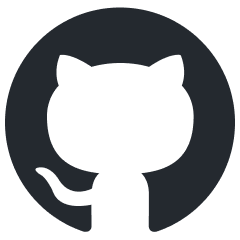
skyvern
Automate browser-based workflows with LLMs and Computer Vision
Stars: 12853
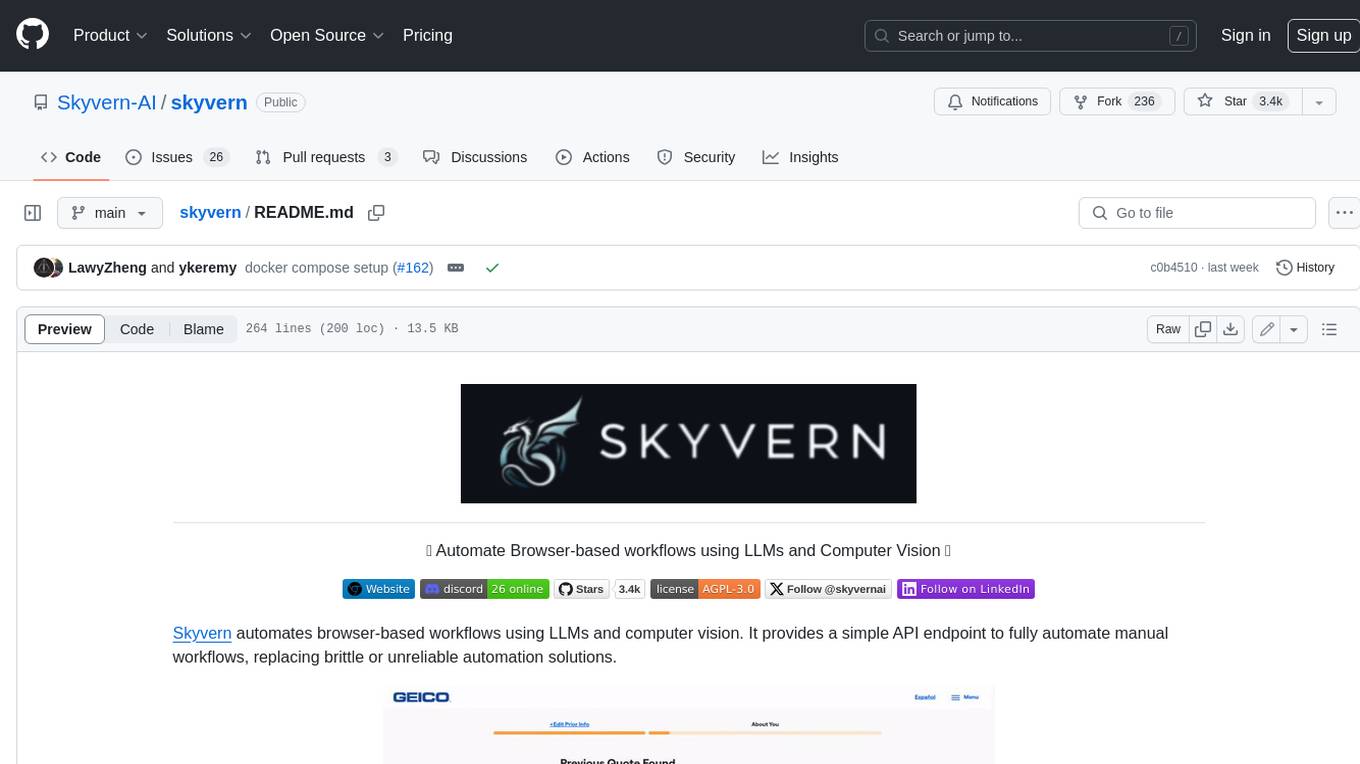
Skyvern automates browser-based workflows using LLMs and computer vision. It provides a simple API endpoint to fully automate manual workflows, replacing brittle or unreliable automation solutions. Traditional approaches to browser automations required writing custom scripts for websites, often relying on DOM parsing and XPath-based interactions which would break whenever the website layouts changed. Instead of only relying on code-defined XPath interactions, Skyvern adds computer vision and LLMs to the mix to parse items in the viewport in real-time, create a plan for interaction and interact with them. This approach gives us a few advantages: 1. Skyvern can operate on websites it’s never seen before, as it’s able to map visual elements to actions necessary to complete a workflow, without any customized code 2. Skyvern is resistant to website layout changes, as there are no pre-determined XPaths or other selectors our system is looking for while trying to navigate 3. Skyvern leverages LLMs to reason through interactions to ensure we can cover complex situations. Examples include: 1. If you wanted to get an auto insurance quote from Geico, the answer to a common question “Were you eligible to drive at 18?” could be inferred from the driver receiving their license at age 16 2. If you were doing competitor analysis, it’s understanding that an Arnold Palmer 22 oz can at 7/11 is almost definitely the same product as a 23 oz can at Gopuff (even though the sizes are slightly different, which could be a rounding error!) Want to see examples of Skyvern in action? Jump to #real-world-examples-of- skyvern
README:
🐉 Automate Browser-based workflows using LLMs and Computer Vision 🐉
Skyvern automates browser-based workflows using LLMs and computer vision. It provides a simple API endpoint to fully automate manual workflows on a large number of websites, replacing brittle or unreliable automation solutions.
Traditional approaches to browser automations required writing custom scripts for websites, often relying on DOM parsing and XPath-based interactions which would break whenever the website layouts changed.
Instead of only relying on code-defined XPath interactions, Skyvern relies on prompts in addition to computer vision and LLMs to parse items in the viewport in real-time, create a plan for interaction and interact with them.
This approach gives us a few advantages:
- Skyvern can operate on websites it’s never seen before, as it’s able to map visual elements to actions necessary to complete a workflow, without any customized code
- Skyvern is resistant to website layout changes, as there are no pre-determined XPaths or other selectors our system is looking for while trying to navigate
- Skyvern is able to take a single workflow and apply it to a large number of websites, as it’s able to reason through the interactions necessary to complete the workflow
- Skyvern leverages LLMs to reason through interactions to ensure we can cover complex situations. Examples include:
- If you wanted to get an auto insurance quote from Geico, the answer to a common question “Were you eligible to drive at 18?” could be inferred from the driver receiving their license at age 16
- If you were doing competitor analysis, it’s understanding that an Arnold Palmer 22 oz can at 7/11 is almost definitely the same product as a 23 oz can at Gopuff (even though the sizes are slightly different, which could be a rounding error!)
Want to see examples of Skyvern in action? Jump to #real-world-examples-of-skyvern
Skyvern was inspired by the Task-Driven autonomous agent design popularized by BabyAGI and AutoGPT -- with one major bonus: we give Skyvern the ability to interact with websites using browser automation libraries like Playwright.
Skyvern uses a swarm of agents to comprehend a website, and plan and execute its actions:
- Interactable Element Agent: This agent is responsible for parsing the HTML of a website and extracting the interactable elements.
- Navigation Agent: This agent is responsible for planning the navigation to complete a task. Examples include clicking buttons, inserting text, selecting options, etc.
- Data Extraction Agent: This agent is responsible for extracting data from a website. It's capable of reading the tables and text on the page, and extracting the output in a user-defined structured format
- Password Agent: This agent is responsible for filling out password forms on a website. It's capable of reading the username and password from a password manager, and filling out the form while preserving the privacy of the user-defined secrets.
- 2FA Agent: This agent is responsible for filling out 2FA forms on a website. It's capable of intercepting website requests for 2FAs, and either requesting user-defined APIs for 2FA codes or waiting for users to feed 2FA codes into it, and then completing the login process.
- Dynamic Auto-complete Agent: This agent is responsible for filling out dynamic auto-complete forms on a website. It's capable of reading the options presented to it, selecting the appropriate option based on the user's input, and adjusting its inputs based on the feedback from inside the form. Popular examples include: Address forms, university dropdowns, and more.

https://github.com/user-attachments/assets/5cab4668-e8e2-4982-8551-aab05ff73a7f
We offer a managed cloud version of Skyvern that allows you to run Skyvern without having to manage the infrastructure. It allows you to run multiple Skyvern instances in parallel and comes bundled with anti-bot detection mechanisms, proxy network, and CAPTCHA solvers.
If you'd like to try it out,
- Navigate to app.skyvern.com
- Create an account & Get $5 of credits on us
- Kick off your first task and see Skyvern in action!
This quickstart guide will walk you through getting Skyvern up and running on your local machine.
⚠️ REQUIREMENT: This project requires Python 3.11⚠️
-
Install Skyvern
pip install skyvern
-
Configure Skyvern Run the setup wizard which will guide you through the configuration process, including Skyvern MCP integration. This will generate a
.env
as the configuration settings file.skyvern init
-
Launch the Skyvern Server
skyvern run server
-
Launch the Skyvern UI
skyvern run ui
- Make sure you have Docker Desktop installed and running on your machine
- Make sure you don't have postgres running locally (Run
docker ps
to check) - Clone the repository and navigate to the root directory
- Fill in the LLM provider key on the docker-compose.yml. If you want to run Skyvern on a remote server, make sure you set the correct server ip for the UI container in docker-compose.yml.
- Run the following command via the commandline:
docker compose up -d
- Navigate to
http://localhost:8080
in your browser to start using the UI
See the MCP documentation here
Here are some tips that may help you on your adventure:
- Skyvern is really good at carrying out a single goal. If you give it too many instructions to do, it has a high likelihood of hallucinating along the way.
- Being really explicit about goals is very important. For example, if you're generating an insurance quote, let it know very clearly how it can identify it has accomplished its goals. Use words like "COMPLETE" or "TERMINATE" to indicate success and failure modes, respectively.
- Workflows can be used if you'd like to do more advanced things such as chaining multiple instructions together, or securely logging in. If you need any help with this, please feel free to book some time with us! We're always happy to help
Skyvern 2.0 is a major overhaul of Skyvern that includes a multi-agent architecture with a planner + validator agent, allowing Skyvern to complete more complex tasks with a zero-shot prompt.
Tasks are the fundamental building block inside Skyvern. Each task is a single request to Skyvern, instructing it to navigate through a website and accomplish a specific goal.
Tasks require you to specify a url
, prompt
, and can optionally include a data schema
(if you want the output to conform to a specific schema) and error codes
(if you want Skyvern to stop running in specific situations).
Workflows are a way to chain multiple tasks together to form a cohesive unit of work.
For example, if you wanted to download all invoices newer than January 1st, you could create a workflow that first navigated to the invoices page, then filtered down to only show invoices newer than January 1st, extracted a list of all eligible invoices, and iterated through each invoice to download it.
Another example is if you wanted to automate purchasing products from an e-commerce store, you could create a workflow that first navigated to the desired product, then added it to a cart. Second, it would navigate to the cart and validate the cart state. Finally, it would go through the checkout process to purchase the items.
Supported workflow features include:
- Navigation
- Action
- Data Extraction
- Loops
- File parsing
- Uploading files to block storage
- Sending emails
- Text Prompts
- Tasks (general)
- (Coming soon) Conditionals
- (Coming soon) Custom Code Block
Skyvern allows you to livestream the viewport of the browser to your local machine so that you can see exactly what Skyvern is doing on the web. This is useful for debugging and understanding how Skyvern is interacting with a website, and intervening when necessary
Skyvern is natively capable of filling out form inputs on websites. Passing in information via the navigation_goal
will allow Skyvern to comprehend the information and fill out the form accordingly.
Skyvern is also capable of extracting data from a website.
You can also specify a data_extraction_schema
directly within the main prompt to tell Skyvern exactly what data you'd like to extract from the website, in jsonc format. Skyvern's output will be structured in accordance to the supplied schema.
Skyvern is also capable of downloading files from a website. All downloaded files are automatically uploaded to block storage (if configured), and you can access them via the UI.
Skyvern supports a number of different authentication methods to make it easier to automate tasks behind a login. If you'd like to try it out, please reach out to us via email or discord.
Skyvern currently supports the following password manager integrations:
- [x] Bitwarden
- [ ] 1Password
- [ ] LastPass
Skyvern supports a number of different 2FA methods to allow you to automate workflows that require 2FA.
Examples include:
- QR-based 2FA (e.g. Google Authenticator, Authy)
- Email based 2FA
- SMS based 2FA
We love to see how Skyvern is being used in the wild. Here are some examples of how Skyvern is being used to automate workflows in the real world. Please open PRs to add your own examples!
⚠️ ⚠️ MAKE SURE YOU ARE USING PYTHON 3.11⚠️ ⚠️ ⚠️ ⚠️ Only well-tested on MacOS⚠️ ⚠️
Before you begin, make sure you have the following installed:
- Brew (if you're on a Mac)
-
Poetry
brew install poetry
- node
- Docker
Note: Our setup script does these two for you, but they are here for reference.
-
Python 3.11
poetry env use 3.11
-
PostgreSQL 14 (if you're on a Mac, setup script will install it for you if you have homebrew installed)
brew install postgresql
- Clone the repository and navigate to the root directory
- Open Docker Desktop (Works for Windows, macOS, and Linux) or run Docker Daemon
- Run the setup script to install the necessary dependencies and setup your environment
skyvern/scripts/setup.sh
- Start the server
./run_skyvern.sh
- You can start sending requests to the server, but we built a simple UI to help you get started. To start the UI, run the following command:
./run_ui.sh
- Navigate to
http://localhost:8080
in your browser to start using the UI
If you're looking to contribute to Skyvern, you'll need to install the pre-commit hooks to ensure code quality and consistency. You can do this by running the following command:
pre-commit install
More extensive documentation can be found on our docs page. Please let us know if something is unclear or missing by opening an issue or reaching out to us via email or discord.
Provider | Supported Models |
---|---|
OpenAI | gpt4-turbo, gpt-4o, gpt-4o-mini |
Anthropic | Claude 3 (Haiku, Sonnet, Opus), Claude 3.5 (Sonnet) |
Azure OpenAI | Any GPT models. Better performance with a multimodal llm (azure/gpt4-o) |
AWS Bedrock | Anthropic Claude 3 (Haiku, Sonnet, Opus), Claude 3.5 (Sonnet) |
Ollama | Coming soon (contributions welcome) |
Gemini | Coming soon (contributions welcome) |
Llama 3.2 | Coming soon (contributions welcome) |
Novita AI | Llama 3.1 (8B, 70B), Llama 3.2 (1B, 3B, 11B Vision) |
Variable | Description | Type | Sample Value |
---|---|---|---|
ENABLE_OPENAI |
Register OpenAI models | Boolean |
true , false
|
ENABLE_ANTHROPIC |
Register Anthropic models | Boolean |
true , false
|
ENABLE_AZURE |
Register Azure OpenAI models | Boolean |
true , false
|
ENABLE_BEDROCK |
Register AWS Bedrock models. To use AWS Bedrock, you need to make sure your AWS configurations are set up correctly first. | Boolean |
true , false
|
ENABLE_GEMINI |
Register Gemini models | Boolean |
true , false
|
ENABLE_NOVITA |
Register Novita AI models | Boolean |
true , false
|
LLM_KEY |
The name of the model you want to use | String | Currently supported llm keys: OPENAI_GPT4_TURBO , OPENAI_GPT4V , OPENAI_GPT4O , OPENAI_GPT4O_MINI , ANTHROPIC_CLAUDE3 , ANTHROPIC_CLAUDE3_OPUS , ANTHROPIC_CLAUDE3_SONNET , ANTHROPIC_CLAUDE3_HAIKU , ANTHROPIC_CLAUDE3.5_SONNET , BEDROCK_ANTHROPIC_CLAUDE3_OPUS , BEDROCK_ANTHROPIC_CLAUDE3_SONNET , BEDROCK_ANTHROPIC_CLAUDE3_HAIKU , BEDROCK_ANTHROPIC_CLAUDE3.5_SONNET , AZURE_OPENAI , GEMINI_PRO , GEMINI_FLASH , BEDROCK_AMAZON_NOVA_PRO , BEDROCK_AMAZON_NOVA_LITE
|
SECONDARY_LLM_KEY |
The name of the model for mini agents skyvern runs with | String | Currently supported llm keys: OPENAI_GPT4_TURBO , OPENAI_GPT4V , OPENAI_GPT4O , OPENAI_GPT4O_MINI , ANTHROPIC_CLAUDE3 , ANTHROPIC_CLAUDE3_OPUS , ANTHROPIC_CLAUDE3_SONNET , ANTHROPIC_CLAUDE3_HAIKU , ANTHROPIC_CLAUDE3.5_SONNET , BEDROCK_ANTHROPIC_CLAUDE3_OPUS , BEDROCK_ANTHROPIC_CLAUDE3_SONNET , BEDROCK_ANTHROPIC_CLAUDE3_HAIKU , BEDROCK_ANTHROPIC_CLAUDE3.5_SONNET , AZURE_OPENAI , GEMINI_PRO , GEMINI_FLASH , NOVITA_DEEPSEEK_R1 , NOVITA_DEEPSEEK_V3 , NOVITA_LLAMA_3_3_70B , NOVITA_LLAMA_3_2_1B , NOVITA_LLAMA_3_2_3B , NOVITA_LLAMA_3_2_11B_VISION , NOVITA_LLAMA_3_1_8B , NOVITA_LLAMA_3_1_70B , NOVITA_LLAMA_3_1_405B , NOVITA_LLAMA_3_8B , NOVITA_LLAMA_3_70B
|
OPENAI_API_KEY |
OpenAI API Key | String | sk-1234567890 |
OPENAI_API_BASE |
OpenAI API Base, optional | String | https://openai.api.base |
OPENAI_ORGANIZATION |
OpenAI Organization ID, optional | String | your-org-id |
ANTHROPIC_API_KEY |
Anthropic API key | String | sk-1234567890 |
AZURE_API_KEY |
Azure deployment API key | String | sk-1234567890 |
AZURE_DEPLOYMENT |
Azure OpenAI Deployment Name | String | skyvern-deployment |
AZURE_API_BASE |
Azure deployment api base url | String | https://skyvern-deployment.openai.azure.com/ |
AZURE_API_VERSION |
Azure API Version | String | 2024-02-01 |
GEMINI_API_KEY |
Gemini API Key | String | your_google_gemini_api_key |
This is our planned roadmap for the next few months. If you have any suggestions or would like to see a feature added, please don't hesitate to reach out to us via email or discord.
- [x] Open Source - Open Source Skyvern's core codebase
- [x] [BETA] Workflow support - Allow support to chain multiple Skyvern calls together
- [x] Improved context - Improve Skyvern's ability to understand content around interactable elements by introducing feeding relevant label context through the text prompt
- [x] Cost Savings - Improve Skyvern's stability and reduce the cost of running Skyvern by optimizing the context tree passed into Skyvern
- [x] Self-serve UI - Deprecate the Streamlit UI in favour of a React-based UI component that allows users to kick off new jobs in Skyvern
- [x] Workflow UI Builder - Introduce a UI to allow users to build and analyze workflows visually
- [x] Chrome Viewport streaming - Introduce a way to live-stream the Chrome viewport to the user's browser (as a part of the self-serve UI)
- [x] Past Runs UI - Deprecate the Streamlit UI in favour of a React-based UI that allows you to visualize past runs and their results
- [X] Auto workflow builder ("Observer") mode - Allow Skyvern to auto-generate workflows as it's navigating the web to make it easier to build new workflows
- [ ] Prompt Caching - Introduce a caching layer to the LLM calls to dramatically reduce the cost of running Skyvern (memorize past actions and repeat them!)
- [ ] Web Evaluation Dataset - Integrate Skyvern with public benchmark tests to track the quality of our models over time
- [ ] Improved Debug mode - Allow Skyvern to plan its actions and get "approval" before running them, allowing you to debug what it's doing and more easily iterate on the prompt
- [ ] Chrome Extension - Allow users to interact with Skyvern through a Chrome extension (incl voice mode, saving tasks, etc.)
- [ ] Skyvern Action Recorder - Allow Skyvern to watch a user complete a task and then automatically generate a workflow for it
- [ ] Interactable Livestream - Allow users to interact with the livestream in real-time to intervene when necessary (such as manually submitting sensitive forms)
- [ ] Integrate LLM Observability tools - Integrate LLM Observability tools to allow back-testing prompt changes with specific data sets + visualize the performance of Skyvern over time
- [ ] Langchain Integration - Create langchain integration in langchain_community to use Skyvern as a "tool".
We welcome PRs and suggestions! Don't hesitate to open a PR/issue or to reach out to us via email or discord. Please have a look at our contribution guide and "Help Wanted" issues to get started!
If you want to chat with the skyvern repository to get a high level overview of how it is structured, how to build off it, and how to resolve usage questions, check out Code Sage.
By Default, Skyvern collects basic usage statistics to help us understand how Skyvern is being used. If you would like to opt-out of telemetry, please set the SKYVERN_TELEMETRY
environment variable to false
.
Skyvern's open source repository is supported via a managed cloud. All of the core logic powering Skyvern is available in this open source repository licensed under the AGPL-3.0 License, with the exception of anti-bot measures available in our managed cloud offering.
If you have any questions or concerns around licensing, please contact us and we would be happy to help.
For Tasks:
Click tags to check more tools for each tasksFor Jobs:
Alternative AI tools for skyvern
Similar Open Source Tools
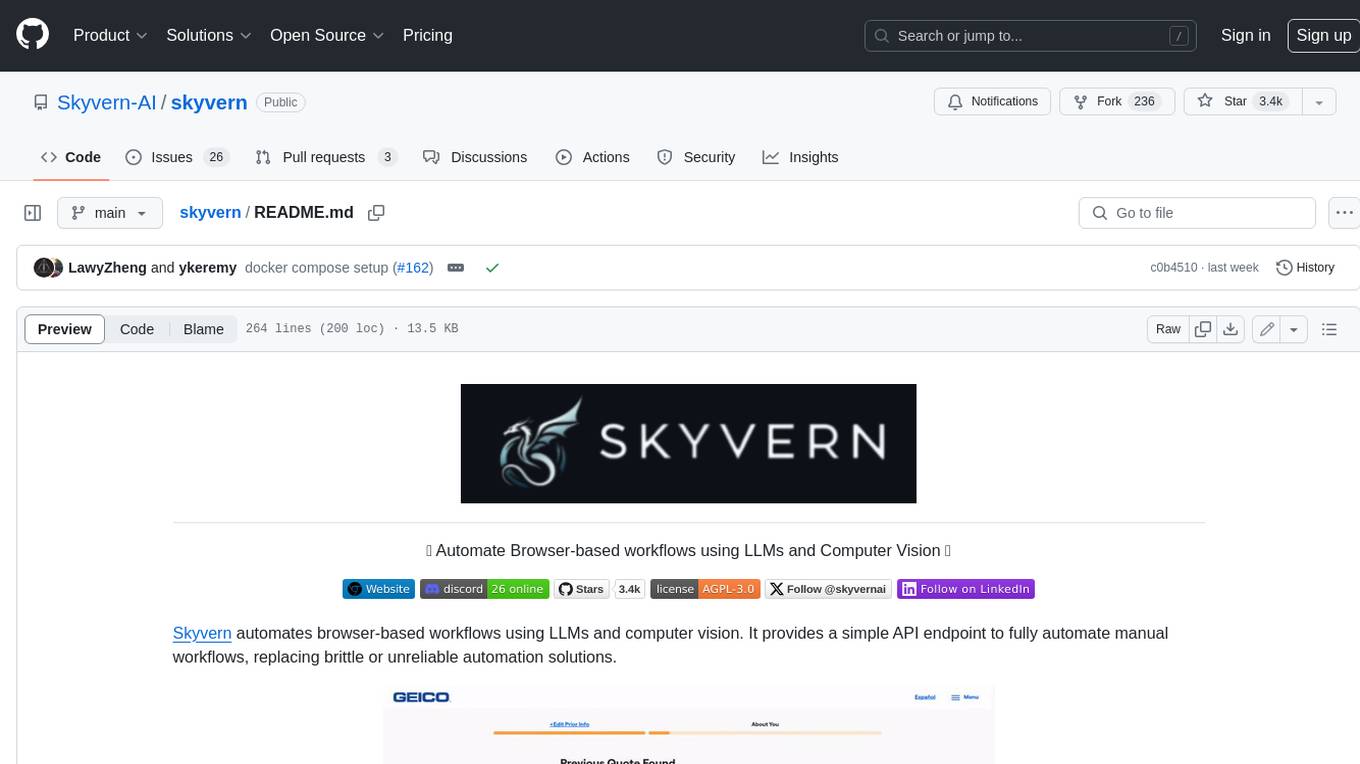
skyvern
Skyvern automates browser-based workflows using LLMs and computer vision. It provides a simple API endpoint to fully automate manual workflows, replacing brittle or unreliable automation solutions. Traditional approaches to browser automations required writing custom scripts for websites, often relying on DOM parsing and XPath-based interactions which would break whenever the website layouts changed. Instead of only relying on code-defined XPath interactions, Skyvern adds computer vision and LLMs to the mix to parse items in the viewport in real-time, create a plan for interaction and interact with them. This approach gives us a few advantages: 1. Skyvern can operate on websites it’s never seen before, as it’s able to map visual elements to actions necessary to complete a workflow, without any customized code 2. Skyvern is resistant to website layout changes, as there are no pre-determined XPaths or other selectors our system is looking for while trying to navigate 3. Skyvern leverages LLMs to reason through interactions to ensure we can cover complex situations. Examples include: 1. If you wanted to get an auto insurance quote from Geico, the answer to a common question “Were you eligible to drive at 18?” could be inferred from the driver receiving their license at age 16 2. If you were doing competitor analysis, it’s understanding that an Arnold Palmer 22 oz can at 7/11 is almost definitely the same product as a 23 oz can at Gopuff (even though the sizes are slightly different, which could be a rounding error!) Want to see examples of Skyvern in action? Jump to #real-world-examples-of- skyvern
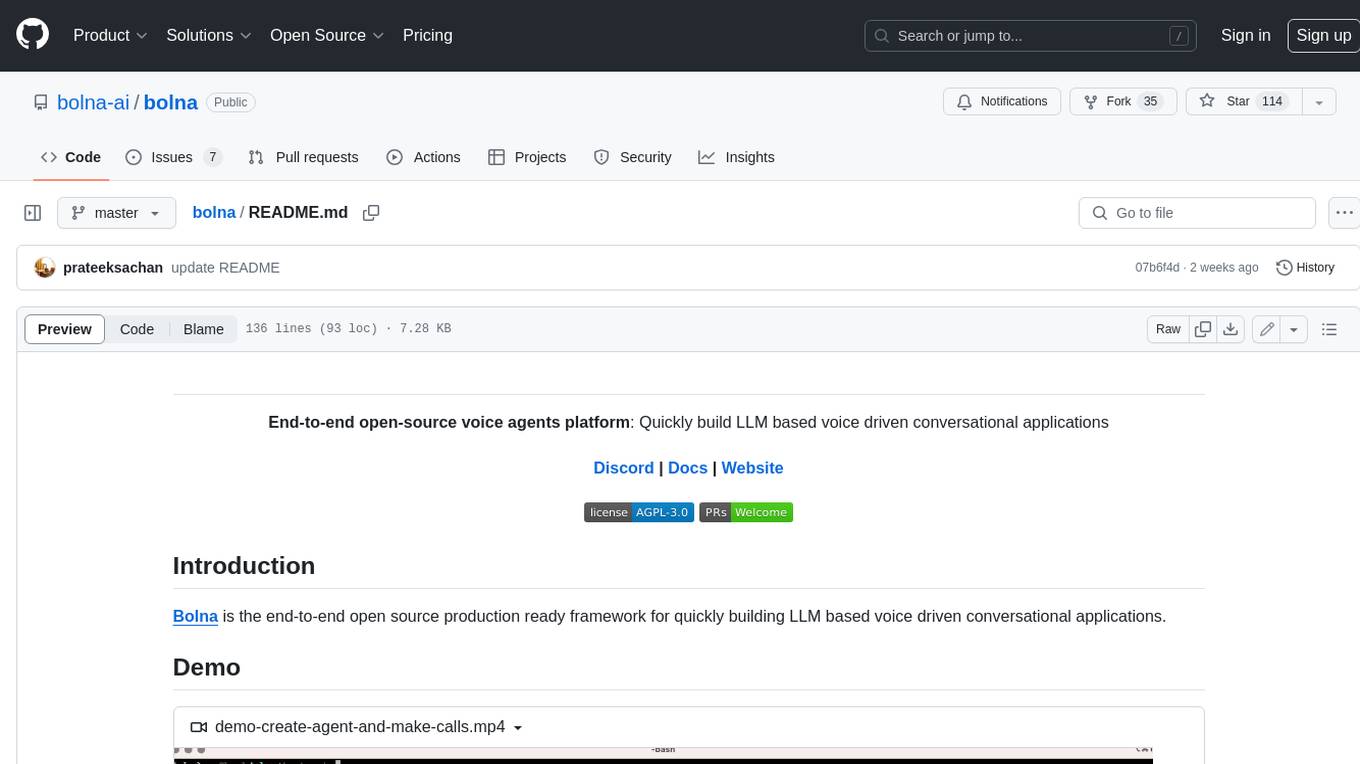
bolna
Bolna is an open-source platform for building voice-driven conversational applications using large language models (LLMs). It provides a comprehensive set of tools and integrations to handle various aspects of voice-based interactions, including telephony, transcription, LLM-based conversation handling, and text-to-speech synthesis. Bolna simplifies the process of creating voice agents that can perform tasks such as initiating phone calls, transcribing conversations, generating LLM-powered responses, and synthesizing speech. It supports multiple providers for each component, allowing users to customize their setup based on their specific needs. Bolna is designed to be easy to use, with a straightforward local setup process and well-documented APIs. It is also extensible, enabling users to integrate with other telephony providers or add custom functionality.
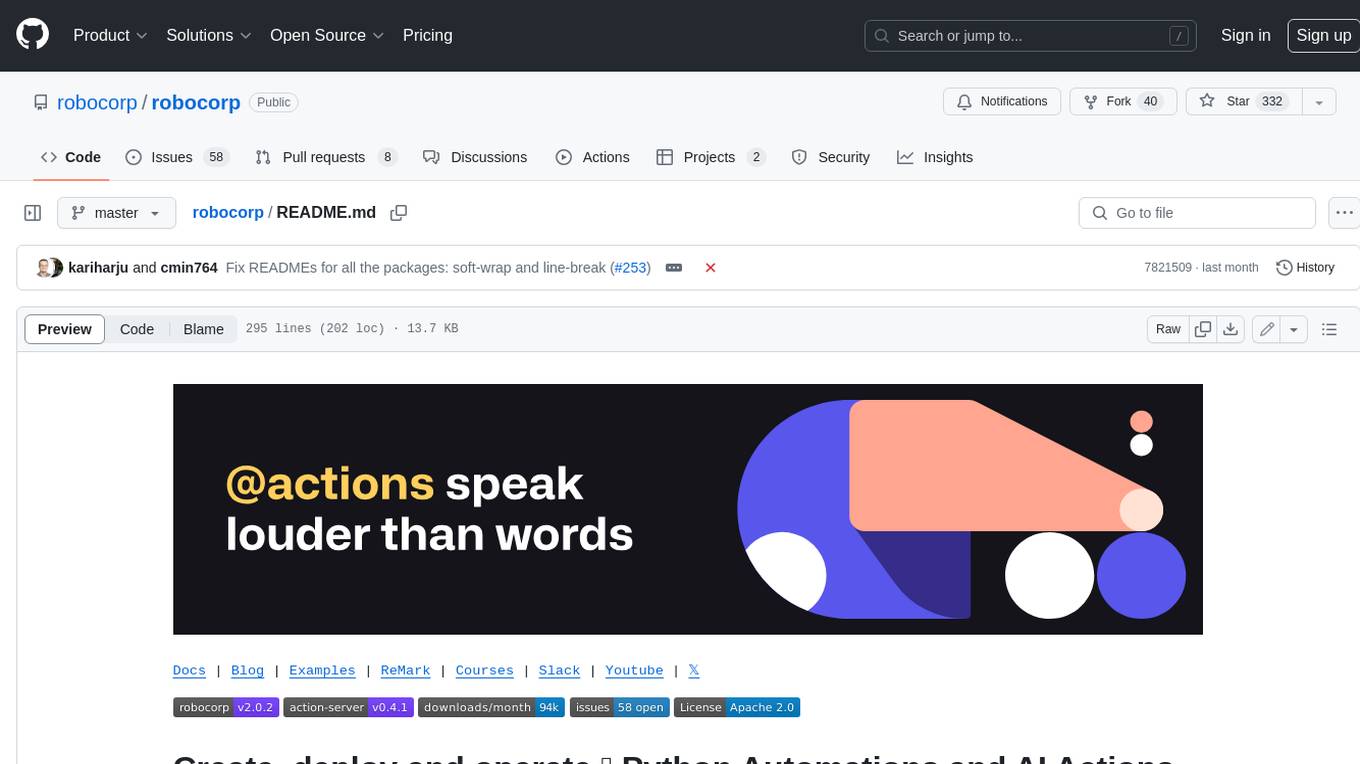
robocorp
Robocorp is a platform that allows users to create, deploy, and operate Python automations and AI actions. It provides an easy way to extend the capabilities of AI agents, assistants, and copilots with custom actions written in Python. Users can create and deploy tools, skills, loaders, and plugins that securely connect any AI Assistant platform to their data and applications. The Robocorp Action Server makes Python scripts compatible with ChatGPT and LangChain by automatically creating and exposing an API based on function declaration, type hints, and docstrings. It simplifies the process of developing and deploying AI actions, enabling users to interact with AI frameworks effortlessly.
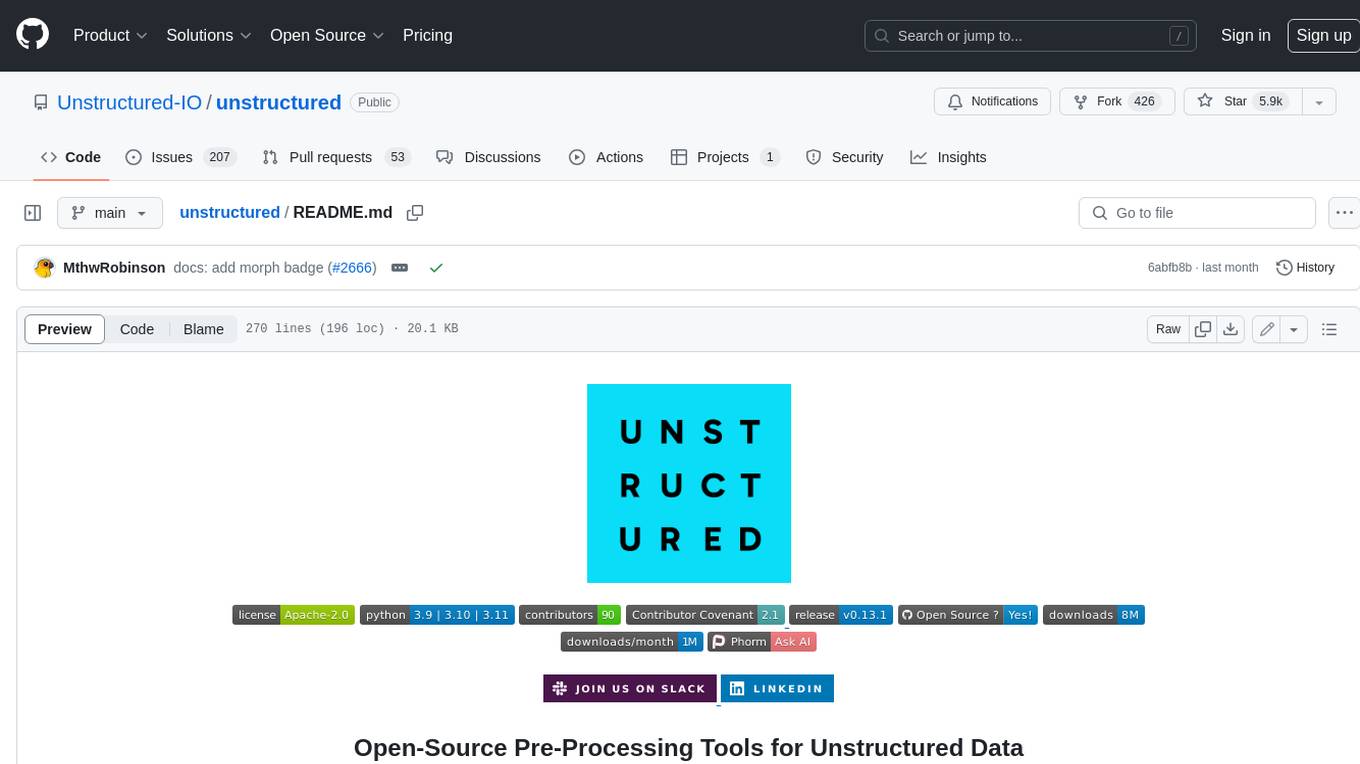
unstructured
The `unstructured` library provides open-source components for ingesting and pre-processing images and text documents, such as PDFs, HTML, Word docs, and many more. The use cases of `unstructured` revolve around streamlining and optimizing the data processing workflow for LLMs. `unstructured` modular functions and connectors form a cohesive system that simplifies data ingestion and pre-processing, making it adaptable to different platforms and efficient in transforming unstructured data into structured outputs.
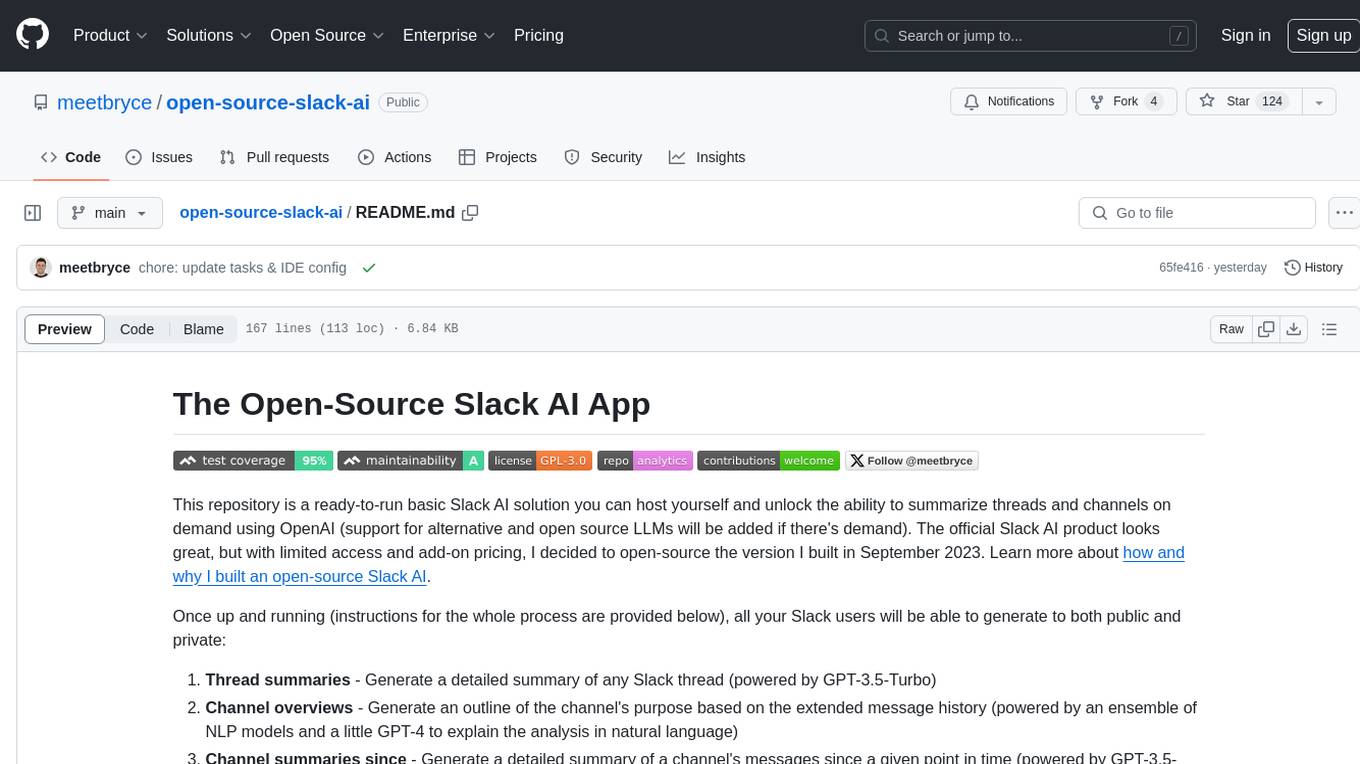
open-source-slack-ai
This repository provides a ready-to-run basic Slack AI solution that allows users to summarize threads and channels using OpenAI. Users can generate thread summaries, channel overviews, channel summaries since a specific time, and full channel summaries. The tool is powered by GPT-3.5-Turbo and an ensemble of NLP models. It requires Python 3.8 or higher, an OpenAI API key, Slack App with associated API tokens, Poetry package manager, and ngrok for local development. Users can customize channel and thread summaries, run tests with coverage using pytest, and contribute to the project for future enhancements.
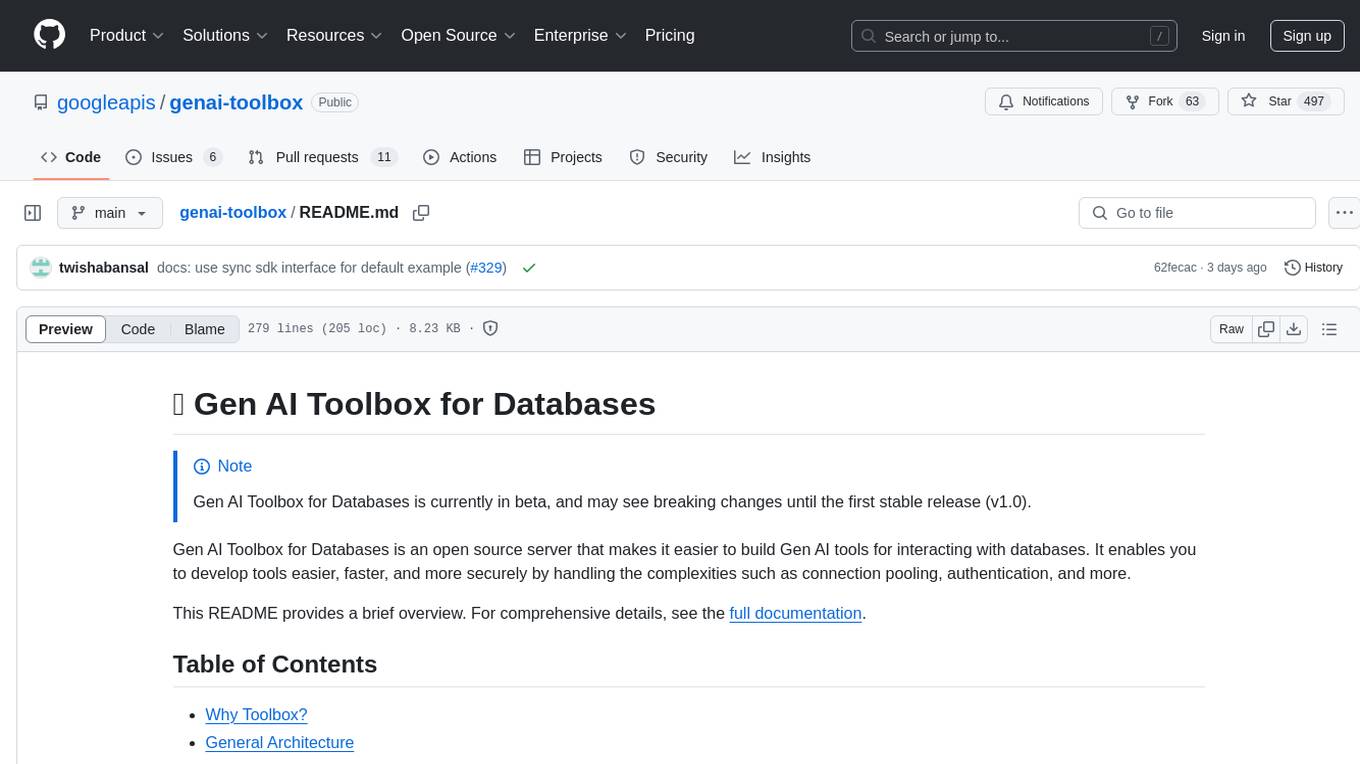
genai-toolbox
Gen AI Toolbox for Databases is an open source server that simplifies building Gen AI tools for interacting with databases. It handles complexities like connection pooling, authentication, and more, enabling easier, faster, and more secure tool development. The toolbox sits between the application's orchestration framework and the database, providing a control plane to modify, distribute, or invoke tools. It offers simplified development, better performance, enhanced security, and end-to-end observability. Users can install the toolbox as a binary, container image, or compile from source. Configuration is done through a 'tools.yaml' file, defining sources, tools, and toolsets. The project follows semantic versioning and welcomes contributions.
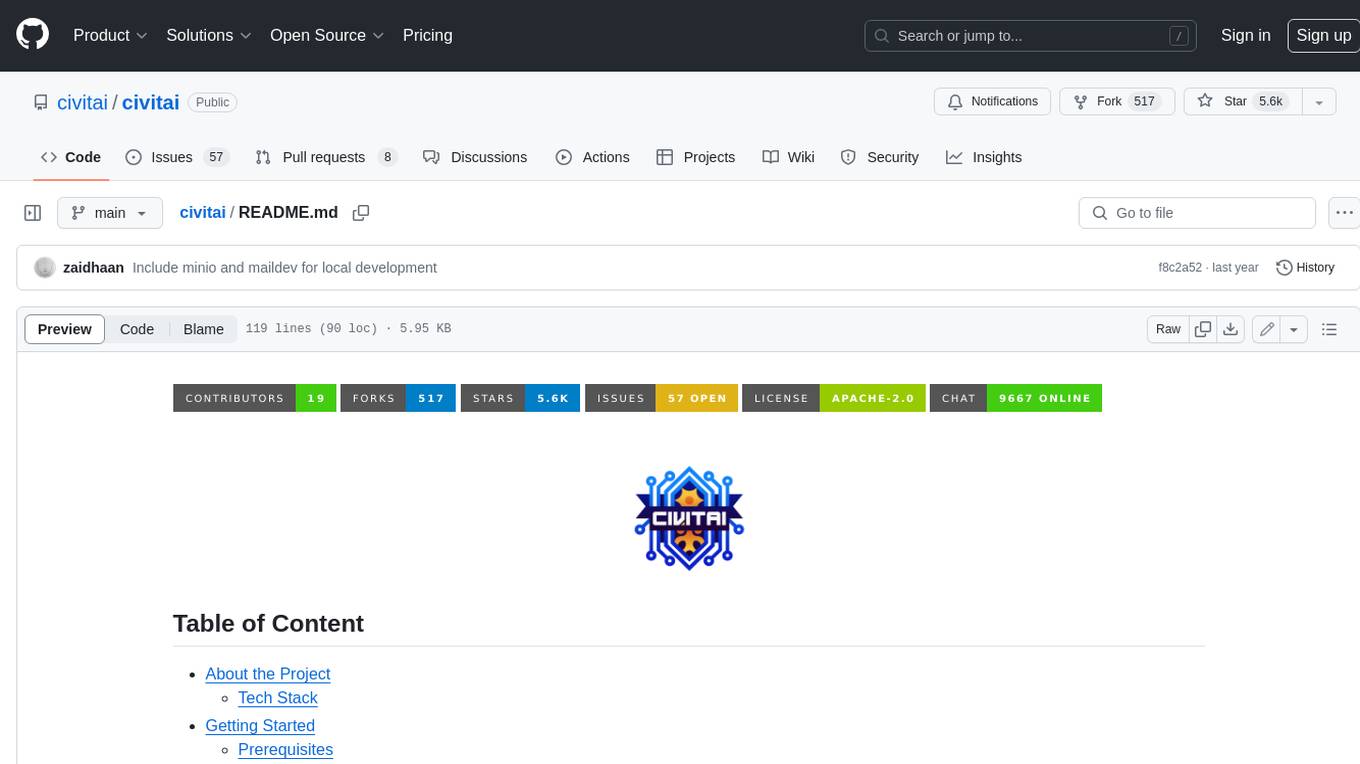
civitai
Civitai is a platform where people can share their stable diffusion models (textual inversions, hypernetworks, aesthetic gradients, VAEs, and any other crazy stuff people do to customize their AI generations), collaborate with others to improve them, and learn from each other's work. The platform allows users to create an account, upload their models, and browse models that have been shared by others. Users can also leave comments and feedback on each other's models to facilitate collaboration and knowledge sharing.
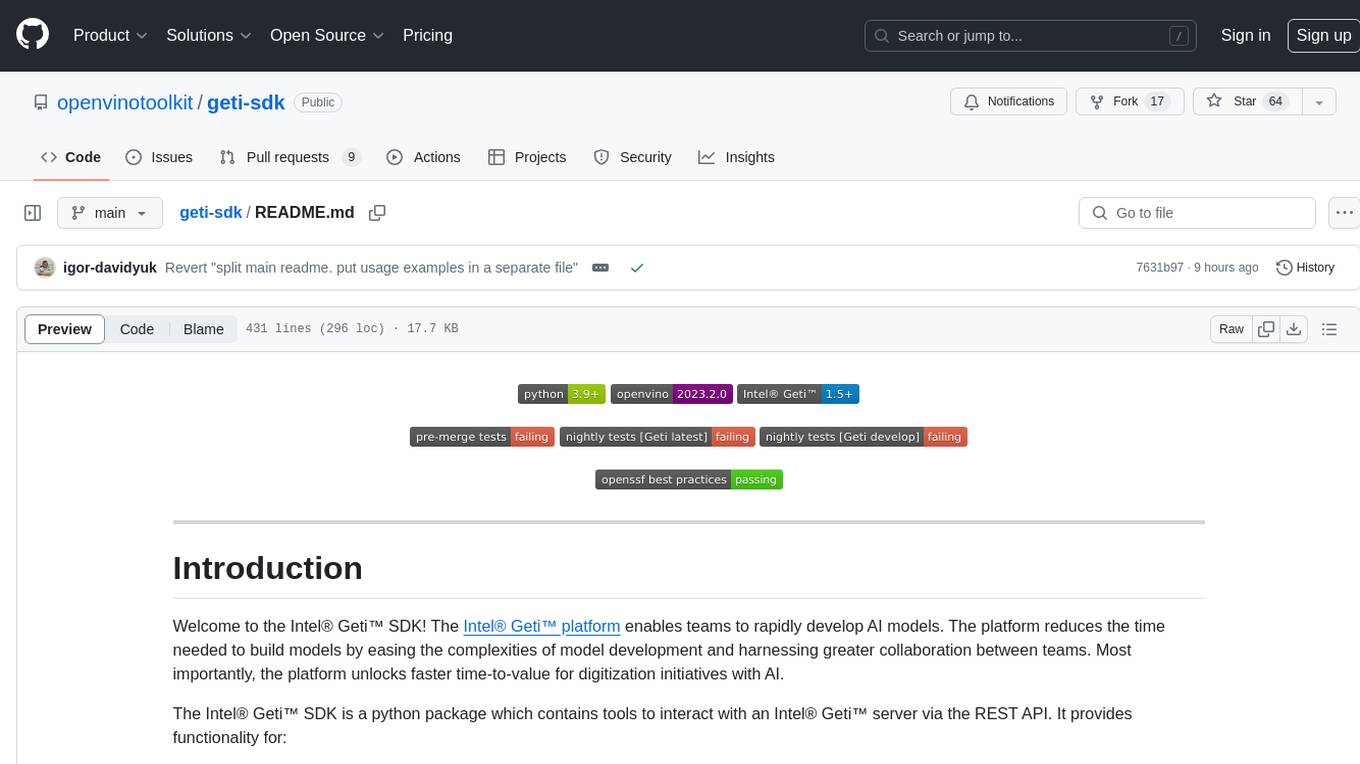
geti-sdk
The Intel® Geti™ SDK is a python package that enables teams to rapidly develop AI models by easing the complexities of model development and enhancing collaboration between teams. It provides tools to interact with an Intel® Geti™ server via the REST API, allowing for project creation, downloading, uploading, deploying for local inference with OpenVINO, setting project and model configuration, launching and monitoring training jobs, and media upload and prediction. The SDK also includes tutorial-style Jupyter notebooks demonstrating its usage.
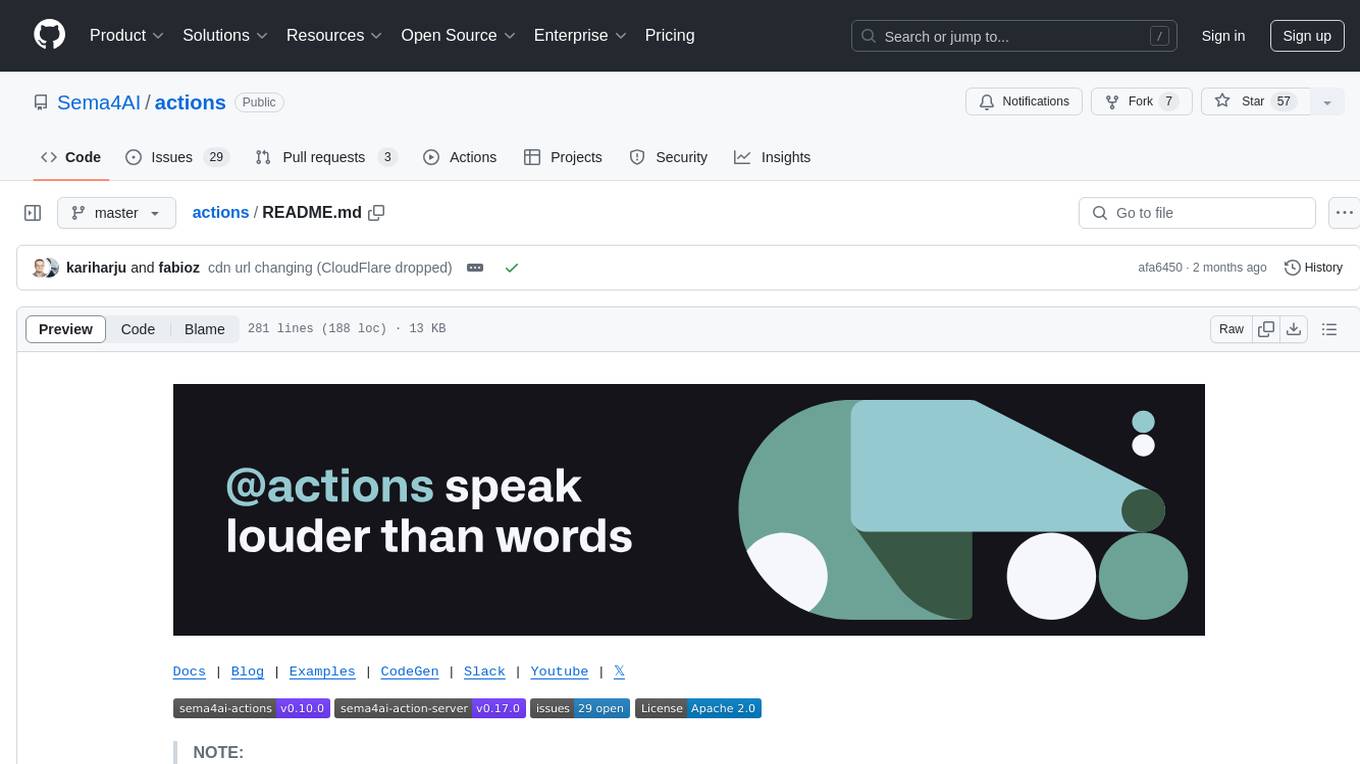
actions
Sema4.ai Action Server is a tool that allows users to build semantic actions in Python to connect AI agents with real-world applications. It enables users to create custom actions, skills, loaders, and plugins that securely connect any AI Assistant platform to data and applications. The tool automatically creates and exposes an API based on function declaration, type hints, and docstrings by adding '@action' to Python scripts. It provides an end-to-end stack supporting various connections between AI and user's apps and data, offering ease of use, security, and scalability.
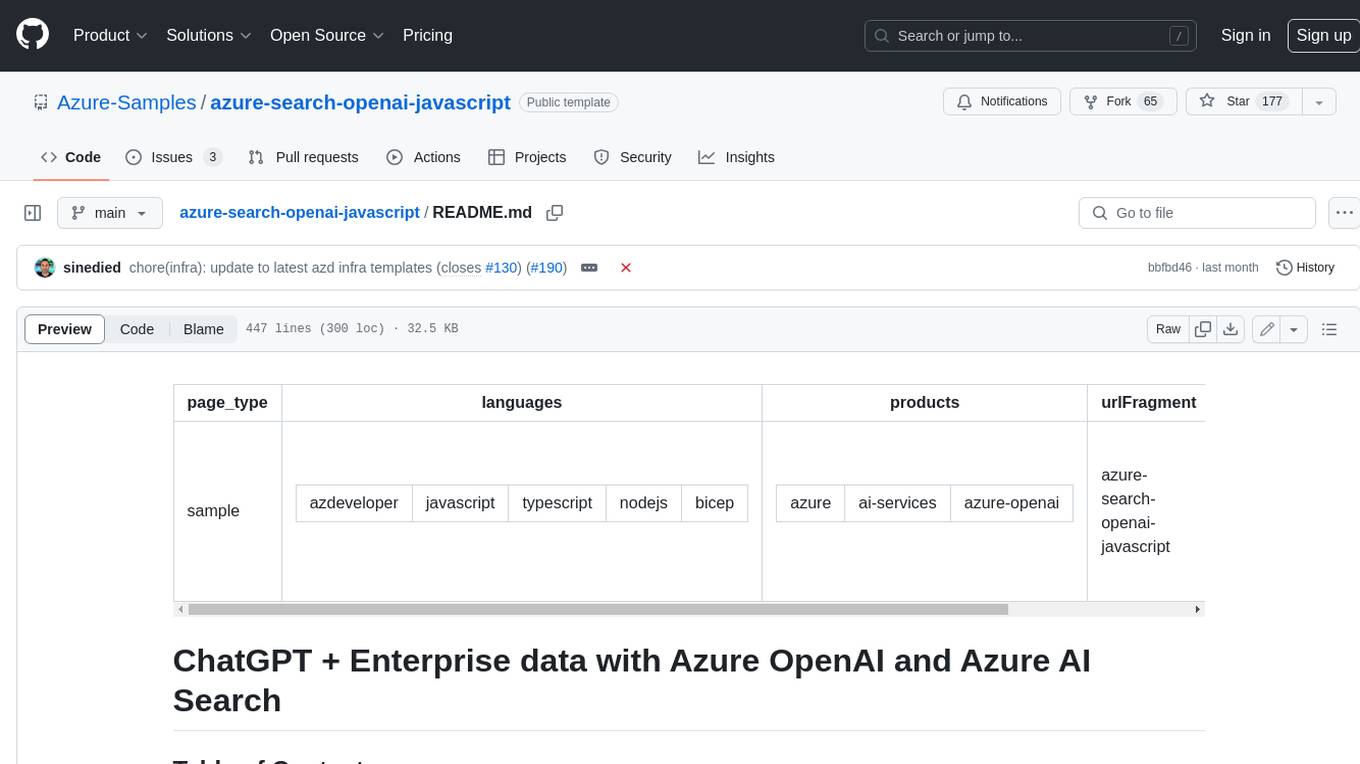
azure-search-openai-javascript
This sample demonstrates a few approaches for creating ChatGPT-like experiences over your own data using the Retrieval Augmented Generation pattern. It uses Azure OpenAI Service to access the ChatGPT model (gpt-35-turbo), and Azure AI Search for data indexing and retrieval.
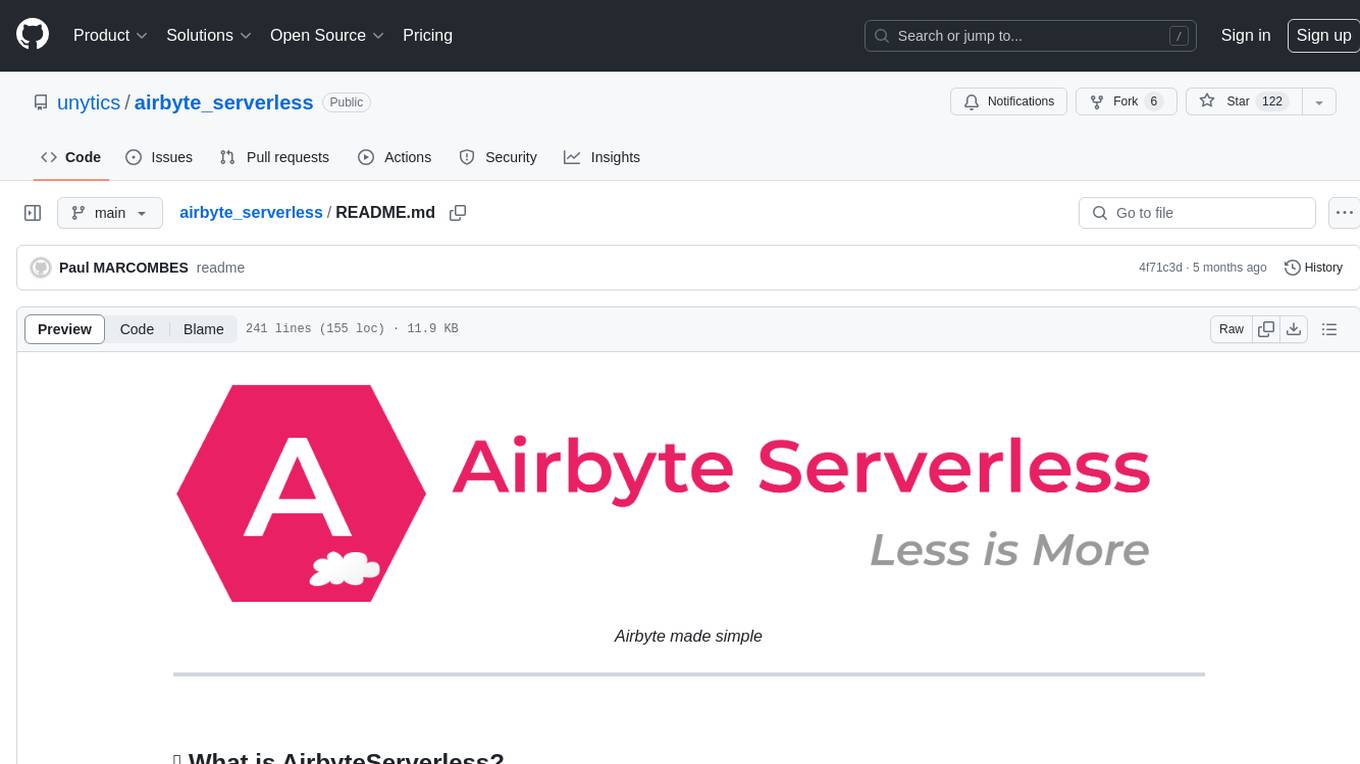
airbyte_serverless
AirbyteServerless is a lightweight tool designed to simplify the management of Airbyte connectors. It offers a serverless mode for running connectors, allowing users to easily move data from any source to their data warehouse. Unlike the full Airbyte-Open-Source-Platform, AirbyteServerless focuses solely on the Extract-Load process without a UI, database, or transform layer. It provides a CLI tool, 'abs', for managing connectors, creating connections, running jobs, selecting specific data streams, handling secrets securely, and scheduling remote runs. The tool is scalable, allowing independent deployment of multiple connectors. It aims to streamline the connector management process and provide a more agile alternative to the comprehensive Airbyte platform.
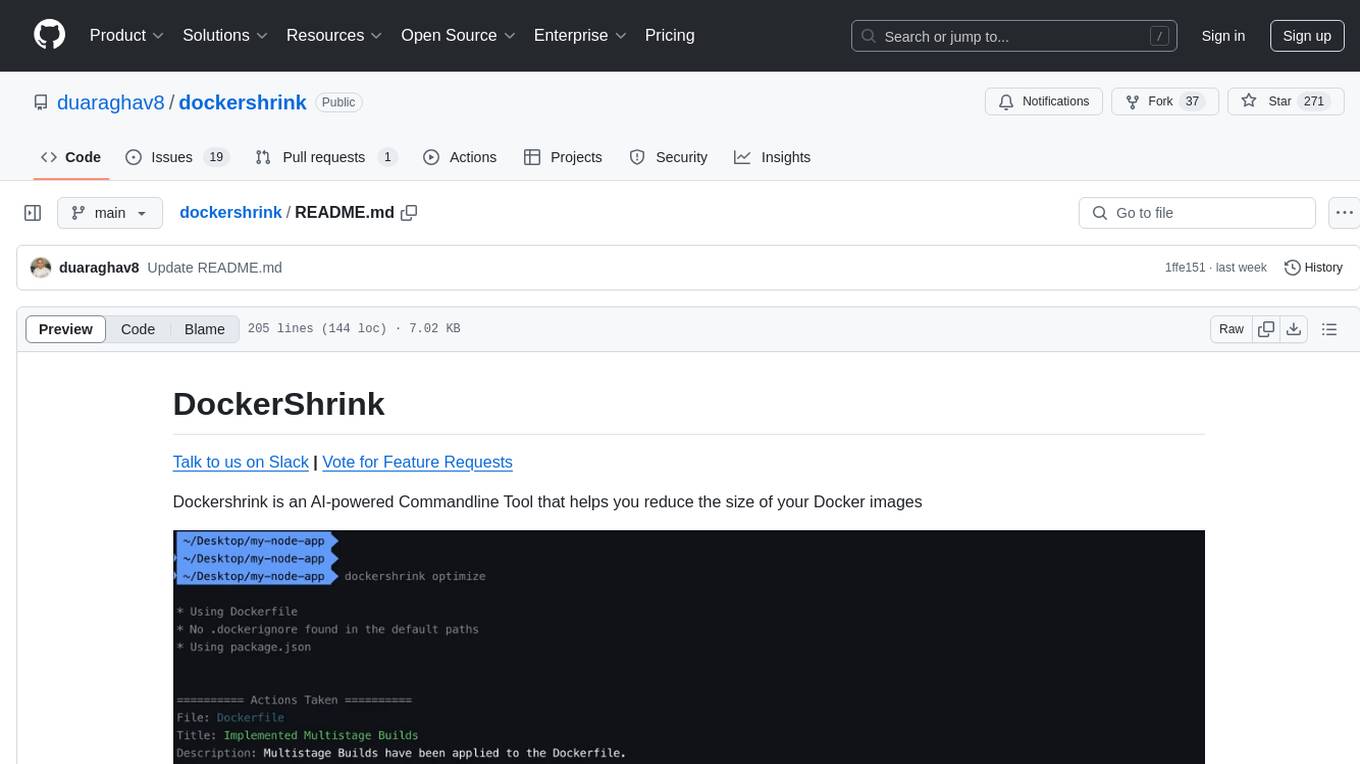
dockershrink
Dockershrink is an AI-powered Commandline Tool designed to help reduce the size of Docker images. It combines traditional Rule-based analysis with Generative AI techniques to optimize Image configurations. The tool supports NodeJS applications and aims to save costs on storage, data transfer, and build times while increasing developer productivity. By automatically applying advanced optimization techniques, Dockershrink simplifies the process for engineers and organizations, resulting in significant savings and efficiency improvements.
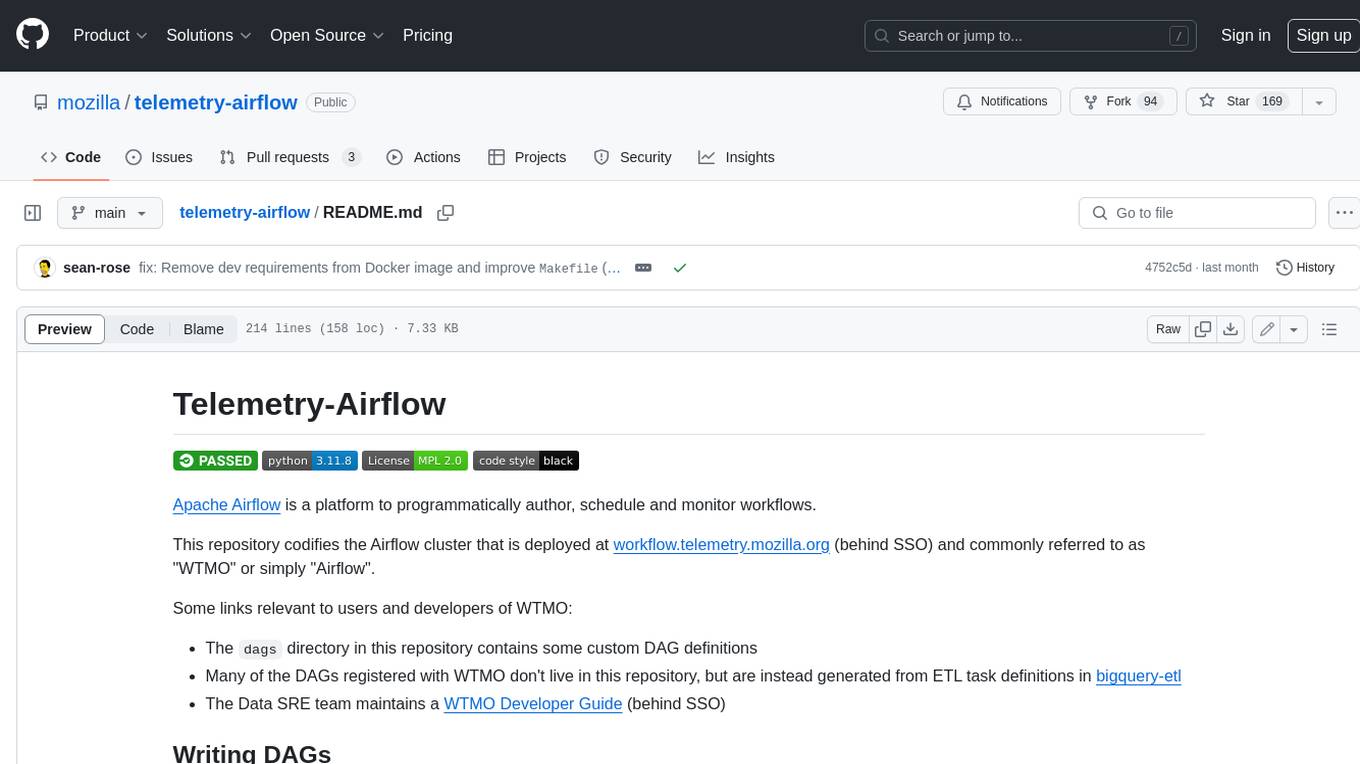
telemetry-airflow
This repository codifies the Airflow cluster that is deployed at workflow.telemetry.mozilla.org (behind SSO) and commonly referred to as "WTMO" or simply "Airflow". Some links relevant to users and developers of WTMO: * The `dags` directory in this repository contains some custom DAG definitions * Many of the DAGs registered with WTMO don't live in this repository, but are instead generated from ETL task definitions in bigquery-etl * The Data SRE team maintains a WTMO Developer Guide (behind SSO)
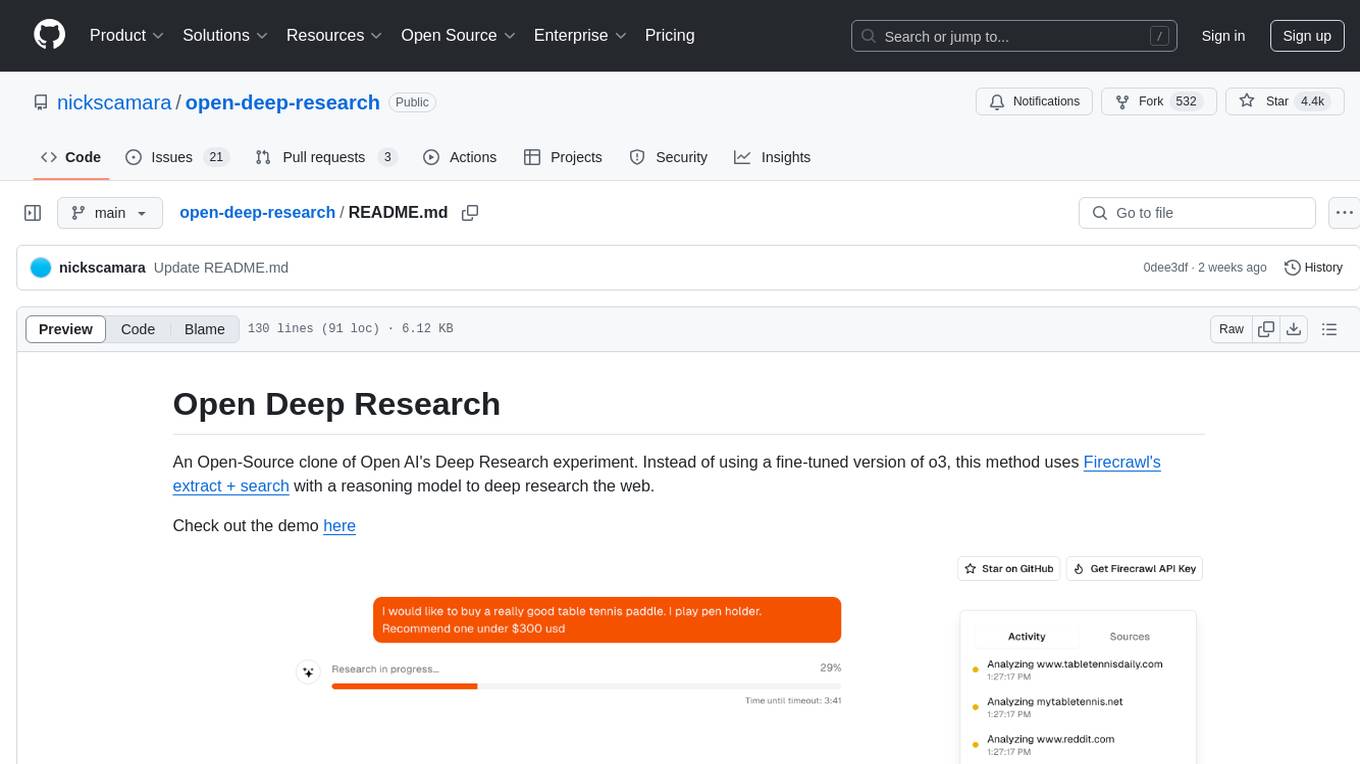
open-deep-research
Open Deep Research is an open-source project that serves as a clone of Open AI's Deep Research experiment. It utilizes Firecrawl's extract and search method along with a reasoning model to conduct in-depth research on the web. The project features Firecrawl Search + Extract, real-time data feeding to AI via search, structured data extraction from multiple websites, Next.js App Router for advanced routing, React Server Components and Server Actions for server-side rendering, AI SDK for generating text and structured objects, support for various model providers, styling with Tailwind CSS, data persistence with Vercel Postgres and Blob, and simple and secure authentication with NextAuth.js.
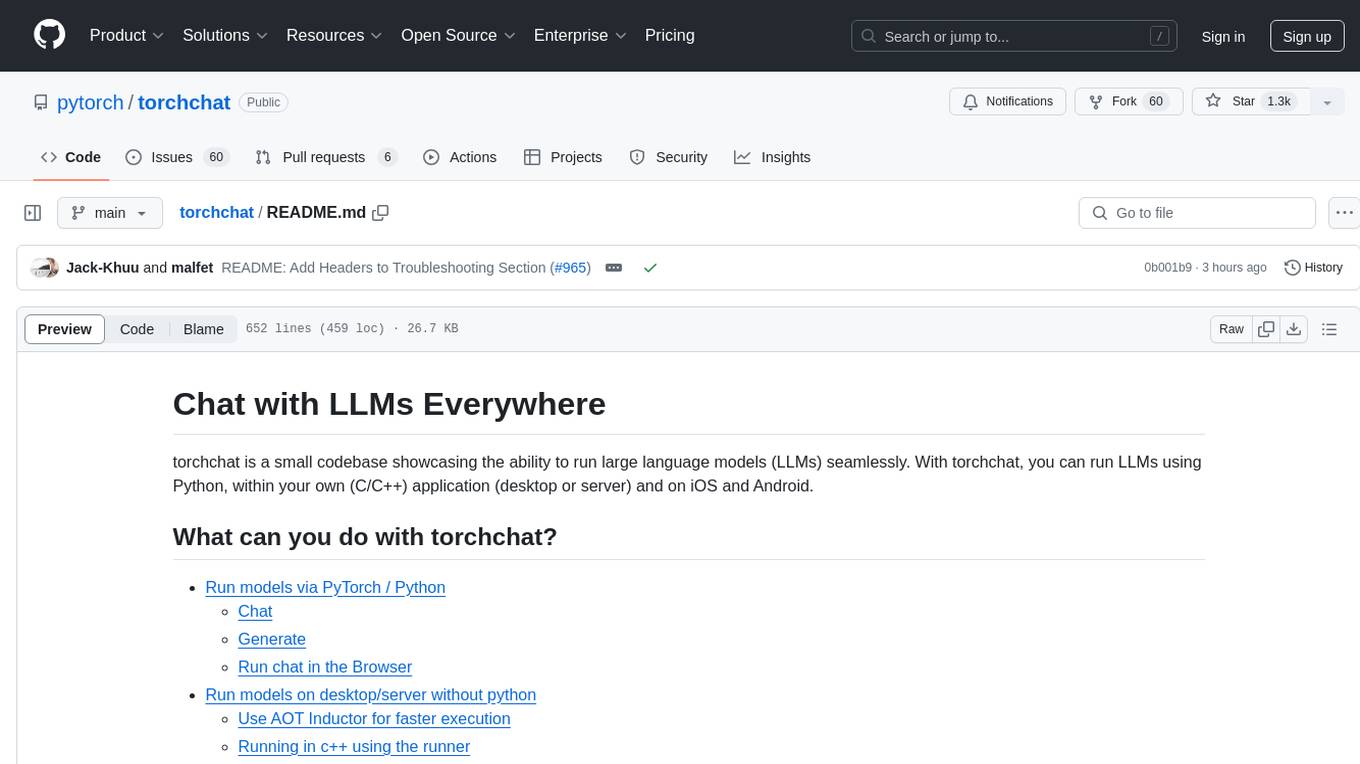
torchchat
torchchat is a codebase showcasing the ability to run large language models (LLMs) seamlessly. It allows running LLMs using Python in various environments such as desktop, server, iOS, and Android. The tool supports running models via PyTorch, chatting, generating text, running chat in the browser, and running models on desktop/server without Python. It also provides features like AOT Inductor for faster execution, running in C++ using the runner, and deploying and running on iOS and Android. The tool supports popular hardware and OS including Linux, Mac OS, Android, and iOS, with various data types and execution modes available.
For similar tasks
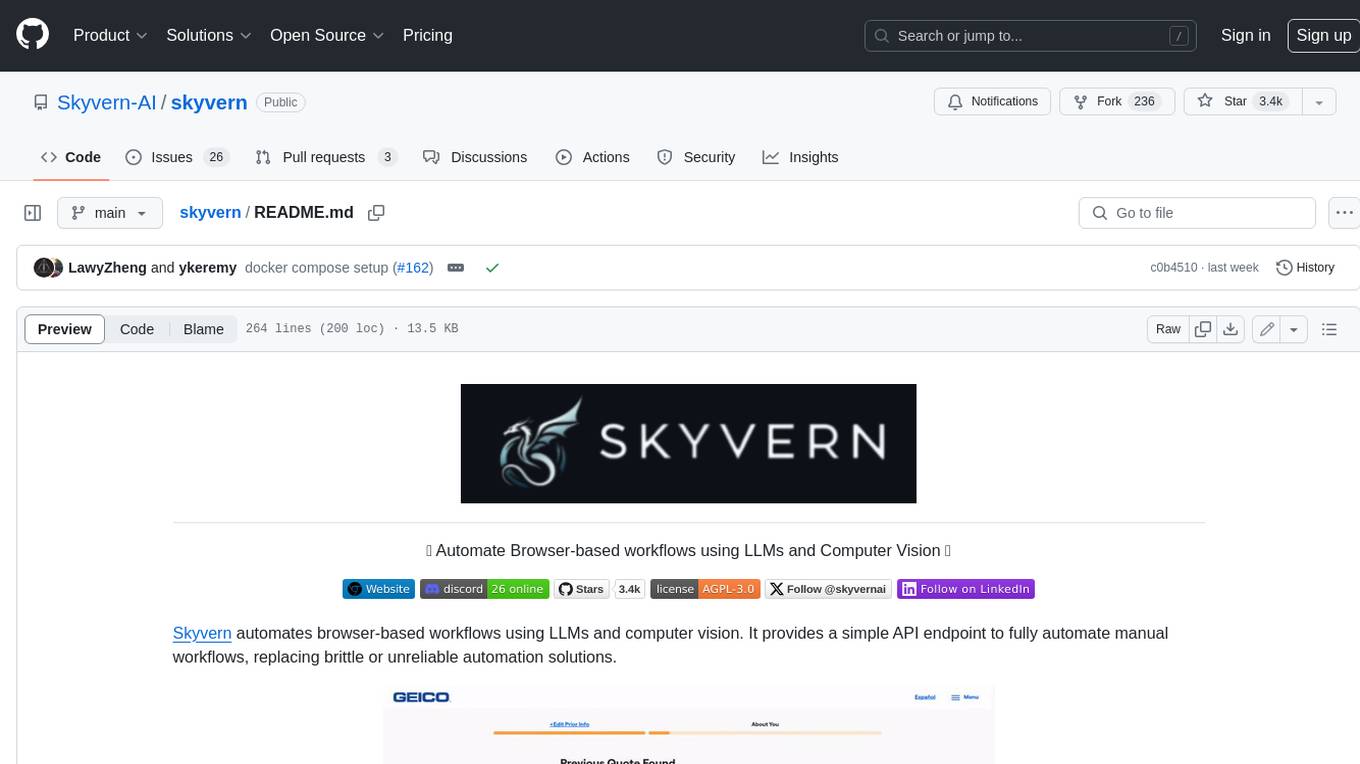
skyvern
Skyvern automates browser-based workflows using LLMs and computer vision. It provides a simple API endpoint to fully automate manual workflows, replacing brittle or unreliable automation solutions. Traditional approaches to browser automations required writing custom scripts for websites, often relying on DOM parsing and XPath-based interactions which would break whenever the website layouts changed. Instead of only relying on code-defined XPath interactions, Skyvern adds computer vision and LLMs to the mix to parse items in the viewport in real-time, create a plan for interaction and interact with them. This approach gives us a few advantages: 1. Skyvern can operate on websites it’s never seen before, as it’s able to map visual elements to actions necessary to complete a workflow, without any customized code 2. Skyvern is resistant to website layout changes, as there are no pre-determined XPaths or other selectors our system is looking for while trying to navigate 3. Skyvern leverages LLMs to reason through interactions to ensure we can cover complex situations. Examples include: 1. If you wanted to get an auto insurance quote from Geico, the answer to a common question “Were you eligible to drive at 18?” could be inferred from the driver receiving their license at age 16 2. If you were doing competitor analysis, it’s understanding that an Arnold Palmer 22 oz can at 7/11 is almost definitely the same product as a 23 oz can at Gopuff (even though the sizes are slightly different, which could be a rounding error!) Want to see examples of Skyvern in action? Jump to #real-world-examples-of- skyvern
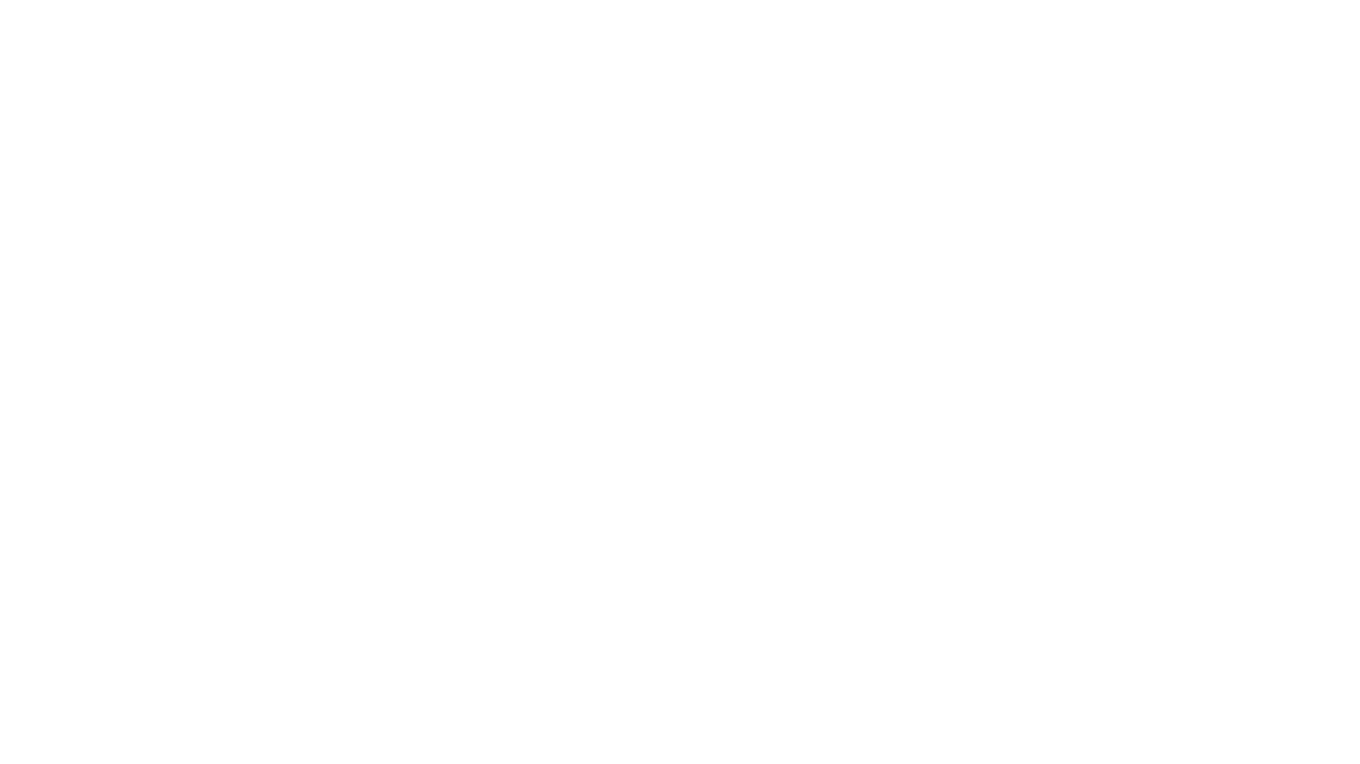
airbyte-connectors
This repository contains Airbyte connectors used in Faros and Faros Community Edition platforms as well as Airbyte Connector Development Kit (CDK) for JavaScript/TypeScript.
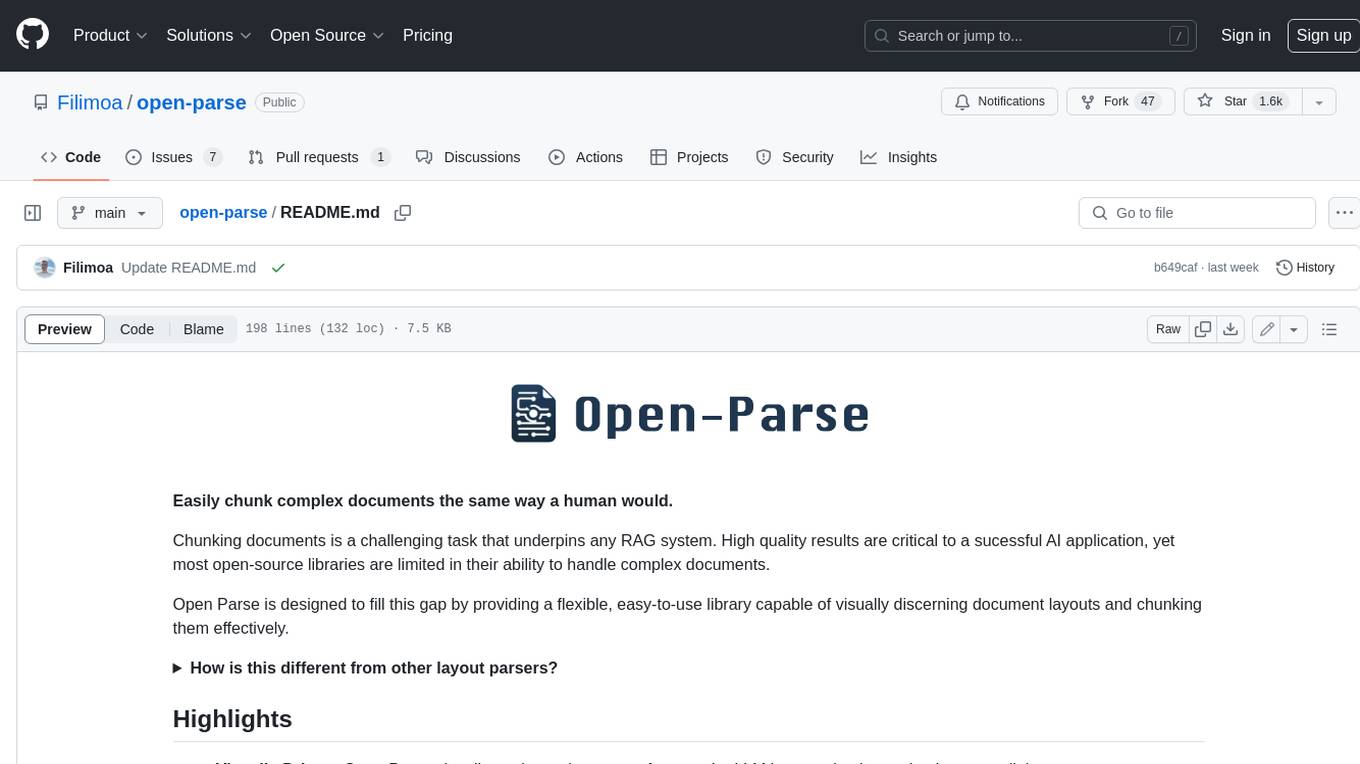
open-parse
Open Parse is a Python library for visually discerning document layouts and chunking them effectively. It is designed to fill the gap in open-source libraries for handling complex documents. Unlike text splitting, which converts a file to raw text and slices it up, Open Parse visually analyzes documents for superior LLM input. It also supports basic markdown for parsing headings, bold, and italics, and has high-precision table support, extracting tables into clean Markdown formats with accuracy that surpasses traditional tools. Open Parse is extensible, allowing users to easily implement their own post-processing steps. It is also intuitive, with great editor support and completion everywhere, making it easy to use and learn.
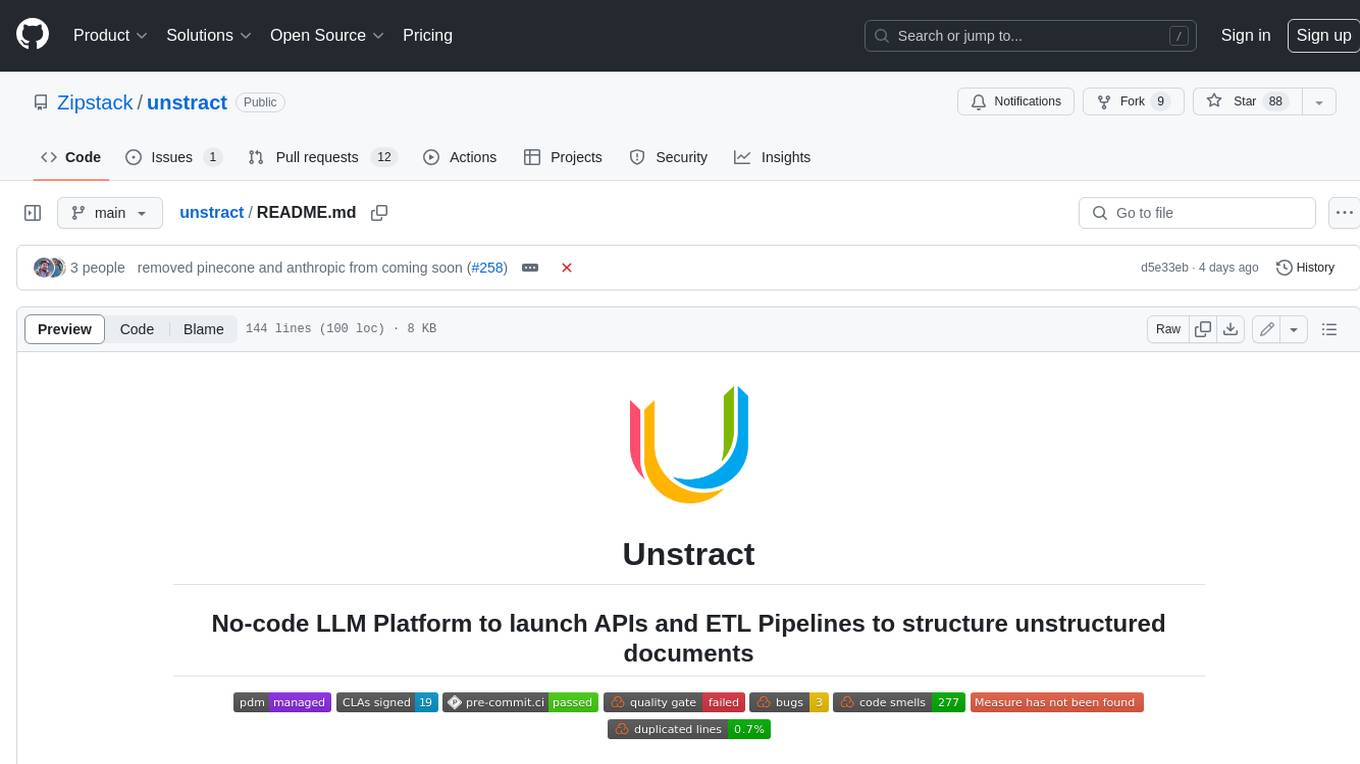
unstract
Unstract is a no-code platform that enables users to launch APIs and ETL pipelines to structure unstructured documents. With Unstract, users can go beyond co-pilots by enabling machine-to-machine automation. Unstract's Prompt Studio provides a simple, no-code approach to creating prompts for LLMs, vector databases, embedding models, and text extractors. Users can then configure Prompt Studio projects as API deployments or ETL pipelines to automate critical business processes that involve complex documents. Unstract supports a wide range of LLM providers, vector databases, embeddings, text extractors, ETL sources, and ETL destinations, providing users with the flexibility to choose the best tools for their needs.
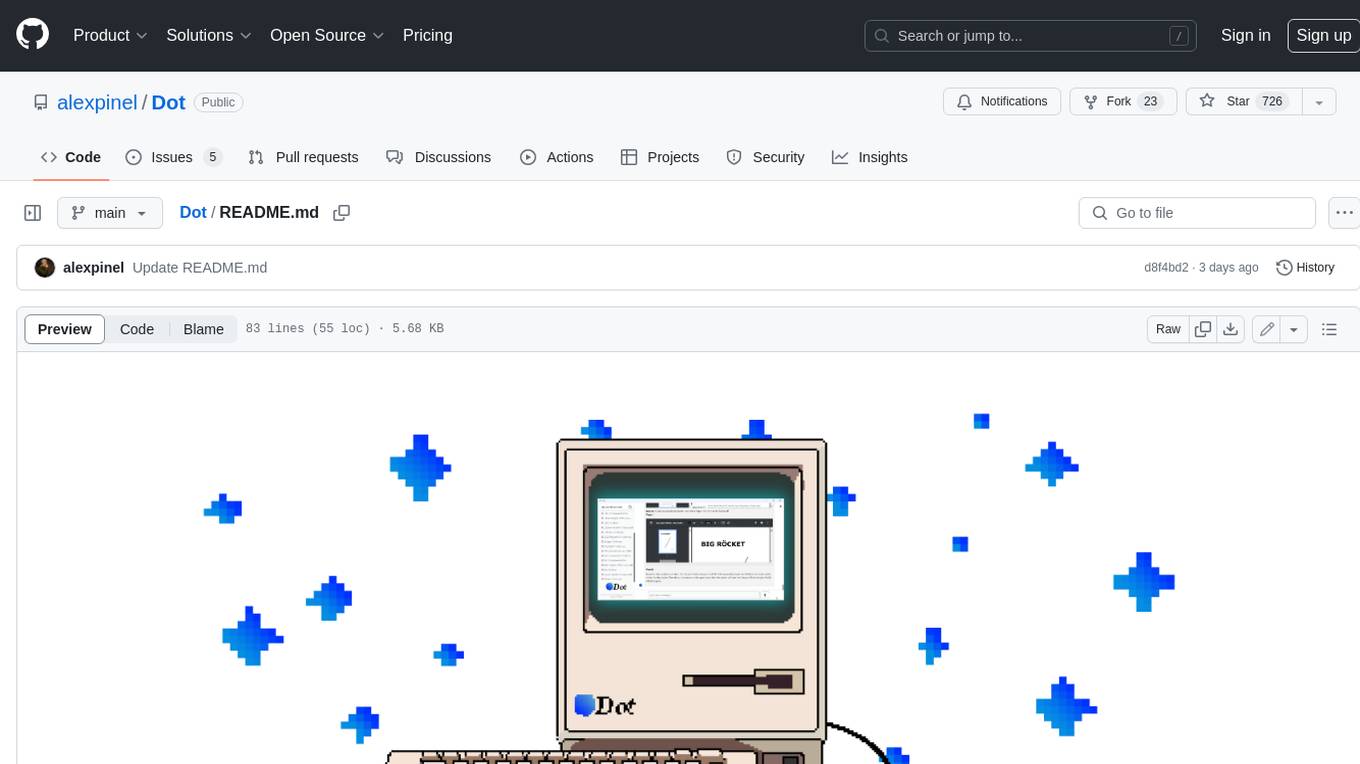
Dot
Dot is a standalone, open-source application designed for seamless interaction with documents and files using local LLMs and Retrieval Augmented Generation (RAG). It is inspired by solutions like Nvidia's Chat with RTX, providing a user-friendly interface for those without a programming background. Pre-packaged with Mistral 7B, Dot ensures accessibility and simplicity right out of the box. Dot allows you to load multiple documents into an LLM and interact with them in a fully local environment. Supported document types include PDF, DOCX, PPTX, XLSX, and Markdown. Users can also engage with Big Dot for inquiries not directly related to their documents, similar to interacting with ChatGPT. Built with Electron JS, Dot encapsulates a comprehensive Python environment that includes all necessary libraries. The application leverages libraries such as FAISS for creating local vector stores, Langchain, llama.cpp & Huggingface for setting up conversation chains, and additional tools for document management and interaction.
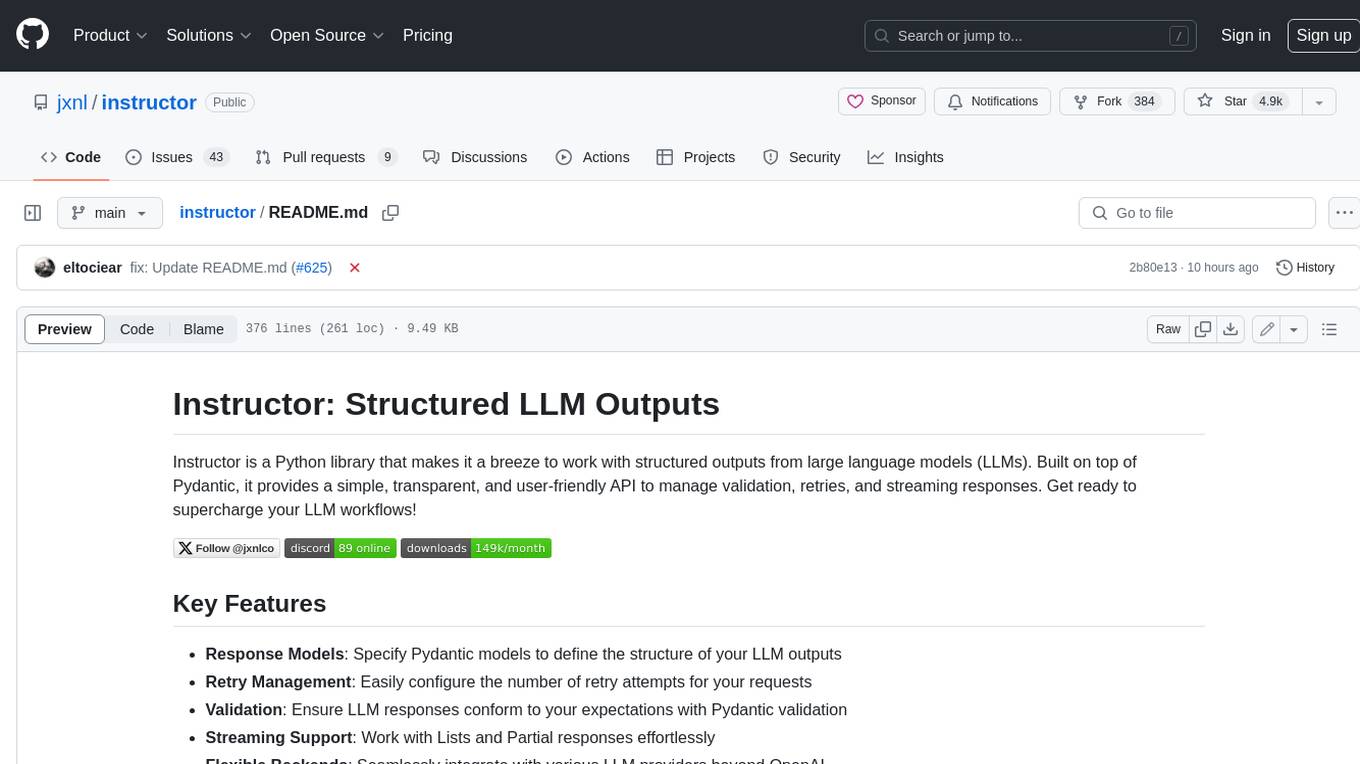
instructor
Instructor is a Python library that makes it a breeze to work with structured outputs from large language models (LLMs). Built on top of Pydantic, it provides a simple, transparent, and user-friendly API to manage validation, retries, and streaming responses. Get ready to supercharge your LLM workflows!
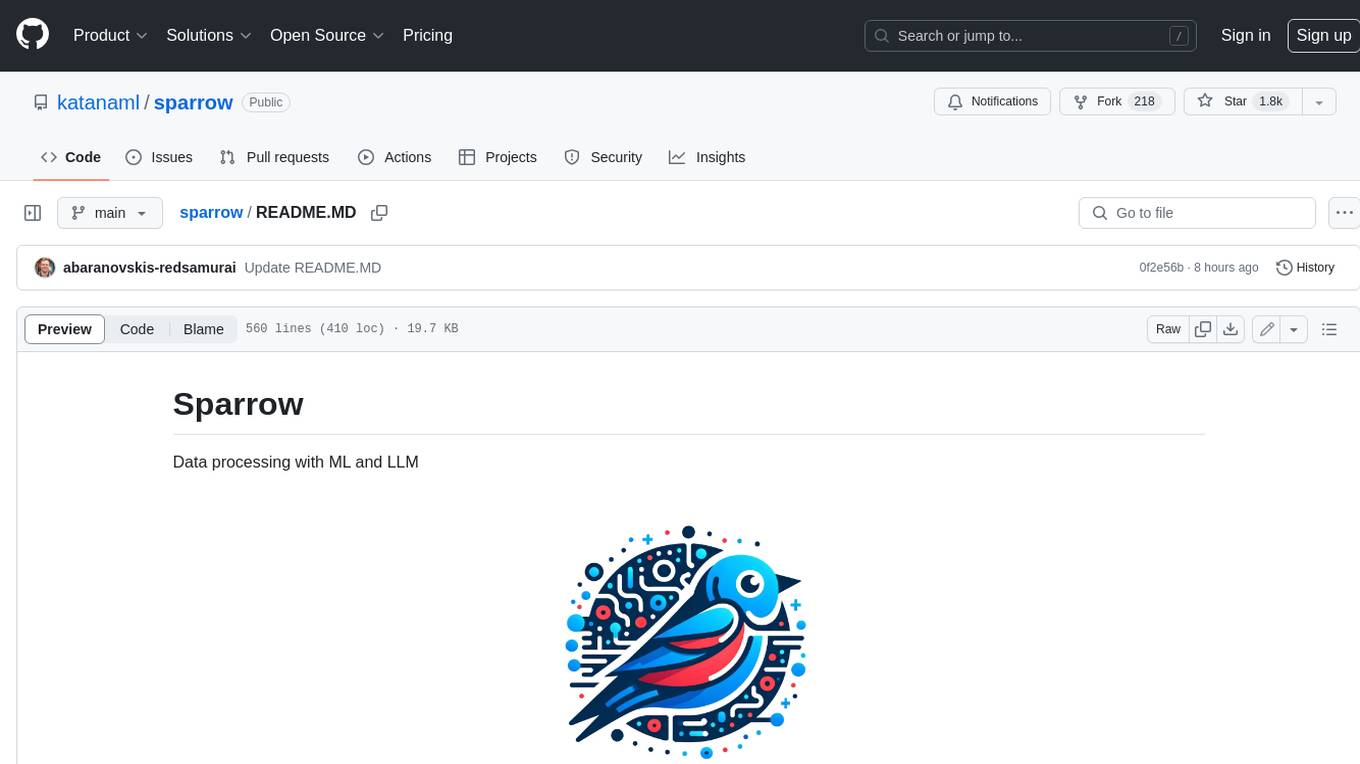
sparrow
Sparrow is an innovative open-source solution for efficient data extraction and processing from various documents and images. It seamlessly handles forms, invoices, receipts, and other unstructured data sources. Sparrow stands out with its modular architecture, offering independent services and pipelines all optimized for robust performance. One of the critical functionalities of Sparrow - pluggable architecture. You can easily integrate and run data extraction pipelines using tools and frameworks like LlamaIndex, Haystack, or Unstructured. Sparrow enables local LLM data extraction pipelines through Ollama or Apple MLX. With Sparrow solution you get API, which helps to process and transform your data into structured output, ready to be integrated with custom workflows. Sparrow Agents - with Sparrow you can build independent LLM agents, and use API to invoke them from your system. **List of available agents:** * **llamaindex** - RAG pipeline with LlamaIndex for PDF processing * **vllamaindex** - RAG pipeline with LLamaIndex multimodal for image processing * **vprocessor** - RAG pipeline with OCR and LlamaIndex for image processing * **haystack** - RAG pipeline with Haystack for PDF processing * **fcall** - Function call pipeline * **unstructured-light** - RAG pipeline with Unstructured and LangChain, supports PDF and image processing * **unstructured** - RAG pipeline with Weaviate vector DB query, Unstructured and LangChain, supports PDF and image processing * **instructor** - RAG pipeline with Unstructured and Instructor libraries, supports PDF and image processing. Works great for JSON response generation
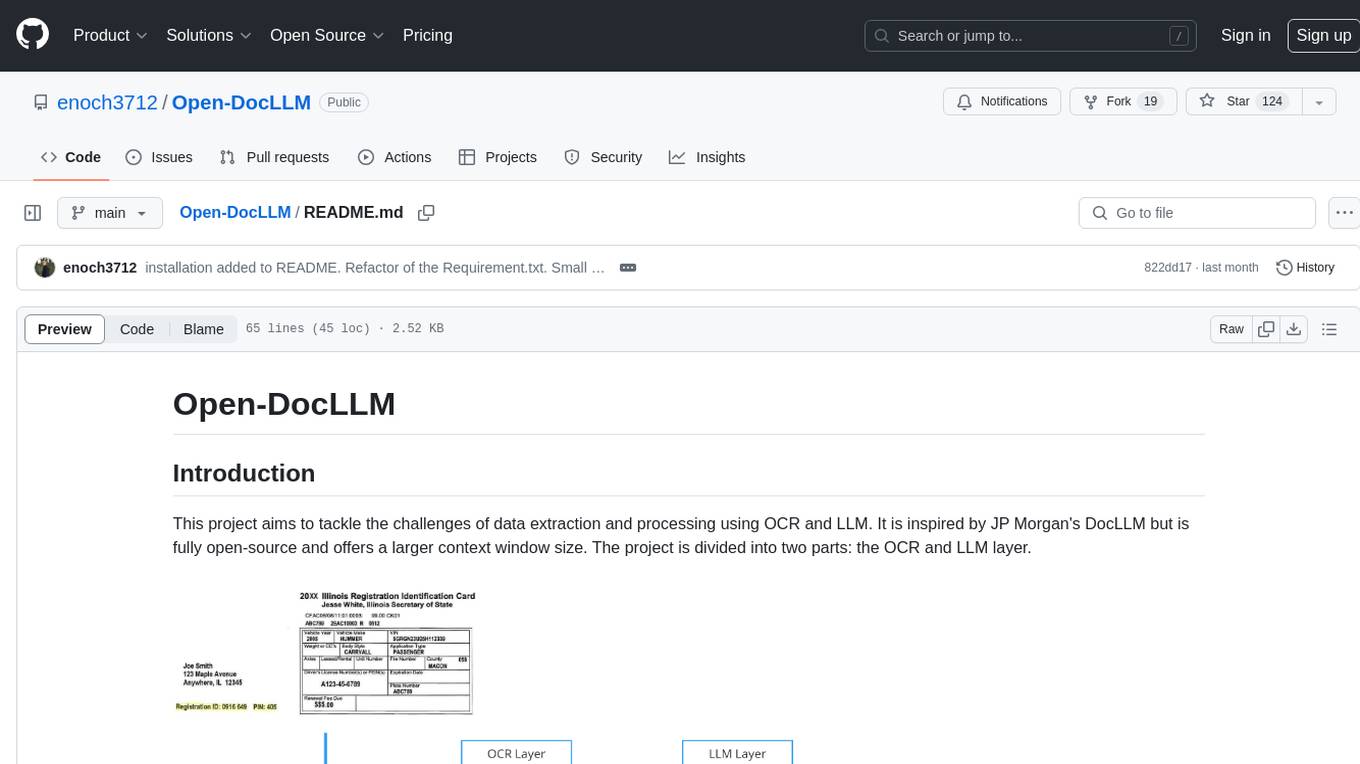
Open-DocLLM
Open-DocLLM is an open-source project that addresses data extraction and processing challenges using OCR and LLM technologies. It consists of two main layers: OCR for reading document content and LLM for extracting specific content in a structured manner. The project offers a larger context window size compared to JP Morgan's DocLLM and integrates tools like Tesseract OCR and Mistral for efficient data analysis. Users can run the models on-premises using LLM studio or Ollama, and the project includes a FastAPI app for testing purposes.
For similar jobs
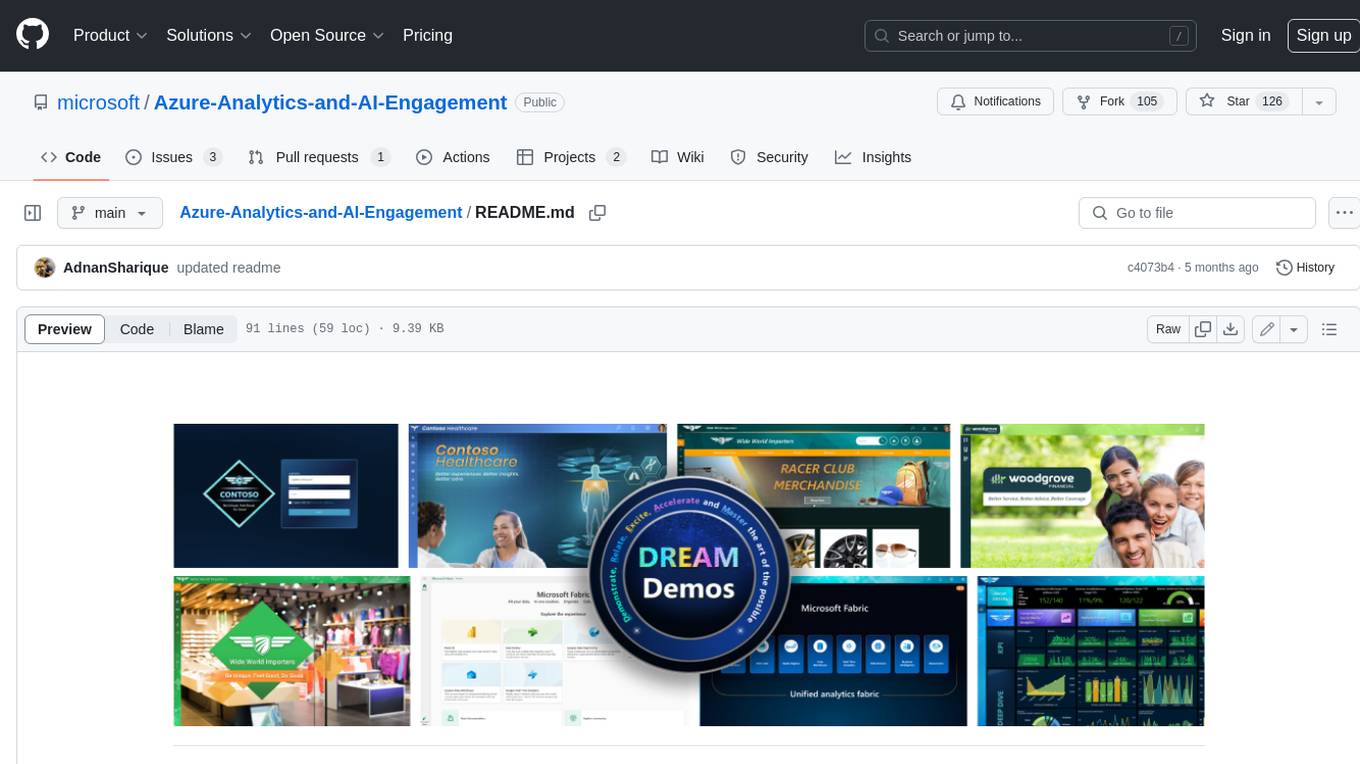
Azure-Analytics-and-AI-Engagement
The Azure-Analytics-and-AI-Engagement repository provides packaged Industry Scenario DREAM Demos with ARM templates (Containing a demo web application, Power BI reports, Synapse resources, AML Notebooks etc.) that can be deployed in a customer’s subscription using the CAPE tool within a matter of few hours. Partners can also deploy DREAM Demos in their own subscriptions using DPoC.
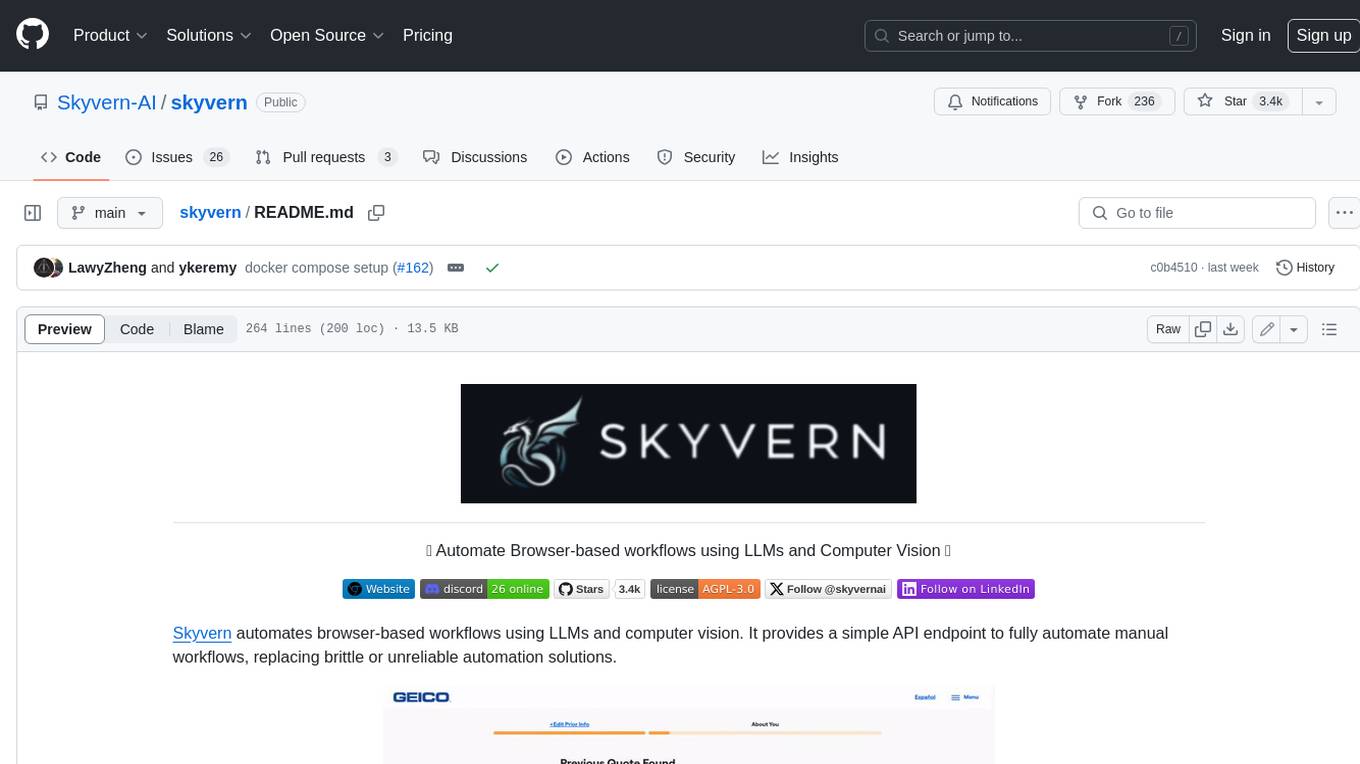
skyvern
Skyvern automates browser-based workflows using LLMs and computer vision. It provides a simple API endpoint to fully automate manual workflows, replacing brittle or unreliable automation solutions. Traditional approaches to browser automations required writing custom scripts for websites, often relying on DOM parsing and XPath-based interactions which would break whenever the website layouts changed. Instead of only relying on code-defined XPath interactions, Skyvern adds computer vision and LLMs to the mix to parse items in the viewport in real-time, create a plan for interaction and interact with them. This approach gives us a few advantages: 1. Skyvern can operate on websites it’s never seen before, as it’s able to map visual elements to actions necessary to complete a workflow, without any customized code 2. Skyvern is resistant to website layout changes, as there are no pre-determined XPaths or other selectors our system is looking for while trying to navigate 3. Skyvern leverages LLMs to reason through interactions to ensure we can cover complex situations. Examples include: 1. If you wanted to get an auto insurance quote from Geico, the answer to a common question “Were you eligible to drive at 18?” could be inferred from the driver receiving their license at age 16 2. If you were doing competitor analysis, it’s understanding that an Arnold Palmer 22 oz can at 7/11 is almost definitely the same product as a 23 oz can at Gopuff (even though the sizes are slightly different, which could be a rounding error!) Want to see examples of Skyvern in action? Jump to #real-world-examples-of- skyvern
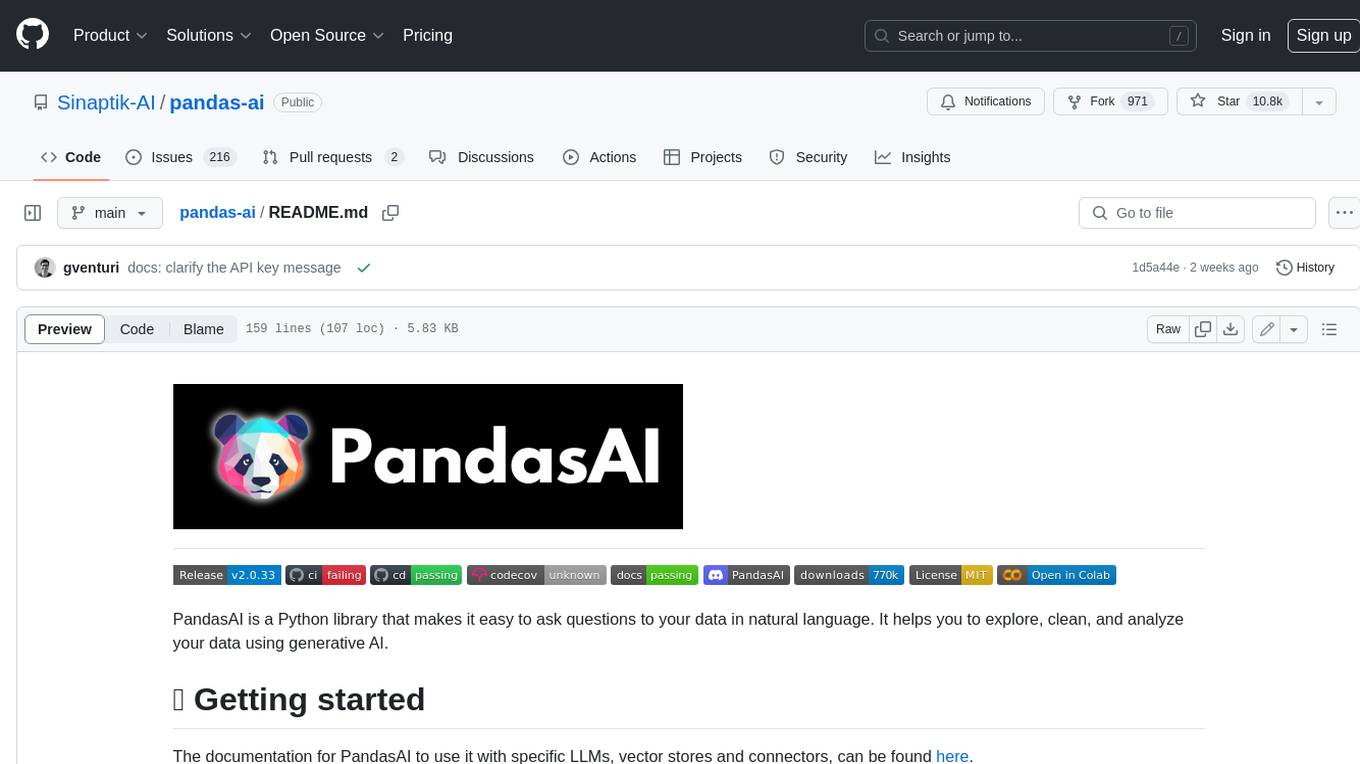
pandas-ai
PandasAI is a Python library that makes it easy to ask questions to your data in natural language. It helps you to explore, clean, and analyze your data using generative AI.
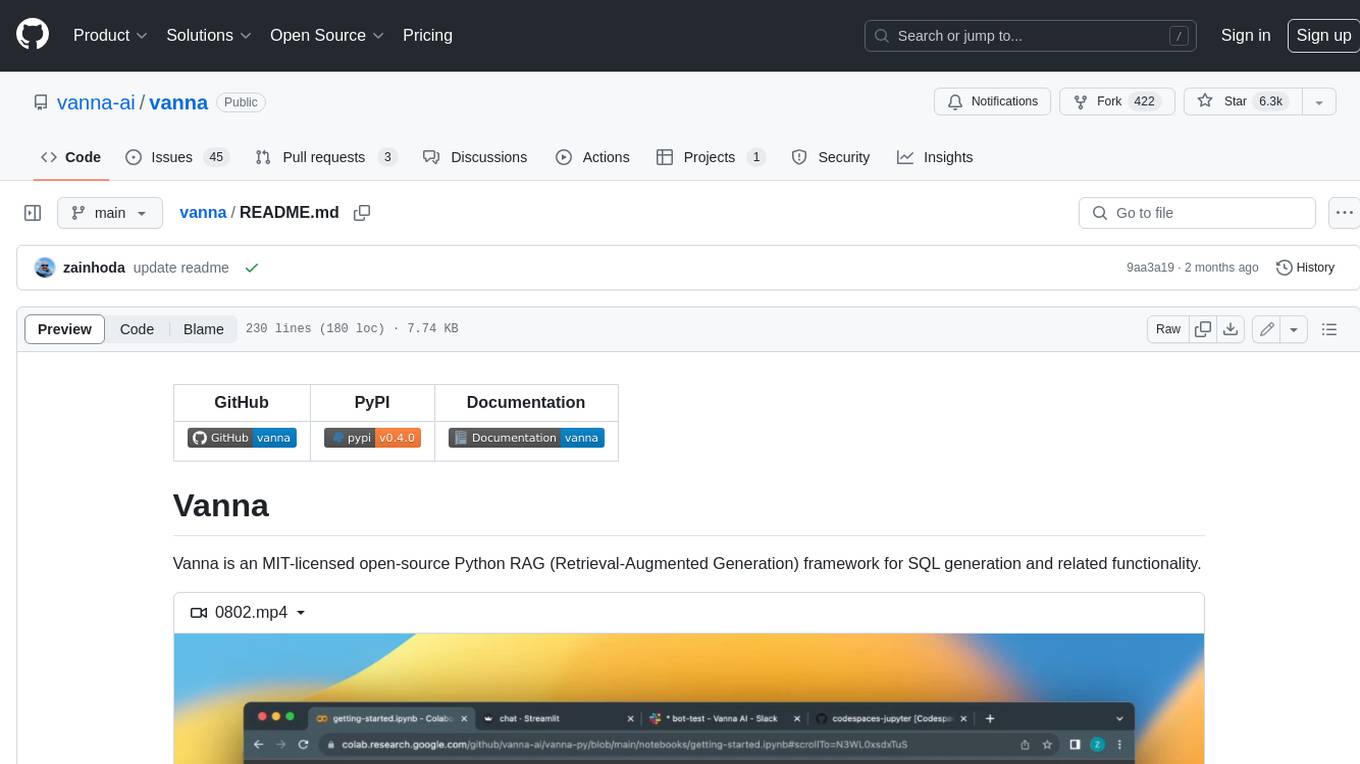
vanna
Vanna is an open-source Python framework for SQL generation and related functionality. It uses Retrieval-Augmented Generation (RAG) to train a model on your data, which can then be used to ask questions and get back SQL queries. Vanna is designed to be portable across different LLMs and vector databases, and it supports any SQL database. It is also secure and private, as your database contents are never sent to the LLM or the vector database.
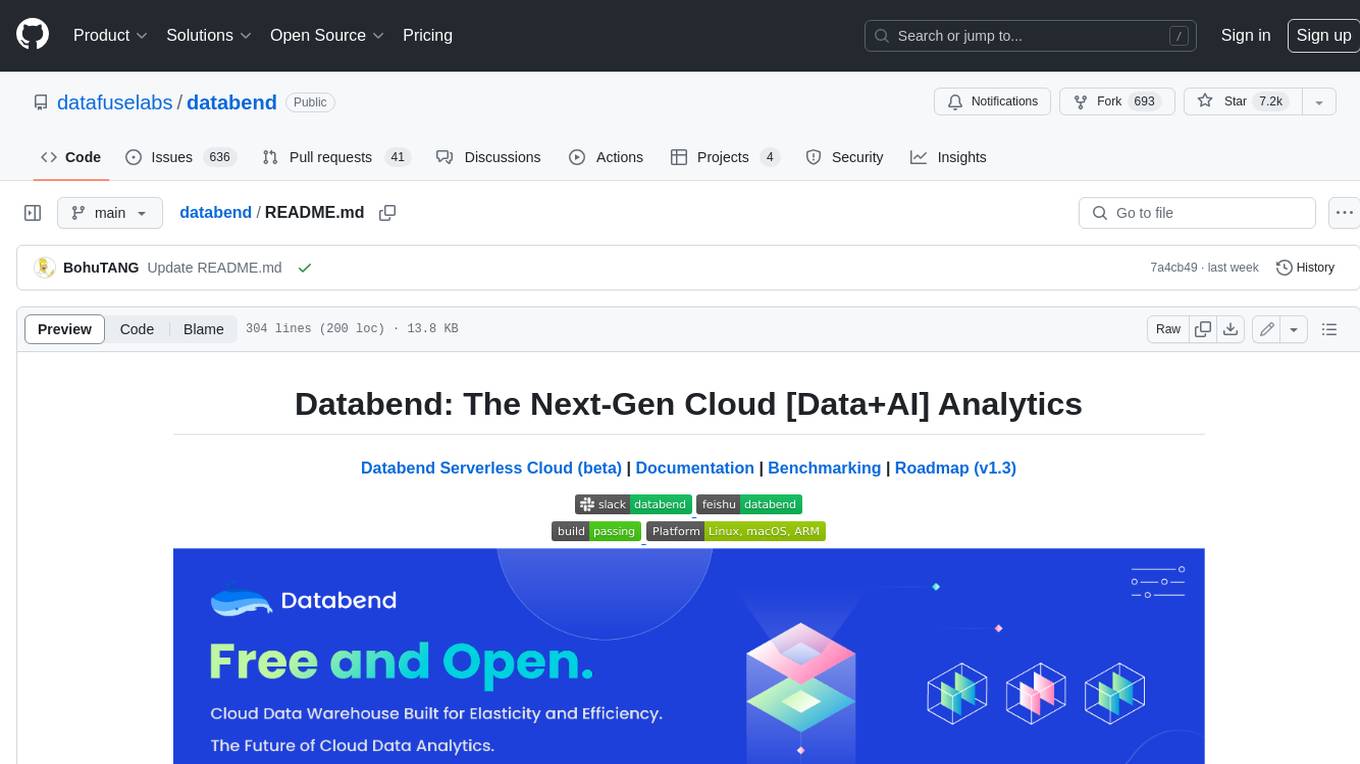
databend
Databend is an open-source cloud data warehouse that serves as a cost-effective alternative to Snowflake. With its focus on fast query execution and data ingestion, it's designed for complex analysis of the world's largest datasets.
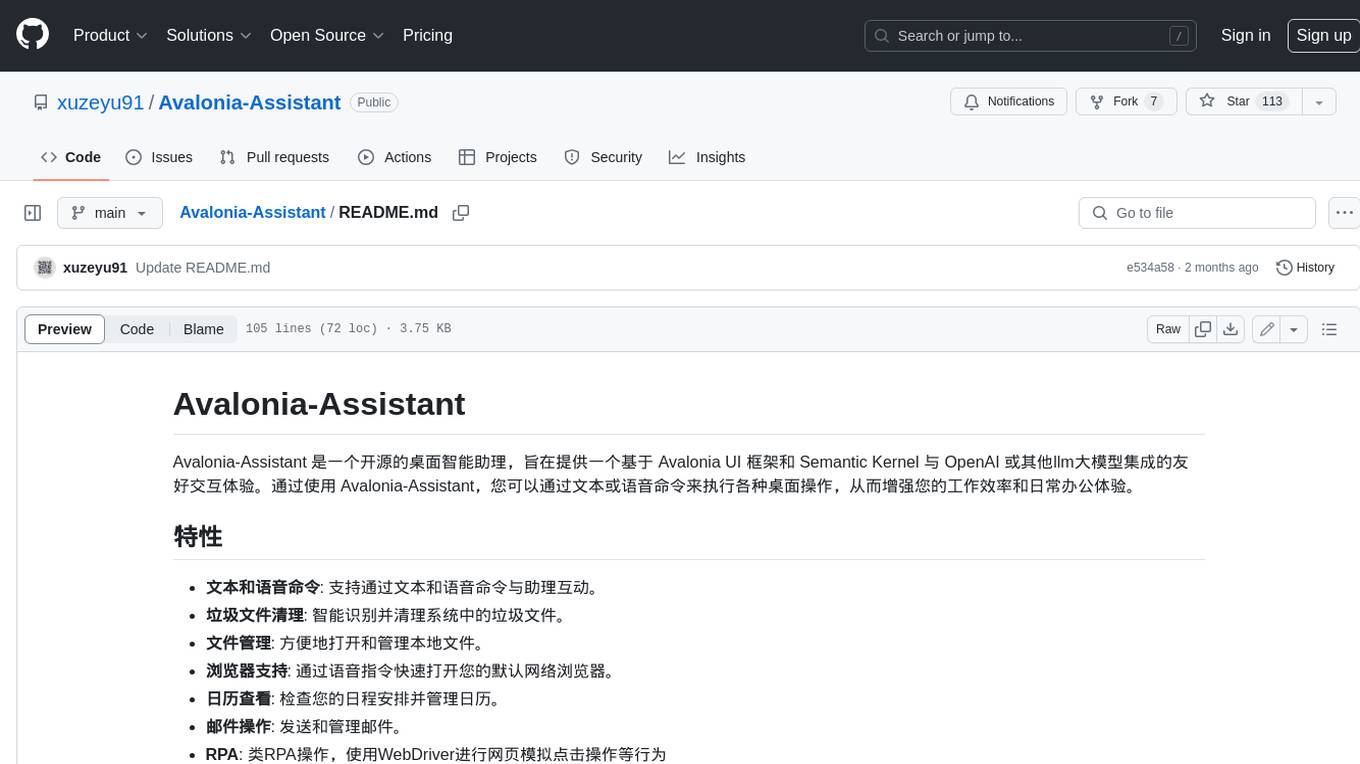
Avalonia-Assistant
Avalonia-Assistant is an open-source desktop intelligent assistant that aims to provide a user-friendly interactive experience based on the Avalonia UI framework and the integration of Semantic Kernel with OpenAI or other large LLM models. By utilizing Avalonia-Assistant, you can perform various desktop operations through text or voice commands, enhancing your productivity and daily office experience.
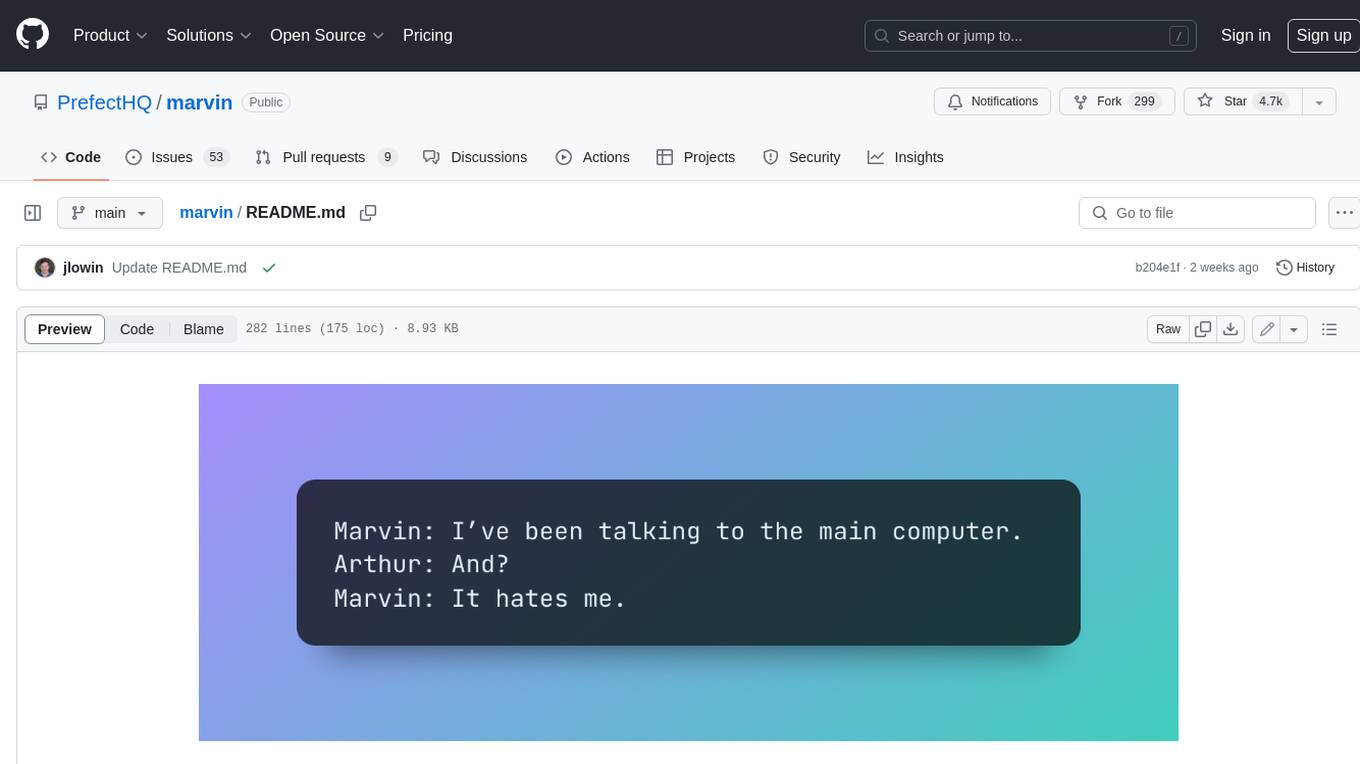
marvin
Marvin is a lightweight AI toolkit for building natural language interfaces that are reliable, scalable, and easy to trust. Each of Marvin's tools is simple and self-documenting, using AI to solve common but complex challenges like entity extraction, classification, and generating synthetic data. Each tool is independent and incrementally adoptable, so you can use them on their own or in combination with any other library. Marvin is also multi-modal, supporting both image and audio generation as well using images as inputs for extraction and classification. Marvin is for developers who care more about _using_ AI than _building_ AI, and we are focused on creating an exceptional developer experience. Marvin users should feel empowered to bring tightly-scoped "AI magic" into any traditional software project with just a few extra lines of code. Marvin aims to merge the best practices for building dependable, observable software with the best practices for building with generative AI into a single, easy-to-use library. It's a serious tool, but we hope you have fun with it. Marvin is open-source, free to use, and made with 💙 by the team at Prefect.
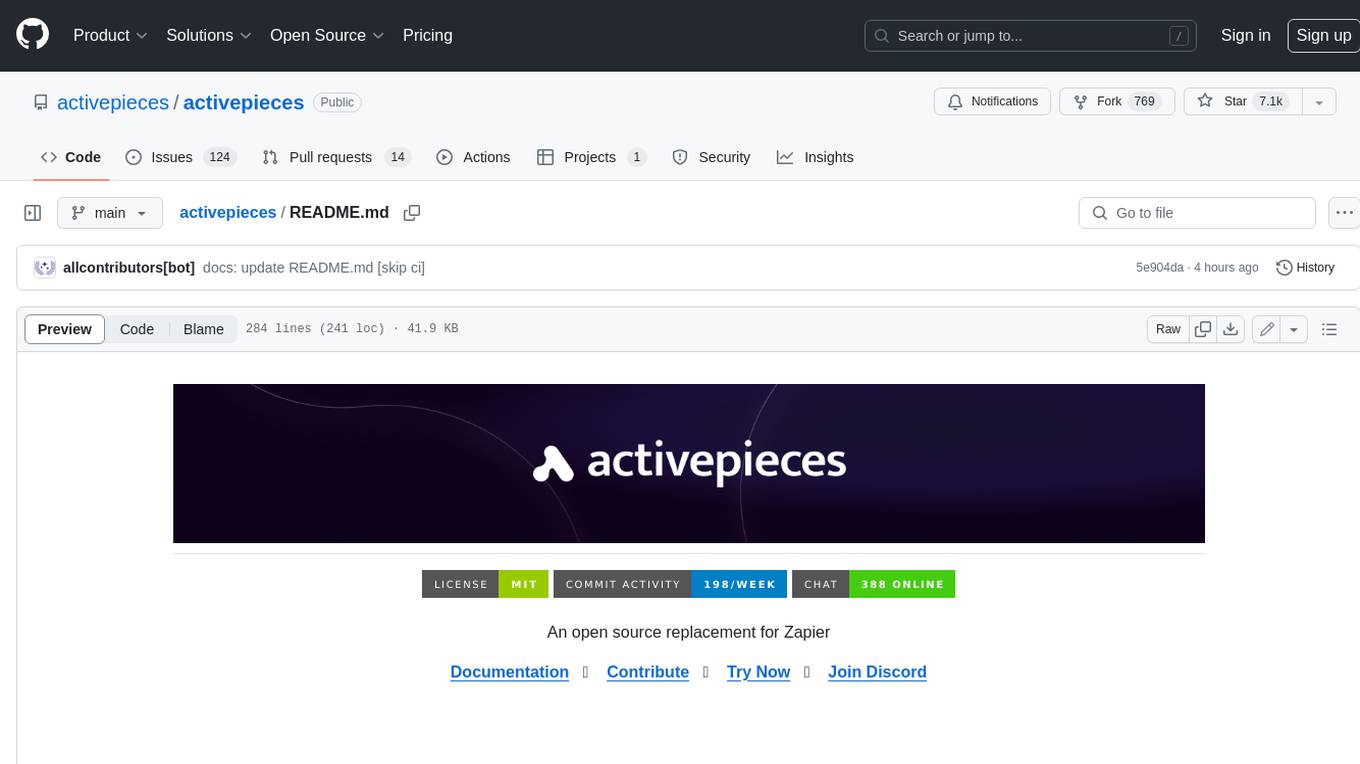
activepieces
Activepieces is an open source replacement for Zapier, designed to be extensible through a type-safe pieces framework written in Typescript. It features a user-friendly Workflow Builder with support for Branches, Loops, and Drag and Drop. Activepieces integrates with Google Sheets, OpenAI, Discord, and RSS, along with 80+ other integrations. The list of supported integrations continues to grow rapidly, thanks to valuable contributions from the community. Activepieces is an open ecosystem; all piece source code is available in the repository, and they are versioned and published directly to npmjs.com upon contributions. If you cannot find a specific piece on the pieces roadmap, please submit a request by visiting the following link: Request Piece Alternatively, if you are a developer, you can quickly build your own piece using our TypeScript framework. For guidance, please refer to the following guide: Contributor's Guide