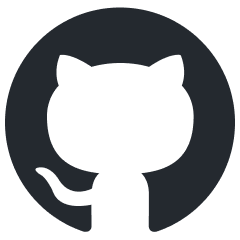
modelfusion
The TypeScript library for building AI applications.
Stars: 918
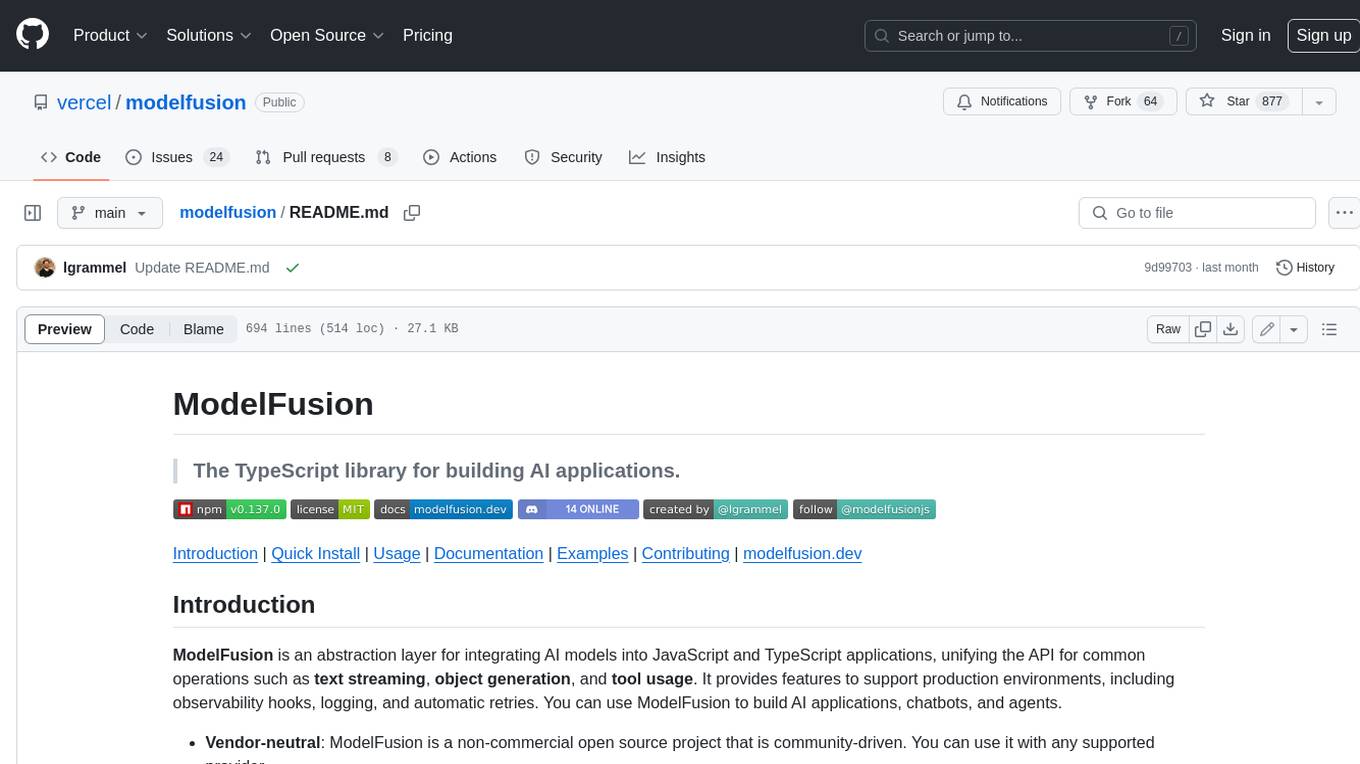
ModelFusion is an abstraction layer for integrating AI models into JavaScript and TypeScript applications, unifying the API for common operations such as text streaming, object generation, and tool usage. It provides features to support production environments, including observability hooks, logging, and automatic retries. You can use ModelFusion to build AI applications, chatbots, and agents. ModelFusion is a non-commercial open source project that is community-driven. You can use it with any supported provider. ModelFusion supports a wide range of models including text generation, image generation, vision, text-to-speech, speech-to-text, and embedding models. ModelFusion infers TypeScript types wherever possible and validates model responses. ModelFusion provides an observer framework and logging support. ModelFusion ensures seamless operation through automatic retries, throttling, and error handling mechanisms. ModelFusion is fully tree-shakeable, can be used in serverless environments, and only uses a minimal set of dependencies.
README:
Introduction | Quick Install | Usage | Documentation | Examples | Contributing | modelfusion.dev
[!IMPORTANT] ModelFusion has joined Vercel and is being integrated into the Vercel AI SDK. We are bringing the best parts of modelfusion to the Vercel AI SDK, starting with text generation, structured object generation, and tool calls. Please check out the AI SDK for the latest developments.
ModelFusion is an abstraction layer for integrating AI models into JavaScript and TypeScript applications, unifying the API for common operations such as text streaming, object generation, and tool usage. It provides features to support production environments, including observability hooks, logging, and automatic retries. You can use ModelFusion to build AI applications, chatbots, and agents.
- Vendor-neutral: ModelFusion is a non-commercial open source project that is community-driven. You can use it with any supported provider.
- Multi-modal: ModelFusion supports a wide range of models including text generation, image generation, vision, text-to-speech, speech-to-text, and embedding models.
- Type inference and validation: ModelFusion infers TypeScript types wherever possible and validates model responses.
- Observability and logging: ModelFusion provides an observer framework and logging support.
- Resilience and robustness: ModelFusion ensures seamless operation through automatic retries, throttling, and error handling mechanisms.
- Built for production: ModelFusion is fully tree-shakeable, can be used in serverless environments, and only uses a minimal set of dependencies.
npm install modelfusion
Or use a starter template:
- ModelFusion terminal app starter
- Next.js, Vercel AI SDK, Llama.cpp & ModelFusion starter
- Next.js, Vercel AI SDK, Ollama & ModelFusion starter
[!TIP] The basic examples are a great way to get started and to explore in parallel with the documentation. You can find them in the examples/basic folder.
You can provide API keys for the different integrations using environment variables (e.g., OPENAI_API_KEY
) or pass them into the model constructors as options.
Generate text using a language model and a prompt. You can stream the text if it is supported by the model. You can use images for multi-modal prompting if the model supports it (e.g. with llama.cpp). You can use prompt styles to use text, instruction, or chat prompts.
import { generateText, openai } from "modelfusion";
const text = await generateText({
model: openai.CompletionTextGenerator({ model: "gpt-3.5-turbo-instruct" }),
prompt: "Write a short story about a robot learning to love:\n\n",
});
Providers: OpenAI, OpenAI compatible, Llama.cpp, Ollama, Mistral, Hugging Face, Cohere
import { streamText, openai } from "modelfusion";
const textStream = await streamText({
model: openai.CompletionTextGenerator({ model: "gpt-3.5-turbo-instruct" }),
prompt: "Write a short story about a robot learning to love:\n\n",
});
for await (const textPart of textStream) {
process.stdout.write(textPart);
}
Providers: OpenAI, OpenAI compatible, Llama.cpp, Ollama, Mistral, Cohere
Multi-modal vision models such as GPT 4 Vision can process images as part of the prompt.
import { streamText, openai } from "modelfusion";
import { readFileSync } from "fs";
const image = readFileSync("./image.png");
const textStream = await streamText({
model: openai
.ChatTextGenerator({ model: "gpt-4-vision-preview" })
.withInstructionPrompt(),
prompt: {
instruction: [
{ type: "text", text: "Describe the image in detail." },
{ type: "image", image, mimeType: "image/png" },
],
},
});
for await (const textPart of textStream) {
process.stdout.write(textPart);
}
Providers: OpenAI, OpenAI compatible, Llama.cpp, Ollama
Generate typed objects using a language model and a schema.
Generate an object that matches a schema.
import {
ollama,
zodSchema,
generateObject,
jsonObjectPrompt,
} from "modelfusion";
const sentiment = await generateObject({
model: ollama
.ChatTextGenerator({
model: "openhermes2.5-mistral",
maxGenerationTokens: 1024,
temperature: 0,
})
.asObjectGenerationModel(jsonObjectPrompt.instruction()),
schema: zodSchema(
z.object({
sentiment: z
.enum(["positive", "neutral", "negative"])
.describe("Sentiment."),
})
),
prompt: {
system:
"You are a sentiment evaluator. " +
"Analyze the sentiment of the following product review:",
instruction:
"After I opened the package, I was met by a very unpleasant smell " +
"that did not disappear even after washing. Never again!",
},
});
Providers: OpenAI, Ollama, Llama.cpp
Stream a object that matches a schema. Partial objects before the final part are untyped JSON.
import { zodSchema, openai, streamObject } from "modelfusion";
const objectStream = await streamObject({
model: openai
.ChatTextGenerator(/* ... */)
.asFunctionCallObjectGenerationModel({
fnName: "generateCharacter",
fnDescription: "Generate character descriptions.",
})
.withTextPrompt(),
schema: zodSchema(
z.object({
characters: z.array(
z.object({
name: z.string(),
class: z
.string()
.describe("Character class, e.g. warrior, mage, or thief."),
description: z.string(),
})
),
})
),
prompt: "Generate 3 character descriptions for a fantasy role playing game.",
});
for await (const { partialObject } of objectStream) {
console.clear();
console.log(partialObject);
}
Providers: OpenAI, Ollama, Llama.cpp
Generate an image from a prompt.
import { generateImage, openai } from "modelfusion";
const image = await generateImage({
model: openai.ImageGenerator({ model: "dall-e-3", size: "1024x1024" }),
prompt:
"the wicked witch of the west in the style of early 19th century painting",
});
Providers: OpenAI (Dall·E), Stability AI, Automatic1111
Synthesize speech (audio) from text. Also called TTS (text-to-speech).
generateSpeech
synthesizes speech from text.
import { generateSpeech, lmnt } from "modelfusion";
// `speech` is a Uint8Array with MP3 audio data
const speech = await generateSpeech({
model: lmnt.SpeechGenerator({
voice: "034b632b-df71-46c8-b440-86a42ffc3cf3", // Henry
}),
text:
"Good evening, ladies and gentlemen! Exciting news on the airwaves tonight " +
"as The Rolling Stones unveil 'Hackney Diamonds,' their first collection of " +
"fresh tunes in nearly twenty years, featuring the illustrious Lady Gaga, the " +
"magical Stevie Wonder, and the final beats from the late Charlie Watts.",
});
Providers: Eleven Labs, LMNT, OpenAI
generateSpeech
generates a stream of speech chunks from text or from a text stream. Depending on the model, this can be fully duplex.
import { streamSpeech, elevenlabs } from "modelfusion";
const textStream: AsyncIterable<string>;
const speechStream = await streamSpeech({
model: elevenlabs.SpeechGenerator({
model: "eleven_turbo_v2",
voice: "pNInz6obpgDQGcFmaJgB", // Adam
optimizeStreamingLatency: 1,
voiceSettings: { stability: 1, similarityBoost: 0.35 },
generationConfig: {
chunkLengthSchedule: [50, 90, 120, 150, 200],
},
}),
text: textStream,
});
for await (const part of speechStream) {
// each part is a Uint8Array with MP3 audio data
}
Providers: Eleven Labs
Transcribe speech (audio) data into text. Also called speech-to-text (STT).
import { generateTranscription, openai } from "modelfusion";
import fs from "node:fs";
const transcription = await generateTranscription({
model: openai.Transcriber({ model: "whisper-1" }),
mimeType: "audio/mp3",
audioData: await fs.promises.readFile("data/test.mp3"),
});
Providers: OpenAI (Whisper), Whisper.cpp
Create embeddings for text and other values. Embeddings are vectors that represent the essence of the values in the context of the model.
import { embed, embedMany, openai } from "modelfusion";
// embed single value:
const embedding = await embed({
model: openai.TextEmbedder({ model: "text-embedding-ada-002" }),
value: "At first, Nox didn't know what to do with the pup.",
});
// embed many values:
const embeddings = await embedMany({
model: openai.TextEmbedder({ model: "text-embedding-ada-002" }),
values: [
"At first, Nox didn't know what to do with the pup.",
"He keenly observed and absorbed everything around him, from the birds in the sky to the trees in the forest.",
],
});
Providers: OpenAI, OpenAI compatible, Llama.cpp, Ollama, Mistral, Hugging Face, Cohere
Classifies a value into a category.
import { classify, EmbeddingSimilarityClassifier, openai } from "modelfusion";
const classifier = new EmbeddingSimilarityClassifier({
embeddingModel: openai.TextEmbedder({ model: "text-embedding-ada-002" }),
similarityThreshold: 0.82,
clusters: [
{
name: "politics" as const,
values: [
"they will save the country!",
// ...
],
},
{
name: "chitchat" as const,
values: [
"how's the weather today?",
// ...
],
},
],
});
// strongly typed result:
const result = await classify({
model: classifier,
value: "don't you love politics?",
});
Classifiers: EmbeddingSimilarityClassifier
Split text into tokens and reconstruct the text from tokens.
const tokenizer = openai.Tokenizer({ model: "gpt-4" });
const text = "At first, Nox didn't know what to do with the pup.";
const tokenCount = await countTokens(tokenizer, text);
const tokens = await tokenizer.tokenize(text);
const tokensAndTokenTexts = await tokenizer.tokenizeWithTexts(text);
const reconstructedText = await tokenizer.detokenize(tokens);
Providers: OpenAI, Llama.cpp, Cohere
Tools are functions (and associated metadata) that can be executed by an AI model. They are useful for building chatbots and agents.
ModelFusion offers several tools out-of-the-box: Math.js, MediaWiki Search, SerpAPI, Google Custom Search. You can also create custom tools.
With runTool
, you can ask a tool-compatible language model (e.g. OpenAI chat) to invoke a single tool. runTool
first generates a tool call and then executes the tool with the arguments.
const { tool, toolCall, args, ok, result } = await runTool({
model: openai.ChatTextGenerator({ model: "gpt-3.5-turbo" }),
too: calculator,
prompt: [openai.ChatMessage.user("What's fourteen times twelve?")],
});
console.log(`Tool call:`, toolCall);
console.log(`Tool:`, tool);
console.log(`Arguments:`, args);
console.log(`Ok:`, ok);
console.log(`Result or Error:`, result);
With runTools
, you can ask a language model to generate several tool calls as well as text. The model will choose which tools (if any) should be called with which arguments. Both the text and the tool calls are optional. This function executes the tools.
const { text, toolResults } = await runTools({
model: openai.ChatTextGenerator({ model: "gpt-3.5-turbo" }),
tools: [calculator /* ... */],
prompt: [openai.ChatMessage.user("What's fourteen times twelve?")],
});
You can use runTools
to implement an agent loop that responds to user messages and executes tools. Learn more.
const texts = [
"A rainbow is an optical phenomenon that can occur under certain meteorological conditions.",
"It is caused by refraction, internal reflection and dispersion of light in water droplets resulting in a continuous spectrum of light appearing in the sky.",
// ...
];
const vectorIndex = new MemoryVectorIndex<string>();
const embeddingModel = openai.TextEmbedder({
model: "text-embedding-ada-002",
});
// update an index - usually done as part of an ingestion process:
await upsertIntoVectorIndex({
vectorIndex,
embeddingModel,
objects: texts,
getValueToEmbed: (text) => text,
});
// retrieve text chunks from the vector index - usually done at query time:
const retrievedTexts = await retrieve(
new VectorIndexRetriever({
vectorIndex,
embeddingModel,
maxResults: 3,
similarityThreshold: 0.8,
}),
"rainbow and water droplets"
);
Available Vector Stores: Memory, SQLite VSS, Pinecone
You can use different prompt styles (such as text, instruction or chat prompts) with ModelFusion text generation models. These prompt styles can be accessed through the methods .withTextPrompt()
, .withChatPrompt()
and .withInstructionPrompt()
:
const text = await generateText({
model: openai
.ChatTextGenerator({
// ...
})
.withTextPrompt(),
prompt: "Write a short story about a robot learning to love",
});
const text = await generateText({
model: llamacpp
.CompletionTextGenerator({
// run https://huggingface.co/TheBloke/Llama-2-7B-Chat-GGUF with llama.cpp
promptTemplate: llamacpp.prompt.Llama2, // Set prompt template
contextWindowSize: 4096, // Llama 2 context window size
maxGenerationTokens: 512,
})
.withInstructionPrompt(),
prompt: {
system: "You are a story writer.",
instruction: "Write a short story about a robot learning to love.",
},
});
const textStream = await streamText({
model: openai
.ChatTextGenerator({
model: "gpt-3.5-turbo",
})
.withChatPrompt(),
prompt: {
system: "You are a celebrated poet.",
messages: [
{
role: "user",
content: "Suggest a name for a robot.",
},
{
role: "assistant",
content: "I suggest the name Robbie",
},
{
role: "user",
content: "Write a short story about Robbie learning to love",
},
],
},
});
You an use prompt templates with image models as well, e.g. to use a basic text prompt. It is available as a shorthand method:
const image = await generateImage({
model: stability
.ImageGenerator({
//...
})
.withTextPrompt(),
prompt:
"the wicked witch of the west in the style of early 19th century painting",
});
Prompt Template | Text Prompt |
---|---|
Automatic1111 | ✅ |
Stability | ✅ |
ModelFusion model functions return rich responses that include the raw (original) response and metadata when you set the fullResponse
argument to true
.
// access the raw response (needs to be typed) and the metadata:
const { text, rawResponse, metadata } = await generateText({
model: openai.CompletionTextGenerator({
model: "gpt-3.5-turbo-instruct",
maxGenerationTokens: 1000,
n: 2, // generate 2 completions
}),
prompt: "Write a short story about a robot learning to love:\n\n",
fullResponse: true,
});
console.log(metadata);
// cast to the raw response type:
for (const choice of (rawResponse as OpenAICompletionResponse).choices) {
console.log(choice.text);
}
ModelFusion provides an observer framework and logging support. You can easily trace runs and call hierarchies, and you can add your own observers.
import { generateText, openai } from "modelfusion";
const text = await generateText({
model: openai.CompletionTextGenerator({ model: "gpt-3.5-turbo-instruct" }),
prompt: "Write a short story about a robot learning to love:\n\n",
logging: "detailed-object",
});
Examples for almost all of the individual functions and objects. Highly recommended to get started.
multi-modal, object streaming, image generation, text to speech, speech to text, text generation, object generation, embeddings
StoryTeller is an exploratory web application that creates short audio stories for pre-school kids.
Next.js app, OpenAI GPT-3.5-turbo, streaming, abort handling
A web chat with an AI assistant, implemented as a Next.js app.
terminal app, PDF parsing, in memory vector indices, retrieval augmented generation, hypothetical document embedding
Ask questions about a PDF document and get answers from the document.
Next.js app, image generation, transcription, object streaming, OpenAI, Stability AI, Ollama
Examples of using ModelFusion with Next.js 14 (App Router):
- image generation
- voice recording & transcription
- object streaming
Speech Streaming, OpenAI, Elevenlabs streaming, Vite, Fastify, ModelFusion Server
Given a prompt, the server returns both a text and a speech stream response.
terminal app, agent, BabyAGI
TypeScript implementation of the BabyAGI classic and BabyBeeAGI.
terminal app, ReAct agent, GPT-4, OpenAI functions, tools
Get answers to questions from Wikipedia, e.g. "Who was born first, Einstein or Picasso?"
terminal app, agent, tools, GPT-4
Small agent that solves middle school math problems. It uses a calculator tool to solve the problems.
terminal app, PDF parsing, recursive information extraction, in memory vector index, _style example retrieval, OpenAI GPT-4, cost calculation
Extracts information about a topic from a PDF and writes a tweet in your own style about it.
Cloudflare, OpenAI
Generate text on a Cloudflare Worker using ModelFusion and OpenAI.
Read the ModelFusion contributing guide to learn about the development process, how to propose bugfixes and improvements, and how to build and test your changes.
For Tasks:
Click tags to check more tools for each tasksFor Jobs:
Alternative AI tools for modelfusion
Similar Open Source Tools
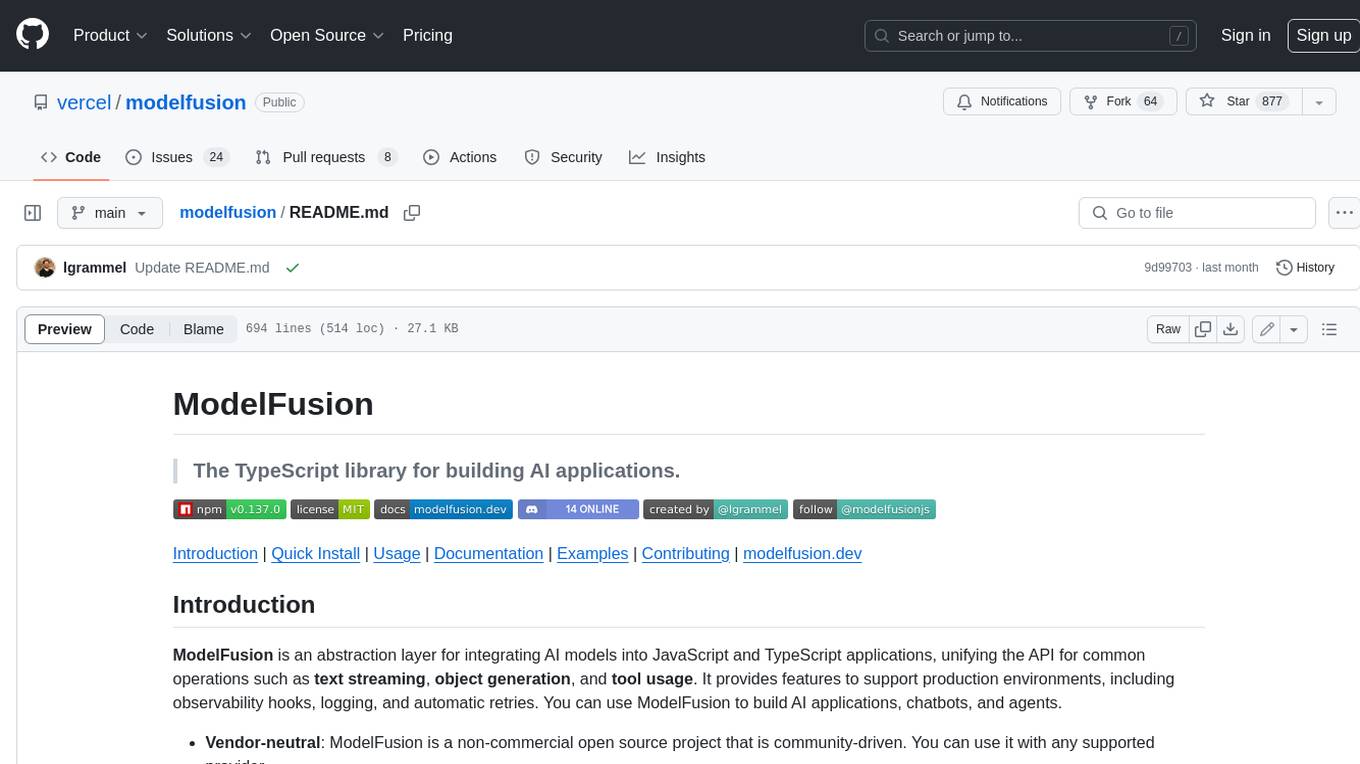
modelfusion
ModelFusion is an abstraction layer for integrating AI models into JavaScript and TypeScript applications, unifying the API for common operations such as text streaming, object generation, and tool usage. It provides features to support production environments, including observability hooks, logging, and automatic retries. You can use ModelFusion to build AI applications, chatbots, and agents. ModelFusion is a non-commercial open source project that is community-driven. You can use it with any supported provider. ModelFusion supports a wide range of models including text generation, image generation, vision, text-to-speech, speech-to-text, and embedding models. ModelFusion infers TypeScript types wherever possible and validates model responses. ModelFusion provides an observer framework and logging support. ModelFusion ensures seamless operation through automatic retries, throttling, and error handling mechanisms. ModelFusion is fully tree-shakeable, can be used in serverless environments, and only uses a minimal set of dependencies.
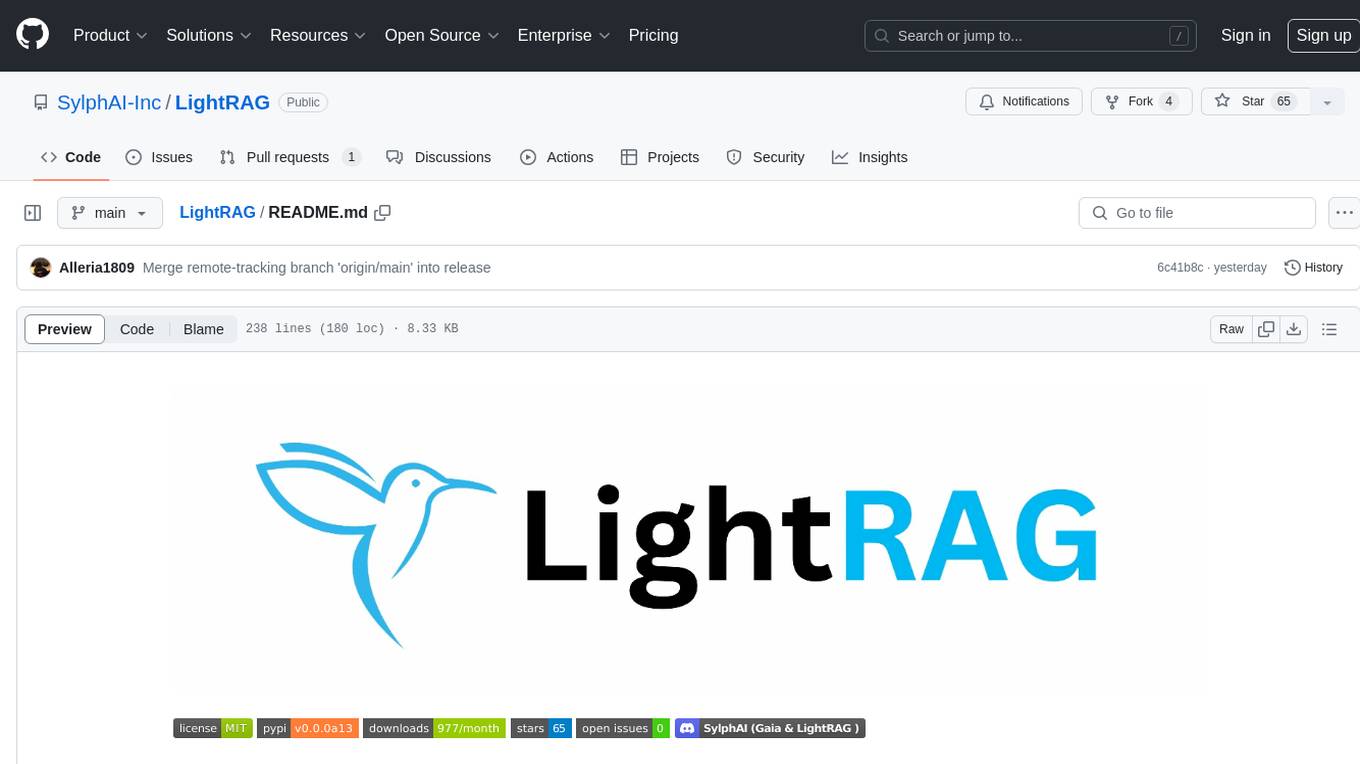
LightRAG
LightRAG is a PyTorch library designed for building and optimizing Retriever-Agent-Generator (RAG) pipelines. It follows principles of simplicity, quality, and optimization, offering developers maximum customizability with minimal abstraction. The library includes components for model interaction, output parsing, and structured data generation. LightRAG facilitates tasks like providing explanations and examples for concepts through a question-answering pipeline.
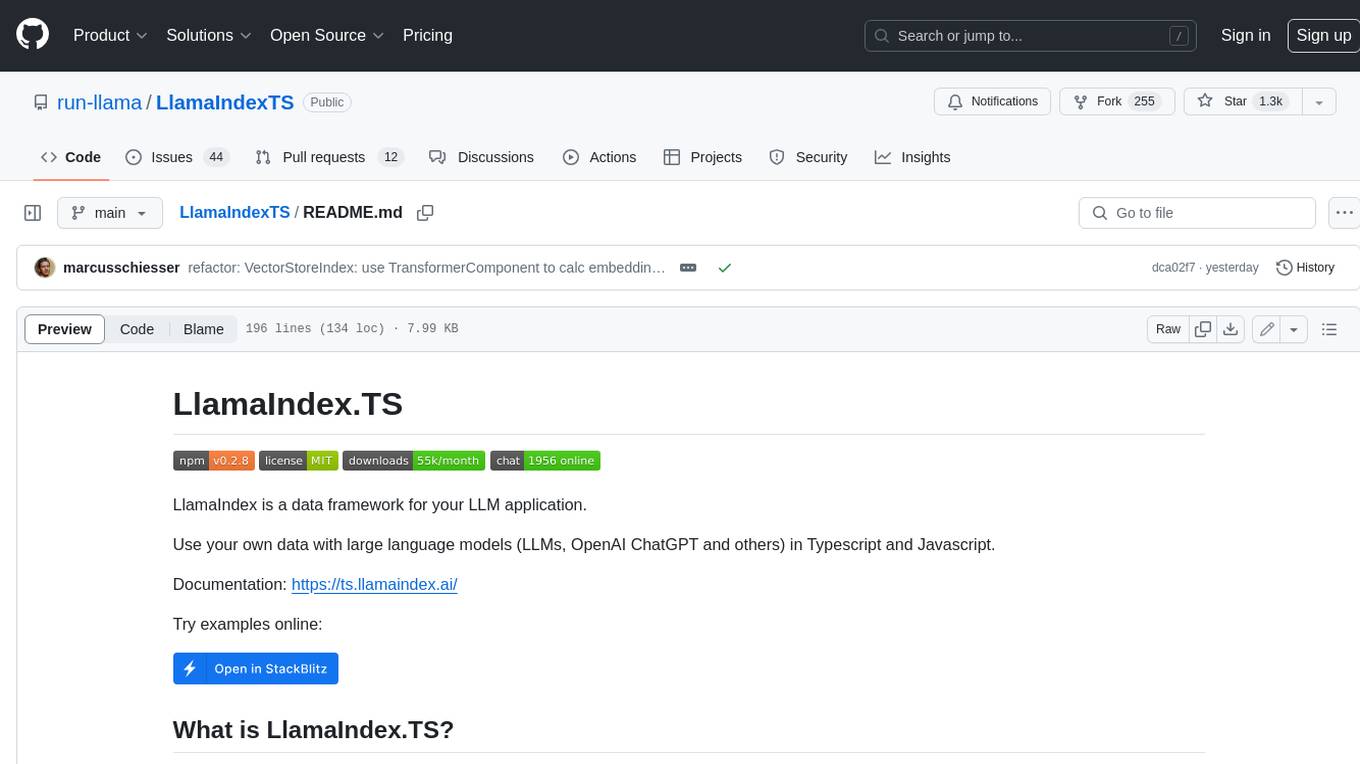
LlamaIndexTS
LlamaIndex.TS is a data framework for your LLM application. Use your own data with large language models (LLMs, OpenAI ChatGPT and others) in Typescript and Javascript.
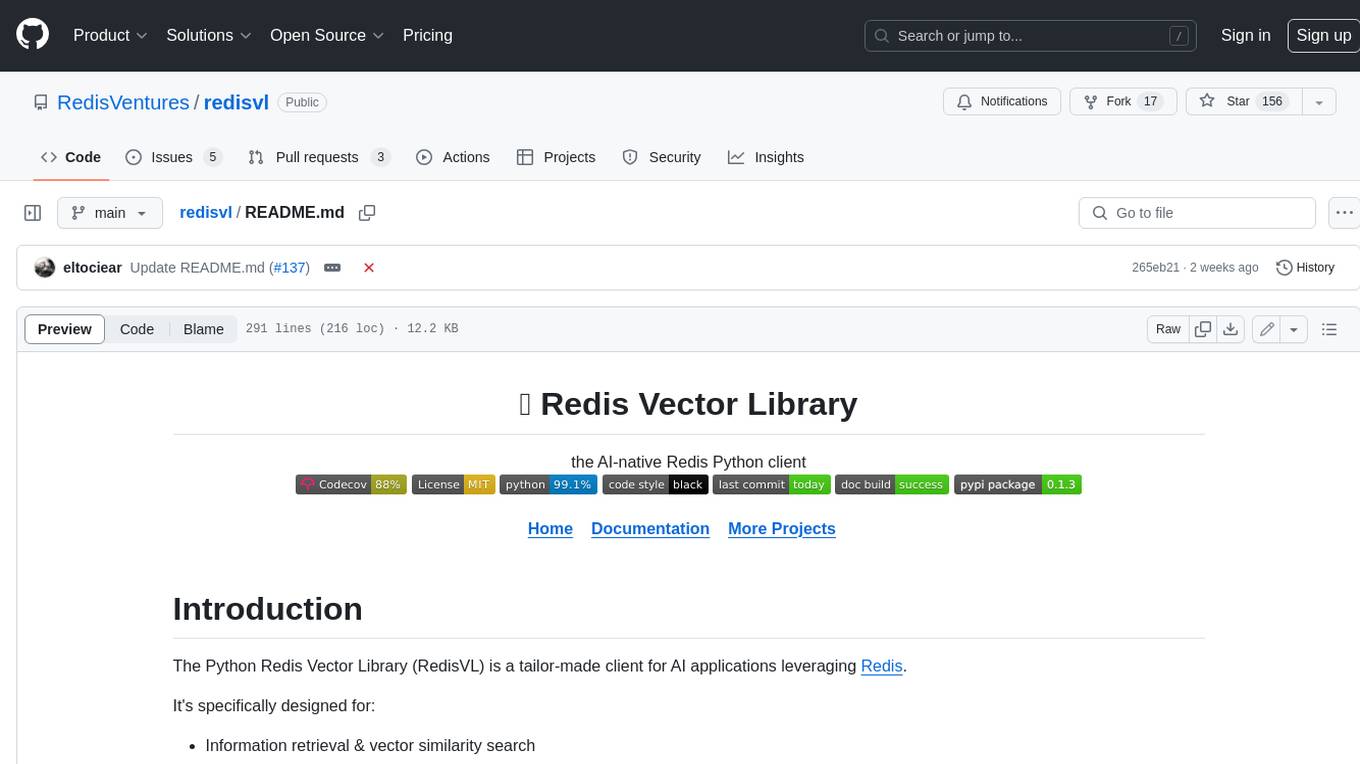
redisvl
Redis Vector Library (RedisVL) is a Python client library for building AI applications on top of Redis. It provides a high-level interface for managing vector indexes, performing vector search, and integrating with popular embedding models and providers. RedisVL is designed to make it easy for developers to build and deploy AI applications that leverage the speed, flexibility, and reliability of Redis.
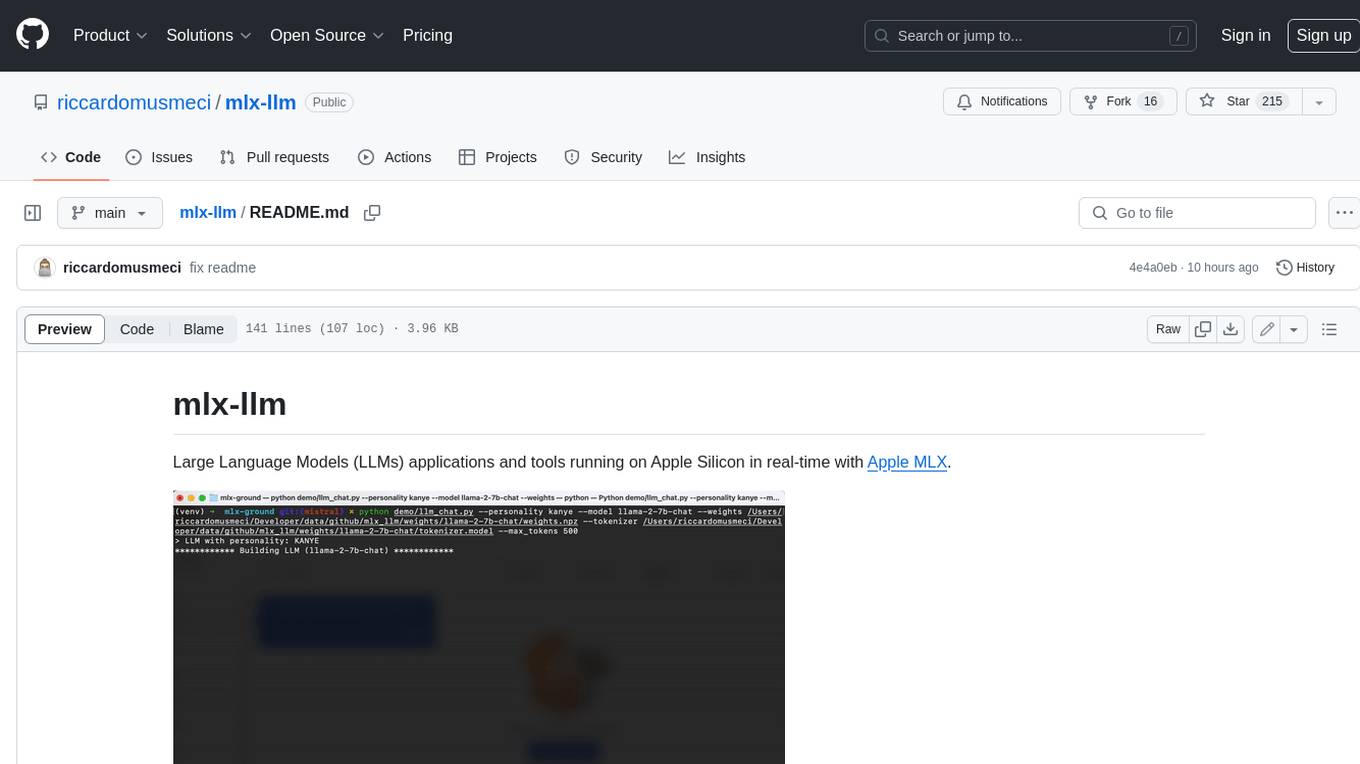
mlx-llm
mlx-llm is a library that allows you to run Large Language Models (LLMs) on Apple Silicon devices in real-time using Apple's MLX framework. It provides a simple and easy-to-use API for creating, loading, and using LLM models, as well as a variety of applications such as chatbots, fine-tuning, and retrieval-augmented generation.
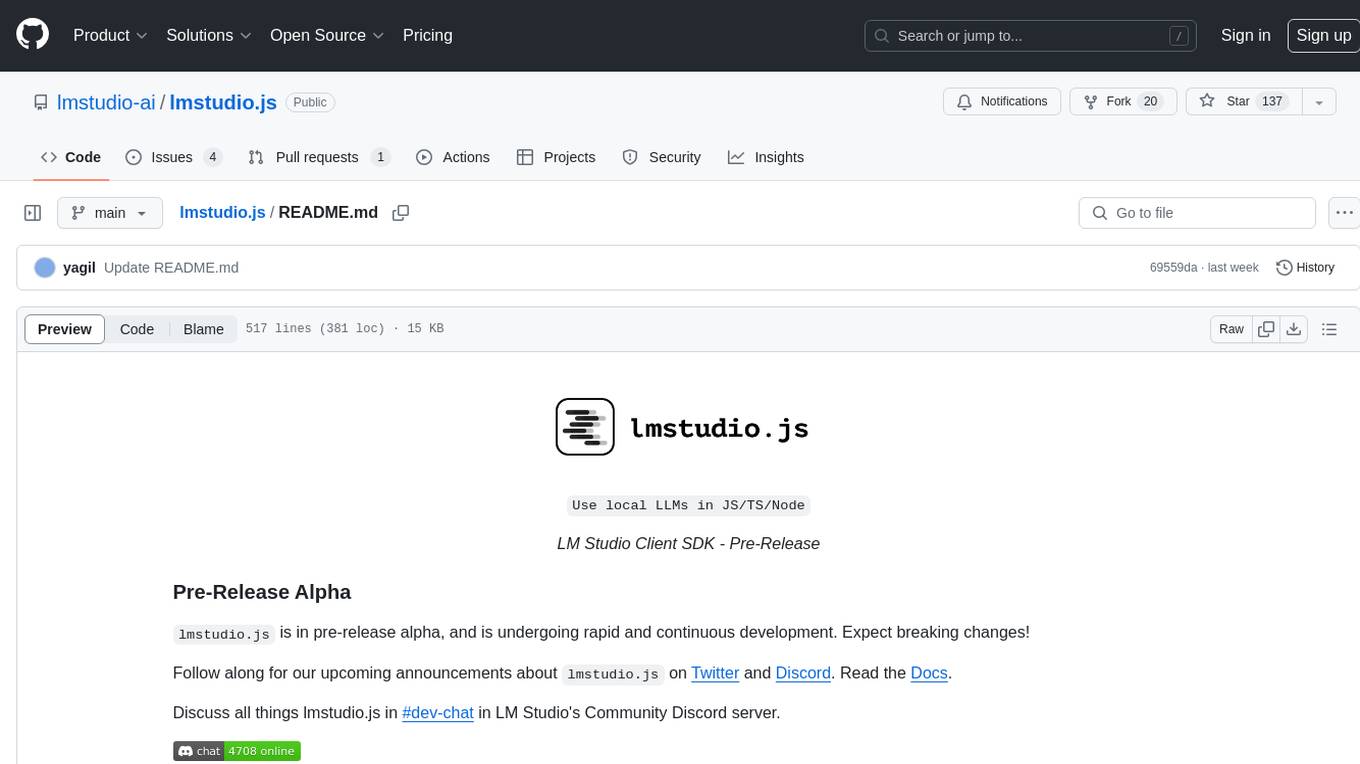
lmstudio.js
lmstudio.js is a pre-release alpha client SDK for LM Studio, allowing users to use local LLMs in JS/TS/Node. It is currently undergoing rapid development with breaking changes expected. Users can follow LM Studio's announcements on Twitter and Discord. The SDK provides API usage for loading models, predicting text, setting up the local LLM server, and more. It supports features like custom loading progress tracking, model unloading, structured output prediction, and cancellation of predictions. Users can interact with LM Studio through the CLI tool 'lms' and perform tasks like text completion, conversation, and getting prediction statistics.
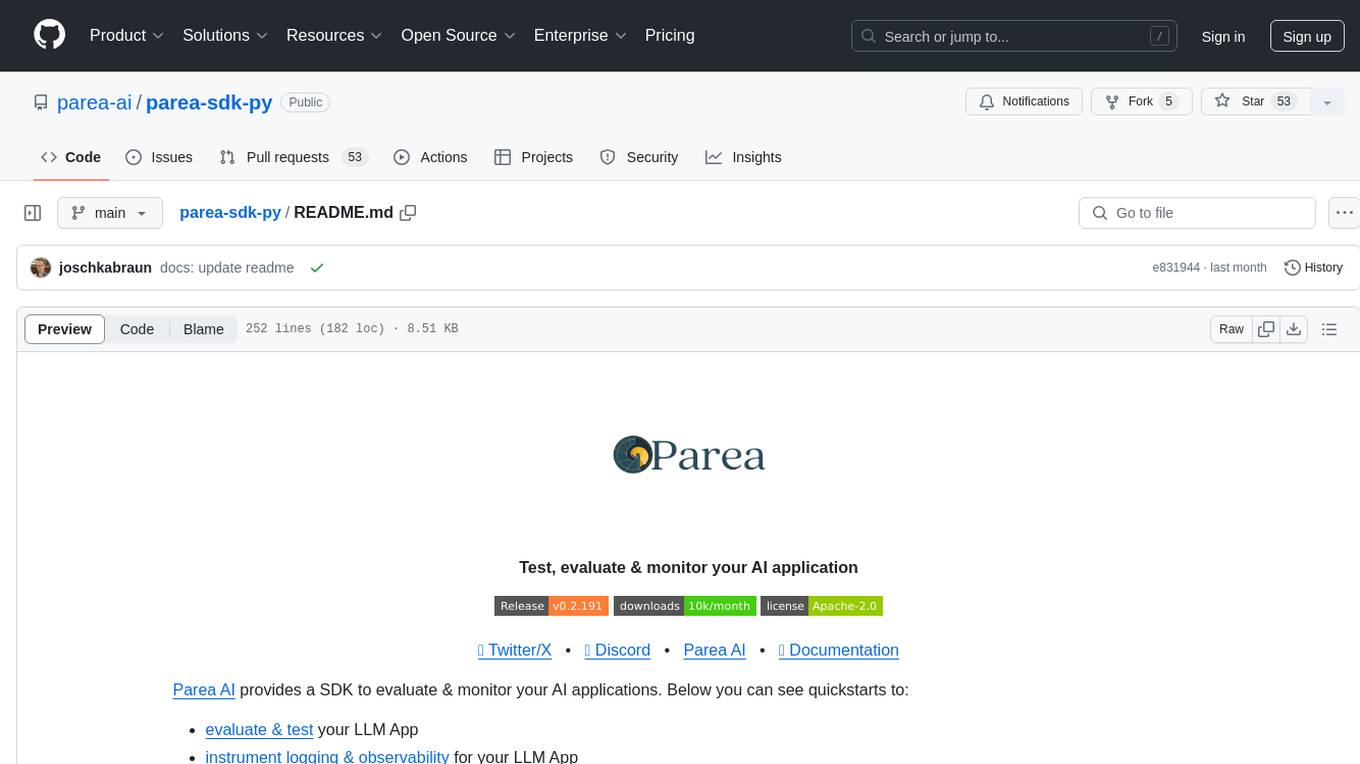
parea-sdk-py
Parea AI provides a SDK to evaluate & monitor AI applications. It allows users to test, evaluate, and monitor their AI models by defining and running experiments. The SDK also enables logging and observability for AI applications, as well as deploying prompts to facilitate collaboration between engineers and subject-matter experts. Users can automatically log calls to OpenAI and Anthropic, create hierarchical traces of their applications, and deploy prompts for integration into their applications.
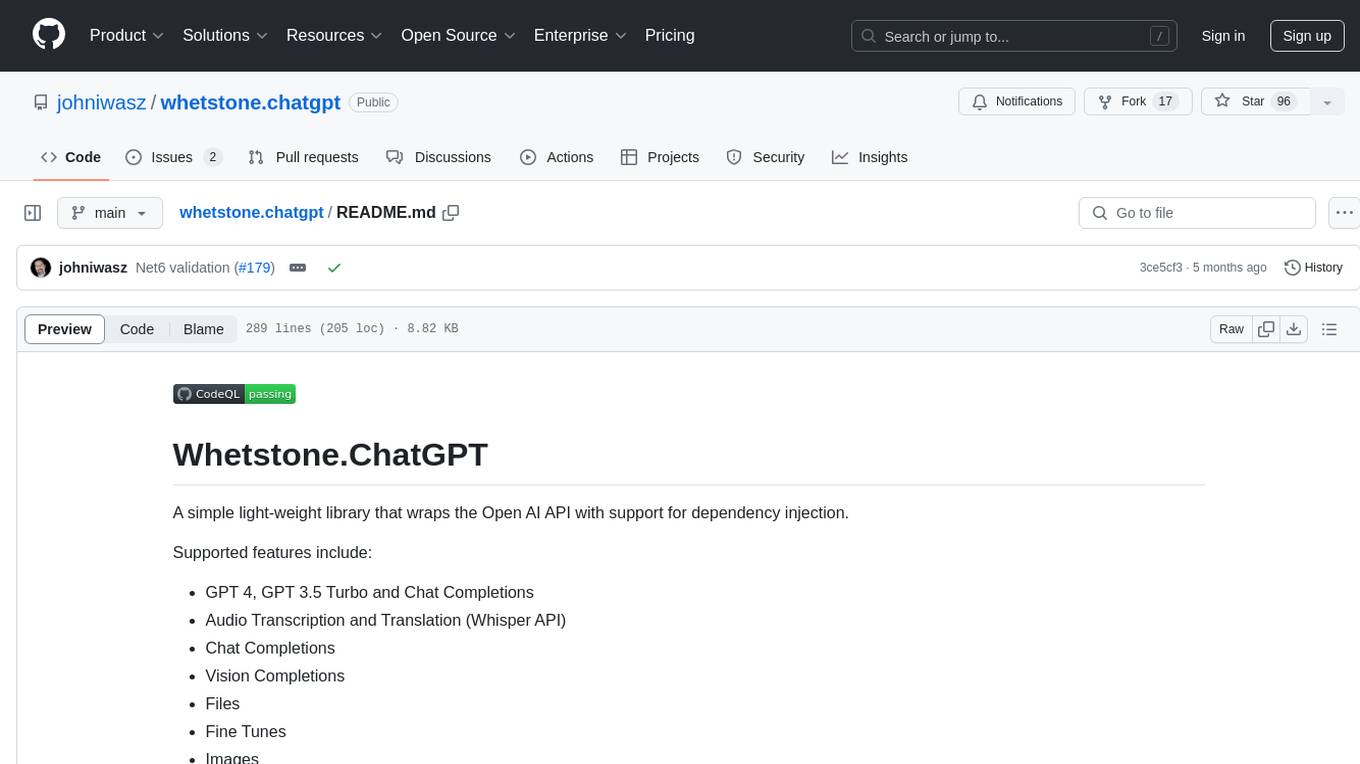
whetstone.chatgpt
Whetstone.ChatGPT is a simple light-weight library that wraps the Open AI API with support for dependency injection. It supports features like GPT 4, GPT 3.5 Turbo, chat completions, audio transcription and translation, vision completions, files, fine tunes, images, embeddings, moderations, and response streaming. The library provides a video walkthrough of a Blazor web app built on it and includes examples such as a command line bot. It offers quickstarts for dependency injection, chat completions, completions, file handling, fine tuning, image generation, and audio transcription.
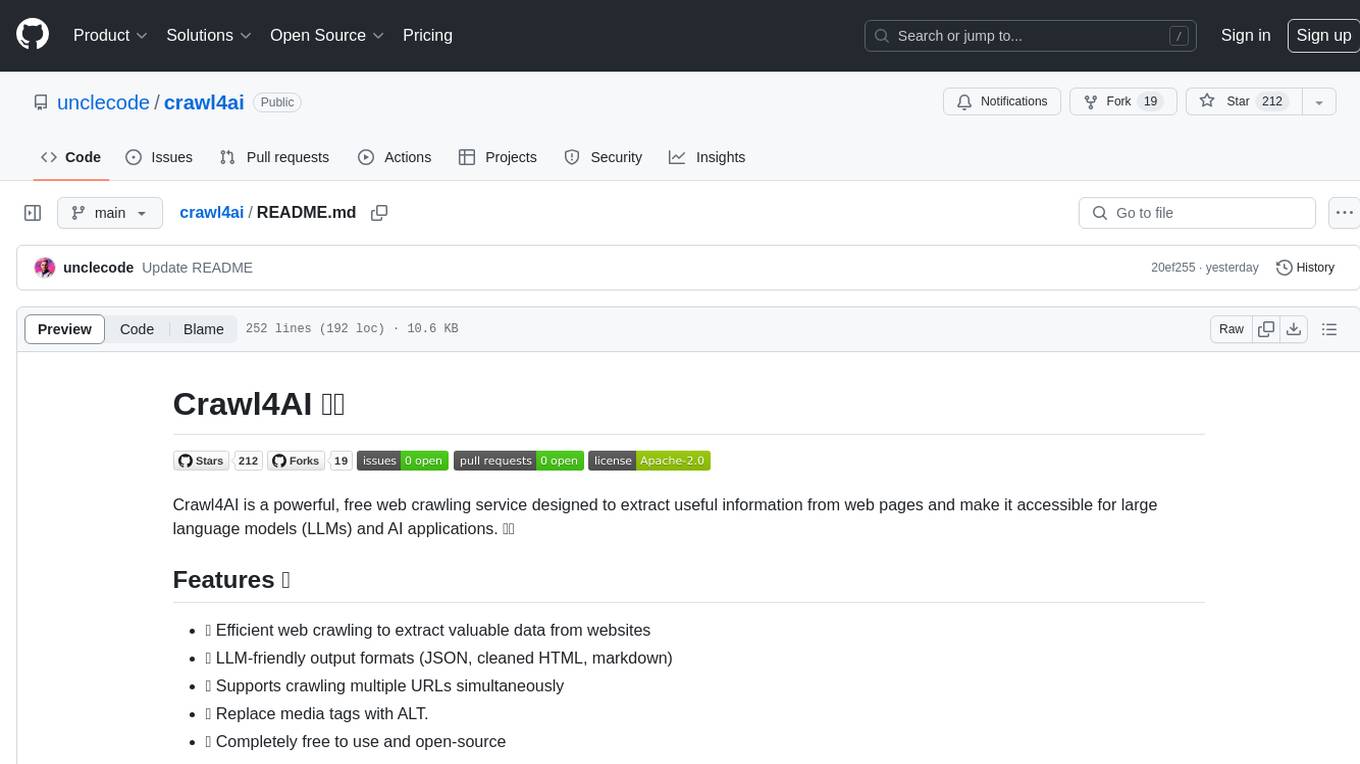
crawl4ai
Crawl4AI is a powerful and free web crawling service that extracts valuable data from websites and provides LLM-friendly output formats. It supports crawling multiple URLs simultaneously, replaces media tags with ALT, and is completely free to use and open-source. Users can integrate Crawl4AI into Python projects as a library or run it as a standalone local server. The tool allows users to crawl and extract data from specified URLs using different providers and models, with options to include raw HTML content, force fresh crawls, and extract meaningful text blocks. Configuration settings can be adjusted in the `crawler/config.py` file to customize providers, API keys, chunk processing, and word thresholds. Contributions to Crawl4AI are welcome from the open-source community to enhance its value for AI enthusiasts and developers.
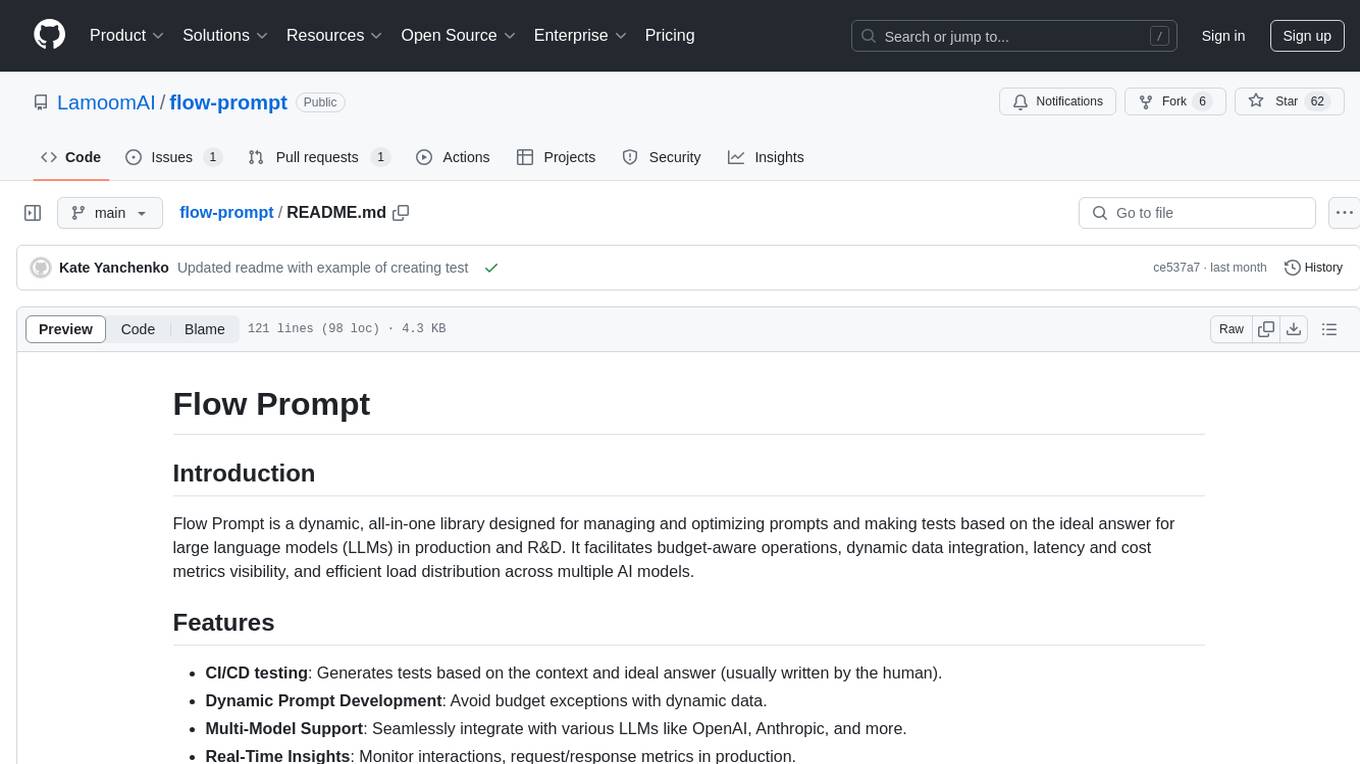
flow-prompt
Flow Prompt is a dynamic library for managing and optimizing prompts for large language models. It facilitates budget-aware operations, dynamic data integration, and efficient load distribution. Features include CI/CD testing, dynamic prompt development, multi-model support, real-time insights, and prompt testing and evolution.
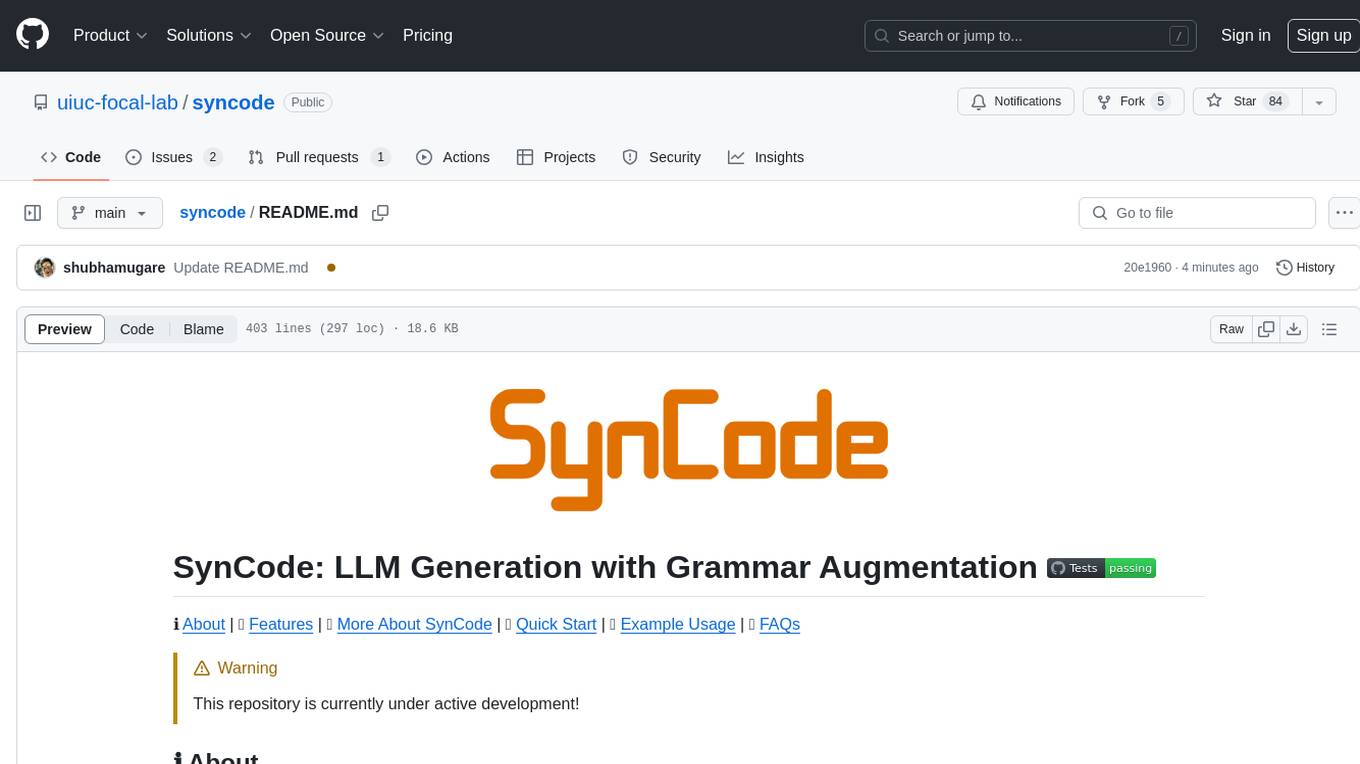
syncode
SynCode is a novel framework for the grammar-guided generation of Large Language Models (LLMs) that ensures syntactically valid output with respect to defined Context-Free Grammar (CFG) rules. It supports general-purpose programming languages like Python, Go, SQL, JSON, and more, allowing users to define custom grammars using EBNF syntax. The tool compares favorably to other constrained decoders and offers features like fast grammar-guided generation, compatibility with HuggingFace Language Models, and the ability to work with various decoding strategies.
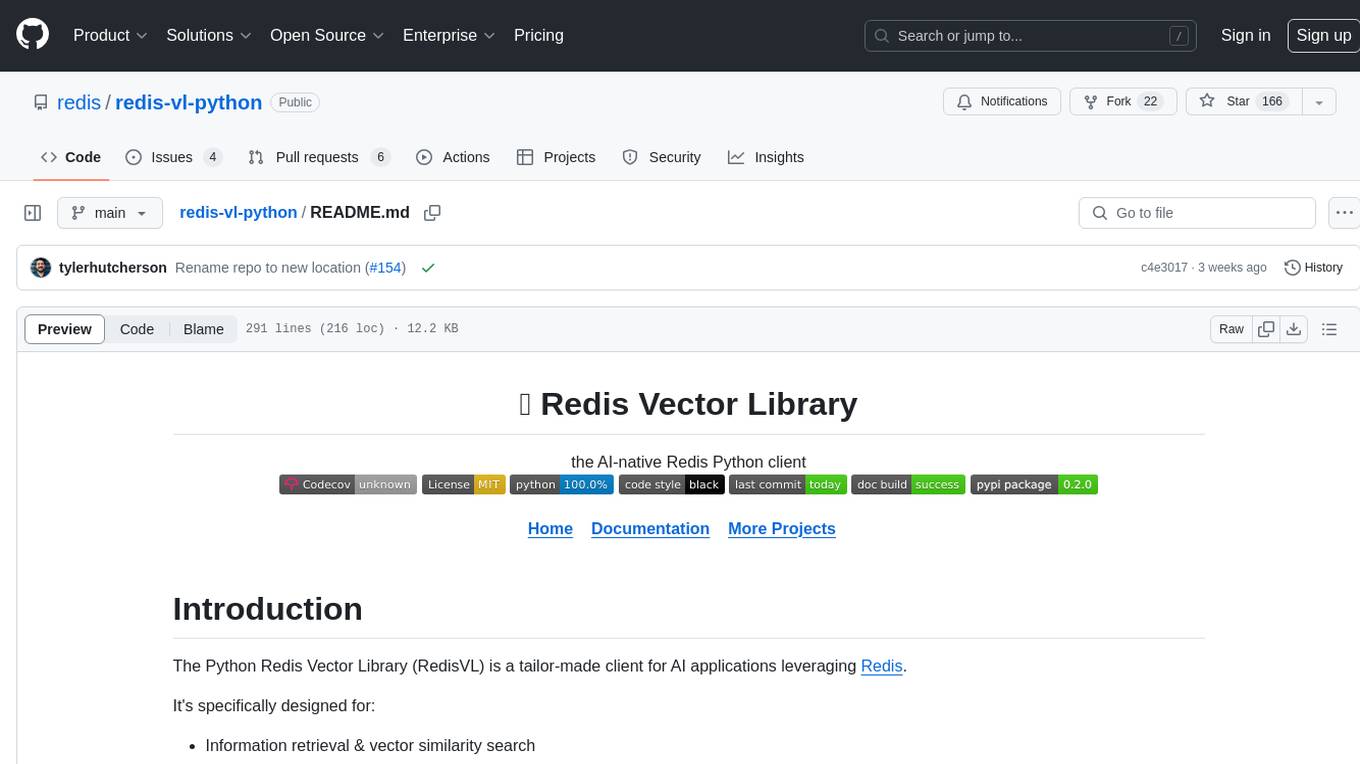
redis-vl-python
The Python Redis Vector Library (RedisVL) is a tailor-made client for AI applications leveraging Redis. It enhances applications with Redis' speed, flexibility, and reliability, incorporating capabilities like vector-based semantic search, full-text search, and geo-spatial search. The library bridges the gap between the emerging AI-native developer ecosystem and the capabilities of Redis by providing a lightweight, elegant, and intuitive interface. It abstracts the features of Redis into a grammar that is more aligned to the needs of today's AI/ML Engineers or Data Scientists.
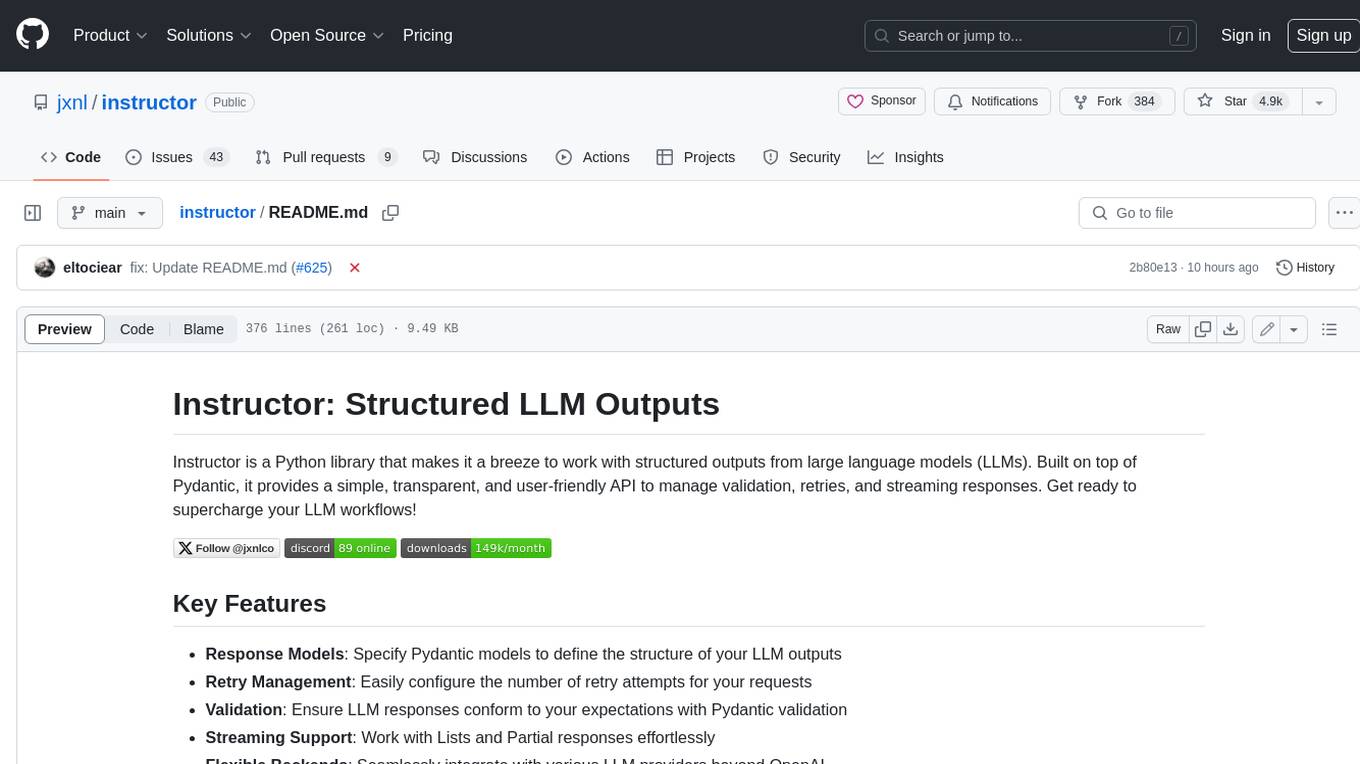
instructor
Instructor is a Python library that makes it a breeze to work with structured outputs from large language models (LLMs). Built on top of Pydantic, it provides a simple, transparent, and user-friendly API to manage validation, retries, and streaming responses. Get ready to supercharge your LLM workflows!
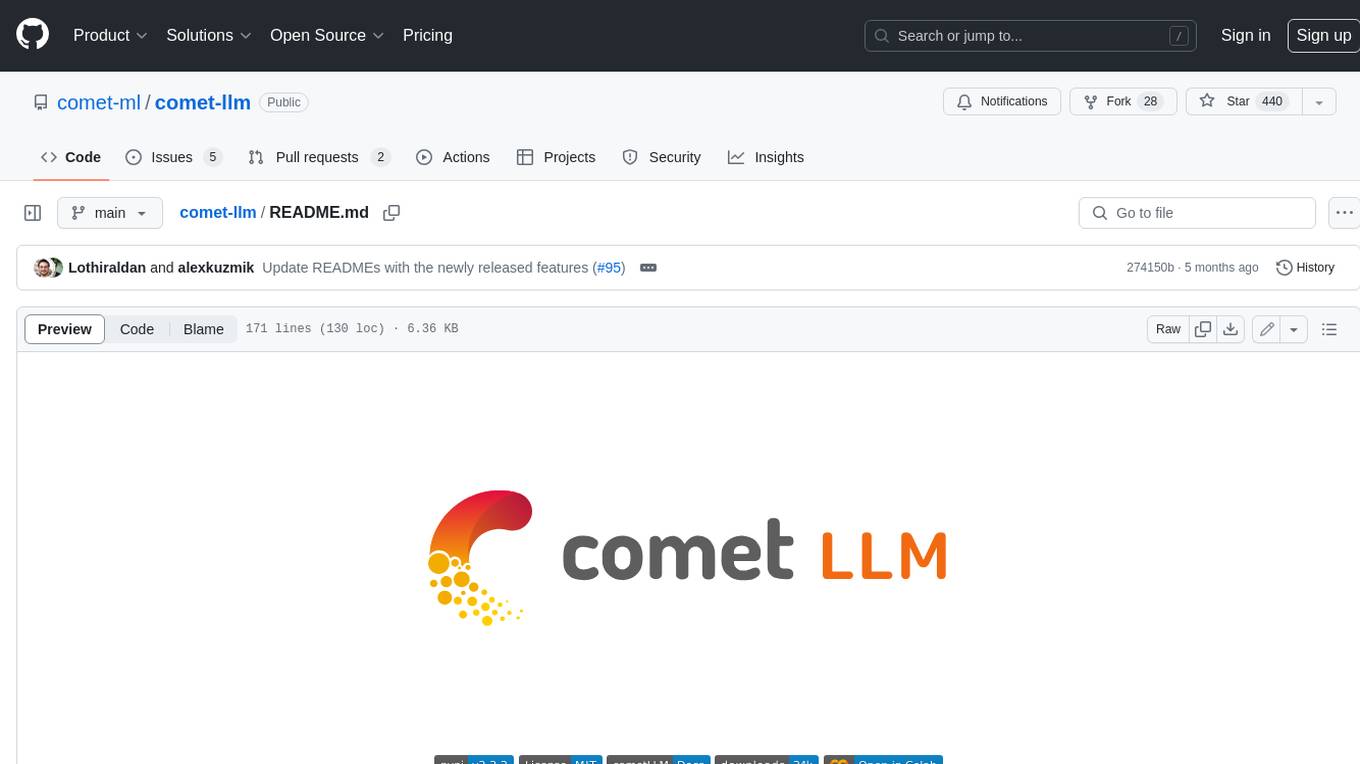
comet-llm
CometLLM is a tool to log and visualize your LLM prompts and chains. Use CometLLM to identify effective prompt strategies, streamline your troubleshooting, and ensure reproducible workflows!
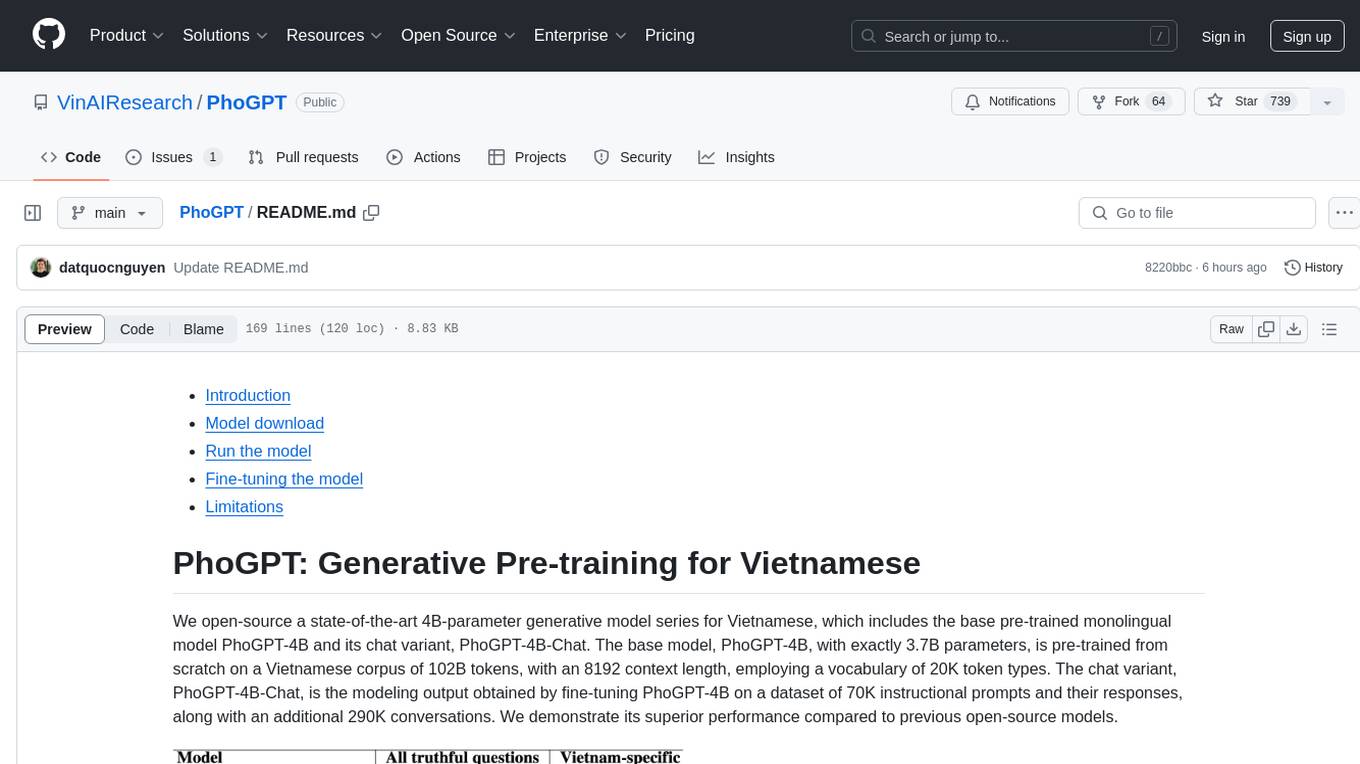
PhoGPT
PhoGPT is an open-source 4B-parameter generative model series for Vietnamese, including the base pre-trained monolingual model PhoGPT-4B and its chat variant, PhoGPT-4B-Chat. PhoGPT-4B is pre-trained from scratch on a Vietnamese corpus of 102B tokens, with an 8192 context length and a vocabulary of 20K token types. PhoGPT-4B-Chat is fine-tuned on instructional prompts and conversations, demonstrating superior performance. Users can run the model with inference engines like vLLM and Text Generation Inference, and fine-tune it using llm-foundry. However, PhoGPT has limitations in reasoning, coding, and mathematics tasks, and may generate harmful or biased responses.
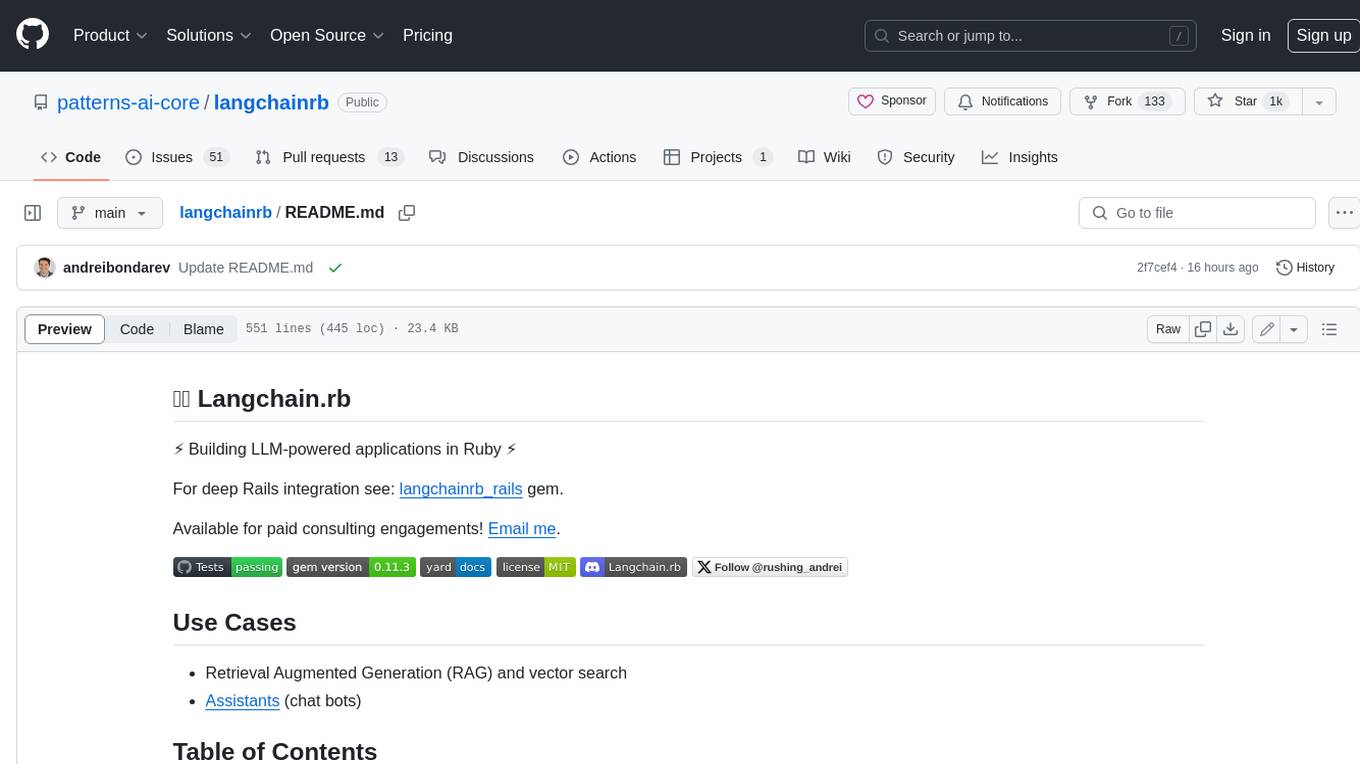
langchainrb
Langchain.rb is a Ruby library that makes it easy to build LLM-powered applications. It provides a unified interface to a variety of LLMs, vector search databases, and other tools, making it easy to build and deploy RAG (Retrieval Augmented Generation) systems and assistants. Langchain.rb is open source and available under the MIT License.
For similar tasks
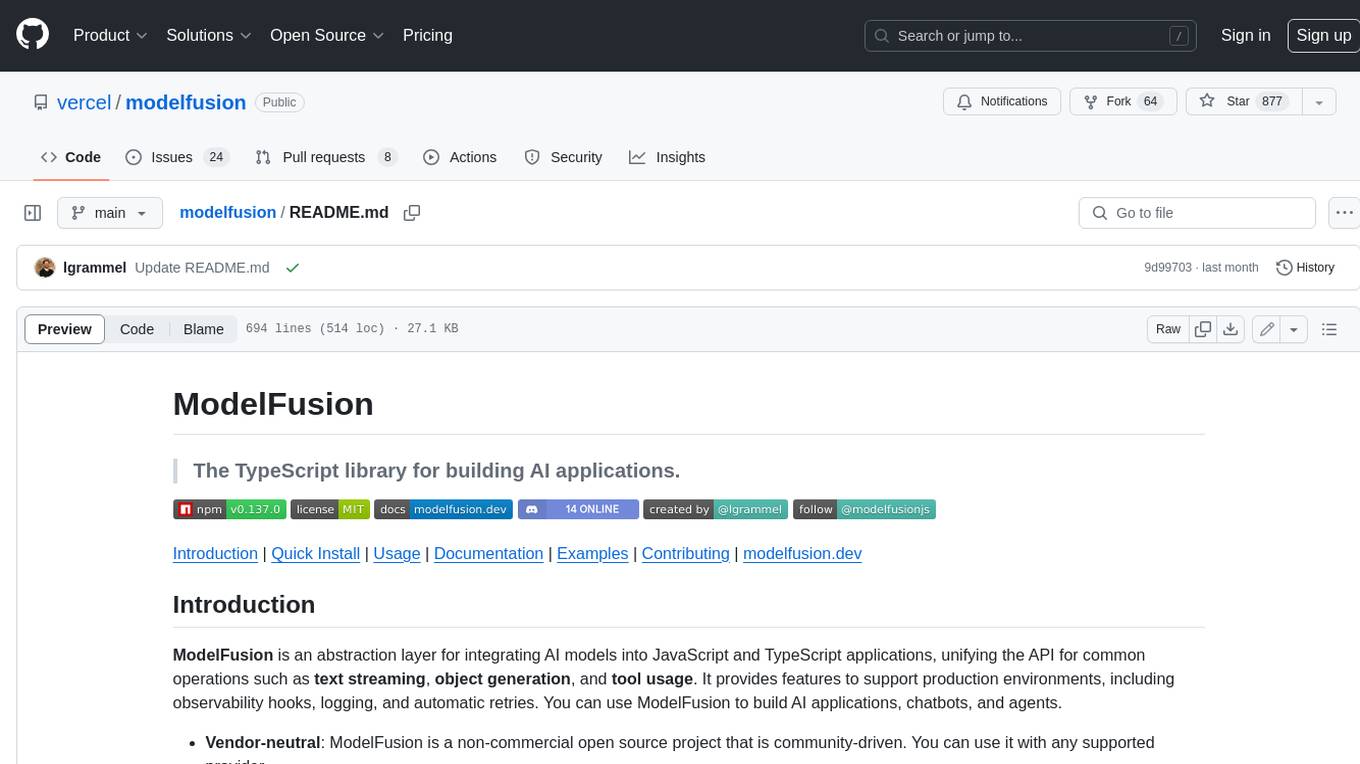
modelfusion
ModelFusion is an abstraction layer for integrating AI models into JavaScript and TypeScript applications, unifying the API for common operations such as text streaming, object generation, and tool usage. It provides features to support production environments, including observability hooks, logging, and automatic retries. You can use ModelFusion to build AI applications, chatbots, and agents. ModelFusion is a non-commercial open source project that is community-driven. You can use it with any supported provider. ModelFusion supports a wide range of models including text generation, image generation, vision, text-to-speech, speech-to-text, and embedding models. ModelFusion infers TypeScript types wherever possible and validates model responses. ModelFusion provides an observer framework and logging support. ModelFusion ensures seamless operation through automatic retries, throttling, and error handling mechanisms. ModelFusion is fully tree-shakeable, can be used in serverless environments, and only uses a minimal set of dependencies.
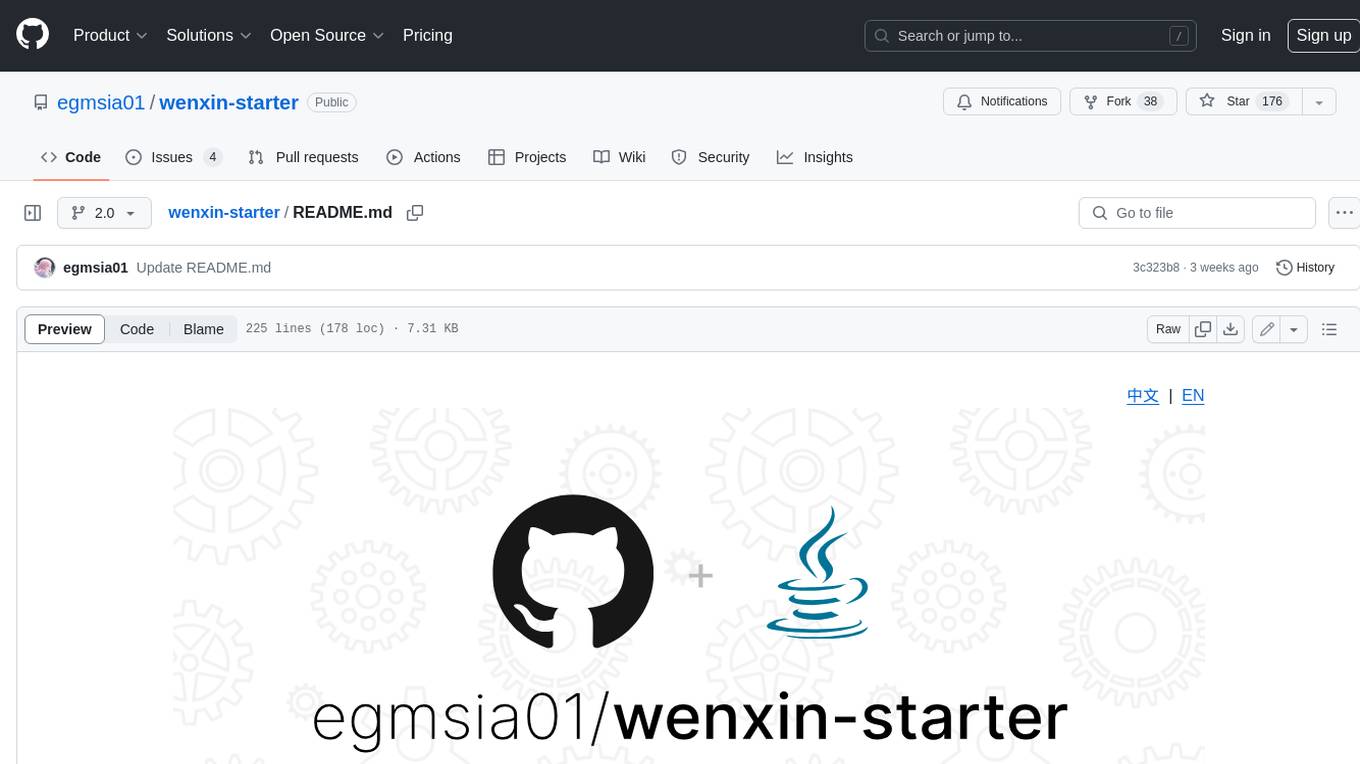
wenxin-starter
WenXin-Starter is a spring-boot-starter for Baidu's "Wenxin Qianfan WENXINWORKSHOP" large model, which can help you quickly access Baidu's AI capabilities. It fully integrates the official API documentation of Wenxin Qianfan. Supports text-to-image generation, built-in dialogue memory, and supports streaming return of dialogue. Supports QPS control of a single model and supports queuing mechanism. Plugins will be added soon.
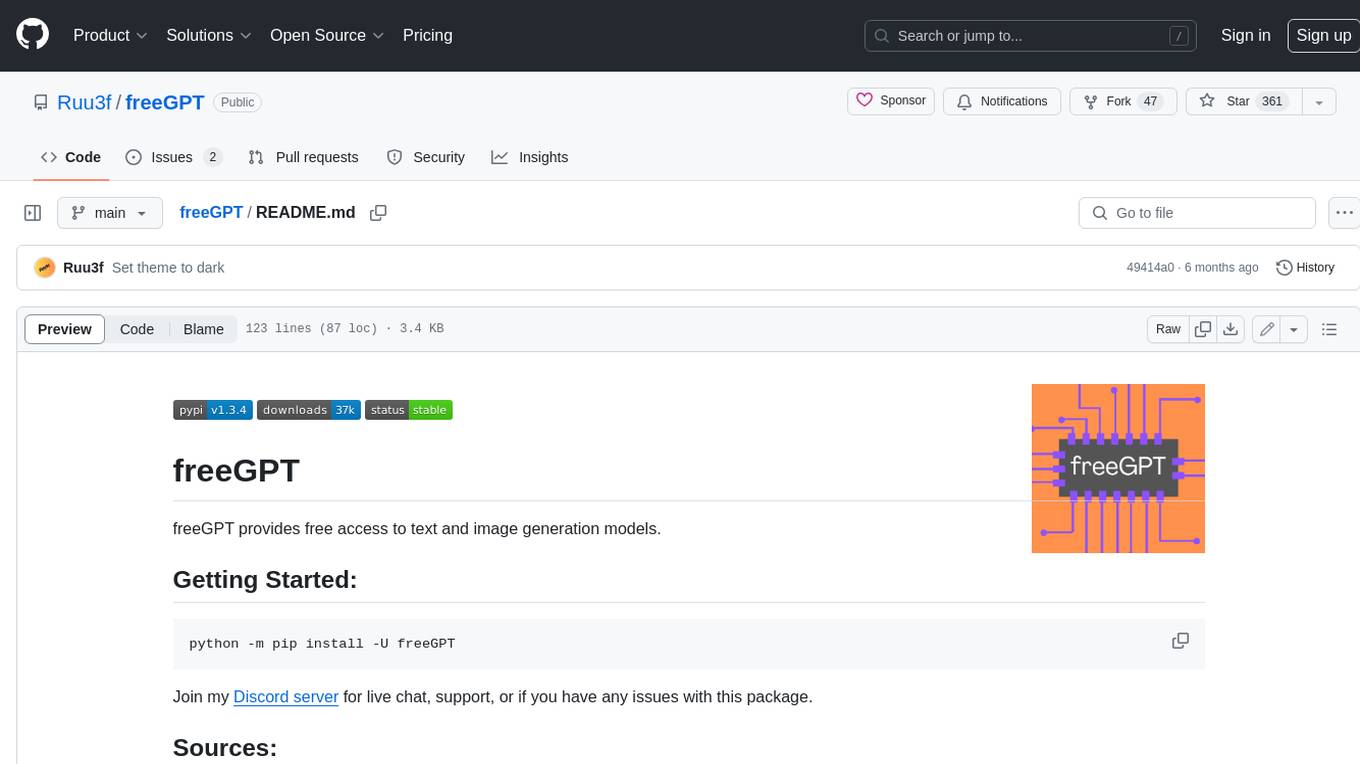
freeGPT
freeGPT provides free access to text and image generation models. It supports various models, including gpt3, gpt4, alpaca_7b, falcon_40b, prodia, and pollinations. The tool offers both asynchronous and non-asynchronous interfaces for text completion and image generation. It also features an interactive Discord bot that provides access to all the models in the repository. The tool is easy to use and can be integrated into various applications.
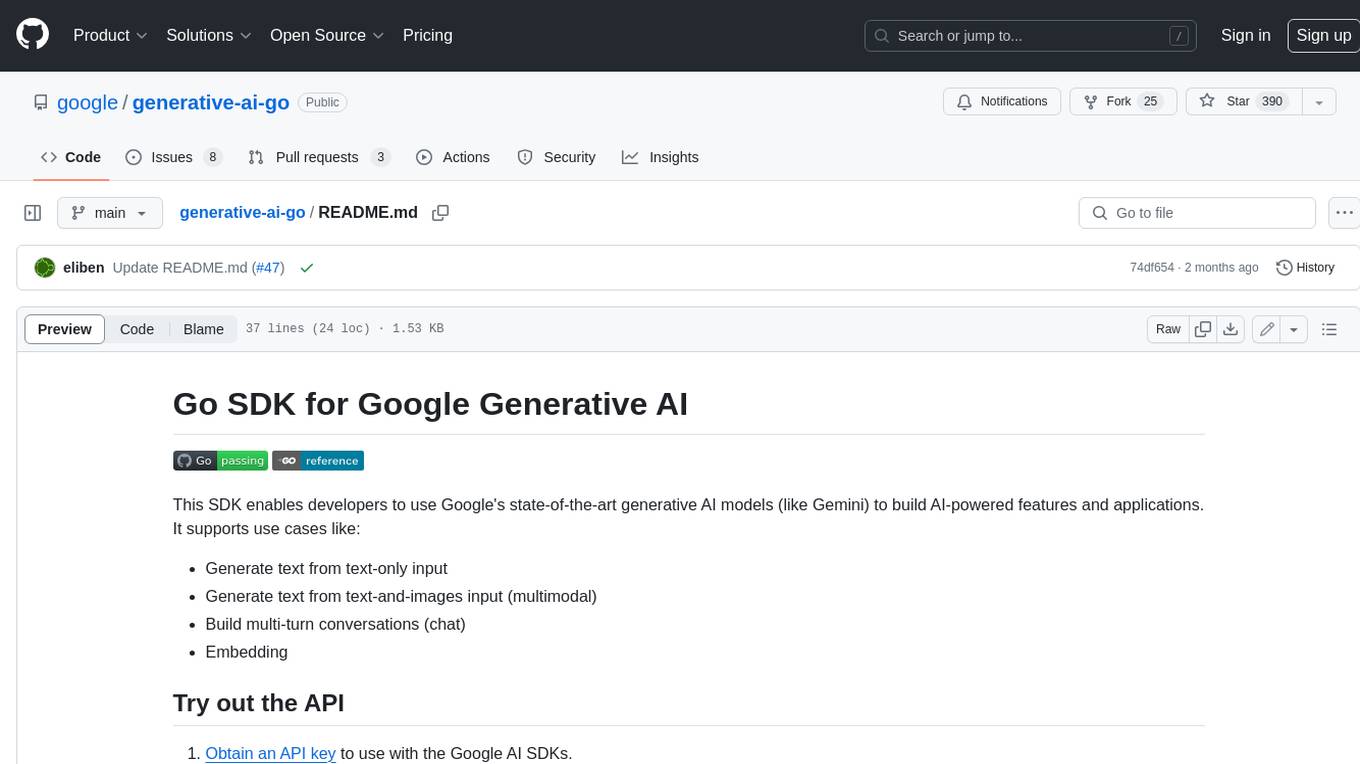
generative-ai-go
The Google AI Go SDK enables developers to use Google's state-of-the-art generative AI models (like Gemini) to build AI-powered features and applications. It supports use cases like generating text from text-only input, generating text from text-and-images input (multimodal), building multi-turn conversations (chat), and embedding.
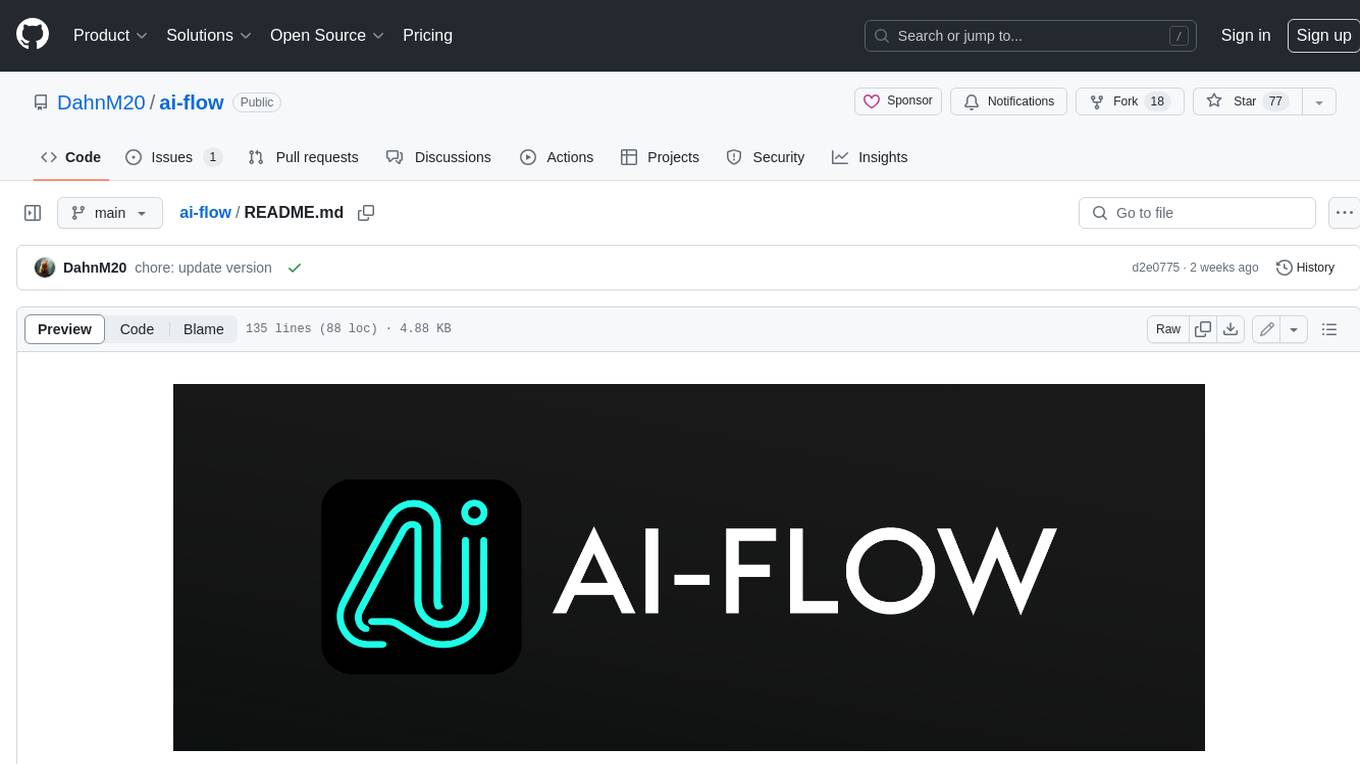
ai-flow
AI Flow is an open-source, user-friendly UI application that empowers you to seamlessly connect multiple AI models together, specifically leveraging the capabilities of multiples AI APIs such as OpenAI, StabilityAI and Replicate. In a nutshell, AI Flow provides a visual platform for crafting and managing AI-driven workflows, thereby facilitating diverse and dynamic AI interactions.
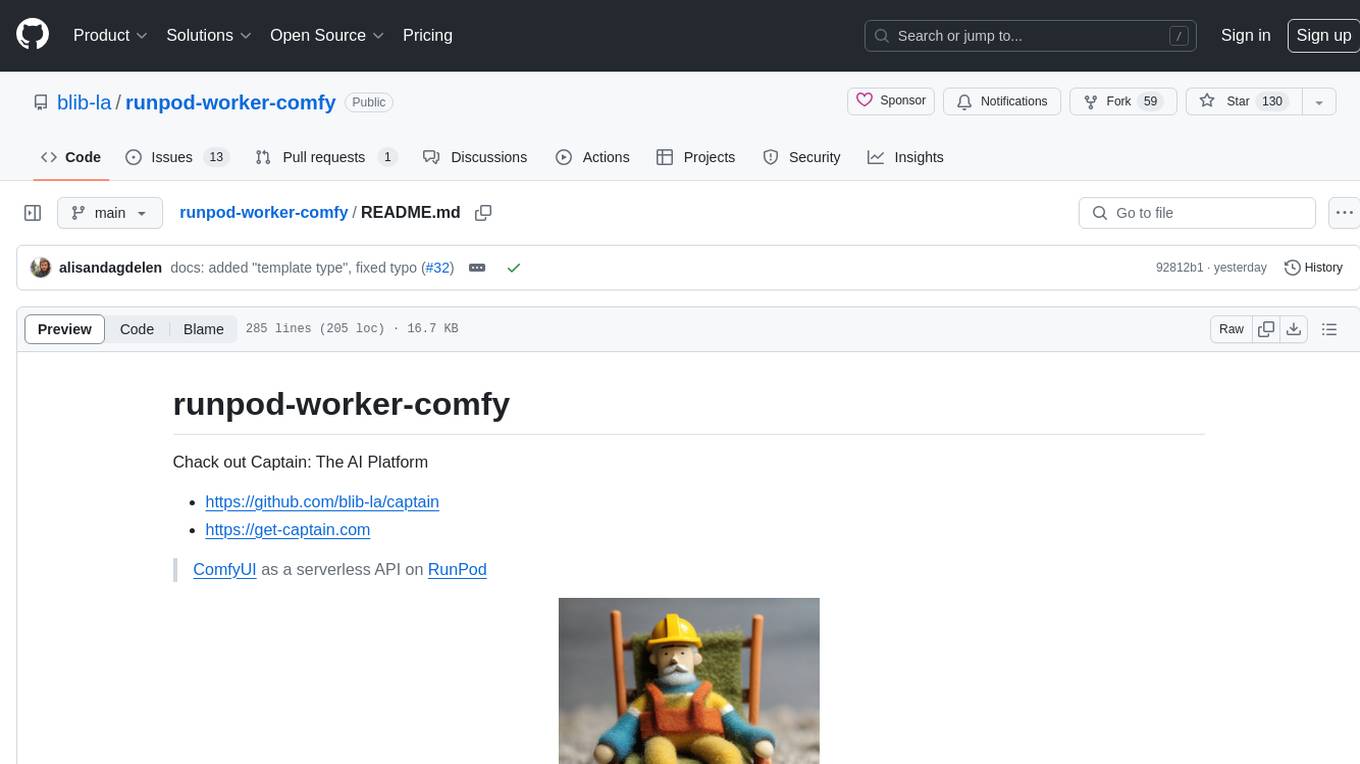
runpod-worker-comfy
runpod-worker-comfy is a serverless API tool that allows users to run any ComfyUI workflow to generate an image. Users can provide input images as base64-encoded strings, and the generated image can be returned as a base64-encoded string or uploaded to AWS S3. The tool is built on Ubuntu + NVIDIA CUDA and provides features like built-in checkpoints and VAE models. Users can configure environment variables to upload images to AWS S3 and interact with the RunPod API to generate images. The tool also supports local testing and deployment to Docker hub using Github Actions.
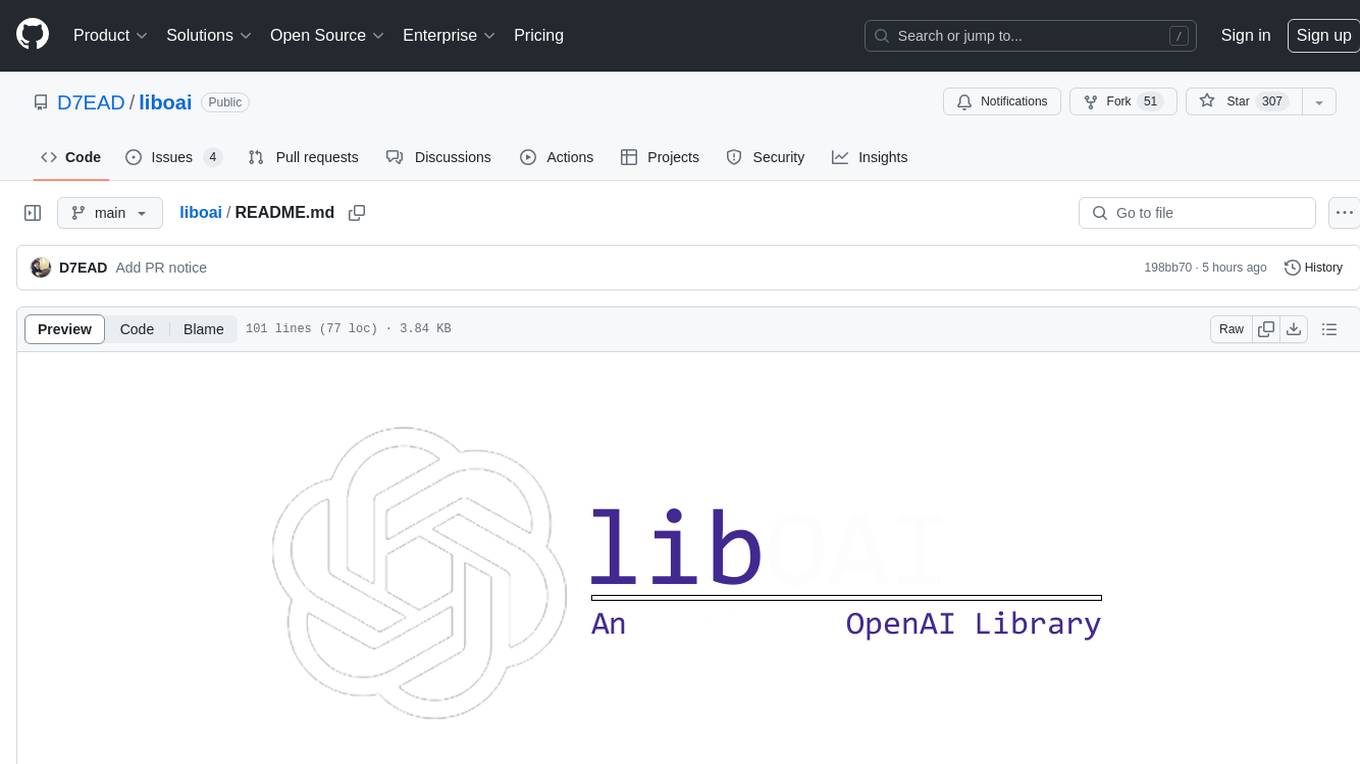
liboai
liboai is a simple C++17 library for the OpenAI API, providing developers with access to OpenAI endpoints through a collection of methods and classes. It serves as a spiritual port of OpenAI's Python library, 'openai', with similar structure and features. The library supports various functionalities such as ChatGPT, Audio, Azure, Functions, Image DALL·E, Models, Completions, Edit, Embeddings, Files, Fine-tunes, Moderation, and Asynchronous Support. Users can easily integrate the library into their C++ projects to interact with OpenAI services.
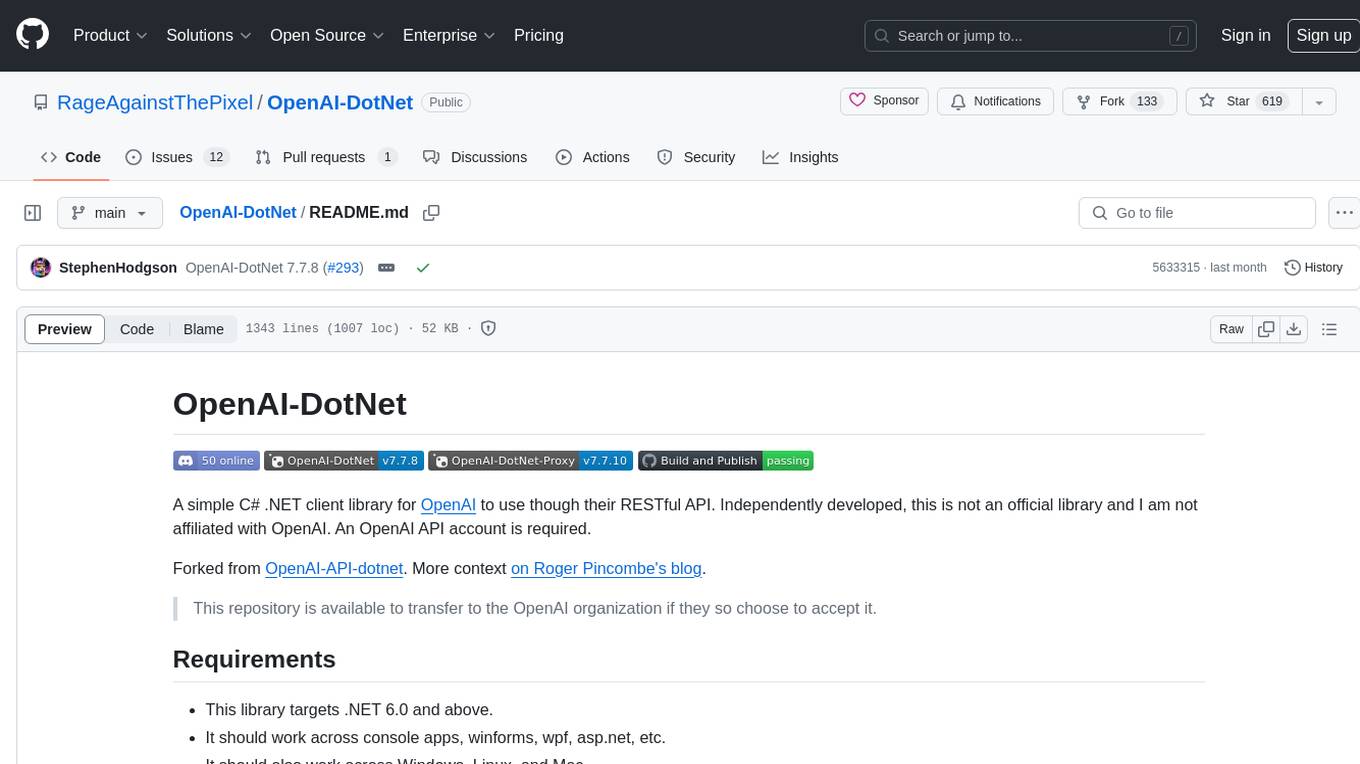
OpenAI-DotNet
OpenAI-DotNet is a simple C# .NET client library for OpenAI to use through their RESTful API. It is independently developed and not an official library affiliated with OpenAI. Users need an OpenAI API account to utilize this library. The library targets .NET 6.0 and above, working across various platforms like console apps, winforms, wpf, asp.net, etc., and on Windows, Linux, and Mac. It provides functionalities for authentication, interacting with models, assistants, threads, chat, audio, images, files, fine-tuning, embeddings, and moderations.
For similar jobs
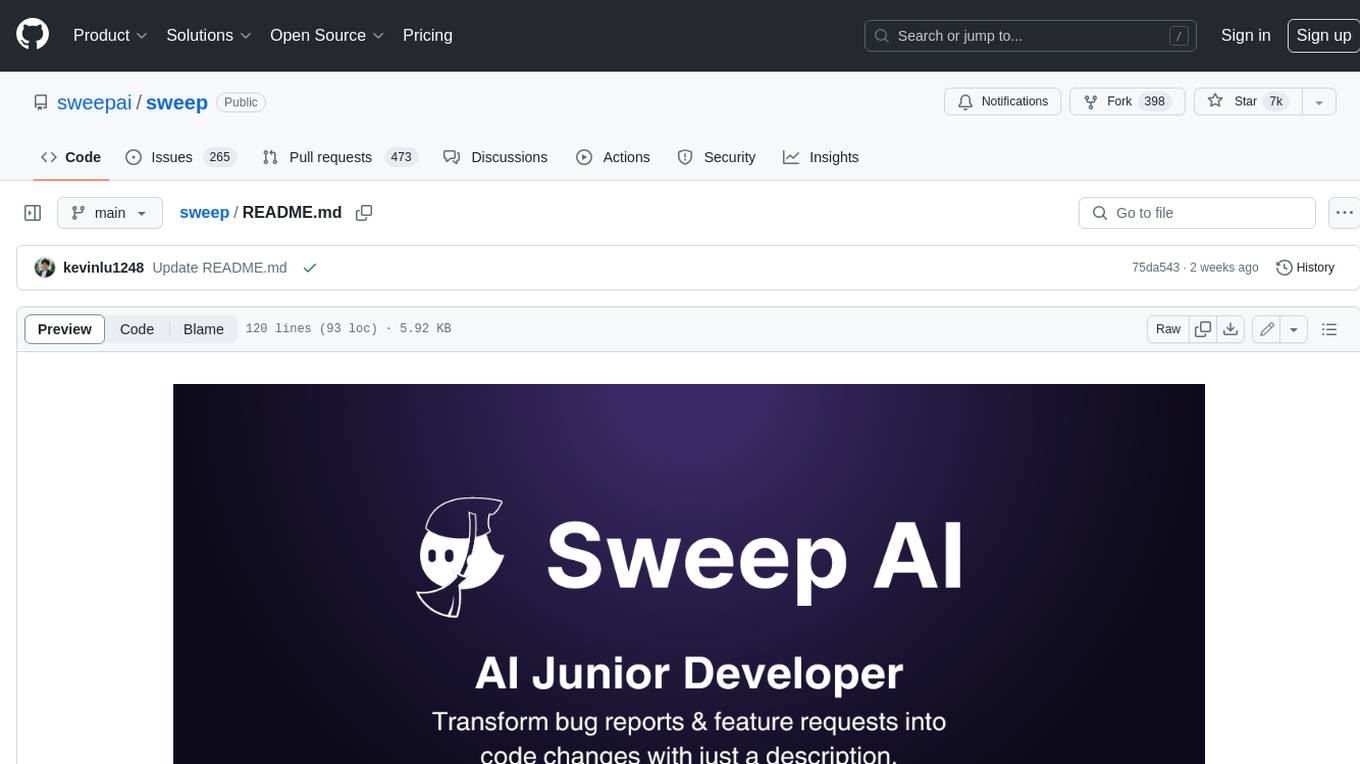
sweep
Sweep is an AI junior developer that turns bugs and feature requests into code changes. It automatically handles developer experience improvements like adding type hints and improving test coverage.
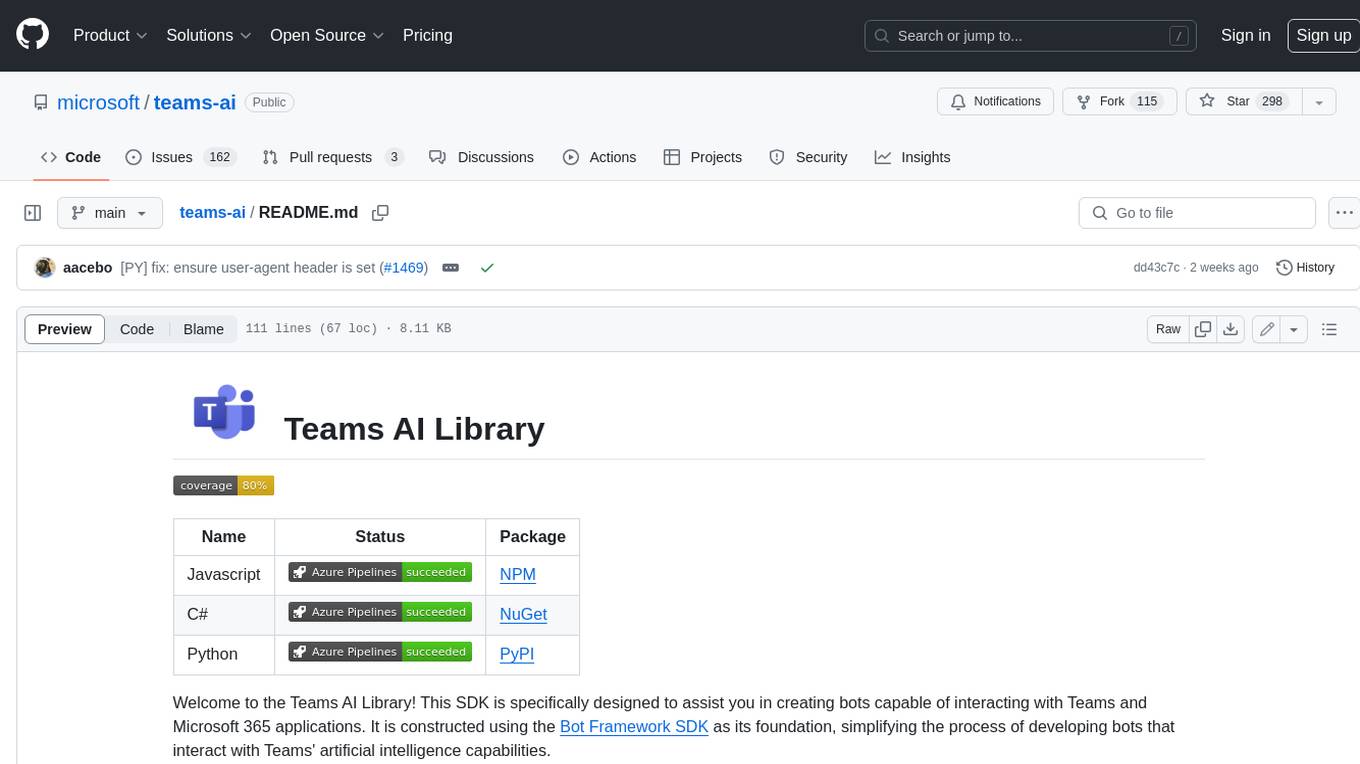
teams-ai
The Teams AI Library is a software development kit (SDK) that helps developers create bots that can interact with Teams and Microsoft 365 applications. It is built on top of the Bot Framework SDK and simplifies the process of developing bots that interact with Teams' artificial intelligence capabilities. The SDK is available for JavaScript/TypeScript, .NET, and Python.
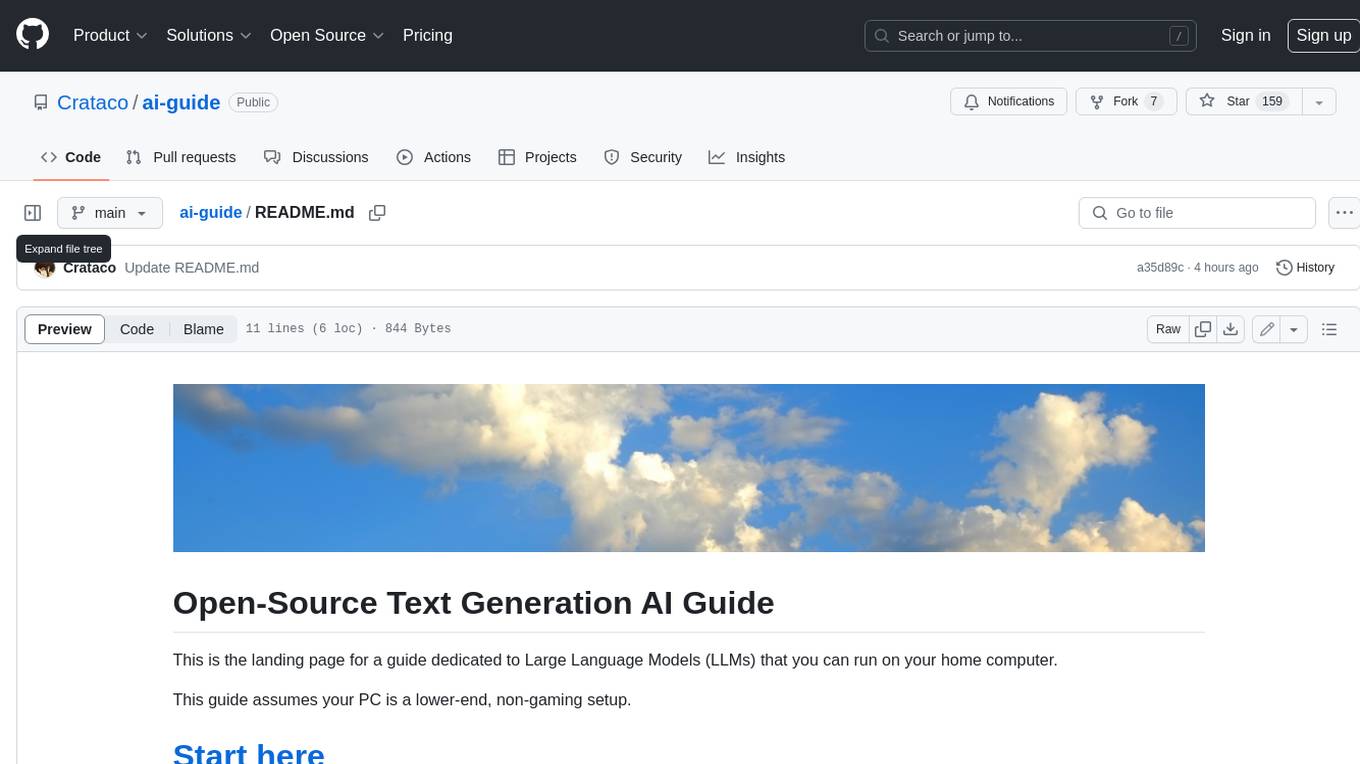
ai-guide
This guide is dedicated to Large Language Models (LLMs) that you can run on your home computer. It assumes your PC is a lower-end, non-gaming setup.
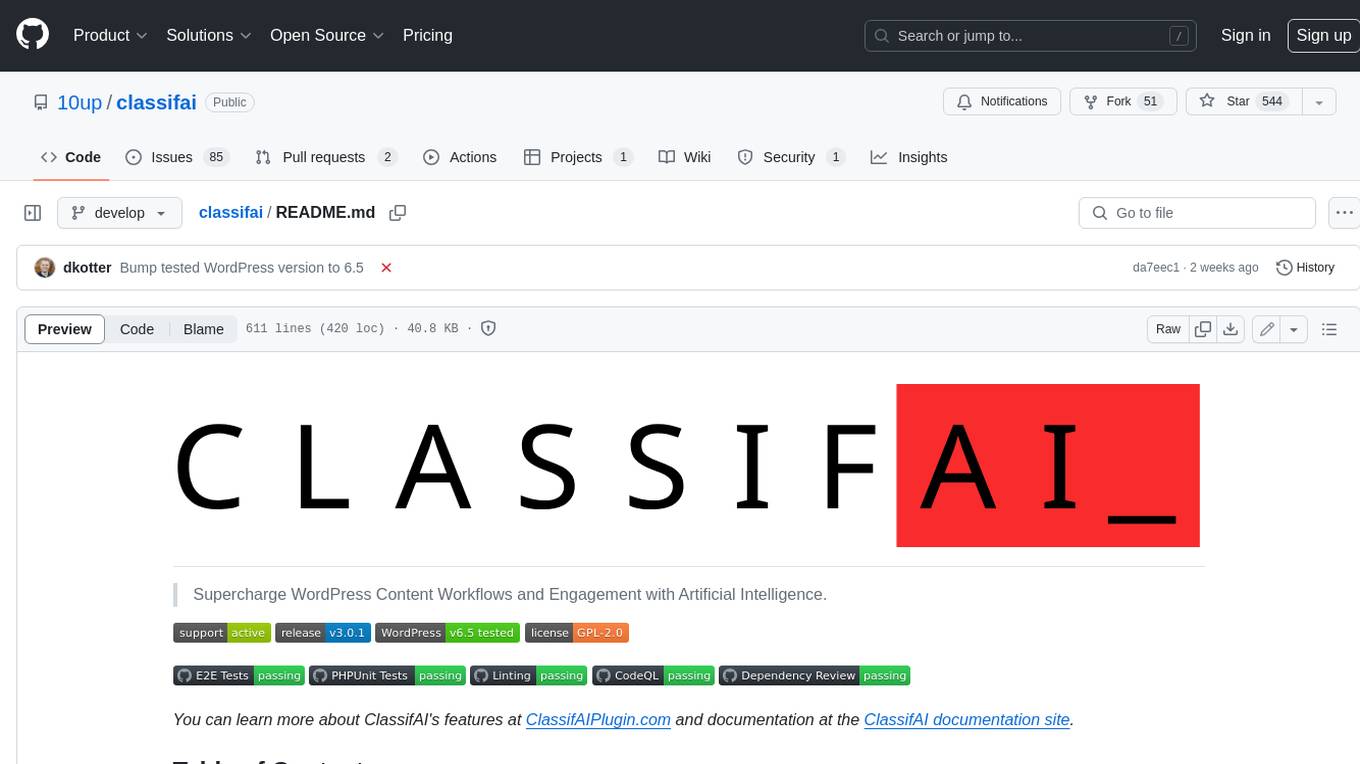
classifai
Supercharge WordPress Content Workflows and Engagement with Artificial Intelligence. Tap into leading cloud-based services like OpenAI, Microsoft Azure AI, Google Gemini and IBM Watson to augment your WordPress-powered websites. Publish content faster while improving SEO performance and increasing audience engagement. ClassifAI integrates Artificial Intelligence and Machine Learning technologies to lighten your workload and eliminate tedious tasks, giving you more time to create original content that matters.
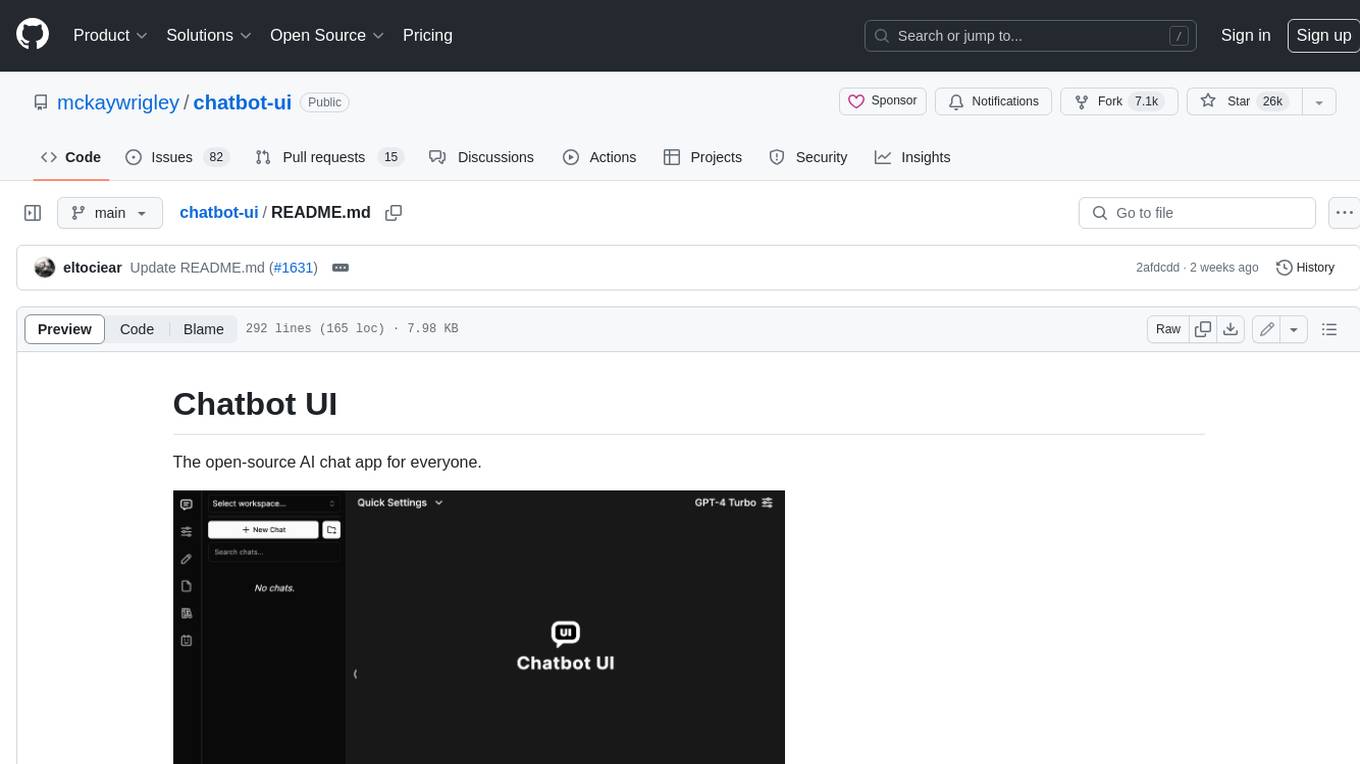
chatbot-ui
Chatbot UI is an open-source AI chat app that allows users to create and deploy their own AI chatbots. It is easy to use and can be customized to fit any need. Chatbot UI is perfect for businesses, developers, and anyone who wants to create a chatbot.
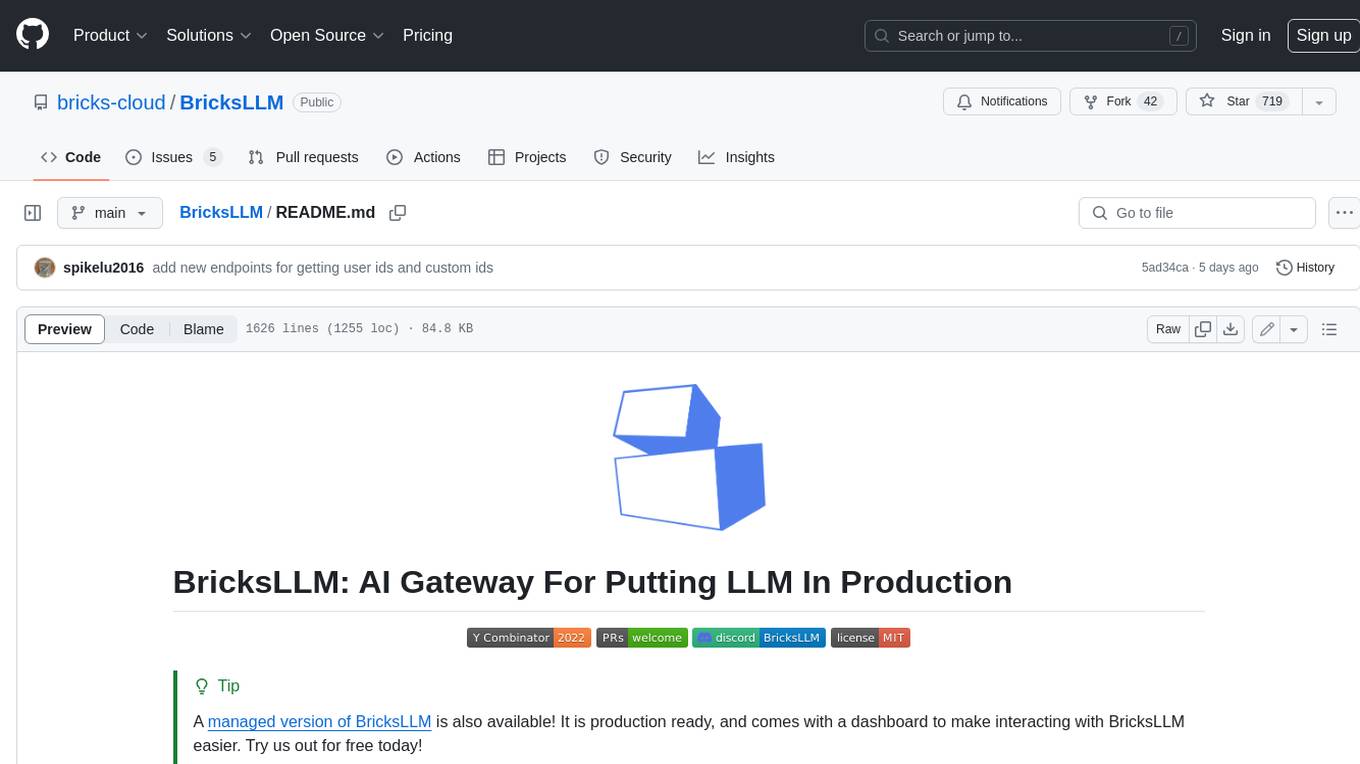
BricksLLM
BricksLLM is a cloud native AI gateway written in Go. Currently, it provides native support for OpenAI, Anthropic, Azure OpenAI and vLLM. BricksLLM aims to provide enterprise level infrastructure that can power any LLM production use cases. Here are some use cases for BricksLLM: * Set LLM usage limits for users on different pricing tiers * Track LLM usage on a per user and per organization basis * Block or redact requests containing PIIs * Improve LLM reliability with failovers, retries and caching * Distribute API keys with rate limits and cost limits for internal development/production use cases * Distribute API keys with rate limits and cost limits for students
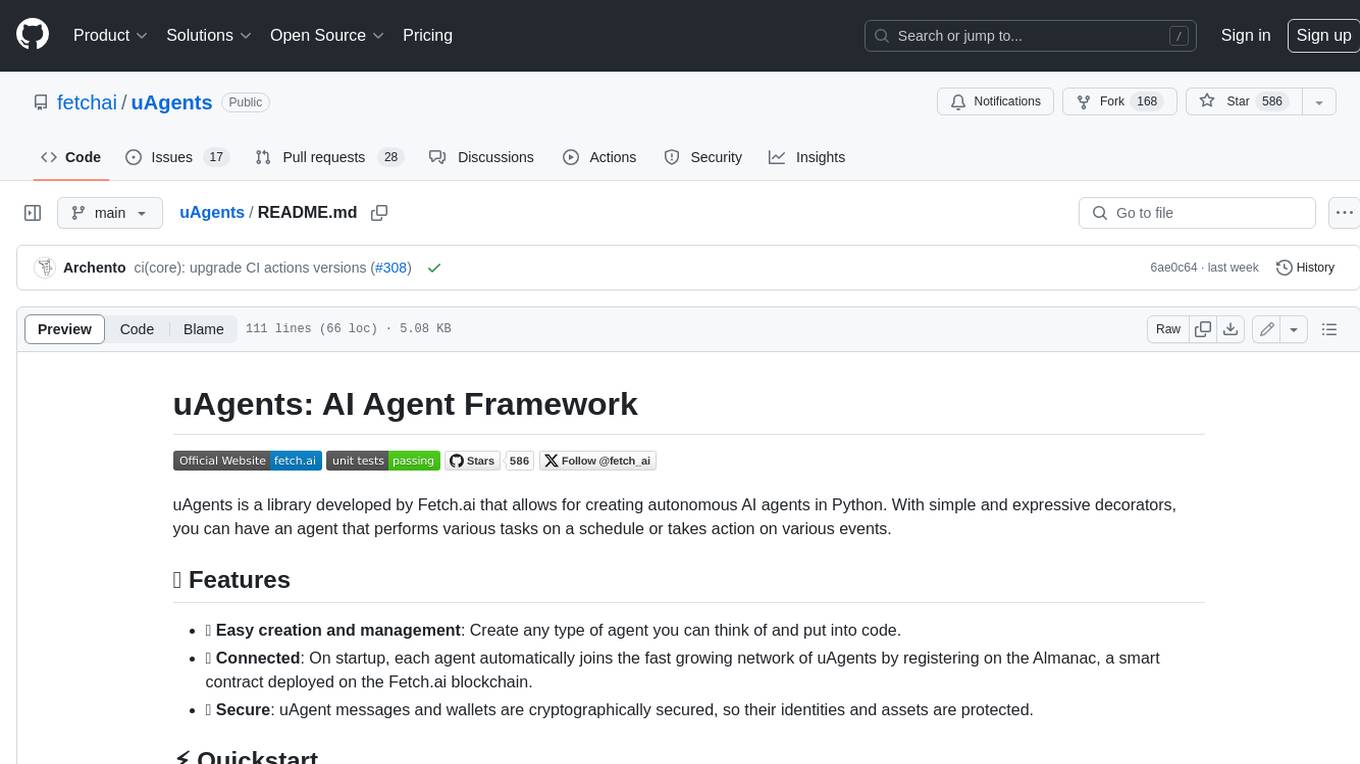
uAgents
uAgents is a Python library developed by Fetch.ai that allows for the creation of autonomous AI agents. These agents can perform various tasks on a schedule or take action on various events. uAgents are easy to create and manage, and they are connected to a fast-growing network of other uAgents. They are also secure, with cryptographically secured messages and wallets.
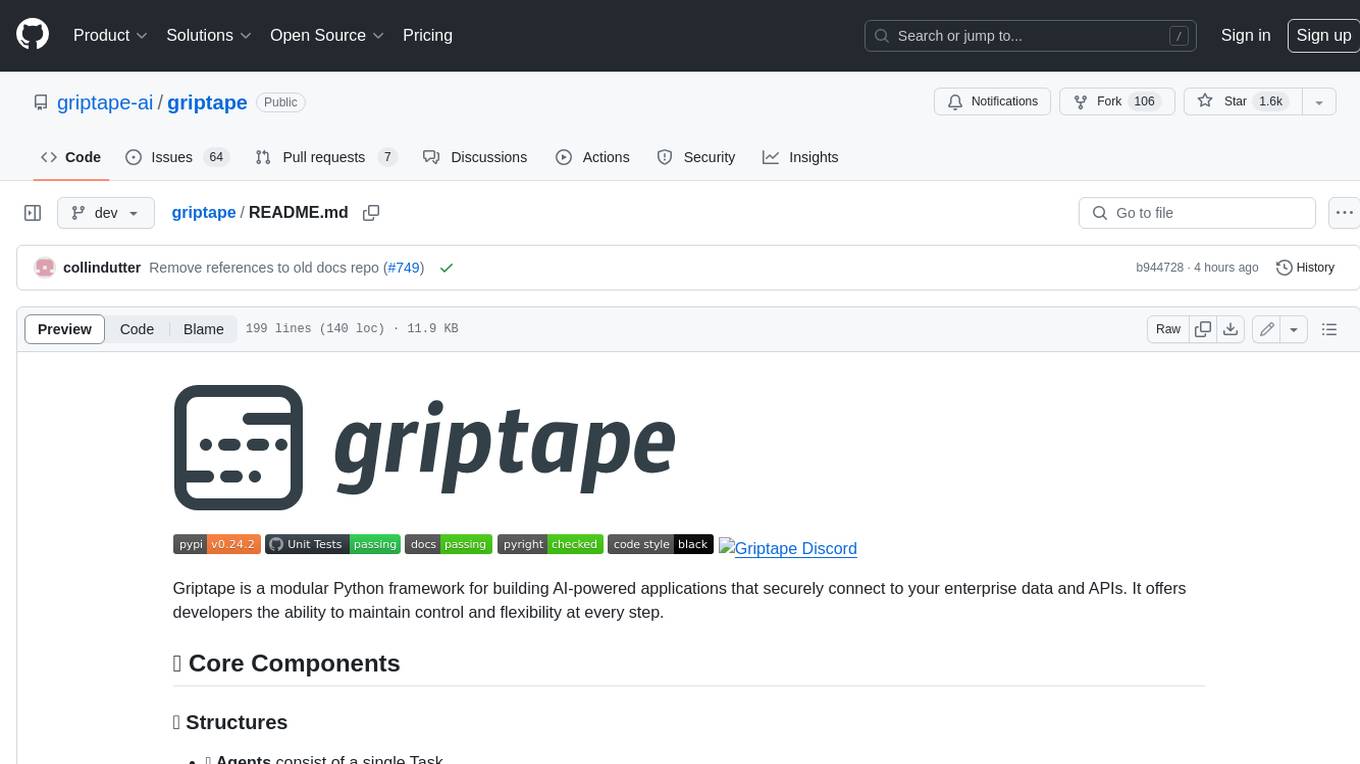
griptape
Griptape is a modular Python framework for building AI-powered applications that securely connect to your enterprise data and APIs. It offers developers the ability to maintain control and flexibility at every step. Griptape's core components include Structures (Agents, Pipelines, and Workflows), Tasks, Tools, Memory (Conversation Memory, Task Memory, and Meta Memory), Drivers (Prompt and Embedding Drivers, Vector Store Drivers, Image Generation Drivers, Image Query Drivers, SQL Drivers, Web Scraper Drivers, and Conversation Memory Drivers), Engines (Query Engines, Extraction Engines, Summary Engines, Image Generation Engines, and Image Query Engines), and additional components (Rulesets, Loaders, Artifacts, Chunkers, and Tokenizers). Griptape enables developers to create AI-powered applications with ease and efficiency.