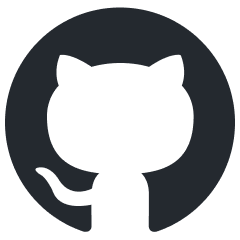
Phi-3-Vision-MLX
Phi-3.5 for Mac: Locally-run Vision and Language Models for Apple Silicon
Stars: 186
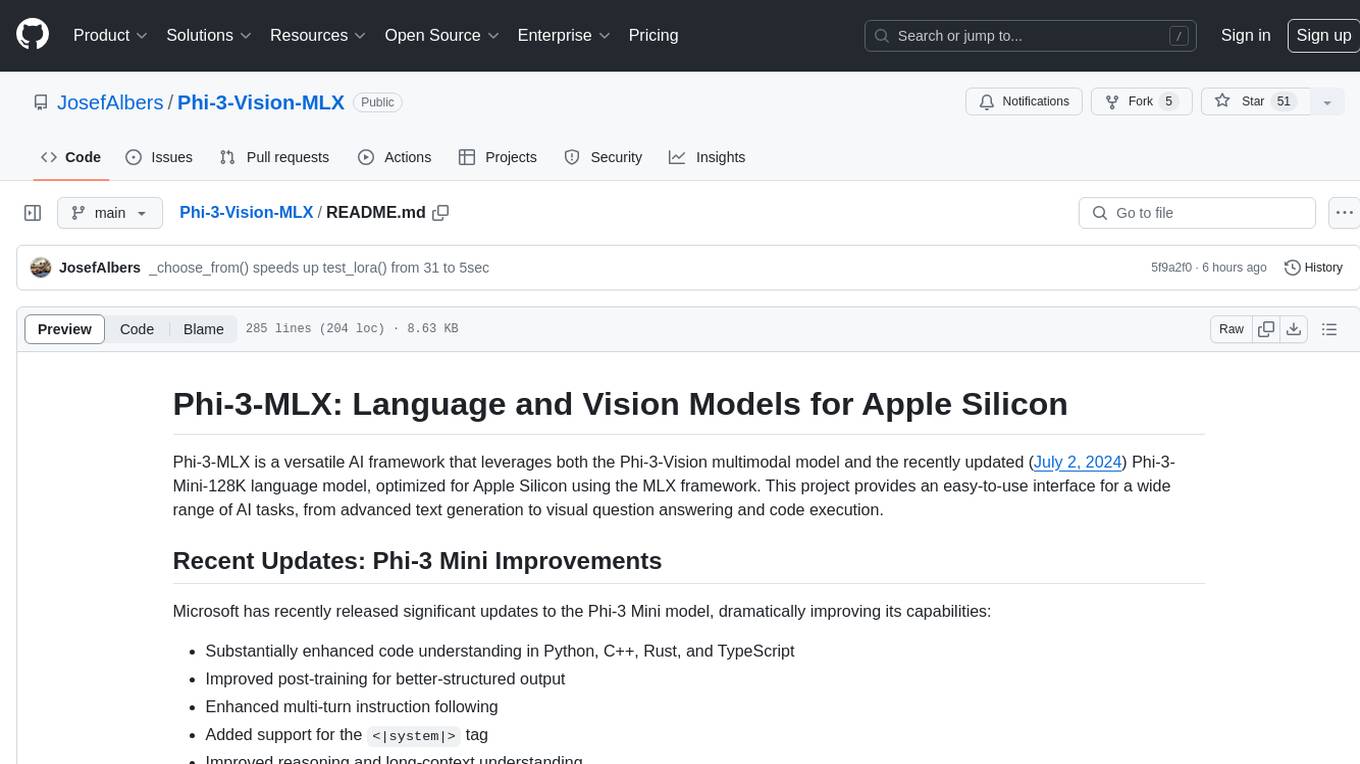
Phi-3-MLX is a versatile AI framework that leverages both the Phi-3-Vision multimodal model and the Phi-3-Mini-128K language model optimized for Apple Silicon using the MLX framework. It provides an easy-to-use interface for a wide range of AI tasks, from advanced text generation to visual question answering and code execution. The project features support for batched generation, flexible agent system, custom toolchains, model quantization, LoRA fine-tuning capabilities, and API integration for extended functionality.
README:
Phi-3-MLX is a versatile AI framework that leverages both the Phi-3-Vision multimodal model and the Phi-3-Mini-128K language model, optimized for Apple Silicon using the MLX framework. This project provides an easy-to-use interface for a wide range of AI tasks, from advanced text generation to visual question answering and code execution.
- Integration with Phi-3.5-vision model
- Support for the Phi-3.5-mini model
- Optimized performance on Apple Silicon using MLX
- Batched generation for processing multiple prompts
- Flexible agent system for various AI tasks
- Custom toolchains for specialized workflows
- Model quantization for improved efficiency
- LoRA fine-tuning capabilities
- API integration for extended functionality (e.g., image generation, text-to-speech)
Phi-3-MLX is designed to run on Apple Silicon Macs. The minimum requirements are:
- Apple Silicon Mac (M1, M2, or later)
- 8GB RAM (with quantization using
quantize_model=True
option)
For optimal performance, especially when working with larger models or datasets, we recommend using a Mac with 16GB RAM or more.
Install and launch Phi-3-MLX from command line:
# Quick install (note: PyPI version may not always be up to date)
pip install phi-3-vision-mlx
phi3v
# For the latest version, you can install directly from the repository:
# git clone https://github.com/JosefAlbers/Phi-3-Vision-MLX.git
# cd Phi-3-Vision-MLX
# pip install -e .
To use the library in a Python script:
from phi_3_vision_mlx import generate
generate('What is shown in this image?', 'https://collectionapi.metmuseum.org/api/collection/v1/iiif/344291/725918/main-image')
# Model quantization
generate("Describe the water cycle.", quantize_model=True)
# Cache quantization
generate("Explain quantum computing.", quantize_cache=True)
# A list of prompts for batch generation
prompts = [
"Write a haiku about spring.",
"Explain the theory of relativity.",
"Describe a futuristic city."
]
# Generate responses using Phi-3-Vision (multimodal model)
generate(prompts, max_tokens=100)
# Generate responses using Phi-3-Mini-128K (language-only model)
generate(prompts, max_tokens=100, blind_model=True)
from phi_3_vision_mlx import constrain
# Use constrain for structured generation (e.g., code, function calls, multiple-choice)
prompts = [
"A 20-year-old woman presents with menorrhagia for the past several years. She says that her menses “have always been heavy”, and she has experienced easy bruising for as long as she can remember. Family history is significant for her mother, who had similar problems with bruising easily. The patient's vital signs include: heart rate 98/min, respiratory rate 14/min, temperature 36.1°C (96.9°F), and blood pressure 110/87 mm Hg. Physical examination is unremarkable. Laboratory tests show the following: platelet count 200,000/mm3, PT 12 seconds, and PTT 43 seconds. Which of the following is the most likely cause of this patient’s symptoms? A: Factor V Leiden B: Hemophilia A C: Lupus anticoagulant D: Protein C deficiency E: Von Willebrand disease",
"A 25-year-old primigravida presents to her physician for a routine prenatal visit. She is at 34 weeks gestation, as confirmed by an ultrasound examination. She has no complaints, but notes that the new shoes she bought 2 weeks ago do not fit anymore. The course of her pregnancy has been uneventful and she has been compliant with the recommended prenatal care. Her medical history is unremarkable. She has a 15-pound weight gain since the last visit 3 weeks ago. Her vital signs are as follows: blood pressure, 148/90 mm Hg; heart rate, 88/min; respiratory rate, 16/min; and temperature, 36.6℃ (97.9℉). The blood pressure on repeat assessment 4 hours later is 151/90 mm Hg. The fetal heart rate is 151/min. The physical examination is significant for 2+ pitting edema of the lower extremity. Which of the following tests o should confirm the probable condition of this patient? A: Bilirubin assessment B: Coagulation studies C: Hematocrit assessment D: Leukocyte count with differential E: 24-hour urine protein"
]
# Define constraints for the generated text
constraints = [(0, '\nThe'), (100, ' The correct answer is'), (1, 'X.')]
# Apply constrained beam decoding
results = constrain(prompts, constraints, blind_model=True, quantize_model=True, use_beam=True)
from phi_3_vision_mlx import choose
# Select best option from choices for given prompts
prompts = [
"What is the largest planet in our solar system? A: Earth B: Mars C: Jupiter D: Saturn",
"Which element has the chemical symbol 'O'? A: Osmium B: Oxygen C: Gold D: Silver"
]
# For multiple-choice or decision-making tasks
choose(prompts, choices='ABCDE')
from phi_3_vision_mlx import train_lora, test_lora
# Train a LoRA adapter
train_lora(
lora_layers=5, # Number of layers to apply LoRA
lora_rank=16, # Rank of the LoRA adaptation
epochs=10, # Number of training epochs
lr=1e-4, # Learning rate
warmup=0.5, # Fraction of steps for learning rate warmup
dataset_path="JosefAlbers/akemiH_MedQA_Reason"
)
# Generate text using the trained LoRA adapter
generate("Describe the potential applications of CRISPR gene editing in medicine.",
blind_model=True,
quantize_model=True,
use_adapter=True)
# Test the performance of the trained LoRA adapter
test_lora()
from phi_3_vision_mlx import Agent
# Create an instance of the Agent
agent = Agent()
# First interaction: Analyze an image
agent('Analyze this image and describe the architectural style:', 'https://images.metmuseum.org/CRDImages/rl/original/DP-19531-075.jpg')
# Second interaction: Follow-up question
agent('What historical period does this architecture likely belong to?')
# End conversation, clear memory for new interaction
agent.end()
# Ask the agent to generate and execute code to create a plot
agent('Plot a Lissajous Curve.')
# Ask the agent to modify the generated code and create a new plot
agent('Modify the code to plot 3:4 frequency')
agent.end()
# Request the agent to generate an image
agent('Draw "A perfectly red apple, 32k HDR, studio lighting"')
agent.end()
# Request the agent to convert text to speech
agent('Speak "People say nothing is impossible, but I do nothing every day."')
agent.end()
from phi_3_vision_mlx import add_text
# Define the toolchain as a string
toolchain = """
prompt = add_text(prompt)
responses = generate(prompt, images)
"""
# Create an Agent instance with the custom toolchain
agent = Agent(toolchain, early_stop=100)
# Run the agent
agent('How to inspect API endpoints? @https://raw.githubusercontent.com/gradio-app/gradio/main/guides/08_gradio-clients-and-lite/01_getting-started-with-the-python-client.md')
from phi_3_vision_mlx import VDB
import datasets
# Simulate user input
user_input = 'Comparison of Sortino Ratio for Bitcoin and Ethereum.'
# Create a custom RAG tool
def rag(prompt, repo_id="JosefAlbers/sharegpt_python_mlx", n_topk=1):
ds = datasets.load_dataset(repo_id, split='train')
vdb = VDB(ds)
context = vdb(prompt, n_topk)[0][0]
return f'{context}\n<|end|>\n<|user|>\nPlot: {prompt}'
# Define the toolchain
toolchain_plot = """
prompt = rag(prompt)
responses = generate(prompt, images)
files = execute(responses, step)
"""
# Create an Agent instance with the RAG toolchain
agent = Agent(toolchain_plot, False)
# Run the agent with the user input
_, images = agent(user_input)
# Continued from Example 2 above
agent_writer = Agent(early_stop=100)
agent_writer(f'Write a stock analysis report on: {user_input}', images)
# Create Agent with Mistral-7B-Instruct-v0.3 instead
agent = Agent(toolchain = "responses, history = mistral_api(prompt, history)")
# Generate a neurology ICU admission note
agent('Write a neurology ICU admission note.')
# Follow-up questions (multi-turn conversation)
agent('Give me the inpatient BP goal for this patient.')
agent('DVT ppx for this patient?')
agent("Patient's prognosis?")
# End
agent.end()
from phi_3_vision_mlx import benchmark
benchmark()
Task | Vanilla Model | Quantized Model | Quantized Cache | LoRA Adapter |
---|---|---|---|---|
Text Generation | 25.02 tps | 61.01 tps | 18.68 tps | 24.72 tps |
Image Captioning | 21.29 tps | 44.26 tps | 5.56 tps | 20.48 tps |
Batched Generation | 236.60 tps | 149.23 tps | 121.92 tps | 232.78 tps |
(On M1 Max 64GB)
API references and additional information are available at:
https://josefalbers.github.io/Phi-3-Vision-MLX/
Also check out our tutorial series available at:
This project is licensed under the MIT License.
For Tasks:
Click tags to check more tools for each tasksFor Jobs:
Alternative AI tools for Phi-3-Vision-MLX
Similar Open Source Tools
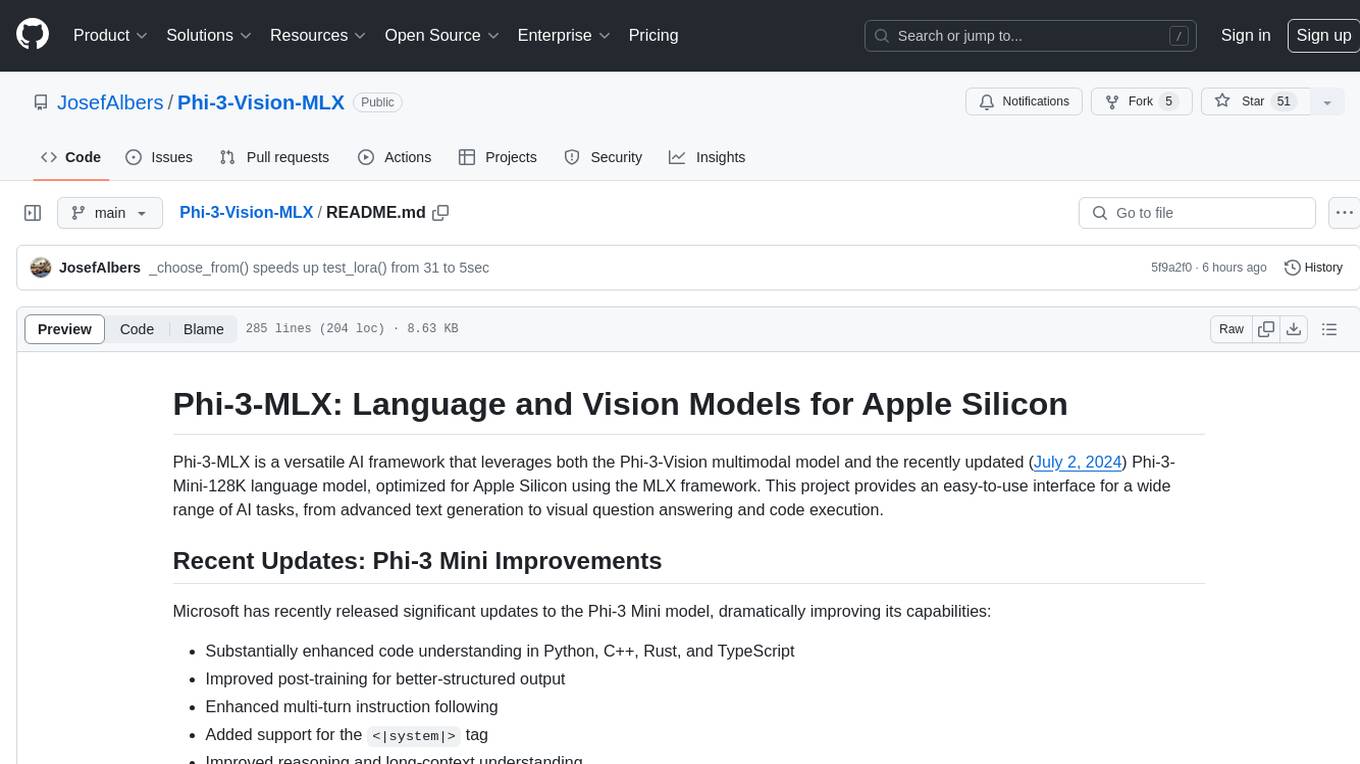
Phi-3-Vision-MLX
Phi-3-MLX is a versatile AI framework that leverages both the Phi-3-Vision multimodal model and the Phi-3-Mini-128K language model optimized for Apple Silicon using the MLX framework. It provides an easy-to-use interface for a wide range of AI tasks, from advanced text generation to visual question answering and code execution. The project features support for batched generation, flexible agent system, custom toolchains, model quantization, LoRA fine-tuning capabilities, and API integration for extended functionality.
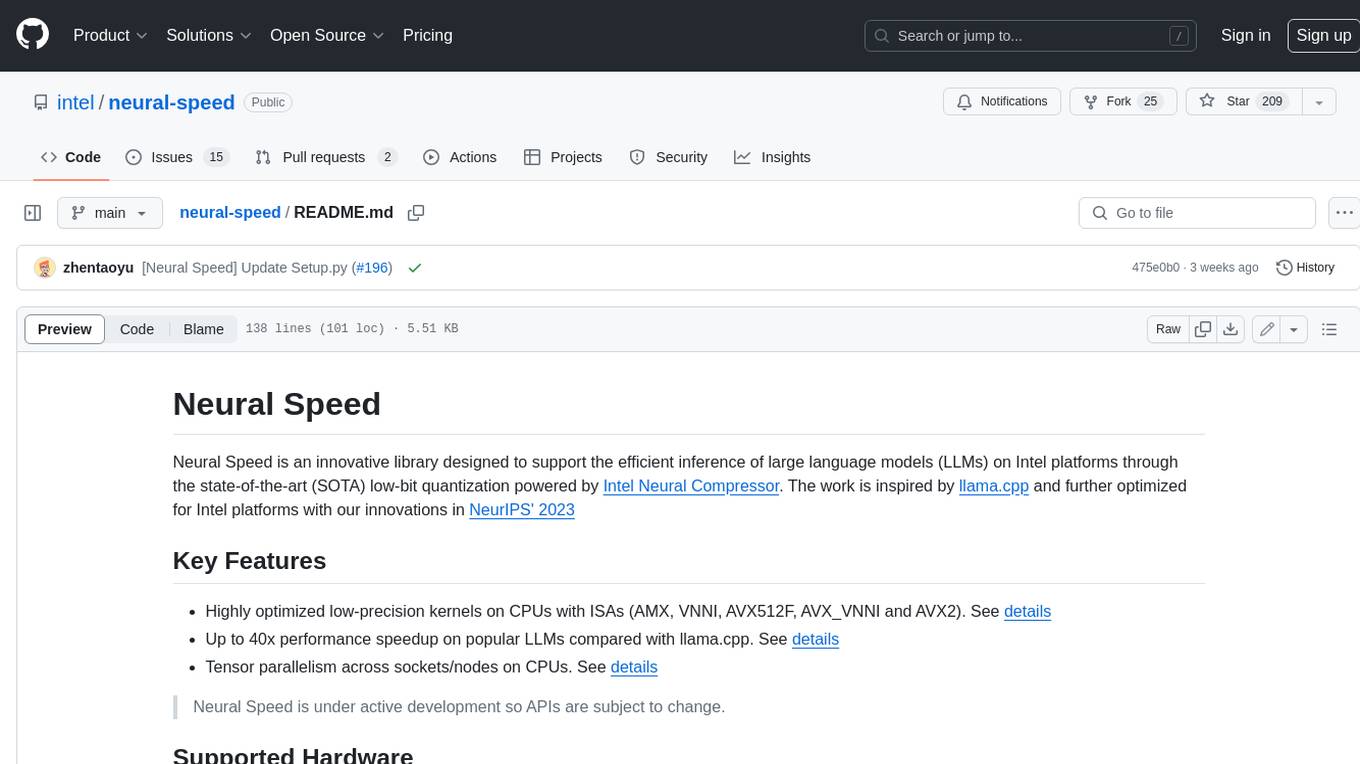
neural-speed
Neural Speed is an innovative library designed to support the efficient inference of large language models (LLMs) on Intel platforms through the state-of-the-art (SOTA) low-bit quantization powered by Intel Neural Compressor. The work is inspired by llama.cpp and further optimized for Intel platforms with our innovations in NeurIPS' 2023
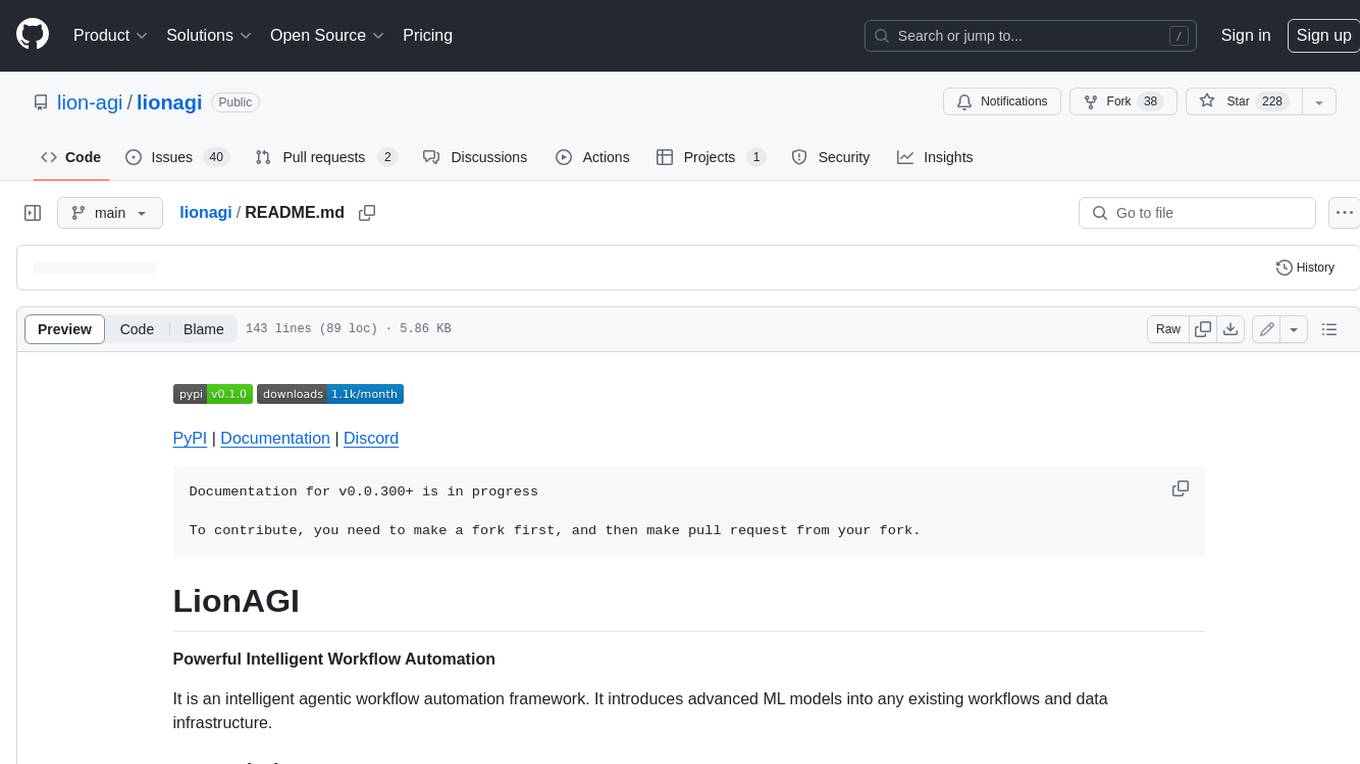
lionagi
LionAGI is a powerful intelligent workflow automation framework that introduces advanced ML models into any existing workflows and data infrastructure. It can interact with almost any model, run interactions in parallel for most models, produce structured pydantic outputs with flexible usage, automate workflow via graph based agents, use advanced prompting techniques, and more. LionAGI aims to provide a centralized agent-managed framework for "ML-powered tools coordination" and to dramatically lower the barrier of entries for creating use-case/domain specific tools. It is designed to be asynchronous only and requires Python 3.10 or higher.
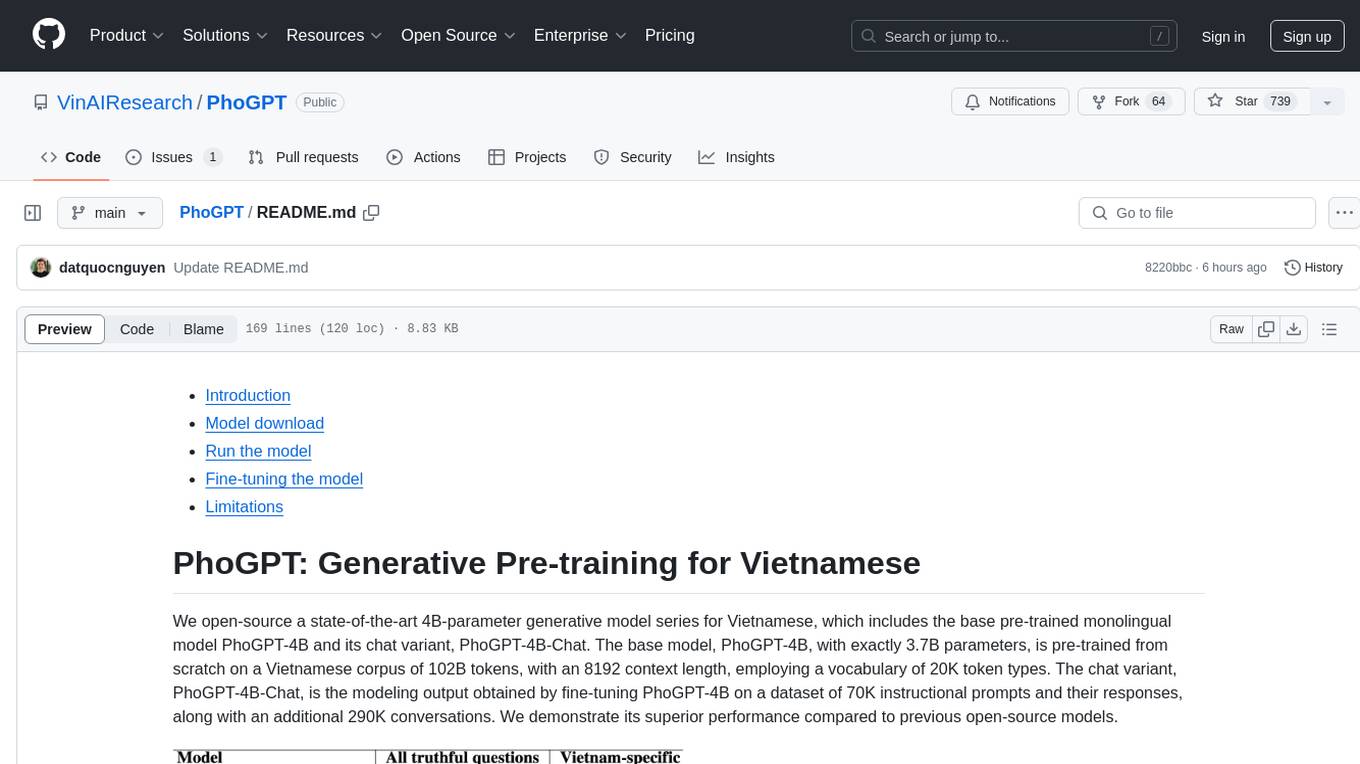
PhoGPT
PhoGPT is an open-source 4B-parameter generative model series for Vietnamese, including the base pre-trained monolingual model PhoGPT-4B and its chat variant, PhoGPT-4B-Chat. PhoGPT-4B is pre-trained from scratch on a Vietnamese corpus of 102B tokens, with an 8192 context length and a vocabulary of 20K token types. PhoGPT-4B-Chat is fine-tuned on instructional prompts and conversations, demonstrating superior performance. Users can run the model with inference engines like vLLM and Text Generation Inference, and fine-tune it using llm-foundry. However, PhoGPT has limitations in reasoning, coding, and mathematics tasks, and may generate harmful or biased responses.
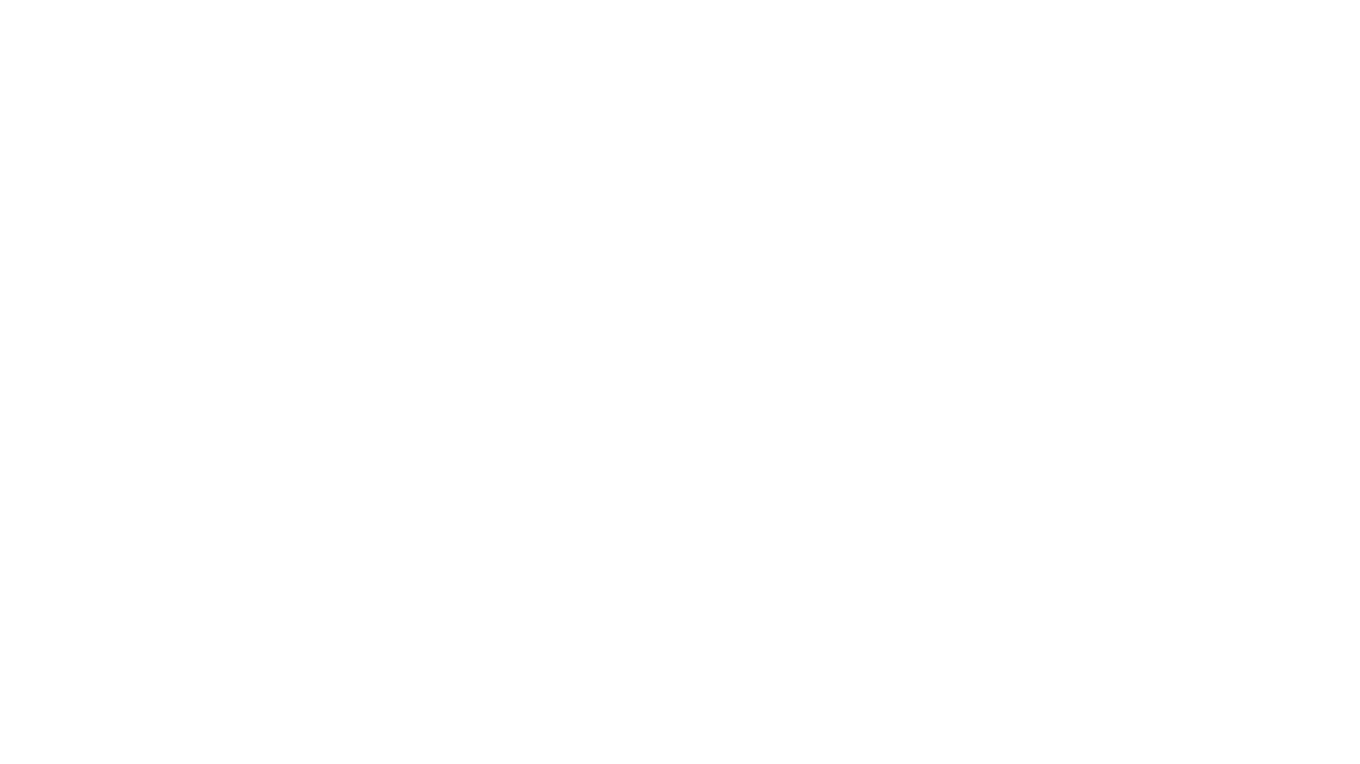
fastrtc
FastRTC is a real-time communication library for Python that allows users to turn any Python function into a real-time audio and video stream over WebRTC or WebSockets. It provides features like automatic voice detection, UI launching, WebRTC support, WebSocket support, telephone support, and customizable backend for production applications. The library offers various examples and usage scenarios for audio and video streaming, object detection, voice APIs, chat applications, and more.
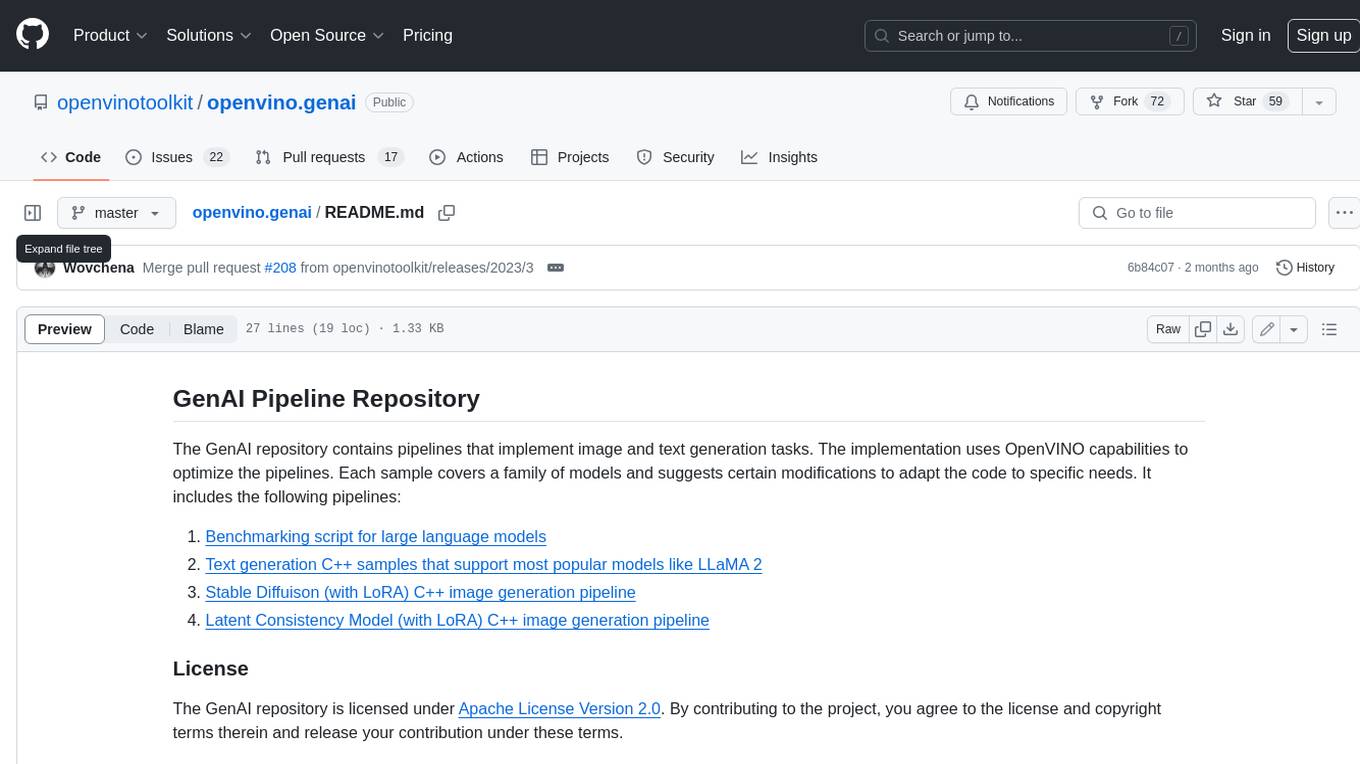
openvino.genai
The GenAI repository contains pipelines that implement image and text generation tasks. The implementation uses OpenVINO capabilities to optimize the pipelines. Each sample covers a family of models and suggests certain modifications to adapt the code to specific needs. It includes the following pipelines: 1. Benchmarking script for large language models 2. Text generation C++ samples that support most popular models like LLaMA 2 3. Stable Diffuison (with LoRA) C++ image generation pipeline 4. Latent Consistency Model (with LoRA) C++ image generation pipeline
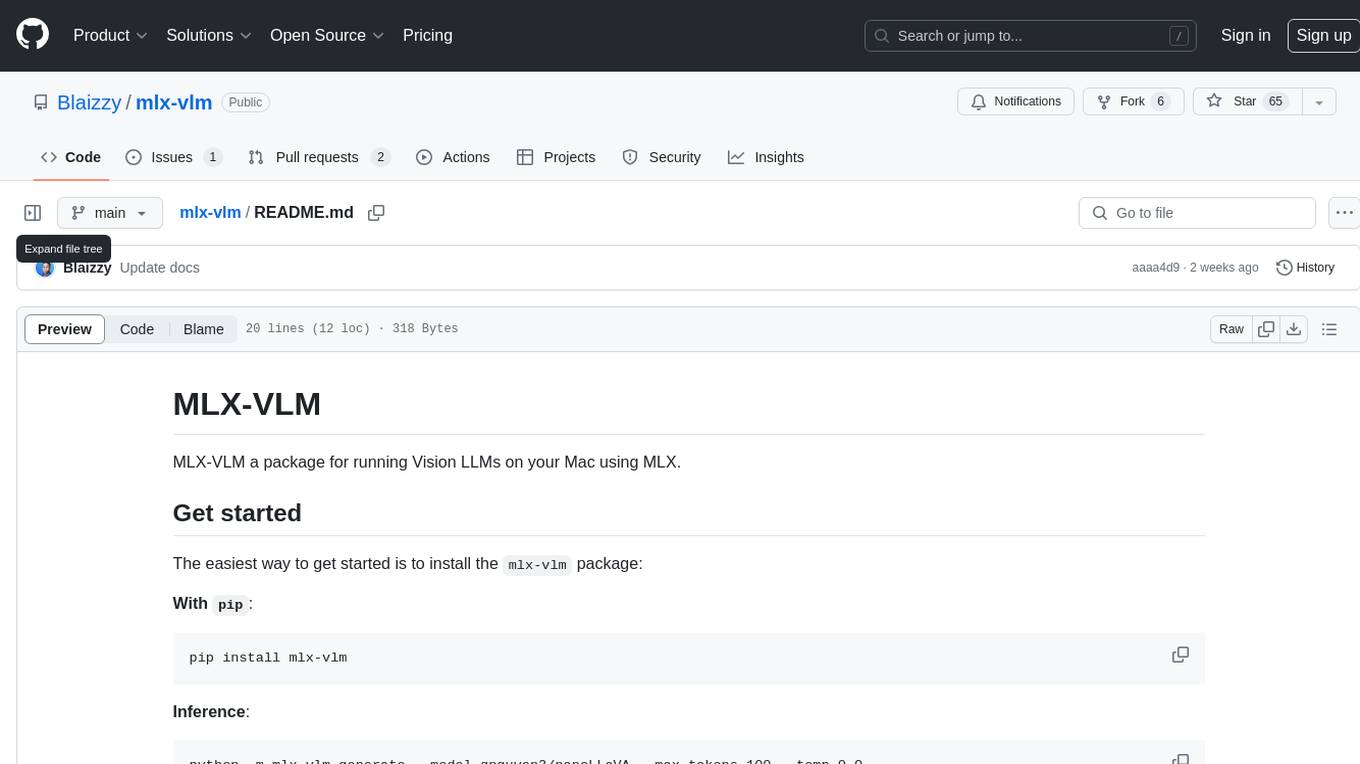
mlx-vlm
MLX-VLM is a package designed for running Vision LLMs on Mac systems using MLX. It provides a convenient way to install and utilize the package for processing large language models related to vision tasks. The tool simplifies the process of running LLMs on Mac computers, offering a seamless experience for users interested in leveraging MLX for vision-related projects.
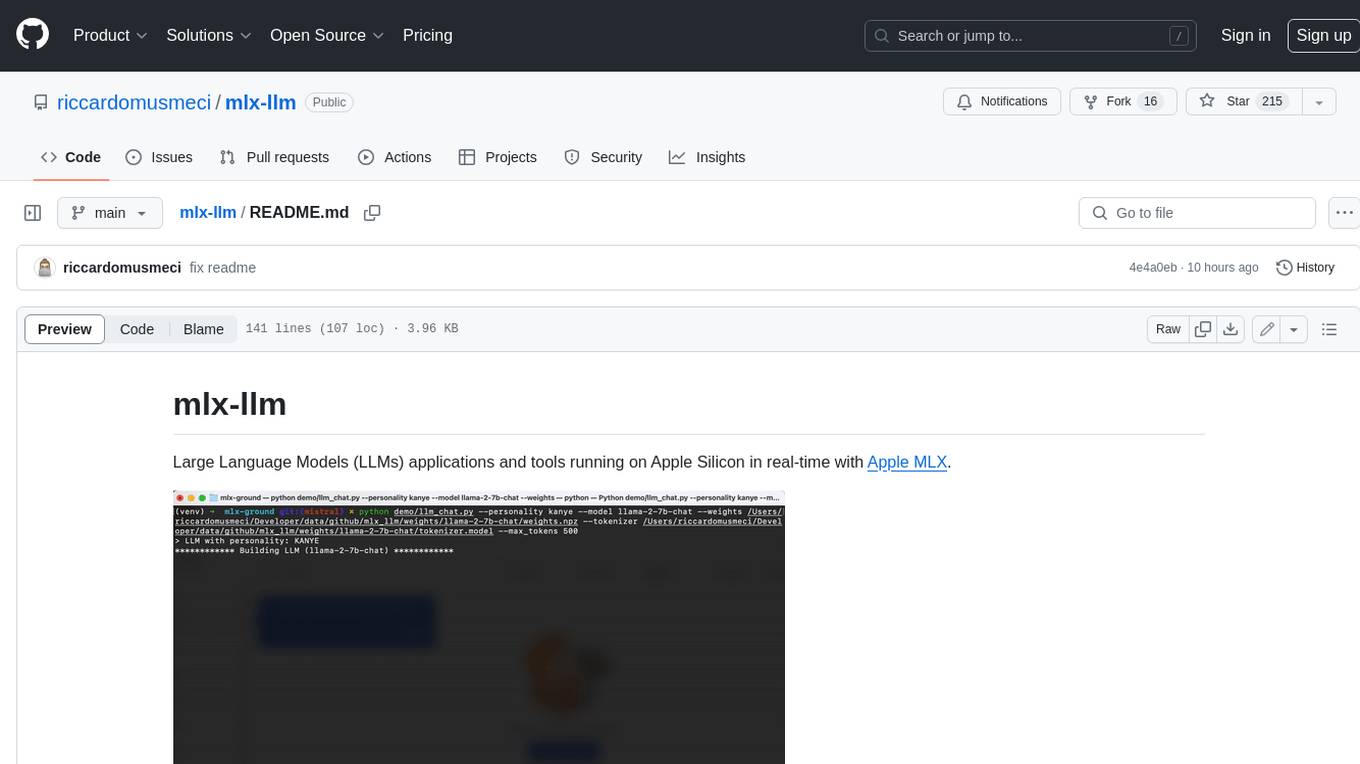
mlx-llm
mlx-llm is a library that allows you to run Large Language Models (LLMs) on Apple Silicon devices in real-time using Apple's MLX framework. It provides a simple and easy-to-use API for creating, loading, and using LLM models, as well as a variety of applications such as chatbots, fine-tuning, and retrieval-augmented generation.
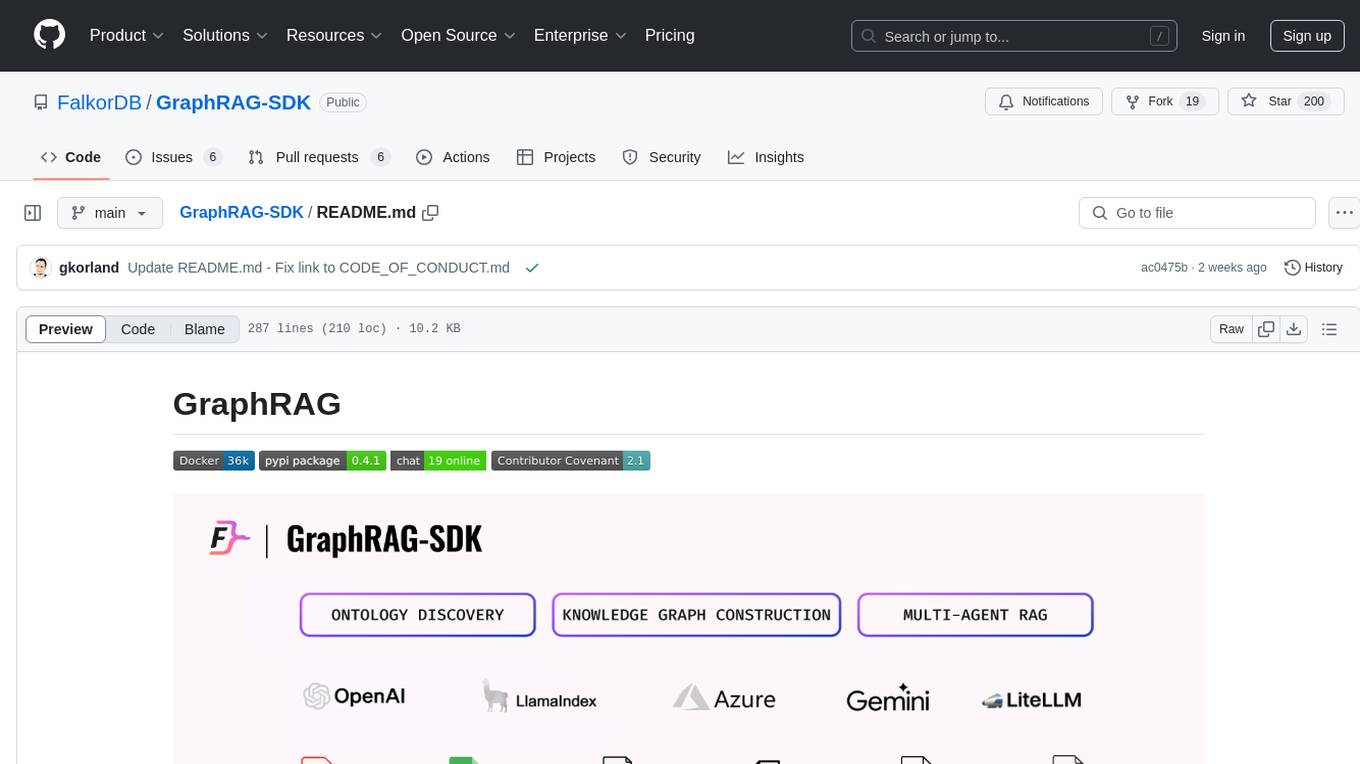
GraphRAG-SDK
Build fast and accurate GenAI applications with GraphRAG SDK, a specialized toolkit for building Graph Retrieval-Augmented Generation (GraphRAG) systems. It integrates knowledge graphs, ontology management, and state-of-the-art LLMs to deliver accurate, efficient, and customizable RAG workflows. The SDK simplifies the development process by automating ontology creation, knowledge graph agent creation, and query handling, enabling users to interact and query their knowledge graphs effectively. It supports multi-agent systems and orchestrates agents specialized in different domains. The SDK is optimized for FalkorDB, ensuring high performance and scalability for large-scale applications. By leveraging knowledge graphs, it enables semantic relationships and ontology-driven queries that go beyond standard vector similarity, enhancing retrieval-augmented generation capabilities.
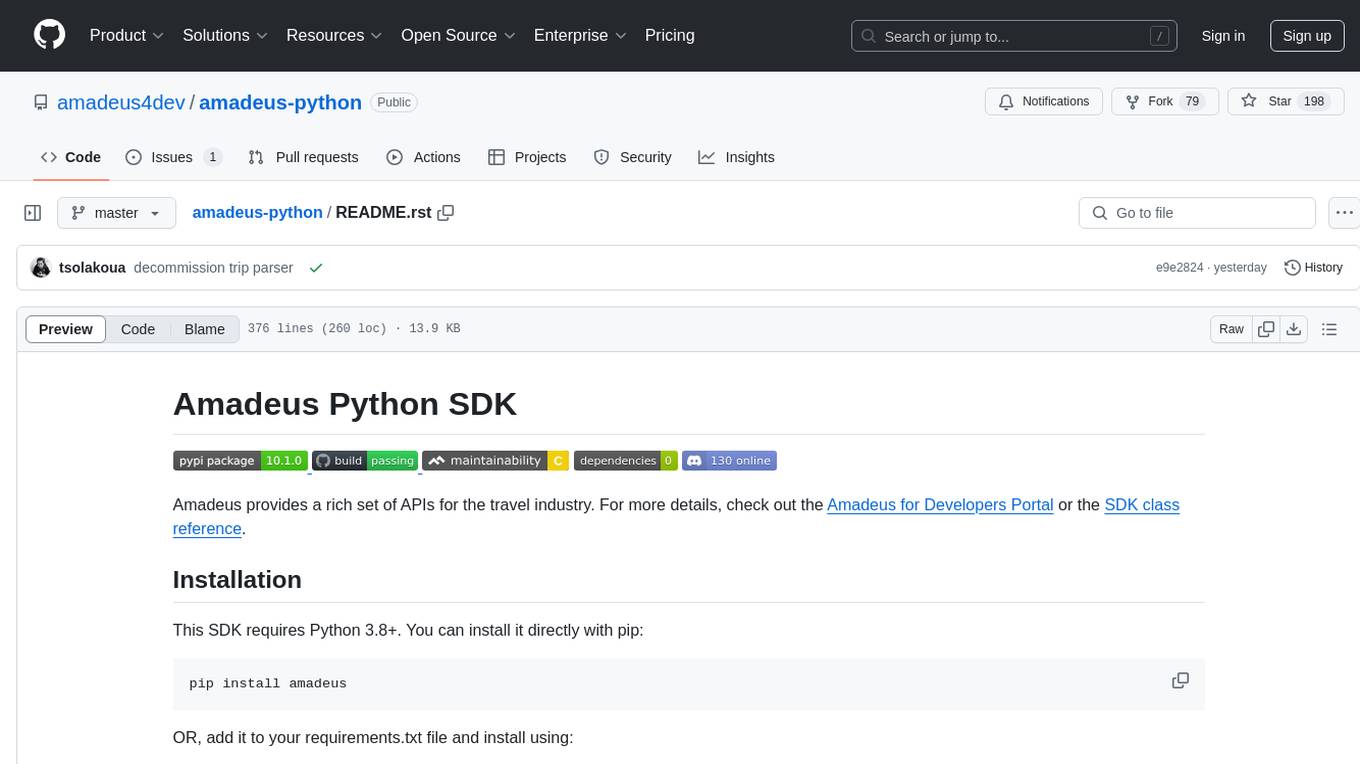
amadeus-python
Amadeus Python SDK provides a rich set of APIs for the travel industry. It allows users to make API calls for various travel-related tasks such as flight offers search, hotel bookings, trip purpose prediction, flight delay prediction, airport on-time performance, travel recommendations, and more. The SDK conveniently maps API paths to similar paths, making it easy to interact with the Amadeus APIs. Users can initialize the client with their API key and secret, make API calls, handle responses, and enable logging for debugging purposes. The SDK documentation includes detailed information about each SDK method, arguments, and return types.
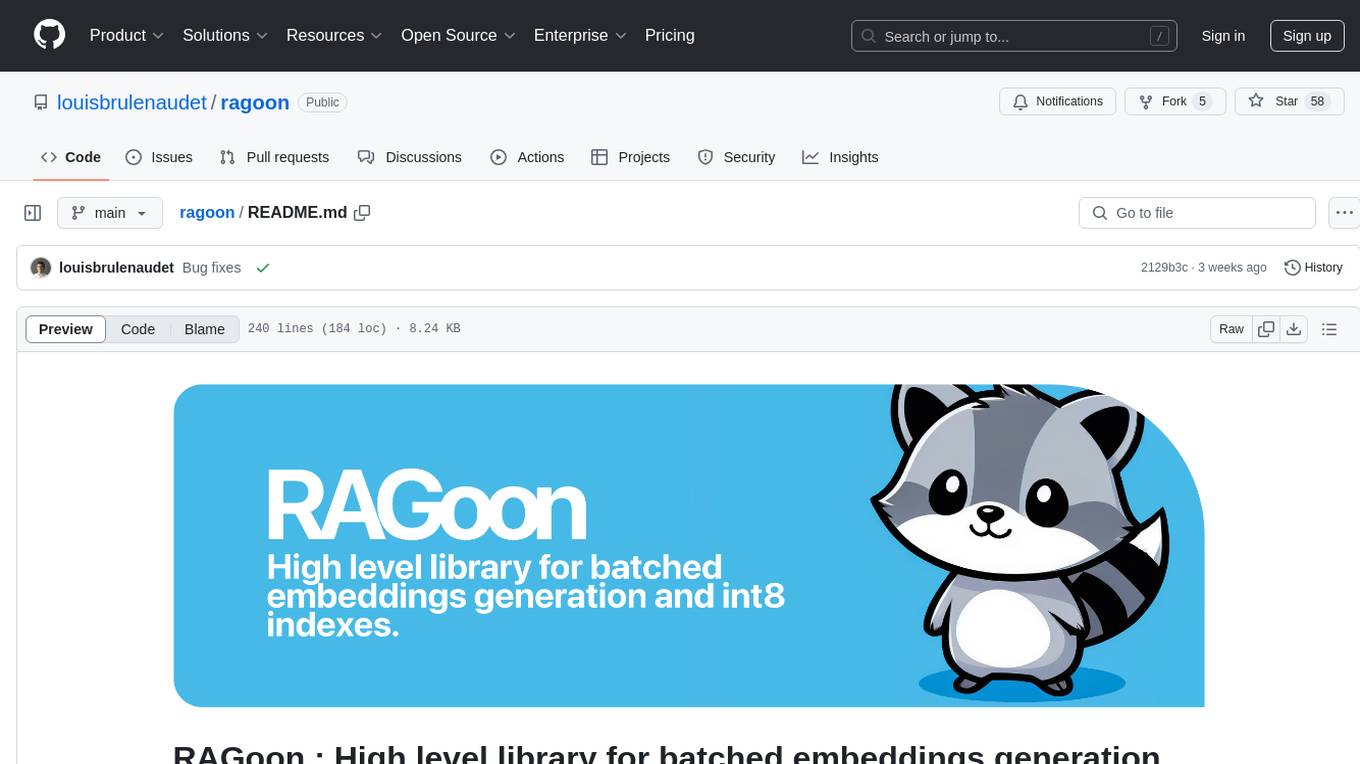
ragoon
RAGoon is a high-level library designed for batched embeddings generation, fast web-based RAG (Retrieval-Augmented Generation) processing, and quantized indexes processing. It provides NLP utilities for multi-model embedding production, high-dimensional vector visualization, and enhancing language model performance through search-based querying, web scraping, and data augmentation techniques.
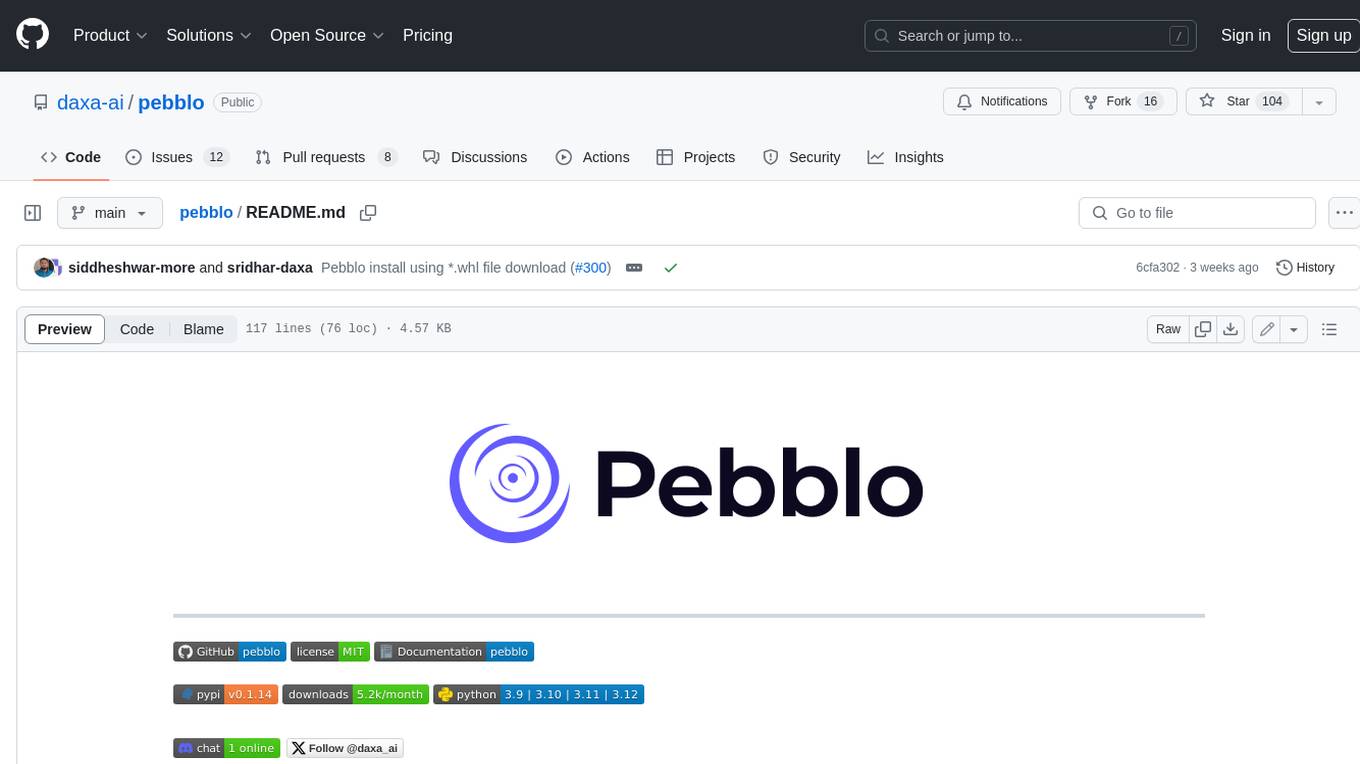
pebblo
Pebblo enables developers to safely load data and promote their Gen AI app to deployment without worrying about the organization’s compliance and security requirements. The project identifies semantic topics and entities found in the loaded data and summarizes them on the UI or a PDF report.
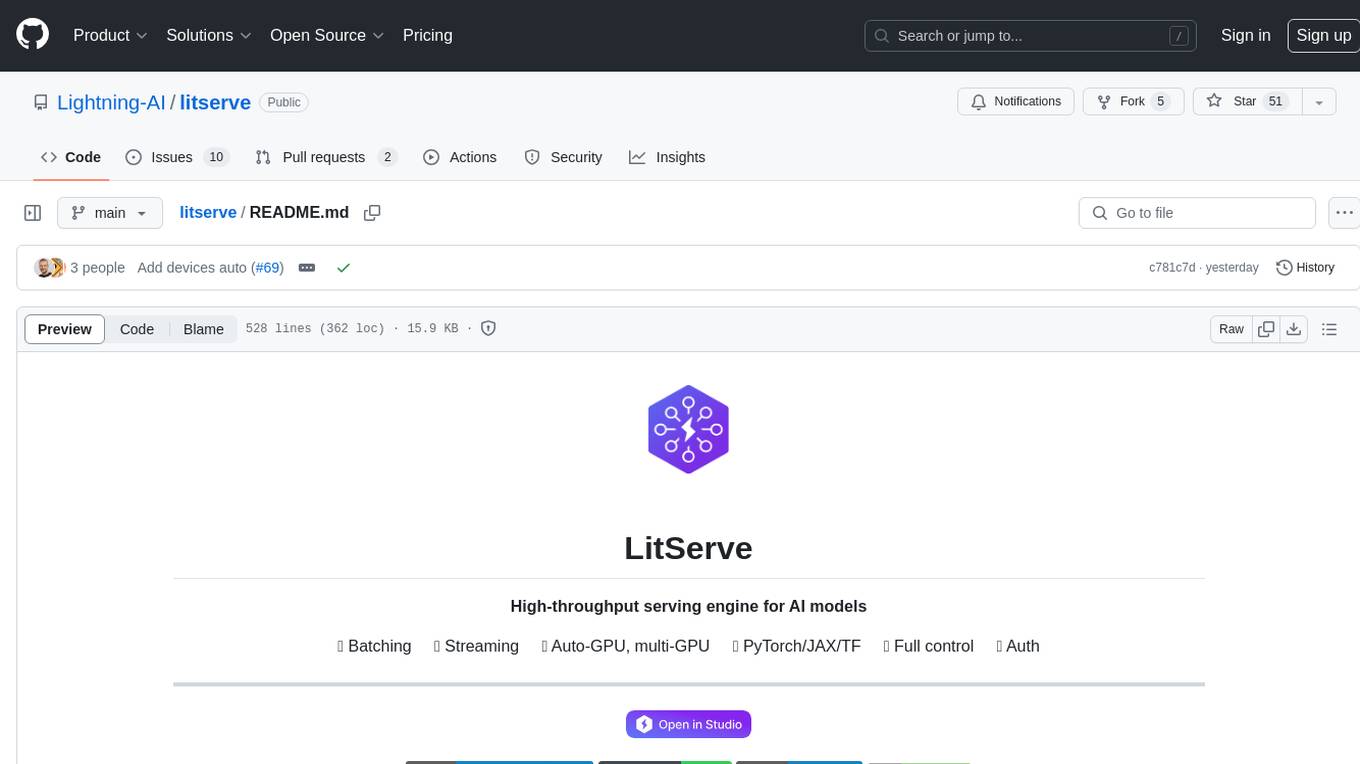
litserve
LitServe is a high-throughput serving engine for deploying AI models at scale. It generates an API endpoint for a model, handles batching, streaming, autoscaling across CPU/GPUs, and more. Built for enterprise scale, it supports every framework like PyTorch, JAX, Tensorflow, and more. LitServe is designed to let users focus on model performance, not the serving boilerplate. It is like PyTorch Lightning for model serving but with broader framework support and scalability.
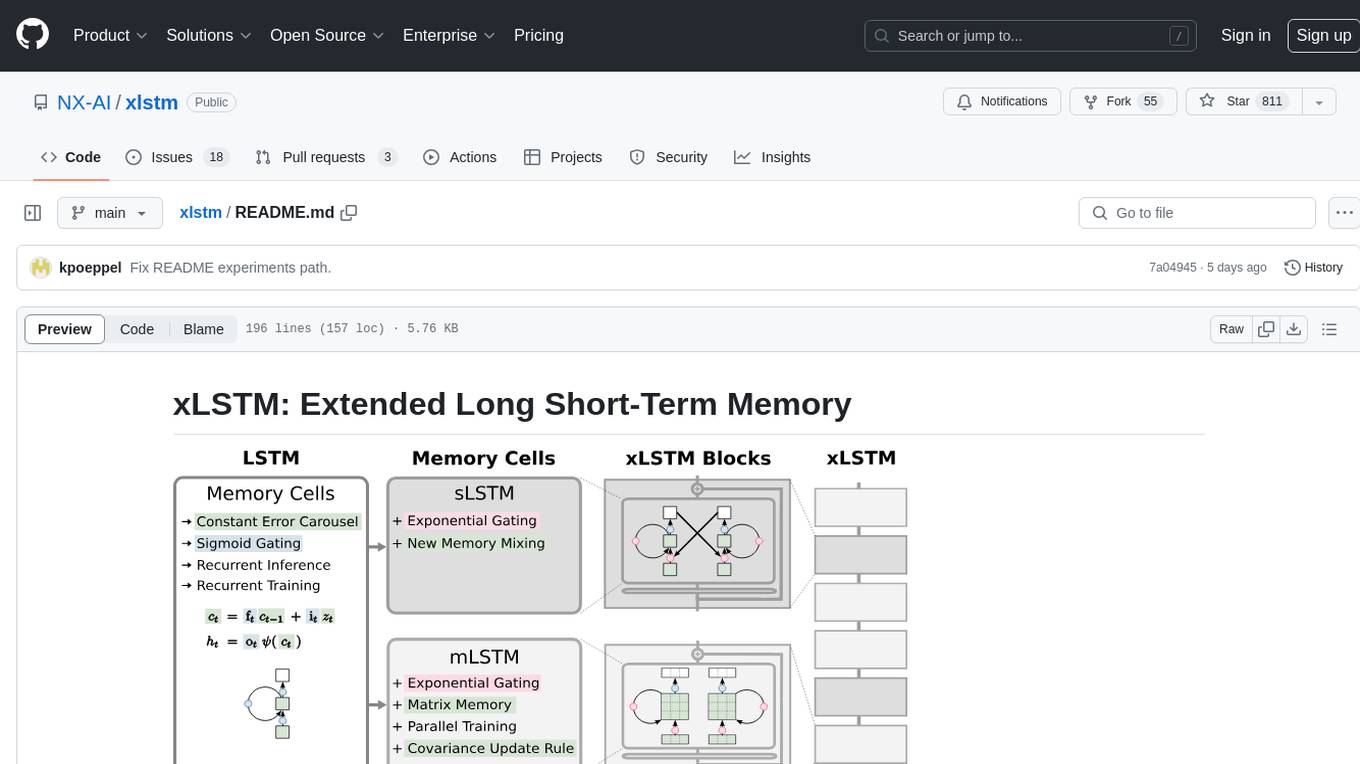
xlstm
xLSTM is a new Recurrent Neural Network architecture based on ideas of the original LSTM. Through Exponential Gating with appropriate normalization and stabilization techniques and a new Matrix Memory it overcomes the limitations of the original LSTM and shows promising performance on Language Modeling when compared to Transformers or State Space Models. The package is based on PyTorch and was tested for versions >=1.8. For the CUDA version of xLSTM, you need Compute Capability >= 8.0. The xLSTM tool provides two main components: xLSTMBlockStack for non-language applications or integrating in other architectures, and xLSTMLMModel for language modeling or other token-based applications.
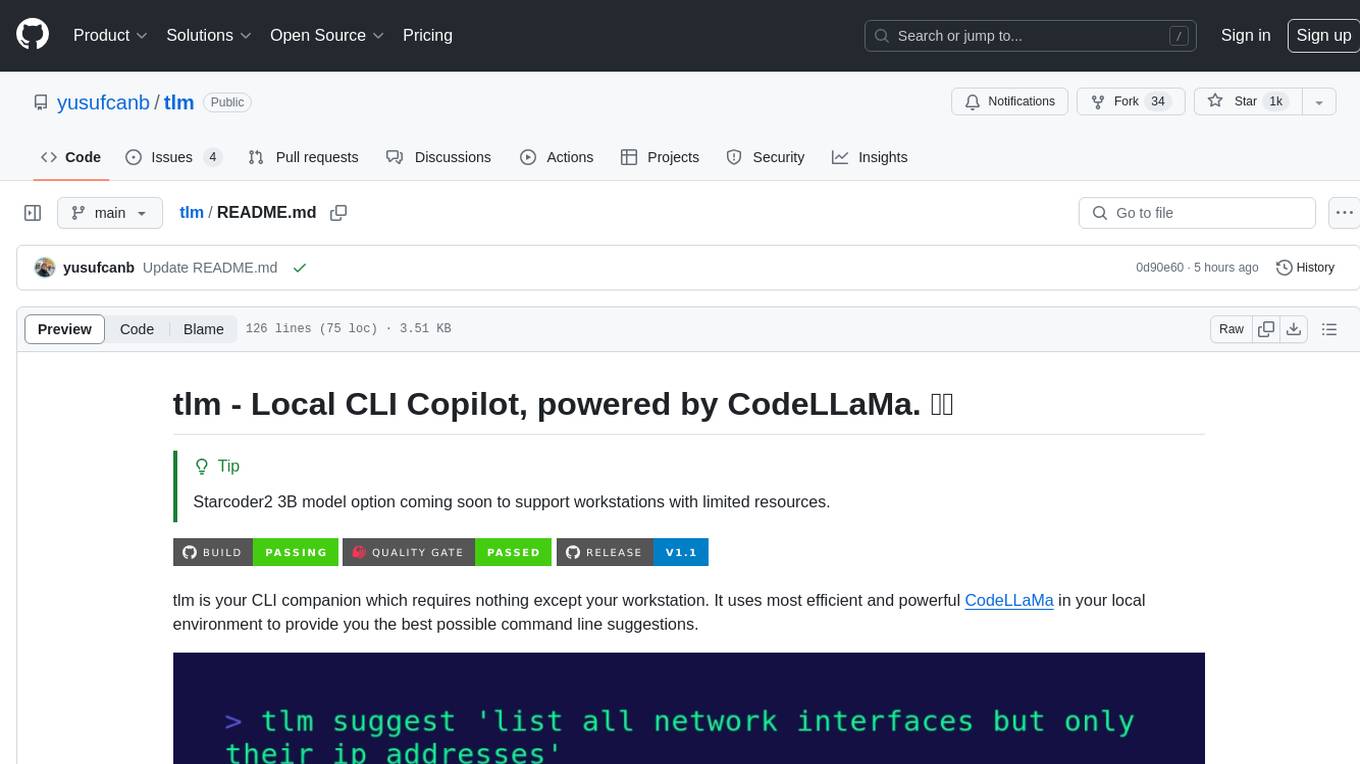
tlm
tlm is a local CLI copilot tool powered by CodeLLaMa, providing efficient command line suggestions without the need for an API key or internet connection. It works on macOS, Linux, and Windows, with automatic shell detection for Powershell, Bash, and Zsh. The tool offers one-liner generation and command explanation, and can be installed via an installation script or using Go Install. Ollama is required to download necessary models, and the tool can be easily deployed and configured. Contributors are welcome to enhance the tool's functionality.
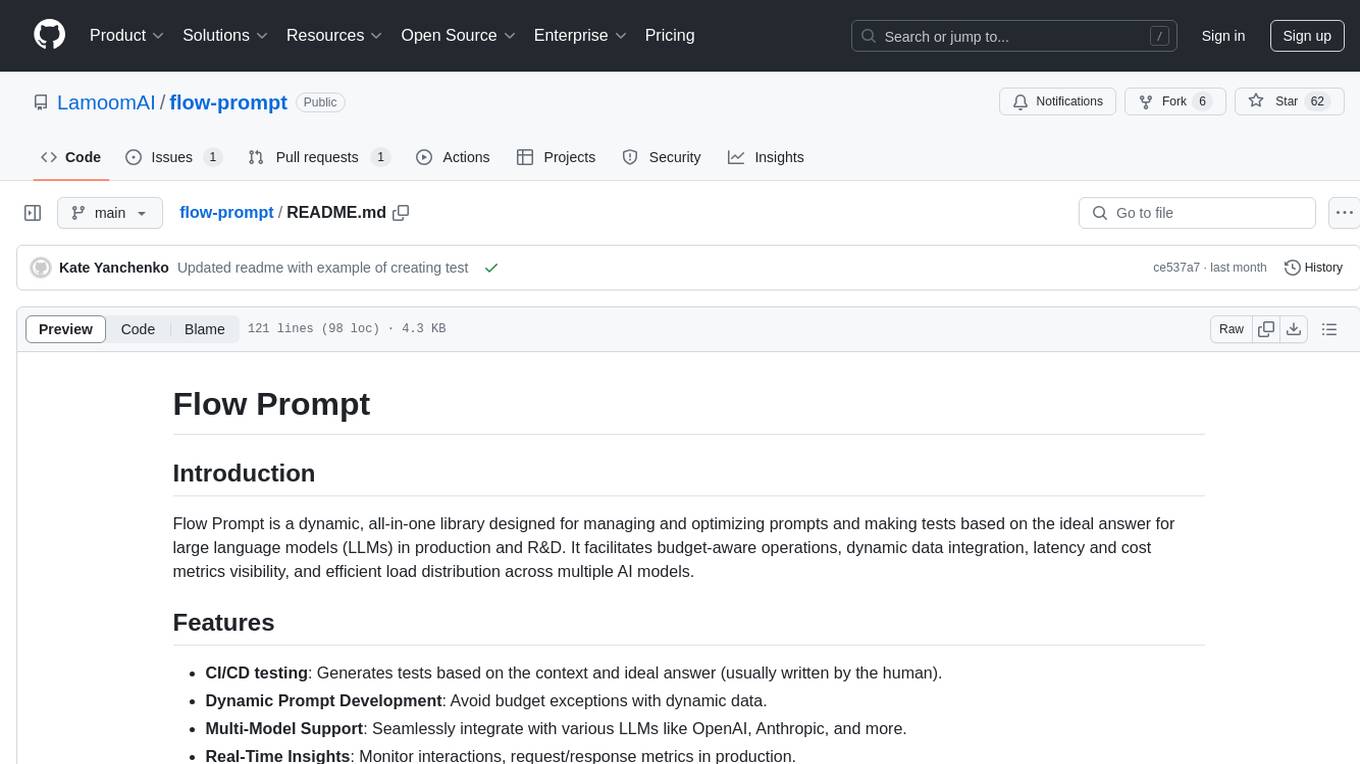
flow-prompt
Flow Prompt is a dynamic library for managing and optimizing prompts for large language models. It facilitates budget-aware operations, dynamic data integration, and efficient load distribution. Features include CI/CD testing, dynamic prompt development, multi-model support, real-time insights, and prompt testing and evolution.
For similar tasks
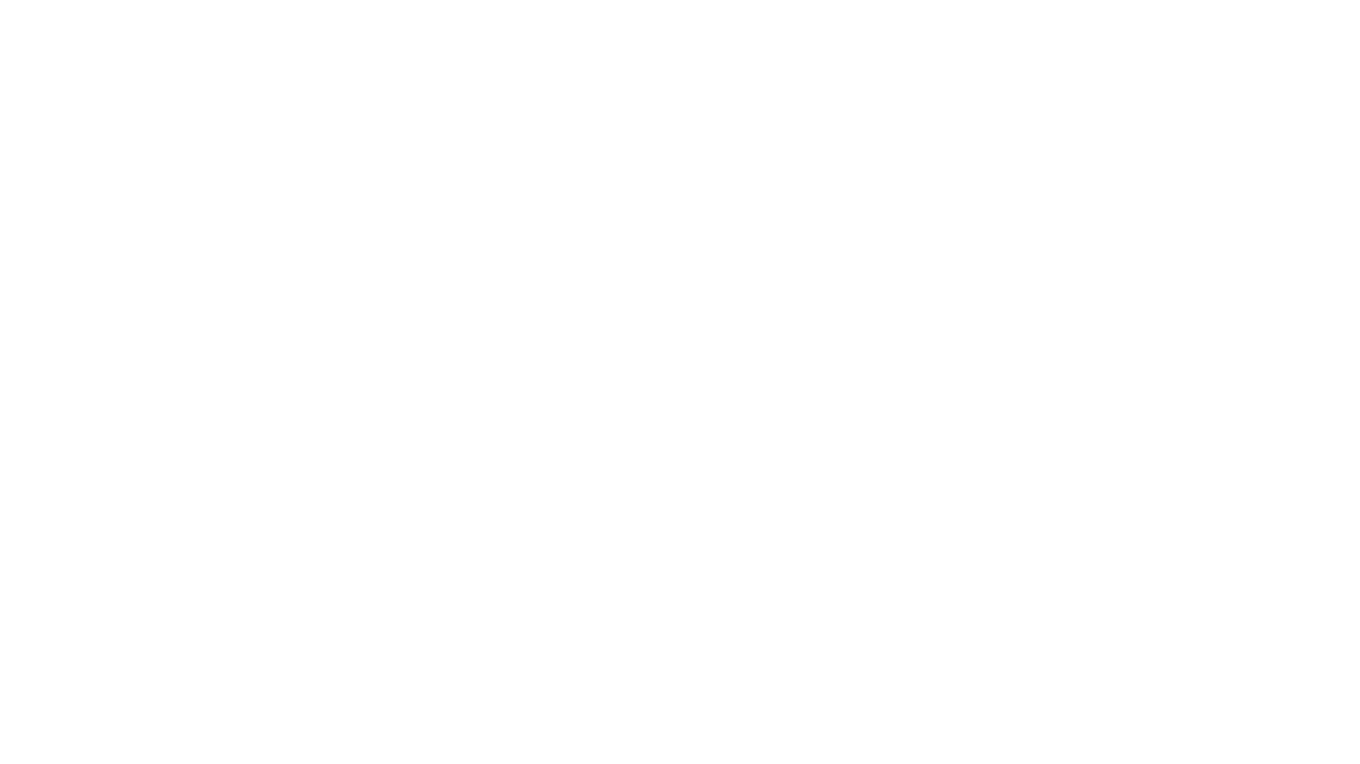
HPT
Hyper-Pretrained Transformers (HPT) is a novel multimodal LLM framework from HyperGAI, trained for vision-language models capable of understanding both textual and visual inputs. The repository contains the open-source implementation of inference code to reproduce the evaluation results of HPT Air on different benchmarks. HPT has achieved competitive results with state-of-the-art models on various multimodal LLM benchmarks. It offers models like HPT 1.5 Air and HPT 1.0 Air, providing efficient solutions for vision-and-language tasks.
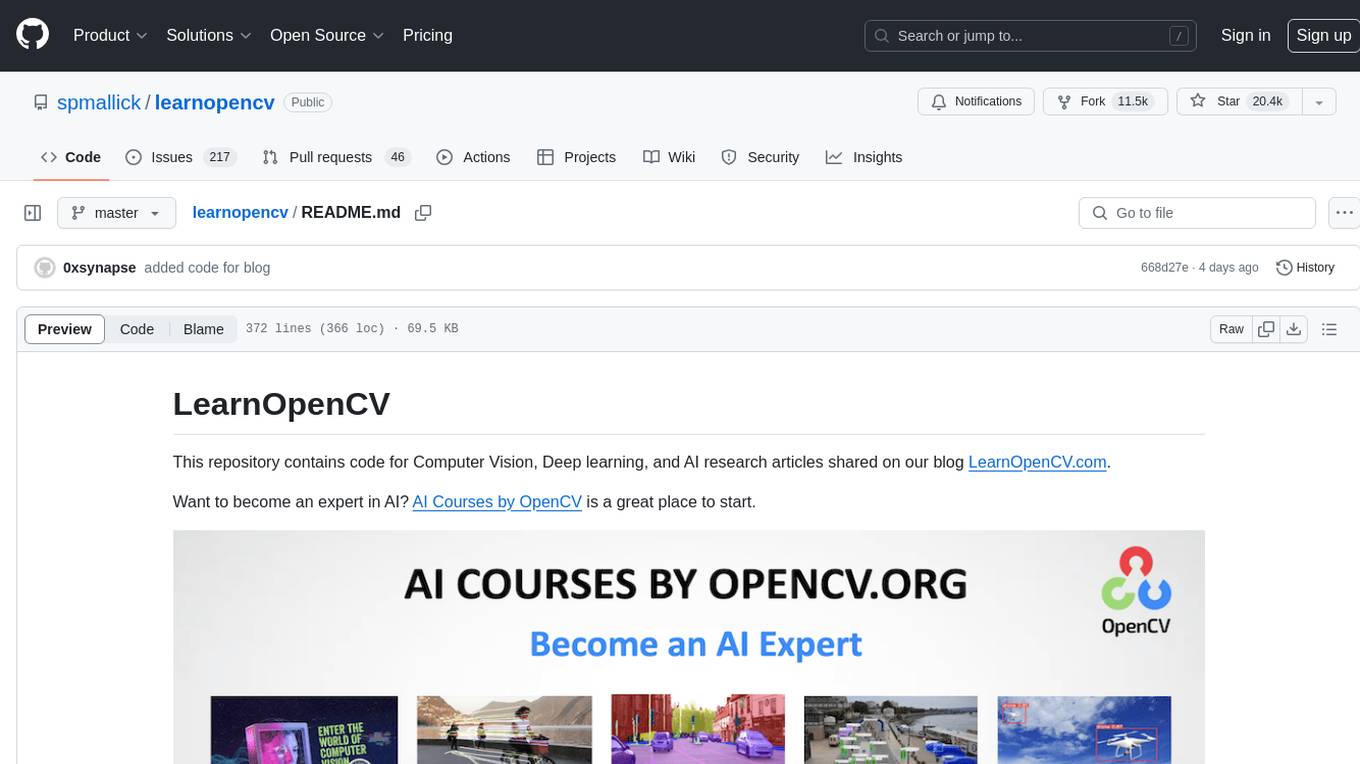
learnopencv
LearnOpenCV is a repository containing code for Computer Vision, Deep learning, and AI research articles shared on the blog LearnOpenCV.com. It serves as a resource for individuals looking to enhance their expertise in AI through various courses offered by OpenCV. The repository includes a wide range of topics such as image inpainting, instance segmentation, robotics, deep learning models, and more, providing practical implementations and code examples for readers to explore and learn from.
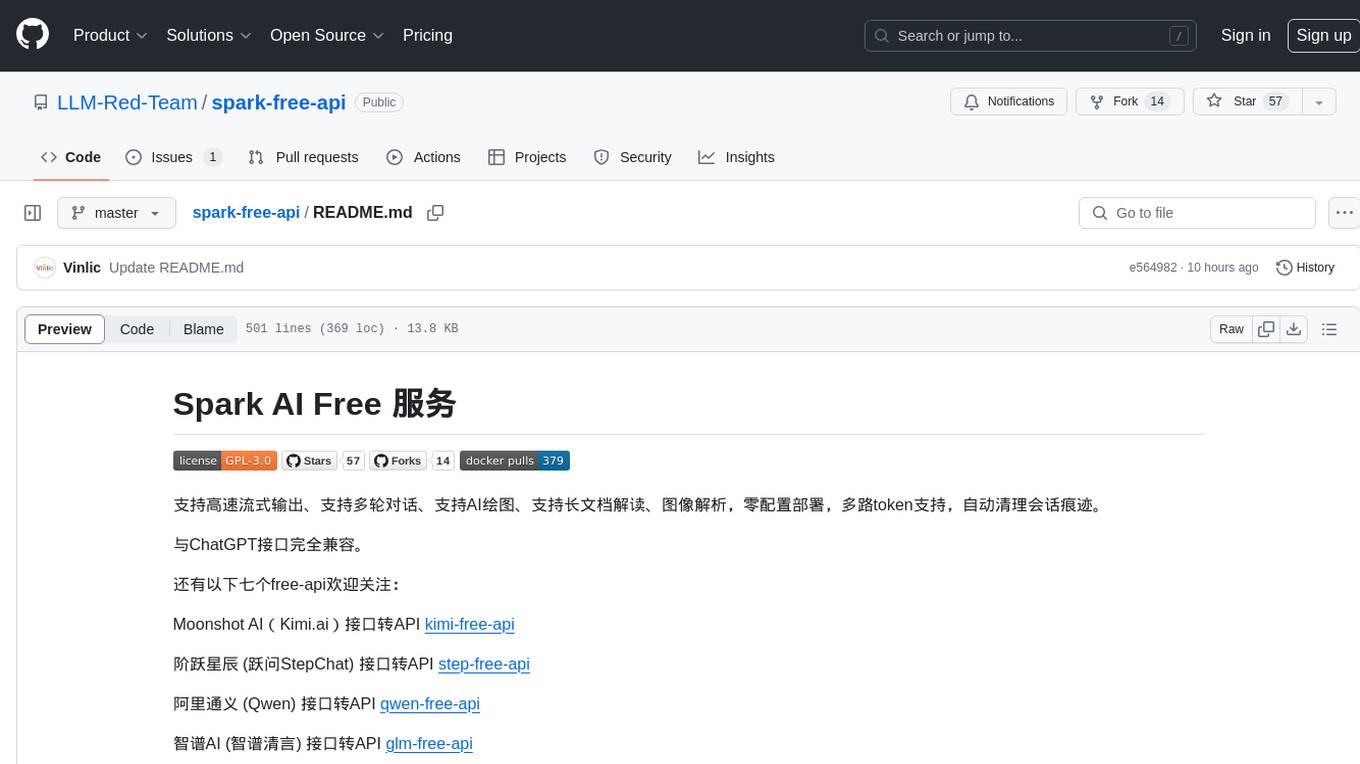
spark-free-api
Spark AI Free 服务 provides high-speed streaming output, multi-turn dialogue support, AI drawing support, long document interpretation, and image parsing. It offers zero-configuration deployment, multi-token support, and automatic session trace cleaning. It is fully compatible with the ChatGPT interface. The repository includes multiple free-api projects for various AI services. Users can access the API for tasks such as chat completions, AI drawing, document interpretation, image analysis, and ssoSessionId live checking. The project also provides guidelines for deployment using Docker, Docker-compose, Render, Vercel, and native deployment methods. It recommends using custom clients for faster and simpler access to the free-api series projects.
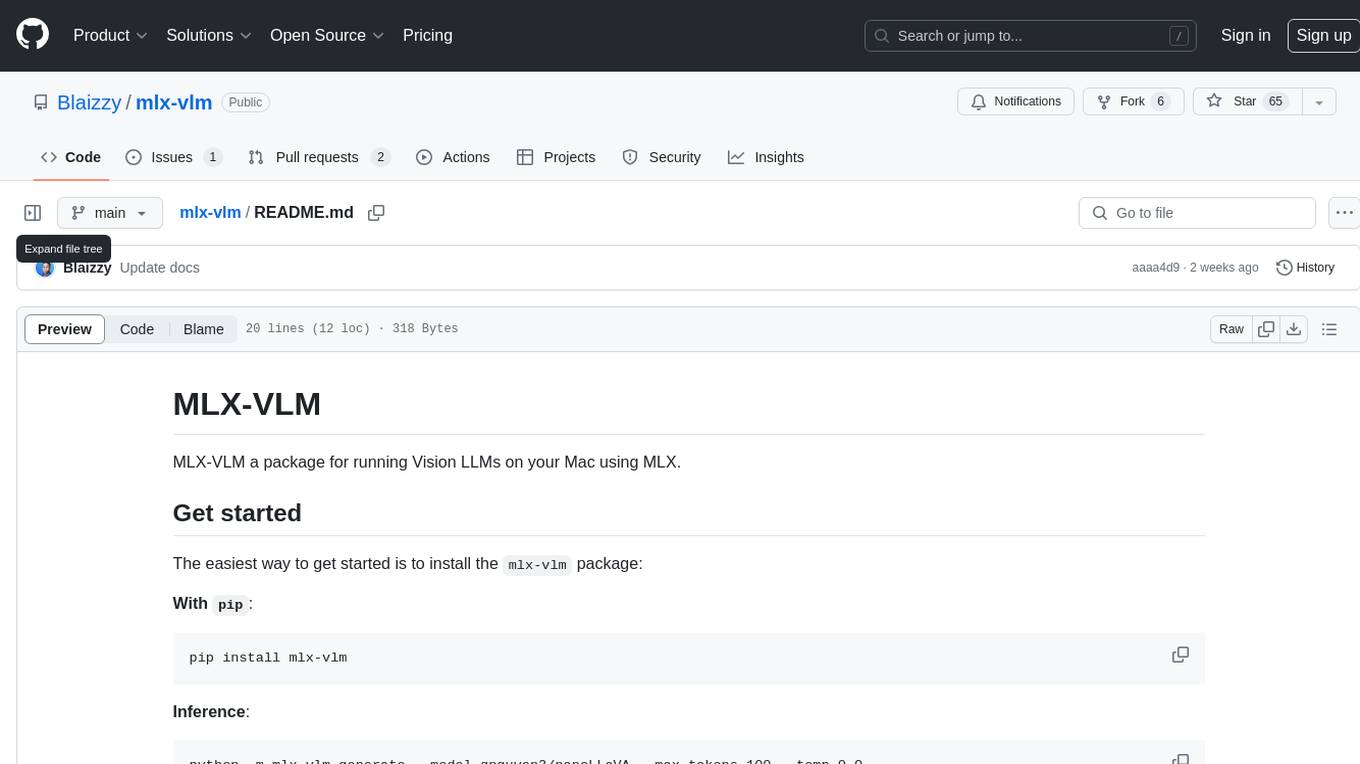
mlx-vlm
MLX-VLM is a package designed for running Vision LLMs on Mac systems using MLX. It provides a convenient way to install and utilize the package for processing large language models related to vision tasks. The tool simplifies the process of running LLMs on Mac computers, offering a seamless experience for users interested in leveraging MLX for vision-related projects.
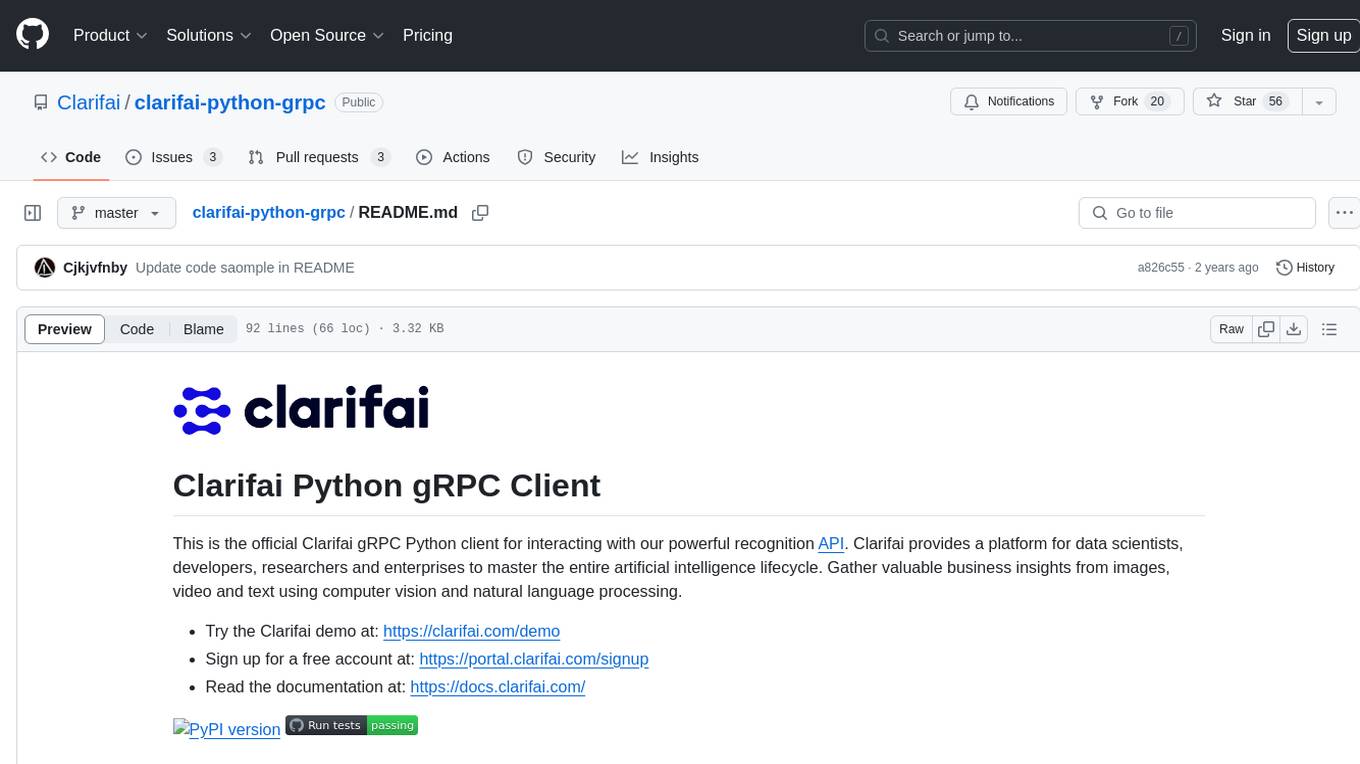
clarifai-python-grpc
This is the official Clarifai gRPC Python client for interacting with their recognition API. Clarifai offers a platform for data scientists, developers, researchers, and enterprises to utilize artificial intelligence for image, video, and text analysis through computer vision and natural language processing. The client allows users to authenticate, predict concepts in images, and access various functionalities provided by the Clarifai API. It follows a versioning scheme that aligns with the backend API updates and includes specific instructions for installation and troubleshooting. Users can explore the Clarifai demo, sign up for an account, and refer to the documentation for detailed information.
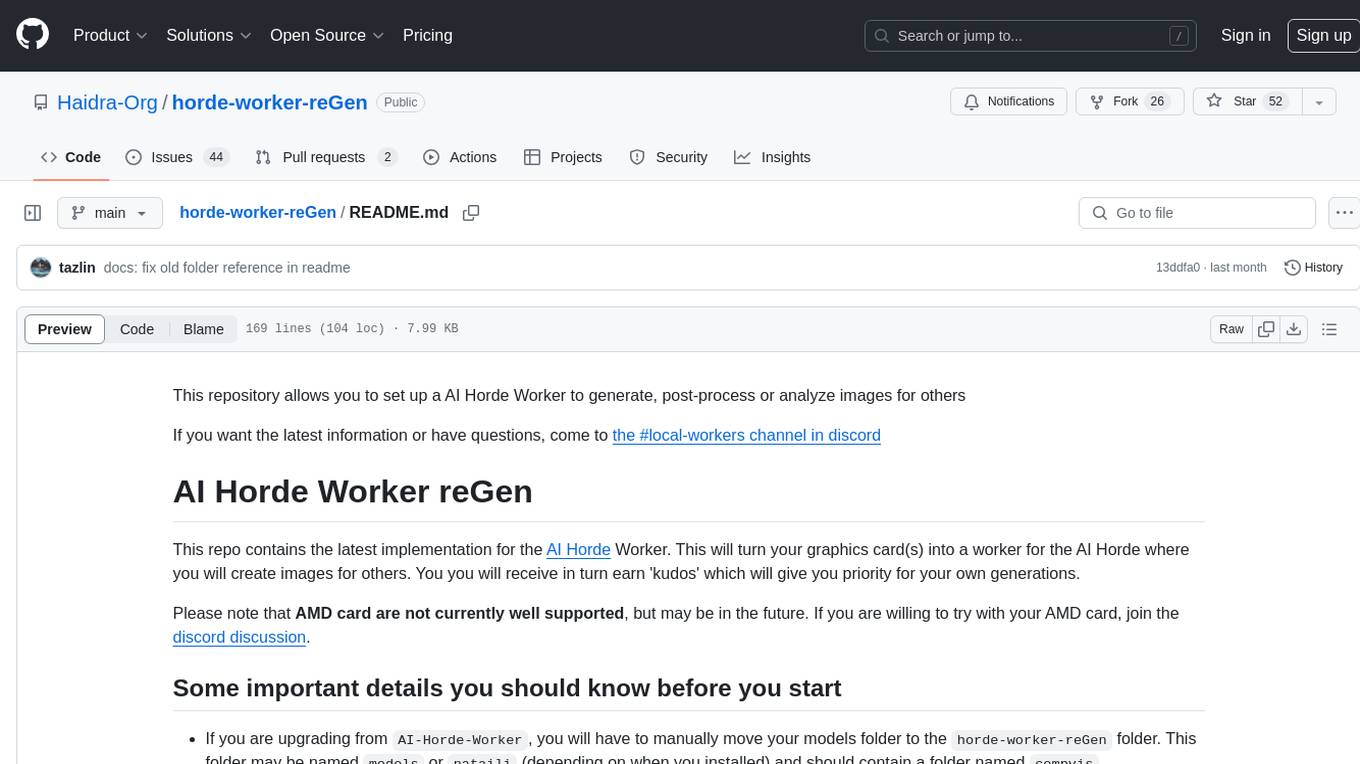
horde-worker-reGen
This repository provides the latest implementation for the AI Horde Worker, allowing users to utilize their graphics card(s) to generate, post-process, or analyze images for others. It offers a platform where users can create images and earn 'kudos' in return, granting priority for their own image generations. The repository includes important details for setup, recommendations for system configurations, instructions for installation on Windows and Linux, basic usage guidelines, and information on updating the AI Horde Worker. Users can also run the worker with multiple GPUs and receive notifications for updates through Discord. Additionally, the repository contains models that are licensed under the CreativeML OpenRAIL License.
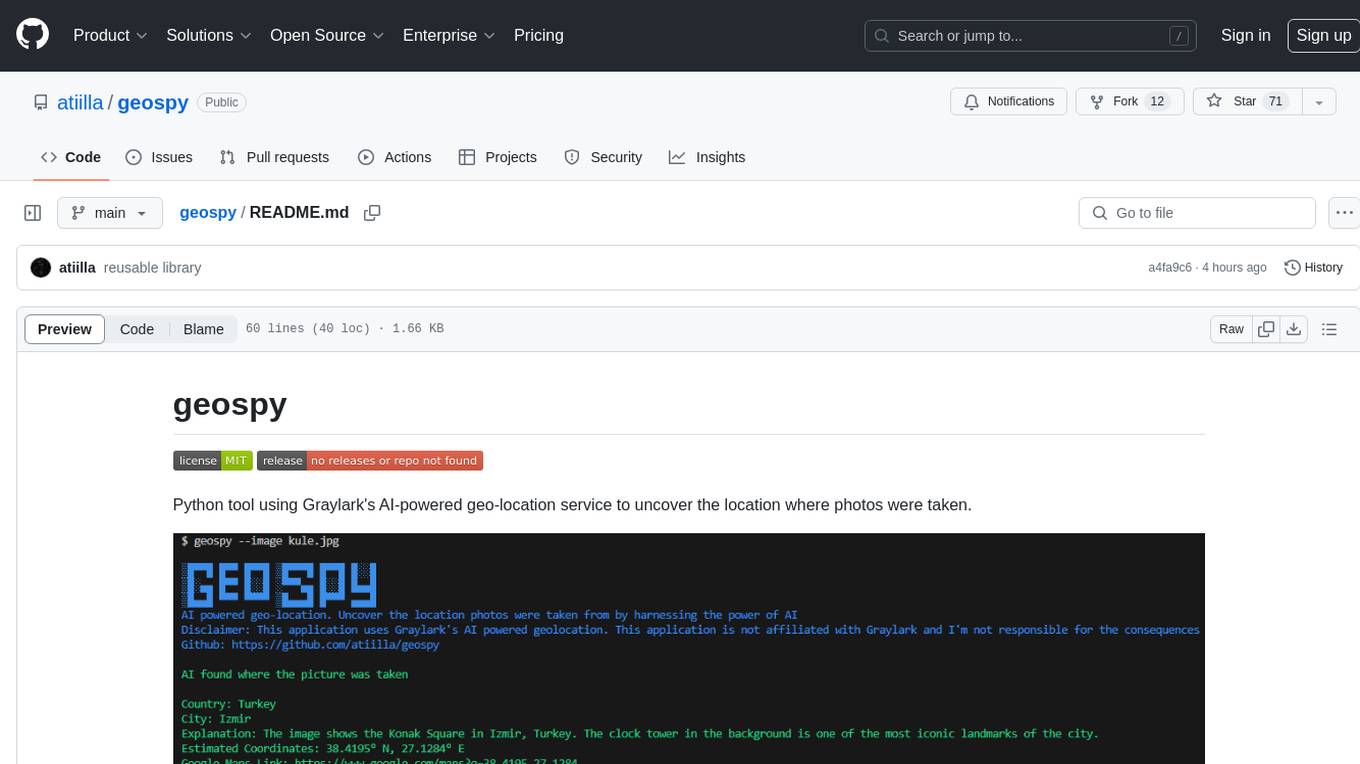
geospy
Geospy is a Python tool that utilizes Graylark's AI-powered geolocation service to determine the location where photos were taken. It allows users to analyze images and retrieve information such as country, city, explanation, coordinates, and Google Maps links. The tool provides a seamless way to integrate geolocation services into various projects and applications.
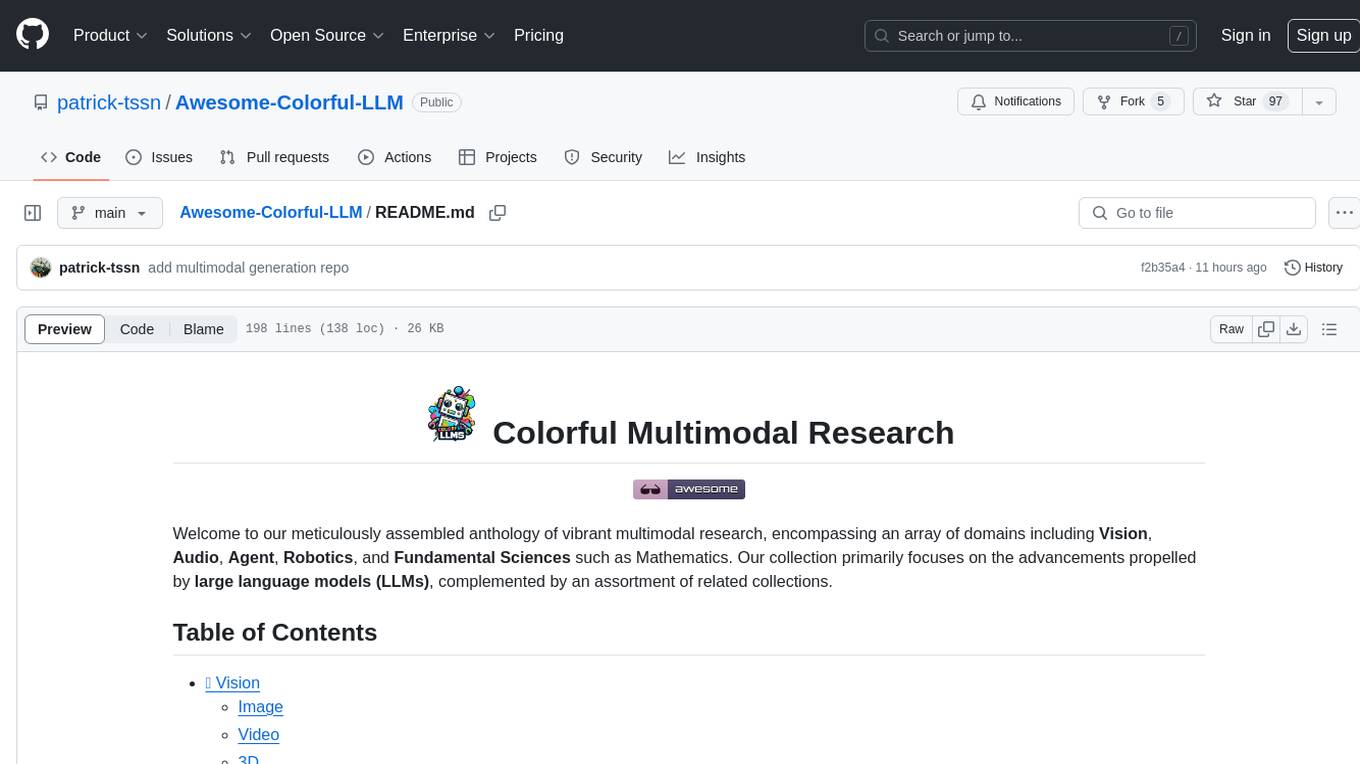
Awesome-Colorful-LLM
Awesome-Colorful-LLM is a meticulously assembled anthology of vibrant multimodal research focusing on advancements propelled by large language models (LLMs) in domains such as Vision, Audio, Agent, Robotics, and Fundamental Sciences like Mathematics. The repository contains curated collections of works, datasets, benchmarks, projects, and tools related to LLMs and multimodal learning. It serves as a comprehensive resource for researchers and practitioners interested in exploring the intersection of language models and various modalities for tasks like image understanding, video pretraining, 3D modeling, document understanding, audio analysis, agent learning, robotic applications, and mathematical research.
For similar jobs
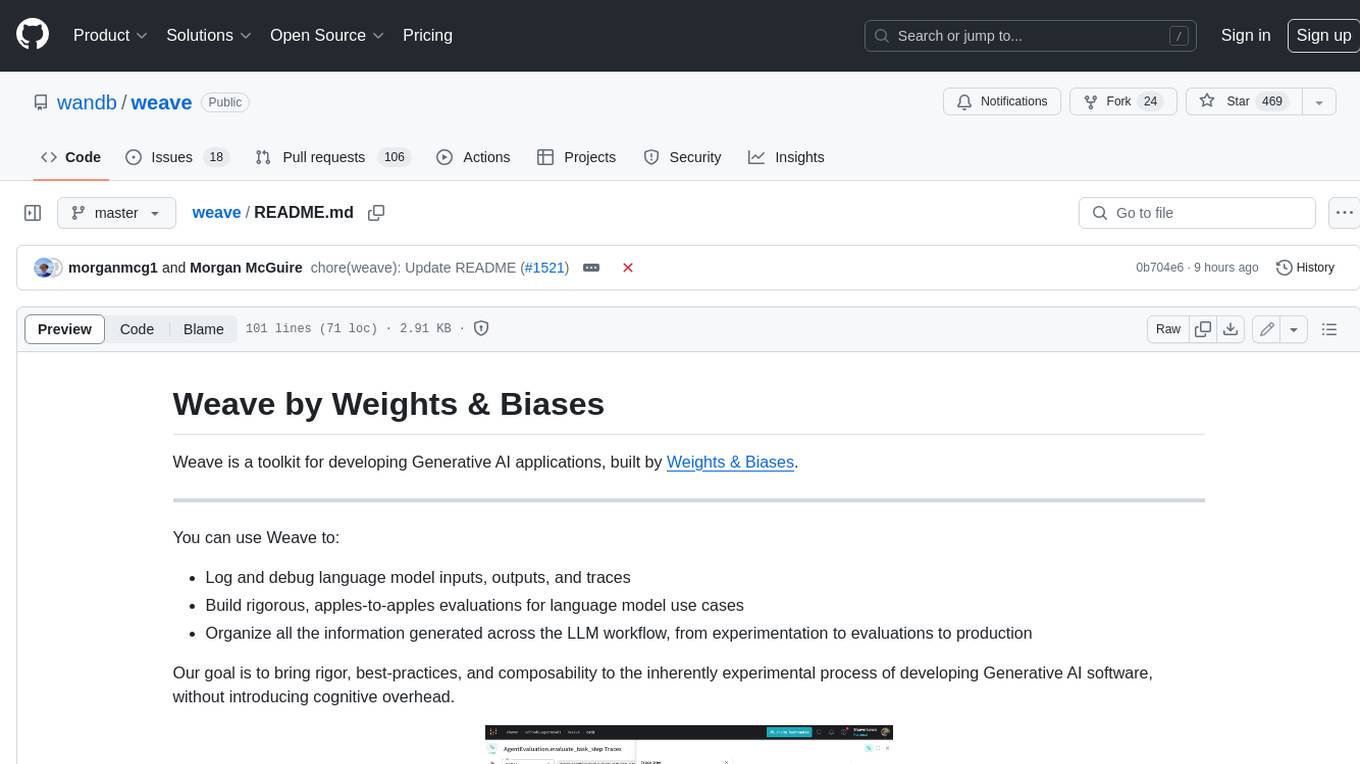
weave
Weave is a toolkit for developing Generative AI applications, built by Weights & Biases. With Weave, you can log and debug language model inputs, outputs, and traces; build rigorous, apples-to-apples evaluations for language model use cases; and organize all the information generated across the LLM workflow, from experimentation to evaluations to production. Weave aims to bring rigor, best-practices, and composability to the inherently experimental process of developing Generative AI software, without introducing cognitive overhead.
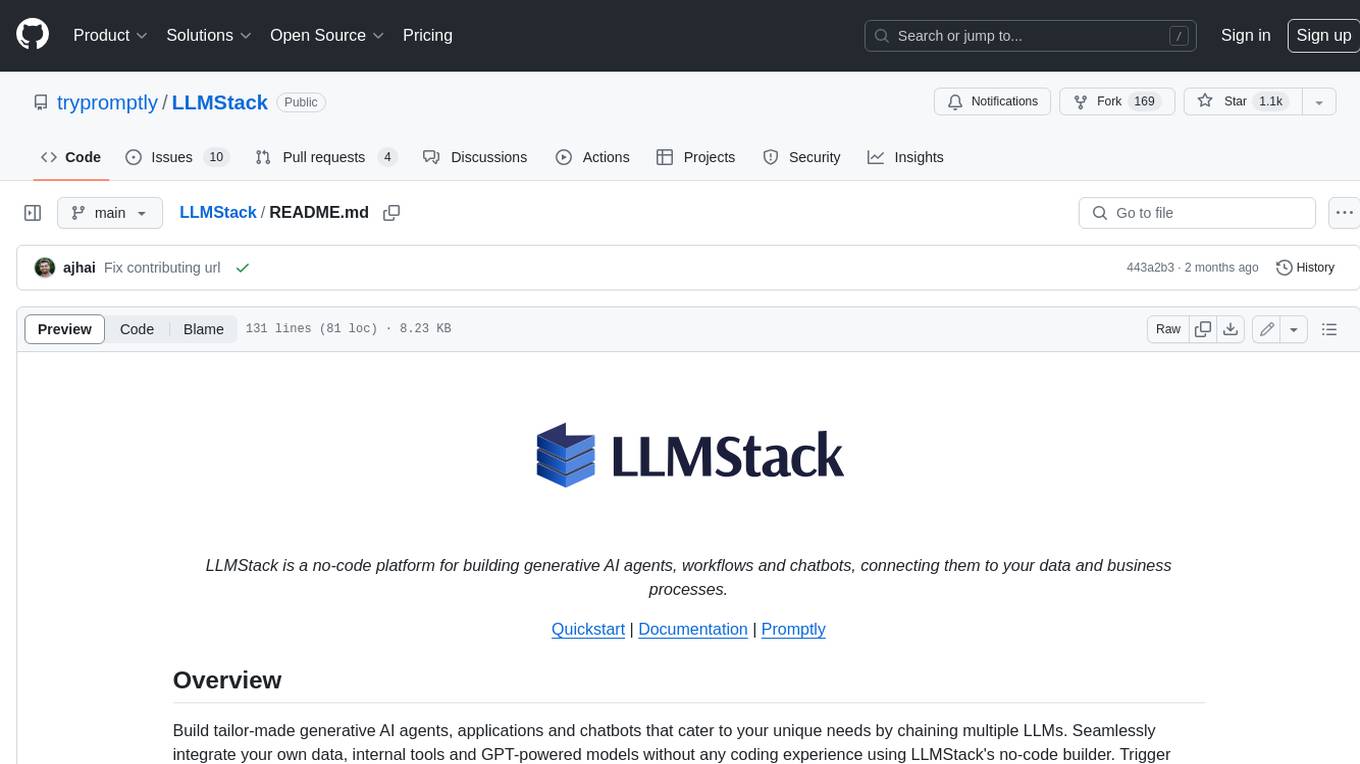
LLMStack
LLMStack is a no-code platform for building generative AI agents, workflows, and chatbots. It allows users to connect their own data, internal tools, and GPT-powered models without any coding experience. LLMStack can be deployed to the cloud or on-premise and can be accessed via HTTP API or triggered from Slack or Discord.
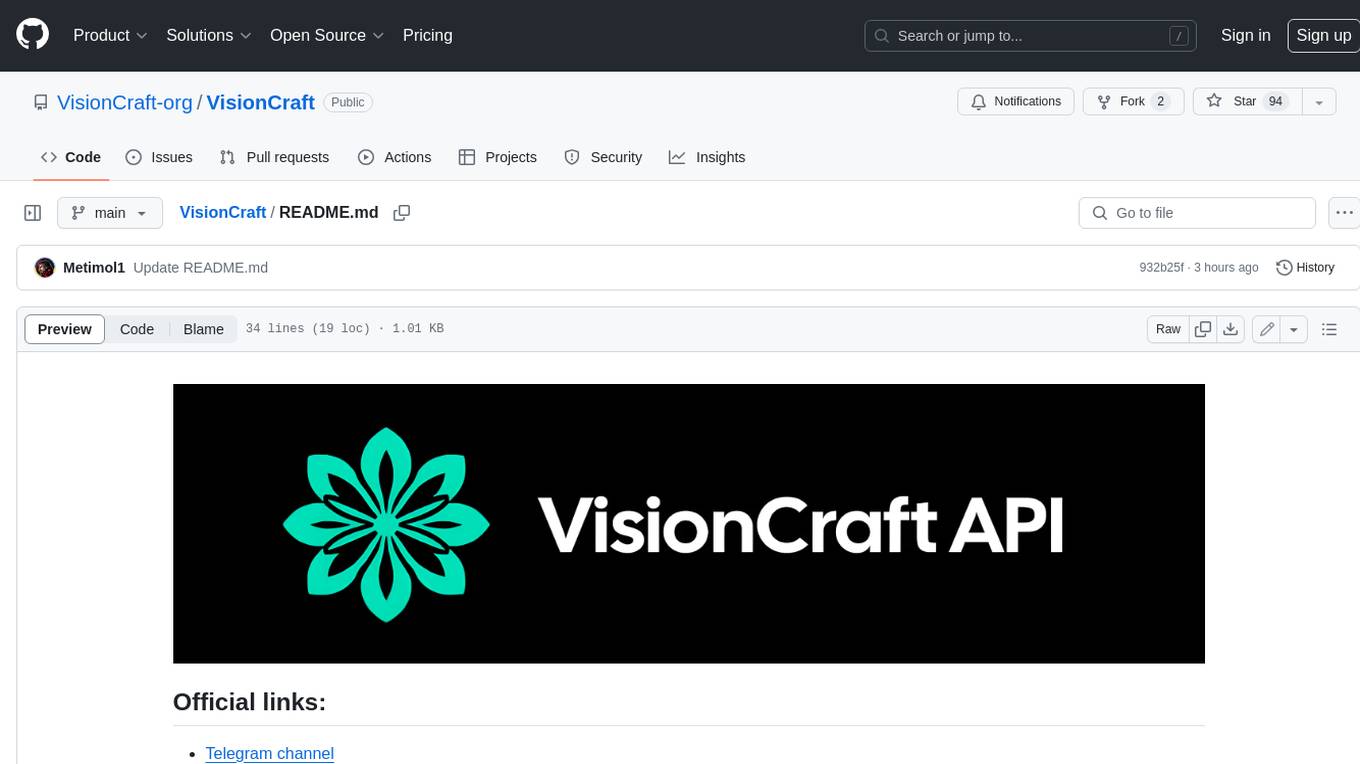
VisionCraft
The VisionCraft API is a free API for using over 100 different AI models. From images to sound.
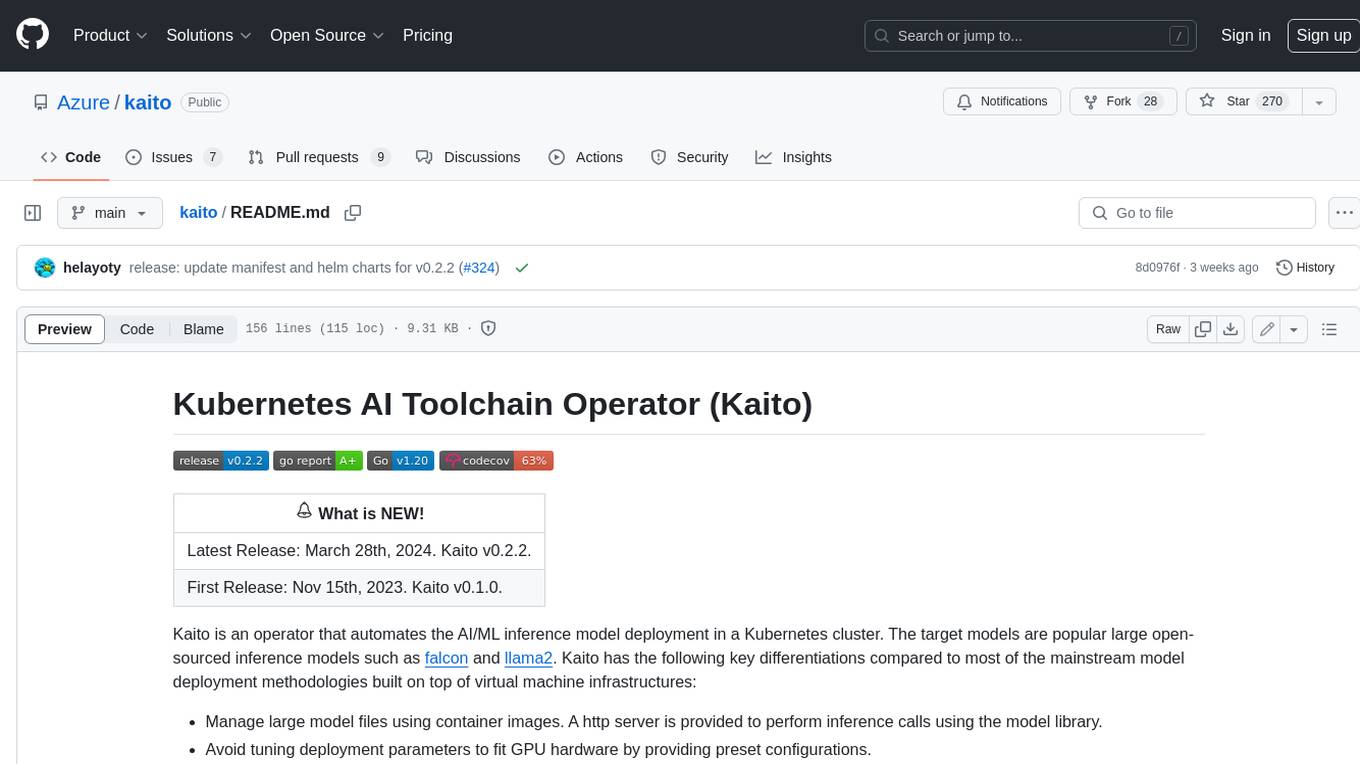
kaito
Kaito is an operator that automates the AI/ML inference model deployment in a Kubernetes cluster. It manages large model files using container images, avoids tuning deployment parameters to fit GPU hardware by providing preset configurations, auto-provisions GPU nodes based on model requirements, and hosts large model images in the public Microsoft Container Registry (MCR) if the license allows. Using Kaito, the workflow of onboarding large AI inference models in Kubernetes is largely simplified.
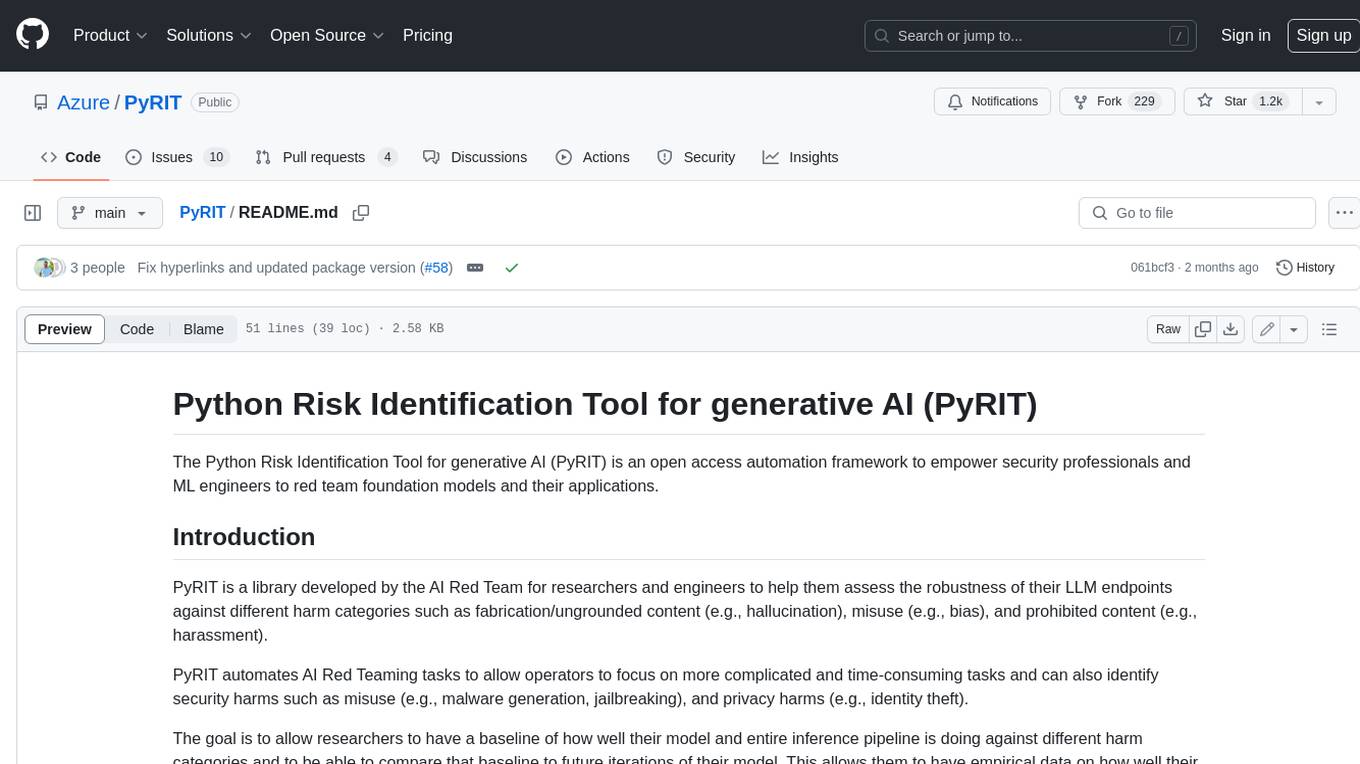
PyRIT
PyRIT is an open access automation framework designed to empower security professionals and ML engineers to red team foundation models and their applications. It automates AI Red Teaming tasks to allow operators to focus on more complicated and time-consuming tasks and can also identify security harms such as misuse (e.g., malware generation, jailbreaking), and privacy harms (e.g., identity theft). The goal is to allow researchers to have a baseline of how well their model and entire inference pipeline is doing against different harm categories and to be able to compare that baseline to future iterations of their model. This allows them to have empirical data on how well their model is doing today, and detect any degradation of performance based on future improvements.
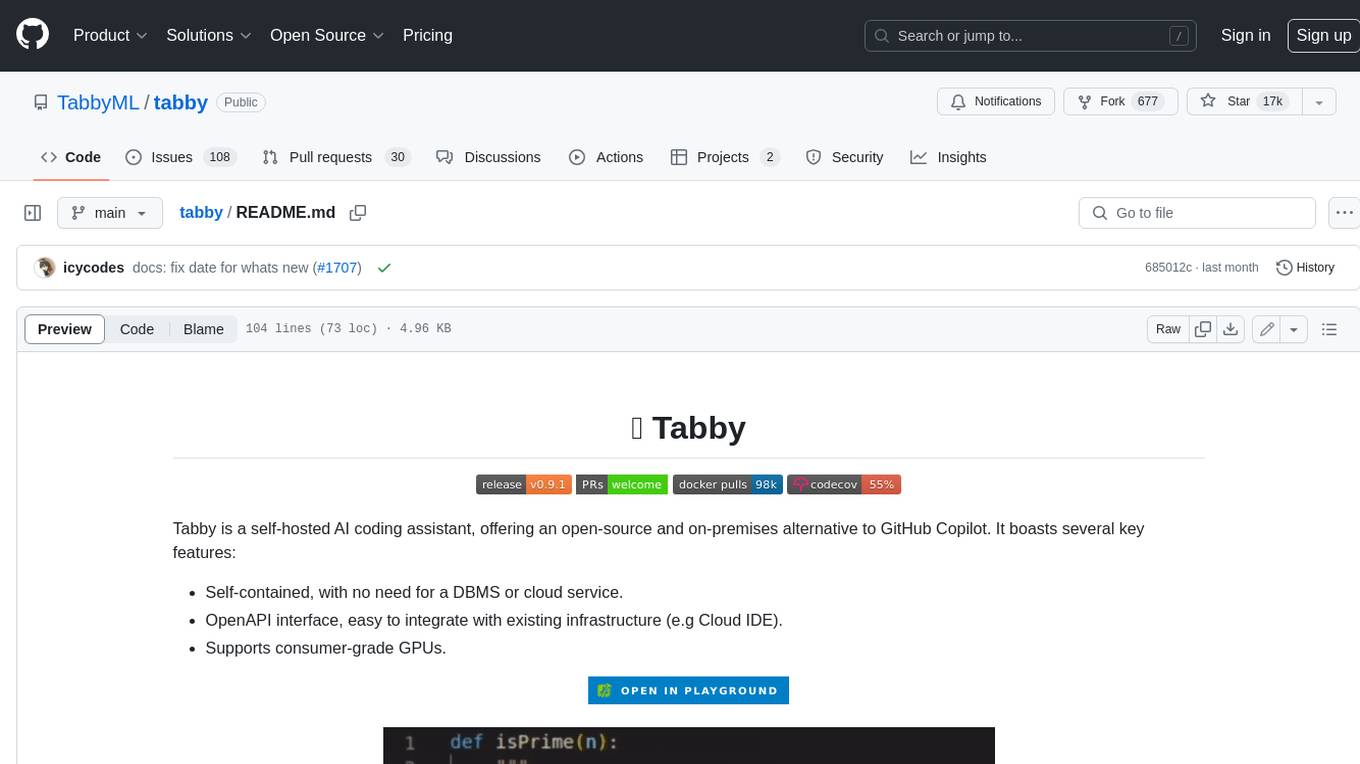
tabby
Tabby is a self-hosted AI coding assistant, offering an open-source and on-premises alternative to GitHub Copilot. It boasts several key features: * Self-contained, with no need for a DBMS or cloud service. * OpenAPI interface, easy to integrate with existing infrastructure (e.g Cloud IDE). * Supports consumer-grade GPUs.
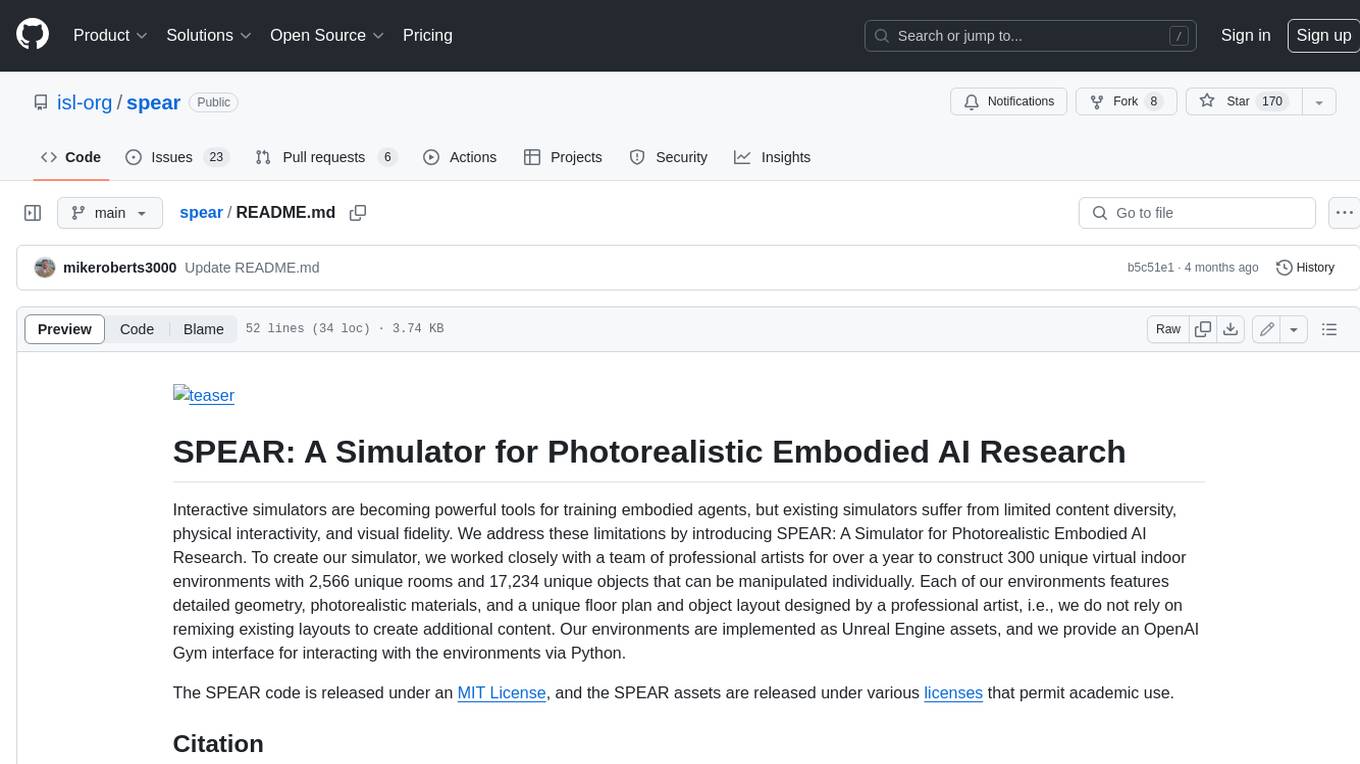
spear
SPEAR (Simulator for Photorealistic Embodied AI Research) is a powerful tool for training embodied agents. It features 300 unique virtual indoor environments with 2,566 unique rooms and 17,234 unique objects that can be manipulated individually. Each environment is designed by a professional artist and features detailed geometry, photorealistic materials, and a unique floor plan and object layout. SPEAR is implemented as Unreal Engine assets and provides an OpenAI Gym interface for interacting with the environments via Python.
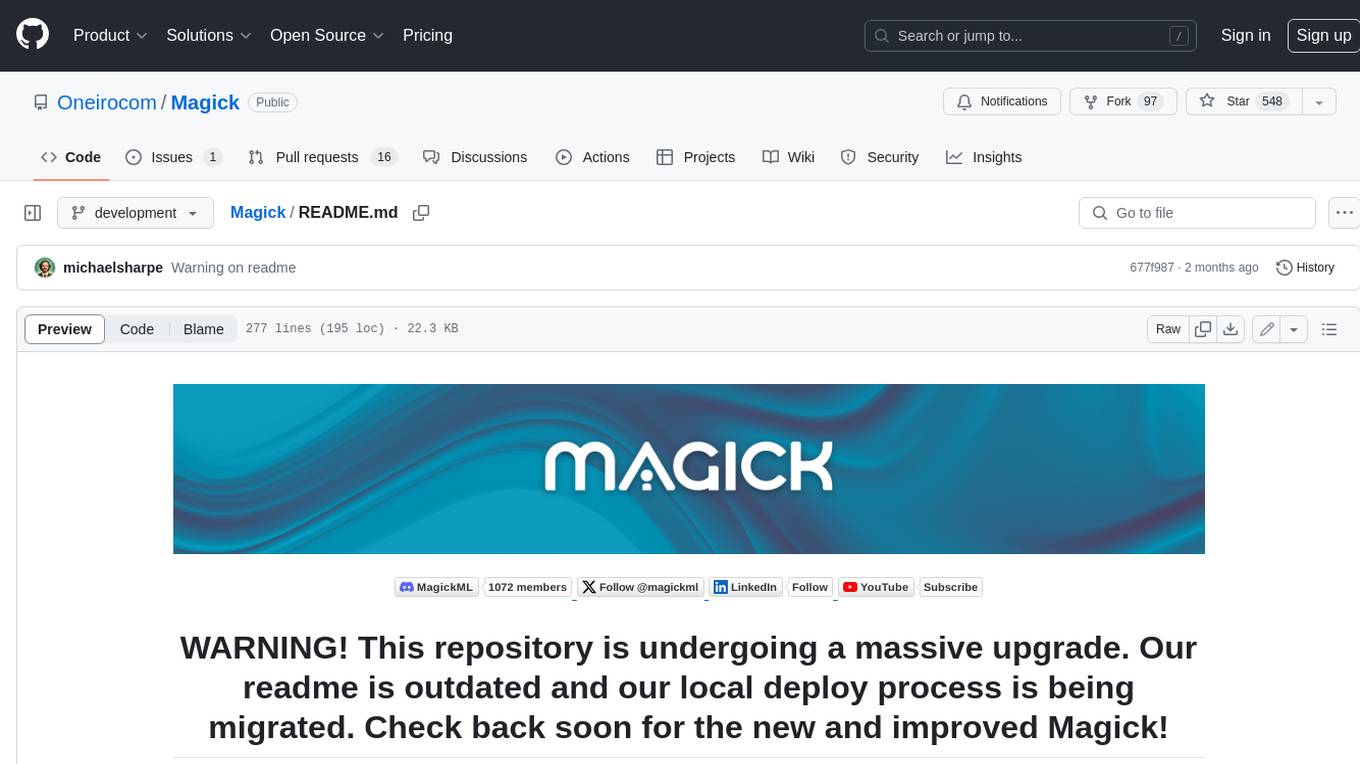
Magick
Magick is a groundbreaking visual AIDE (Artificial Intelligence Development Environment) for no-code data pipelines and multimodal agents. Magick can connect to other services and comes with nodes and templates well-suited for intelligent agents, chatbots, complex reasoning systems and realistic characters.