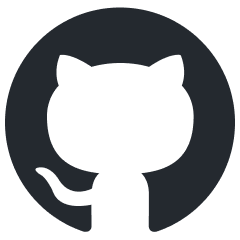
AI-Scientist
The AI Scientist: Towards Fully Automated Open-Ended Scientific Discovery 🧑🔬
Stars: 10244
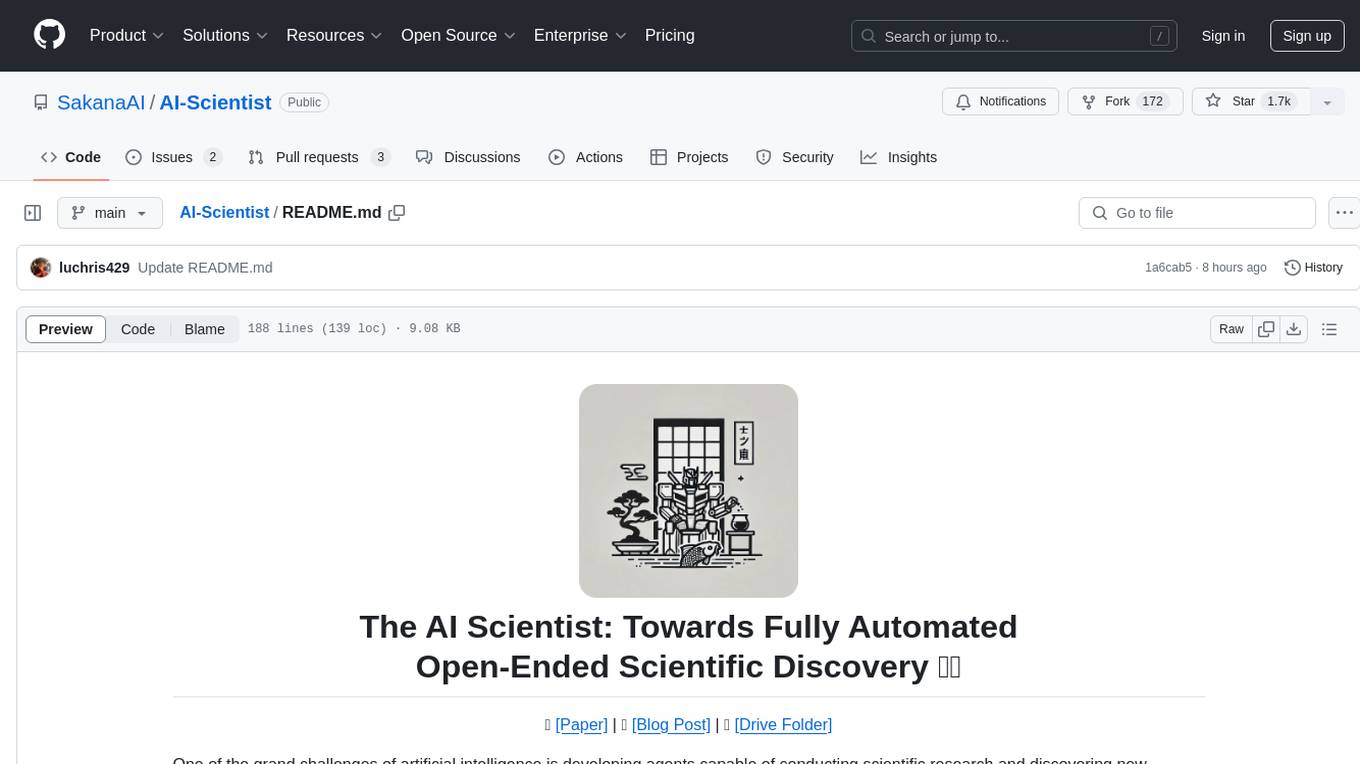
The AI Scientist is a comprehensive system for fully automatic scientific discovery, enabling Foundation Models to perform research independently. It aims to tackle the grand challenge of developing agents capable of conducting scientific research and discovering new knowledge. The tool generates papers on various topics using Large Language Models (LLMs) and provides a platform for exploring new research ideas. Users can create their own templates for specific areas of study and run experiments to generate papers. However, caution is advised as the codebase executes LLM-written code, which may pose risks such as the use of potentially dangerous packages and web access.
README:
📚 [Paper] | 📝 [Blog Post] | 📂 [Drive Folder]
One of the grand challenges of artificial intelligence is developing agents capable of conducting scientific research and discovering new knowledge. While frontier models have already been used to aid human scientists—for example, for brainstorming ideas or writing code—they still require extensive manual supervision or are heavily constrained to specific tasks.
We're excited to introduce The AI Scientist, the first comprehensive system for fully automatic scientific discovery, enabling Foundation Models such as Large Language Models (LLMs) to perform research independently.
We provide all runs and data from our paper here, where we run each base model on each template for approximately 50 ideas. We highly recommend reading through some of the Claude papers to get a sense of the system's strengths and weaknesses. Here are some example papers generated by The AI Scientist 📝:
- DualScale Diffusion: Adaptive Feature Balancing for Low-Dimensional Generative Models
- Multi-scale Grid Noise Adaptation: Enhancing Diffusion Models For Low-dimensional Data
- GAN-Enhanced Diffusion: Boosting Sample Quality and Diversity
- DualDiff: Enhancing Mode Capture in Low-dimensional Diffusion Models via Dual-expert Denoising
- StyleFusion: Adaptive Multi-style Generation in Character-Level Language Models
- Adaptive Learning Rates for Transformers via Q-Learning
- Unlocking Grokking: A Comparative Study of Weight Initialization Strategies in Transformer Models
- Grokking Accelerated: Layer-wise Learning Rates for Transformer Generalization
- Grokking Through Compression: Unveiling Sudden Generalization via Minimal Description Length
- Accelerating Mathematical Insight: Boosting Grokking Through Strategic Data Augmentation
Note:
Caution! This codebase will execute LLM-written code. There are various risks and challenges associated with this autonomy, including the use of potentially dangerous packages, web access, and potential spawning of processes. Use at your own discretion. Please make sure to containerize and restrict web access appropriately.
- Introduction
- Requirements
- Setting Up the Templates
- Run AI Scientist Paper Generation Experiments
- Getting an LLM-Generated Paper Review
- Making Your Own Template
- Template Resources
- Citing The AI Scientist
- Frequently Asked Questions
- Containerization
We provide three templates, which were used in our paper, covering the following domains: NanoGPT, 2D Diffusion, and Grokking. These templates enable The AI Scientist to generate ideas and conduct experiments in these areas. We accept contributions of new templates from the community, but please note that they are not maintained by us. All other templates beyond the three provided are community contributions.
This code is designed to run on Linux with NVIDIA GPUs using CUDA and PyTorch. Support for other GPU architectures may be possible by following the PyTorch guidelines. The current templates would likely take an infeasible amount of time on CPU-only machines. Running on other operating systems may require significant adjustments.
conda create -n ai_scientist python=3.11
conda activate ai_scientist
# Install pdflatex
sudo apt-get install texlive-full
# Install PyPI requirements
pip install -r requirements.txt
Note: Installing texlive-full
can take a long time. You may need to hold Enter during the installation.
We support a wide variety of models, including open-weight and API-only models. In general, we recommend using only frontier models above the capability of the original GPT-4. To see a full list of supported models, see here.
By default, this uses the OPENAI_API_KEY
environment variable.
By default, this uses the ANTHROPIC_API_KEY
environment variable.
For Claude models provided by Amazon Bedrock, please install these additional packages:
pip install anthropic[bedrock]
Next, specify a set of valid AWS Credentials and the target AWS Region:
Set the environment variables: AWS_ACCESS_KEY_ID
, AWS_SECRET_ACCESS_KEY
, AWS_REGION_NAME
.
For Claude models provided by Vertex AI Model Garden, please install these additional packages:
pip install google-cloud-aiplatform
pip install anthropic[vertex]
Next, set up valid authentication for a Google Cloud project, for example by providing the region and project ID:
export CLOUD_ML_REGION="REGION" # for Model Garden call
export ANTHROPIC_VERTEX_PROJECT_ID="PROJECT_ID" # for Model Garden call
export VERTEXAI_LOCATION="REGION" # for Aider/LiteLLM call
export VERTEXAI_PROJECT="PROJECT_ID" # for Aider/LiteLLM call
By default, this uses the DEEPSEEK_API_KEY
environment variable.
By default, this uses the OPENROUTER_API_KEY
environment variable.
We support Google Gemini models (e.g., "gemini-1.5-flash", "gemini-1.5-pro") via the google-generativeai Python library. By default, it uses the environment variable:
export GEMINI_API_KEY="YOUR GEMINI API KEY"
Our code can also optionally use a Semantic Scholar API Key (S2_API_KEY
) for higher throughput if you have one, though it should work without it in principle. If you have problems with Semantic Scholar, you can skip the literature search and citation phases of paper generation.
Be sure to provide the key for the model used for your runs, e.g.:
export OPENAI_API_KEY="YOUR KEY HERE"
export S2_API_KEY="YOUR KEY HERE"
OpenAlex API can be used as an alternative if you do not have a Semantic Scholar API Key. OpenAlex does not require API key.
pip install pyalex
export OPENALEX_MAIL_ADDRESS="YOUR EMAIL ADDRESS"
And specify --engine openalex
when you execute the AI Scientist code.
Note that this is experimental for those who do not have a Semantic Scholar API Key.
This section provides instructions for setting up each of the three templates used in our paper. Before running The AI Scientist experiments, please ensure you have completed the setup steps for the templates you are interested in.
Description: This template investigates transformer-based autoregressive next-token prediction tasks.
Setup Steps:
-
Prepare the data:
python data/enwik8/prepare.py python data/shakespeare_char/prepare.py python data/text8/prepare.py
-
Create baseline runs (machine dependent):
# Set up NanoGPT baseline run # NOTE: YOU MUST FIRST RUN THE PREPARE SCRIPTS ABOVE! cd templates/nanoGPT python experiment.py --out_dir run_0 python plot.py
Description: This template studies improving the performance of diffusion generative models on low-dimensional datasets.
Setup Steps:
-
Install dependencies:
# Set up 2D Diffusion git clone https://github.com/gregversteeg/NPEET.git cd NPEET pip install . pip install scikit-learn
-
Create baseline runs:
# Set up 2D Diffusion baseline run cd templates/2d_diffusion python experiment.py --out_dir run_0 python plot.py
Description: This template investigates questions about generalization and learning speed in deep neural networks.
Setup Steps:
-
Install dependencies:
# Set up Grokking pip install einops
-
Create baseline runs:
# Set up Grokking baseline run cd templates/grokking python experiment.py --out_dir run_0 python plot.py
Note: Please ensure the setup steps above are completed before running these experiments.
conda activate ai_scientist
# Run the paper generation.
python launch_scientist.py --model "gpt-4o-2024-05-13" --experiment nanoGPT_lite --num-ideas 2
python launch_scientist.py --model "claude-3-5-sonnet-20241022" --experiment nanoGPT_lite --num-ideas 2
If you have more than one GPU, use the --parallel
option to parallelize ideas across multiple GPUs.
import openai
from ai_scientist.perform_review import load_paper, perform_review
client = openai.OpenAI()
model = "gpt-4o-2024-05-13"
# Load paper from PDF file (raw text)
paper_txt = load_paper("report.pdf")
# Get the review dictionary
review = perform_review(
paper_txt,
model,
client,
num_reflections=5,
num_fs_examples=1,
num_reviews_ensemble=5,
temperature=0.1,
)
# Inspect review results
review["Overall"] # Overall score (1-10)
review["Decision"] # 'Accept' or 'Reject'
review["Weaknesses"] # List of weaknesses (strings)
To run batch analysis:
cd review_iclr_bench
python iclr_analysis.py --num_reviews 500 --batch_size 100 --num_fs_examples 1 --num_reflections 5 --temperature 0.1 --num_reviews_ensemble 5
If there is an area of study you would like The AI Scientist to explore, it is straightforward to create your own templates. In general, follow the structure of the existing templates, which consist of:
-
experiment.py
— This is the main script where the core content is. It takes an argument--out_dir
, which specifies where it should create the folder and save the relevant information from the run. -
plot.py
— This script takes the information from therun
folders and creates plots. The code should be clear and easy to edit. -
prompt.json
— Put information about your template here. -
seed_ideas.json
— Place example ideas here. You can also try to generate ideas without any examples and then pick the best one or two to put here. -
latex/template.tex
— We recommend using our LaTeX folder but be sure to replace the pre-loaded citations with ones that you expect to be more relevant.
The key to making new templates work is matching the base filenames and output JSONs to the existing format; everything else is free to change.
You should also ensure that the template.tex
file is updated to use the correct citation style / base plots for your template.
We welcome community contributions in the form of new templates. While these are not maintained by us, we are delighted to highlight your templates to others. Below, we list community-contributed templates along with links to their pull requests (PRs):
- Infectious Disease Modeling (
seir
) - PR #137 - Image Classification with MobileNetV3 (
mobilenetV3
) - PR #141 - Sketch RNN (
sketch_rnn
) - PR #143 - AI in Quantum Chemistry (
MACE
) - PR#157 - Earthquake Prediction (
earthquake-prediction
) - PR #167 - Tensorial Radiance Fields (
tensorf
) - PR #175
This section is reserved for community contributions. Please submit a pull request to add your template to the list! Please describe the template in the PR description, and also show examples of the generated papers.
We provide three templates, which heavily use code from other repositories, credited below:
- NanoGPT Template uses code from NanoGPT and this PR.
- 2D Diffusion Template uses code from tiny-diffusion, ema-pytorch, and Datasaur.
- Grokking Template uses code from Sea-Snell/grokking and danielmamay/grokking.
We would like to thank the developers of the open-source models and packages for their contributions and for making their work available.
If you use The AI Scientist in your research, please cite it as follows:
@article{lu2024aiscientist,
title={The {AI} {S}cientist: Towards Fully Automated Open-Ended Scientific Discovery},
author={Lu, Chris and Lu, Cong and Lange, Robert Tjarko and Foerster, Jakob and Clune, Jeff and Ha, David},
journal={arXiv preprint arXiv:2408.06292},
year={2024}
}
We recommend reading our paper first for any questions you have on The AI Scientist.
Why am I missing files when running The AI Scientist?
Ensure you have completed all the setup and preparation steps before the main experiment script.
Why has a PDF or a review not been generated?
The AI Scientist finishes an idea with a success rate that depends on the template, the base foundation model, and the complexity of the idea. We advise referring to our main paper. The highest success rates are observed with Claude Sonnet 3.5. Reviews are best done with GPT-4o; all other models have issues with positivity bias or failure to conform to required outputs.
What is the cost of each idea generated?
Typically less than $15 per paper with Claude Sonnet 3.5. We recommend DeepSeek Coder V2 for a much more cost-effective approach. A good place to look for new models is the Aider leaderboard.
How do I change the base conference format associated with the write-ups?
Change the base template.tex
files contained within each template.
How do I run The AI Scientist for different subject fields?
Please refer to the instructions for different templates. In this current iteration, this is restricted to ideas that can be expressed in code. However, lifting this restriction would represent exciting future work! :)
How do I add support for a new foundation model?
You may modify ai_scientist/llm.py
to add support for a new foundation model. We do not advise using any model that is significantly weaker than GPT-4 level for The AI Scientist.
Why do I need to run the baseline runs myself?
These appear as run_0
and should be run per machine you execute The AI Scientist on for accurate run-time comparisons due to hardware differences.
What if I have problems accessing the Semantic Scholar API?
We use the Semantic Scholar API to check ideas for novelty and collect citations for the paper write-up. You may be able to skip these phases if you don't have an API key or the API is slow to access.
We include a community-contributed Docker image that may assist with your containerization efforts in experimental/Dockerfile
.
You can use this image like this:
# Endpoint Script
docker run -e OPENAI_API_KEY=$OPENAI_API_KEY -v `pwd`/templates:/app/AI-Scientist/templates <AI_SCIENTIST_IMAGE> \
--model gpt-4o-2024-05-13 \
--experiment 2d_diffusion \
--num-ideas 2
# Interactive
docker run -it -e OPENAI_API_KEY=$OPENAI_API_KEY \
--entrypoint /bin/bash \
<AI_SCIENTIST_IMAGE>
For Tasks:
Click tags to check more tools for each tasksFor Jobs:
Alternative AI tools for AI-Scientist
Similar Open Source Tools
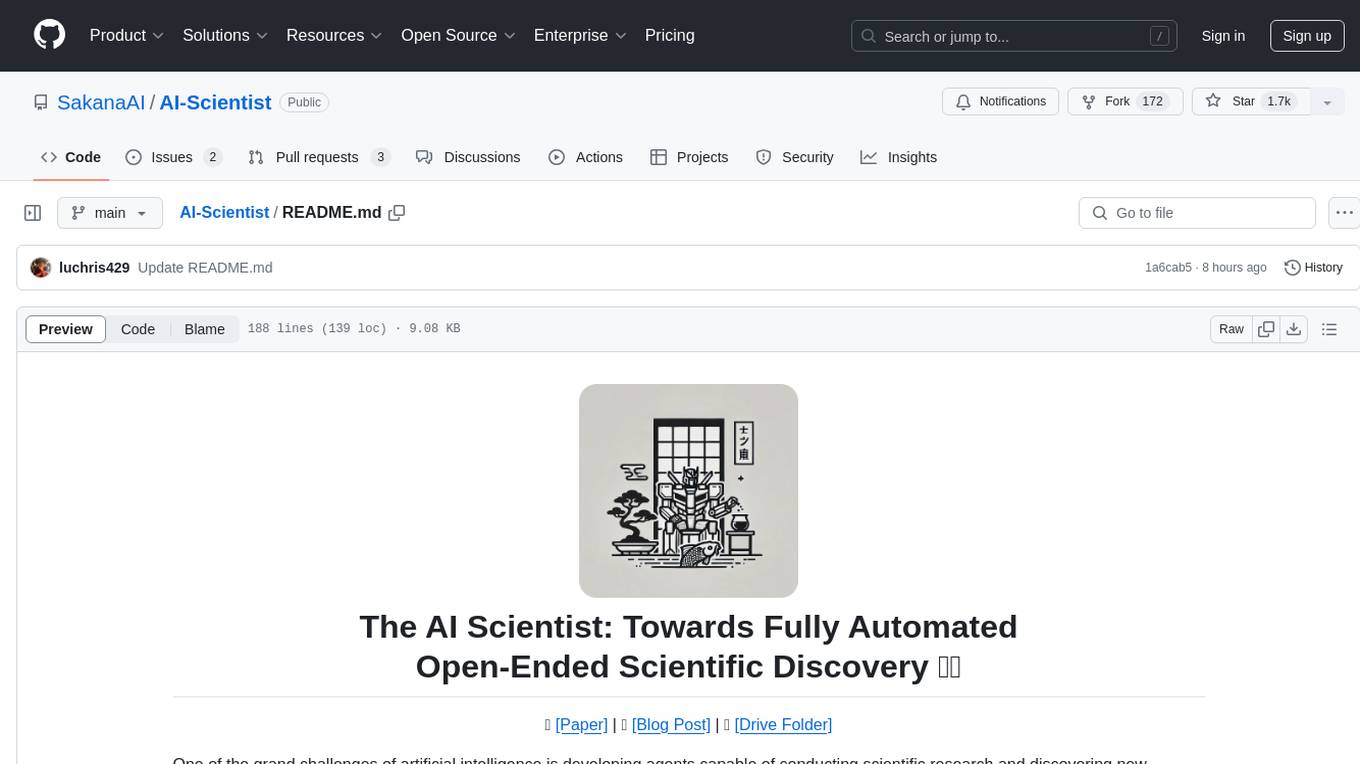
AI-Scientist
The AI Scientist is a comprehensive system for fully automatic scientific discovery, enabling Foundation Models to perform research independently. It aims to tackle the grand challenge of developing agents capable of conducting scientific research and discovering new knowledge. The tool generates papers on various topics using Large Language Models (LLMs) and provides a platform for exploring new research ideas. Users can create their own templates for specific areas of study and run experiments to generate papers. However, caution is advised as the codebase executes LLM-written code, which may pose risks such as the use of potentially dangerous packages and web access.
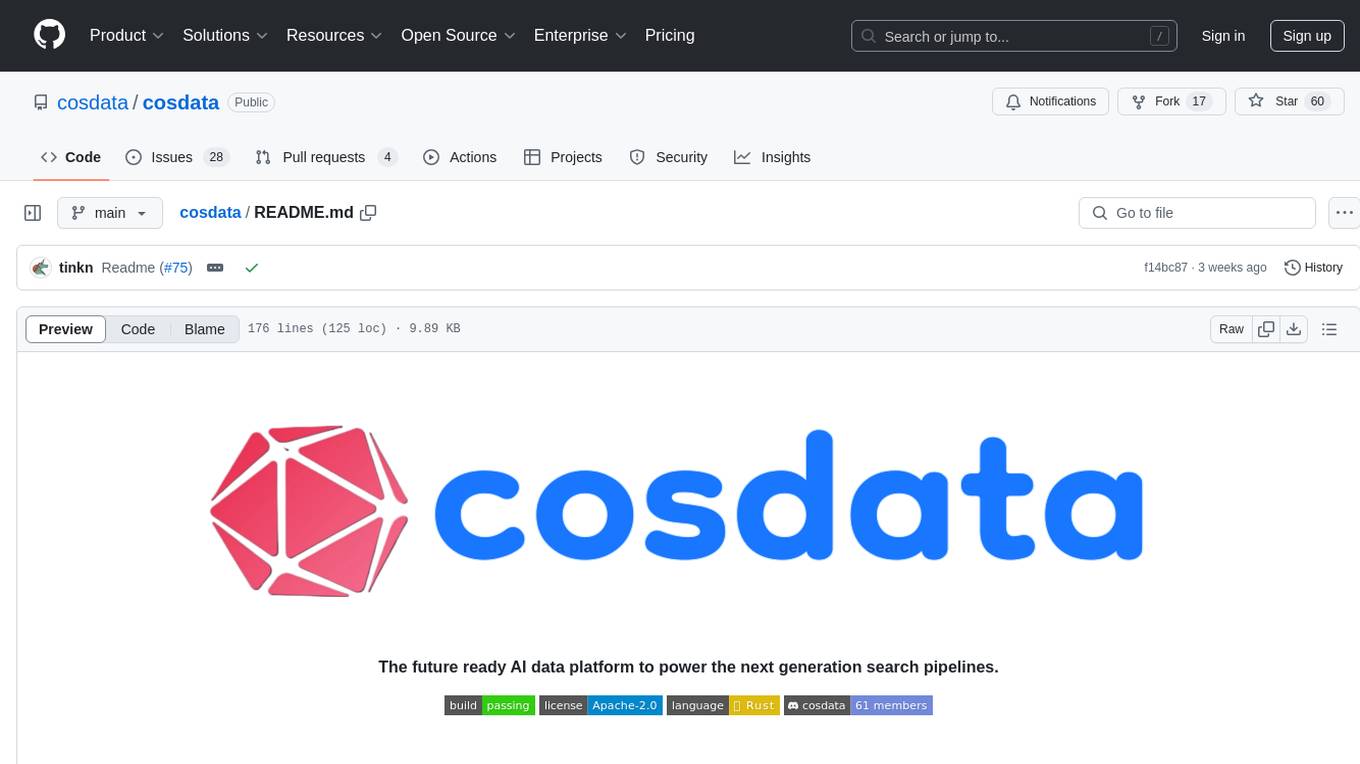
cosdata
Cosdata is a cutting-edge AI data platform designed to power the next generation search pipelines. It features immutability, version control, and excels in semantic search, structured knowledge graphs, hybrid search capabilities, real-time search at scale, and ML pipeline integration. The platform is customizable, scalable, efficient, enterprise-grade, easy to use, and can manage multi-modal data. It offers high performance, indexing, low latency, and high requests per second. Cosdata is designed to meet the demands of modern search applications, empowering businesses to harness the full potential of their data.
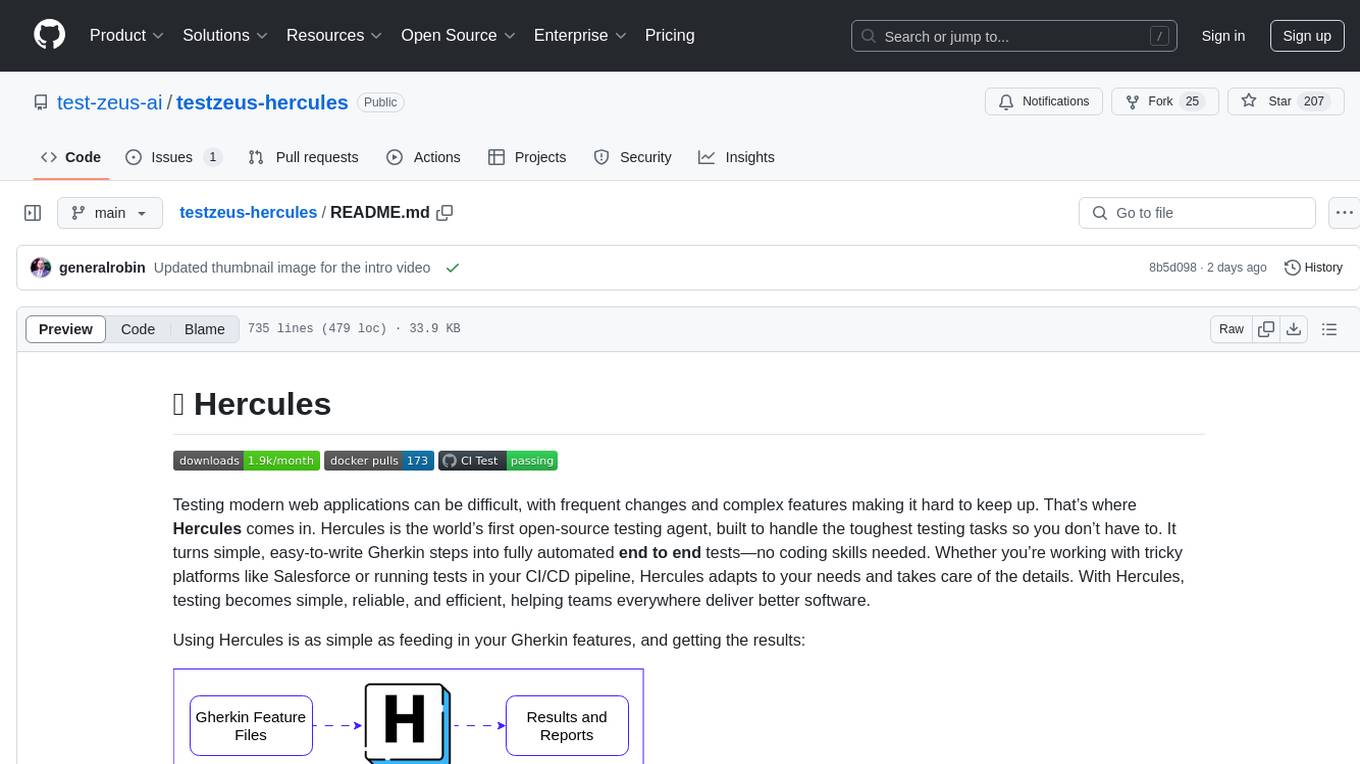
testzeus-hercules
Hercules is the world’s first open-source testing agent designed to handle the toughest testing tasks for modern web applications. It turns simple Gherkin steps into fully automated end-to-end tests, making testing simple, reliable, and efficient. Hercules adapts to various platforms like Salesforce and is suitable for CI/CD pipelines. It aims to democratize and disrupt test automation, making top-tier testing accessible to everyone. The tool is transparent, reliable, and community-driven, empowering teams to deliver better software. Hercules offers multiple ways to get started, including using PyPI package, Docker, or building and running from source code. It supports various AI models, provides detailed installation and usage instructions, and integrates with Nuclei for security testing and WCAG for accessibility testing. The tool is production-ready, open core, and open source, with plans for enhanced LLM support, advanced tooling, improved DOM distillation, community contributions, extensive documentation, and a bounty program.
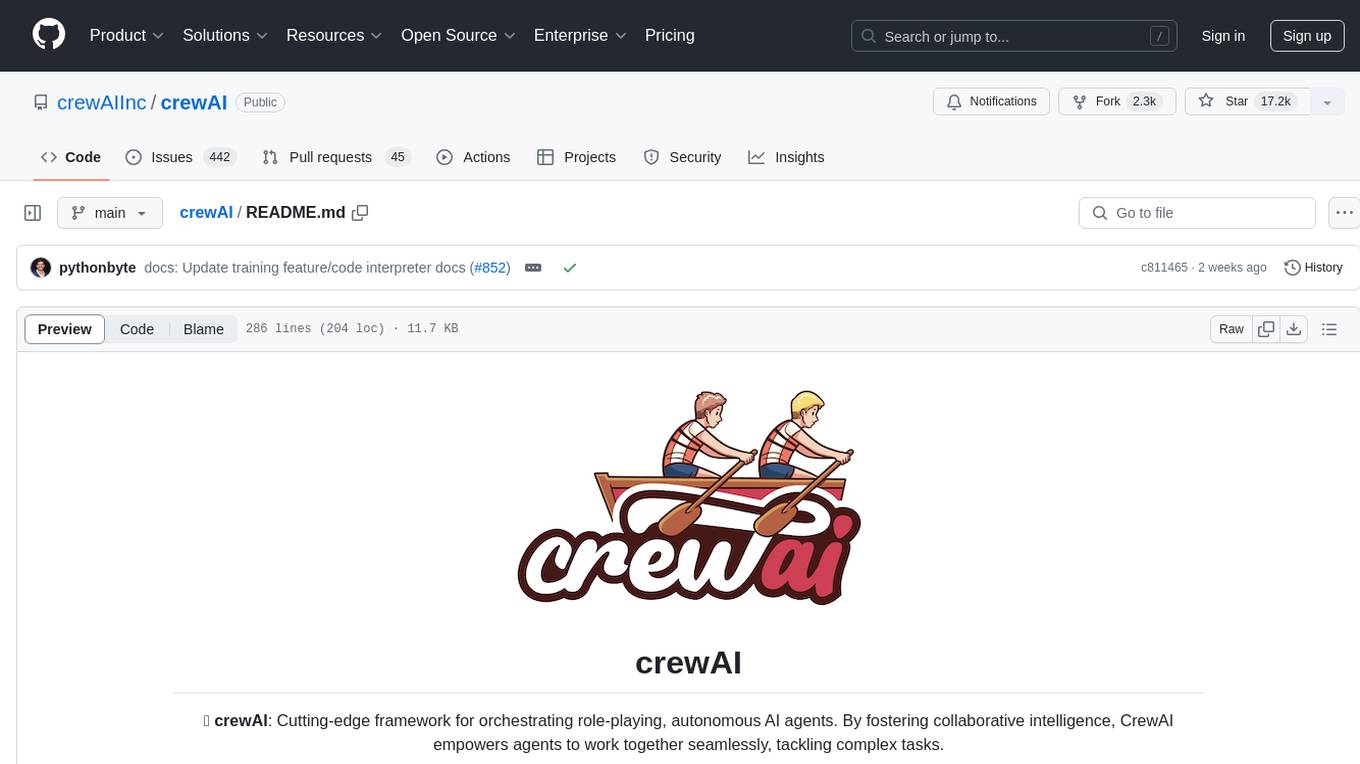
crewAI
CrewAI is a cutting-edge framework designed to orchestrate role-playing autonomous AI agents. By fostering collaborative intelligence, CrewAI empowers agents to work together seamlessly, tackling complex tasks. It enables AI agents to assume roles, share goals, and operate in a cohesive unit, much like a well-oiled crew. Whether you're building a smart assistant platform, an automated customer service ensemble, or a multi-agent research team, CrewAI provides the backbone for sophisticated multi-agent interactions. With features like role-based agent design, autonomous inter-agent delegation, flexible task management, and support for various LLMs, CrewAI offers a dynamic and adaptable solution for both development and production workflows.
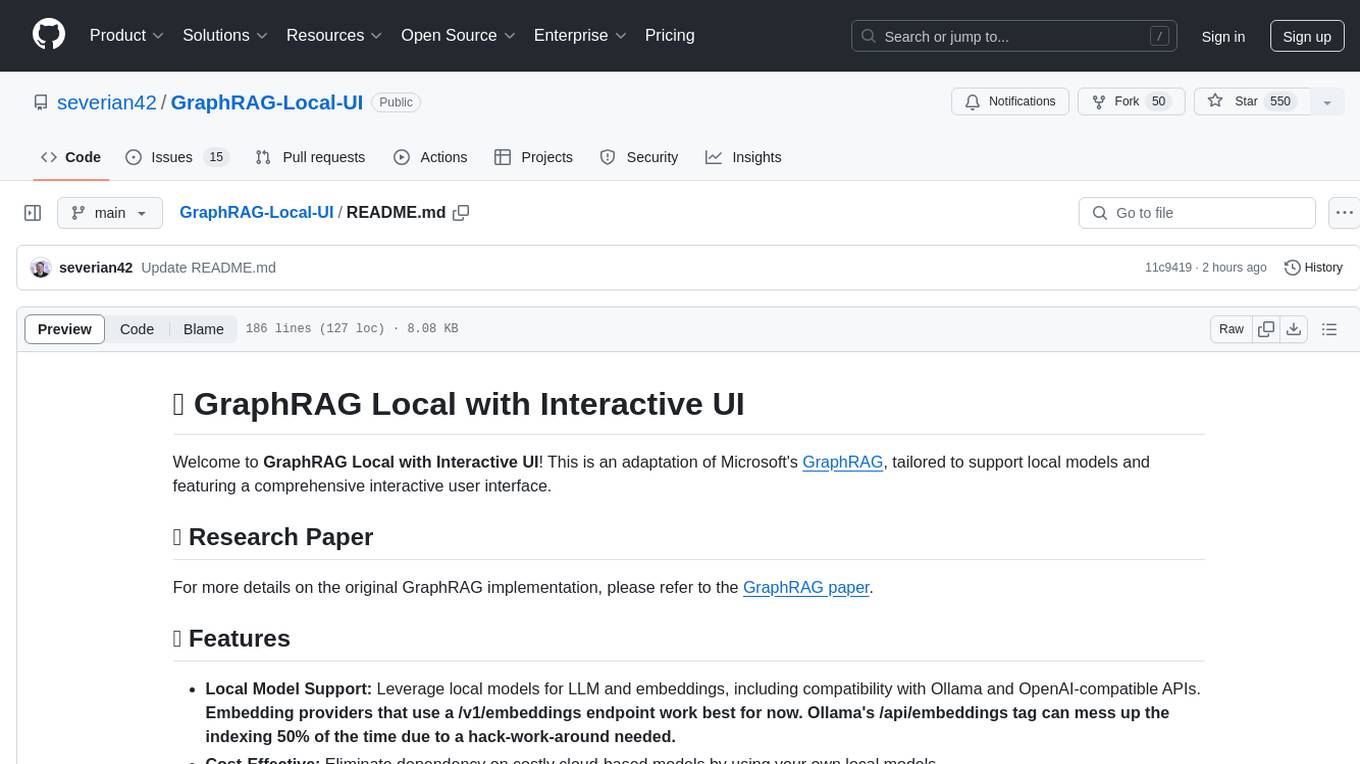
GraphRAG-Local-UI
GraphRAG Local with Interactive UI is an adaptation of Microsoft's GraphRAG, tailored to support local models and featuring a comprehensive interactive user interface. It allows users to leverage local models for LLM and embeddings, visualize knowledge graphs in 2D or 3D, manage files, settings, and queries, and explore indexing outputs. The tool aims to be cost-effective by eliminating dependency on costly cloud-based models and offers flexible querying options for global, local, and direct chat queries.
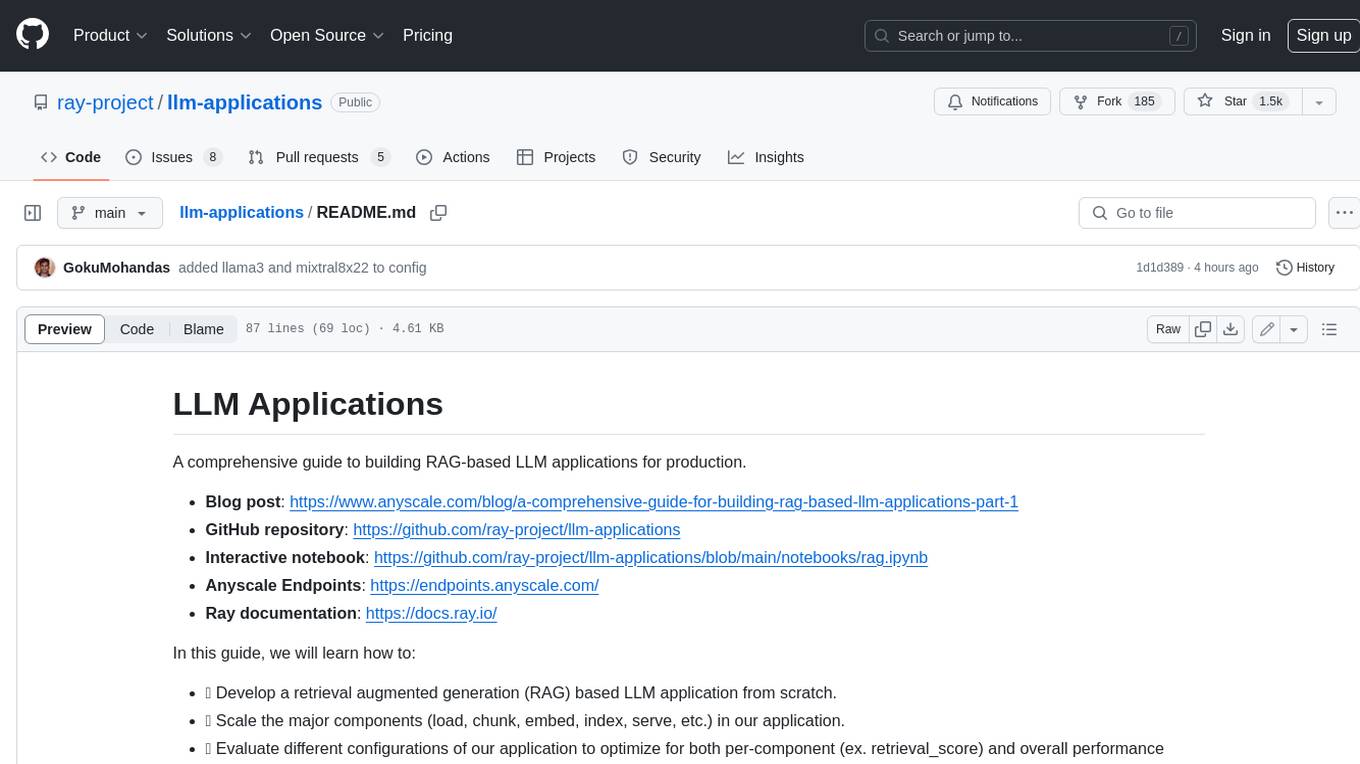
llm-applications
A comprehensive guide to building Retrieval Augmented Generation (RAG)-based LLM applications for production. This guide covers developing a RAG-based LLM application from scratch, scaling the major components, evaluating different configurations, implementing LLM hybrid routing, serving the application in a highly scalable and available manner, and sharing the impacts LLM applications have had on products.
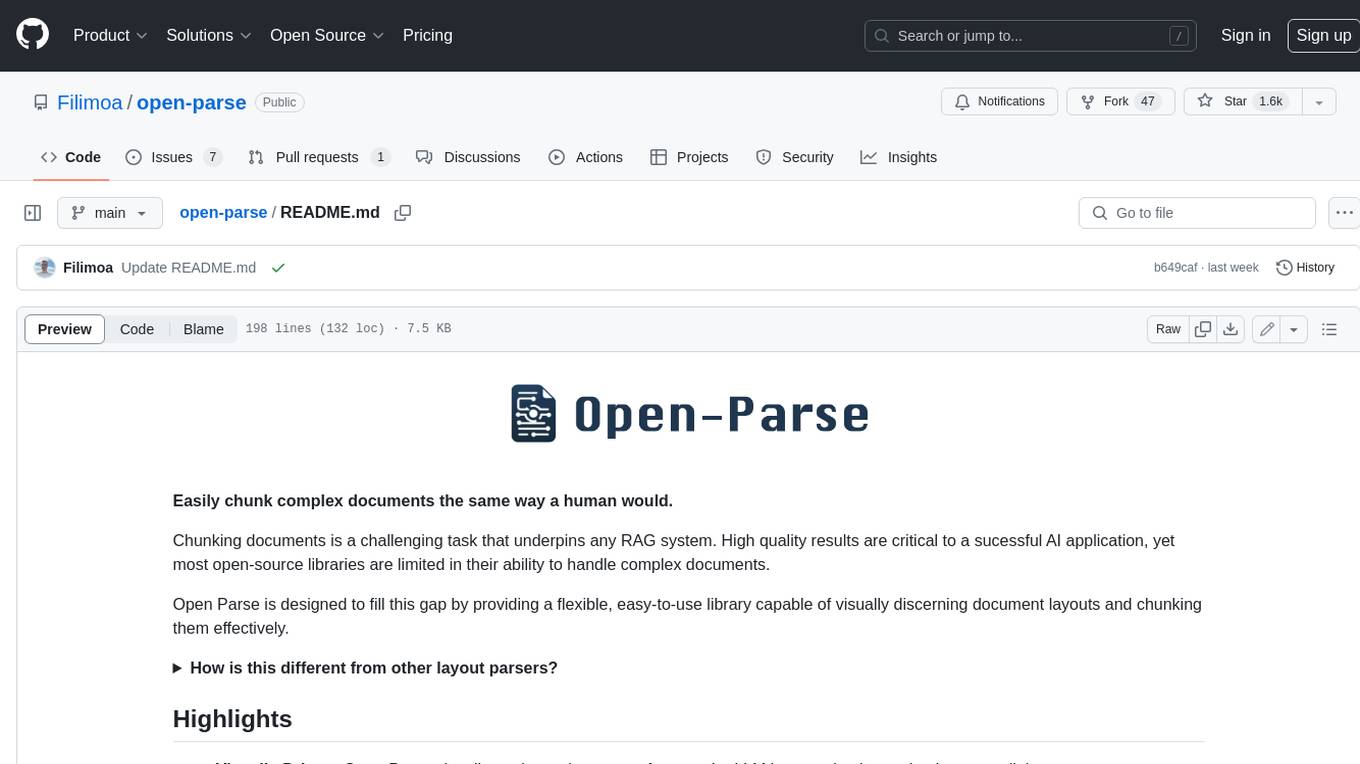
open-parse
Open Parse is a Python library for visually discerning document layouts and chunking them effectively. It is designed to fill the gap in open-source libraries for handling complex documents. Unlike text splitting, which converts a file to raw text and slices it up, Open Parse visually analyzes documents for superior LLM input. It also supports basic markdown for parsing headings, bold, and italics, and has high-precision table support, extracting tables into clean Markdown formats with accuracy that surpasses traditional tools. Open Parse is extensible, allowing users to easily implement their own post-processing steps. It is also intuitive, with great editor support and completion everywhere, making it easy to use and learn.
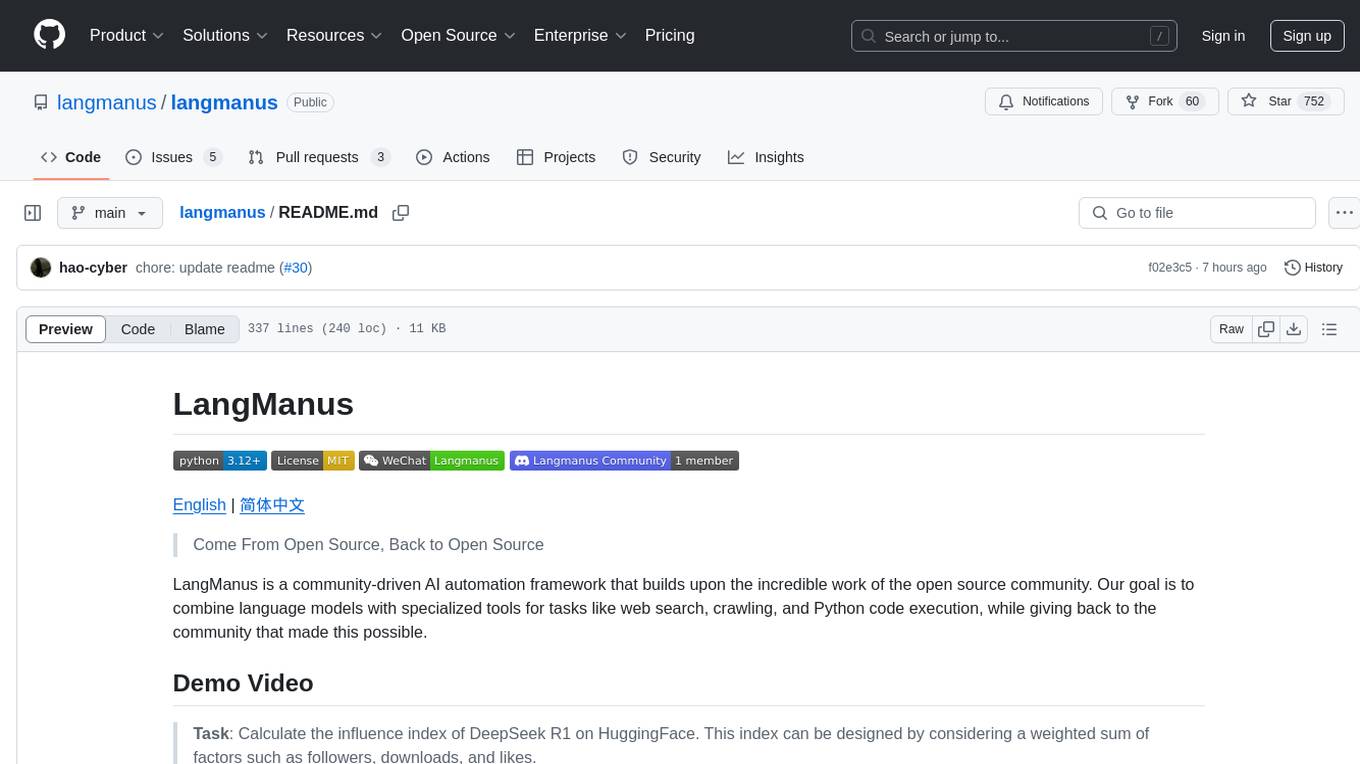
langmanus
LangManus is a community-driven AI automation framework that combines language models with specialized tools for tasks like web search, crawling, and Python code execution. It implements a hierarchical multi-agent system with agents like Coordinator, Planner, Supervisor, Researcher, Coder, Browser, and Reporter. The framework supports LLM integration, search and retrieval tools, Python integration, workflow management, and visualization. LangManus aims to give back to the open-source community and welcomes contributions in various forms.
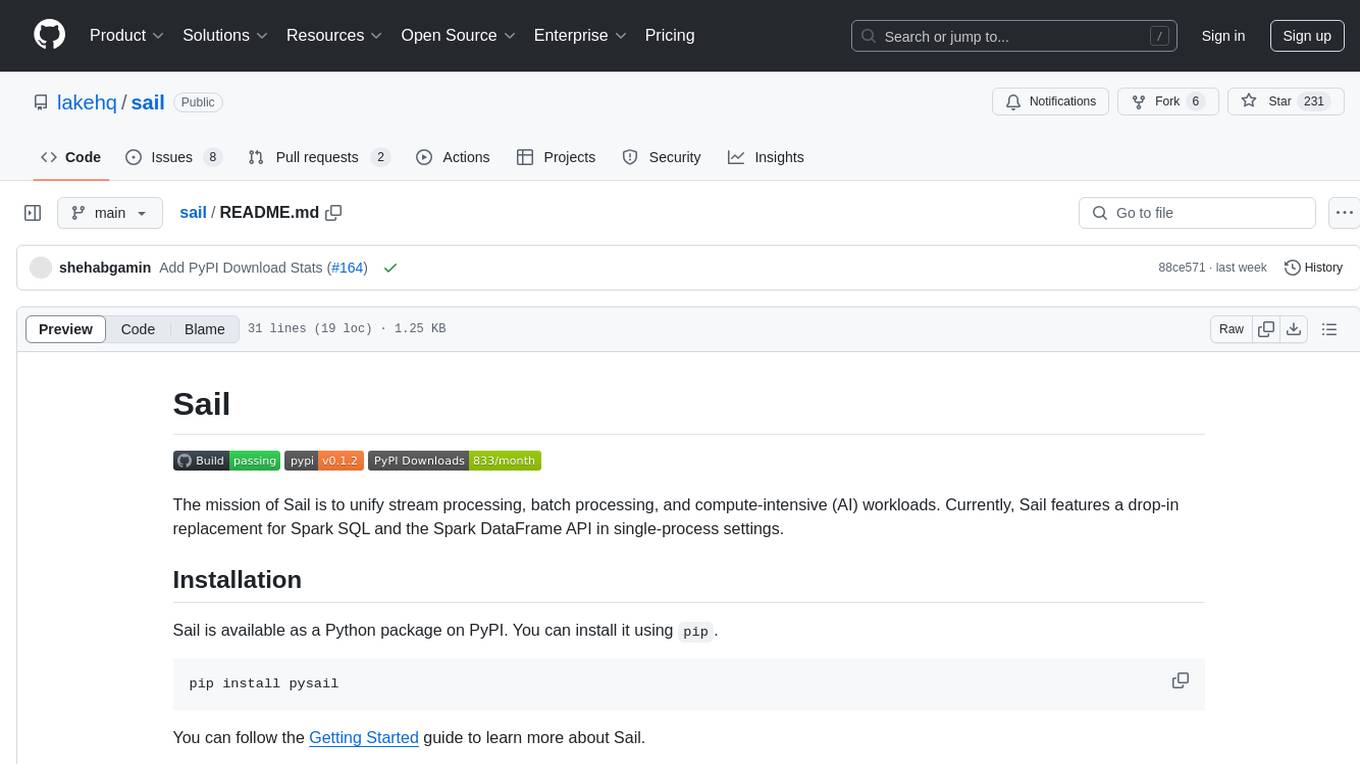
sail
Sail is a tool designed to unify stream processing, batch processing, and compute-intensive workloads, serving as a drop-in replacement for Spark SQL and the Spark DataFrame API in single-process settings. It aims to streamline data processing tasks and facilitate AI workloads.
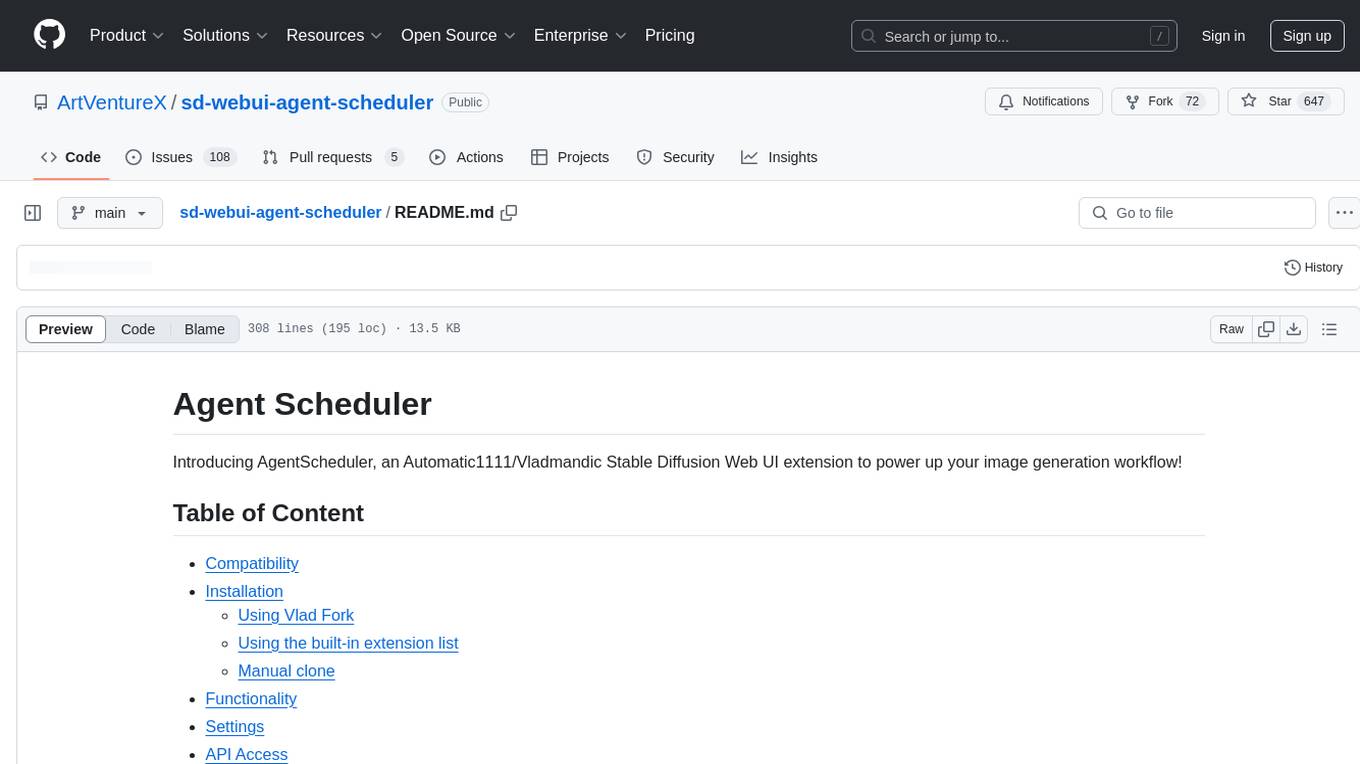
sd-webui-agent-scheduler
AgentScheduler is an Automatic/Vladmandic Stable Diffusion Web UI extension designed to enhance image generation workflows. It allows users to enqueue prompts, settings, and controlnets, manage queued tasks, prioritize, pause, resume, and delete tasks, view generation results, and more. The extension offers hidden features like queuing checkpoints, editing queued tasks, and custom checkpoint selection. Users can access the functionality through HTTP APIs and API callbacks. Troubleshooting steps are provided for common errors. The extension is compatible with latest versions of A1111 and Vladmandic. It is licensed under Apache License 2.0.
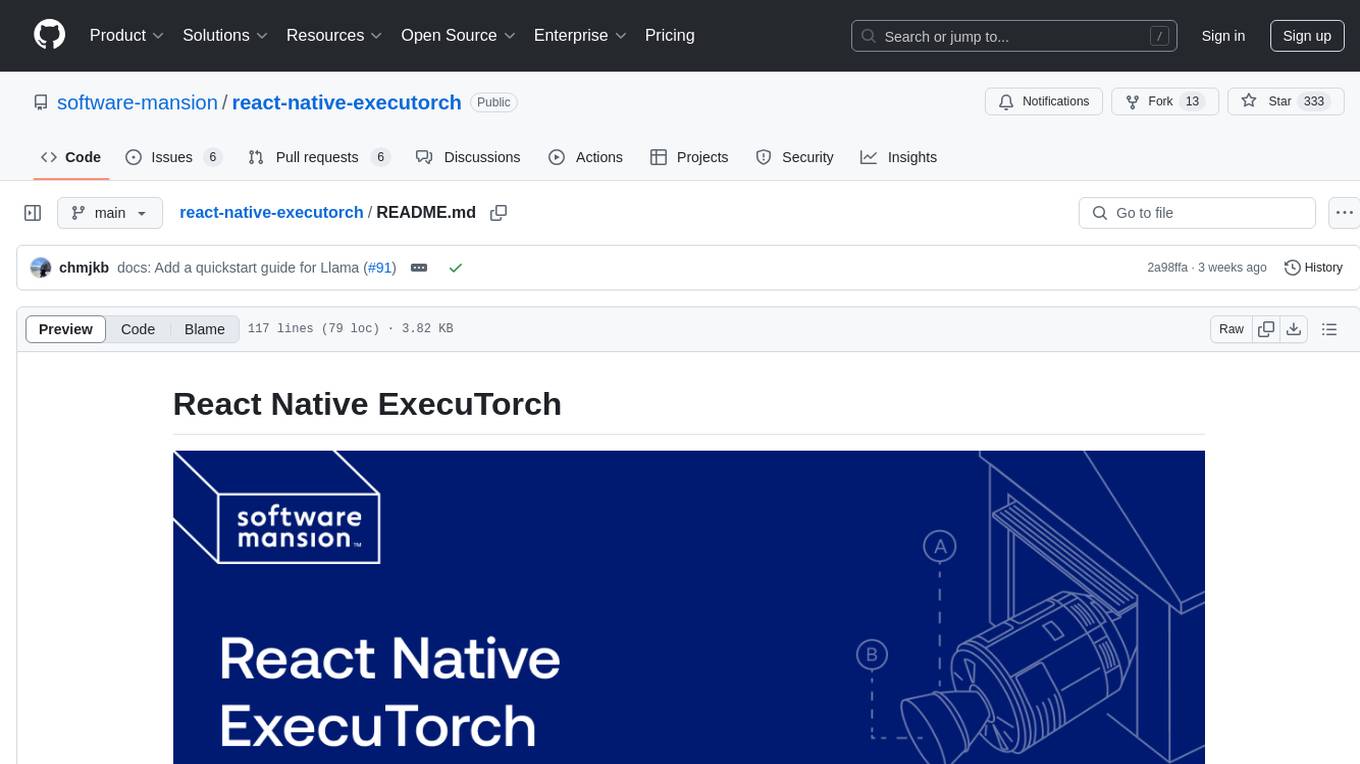
react-native-executorch
React Native ExecuTorch is a framework that allows developers to run AI models on mobile devices using React Native. It bridges the gap between React Native and native platform capabilities, providing high-performance AI model execution without requiring deep knowledge of native code or machine learning internals. The tool supports ready-made models in `.pte` format and offers a Python API for custom models. It is designed to simplify the integration of AI features into React Native apps.
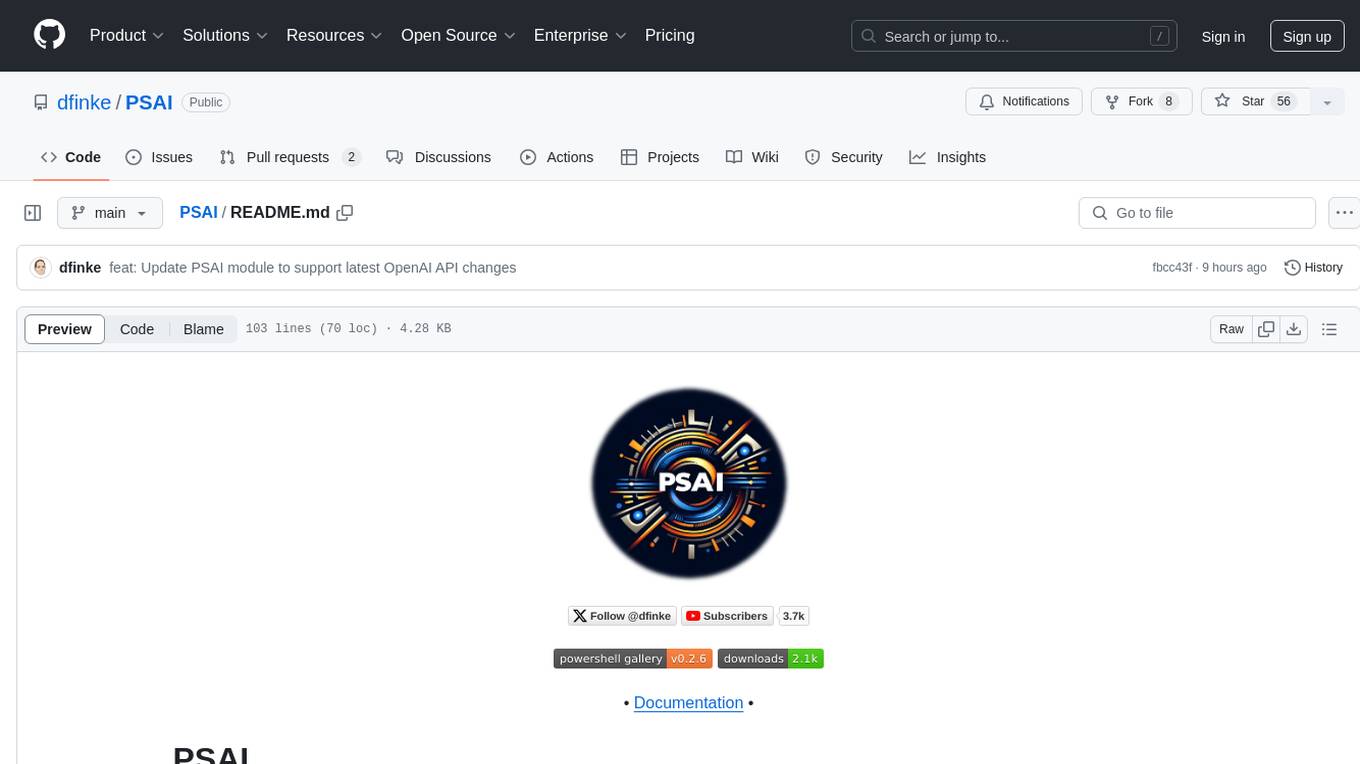
PSAI
PSAI is a PowerShell module that empowers scripts with the intelligence of OpenAI, bridging the gap between PowerShell and AI. It enables seamless integration for tasks like file searches and data analysis, revolutionizing automation possibilities with just a few lines of code. The module supports the latest OpenAI API changes, offering features like improved file search, vector store objects, token usage control, message limits, tool choice parameter, custom conversation histories, and model configuration parameters.
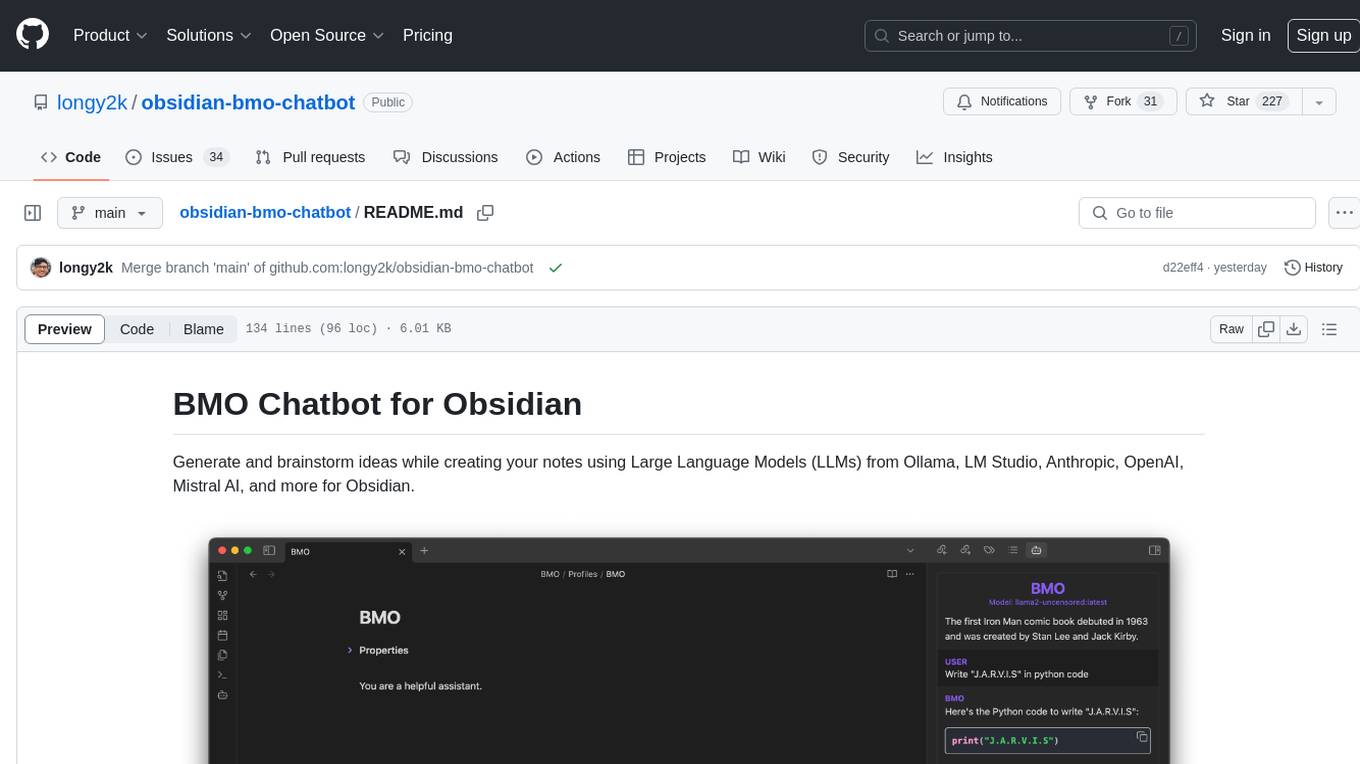
obsidian-bmo-chatbot
Obsidian BMO Chatbot is a plugin that allows users to generate and brainstorm ideas while creating notes using Large Language Models (LLMs) from various providers like Ollama, LM Studio, Anthropic, OpenAI, Mistral AI, and more. Users can interact with self-hosted LLMs, create chatbots with specific knowledge and personalities, chat from anywhere within Obsidian, and receive formatted responses in Obsidian Markdown. The plugin also offers features like customizable bot name, prompt selection, saving chat history as markdown, and more. Users can activate the plugin through Obsidian Community plugins or by installing it manually. Supported models include Ollama, LM Studio, Anthropic, Mistral AI, Google Gemini Pro, OpenAI, and Openrouter provided models.
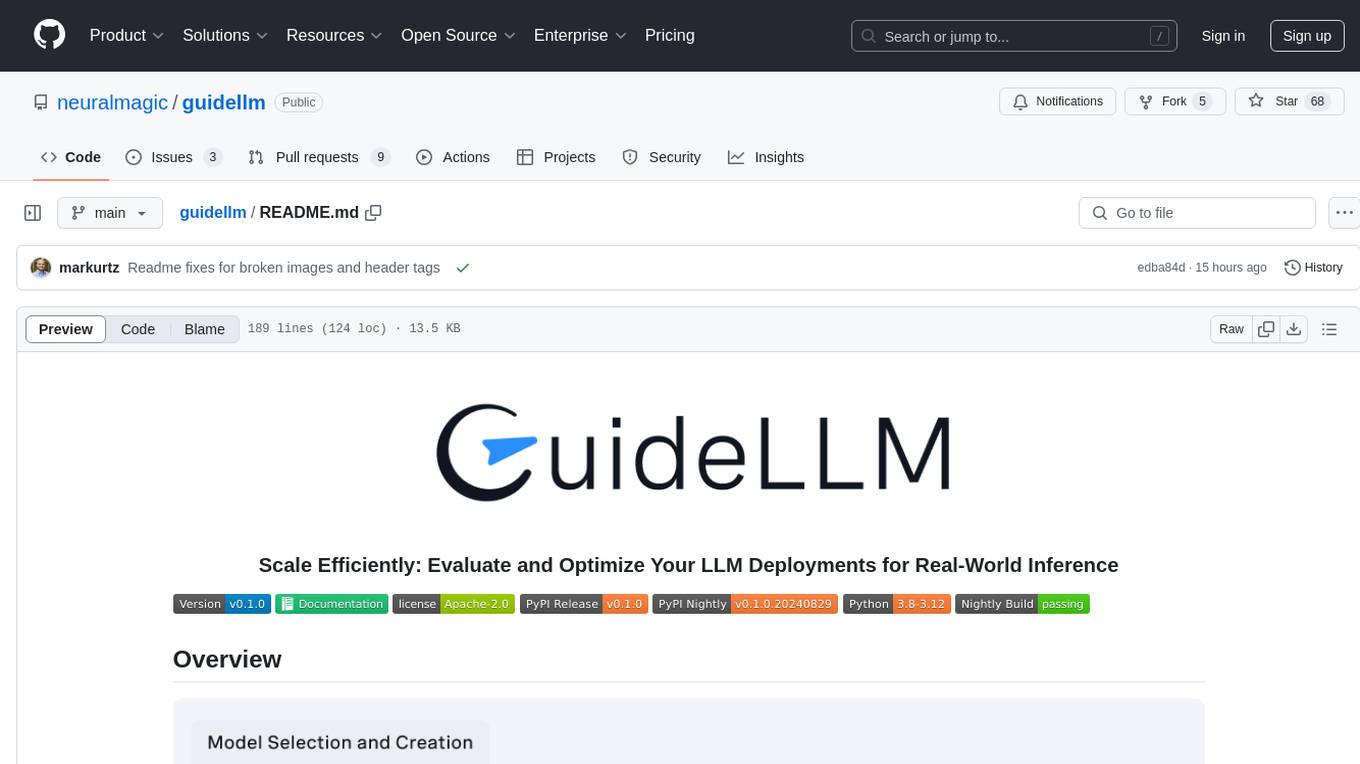
guidellm
GuideLLM is a powerful tool for evaluating and optimizing the deployment of large language models (LLMs). By simulating real-world inference workloads, GuideLLM helps users gauge the performance, resource needs, and cost implications of deploying LLMs on various hardware configurations. This approach ensures efficient, scalable, and cost-effective LLM inference serving while maintaining high service quality. Key features include performance evaluation, resource optimization, cost estimation, and scalability testing.
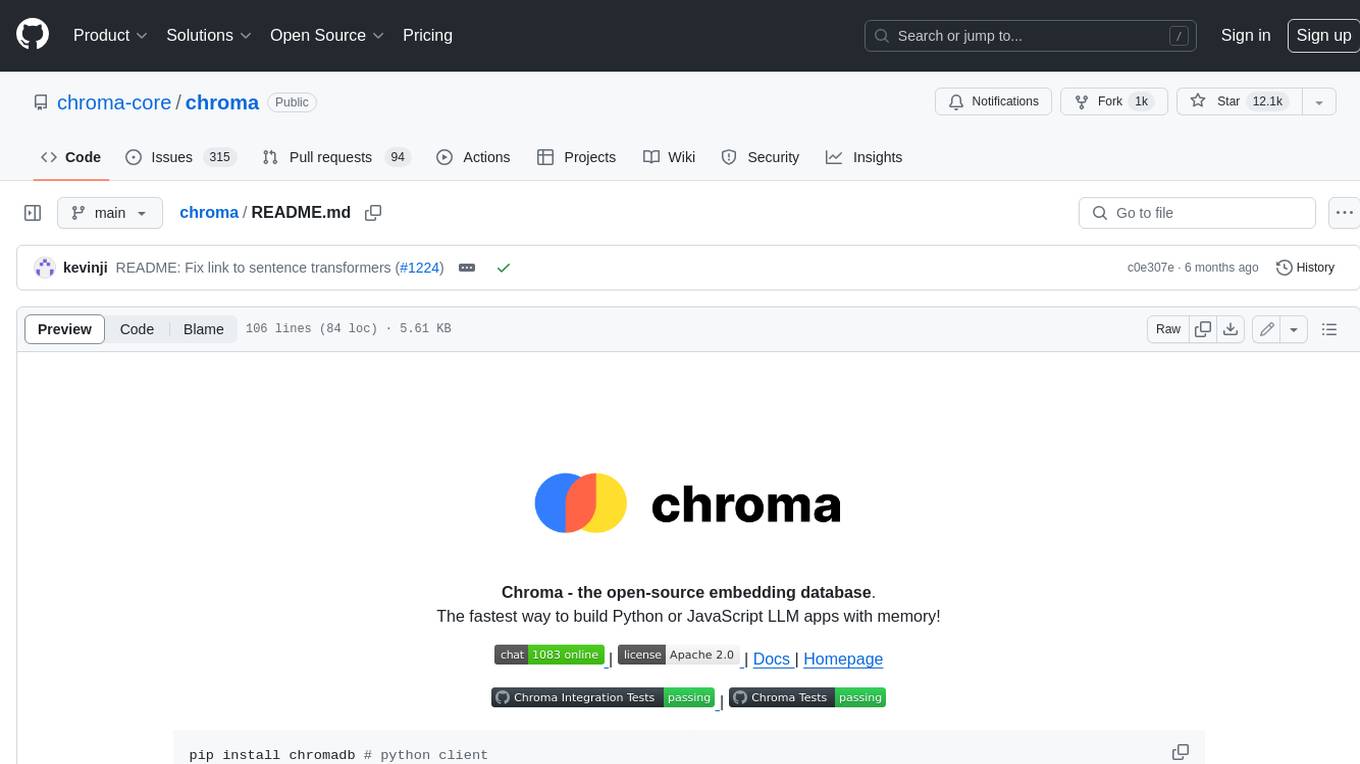
chroma
Chroma is an open-source embedding database that provides a simple, scalable, and feature-rich way to build Python or JavaScript LLM apps with memory. It offers a fully-typed, fully-tested, and fully-documented API that makes it easy to get started and scale your applications. Chroma also integrates with popular tools like LangChain and LlamaIndex, and supports a variety of embedding models, including Sentence Transformers, OpenAI embeddings, and Cohere embeddings. With Chroma, you can easily add documents to your database, query relevant documents with natural language, and compose documents into the context window of an LLM like GPT3 for additional summarization or analysis.
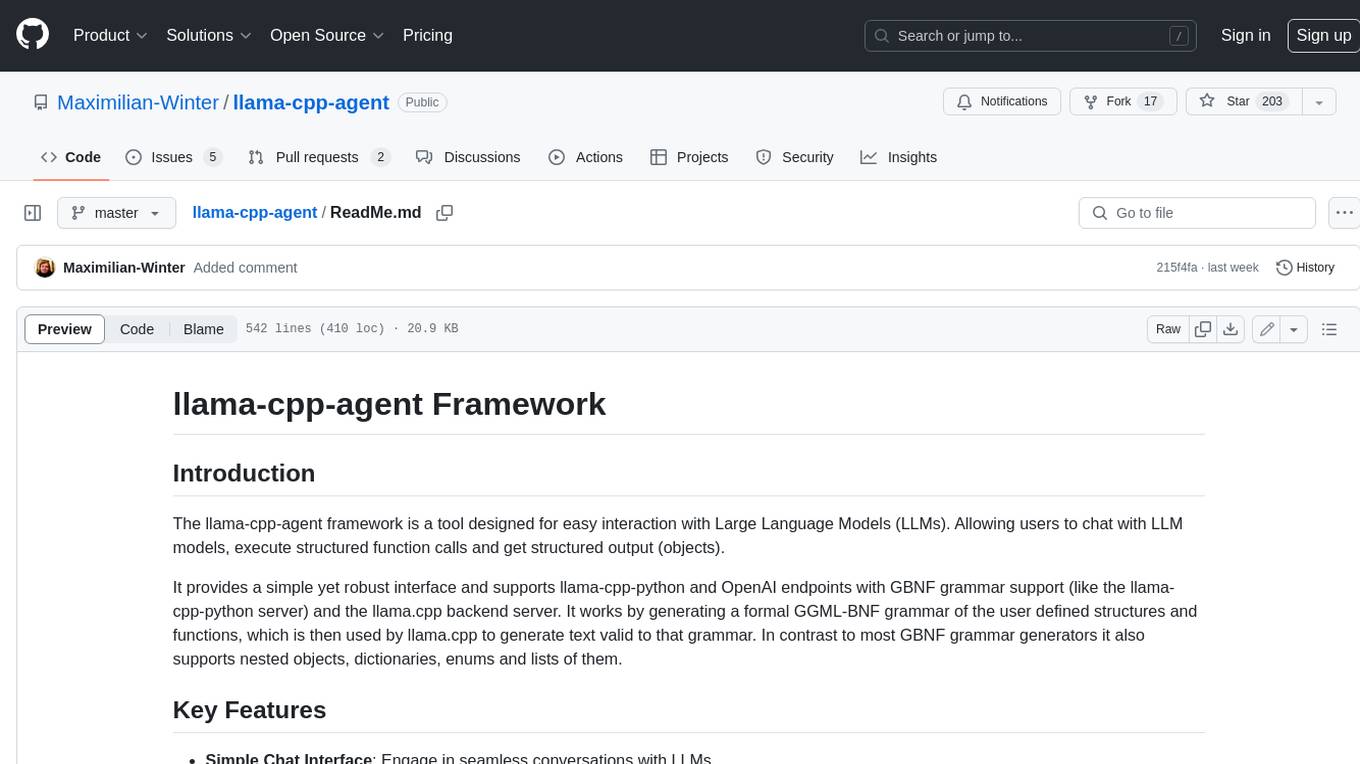
llama-cpp-agent
The llama-cpp-agent framework is a tool designed for easy interaction with Large Language Models (LLMs). Allowing users to chat with LLM models, execute structured function calls and get structured output (objects). It provides a simple yet robust interface and supports llama-cpp-python and OpenAI endpoints with GBNF grammar support (like the llama-cpp-python server) and the llama.cpp backend server. It works by generating a formal GGML-BNF grammar of the user defined structures and functions, which is then used by llama.cpp to generate text valid to that grammar. In contrast to most GBNF grammar generators it also supports nested objects, dictionaries, enums and lists of them.
For similar tasks
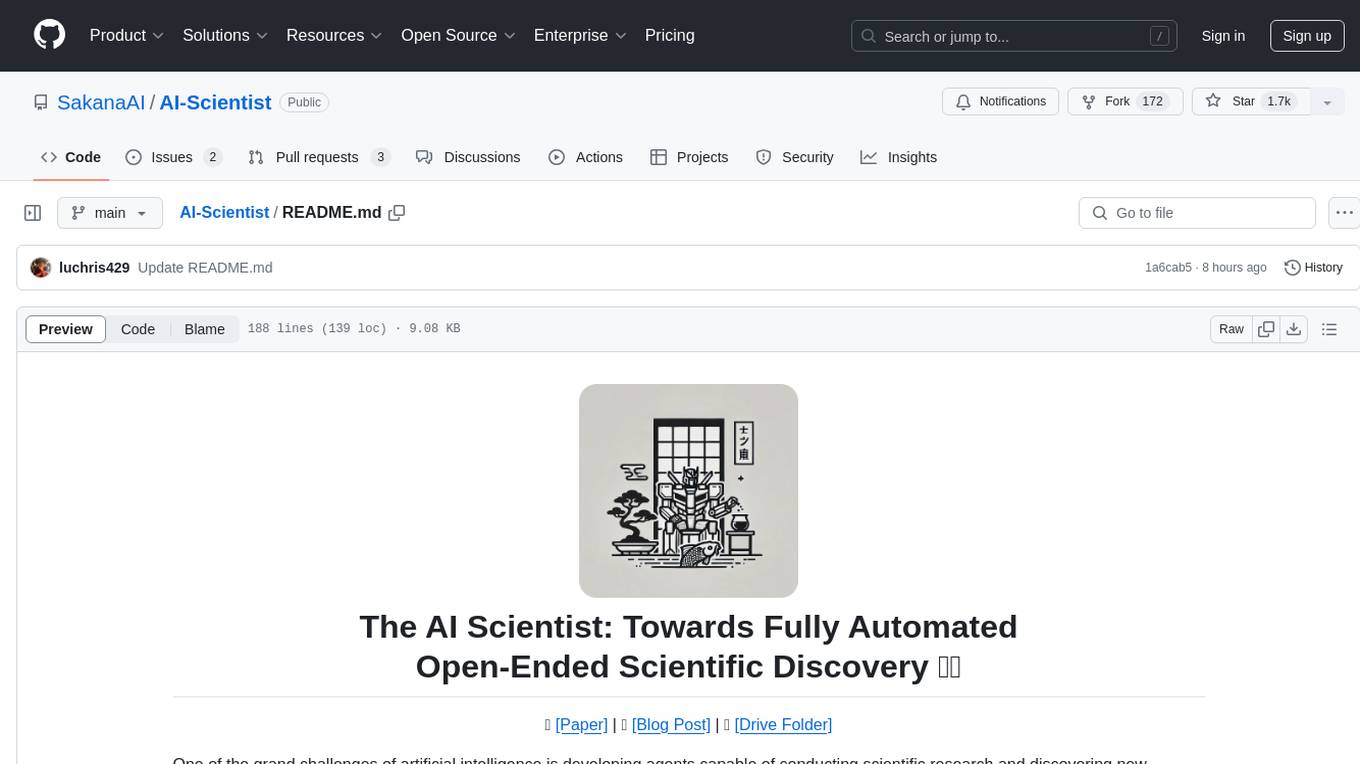
AI-Scientist
The AI Scientist is a comprehensive system for fully automatic scientific discovery, enabling Foundation Models to perform research independently. It aims to tackle the grand challenge of developing agents capable of conducting scientific research and discovering new knowledge. The tool generates papers on various topics using Large Language Models (LLMs) and provides a platform for exploring new research ideas. Users can create their own templates for specific areas of study and run experiments to generate papers. However, caution is advised as the codebase executes LLM-written code, which may pose risks such as the use of potentially dangerous packages and web access.
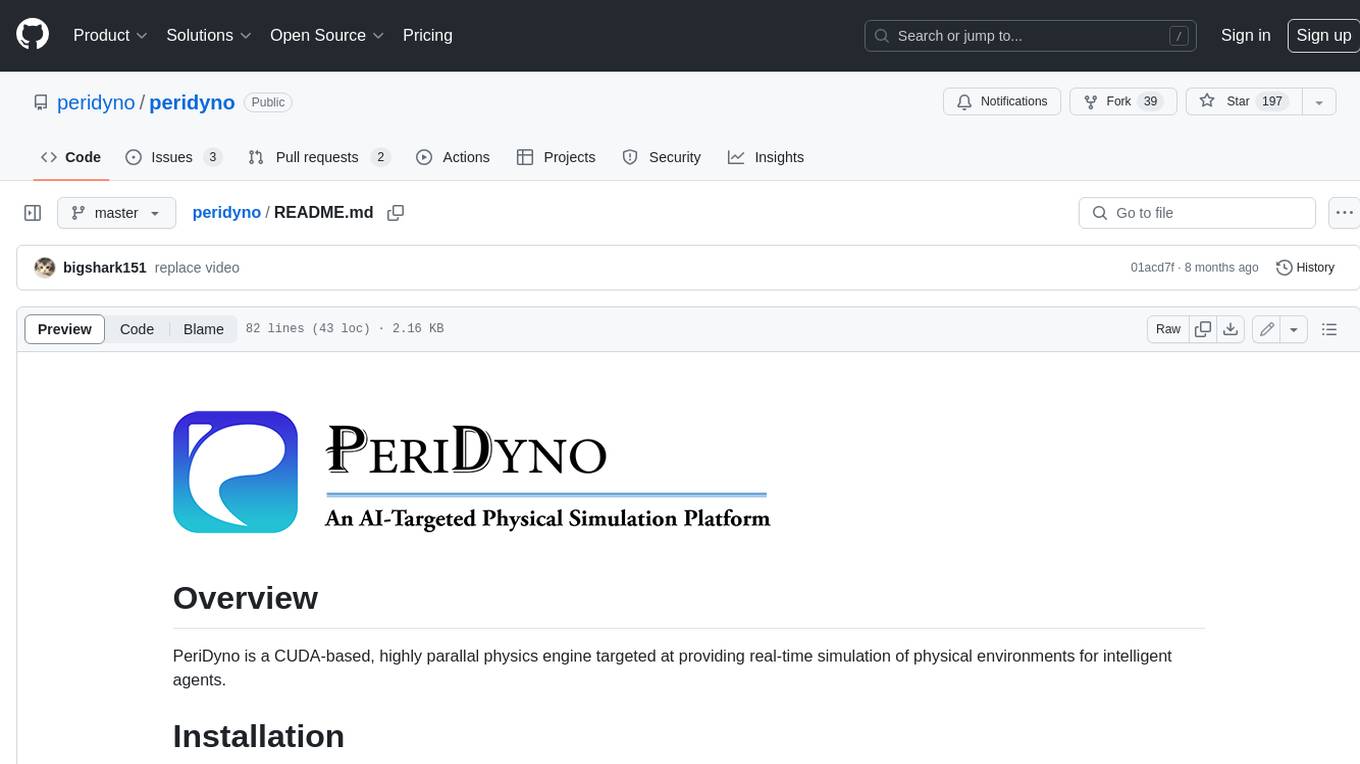
peridyno
PeriDyno is a CUDA-based, highly parallel physics engine targeted at providing real-time simulation of physical environments for intelligent agents. It is designed to be easy to use and integrate into existing projects, and it provides a wide range of features for simulating a variety of physical phenomena. PeriDyno is open source and available under the Apache 2.0 license.
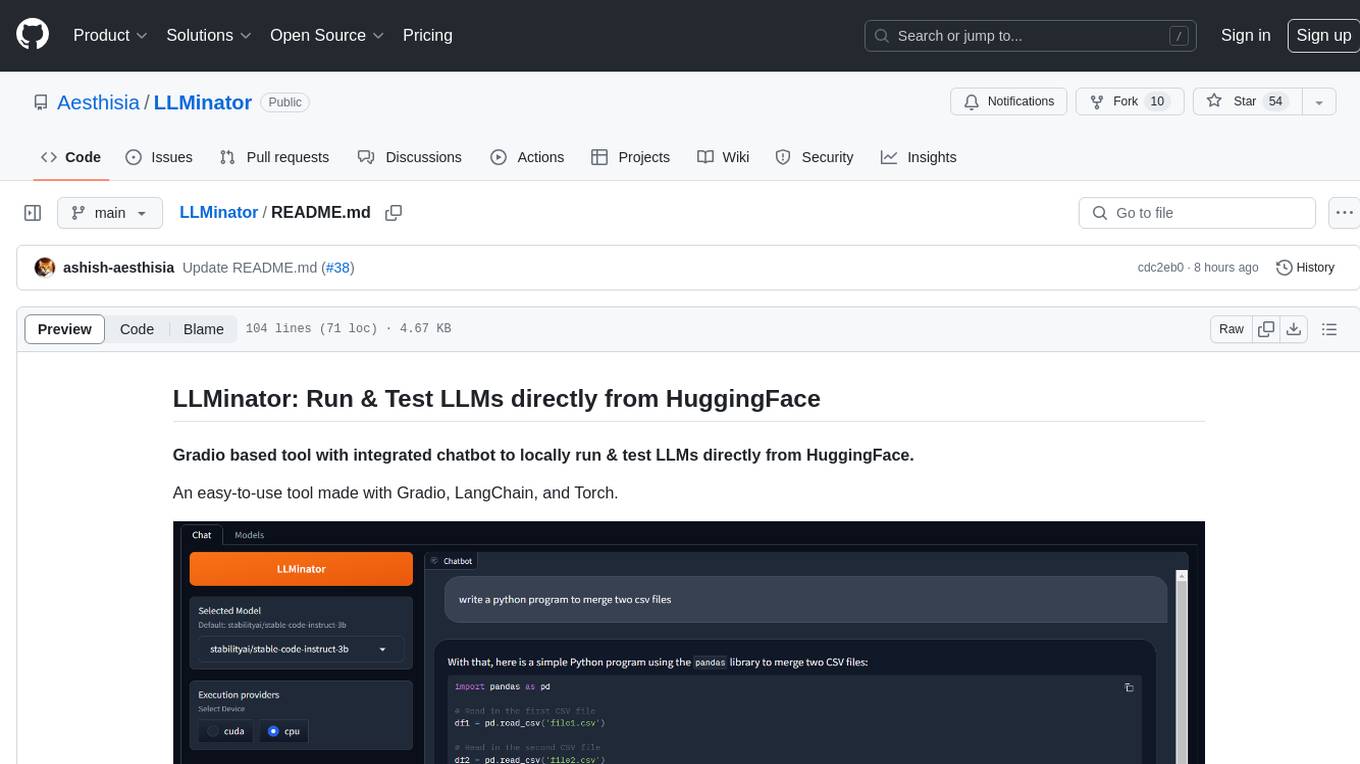
LLMinator
LLMinator is a Gradio-based tool with an integrated chatbot designed to locally run and test Language Model Models (LLMs) directly from HuggingFace. It provides an easy-to-use interface made with Gradio, LangChain, and Torch, offering features such as context-aware streaming chatbot, inbuilt code syntax highlighting, loading any LLM repo from HuggingFace, support for both CPU and CUDA modes, enabling LLM inference with llama.cpp, and model conversion capabilities.
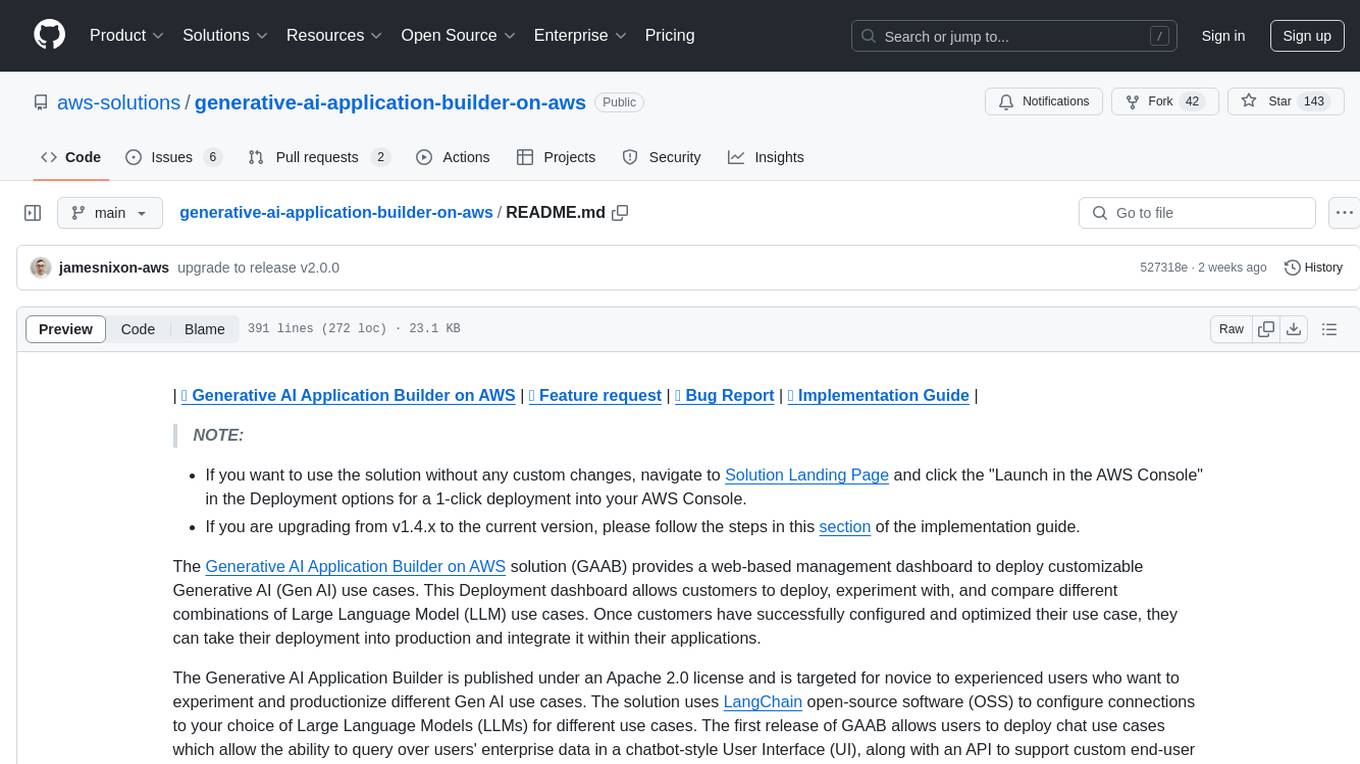
generative-ai-application-builder-on-aws
The Generative AI Application Builder on AWS (GAAB) is a solution that provides a web-based management dashboard for deploying customizable Generative AI (Gen AI) use cases. Users can experiment with and compare different combinations of Large Language Model (LLM) use cases, configure and optimize their use cases, and integrate them into their applications for production. The solution is targeted at novice to experienced users who want to experiment and productionize different Gen AI use cases. It uses LangChain open-source software to configure connections to Large Language Models (LLMs) for various use cases, with the ability to deploy chat use cases that allow querying over users' enterprise data in a chatbot-style User Interface (UI) and support custom end-user implementations through an API.
For similar jobs
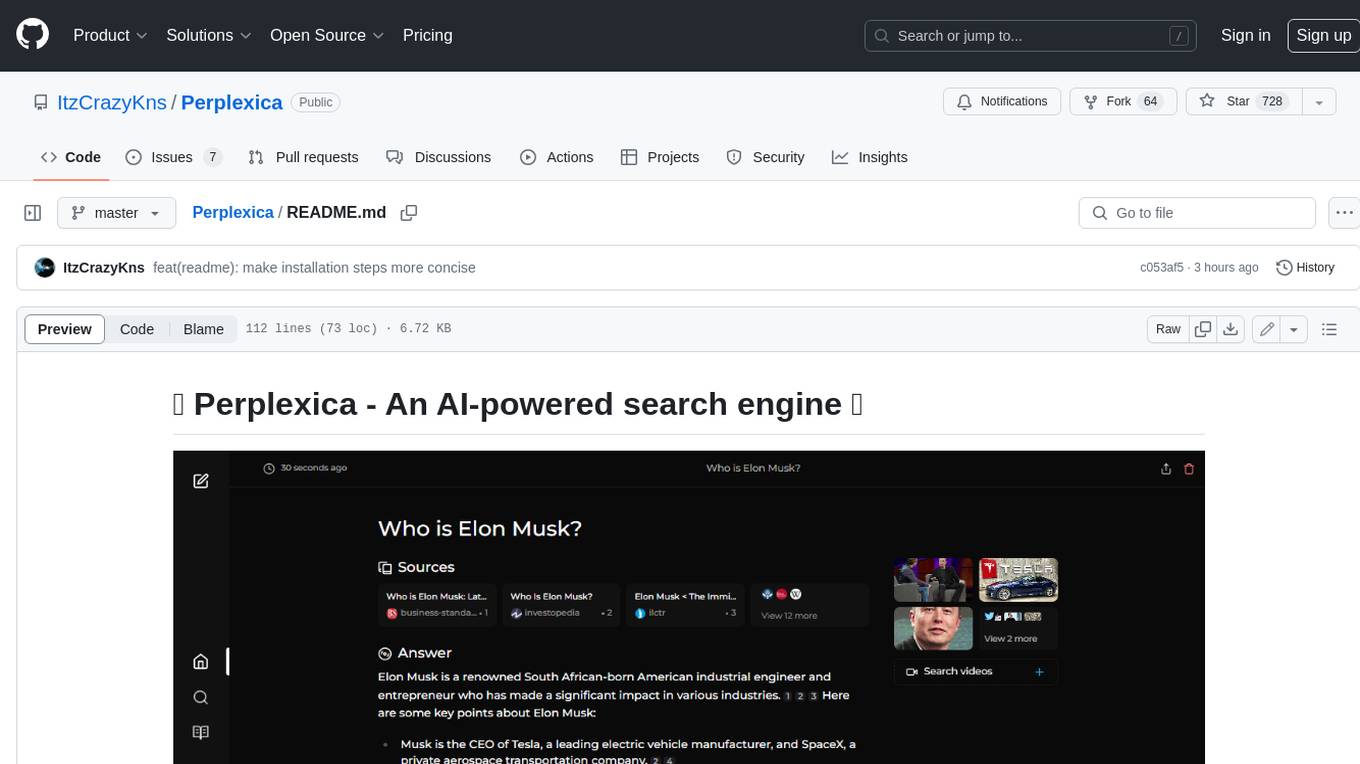
Perplexica
Perplexica is an open-source AI-powered search engine that utilizes advanced machine learning algorithms to provide clear answers with sources cited. It offers various modes like Copilot Mode, Normal Mode, and Focus Modes for specific types of questions. Perplexica ensures up-to-date information by using SearxNG metasearch engine. It also features image and video search capabilities and upcoming features include finalizing Copilot Mode and adding Discover and History Saving features.
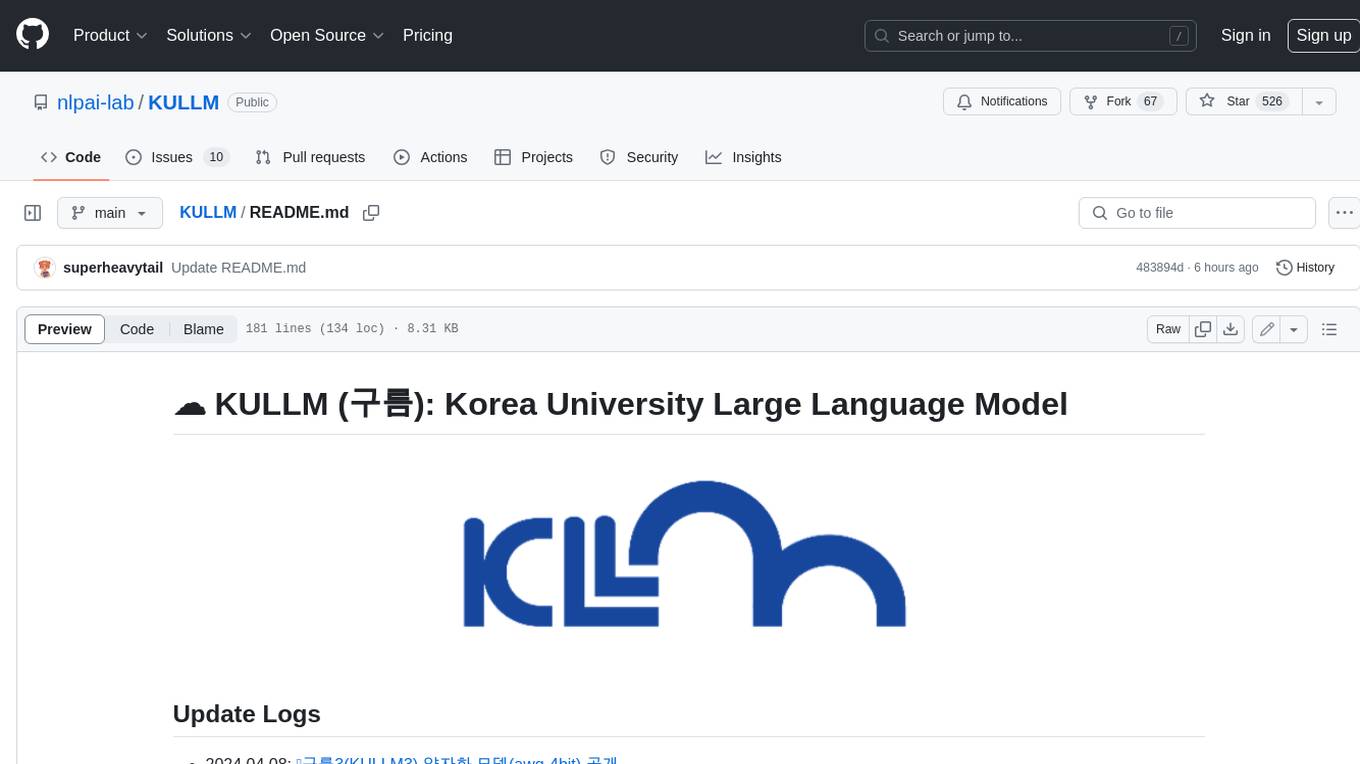
KULLM
KULLM (구름) is a Korean Large Language Model developed by Korea University NLP & AI Lab and HIAI Research Institute. It is based on the upstage/SOLAR-10.7B-v1.0 model and has been fine-tuned for instruction. The model has been trained on 8×A100 GPUs and is capable of generating responses in Korean language. KULLM exhibits hallucination and repetition phenomena due to its decoding strategy. Users should be cautious as the model may produce inaccurate or harmful results. Performance may vary in benchmarks without a fixed system prompt.
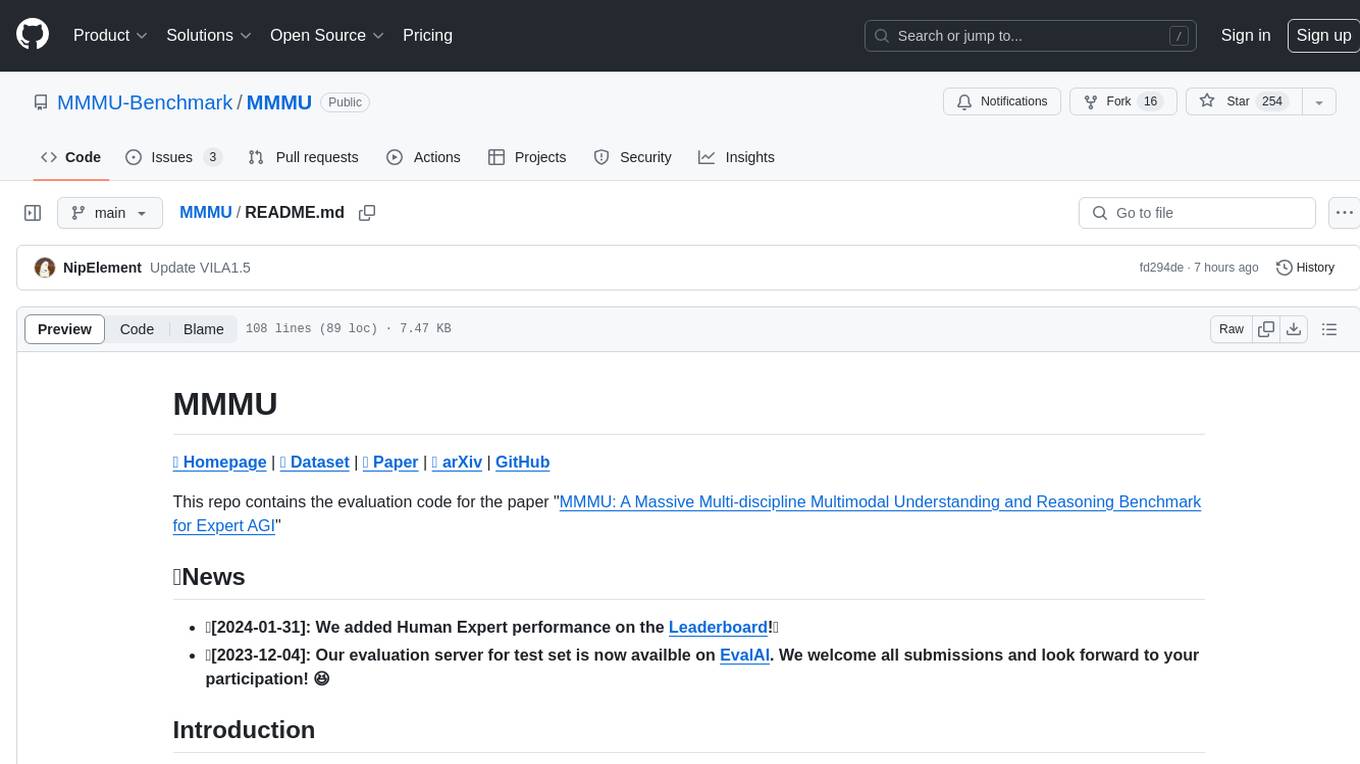
MMMU
MMMU is a benchmark designed to evaluate multimodal models on college-level subject knowledge tasks, covering 30 subjects and 183 subfields with 11.5K questions. It focuses on advanced perception and reasoning with domain-specific knowledge, challenging models to perform tasks akin to those faced by experts. The evaluation of various models highlights substantial challenges, with room for improvement to stimulate the community towards expert artificial general intelligence (AGI).
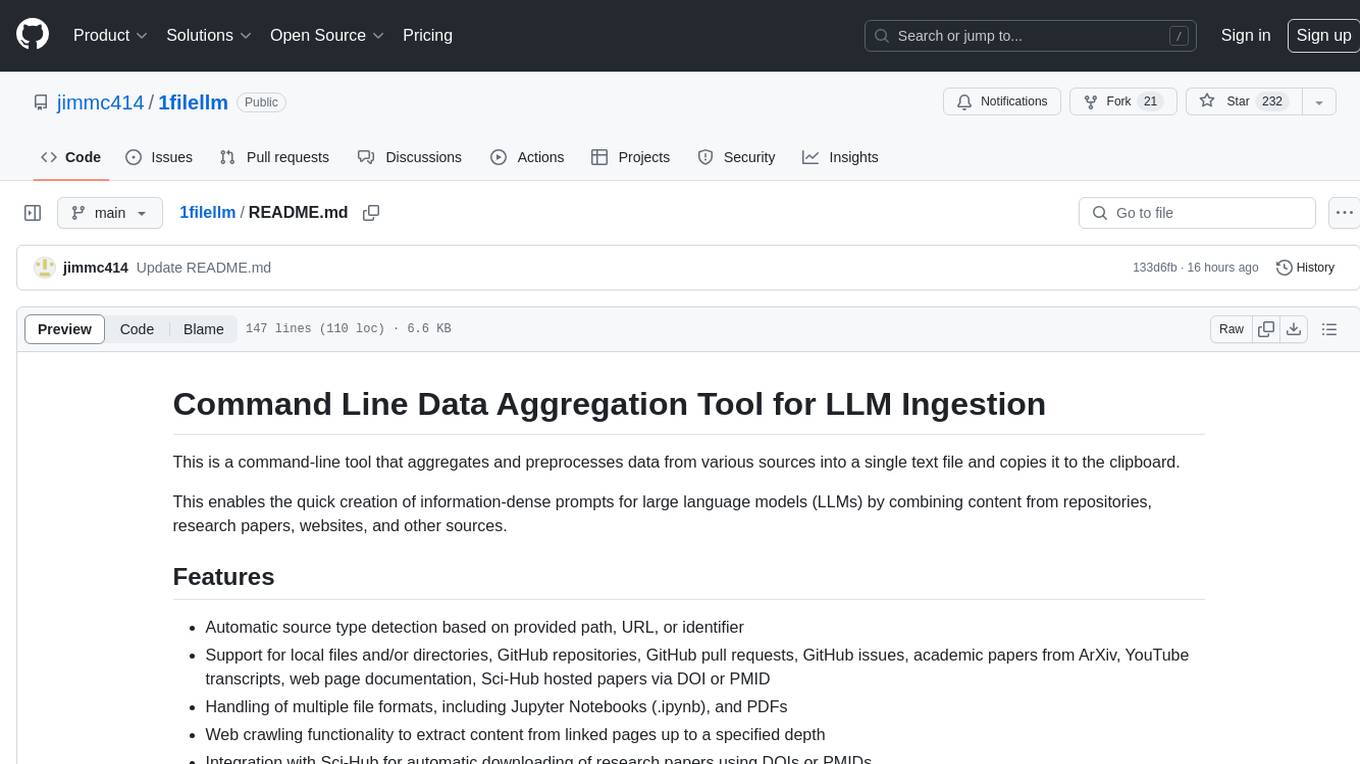
1filellm
1filellm is a command-line data aggregation tool designed for LLM ingestion. It aggregates and preprocesses data from various sources into a single text file, facilitating the creation of information-dense prompts for large language models. The tool supports automatic source type detection, handling of multiple file formats, web crawling functionality, integration with Sci-Hub for research paper downloads, text preprocessing, and token count reporting. Users can input local files, directories, GitHub repositories, pull requests, issues, ArXiv papers, YouTube transcripts, web pages, Sci-Hub papers via DOI or PMID. The tool provides uncompressed and compressed text outputs, with the uncompressed text automatically copied to the clipboard for easy pasting into LLMs.
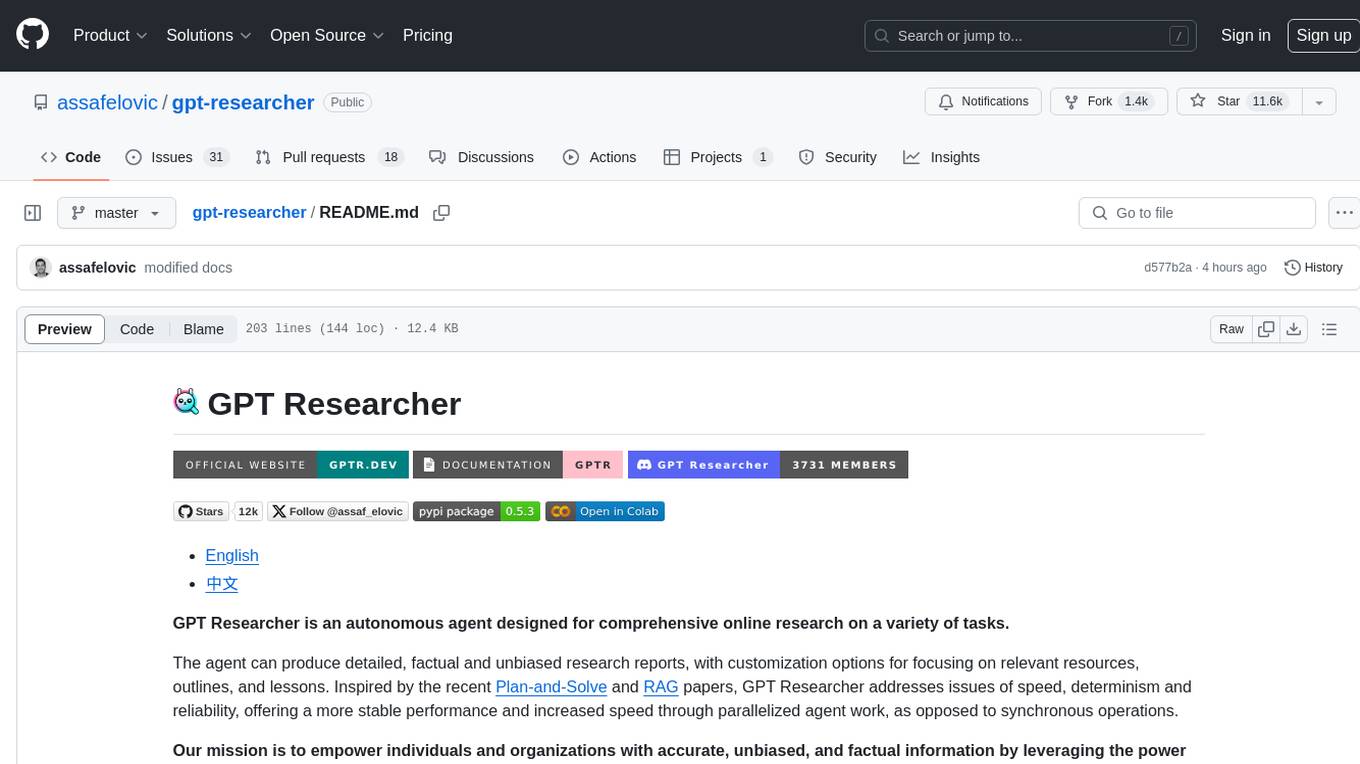
gpt-researcher
GPT Researcher is an autonomous agent designed for comprehensive online research on a variety of tasks. It can produce detailed, factual, and unbiased research reports with customization options. The tool addresses issues of speed, determinism, and reliability by leveraging parallelized agent work. The main idea involves running 'planner' and 'execution' agents to generate research questions, seek related information, and create research reports. GPT Researcher optimizes costs and completes tasks in around 3 minutes. Features include generating long research reports, aggregating web sources, an easy-to-use web interface, scraping web sources, and exporting reports to various formats.
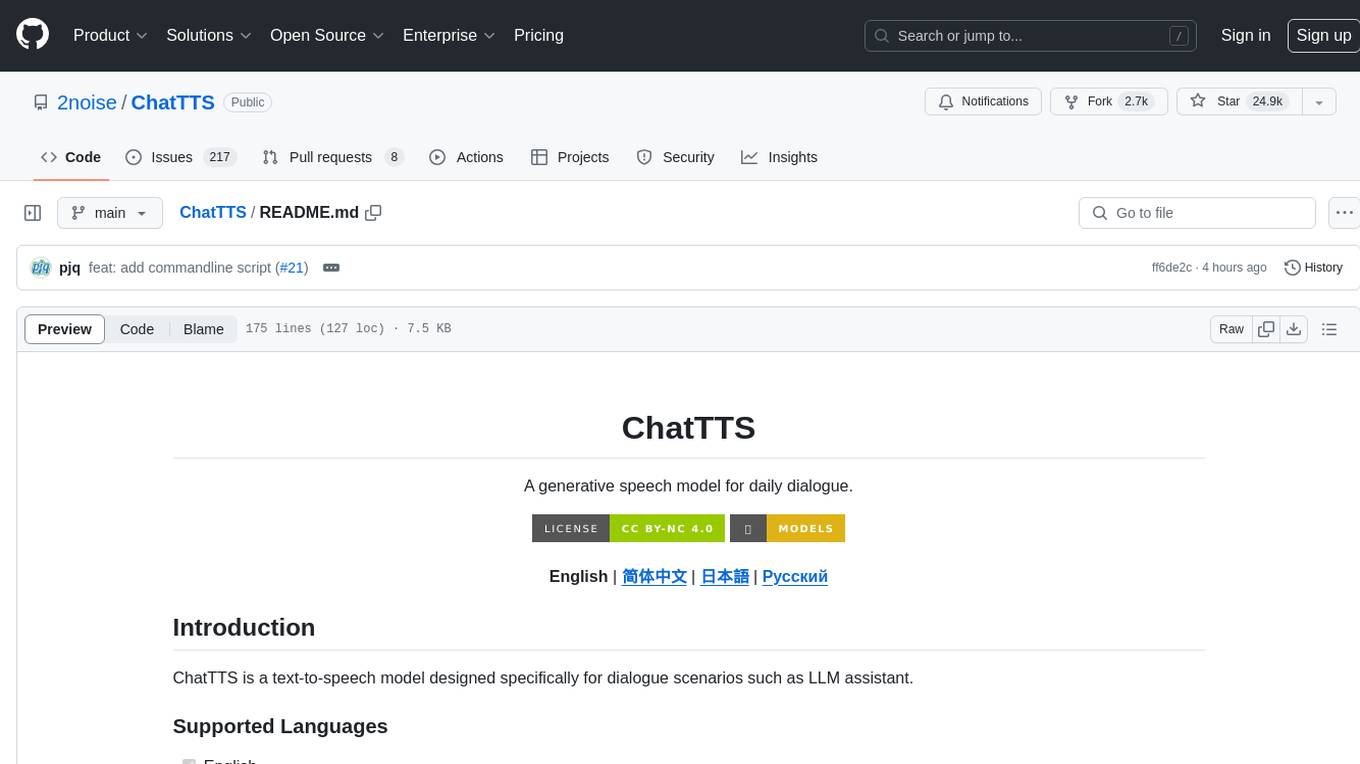
ChatTTS
ChatTTS is a generative speech model optimized for dialogue scenarios, providing natural and expressive speech synthesis with fine-grained control over prosodic features. It supports multiple speakers and surpasses most open-source TTS models in terms of prosody. The model is trained with 100,000+ hours of Chinese and English audio data, and the open-source version on HuggingFace is a 40,000-hour pre-trained model without SFT. The roadmap includes open-sourcing additional features like VQ encoder, multi-emotion control, and streaming audio generation. The tool is intended for academic and research use only, with precautions taken to limit potential misuse.
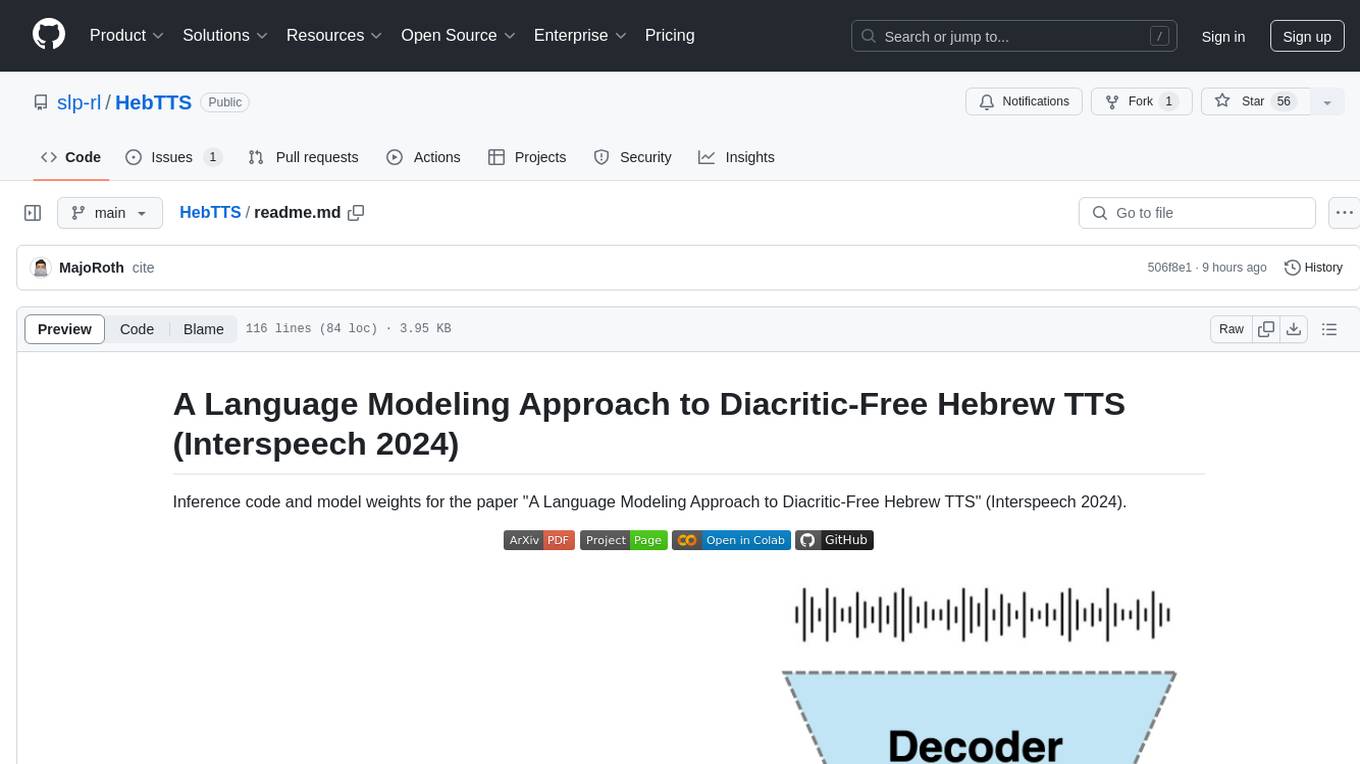
HebTTS
HebTTS is a language modeling approach to diacritic-free Hebrew text-to-speech (TTS) system. It addresses the challenge of accurately mapping text to speech in Hebrew by proposing a language model that operates on discrete speech representations and is conditioned on a word-piece tokenizer. The system is optimized using weakly supervised recordings and outperforms diacritic-based Hebrew TTS systems in terms of content preservation and naturalness of generated speech.
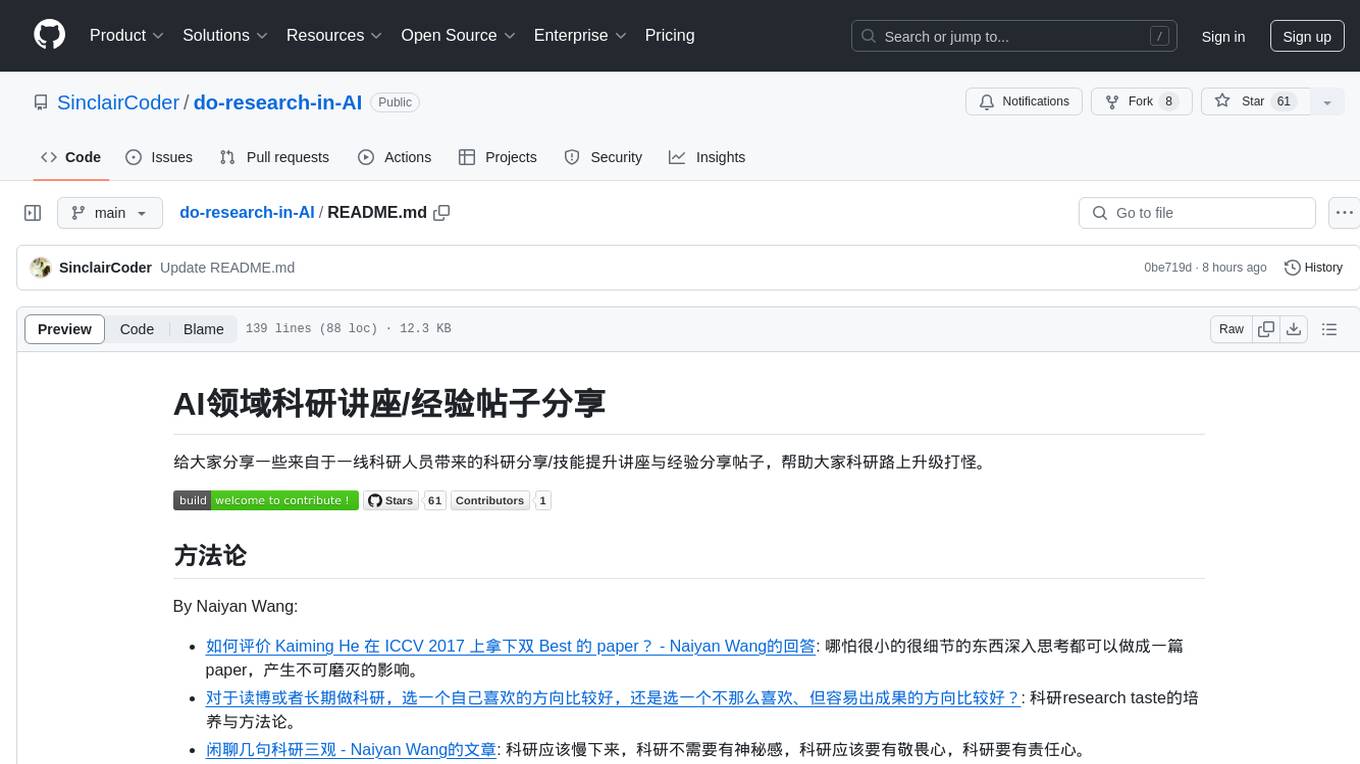
do-research-in-AI
This repository is a collection of research lectures and experience sharing posts from frontline researchers in the field of AI. It aims to help individuals upgrade their research skills and knowledge through insightful talks and experiences shared by experts. The content covers various topics such as evaluating research papers, choosing research directions, research methodologies, and tips for writing high-quality scientific papers. The repository also includes discussions on academic career paths, research ethics, and the emotional aspects of research work. Overall, it serves as a valuable resource for individuals interested in advancing their research capabilities in the field of AI.