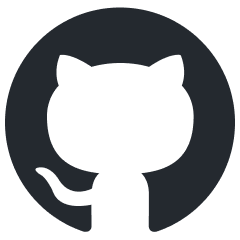
cgft-llm
Practice to LLM.
Stars: 739
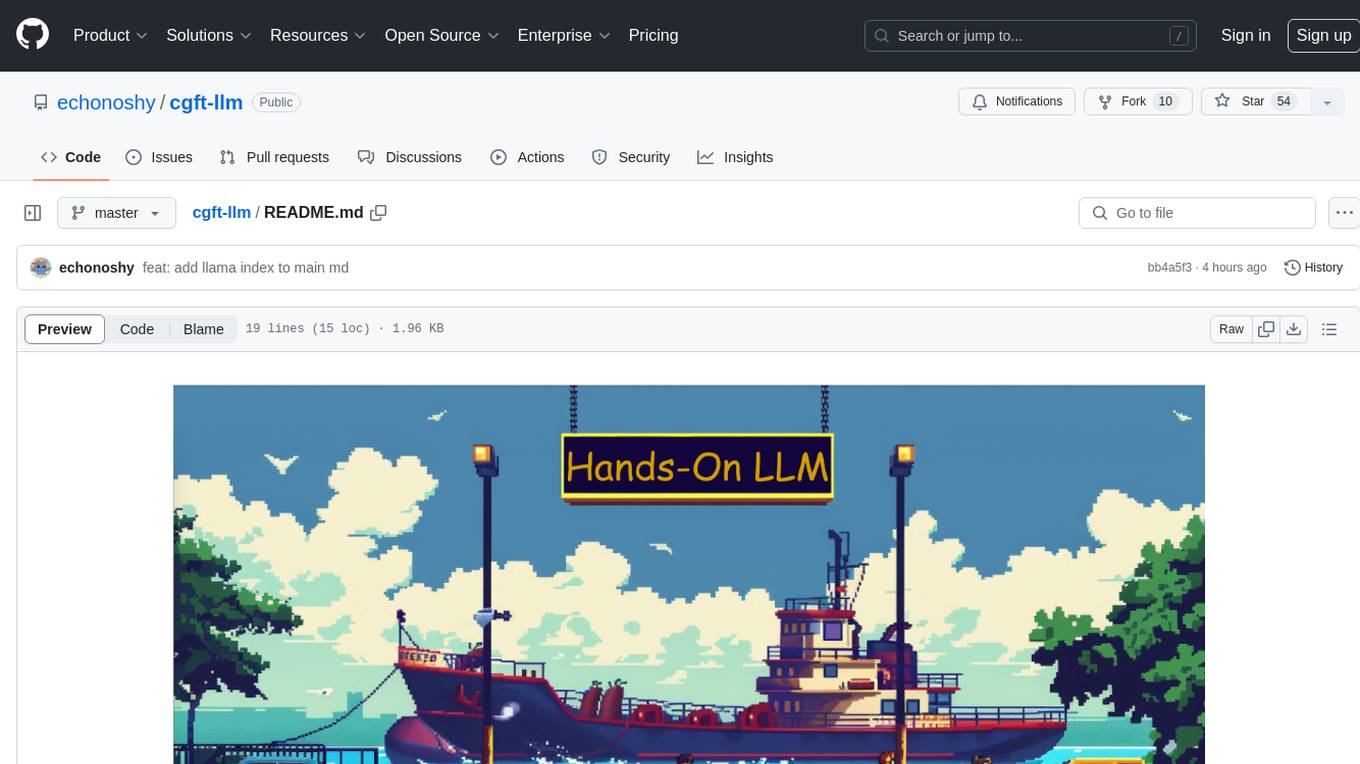
The cgft-llm repository is a collection of video tutorials and documentation for implementing large models. It provides guidance on topics such as fine-tuning llama3 with llama-factory, lightweight deployment and quantization using llama.cpp, speech generation with ChatTTS, introduction to Ollama for large model deployment, deployment tools for vllm and paged attention, and implementing RAG with llama-index. Users can find detailed code documentation and video tutorials for each project in the repository.
README:
如果你在实操的过程中遇到问题,请在对应的视频下方留言。 复现遇到bug,请描述:
- 🎯 运行环境
- 🧩 对应的代码、日志和报错截图
有感兴趣的内容或问题,点击这里加入讨论🎉。
如果你不太了解如何提出一个好问题,请花几分钟阅读一下这个,相信我,可能并不能改变什么🤫(不是)。
How-To-Ask-Questions-The-Smart-Way
序号 | 项目 | 代码文档 | 视频时长 | 视频教程 |
---|---|---|---|---|
1 | 使用llama-factory微调llama3 | llama-factory |
|
|
2 | 训练数据整理以及微调优化建议 | training-dataset |
|
|
3 | llama.cpp进行轻量化部署和量化 | llama-cpp |
|
|
4 | Ollama大模型部署工具介绍 | ollama |
|
|
5 | vllm部署工具及paged attention | vllm |
|
|
6 | llama-index实现RAG | llama-index |
|
|
7 | graph-rag本地部署 | graph-rag |
|
|
8 | mkdocs+readthedocs部署项目文档 | mkdocs |
|
|
9 | function-calling 自动发邮件 | function-calling |
|
|
10 | 大模型学习路径及面试 | llm-roadmap |
|
|
11 | 大模型算法岗非技术答疑 | llm-no-tec-qa |
|
|
12 | AI标注流程及label studio框架 | label-studio |
|
|
13 | AI算法项目开发流程及实例 | AI-project-workflow |
|
|
14 | Dify实现Agent和LLM Workflow | Agent llm-workflow |
|
专题系列会以多个视频介绍同一个主题,分P的形式展示,即共用一个video url。
序号 | 项目 | 代码文档 | 视频时长 | 视频教程 |
---|---|---|---|---|
1 | kaggle 大模型竞赛系列 (🏊更新至01期) | kaggle |
|
|
2 | gradio 使用python构建并分享AI应用(🏊已完结) | gradio |
|
|
3 | docker 容器化部署 (🏊已完结) | docker |
|
序号 | 项目 | 代码文档 | 视频时长 | 视频教程 |
---|---|---|---|---|
1 | B站实现动态转发抽奖 | Bilibili-lottery |
|
1. LLM 主线知识
- [ ] llama.cpp更新版本
- [x] AI agent
- [x] label studio: 自建打标平台
2. AI 专题
- [x] gradio
- [ ] kaggle
- [x] docker 容器化部署
3. AI工程
-
语音类
- [ ] 流式变声器
- [ ] 端到端音频大模型
- [x] 声音克隆
-
文本类
- [ ] AI播客
-
图像类
For Tasks:
Click tags to check more tools for each tasksFor Jobs:
Alternative AI tools for cgft-llm
Similar Open Source Tools
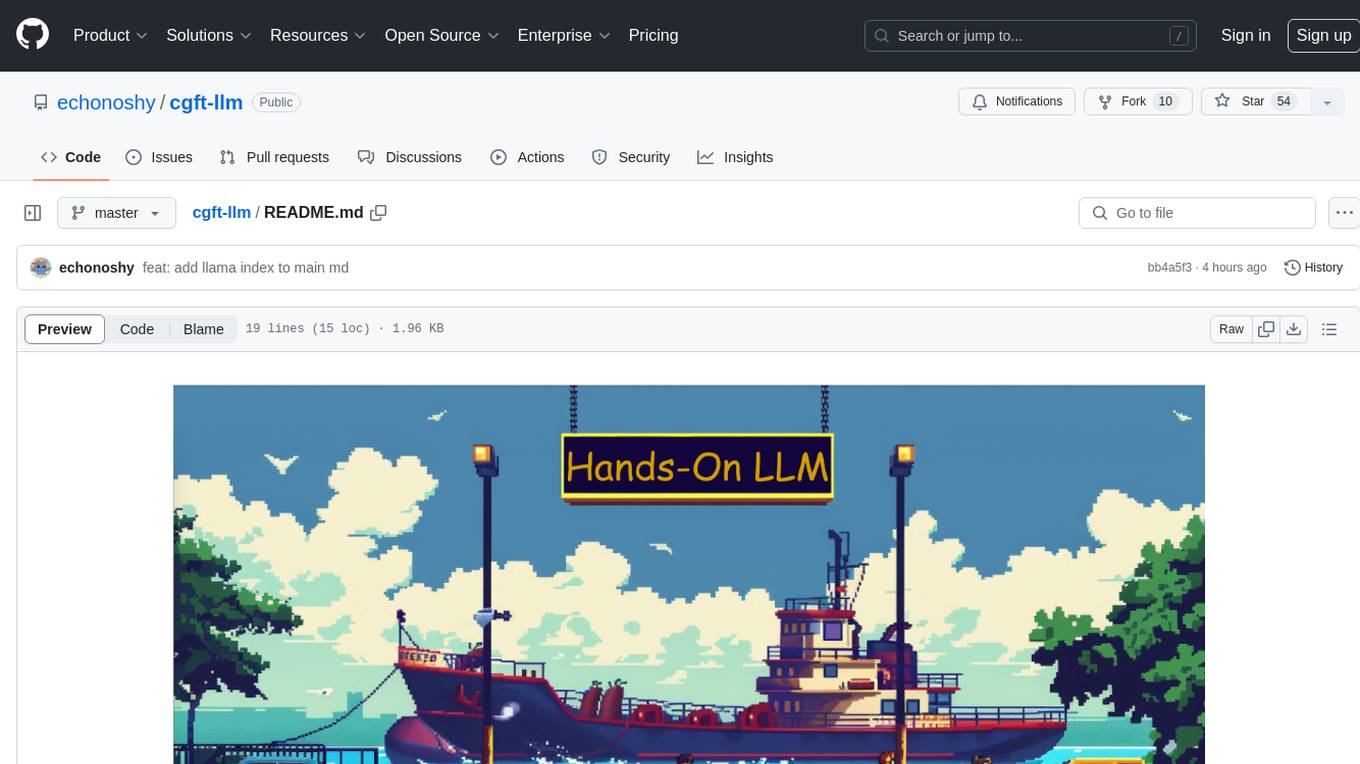
cgft-llm
The cgft-llm repository is a collection of video tutorials and documentation for implementing large models. It provides guidance on topics such as fine-tuning llama3 with llama-factory, lightweight deployment and quantization using llama.cpp, speech generation with ChatTTS, introduction to Ollama for large model deployment, deployment tools for vllm and paged attention, and implementing RAG with llama-index. Users can find detailed code documentation and video tutorials for each project in the repository.
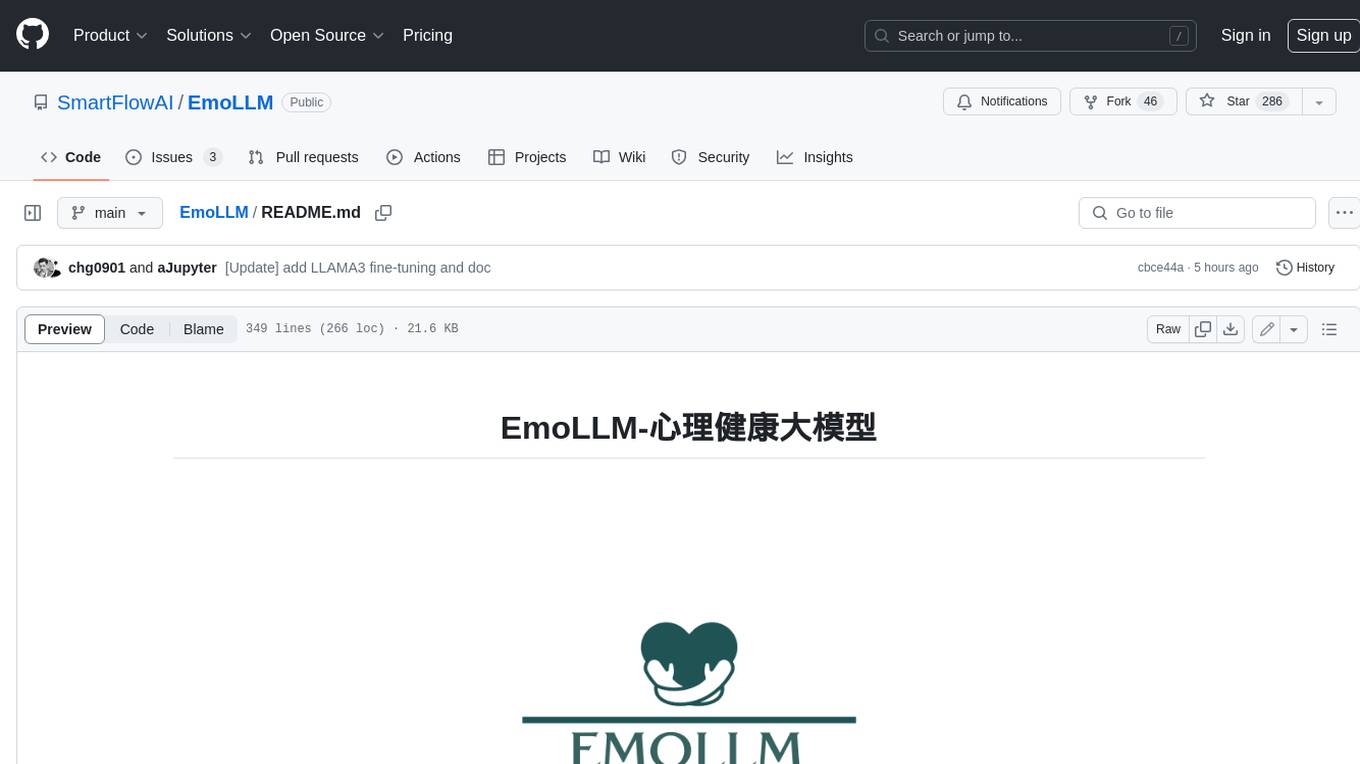
EmoLLM
EmoLLM is a series of large-scale psychological health counseling models that can support **understanding-supporting-helping users** in the psychological health counseling chain, which is fine-tuned from `LLM` instructions. Welcome everyone to star~⭐⭐. The currently open source `LLM` fine-tuning configurations are as follows:
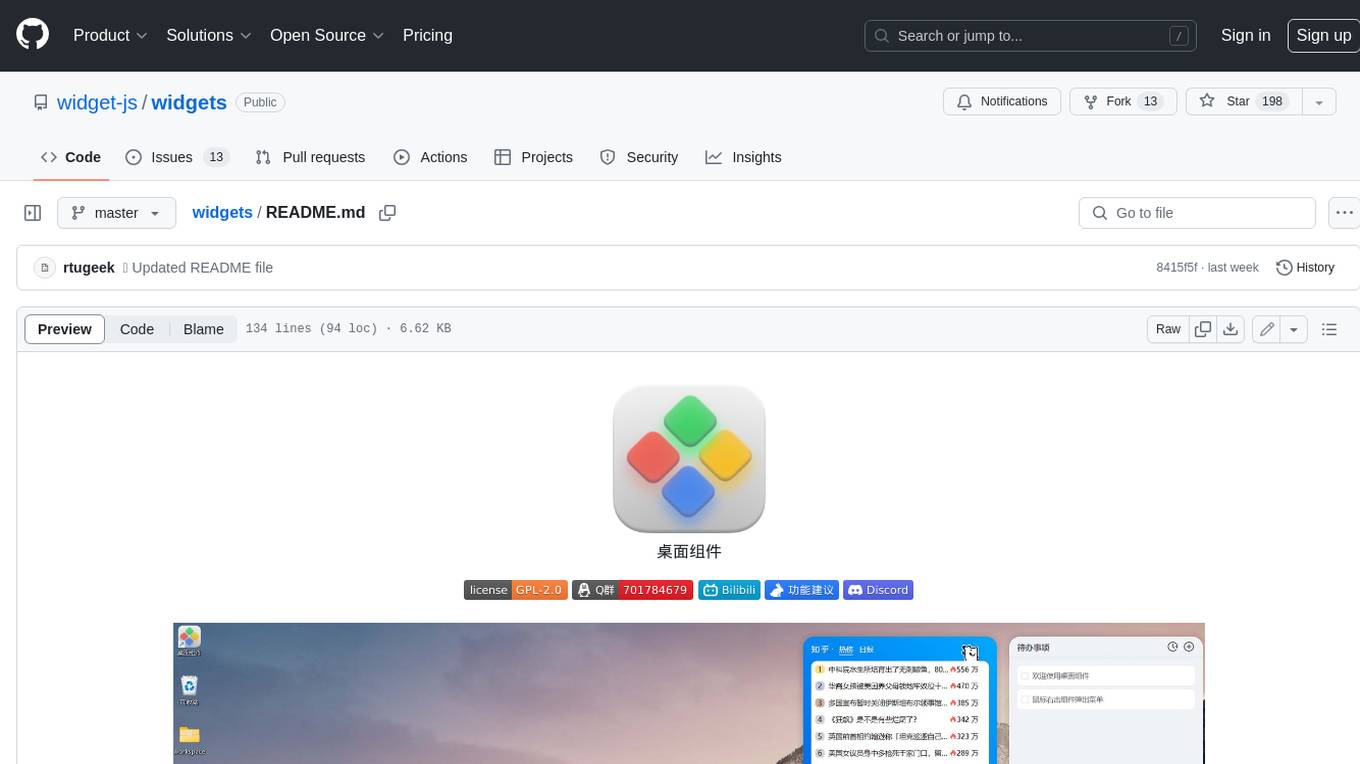
widgets
Widgets is a desktop component front-end open source component. The project is still being continuously improved. The desktop component client can be downloaded and run in two ways: 1. https://www.microsoft.com/store/productId/9NPR50GQ7T53 2. https://widgetjs.cn After cloning the code, you need to download the dependency in the project directory: `shell pnpm install` and run: `shell pnpm serve`
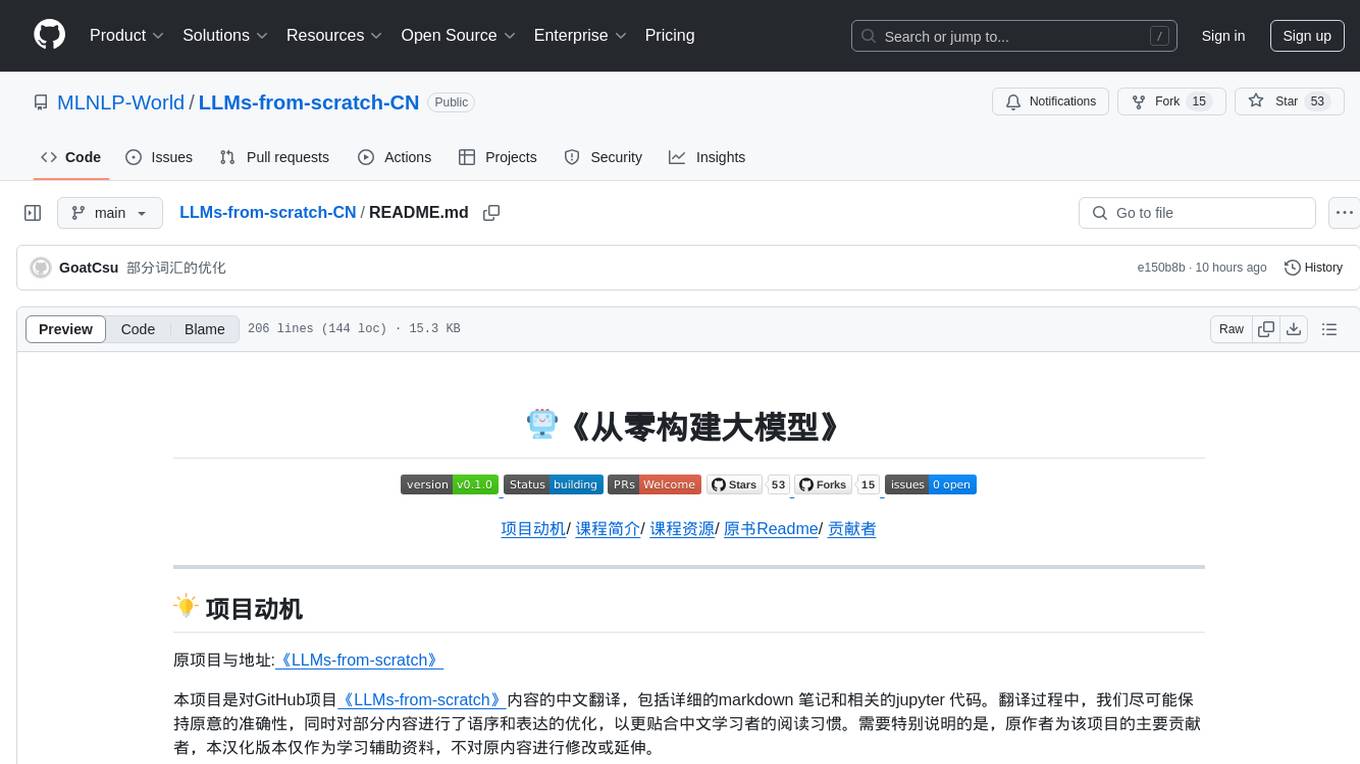
LLMs-from-scratch-CN
This repository is a Chinese translation of the GitHub project 'LLMs-from-scratch', including detailed markdown notes and related Jupyter code. The translation process aims to maintain the accuracy of the original content while optimizing the language and expression to better suit Chinese learners' reading habits. The repository features detailed Chinese annotations for all Jupyter code, aiding users in practical implementation. It also provides various supplementary materials to expand knowledge. The project focuses on building Large Language Models (LLMs) from scratch, covering fundamental constructions like Transformer architecture, sequence modeling, and delving into deep learning models such as GPT and BERT. Each part of the project includes detailed code implementations and learning resources to help users construct LLMs from scratch and master their core technologies.
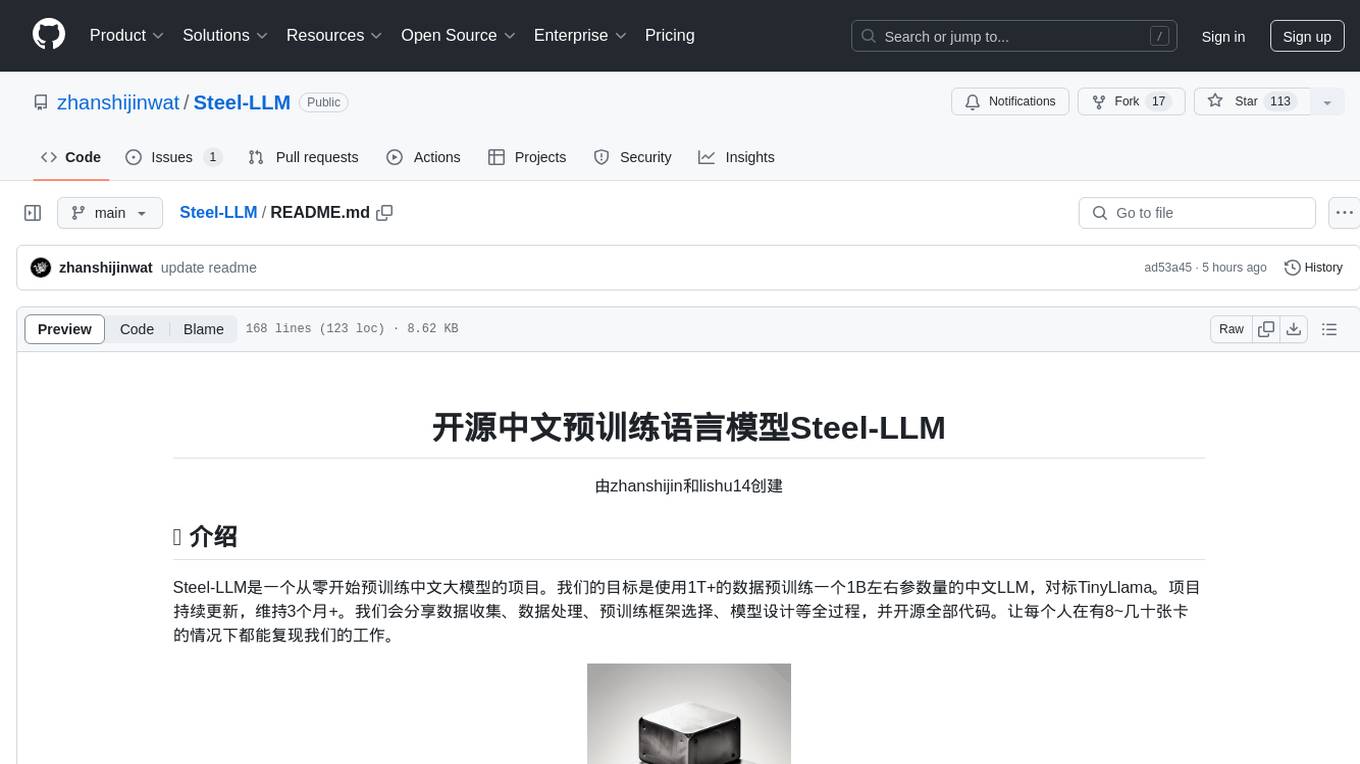
Steel-LLM
Steel-LLM is a project to pre-train a large Chinese language model from scratch using over 1T of data to achieve a parameter size of around 1B, similar to TinyLlama. The project aims to share the entire process including data collection, data processing, pre-training framework selection, model design, and open-source all the code. The goal is to enable reproducibility of the work even with limited resources. The name 'Steel' is inspired by a band '万能青年旅店' and signifies the desire to create a strong model despite limited conditions. The project involves continuous data collection of various cultural elements, trivia, lyrics, niche literature, and personal secrets to train the LLM. The ultimate aim is to fill the model with diverse data and leave room for individual input, fostering collaboration among users.
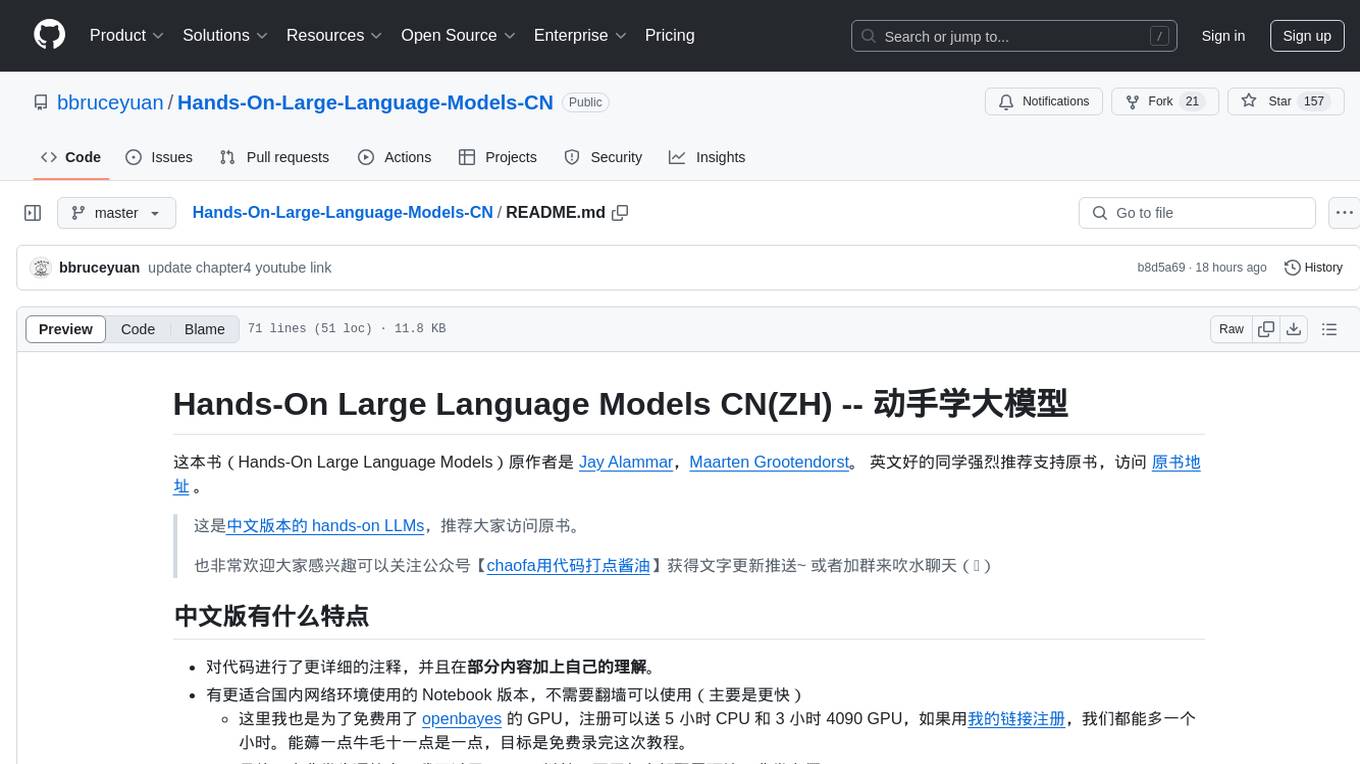
Hands-On-Large-Language-Models-CN
Hands-On Large Language Models CN(ZH) is a Chinese version of the book 'Hands-On Large Language Models' by Jay Alammar and Maarten Grootendorst. It provides detailed code annotations and additional insights, offers Notebook versions suitable for Chinese network environments, utilizes openbayes for free GPU access, allows convenient environment setup with vscode, and includes accompanying Chinese language videos on platforms like Bilibili and YouTube. The book covers various chapters on topics like Tokens and Embeddings, Transformer LLMs, Text Classification, Text Clustering, Prompt Engineering, Text Generation, Semantic Search, Multimodal LLMs, Text Embedding Models, Fine-tuning Models, and more.
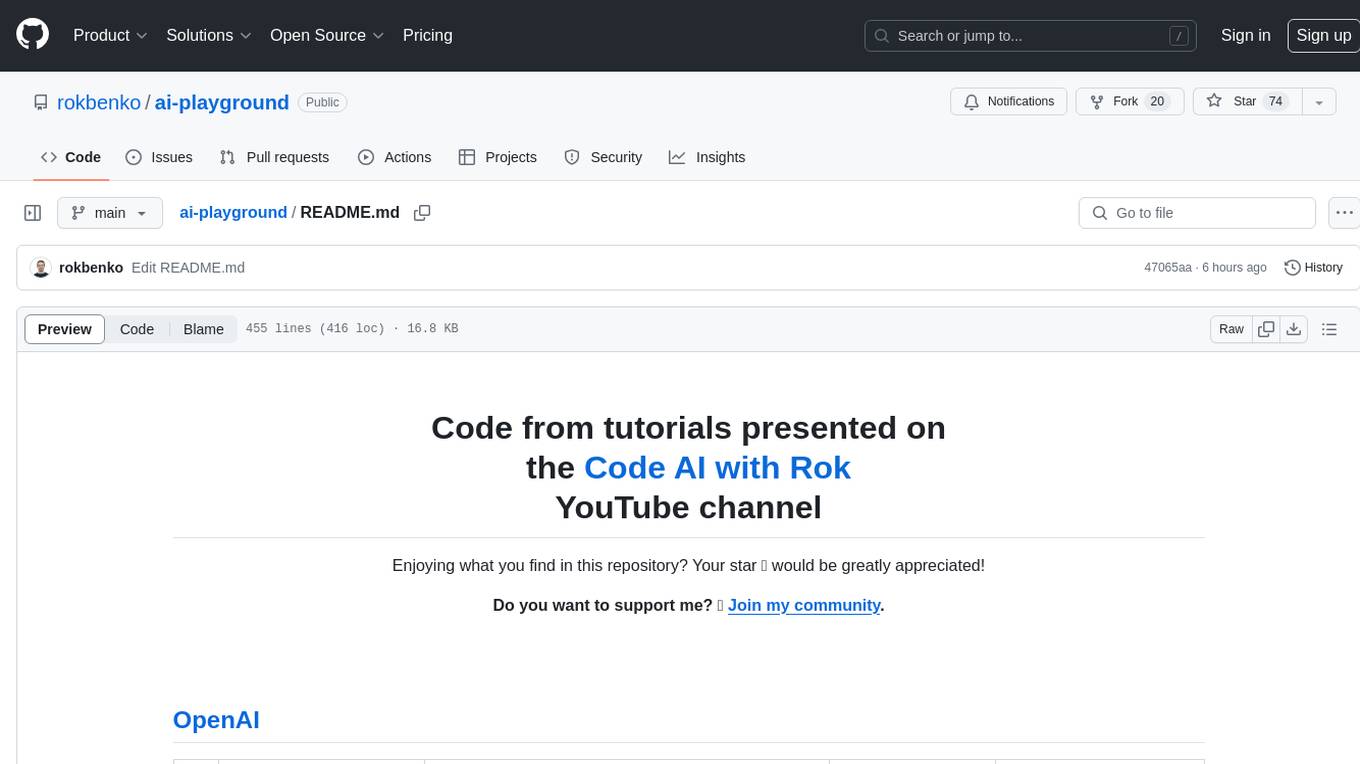
ai-playground
The ai-playground repository contains code from tutorials presented on the Code AI with Rok YouTube channel. It includes tutorials on using the OpenAI Assistants API v1 beta to build personal math tutors, customer support chatbots, and more. Additionally, there are tutorials on using Gemini Pro API, Snowflake Cortex LLM functions, LlamaIndex chat streaming app, Fetch.ai uAgents, Milvus Standalone, spaCy for NER, and more. The repository aims to provide practical examples and guides for developers interested in AI-related projects and tools.
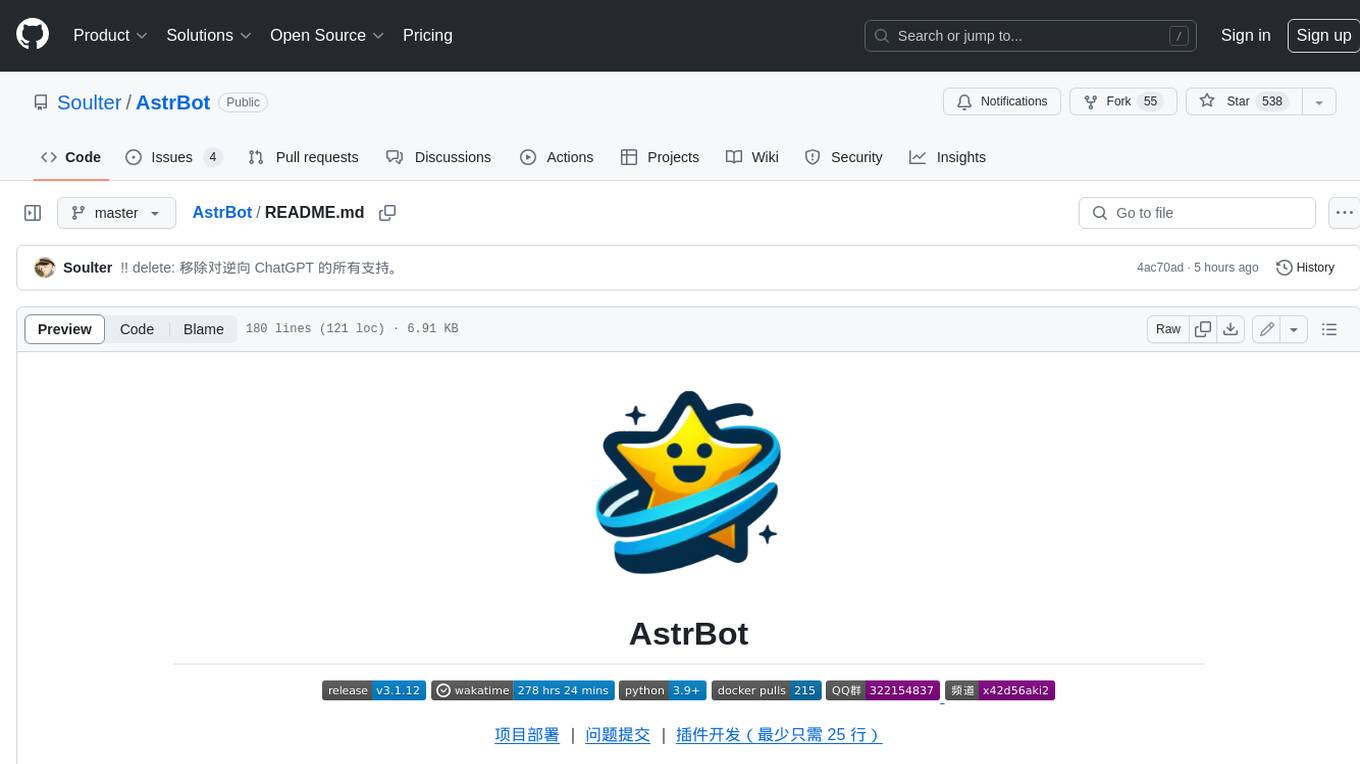
AstrBot
AstrBot is a powerful and versatile tool that leverages the capabilities of large language models (LLMs) like GPT-3, GPT-3.5, and GPT-4 to enhance communication and automate tasks. It seamlessly integrates with popular messaging platforms such as QQ, QQ Channel, and Telegram, enabling users to harness the power of AI within their daily conversations and workflows.
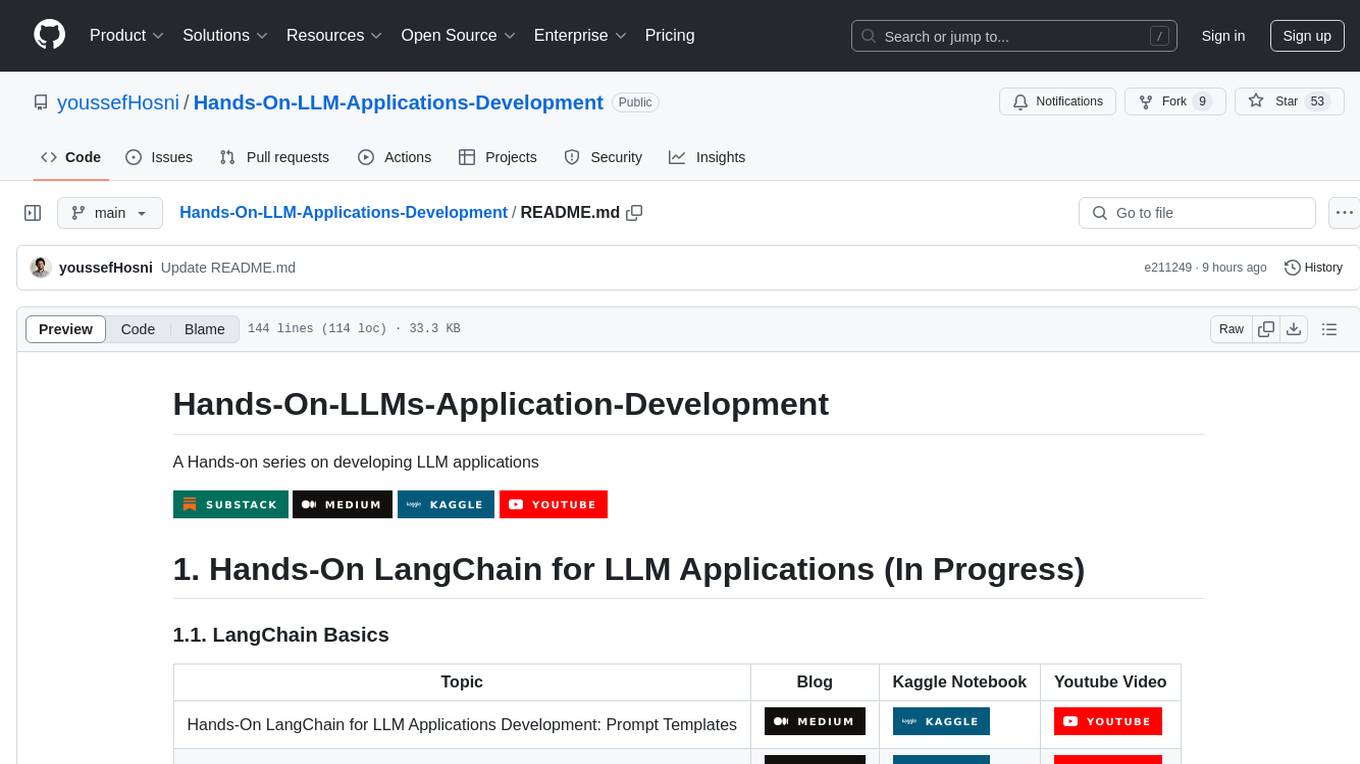
Hands-On-LLM-Applications-Development
Hands-On-LLM-Applications-Development is a repository focused on developing applications using Large Language Models (LLMs). The repository provides hands-on tutorials, guides, and resources for building various applications such as LangChain for LLM applications, Retrieval Augmented Generation (RAG) with LangChain, building LLM agents with LangGraph, and advanced LangChain with OpenAI. It covers topics like prompt engineering for LLMs, building applications using HuggingFace open-source models, LLM fine-tuning, and advanced RAG applications.
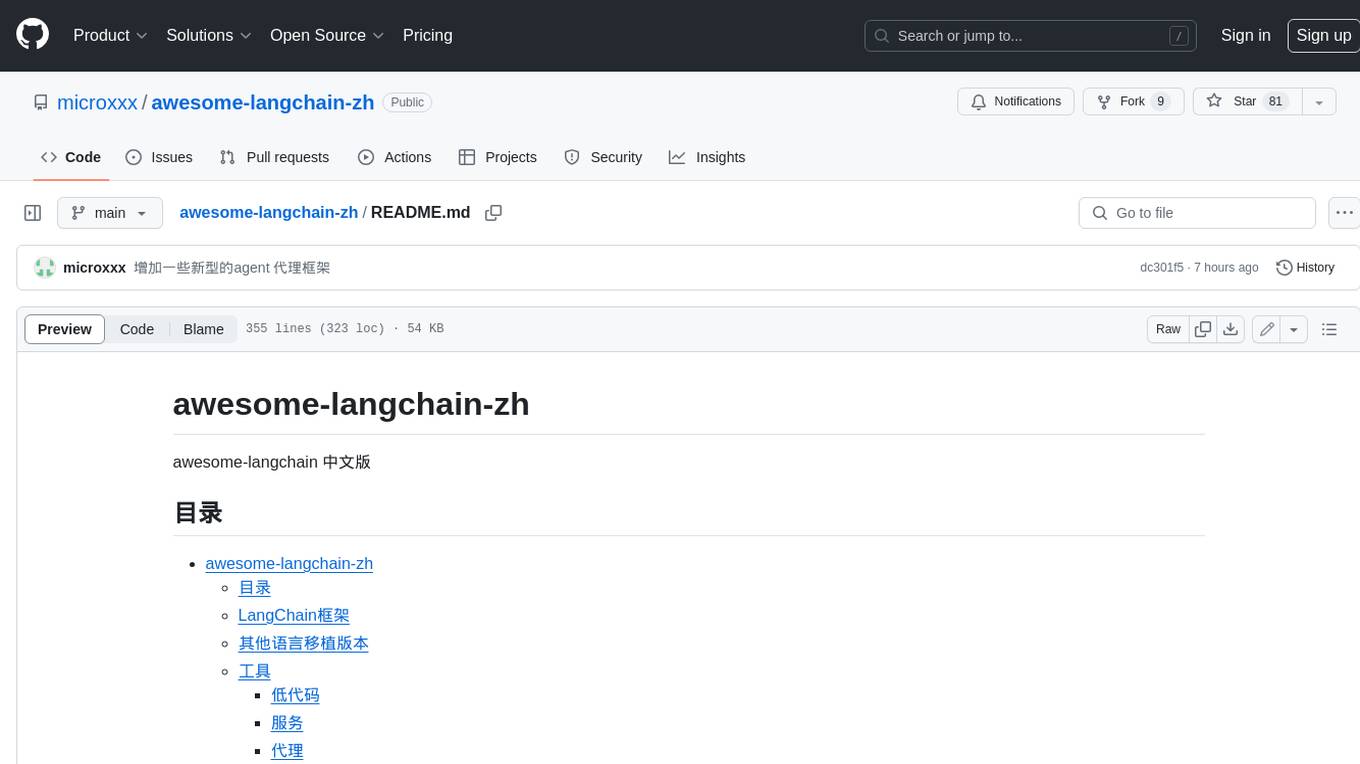
awesome-langchain-zh
The awesome-langchain-zh repository is a collection of resources related to LangChain, a framework for building AI applications using large language models (LLMs). The repository includes sections on the LangChain framework itself, other language ports of LangChain, tools for low-code development, services, agents, templates, platforms, open-source projects related to knowledge management and chatbots, as well as learning resources such as notebooks, videos, and articles. It also covers other LLM frameworks and provides additional resources for exploring and working with LLMs. The repository serves as a comprehensive guide for developers and AI enthusiasts interested in leveraging LangChain and LLMs for various applications.
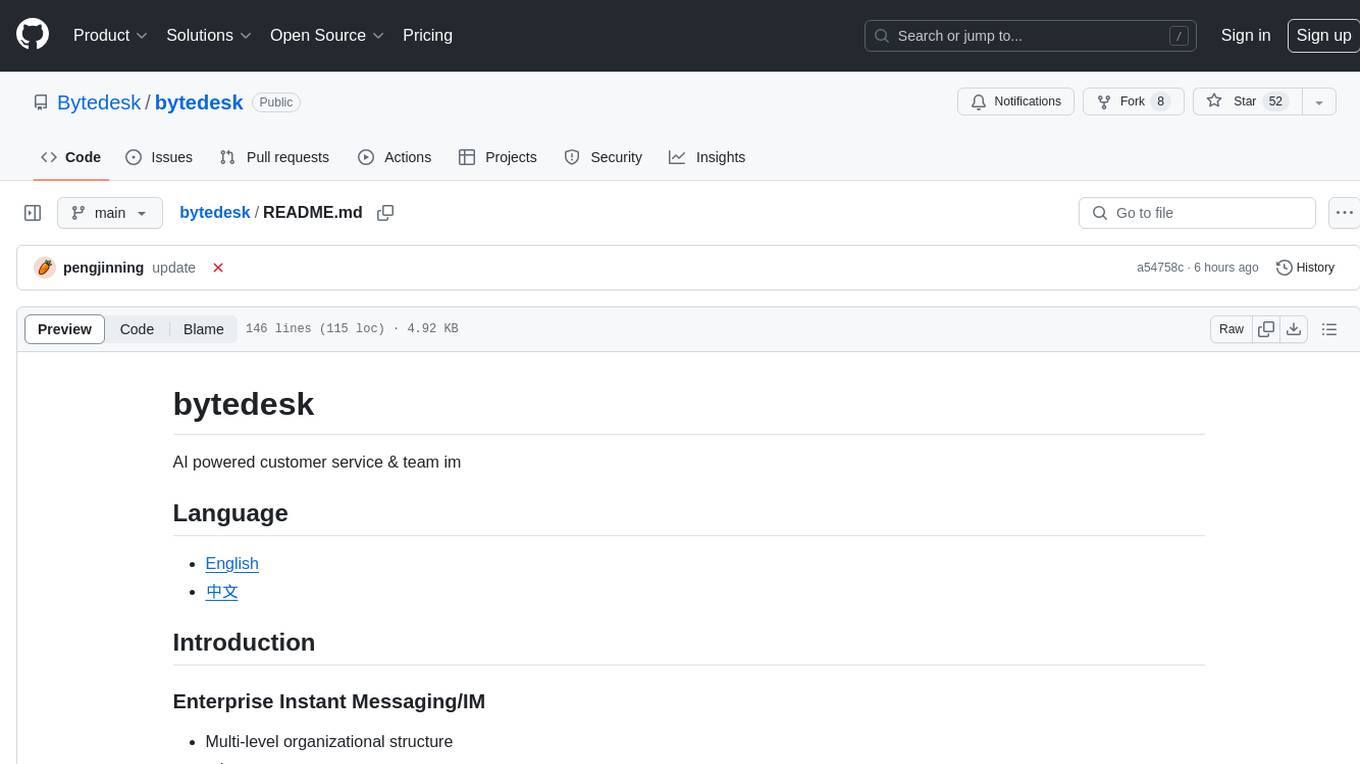
bytedesk
Bytedesk is an AI-powered customer service and team instant messaging tool that offers features like enterprise instant messaging, online customer service, large model AI assistant, and local area network file transfer. It supports multi-level organizational structure, role management, permission management, chat record management, seating workbench, work order system, seat management, data dashboard, manual knowledge base, skill group management, real-time monitoring, announcements, sensitive words, CRM, report function, and integrated customer service workbench services. The tool is designed for team use with easy configuration throughout the company, and it allows file transfer across platforms using WiFi/hotspots without the need for internet connection.
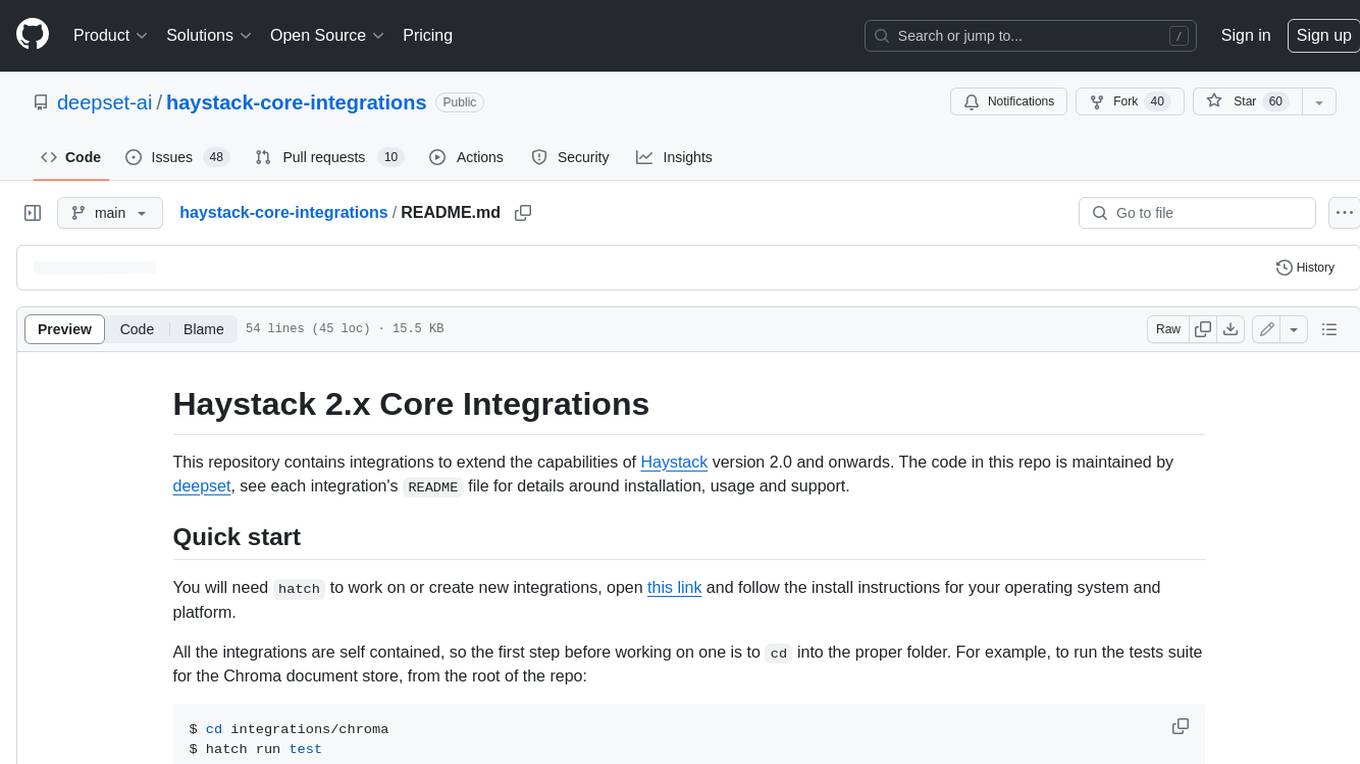
haystack-core-integrations
This repository contains integrations to extend the capabilities of Haystack version 2.0 and onwards. The code in this repo is maintained by deepset, see each integration's `README` file for details around installation, usage and support.
For similar tasks
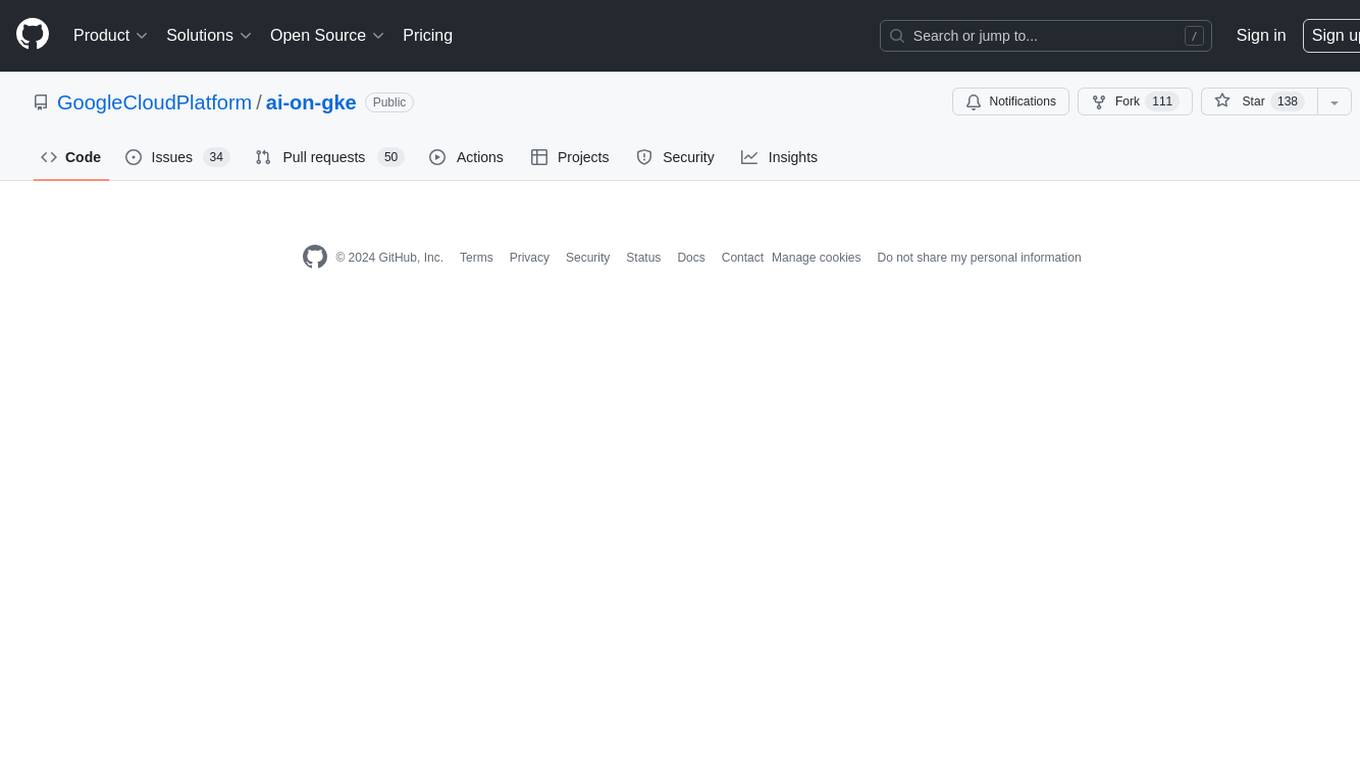
ai-on-gke
This repository contains assets related to AI/ML workloads on Google Kubernetes Engine (GKE). Run optimized AI/ML workloads with Google Kubernetes Engine (GKE) platform orchestration capabilities. A robust AI/ML platform considers the following layers: Infrastructure orchestration that support GPUs and TPUs for training and serving workloads at scale Flexible integration with distributed computing and data processing frameworks Support for multiple teams on the same infrastructure to maximize utilization of resources
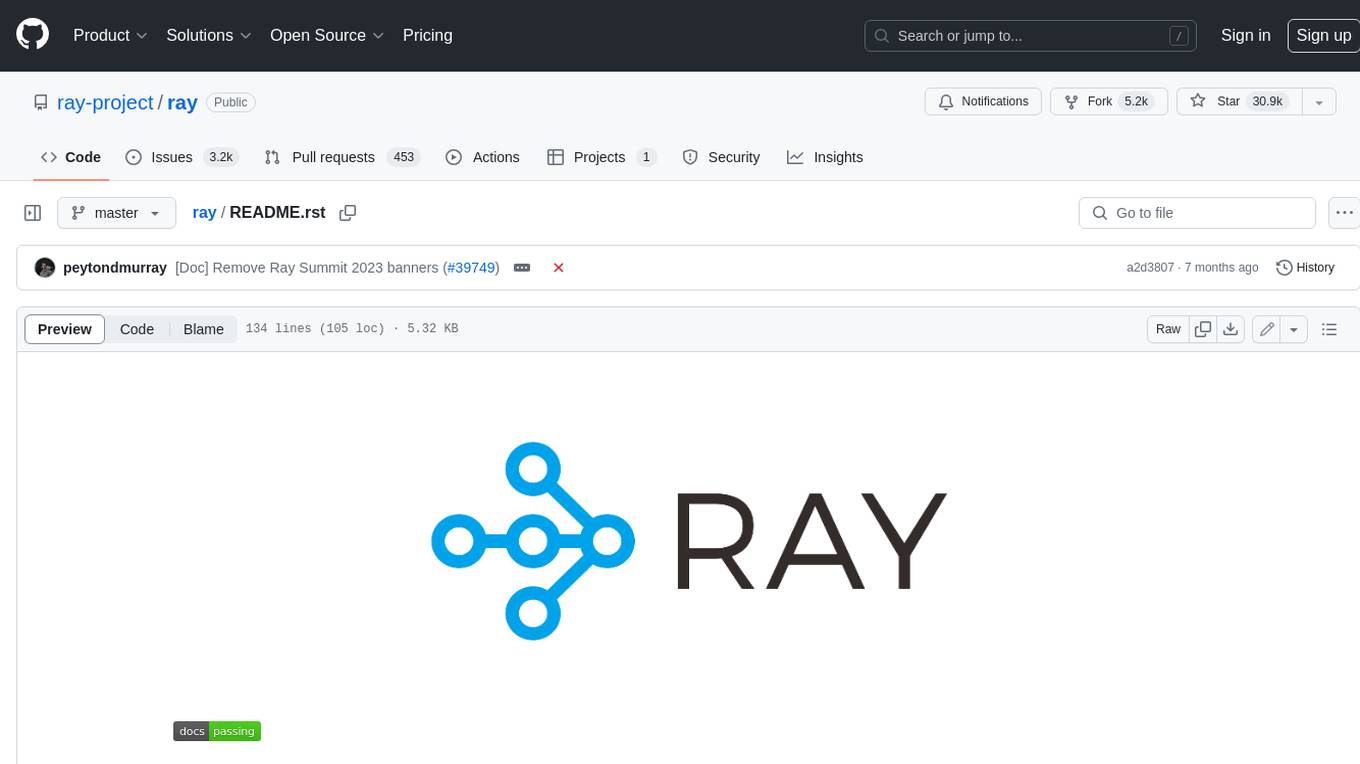
ray
Ray is a unified framework for scaling AI and Python applications. It consists of a core distributed runtime and a set of AI libraries for simplifying ML compute, including Data, Train, Tune, RLlib, and Serve. Ray runs on any machine, cluster, cloud provider, and Kubernetes, and features a growing ecosystem of community integrations. With Ray, you can seamlessly scale the same code from a laptop to a cluster, making it easy to meet the compute-intensive demands of modern ML workloads.
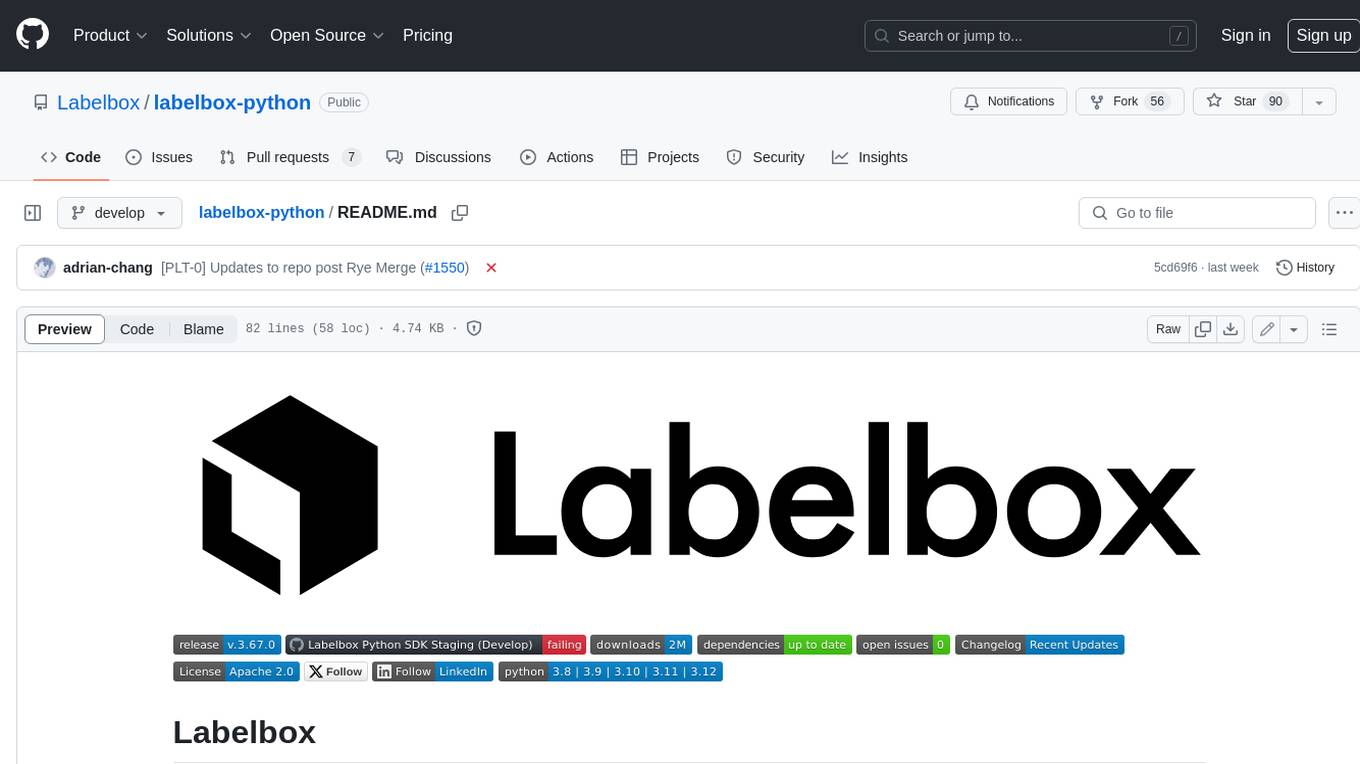
labelbox-python
Labelbox is a data-centric AI platform for enterprises to develop, optimize, and use AI to solve problems and power new products and services. Enterprises use Labelbox to curate data, generate high-quality human feedback data for computer vision and LLMs, evaluate model performance, and automate tasks by combining AI and human-centric workflows. The academic & research community uses Labelbox for cutting-edge AI research.
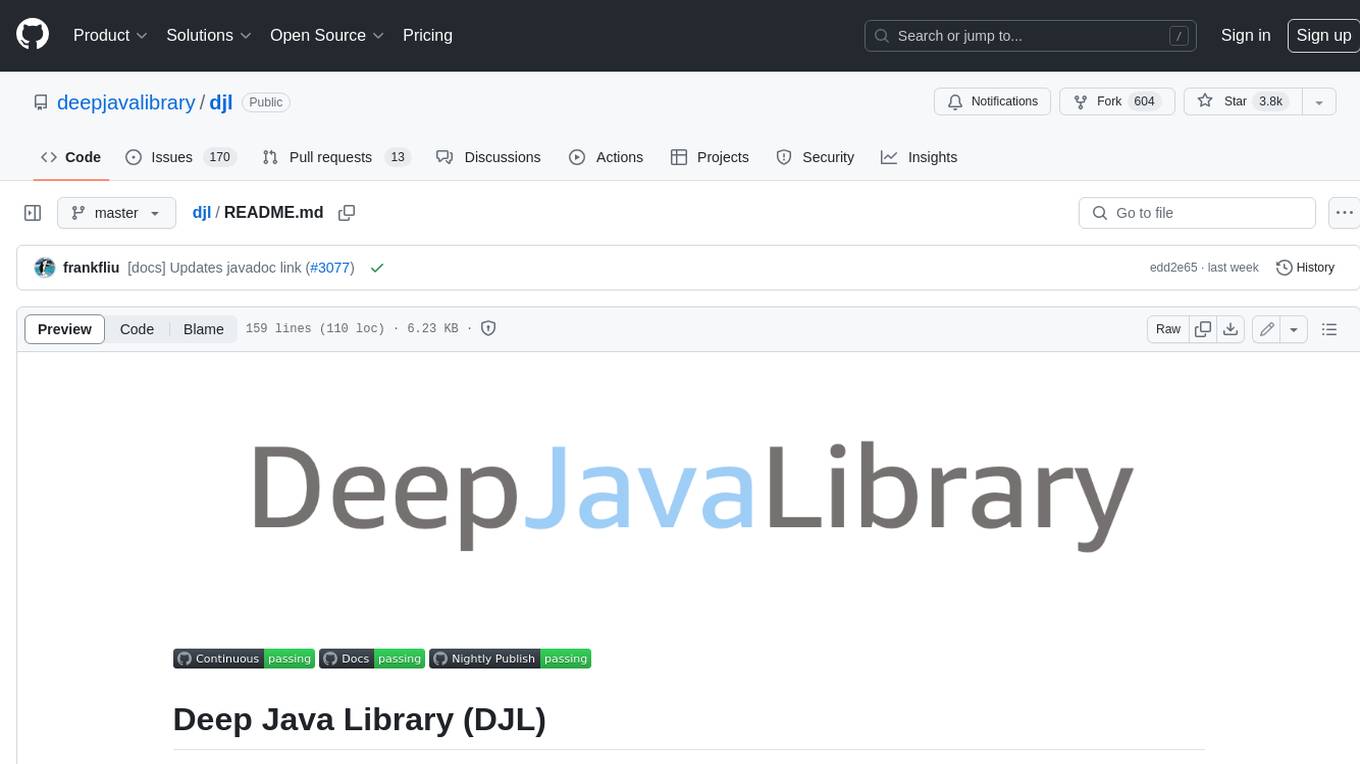
djl
Deep Java Library (DJL) is an open-source, high-level, engine-agnostic Java framework for deep learning. It is designed to be easy to get started with and simple to use for Java developers. DJL provides a native Java development experience and allows users to integrate machine learning and deep learning models with their Java applications. The framework is deep learning engine agnostic, enabling users to switch engines at any point for optimal performance. DJL's ergonomic API interface guides users with best practices to accomplish deep learning tasks, such as running inference and training neural networks.
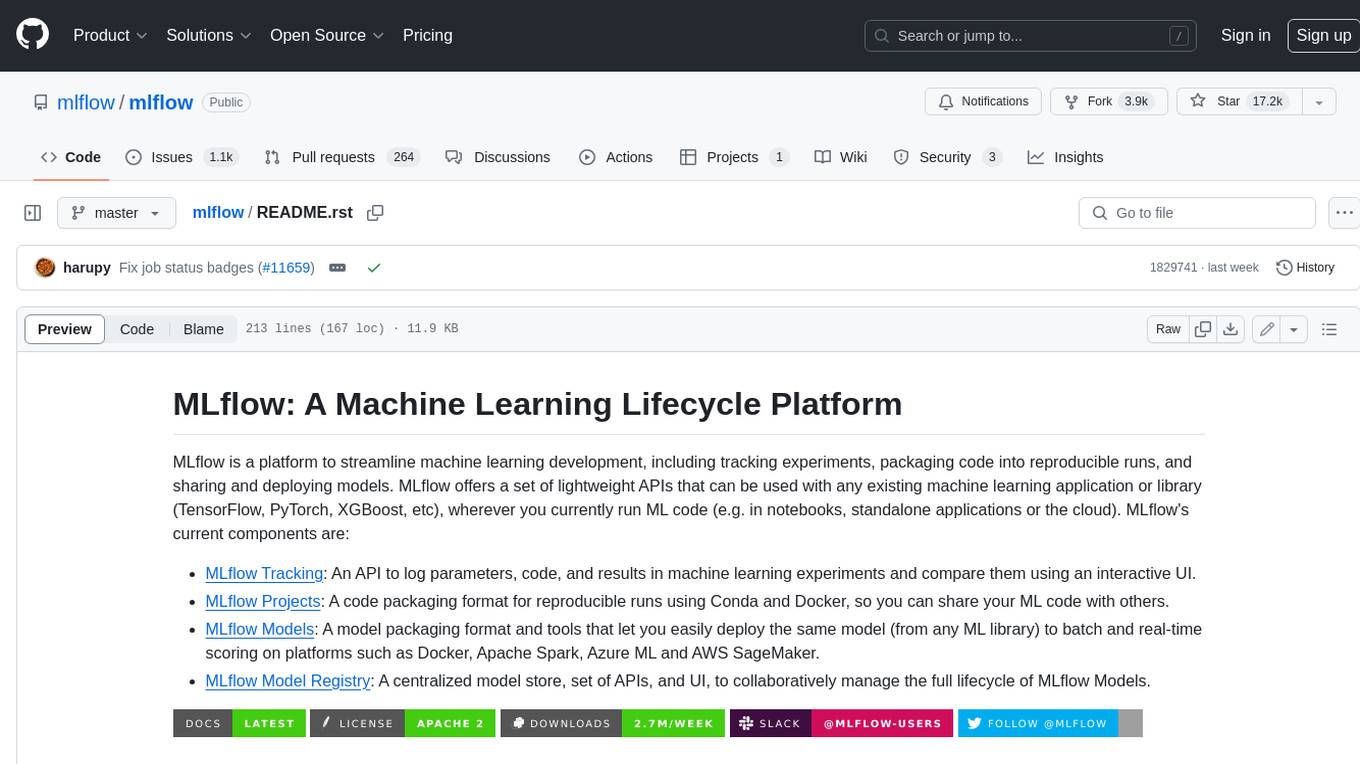
mlflow
MLflow is a platform to streamline machine learning development, including tracking experiments, packaging code into reproducible runs, and sharing and deploying models. MLflow offers a set of lightweight APIs that can be used with any existing machine learning application or library (TensorFlow, PyTorch, XGBoost, etc), wherever you currently run ML code (e.g. in notebooks, standalone applications or the cloud). MLflow's current components are:
* `MLflow Tracking
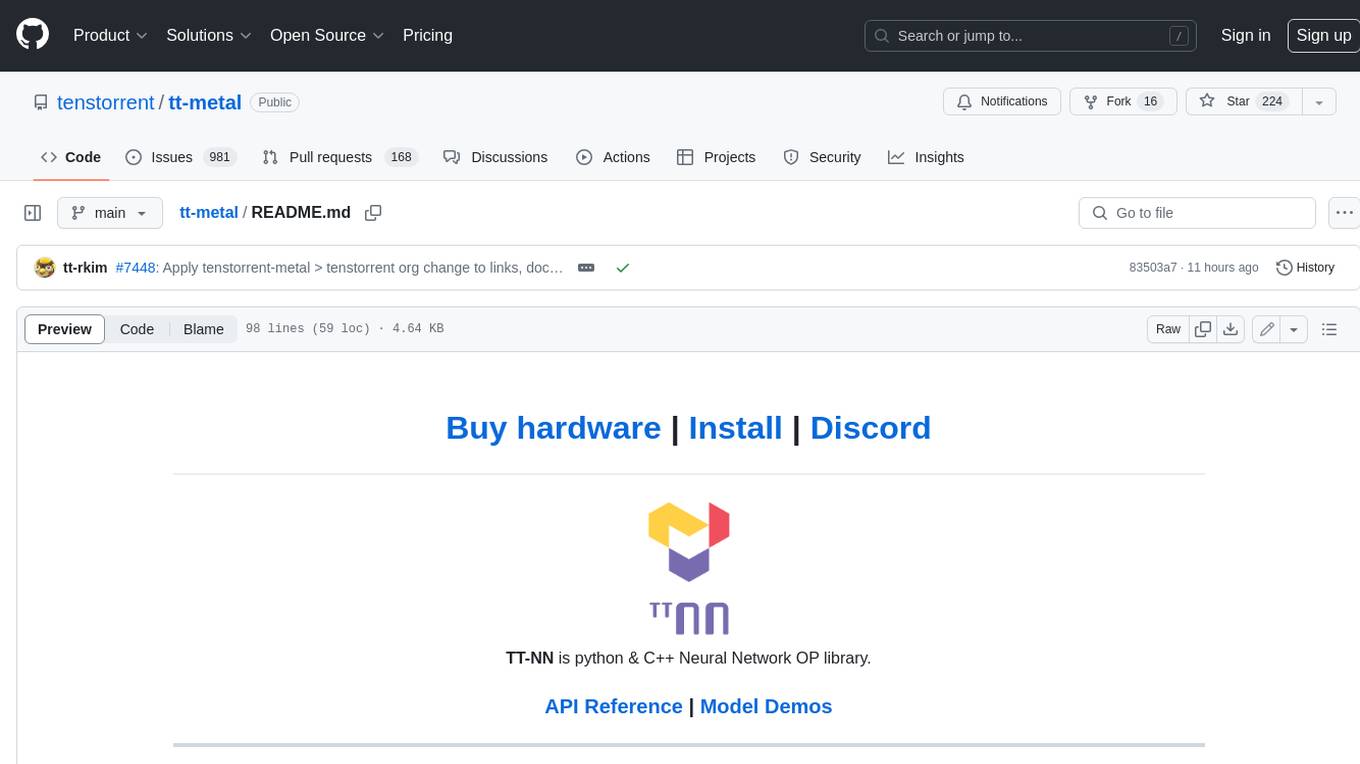
tt-metal
TT-NN is a python & C++ Neural Network OP library. It provides a low-level programming model, TT-Metalium, enabling kernel development for Tenstorrent hardware.
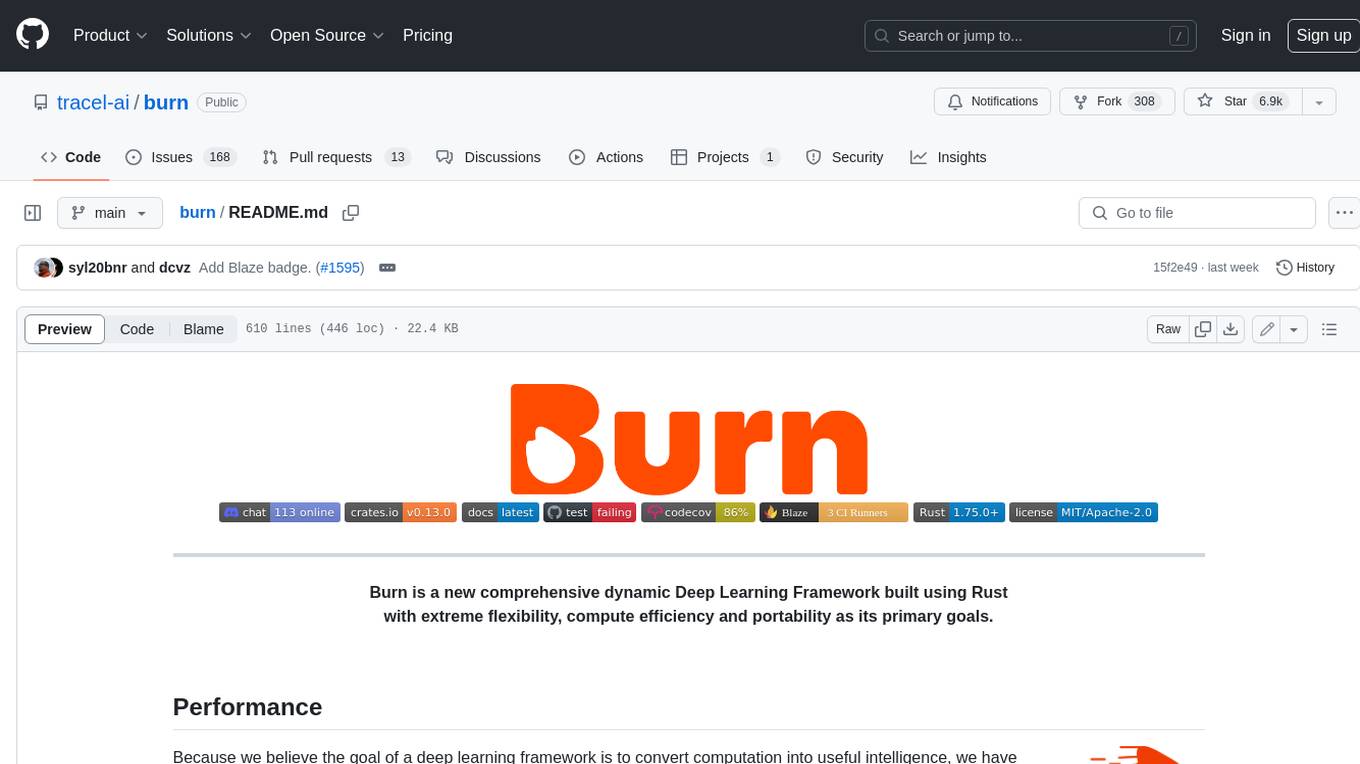
burn
Burn is a new comprehensive dynamic Deep Learning Framework built using Rust with extreme flexibility, compute efficiency and portability as its primary goals.
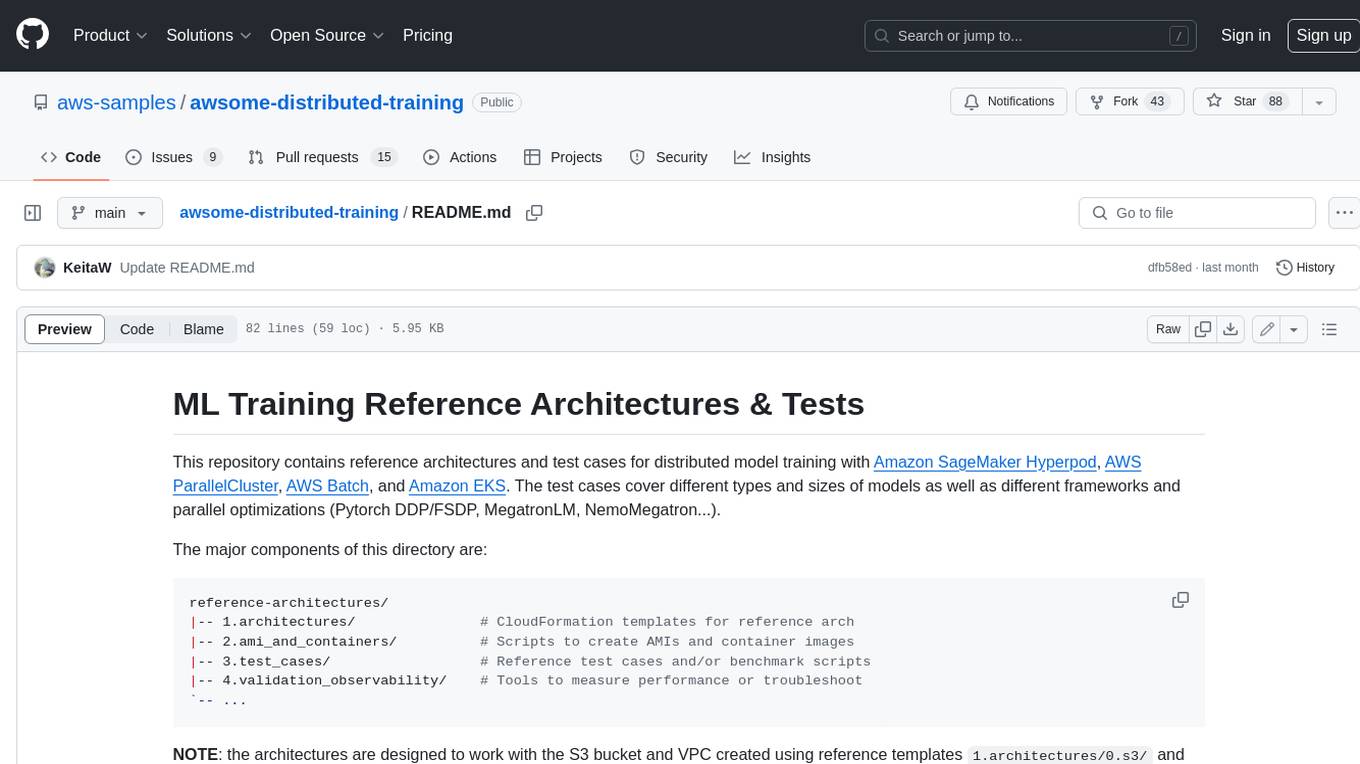
awsome-distributed-training
This repository contains reference architectures and test cases for distributed model training with Amazon SageMaker Hyperpod, AWS ParallelCluster, AWS Batch, and Amazon EKS. The test cases cover different types and sizes of models as well as different frameworks and parallel optimizations (Pytorch DDP/FSDP, MegatronLM, NemoMegatron...).
For similar jobs
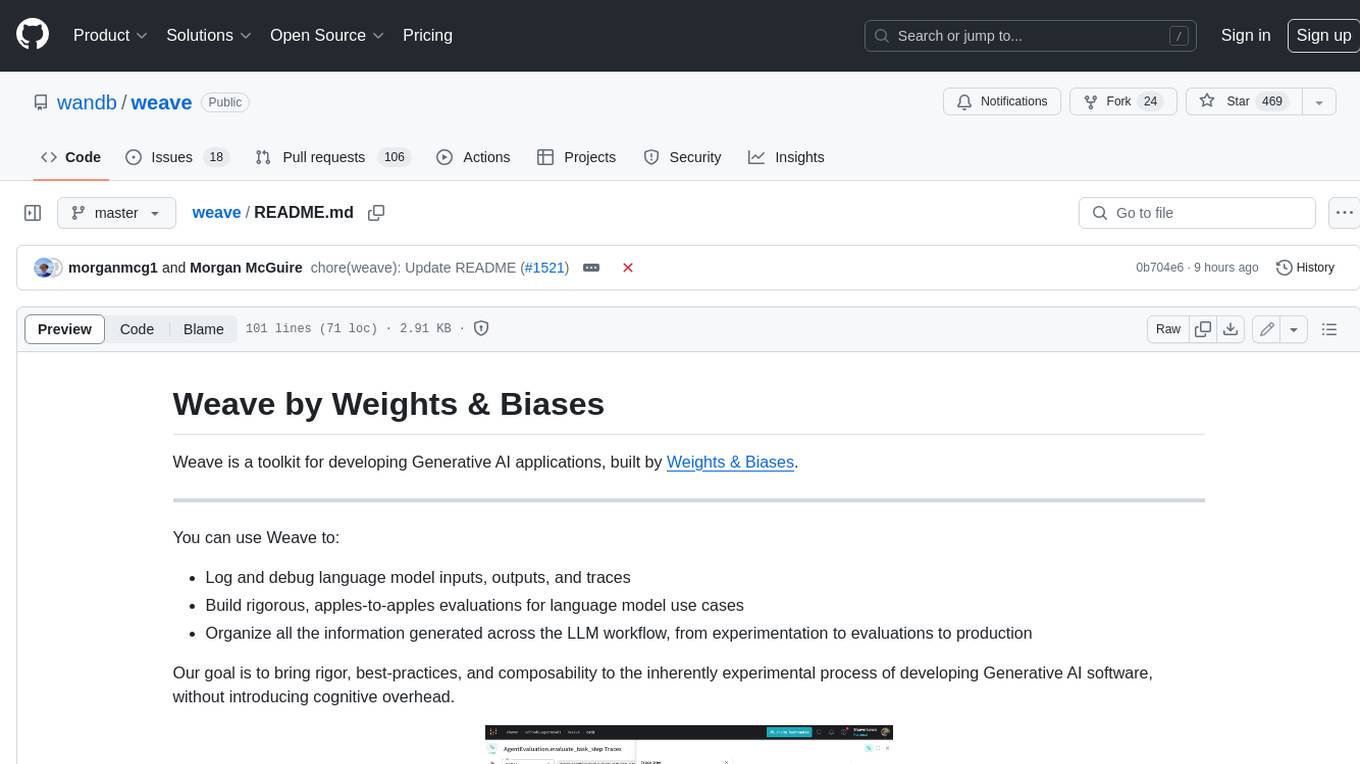
weave
Weave is a toolkit for developing Generative AI applications, built by Weights & Biases. With Weave, you can log and debug language model inputs, outputs, and traces; build rigorous, apples-to-apples evaluations for language model use cases; and organize all the information generated across the LLM workflow, from experimentation to evaluations to production. Weave aims to bring rigor, best-practices, and composability to the inherently experimental process of developing Generative AI software, without introducing cognitive overhead.
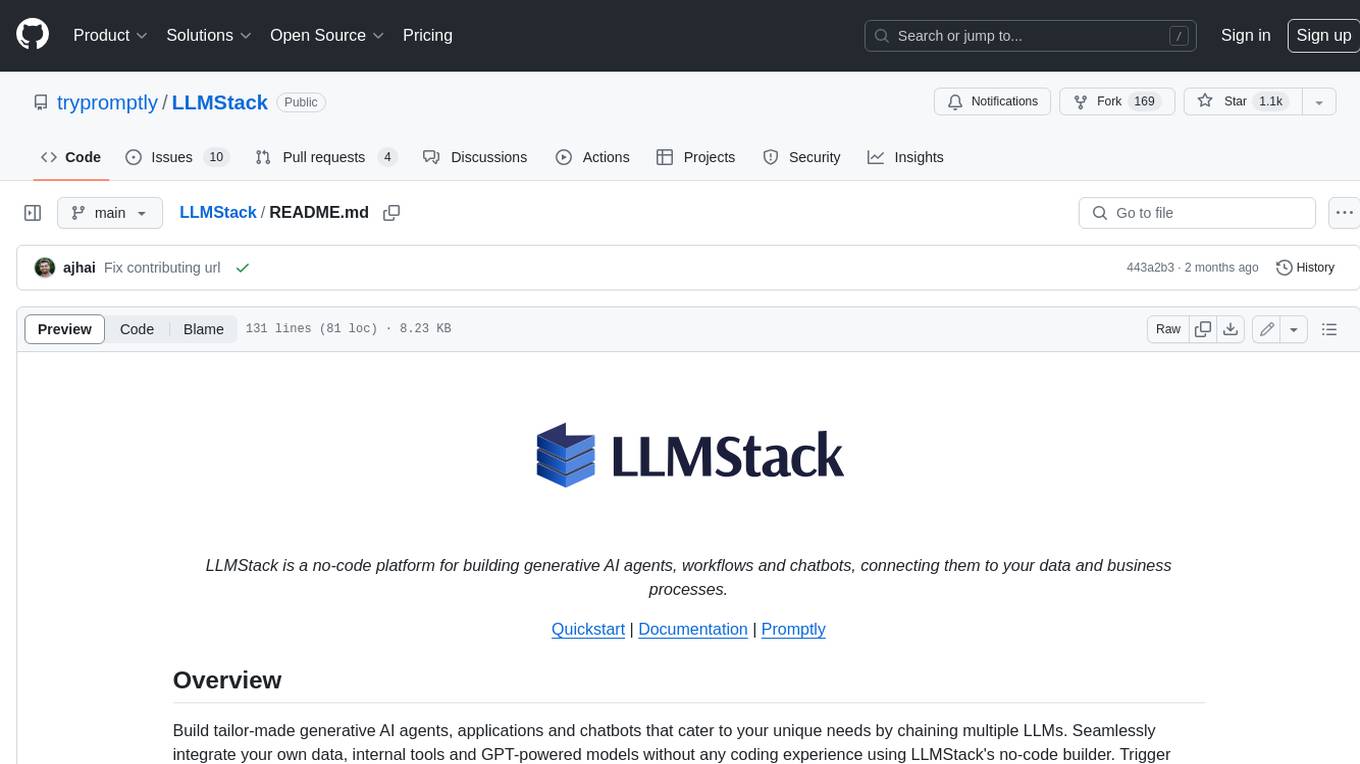
LLMStack
LLMStack is a no-code platform for building generative AI agents, workflows, and chatbots. It allows users to connect their own data, internal tools, and GPT-powered models without any coding experience. LLMStack can be deployed to the cloud or on-premise and can be accessed via HTTP API or triggered from Slack or Discord.
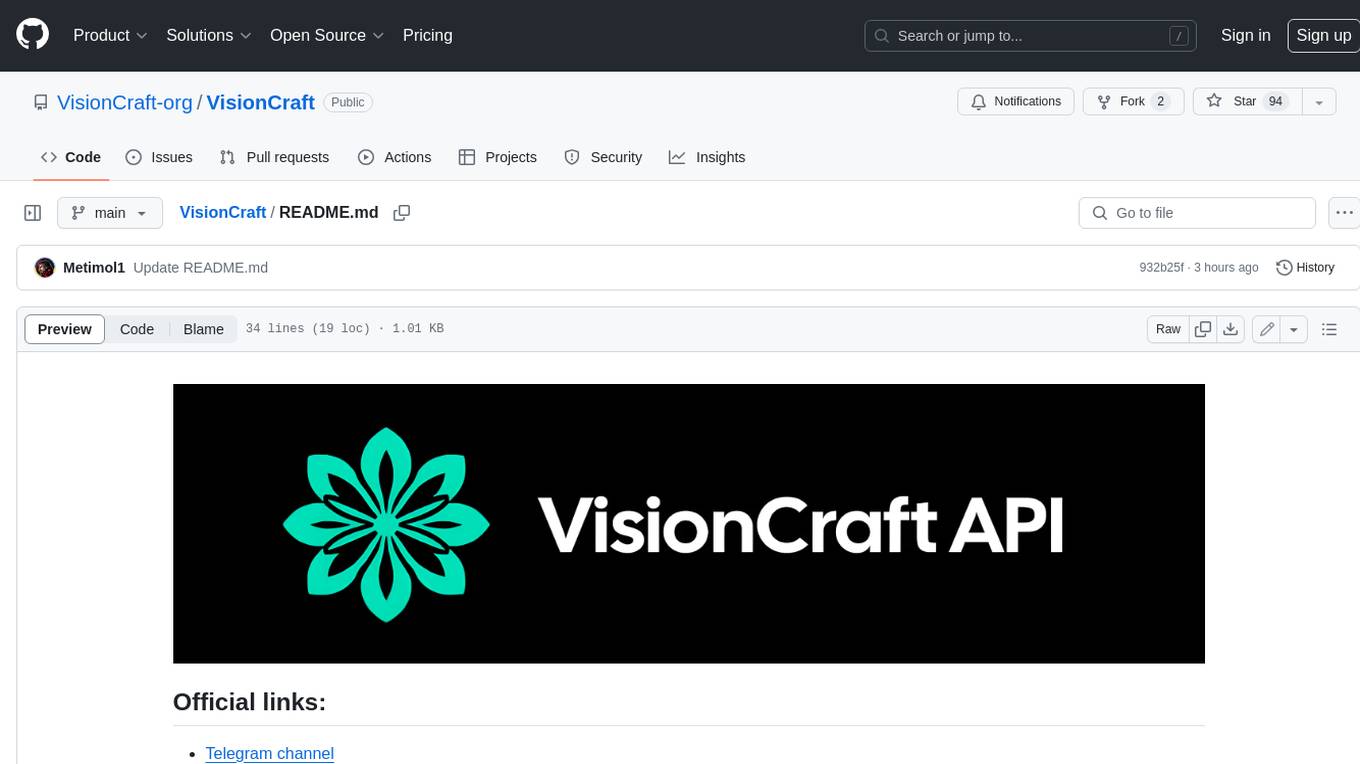
VisionCraft
The VisionCraft API is a free API for using over 100 different AI models. From images to sound.
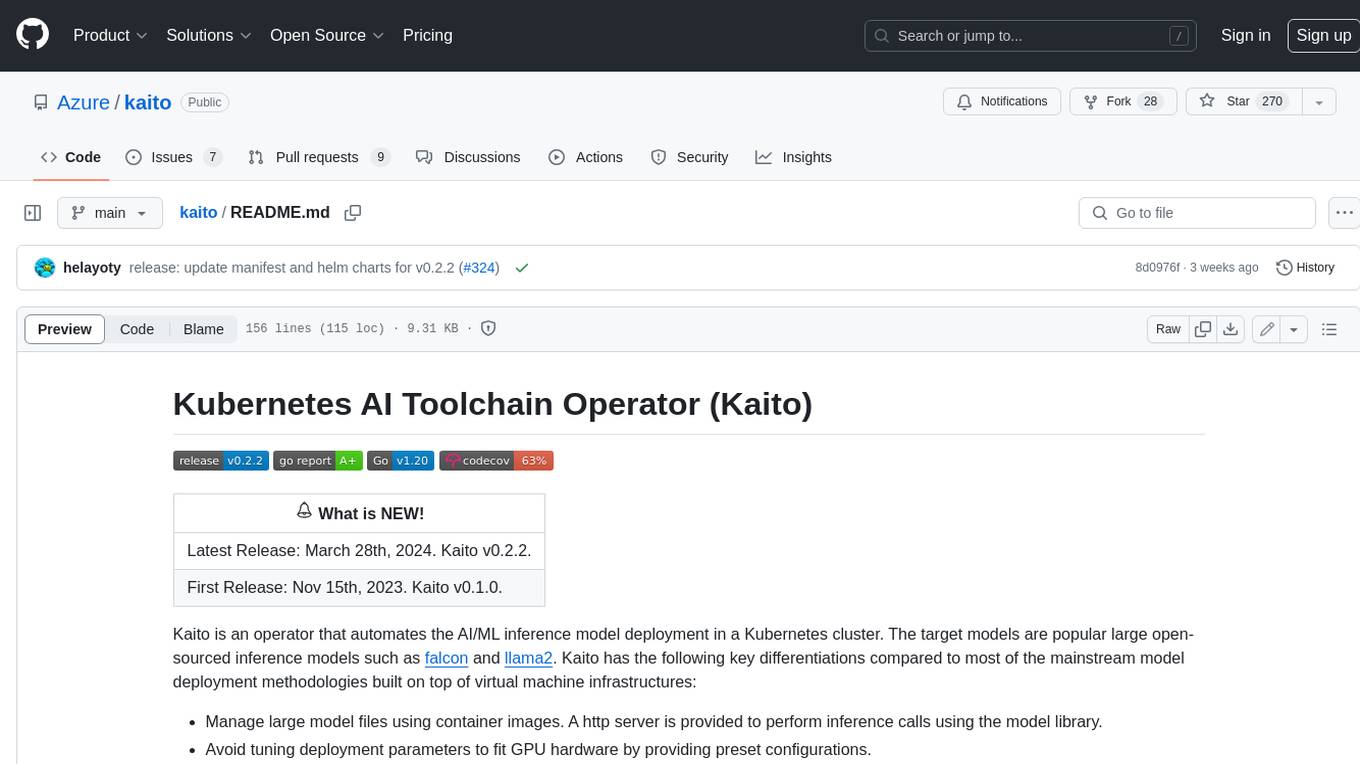
kaito
Kaito is an operator that automates the AI/ML inference model deployment in a Kubernetes cluster. It manages large model files using container images, avoids tuning deployment parameters to fit GPU hardware by providing preset configurations, auto-provisions GPU nodes based on model requirements, and hosts large model images in the public Microsoft Container Registry (MCR) if the license allows. Using Kaito, the workflow of onboarding large AI inference models in Kubernetes is largely simplified.
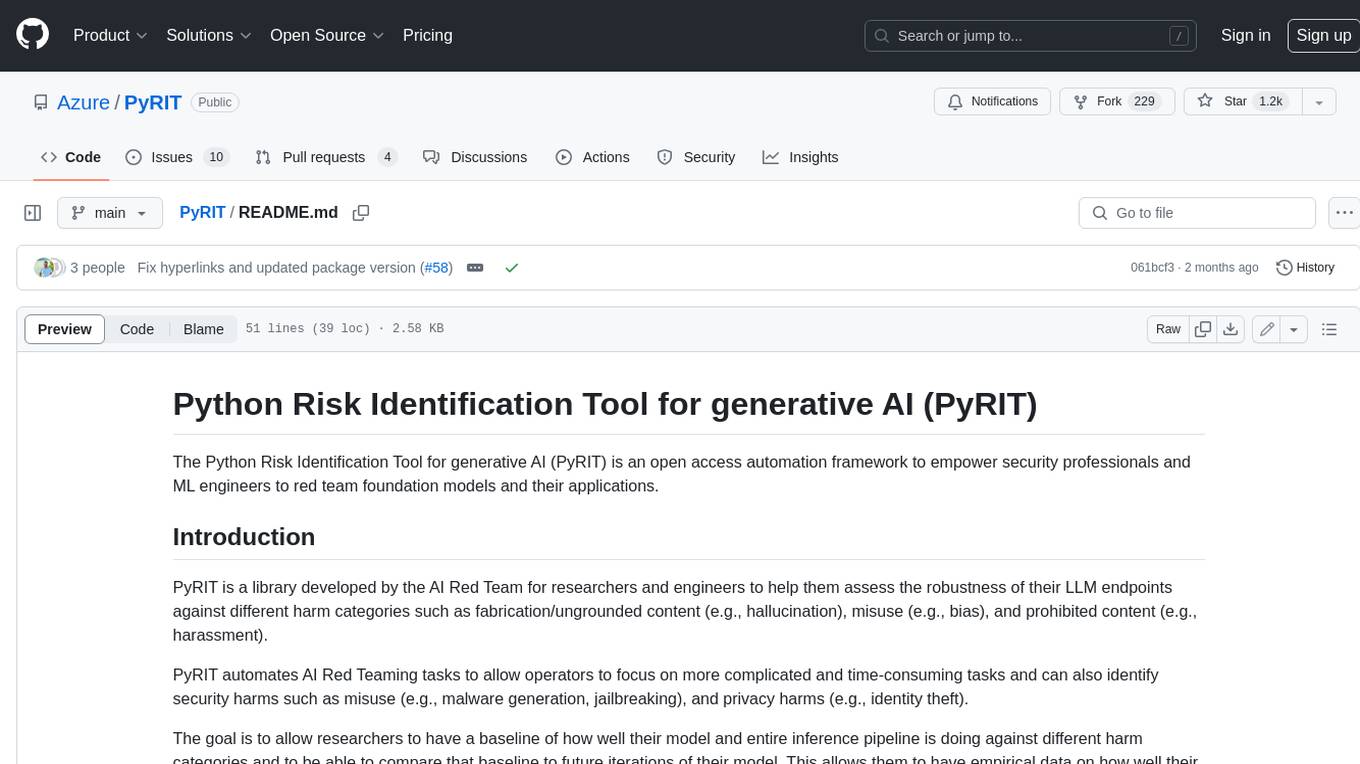
PyRIT
PyRIT is an open access automation framework designed to empower security professionals and ML engineers to red team foundation models and their applications. It automates AI Red Teaming tasks to allow operators to focus on more complicated and time-consuming tasks and can also identify security harms such as misuse (e.g., malware generation, jailbreaking), and privacy harms (e.g., identity theft). The goal is to allow researchers to have a baseline of how well their model and entire inference pipeline is doing against different harm categories and to be able to compare that baseline to future iterations of their model. This allows them to have empirical data on how well their model is doing today, and detect any degradation of performance based on future improvements.
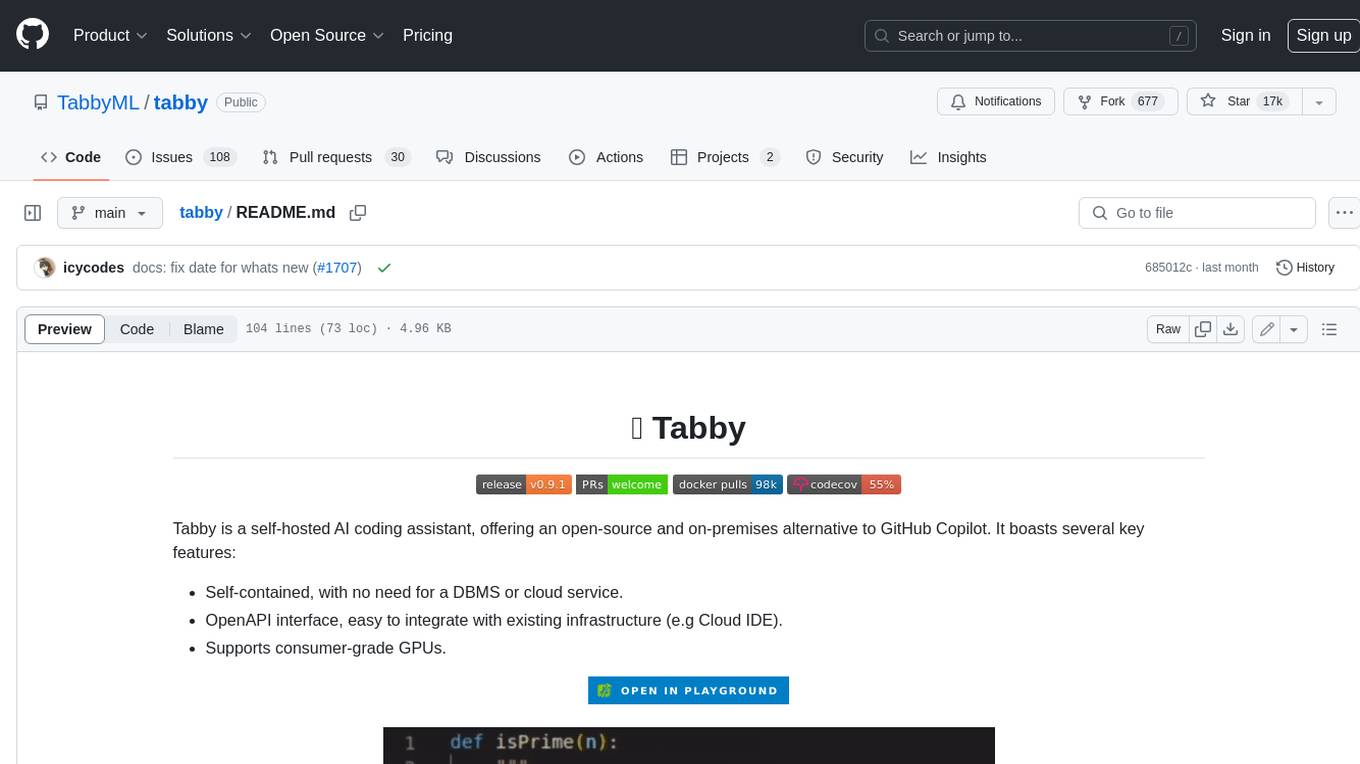
tabby
Tabby is a self-hosted AI coding assistant, offering an open-source and on-premises alternative to GitHub Copilot. It boasts several key features: * Self-contained, with no need for a DBMS or cloud service. * OpenAPI interface, easy to integrate with existing infrastructure (e.g Cloud IDE). * Supports consumer-grade GPUs.
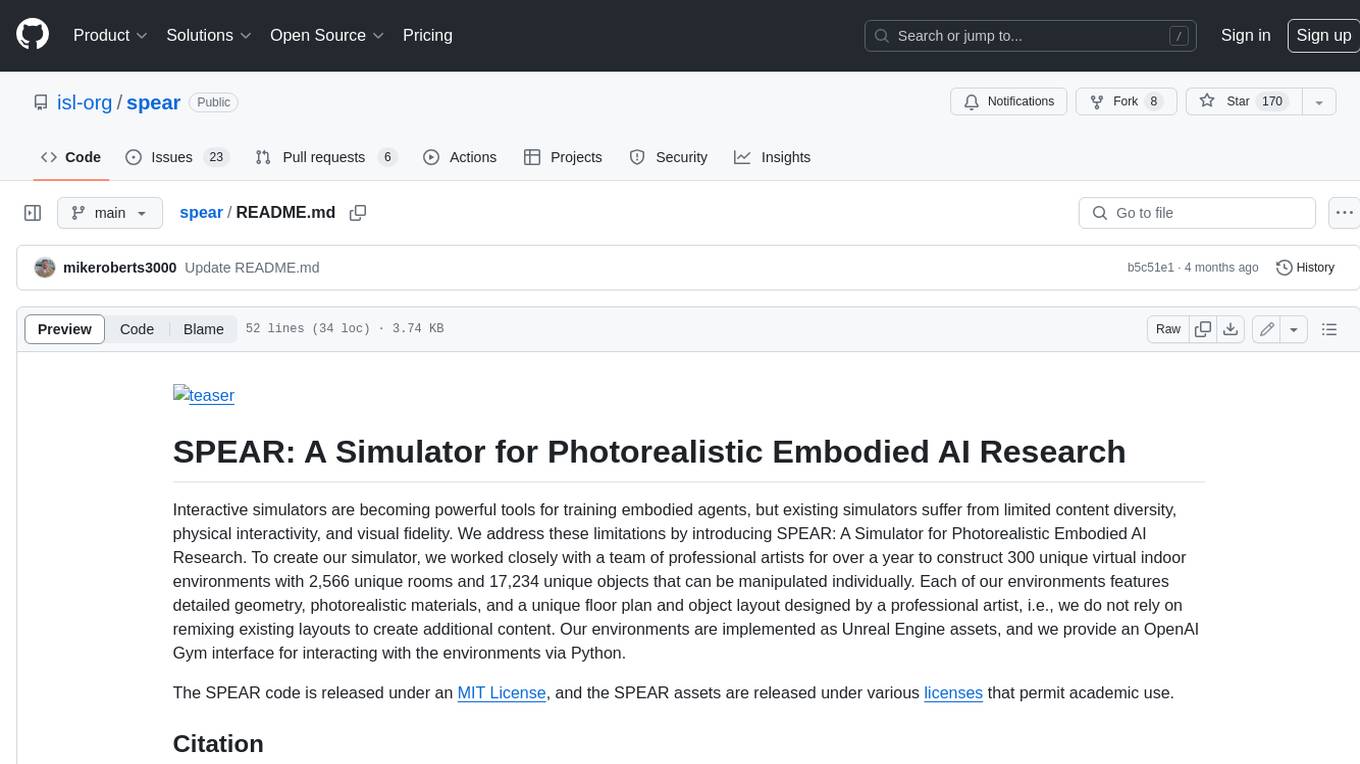
spear
SPEAR (Simulator for Photorealistic Embodied AI Research) is a powerful tool for training embodied agents. It features 300 unique virtual indoor environments with 2,566 unique rooms and 17,234 unique objects that can be manipulated individually. Each environment is designed by a professional artist and features detailed geometry, photorealistic materials, and a unique floor plan and object layout. SPEAR is implemented as Unreal Engine assets and provides an OpenAI Gym interface for interacting with the environments via Python.
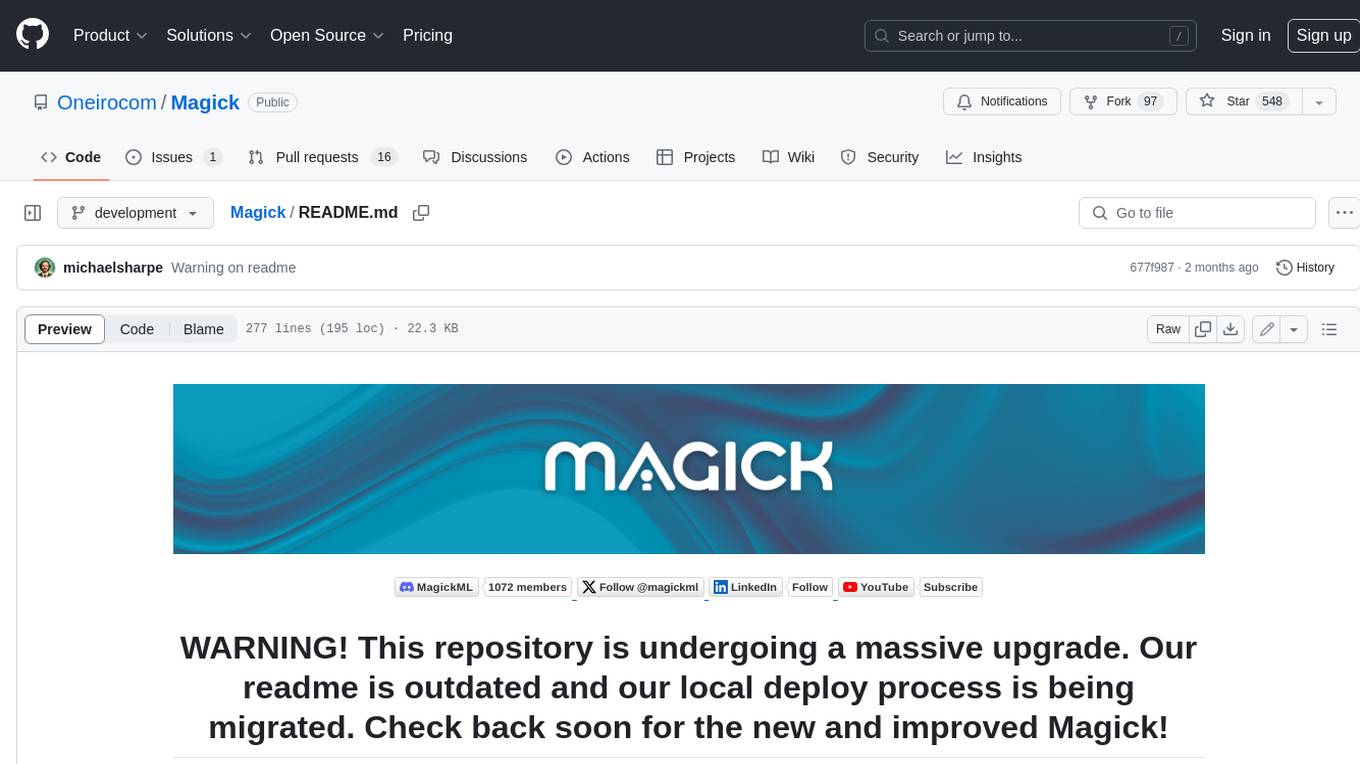
Magick
Magick is a groundbreaking visual AIDE (Artificial Intelligence Development Environment) for no-code data pipelines and multimodal agents. Magick can connect to other services and comes with nodes and templates well-suited for intelligent agents, chatbots, complex reasoning systems and realistic characters.