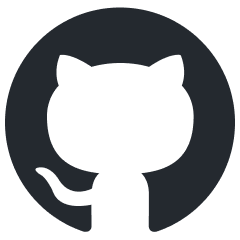
Upsonic
The most reliable AI agent framework that supports MCP.
Stars: 7238
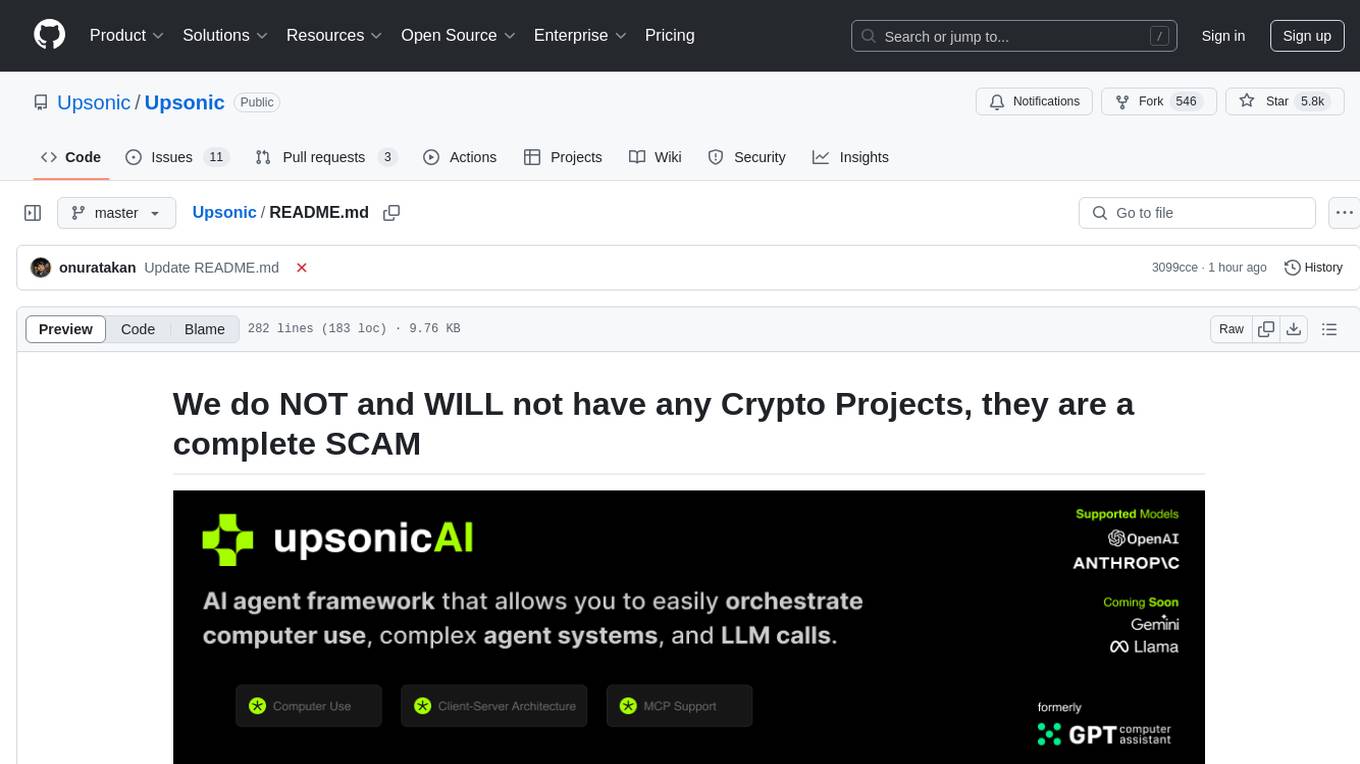
Upsonic offers a cutting-edge enterprise-ready framework for orchestrating LLM calls, agents, and computer use to complete tasks cost-effectively. It provides reliable systems, scalability, and a task-oriented structure for real-world cases. Key features include production-ready scalability, task-centric design, MCP server support, tool-calling server, computer use integration, and easy addition of custom tools. The framework supports client-server architecture and allows seamless deployment on AWS, GCP, or locally using Docker.
README:
Upsonic is a reliability-focused framework designed for real-world applications. It enables trusted agent workflows in your organization through advanced reliability features, including verification layers, triangular architecture, validator agents, and output evaluation systems.
Upsonic is a next-generation framework that makes agents production-ready by solving three critical challenges:
1- Reliability: While other frameworks require expertise and complex coding for reliability features, Upsonic offers easy-to-activate reliability layers without disrupting functionality.
2- Model Context Protocol (MCP): The MCP allows you to leverage tools with various functionalities developed both officially and by third parties without requiring you to build custom tools from scratch.
3- Integrated Browser Use and Computer Use: Directly use and deploy agents that works on non-API systems.
4- Secure Runtime: Isolated environment to run agents
LLM output reliability is critical, particularly for numerical operations and action execution. Upsonic addresses this through a multi-layered reliability system, enabling control agents and verification rounds to ensure output accuracy.
Verifier Agent: Validates outputs, tasks, and formats - detecting inconsistencies, numerical errors, and hallucinations
Editor Agent: Works with verifier feedback to revise and refine outputs until they meet quality standards
Rounds: Implements iterative quality improvement through scored verification cycles
Loops: Ensures accuracy through controlled feedback loops at critical reliability checkpoints
Upsonic is a reliability-focused framework. The results in the table were generated with a small dataset. They show success rates in the transformation of JSON keys. No hard-coded changes were made to the frameworks during testing; only the existing features of each framework were activated and run. GPT-4o was used in the tests.
10 transfers were performed for each section. The numbers show the error count. So if it says 7, it means 7 out of 10 were done incorrectly. The table has been created based on initial results. We are expanding the dataset. The tests will become more reliable after creating a larger test set. Reliability benchmark repo
Name | Reliability Score % | ASIN Code | HS Code | CIS Code | Marketing URL | Usage URL | Warranty Time | Policy Link | Policy Description |
---|---|---|---|---|---|---|---|---|---|
Upsonic | 99.3 | 0 | 1 | 0 | 0 | 0 | 0 | 0 | 0 |
CrewAI | 87.5 | 0 | 3 | 2 | 1 | 1 | 0 | 1 | 2 |
Langgraph | 6.3 | 10 | 10 | 7 | 10 | 8 | 10 | 10 | 10 |
class ReliabilityLayer:
prevent_hallucination = 10
agent = Agent("Coder", reliability_layer=ReliabilityLayer, model="openai/gpt4o")
Key features:
- Production-Ready Scalability: Deploy seamlessly on AWS, GCP, or locally using Docker.
-
Task-Centric Design: Focus on practical task execution, with options for:
- Basic tasks via LLM calls.
- Advanced tasks with V1 agents.
- Complex automation using V2 agents with MCP integration.
- MCP Server Support: Utilize multi-client processing for high-performance tasks.
- Tool-Calling Server: Exception-secure tool management with robust server API interactions.
- Computer Use Integration: Execute human-like tasks using Anthropic’s ‘Computer Use’ capabilities.
- Easily adding tools: You can add your custom tools and MCP tools with a single line of code.
You can access our documentation at docs.upsonic.ai All concepts and examples are available there.
- Python 3.10 or higher
- Access to OpenAI or Anthropic API keys (Azure and Bedrock Supported)
pip install upsonic
Set your OPENAI_API_KEY
export OPENAI_API_KEY=sk-***
Start the agent
from upsonic import Task, Agent
task = Task("Who developed you?")
agent = Agent("Coder")
agent.print_do(task)
Upsonic officially supports Model Context Protocol (MCP) and custom tools. You can use hundreds of MCP servers at glama or mcprun We also support Python functions inside a class as a tool. You can easily generate your integrations with that.
from upsonic import Agent, Task, ObjectResponse
# Define Fetch MCP configuration
class FetchMCP:
command = "uvx"
args = ["mcp-server-fetch"]
# Create response format for web content
class WebContent(ObjectResponse):
title: str
content: str
summary: str
word_count: int
# Initialize agent
web_agent = Agent(
"Web Content Analyzer",
model="openai/gpt-4o", # You can use other models
)
# Create a task to analyze a web page
task = Task(
description="Fetch and analyze the content from url. Extract the main content, title, and create a brief summary.",
context=["https://upsonic.ai"],
tools=[FetchMCP],
response_format=WebContent
)
# Usage
result = web_agent.print_do(task)
print(result.title)
print(result.summary)
Distribute tasks effectively across agents with our automated task distribution mechanism. This tool matches tasks based on the relationship between agent and task, ensuring collaborative problem-solving across agents and tasks. The output is essential for deploying an AI agent across apps or as a service. Upsonic uses Pydantic BaseClass to define structured outputs for tasks, allowing developers to specify exact response formats for their AI agent tasks.
from upsonic import Agent, Task, MultiAgent, ObjectResponse
from upsonic.tools import Search
from typing import List
# Targeted Company and Our Company
our_company = "https://redis.io/"
targeted_url = "https://upsonic.ai/"
# Response formats
class CompanyResearch(ObjectResponse):
industry: str
product_focus: str
company_values: List[str]
recent_news: List[str]
class Mail(ObjectResponse):
subject: str
content: str
# Creating Agents
researcher = Agent(
"Company Researcher",
company_url=our_company
)
strategist = Agent(
"Outreach Strategist",
company_url=our_company
)
# Creating Tasks and connect
company_task = Task(
"Research company website and analyze key information",
context=[targeted_url],
tools=[Search],
response_format=CompanyResearch
)
position_task = Task(
"Analyze Senior Developer position context and requirements",
context=[company_task, targeted_url],
)
message_task = Task(
"Create personalized outreach message using research",
context=[company_task, position_task, targeted_url],
response_format=Mail
)
# Run the Tasks over agents
results = MultiAgent.do(
[researcher, strategist],
[company_task, position_task, message_task]
)
# Print the results
print(f"Company Industry: {company_task.response.industry}")
print(f"Company Focus: {company_task.response.product_focus}")
print(f"Company Values: {company_task.response.company_values}")
print(f"Company Recent News: {company_task.response.recent_news}")
print(f"Position Analyze: {position_task.response}")
print(f"Outreach Message Subject: {message_task.response.subject}")
print(f"Outreach Message Content: {message_task.response.content}")
Direct LLM calls offer faster, cheaper solutions for simple tasks. In Upsonic, you can make calls to model providers without any abstraction level and organize structured outputs. You can also use tools with LLM calls.
from upsonic import Task, Direct
direct = Direct(model="openai/gpt-4o")
task = Task("Where can I use agents in real life?")
direct.print_do(task)
You can check out many examples showing how to build agents using MCP tools and browser use with Upsonic.
We use anonymous telemetry to collect usage data. We do this to focus our developments on more accurate points. You can disable it by setting the UPSONIC_TELEMETRY environment variable to false.
import os
os.environ["UPSONIC_TELEMETRY"] = "False"
For Tasks:
Click tags to check more tools for each tasksFor Jobs:
Alternative AI tools for Upsonic
Similar Open Source Tools
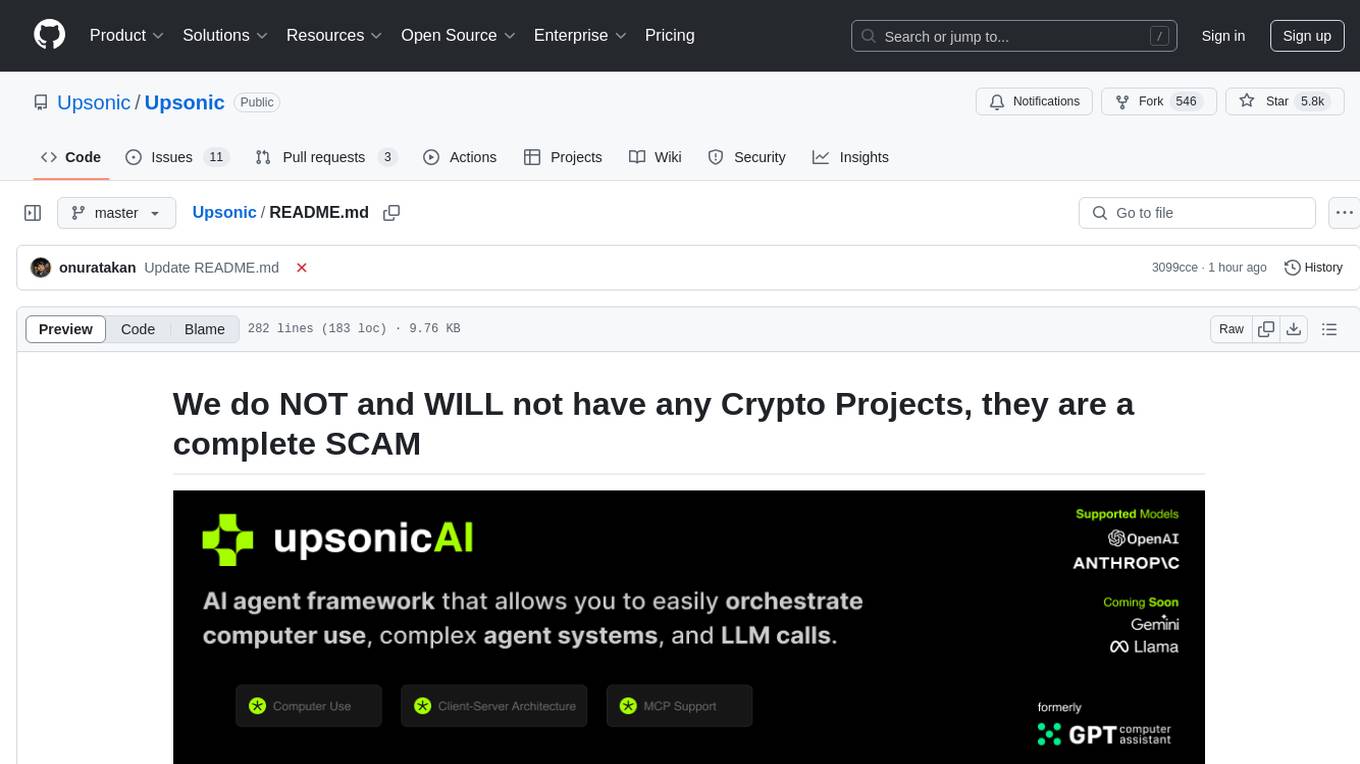
Upsonic
Upsonic offers a cutting-edge enterprise-ready framework for orchestrating LLM calls, agents, and computer use to complete tasks cost-effectively. It provides reliable systems, scalability, and a task-oriented structure for real-world cases. Key features include production-ready scalability, task-centric design, MCP server support, tool-calling server, computer use integration, and easy addition of custom tools. The framework supports client-server architecture and allows seamless deployment on AWS, GCP, or locally using Docker.
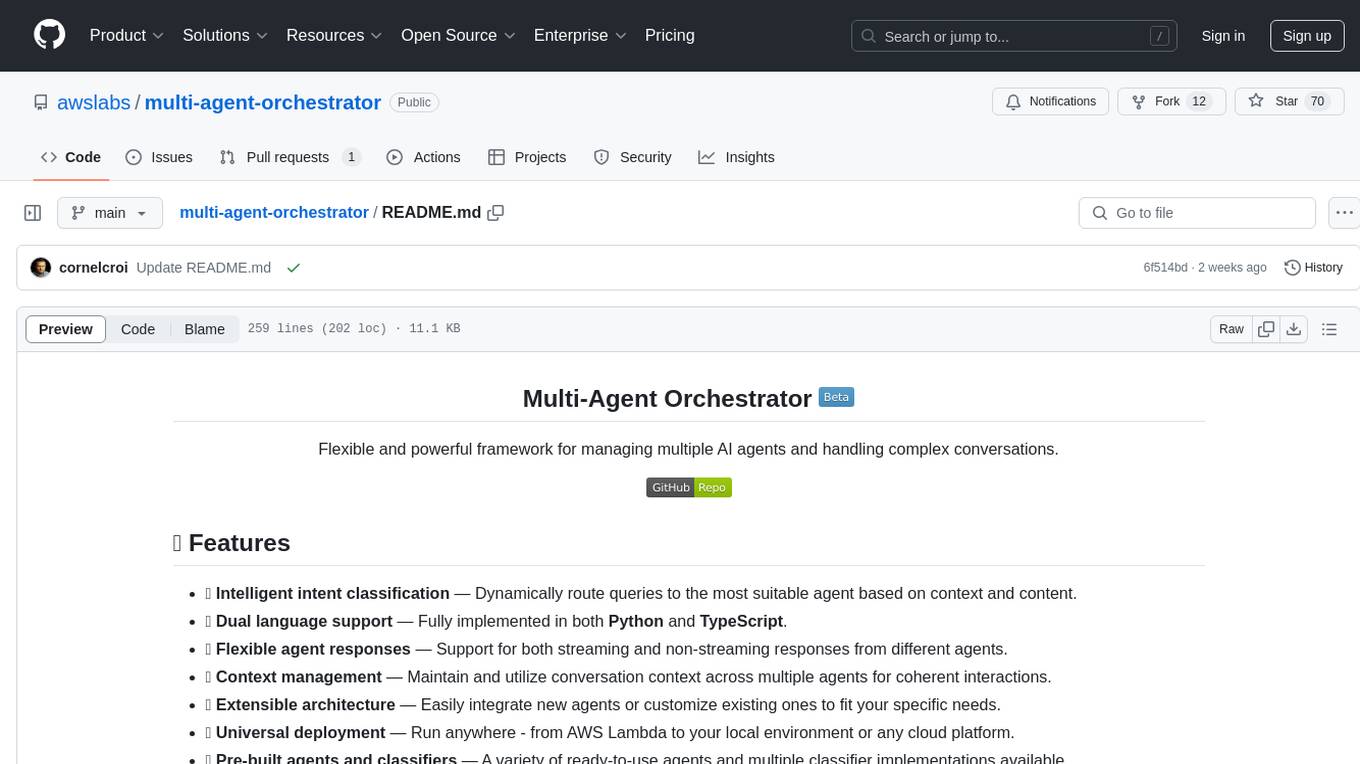
multi-agent-orchestrator
Multi-Agent Orchestrator is a flexible and powerful framework for managing multiple AI agents and handling complex conversations. It intelligently routes queries to the most suitable agent based on context and content, supports dual language implementation in Python and TypeScript, offers flexible agent responses, context management across agents, extensible architecture for customization, universal deployment options, and pre-built agents and classifiers. It is suitable for various applications, from simple chatbots to sophisticated AI systems, accommodating diverse requirements and scaling efficiently.
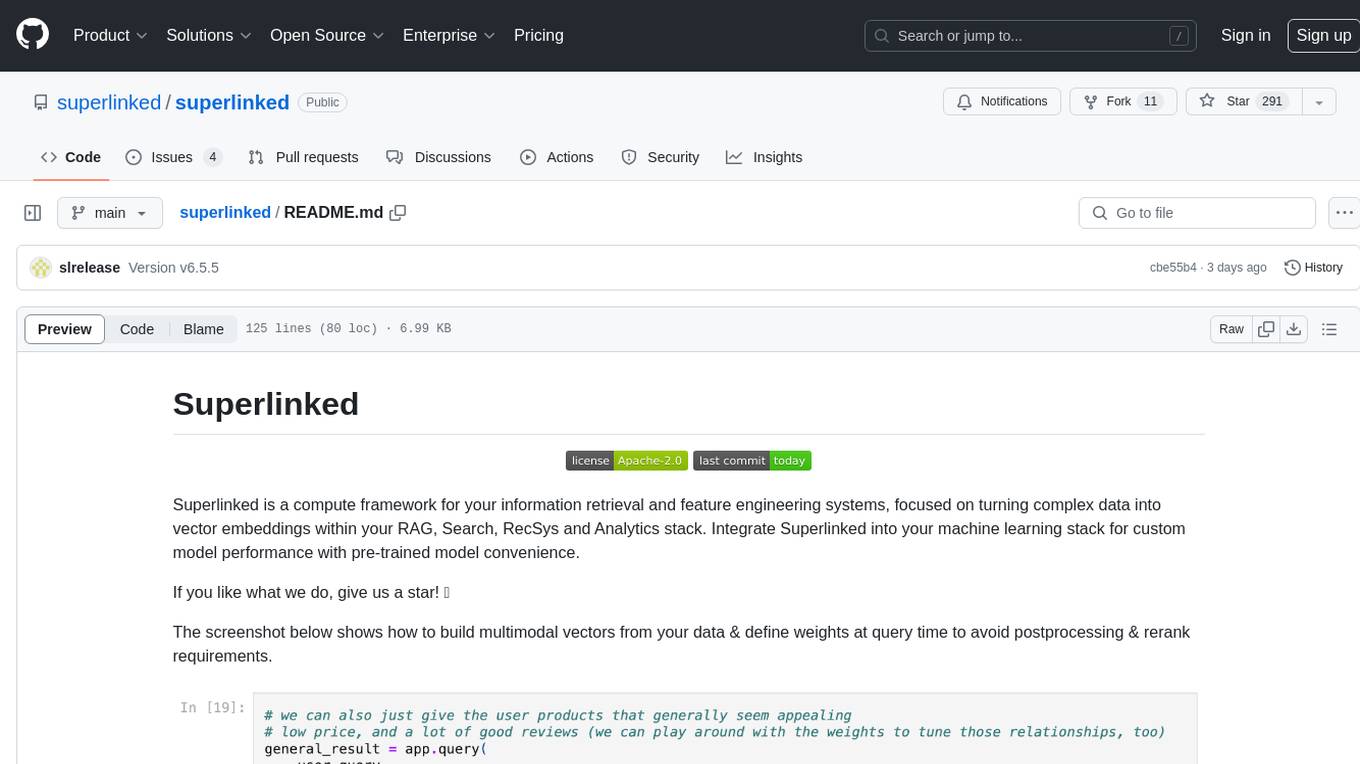
superlinked
Superlinked is a compute framework for information retrieval and feature engineering systems, focusing on converting complex data into vector embeddings for RAG, Search, RecSys, and Analytics stack integration. It enables custom model performance in machine learning with pre-trained model convenience. The tool allows users to build multimodal vectors, define weights at query time, and avoid postprocessing & rerank requirements. Users can explore the computational model through simple scripts and python notebooks, with a future release planned for production usage with built-in data infra and vector database integrations.
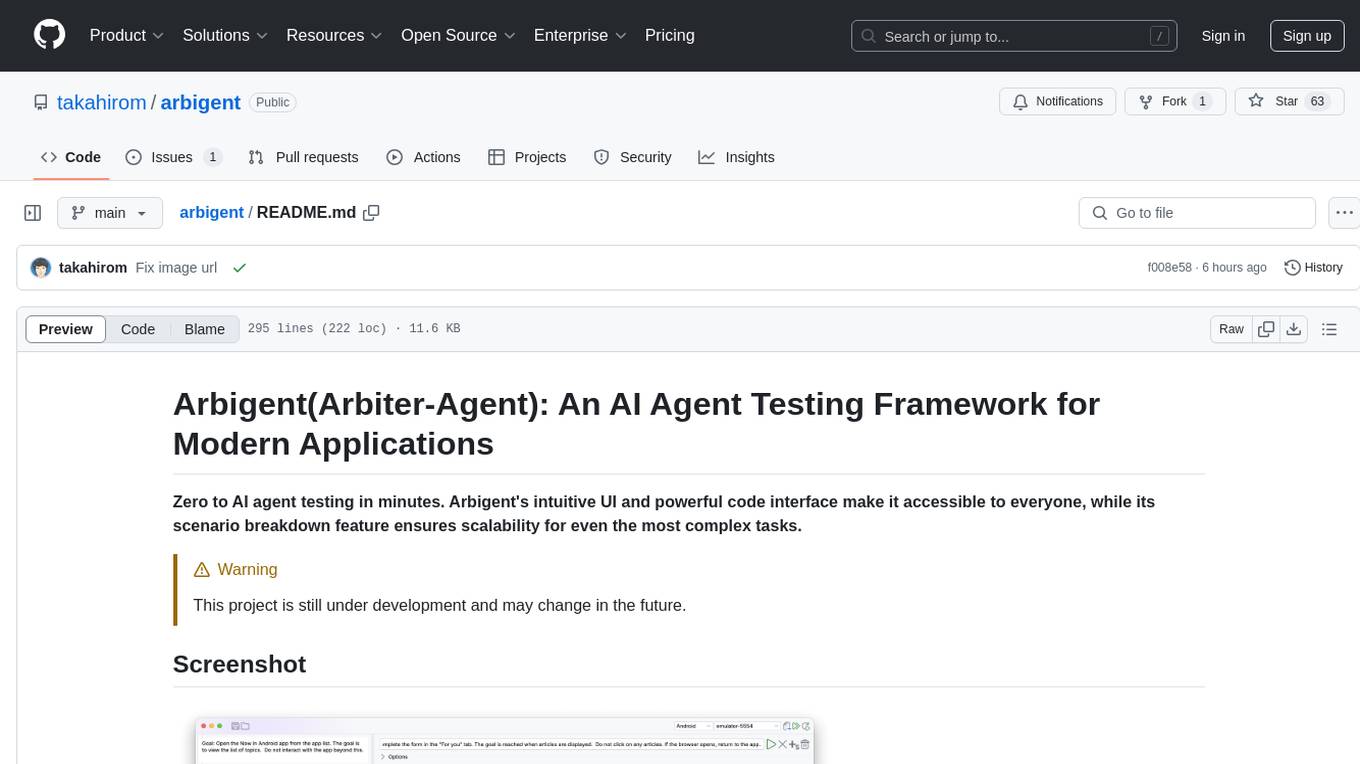
arbigent
Arbigent (Arbiter-Agent) is an AI agent testing framework designed to make AI agent testing practical for modern applications. It addresses challenges faced by traditional UI testing frameworks and AI agents by breaking down complex tasks into smaller, dependent scenarios. The framework is customizable for various AI providers, operating systems, and form factors, empowering users with extensive customization capabilities. Arbigent offers an intuitive UI for scenario creation and a powerful code interface for seamless test execution. It supports multiple form factors, optimizes UI for AI interaction, and is cost-effective by utilizing models like GPT-4o mini. With a flexible code interface and open-source nature, Arbigent aims to revolutionize AI agent testing in modern applications.
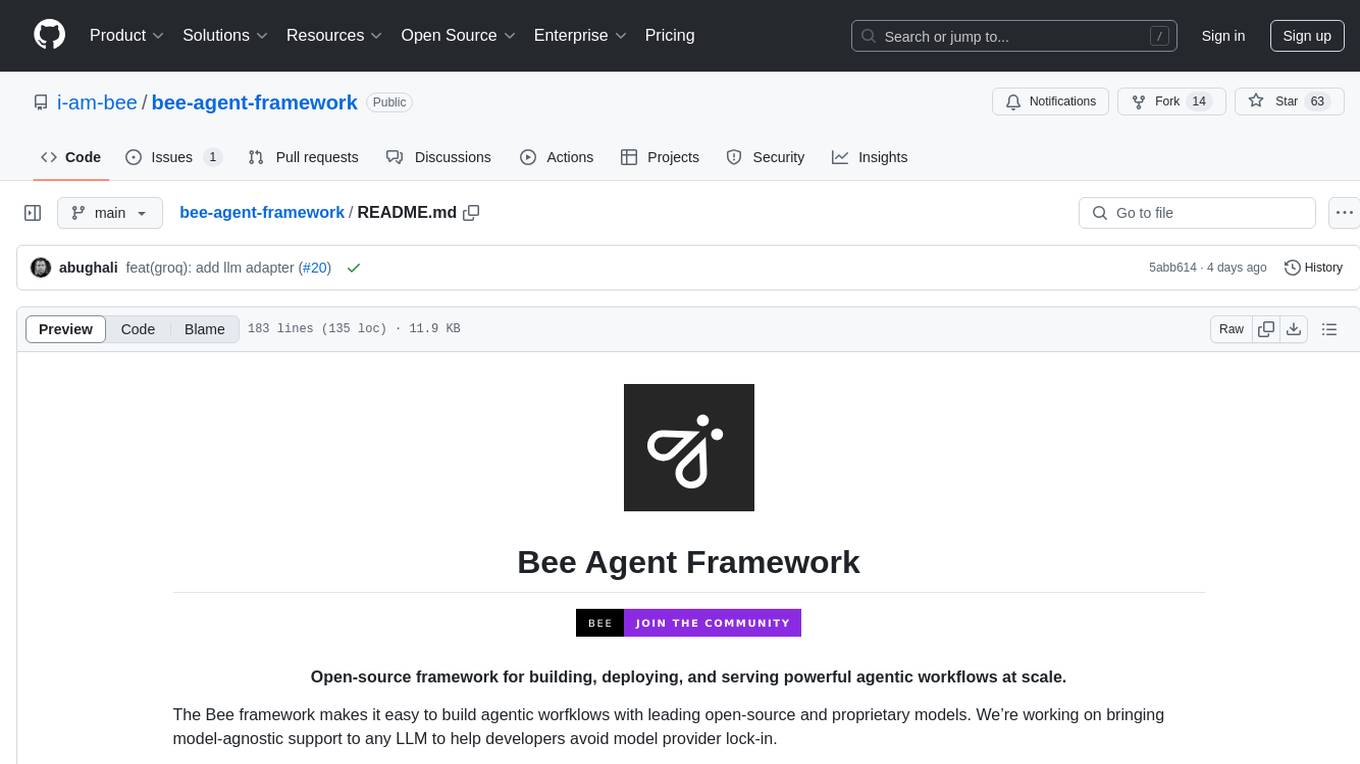
bee-agent-framework
The Bee Agent Framework is an open-source tool for building, deploying, and serving powerful agentic workflows at scale. It provides AI agents, tools for creating workflows in Javascript/Python, a code interpreter, memory optimization strategies, serialization for pausing/resuming workflows, traceability features, production-level control, and upcoming features like model-agnostic support and a chat UI. The framework offers various modules for agents, llms, memory, tools, caching, errors, adapters, logging, serialization, and more, with a roadmap including MLFlow integration, JSON support, structured outputs, chat client, base agent improvements, guardrails, and evaluation.
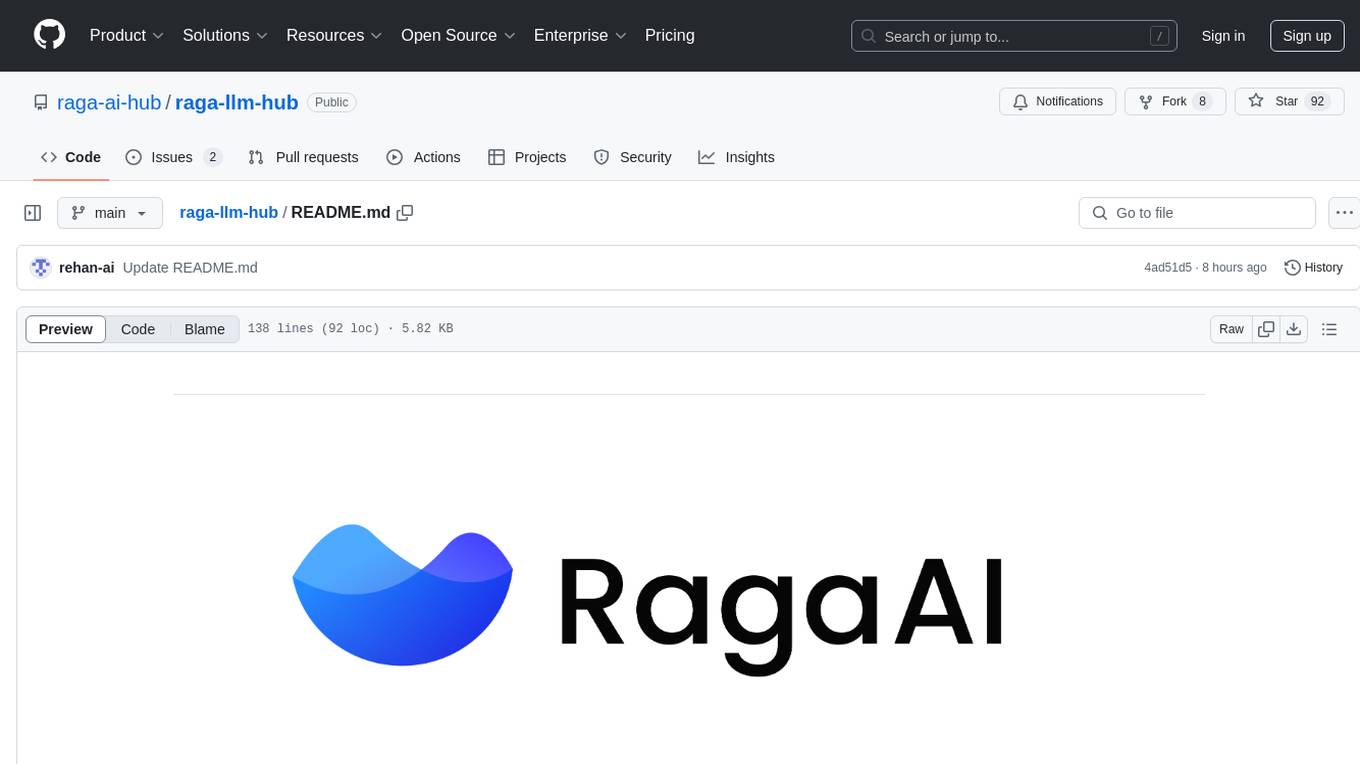
raga-llm-hub
Raga LLM Hub is a comprehensive evaluation toolkit for Language and Learning Models (LLMs) with over 100 meticulously designed metrics. It allows developers and organizations to evaluate and compare LLMs effectively, establishing guardrails for LLMs and Retrieval Augmented Generation (RAG) applications. The platform assesses aspects like Relevance & Understanding, Content Quality, Hallucination, Safety & Bias, Context Relevance, Guardrails, and Vulnerability scanning, along with Metric-Based Tests for quantitative analysis. It helps teams identify and fix issues throughout the LLM lifecycle, revolutionizing reliability and trustworthiness.
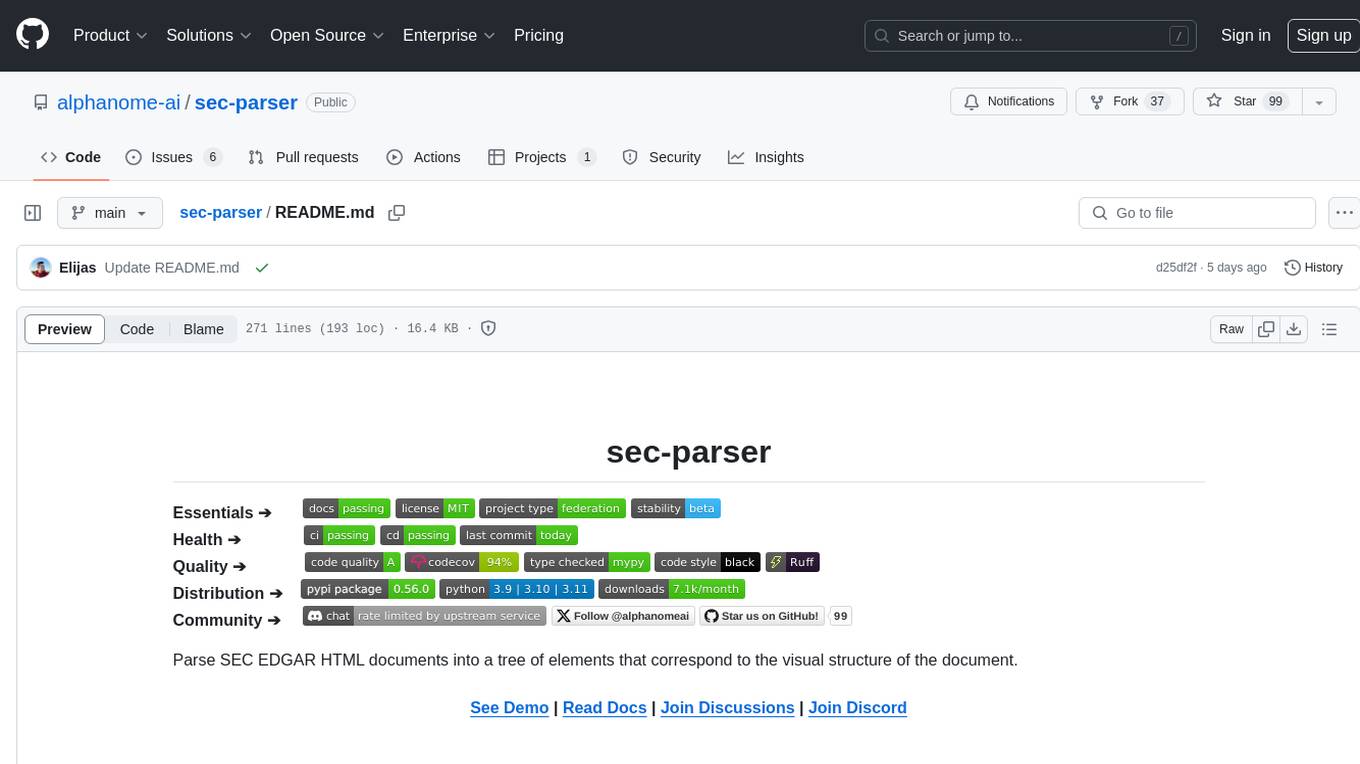
sec-parser
The `sec-parser` project simplifies extracting meaningful information from SEC EDGAR HTML documents by organizing them into semantic elements and a tree structure. It helps in parsing SEC filings for financial and regulatory analysis, analytics and data science, AI and machine learning, causal AI, and large language models. The tool is especially beneficial for AI, ML, and LLM applications by streamlining data pre-processing and feature extraction.
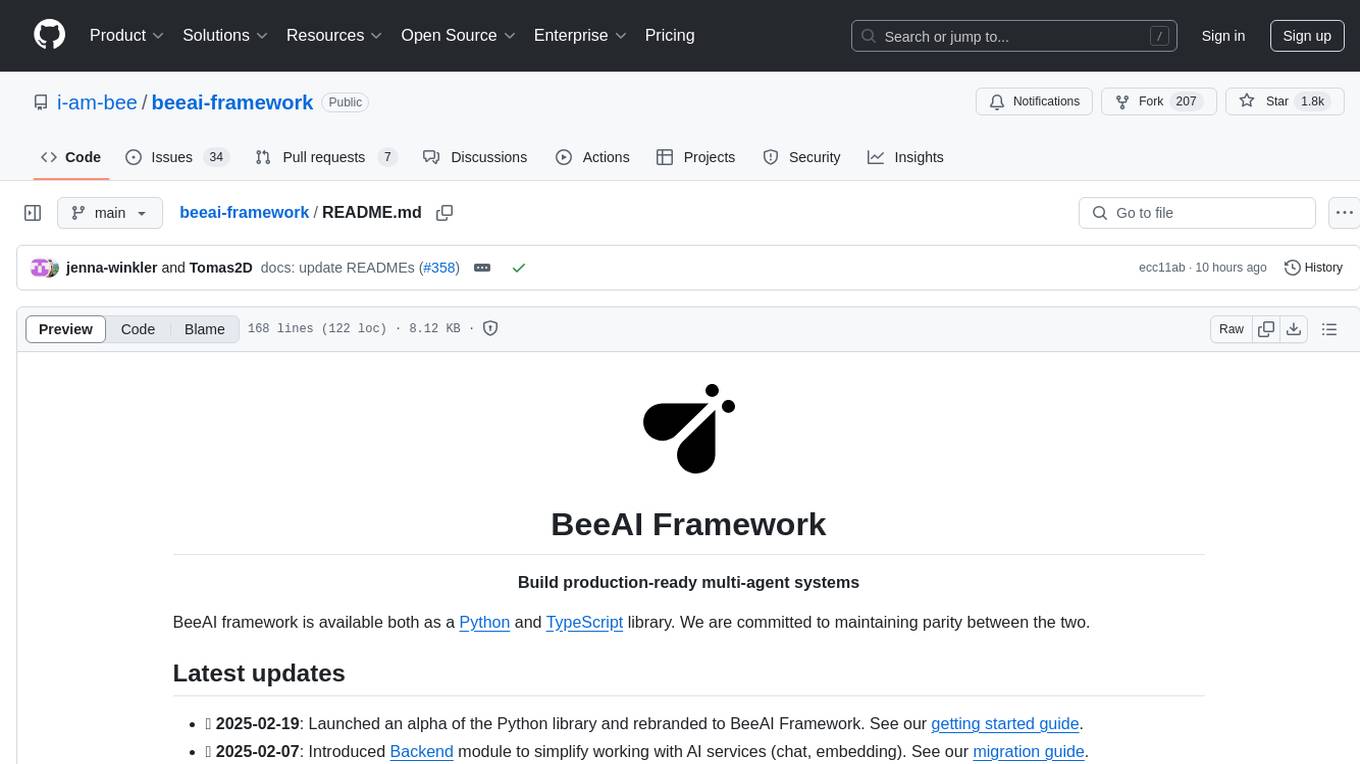
beeai-framework
BeeAI Framework is a versatile tool for building production-ready multi-agent systems. It offers flexibility in orchestrating agents, seamless integration with various models and tools, and production-grade controls for scaling. The framework supports Python and TypeScript libraries, enabling users to implement simple to complex multi-agent patterns, connect with AI services, and optimize token usage and resource management.
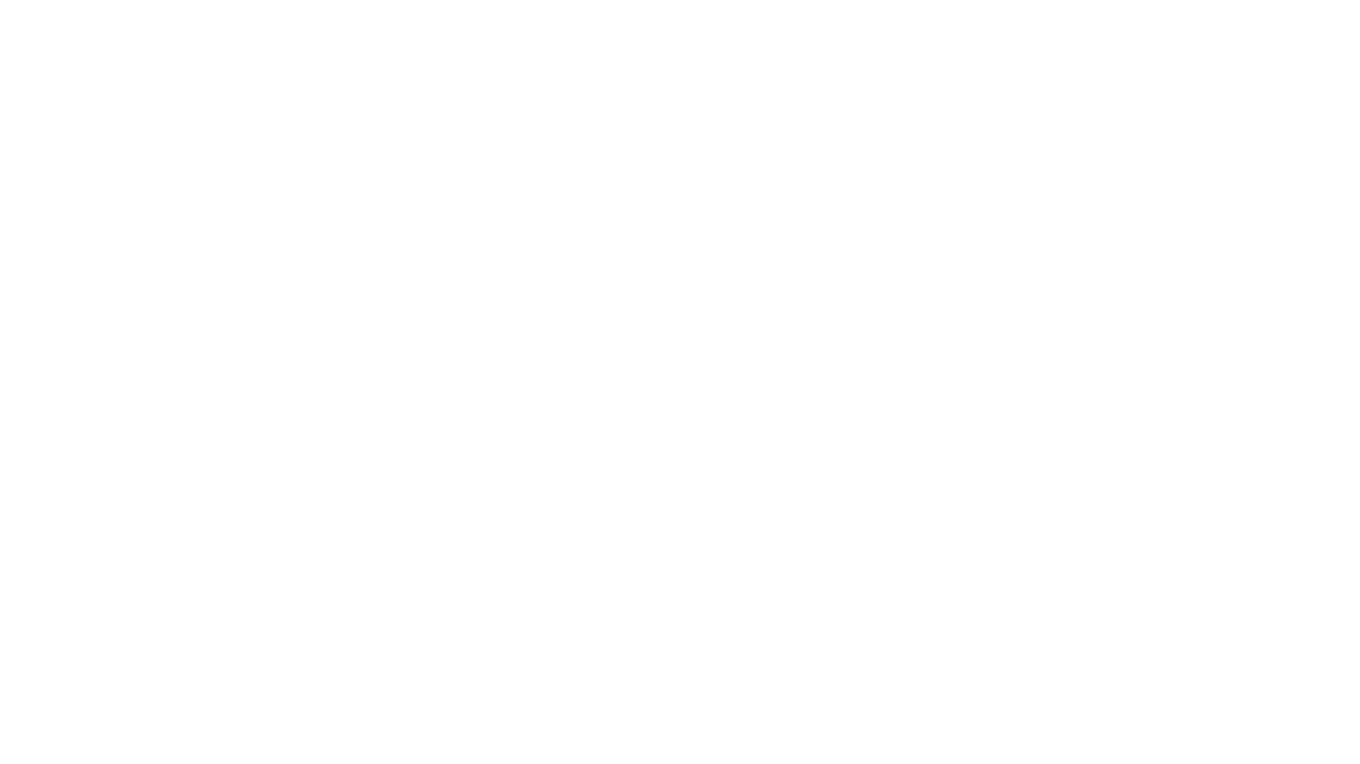
motia
Motia is an AI agent framework designed for software engineers to create, test, and deploy production-ready AI agents quickly. It provides a code-first approach, allowing developers to write agent logic in familiar languages and visualize execution in real-time. With Motia, developers can focus on business logic rather than infrastructure, offering zero infrastructure headaches, multi-language support, composable steps, built-in observability, instant APIs, and full control over AI logic. Ideal for building sophisticated agents and intelligent automations, Motia's event-driven architecture and modular steps enable the creation of GenAI-powered workflows, decision-making systems, and data processing pipelines.
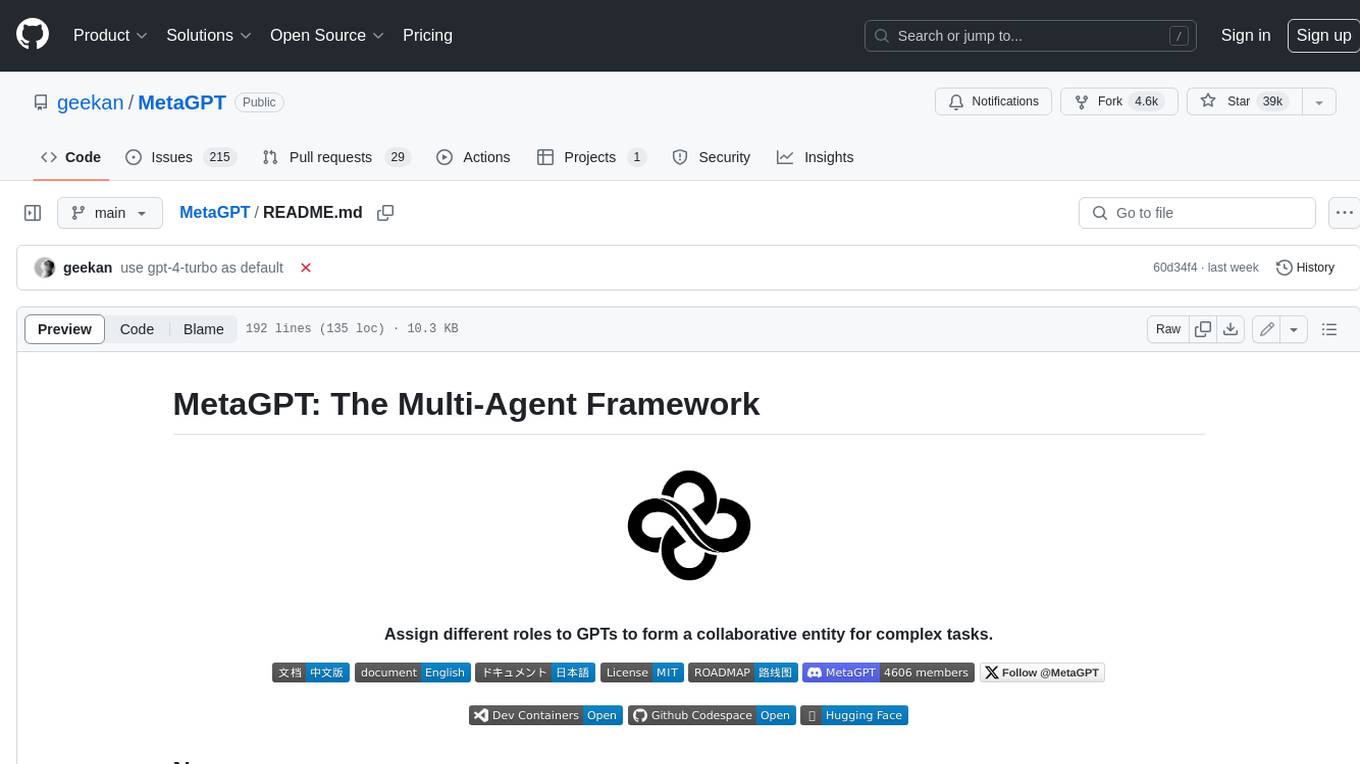
MetaGPT
MetaGPT is a multi-agent framework that enables GPT to work in a software company, collaborating to tackle more complex tasks. It assigns different roles to GPTs to form a collaborative entity for complex tasks. MetaGPT takes a one-line requirement as input and outputs user stories, competitive analysis, requirements, data structures, APIs, documents, etc. Internally, MetaGPT includes product managers, architects, project managers, and engineers. It provides the entire process of a software company along with carefully orchestrated SOPs. MetaGPT's core philosophy is "Code = SOP(Team)", materializing SOP and applying it to teams composed of LLMs.
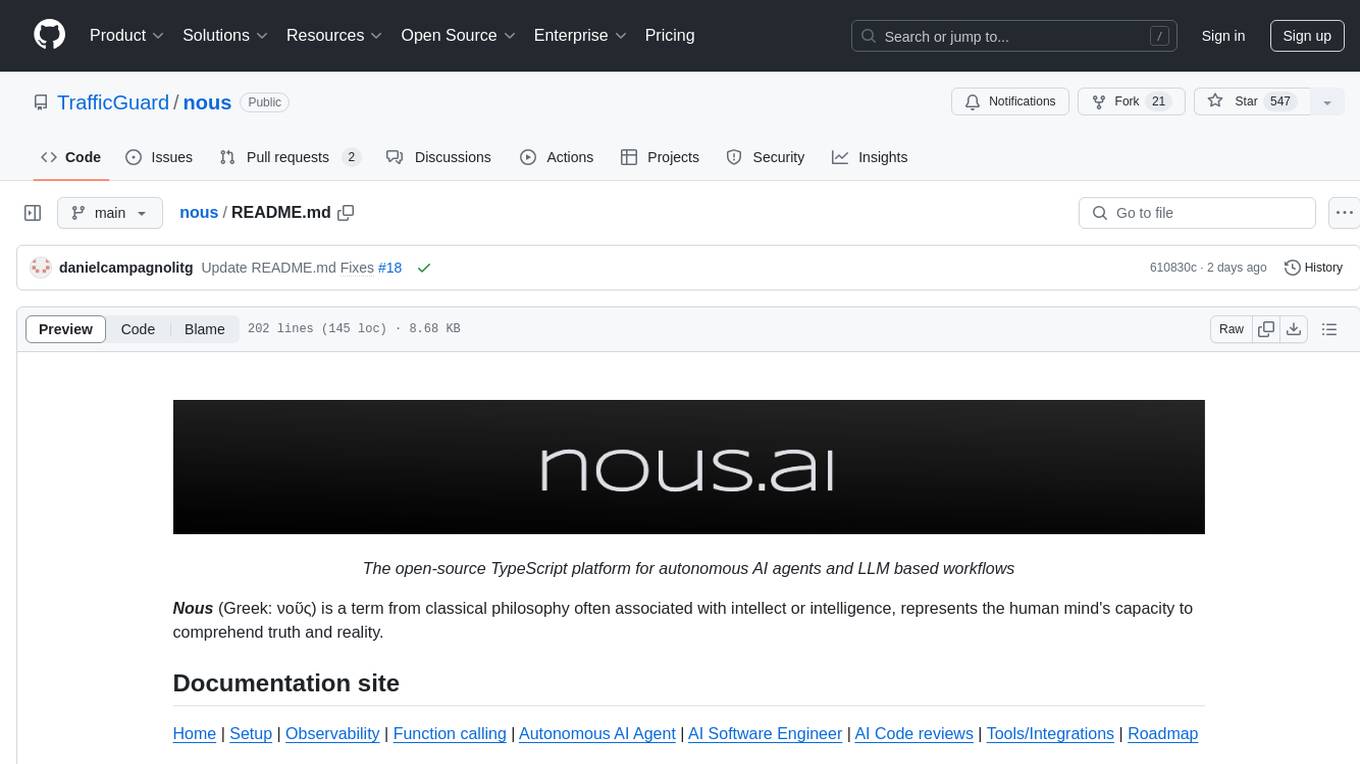
nous
Nous is an open-source TypeScript platform for autonomous AI agents and LLM based workflows. It aims to automate processes, support requests, review code, assist with refactorings, and more. The platform supports various integrations, multiple LLMs/services, CLI and web interface, human-in-the-loop interactions, flexible deployment options, observability with OpenTelemetry tracing, and specific agents for code editing, software engineering, and code review. It offers advanced features like reasoning/planning, memory and function call history, hierarchical task decomposition, and control-loop function calling options. Nous is designed to be a flexible platform for the TypeScript community to expand and support different use cases and integrations.
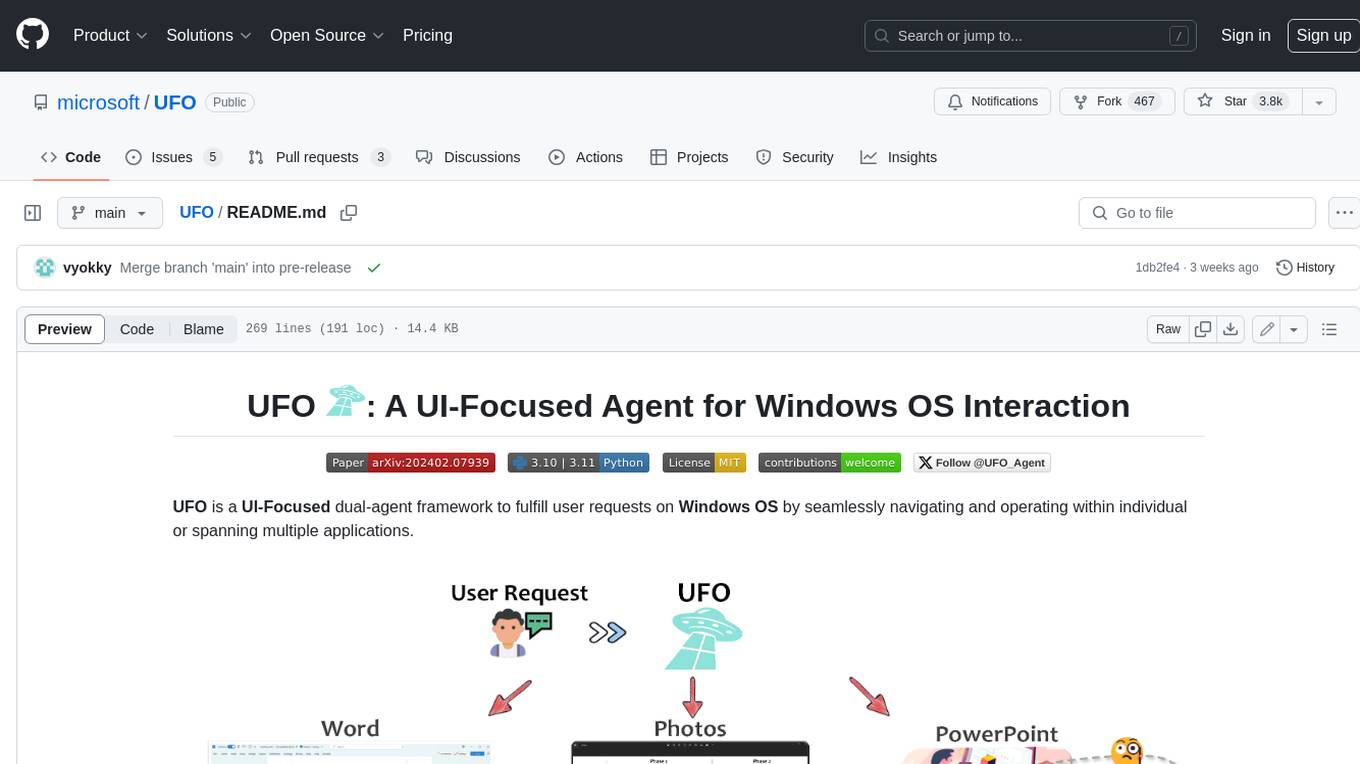
UFO
UFO is a UI-focused dual-agent framework to fulfill user requests on Windows OS by seamlessly navigating and operating within individual or spanning multiple applications.
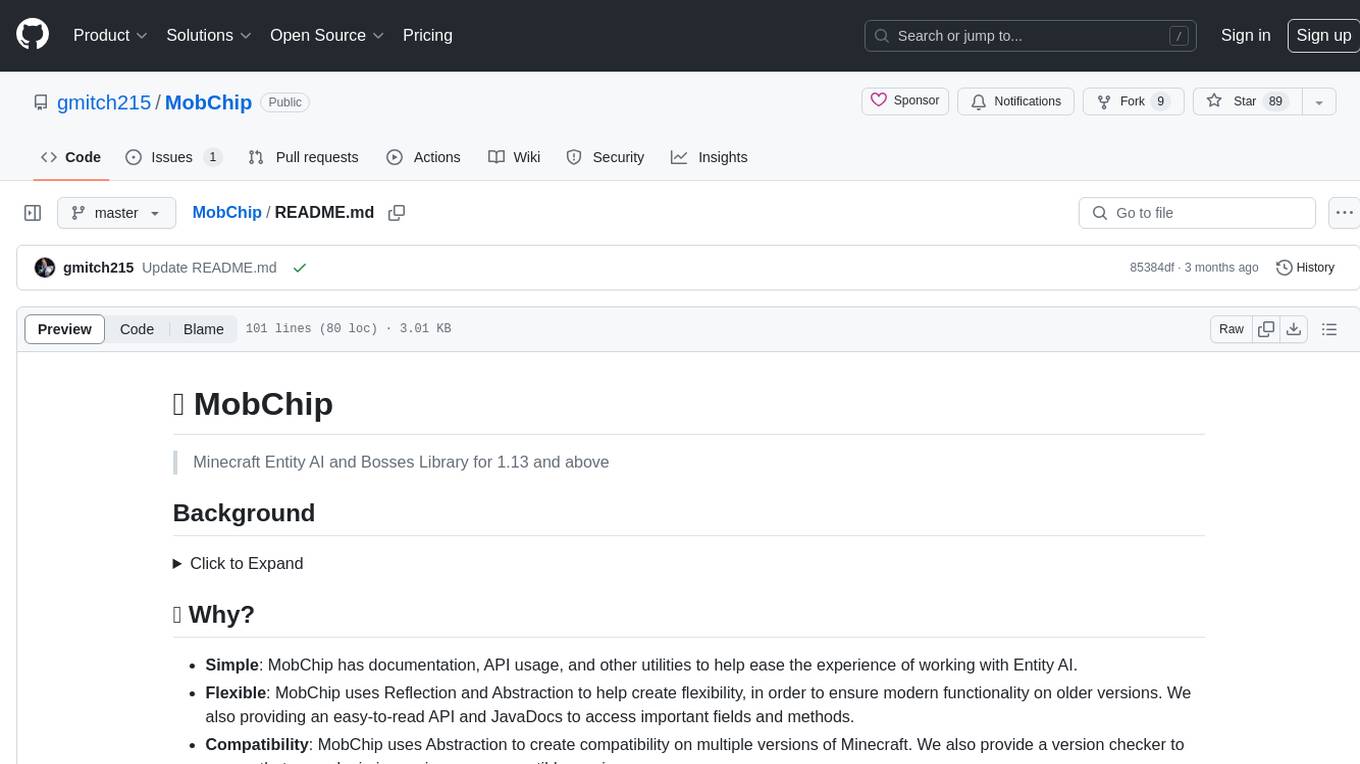
MobChip
MobChip is an all-in-one Entity AI and Bosses Library for Minecraft 1.13 and above. It simplifies the implementation of Minecraft's native entity AI into plugins, offering documentation, API usage, and utilities for ease of use. The library is flexible, using Reflection and Abstraction for modern functionality on older versions, and ensuring compatibility across multiple Minecraft versions. MobChip is open source, providing features like Bosses Library, Pathfinder Goals, Behaviors, Villager Gossip, Ender Dragon Phases, and more.
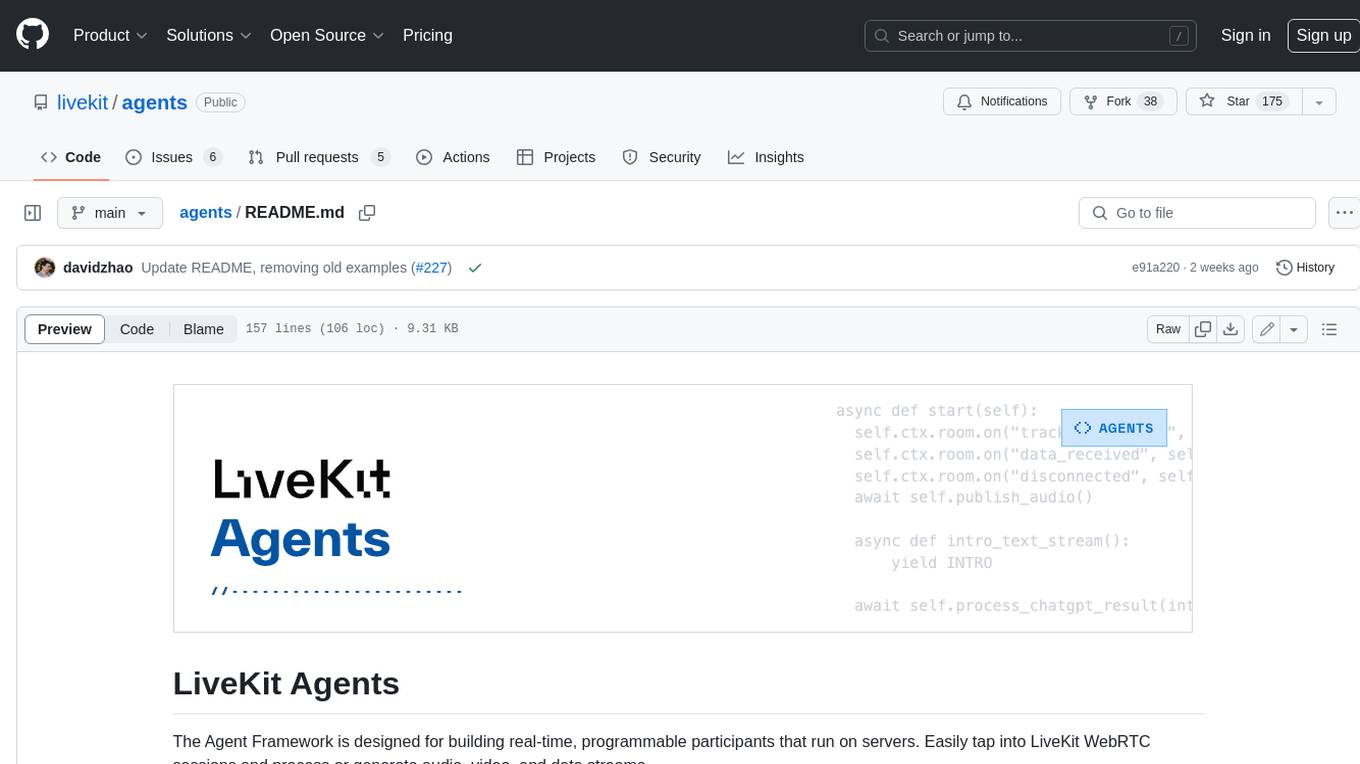
agents
The LiveKit Agent Framework is designed for building real-time, programmable participants that run on servers. Easily tap into LiveKit WebRTC sessions and process or generate audio, video, and data streams. The framework includes plugins for common workflows, such as voice activity detection and speech-to-text. Agents integrates seamlessly with LiveKit server, offloading job queuing and scheduling responsibilities to it. This eliminates the need for additional queuing infrastructure. Agent code developed on your local machine can scale to support thousands of concurrent sessions when deployed to a server in production.
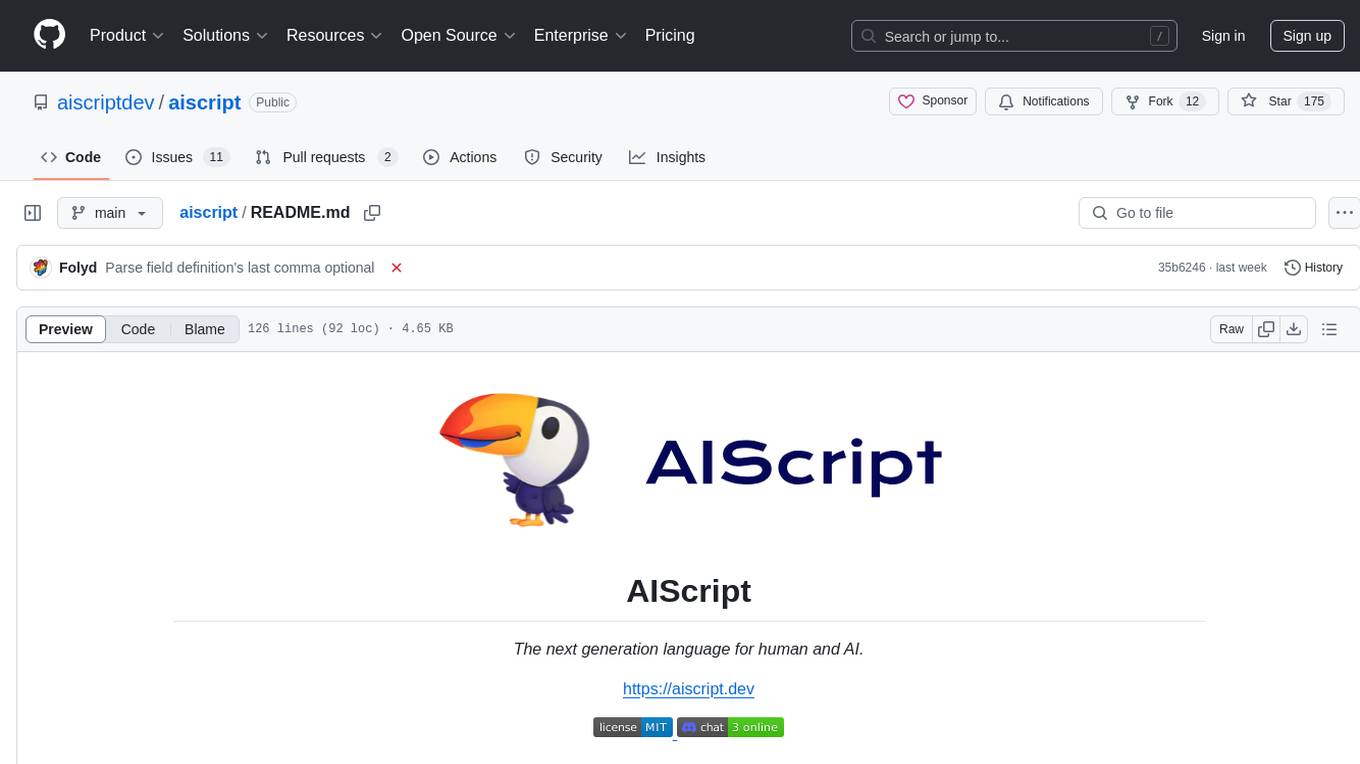
aiscript
AIScript is a unique programming language and web framework written in Rust, designed to help developers effortlessly build AI applications. It combines the strengths of Python, JavaScript, and Rust to create an intuitive, powerful, and easy-to-use tool. The language features first-class functions, built-in AI primitives, dynamic typing with static type checking, data validation, error handling inspired by Rust, a rich standard library, and automatic garbage collection. The web framework offers an elegant route DSL, automatic parameter validation, OpenAPI schema generation, database modules, authentication capabilities, and more. AIScript excels in AI-powered APIs, prototyping, microservices, data validation, and building internal tools.
For similar tasks
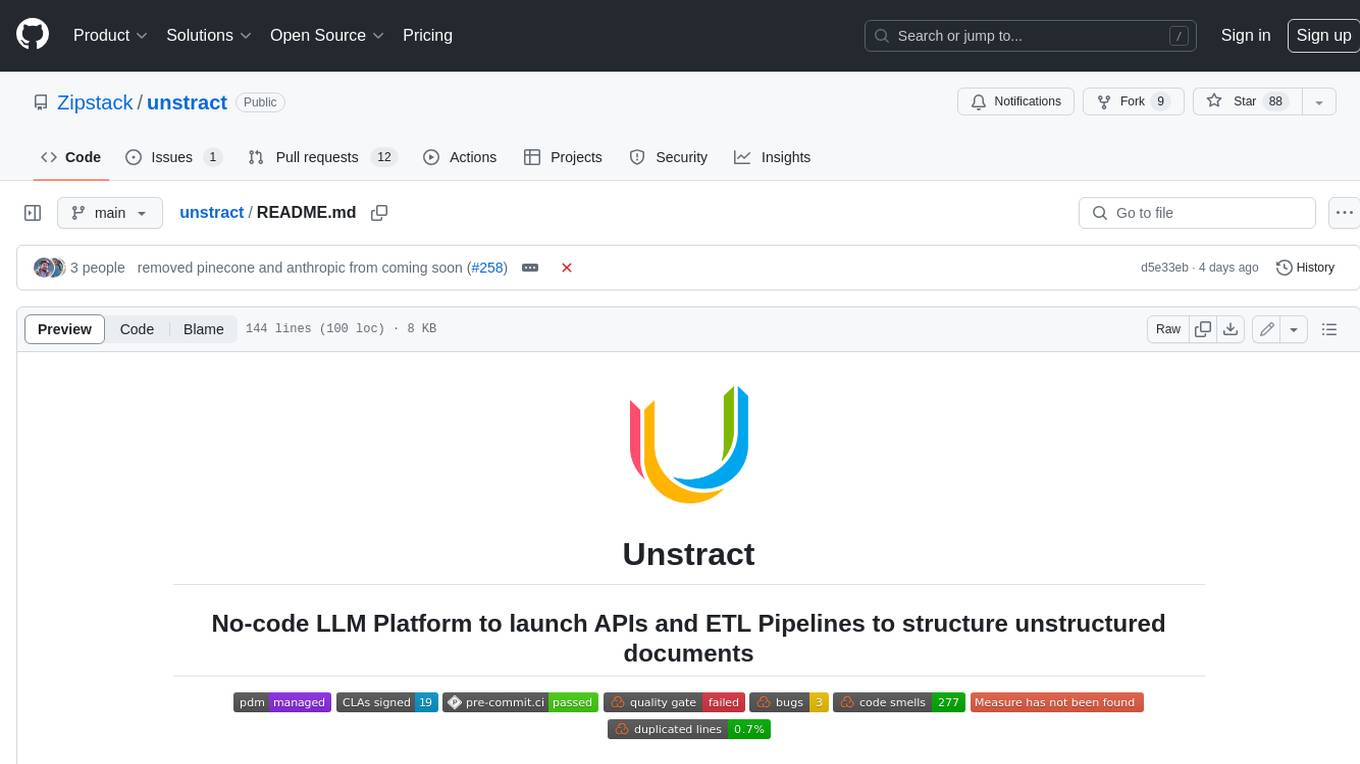
unstract
Unstract is a no-code platform that enables users to launch APIs and ETL pipelines to structure unstructured documents. With Unstract, users can go beyond co-pilots by enabling machine-to-machine automation. Unstract's Prompt Studio provides a simple, no-code approach to creating prompts for LLMs, vector databases, embedding models, and text extractors. Users can then configure Prompt Studio projects as API deployments or ETL pipelines to automate critical business processes that involve complex documents. Unstract supports a wide range of LLM providers, vector databases, embeddings, text extractors, ETL sources, and ETL destinations, providing users with the flexibility to choose the best tools for their needs.
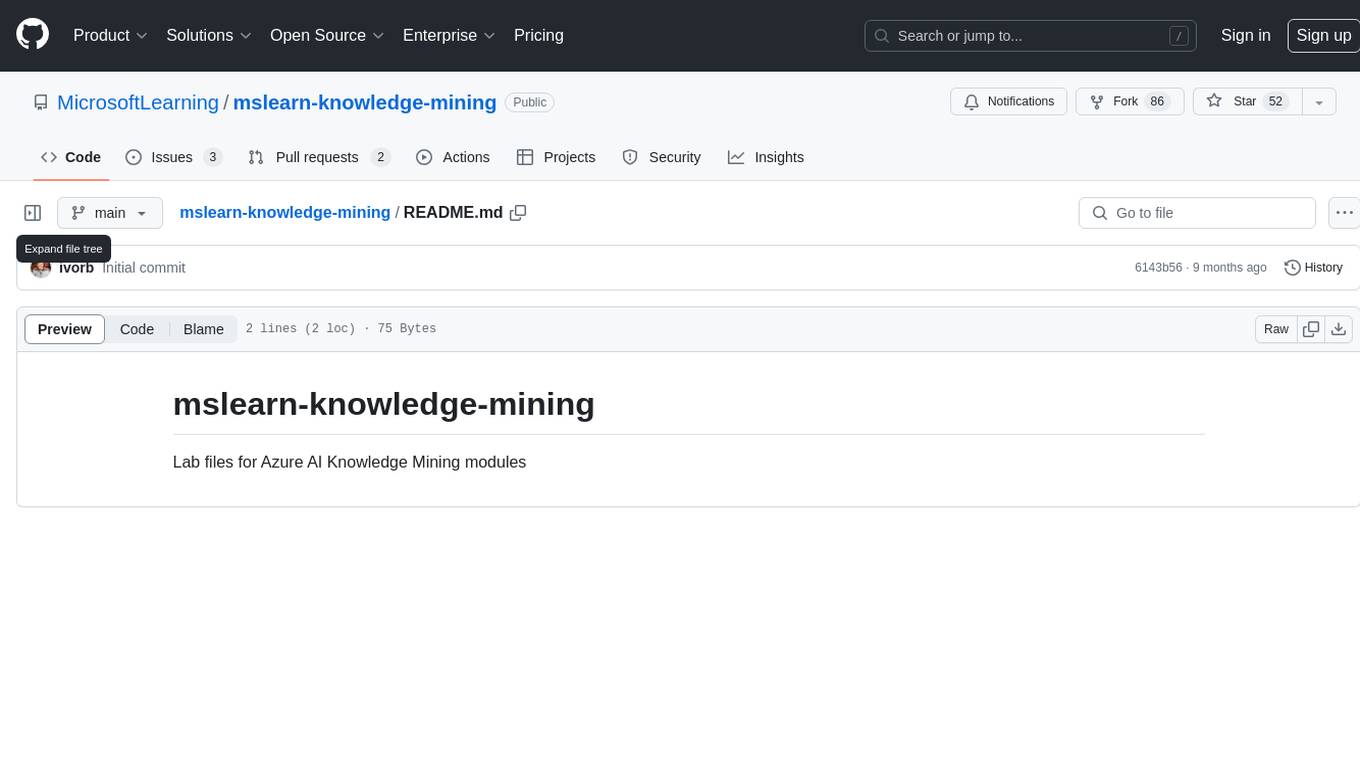
mslearn-knowledge-mining
The mslearn-knowledge-mining repository contains lab files for Azure AI Knowledge Mining modules. It provides resources for learning and implementing knowledge mining techniques using Azure AI services. The repository is designed to help users explore and understand how to leverage AI for knowledge mining purposes within the Azure ecosystem.
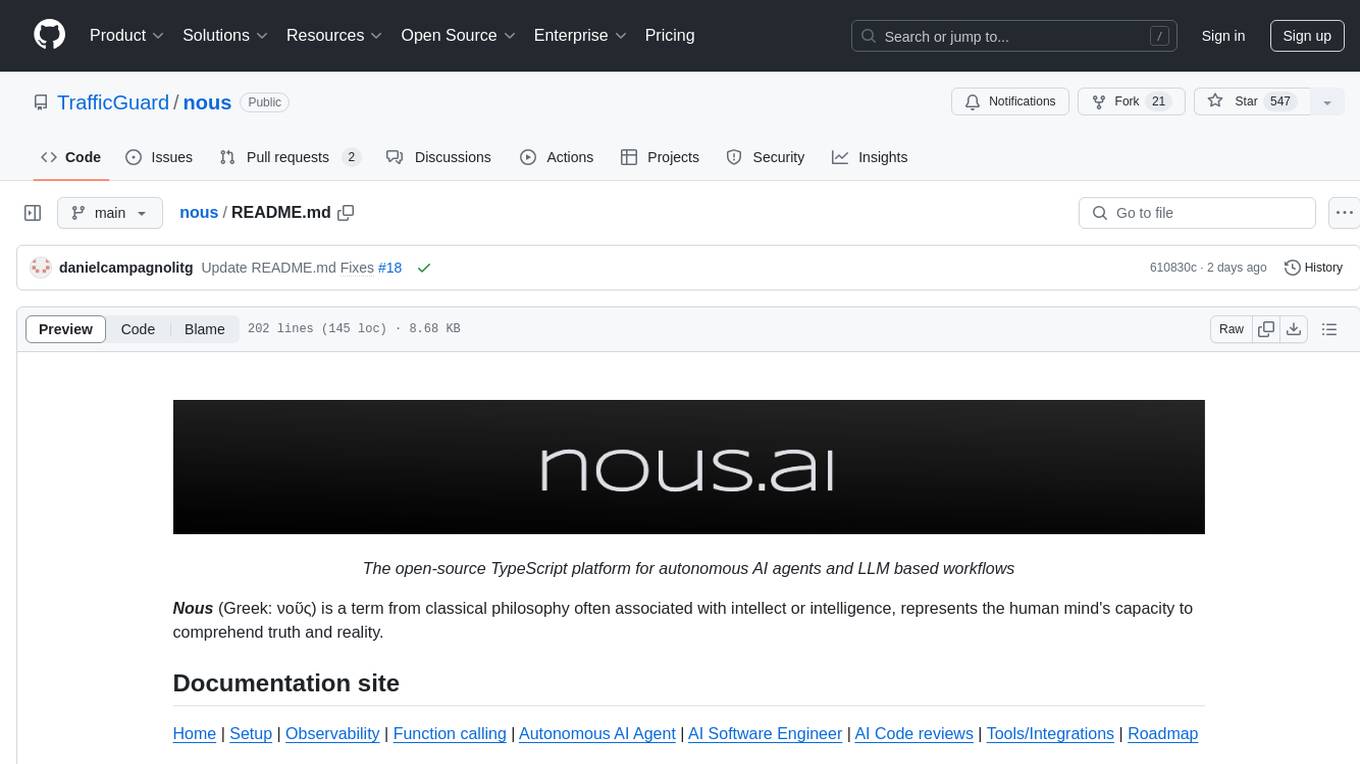
nous
Nous is an open-source TypeScript platform for autonomous AI agents and LLM based workflows. It aims to automate processes, support requests, review code, assist with refactorings, and more. The platform supports various integrations, multiple LLMs/services, CLI and web interface, human-in-the-loop interactions, flexible deployment options, observability with OpenTelemetry tracing, and specific agents for code editing, software engineering, and code review. It offers advanced features like reasoning/planning, memory and function call history, hierarchical task decomposition, and control-loop function calling options. Nous is designed to be a flexible platform for the TypeScript community to expand and support different use cases and integrations.
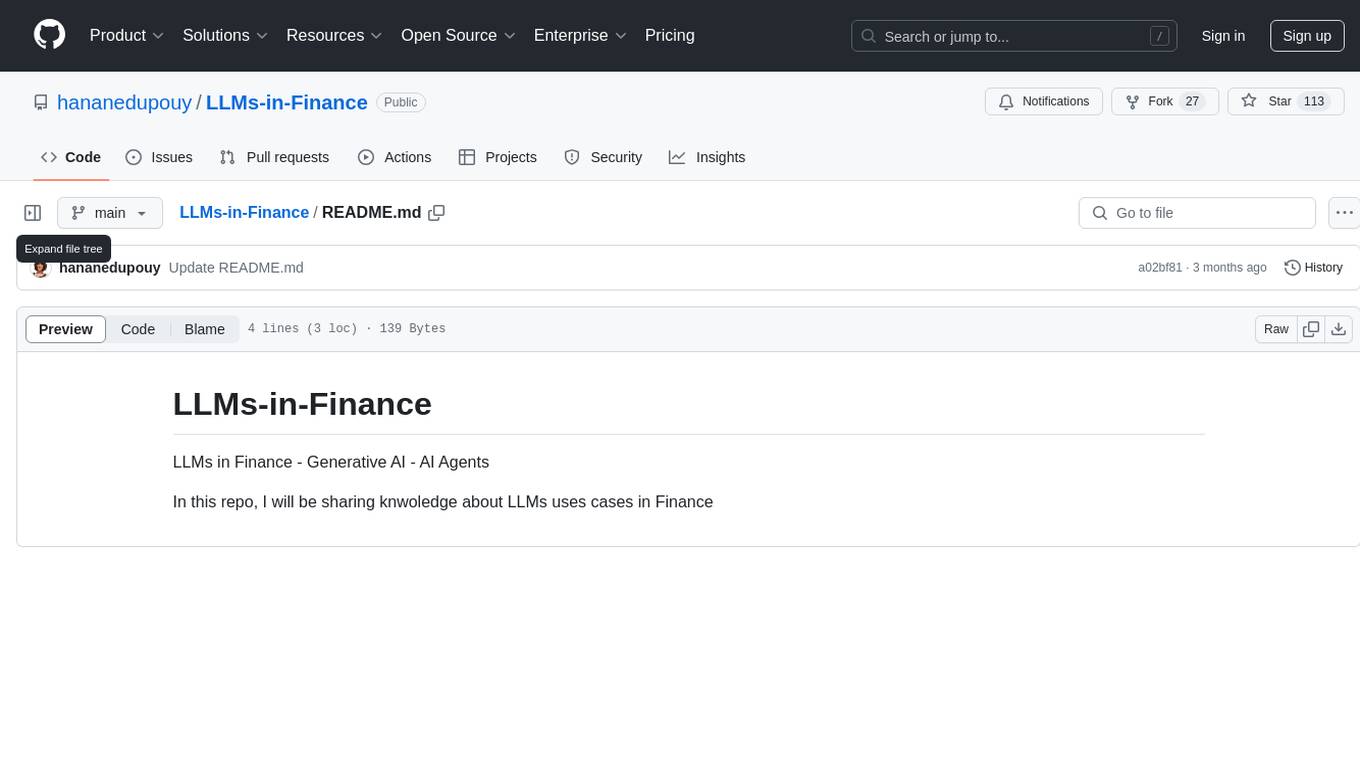
LLMs-in-Finance
This repository focuses on the application of Large Language Models (LLMs) in the field of finance. It provides insights and knowledge about how LLMs can be utilized in various scenarios within the finance industry, particularly in generating AI agents. The repository aims to explore the potential of LLMs to enhance financial processes and decision-making through the use of advanced natural language processing techniques.
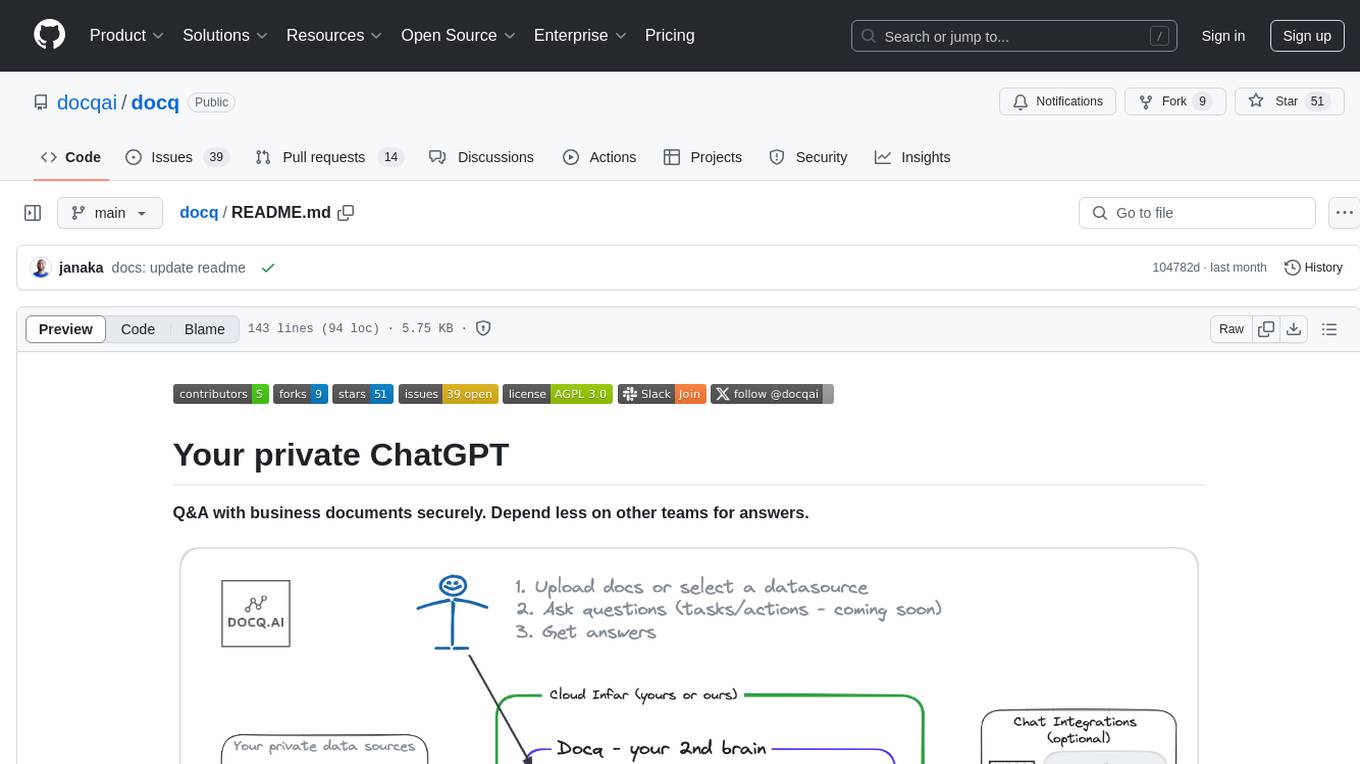
docq
Docq is a private and secure GenAI tool designed to extract knowledge from business documents, enabling users to find answers independently. It allows data to stay within organizational boundaries, supports self-hosting with various cloud vendors, and offers multi-model and multi-modal capabilities. Docq is extensible, open-source (AGPLv3), and provides commercial licensing options. The tool aims to be a turnkey solution for organizations to adopt AI innovation safely, with plans for future features like more data ingestion options and model fine-tuning.
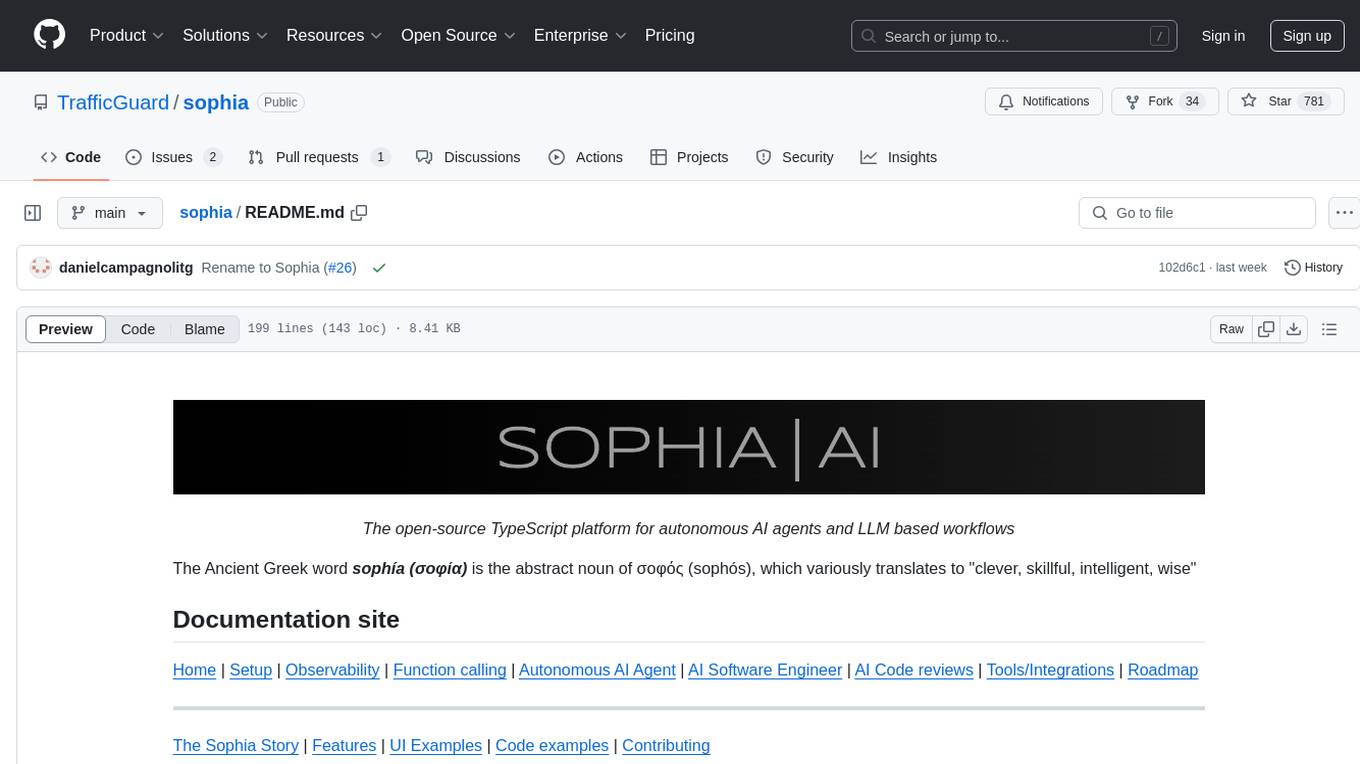
sophia
Sophia is an open-source TypeScript platform designed for autonomous AI agents and LLM based workflows. It aims to automate processes, review code, assist with refactorings, and support various integrations. The platform offers features like advanced autonomous agents, reasoning/planning inspired by Google's Self-Discover paper, memory and function call history, adaptive iterative planning, and more. Sophia supports multiple LLMs/services, CLI and web interface, human-in-the-loop interactions, flexible deployment options, observability with OpenTelemetry tracing, and specific agents for code editing, software engineering, and code review. It provides a flexible platform for the TypeScript community to expand and support various use cases and integrations.
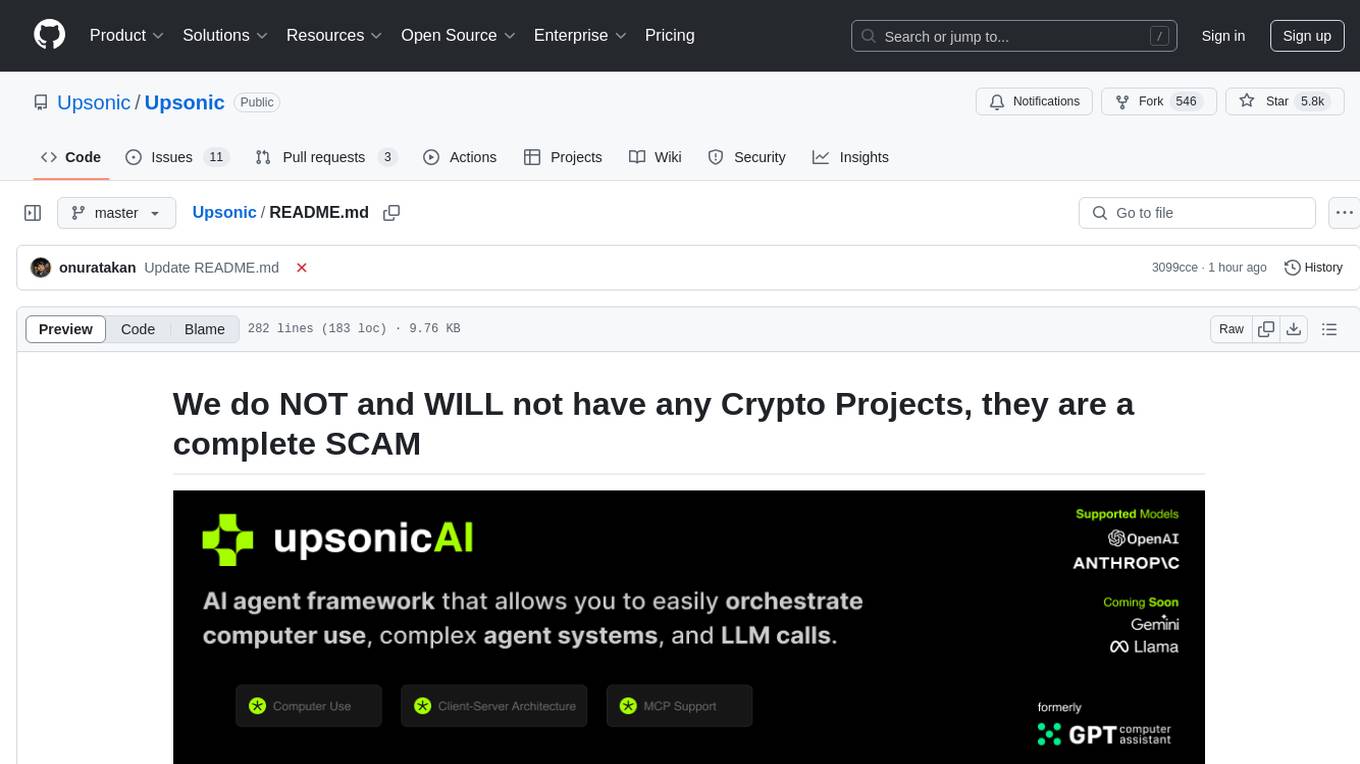
Upsonic
Upsonic offers a cutting-edge enterprise-ready framework for orchestrating LLM calls, agents, and computer use to complete tasks cost-effectively. It provides reliable systems, scalability, and a task-oriented structure for real-world cases. Key features include production-ready scalability, task-centric design, MCP server support, tool-calling server, computer use integration, and easy addition of custom tools. The framework supports client-server architecture and allows seamless deployment on AWS, GCP, or locally using Docker.
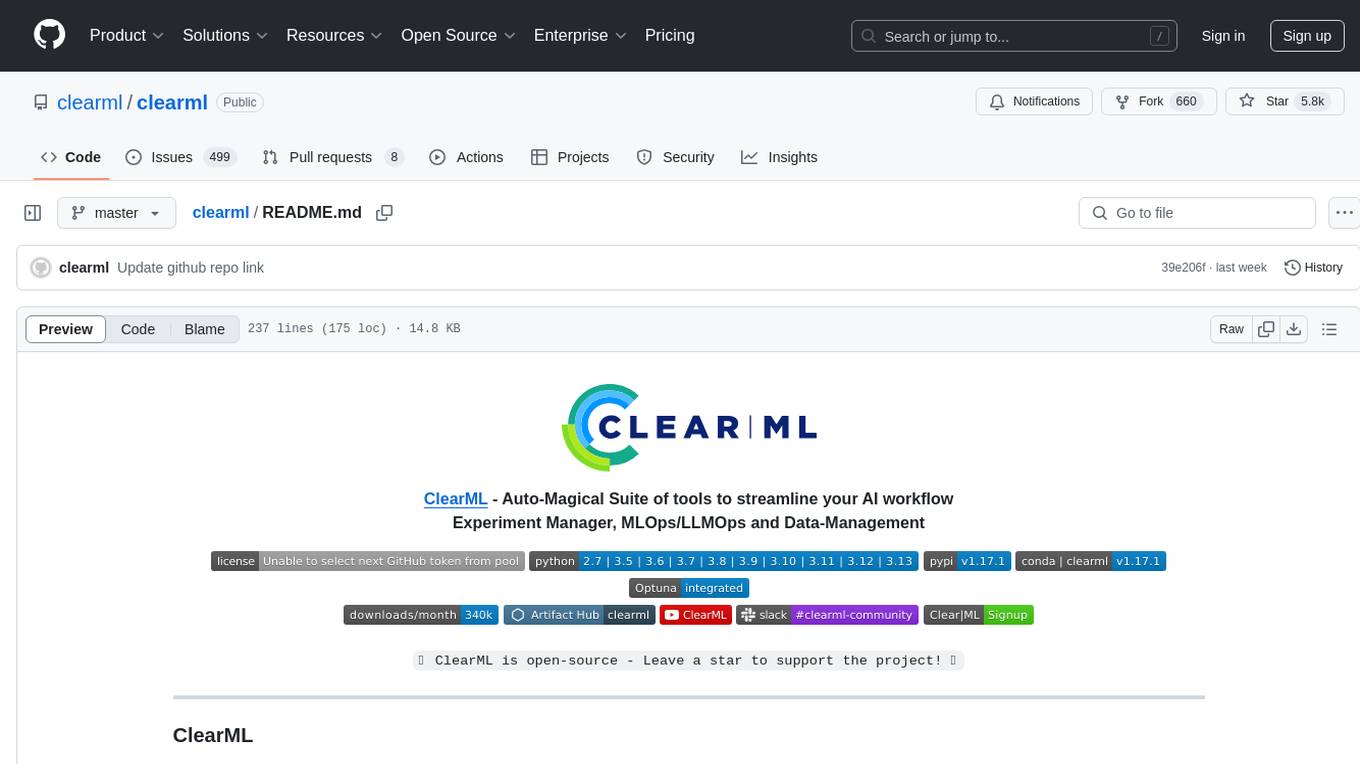
clearml
ClearML is an auto-magical suite of tools designed to streamline AI workflows. It includes modules for experiment management, MLOps/LLMOps, data management, model serving, and more. ClearML offers features like experiment tracking, model serving, orchestration, and automation. It supports various ML/DL frameworks and integrates with Jupyter Notebook and PyCharm for remote debugging. ClearML aims to simplify collaboration, automate processes, and enhance visibility in AI projects.
For similar jobs
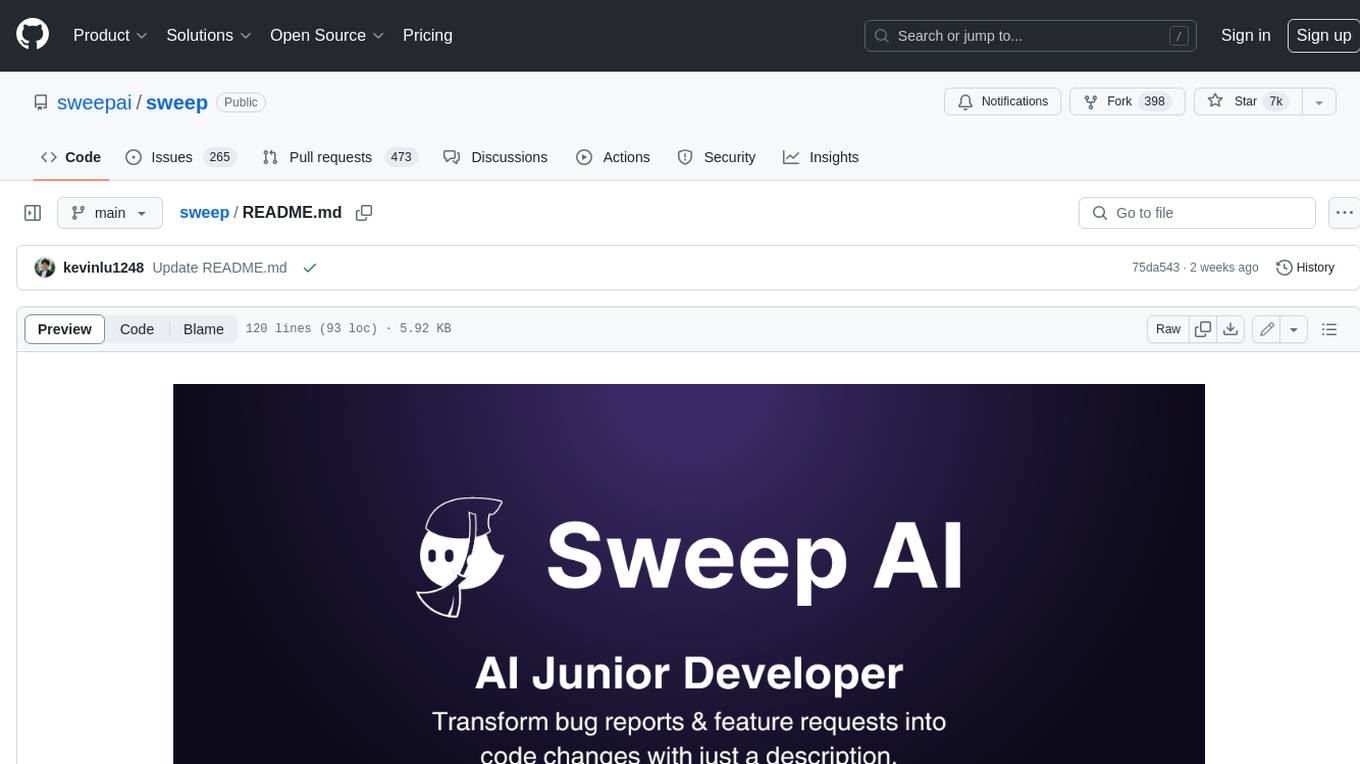
sweep
Sweep is an AI junior developer that turns bugs and feature requests into code changes. It automatically handles developer experience improvements like adding type hints and improving test coverage.
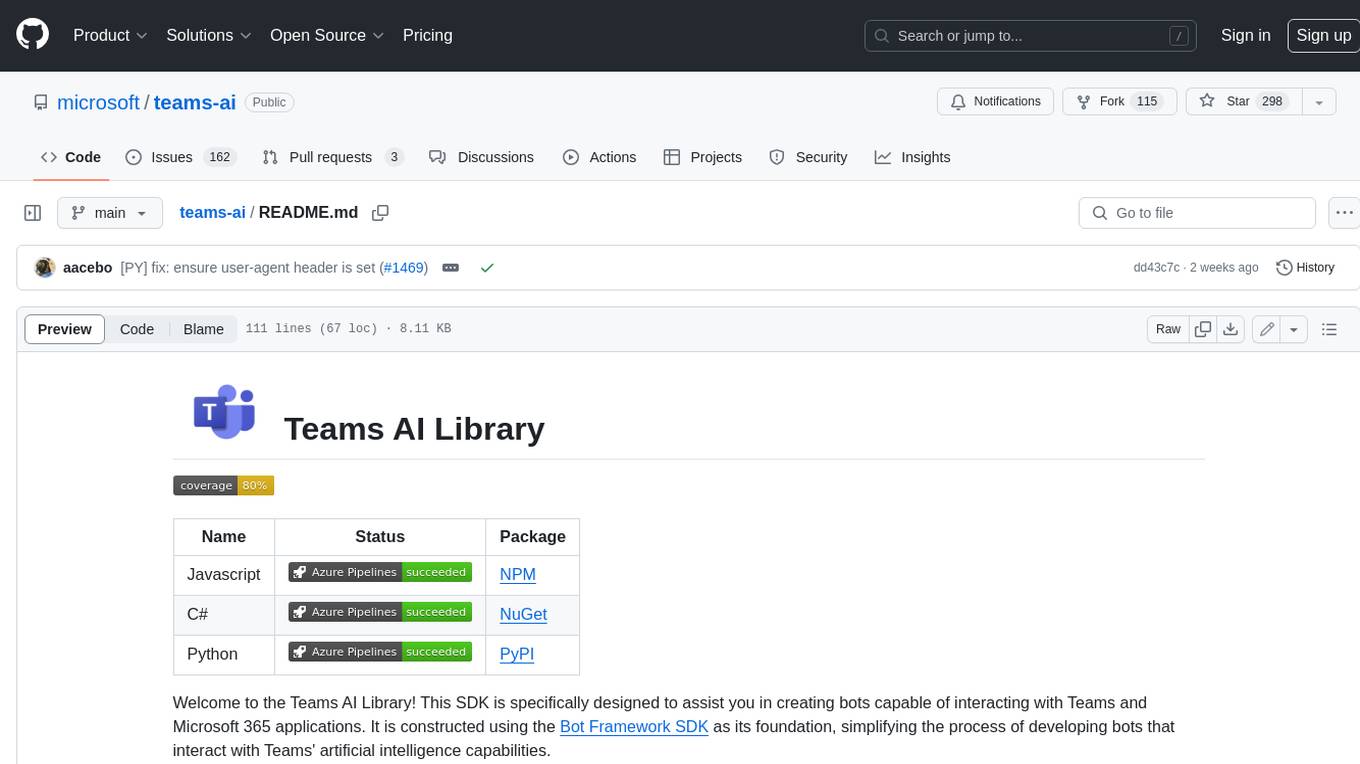
teams-ai
The Teams AI Library is a software development kit (SDK) that helps developers create bots that can interact with Teams and Microsoft 365 applications. It is built on top of the Bot Framework SDK and simplifies the process of developing bots that interact with Teams' artificial intelligence capabilities. The SDK is available for JavaScript/TypeScript, .NET, and Python.
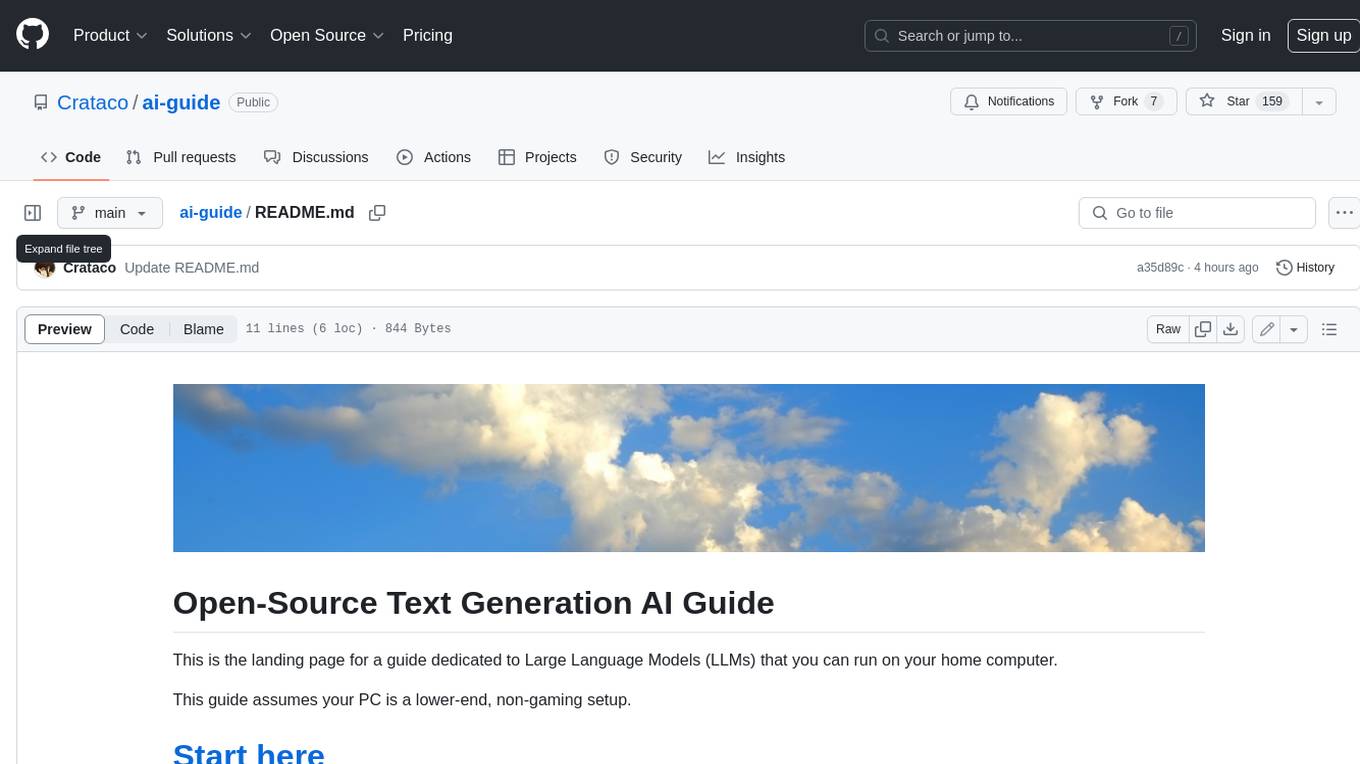
ai-guide
This guide is dedicated to Large Language Models (LLMs) that you can run on your home computer. It assumes your PC is a lower-end, non-gaming setup.
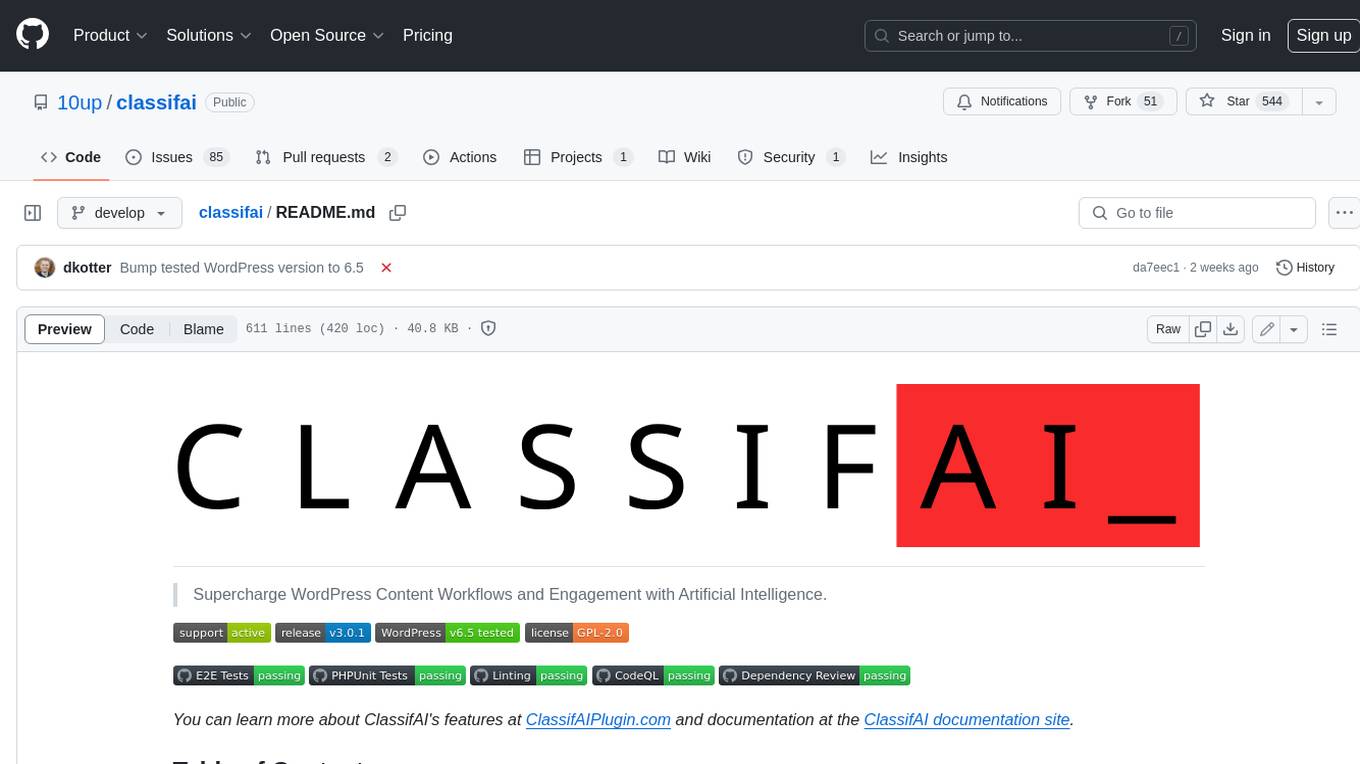
classifai
Supercharge WordPress Content Workflows and Engagement with Artificial Intelligence. Tap into leading cloud-based services like OpenAI, Microsoft Azure AI, Google Gemini and IBM Watson to augment your WordPress-powered websites. Publish content faster while improving SEO performance and increasing audience engagement. ClassifAI integrates Artificial Intelligence and Machine Learning technologies to lighten your workload and eliminate tedious tasks, giving you more time to create original content that matters.
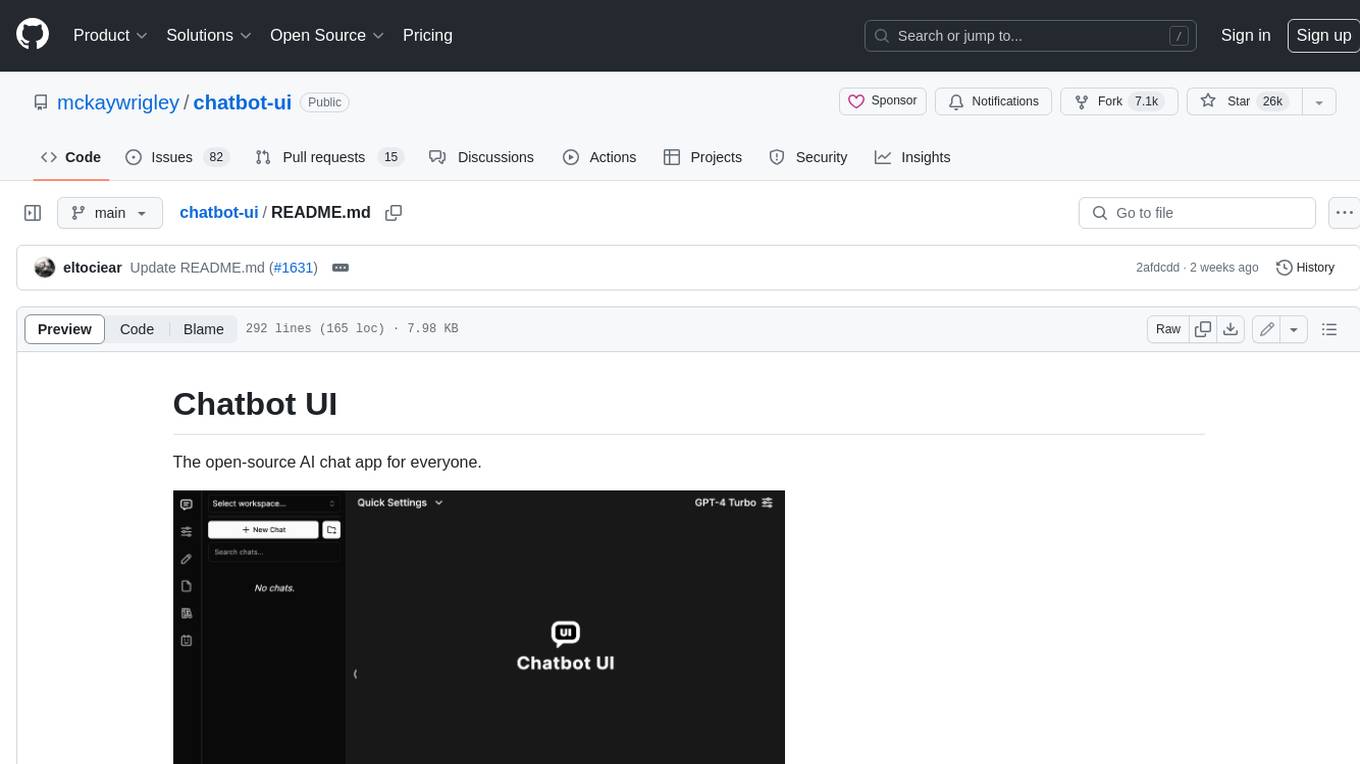
chatbot-ui
Chatbot UI is an open-source AI chat app that allows users to create and deploy their own AI chatbots. It is easy to use and can be customized to fit any need. Chatbot UI is perfect for businesses, developers, and anyone who wants to create a chatbot.
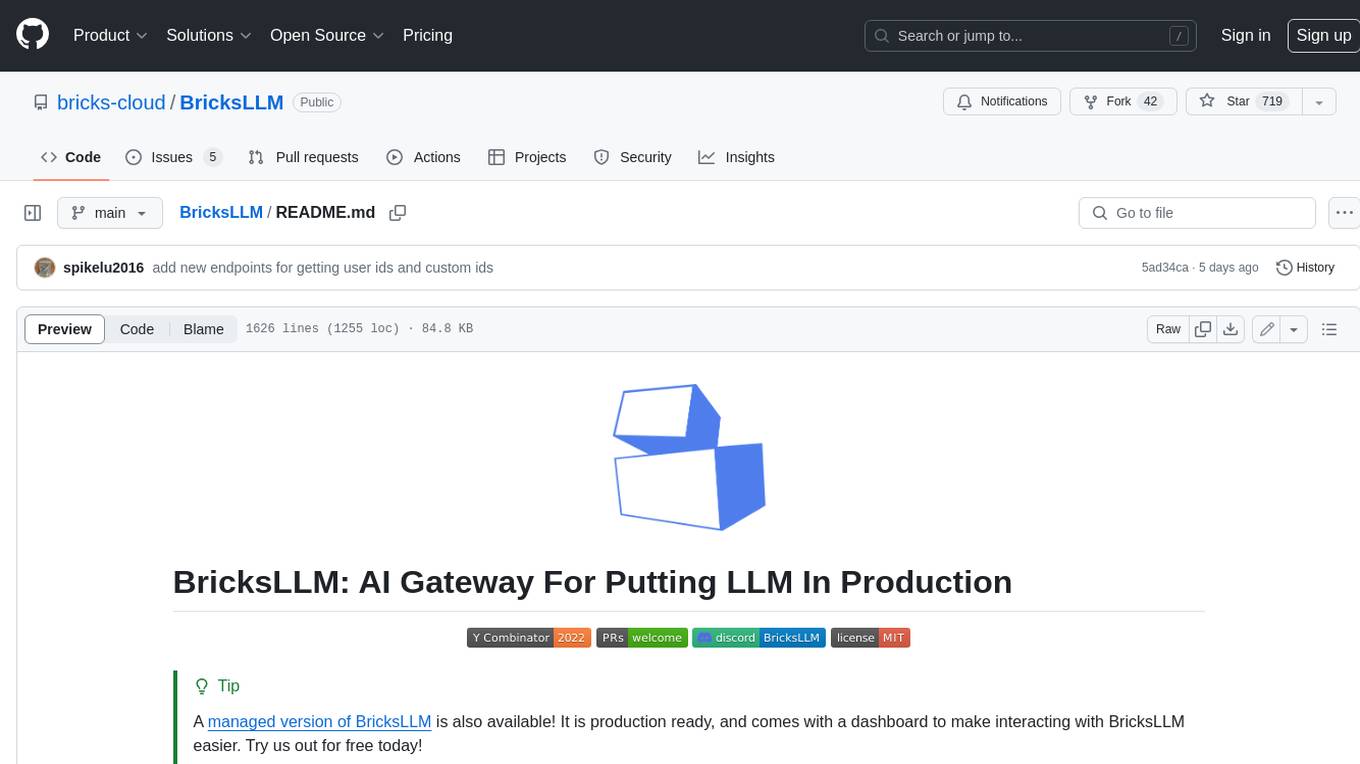
BricksLLM
BricksLLM is a cloud native AI gateway written in Go. Currently, it provides native support for OpenAI, Anthropic, Azure OpenAI and vLLM. BricksLLM aims to provide enterprise level infrastructure that can power any LLM production use cases. Here are some use cases for BricksLLM: * Set LLM usage limits for users on different pricing tiers * Track LLM usage on a per user and per organization basis * Block or redact requests containing PIIs * Improve LLM reliability with failovers, retries and caching * Distribute API keys with rate limits and cost limits for internal development/production use cases * Distribute API keys with rate limits and cost limits for students
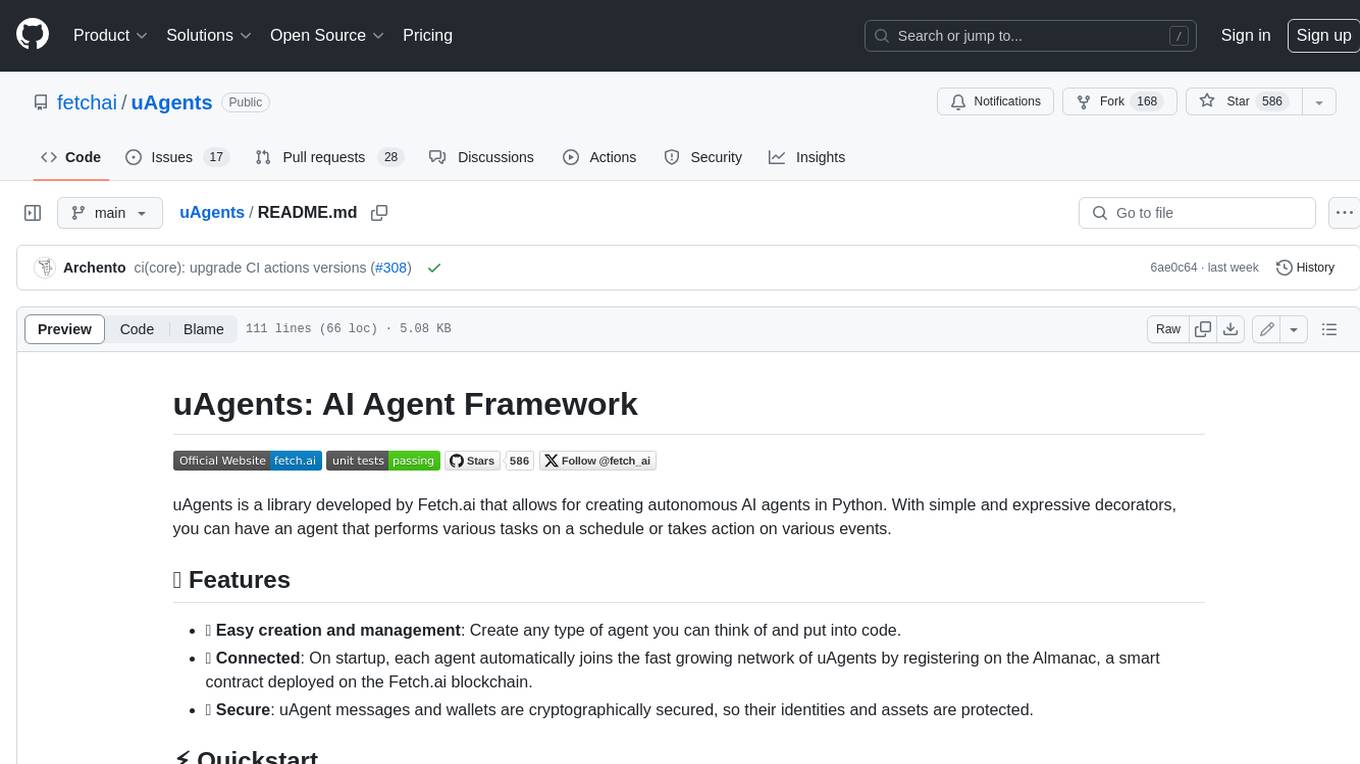
uAgents
uAgents is a Python library developed by Fetch.ai that allows for the creation of autonomous AI agents. These agents can perform various tasks on a schedule or take action on various events. uAgents are easy to create and manage, and they are connected to a fast-growing network of other uAgents. They are also secure, with cryptographically secured messages and wallets.
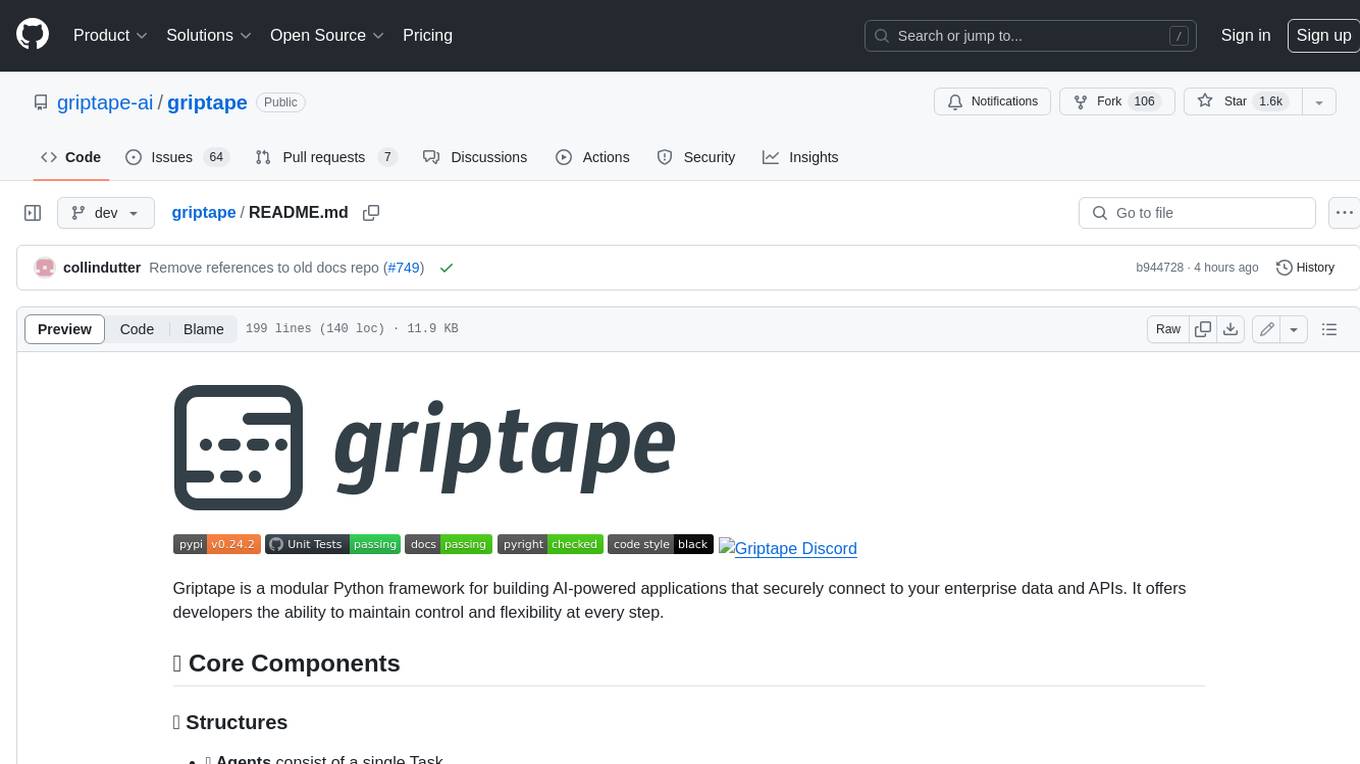
griptape
Griptape is a modular Python framework for building AI-powered applications that securely connect to your enterprise data and APIs. It offers developers the ability to maintain control and flexibility at every step. Griptape's core components include Structures (Agents, Pipelines, and Workflows), Tasks, Tools, Memory (Conversation Memory, Task Memory, and Meta Memory), Drivers (Prompt and Embedding Drivers, Vector Store Drivers, Image Generation Drivers, Image Query Drivers, SQL Drivers, Web Scraper Drivers, and Conversation Memory Drivers), Engines (Query Engines, Extraction Engines, Summary Engines, Image Generation Engines, and Image Query Engines), and additional components (Rulesets, Loaders, Artifacts, Chunkers, and Tokenizers). Griptape enables developers to create AI-powered applications with ease and efficiency.