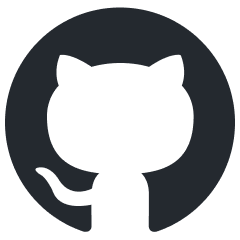
multi-agent-orchestrator
Flexible and powerful framework for managing multiple AI agents and handling complex conversations
Stars: 4568
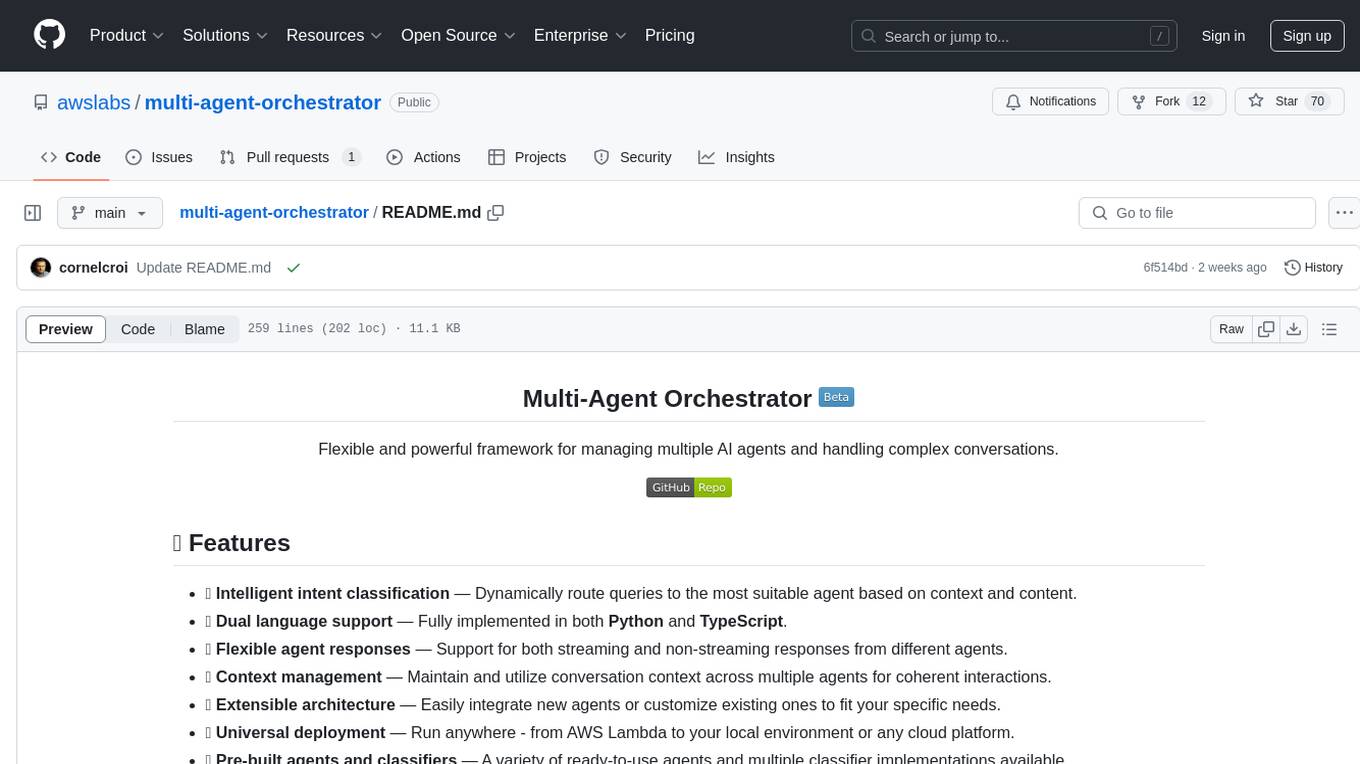
Multi-Agent Orchestrator is a flexible and powerful framework for managing multiple AI agents and handling complex conversations. It intelligently routes queries to the most suitable agent based on context and content, supports dual language implementation in Python and TypeScript, offers flexible agent responses, context management across agents, extensible architecture for customization, universal deployment options, and pre-built agents and classifiers. It is suitable for various applications, from simple chatbots to sophisticated AI systems, accommodating diverse requirements and scaling efficiently.
README:
Flexible, lightweight open-source framework for orchestrating multiple AI agents to handle complex conversations.
π Explore Full Documentation
- π§ Intelligent intent classification β Dynamically route queries to the most suitable agent based on context and content.
- π€ Dual language support β Fully implemented in both Python and TypeScript.
- π Flexible agent responses β Support for both streaming and non-streaming responses from different agents.
- π Context management β Maintain and utilize conversation context across multiple agents for coherent interactions.
- π§ Extensible architecture β Easily integrate new agents or customize existing ones to fit your specific needs.
- π Universal deployment β Run anywhere - from AWS Lambda to your local environment or any cloud platform.
- π¦ Pre-built agents and classifiers β A variety of ready-to-use agents and multiple classifier implementations available.
The Multi-Agent Orchestrator is a flexible framework for managing multiple AI agents and handling complex conversations. It intelligently routes queries and maintains context across interactions.
The system offers pre-built components for quick deployment, while also allowing easy integration of custom agents and conversation messages storage solutions.
This adaptability makes it suitable for a wide range of applications, from simple chatbots to sophisticated AI systems, accommodating diverse requirements and scaling efficiently.
π€ Looking for details on Amazon Bedrock's multi-agent collaboration capability announced during Matt Garman's keynote at re:Invent 2024?
π Visit the Amazon Bedrock Agents page to explore how multi-agent collaboration enables developers to build, deploy, and manage specialized agents designed for tackling complex workflows efficiently and accurately. β‘
- The process begins with user input, which is analyzed by a Classifier.
- The Classifier leverages both Agents' Characteristics and Agents' Conversation history to select the most appropriate agent for the task.
- Once an agent is selected, it processes the user input.
- The orchestrator then saves the conversation, updating the Agents' Conversation history, before delivering the response back to the user.
The Multi-Agent Orchestrator now includes a powerful new SupervisorAgent that enables sophisticated team coordination between multiple specialized agents. This new component implements a "agent-as-tools" architecture, allowing a lead agent to coordinate a team of specialized agents in parallel, maintaining context and delivering coherent responses.
Key capabilities:
- π€ Team Coordination - Coordonate multiple specialized agents working together on complex tasks
- β‘ Parallel Processing - Execute multiple agent queries simultaneously
- π§ Smart Context Management - Maintain conversation history across all team members
- π Dynamic Delegation - Intelligently distribute subtasks to appropriate team members
- π€ Agent Compatibility - Works with all agent types (Bedrock, Anthropic, Lex, etc.)
The SupervisorAgent can be used in two powerful ways:
- Direct Usage - Call it directly when you need dedicated team coordination for specific tasks
- Classifier Integration - Add it as an agent within the classifier to build complex hierarchical systems with multiple specialized teams
Here are just a few examples where this agent can be used:
- Customer Support Teams with specialized sub-teams
- AI Movie Production Studios
- Travel Planning Services
- Product Development Teams
- Healthcare Coordination Systems
Learn more about SupervisorAgent β
In the screen recording below, we demonstrate an extended version of the demo app that uses 6 specialized agents:
- Travel Agent: Powered by an Amazon Lex Bot
- Weather Agent: Utilizes a Bedrock LLM Agent with a tool to query the open-meteo API
- Restaurant Agent: Implemented as an Amazon Bedrock Agent
- Math Agent: Utilizes a Bedrock LLM Agent with two tools for executing mathematical operations
- Tech Agent: A Bedrock LLM Agent designed to answer questions on technical topics
- Health Agent: A Bedrock LLM Agent focused on addressing health-related queries
Watch as the system seamlessly switches context between diverse topics, from booking flights to checking weather, solving math problems, and providing health information. Notice how the appropriate agent is selected for each query, maintaining coherence even with brief follow-up inputs.
The demo highlights the system's ability to handle complex, multi-turn conversations while preserving context and leveraging specialized agents across various domains.
Get hands-on experience with the Multi-Agent Orchestrator through our diverse set of examples:
-
Demo Applications:
-
Streamlit Global Demo: A single Streamlit application showcasing multiple demos, including:
- AI Movie Production Studio
- AI Travel Planner
-
Chat Demo App:
- Explore multiple specialized agents handling various domains like travel, weather, math, and health
-
E-commerce Support Simulator: Experience AI-powered customer support with:
- Automated response generation for common queries
- Intelligent routing of complex issues to human support
- Real-time chat and email-style communication
- Human-in-the-loop interactions for complex cases
-
Streamlit Global Demo: A single Streamlit application showcasing multiple demos, including:
-
Sample Projects: Explore our example implementations in the
examples
folder:-
chat-demo-app
: Web-based chat interface with multiple specialized agents -
ecommerce-support-simulator
: AI-powered customer support system -
chat-chainlit-app
: Chat application built with Chainlit -
fast-api-streaming
: FastAPI implementation with streaming support -
text-2-structured-output
: Natural Language to Structured Data -
bedrock-inline-agents
: Bedrock Inline Agents sample -
bedrock-prompt-routing
: Bedrock Prompt Routing sample code
-
Examples are available in both Python and TypeScript. Check out our documentation for comprehensive guides on setting up and using the Multi-Agent Orchestrator framework!
Discover creative implementations and diverse applications of the Multi-Agent Orchestrator:
-
From 'Bonjour' to 'Boarding Pass': Multilingual AI Chatbot for Flight Reservations
This article demonstrates how to build a multilingual chatbot using the Multi-Agent Orchestrator framework. The article explains how to use an Amazon Lex bot as an agent, along with 2 other new agents to make it work in many languages with just a few lines of code.
-
Beyond Auto-Replies: Building an AI-Powered E-commerce Support system
This article demonstrates how to build an AI-driven multi-agent system for automated e-commerce customer email support. It covers the architecture and setup of specialized AI agents using the Multi-Agent Orchestrator framework, integrating automated processing with human-in-the-loop oversight. The guide explores email ingestion, intelligent routing, automated response generation, and human verification, providing a comprehensive approach to balancing AI efficiency with human expertise in customer support.
-
Speak Up, AI: Voicing Your Agents with Amazon Connect, Lex, and Bedrock
This article demonstrates how to build an AI customer call center. It covers the architecture and setup of specialized AI agents using the Multi-Agent Orchestrator framework interacting with voice via Amazon Connect and Amazon Lex.
-
Unlock Bedrock InvokeInlineAgent API's Hidden Potential
Learn how to scale Amazon Bedrock Agents beyond knowledge base limitations using the Multi-Agent Orchestrator framework and InvokeInlineAgent API. This article demonstrates dynamic agent creation and knowledge base selection for enterprise-scale AI applications.
-
Supercharging Amazon Bedrock Flows
Learn how to enhance Amazon Bedrock Flows with conversation memory and multi-flow orchestration using the Multi-Agent Orchestrator framework. This guide shows how to overcome Bedrock Flows' limitations to build more sophisticated AI workflows with persistent memory and intelligent routing between flows.
-
π«π· Podcast (French): L'orchestrateur multi-agents : Un orchestrateur open source pour vos agents IA
- Platforms:
-
π¬π§ Podcast (English): An Orchestrator for Your AI Agents
- Platforms:
npm install multi-agent-orchestrator
The following example demonstrates how to use the Multi-Agent Orchestrator with two different types of agents: a Bedrock LLM Agent with Converse API support and a Lex Bot Agent. This showcases the flexibility of the system in integrating various AI services.
import { MultiAgentOrchestrator, BedrockLLMAgent, LexBotAgent } from "multi-agent-orchestrator";
const orchestrator = new MultiAgentOrchestrator();
// Add a Bedrock LLM Agent with Converse API support
orchestrator.addAgent(
new BedrockLLMAgent({
name: "Tech Agent",
description:
"Specializes in technology areas including software development, hardware, AI, cybersecurity, blockchain, cloud computing, emerging tech innovations, and pricing/costs related to technology products and services.",
streaming: true
})
);
// Add a Lex Bot Agent for handling travel-related queries
orchestrator.addAgent(
new LexBotAgent({
name: "Travel Agent",
description: "Helps users book and manage their flight reservations",
botId: process.env.LEX_BOT_ID,
botAliasId: process.env.LEX_BOT_ALIAS_ID,
localeId: "en_US",
})
);
// Example usage
const response = await orchestrator.routeRequest(
"I want to book a flight",
'user123',
'session456'
);
// Handle the response (streaming or non-streaming)
if (response.streaming == true) {
console.log("\n** RESPONSE STREAMING ** \n");
// Send metadata immediately
console.log(`> Agent ID: ${response.metadata.agentId}`);
console.log(`> Agent Name: ${response.metadata.agentName}`);
console.log(`> User Input: ${response.metadata.userInput}`);
console.log(`> User ID: ${response.metadata.userId}`);
console.log(`> Session ID: ${response.metadata.sessionId}`);
console.log(
`> Additional Parameters:`,
response.metadata.additionalParams
);
console.log(`\n> Response: `);
// Stream the content
for await (const chunk of response.output) {
if (typeof chunk === "string") {
process.stdout.write(chunk);
} else {
console.error("Received unexpected chunk type:", typeof chunk);
}
}
} else {
// Handle non-streaming response (AgentProcessingResult)
console.log("\n** RESPONSE ** \n");
console.log(`> Agent ID: ${response.metadata.agentId}`);
console.log(`> Agent Name: ${response.metadata.agentName}`);
console.log(`> User Input: ${response.metadata.userInput}`);
console.log(`> User ID: ${response.metadata.userId}`);
console.log(`> Session ID: ${response.metadata.sessionId}`);
console.log(
`> Additional Parameters:`,
response.metadata.additionalParams
);
console.log(`\n> Response: ${response.output}`);
}
# Optional: Set up a virtual environment
python -m venv venv
source venv/bin/activate # On Windows use `venv\Scripts\activate`
pip install multi-agent-orchestrator[aws]
Here's an equivalent Python example demonstrating the use of the Multi-Agent Orchestrator with a Bedrock LLM Agent and a Lex Bot Agent:
import sys
import asyncio
from multi_agent_orchestrator.orchestrator import MultiAgentOrchestrator
from multi_agent_orchestrator.agents import BedrockLLMAgent, BedrockLLMAgentOptions, AgentStreamResponse
orchestrator = MultiAgentOrchestrator()
tech_agent = BedrockLLMAgent(BedrockLLMAgentOptions(
name="Tech Agent",
streaming=True,
description="Specializes in technology areas including software development, hardware, AI, \
cybersecurity, blockchain, cloud computing, emerging tech innovations, and pricing/costs \
related to technology products and services.",
model_id="anthropic.claude-3-sonnet-20240229-v1:0",
))
orchestrator.add_agent(tech_agent)
health_agent = BedrockLLMAgent(BedrockLLMAgentOptions(
name="Health Agent",
streaming=True,
description="Specializes in health and well being",
))
orchestrator.add_agent(health_agent)
async def main():
# Example usage
response = await orchestrator.route_request(
"What is AWS Lambda?",
'user123',
'session456',
{},
True
)
# Handle the response (streaming or non-streaming)
if response.streaming:
print("\n** RESPONSE STREAMING ** \n")
# Send metadata immediately
print(f"> Agent ID: {response.metadata.agent_id}")
print(f"> Agent Name: {response.metadata.agent_name}")
print(f"> User Input: {response.metadata.user_input}")
print(f"> User ID: {response.metadata.user_id}")
print(f"> Session ID: {response.metadata.session_id}")
print(f"> Additional Parameters: {response.metadata.additional_params}")
print("\n> Response: ")
# Stream the content
async for chunk in response.output:
async for chunk in response.output:
if isinstance(chunk, AgentStreamResponse):
print(chunk.text, end='', flush=True)
else:
print(f"Received unexpected chunk type: {type(chunk)}", file=sys.stderr)
else:
# Handle non-streaming response (AgentProcessingResult)
print("\n** RESPONSE ** \n")
print(f"> Agent ID: {response.metadata.agent_id}")
print(f"> Agent Name: {response.metadata.agent_name}")
print(f"> User Input: {response.metadata.user_input}")
print(f"> User ID: {response.metadata.user_id}")
print(f"> Session ID: {response.metadata.session_id}")
print(f"> Additional Parameters: {response.metadata.additional_params}")
print(f"\n> Response: {response.output.content}")
if __name__ == "__main__":
asyncio.run(main())
These examples showcase:
- The use of a Bedrock LLM Agent with Converse API support, allowing for multi-turn conversations.
- Integration of a Lex Bot Agent for specialized tasks (in this case, travel-related queries).
- The orchestrator's ability to route requests to the most appropriate agent based on the input.
- Handling of both streaming and non-streaming responses from different types of agents.
The Multi-Agent Orchestrator is designed with a modular architecture, allowing you to install only the components you need while ensuring you always get the core functionality.
1. AWS Integration:
pip install "multi-agent-orchestrator[aws]"
Includes core orchestration functionality with comprehensive AWS service integrations (BedrockLLMAgent
, AmazonBedrockAgent
, LambdaAgent
, etc.)
2. Anthropic Integration:
pip install "multi-agent-orchestrator[anthropic]"
3. OpenAI Integration:
pip install "multi-agent-orchestrator[openai]"
Adds OpenAI's GPT models for agents and classification, along with core packages.
4. Full Installation:
pip install "multi-agent-orchestrator[all]"
Includes all optional dependencies for maximum flexibility.
Have something to share, discuss, or brainstorm? Weβd love to connect with you and hear about your journey with the Multi-Agent Orchestrator framework. Hereβs how you can get involved:
-
π Show & Tell: Got a success story, cool project, or creative implementation? Share it with us in the Show and Tell section. Your work might inspire the entire community! π
-
π¬ General Discussion: Have questions, feedback, or suggestions? Join the conversation in our General Discussions section. Itβs the perfect place to connect with other users and contributors.
-
π‘ Ideas: Thinking of a new feature or improvement? Share your thoughts in the Ideas section. Weβre always open to exploring innovative ways to make the orchestrator even better!
Letβs collaborate, learn from each other, and build something incredible together! π
This repository follows an Issue-First policy:
- Every pull request must be linked to an existing issue
- If there isn't an issue for the changes you want to make, please create one first
- Use the issue to discuss proposed changes before investing time in implementation
When creating a pull request, you must link it to an issue using one of these methods:
-
Include a reference in the PR description using keywords:
Fixes #123
Resolves #123
Closes #123
-
Manually link the PR to an issue through GitHub's UI:
- On the right sidebar of your PR, click "Development" and then "Link an issue"
We use GitHub Actions to automatically verify that each PR is linked to an issue. PRs without linked issues will not pass required checks and cannot be merged.
This policy helps us:
- Maintain clear documentation of changes and their purposes
- Ensure community discussion before implementation
- Keep a structured development process
- Make project history more traceable and understandable
Once your proposal is approved, here are the next steps:
- π Review our Contributing Guide
- π‘ Create a GitHub Issue
- π¨ Submit a pull request
β Follow existing project structure and include documentation for new features.
π Stay Updated: Star the repository to be notified about new features, improvements, and exciting developments in the Multi-Agent Orchestrator framework!
Big shout out to our awesome contributors! Thank you for making this project better! π β π
Please see our contributing guide for guidelines on how to propose bugfixes and improvements.
This project is licensed under the Apache 2.0 licence - see the LICENSE file for details.
This project uses the JetBrainsMono NF font, licensed under the SIL Open Font License 1.1. For full license details, see FONT-LICENSE.md.
For Tasks:
Click tags to check more tools for each tasksFor Jobs:
Alternative AI tools for multi-agent-orchestrator
Similar Open Source Tools
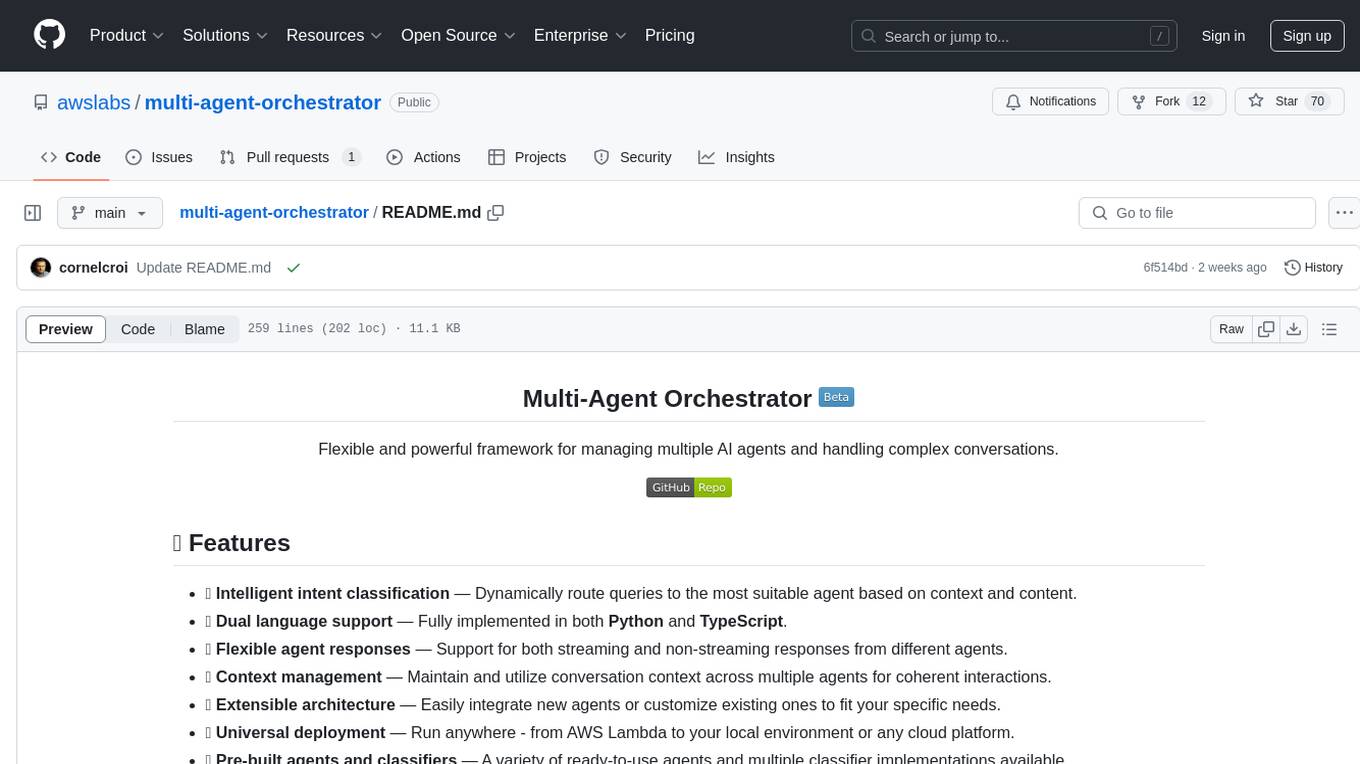
multi-agent-orchestrator
Multi-Agent Orchestrator is a flexible and powerful framework for managing multiple AI agents and handling complex conversations. It intelligently routes queries to the most suitable agent based on context and content, supports dual language implementation in Python and TypeScript, offers flexible agent responses, context management across agents, extensible architecture for customization, universal deployment options, and pre-built agents and classifiers. It is suitable for various applications, from simple chatbots to sophisticated AI systems, accommodating diverse requirements and scaling efficiently.
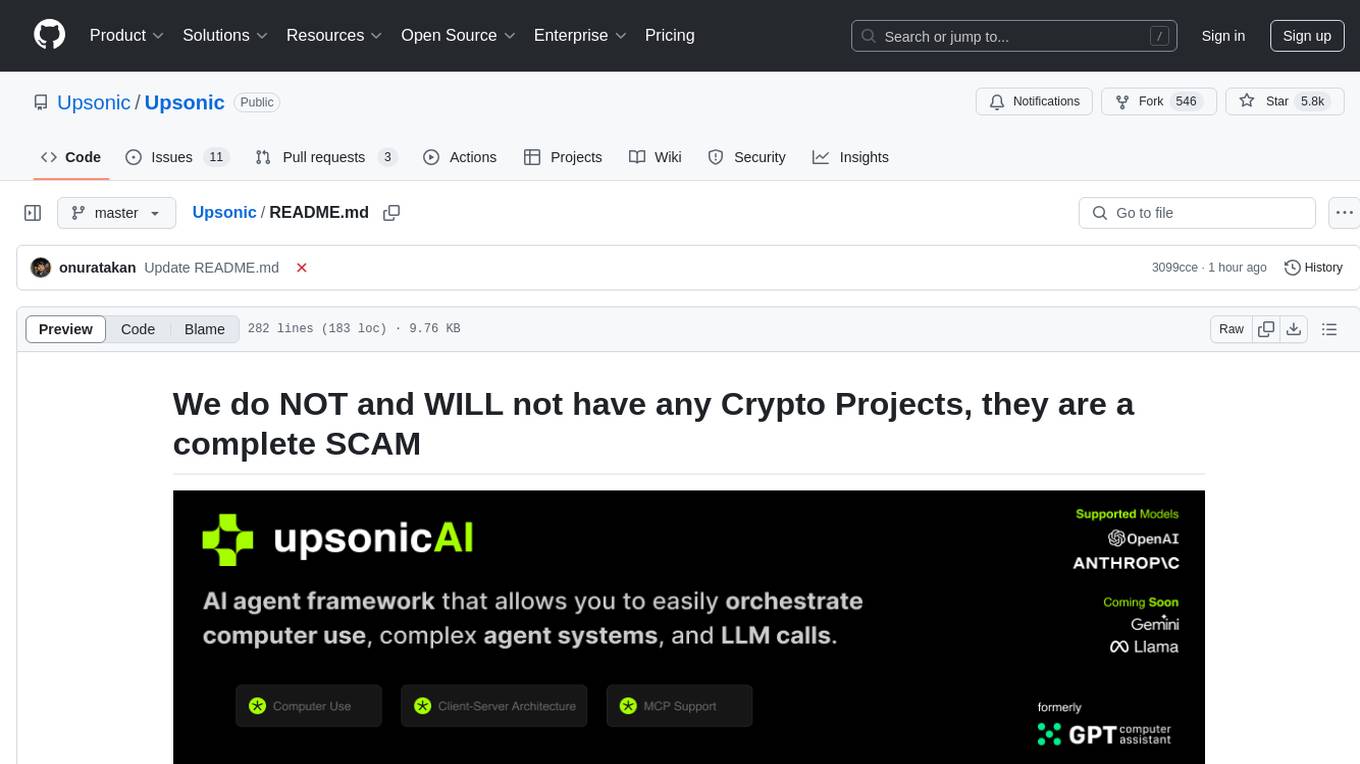
Upsonic
Upsonic offers a cutting-edge enterprise-ready framework for orchestrating LLM calls, agents, and computer use to complete tasks cost-effectively. It provides reliable systems, scalability, and a task-oriented structure for real-world cases. Key features include production-ready scalability, task-centric design, MCP server support, tool-calling server, computer use integration, and easy addition of custom tools. The framework supports client-server architecture and allows seamless deployment on AWS, GCP, or locally using Docker.
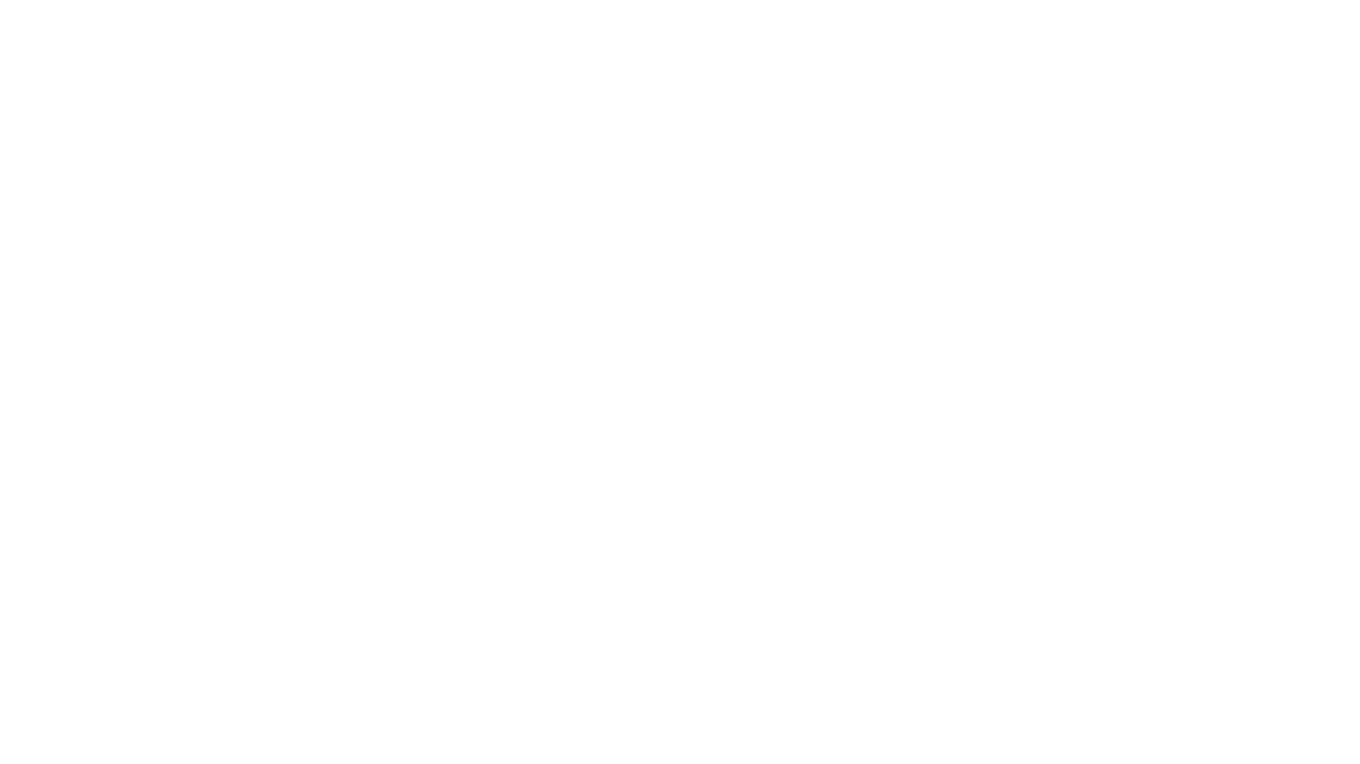
motia
Motia is an AI agent framework designed for software engineers to create, test, and deploy production-ready AI agents quickly. It provides a code-first approach, allowing developers to write agent logic in familiar languages and visualize execution in real-time. With Motia, developers can focus on business logic rather than infrastructure, offering zero infrastructure headaches, multi-language support, composable steps, built-in observability, instant APIs, and full control over AI logic. Ideal for building sophisticated agents and intelligent automations, Motia's event-driven architecture and modular steps enable the creation of GenAI-powered workflows, decision-making systems, and data processing pipelines.
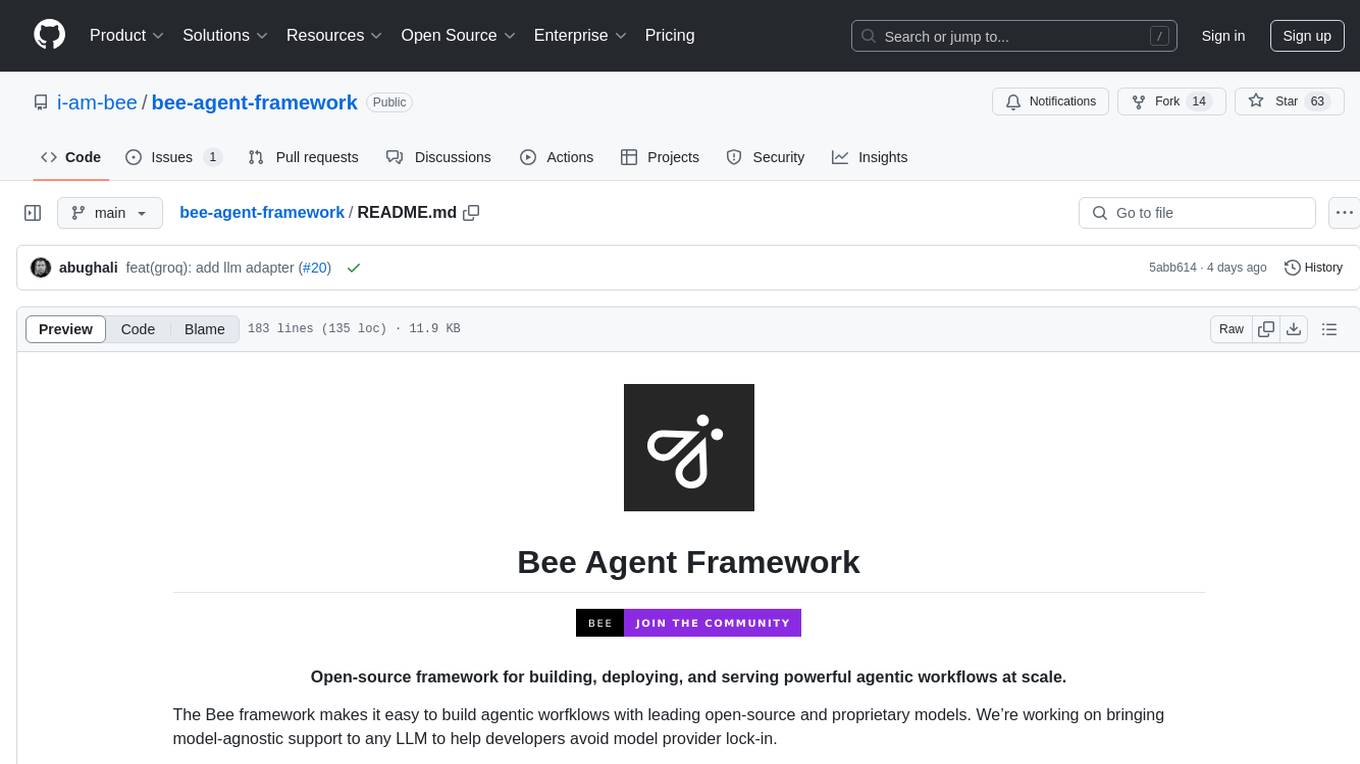
bee-agent-framework
The Bee Agent Framework is an open-source tool for building, deploying, and serving powerful agentic workflows at scale. It provides AI agents, tools for creating workflows in Javascript/Python, a code interpreter, memory optimization strategies, serialization for pausing/resuming workflows, traceability features, production-level control, and upcoming features like model-agnostic support and a chat UI. The framework offers various modules for agents, llms, memory, tools, caching, errors, adapters, logging, serialization, and more, with a roadmap including MLFlow integration, JSON support, structured outputs, chat client, base agent improvements, guardrails, and evaluation.
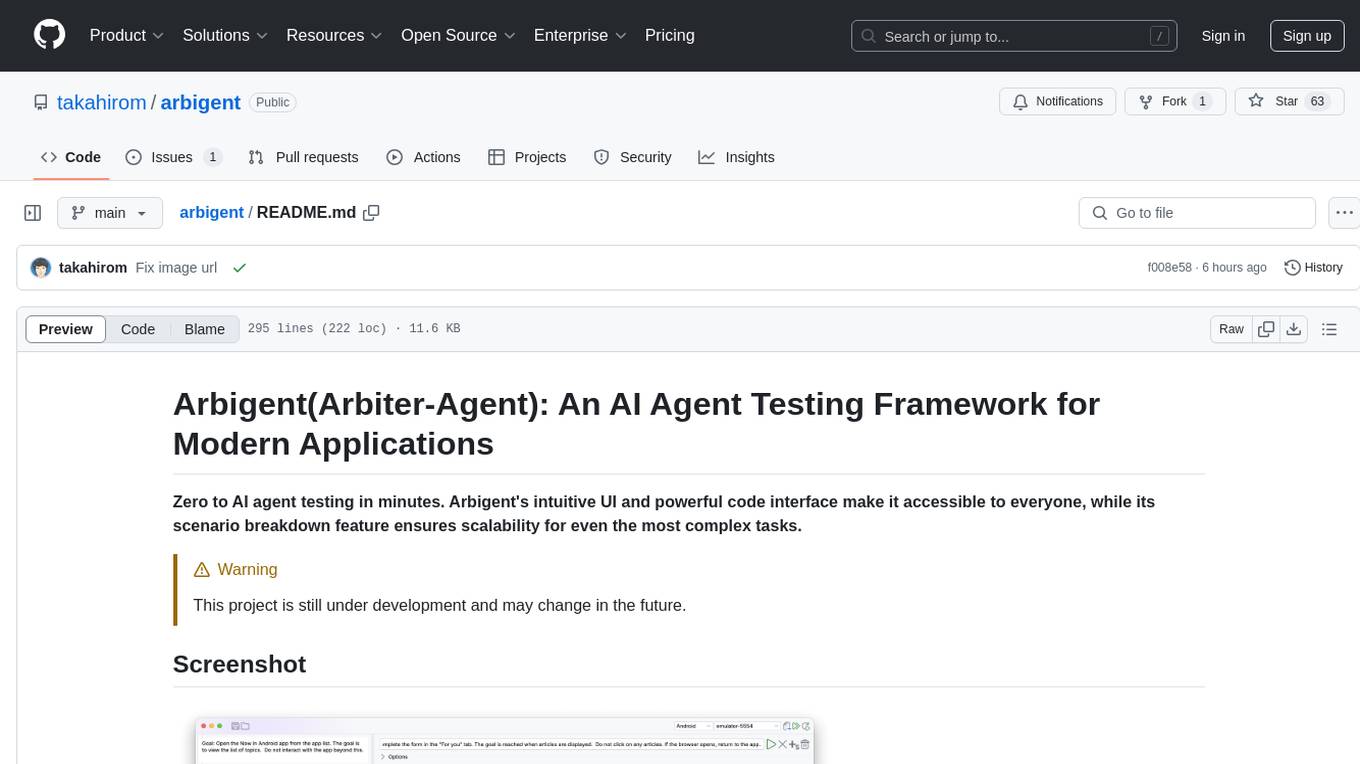
arbigent
Arbigent (Arbiter-Agent) is an AI agent testing framework designed to make AI agent testing practical for modern applications. It addresses challenges faced by traditional UI testing frameworks and AI agents by breaking down complex tasks into smaller, dependent scenarios. The framework is customizable for various AI providers, operating systems, and form factors, empowering users with extensive customization capabilities. Arbigent offers an intuitive UI for scenario creation and a powerful code interface for seamless test execution. It supports multiple form factors, optimizes UI for AI interaction, and is cost-effective by utilizing models like GPT-4o mini. With a flexible code interface and open-source nature, Arbigent aims to revolutionize AI agent testing in modern applications.
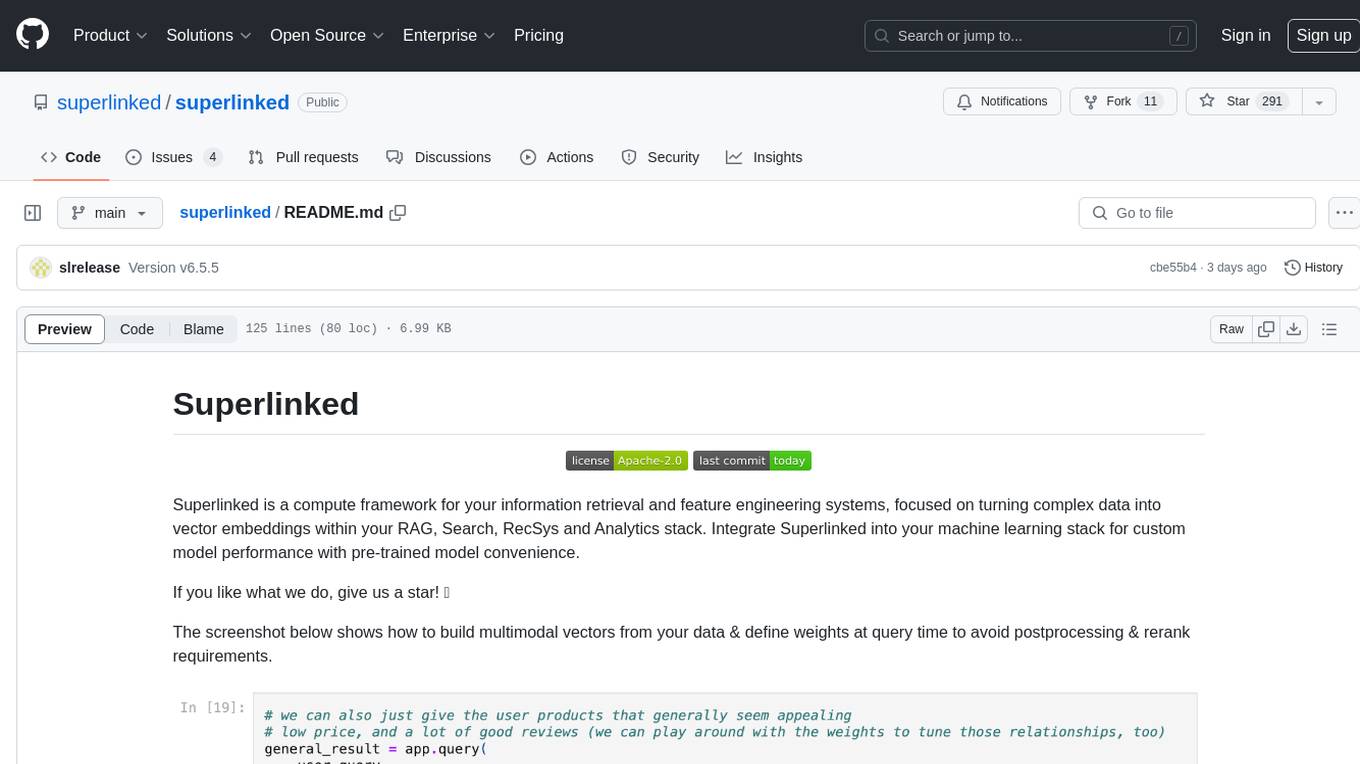
superlinked
Superlinked is a compute framework for information retrieval and feature engineering systems, focusing on converting complex data into vector embeddings for RAG, Search, RecSys, and Analytics stack integration. It enables custom model performance in machine learning with pre-trained model convenience. The tool allows users to build multimodal vectors, define weights at query time, and avoid postprocessing & rerank requirements. Users can explore the computational model through simple scripts and python notebooks, with a future release planned for production usage with built-in data infra and vector database integrations.
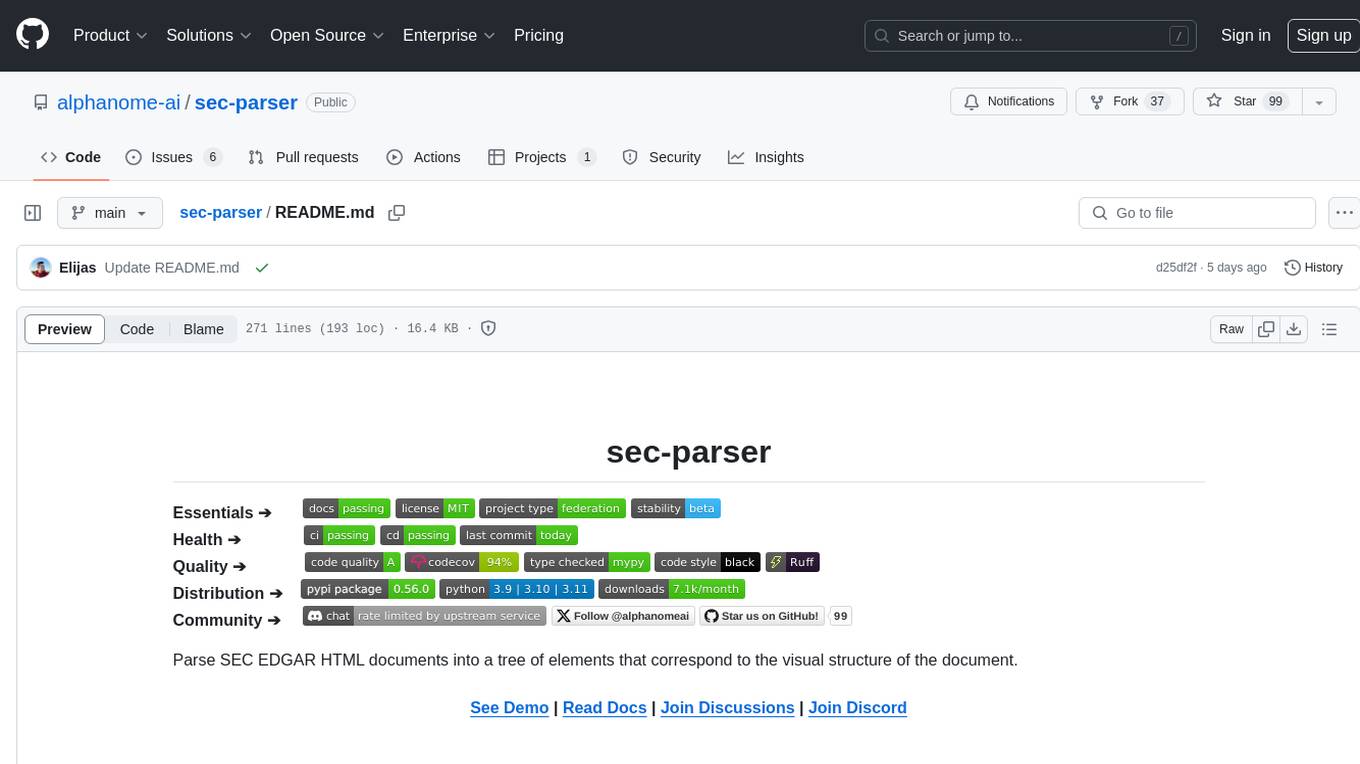
sec-parser
The `sec-parser` project simplifies extracting meaningful information from SEC EDGAR HTML documents by organizing them into semantic elements and a tree structure. It helps in parsing SEC filings for financial and regulatory analysis, analytics and data science, AI and machine learning, causal AI, and large language models. The tool is especially beneficial for AI, ML, and LLM applications by streamlining data pre-processing and feature extraction.
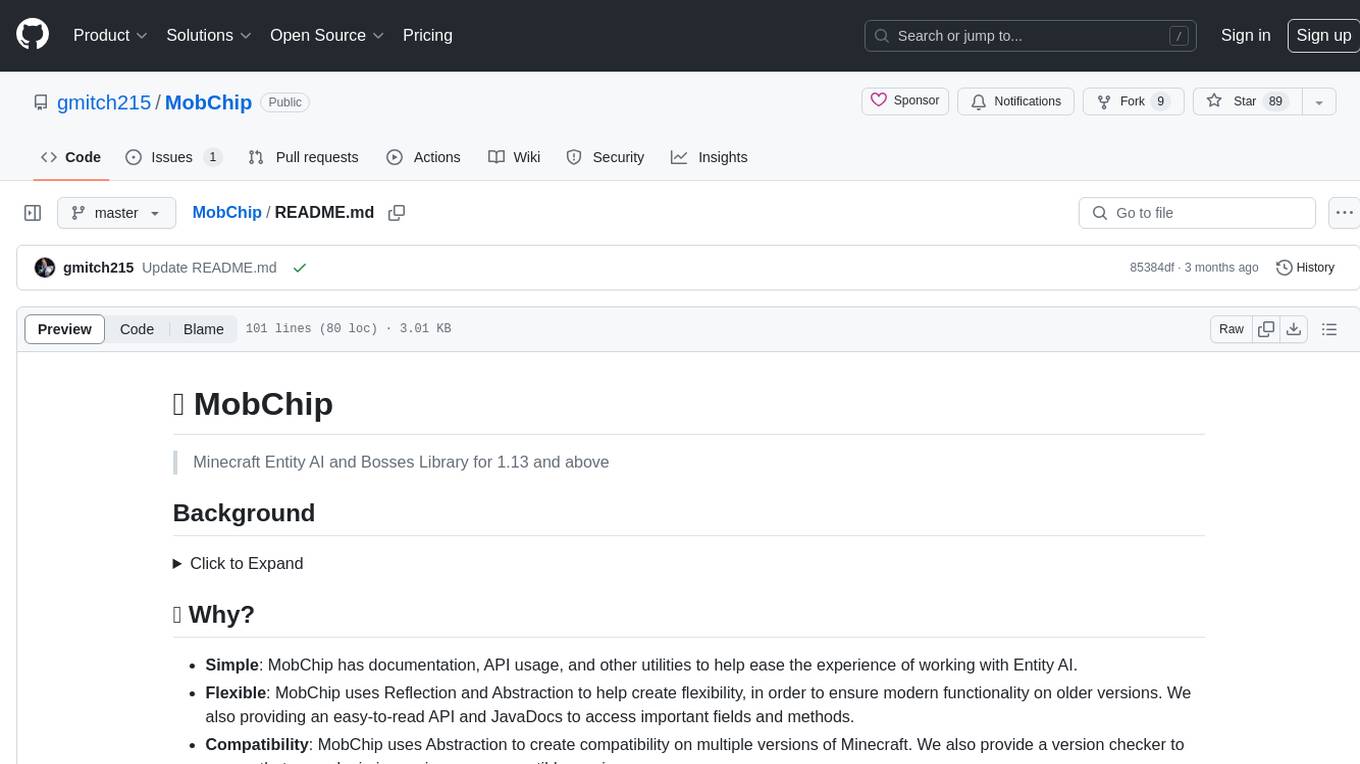
MobChip
MobChip is an all-in-one Entity AI and Bosses Library for Minecraft 1.13 and above. It simplifies the implementation of Minecraft's native entity AI into plugins, offering documentation, API usage, and utilities for ease of use. The library is flexible, using Reflection and Abstraction for modern functionality on older versions, and ensuring compatibility across multiple Minecraft versions. MobChip is open source, providing features like Bosses Library, Pathfinder Goals, Behaviors, Villager Gossip, Ender Dragon Phases, and more.
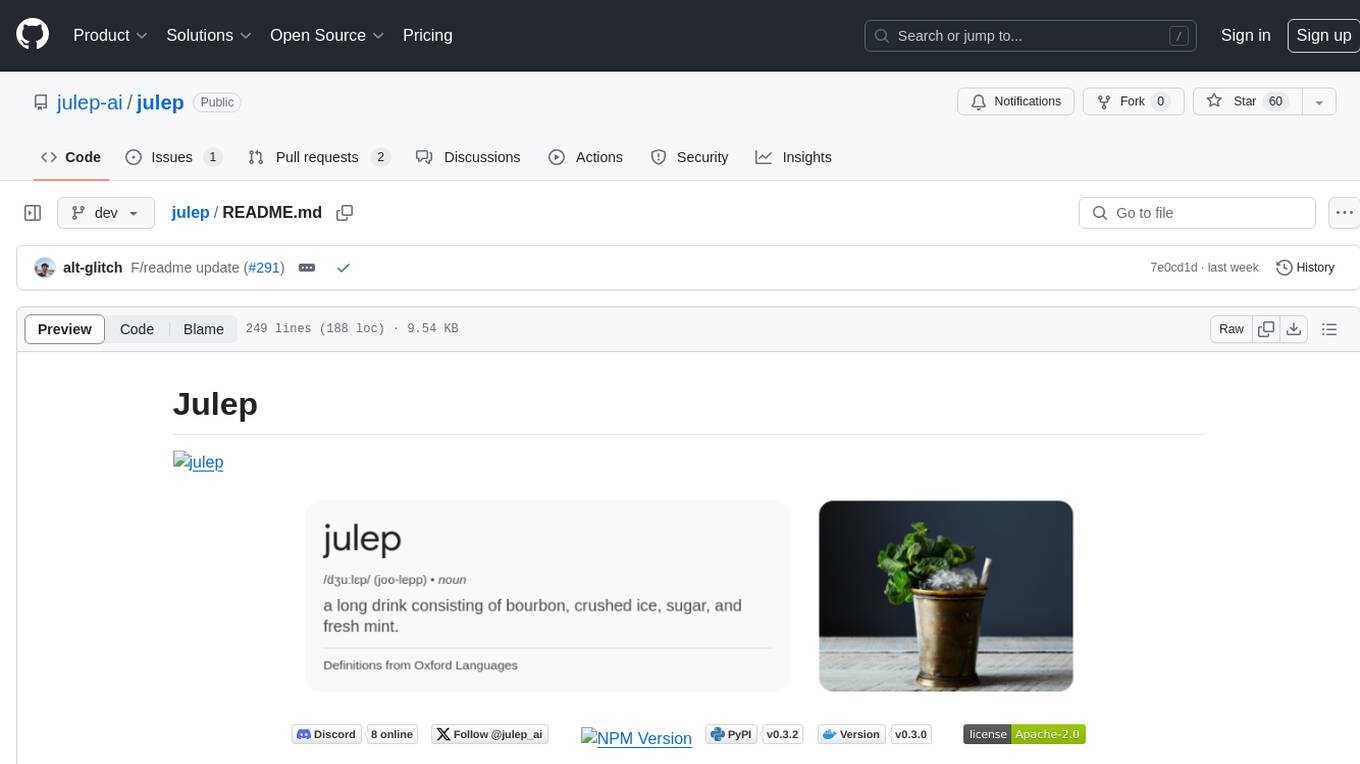
julep
Julep is an advanced platform for creating stateful and functional AI apps powered by large language models. It offers features like statefulness by design, automatic function calling, production-ready deployment, cron-like asynchronous functions, 90+ built-in tools, and the ability to switch between different LLMs easily. Users can build AI applications without the need to write code for embedding, saving, and retrieving conversation history, and can connect to third-party applications using Composio. Julep simplifies the process of getting started with AI apps, whether they are conversational, functional, or agentic.
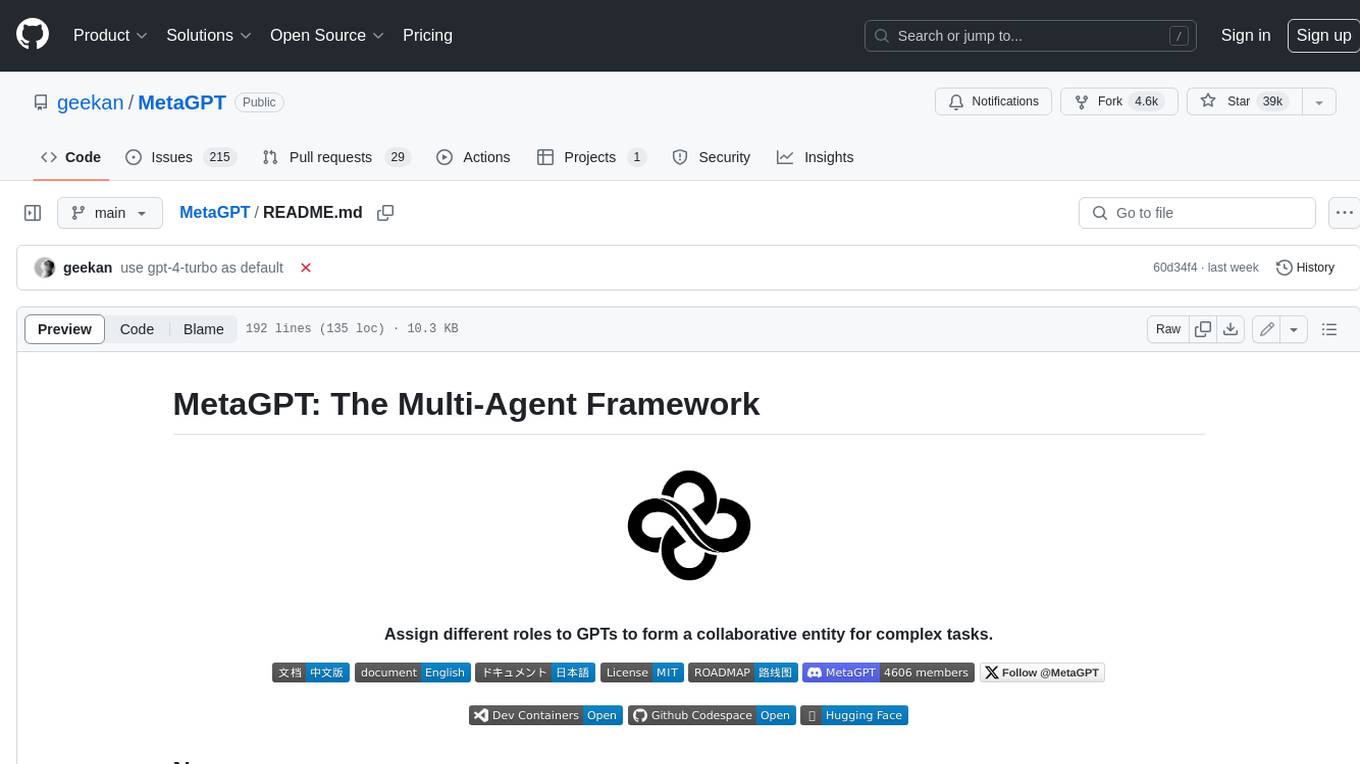
MetaGPT
MetaGPT is a multi-agent framework that enables GPT to work in a software company, collaborating to tackle more complex tasks. It assigns different roles to GPTs to form a collaborative entity for complex tasks. MetaGPT takes a one-line requirement as input and outputs user stories, competitive analysis, requirements, data structures, APIs, documents, etc. Internally, MetaGPT includes product managers, architects, project managers, and engineers. It provides the entire process of a software company along with carefully orchestrated SOPs. MetaGPT's core philosophy is "Code = SOP(Team)", materializing SOP and applying it to teams composed of LLMs.
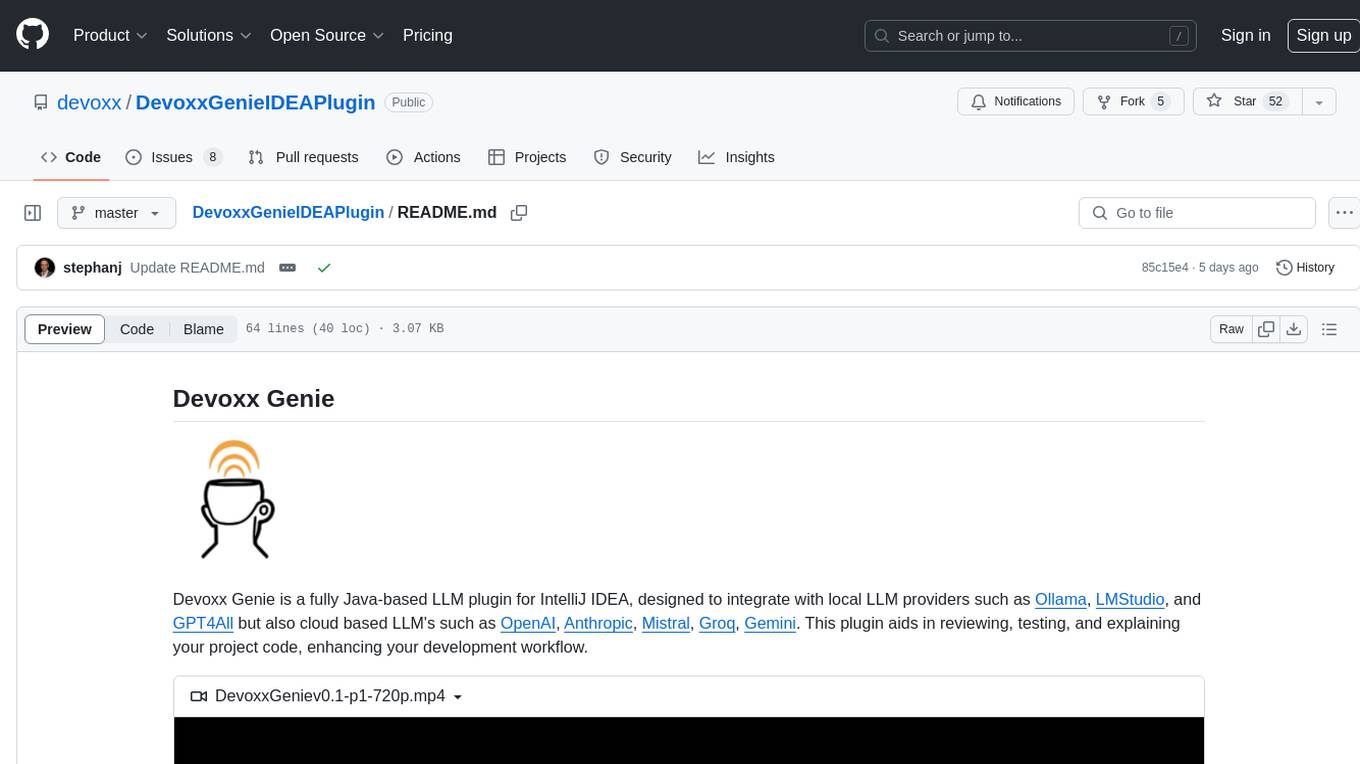
DevoxxGenieIDEAPlugin
Devoxx Genie is a Java-based IntelliJ IDEA plugin that integrates with local and cloud-based LLM providers to aid in reviewing, testing, and explaining project code. It supports features like code highlighting, chat conversations, and adding files/code snippets to context. Users can modify REST endpoints and LLM parameters in settings, including support for cloud-based LLMs. The plugin requires IntelliJ version 2023.3.4 and JDK 17. Building and publishing the plugin is done using Gradle tasks. Users can select an LLM provider, choose code, and use commands like review, explain, or generate unit tests for code analysis.
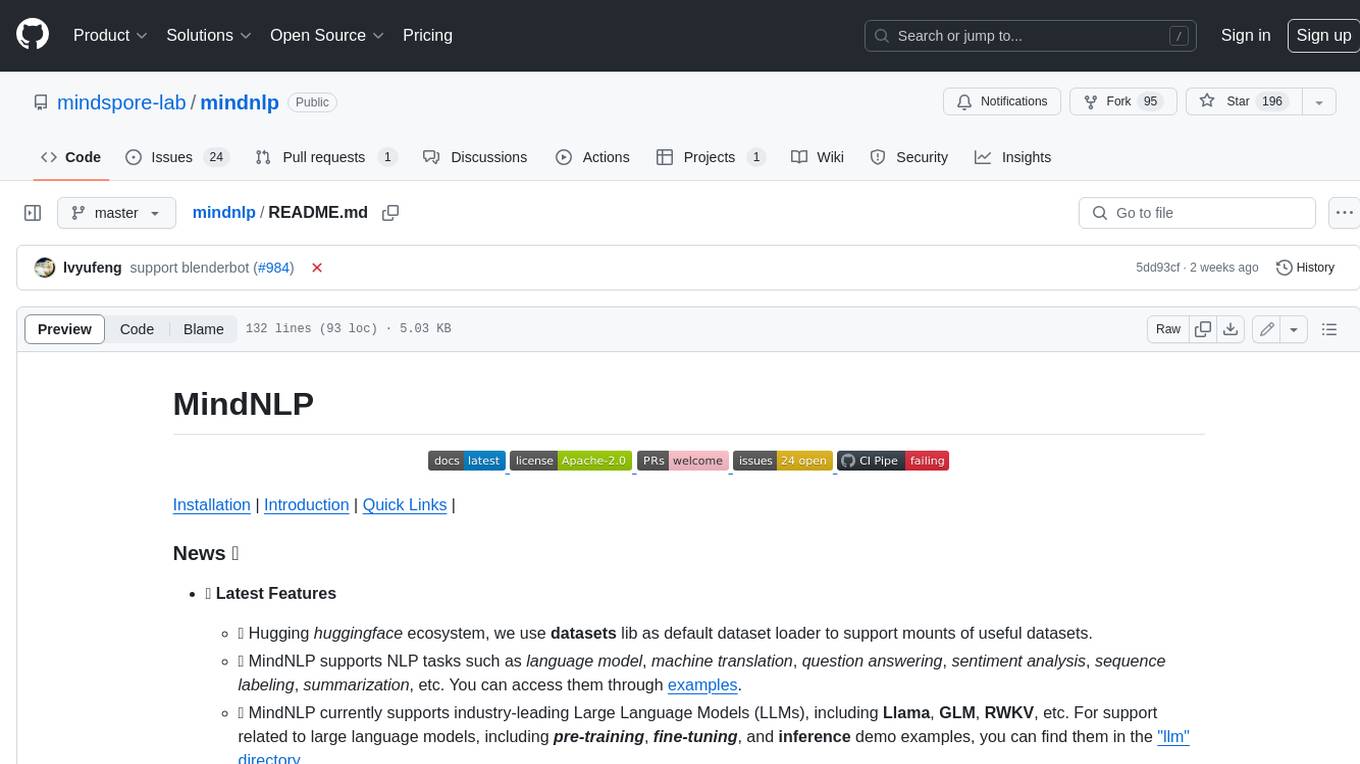
mindnlp
MindNLP is an open-source NLP library based on MindSpore. It provides a platform for solving natural language processing tasks, containing many common approaches in NLP. It can help researchers and developers to construct and train models more conveniently and rapidly. Key features of MindNLP include: * Comprehensive data processing: Several classical NLP datasets are packaged into a friendly module for easy use, such as Multi30k, SQuAD, CoNLL, etc. * Friendly NLP model toolset: MindNLP provides various configurable components. It is friendly to customize models using MindNLP. * Easy-to-use engine: MindNLP simplified complicated training process in MindSpore. It supports Trainer and Evaluator interfaces to train and evaluate models easily. MindNLP supports a wide range of NLP tasks, including: * Language modeling * Machine translation * Question answering * Sentiment analysis * Sequence labeling * Summarization MindNLP also supports industry-leading Large Language Models (LLMs), including Llama, GLM, RWKV, etc. For support related to large language models, including pre-training, fine-tuning, and inference demo examples, you can find them in the "llm" directory. To install MindNLP, you can either install it from Pypi, download the daily build wheel, or install it from source. The installation instructions are provided in the documentation. MindNLP is released under the Apache 2.0 license. If you find this project useful in your research, please consider citing the following paper: @misc{mindnlp2022, title={{MindNLP}: a MindSpore NLP library}, author={MindNLP Contributors}, howpublished = {\url{https://github.com/mindlab-ai/mindnlp}}, year={2022} }
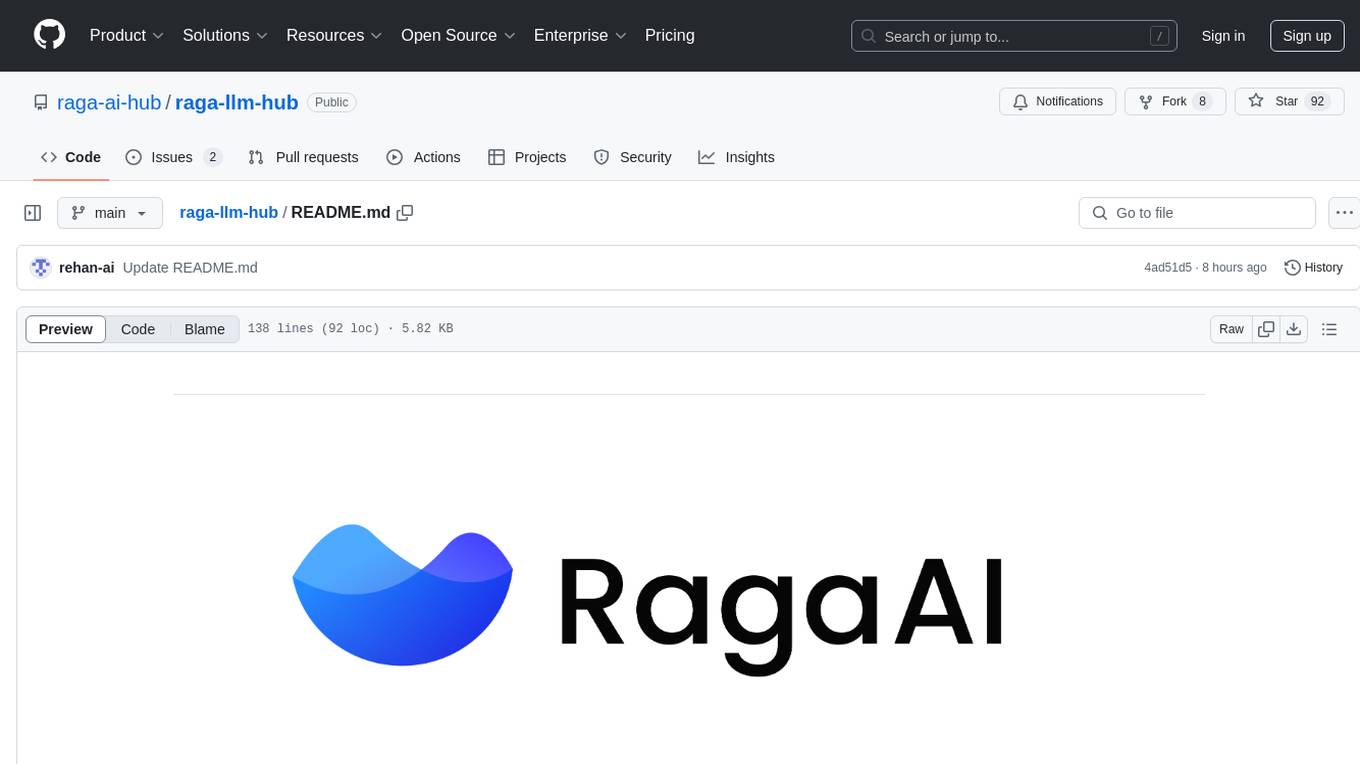
raga-llm-hub
Raga LLM Hub is a comprehensive evaluation toolkit for Language and Learning Models (LLMs) with over 100 meticulously designed metrics. It allows developers and organizations to evaluate and compare LLMs effectively, establishing guardrails for LLMs and Retrieval Augmented Generation (RAG) applications. The platform assesses aspects like Relevance & Understanding, Content Quality, Hallucination, Safety & Bias, Context Relevance, Guardrails, and Vulnerability scanning, along with Metric-Based Tests for quantitative analysis. It helps teams identify and fix issues throughout the LLM lifecycle, revolutionizing reliability and trustworthiness.
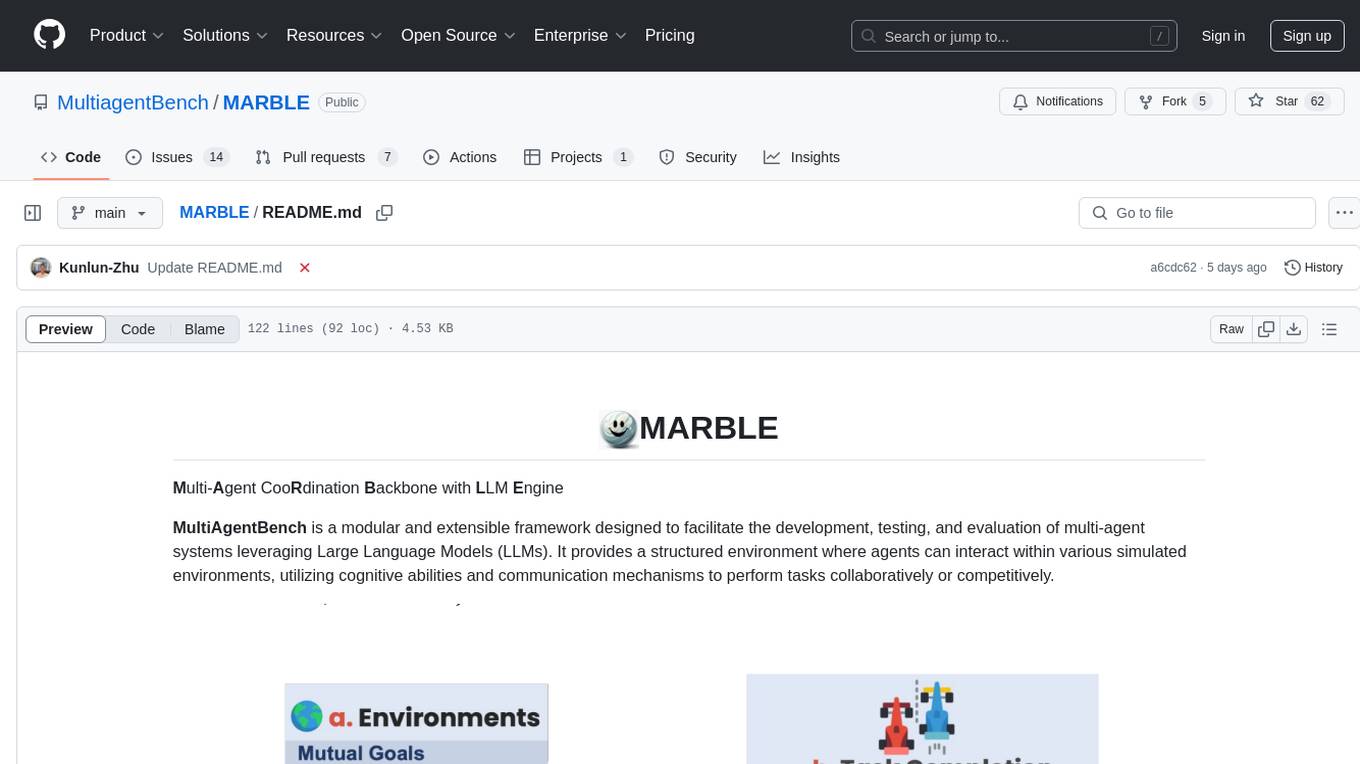
MARBLE
MARBLE (Multi-Agent Coordination Backbone with LLM Engine) is a modular framework for developing, testing, and evaluating multi-agent systems leveraging Large Language Models. It provides a structured environment for agents to interact in simulated environments, utilizing cognitive abilities and communication mechanisms for collaborative or competitive tasks. The framework features modular design, multi-agent support, LLM integration, shared memory, flexible environments, metrics and evaluation, industrial coding standards, and Docker support.
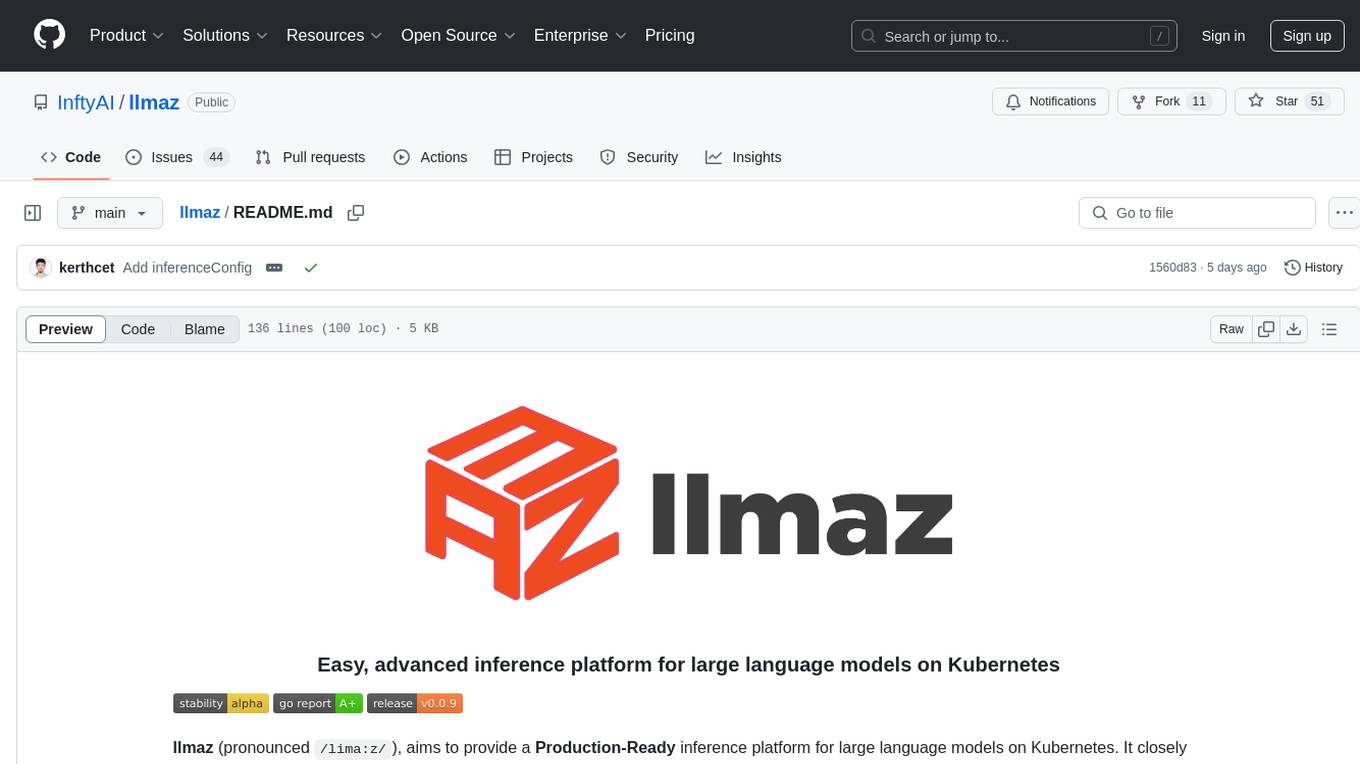
llmaz
llmaz is an easy, advanced inference platform for large language models on Kubernetes. It aims to provide a production-ready solution that integrates with state-of-the-art inference backends. The platform supports efficient model distribution, accelerator fungibility, SOTA inference, various model providers, multi-host support, and scaling efficiency. Users can quickly deploy LLM services with minimal configurations and benefit from a wide range of advanced inference backends. llmaz is designed to optimize cost and performance while supporting cutting-edge researches like Speculative Decoding or Splitwise on Kubernetes.
For similar tasks
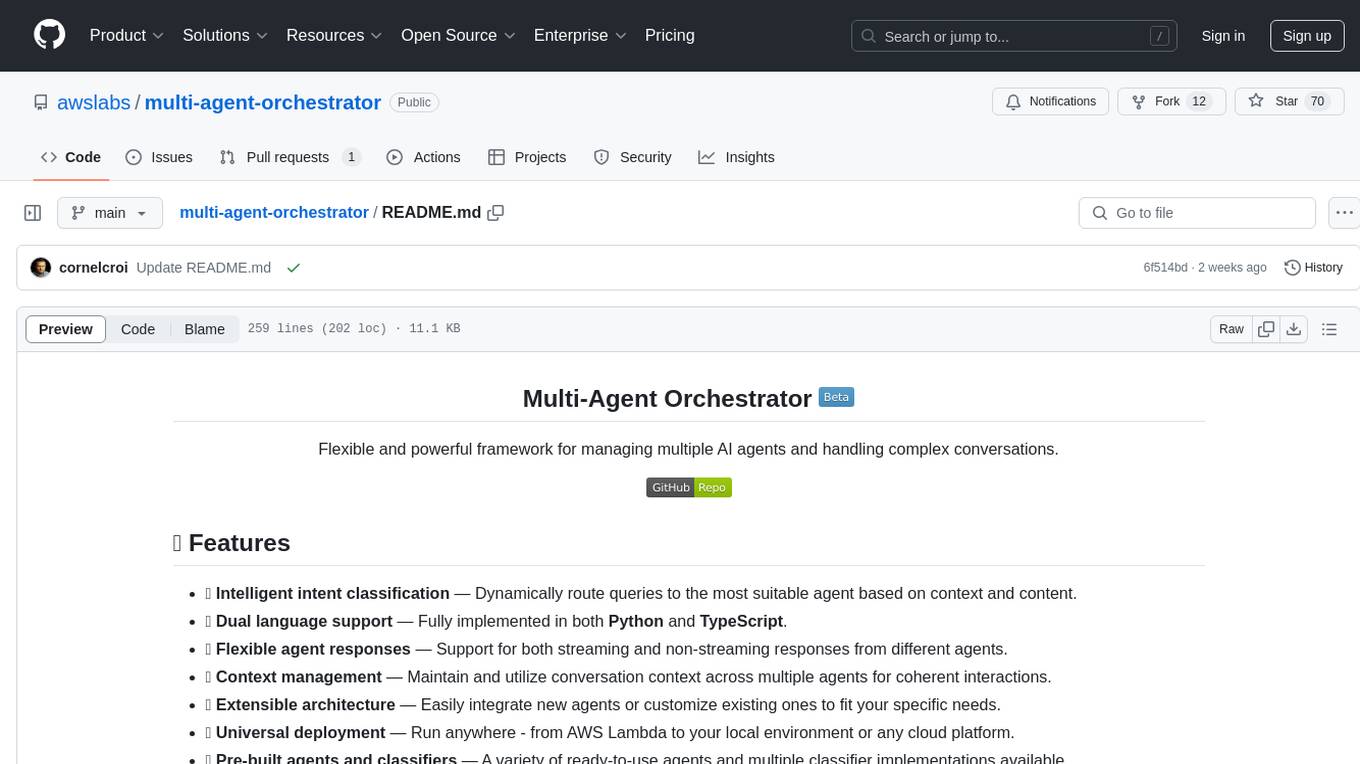
multi-agent-orchestrator
Multi-Agent Orchestrator is a flexible and powerful framework for managing multiple AI agents and handling complex conversations. It intelligently routes queries to the most suitable agent based on context and content, supports dual language implementation in Python and TypeScript, offers flexible agent responses, context management across agents, extensible architecture for customization, universal deployment options, and pre-built agents and classifiers. It is suitable for various applications, from simple chatbots to sophisticated AI systems, accommodating diverse requirements and scaling efficiently.
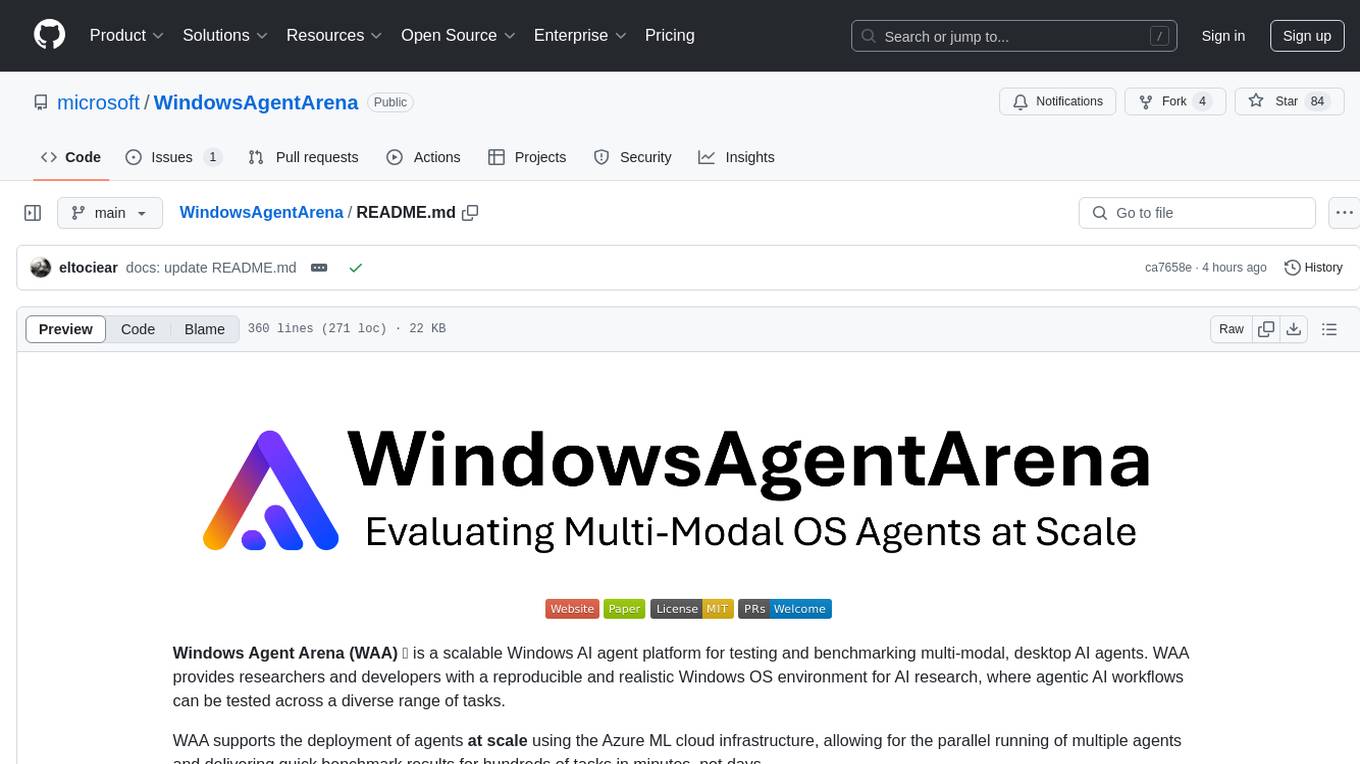
WindowsAgentArena
Windows Agent Arena (WAA) is a scalable Windows AI agent platform designed for testing and benchmarking multi-modal, desktop AI agents. It provides researchers and developers with a reproducible and realistic Windows OS environment for AI research, enabling testing of agentic AI workflows across various tasks. WAA supports deploying agents at scale using Azure ML cloud infrastructure, allowing parallel running of multiple agents and delivering quick benchmark results for hundreds of tasks in minutes.
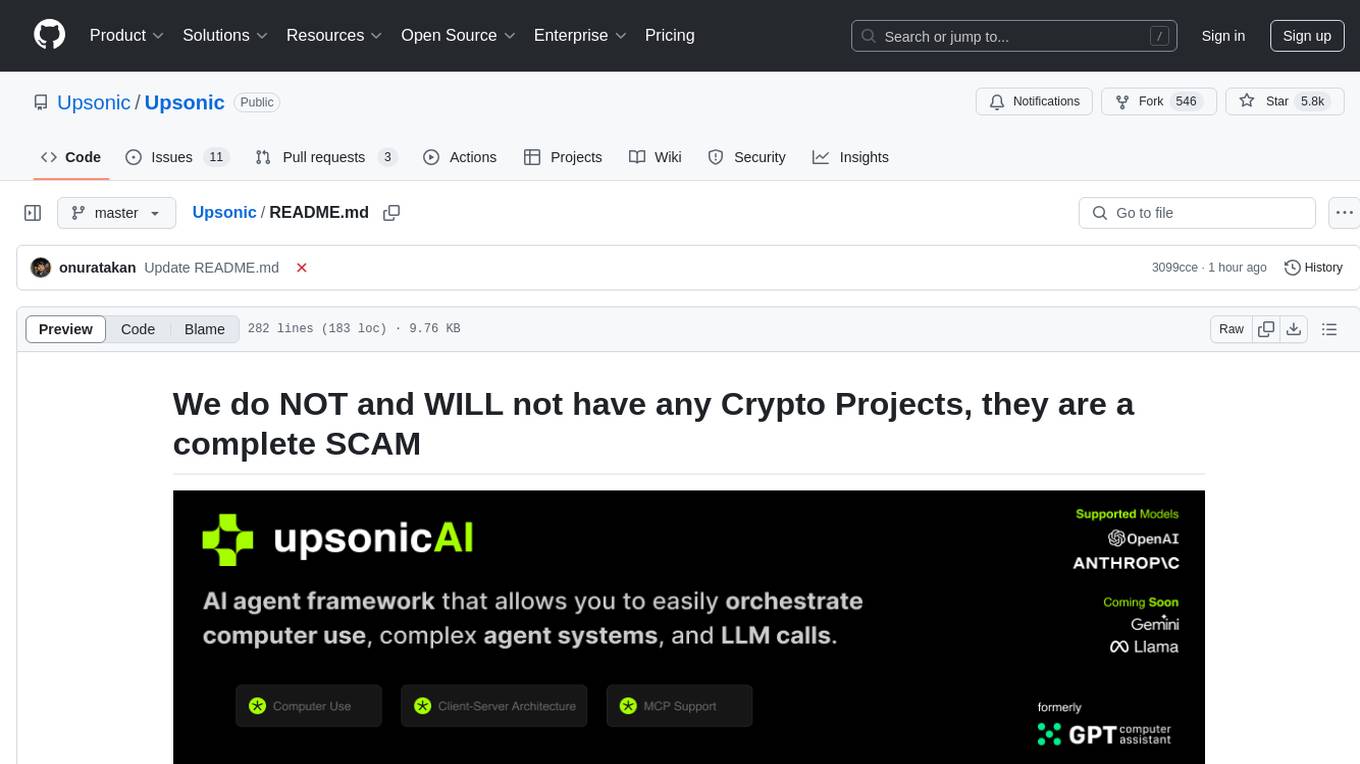
Upsonic
Upsonic offers a cutting-edge enterprise-ready framework for orchestrating LLM calls, agents, and computer use to complete tasks cost-effectively. It provides reliable systems, scalability, and a task-oriented structure for real-world cases. Key features include production-ready scalability, task-centric design, MCP server support, tool-calling server, computer use integration, and easy addition of custom tools. The framework supports client-server architecture and allows seamless deployment on AWS, GCP, or locally using Docker.
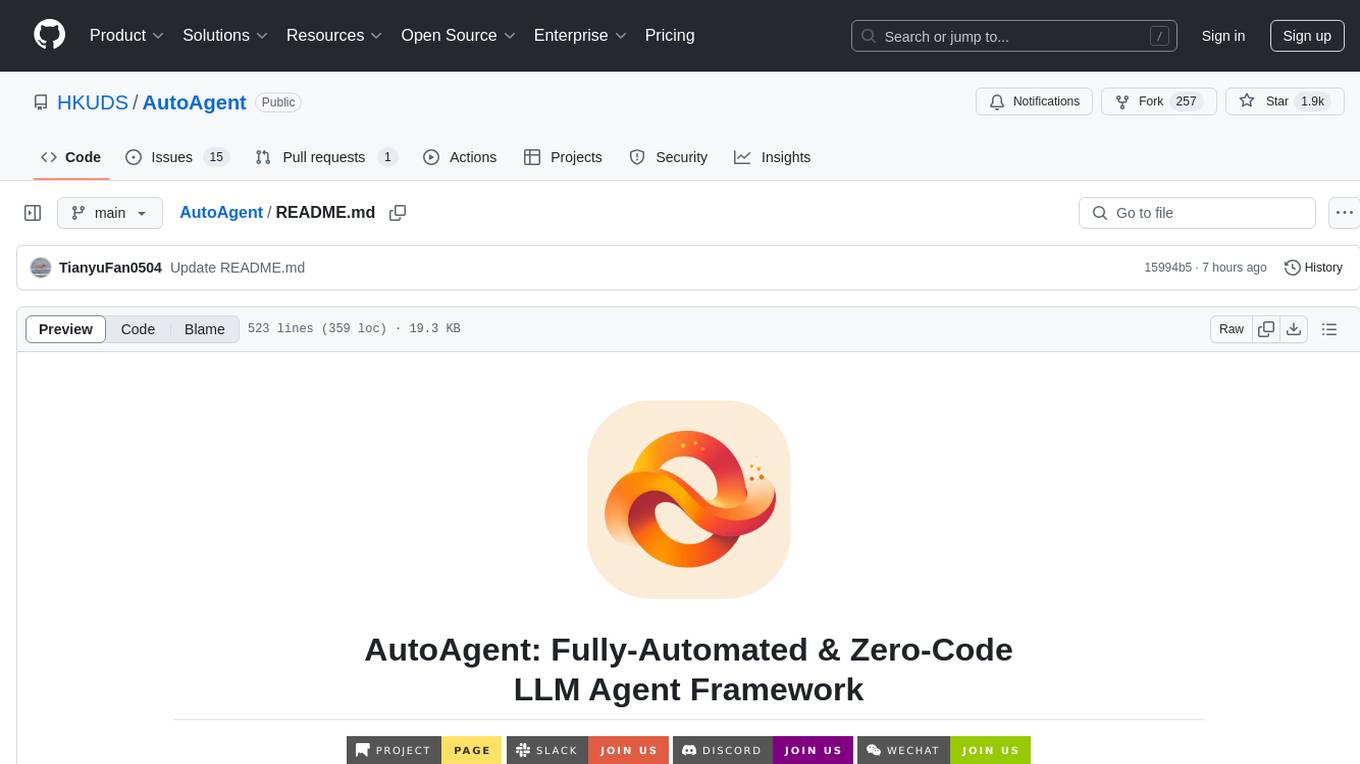
AutoAgent
AutoAgent is a fully-automated and zero-code framework that enables users to create and deploy LLM agents through natural language alone. It is a top performer on the GAIA Benchmark, equipped with a native self-managing vector database, and allows for easy creation of tools, agents, and workflows without any coding. AutoAgent seamlessly integrates with a wide range of LLMs and supports both function-calling and ReAct interaction modes. It is designed to be dynamic, extensible, customized, and lightweight, serving as a personal AI assistant.
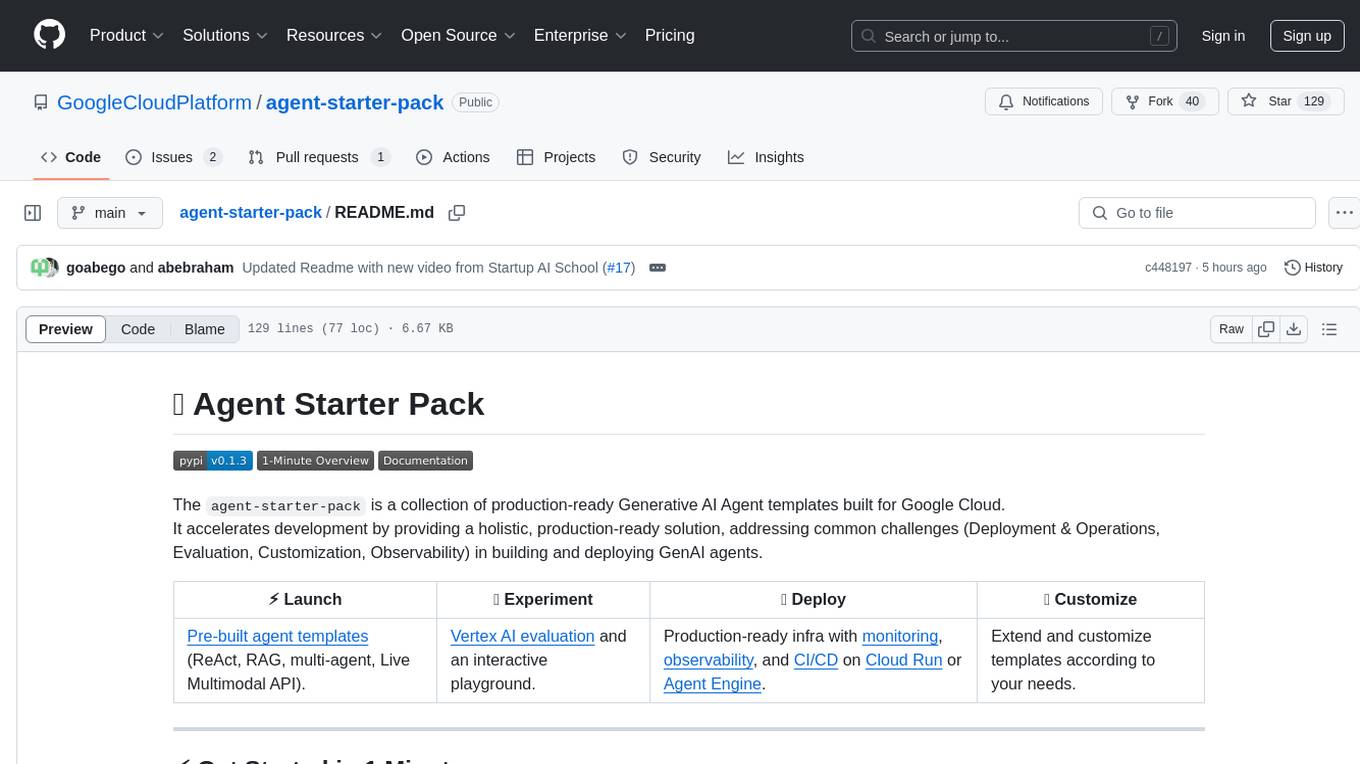
agent-starter-pack
The agent-starter-pack is a collection of production-ready Generative AI Agent templates built for Google Cloud. It accelerates development by providing a holistic, production-ready solution, addressing common challenges in building and deploying GenAI agents. The tool offers pre-built agent templates, evaluation tools, production-ready infrastructure, and customization options. It also provides CI/CD automation and data pipeline integration for RAG agents. The starter pack covers all aspects of agent development, from prototyping and evaluation to deployment and monitoring. It is designed to simplify project creation, template selection, and deployment for agent development on Google Cloud.
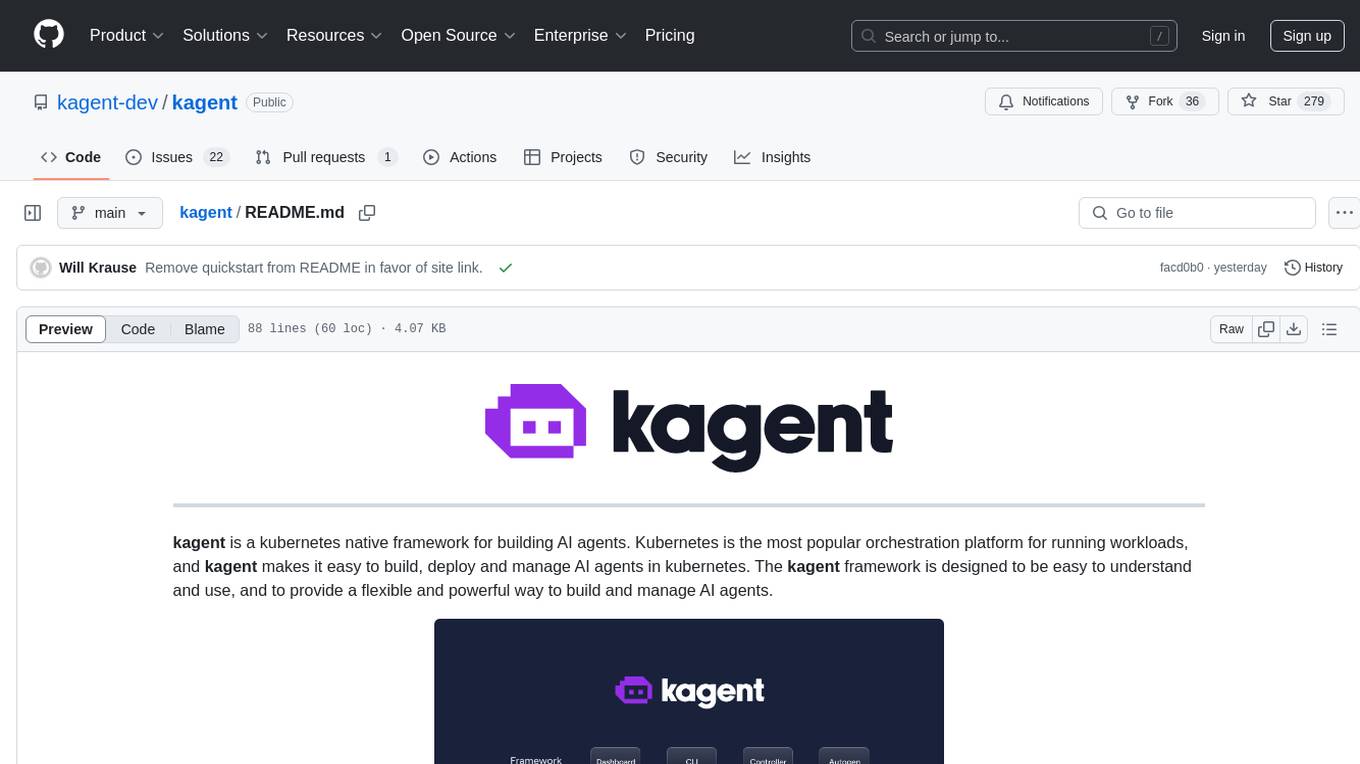
kagent
Kagent is a Kubernetes native framework for building AI agents, designed to be easy to understand and use. It provides a flexible and powerful way to build, deploy, and manage AI agents in Kubernetes. The framework consists of agents, tools, and model configurations defined as Kubernetes custom resources, making them easy to manage and modify. Kagent is extensible, flexible, observable, declarative, testable, and has core components like a controller, UI, engine, and CLI.
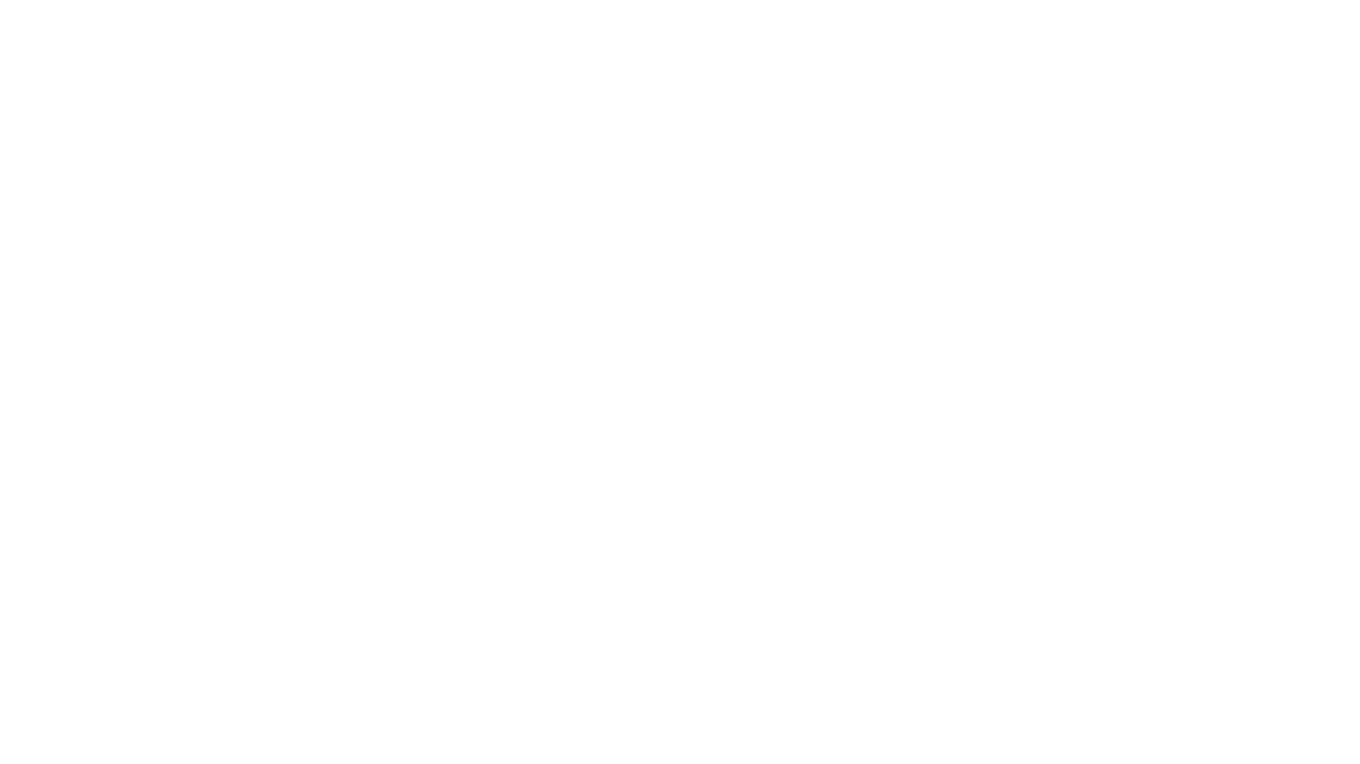
motia
Motia is an AI agent framework designed for software engineers to create, test, and deploy production-ready AI agents quickly. It provides a code-first approach, allowing developers to write agent logic in familiar languages and visualize execution in real-time. With Motia, developers can focus on business logic rather than infrastructure, offering zero infrastructure headaches, multi-language support, composable steps, built-in observability, instant APIs, and full control over AI logic. Ideal for building sophisticated agents and intelligent automations, Motia's event-driven architecture and modular steps enable the creation of GenAI-powered workflows, decision-making systems, and data processing pipelines.
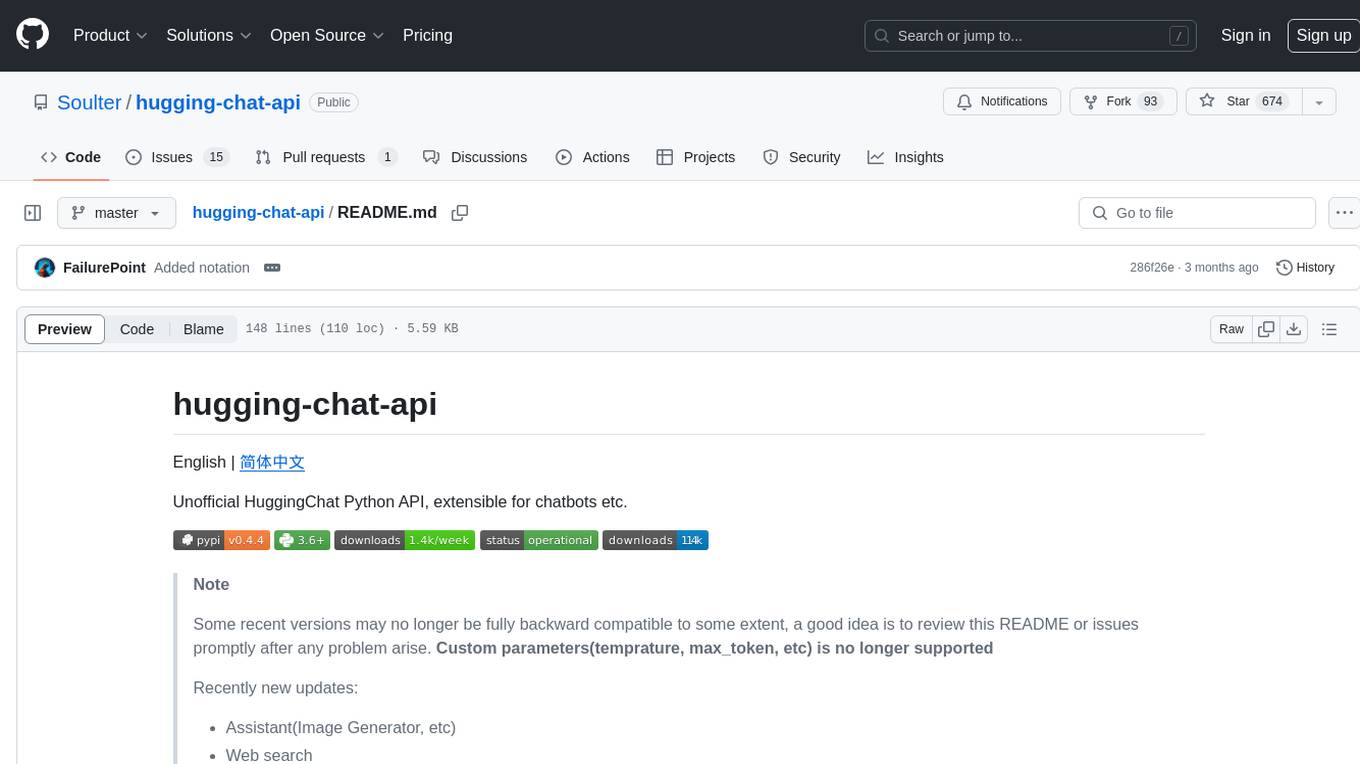
hugging-chat-api
Unofficial HuggingChat Python API for creating chatbots, supporting features like image generation, web search, memorizing context, and changing LLMs. Users can log in, chat with the ChatBot, perform web searches, create new conversations, manage conversations, switch models, get conversation info, use assistants, and delete conversations. The API also includes a CLI mode with various commands for interacting with the tool. Users are advised not to use the application for high-stakes decisions or advice and to avoid high-frequency requests to preserve server resources.
For similar jobs
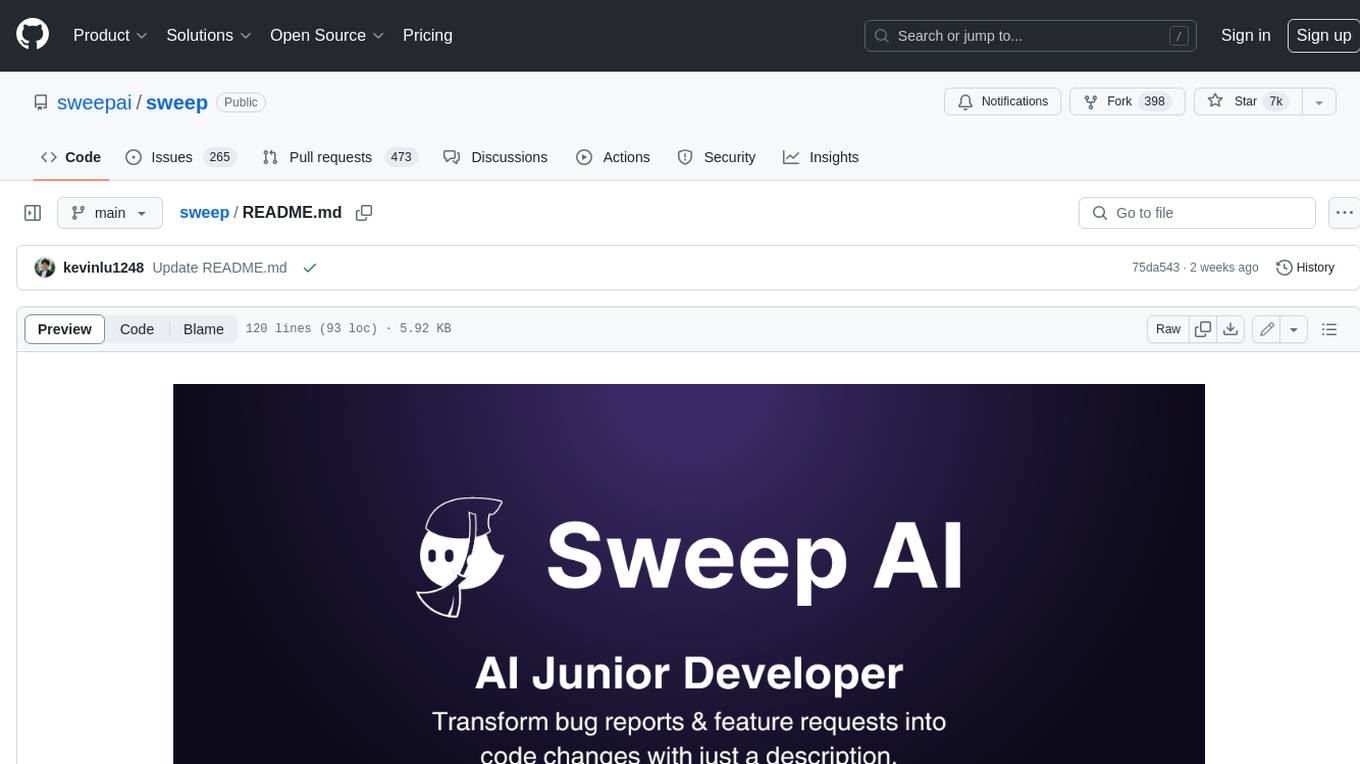
sweep
Sweep is an AI junior developer that turns bugs and feature requests into code changes. It automatically handles developer experience improvements like adding type hints and improving test coverage.
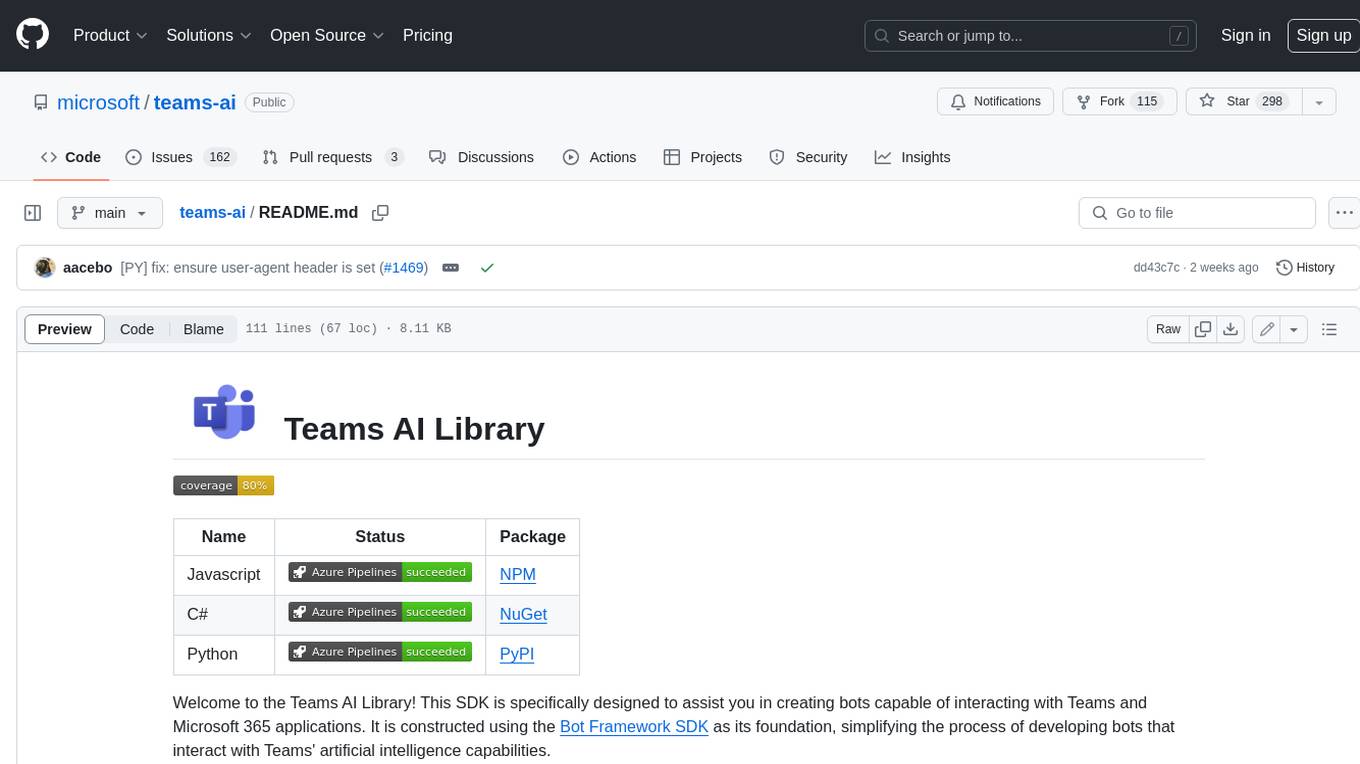
teams-ai
The Teams AI Library is a software development kit (SDK) that helps developers create bots that can interact with Teams and Microsoft 365 applications. It is built on top of the Bot Framework SDK and simplifies the process of developing bots that interact with Teams' artificial intelligence capabilities. The SDK is available for JavaScript/TypeScript, .NET, and Python.
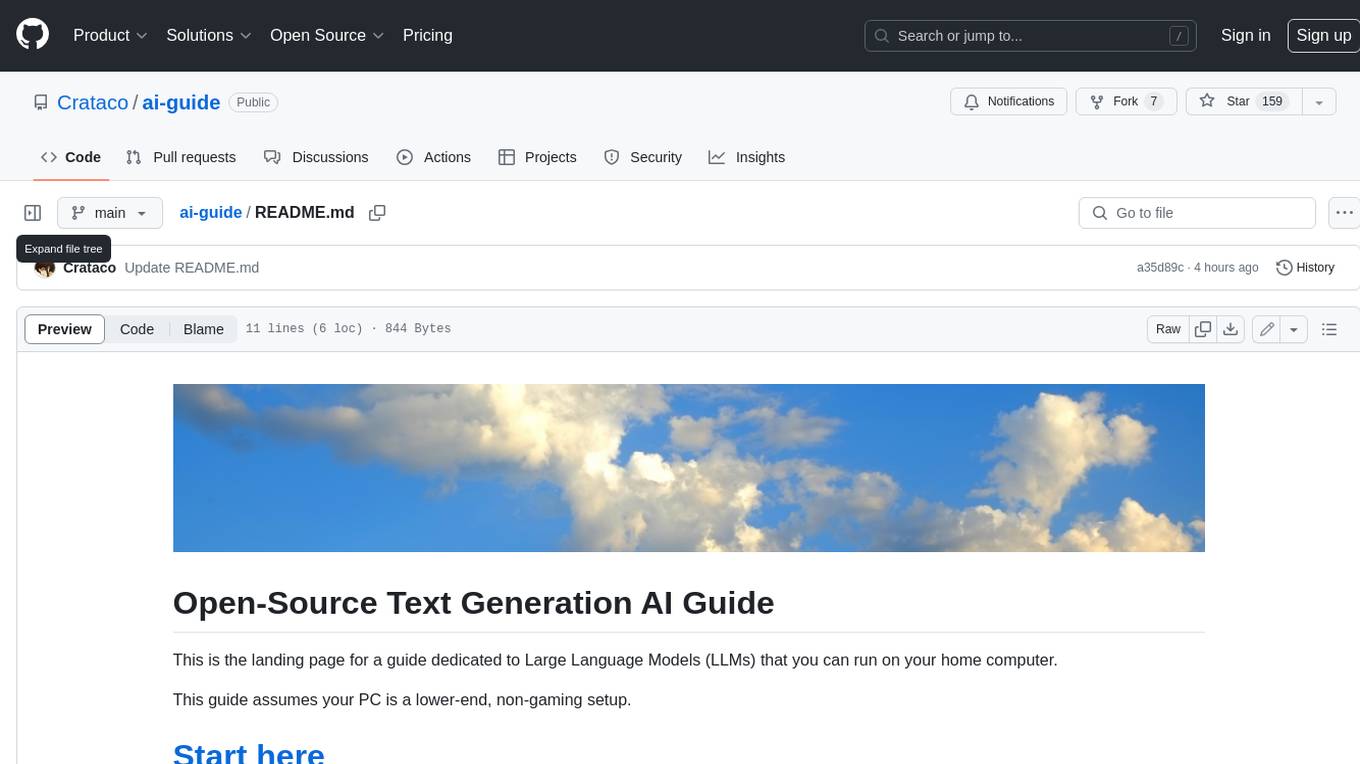
ai-guide
This guide is dedicated to Large Language Models (LLMs) that you can run on your home computer. It assumes your PC is a lower-end, non-gaming setup.
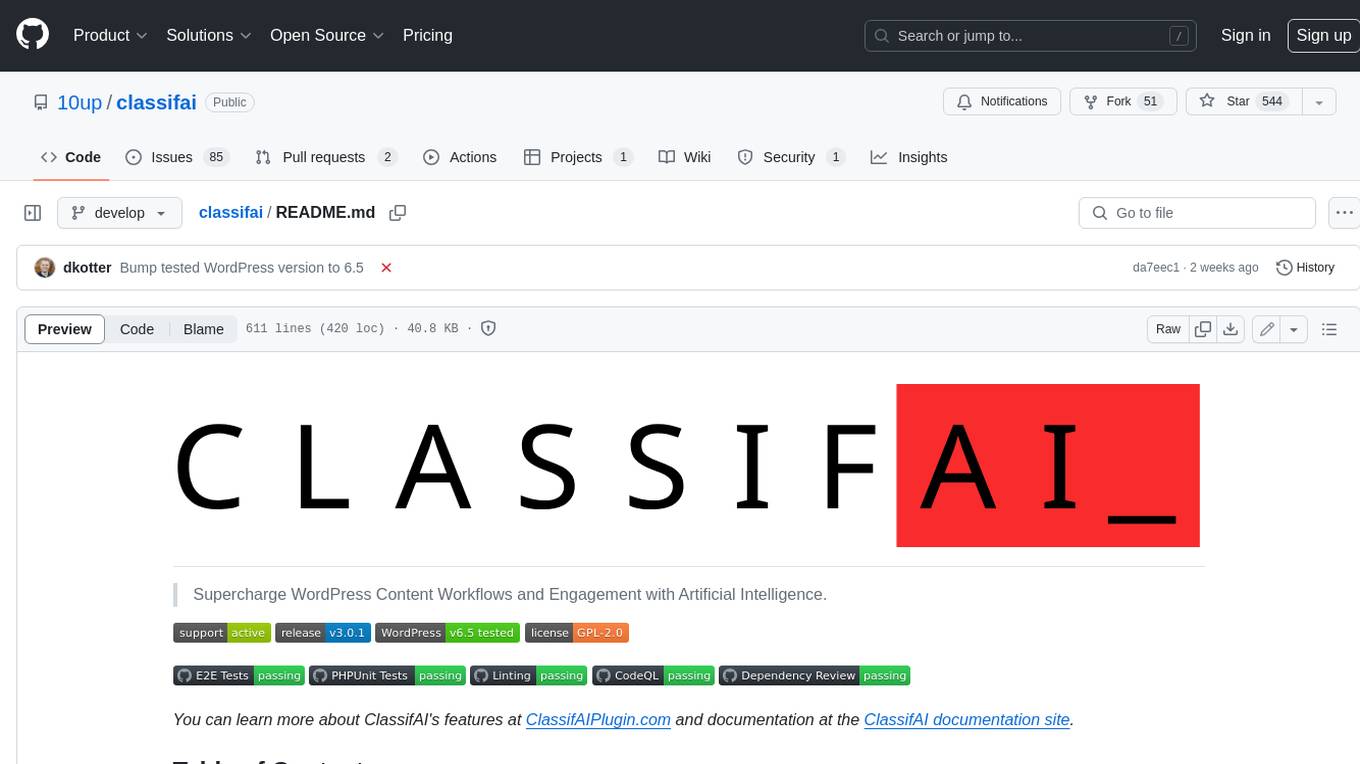
classifai
Supercharge WordPress Content Workflows and Engagement with Artificial Intelligence. Tap into leading cloud-based services like OpenAI, Microsoft Azure AI, Google Gemini and IBM Watson to augment your WordPress-powered websites. Publish content faster while improving SEO performance and increasing audience engagement. ClassifAI integrates Artificial Intelligence and Machine Learning technologies to lighten your workload and eliminate tedious tasks, giving you more time to create original content that matters.
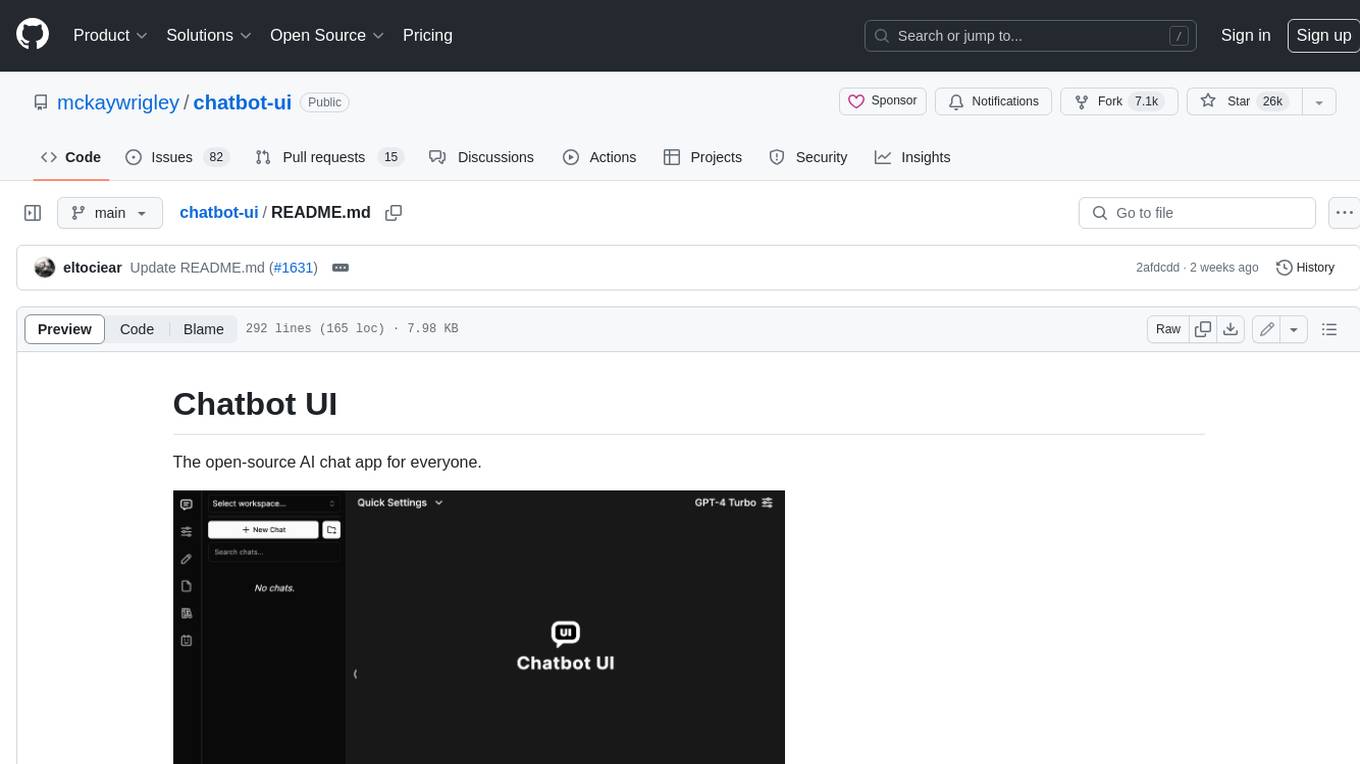
chatbot-ui
Chatbot UI is an open-source AI chat app that allows users to create and deploy their own AI chatbots. It is easy to use and can be customized to fit any need. Chatbot UI is perfect for businesses, developers, and anyone who wants to create a chatbot.
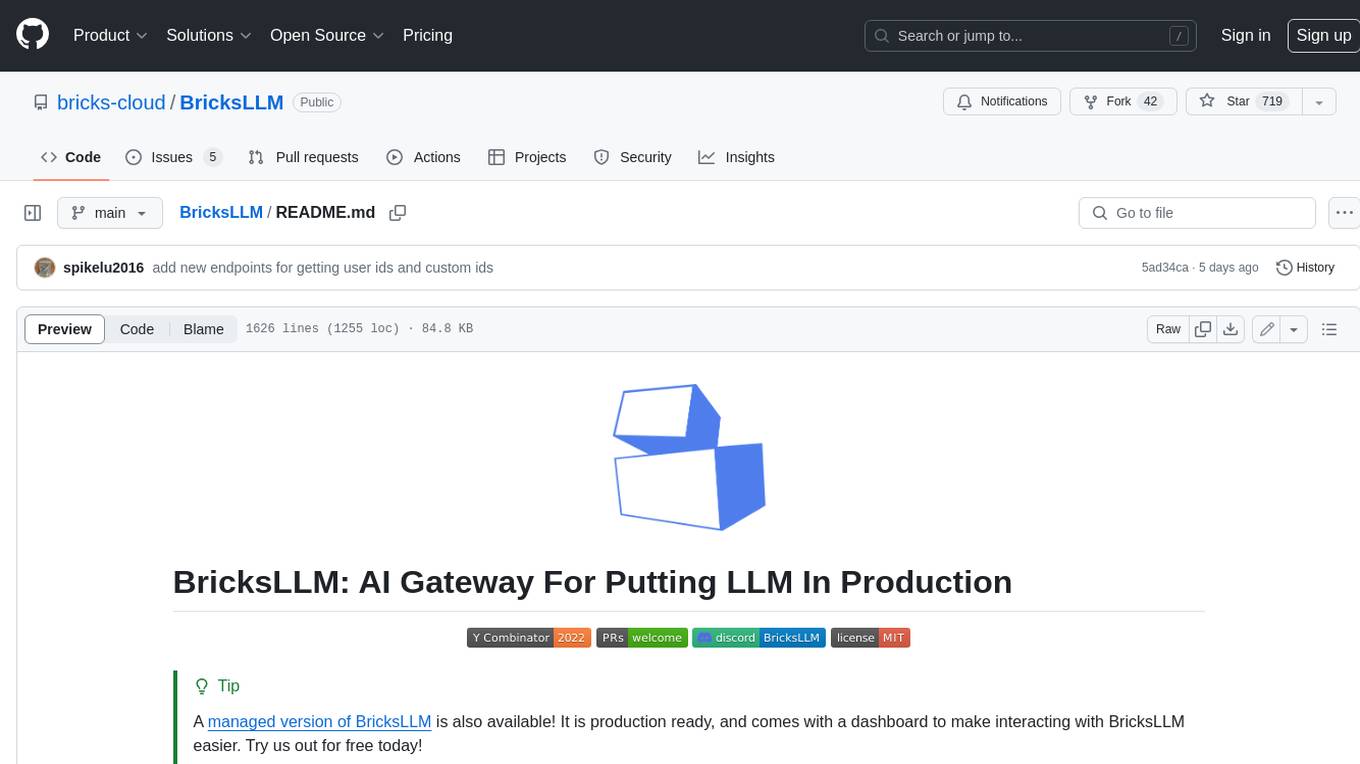
BricksLLM
BricksLLM is a cloud native AI gateway written in Go. Currently, it provides native support for OpenAI, Anthropic, Azure OpenAI and vLLM. BricksLLM aims to provide enterprise level infrastructure that can power any LLM production use cases. Here are some use cases for BricksLLM: * Set LLM usage limits for users on different pricing tiers * Track LLM usage on a per user and per organization basis * Block or redact requests containing PIIs * Improve LLM reliability with failovers, retries and caching * Distribute API keys with rate limits and cost limits for internal development/production use cases * Distribute API keys with rate limits and cost limits for students
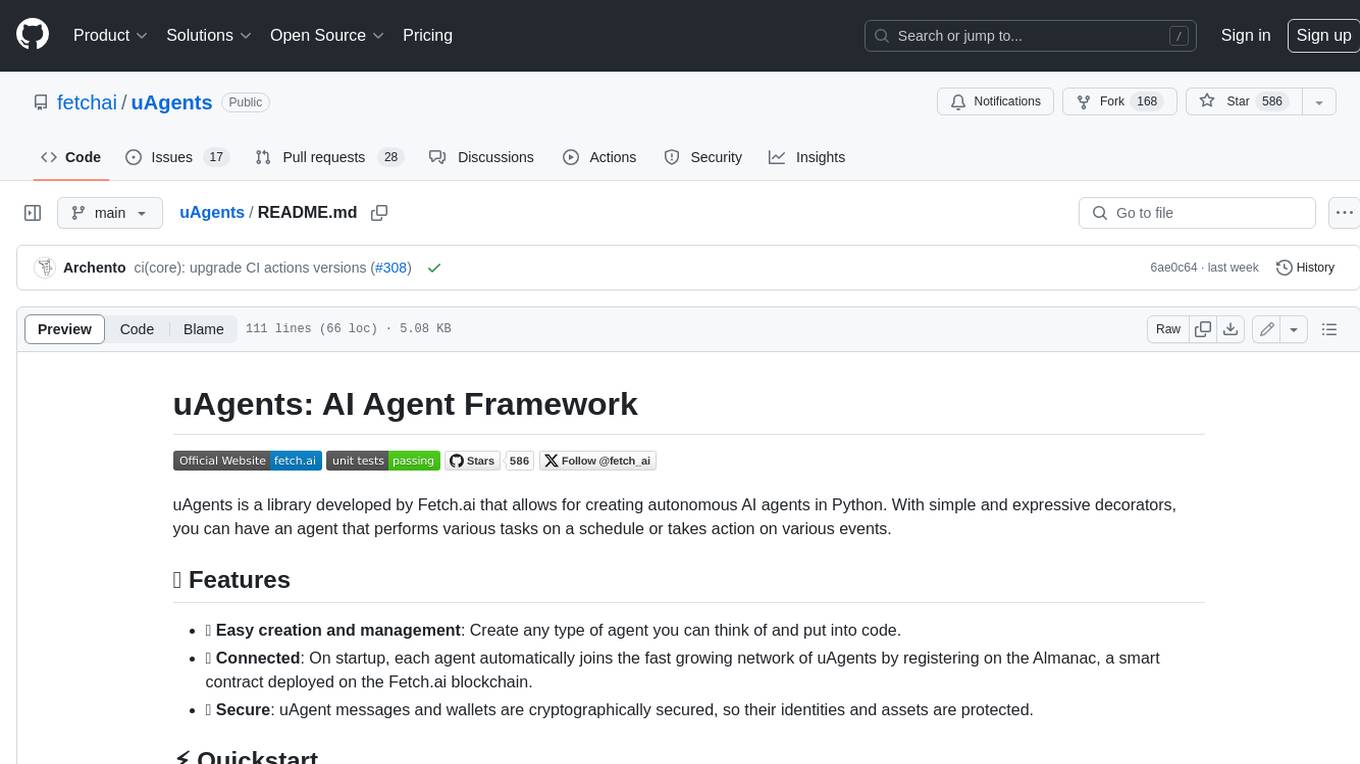
uAgents
uAgents is a Python library developed by Fetch.ai that allows for the creation of autonomous AI agents. These agents can perform various tasks on a schedule or take action on various events. uAgents are easy to create and manage, and they are connected to a fast-growing network of other uAgents. They are also secure, with cryptographically secured messages and wallets.
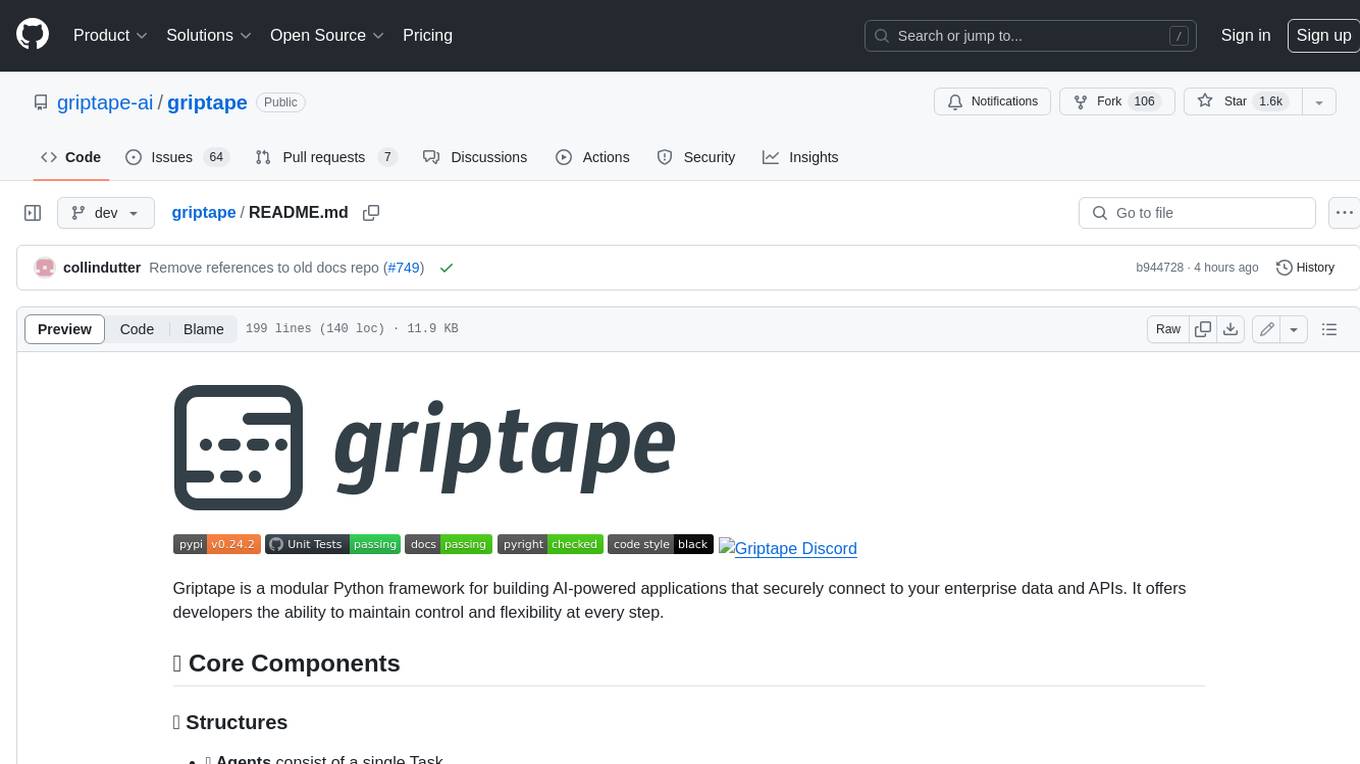
griptape
Griptape is a modular Python framework for building AI-powered applications that securely connect to your enterprise data and APIs. It offers developers the ability to maintain control and flexibility at every step. Griptape's core components include Structures (Agents, Pipelines, and Workflows), Tasks, Tools, Memory (Conversation Memory, Task Memory, and Meta Memory), Drivers (Prompt and Embedding Drivers, Vector Store Drivers, Image Generation Drivers, Image Query Drivers, SQL Drivers, Web Scraper Drivers, and Conversation Memory Drivers), Engines (Query Engines, Extraction Engines, Summary Engines, Image Generation Engines, and Image Query Engines), and additional components (Rulesets, Loaders, Artifacts, Chunkers, and Tokenizers). Griptape enables developers to create AI-powered applications with ease and efficiency.