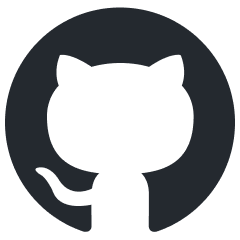
lionagi
An Intelligence Operating System
Stars: 322
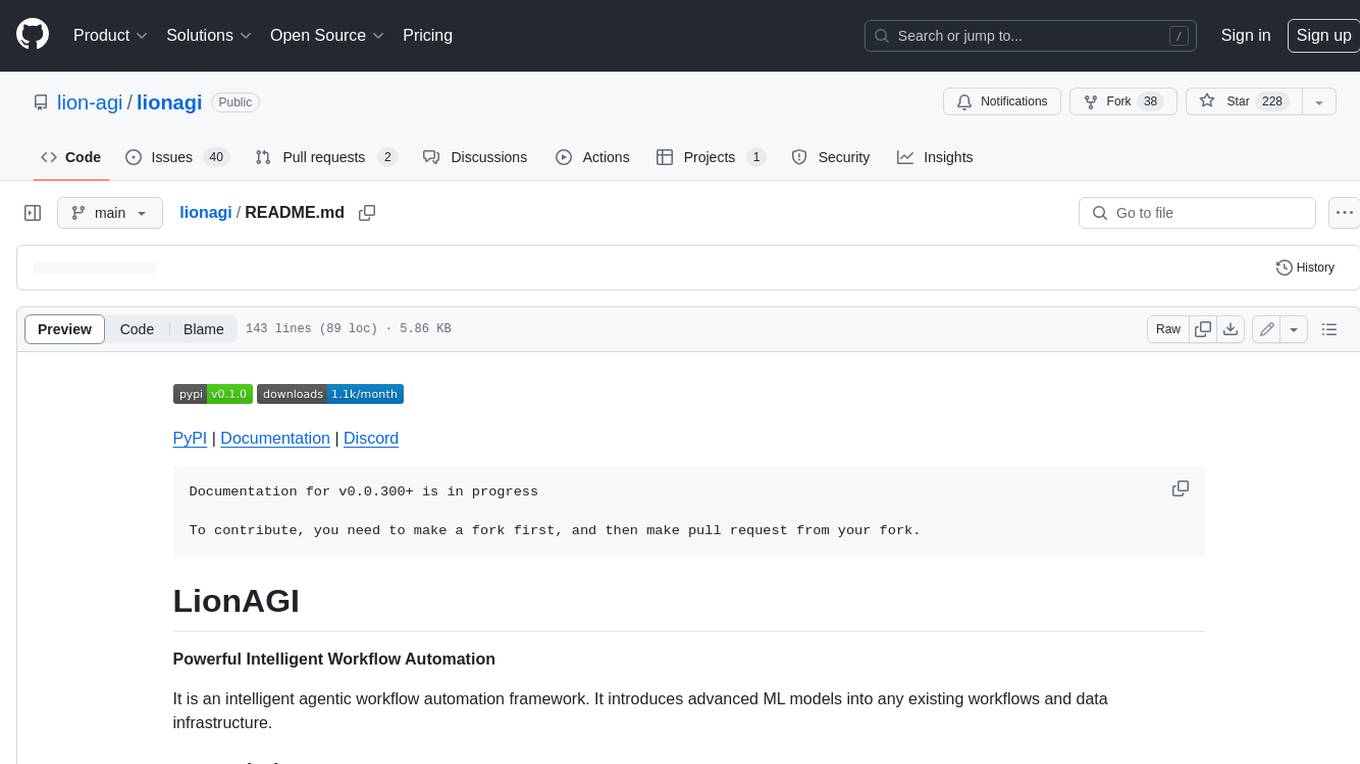
LionAGI is a powerful intelligent workflow automation framework that introduces advanced ML models into any existing workflows and data infrastructure. It can interact with almost any model, run interactions in parallel for most models, produce structured pydantic outputs with flexible usage, automate workflow via graph based agents, use advanced prompting techniques, and more. LionAGI aims to provide a centralized agent-managed framework for "ML-powered tools coordination" and to dramatically lower the barrier of entries for creating use-case/domain specific tools. It is designed to be asynchronous only and requires Python 3.10 or higher.
README:
Documentation | Discord | PyPI | Roadmap
LionAGI is a robust framework for orchestrating multi-step AI operations with precise control. Bring together multiple models, advanced ReAct reasoning, tool integrations, and custom validations in a single coherent pipeline.
- Structured: LLM interactions are validated and typed (via Pydantic).
- Expandable: Integrate multiple providers (OpenAI, Anthropic, Perplexity, custom) with minimal friction.
- Controlled: Built-in safety checks, concurrency strategies, and advanced multi-step flows—like ReAct with verbose outputs.
- Transparent: Real-time logging, message introspection, and easy debugging of tool usage.
pip install lionagi
Dependencies: • aiocahce • aiohttp • jinja2 • pandas • pillow • pydantic • python-dotenv • tiktoken
from lionagi import Branch, iModel
# Pick a model
gpt4o = iModel(provider="openai", model="gpt-4o")
# Create a Branch (conversation context)
hunter = Branch(
system="you are a hilarious dragon hunter who responds in 10 words rhymes.",
chat_model=gpt4o,
)
# Communicate asynchronously
response = await hunter.communicate("I am a dragon")
print(response)
You claim to be a dragon, oh what a braggin'!
Use Pydantic to keep outputs structured:
from pydantic import BaseModel
class Joke(BaseModel):
joke: str
res = await hunter.communicate(
"Tell me a short dragon joke",
response_format=Joke
)
print(type(response))
print(response.joke)
<class '__main__.Joke'>
With fiery claws, dragons hide their laughter flaws!
LionAGI supports advanced multi-step reasoning with ReAct. Tools let the LLM invoke external actions:
from lionagi.tools.types import ReaderTool
branch = Branch(chat_model=gpt4o, tools=ReaderTool)
result = await branch.ReAct(
instruct={
"instruction": "Summarize my PDF and compare with relevant papers.",
"context": {"paper_file_path": "/path/to/paper.pdf"},
},
extension_allowed=True, # allow multi-round expansions
max_extensions=5,
verbose=True, # see step-by-step chain-of-thought
)
print(result)
The LLM can now open the PDF, read in slices, fetch references, and produce a final structured summary.
- Inspect messages:
df = branch.to_df()
print(df.tail())
- Action logs show each tool call, arguments, and outcomes.
- Verbose ReAct provides chain-of-thought analysis (helpful for debugging multi-step flows).
from lionagi import Branch, iModel
gpt4o = iModel(provider="openai", model="gpt-4o")
sonnet = iModel(
provider="anthropic",
model="claude-3-5-sonnet-20241022",
max_tokens=1000, # max_tokens is required for anthropic models
)
branch = Branch(chat_model=gpt4o)
# Switch mid-flow
analysis = await branch.communicate("Analyze these stats", imodel=sonnet)
Seamlessly route to different models in the same workflow.
pip install "lionagi[tools]"
pip install "lionagi[llms]"
pip install "lionagi[ollama]"
We welcome issues, ideas, and pull requests:
- Discord: Join to chat or get help
- Issues / PRs: GitHub
@software{Li_LionAGI_2023,
author = {Haiyang Li},
month = {12},
year = {2023},
title = {LionAGI: Towards Automated General Intelligence},
url = {https://github.com/lion-agi/lionagi},
}
🦁 LionAGI
Because real AI orchestration demands more than a single prompt. Try it out and discover the next evolution in structured, multi-model, safe AI.
For Tasks:
Click tags to check more tools for each tasksFor Jobs:
Alternative AI tools for lionagi
Similar Open Source Tools
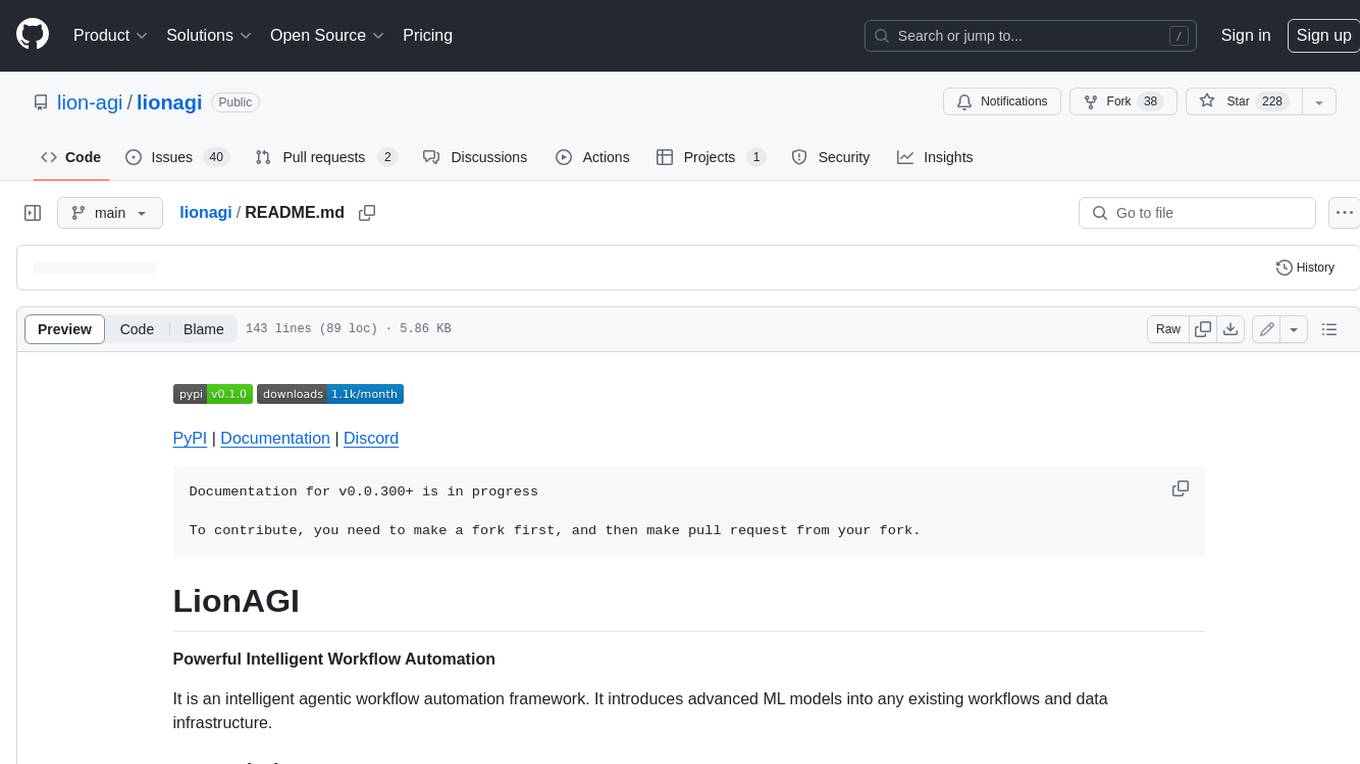
lionagi
LionAGI is a powerful intelligent workflow automation framework that introduces advanced ML models into any existing workflows and data infrastructure. It can interact with almost any model, run interactions in parallel for most models, produce structured pydantic outputs with flexible usage, automate workflow via graph based agents, use advanced prompting techniques, and more. LionAGI aims to provide a centralized agent-managed framework for "ML-powered tools coordination" and to dramatically lower the barrier of entries for creating use-case/domain specific tools. It is designed to be asynchronous only and requires Python 3.10 or higher.
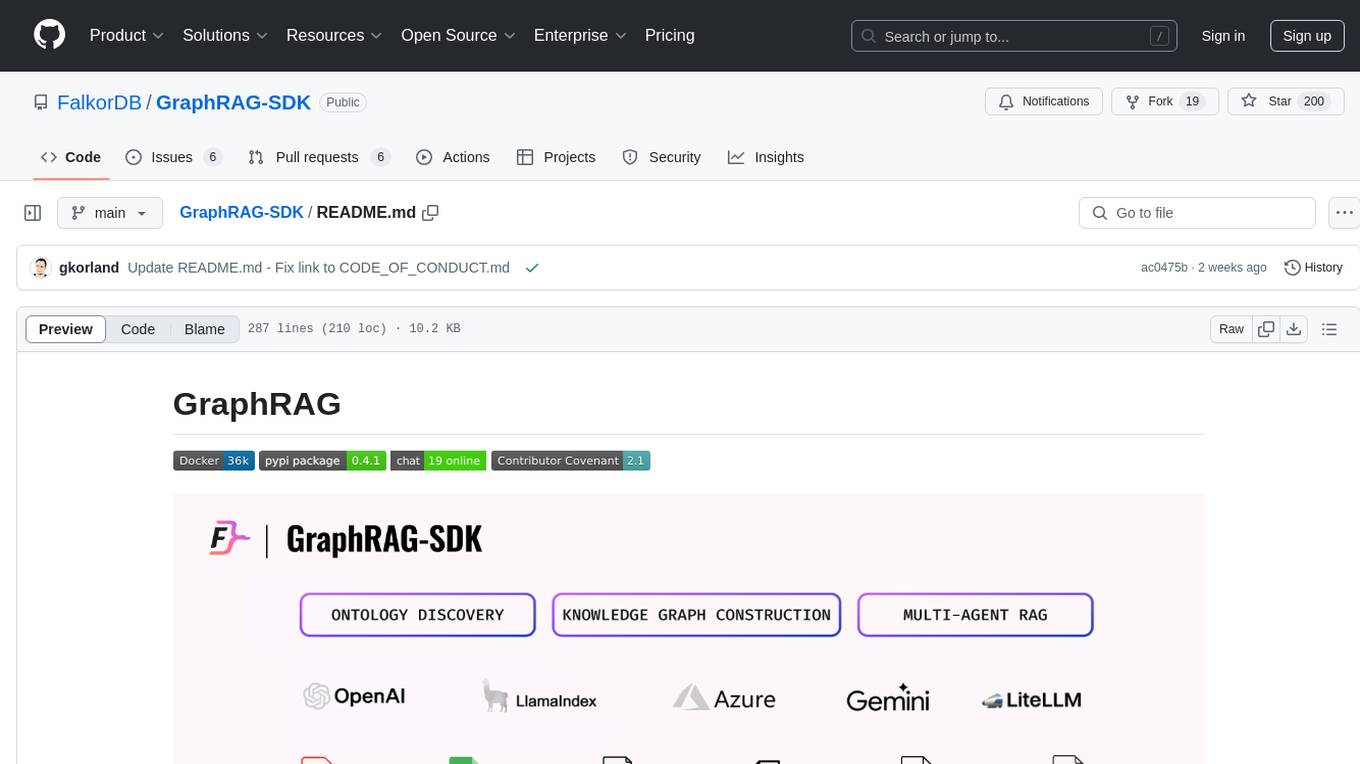
GraphRAG-SDK
Build fast and accurate GenAI applications with GraphRAG SDK, a specialized toolkit for building Graph Retrieval-Augmented Generation (GraphRAG) systems. It integrates knowledge graphs, ontology management, and state-of-the-art LLMs to deliver accurate, efficient, and customizable RAG workflows. The SDK simplifies the development process by automating ontology creation, knowledge graph agent creation, and query handling, enabling users to interact and query their knowledge graphs effectively. It supports multi-agent systems and orchestrates agents specialized in different domains. The SDK is optimized for FalkorDB, ensuring high performance and scalability for large-scale applications. By leveraging knowledge graphs, it enables semantic relationships and ontology-driven queries that go beyond standard vector similarity, enhancing retrieval-augmented generation capabilities.
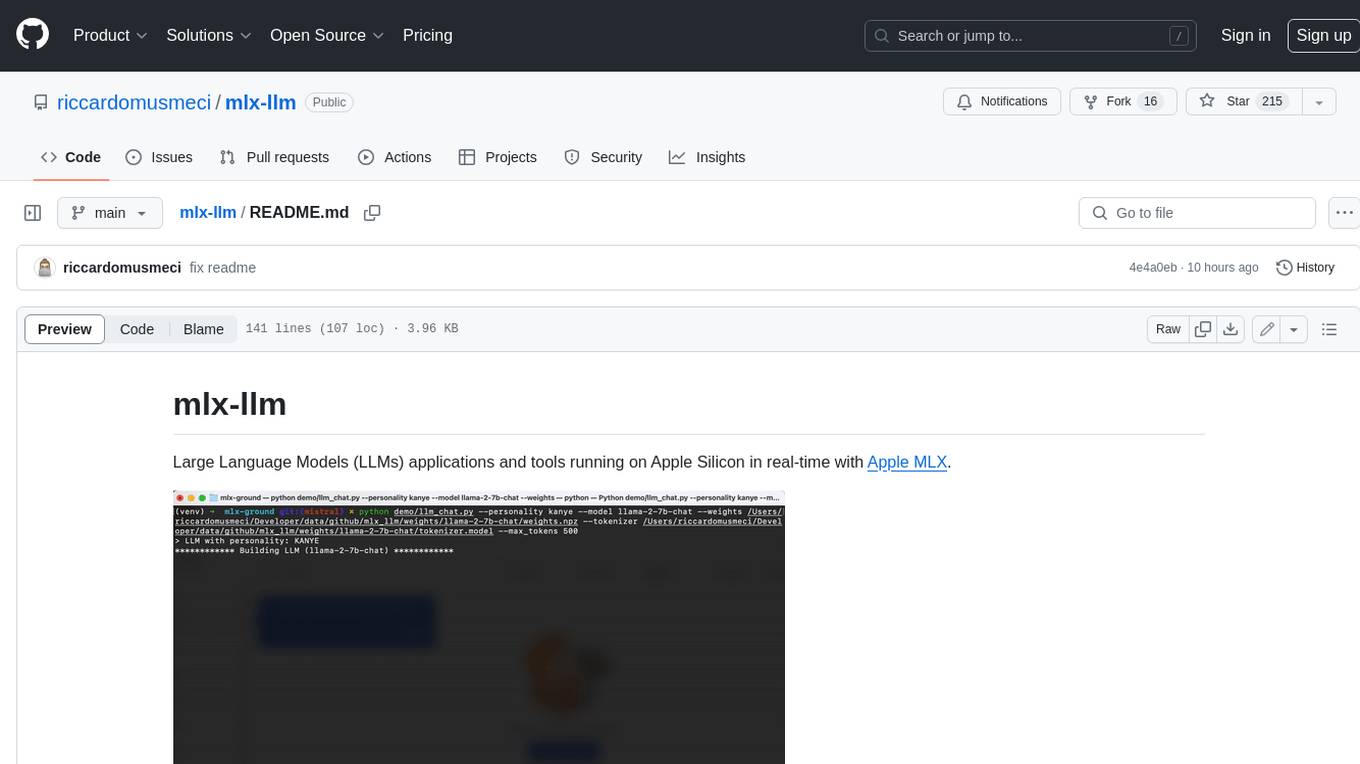
mlx-llm
mlx-llm is a library that allows you to run Large Language Models (LLMs) on Apple Silicon devices in real-time using Apple's MLX framework. It provides a simple and easy-to-use API for creating, loading, and using LLM models, as well as a variety of applications such as chatbots, fine-tuning, and retrieval-augmented generation.
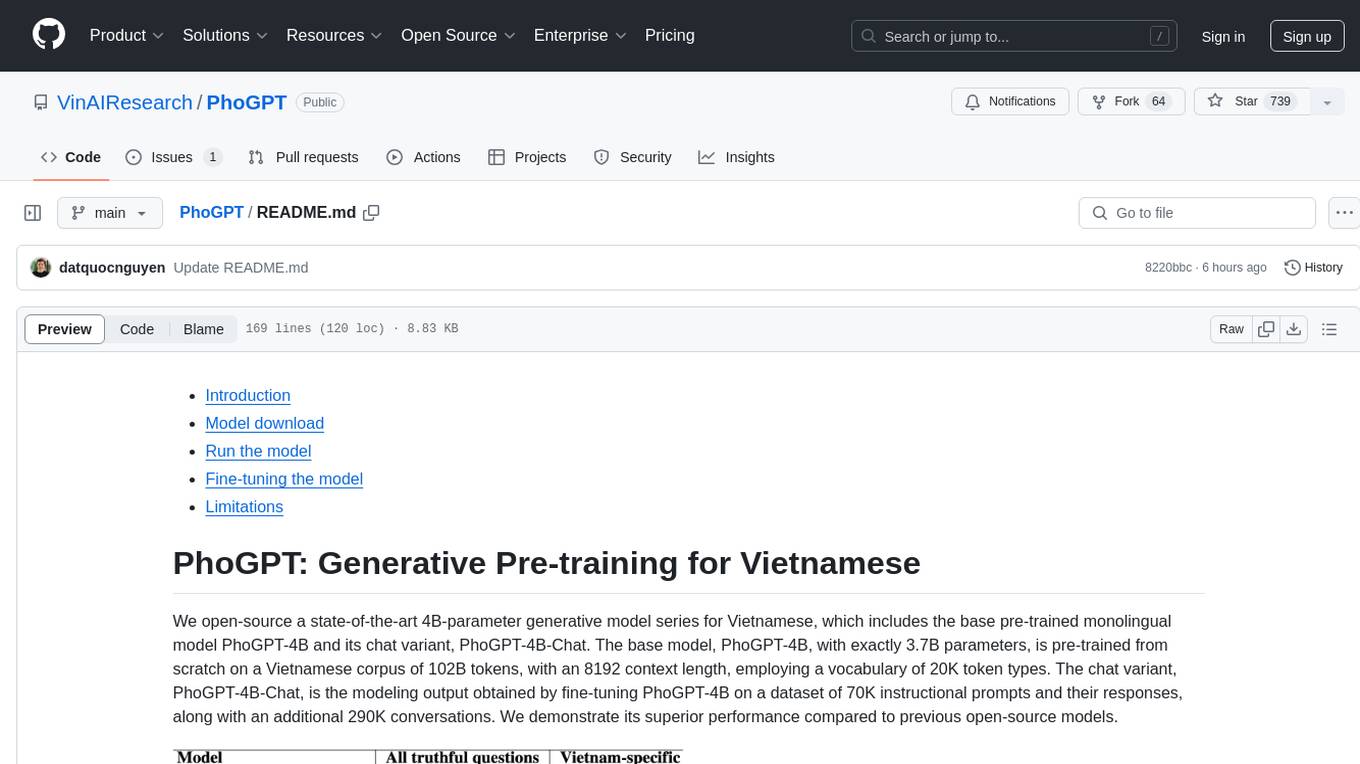
PhoGPT
PhoGPT is an open-source 4B-parameter generative model series for Vietnamese, including the base pre-trained monolingual model PhoGPT-4B and its chat variant, PhoGPT-4B-Chat. PhoGPT-4B is pre-trained from scratch on a Vietnamese corpus of 102B tokens, with an 8192 context length and a vocabulary of 20K token types. PhoGPT-4B-Chat is fine-tuned on instructional prompts and conversations, demonstrating superior performance. Users can run the model with inference engines like vLLM and Text Generation Inference, and fine-tune it using llm-foundry. However, PhoGPT has limitations in reasoning, coding, and mathematics tasks, and may generate harmful or biased responses.
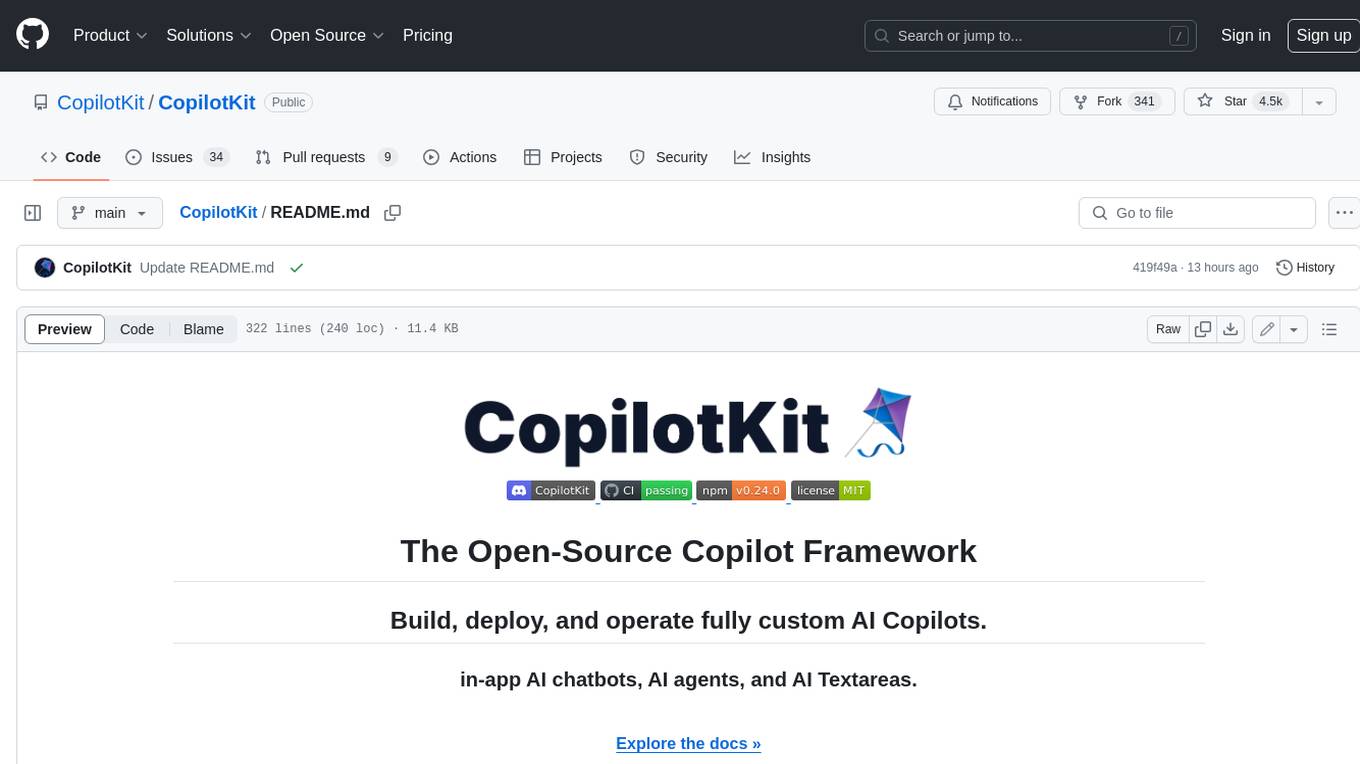
CopilotKit
CopilotKit is an open-source framework for building, deploying, and operating fully custom AI Copilots, including in-app AI chatbots, AI agents, and AI Textareas. It provides a set of components and entry points that allow developers to easily integrate AI capabilities into their applications. CopilotKit is designed to be flexible and extensible, so developers can tailor it to their specific needs. It supports a variety of use cases, including providing app-aware AI chatbots that can interact with the application state and take action, drop-in replacements for textareas with AI-assisted text generation, and in-app agents that can access real-time application context and take action within the application.
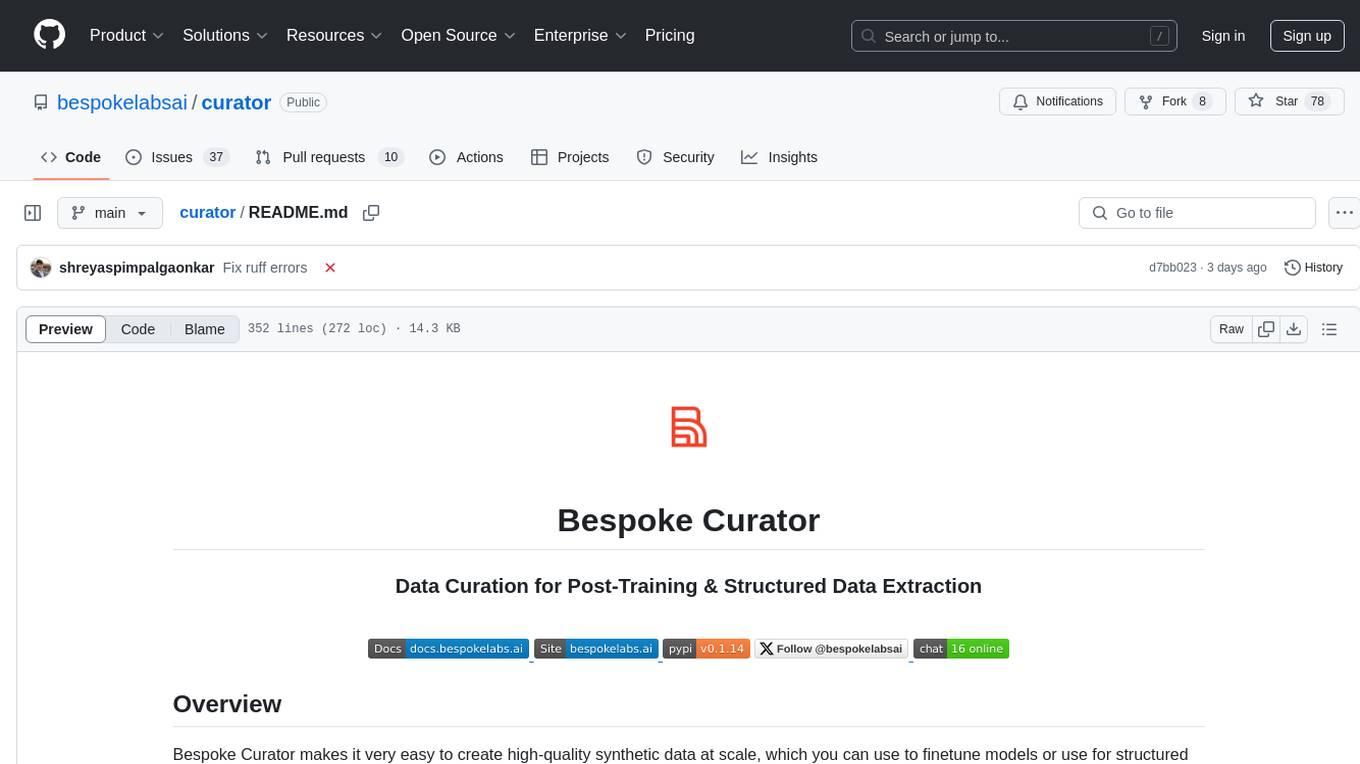
curator
Bespoke Curator is an open-source tool for data curation and structured data extraction. It provides a Python library for generating synthetic data at scale, with features like programmability, performance optimization, caching, and integration with HuggingFace Datasets. The tool includes a Curator Viewer for dataset visualization and offers a rich set of functionalities for creating and refining data generation strategies.
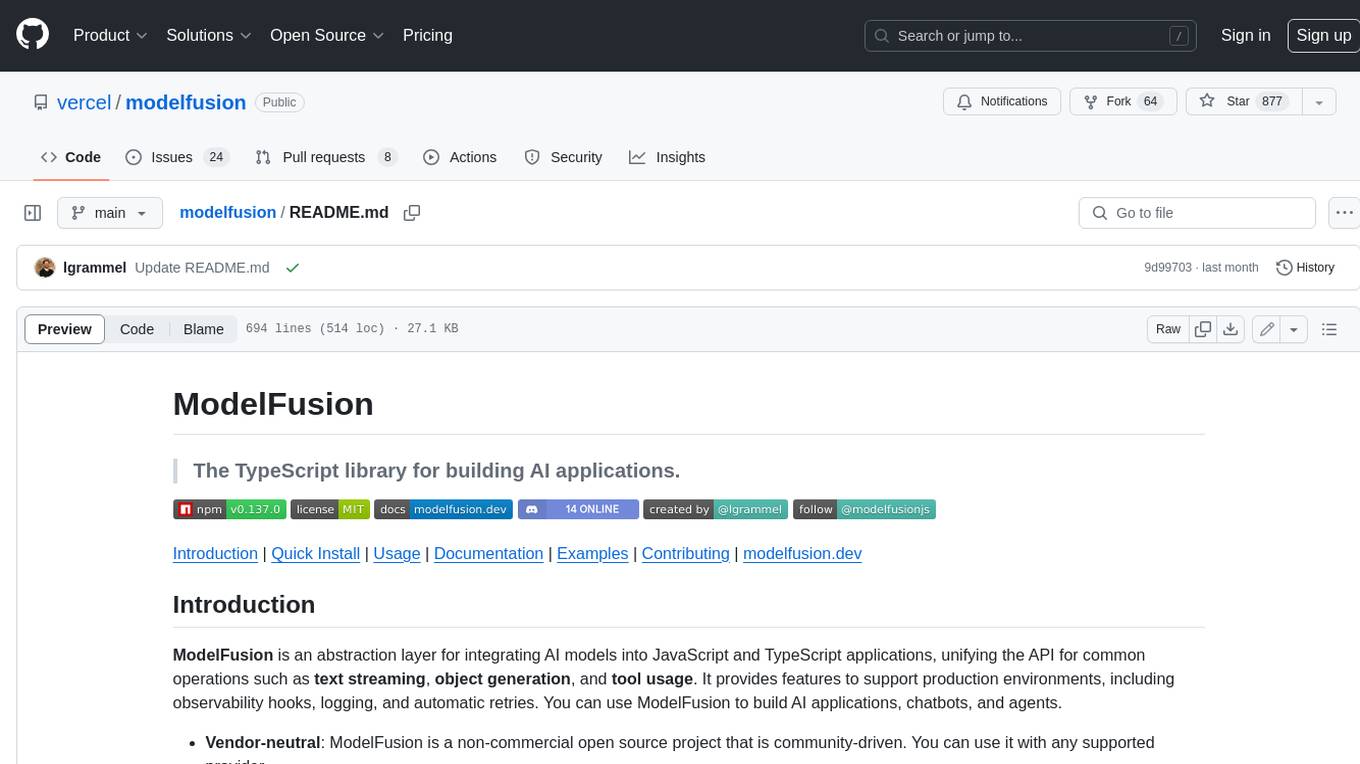
modelfusion
ModelFusion is an abstraction layer for integrating AI models into JavaScript and TypeScript applications, unifying the API for common operations such as text streaming, object generation, and tool usage. It provides features to support production environments, including observability hooks, logging, and automatic retries. You can use ModelFusion to build AI applications, chatbots, and agents. ModelFusion is a non-commercial open source project that is community-driven. You can use it with any supported provider. ModelFusion supports a wide range of models including text generation, image generation, vision, text-to-speech, speech-to-text, and embedding models. ModelFusion infers TypeScript types wherever possible and validates model responses. ModelFusion provides an observer framework and logging support. ModelFusion ensures seamless operation through automatic retries, throttling, and error handling mechanisms. ModelFusion is fully tree-shakeable, can be used in serverless environments, and only uses a minimal set of dependencies.
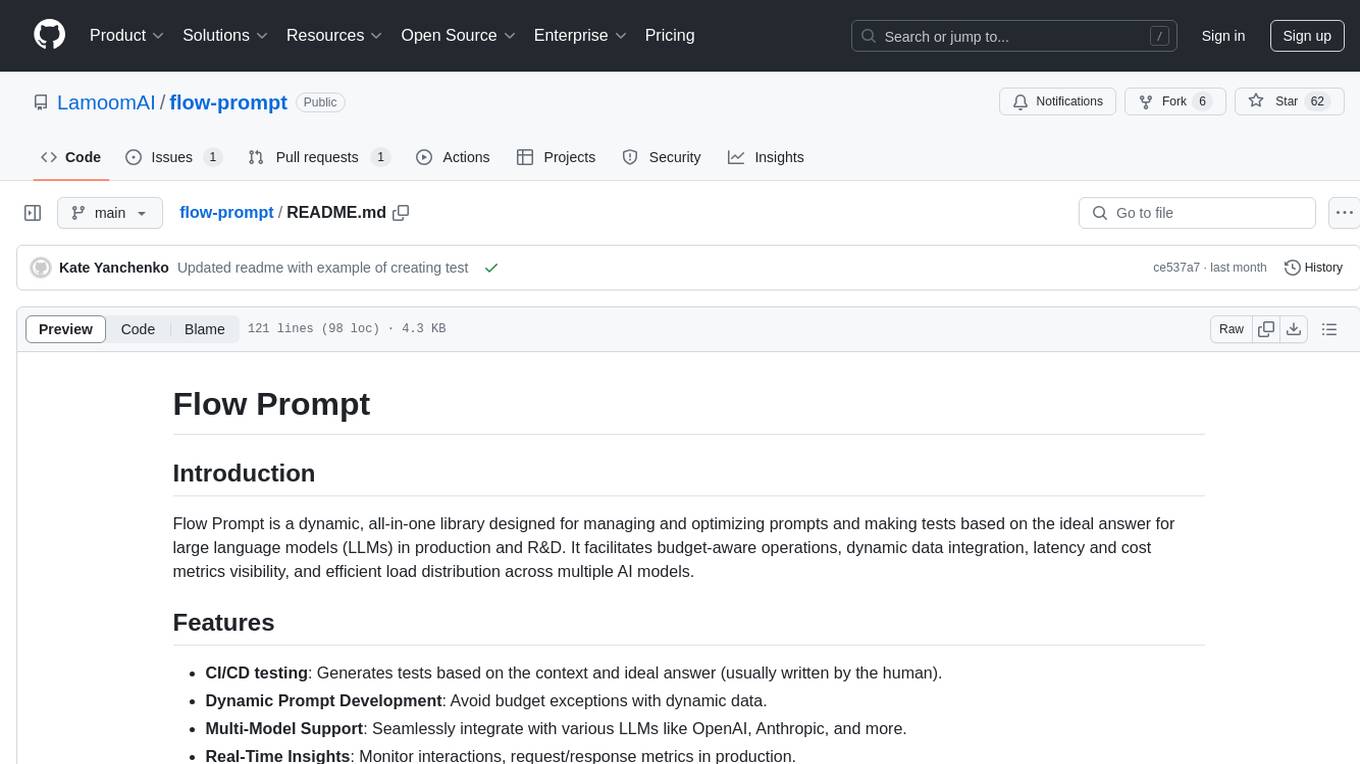
flow-prompt
Flow Prompt is a dynamic library for managing and optimizing prompts for large language models. It facilitates budget-aware operations, dynamic data integration, and efficient load distribution. Features include CI/CD testing, dynamic prompt development, multi-model support, real-time insights, and prompt testing and evolution.
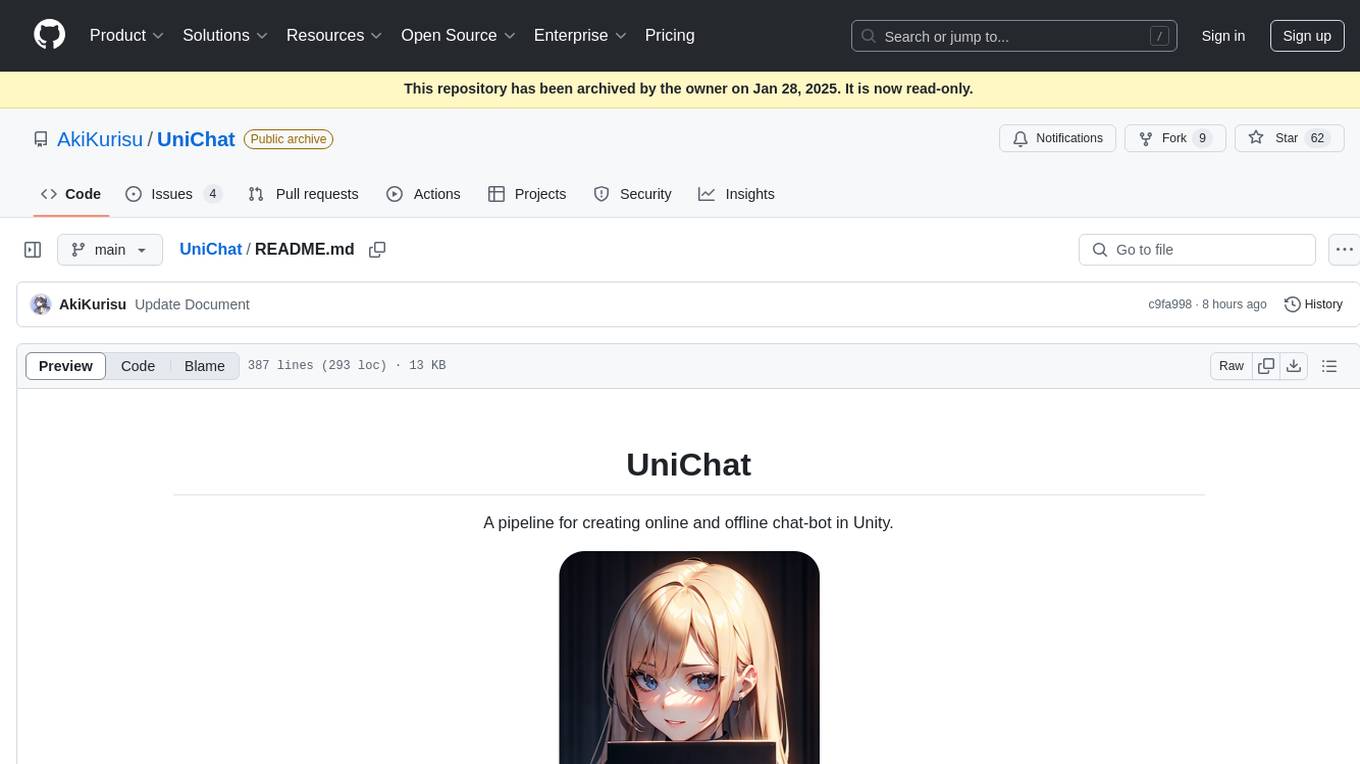
UniChat
UniChat is a pipeline tool for creating online and offline chat-bots in Unity. It leverages Unity.Sentis and text vector embedding technology to enable offline mode text content search based on vector databases. The tool includes a chain toolkit for embedding LLM and Agent in games, along with middleware components for Text to Speech, Speech to Text, and Sub-classifier functionalities. UniChat also offers a tool for invoking tools based on ReActAgent workflow, allowing users to create personalized chat scenarios and character cards. The tool provides a comprehensive solution for designing flexible conversations in games while maintaining developer's ideas.
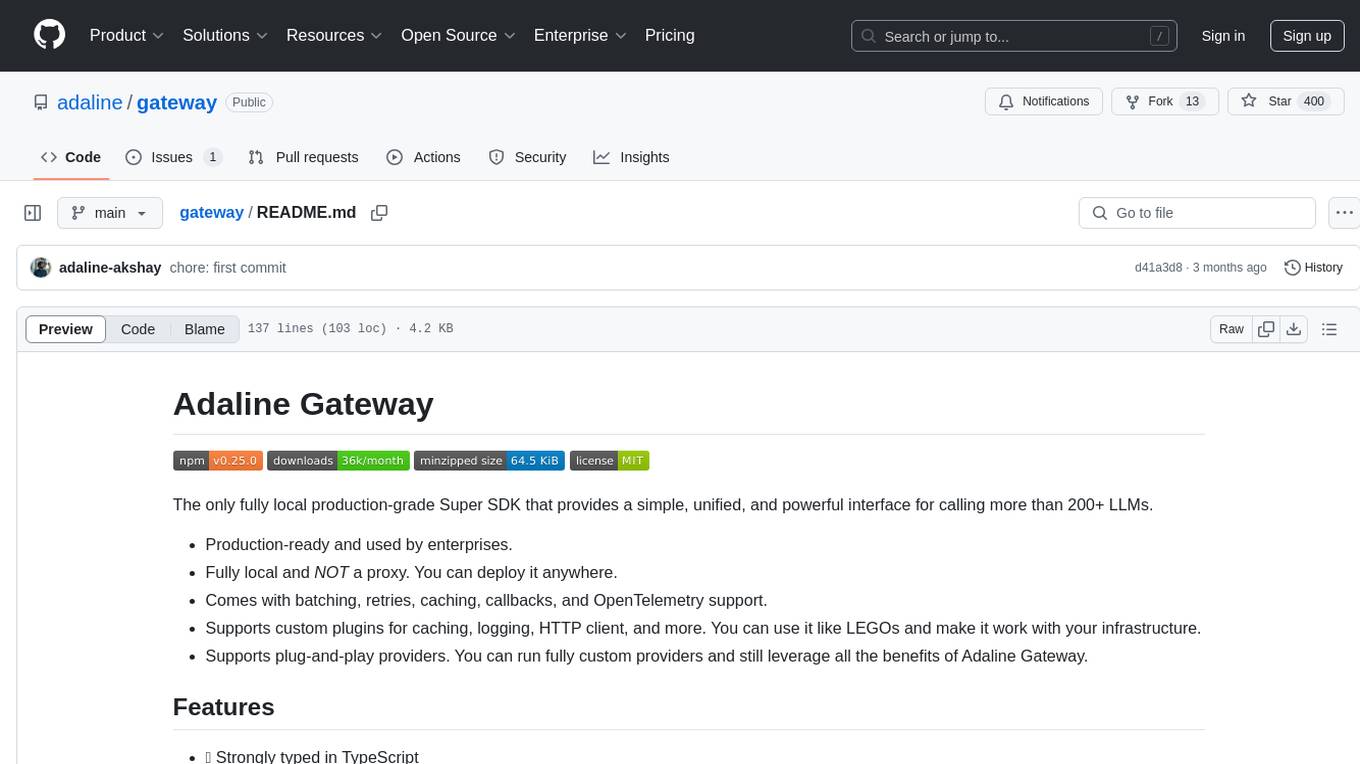
gateway
Adaline Gateway is a fully local production-grade Super SDK that offers a unified interface for calling over 200+ LLMs. It is production-ready, supports batching, retries, caching, callbacks, and OpenTelemetry. Users can create custom plugins and providers for seamless integration with their infrastructure.
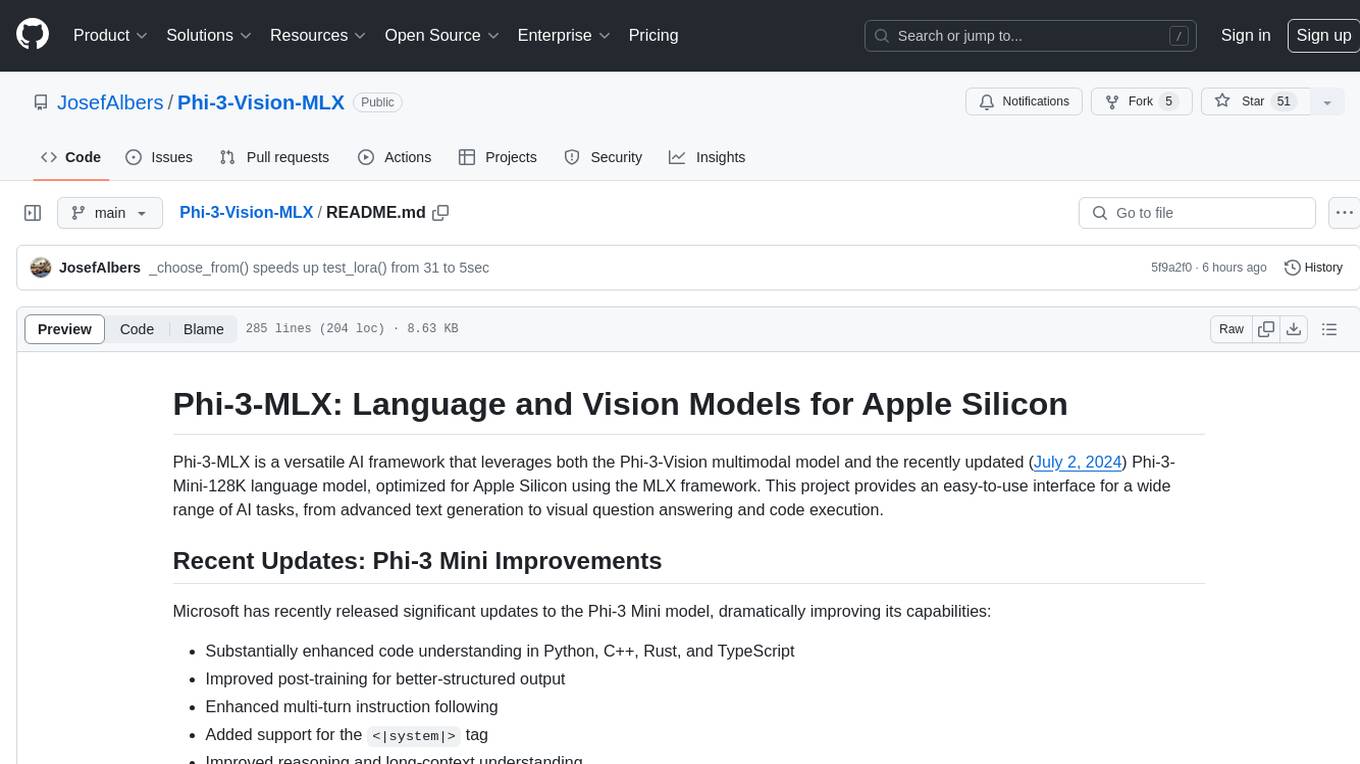
Phi-3-Vision-MLX
Phi-3-MLX is a versatile AI framework that leverages both the Phi-3-Vision multimodal model and the Phi-3-Mini-128K language model optimized for Apple Silicon using the MLX framework. It provides an easy-to-use interface for a wide range of AI tasks, from advanced text generation to visual question answering and code execution. The project features support for batched generation, flexible agent system, custom toolchains, model quantization, LoRA fine-tuning capabilities, and API integration for extended functionality.
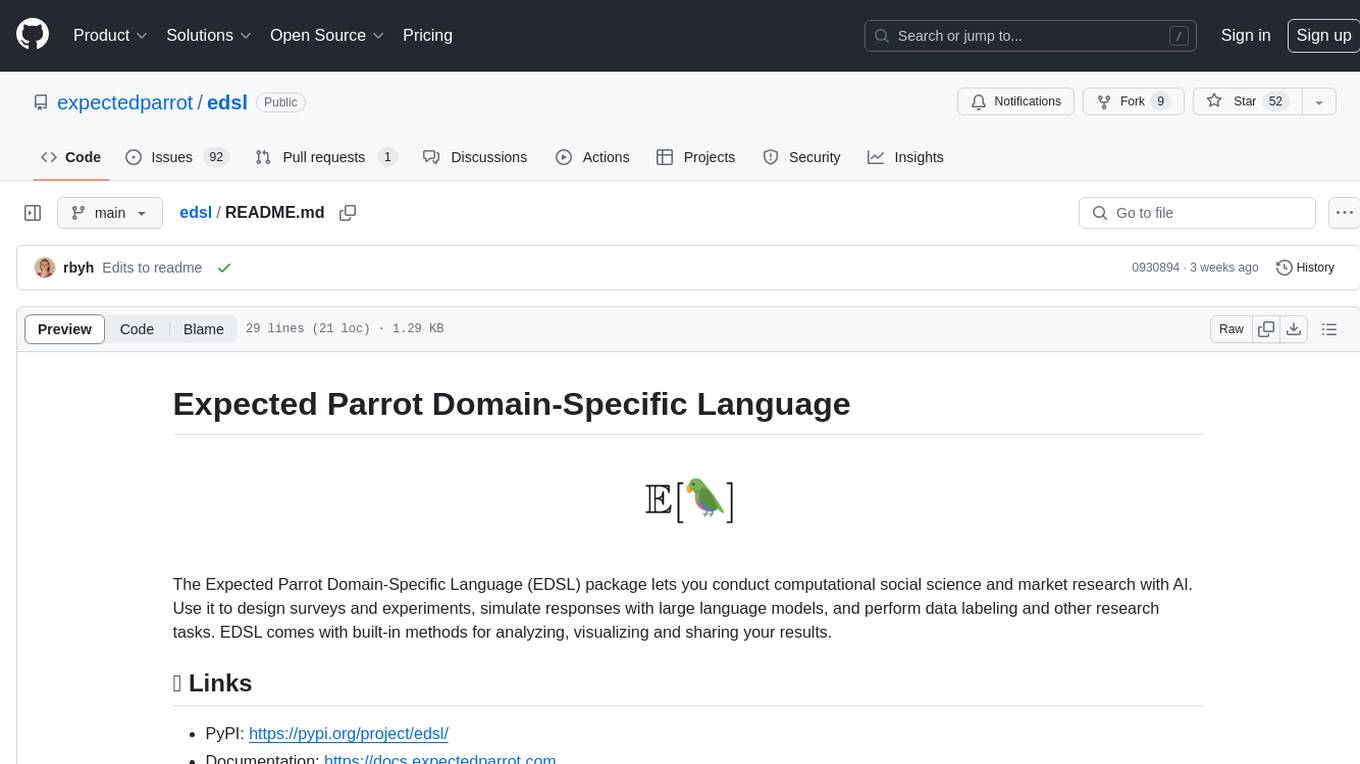
edsl
The Expected Parrot Domain-Specific Language (EDSL) package enables users to conduct computational social science and market research with AI. It facilitates designing surveys and experiments, simulating responses using large language models, and performing data labeling and other research tasks. EDSL includes built-in methods for analyzing, visualizing, and sharing research results. It is compatible with Python 3.9 - 3.11 and requires API keys for LLMs stored in a `.env` file.
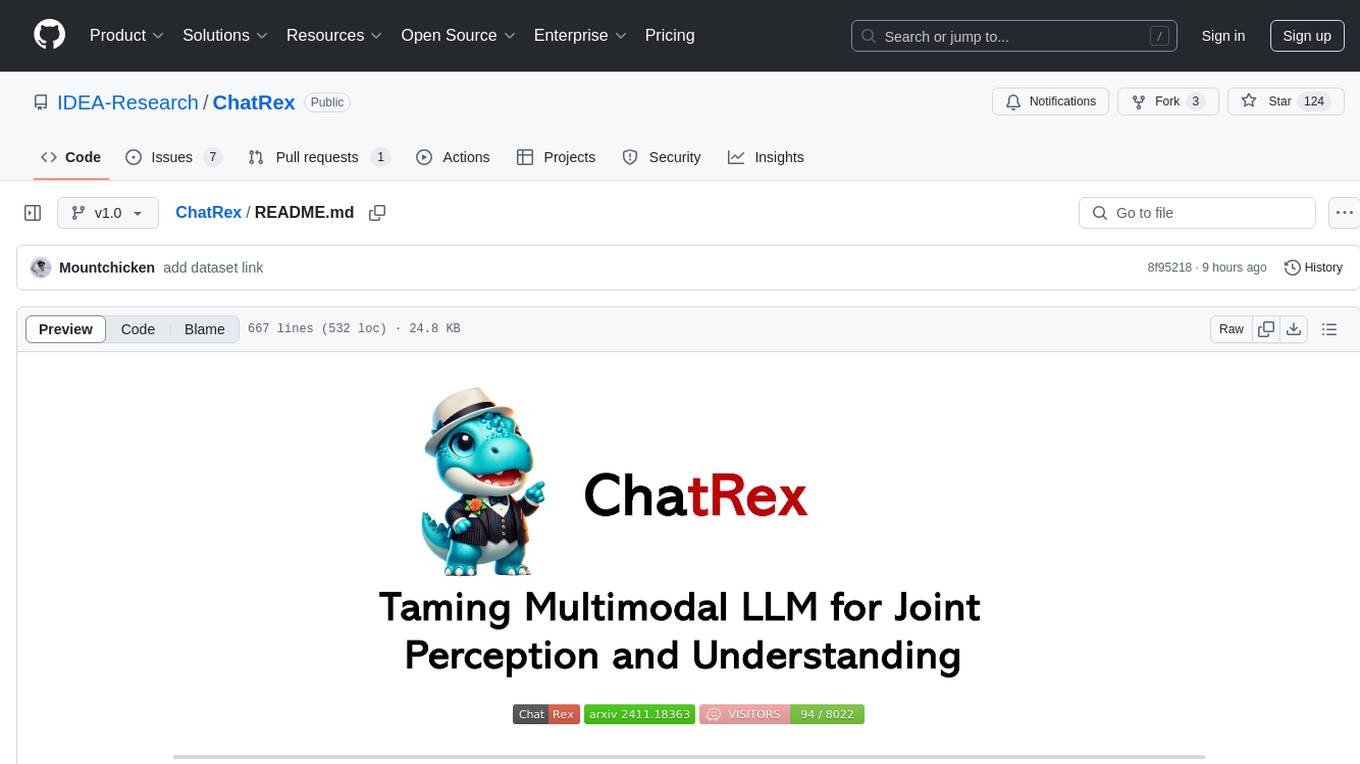
ChatRex
ChatRex is a Multimodal Large Language Model (MLLM) designed to seamlessly integrate fine-grained object perception and robust language understanding. By adopting a decoupled architecture with a retrieval-based approach for object detection and leveraging high-resolution visual inputs, ChatRex addresses key challenges in perception tasks. It is powered by the Rexverse-2M dataset with diverse image-region-text annotations. ChatRex can be applied to various scenarios requiring fine-grained perception, such as object detection, grounded conversation, grounded image captioning, and region understanding.
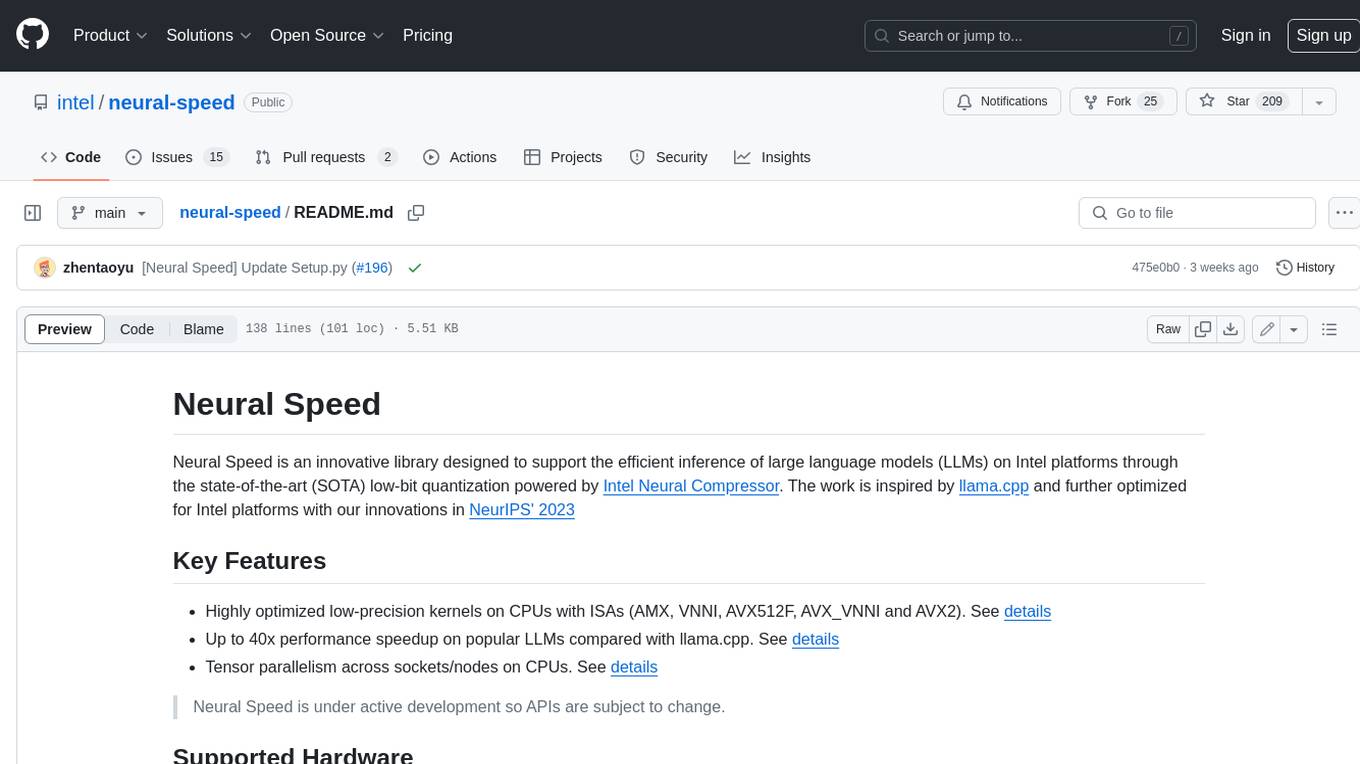
neural-speed
Neural Speed is an innovative library designed to support the efficient inference of large language models (LLMs) on Intel platforms through the state-of-the-art (SOTA) low-bit quantization powered by Intel Neural Compressor. The work is inspired by llama.cpp and further optimized for Intel platforms with our innovations in NeurIPS' 2023
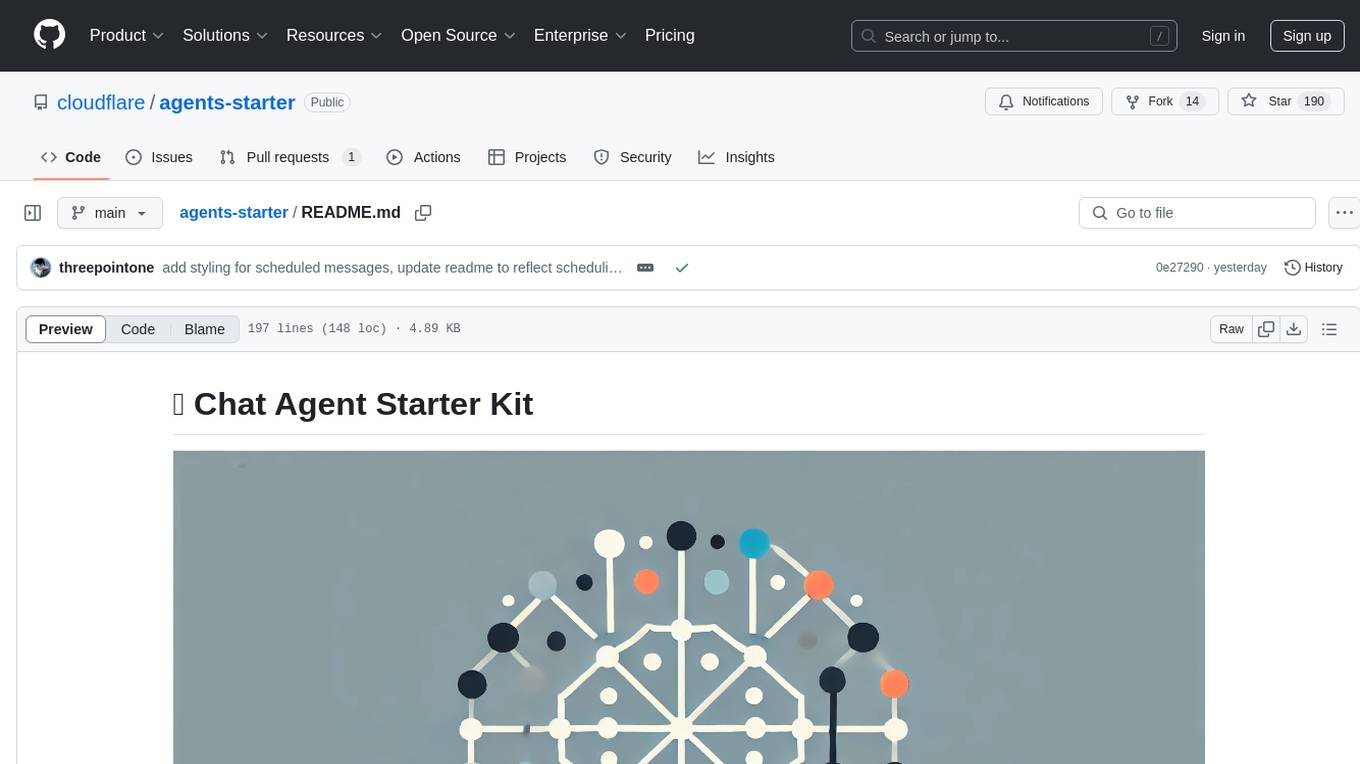
agents-starter
A starter template for building AI-powered chat agents using Cloudflare's Agent platform, powered by agents-sdk. It provides a foundation for creating interactive chat experiences with AI, complete with a modern UI and tool integration capabilities. Features include interactive chat interface with AI, built-in tool system with human-in-the-loop confirmation, advanced task scheduling, dark/light theme support, real-time streaming responses, state management, and chat history. Prerequisites include a Cloudflare account and OpenAI API key. The project structure includes components for chat UI implementation, chat agent logic, tool definitions, and helper functions. Customization guide covers adding new tools, modifying the UI, and example use cases for customer support, development assistant, data analysis assistant, personal productivity assistant, and scheduling assistant.
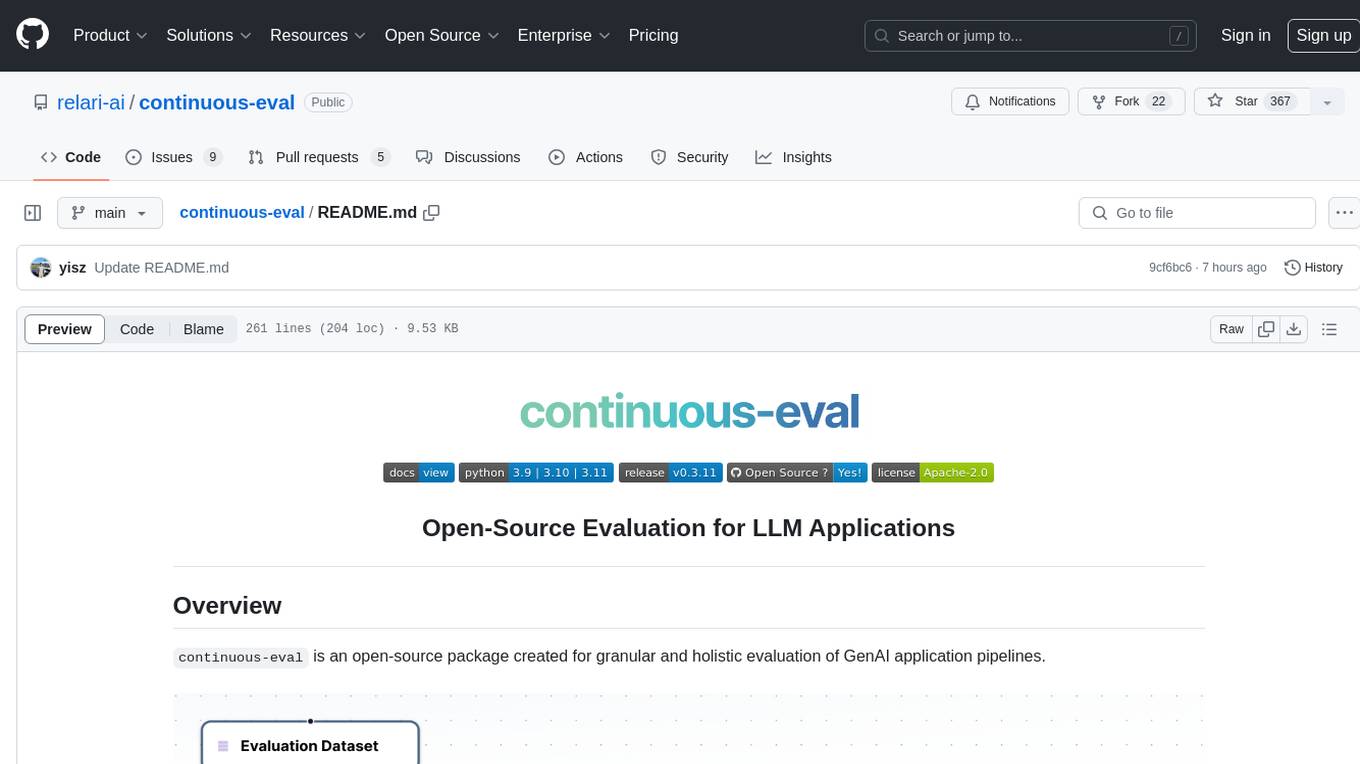
continuous-eval
Open-Source Evaluation for LLM Applications. `continuous-eval` is an open-source package created for granular and holistic evaluation of GenAI application pipelines. It offers modularized evaluation, a comprehensive metric library covering various LLM use cases, the ability to leverage user feedback in evaluation, and synthetic dataset generation for testing pipelines. Users can define their own metrics by extending the Metric class. The tool allows running evaluation on a pipeline defined with modules and corresponding metrics. Additionally, it provides synthetic data generation capabilities to create user interaction data for evaluation or training purposes.
For similar tasks
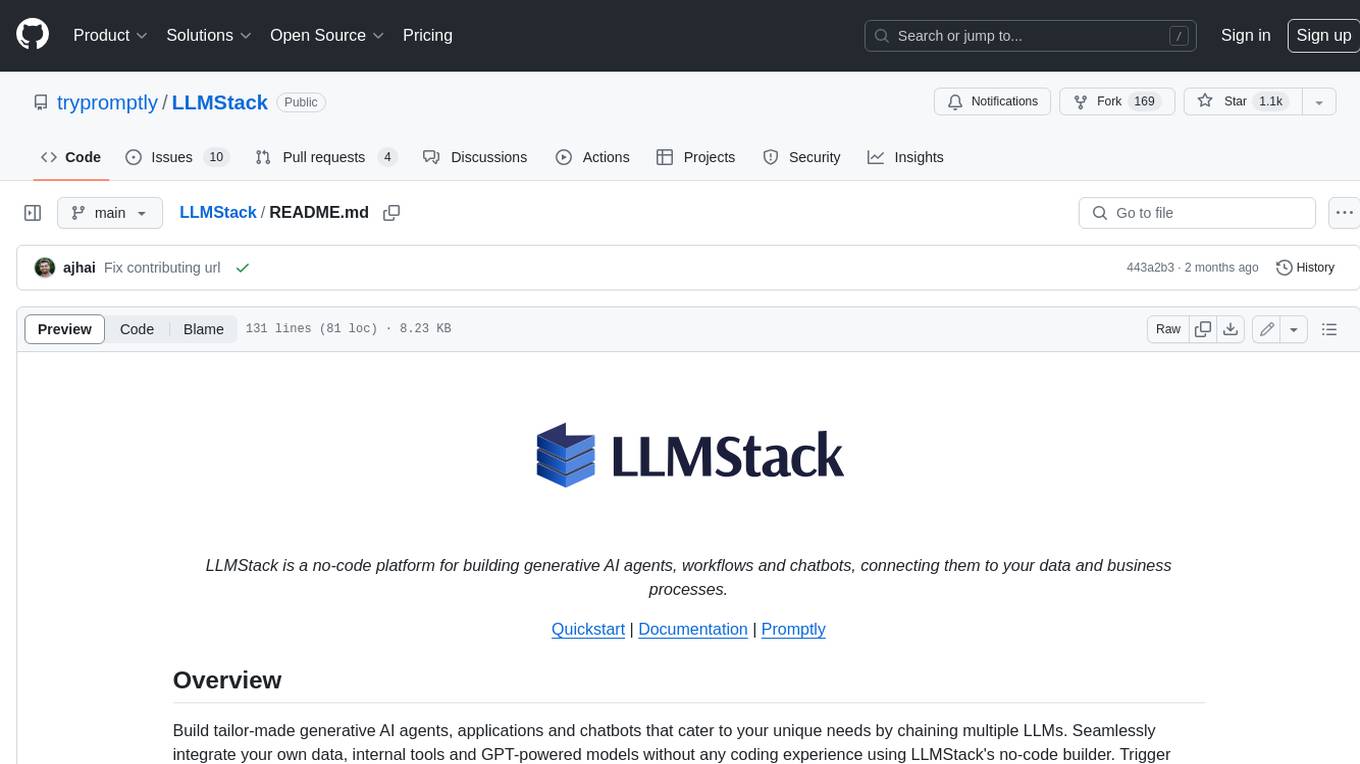
LLMStack
LLMStack is a no-code platform for building generative AI agents, workflows, and chatbots. It allows users to connect their own data, internal tools, and GPT-powered models without any coding experience. LLMStack can be deployed to the cloud or on-premise and can be accessed via HTTP API or triggered from Slack or Discord.
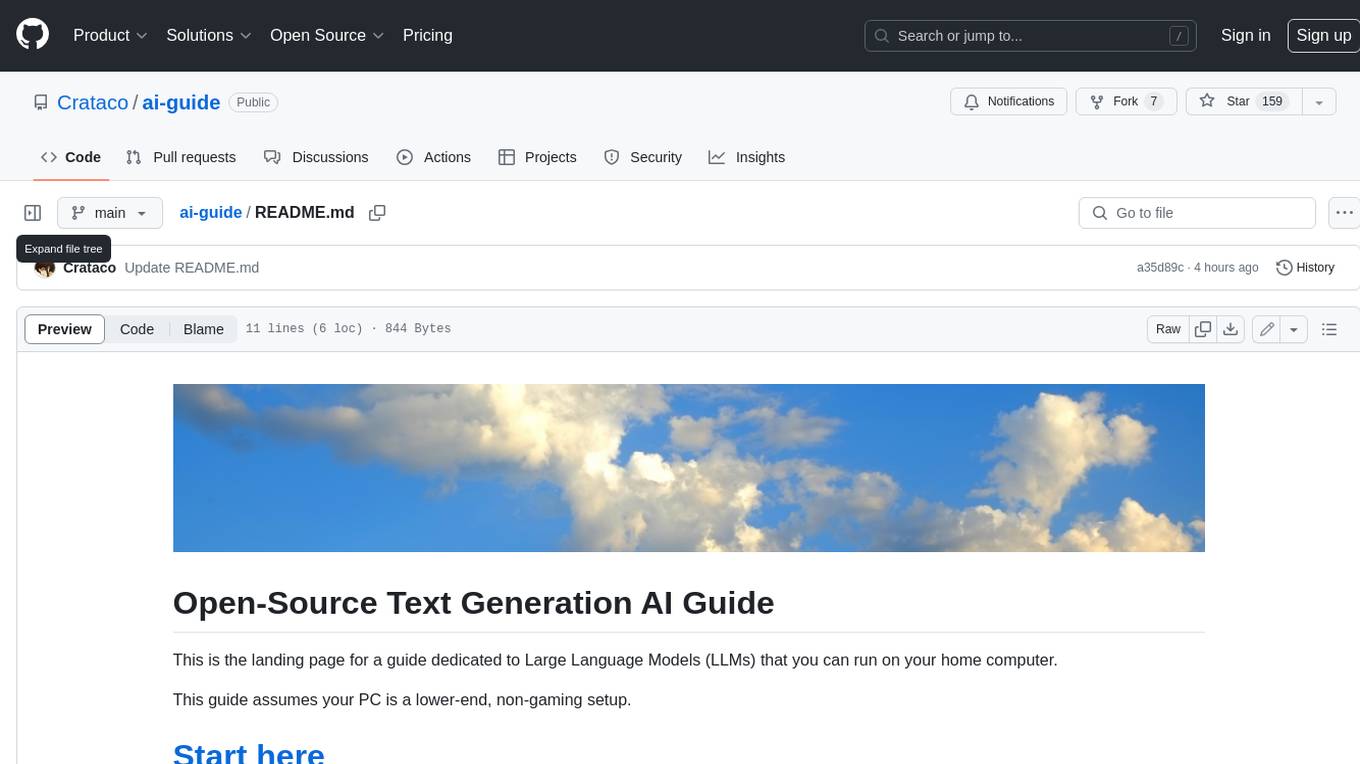
ai-guide
This guide is dedicated to Large Language Models (LLMs) that you can run on your home computer. It assumes your PC is a lower-end, non-gaming setup.
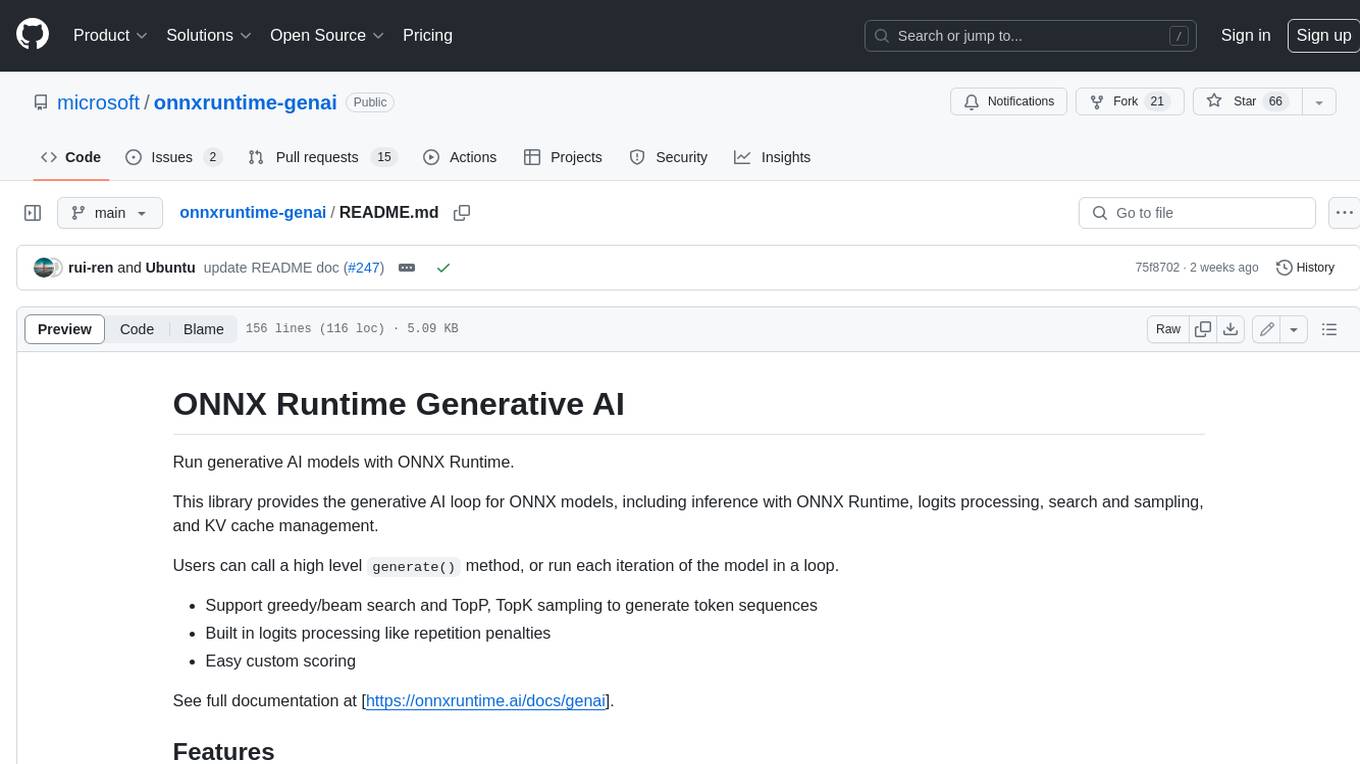
onnxruntime-genai
ONNX Runtime Generative AI is a library that provides the generative AI loop for ONNX models, including inference with ONNX Runtime, logits processing, search and sampling, and KV cache management. Users can call a high level `generate()` method, or run each iteration of the model in a loop. It supports greedy/beam search and TopP, TopK sampling to generate token sequences, has built in logits processing like repetition penalties, and allows for easy custom scoring.
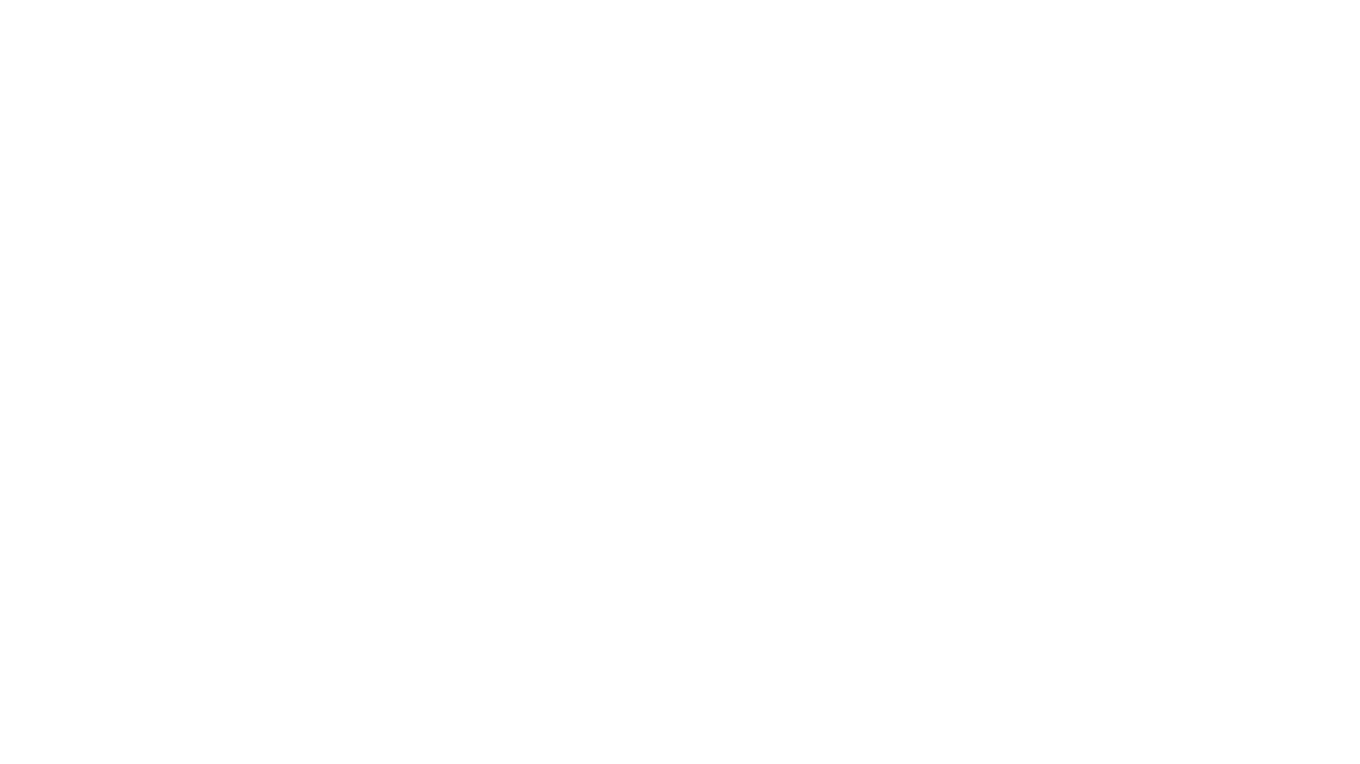
jupyter-ai
Jupyter AI connects generative AI with Jupyter notebooks. It provides a user-friendly and powerful way to explore generative AI models in notebooks and improve your productivity in JupyterLab and the Jupyter Notebook. Specifically, Jupyter AI offers: * An `%%ai` magic that turns the Jupyter notebook into a reproducible generative AI playground. This works anywhere the IPython kernel runs (JupyterLab, Jupyter Notebook, Google Colab, Kaggle, VSCode, etc.). * A native chat UI in JupyterLab that enables you to work with generative AI as a conversational assistant. * Support for a wide range of generative model providers, including AI21, Anthropic, AWS, Cohere, Gemini, Hugging Face, NVIDIA, and OpenAI. * Local model support through GPT4All, enabling use of generative AI models on consumer grade machines with ease and privacy.
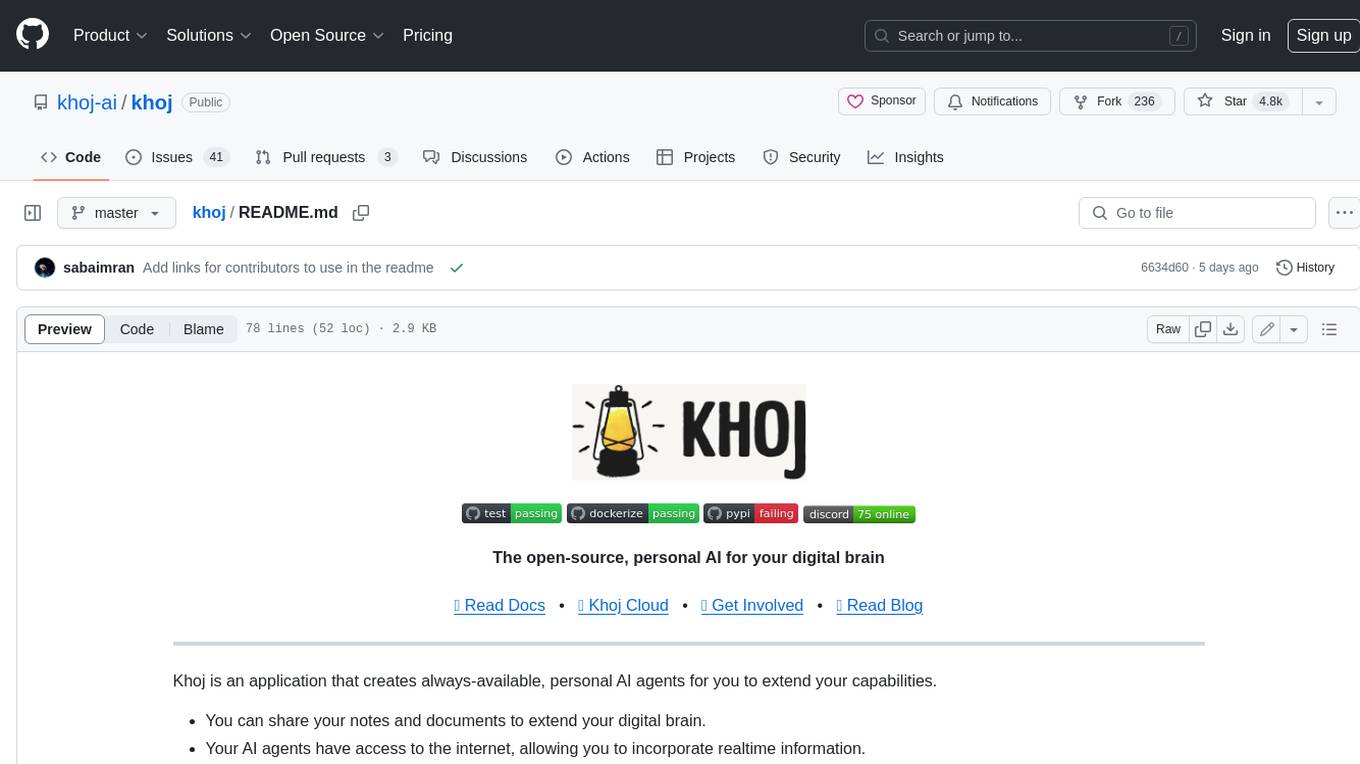
khoj
Khoj is an open-source, personal AI assistant that extends your capabilities by creating always-available AI agents. You can share your notes and documents to extend your digital brain, and your AI agents have access to the internet, allowing you to incorporate real-time information. Khoj is accessible on Desktop, Emacs, Obsidian, Web, and Whatsapp, and you can share PDF, markdown, org-mode, notion files, and GitHub repositories. You'll get fast, accurate semantic search on top of your docs, and your agents can create deeply personal images and understand your speech. Khoj is self-hostable and always will be.
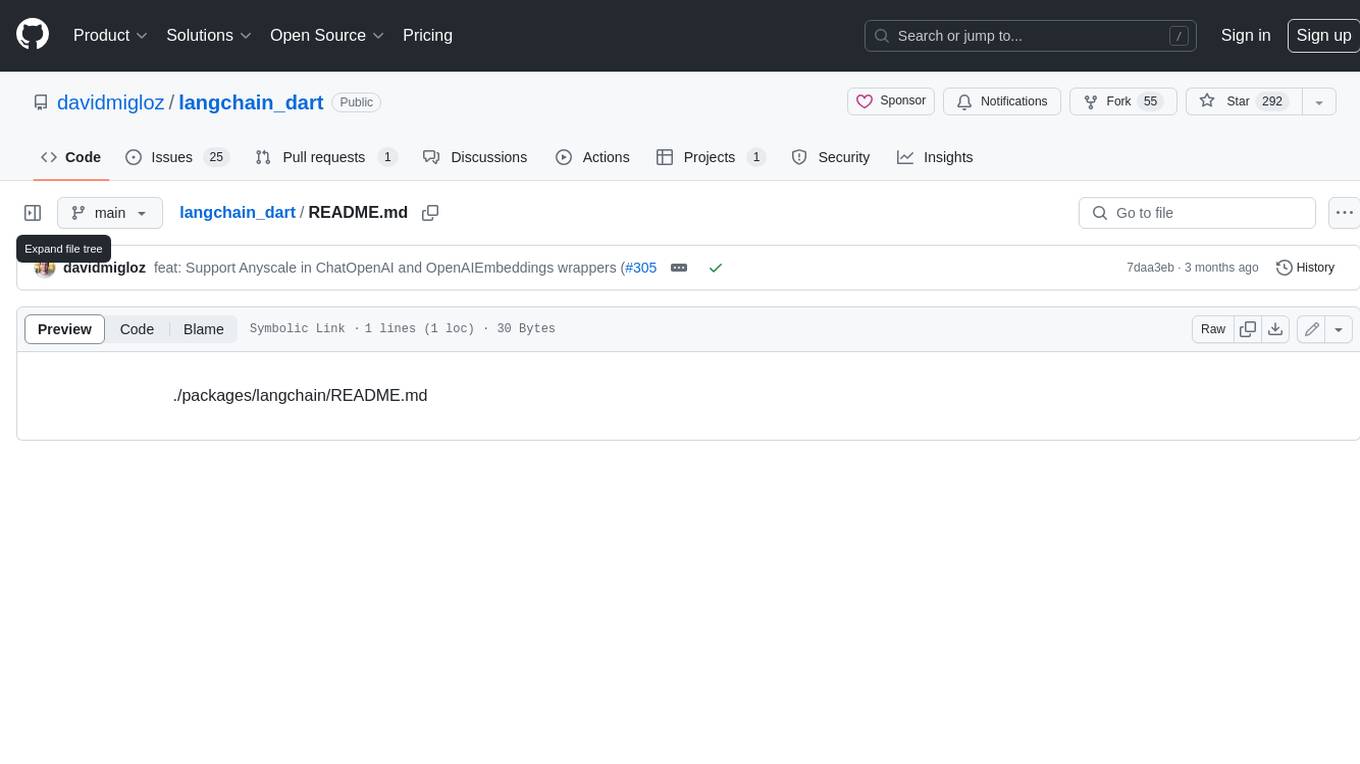
langchain_dart
LangChain.dart is a Dart port of the popular LangChain Python framework created by Harrison Chase. LangChain provides a set of ready-to-use components for working with language models and a standard interface for chaining them together to formulate more advanced use cases (e.g. chatbots, Q&A with RAG, agents, summarization, extraction, etc.). The components can be grouped into a few core modules: * **Model I/O:** LangChain offers a unified API for interacting with various LLM providers (e.g. OpenAI, Google, Mistral, Ollama, etc.), allowing developers to switch between them with ease. Additionally, it provides tools for managing model inputs (prompt templates and example selectors) and parsing the resulting model outputs (output parsers). * **Retrieval:** assists in loading user data (via document loaders), transforming it (with text splitters), extracting its meaning (using embedding models), storing (in vector stores) and retrieving it (through retrievers) so that it can be used to ground the model's responses (i.e. Retrieval-Augmented Generation or RAG). * **Agents:** "bots" that leverage LLMs to make informed decisions about which available tools (such as web search, calculators, database lookup, etc.) to use to accomplish the designated task. The different components can be composed together using the LangChain Expression Language (LCEL).
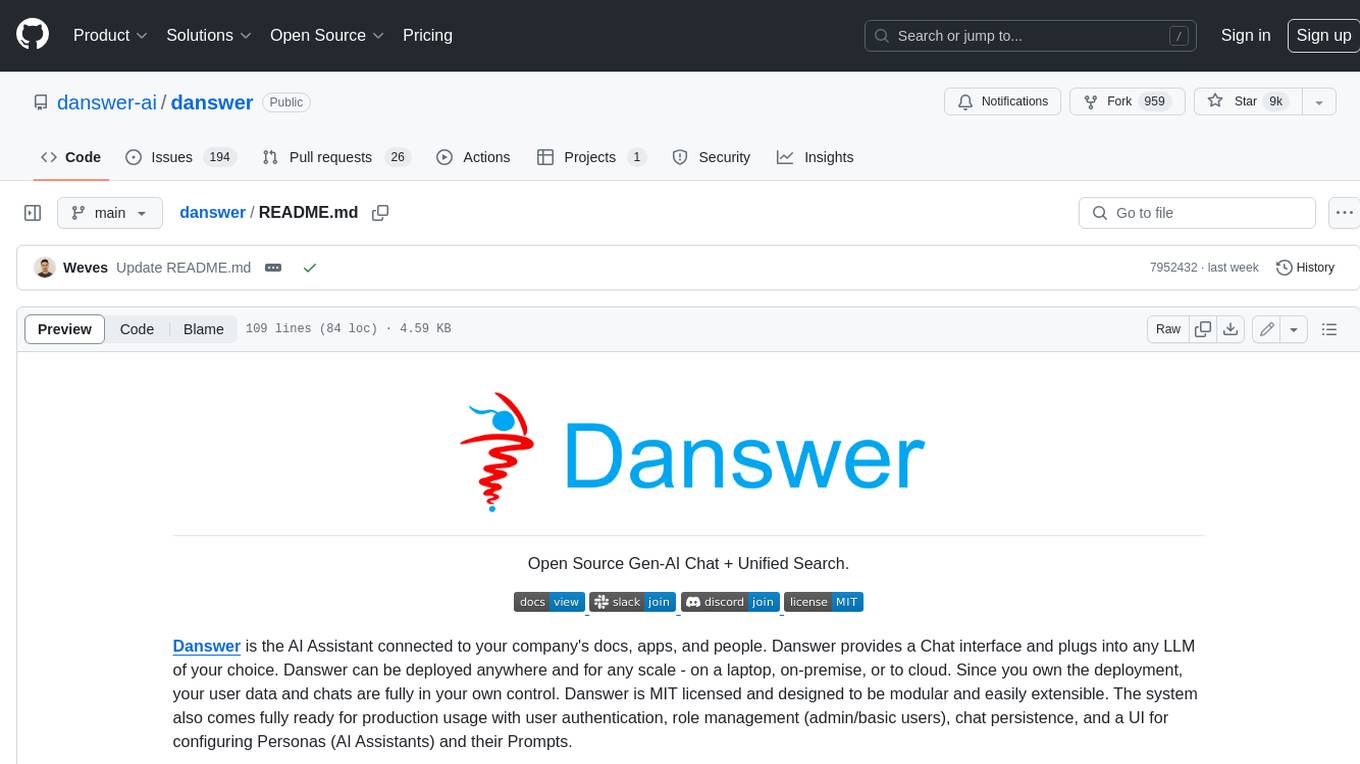
danswer
Danswer is an open-source Gen-AI Chat and Unified Search tool that connects to your company's docs, apps, and people. It provides a Chat interface and plugs into any LLM of your choice. Danswer can be deployed anywhere and for any scale - on a laptop, on-premise, or to cloud. Since you own the deployment, your user data and chats are fully in your own control. Danswer is MIT licensed and designed to be modular and easily extensible. The system also comes fully ready for production usage with user authentication, role management (admin/basic users), chat persistence, and a UI for configuring Personas (AI Assistants) and their Prompts. Danswer also serves as a Unified Search across all common workplace tools such as Slack, Google Drive, Confluence, etc. By combining LLMs and team specific knowledge, Danswer becomes a subject matter expert for the team. Imagine ChatGPT if it had access to your team's unique knowledge! It enables questions such as "A customer wants feature X, is this already supported?" or "Where's the pull request for feature Y?"
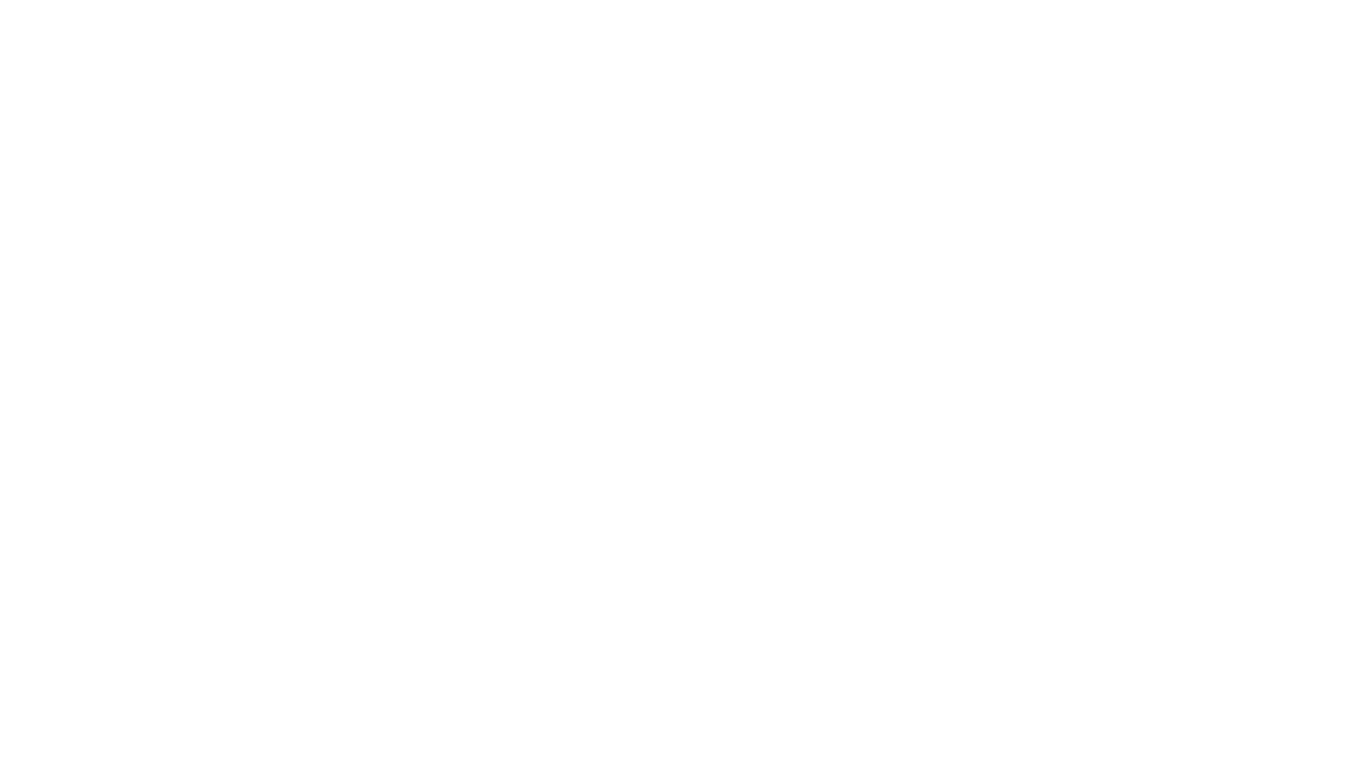
infinity
Infinity is an AI-native database designed for LLM applications, providing incredibly fast full-text and vector search capabilities. It supports a wide range of data types, including vectors, full-text, and structured data, and offers a fused search feature that combines multiple embeddings and full text. Infinity is easy to use, with an intuitive Python API and a single-binary architecture that simplifies deployment. It achieves high performance, with 0.1 milliseconds query latency on million-scale vector datasets and up to 15K QPS.
For similar jobs
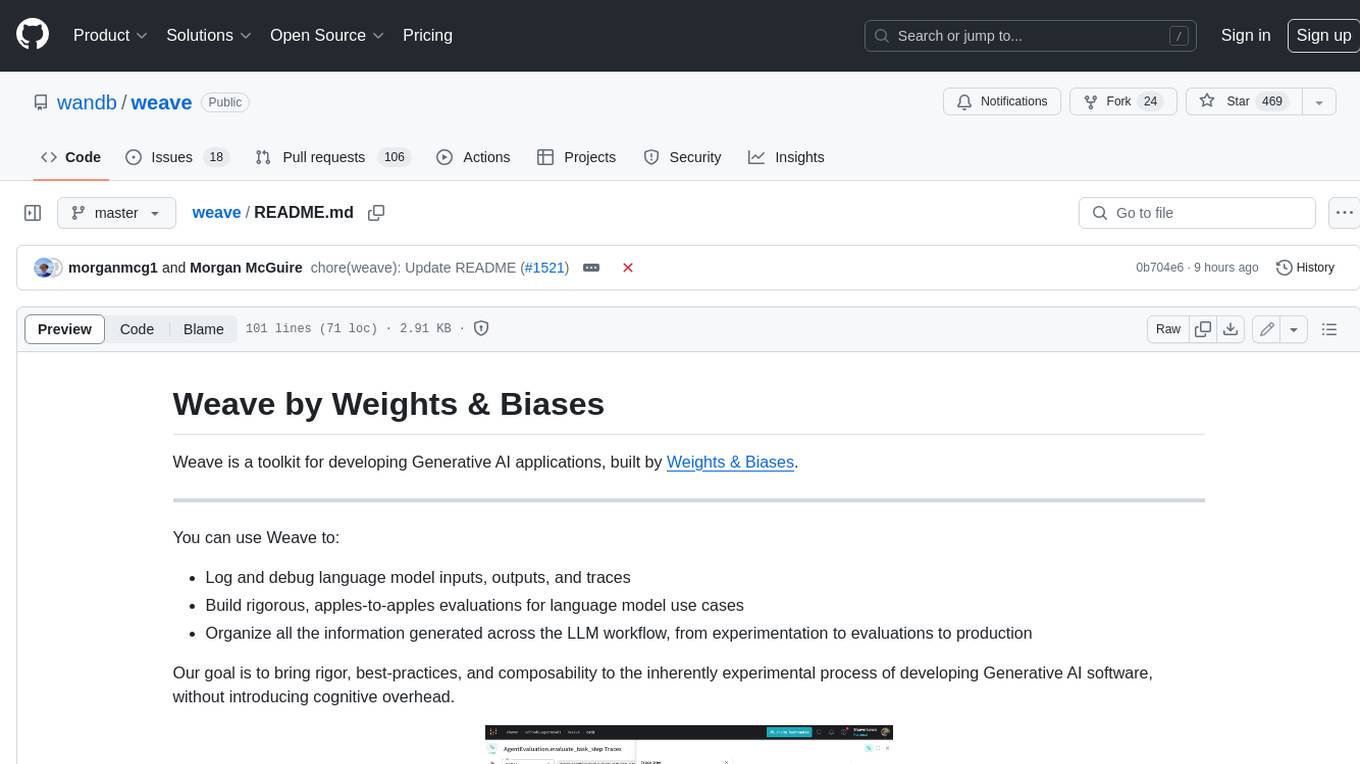
weave
Weave is a toolkit for developing Generative AI applications, built by Weights & Biases. With Weave, you can log and debug language model inputs, outputs, and traces; build rigorous, apples-to-apples evaluations for language model use cases; and organize all the information generated across the LLM workflow, from experimentation to evaluations to production. Weave aims to bring rigor, best-practices, and composability to the inherently experimental process of developing Generative AI software, without introducing cognitive overhead.
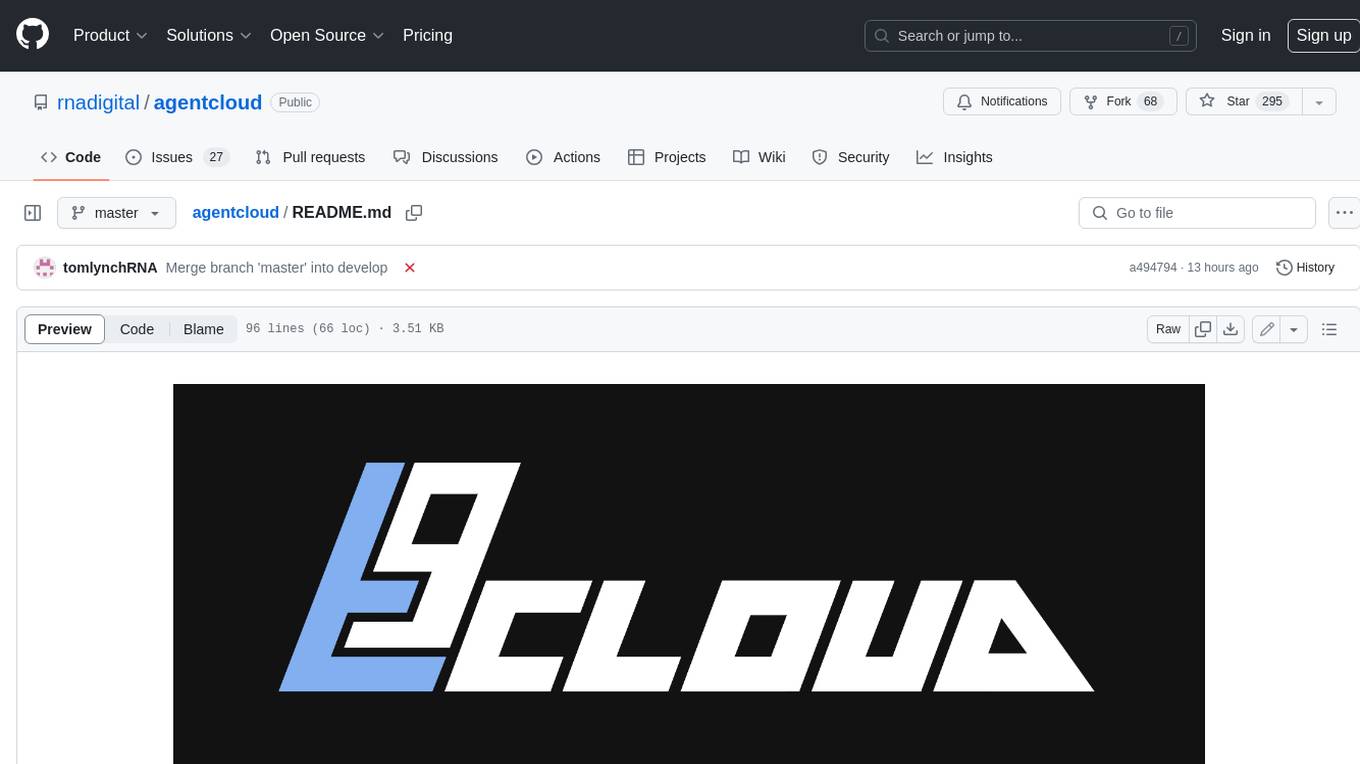
agentcloud
AgentCloud is an open-source platform that enables companies to build and deploy private LLM chat apps, empowering teams to securely interact with their data. It comprises three main components: Agent Backend, Webapp, and Vector Proxy. To run this project locally, clone the repository, install Docker, and start the services. The project is licensed under the GNU Affero General Public License, version 3 only. Contributions and feedback are welcome from the community.
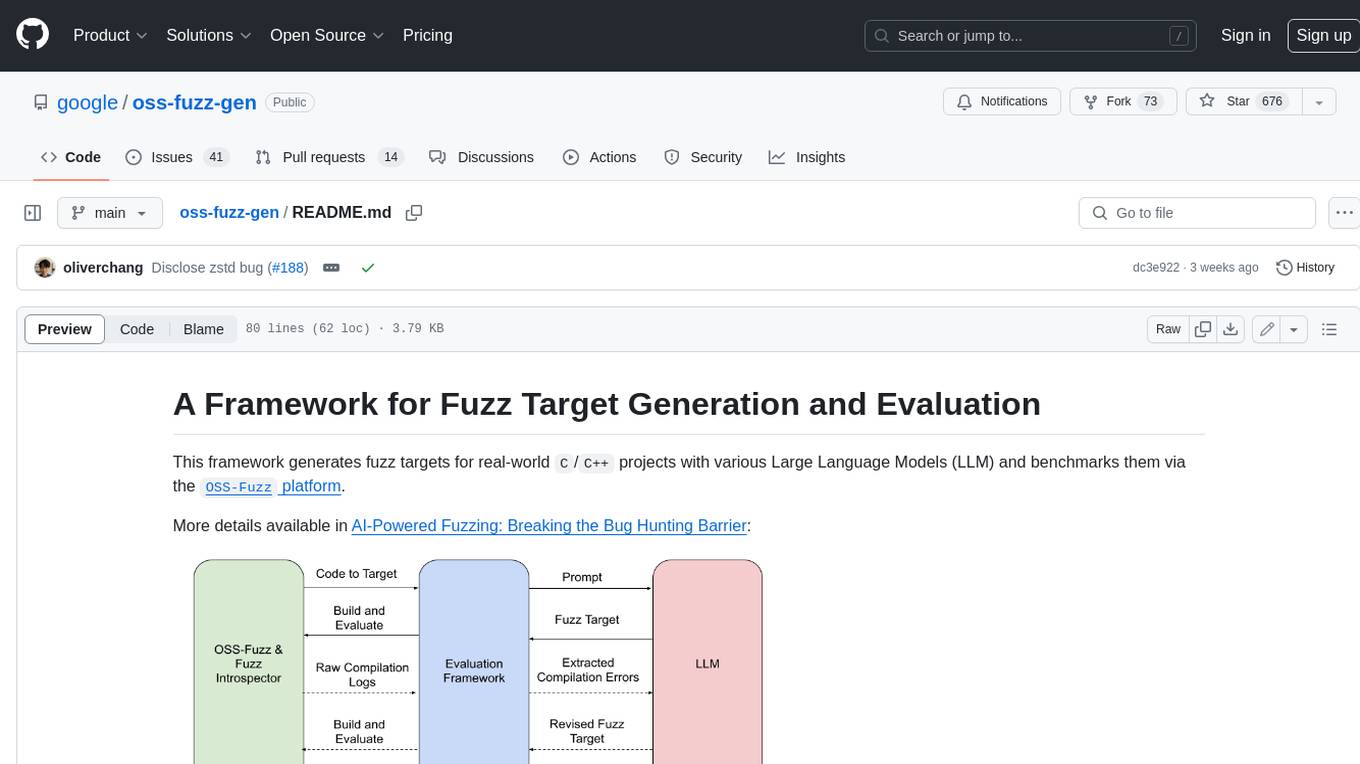
oss-fuzz-gen
This framework generates fuzz targets for real-world `C`/`C++` projects with various Large Language Models (LLM) and benchmarks them via the `OSS-Fuzz` platform. It manages to successfully leverage LLMs to generate valid fuzz targets (which generate non-zero coverage increase) for 160 C/C++ projects. The maximum line coverage increase is 29% from the existing human-written targets.
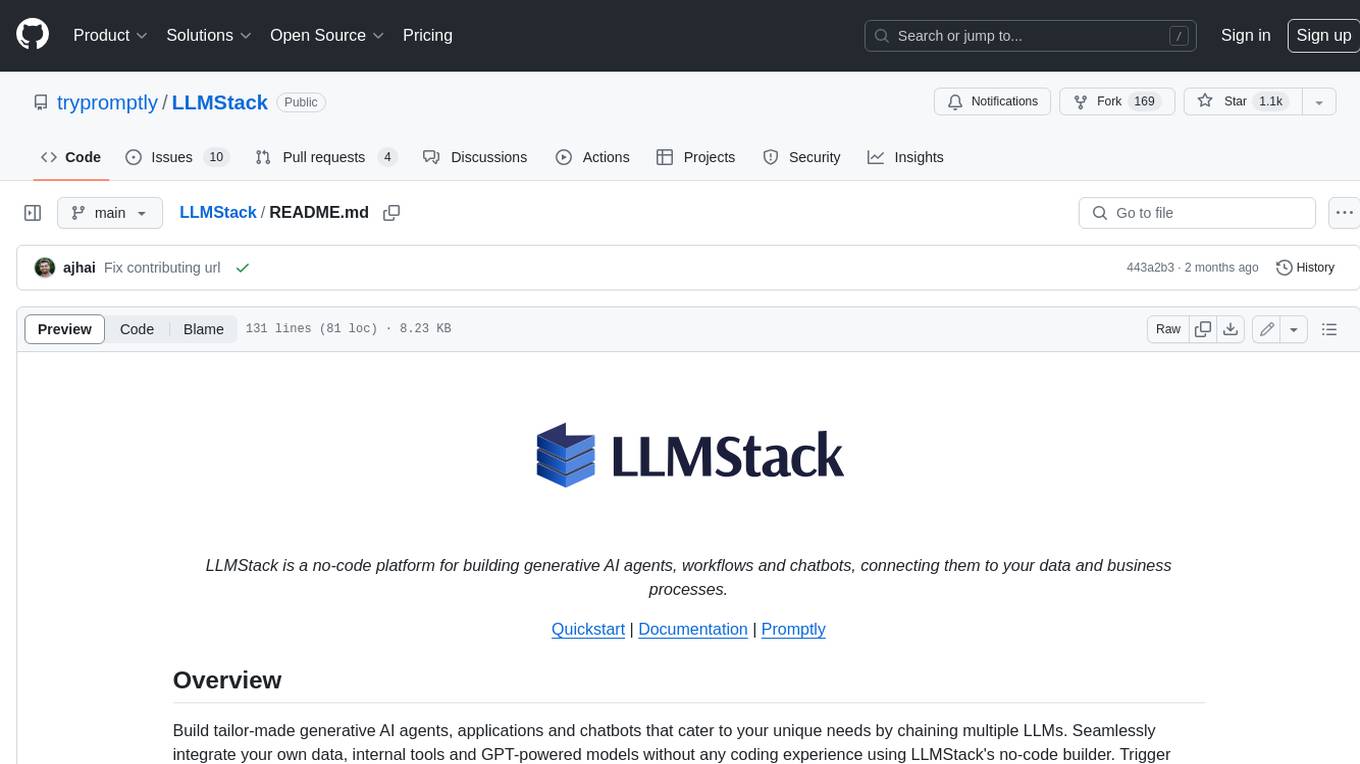
LLMStack
LLMStack is a no-code platform for building generative AI agents, workflows, and chatbots. It allows users to connect their own data, internal tools, and GPT-powered models without any coding experience. LLMStack can be deployed to the cloud or on-premise and can be accessed via HTTP API or triggered from Slack or Discord.
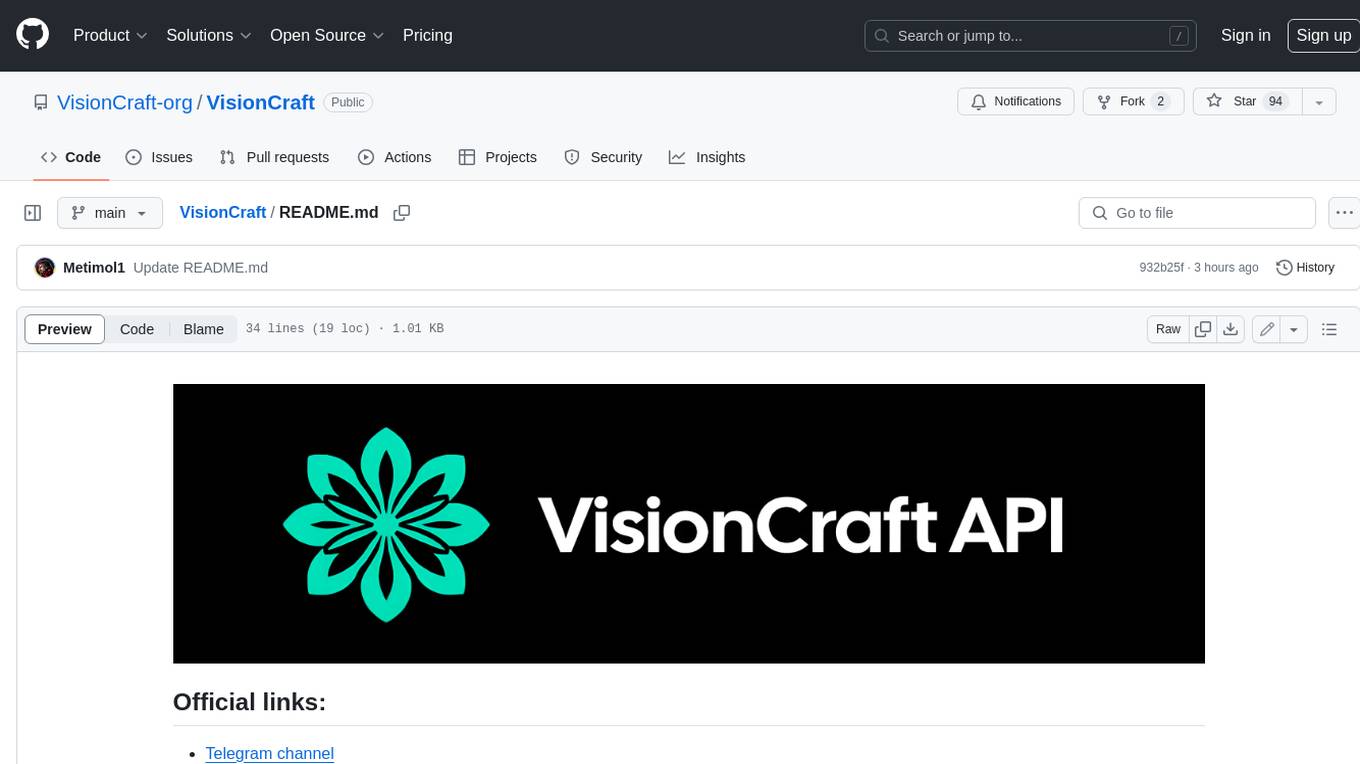
VisionCraft
The VisionCraft API is a free API for using over 100 different AI models. From images to sound.
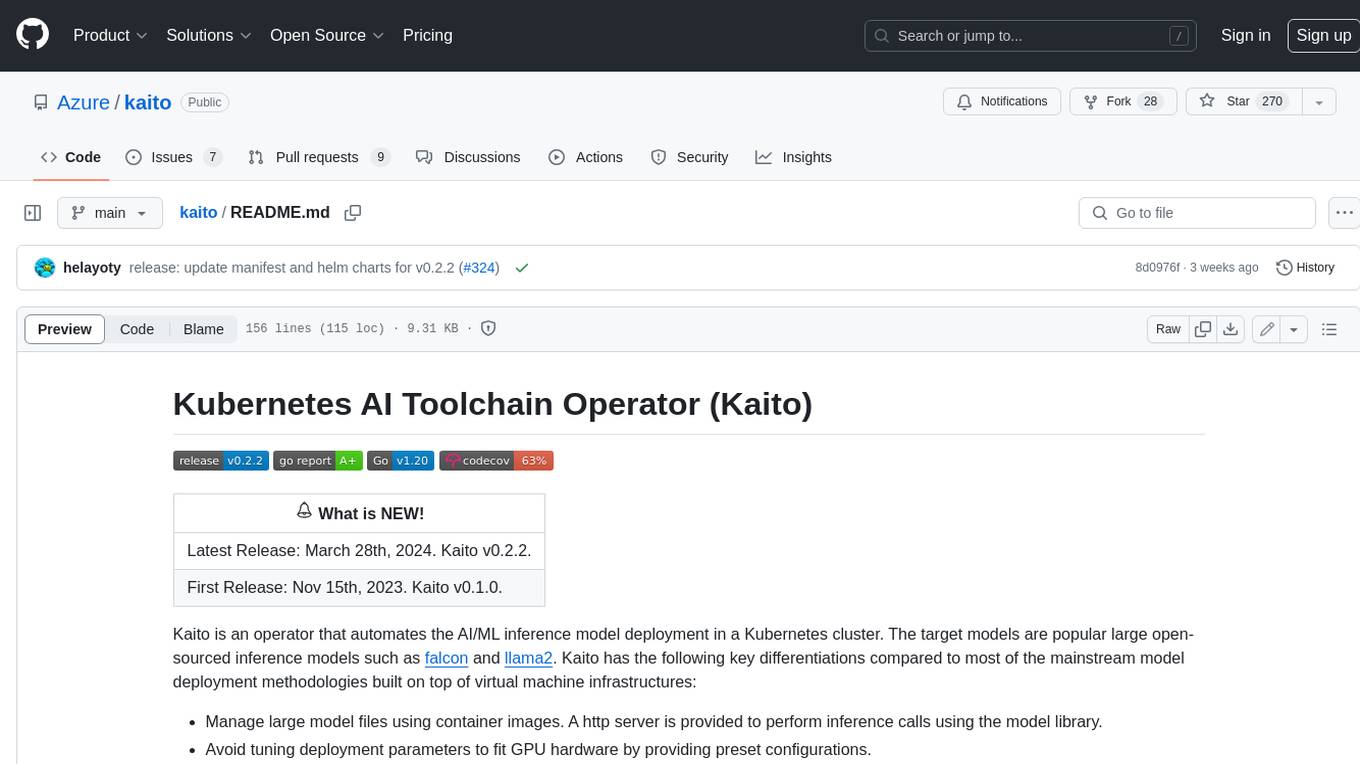
kaito
Kaito is an operator that automates the AI/ML inference model deployment in a Kubernetes cluster. It manages large model files using container images, avoids tuning deployment parameters to fit GPU hardware by providing preset configurations, auto-provisions GPU nodes based on model requirements, and hosts large model images in the public Microsoft Container Registry (MCR) if the license allows. Using Kaito, the workflow of onboarding large AI inference models in Kubernetes is largely simplified.
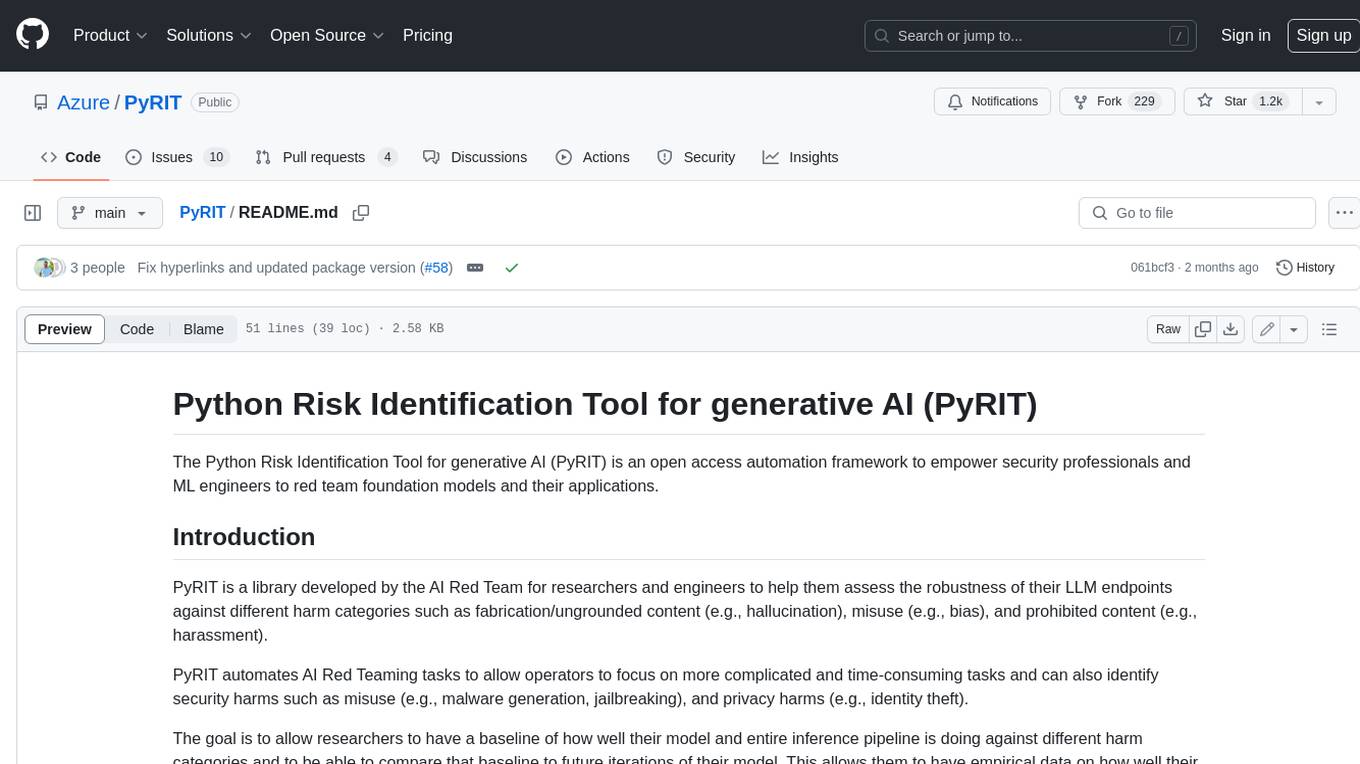
PyRIT
PyRIT is an open access automation framework designed to empower security professionals and ML engineers to red team foundation models and their applications. It automates AI Red Teaming tasks to allow operators to focus on more complicated and time-consuming tasks and can also identify security harms such as misuse (e.g., malware generation, jailbreaking), and privacy harms (e.g., identity theft). The goal is to allow researchers to have a baseline of how well their model and entire inference pipeline is doing against different harm categories and to be able to compare that baseline to future iterations of their model. This allows them to have empirical data on how well their model is doing today, and detect any degradation of performance based on future improvements.
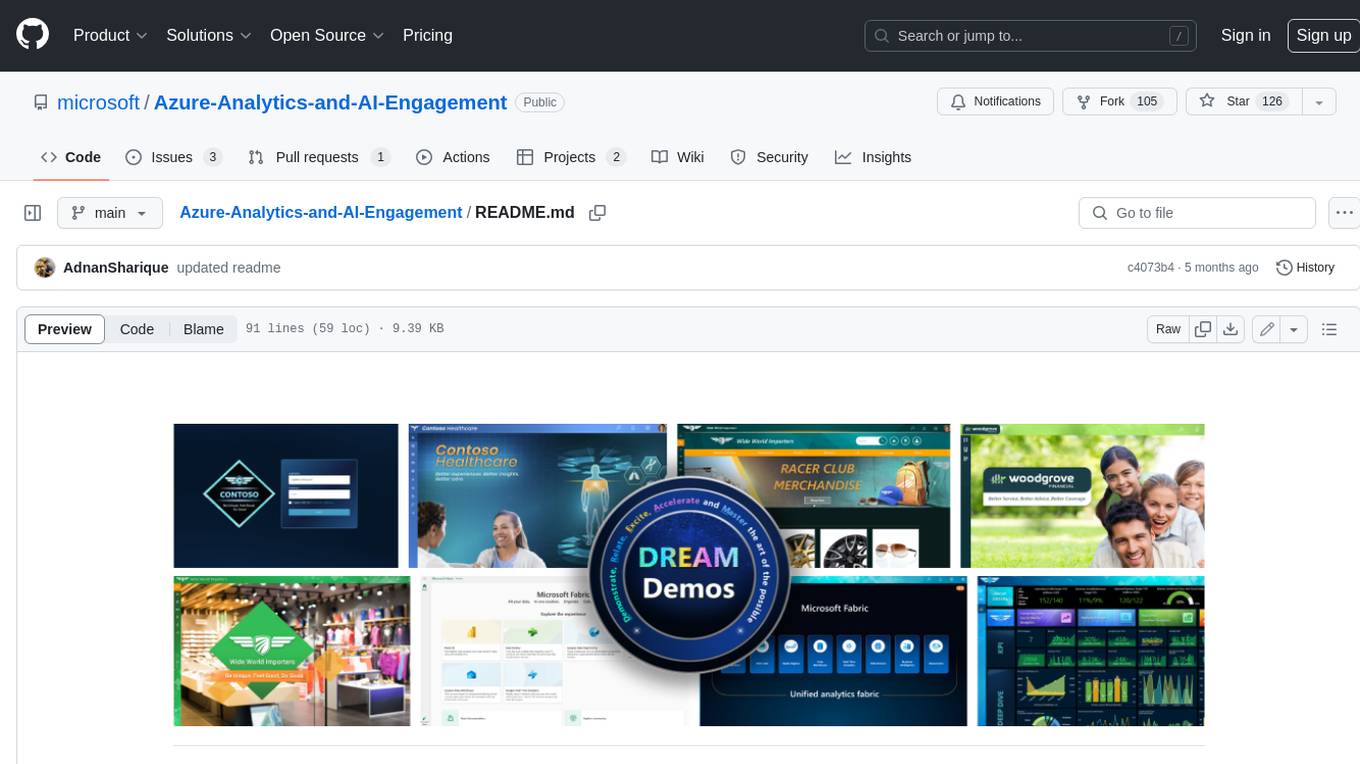
Azure-Analytics-and-AI-Engagement
The Azure-Analytics-and-AI-Engagement repository provides packaged Industry Scenario DREAM Demos with ARM templates (Containing a demo web application, Power BI reports, Synapse resources, AML Notebooks etc.) that can be deployed in a customer’s subscription using the CAPE tool within a matter of few hours. Partners can also deploy DREAM Demos in their own subscriptions using DPoC.