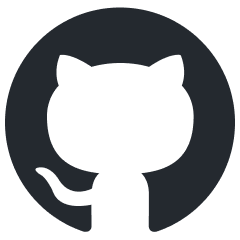
cosdata
Cosdata: A cutting-edge AI data platform for next-gen search pipelines. Features semantic search, knowledge graphs, hybrid capabilities, real-time scalability, and ML integration. Designed for immutability and version control to enhance AI projects.
Stars: 110
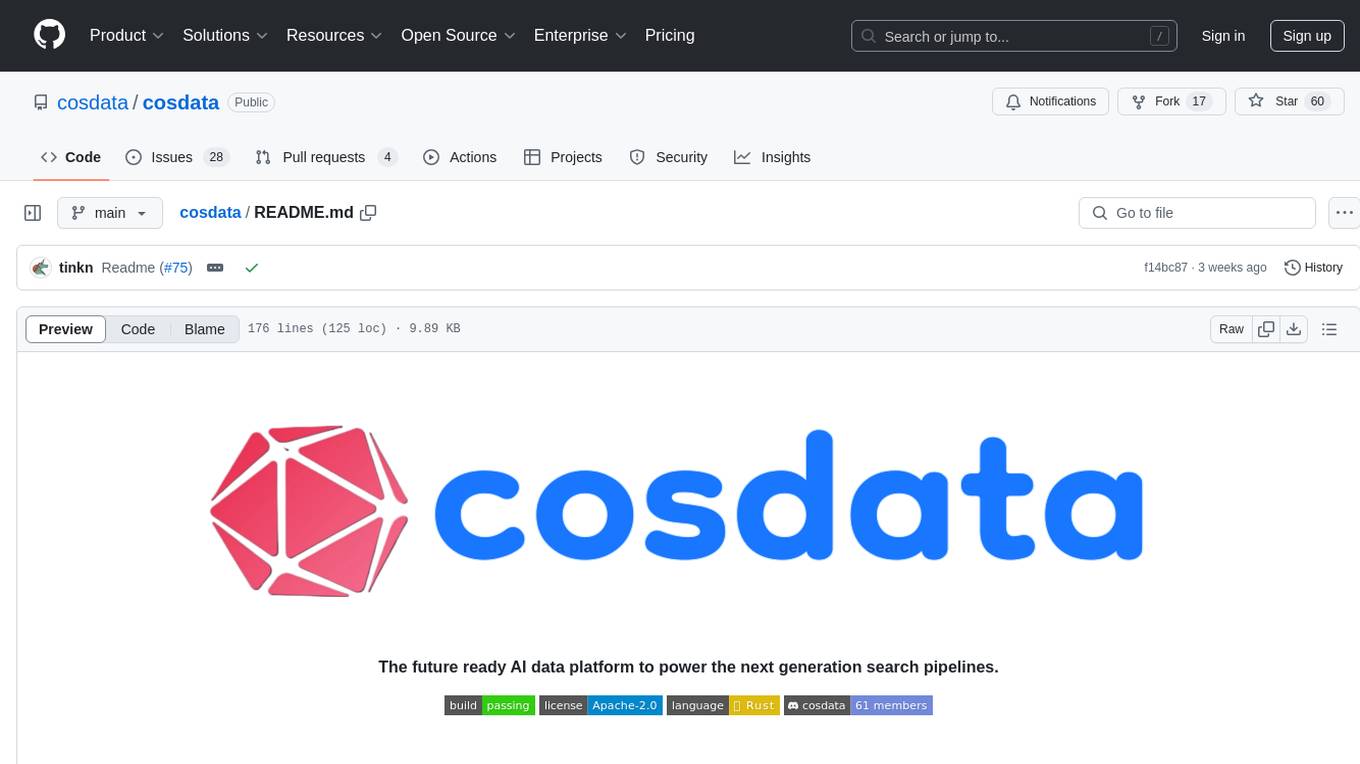
Cosdata is a cutting-edge AI data platform designed to power the next generation search pipelines. It features immutability, version control, and excels in semantic search, structured knowledge graphs, hybrid search capabilities, real-time search at scale, and ML pipeline integration. The platform is customizable, scalable, efficient, enterprise-grade, easy to use, and can manage multi-modal data. It offers high performance, indexing, low latency, and high requests per second. Cosdata is designed to meet the demands of modern search applications, empowering businesses to harness the full potential of their data.
README:
Next-gen vector database delivering lightning-fast performance at billion-vector scale.
Cosdata is cutting-edge AI data platform engineered for exceptional performance. Featuring immutability and version control, it's designed to elevate a wide range of AI and machine learning projects.
Cosdata is at the forefront of advancing search technology, excelling in critical areas that will define the next generation of search pipelines:
- Semantic Search: Leverage embedding-based hybrid search, seamlessly managing both dense and sparse vectors to deliver deep semantic analysis.
- Structured Knowledge Graphs: Sophisticated context retrieval by leveraging structured knowledge graphs.
- Hybrid Search Capabilities: Combine explicit relationship queries with vector similarity search.
- Real-Time Search at Scale: Execute real-time vector search with unmatched scalability, ensuring that your search pipeline performs flawlessly under any load.
- ML Pipeline Integration: Enjoy seamless integration with your existing machine learning pipeline, enhancing your search capabilities without disrupting your workflow.
Cosdata is designed to meet the demands of modern search applications, empowering businesses to harness the full potential of their data.
For a simple one-step installation on Linux, run:
curl -sL https://cosdata.io/install.sh | bash
This script will handle all dependencies and set up Cosdata automatically.
For Mac & Windows, use our Docker-based installation:
- Ensure Docker is installed and running
- Pull the latest image from Ducker Hub:
docker pull cosdatateam/cosdata:latest
- Run the container
docker run -it \
--name cosdata-server \
-p 8443:8443 \
-p 50051:50051 \
cosdatateam/cosdata:latest
The server will be available at http://localhost:8443
.
For developers looking to modify or contribute to Cosdata:
- Git
- Rust (version 1.81.0 or higher)
- Cargo (Rust's package manager)
- C++ compiler (GCC 4.8+ or Clang 3.4+)
# Clone the repository
git clone https://github.com/cosdata/cosdata.git
cd cosdata
# Build the project
cargo build --release
# Run Cosdata
./target/release/cosdata --admin-key your_admin_key
You should see output similar to:
[2025-02-21T02:30:29Z INFO cosdata::web_server] starting HTTP server at http://127.0.0.1:8443
[2025-02-21T02:30:29Z INFO actix_server::builder] starting 20 workers
[2025-02-21T02:30:29Z INFO actix_server::server] Actix runtime found; starting in Actix runtime
[2025-02-21T02:30:29Z INFO actix_server::server] starting service: "actix-web-service-127.0.0.1:8443"
[2025-02-21T02:30:29Z INFO cosdata::grpc::server] gRPC server listening on [::1]:50051
To quickly get started with Cosdata, you can run the test.py
file available in the top level directory. The test.py
script -
- Creates a test collection and a Dense HNSW Index.
- In a transaction, submits batches of Random vectors to be stored on the server.
- Uses about 10% of the vectors from the above set as query vectors by adding small perturbations.
- Issues query to the server to perform the search against the query vectors and performs a brute force search locally using
cosine
distance. - Compares the results
In order to run the test.py
file a few dependencies need to be added. The description here uses uv
for downloading and setting up required dependencies. Perform following steps.
# Run uv sync to setup the Python virtual Env and download and install dependencies using `pyproject.toml` file.
uv sync
# Run the test.py file
uv run test.py
The script will then perform the steps described above and display the summary of execution
You can test Cosdata server using real world datasets. This is performed using test-dataset.py
. (TODO: Add details for this).
It's recommended to run Cosdata server in HTTPS mode i.e. with TLS
support. However, during development it might be easier to get it
running without TLS. To do so, set server.mode=http
in the
config.toml file.
Alternately, you may use self-signed certificates for testing the APIs. The paths to the certificate and private key files are configured in the config.toml file.
This sections mentions how you can generate and setup the certificates.
Run the following commands in sequence to get the private key and certificate
openssl req -newkey rsa:2048 -nodes -keyout private_key.pem -x509 -days 365 -out self_signed_certificate.crt
# Convert the private key to PKCS#8 format
openssl pkcs8 -topk8 -inform PEM -outform PEM -in private_key.pem -out private_key_pkcs8.pem -nocrypt
Set the SSL_CERT_DIR
environment variable to the folder where you're gonna store the certificates:
export SSL_CERT_DIR="/etc/ssl"
Move certificates to appropriate folders and set permissions:
# Create directories if don't exist
sudo mkdir -p $SSL_CERT_DIR/{certs,private}
# Move certificates
sudo mv self_signed_certificate.crt $SSL_CERT_DIR/certs/cosdata-ssl.crt
sudo mv private_key_pkcs8.pem $SSL_CERT_DIR/private/cosdata-ssl.key
# Create 'ssl-cert' group (if if doesn't exist already)
sudo groupadd ssl-cert
# Change private key file permissions
sudo chgrp ssl-cert $SSL_CERT_DIR/private/cosdata-ssl.key
sudo chmod 640 $SSL_CERT_DIR/private/cosdata-ssl.key
sudo usermod -aG ssl-cert $USER
# Change private key folder permissions
sudo chmod 750 $SSL_CERT_DIR/private
sudo chgrp ssl-cert $SSL_CERT_DIR/private
# Add yourself to ssl-cert group (you may need to re-login after this)
newgrp ssl-cert
Cosdata provides official client SDKs for easy integration:
Install using pip:
pip install cosdata-client
Example usage:
from cosdata.client import Client
# Initialize the client
client = Client(
host="http://127.0.0.1:8443",
admin_key="your_admin_key"
)
# Create a collection
collection = client.create_collection(
name="my_collection",
dimension=768
)
View Python SDK Documentation →
Install using npm:
npm install cosdata-sdk
Example usage:
import { Client } from 'cosdata';
// Initialize the client
const client = new Client({
host: 'http://127.0.0.1:8443',
admin_key: 'your_admin_key'
});
// Create a collection
const collection = await client.createCollection({
name: 'my_collection',
dimension: 768
});
View Node.js SDK Documentation →
- Hybrid Search: Enhance search precision with our vector database, leveraging the power of combined sparse and dense vector searches to deliver highly relevant, context-rich results for complex queries.
- Knowledge-Graph: Improve the relevance of your search results by seamlessly combining structured information from knowledge graphs with the nuanced semantics of vector embedding, enabling our vector database to deliver richer and more relevant insights.
- Indexing: Experience lightning-fast indexing with our vector database, optimized for handling high-dimensional data at scale. Our advanced indexing algorithms ensure that your sparse and dense vectors are always ready for instant querying, no matter how large or complex your dataset grows.
- Latency: Power your applications with lightning-fast search performance—our vector database is engineered to deliver exceptionally fast query responses.
- Requests per second: Achieve industry-leading concurrent requests per second (RPS) across different indices with an advanced architecture designed for optimal performance under heavy loads.
- Configurability: Gain precise control over your setup with manual configuration of all indexing and querying hyperparameters, enabling you to optimize performance, resource utilization and tailor results to your exact specifications.
- Dense Vector indexing: Achieve efficient and precise indexing with our vector database's optimized HNSW (Hierarchical Navigable Small World) algorithm, designed to enhance search performance and accuracy for large-scale data sets.
- Sparse vectors: Expertly designed to work seamlessly with SPLADE-generated sparse vectors, our solution offers superior performance compared to BM25 indices for more precise and meaningful insights.
- Unlock unbounded scalability with our vector database, engineered to grow alongside your data and query demands. Whether you're handling millions of records or scaling up to massive datasets, enjoy consistent, high-speed performance without compromise
- Achieve predictable and efficient query performance with our vector database, engineered for near-linear scalability that ensures fast results, even as your data expands.
- Resource utilization: Efficiency is at the core of our vector database, where ingenious provably efficient data structures and algorithms ensure outstanding performance while providing increasingly relevant search results.
- Scalar quantization: Configure finer quantization resolutions, including quarter-nary (2-bit) and octal (3-bit), for enhanced compression and improved recall trade-offs, giving you more control over data efficiency and performance.
- Product quantization: A pioneering product quantization approach to not only compress data more effectively but also enhance recall performance beyond what scalar quantization offers, optimizing both data efficiency and retrieval recall.
- Data isolation: Experience enterprise-grade privacy with our vector database, providing robust data isolation to ensure secure and consistent access.
- Data security: Achieve reliable data security with our vector database, designed with robust safeguards such as role-based-access-control to protect your information and maintain its integrity against unauthorized access and threats.
- Multiple deployment modes: Deploy our vector database in various environments—whether at the edge, in a private cloud, public cloud, or serverless setup—providing you with flexible, scalable solutions to meet your unique operational needs.
- Reliability: Cosdata delivers reliable performance and data integrity with fault-tolerance, backup, and recovery features, designed to meet enterprise demands and ensure uninterrupted operation.
- Versioning: Experience Git-like version control with our vector database, enabling you to compare search performance, use time travel to access past states, audit data, and effortlessly roll back when necessary.
- Auto-configuration of hyper-parameters: Achieve peak performance with our vector database, utilizing insights-driven auto-configuration of hyperparameters to automatically fine-tune your system for the best results, no manual adjustments needed.
- Intuitive API: Elegantly crafted HTTP Restful APIs featuring "Transactions as a resource". Manage all functions of our vector database effortlessly with intuitive HTTP RESTful APIs.
- Client SDKs in your favourite language: Access our vector database effortlessly with client SDKs available in multiple programming languages.
- Powerful and expressive cosQuery language: Leverage cosQuery, a powerful and expressive declarative query language, to seamlessly query data across vector embedding and knowledge graph, enabling deep and nuanced insights into your data.
- Supports real-time querying and dynamic index updates, ensuring that new multi-modal data (text, images, audio, etc.) is immediately searchable without downtime or delays.
- Want to learn more and/or contribute to the project? Join our Discord channel
- For business inquiries, please reach us at [email protected]
⭐️ If you find this project useful, please give it a star on GitHub! ⭐️
Your support helps us improve and continue developing. Thank you!
For Tasks:
Click tags to check more tools for each tasksFor Jobs:
Alternative AI tools for cosdata
Similar Open Source Tools
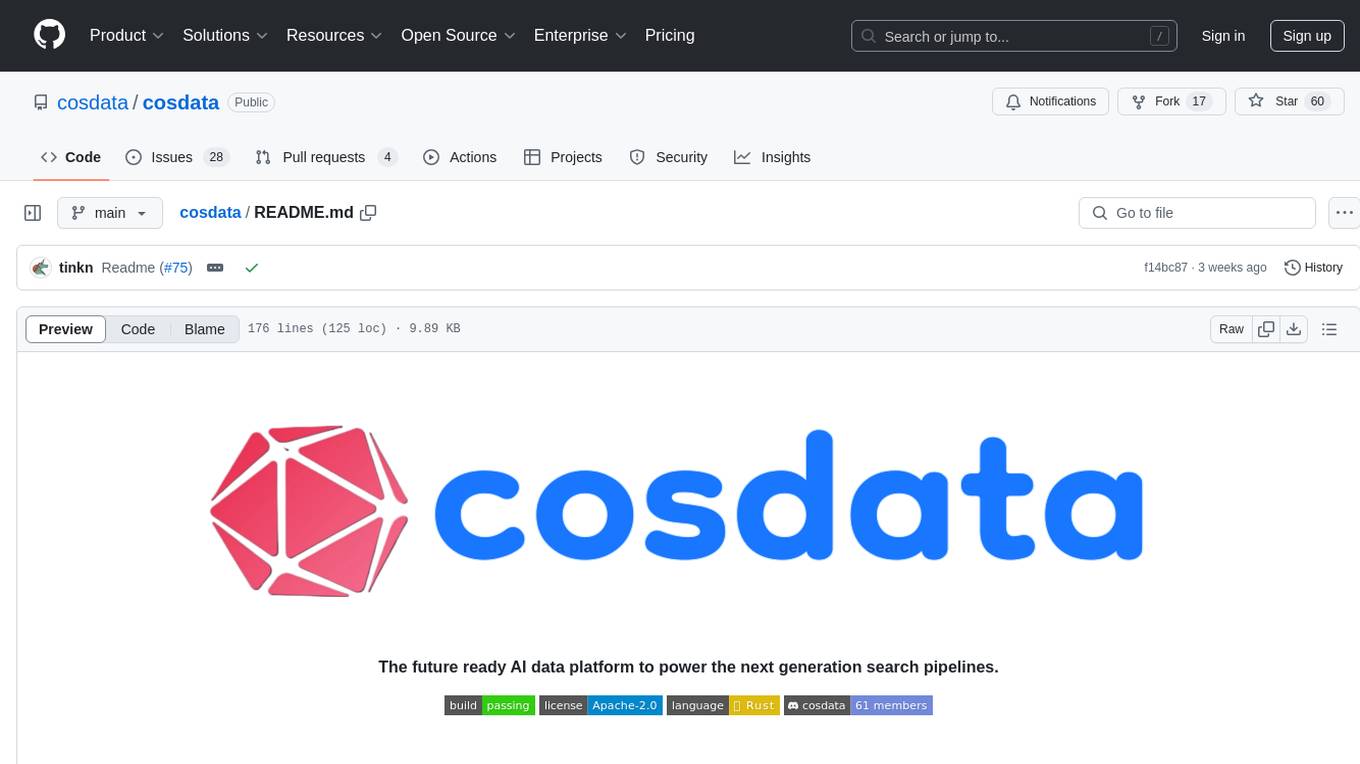
cosdata
Cosdata is a cutting-edge AI data platform designed to power the next generation search pipelines. It features immutability, version control, and excels in semantic search, structured knowledge graphs, hybrid search capabilities, real-time search at scale, and ML pipeline integration. The platform is customizable, scalable, efficient, enterprise-grade, easy to use, and can manage multi-modal data. It offers high performance, indexing, low latency, and high requests per second. Cosdata is designed to meet the demands of modern search applications, empowering businesses to harness the full potential of their data.
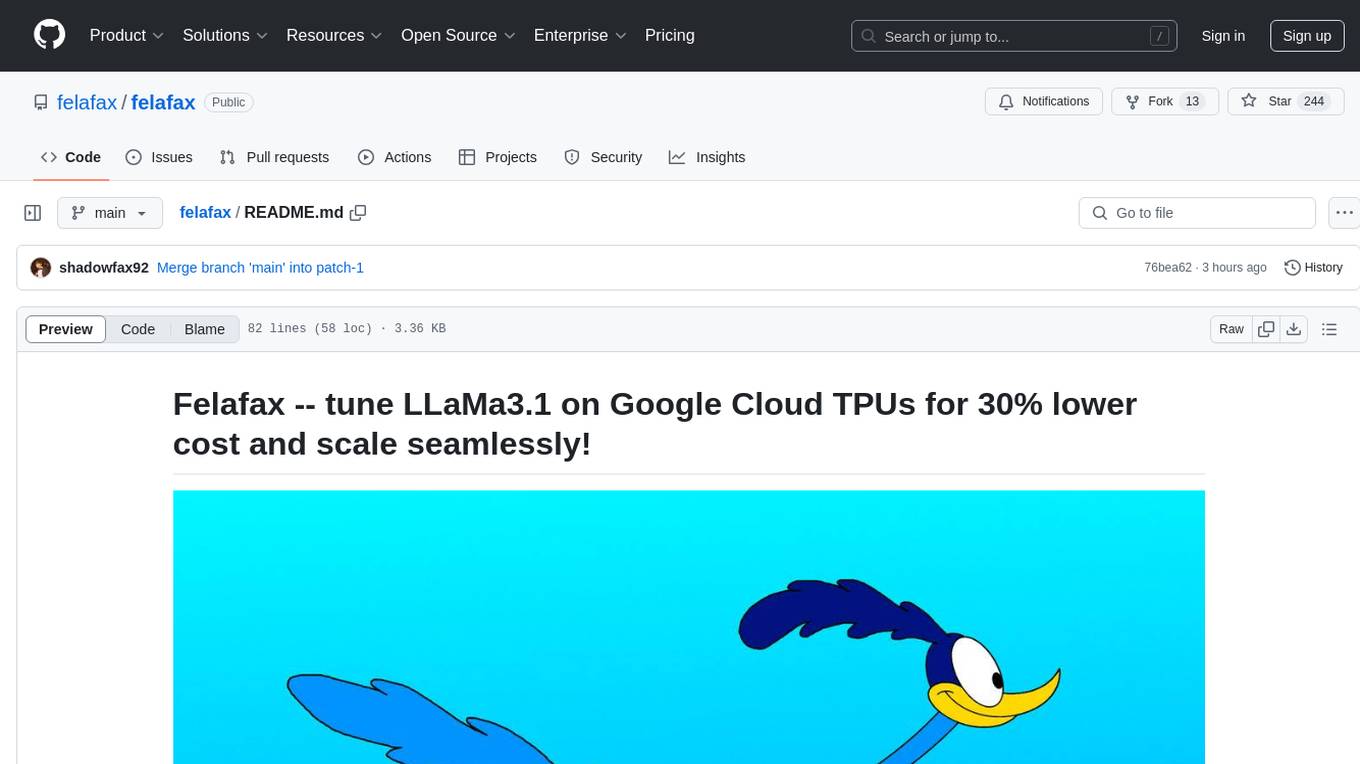
felafax
Felafax is a framework designed to tune LLaMa3.1 on Google Cloud TPUs for cost efficiency and seamless scaling. It provides a Jupyter notebook for continued-training and fine-tuning open source LLMs using XLA runtime. The goal of Felafax is to simplify running AI workloads on non-NVIDIA hardware such as TPUs, AWS Trainium, AMD GPU, and Intel GPU. It supports various models like LLaMa-3.1 JAX Implementation, LLaMa-3/3.1 PyTorch XLA, and Gemma2 Models optimized for Cloud TPUs with full-precision training support.
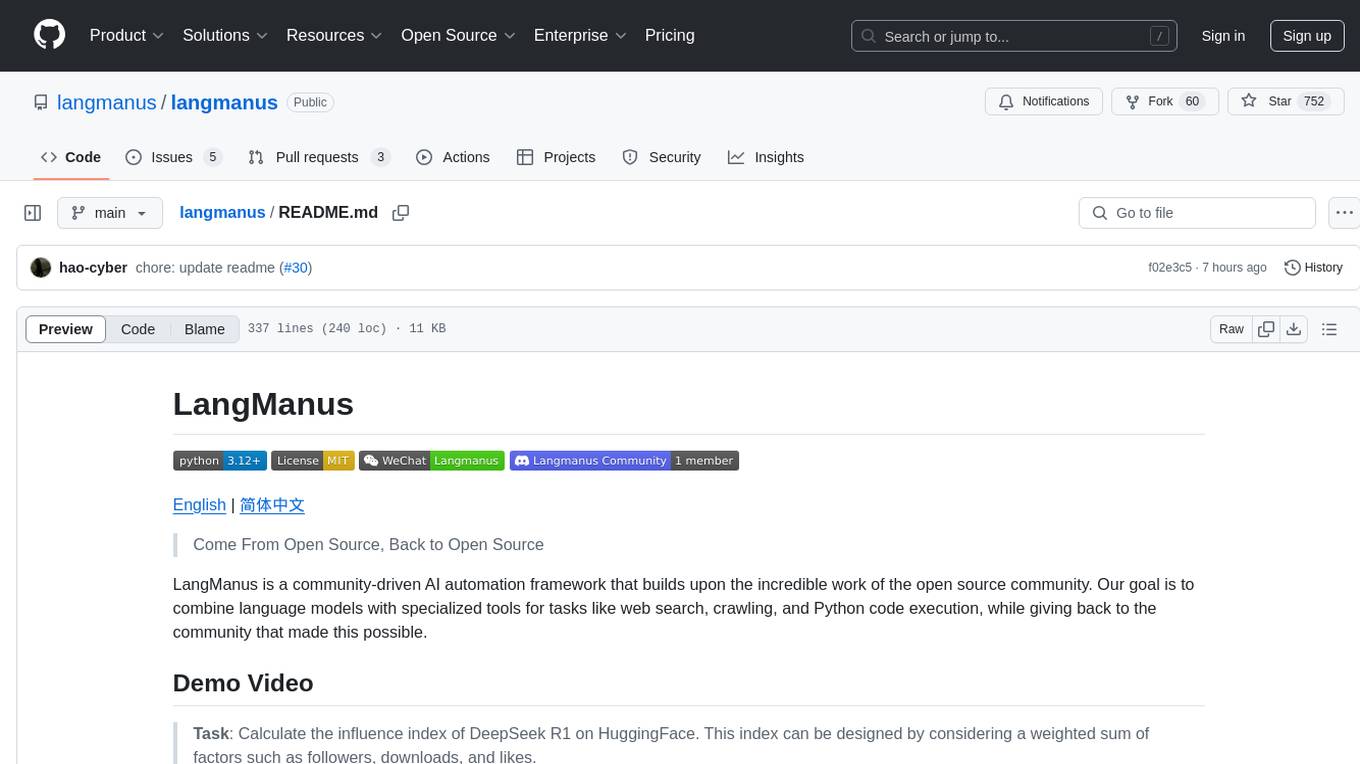
langmanus
LangManus is a community-driven AI automation framework that combines language models with specialized tools for tasks like web search, crawling, and Python code execution. It implements a hierarchical multi-agent system with agents like Coordinator, Planner, Supervisor, Researcher, Coder, Browser, and Reporter. The framework supports LLM integration, search and retrieval tools, Python integration, workflow management, and visualization. LangManus aims to give back to the open-source community and welcomes contributions in various forms.
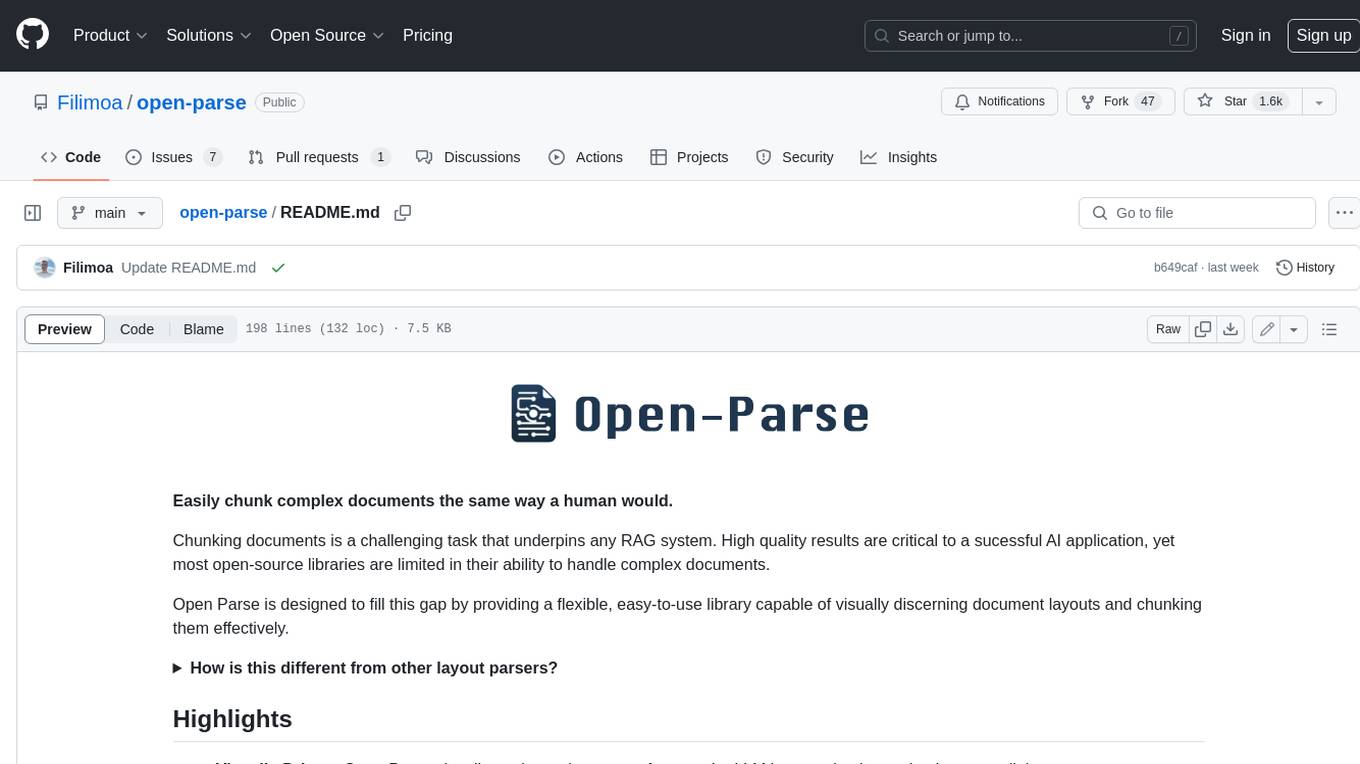
open-parse
Open Parse is a Python library for visually discerning document layouts and chunking them effectively. It is designed to fill the gap in open-source libraries for handling complex documents. Unlike text splitting, which converts a file to raw text and slices it up, Open Parse visually analyzes documents for superior LLM input. It also supports basic markdown for parsing headings, bold, and italics, and has high-precision table support, extracting tables into clean Markdown formats with accuracy that surpasses traditional tools. Open Parse is extensible, allowing users to easily implement their own post-processing steps. It is also intuitive, with great editor support and completion everywhere, making it easy to use and learn.
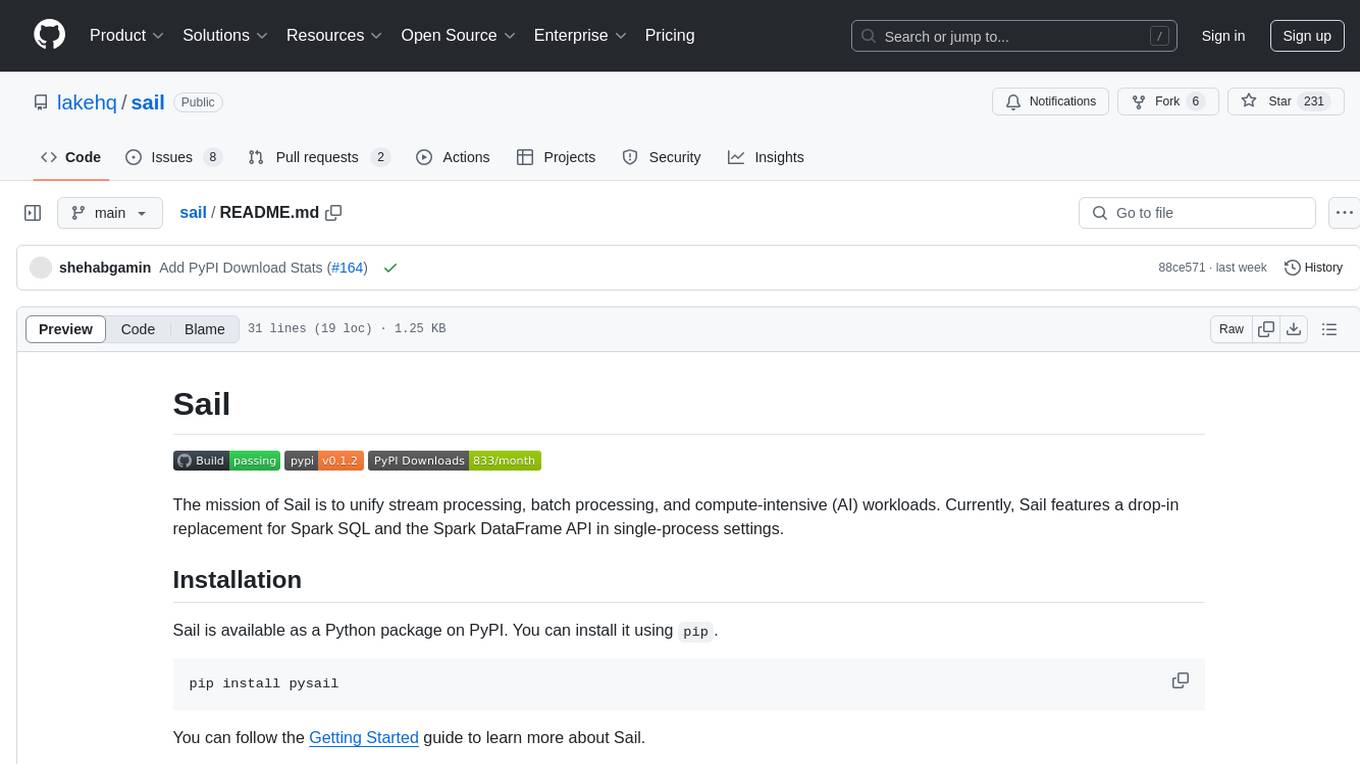
sail
Sail is a tool designed to unify stream processing, batch processing, and compute-intensive workloads, serving as a drop-in replacement for Spark SQL and the Spark DataFrame API in single-process settings. It aims to streamline data processing tasks and facilitate AI workloads.
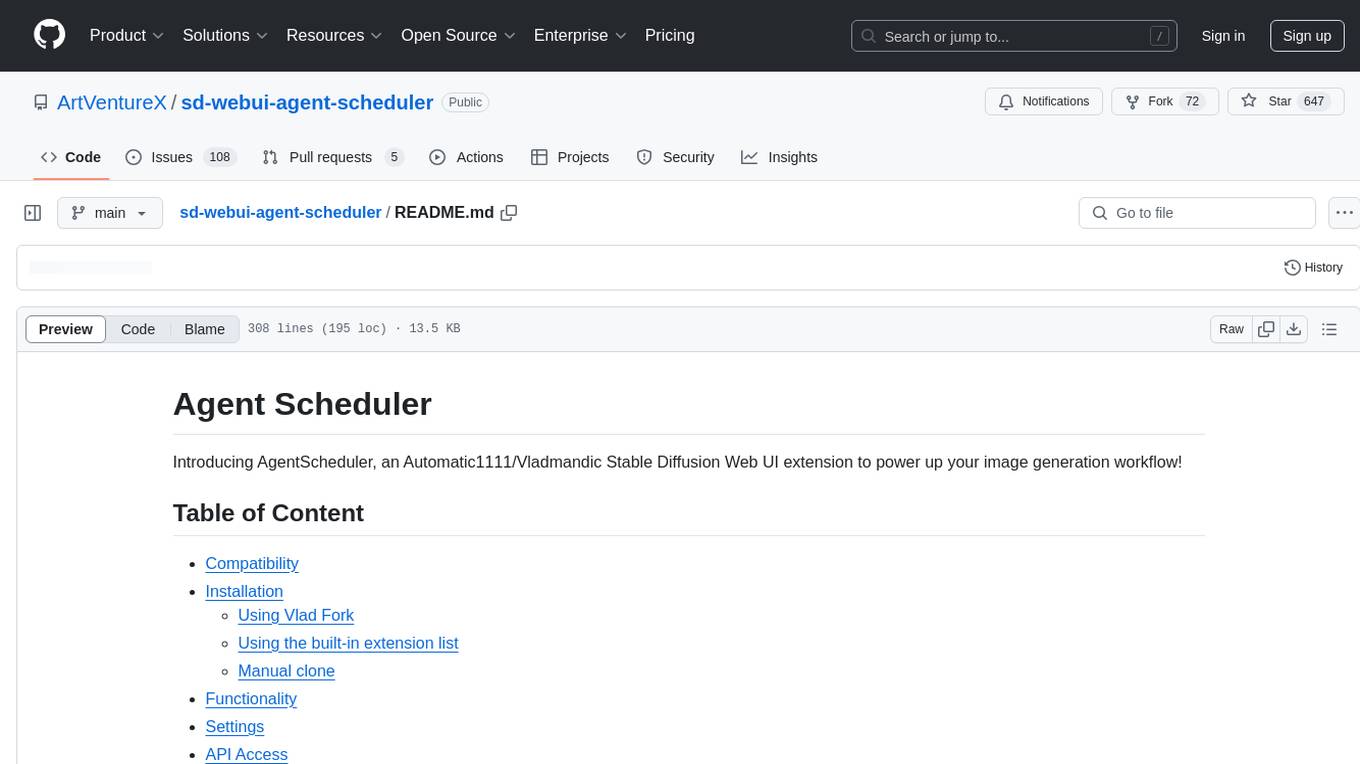
sd-webui-agent-scheduler
AgentScheduler is an Automatic/Vladmandic Stable Diffusion Web UI extension designed to enhance image generation workflows. It allows users to enqueue prompts, settings, and controlnets, manage queued tasks, prioritize, pause, resume, and delete tasks, view generation results, and more. The extension offers hidden features like queuing checkpoints, editing queued tasks, and custom checkpoint selection. Users can access the functionality through HTTP APIs and API callbacks. Troubleshooting steps are provided for common errors. The extension is compatible with latest versions of A1111 and Vladmandic. It is licensed under Apache License 2.0.
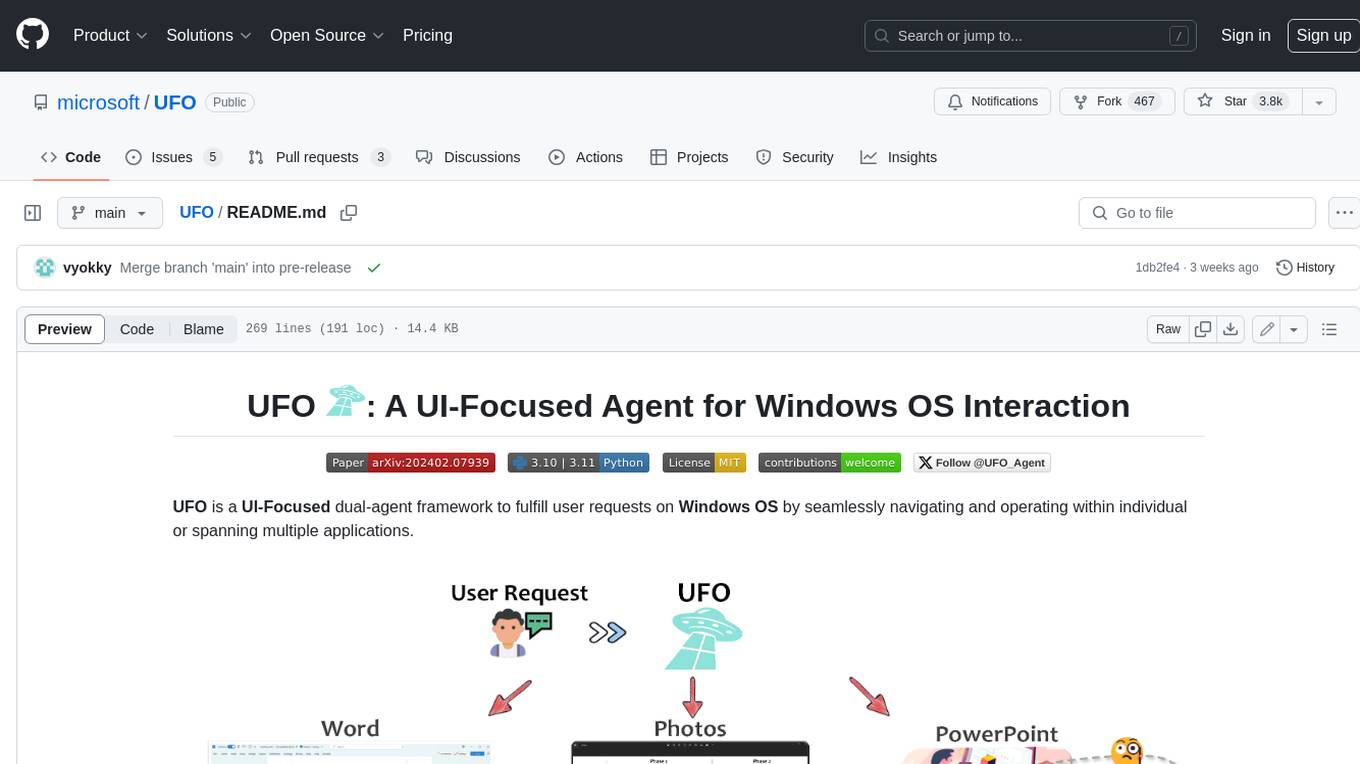
UFO
UFO is a UI-focused dual-agent framework to fulfill user requests on Windows OS by seamlessly navigating and operating within individual or spanning multiple applications.
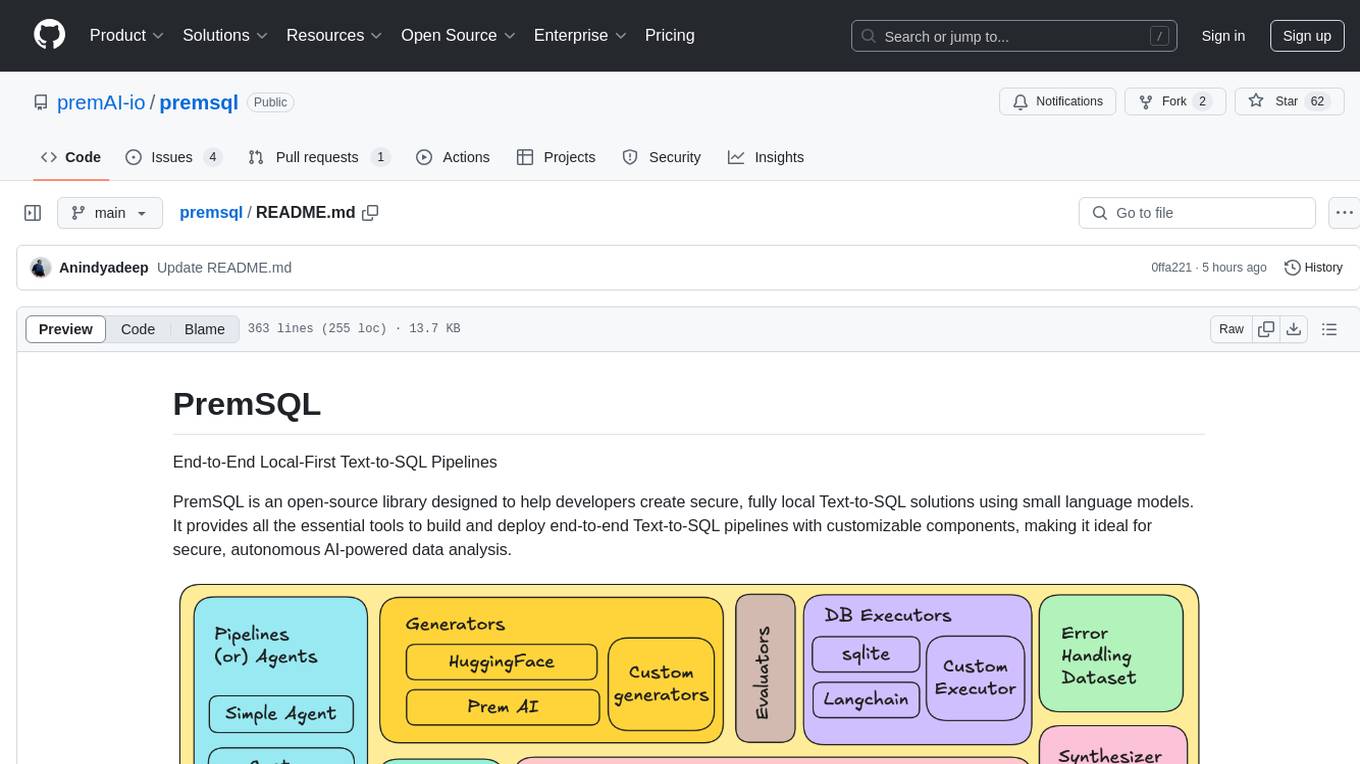
premsql
PremSQL is an open-source library designed to help developers create secure, fully local Text-to-SQL solutions using small language models. It provides essential tools for building and deploying end-to-end Text-to-SQL pipelines with customizable components, ideal for secure, autonomous AI-powered data analysis. The library offers features like Local-First approach, Customizable Datasets, Robust Executors and Evaluators, Advanced Generators, Error Handling and Self-Correction, Fine-Tuning Support, and End-to-End Pipelines. Users can fine-tune models, generate SQL queries from natural language inputs, handle errors, and evaluate model performance against predefined metrics. PremSQL is extendible for customization and private data usage.
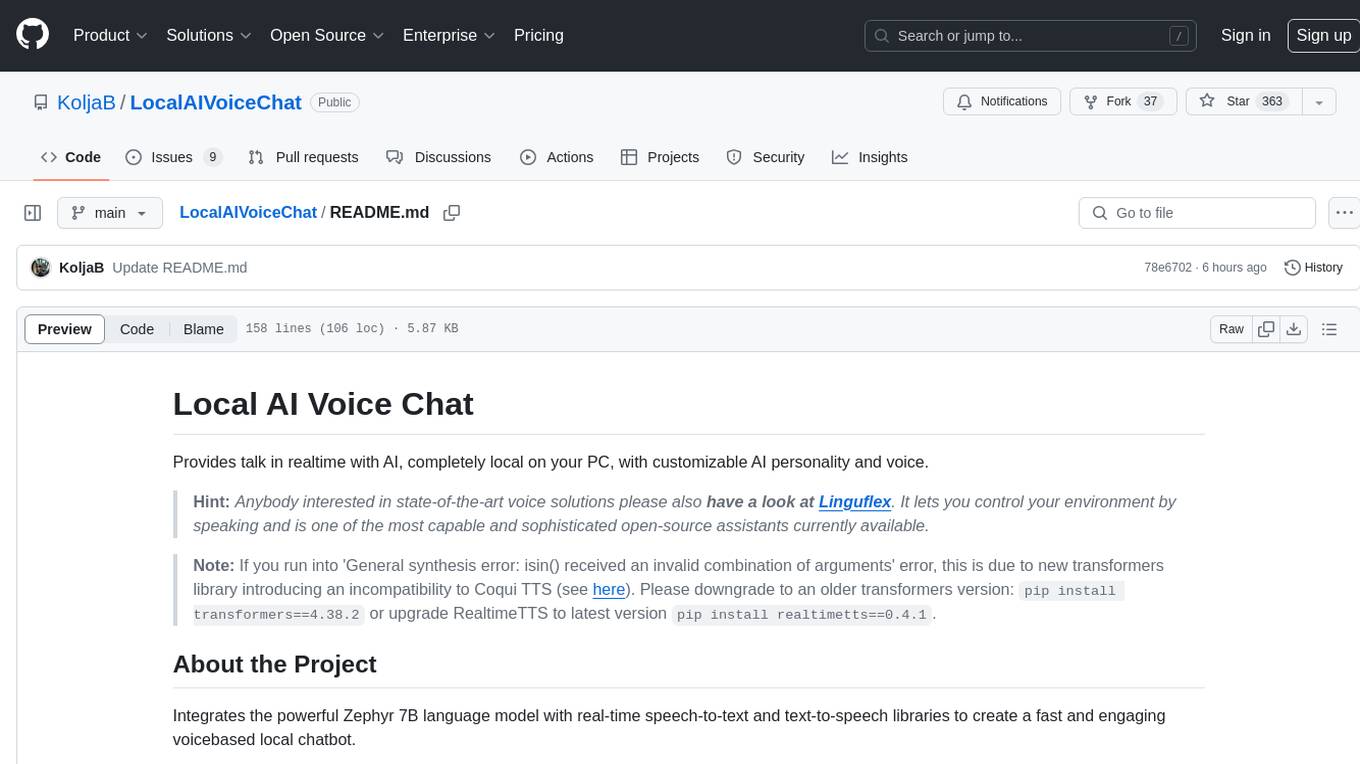
LocalAIVoiceChat
LocalAIVoiceChat is an experimental alpha software that enables real-time voice chat with a customizable AI personality and voice on your PC. It integrates Zephyr 7B language model with speech-to-text and text-to-speech libraries. The tool is designed for users interested in state-of-the-art voice solutions and provides an early version of a local real-time chatbot.
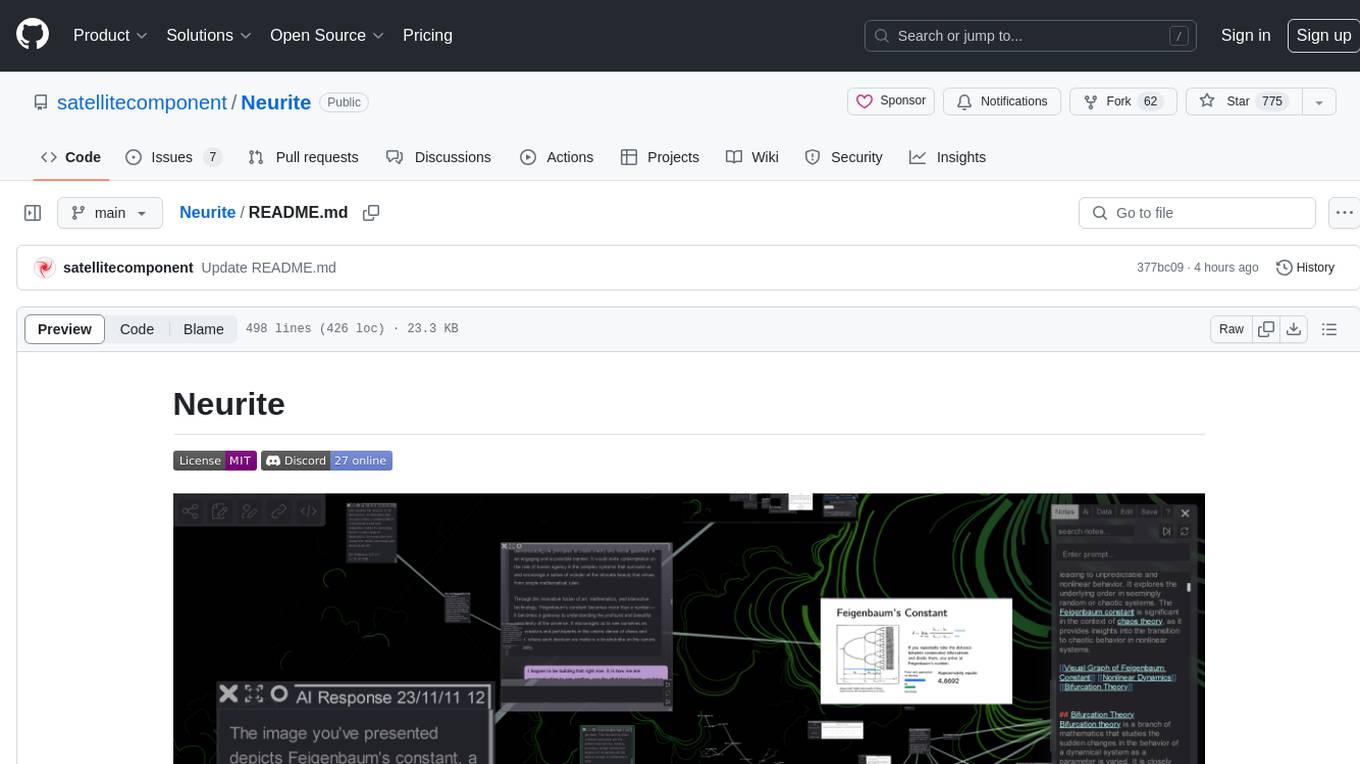
Neurite
Neurite is an innovative project that combines chaos theory and graph theory to create a digital interface that explores hidden patterns and connections for creative thinking. It offers a unique workspace blending fractals with mind mapping techniques, allowing users to navigate the Mandelbrot set in real-time. Nodes in Neurite represent various content types like text, images, videos, code, and AI agents, enabling users to create personalized microcosms of thoughts and inspirations. The tool supports synchronized knowledge management through bi-directional synchronization between mind-mapping and text-based hyperlinking. Neurite also features FractalGPT for modular conversation with AI, local AI capabilities for multi-agent chat networks, and a Neural API for executing code and sequencing animations. The project is actively developed with plans for deeper fractal zoom, advanced control over node placement, and experimental features.
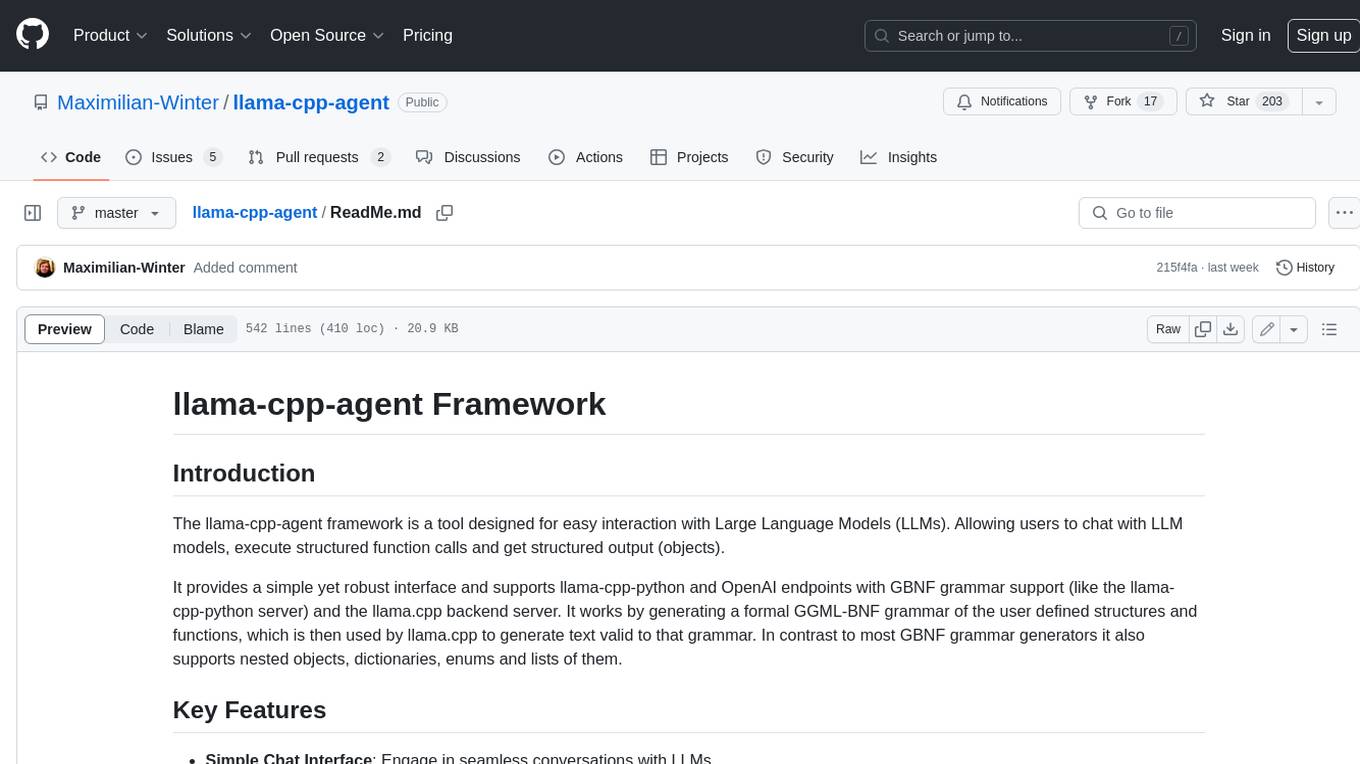
llama-cpp-agent
The llama-cpp-agent framework is a tool designed for easy interaction with Large Language Models (LLMs). Allowing users to chat with LLM models, execute structured function calls and get structured output (objects). It provides a simple yet robust interface and supports llama-cpp-python and OpenAI endpoints with GBNF grammar support (like the llama-cpp-python server) and the llama.cpp backend server. It works by generating a formal GGML-BNF grammar of the user defined structures and functions, which is then used by llama.cpp to generate text valid to that grammar. In contrast to most GBNF grammar generators it also supports nested objects, dictionaries, enums and lists of them.
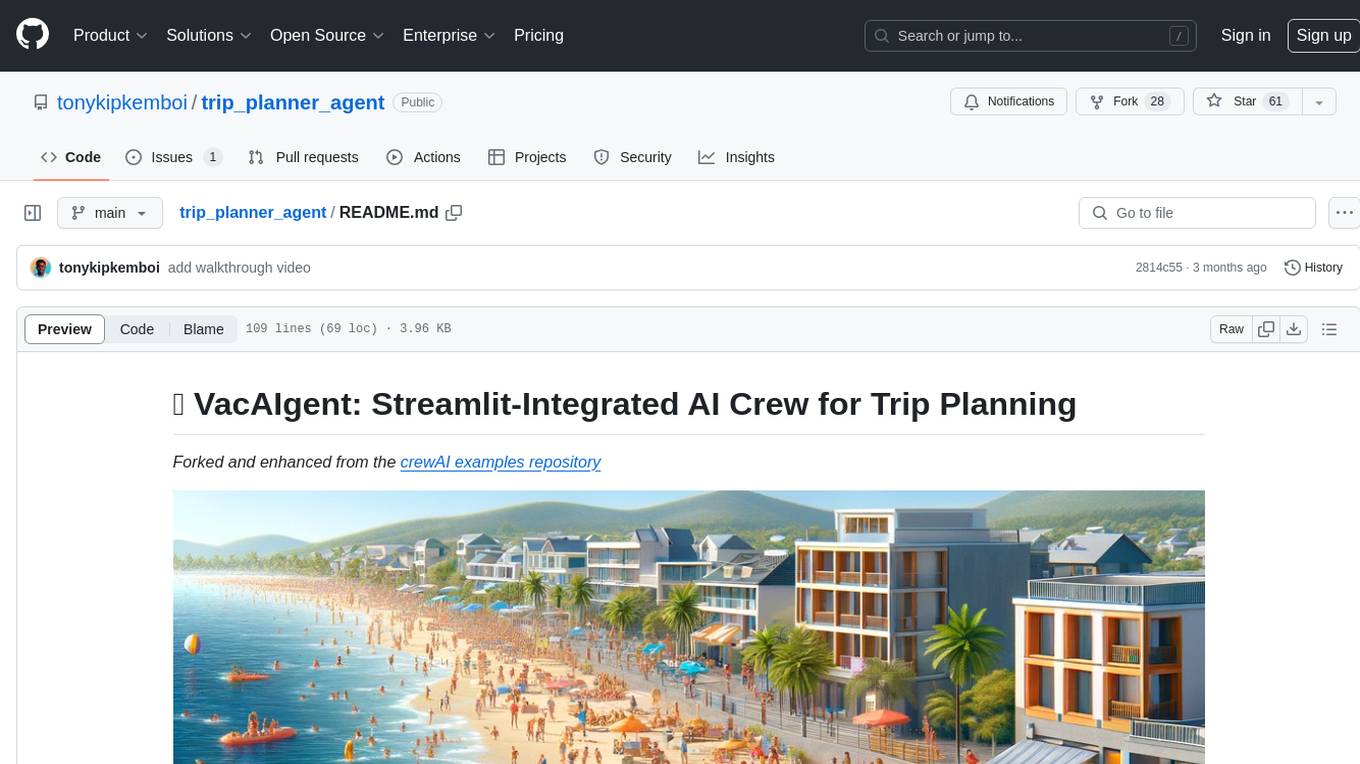
trip_planner_agent
VacAIgent is an AI tool that automates and enhances trip planning by leveraging the CrewAI framework. It integrates a user-friendly Streamlit interface for interactive travel planning. Users can input preferences and receive tailored travel plans with the help of autonomous AI agents. The tool allows for collaborative decision-making on cities and crafting complete itineraries based on specified preferences, all accessible via a streamlined Streamlit user interface. VacAIgent can be customized to use different AI models like GPT-3.5 or local models like Ollama for enhanced privacy and customization.
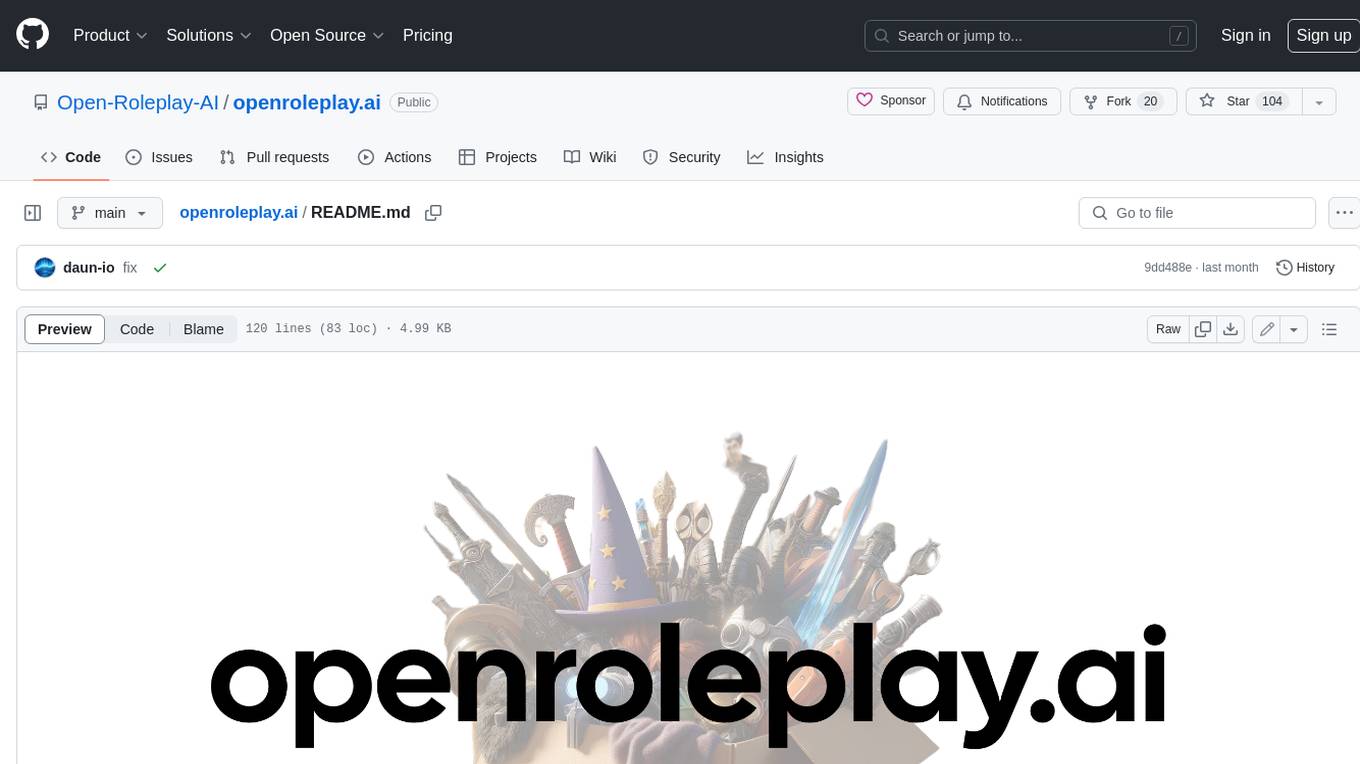
openroleplay.ai
Open Roleplay is an open-source alternative to Character.ai. It allows users to create their own AI characters, customize them, and generate images and voices for them. Open Roleplay also supports group chat and automatic translation. The tool is built with Next.js, React.js, Tailwind CSS, Vercel, Convex, and Clerk.
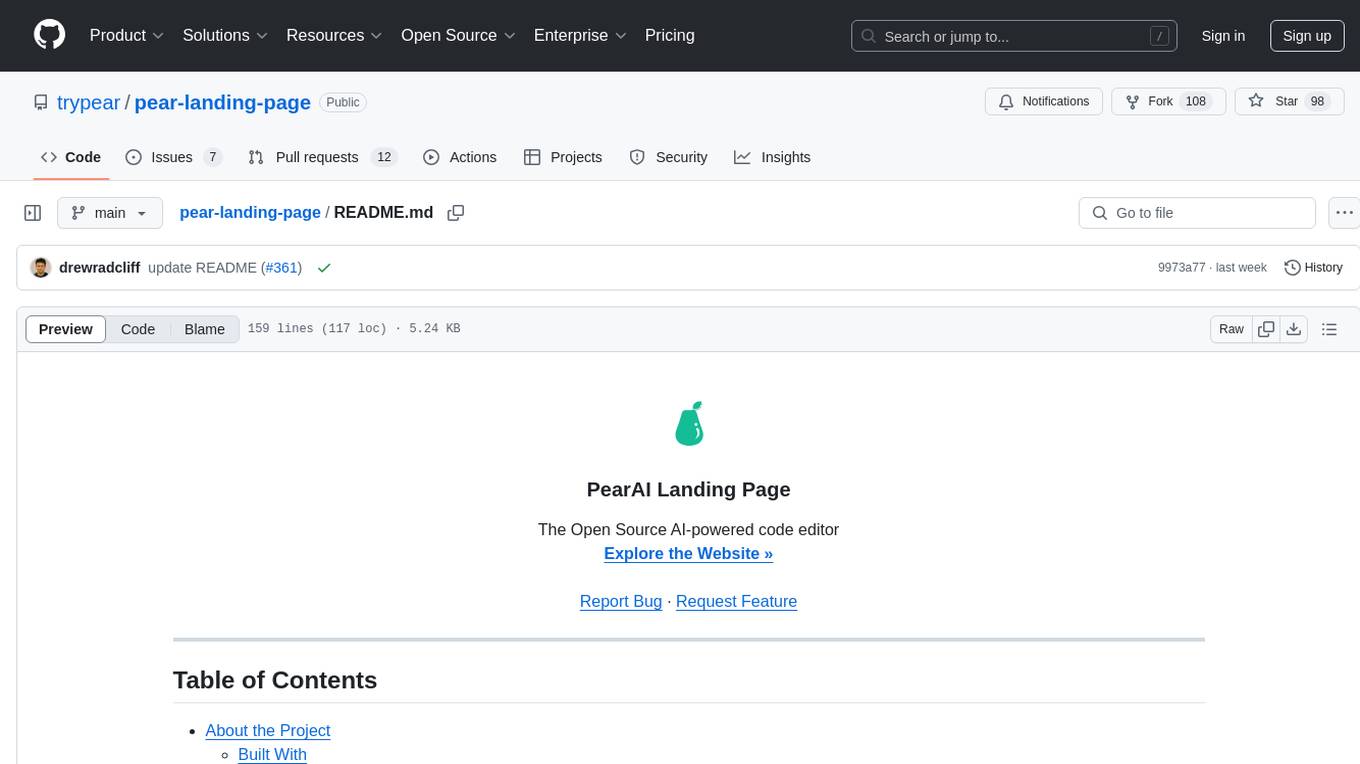
pear-landing-page
PearAI Landing Page is an open-source AI-powered code editor managed by Nang and Pan. It is built with Next.js, Vercel, Tailwind CSS, and TypeScript. The project requires setting up environment variables for proper configuration. Users can run the project locally by starting the development server and visiting the specified URL in the browser. Recommended extensions include Prettier, ESLint, and JavaScript and TypeScript Nightly. Contributions to the project are welcomed and appreciated.
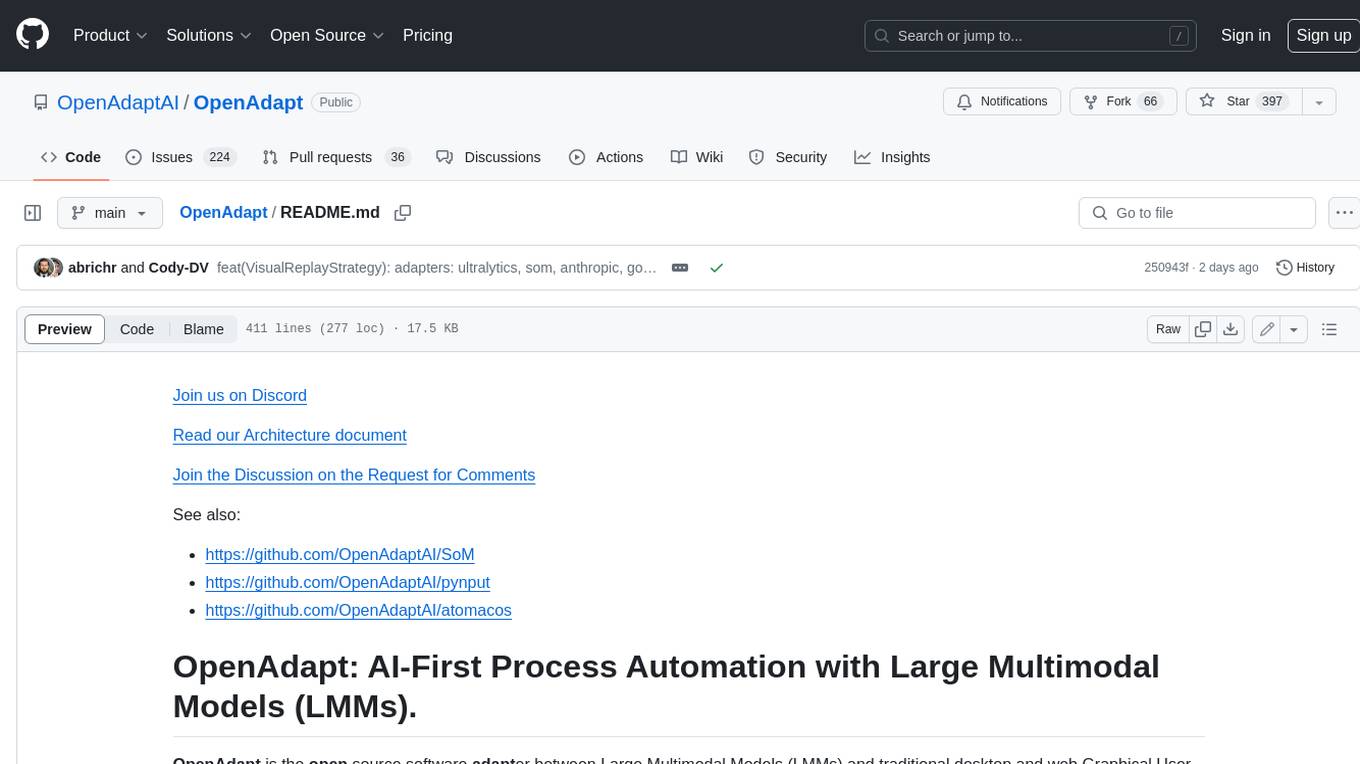
OpenAdapt
OpenAdapt is an open-source software adapter between Large Multimodal Models (LMMs) and traditional desktop and web Graphical User Interfaces (GUIs). It aims to automate repetitive GUI workflows by leveraging the power of LMMs. OpenAdapt records user input and screenshots, converts them into tokenized format, and generates synthetic input via transformer model completions. It also analyzes recordings to generate task trees and replay synthetic input to complete tasks. OpenAdapt is model agnostic and generates prompts automatically by learning from human demonstration, ensuring that agents are grounded in existing processes and mitigating hallucinations. It works with all types of desktop GUIs, including virtualized and web, and is open source under the MIT license.
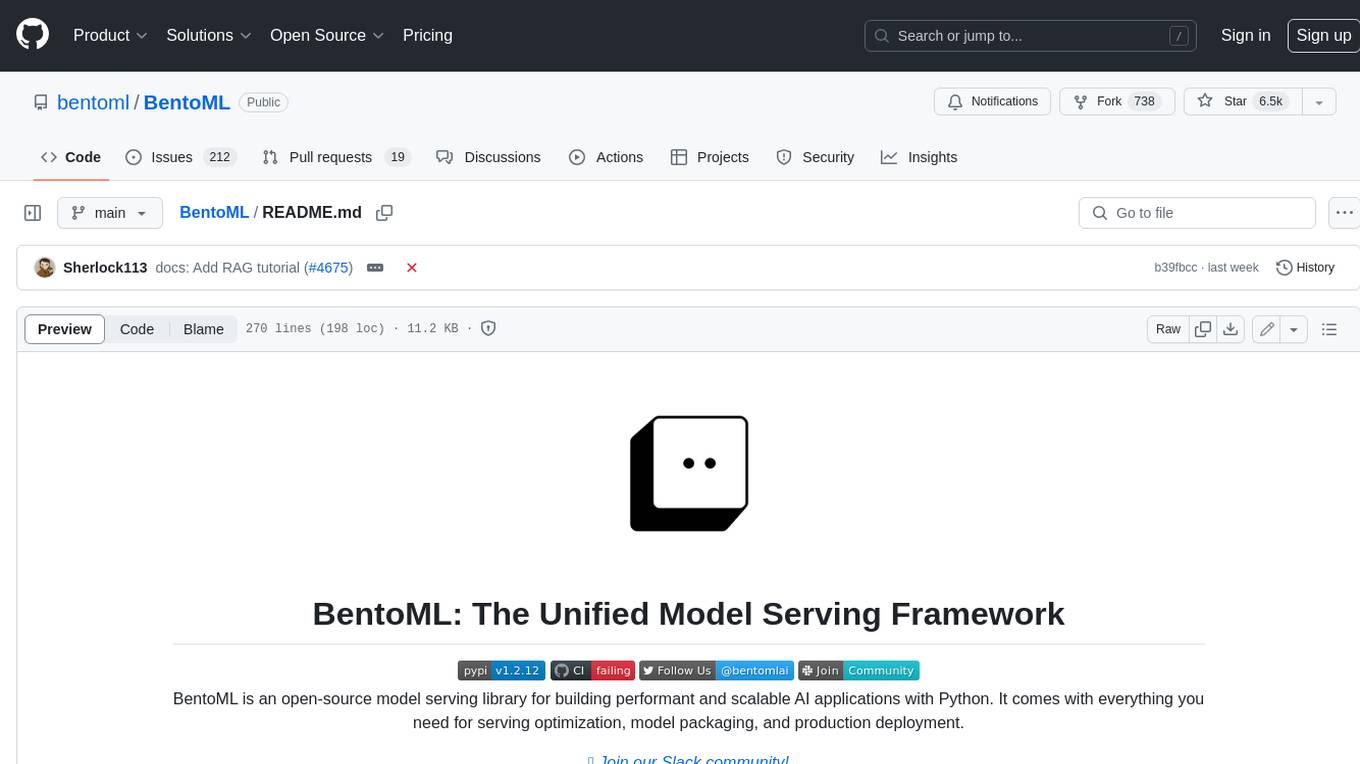
BentoML
BentoML is an open-source model serving library for building performant and scalable AI applications with Python. It comes with everything you need for serving optimization, model packaging, and production deployment.
For similar tasks
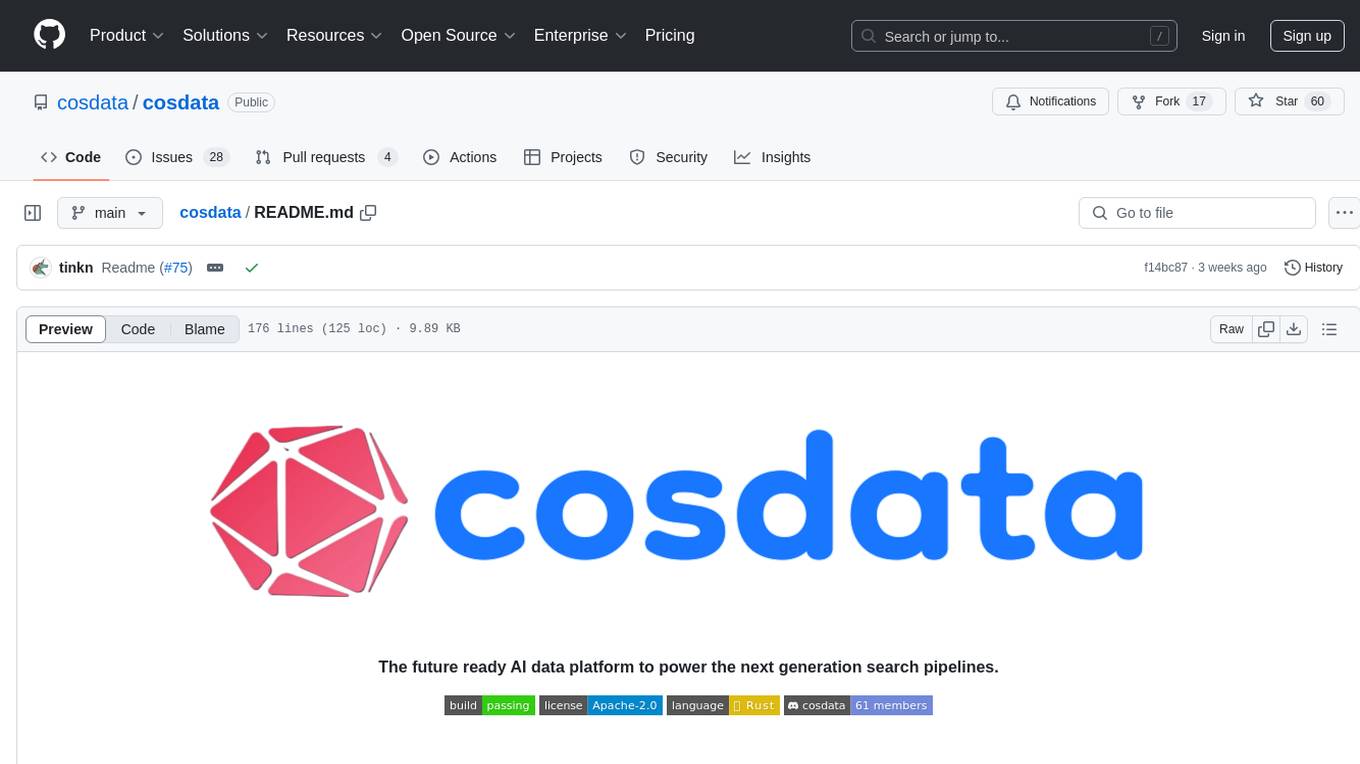
cosdata
Cosdata is a cutting-edge AI data platform designed to power the next generation search pipelines. It features immutability, version control, and excels in semantic search, structured knowledge graphs, hybrid search capabilities, real-time search at scale, and ML pipeline integration. The platform is customizable, scalable, efficient, enterprise-grade, easy to use, and can manage multi-modal data. It offers high performance, indexing, low latency, and high requests per second. Cosdata is designed to meet the demands of modern search applications, empowering businesses to harness the full potential of their data.
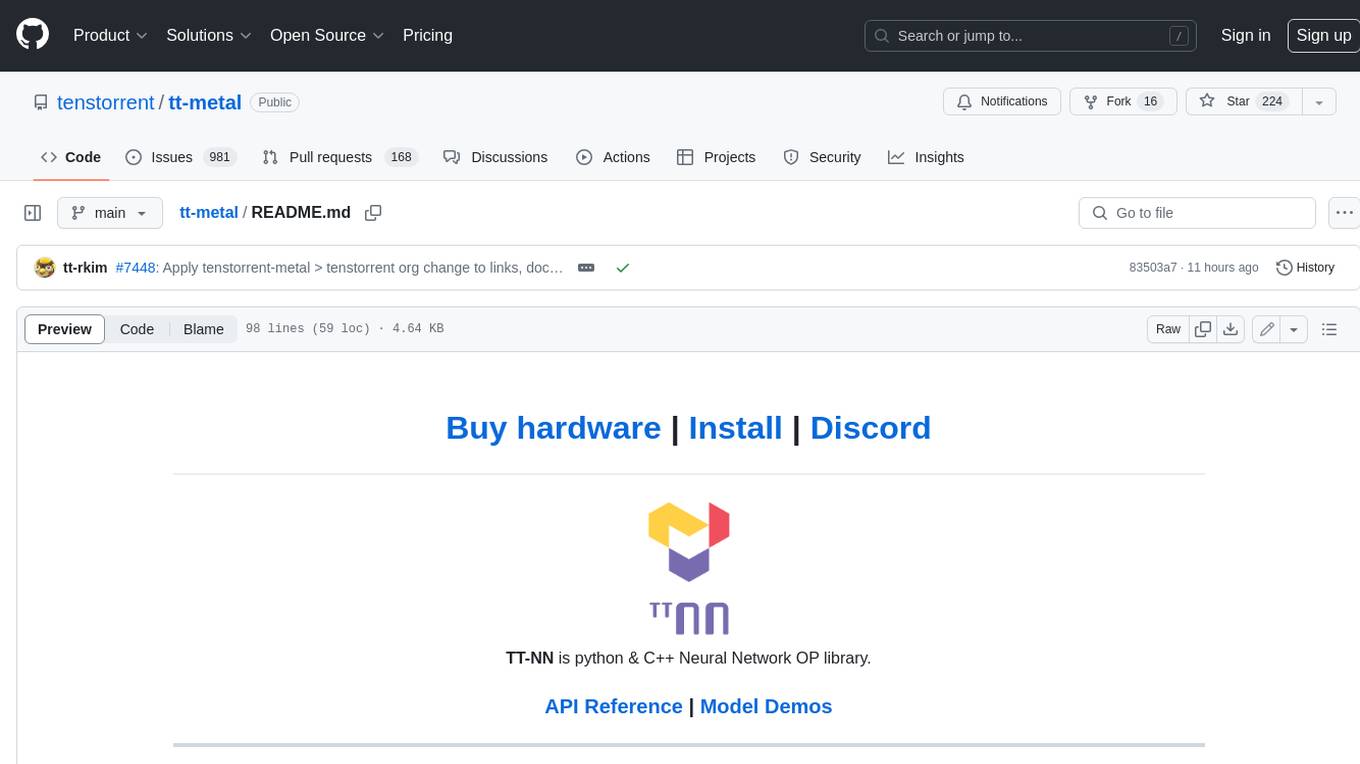
tt-metal
TT-NN is a python & C++ Neural Network OP library. It provides a low-level programming model, TT-Metalium, enabling kernel development for Tenstorrent hardware.
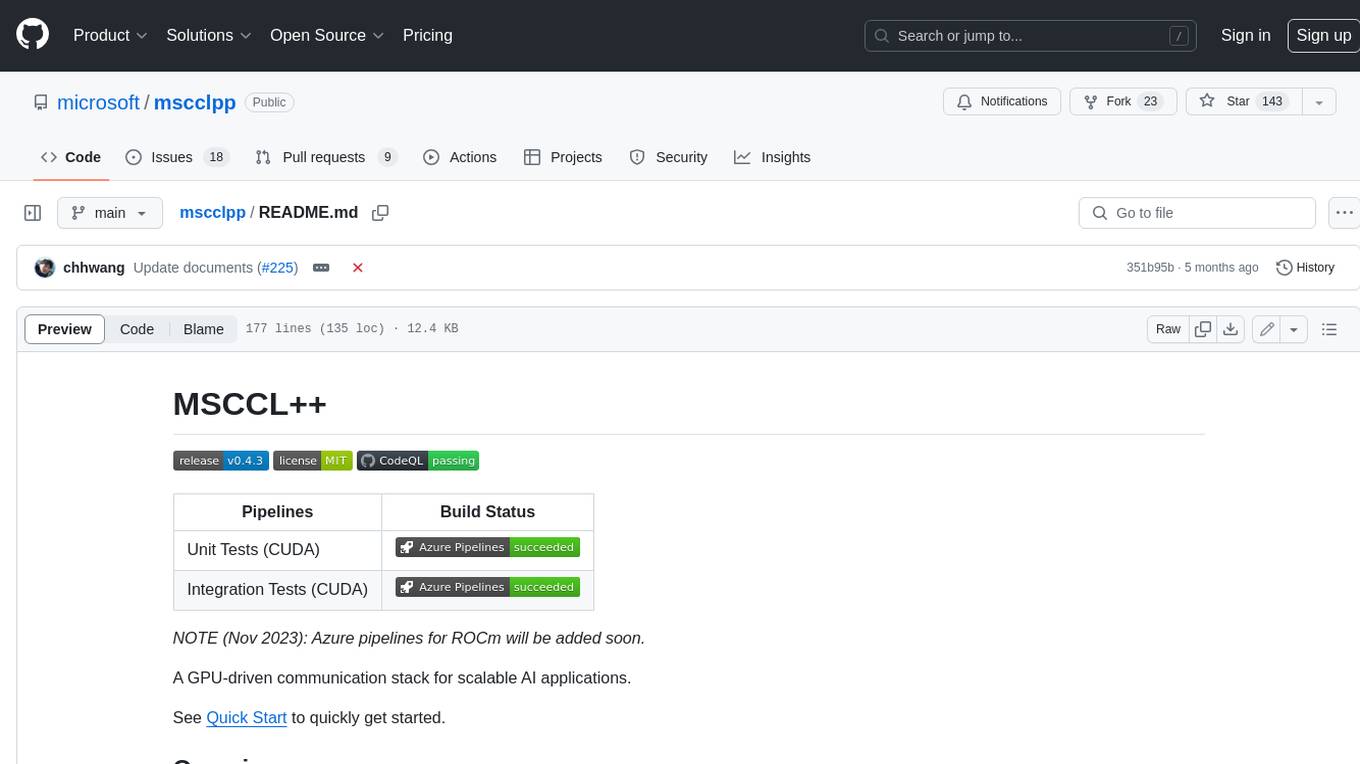
mscclpp
MSCCL++ is a GPU-driven communication stack for scalable AI applications. It provides a highly efficient and customizable communication stack for distributed GPU applications. MSCCL++ redefines inter-GPU communication interfaces, delivering a highly efficient and customizable communication stack for distributed GPU applications. Its design is specifically tailored to accommodate diverse performance optimization scenarios often encountered in state-of-the-art AI applications. MSCCL++ provides communication abstractions at the lowest level close to hardware and at the highest level close to application API. The lowest level of abstraction is ultra light weight which enables a user to implement logics of data movement for a collective operation such as AllReduce inside a GPU kernel extremely efficiently without worrying about memory ordering of different ops. The modularity of MSCCL++ enables a user to construct the building blocks of MSCCL++ in a high level abstraction in Python and feed them to a CUDA kernel in order to facilitate the user's productivity. MSCCL++ provides fine-grained synchronous and asynchronous 0-copy 1-sided abstracts for communication primitives such as `put()`, `get()`, `signal()`, `flush()`, and `wait()`. The 1-sided abstractions allows a user to asynchronously `put()` their data on the remote GPU as soon as it is ready without requiring the remote side to issue any receive instruction. This enables users to easily implement flexible communication logics, such as overlapping communication with computation, or implementing customized collective communication algorithms without worrying about potential deadlocks. Additionally, the 0-copy capability enables MSCCL++ to directly transfer data between user's buffers without using intermediate internal buffers which saves GPU bandwidth and memory capacity. MSCCL++ provides consistent abstractions regardless of the location of the remote GPU (either on the local node or on a remote node) or the underlying link (either NVLink/xGMI or InfiniBand). This simplifies the code for inter-GPU communication, which is often complex due to memory ordering of GPU/CPU read/writes and therefore, is error-prone.
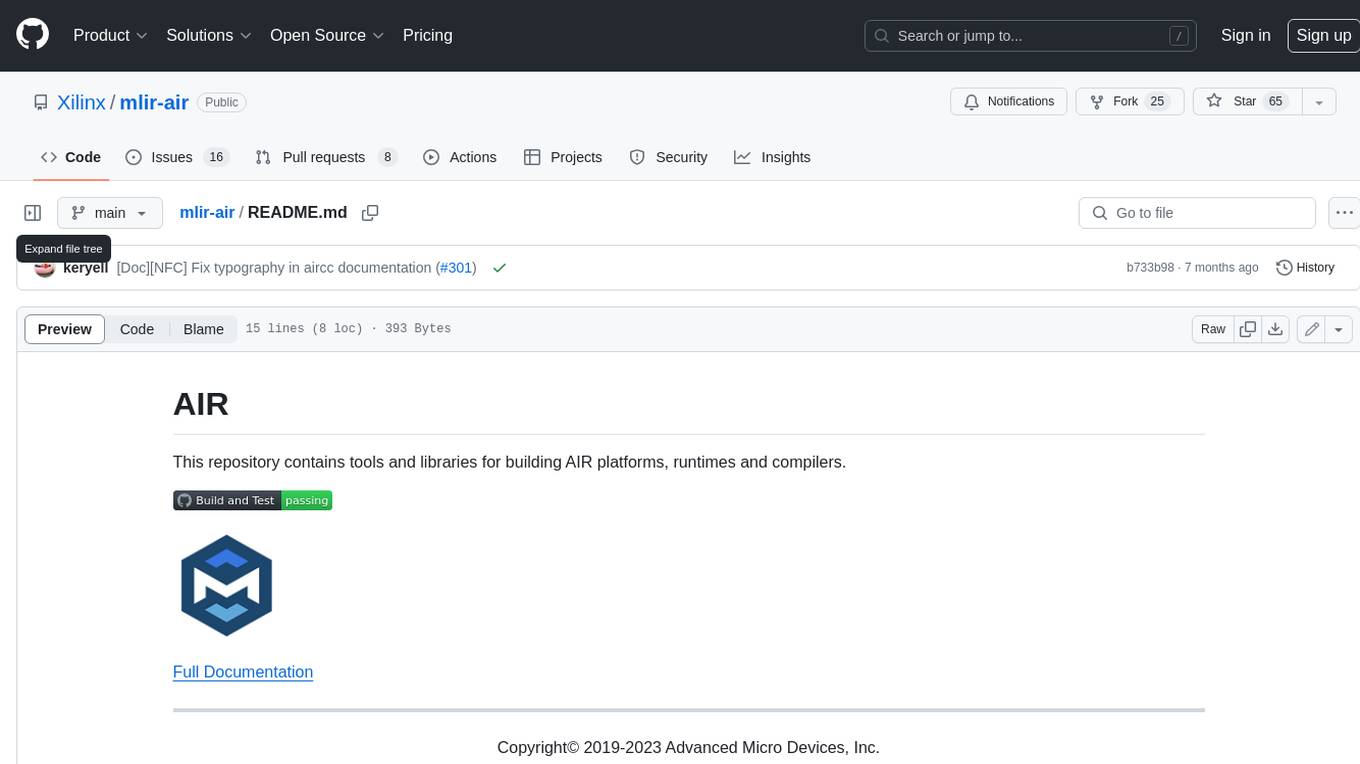
mlir-air
This repository contains tools and libraries for building AIR platforms, runtimes and compilers.
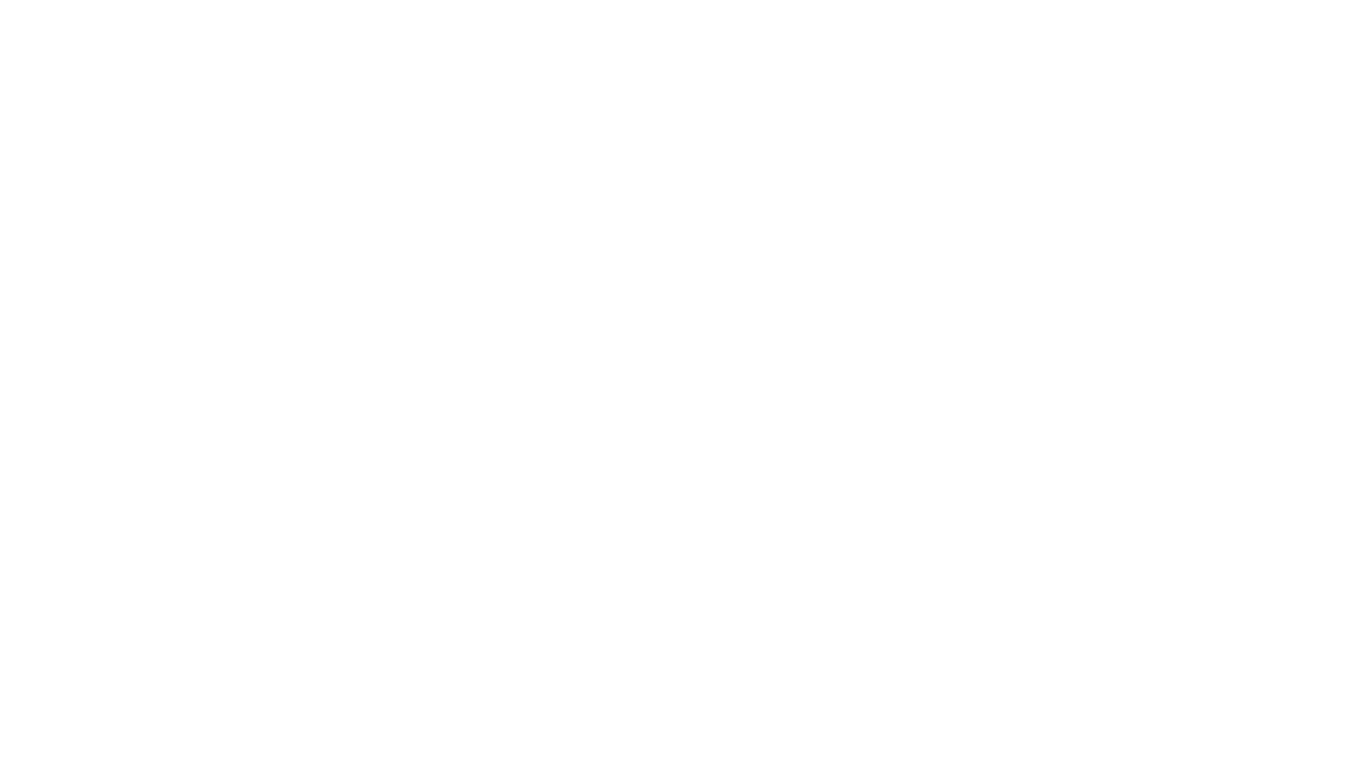
free-for-life
A massive list including a huge amount of products and services that are completely free! ⭐ Star on GitHub • 🤝 Contribute # Table of Contents * APIs, Data & ML * Artificial Intelligence * BaaS * Code Editors * Code Generation * DNS * Databases * Design & UI * Domains * Email * Font * For Students * Forms * Linux Distributions * Messaging & Streaming * PaaS * Payments & Billing * SSL
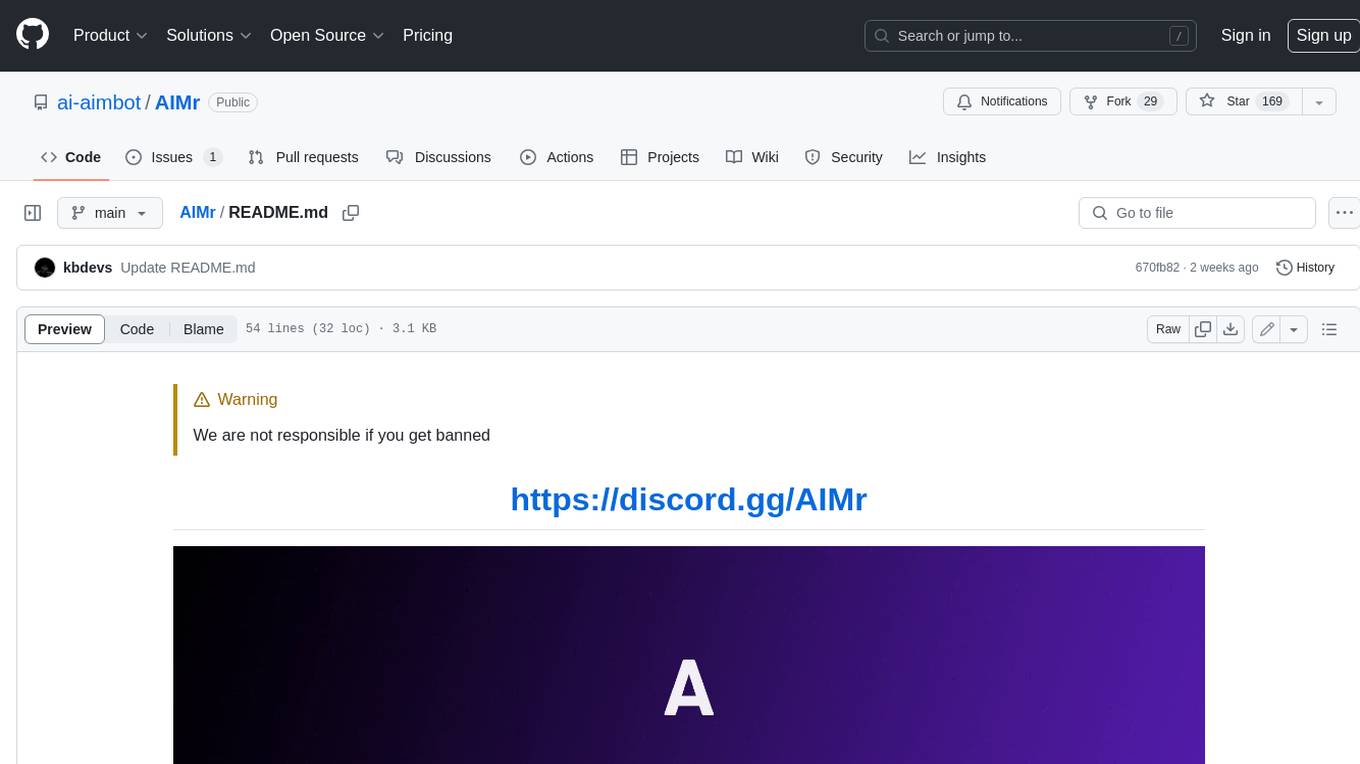
AIMr
AIMr is an AI aimbot tool written in Python that leverages modern technologies to achieve an undetected system with a pleasing appearance. It works on any game that uses human-shaped models. To optimize its performance, users should build OpenCV with CUDA. For Valorant, additional perks in the Discord and an Arduino Leonardo R3 are required.
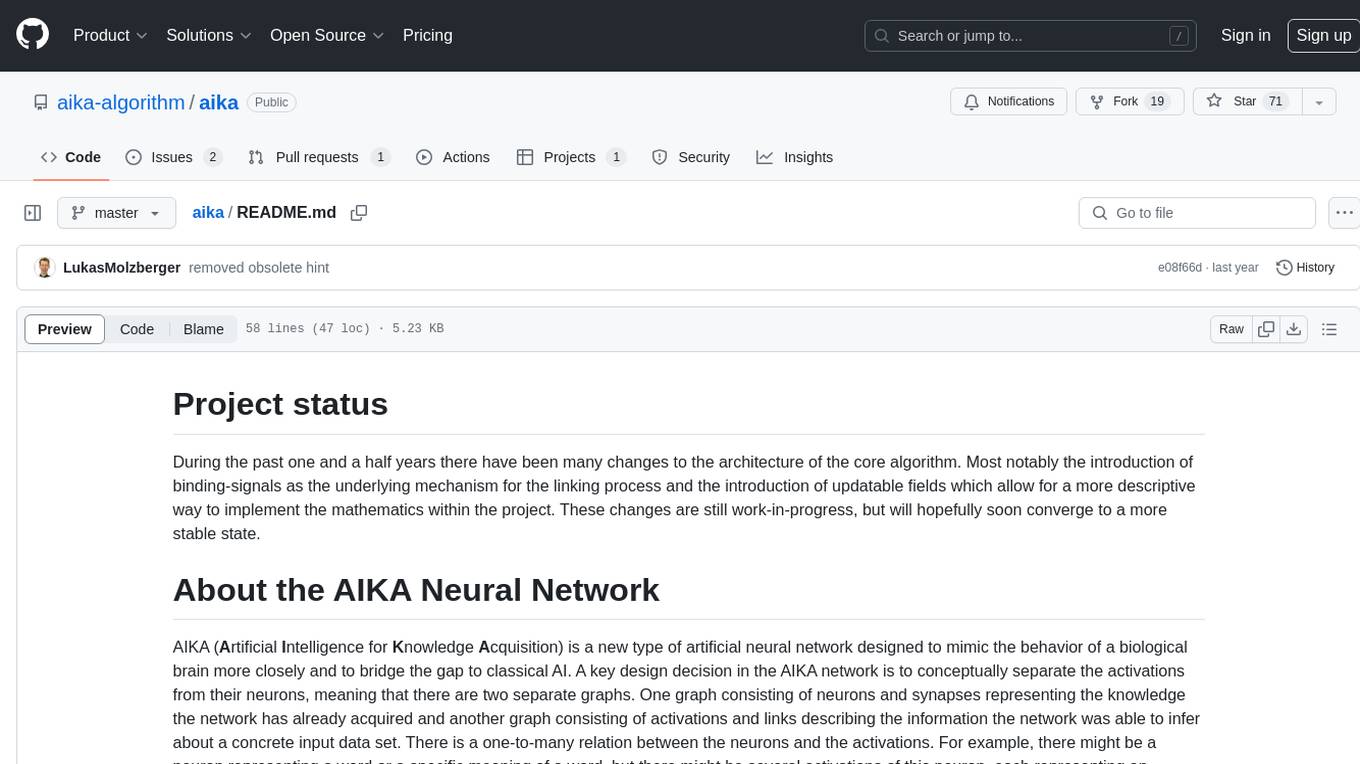
aika
AIKA (Artificial Intelligence for Knowledge Acquisition) is a new type of artificial neural network designed to mimic the behavior of a biological brain more closely and bridge the gap to classical AI. The network conceptually separates activations from neurons, creating two separate graphs to represent acquired knowledge and inferred information. It uses different types of neurons and synapses to propagate activation values, binding signals, causal relations, and training gradients. The network structure allows for flexible topology and supports the gradual population of neurons and synapses during training.
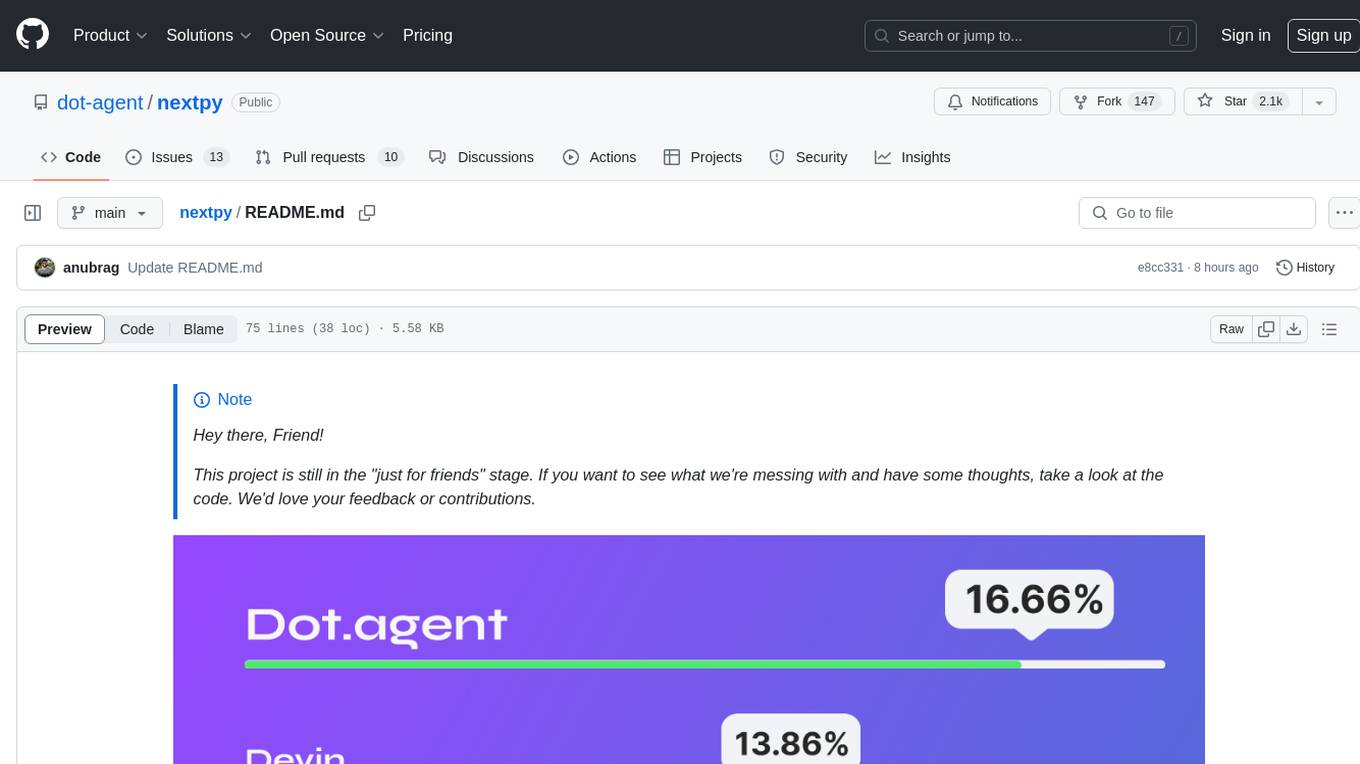
nextpy
Nextpy is a cutting-edge software development framework optimized for AI-based code generation. It provides guardrails for defining AI system boundaries, structured outputs for prompt engineering, a powerful prompt engine for efficient processing, better AI generations with precise output control, modularity for multiplatform and extensible usage, developer-first approach for transferable knowledge, and containerized & scalable deployment options. It offers 4-10x faster performance compared to Streamlit apps, with a focus on cooperation within the open-source community and integration of key components from various projects.
For similar jobs
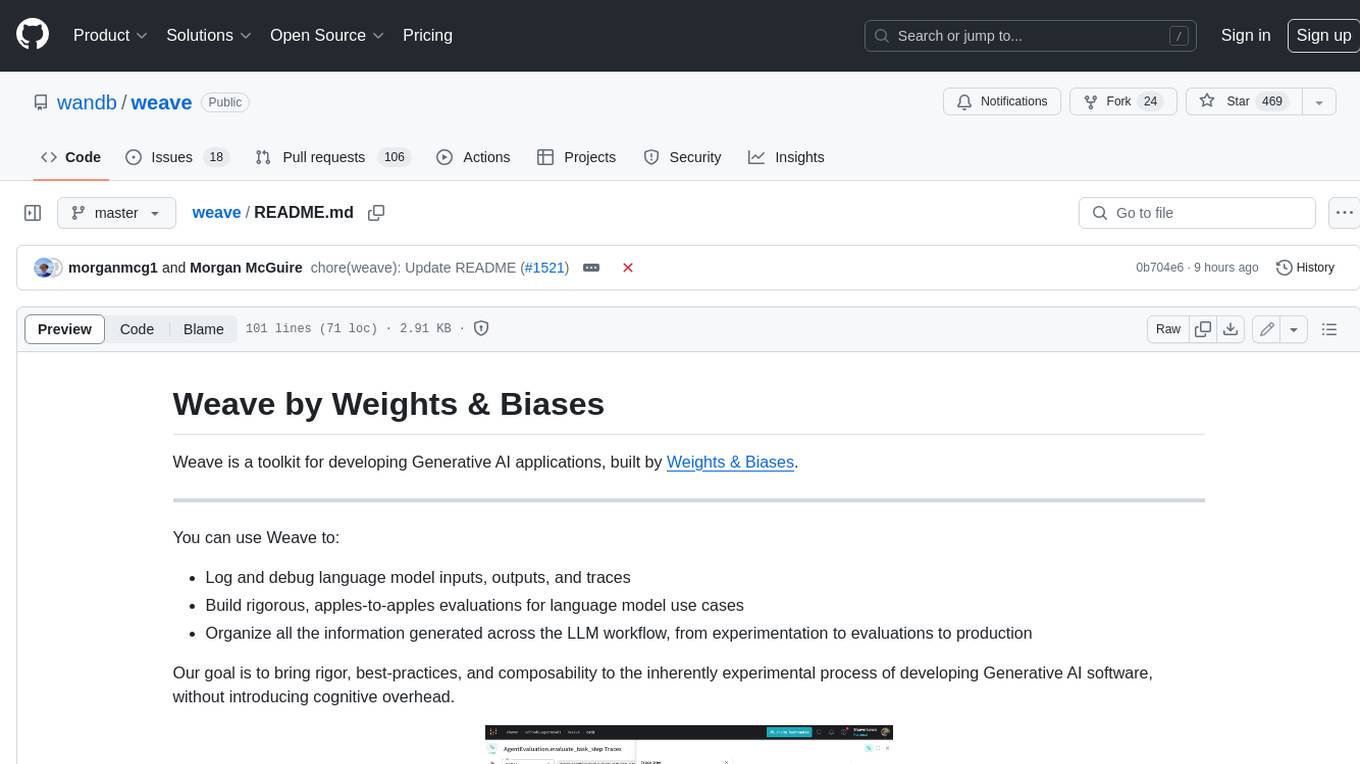
weave
Weave is a toolkit for developing Generative AI applications, built by Weights & Biases. With Weave, you can log and debug language model inputs, outputs, and traces; build rigorous, apples-to-apples evaluations for language model use cases; and organize all the information generated across the LLM workflow, from experimentation to evaluations to production. Weave aims to bring rigor, best-practices, and composability to the inherently experimental process of developing Generative AI software, without introducing cognitive overhead.
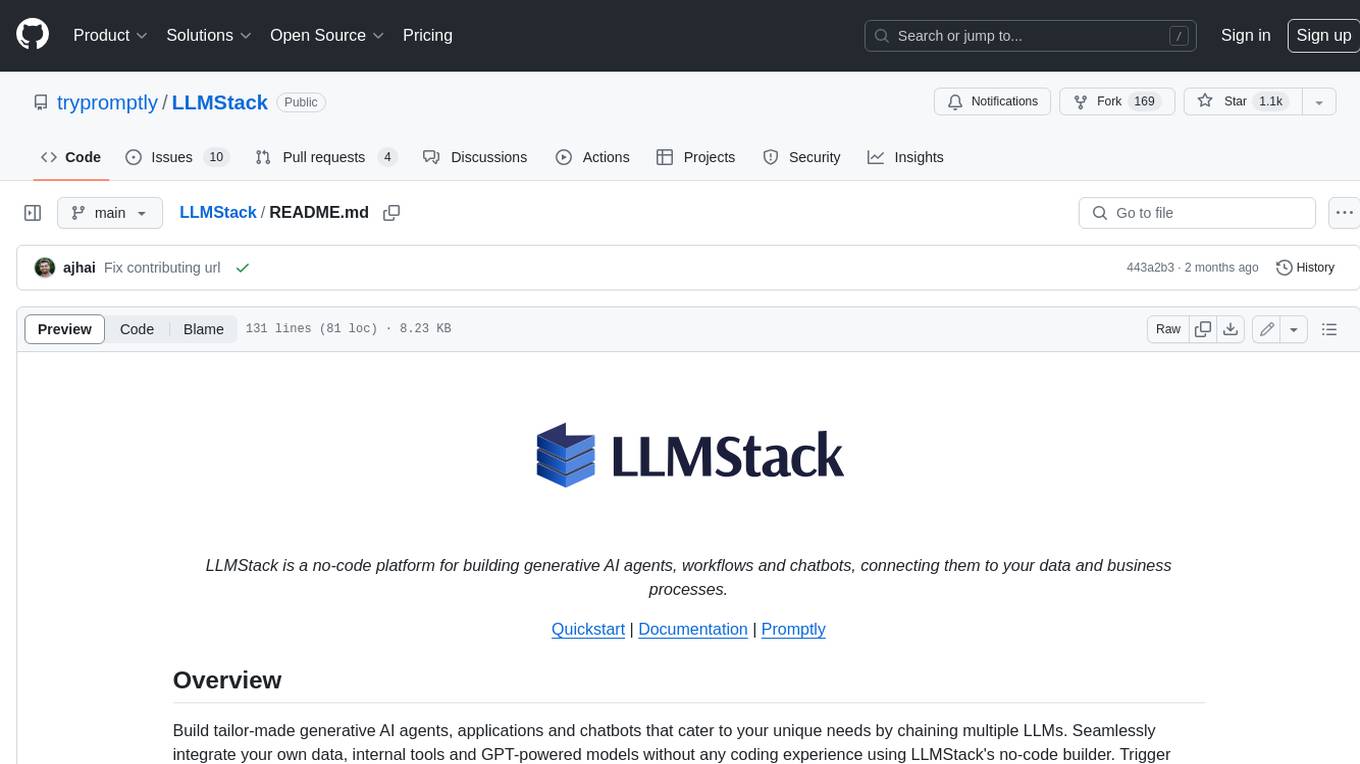
LLMStack
LLMStack is a no-code platform for building generative AI agents, workflows, and chatbots. It allows users to connect their own data, internal tools, and GPT-powered models without any coding experience. LLMStack can be deployed to the cloud or on-premise and can be accessed via HTTP API or triggered from Slack or Discord.
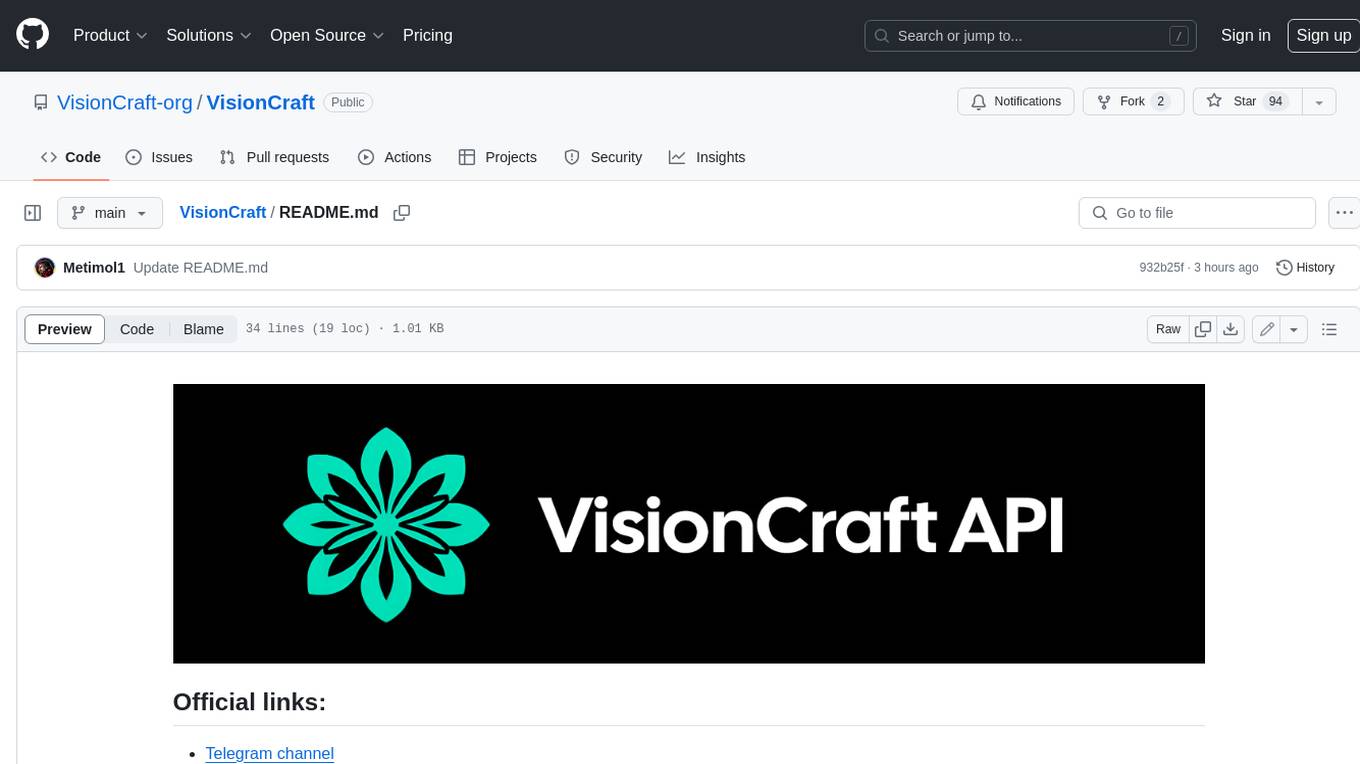
VisionCraft
The VisionCraft API is a free API for using over 100 different AI models. From images to sound.
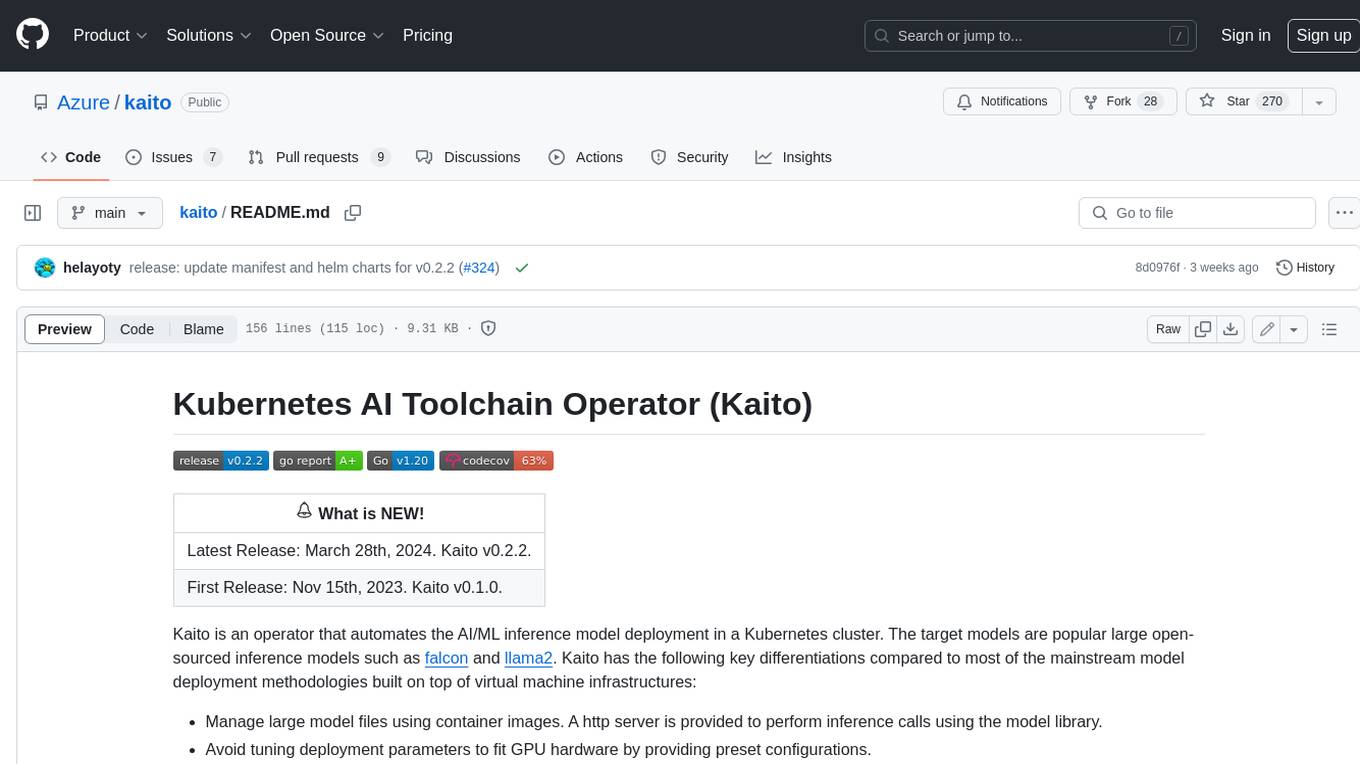
kaito
Kaito is an operator that automates the AI/ML inference model deployment in a Kubernetes cluster. It manages large model files using container images, avoids tuning deployment parameters to fit GPU hardware by providing preset configurations, auto-provisions GPU nodes based on model requirements, and hosts large model images in the public Microsoft Container Registry (MCR) if the license allows. Using Kaito, the workflow of onboarding large AI inference models in Kubernetes is largely simplified.
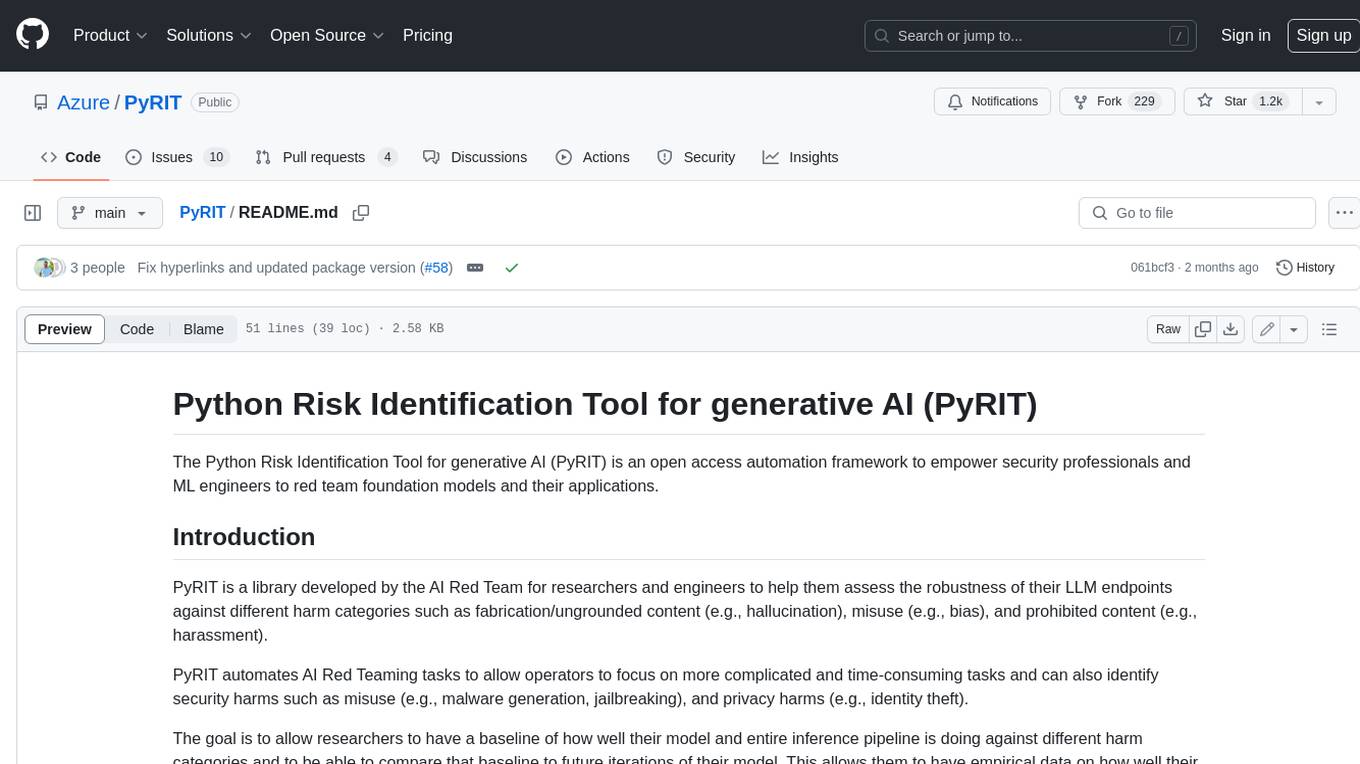
PyRIT
PyRIT is an open access automation framework designed to empower security professionals and ML engineers to red team foundation models and their applications. It automates AI Red Teaming tasks to allow operators to focus on more complicated and time-consuming tasks and can also identify security harms such as misuse (e.g., malware generation, jailbreaking), and privacy harms (e.g., identity theft). The goal is to allow researchers to have a baseline of how well their model and entire inference pipeline is doing against different harm categories and to be able to compare that baseline to future iterations of their model. This allows them to have empirical data on how well their model is doing today, and detect any degradation of performance based on future improvements.
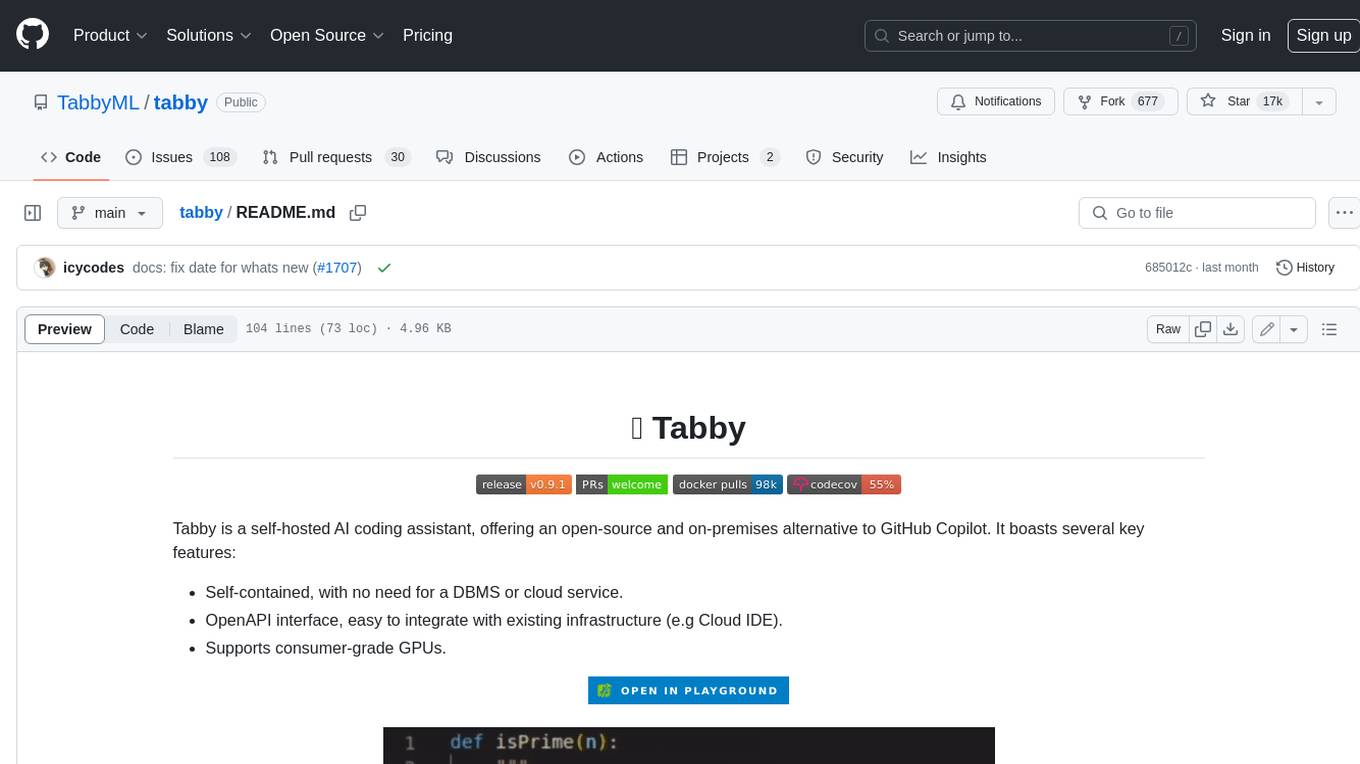
tabby
Tabby is a self-hosted AI coding assistant, offering an open-source and on-premises alternative to GitHub Copilot. It boasts several key features: * Self-contained, with no need for a DBMS or cloud service. * OpenAPI interface, easy to integrate with existing infrastructure (e.g Cloud IDE). * Supports consumer-grade GPUs.
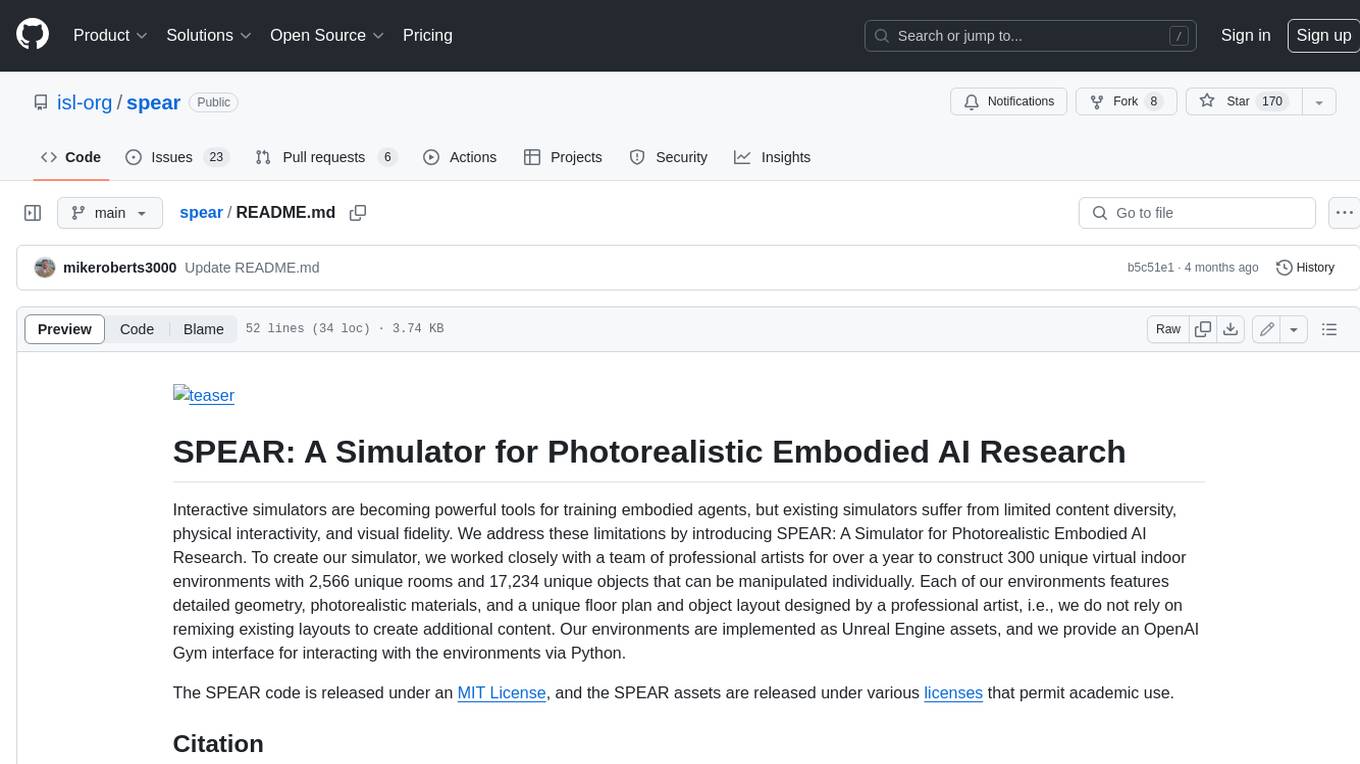
spear
SPEAR (Simulator for Photorealistic Embodied AI Research) is a powerful tool for training embodied agents. It features 300 unique virtual indoor environments with 2,566 unique rooms and 17,234 unique objects that can be manipulated individually. Each environment is designed by a professional artist and features detailed geometry, photorealistic materials, and a unique floor plan and object layout. SPEAR is implemented as Unreal Engine assets and provides an OpenAI Gym interface for interacting with the environments via Python.
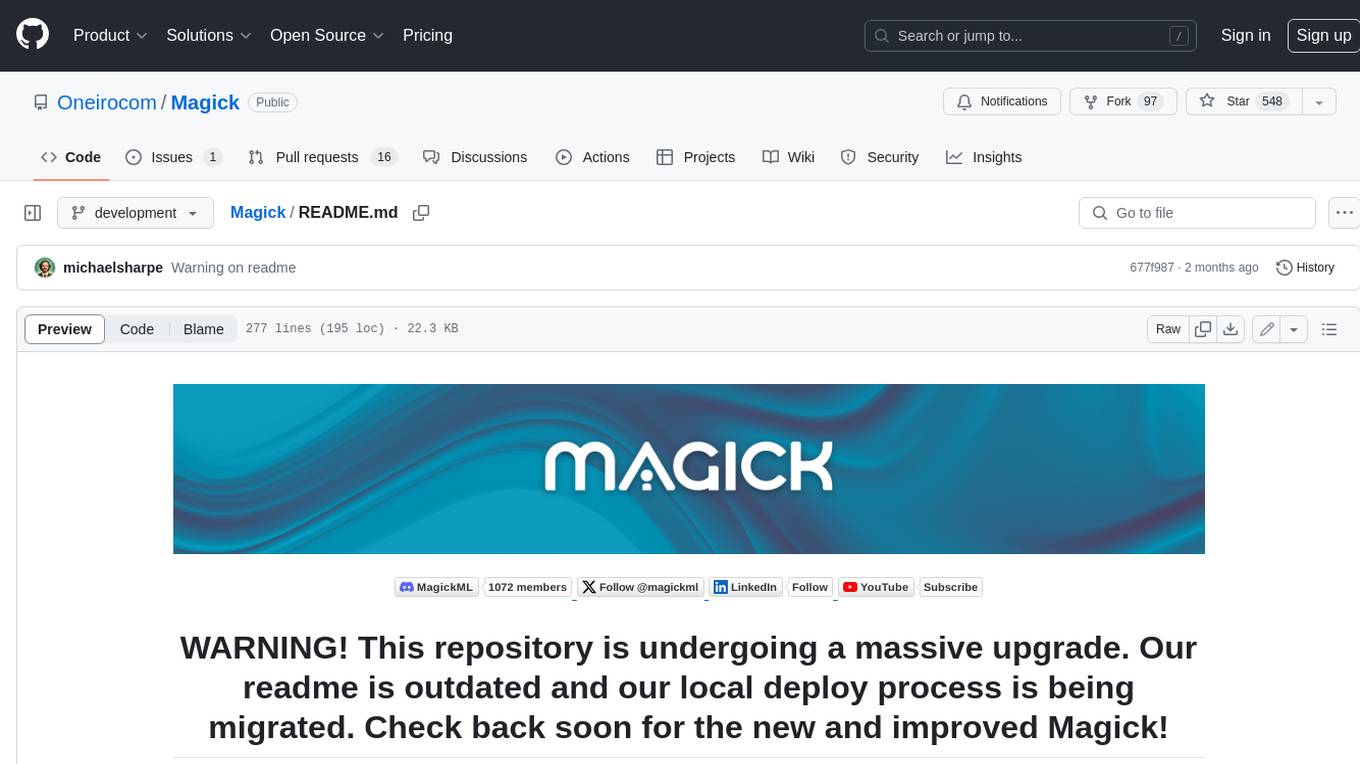
Magick
Magick is a groundbreaking visual AIDE (Artificial Intelligence Development Environment) for no-code data pipelines and multimodal agents. Magick can connect to other services and comes with nodes and templates well-suited for intelligent agents, chatbots, complex reasoning systems and realistic characters.