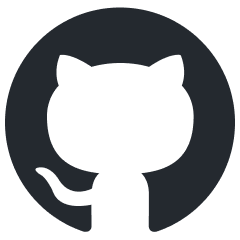
GraphRAG-Local-UI
GraphRAG using Local LLMs - Features robust API and multiple apps for Indexing/Prompt Tuning/Query/Chat/Visualizing/Etc. This is meant to be the ultimate GraphRAG/KG local LLM app.
Stars: 800
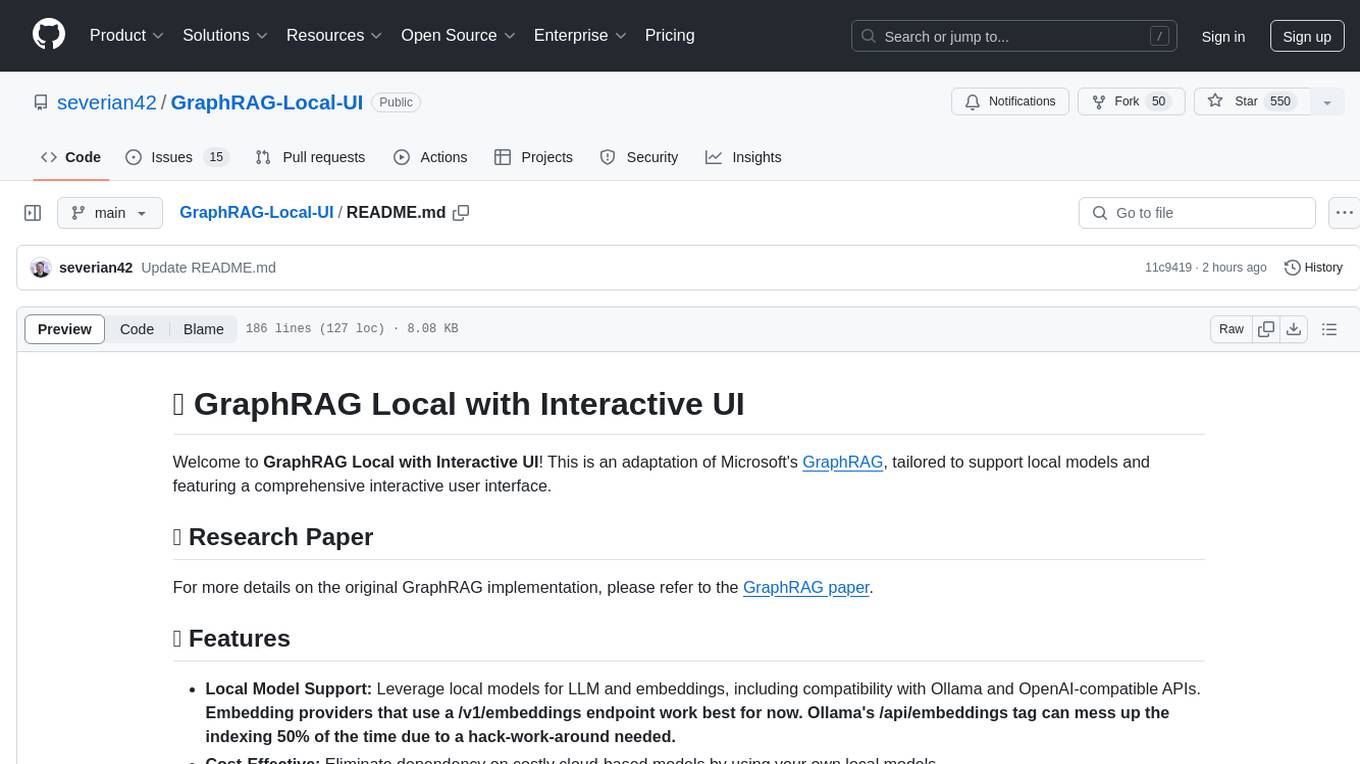
GraphRAG Local with Interactive UI is an adaptation of Microsoft's GraphRAG, tailored to support local models and featuring a comprehensive interactive user interface. It allows users to leverage local models for LLM and embeddings, visualize knowledge graphs in 2D or 3D, manage files, settings, and queries, and explore indexing outputs. The tool aims to be cost-effective by eliminating dependency on costly cloud-based models and offers flexible querying options for global, local, and direct chat queries.
README:
Welcome to GraphRAG Local with Index/Prompt-Tuning and Querying/Chat UIs! This project is an adaptation of Microsoft's GraphRAG, tailored to support local models and featuring a comprehensive interactive user interface ecosystem.
For more details on the original GraphRAG implementation, please refer to the GraphRAG paper.
-
API-Centric Architecture: A robust FastAPI-based server (
api.py
) serving as the core of the GraphRAG operations. -
Dedicated Indexing and Prompt Tuning UI: A separate Gradio-based interface (
index_app.py
) for managing indexing and prompt tuning processes. - Local Model Support: Leverage local models for LLM and embeddings, including compatibility with Ollama and OpenAI-compatible APIs.
- Cost-Effective: Eliminate dependency on costly cloud-based models by using your own local models.
- Interactive UI: User-friendly interface for managing data, running queries, and visualizing results (main app).
- Real-time Graph Visualization: Visualize your knowledge graph in 2D or 3D using Plotly (main app).
- File Management: Upload, view, edit, and delete input files directly from the UI.
- Settings Management: Easily update and manage your GraphRAG settings through the UI.
- Output Exploration: Browse and view indexing outputs and artifacts.
- Logging: Real-time logging for better debugging and monitoring.
- Flexible Querying: Support for global, local, and direct chat queries with customizable parameters (main app).
- Customizable Visualization: Adjust graph layout, node sizes, colors, and more to suit your preferences (main app).
Important Note: The GraphRAG Local UI ecosystem is currently undergoing a major transition. While the main app remains functional, I am actively developing separate applications for Indexing/Prompt Tuning and Querying/Chat, all built around a robust central API. Users should expect some changes and potential instability during this transition period.
While it is currently functional, it has only been primarily tested on a Mac Studio M2.
My vision for the GraphRAG Local UI ecosystem is to become the ultimate set of tools for working with GraphRAG and local LLMs, incorporating as many cool features and knowledge graph tools as possible. I am continuously working on improvements and new features.
- [x] New API-centric architecture (
api.py
) - [x] Dedicated Indexing and Prompt Tuning UI (
index_app.py
) - [x] Improved file management and output exploration
- [x] Background task handling for long-running operations
- [x] Enhanced configuration options through environment variables and YAML files
- [ ] Dedicated Querying/Chat UI that interacts with the API
- [ ] Dockerfile for easier deployment
- [ ] Launch your own GraphRAG API server for use in external applications
- [ ] Experimental: Mixture of Agents for Indexing/Query of knowledge graph
- [ ] Support for more file formats (CSV, PDF, etc.)
- [ ] Web search/Scraping capabilities
- [ ] Advanced graph analysis tools
- [ ] Integration with popular knowledge management tools
- [ ] Collaborative features for team-based knowledge graph building
I am committed to making the GraphRAG Local UI ecosystem the most comprehensive and user-friendly toolset for working with knowledge graphs and LLMs. Your feedback and suggestions are much needed in shaping the future of this project.
Feel free to open an Issue if you run into an error, and I will try to address it as soon as possible to minimize any downtime you might experience.
Follow these steps to set up and run the GraphRAG Local UI ecosystem:
-
Create and activate a new conda environment:
conda create -n graphrag-local -y conda activate graphrag-local
-
Install the required packages:
First install the GraphRAG dir from this repo (has changes not present in the Microsoft repo):
pip install -e ./graphrag
Then install the rest of the dependencies:
pip install -r requirements.txt
-
Launch the API server:
python api.py --host 0.0.0.0 --port 8012 --reload
-
If using Ollama for embeddings, launch the embedding proxy:
python embedding_proxy.py --port 11435 --host http://localhost:11434
Note: For detailed instructions on using Ollama embeddings with GraphRAG, refer to the EMBEDDING_PROXY_README.md file.
-
Launch the Indexing and Prompt Tuning UI:
gradio index_app.py
-
Launch the main interactive UI (legacy app):
gradio app.py
or
python app.py
-
Access the UIs:
- Indexing and Prompt Tuning UI: Open your web browser and navigate to
http://localhost:7861
- Main UI (legacy): Open your web browser and navigate to
http://localhost:7860
- Indexing and Prompt Tuning UI: Open your web browser and navigate to
GraphRAG is designed for flexibility, allowing you to quickly create and initialize your own indexing directory. Follow these steps to set up your environment:
This repo comes with a pre-made Indexing folder but you may want to make your own, so here are the steps. First, create the required directory structure for your input data and indexing results:
mkdir -p ./indexing/input
This directory will store:
- Input .txt files for indexing
- Output results
- Prompts for Prompt Tuning
If you want to start with sample data, copy it to your new input directory:
cp input/* ./indexing/input
You can also add your own .txt files to this directory for indexing.
Run the following command to initialize the ./indexing folder with the required files:
python -m graphrag.index --init --root ./indexing
Move the pre-configured settings.yaml
file to your indexing directory:
mv settings.yaml ./indexing
This file contains the main configuration, pre-set for use with local models.
You can customize your setup by modifying the following environment variables:
-
ROOT_DIR
: Points to your main indexing directory -
INPUT_DIR
: Specifies the location of your input files
For more detailed information and advanced usage, refer to the official GraphRAG documentation.
The GraphRAG Local UI ecosystem consists of three main components, each serving a specific purpose in the knowledge graph creation and querying process:
The api.py
file serves as the backbone of the GraphRAG system, providing a robust FastAPI-based server that handles all core operations.
Key features:
- Manages indexing and prompt tuning processes
- Handles various query types (local, global, and direct chat)
- Integrates with local LLM and embedding models
- Provides endpoints for file management and system configuration
Usage:
python api.py --host 0.0.0.0 --port 8012 --reload
Note: If using Ollama for embeddings, make sure to run the embedding proxy (embedding_proxy.py
) alongside api.py
. Refer to the EMBEDDING_PROXY_README.md for detailed instructions.
- Start the Core API (
api.py
) to enable backend functionality. - If using Ollama for embeddings, start the embedding proxy (
embedding_proxy.py
). - Use the Indexing and Prompt Tuning UI (
index_app.py
) to prepare your data and fine-tune the system. - (Optional) Use the Main Interactive UI (
app.py
) for visualization and legacy features.
This modular approach allows for greater flexibility and easier maintenance of the GraphRAG system. As development continues, the functionality of app.py
will be gradually integrated into new, specialized interfaces that interact with the core API.
The index_app.py
file provides a user-friendly Gradio interface for managing the indexing and prompt tuning processes.
Key features:
- Configure and run indexing tasks
- Set up and execute prompt tuning
- Manage input files and explore output data
- Adjust LLM and embedding settings
Usage:
python index_app.py
Access the UI at http://localhost:7861
The app.py
file is the pre-existing main application, which is being phased out but still provides useful functionality.
Key features:
- Visualize knowledge graphs in 2D or 3D
- Run queries and view results
- Manage GraphRAG settings
- Explore indexed data
Usage:
python app.py
or
gradio app.py
Access the UI at http://localhost:7860
- Start the Core API (
api.py
) to enable backend functionality. - Use the Indexing and Prompt Tuning UI (
index_app.py
) to prepare your data and fine-tune the system. - (Optional) Use the Main Interactive UI (
app.py
) for visualization and legacy features.
This modular approach allows for greater flexibility and easier maintenance of the GraphRAG system. As development continues, the functionality of app.py
will be gradually integrated into new, specialized interfaces that interact with the core API.
- Original GraphRAG repository by Microsoft: GraphRAG
- This project took inspiration and used the GraphRAG4OpenWebUI repository by win4r (https://github.com/win4r/GraphRAG4OpenWebUI) as a starting point for the API implementation.
- If you encounter any issues with the new API or Indexing UI, please check the console logs for detailed error messages.
- For the main app, if you can't run
gradio app.py
, try runningpip install --upgrade gradio
and then exit out and start a new terminal. It should then load and launch properly as a Gradio app. - On Windows, if you run into an encoding/UTF error, you can change it to the correct format in the YAML Settings menu.
For any issues or feature requests, please open an issue on the GitHub repository. Happy knowledge graphing!
For Tasks:
Click tags to check more tools for each tasksFor Jobs:
Alternative AI tools for GraphRAG-Local-UI
Similar Open Source Tools
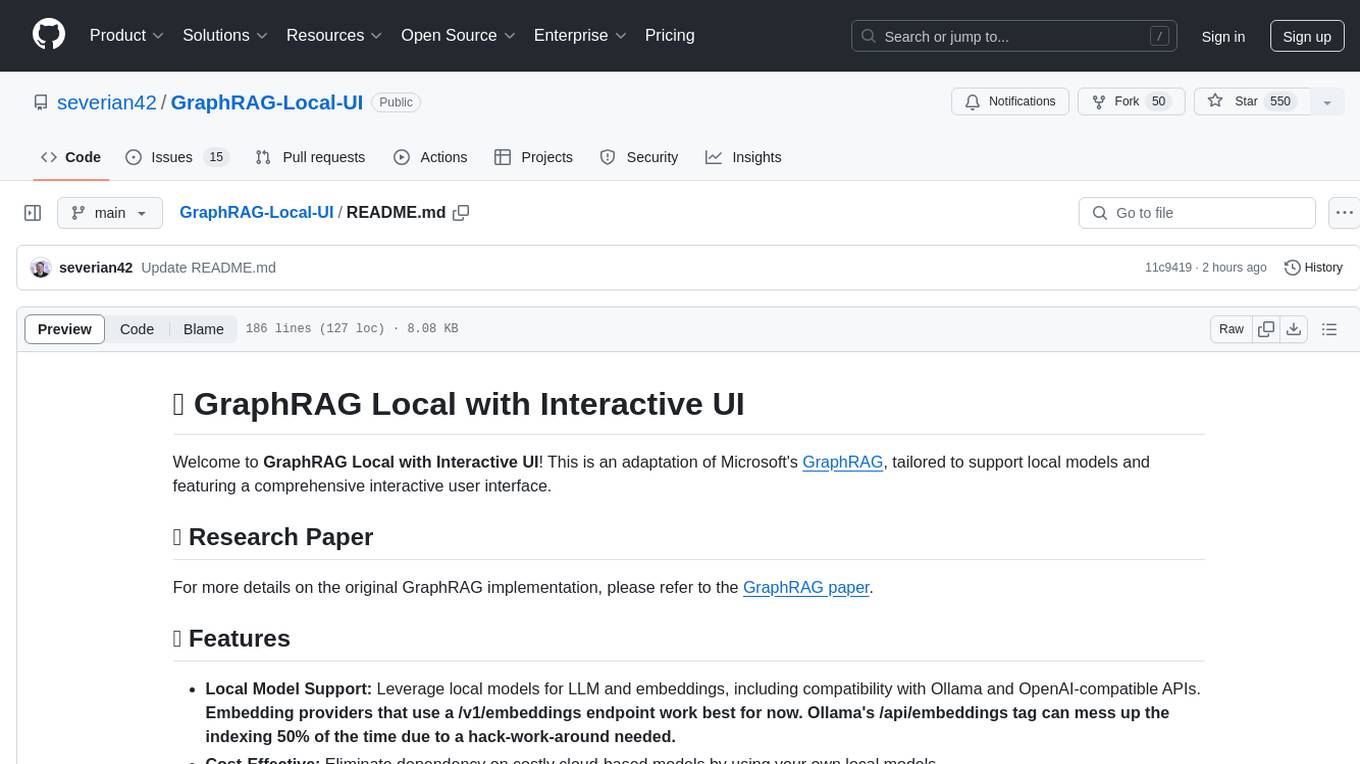
GraphRAG-Local-UI
GraphRAG Local with Interactive UI is an adaptation of Microsoft's GraphRAG, tailored to support local models and featuring a comprehensive interactive user interface. It allows users to leverage local models for LLM and embeddings, visualize knowledge graphs in 2D or 3D, manage files, settings, and queries, and explore indexing outputs. The tool aims to be cost-effective by eliminating dependency on costly cloud-based models and offers flexible querying options for global, local, and direct chat queries.
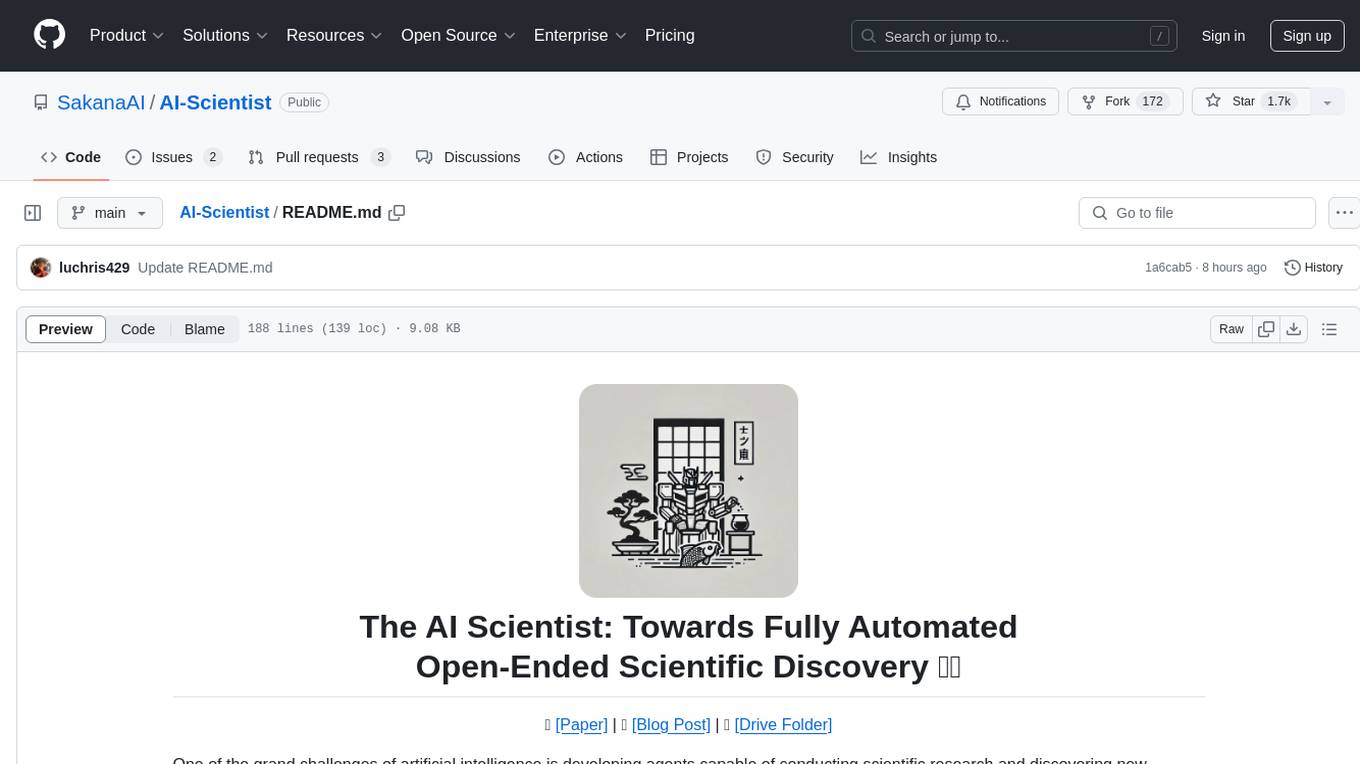
AI-Scientist
The AI Scientist is a comprehensive system for fully automatic scientific discovery, enabling Foundation Models to perform research independently. It aims to tackle the grand challenge of developing agents capable of conducting scientific research and discovering new knowledge. The tool generates papers on various topics using Large Language Models (LLMs) and provides a platform for exploring new research ideas. Users can create their own templates for specific areas of study and run experiments to generate papers. However, caution is advised as the codebase executes LLM-written code, which may pose risks such as the use of potentially dangerous packages and web access.
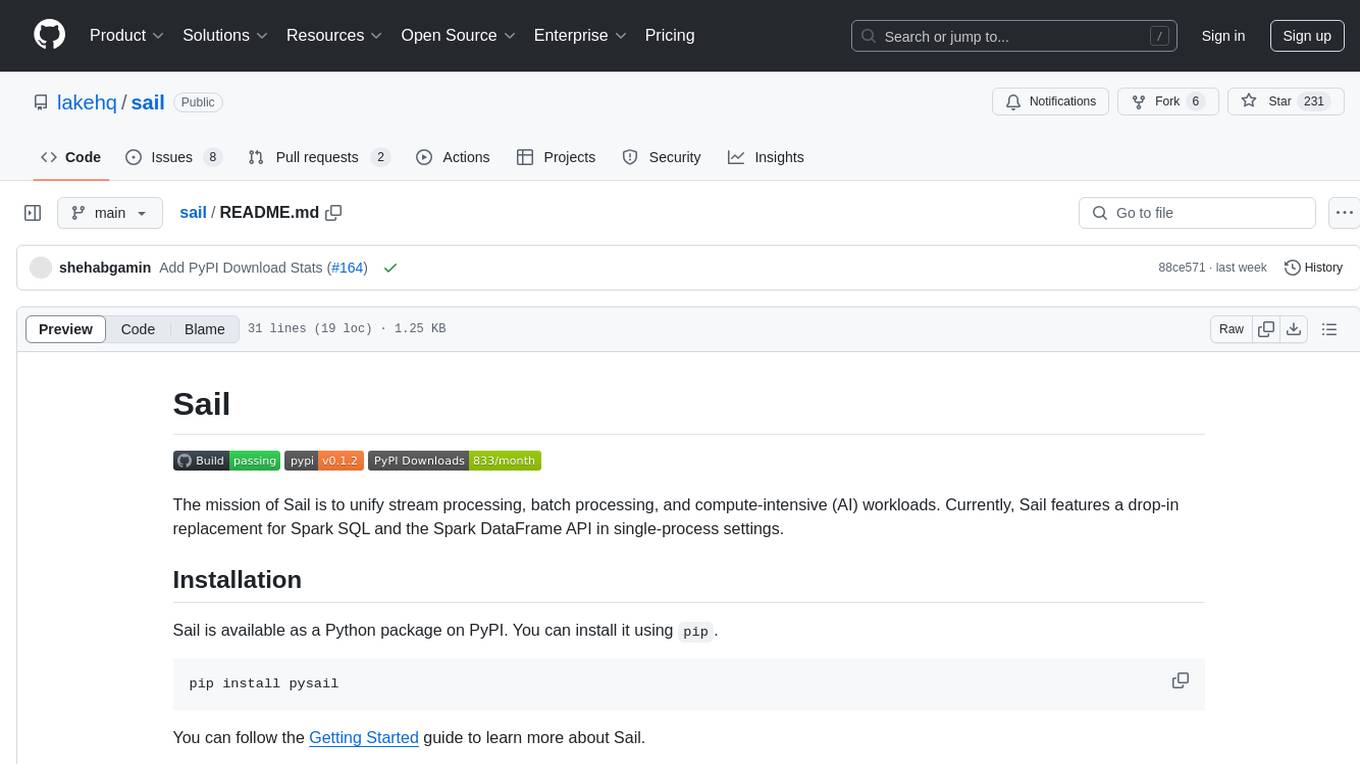
sail
Sail is a tool designed to unify stream processing, batch processing, and compute-intensive workloads, serving as a drop-in replacement for Spark SQL and the Spark DataFrame API in single-process settings. It aims to streamline data processing tasks and facilitate AI workloads.
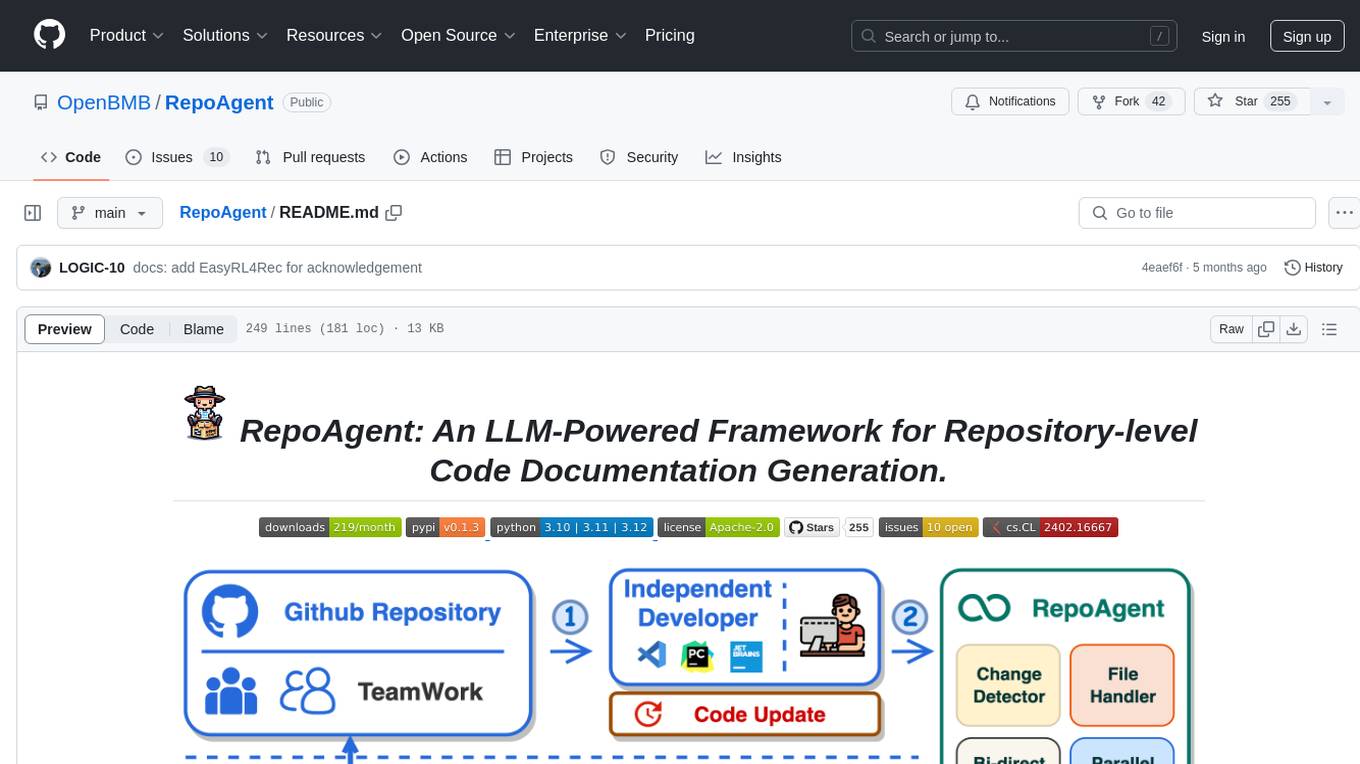
RepoAgent
RepoAgent is an LLM-powered framework designed for repository-level code documentation generation. It automates the process of detecting changes in Git repositories, analyzing code structure through AST, identifying inter-object relationships, replacing Markdown content, and executing multi-threaded operations. The tool aims to assist developers in understanding and maintaining codebases by providing comprehensive documentation, ultimately improving efficiency and saving time.
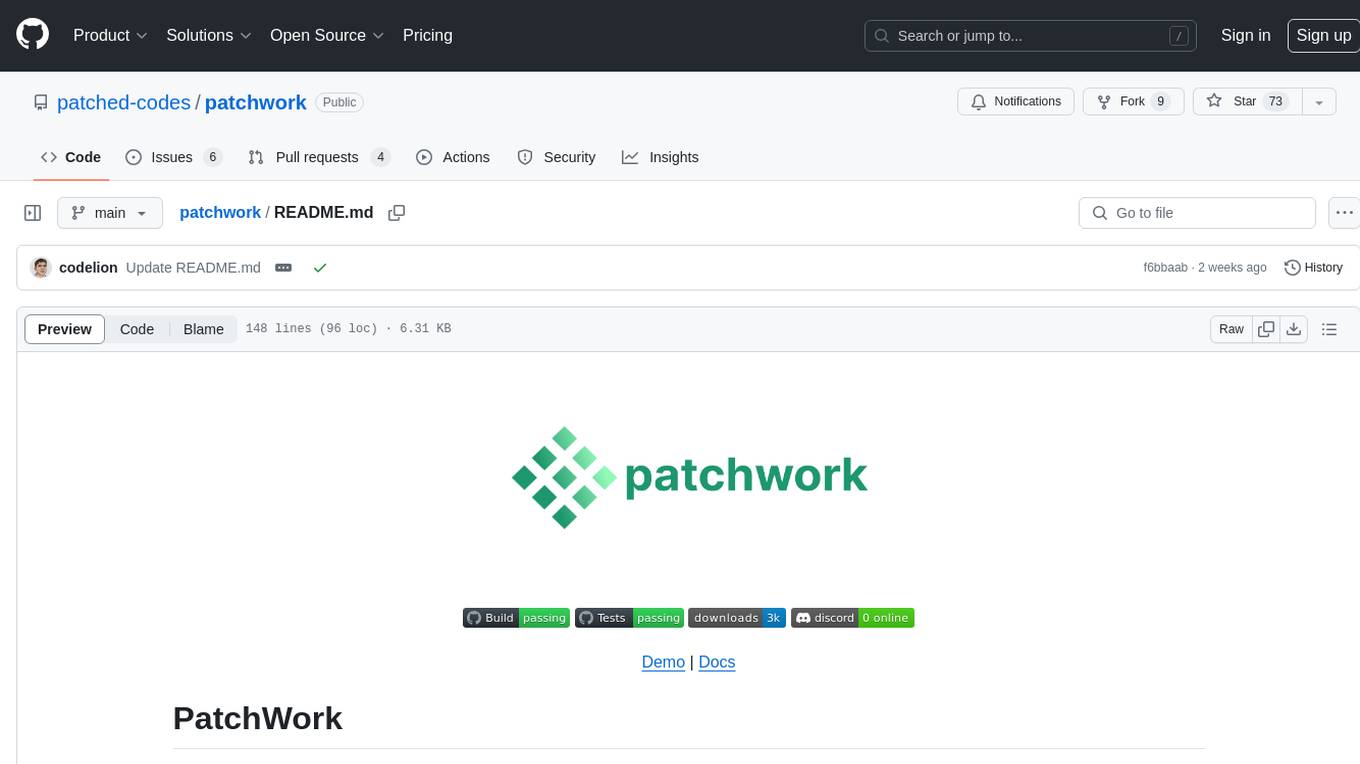
patchwork
PatchWork is an open-source framework designed for automating development tasks using large language models. It enables users to automate workflows such as PR reviews, bug fixing, security patching, and more through a self-hosted CLI agent and preferred LLMs. The framework consists of reusable atomic actions called Steps, customizable LLM prompts known as Prompt Templates, and LLM-assisted automations called Patchflows. Users can run Patchflows locally in their CLI/IDE or as part of CI/CD pipelines. PatchWork offers predefined patchflows like AutoFix, PRReview, GenerateREADME, DependencyUpgrade, and ResolveIssue, with the flexibility to create custom patchflows. Prompt templates are used to pass queries to LLMs and can be customized. Contributions to new patchflows, steps, and the core framework are encouraged, with chat assistants available to aid in the process. The roadmap includes expanding the patchflow library, introducing a debugger and validation module, supporting large-scale code embeddings, parallelization, fine-tuned models, and an open-source GUI. PatchWork is licensed under AGPL-3.0 terms, while custom patchflows and steps can be shared using the Apache-2.0 licensed patchwork template repository.
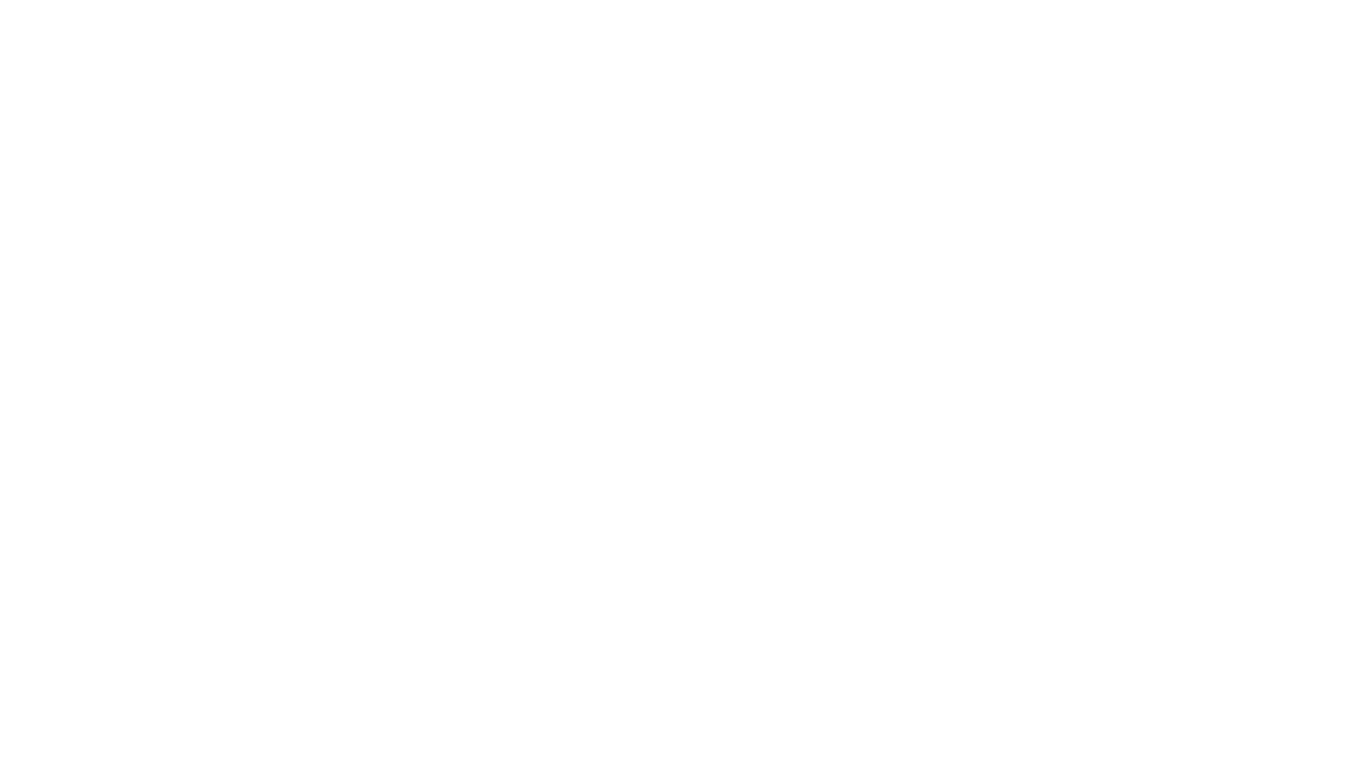
cognita
Cognita is an open-source framework to organize your RAG codebase along with a frontend to play around with different RAG customizations. It provides a simple way to organize your codebase so that it becomes easy to test it locally while also being able to deploy it in a production ready environment. The key issues that arise while productionizing RAG system from a Jupyter Notebook are: 1. **Chunking and Embedding Job** : The chunking and embedding code usually needs to be abstracted out and deployed as a job. Sometimes the job will need to run on a schedule or be trigerred via an event to keep the data updated. 2. **Query Service** : The code that generates the answer from the query needs to be wrapped up in a api server like FastAPI and should be deployed as a service. This service should be able to handle multiple queries at the same time and also autoscale with higher traffic. 3. **LLM / Embedding Model Deployment** : Often times, if we are using open-source models, we load the model in the Jupyter notebook. This will need to be hosted as a separate service in production and model will need to be called as an API. 4. **Vector DB deployment** : Most testing happens on vector DBs in memory or on disk. However, in production, the DBs need to be deployed in a more scalable and reliable way. Cognita makes it really easy to customize and experiment everything about a RAG system and still be able to deploy it in a good way. It also ships with a UI that makes it easier to try out different RAG configurations and see the results in real time. You can use it locally or with/without using any Truefoundry components. However, using Truefoundry components makes it easier to test different models and deploy the system in a scalable way. Cognita allows you to host multiple RAG systems using one app. ### Advantages of using Cognita are: 1. A central reusable repository of parsers, loaders, embedders and retrievers. 2. Ability for non-technical users to play with UI - Upload documents and perform QnA using modules built by the development team. 3. Fully API driven - which allows integration with other systems. > If you use Cognita with Truefoundry AI Gateway, you can get logging, metrics and feedback mechanism for your user queries. ### Features: 1. Support for multiple document retrievers that use `Similarity Search`, `Query Decompostion`, `Document Reranking`, etc 2. Support for SOTA OpenSource embeddings and reranking from `mixedbread-ai` 3. Support for using LLMs using `Ollama` 4. Support for incremental indexing that ingests entire documents in batches (reduces compute burden), keeps track of already indexed documents and prevents re-indexing of those docs.
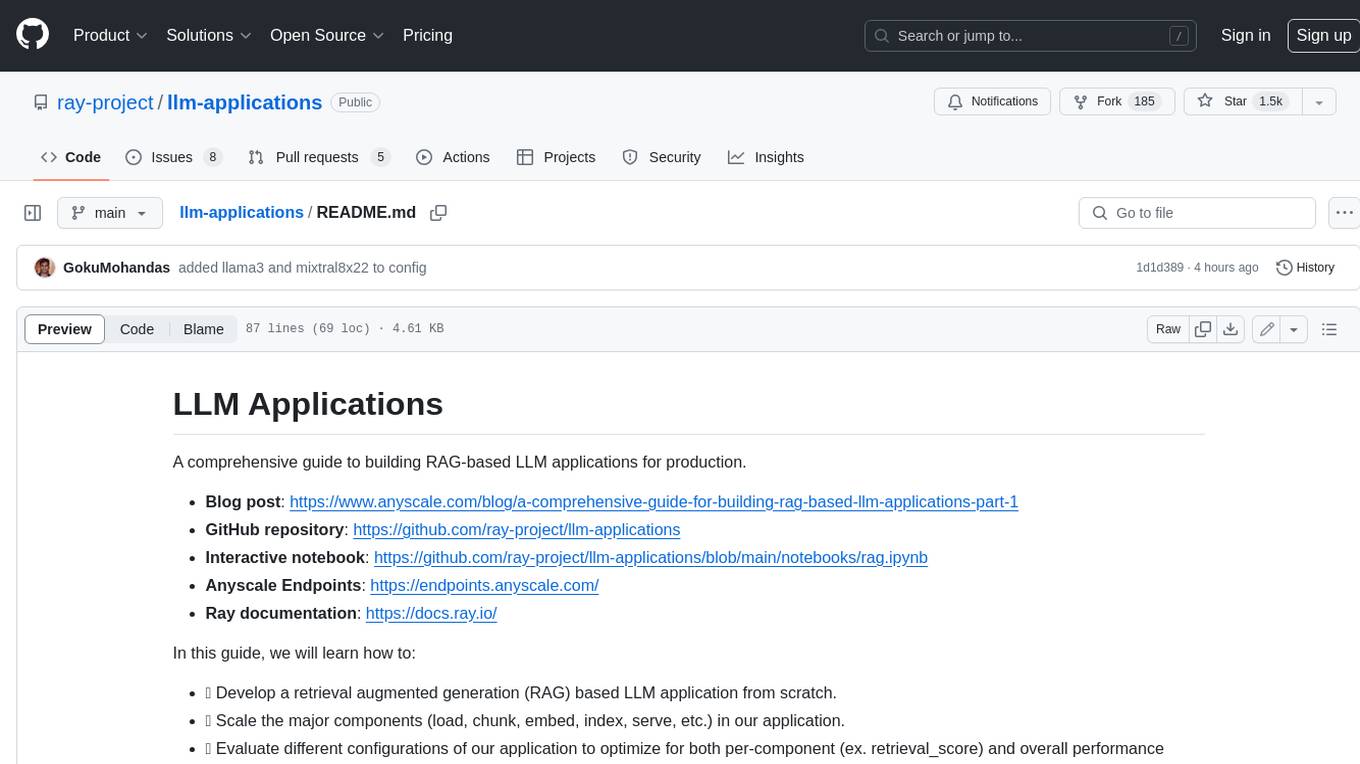
llm-applications
A comprehensive guide to building Retrieval Augmented Generation (RAG)-based LLM applications for production. This guide covers developing a RAG-based LLM application from scratch, scaling the major components, evaluating different configurations, implementing LLM hybrid routing, serving the application in a highly scalable and available manner, and sharing the impacts LLM applications have had on products.
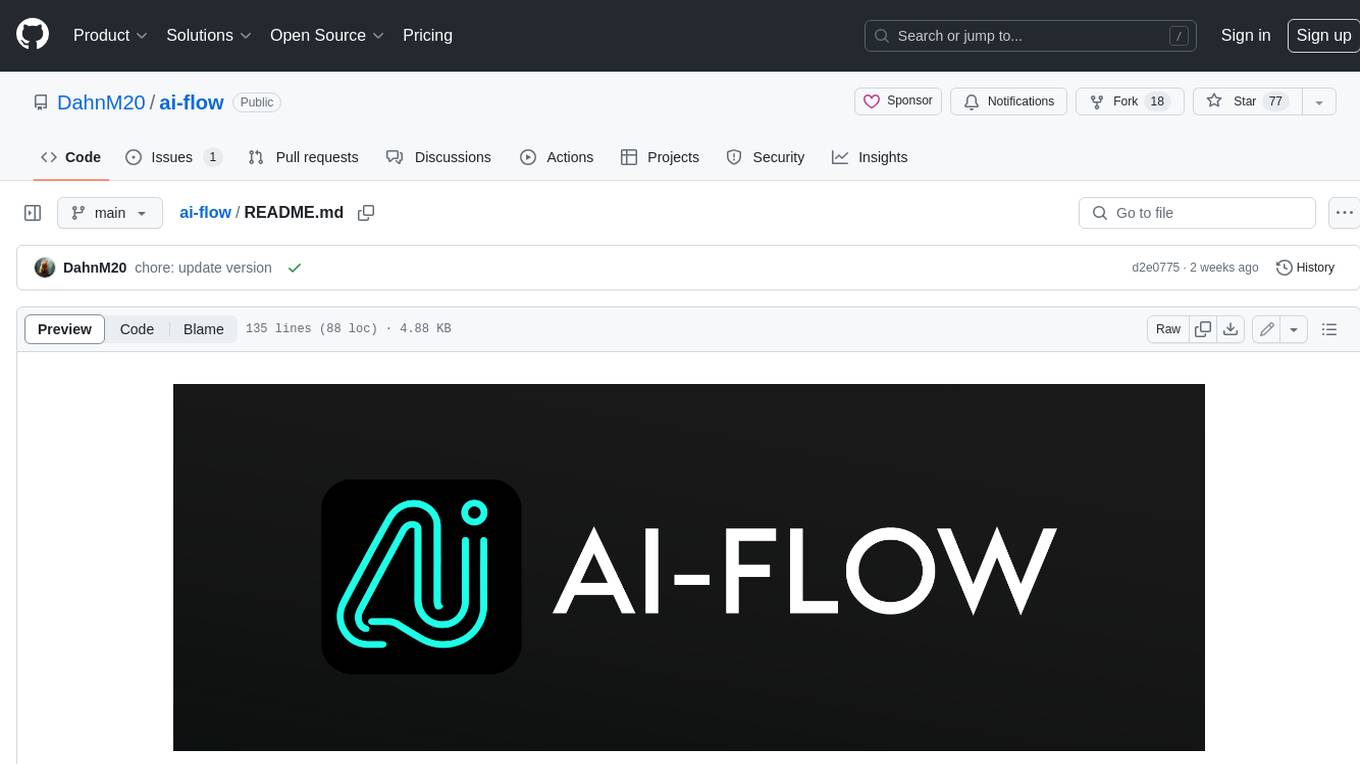
ai-flow
AI Flow is an open-source, user-friendly UI application that empowers you to seamlessly connect multiple AI models together, specifically leveraging the capabilities of multiples AI APIs such as OpenAI, StabilityAI and Replicate. In a nutshell, AI Flow provides a visual platform for crafting and managing AI-driven workflows, thereby facilitating diverse and dynamic AI interactions.
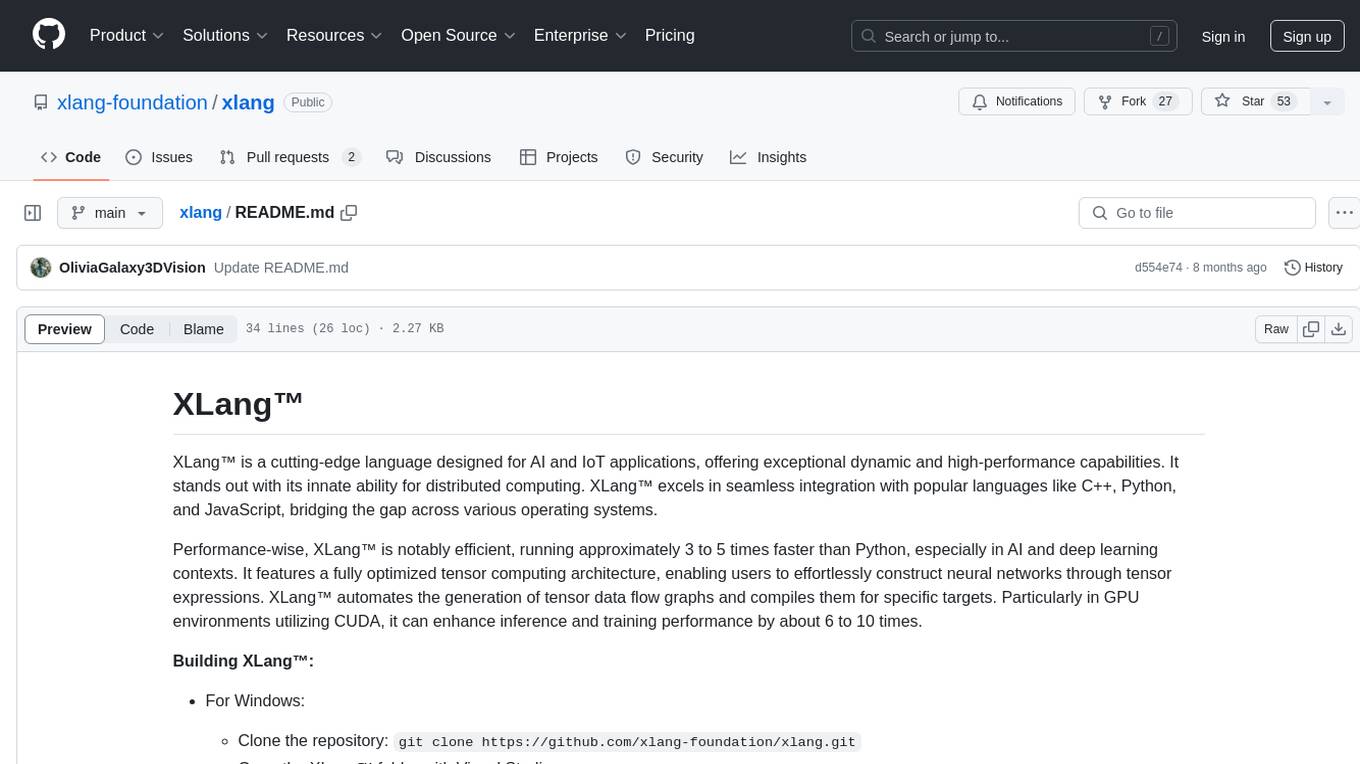
xlang
XLangβ’ is a cutting-edge language designed for AI and IoT applications, offering exceptional dynamic and high-performance capabilities. It excels in distributed computing and seamless integration with popular languages like C++, Python, and JavaScript. Notably efficient, running 3 to 5 times faster than Python in AI and deep learning contexts. Features optimized tensor computing architecture for constructing neural networks through tensor expressions. Automates tensor data flow graph generation and compilation for specific targets, enhancing GPU performance by 6 to 10 times in CUDA environments.
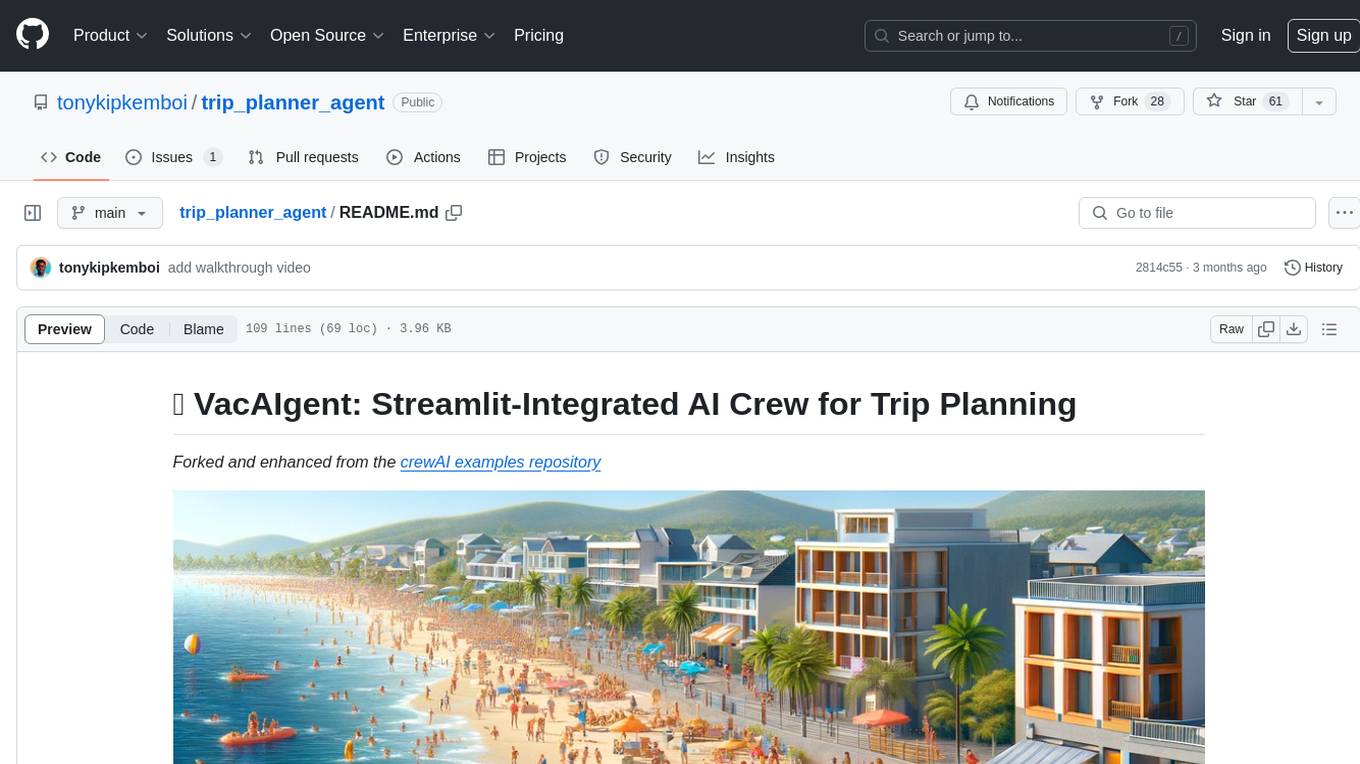
trip_planner_agent
VacAIgent is an AI tool that automates and enhances trip planning by leveraging the CrewAI framework. It integrates a user-friendly Streamlit interface for interactive travel planning. Users can input preferences and receive tailored travel plans with the help of autonomous AI agents. The tool allows for collaborative decision-making on cities and crafting complete itineraries based on specified preferences, all accessible via a streamlined Streamlit user interface. VacAIgent can be customized to use different AI models like GPT-3.5 or local models like Ollama for enhanced privacy and customization.
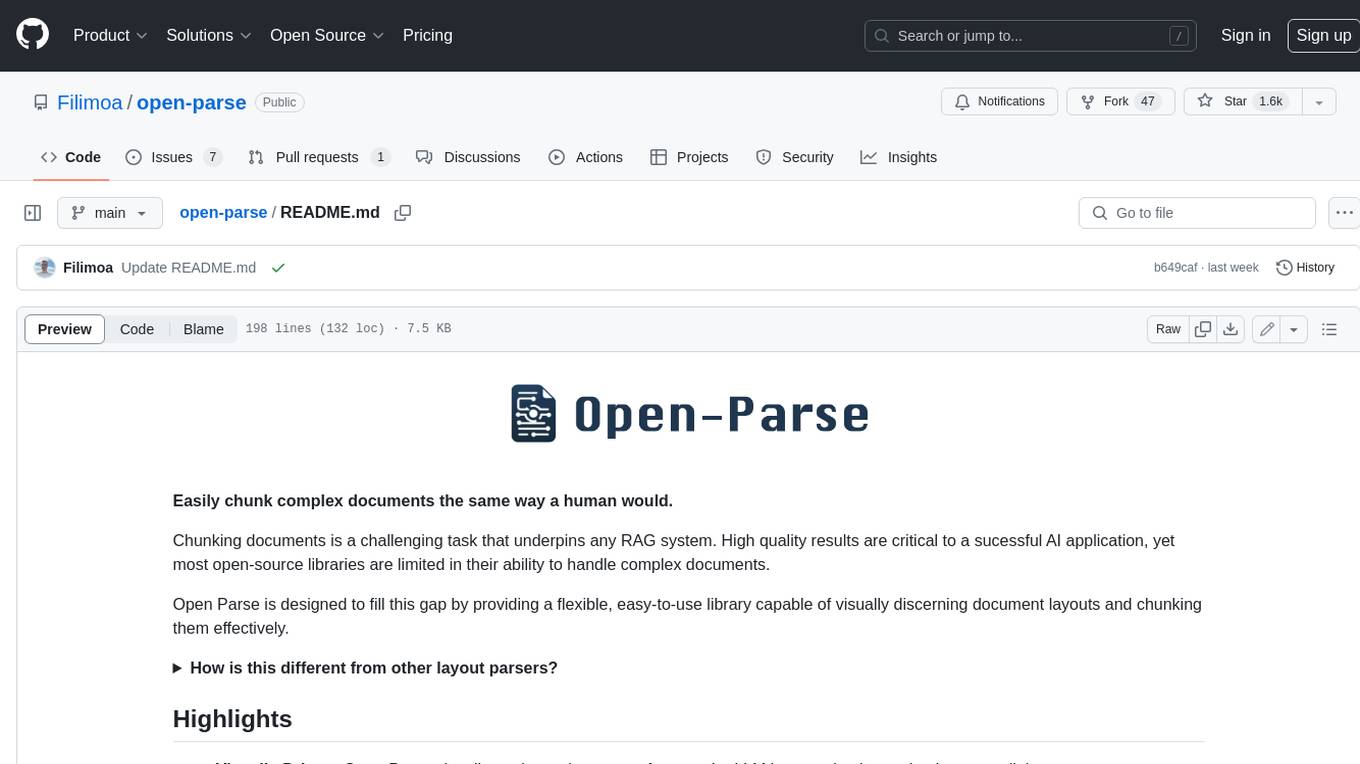
open-parse
Open Parse is a Python library for visually discerning document layouts and chunking them effectively. It is designed to fill the gap in open-source libraries for handling complex documents. Unlike text splitting, which converts a file to raw text and slices it up, Open Parse visually analyzes documents for superior LLM input. It also supports basic markdown for parsing headings, bold, and italics, and has high-precision table support, extracting tables into clean Markdown formats with accuracy that surpasses traditional tools. Open Parse is extensible, allowing users to easily implement their own post-processing steps. It is also intuitive, with great editor support and completion everywhere, making it easy to use and learn.
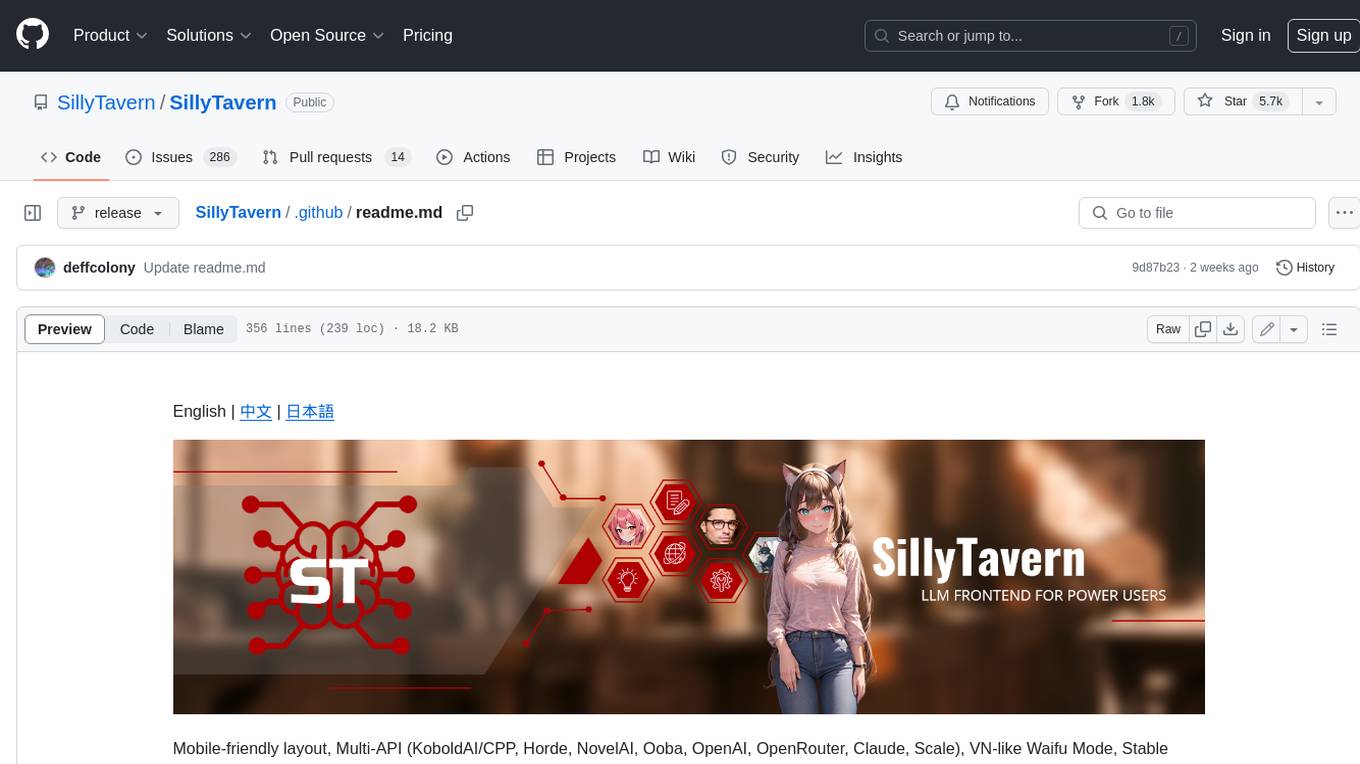
SillyTavern
SillyTavern is a user interface you can install on your computer (and Android phones) that allows you to interact with text generation AIs and chat/roleplay with characters you or the community create. SillyTavern is a fork of TavernAI 1.2.8 which is under more active development and has added many major features. At this point, they can be thought of as completely independent programs.
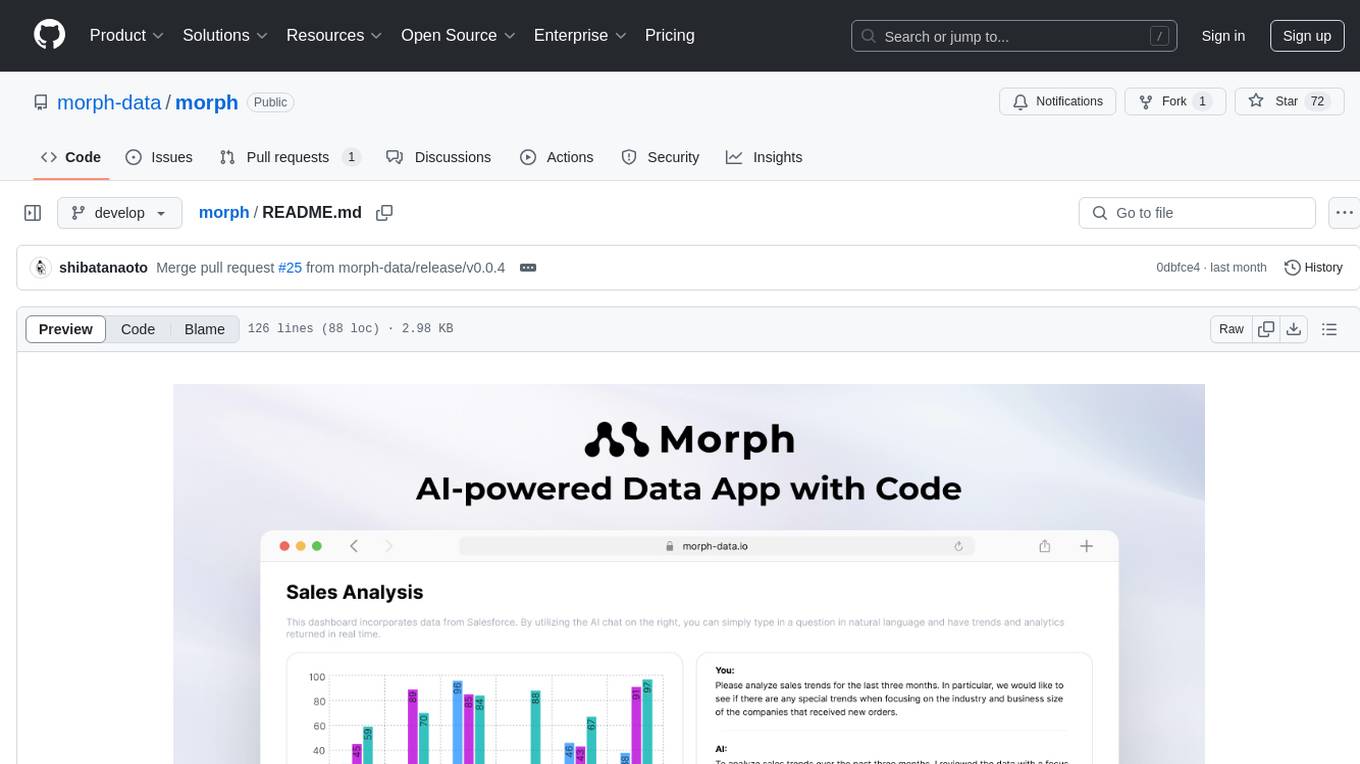
morph
Morph is a python-centric full-stack framework for building and deploying data apps. It is fast to start, deploy and operate, requires no HTML/CSS knowledge, and is customizable with Python and SQL for advanced data workflows. With Markdown-based syntax and pre-made components, users can create visually appealing designs without writing HTML or CSS.
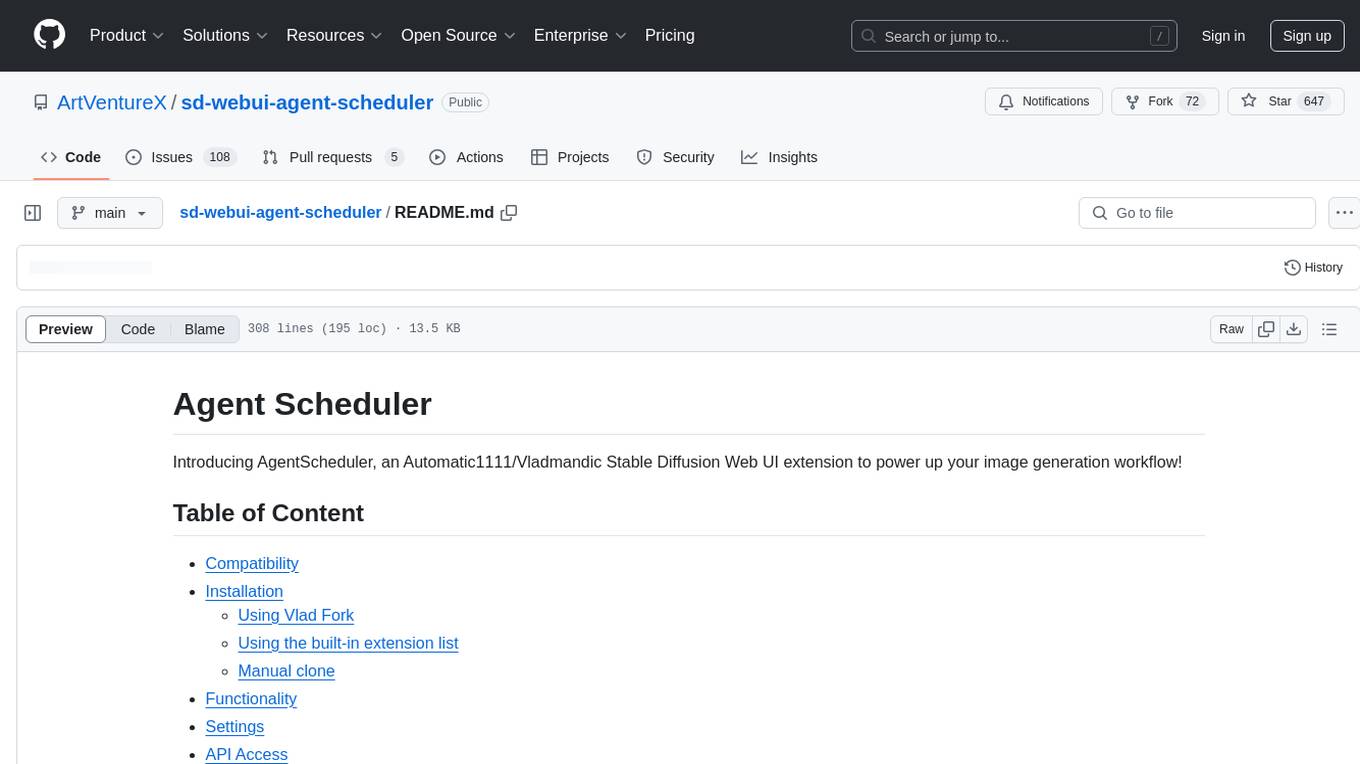
sd-webui-agent-scheduler
AgentScheduler is an Automatic/Vladmandic Stable Diffusion Web UI extension designed to enhance image generation workflows. It allows users to enqueue prompts, settings, and controlnets, manage queued tasks, prioritize, pause, resume, and delete tasks, view generation results, and more. The extension offers hidden features like queuing checkpoints, editing queued tasks, and custom checkpoint selection. Users can access the functionality through HTTP APIs and API callbacks. Troubleshooting steps are provided for common errors. The extension is compatible with latest versions of A1111 and Vladmandic. It is licensed under Apache License 2.0.
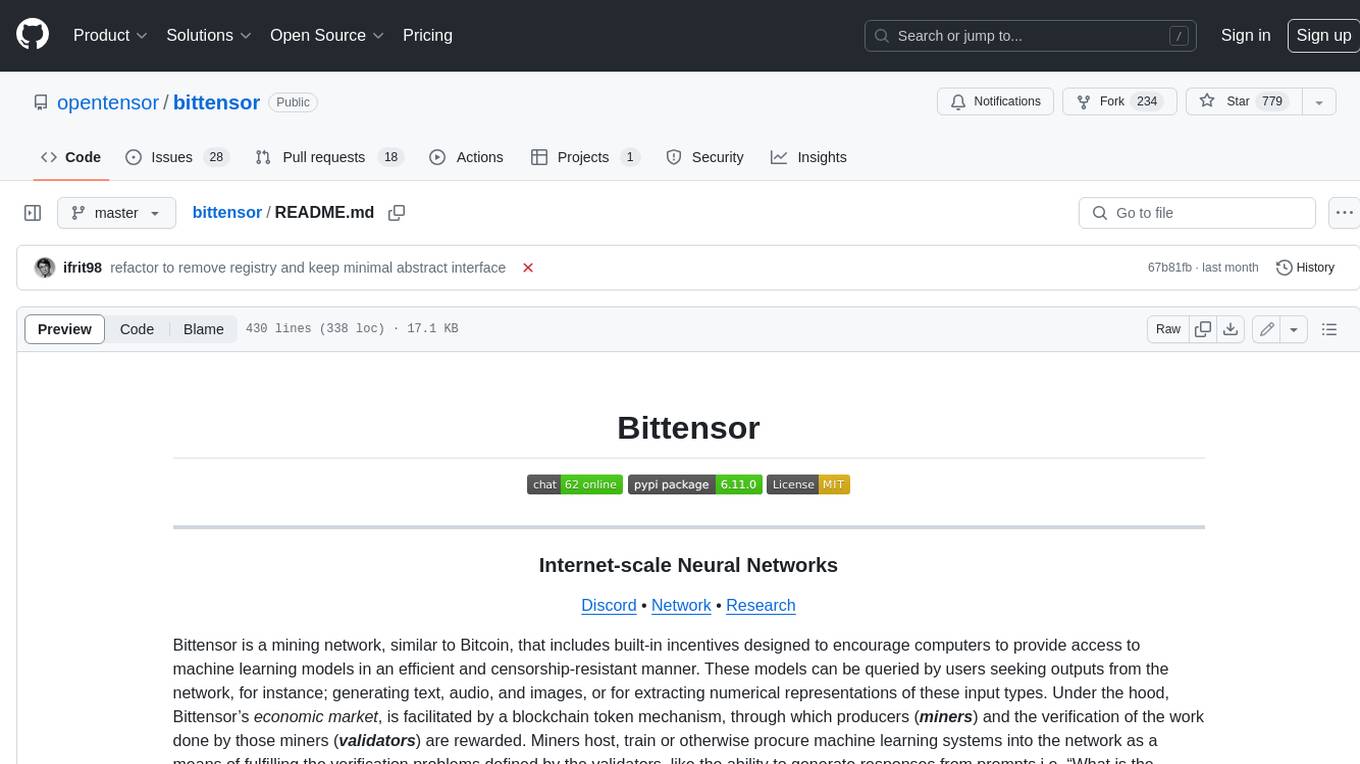
bittensor
Bittensor is an internet-scale neural network that incentivizes computers to provide access to machine learning models in a decentralized and censorship-resistant manner. It operates through a token-based mechanism where miners host, train, and procure machine learning systems to fulfill verification problems defined by validators. The network rewards miners and validators for their contributions, ensuring continuous improvement in knowledge output. Bittensor allows anyone to participate, extract value, and govern the network without centralized control. It supports tasks such as generating text, audio, images, and extracting numerical representations.
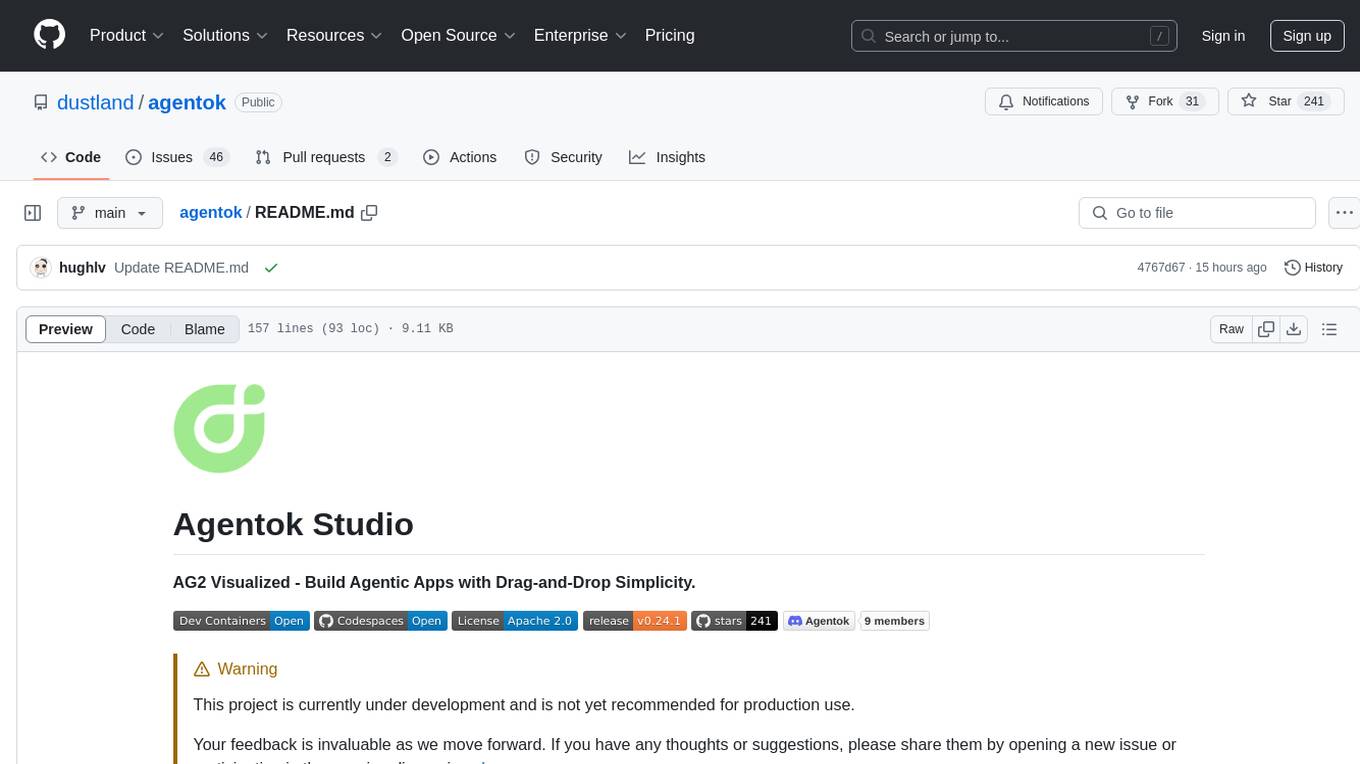
agentok
Agentok Studio is a tool built upon AG2, a powerful agent framework from Microsoft, offering intuitive visual tools to streamline the creation and management of complex agent-based workflows. It simplifies the process for creators and developers by generating native Python code with minimal dependencies, enabling users to create self-contained code that can be executed anywhere. The tool is currently under development and not recommended for production use, but contributions are welcome from the community to enhance its capabilities and functionalities.
For similar tasks
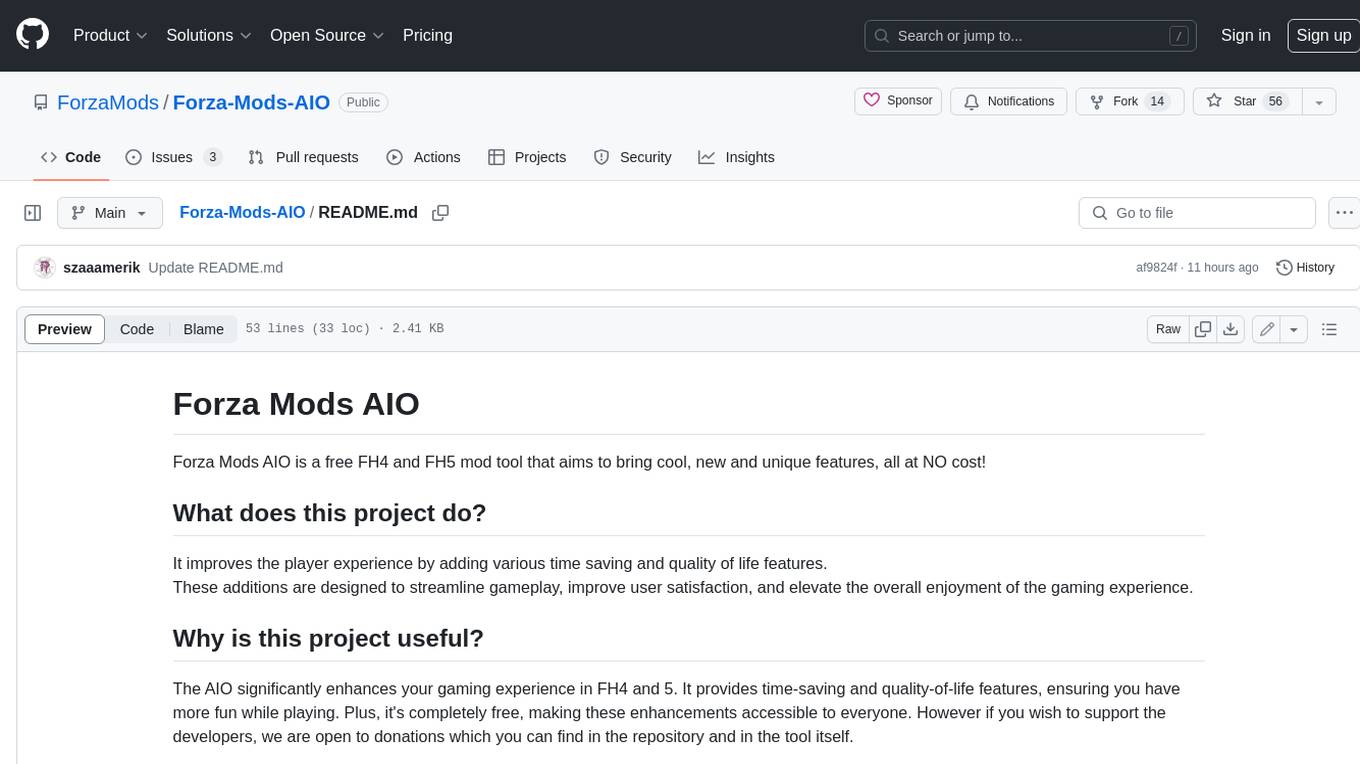
Forza-Mods-AIO
Forza Mods AIO is a free and open-source tool that enhances the gaming experience in Forza Horizon 4 and 5. It offers a range of time-saving and quality-of-life features, making gameplay more enjoyable and efficient. The tool is designed to streamline various aspects of the game, improving user satisfaction and overall enjoyment.
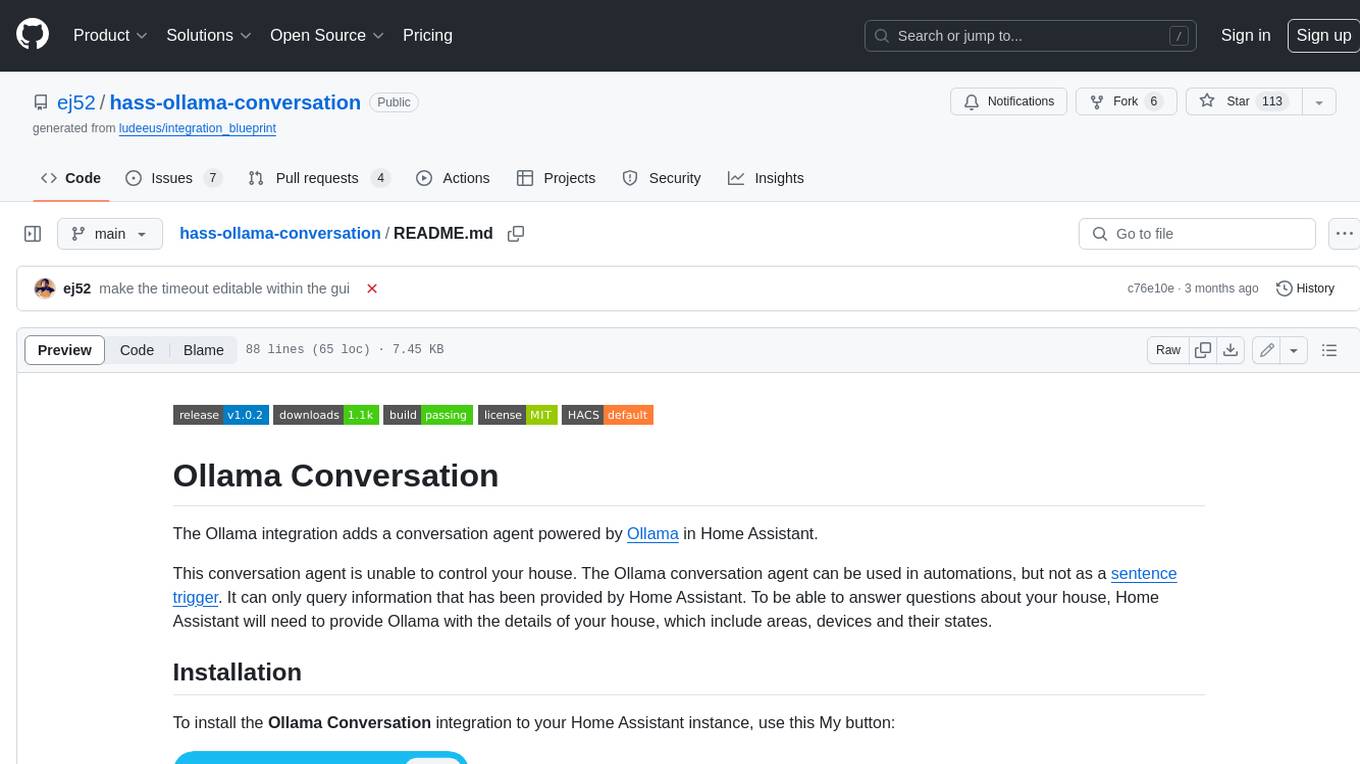
hass-ollama-conversation
The Ollama Conversation integration adds a conversation agent powered by Ollama in Home Assistant. This agent can be used in automations to query information provided by Home Assistant about your house, including areas, devices, and their states. Users can install the integration via HACS and configure settings such as API timeout, model selection, context size, maximum tokens, and other parameters to fine-tune the responses generated by the AI language model. Contributions to the project are welcome, and discussions can be held on the Home Assistant Community platform.
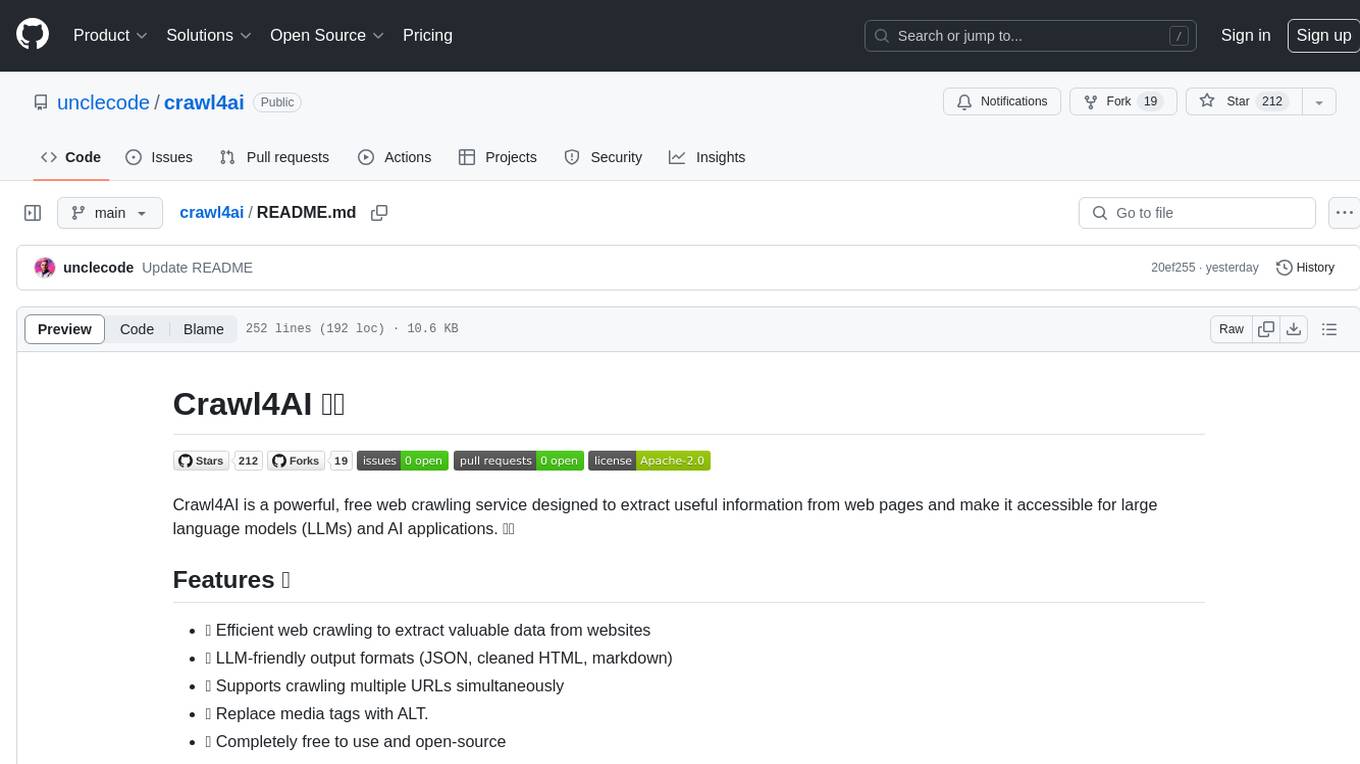
crawl4ai
Crawl4AI is a powerful and free web crawling service that extracts valuable data from websites and provides LLM-friendly output formats. It supports crawling multiple URLs simultaneously, replaces media tags with ALT, and is completely free to use and open-source. Users can integrate Crawl4AI into Python projects as a library or run it as a standalone local server. The tool allows users to crawl and extract data from specified URLs using different providers and models, with options to include raw HTML content, force fresh crawls, and extract meaningful text blocks. Configuration settings can be adjusted in the `crawler/config.py` file to customize providers, API keys, chunk processing, and word thresholds. Contributions to Crawl4AI are welcome from the open-source community to enhance its value for AI enthusiasts and developers.
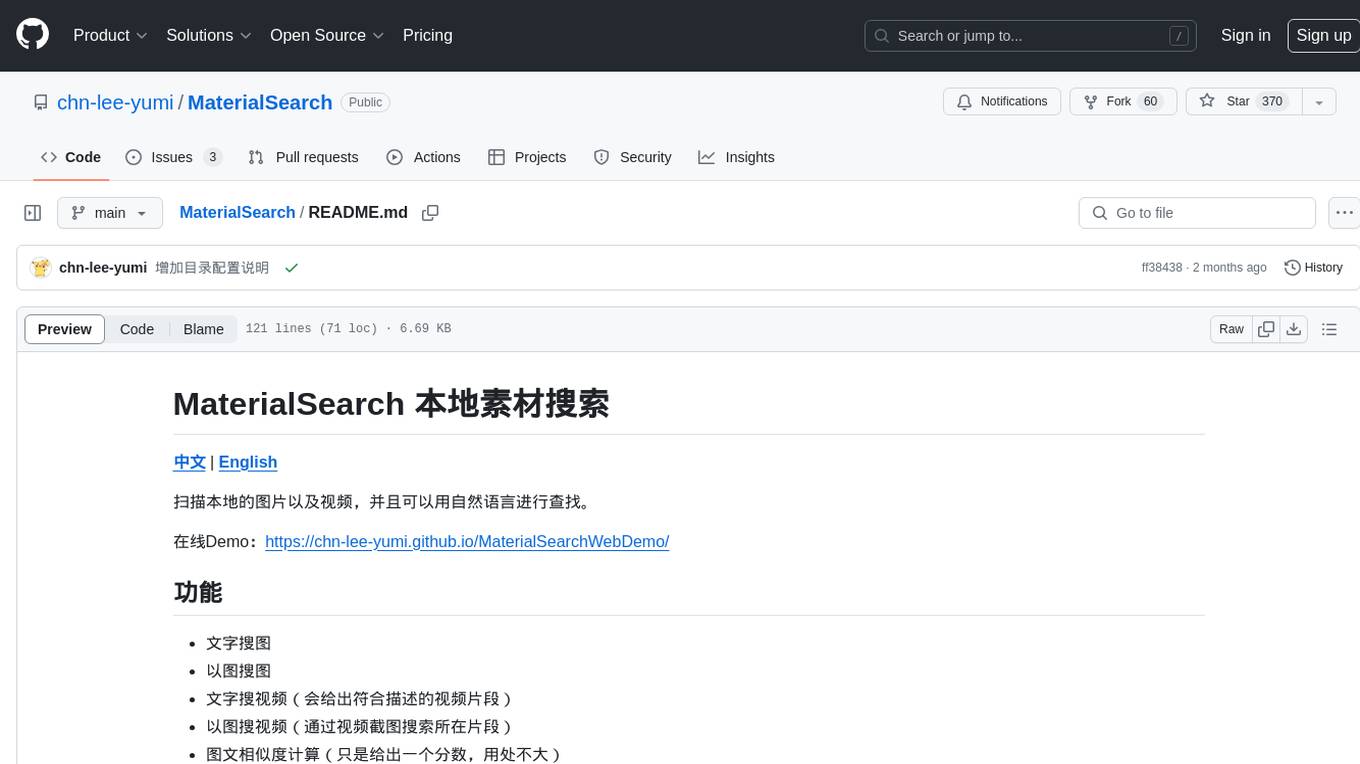
MaterialSearch
MaterialSearch is a tool for searching local images and videos using natural language. It provides functionalities such as text search for images, image search for images, text search for videos (providing matching video clips), image search for videos (searching for the segment in a video through a screenshot), image-text similarity calculation, and Pexels video search. The tool can be deployed through the source code or Docker image, and it supports GPU acceleration. Users can configure the tool through environment variables or a .env file. The tool is still under development, and configurations may change frequently. Users can report issues or suggest improvements through issues or pull requests.
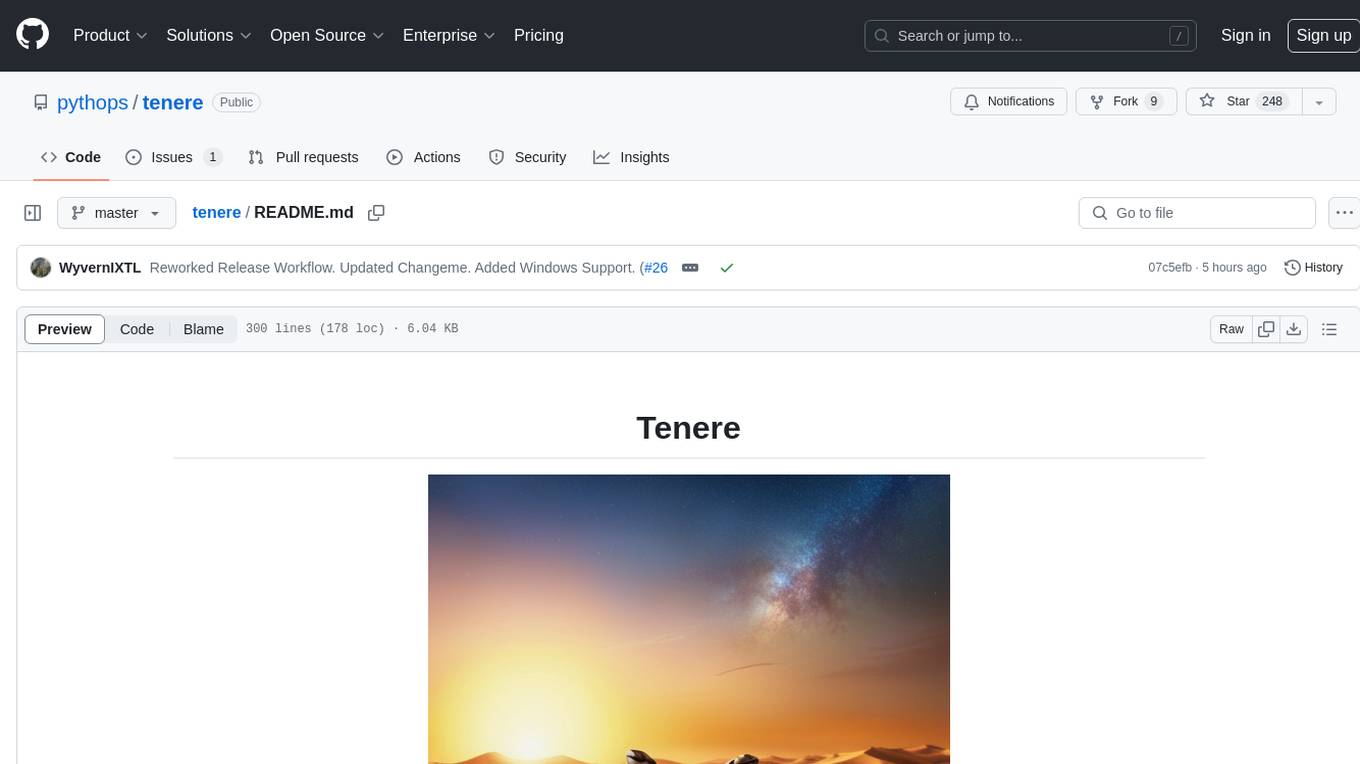
tenere
Tenere is a TUI interface for Language Model Libraries (LLMs) written in Rust. It provides syntax highlighting, chat history, saving chats to files, Vim keybindings, copying text from/to clipboard, and supports multiple backends. Users can configure Tenere using a TOML configuration file, set key bindings, and use different LLMs such as ChatGPT, llama.cpp, and ollama. Tenere offers default key bindings for global and prompt modes, with features like starting a new chat, saving chats, scrolling, showing chat history, and quitting the app. Users can interact with the prompt in different modes like Normal, Visual, and Insert, with various key bindings for navigation, editing, and text manipulation.
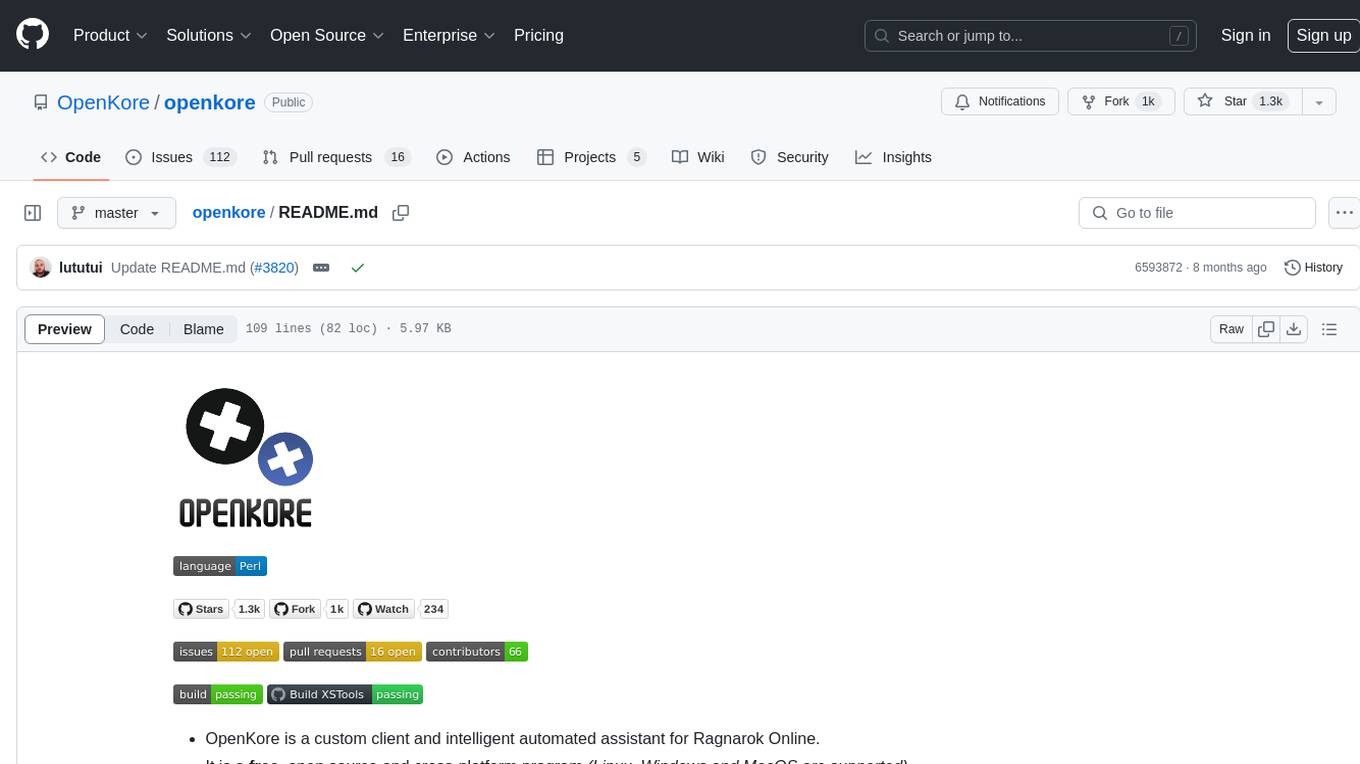
openkore
OpenKore is a custom client and intelligent automated assistant for Ragnarok Online. It is a free, open source, and cross-platform program (Linux, Windows, and MacOS are supported). To run OpenKore, you need to download and extract it or clone the repository using Git. Configure OpenKore according to the documentation and run openkore.pl to start. The tool provides a FAQ section for troubleshooting, guidelines for reporting issues, and information about botting status on official servers. OpenKore is developed by a global team, and contributions are welcome through pull requests. Various community resources are available for support and communication. Users are advised to comply with the GNU General Public License when using and distributing the software.
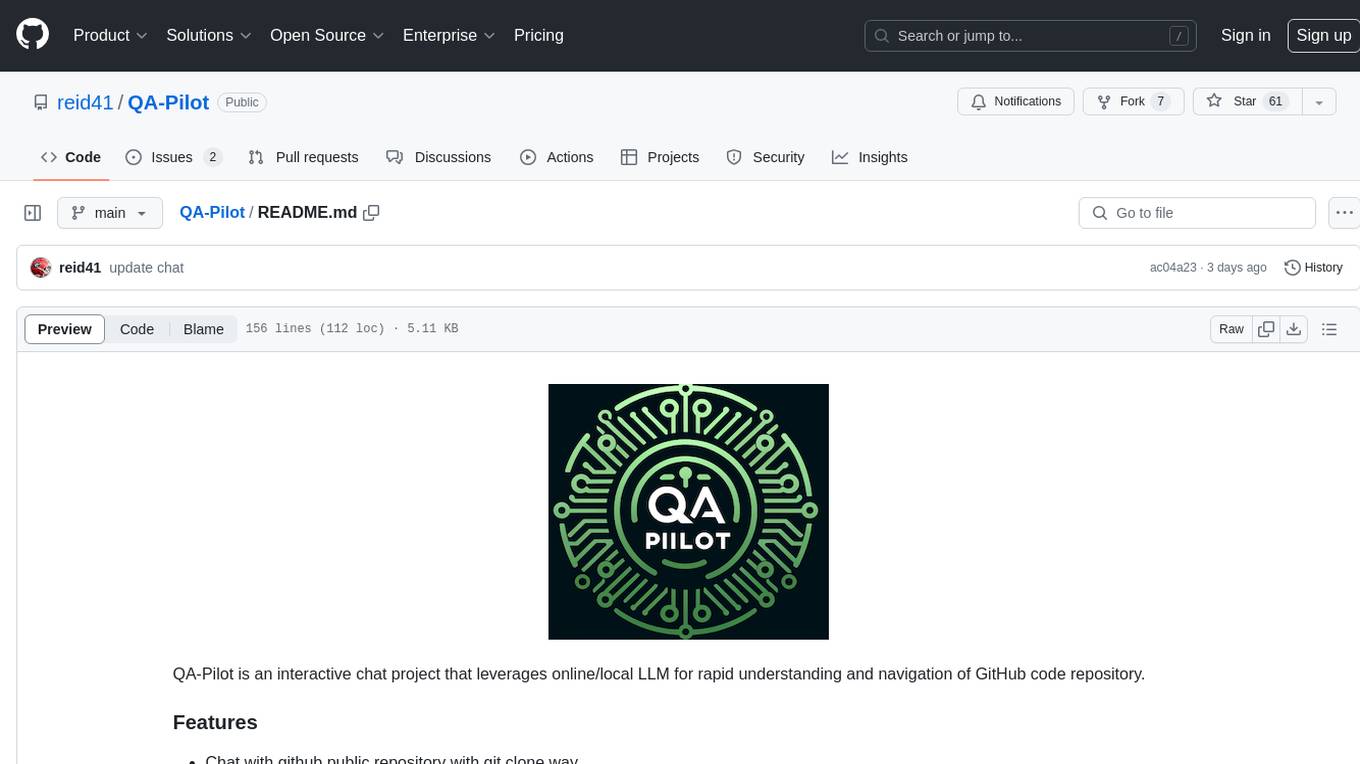
QA-Pilot
QA-Pilot is an interactive chat project that leverages online/local LLM for rapid understanding and navigation of GitHub code repository. It allows users to chat with GitHub public repositories using a git clone approach, store chat history, configure settings easily, manage multiple chat sessions, and quickly locate sessions with a search function. The tool integrates with `codegraph` to view Python files and supports various LLM models such as ollama, openai, mistralai, and localai. The project is continuously updated with new features and improvements, such as converting from `flask` to `fastapi`, adding `localai` API support, and upgrading dependencies like `langchain` and `Streamlit` to enhance performance.
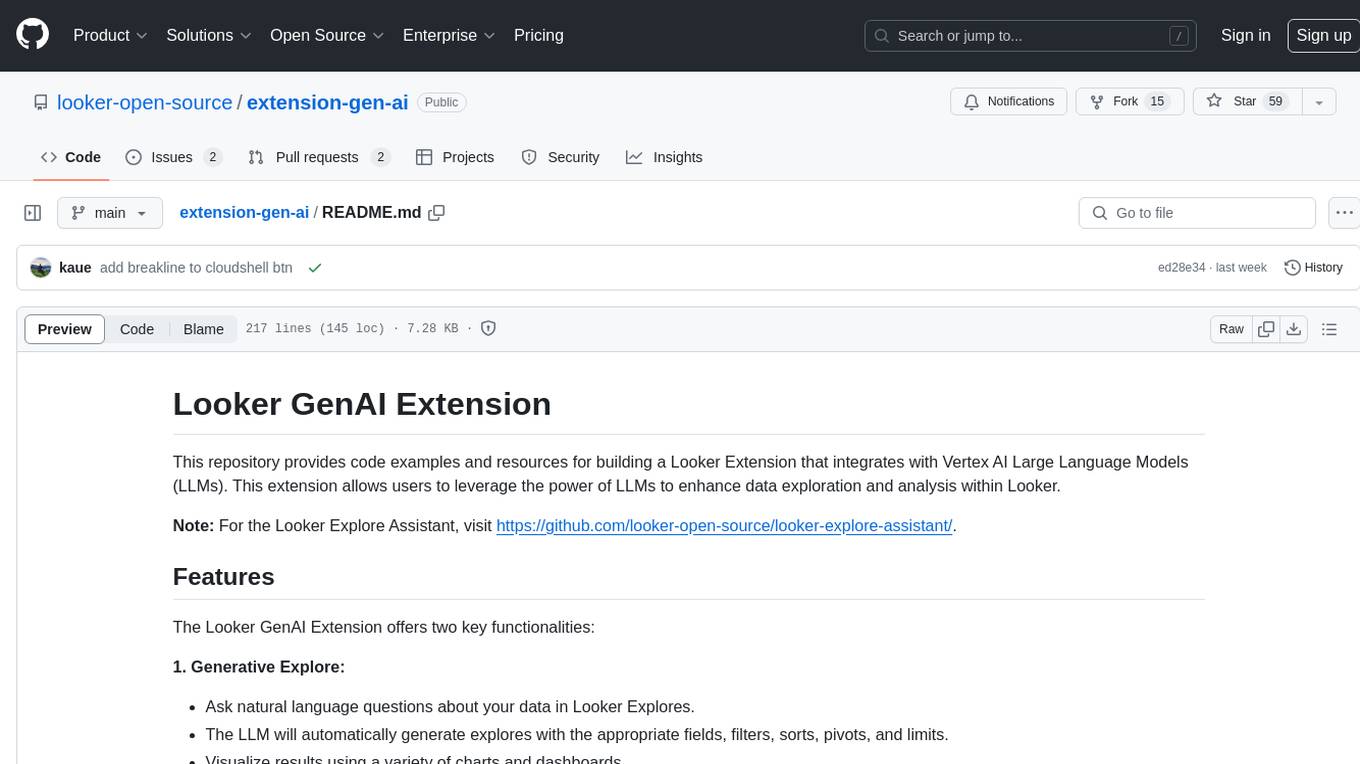
extension-gen-ai
The Looker GenAI Extension provides code examples and resources for building a Looker Extension that integrates with Vertex AI Large Language Models (LLMs). Users can leverage the power of LLMs to enhance data exploration and analysis within Looker. The extension offers generative explore functionality to ask natural language questions about data and generative insights on dashboards to analyze data by asking questions. It leverages components like BQML Remote Models, BQML Remote UDF with Vertex AI, and Custom Fine Tune Model for different integration options. Deployment involves setting up infrastructure with Terraform and deploying the Looker Extension by creating a Looker project, copying extension files, configuring BigQuery connection, connecting to Git, and testing the extension. Users can save example prompts and configure user settings for the extension. Development of the Looker Extension environment includes installing dependencies, starting the development server, and building for production.
For similar jobs
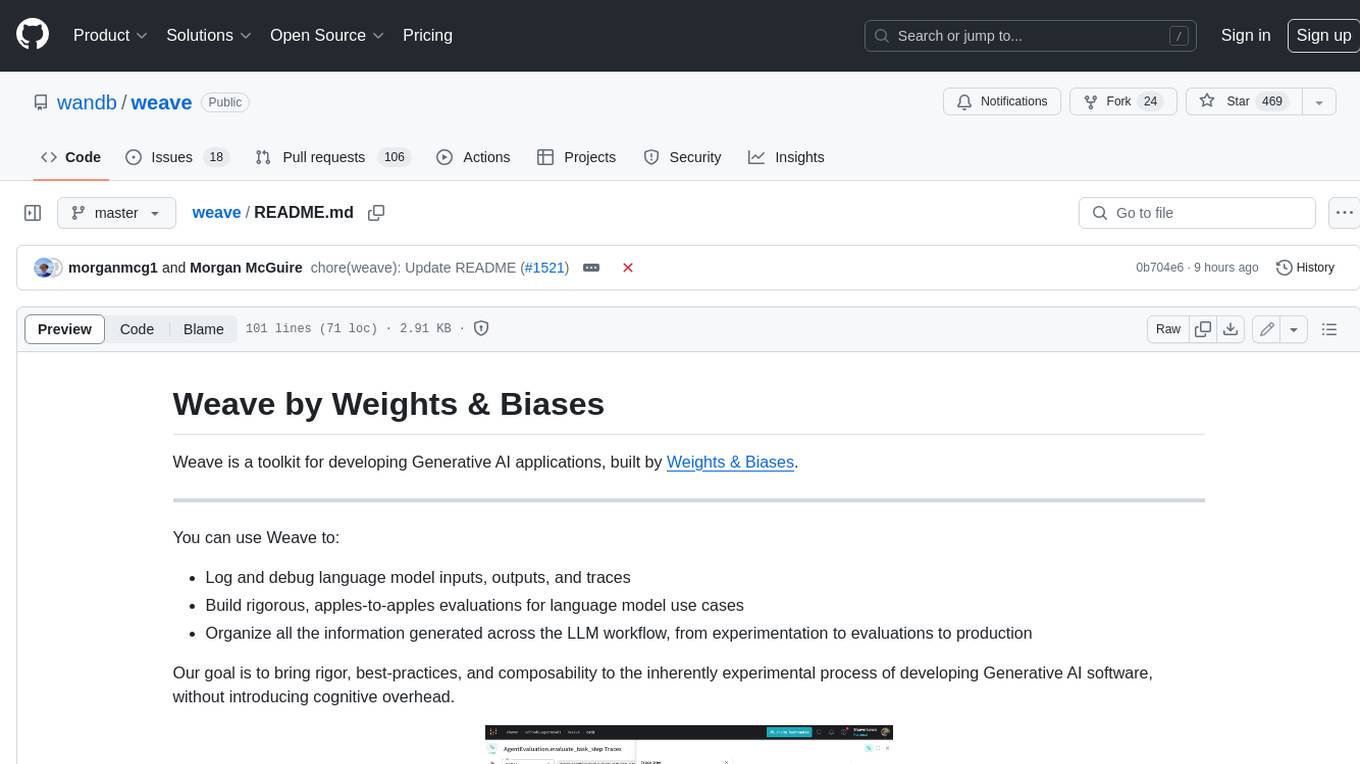
weave
Weave is a toolkit for developing Generative AI applications, built by Weights & Biases. With Weave, you can log and debug language model inputs, outputs, and traces; build rigorous, apples-to-apples evaluations for language model use cases; and organize all the information generated across the LLM workflow, from experimentation to evaluations to production. Weave aims to bring rigor, best-practices, and composability to the inherently experimental process of developing Generative AI software, without introducing cognitive overhead.
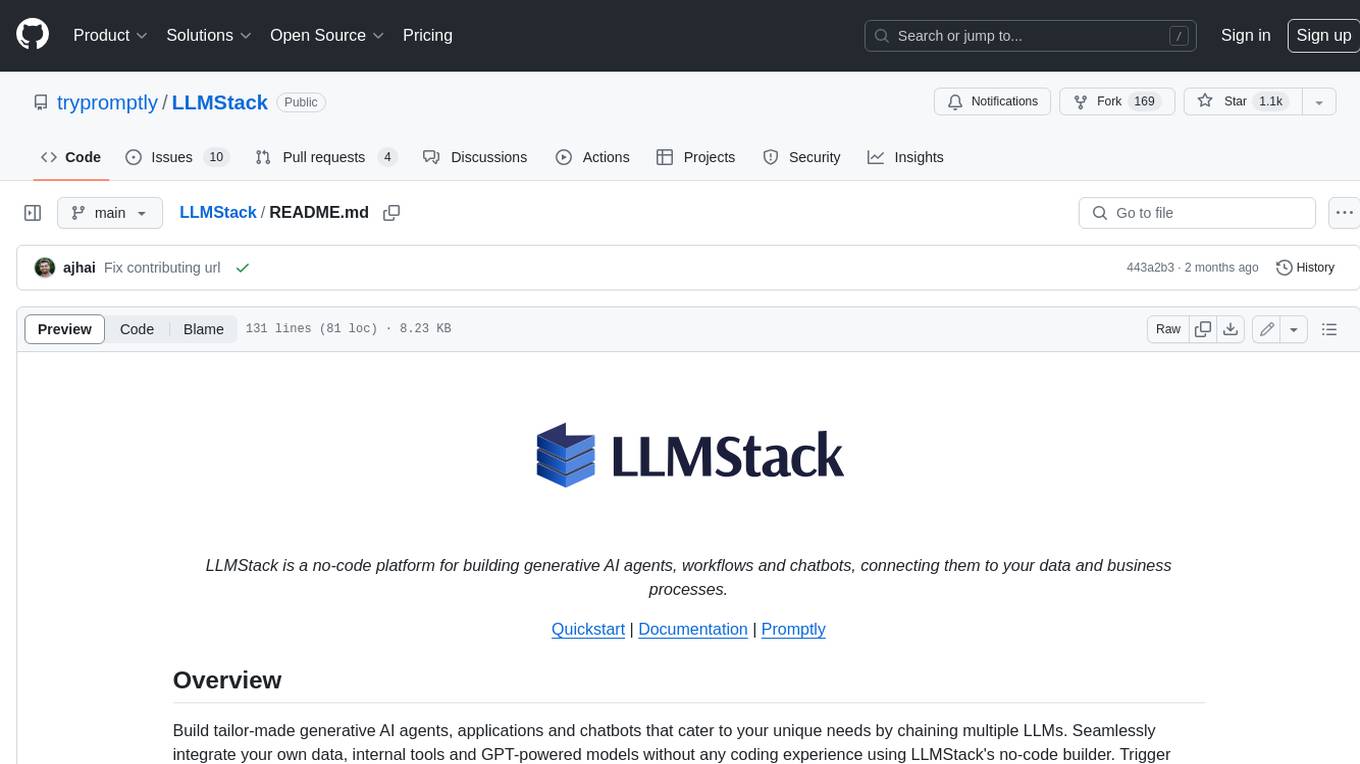
LLMStack
LLMStack is a no-code platform for building generative AI agents, workflows, and chatbots. It allows users to connect their own data, internal tools, and GPT-powered models without any coding experience. LLMStack can be deployed to the cloud or on-premise and can be accessed via HTTP API or triggered from Slack or Discord.
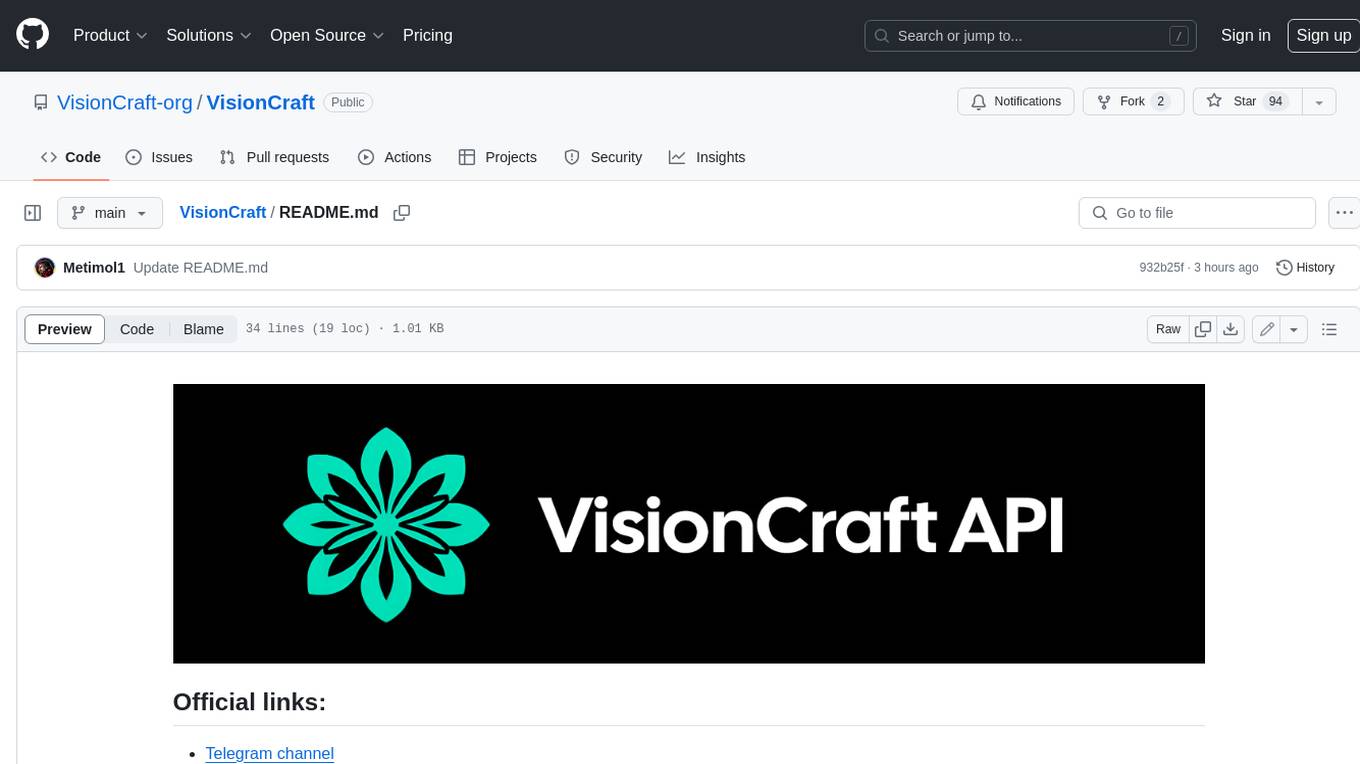
VisionCraft
The VisionCraft API is a free API for using over 100 different AI models. From images to sound.
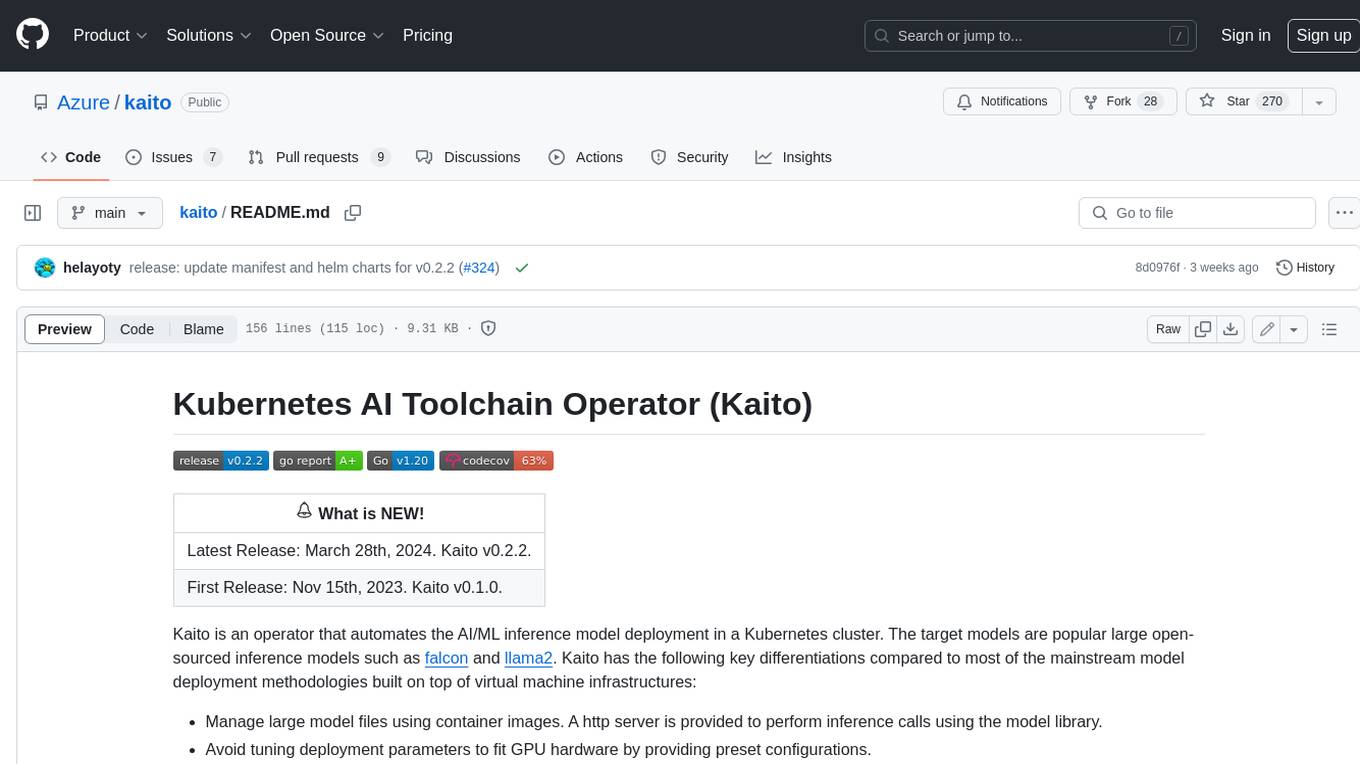
kaito
Kaito is an operator that automates the AI/ML inference model deployment in a Kubernetes cluster. It manages large model files using container images, avoids tuning deployment parameters to fit GPU hardware by providing preset configurations, auto-provisions GPU nodes based on model requirements, and hosts large model images in the public Microsoft Container Registry (MCR) if the license allows. Using Kaito, the workflow of onboarding large AI inference models in Kubernetes is largely simplified.
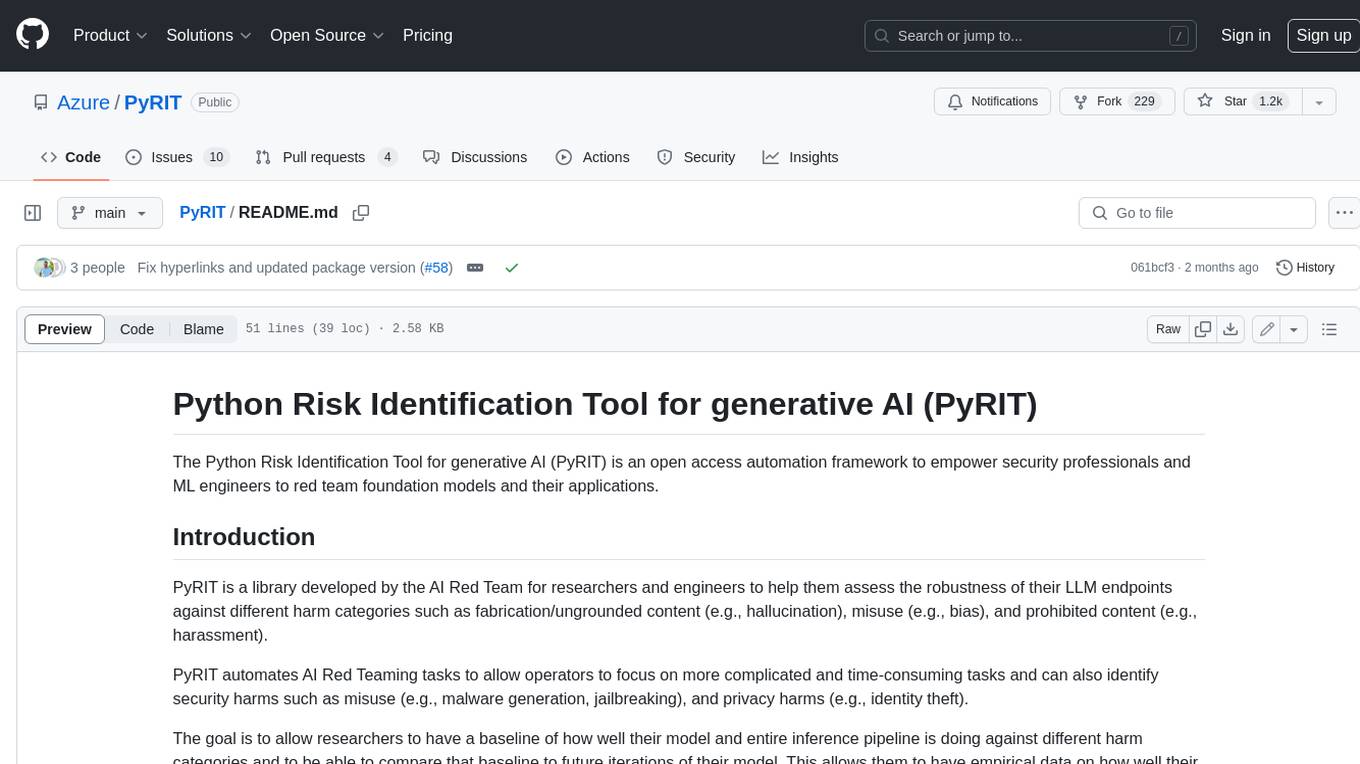
PyRIT
PyRIT is an open access automation framework designed to empower security professionals and ML engineers to red team foundation models and their applications. It automates AI Red Teaming tasks to allow operators to focus on more complicated and time-consuming tasks and can also identify security harms such as misuse (e.g., malware generation, jailbreaking), and privacy harms (e.g., identity theft). The goal is to allow researchers to have a baseline of how well their model and entire inference pipeline is doing against different harm categories and to be able to compare that baseline to future iterations of their model. This allows them to have empirical data on how well their model is doing today, and detect any degradation of performance based on future improvements.
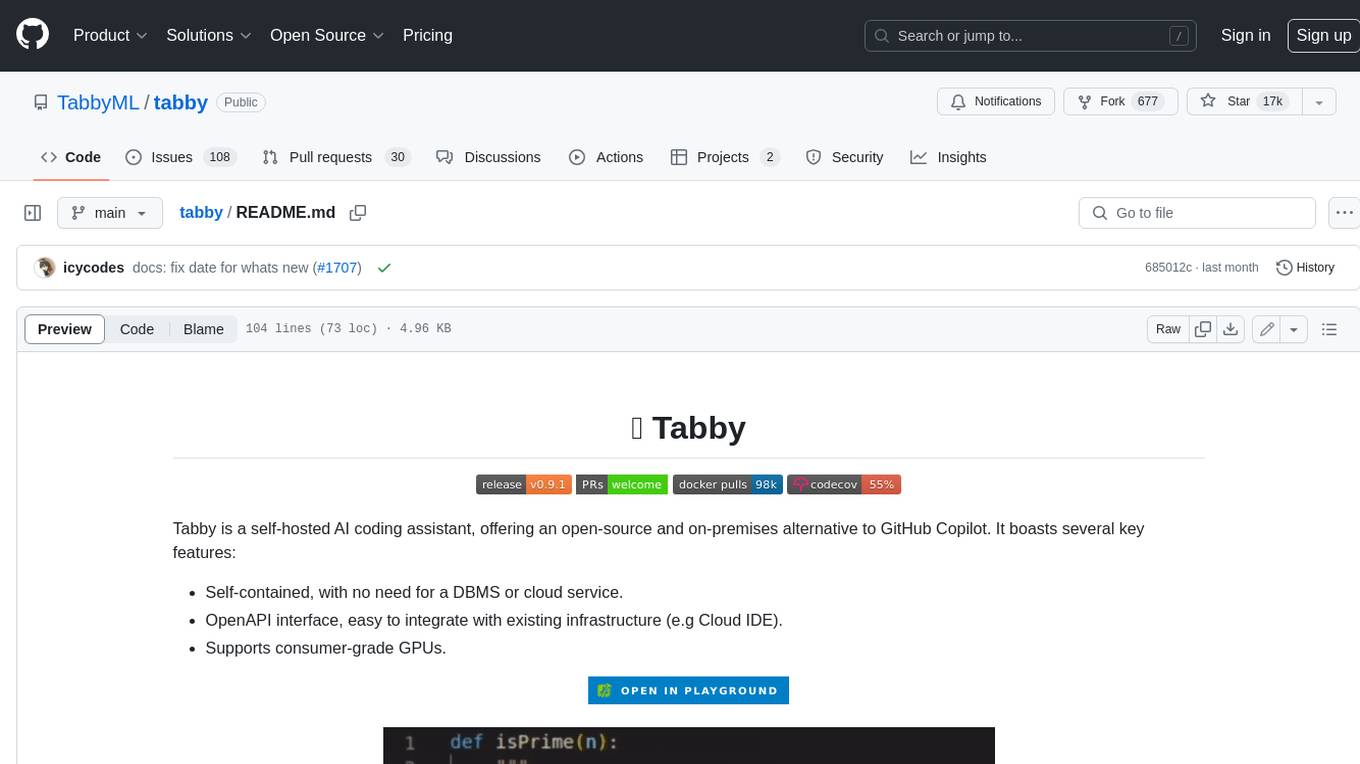
tabby
Tabby is a self-hosted AI coding assistant, offering an open-source and on-premises alternative to GitHub Copilot. It boasts several key features: * Self-contained, with no need for a DBMS or cloud service. * OpenAPI interface, easy to integrate with existing infrastructure (e.g Cloud IDE). * Supports consumer-grade GPUs.
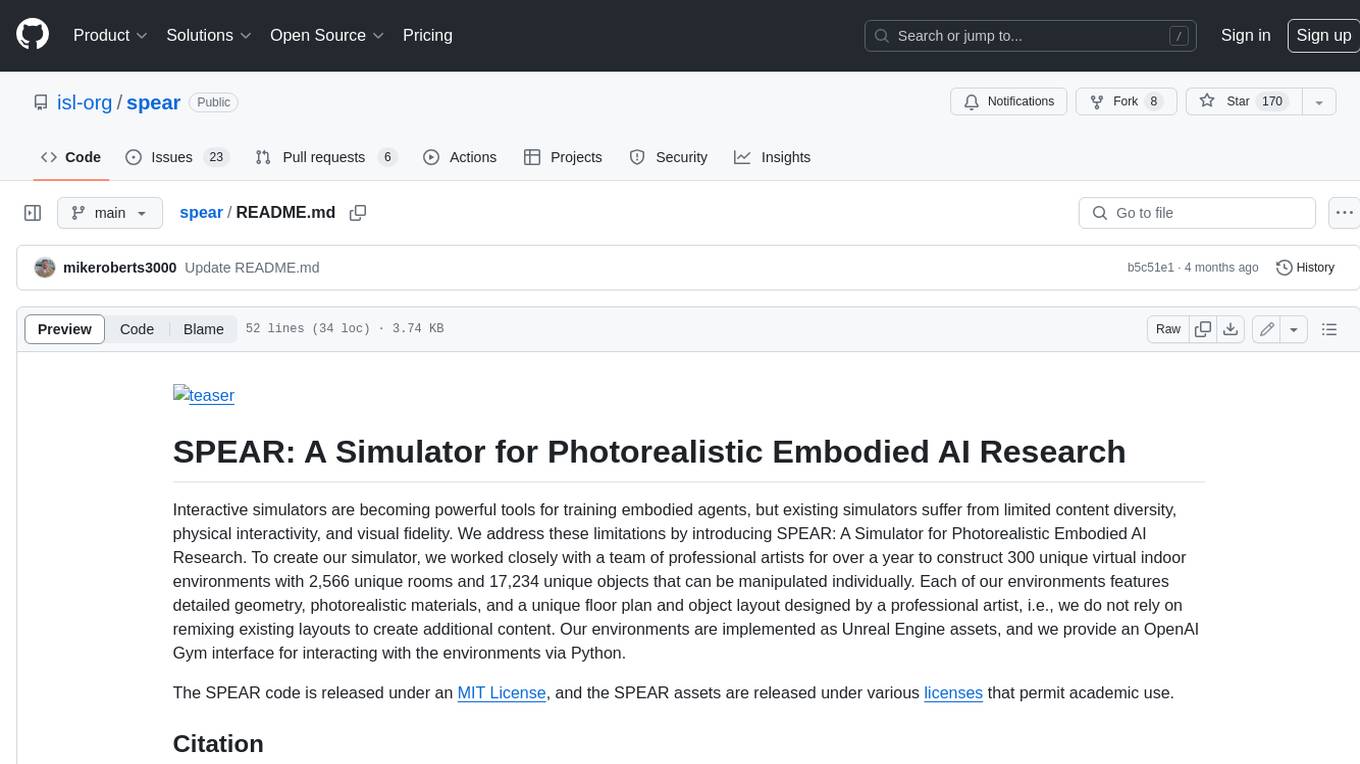
spear
SPEAR (Simulator for Photorealistic Embodied AI Research) is a powerful tool for training embodied agents. It features 300 unique virtual indoor environments with 2,566 unique rooms and 17,234 unique objects that can be manipulated individually. Each environment is designed by a professional artist and features detailed geometry, photorealistic materials, and a unique floor plan and object layout. SPEAR is implemented as Unreal Engine assets and provides an OpenAI Gym interface for interacting with the environments via Python.
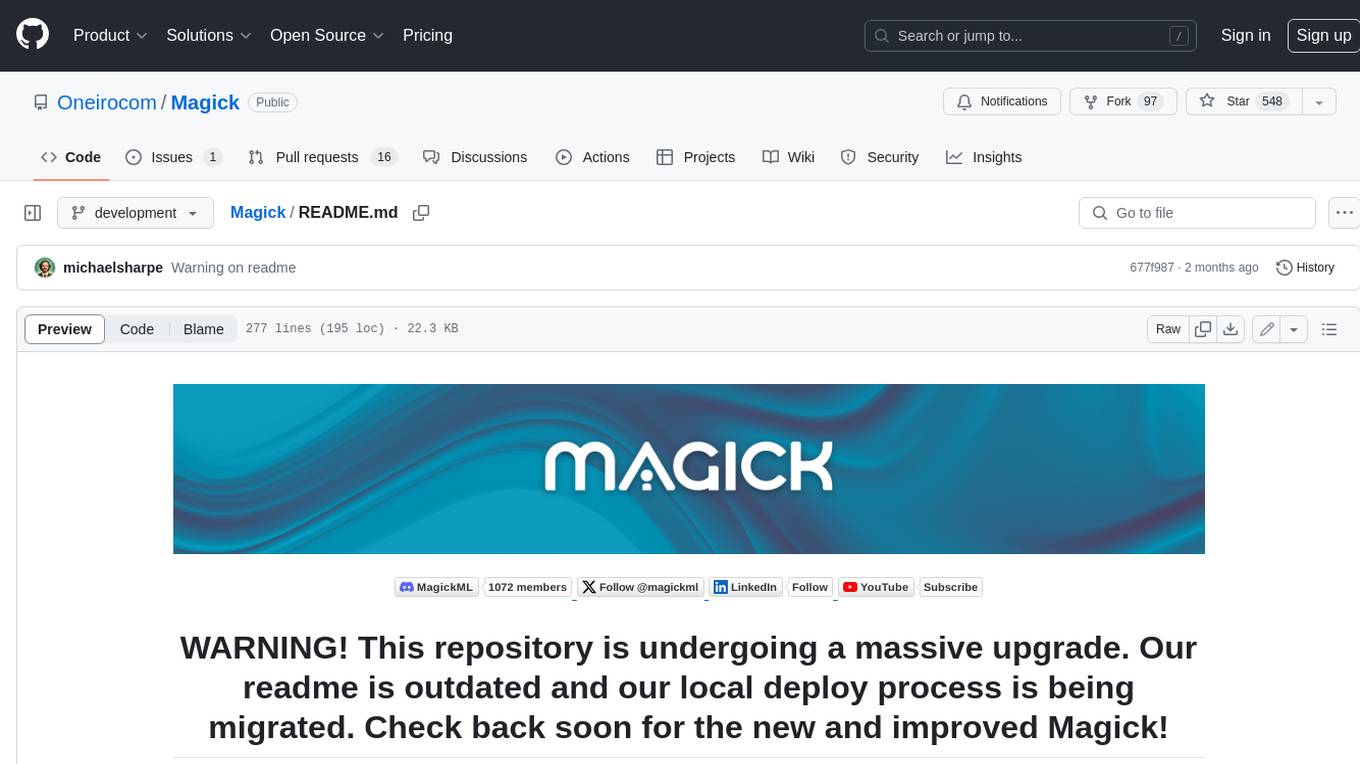
Magick
Magick is a groundbreaking visual AIDE (Artificial Intelligence Development Environment) for no-code data pipelines and multimodal agents. Magick can connect to other services and comes with nodes and templates well-suited for intelligent agents, chatbots, complex reasoning systems and realistic characters.