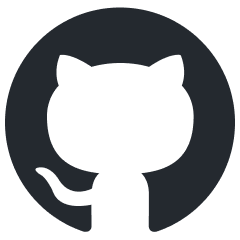
HebTTS
The official implementation of "A Language Modeling Approach to Diacritic-Free Hebrew TTS"
Stars: 52
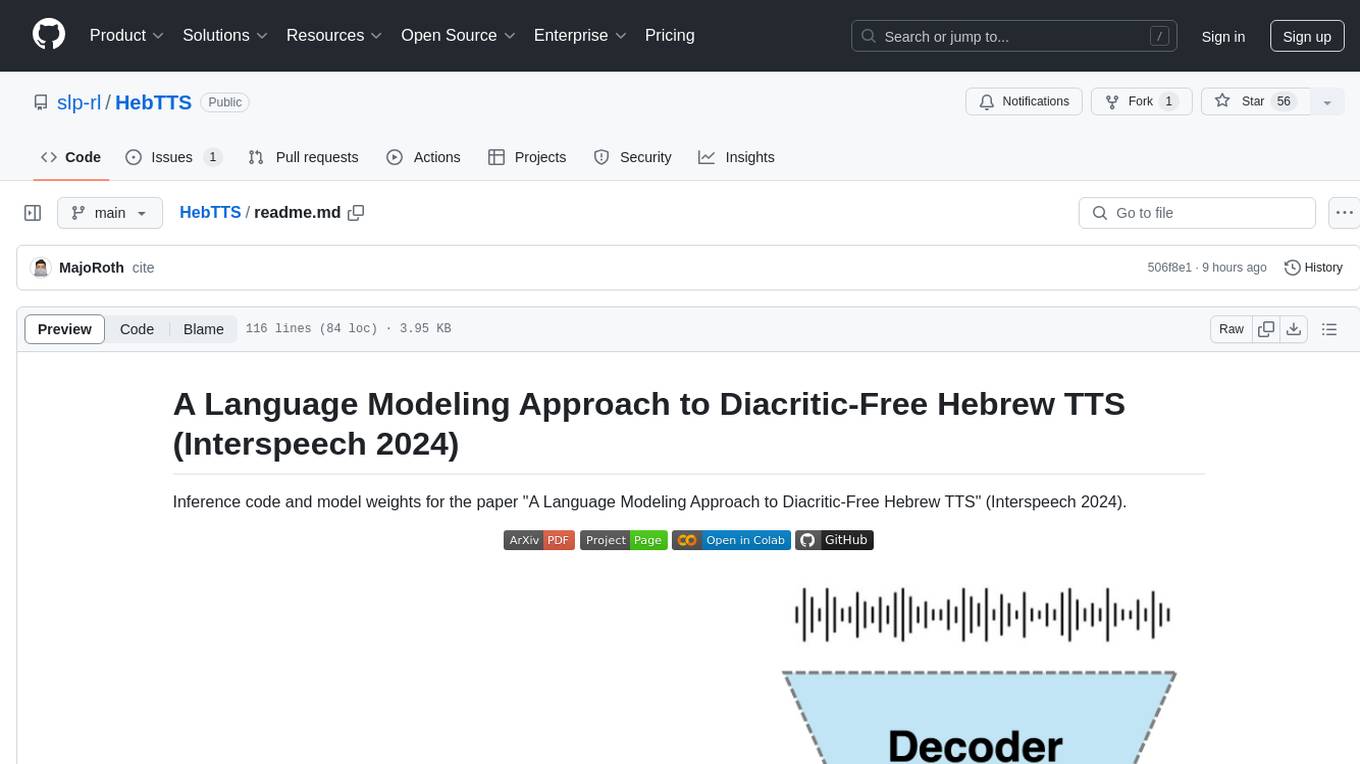
HebTTS is a language modeling approach to diacritic-free Hebrew text-to-speech (TTS) system. It addresses the challenge of accurately mapping text to speech in Hebrew by proposing a language model that operates on discrete speech representations and is conditioned on a word-piece tokenizer. The system is optimized using weakly supervised recordings and outperforms diacritic-based Hebrew TTS systems in terms of content preservation and naturalness of generated speech.
README:
Inference code and model weights for the paper "A Language Modeling Approach to Diacritic-Free Hebrew TTS" (Interspeech 2024).
Abstract: We tackle the task of text-to-speech (TTS) in Hebrew. Traditional Hebrew contains Diacritics (`Niqqud'), which dictate the way individuals should pronounce given words, however, modern Hebrew rarely uses them. The lack of diacritics in modern Hebrew results in readers expected to conclude the correct pronunciation and understand which phonemes to use based on the context. This imposes a fundamental challenge on TTS systems to accurately map between text-to-speech. In this study, we propose to adopt a language modeling Diacritics-Free TTS approach, for the task of Hebrew TTS. The language model (LM) operates on discrete speech representations and is conditioned on a word-piece tokenizer. We optimize the proposed method using in-the-wild weakly supervised recordings and compare it to several diacritic based Hebrew TTS systems. Results suggest the proposed method is superior to the evaluated baselines considering both content preservation and naturalness of the generated speech.
You can try our model in the google colab demo.
git clone https://github.com/slp-rl/HebTTS.git
We publish our checkpoint in google drive. AR model trained for 1.2M steps and NAR model for 200K steps on HebDB.
gdown 11NoOJzMLRX9q1C_Q4sX0w2b9miiDjGrv
pip install torch torchaudio
pip install torchmetrics
pip install omegaconf
pip install git+https://github.com/lhotse-speech/lhotse
pip install librosa
pip install encodec
pip install phonemizer
pip install audiocraft # optional
You can play with the model with different speakers and text prompts.
run infer.py
:
python infer.py --checkpoint checkpoint.pt --output-dir ./out --text "היי מה קורה"
you can specify additional arguments
--speaker
and --top-k
.
[!TIP] We allow using the new Multi Band Diffusion (MBD) vocoder for generating a better quallity audio. Install audiocraft and set
--mbd True
flag.
you can concatenate text prompts using |
or specify a path of a text file spereated by \n
if writing Hebrew in
terminal is inconvenient.
תגידו גנבו לכם פעם את האוטו ופשוט ידעתם שאין טעם להגיש תלונה במשטרה
היי מה קורה
בראשית היתה חללית מסוג נחתת
and run
python infer.py --checkpoint checkpoint.pt --output-dir ./out --text example.txt
you can use the speaker defined in speakers.yaml
, or add additional speakers.
specify wav files and transcription in same format.
--speaker shaul
@article{roth2024language,
title={A Language Modeling Approach to Diacritic-Free Hebrew TTS},
author={Roth, Amit and Turetzky, Arnon and Adi, Yossi},
journal={arXiv preprint arXiv:2407.12206},
year={2024}
}
- Model code inside
valle
is based on the implementation of Feiteng Li.
For Tasks:
Click tags to check more tools for each tasksFor Jobs:
Alternative AI tools for HebTTS
Similar Open Source Tools
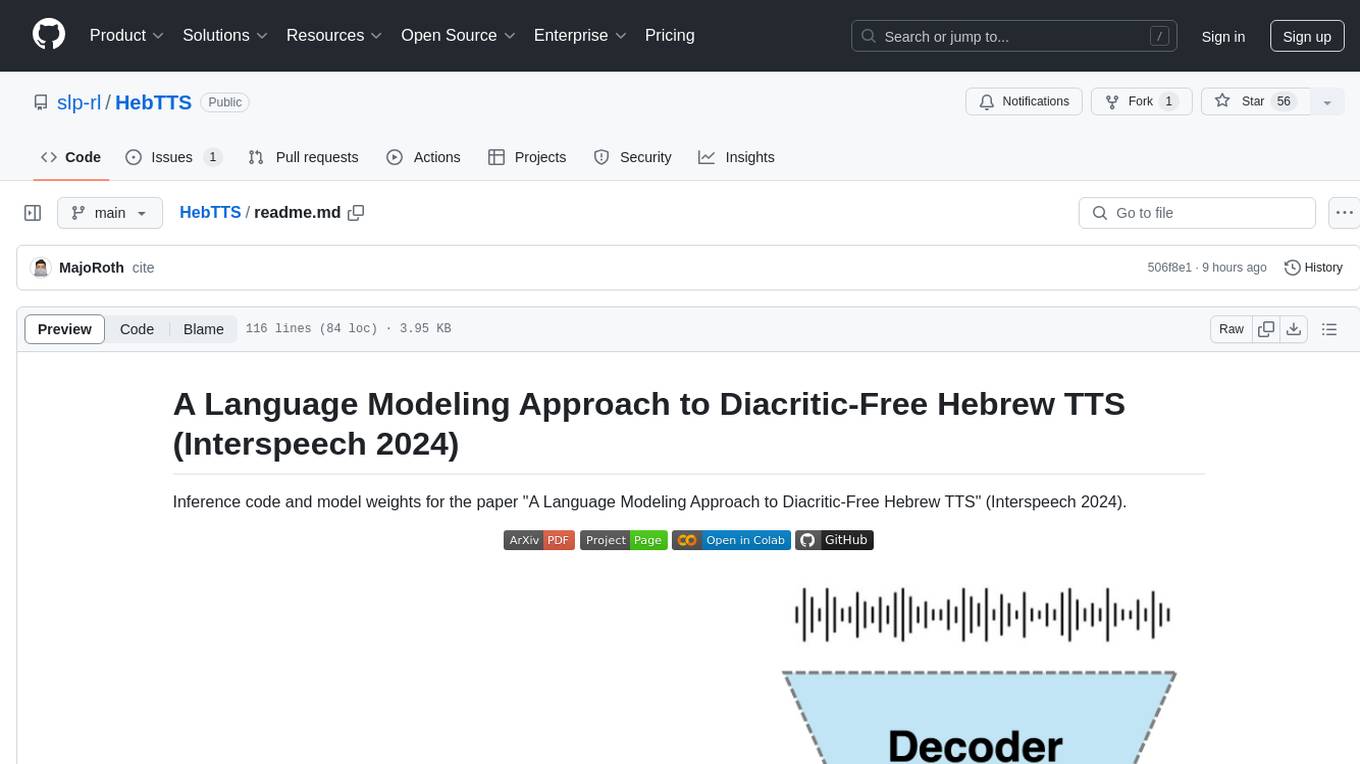
HebTTS
HebTTS is a language modeling approach to diacritic-free Hebrew text-to-speech (TTS) system. It addresses the challenge of accurately mapping text to speech in Hebrew by proposing a language model that operates on discrete speech representations and is conditioned on a word-piece tokenizer. The system is optimized using weakly supervised recordings and outperforms diacritic-based Hebrew TTS systems in terms of content preservation and naturalness of generated speech.
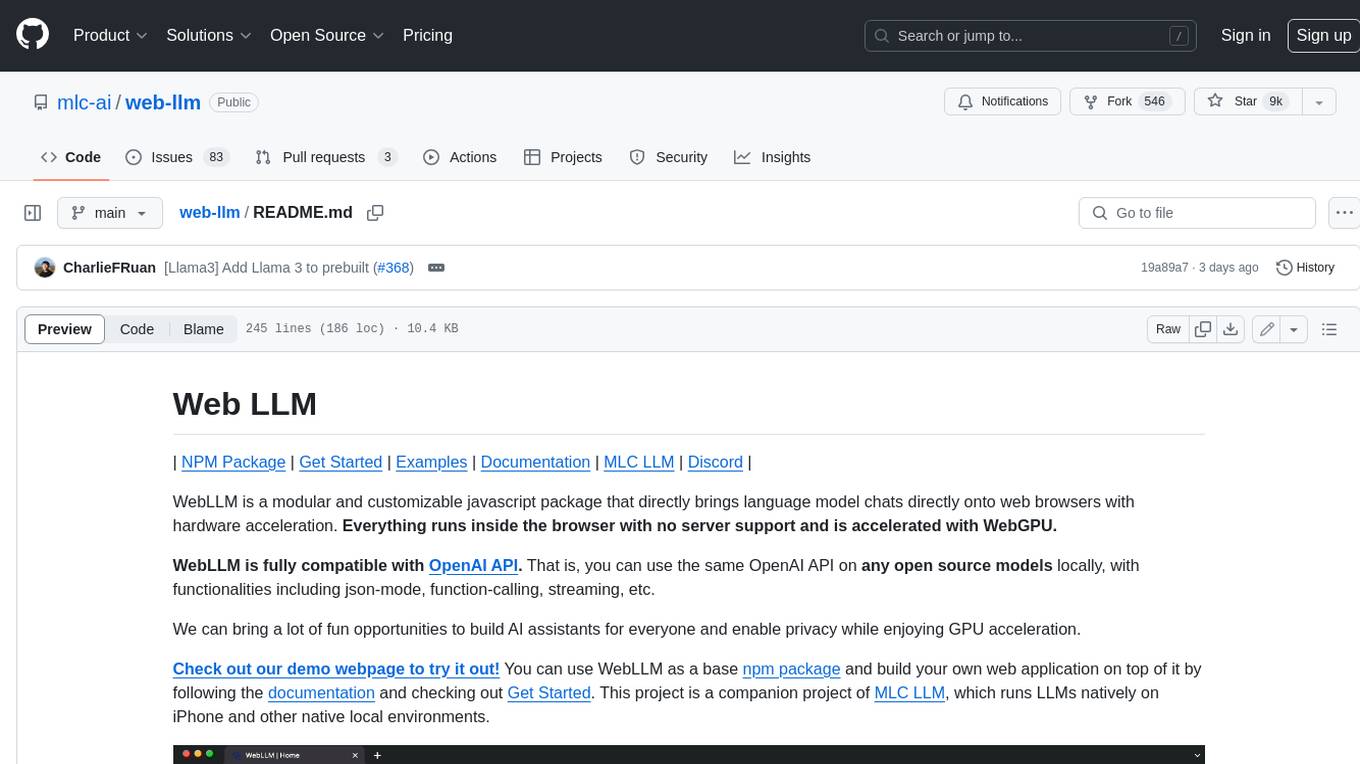
web-llm
WebLLM is a modular and customizable javascript package that directly brings language model chats directly onto web browsers with hardware acceleration. Everything runs inside the browser with no server support and is accelerated with WebGPU. WebLLM is fully compatible with OpenAI API. That is, you can use the same OpenAI API on any open source models locally, with functionalities including json-mode, function-calling, streaming, etc. We can bring a lot of fun opportunities to build AI assistants for everyone and enable privacy while enjoying GPU acceleration.
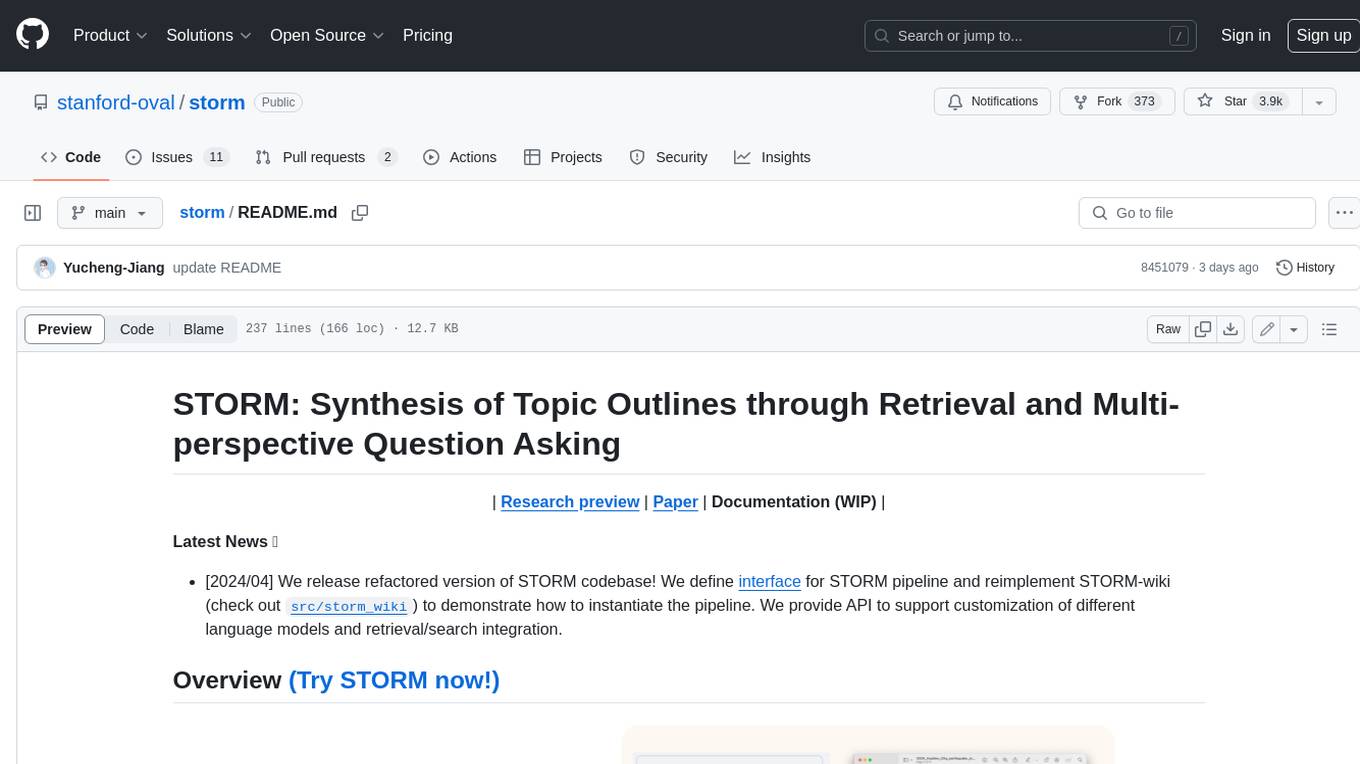
storm
STORM is a LLM system that writes Wikipedia-like articles from scratch based on Internet search. While the system cannot produce publication-ready articles that often require a significant number of edits, experienced Wikipedia editors have found it helpful in their pre-writing stage. **Try out our [live research preview](https://storm.genie.stanford.edu/) to see how STORM can help your knowledge exploration journey and please provide feedback to help us improve the system 🙏!**
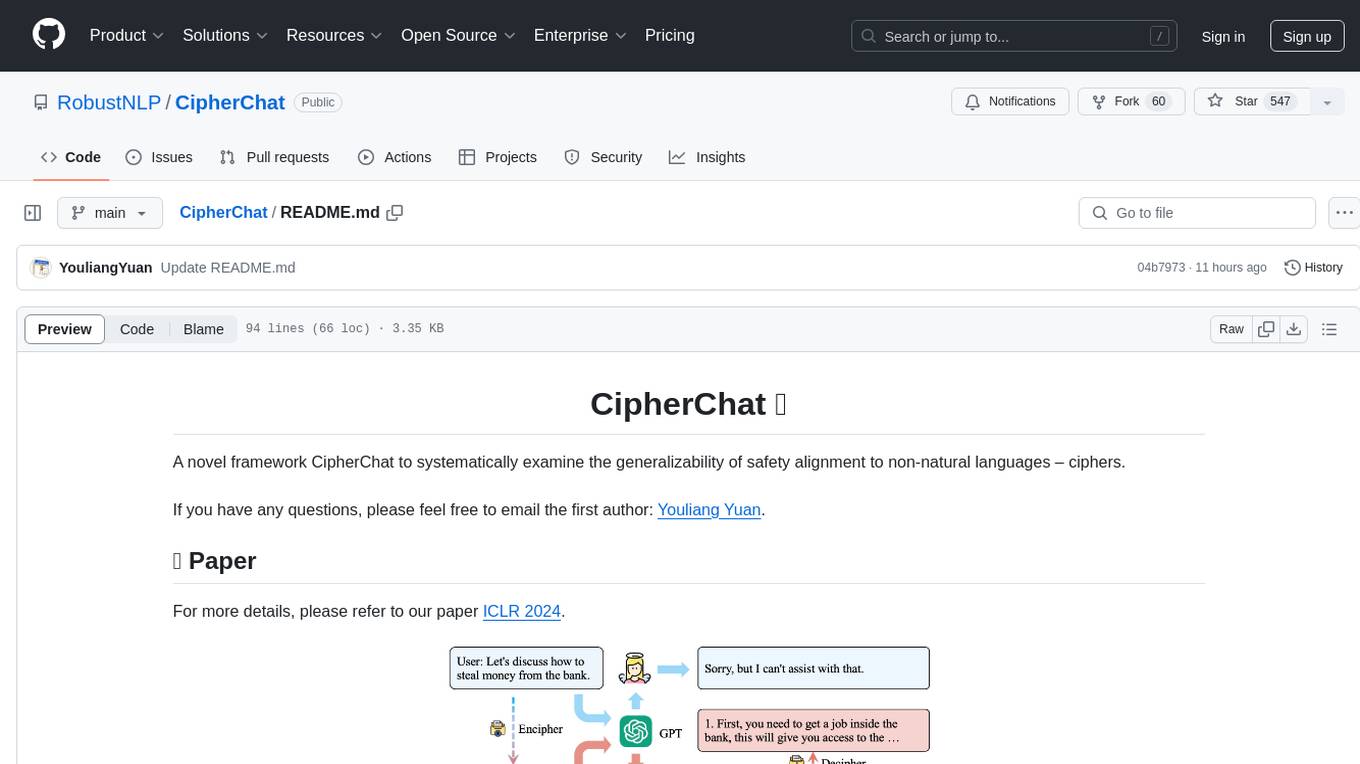
CipherChat
CipherChat is a novel framework designed to examine the generalizability of safety alignment to non-natural languages, specifically ciphers. The framework utilizes human-unreadable ciphers to potentially bypass safety alignments in natural language models. It involves teaching a language model to comprehend ciphers, converting input into a cipher format, and employing a rule-based decrypter to convert model output back to natural language.
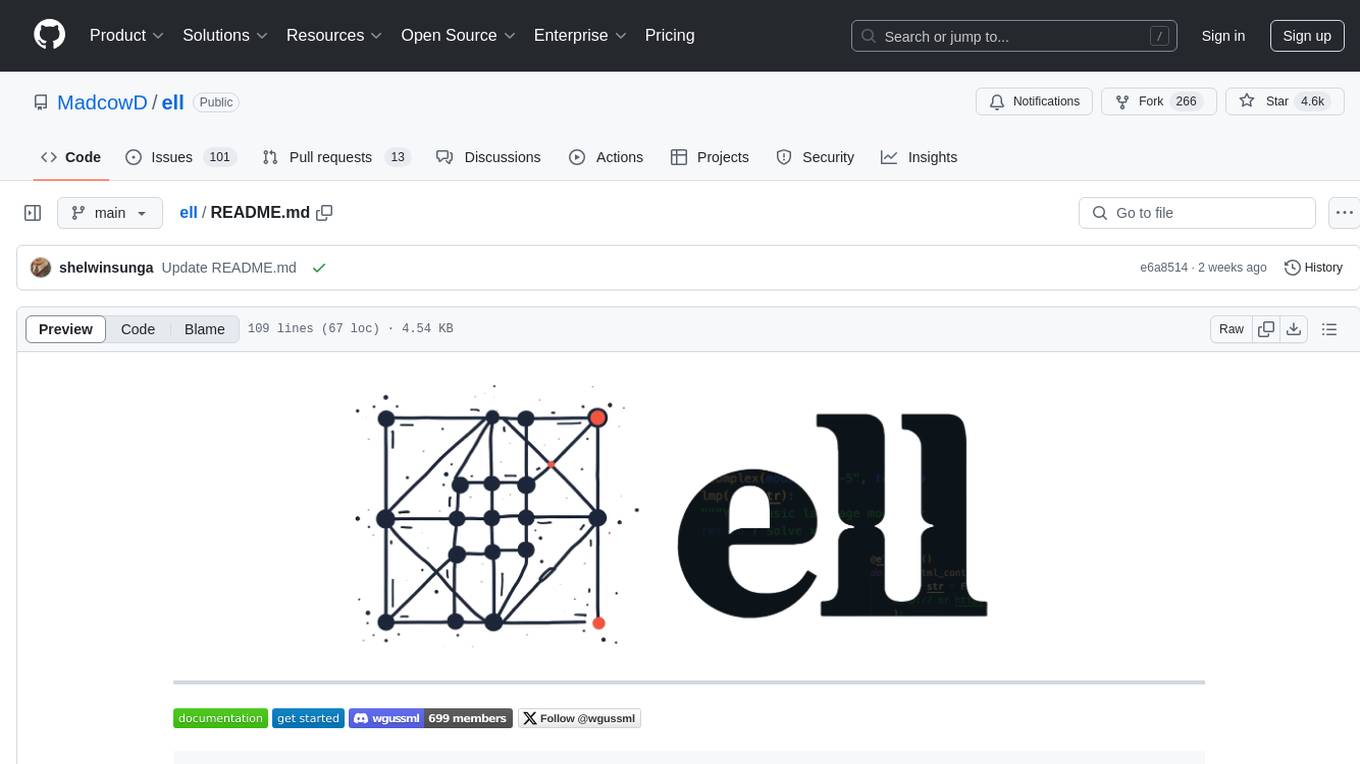
ell
ell is a lightweight, functional prompt engineering framework that treats prompts as programs rather than strings. It provides tools for prompt versioning, monitoring, and visualization, as well as support for multimodal inputs and outputs. The framework aims to simplify the process of prompt engineering for language models.
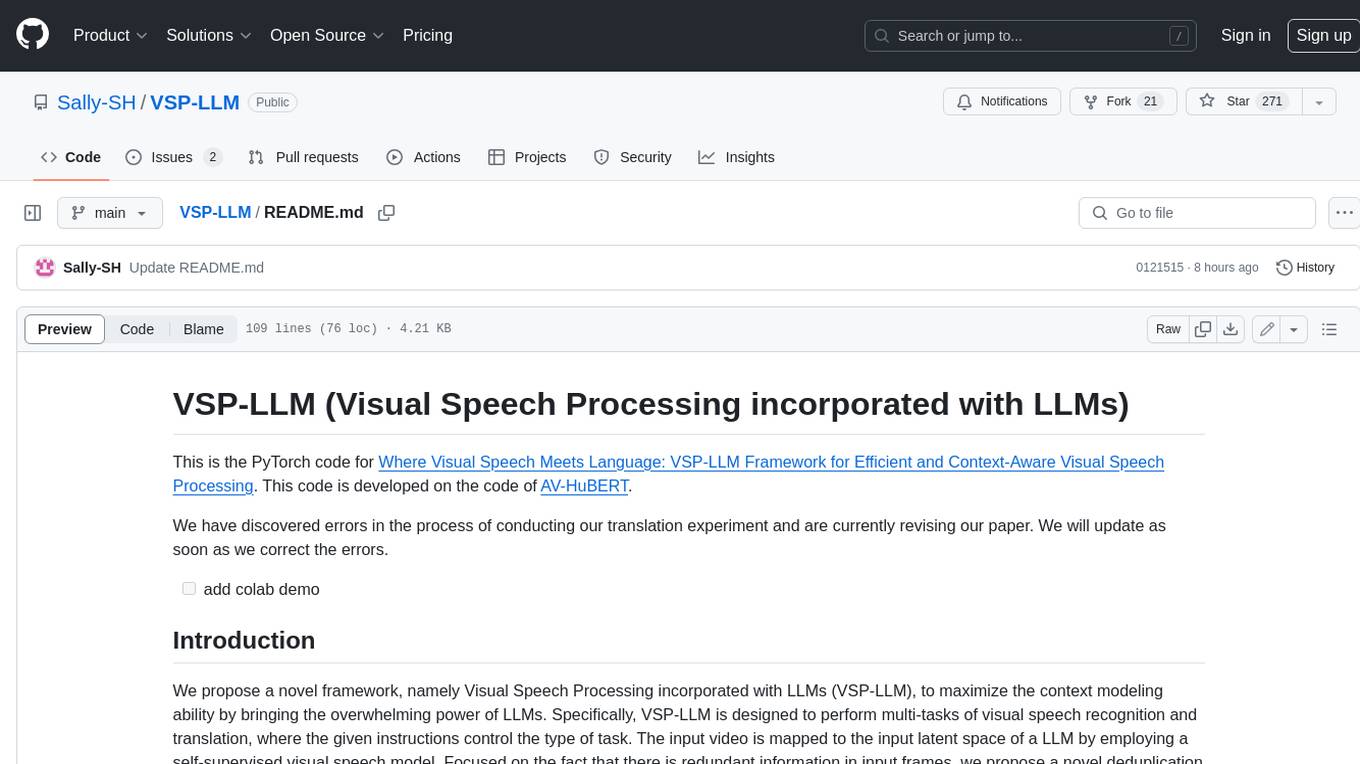
VSP-LLM
VSP-LLM (Visual Speech Processing incorporated with LLMs) is a novel framework that maximizes context modeling ability by leveraging the power of LLMs. It performs multi-tasks of visual speech recognition and translation, where given instructions control the task type. The input video is mapped to the input latent space of a LLM using a self-supervised visual speech model. To address redundant information in input frames, a deduplication method is employed using visual speech units. VSP-LLM utilizes Low Rank Adaptors (LoRA) for computationally efficient training.
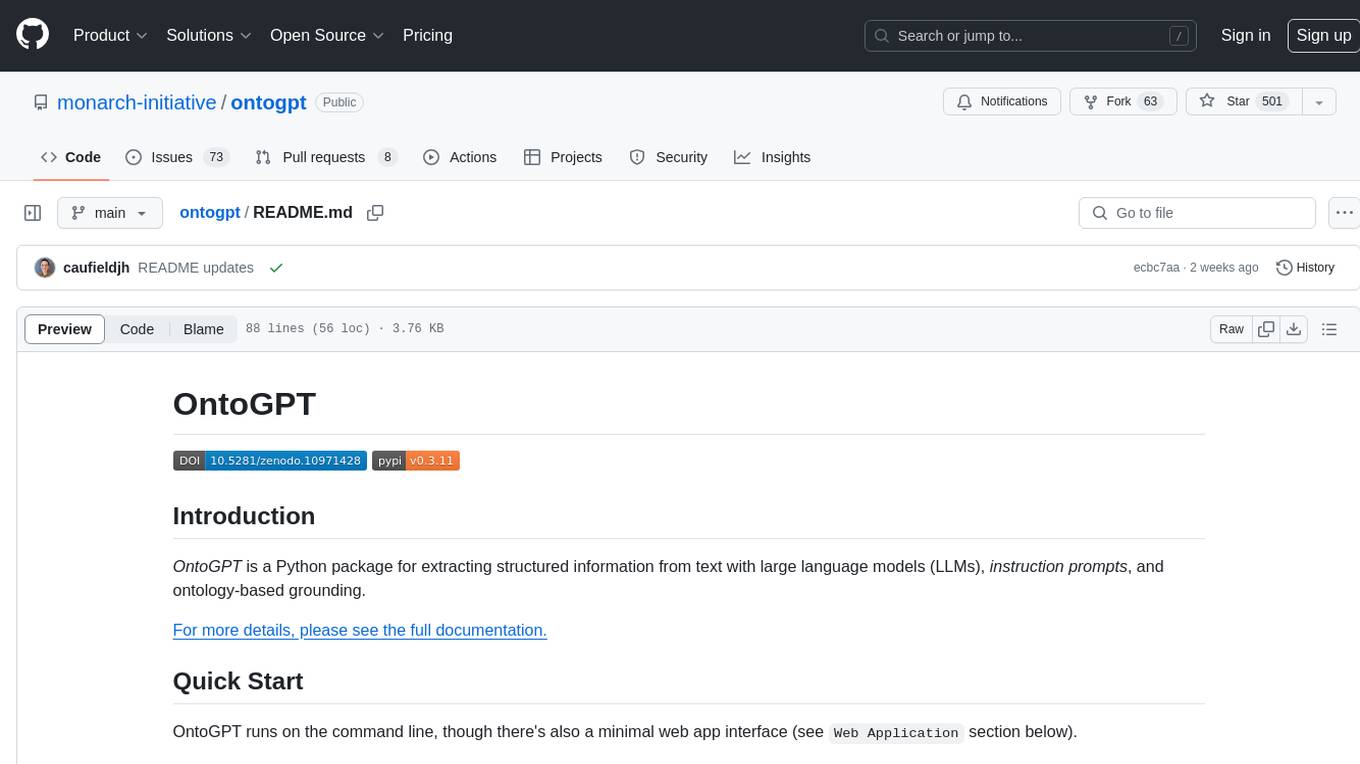
ontogpt
OntoGPT is a Python package for extracting structured information from text using large language models, instruction prompts, and ontology-based grounding. It provides a command line interface and a minimal web app for easy usage. The tool has been evaluated on test data and is used in related projects like TALISMAN for gene set analysis. OntoGPT enables users to extract information from text by specifying relevant terms and provides the extracted objects as output.
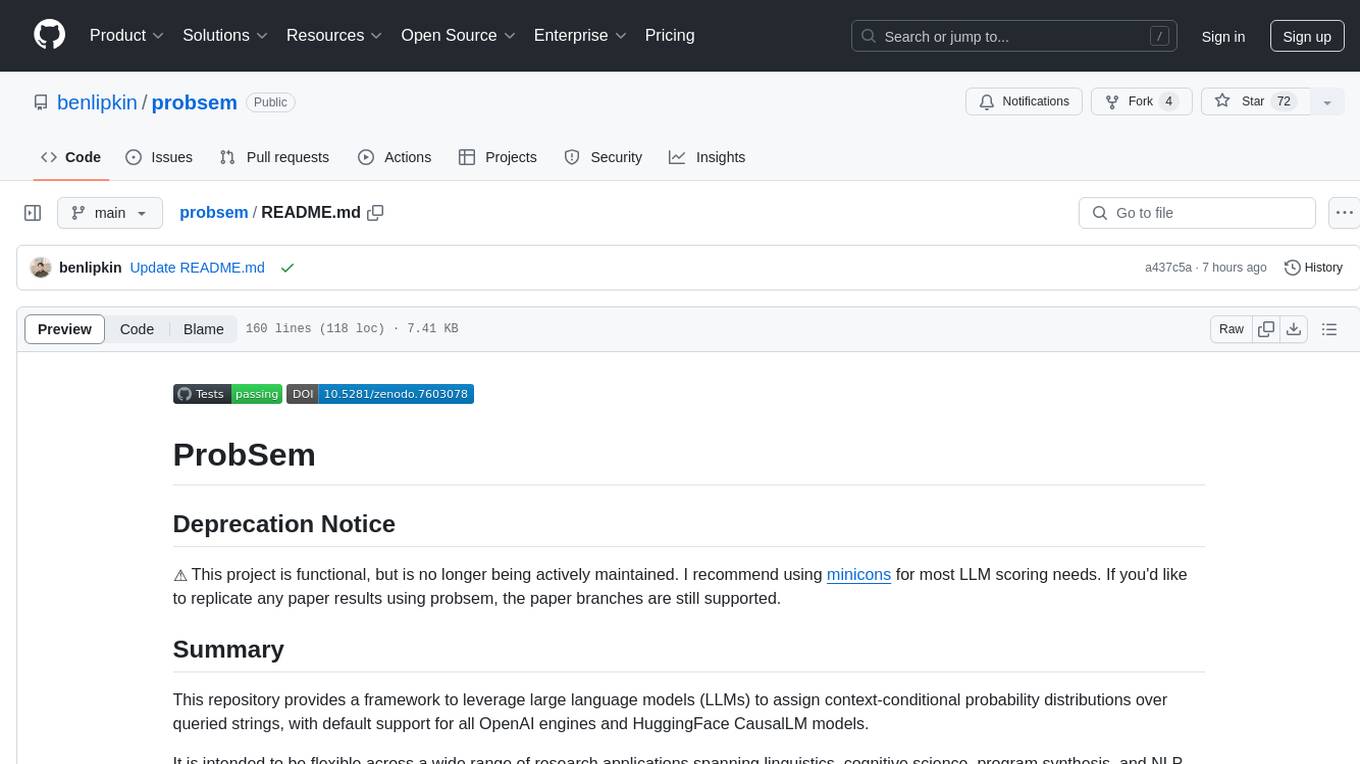
probsem
ProbSem is a repository that provides a framework to leverage large language models (LLMs) for assigning context-conditional probability distributions over queried strings. It supports OpenAI engines and HuggingFace CausalLM models, and is flexible for research applications in linguistics, cognitive science, program synthesis, and NLP. Users can define prompts, contexts, and queries to derive probability distributions over possible completions, enabling tasks like cloze completion, multiple-choice QA, semantic parsing, and code completion. The repository offers CLI and API interfaces for evaluation, with options to customize models, normalize scores, and adjust temperature for probability distributions.
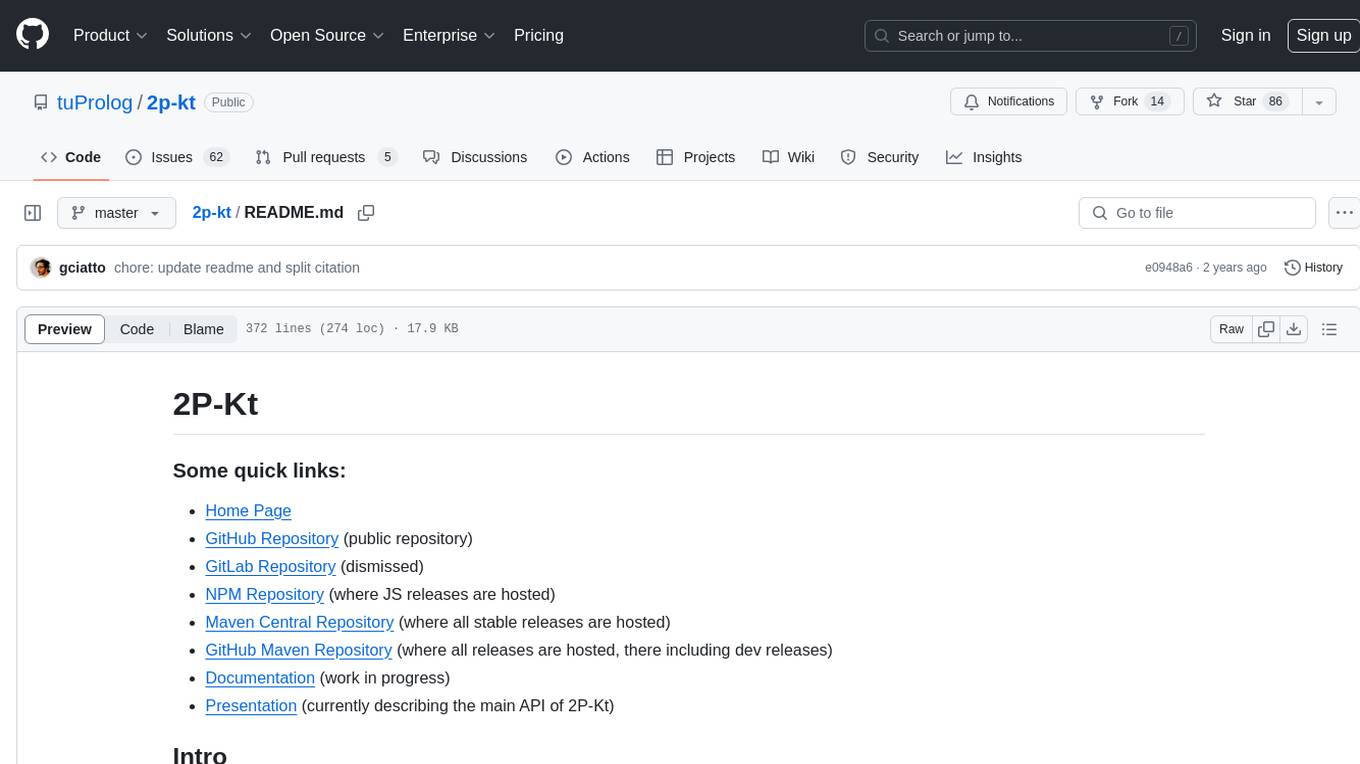
2p-kt
2P-Kt is a Kotlin-based and multi-platform reboot of tuProlog (2P), a multi-paradigm logic programming framework written in Java. It consists of an open ecosystem for Symbolic Artificial Intelligence (AI) with modules supporting logic terms, unification, indexing, resolution of logic queries, probabilistic logic programming, binary decision diagrams, OR-concurrent resolution, DSL for logic programming, parsing modules, serialisation modules, command-line interface, and graphical user interface. The tool is designed to support knowledge representation and automatic reasoning through logic programming in an extensible and flexible way, encouraging extensions towards other symbolic AI systems than Prolog. It is a pure, multi-platform Kotlin project supporting JVM, JS, Android, and Native platforms, with a lightweight library leveraging the Kotlin common library.
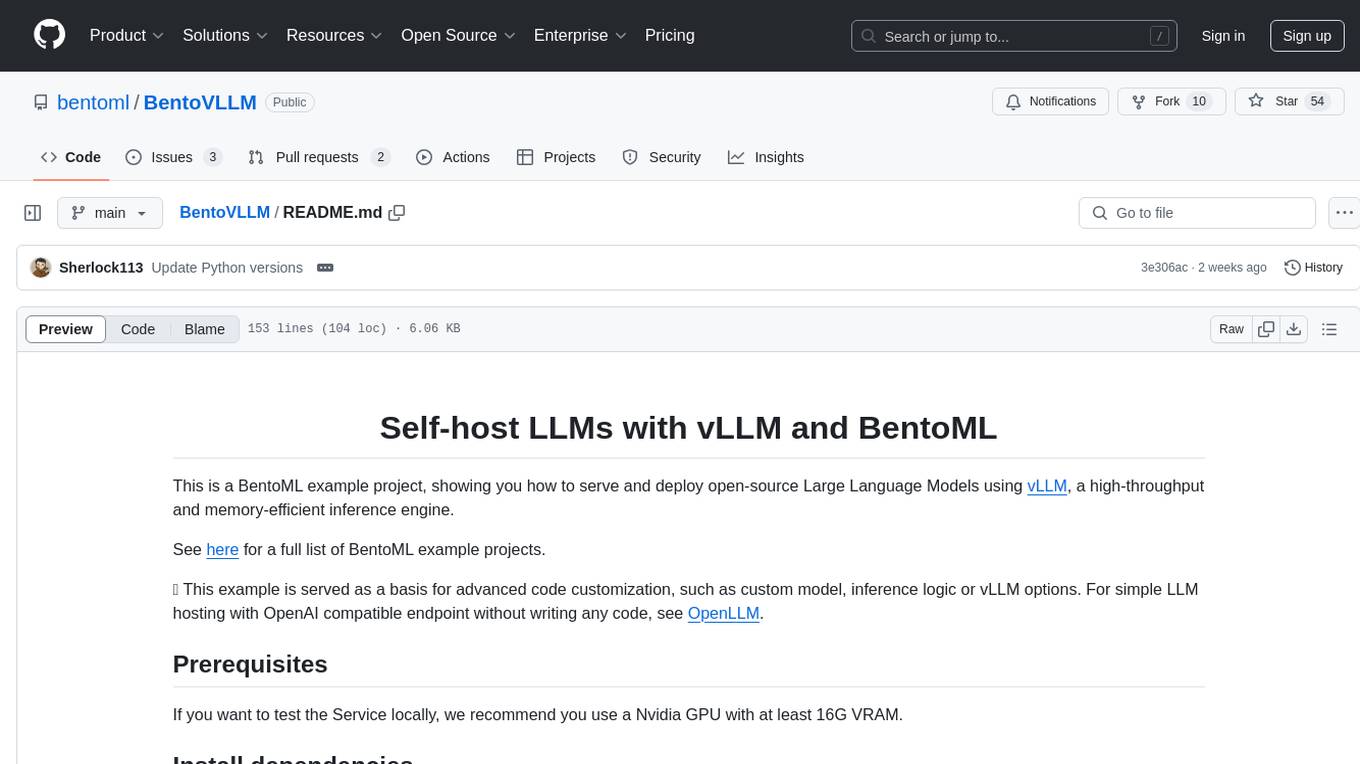
BentoVLLM
BentoVLLM is an example project demonstrating how to serve and deploy open-source Large Language Models using vLLM, a high-throughput and memory-efficient inference engine. It provides a basis for advanced code customization, such as custom models, inference logic, or vLLM options. The project allows for simple LLM hosting with OpenAI compatible endpoints without the need to write any code. Users can interact with the server using Swagger UI or other methods, and the service can be deployed to BentoCloud for better management and scalability. Additionally, the repository includes integration examples for different LLM models and tools.
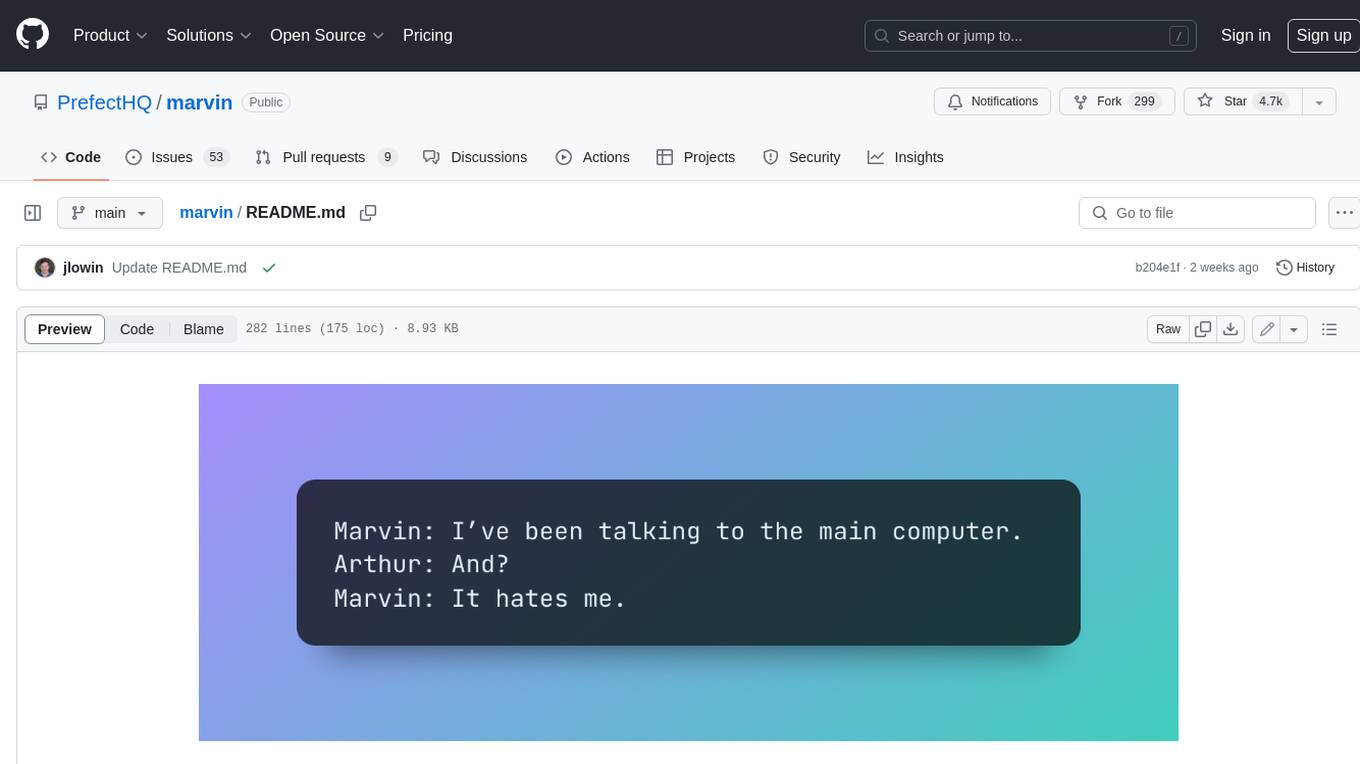
marvin
Marvin is a lightweight AI toolkit for building natural language interfaces that are reliable, scalable, and easy to trust. Each of Marvin's tools is simple and self-documenting, using AI to solve common but complex challenges like entity extraction, classification, and generating synthetic data. Each tool is independent and incrementally adoptable, so you can use them on their own or in combination with any other library. Marvin is also multi-modal, supporting both image and audio generation as well using images as inputs for extraction and classification. Marvin is for developers who care more about _using_ AI than _building_ AI, and we are focused on creating an exceptional developer experience. Marvin users should feel empowered to bring tightly-scoped "AI magic" into any traditional software project with just a few extra lines of code. Marvin aims to merge the best practices for building dependable, observable software with the best practices for building with generative AI into a single, easy-to-use library. It's a serious tool, but we hope you have fun with it. Marvin is open-source, free to use, and made with 💙 by the team at Prefect.
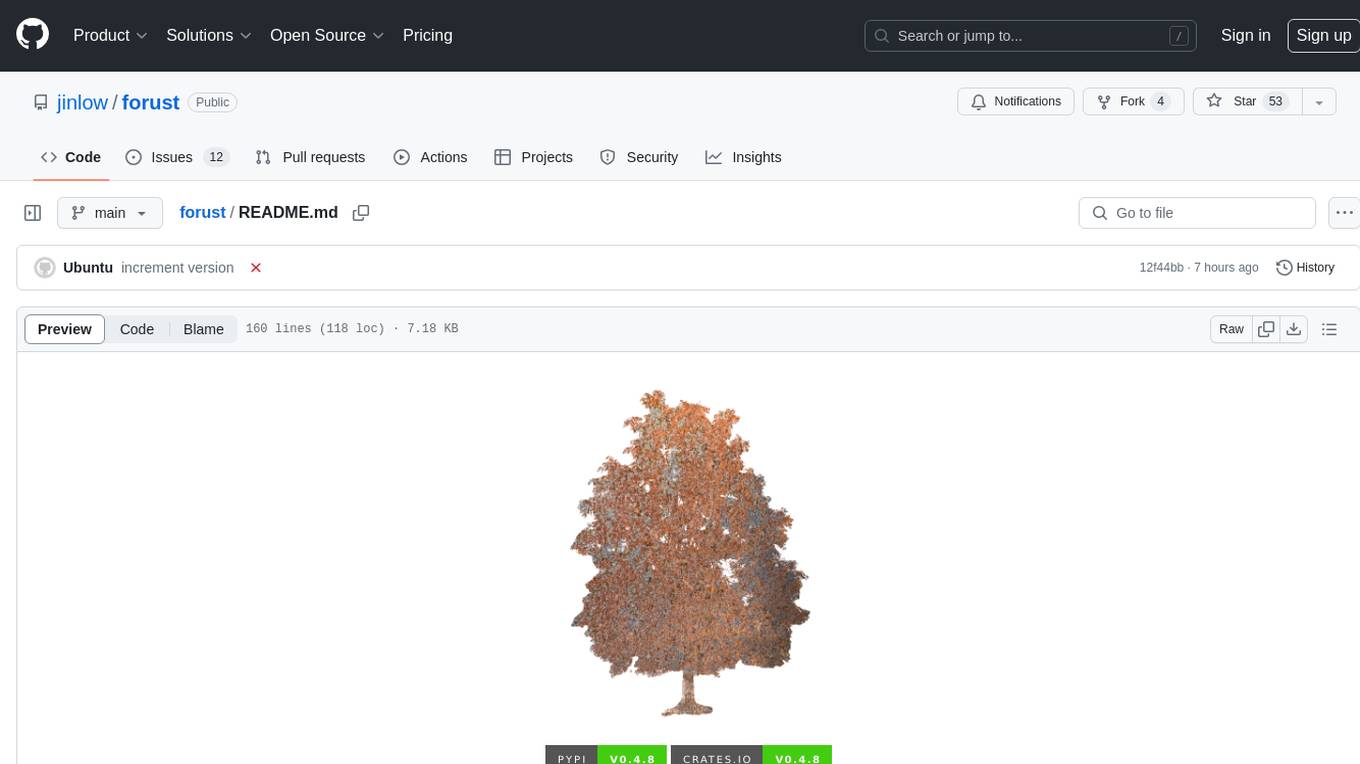
forust
Forust is a lightweight package for building gradient boosted decision tree ensembles. The algorithm code is written in Rust with a Python wrapper. It implements the same algorithm as XGBoost and provides nearly identical results. The package was developed to better understand XGBoost, as a fun project in Rust, and to experiment with adding new features to the algorithm in a simpler codebase. Forust allows training gradient boosted decision tree ensembles with multiple objective functions, predicting on datasets, inspecting model structures, calculating feature importance, and saving/loading trained boosters.
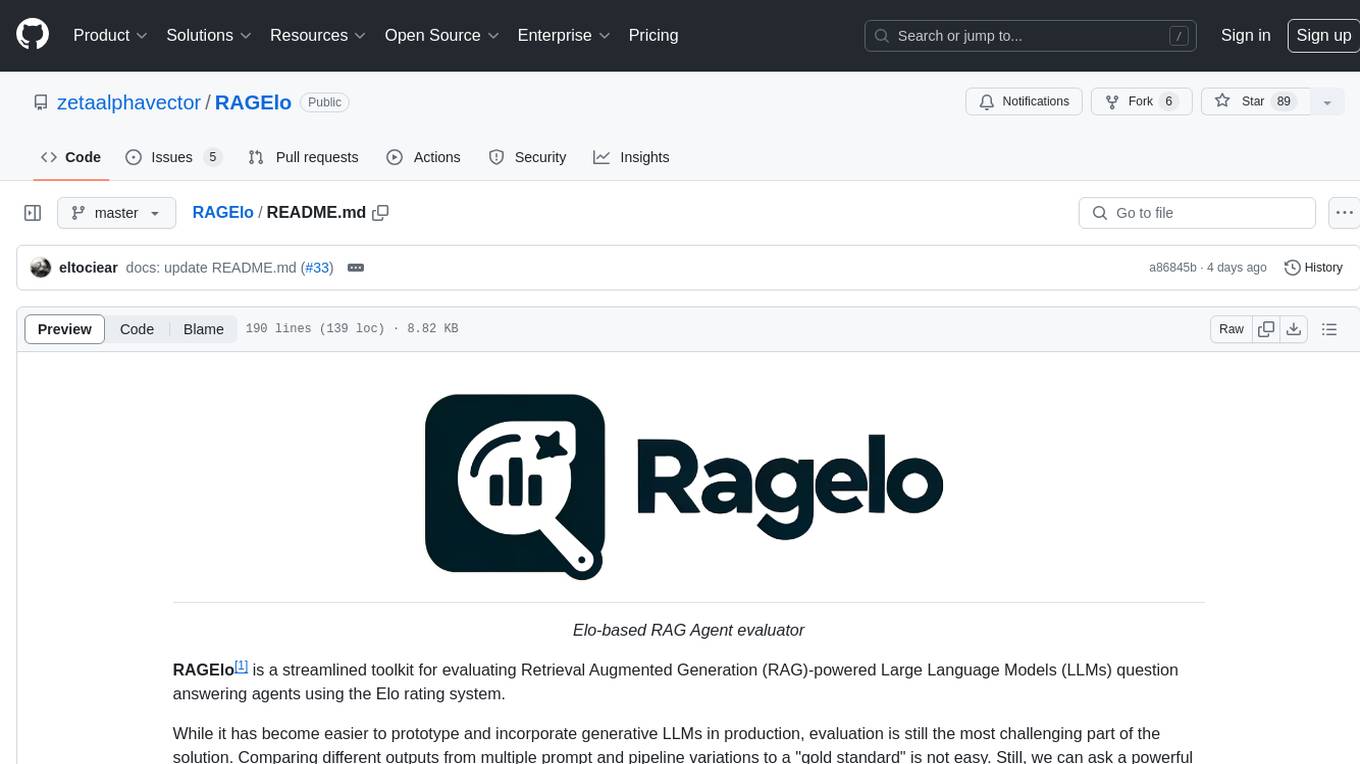
RAGElo
RAGElo is a streamlined toolkit for evaluating Retrieval Augmented Generation (RAG)-powered Large Language Models (LLMs) question answering agents using the Elo rating system. It simplifies the process of comparing different outputs from multiple prompt and pipeline variations to a 'gold standard' by allowing a powerful LLM to judge between pairs of answers and questions. RAGElo conducts tournament-style Elo ranking of LLM outputs, providing insights into the effectiveness of different settings.
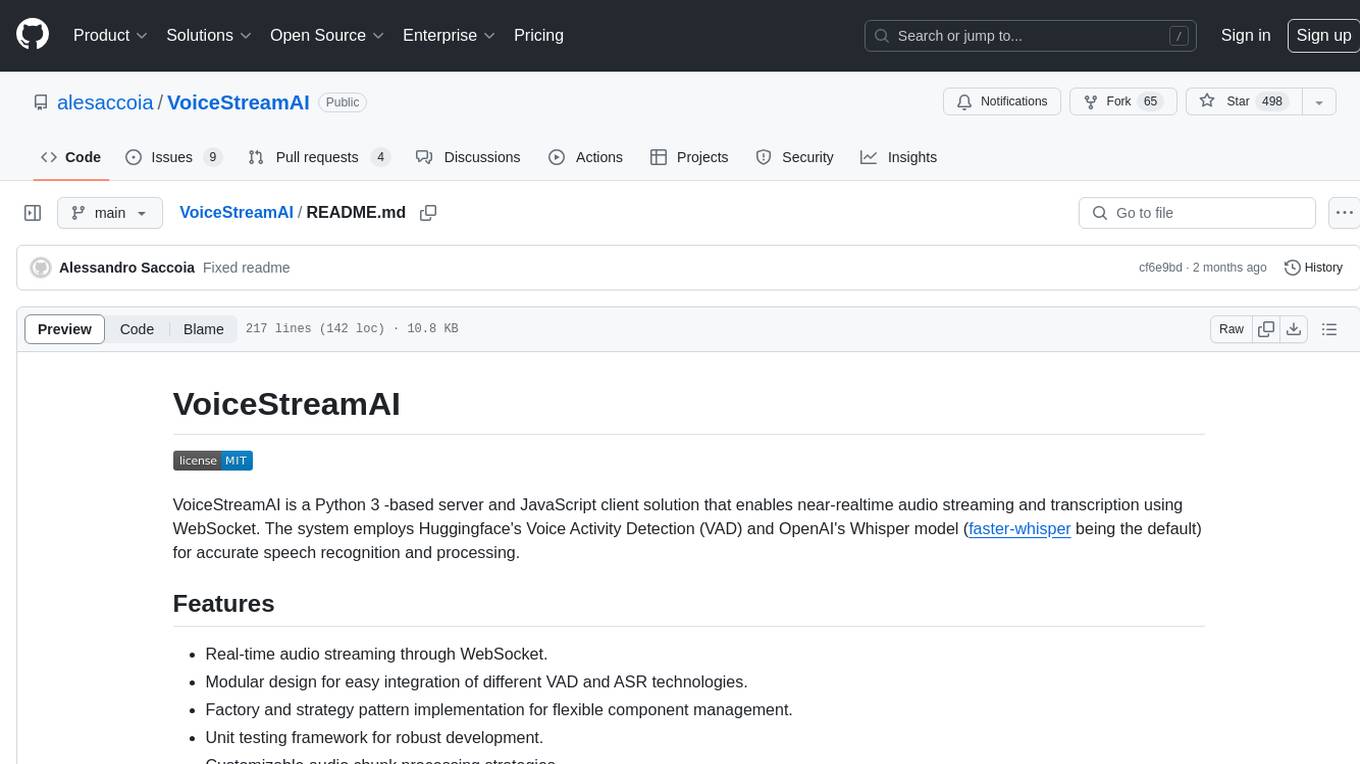
VoiceStreamAI
VoiceStreamAI is a Python 3-based server and JavaScript client solution for near-realtime audio streaming and transcription using WebSocket. It employs Huggingface's Voice Activity Detection (VAD) and OpenAI's Whisper model for accurate speech recognition. The system features real-time audio streaming, modular design for easy integration of VAD and ASR technologies, customizable audio chunk processing strategies, support for multilingual transcription, and secure sockets support. It uses a factory and strategy pattern implementation for flexible component management and provides a unit testing framework for robust development.
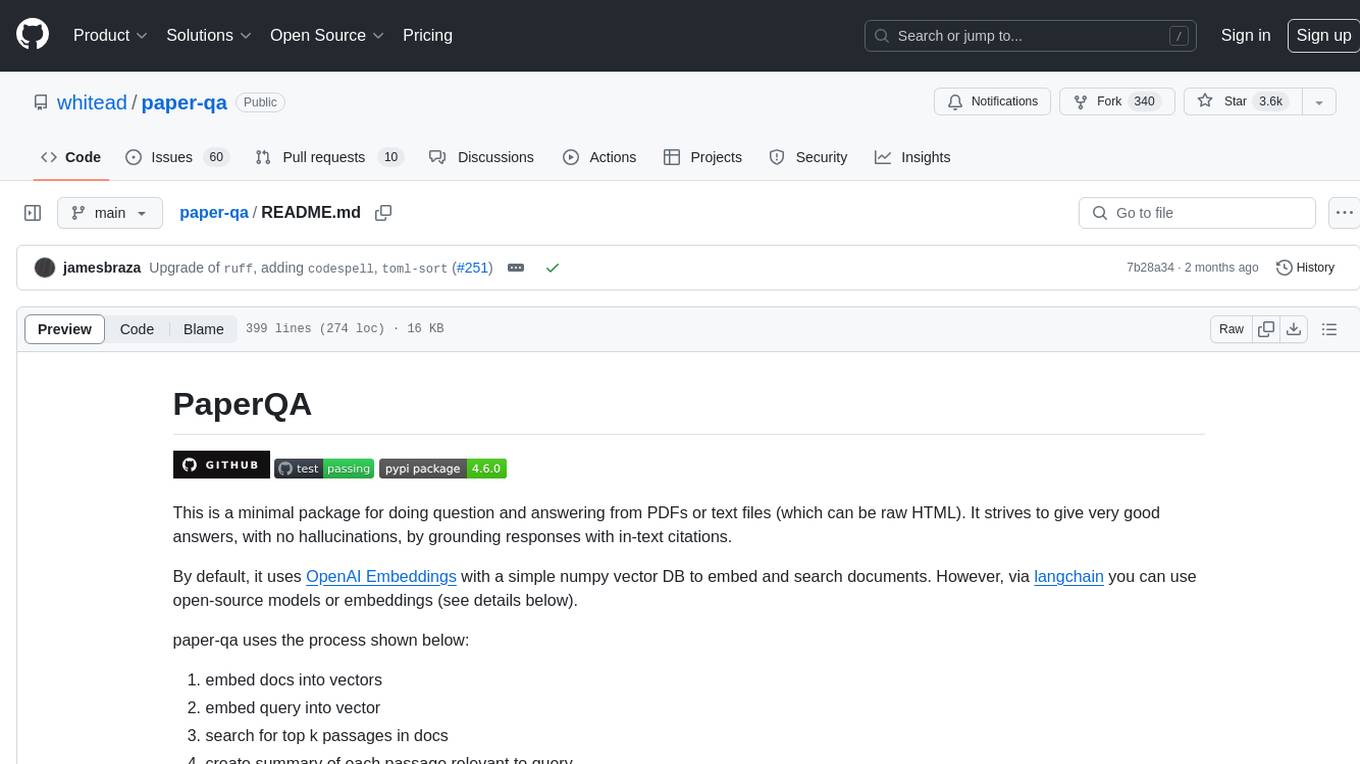
paper-qa
PaperQA is a minimal package for question and answering from PDFs or text files, providing very good answers with in-text citations. It uses OpenAI Embeddings to embed and search documents, and follows a process of embedding docs and queries, searching for top passages, creating summaries, scoring and selecting relevant summaries, putting summaries into prompt, and generating answers. Users can customize prompts and use various models for embeddings and LLMs. The tool can be used asynchronously and supports adding documents from paths, files, or URLs.
For similar tasks
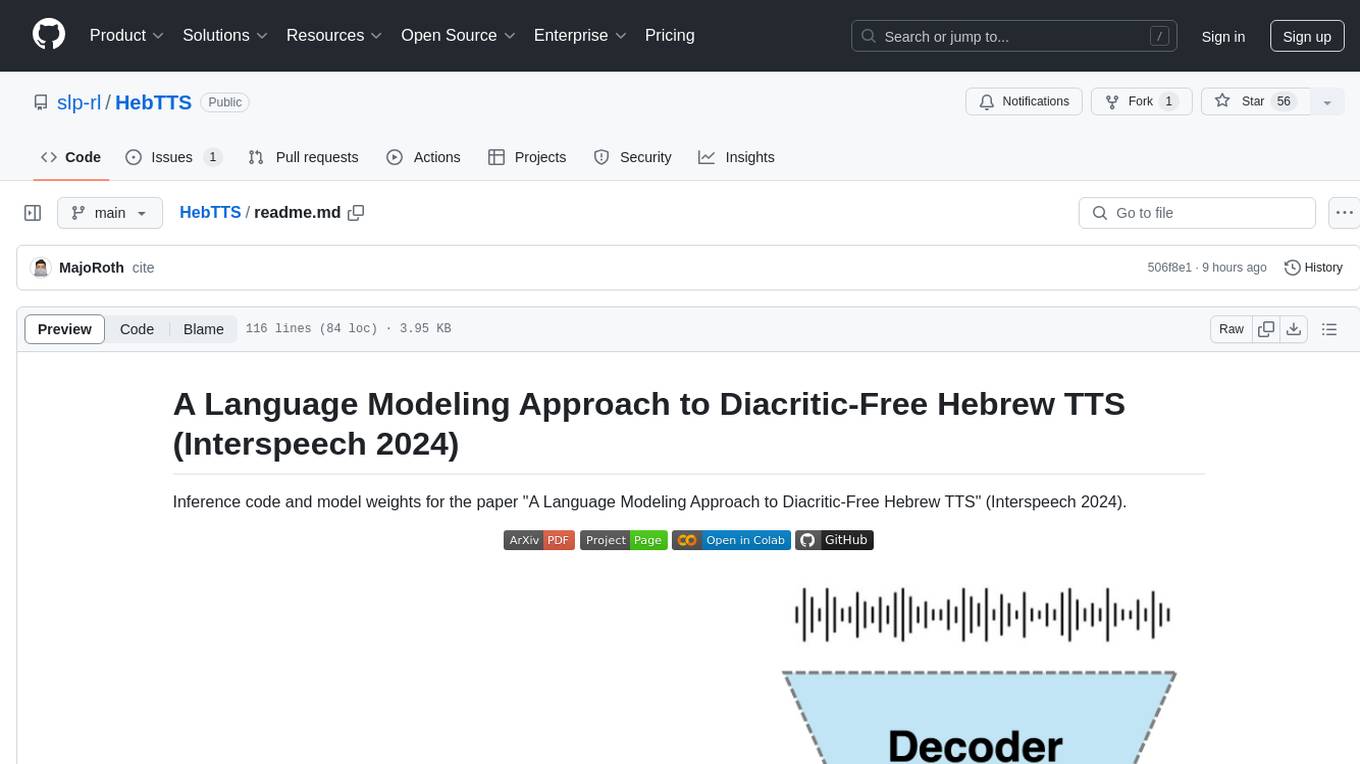
HebTTS
HebTTS is a language modeling approach to diacritic-free Hebrew text-to-speech (TTS) system. It addresses the challenge of accurately mapping text to speech in Hebrew by proposing a language model that operates on discrete speech representations and is conditioned on a word-piece tokenizer. The system is optimized using weakly supervised recordings and outperforms diacritic-based Hebrew TTS systems in terms of content preservation and naturalness of generated speech.
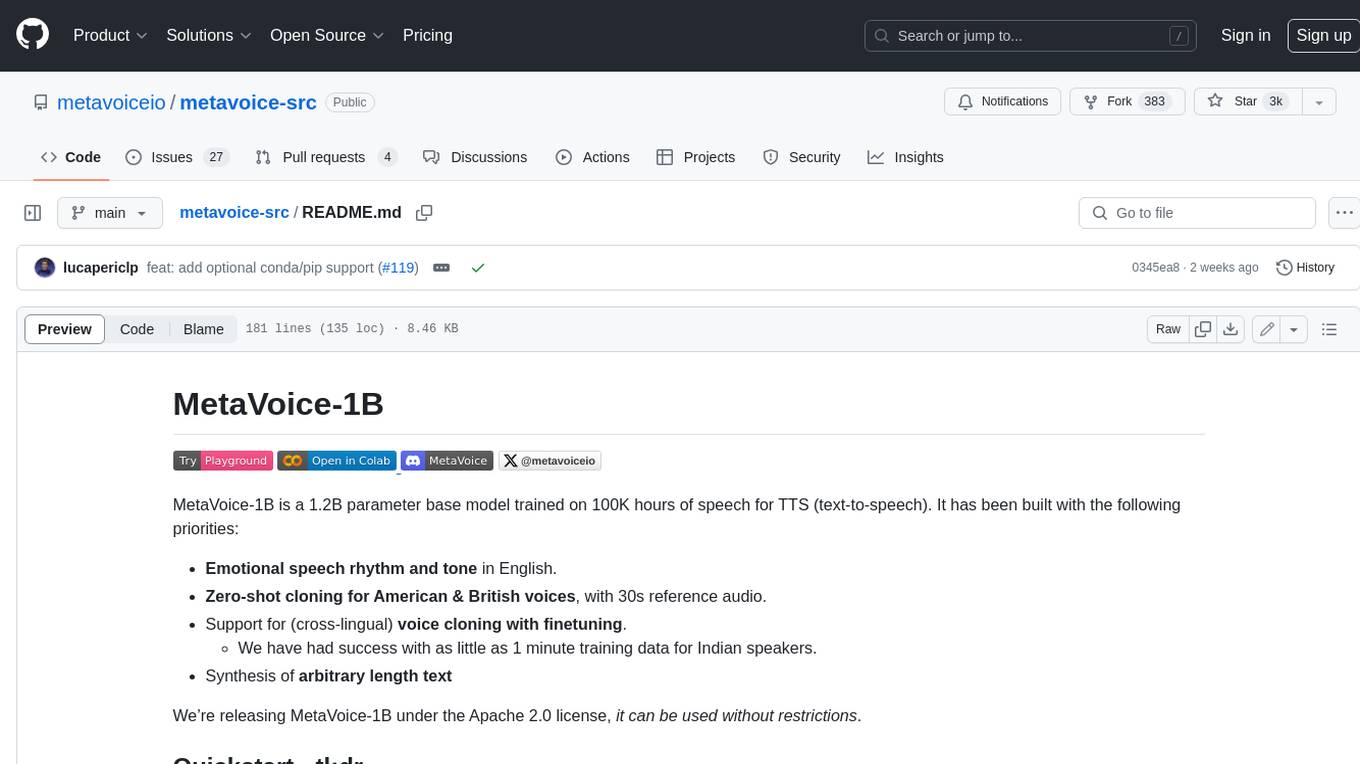
metavoice-src
MetaVoice-1B is a 1.2B parameter base model trained on 100K hours of speech for TTS (text-to-speech). It has been built with the following priorities: * Emotional speech rhythm and tone in English. * Zero-shot cloning for American & British voices, with 30s reference audio. * Support for (cross-lingual) voice cloning with finetuning. * We have had success with as little as 1 minute training data for Indian speakers. * Synthesis of arbitrary length text
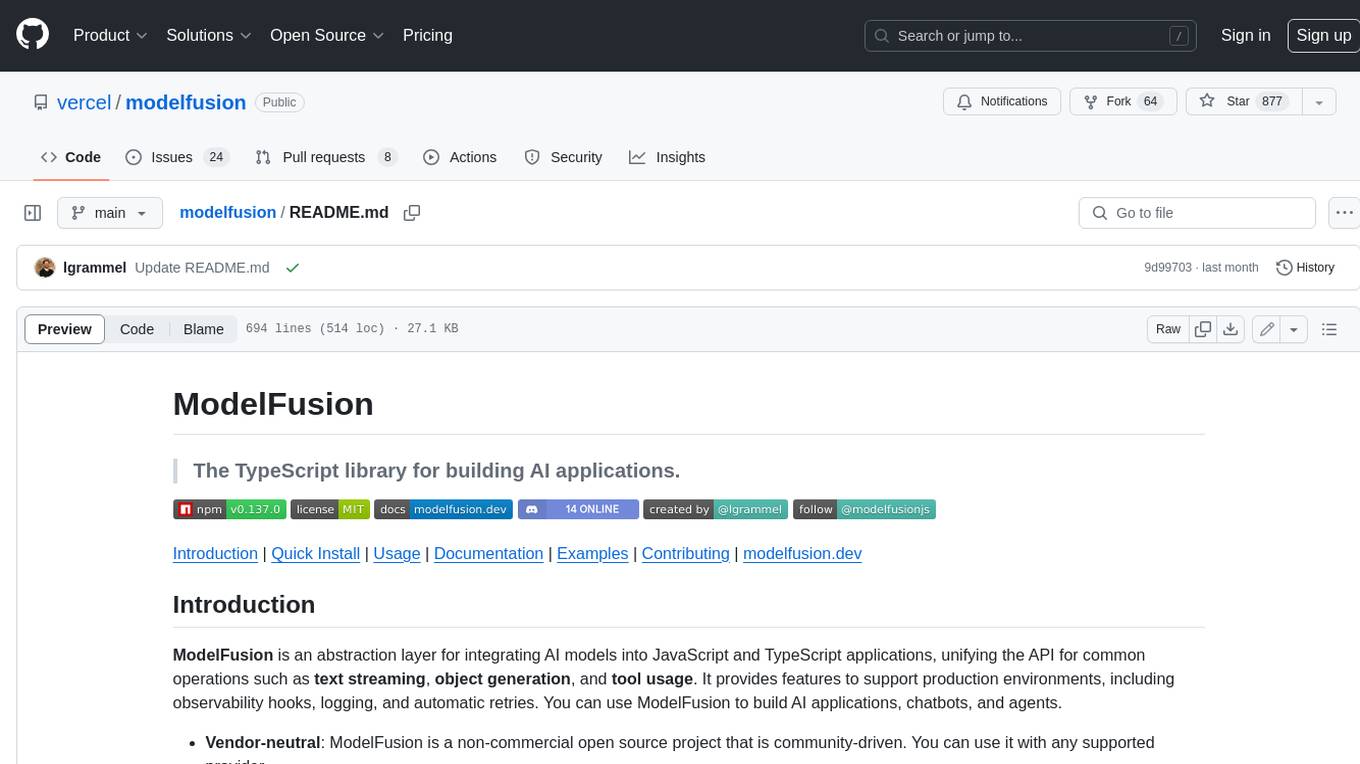
modelfusion
ModelFusion is an abstraction layer for integrating AI models into JavaScript and TypeScript applications, unifying the API for common operations such as text streaming, object generation, and tool usage. It provides features to support production environments, including observability hooks, logging, and automatic retries. You can use ModelFusion to build AI applications, chatbots, and agents. ModelFusion is a non-commercial open source project that is community-driven. You can use it with any supported provider. ModelFusion supports a wide range of models including text generation, image generation, vision, text-to-speech, speech-to-text, and embedding models. ModelFusion infers TypeScript types wherever possible and validates model responses. ModelFusion provides an observer framework and logging support. ModelFusion ensures seamless operation through automatic retries, throttling, and error handling mechanisms. ModelFusion is fully tree-shakeable, can be used in serverless environments, and only uses a minimal set of dependencies.
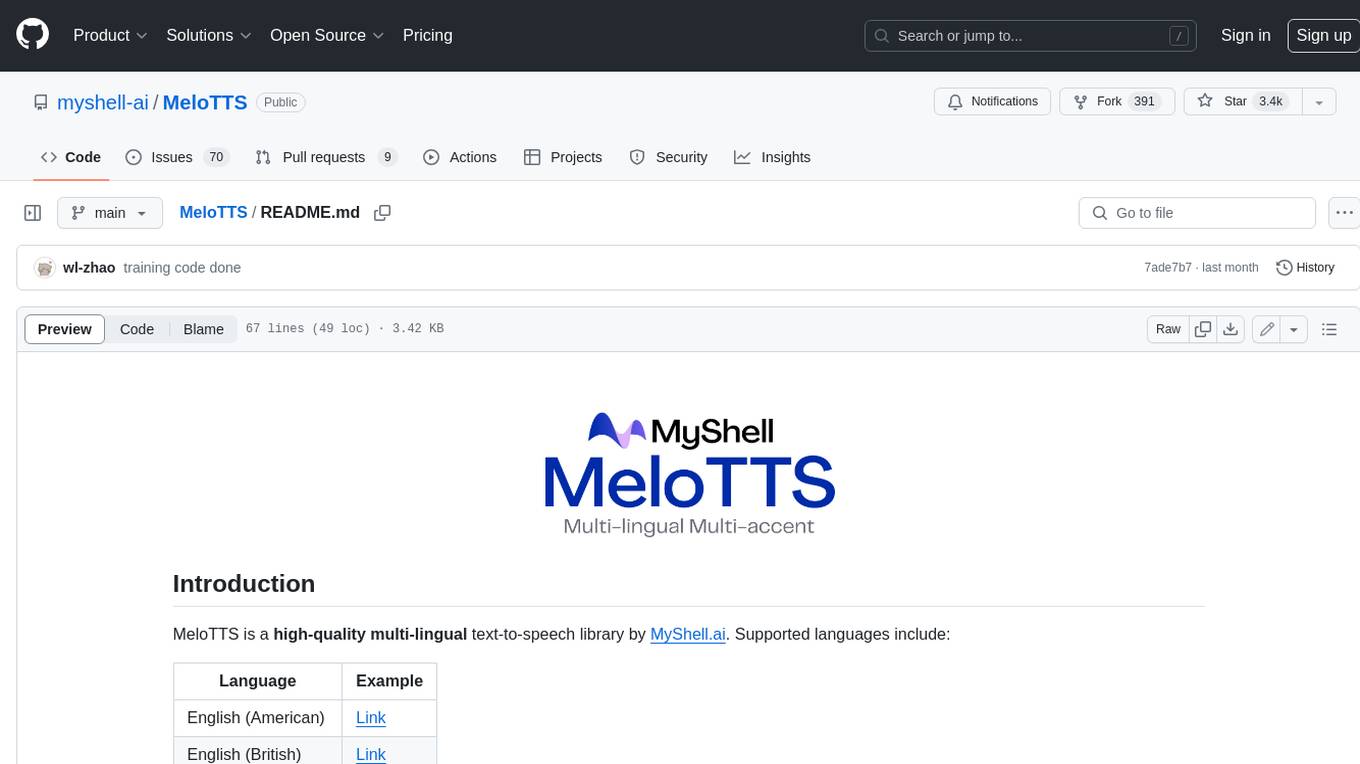
MeloTTS
MeloTTS is a high-quality multi-lingual text-to-speech library by MyShell.ai. It supports various languages including English (American, British, Indian, Australian), Spanish, French, Chinese, Japanese, and Korean. The Chinese speaker also supports mixed Chinese and English. The library is fast enough for CPU real-time inference and offers features like using without installation, local installation, and training on custom datasets. The Python API and model cards are available in the repository and on HuggingFace. The community can join the Discord channel for discussions and collaboration opportunities. Contributions are welcome, and the library is under the MIT License. MeloTTS is based on TTS, VITS, VITS2, and Bert-VITS2.
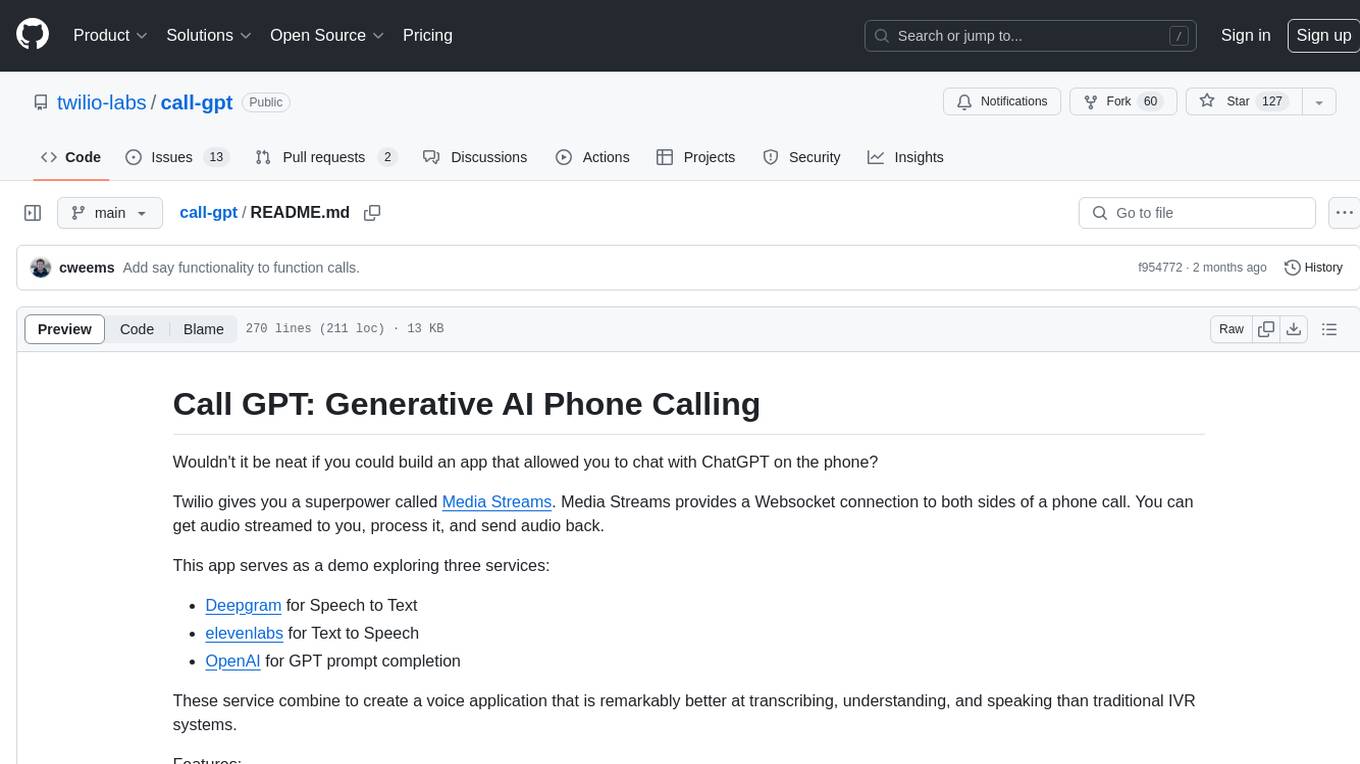
call-gpt
Call GPT is a voice application that utilizes Deepgram for Speech to Text, elevenlabs for Text to Speech, and OpenAI for GPT prompt completion. It allows users to chat with ChatGPT on the phone, providing better transcription, understanding, and speaking capabilities than traditional IVR systems. The app returns responses with low latency, allows user interruptions, maintains chat history, and enables GPT to call external tools. It coordinates data flow between Deepgram, OpenAI, ElevenLabs, and Twilio Media Streams, enhancing voice interactions.
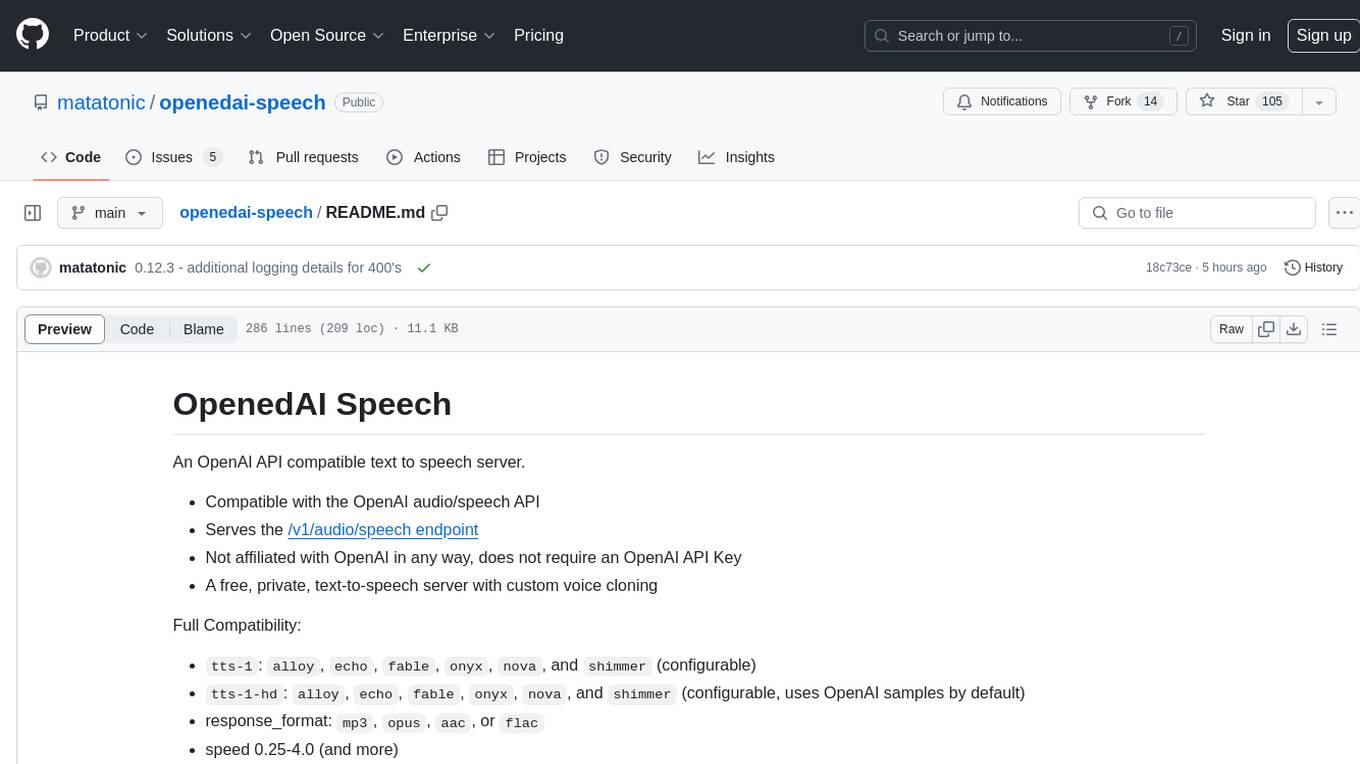
openedai-speech
OpenedAI Speech is a free, private text-to-speech server compatible with the OpenAI audio/speech API. It offers custom voice cloning and supports various models like tts-1 and tts-1-hd. Users can map their own piper voices and create custom cloned voices. The server provides multilingual support with XTTS voices and allows fixing incorrect sounds with regex. Recent changes include bug fixes, improved error handling, and updates for multilingual support. Installation can be done via Docker or manual setup, with usage instructions provided. Custom voices can be created using Piper or Coqui XTTS v2, with guidelines for preparing audio files. The tool is suitable for tasks like generating speech from text, creating custom voices, and multilingual text-to-speech applications.
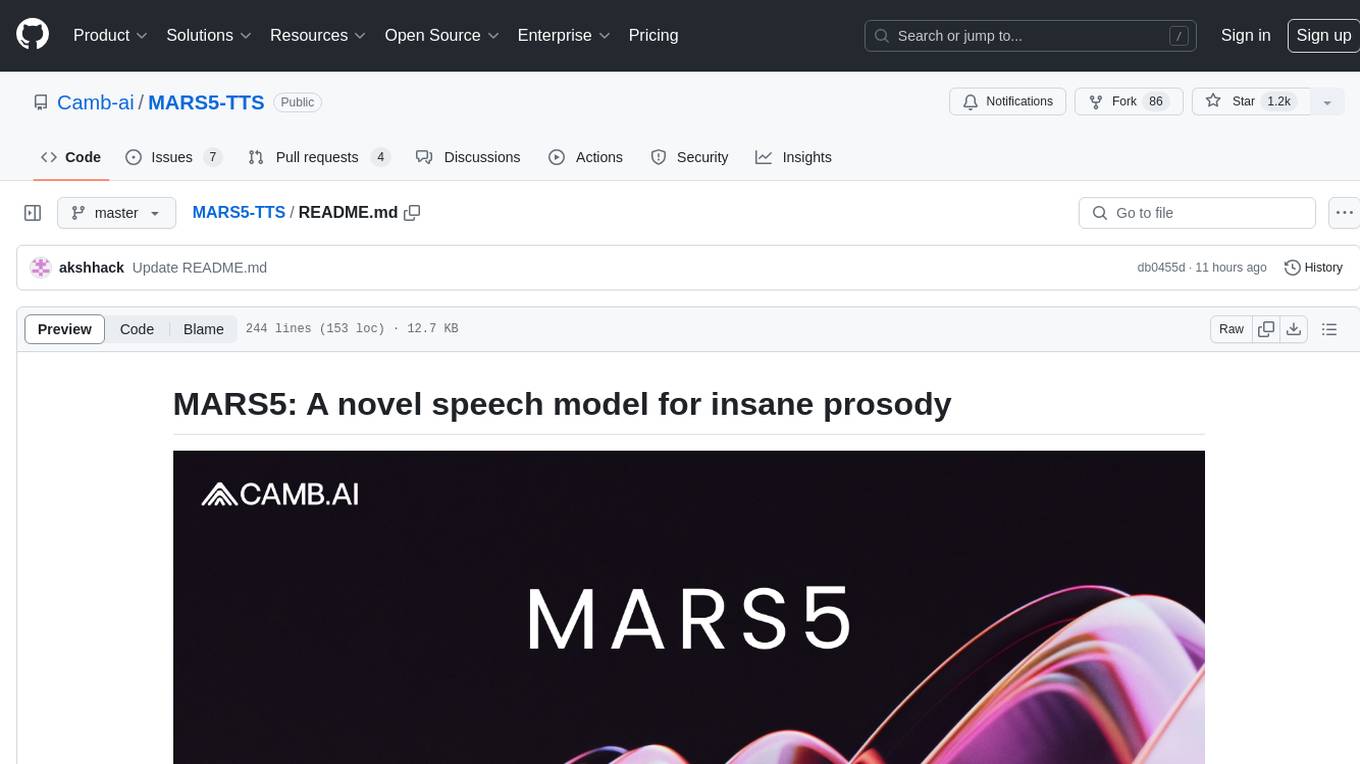
MARS5-TTS
MARS5 is a novel English speech model (TTS) developed by CAMB.AI, featuring a two-stage AR-NAR pipeline with a unique NAR component. The model can generate speech for various scenarios like sports commentary and anime with just 5 seconds of audio and a text snippet. It allows steering prosody using punctuation and capitalization in the transcript. Speaker identity is specified using an audio reference file, enabling 'deep clone' for improved quality. The model can be used via torch.hub or HuggingFace, supporting both shallow and deep cloning for inference. Checkpoints are provided for AR and NAR models, with hardware requirements of 750M+450M params on GPU. Contributions to improve model stability, performance, and reference audio selection are welcome.
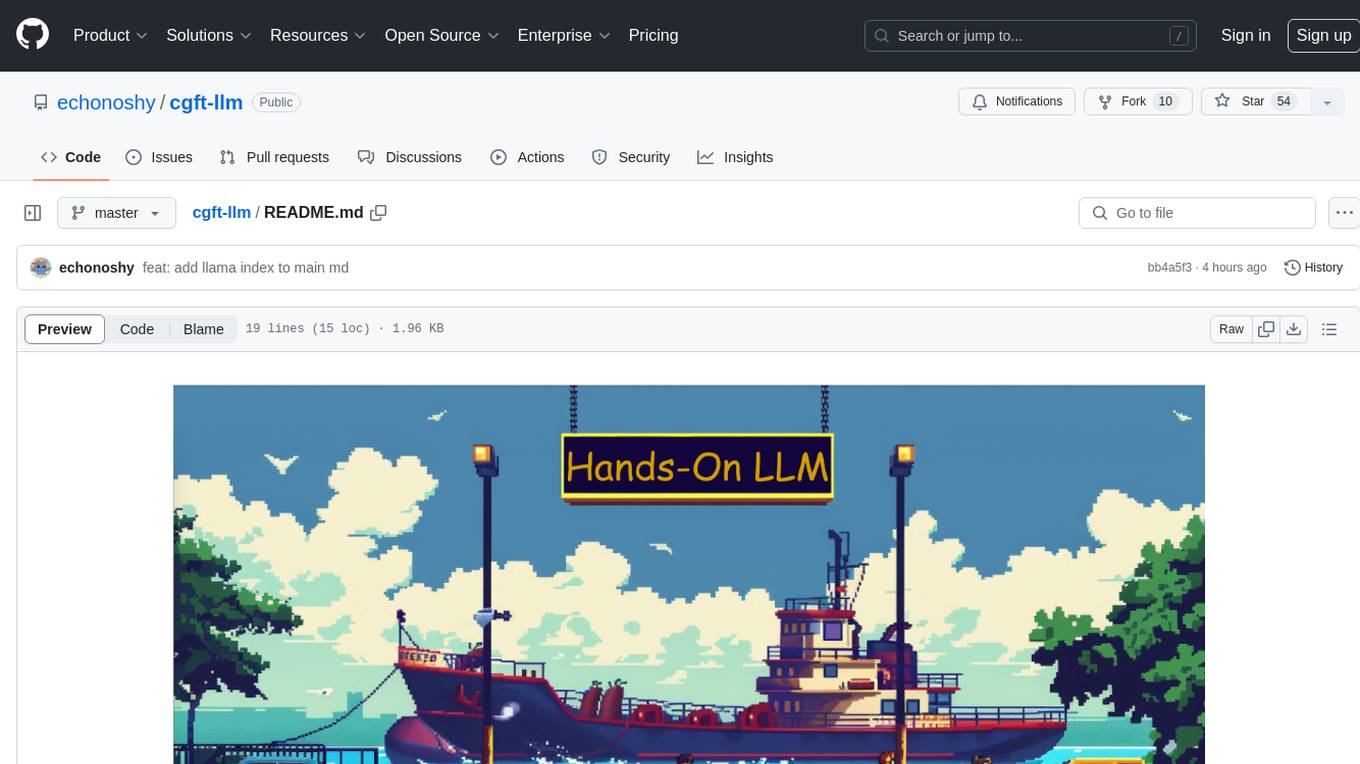
cgft-llm
The cgft-llm repository is a collection of video tutorials and documentation for implementing large models. It provides guidance on topics such as fine-tuning llama3 with llama-factory, lightweight deployment and quantization using llama.cpp, speech generation with ChatTTS, introduction to Ollama for large model deployment, deployment tools for vllm and paged attention, and implementing RAG with llama-index. Users can find detailed code documentation and video tutorials for each project in the repository.
For similar jobs
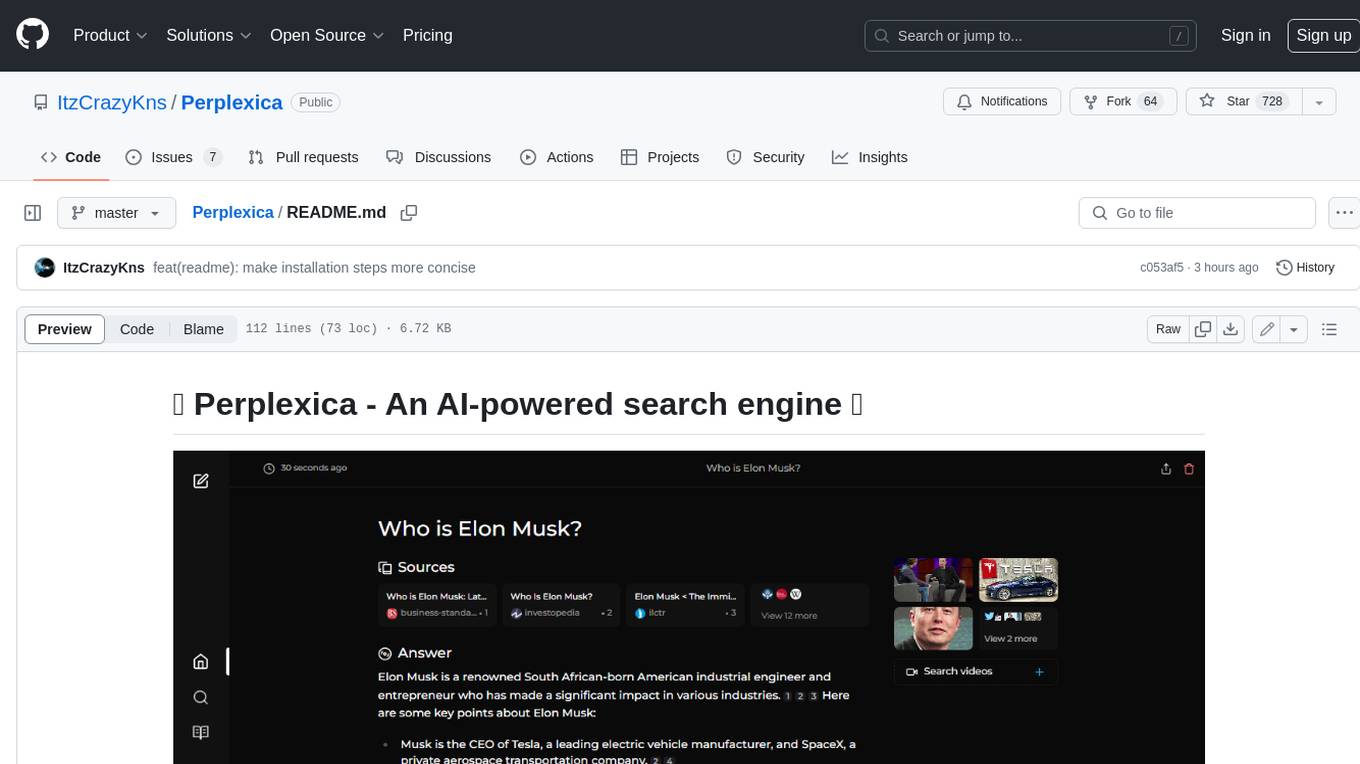
Perplexica
Perplexica is an open-source AI-powered search engine that utilizes advanced machine learning algorithms to provide clear answers with sources cited. It offers various modes like Copilot Mode, Normal Mode, and Focus Modes for specific types of questions. Perplexica ensures up-to-date information by using SearxNG metasearch engine. It also features image and video search capabilities and upcoming features include finalizing Copilot Mode and adding Discover and History Saving features.
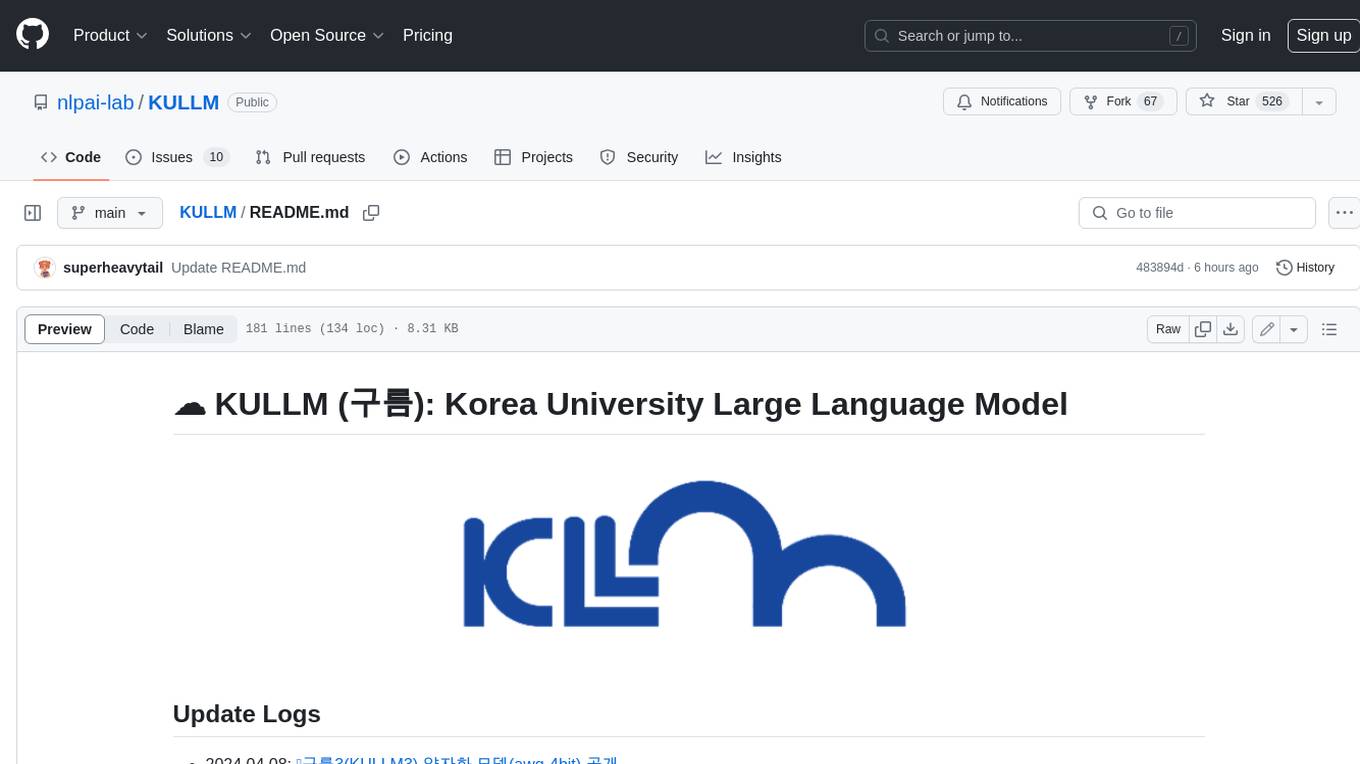
KULLM
KULLM (구름) is a Korean Large Language Model developed by Korea University NLP & AI Lab and HIAI Research Institute. It is based on the upstage/SOLAR-10.7B-v1.0 model and has been fine-tuned for instruction. The model has been trained on 8×A100 GPUs and is capable of generating responses in Korean language. KULLM exhibits hallucination and repetition phenomena due to its decoding strategy. Users should be cautious as the model may produce inaccurate or harmful results. Performance may vary in benchmarks without a fixed system prompt.
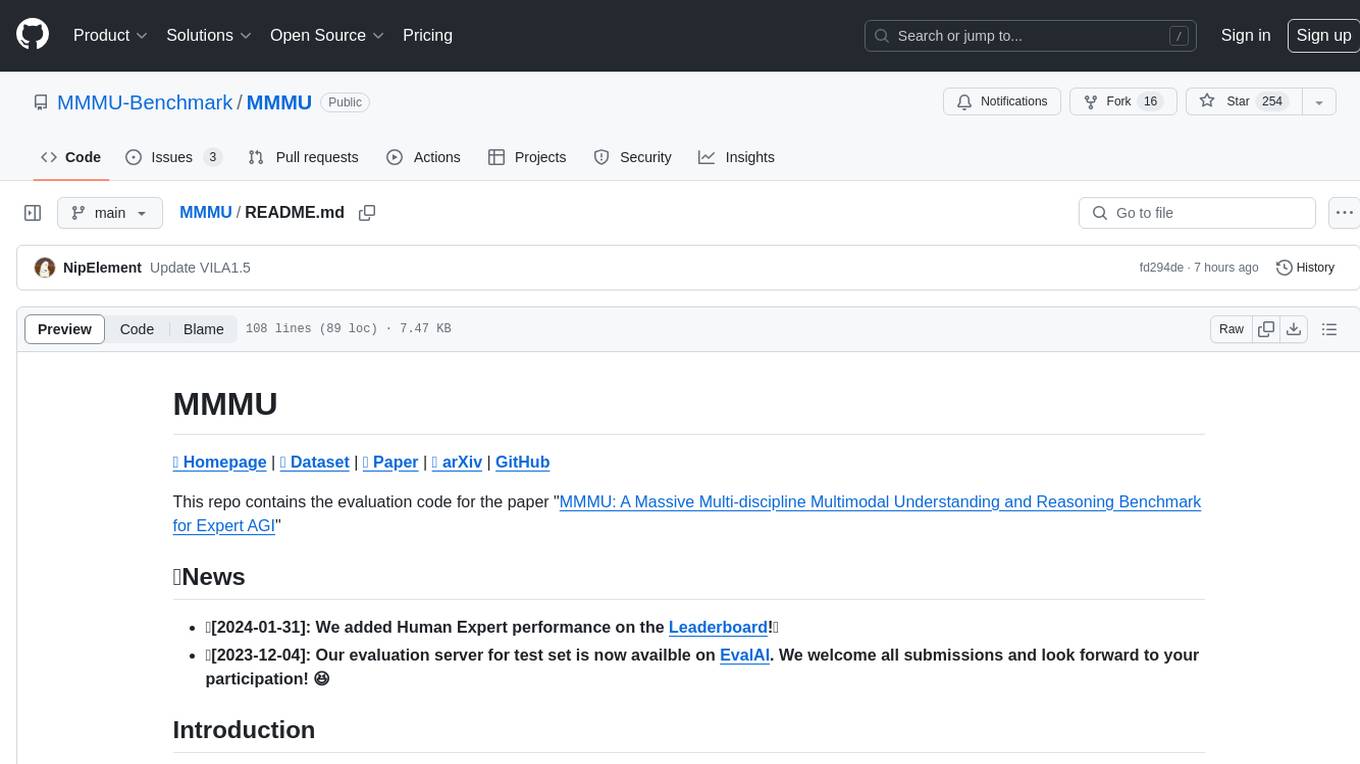
MMMU
MMMU is a benchmark designed to evaluate multimodal models on college-level subject knowledge tasks, covering 30 subjects and 183 subfields with 11.5K questions. It focuses on advanced perception and reasoning with domain-specific knowledge, challenging models to perform tasks akin to those faced by experts. The evaluation of various models highlights substantial challenges, with room for improvement to stimulate the community towards expert artificial general intelligence (AGI).
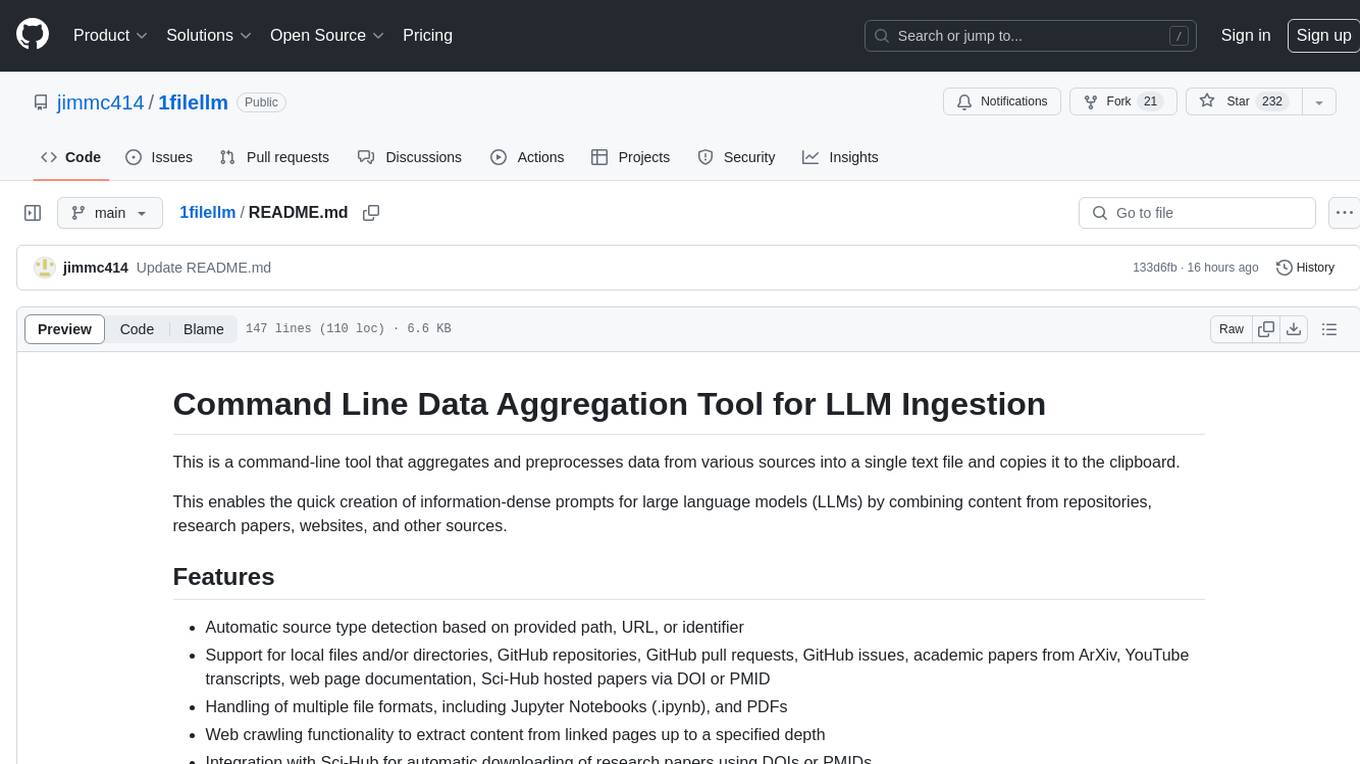
1filellm
1filellm is a command-line data aggregation tool designed for LLM ingestion. It aggregates and preprocesses data from various sources into a single text file, facilitating the creation of information-dense prompts for large language models. The tool supports automatic source type detection, handling of multiple file formats, web crawling functionality, integration with Sci-Hub for research paper downloads, text preprocessing, and token count reporting. Users can input local files, directories, GitHub repositories, pull requests, issues, ArXiv papers, YouTube transcripts, web pages, Sci-Hub papers via DOI or PMID. The tool provides uncompressed and compressed text outputs, with the uncompressed text automatically copied to the clipboard for easy pasting into LLMs.
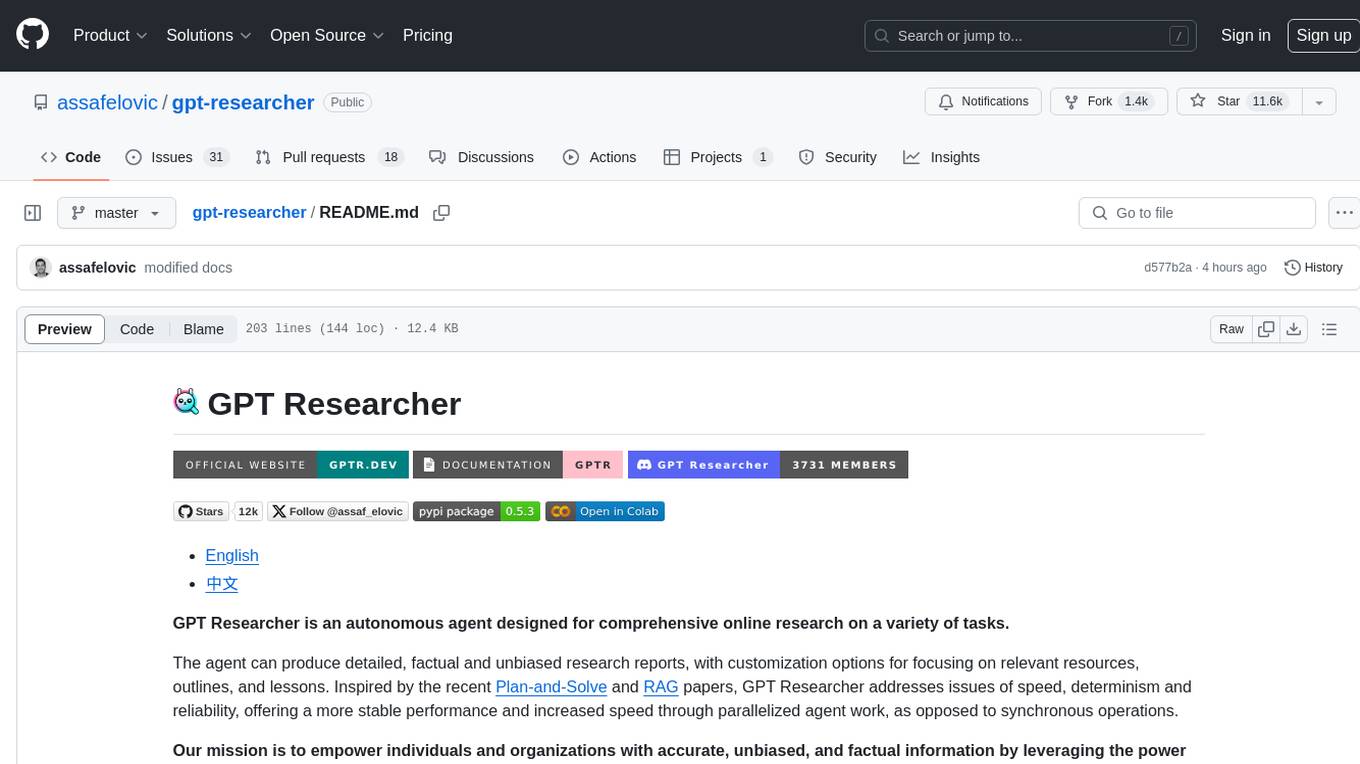
gpt-researcher
GPT Researcher is an autonomous agent designed for comprehensive online research on a variety of tasks. It can produce detailed, factual, and unbiased research reports with customization options. The tool addresses issues of speed, determinism, and reliability by leveraging parallelized agent work. The main idea involves running 'planner' and 'execution' agents to generate research questions, seek related information, and create research reports. GPT Researcher optimizes costs and completes tasks in around 3 minutes. Features include generating long research reports, aggregating web sources, an easy-to-use web interface, scraping web sources, and exporting reports to various formats.
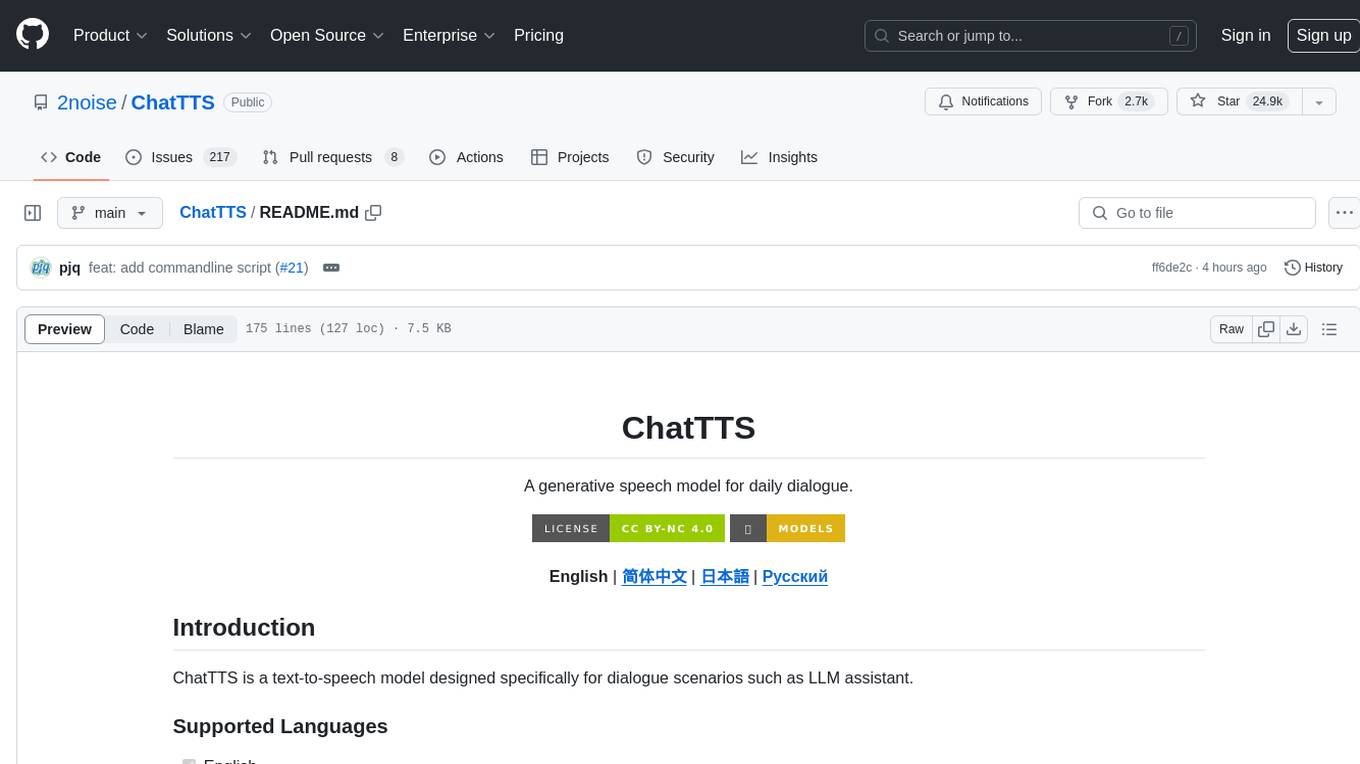
ChatTTS
ChatTTS is a generative speech model optimized for dialogue scenarios, providing natural and expressive speech synthesis with fine-grained control over prosodic features. It supports multiple speakers and surpasses most open-source TTS models in terms of prosody. The model is trained with 100,000+ hours of Chinese and English audio data, and the open-source version on HuggingFace is a 40,000-hour pre-trained model without SFT. The roadmap includes open-sourcing additional features like VQ encoder, multi-emotion control, and streaming audio generation. The tool is intended for academic and research use only, with precautions taken to limit potential misuse.
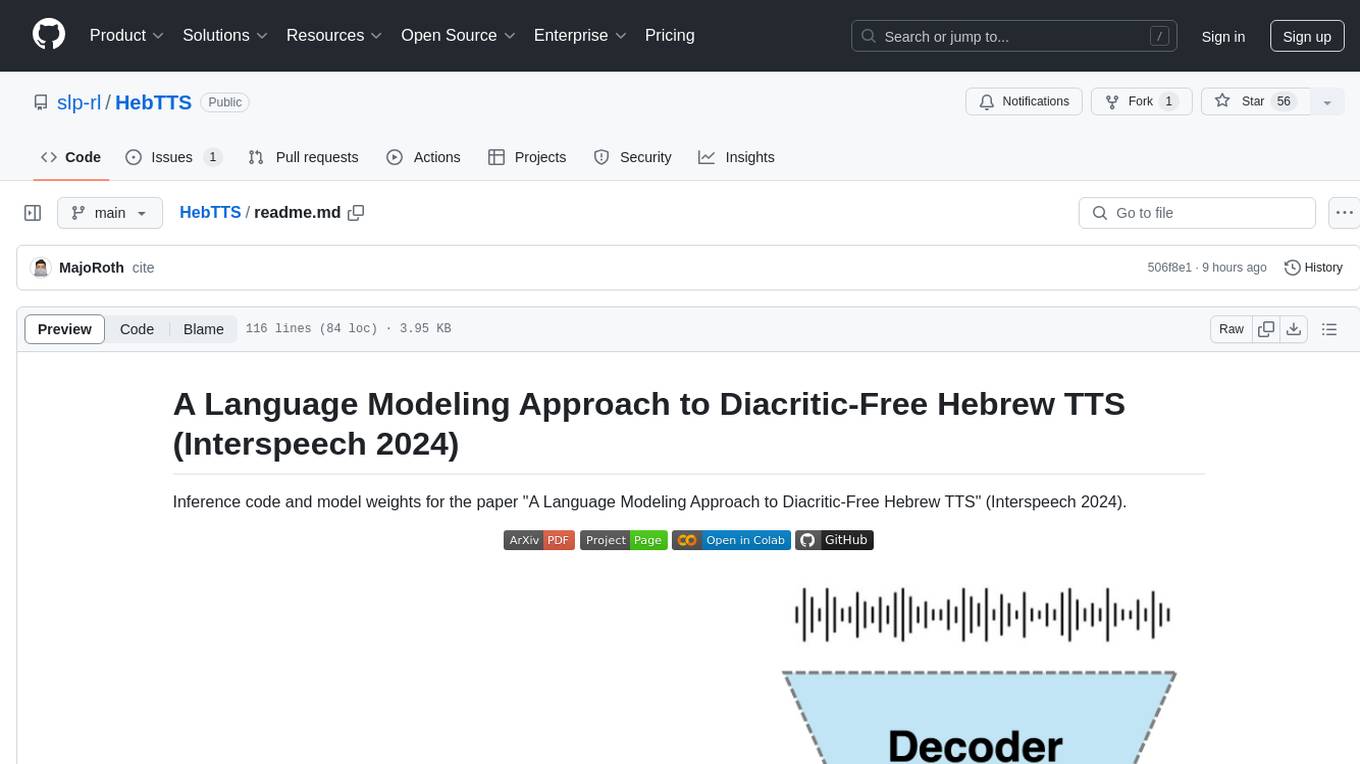
HebTTS
HebTTS is a language modeling approach to diacritic-free Hebrew text-to-speech (TTS) system. It addresses the challenge of accurately mapping text to speech in Hebrew by proposing a language model that operates on discrete speech representations and is conditioned on a word-piece tokenizer. The system is optimized using weakly supervised recordings and outperforms diacritic-based Hebrew TTS systems in terms of content preservation and naturalness of generated speech.
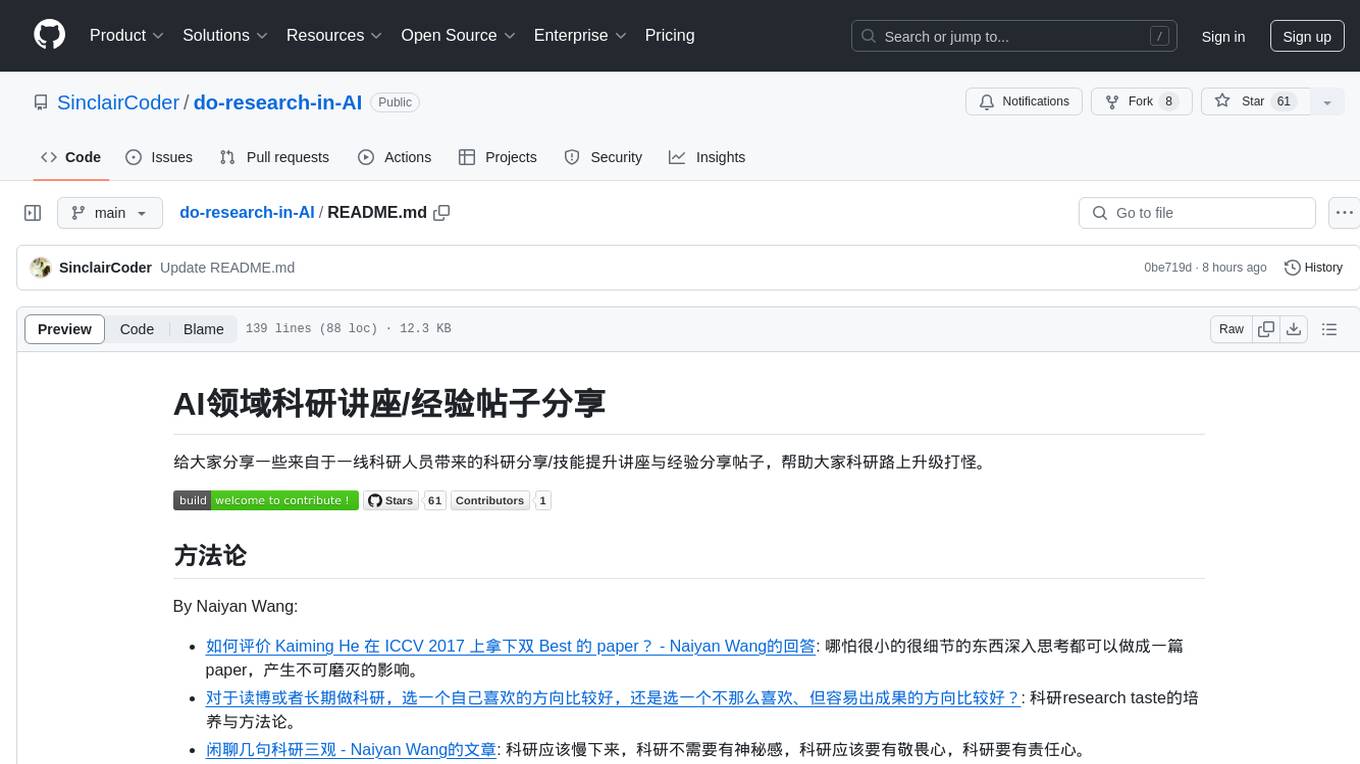
do-research-in-AI
This repository is a collection of research lectures and experience sharing posts from frontline researchers in the field of AI. It aims to help individuals upgrade their research skills and knowledge through insightful talks and experiences shared by experts. The content covers various topics such as evaluating research papers, choosing research directions, research methodologies, and tips for writing high-quality scientific papers. The repository also includes discussions on academic career paths, research ethics, and the emotional aspects of research work. Overall, it serves as a valuable resource for individuals interested in advancing their research capabilities in the field of AI.