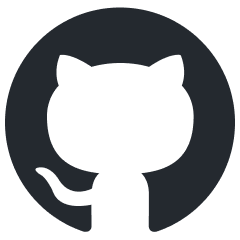
arbigent
AI Agent for testing Android, iOS, and Web apps. Get Started in 5 Minutes. Arbigent's intuitive UI and powerful code interface make it accessible to everyone, while its scenario breakdown feature ensures scalability for even the most complex tasks.
Stars: 221
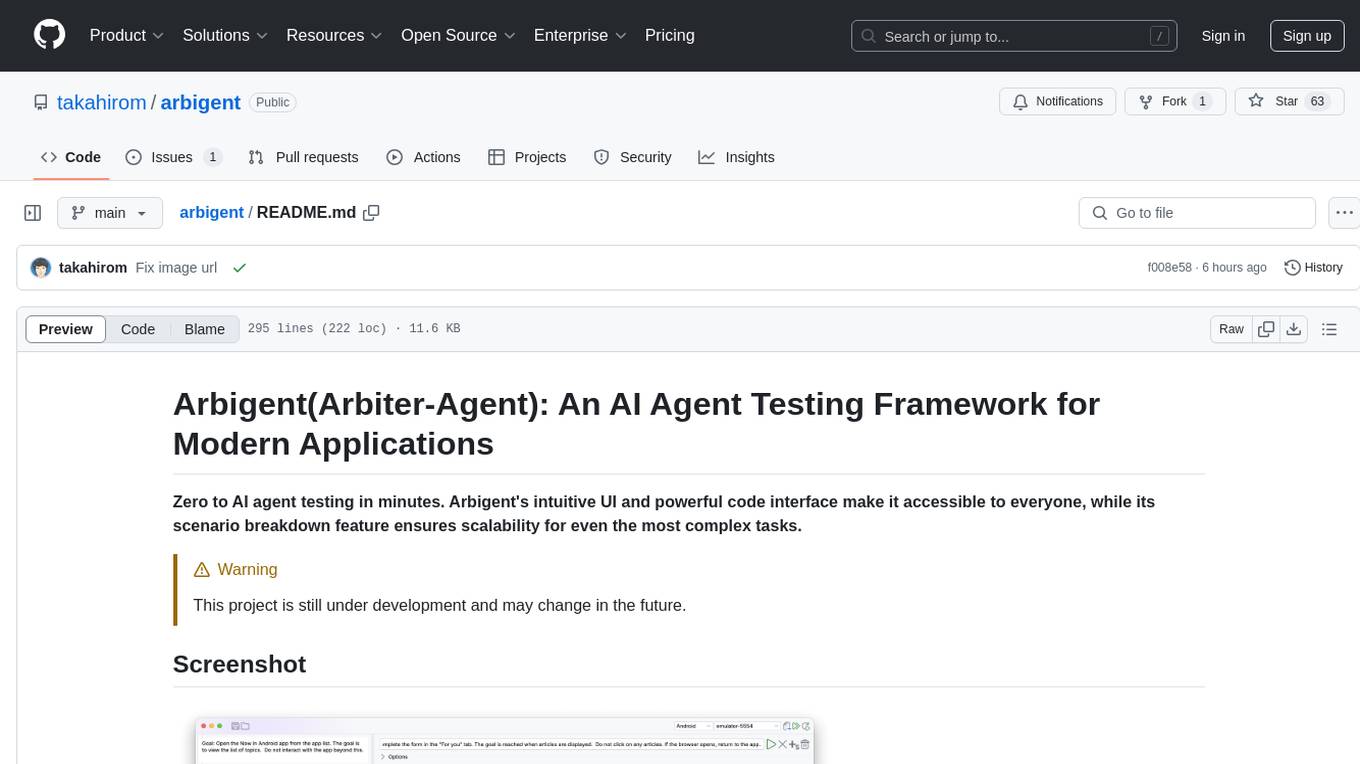
Arbigent (Arbiter-Agent) is an AI agent testing framework designed to make AI agent testing practical for modern applications. It addresses challenges faced by traditional UI testing frameworks and AI agents by breaking down complex tasks into smaller, dependent scenarios. The framework is customizable for various AI providers, operating systems, and form factors, empowering users with extensive customization capabilities. Arbigent offers an intuitive UI for scenario creation and a powerful code interface for seamless test execution. It supports multiple form factors, optimizes UI for AI interaction, and is cost-effective by utilizing models like GPT-4o mini. With a flexible code interface and open-source nature, Arbigent aims to revolutionize AI agent testing in modern applications.
README:
Zero to AI agent testing in minutes. Arbigent's intuitive UI and powerful code interface make it accessible to everyone, while its scenario breakdown feature ensures scalability for even the most complex tasks.
[!WARNING] There seems to be a spam account posing as Arbigent, but the account is not related to me. The creator's accounts are
https://x.com/_takahirom_
andhttps://x.com/new_runnable
.
https://github.com/user-attachments/assets/ec582760-5d6a-4ee3-8067-87cb2b673c8d
Traditional UI testing often relies on brittle methods that are easily disrupted by even minor UI changes. A/B tests, updated tutorials, unexpected dialogs, dynamic advertising, or ever-changing user-generated content can cause tests to fail.
AI agents emerged as a solution, but testing with AI agents also presents challenges. AI agents often don't work as intended; for example, the agents might open other apps or click on the wrong button due to the complexity of the task.
To address these challenges, I created Arbigent, an AI agent testing framework that can break down complex tasks into smaller, dependent scenarios. By decomposing tasks, Arbigent enables more predictable and scalable testing of AI agents in modern applications.
I believe many AI Agent testing frameworks will emerge in the future. However, widespread adoption might be delayed due to limitations in customization. For instance:
- Limited AI Provider Support: Frameworks might be locked to specific AI providers, excluding those used internally by companies.
- Slow OS Adoption: Support for different operating systems (like iOS and Android) could lag.
- Delayed Form Factor Support: Expanding to form factors beyond phones, such as Android TV, might take considerable time.
To address these issues, I aimed to create a framework that empowers users with extensive customization capabilities. Inspired by OkHttp's interceptor pattern, Arbigent provides interfaces for flexible customization, allowing users to adapt the framework to their specific needs, such as those listed above.
Furthermore, I wanted to make Arbigent accessible to QA engineers by offering a user-friendly UI. This allows for scenario creation within the UI and seamless test execution via the code interface.
I. Core Functionality & Design
-
Complex Task Management:
- Scenario Dependencies: Breaks down complex goals into smaller, manageable scenarios that depend on each other (e.g., login -> search).
- Orchestration: Acts as a mediator, managing the execution flow of AI agents across multiple, interconnected scenarios.
-
Hybrid Development Workflow:
- UI-Driven Scenario Creation: Allows non-programmers (e.g., QA engineers) to visually design test scenarios through a user-friendly interface.
- Code-Based Execution: Enables software engineers to execute the saved scenarios programmatically (YAML files), allowing for integration with existing testing infrastructure.
II. Cross-Platform & Device Support
-
Multi-Platform Compatibility:
- Mobile & TV: Supports testing on iOS, Android, Web, and TV interfaces.
- D-Pad Navigation: Handles TV interfaces that rely on D-pad navigation.
III. AI Optimization & Efficiency
-
Enhanced AI Understanding:
- UI Tree Optimization: Simplifies and filters the UI tree to improve AI comprehension and performance.
- Accessibility-Independent: Provides annotated screenshots to assist AI in understanding UIs that lack accessibility information.
-
Cost Savings:
- Open Source: Free to use, modify, and distribute, eliminating licensing costs.
-
Efficient Model Usage: Compatible with cost-effective models like
GPT-4o mini
, reducing operational expenses.
IV. Robustness & Reliability
- Double Check with AI-Powered Image Assertion: Integrates Roborazzi's feature to verify AI decisions using image-based prompts and allows the AI to re-evaluate if needed.
- Stuck Screen Detection: Identifies and recovers from situations where the AI agent gets stuck on the same screen, prompting it to reconsider its actions.
V. Advanced Features & Customization
-
Flexible Code Interface:
- Custom Hooks: Offers a code interface for adding custom initialization and cleanup methods, providing greater control over scenario execution.
-
Model Context Protocol (MCP) Support:
- Introduced initial support for MCP, enabling Arbigent to leverage external tools and services defined via MCP servers. This significantly extends testing capabilities beyond direct UI interaction.
- You can configure MCP servers using a JSON string in the Project Settings.
-
Example MCP Use Cases:
- Install and launch applications
- Check server logs (e.g., user behavior) using external tools
- Retrieve debug logs
- Interact with various other custom tools and services
VI. Community & Open Source
-
Open Source Nature:
- Free & Open: Freely available for use, modification, and distribution.
- Community Driven: Welcomes contributions from the community to enhance and expand the framework.
Arbigent's Strengths and Weaknesses Based on SMURF
I categorized automated testing frameworks into five levels using the SMURF framework. Here's how Arbigent stacks up:
-
Speed (1/5): Arbigent's speed is currently limited by the underlying AI technology and the need to interact with the application's UI in real-time. This makes it slower than traditional unit or integration tests.
- We have introduced some mechanisms to address this:
- Tests can be parallelized using the
--shard
option to speed up execution. - AI result caching can be utilized when the UI tree and goal are identical, which is configurable in the project settings.
- Tests can be parallelized using the
- We have introduced some mechanisms to address this:
- Maintainability (4/5): Arbigent excels in maintainability. The underlying AI model can adapt to minor UI changes, minimizing the need to rewrite tests for every small update, thus reducing maintenance effort. You can write tests in natural language (e.g., "Complete the tutorial"), making them resilient to UI changes. The task decomposition feature also reduces duplication, further enhancing maintainability. Maintenance can be done by non-engineers, thanks to the natural language interface.
- Utilization (1/5): Arbigent requires both device resources (emulators or physical devices) and AI resources, which can be costly. (AI cost can be around $0.005 per step and $0.02 per task when using GPT-4o.)
-
Reliability (3/5): Arbigent has several features to improve reliability. It automatically waits during loading screens, handles unexpected dialogs, and even attempts self-correction. However, external factors like emulator flakiness can still impact reliability.
- Recently I found Arbigent has retry feature and can execute the scenario from the beginning. But, even without retry, Arbigent works fine without failures thanks to the flexibility of AI.
- Fidelity (5/5): Arbigent provides high fidelity by testing on real or emulated devices with the actual application. It can even assess aspects that were previously difficult to test, such as verifying video playback by checking for visual changes on the screen.
I believe that many of its current limitations, such as speed, maintainability, utilization, and reliability, will be addressed as AI technology continues to evolve. The need for extensive prompt engineering will likely diminish as AI models become more capable.
Install the Arbigent UI binary from the Release page.
If you encounter security warnings when opening the app: Refer to Apple's guide on opening apps from unidentified developers.
This Open Anyway
button is available for about an hour after you try to open the app.
- Connect your device to your PC.
- In the Arbigent UI, select your connected device from the list of available devices. This will establish a connection.
- Enter your AI provider's API key in the designated field within the Arbigent UI.
Use the intuitive UI to define scenarios. Simply specify the desired goal for the AI agent.
Run tests either directly through the UI or programmatically via the code interface or CLI.
You can install the CLI via Homebrew and run a saved YAML file.
brew tap takahirom/homebrew-repo
brew install takahirom/repo/arbigent
Usage: arbigent [<options>]
Options for OpenAI API AI:
--open-ai-endpoint=<text> Endpoint URL (default: https://api.openai.com/v1/)
--open-ai-model-name=<text> Model name (default: gpt-4o-mini)
Options for Gemini API AI:
--gemini-endpoint=<text> Endpoint URL (default: https://generativelanguage.googleapis.com/v1beta/openai/)
--gemini-model-name=<text> Model name (default: gemini-1.5-flash)
Options for Azure OpenAI:
--azure-open-aiendpoint=<text> Endpoint URL
--azure-open-aiapi-version=<text> API version
--azure-open-aimodel-name=<text> Model name (default: gpt-4o-mini)
Options:
--ai-type=(openai|gemini|azureopenai) Type of AI to use
--os=(android|ios|web) Target operating system
--project-file=<text> Path to the project YAML file
--log-level=(debug|info|warn|error) Log level
--shard=<value> Shard specification (e.g., 1/5)
-h, --help Show this message and exit
You can run tests separately with the --shard
option. This allows you to split your test suite and run tests in parallel, reducing overall test execution time.
Example:
arbigent --shard=1/4
This command will run the first quarter of your test suite.
Integrating with GitHub Actions:
Here's an example of how to integrate the --shard
option with GitHub Actions to run parallel tests on multiple Android emulators:
cli-e2e-android:
runs-on: ubuntu-latest
strategy:
fail-fast: false
matrix:
shardIndex: [ 1, 2, 3, 4 ]
shardTotal: [ 4 ]
steps:
...
- name: CLI E2E test
uses: reactivecircus/android-emulator-runner@v2
...
script: |
arbigent --shard=${{ matrix.shardIndex }}/${{ matrix.shardTotal }} --os=android --project-file=sample-test/src/main/resources/projects/e2e-test-android.yaml --ai-type=gemini --gemini-model-name=gemini-2.0-flash-exp
...
- uses: actions/upload-artifact@b4b15b8c7c6ac21ea08fcf65892d2ee8f75cf882 # v4
if: ${{ always() }}
with:
name: cli-report-android-${{ matrix.shardIndex }}-${{ matrix.shardTotal }}
path: |
arbigent-result/*
retention-days: 90
You can use the CLI in GitHub Actions like in this sample. There are only two files: .github/workflows/arbigent-test.yaml
and arbigent-project.yaml
. This example demonstrates GitHub Actions and an arbigent-project.yaml
file created by the Arbigent UI.
https://github.com/takahirom/arbigent-sample
AI Provider | Supported |
---|---|
OpenAI | Yes |
Gemini | Yes |
OpenAI based APIs like Ollama | Yes |
You can add AI providers by implementing the ArbigentAi
interface.
OS | Supported | Test Status in the Arbigent repository |
---|---|---|
Android | Yes | End-to-End including Android emulator and real AI |
iOS | Yes | End-to-End including iOS simulator and real AI |
Web(Chrome) | Yes | Currently, Testing not yet conducted |
You can add OSes by implementing the ArbigentDevice
interface. Thanks to the excellent Maestro library, we are able to support multiple OSes.
Form Factor | Supported |
---|---|
Phone / Tablet | Yes |
TV(D-Pad) | Yes |
The execution flow involves the UI, Arbigent, ArbigentDevice, and ArbigentAi. The UI sends a project creation request to Arbigent, which fetches the UI tree from ArbigentDevice. ArbigentAi then decides on an action based on the goal and UI tree. The action is performed by ArbigentDevice, and the results are returned to the UI for display.
sequenceDiagram
participant UI(or Tests)
participant ArbigentAgent
participant ArbigentDevice
participant ArbigentAi
UI(or Tests)->>ArbigentAgent: Execute
loop
ArbigentAgent->>ArbigentDevice: Fetch UI tree
ArbigentDevice->>ArbigentAgent: Return UI tree
ArbigentAgent->>ArbigentAi: Decide Action by goal and UI tree and histories
ArbigentAi->>ArbigentAgent: Return Action
ArbigentAgent->>ArbigentDevice: Perform actions
ArbigentDevice->>ArbigentAgent: Return results
end
ArbigentAgent->>UI(or Tests): Display results
The class diagram illustrates the relationships between ArbigentProject, ArbigentScenario, ArbigentTask, ArbigentAgent, ArbigentScenarioExecutor, ArbigentAi, ArbigentDevice, and ArbigentInterceptor.
classDiagram
direction TB
class ArbigentProject {
+List~ArbigentScenario~ scenarios
+execute()
}
class ArbigentAgentTask {
+String goal
}
class ArbigentAgent {
+ArbigentAi ai
+ArbigentDevice device
+List~ArbigentInterceptor~ interceptors
+execute(arbigentAgentTask)
}
class ArbigentScenarioExecutor {
+execute(arbigentScenario)
}
class ArbigentScenario {
+List~ArbigentAgentTask~ agentTasks
}
ArbigentProject o--"*" ArbigentScenarioExecutor
ArbigentScenarioExecutor o--"*" ArbigentAgent
ArbigentScenario o--"*" ArbigentAgentTask
ArbigentProject o--"*" ArbigentScenario
[!WARNING] The yaml format is still under development and may change in the future.
The project file is saved in YAML format and contains scenarios with goals, initialization methods, and cleanup data. Dependencies between scenarios are also defined. You can write a project file in YAML format by hand or create it using the Arbigent UI.
The id is auto-generated UUID by Arbigent UI but you can change it to any string.
scenarios:
- id: "7788d7f4-7276-4cb3-8e98-7d3ad1d1cd47"
goal: "Open the Now in Android app from the app list. The goal is to view the list\
\ of topics. Do not interact with the app beyond this."
initializationMethods:
- type: "CleanupData"
packageName: "com.google.samples.apps.nowinandroid"
- type: "LaunchApp"
packageName: "com.google.samples.apps.nowinandroid"
- id: "f0ef0129-c764-443f-897d-fc4408e5952b"
goal: "In the Now in Android app, select an tech topic and complete the form in\
\ the \"For you\" tab. The goal is reached when articles are displayed. Do not\
\ click on any articles. If the browser opens, return to the app."
dependency: "7788d7f4-7276-4cb3-8e98-7d3ad1d1cd47"
imageAssertions:
- assertionPrompt: "Articles are visible on the screen"
- id: "73c785f7-0f45-4709-97b5-601b6803eb0d"
goal: "Save an article using the Bookmark button."
dependency: "f0ef0129-c764-443f-897d-fc4408e5952b"
- id: "797514d2-fb04-4b92-9c07-09d46cd8f931"
goal: "Check if a saved article appears in the Saved tab."
dependency: "73c785f7-0f45-4709-97b5-601b6803eb0d"
imageAssertions:
- assertionPrompt: "The screen is showing Saved tab"
- assertionPrompt: "There is an article in the screen"
[!WARNING] The code interface is still under development and may change in the future.
Arbigent provides a code interface for executing tests programmatically. Here's an example of how to run a test:
Stay tuned for the release of Arbigent on Maven Central.
You can load a project yaml file and execute it using the following code:
class ArbigentTest {
private val scenarioFile = File(this::class.java.getResource("/projects/nowinandroidsample.yaml").toURI())
@Test
fun tests() = runTest(
timeout = 10.minutes
) {
val arbigentProject = ArbigentProject(
file = scenarioFile,
aiFactory = {
OpenAIAi(
apiKey = System.getenv("OPENAI_API_KEY")
)
},
deviceFactory = {
AvailableDevice.Android(
dadb = Dadb.discover()!!
).connectToDevice()
}
)
arbigentProject.execute()
}
}
val agentConfig = AgentConfig {
deviceFactory { FakeDevice() }
ai(FakeAi())
}
val arbigentScenarioExecutor = ArbigentScenarioExecutor {
}
val arbigentScenario = ArbigentScenario(
id = "id2",
agentTasks = listOf(
ArbigentAgentTask("id1", "Login in the app and see the home tab.", agentConfig),
ArbigentAgentTask("id2", "Search an episode and open detail", agentConfig)
),
maxStepCount = 10,
)
arbigentScenarioExecutor.execute(
arbigentScenario
)
val agentConfig = AgentConfig {
deviceFactory { FakeDevice() }
ai(FakeAi())
}
val task = ArbigentAgentTask("id1", "Login in the app and see the home tab.", agentConfig)
ArbigentAgent(agentConfig)
.execute(task)
For Tasks:
Click tags to check more tools for each tasksFor Jobs:
Alternative AI tools for arbigent
Similar Open Source Tools
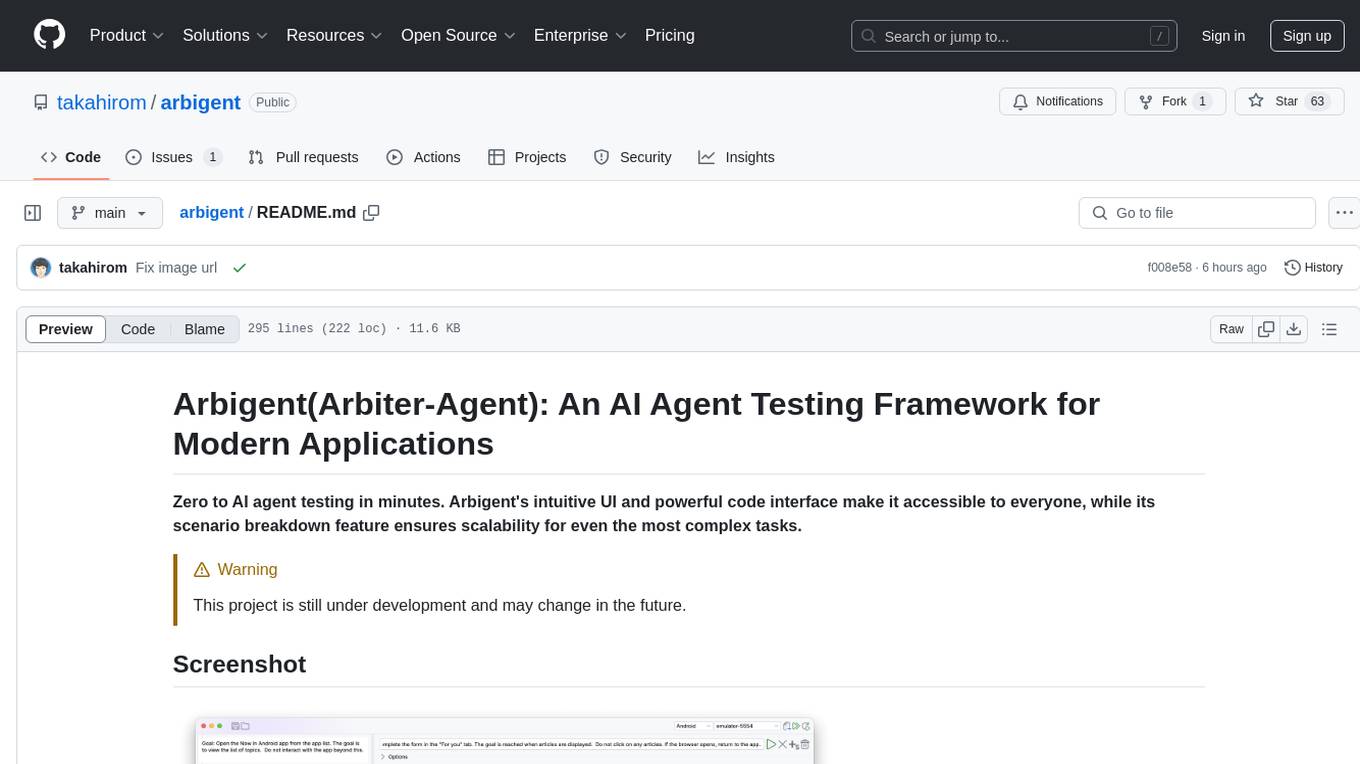
arbigent
Arbigent (Arbiter-Agent) is an AI agent testing framework designed to make AI agent testing practical for modern applications. It addresses challenges faced by traditional UI testing frameworks and AI agents by breaking down complex tasks into smaller, dependent scenarios. The framework is customizable for various AI providers, operating systems, and form factors, empowering users with extensive customization capabilities. Arbigent offers an intuitive UI for scenario creation and a powerful code interface for seamless test execution. It supports multiple form factors, optimizes UI for AI interaction, and is cost-effective by utilizing models like GPT-4o mini. With a flexible code interface and open-source nature, Arbigent aims to revolutionize AI agent testing in modern applications.
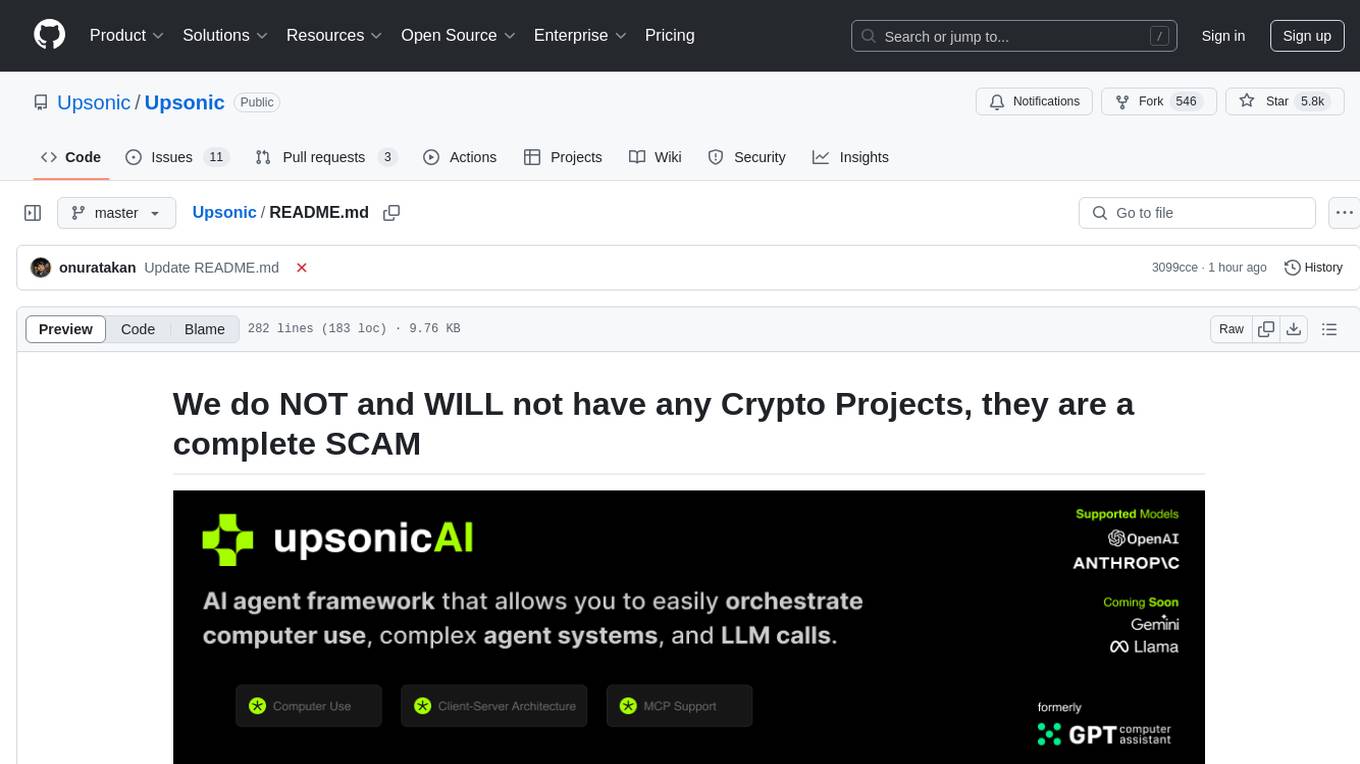
Upsonic
Upsonic offers a cutting-edge enterprise-ready framework for orchestrating LLM calls, agents, and computer use to complete tasks cost-effectively. It provides reliable systems, scalability, and a task-oriented structure for real-world cases. Key features include production-ready scalability, task-centric design, MCP server support, tool-calling server, computer use integration, and easy addition of custom tools. The framework supports client-server architecture and allows seamless deployment on AWS, GCP, or locally using Docker.
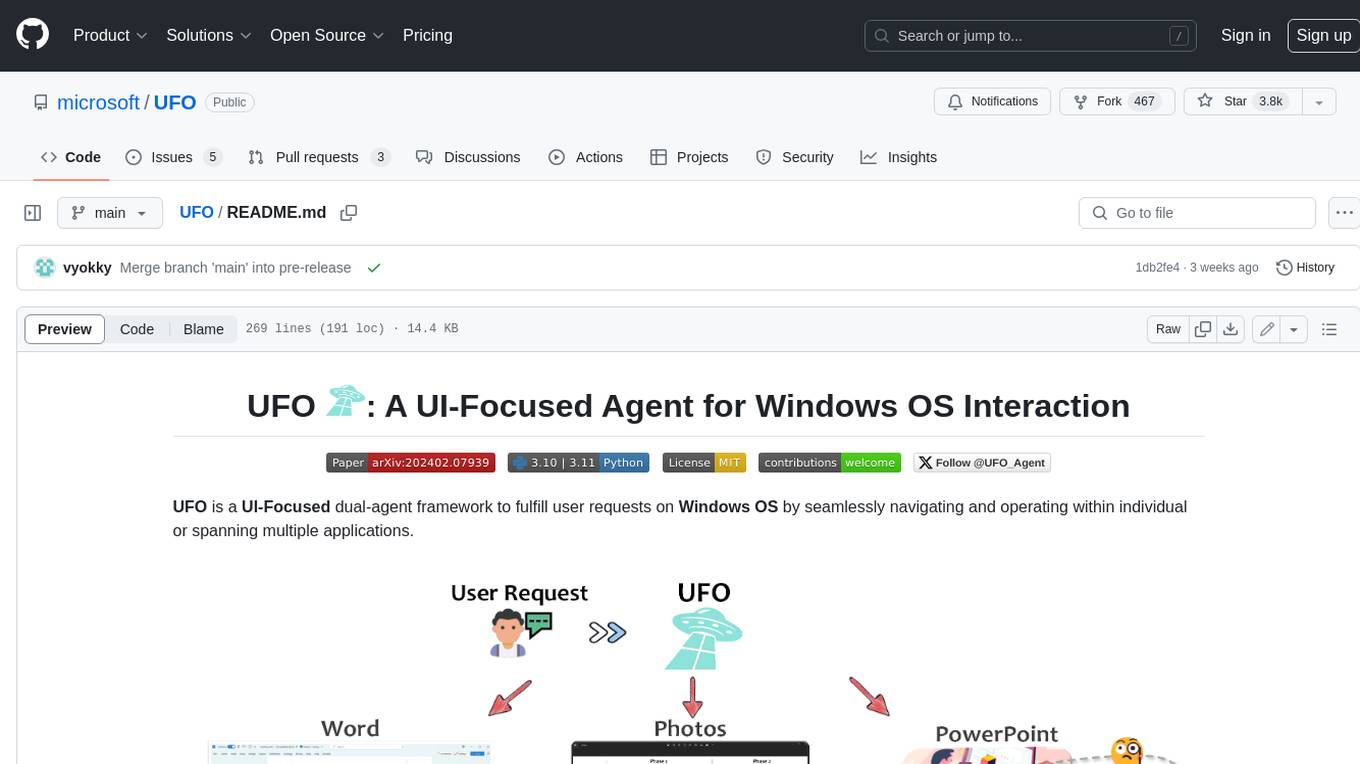
UFO
UFO is a UI-focused dual-agent framework to fulfill user requests on Windows OS by seamlessly navigating and operating within individual or spanning multiple applications.
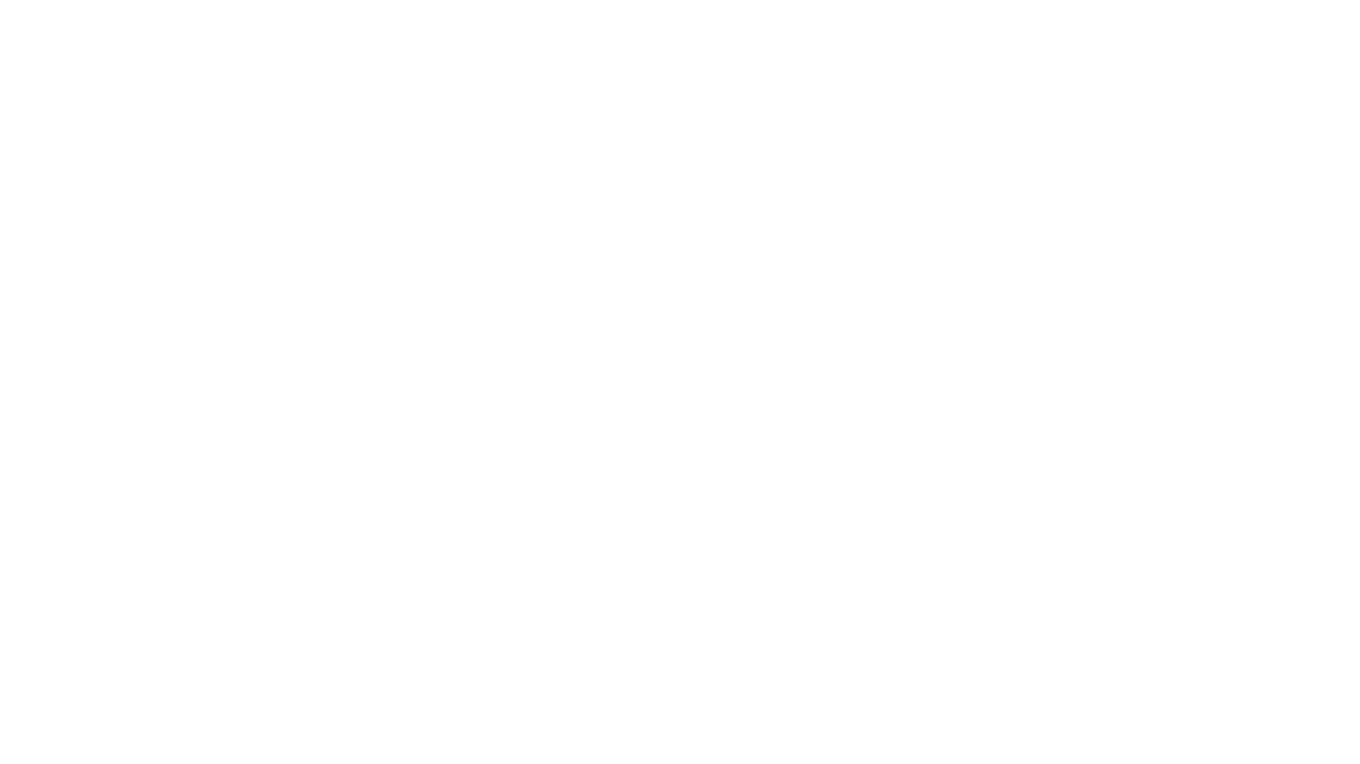
motia
Motia is an AI agent framework designed for software engineers to create, test, and deploy production-ready AI agents quickly. It provides a code-first approach, allowing developers to write agent logic in familiar languages and visualize execution in real-time. With Motia, developers can focus on business logic rather than infrastructure, offering zero infrastructure headaches, multi-language support, composable steps, built-in observability, instant APIs, and full control over AI logic. Ideal for building sophisticated agents and intelligent automations, Motia's event-driven architecture and modular steps enable the creation of GenAI-powered workflows, decision-making systems, and data processing pipelines.
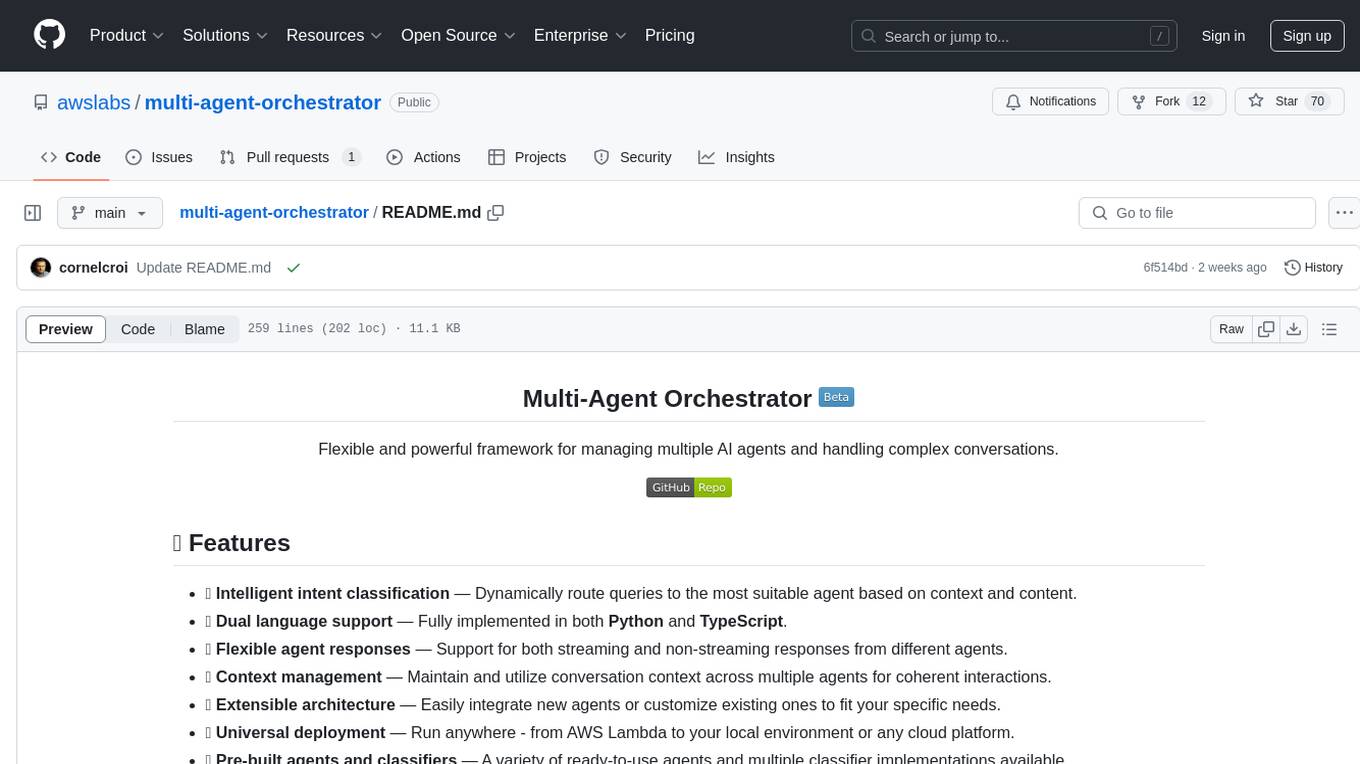
multi-agent-orchestrator
Multi-Agent Orchestrator is a flexible and powerful framework for managing multiple AI agents and handling complex conversations. It intelligently routes queries to the most suitable agent based on context and content, supports dual language implementation in Python and TypeScript, offers flexible agent responses, context management across agents, extensible architecture for customization, universal deployment options, and pre-built agents and classifiers. It is suitable for various applications, from simple chatbots to sophisticated AI systems, accommodating diverse requirements and scaling efficiently.
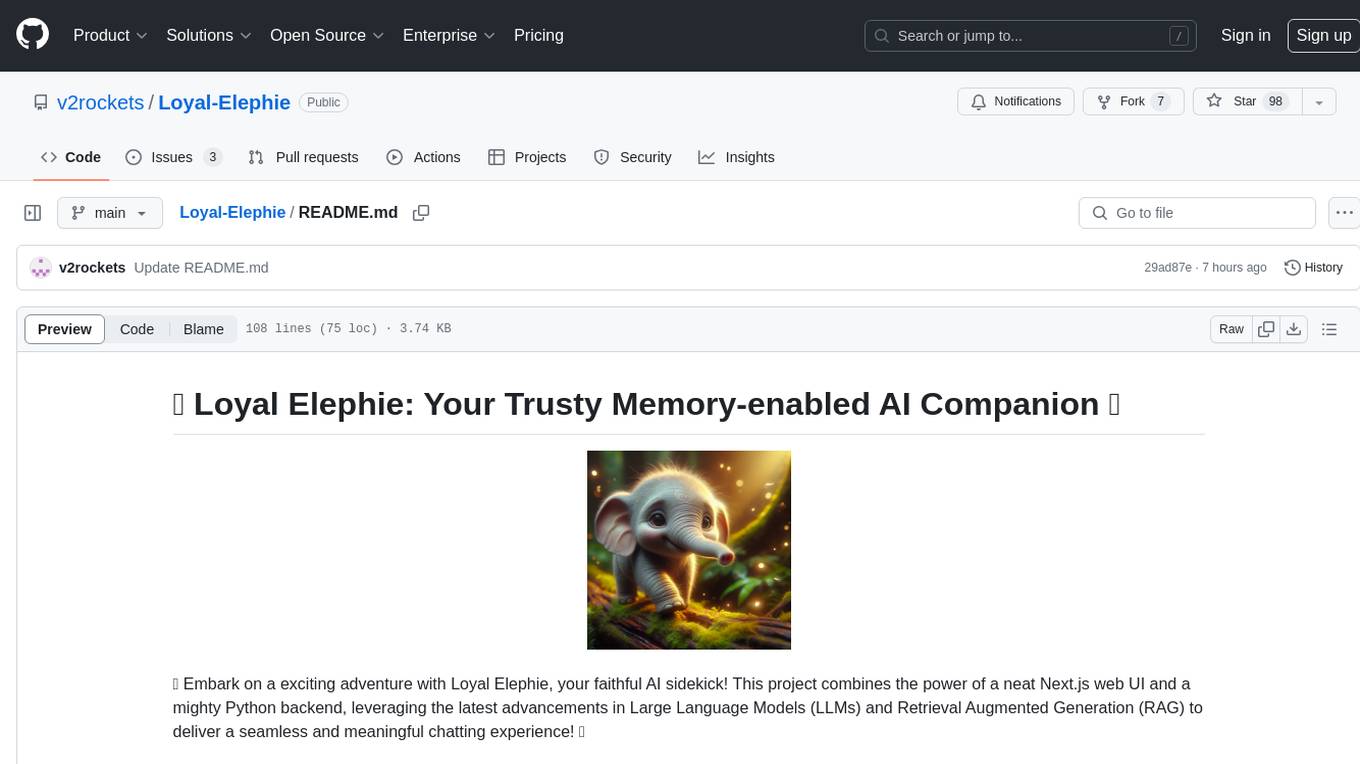
Loyal-Elephie
Embark on an exciting adventure with Loyal Elephie, your faithful AI sidekick! This project combines the power of a neat Next.js web UI and a mighty Python backend, leveraging the latest advancements in Large Language Models (LLMs) and Retrieval Augmented Generation (RAG) to deliver a seamless and meaningful chatting experience. Features include controllable memory, hybrid search, secure web access, streamlined LLM agent, and optional Markdown editor integration. Loyal Elephie supports both open and proprietary LLMs and embeddings serving as OpenAI compatible APIs.
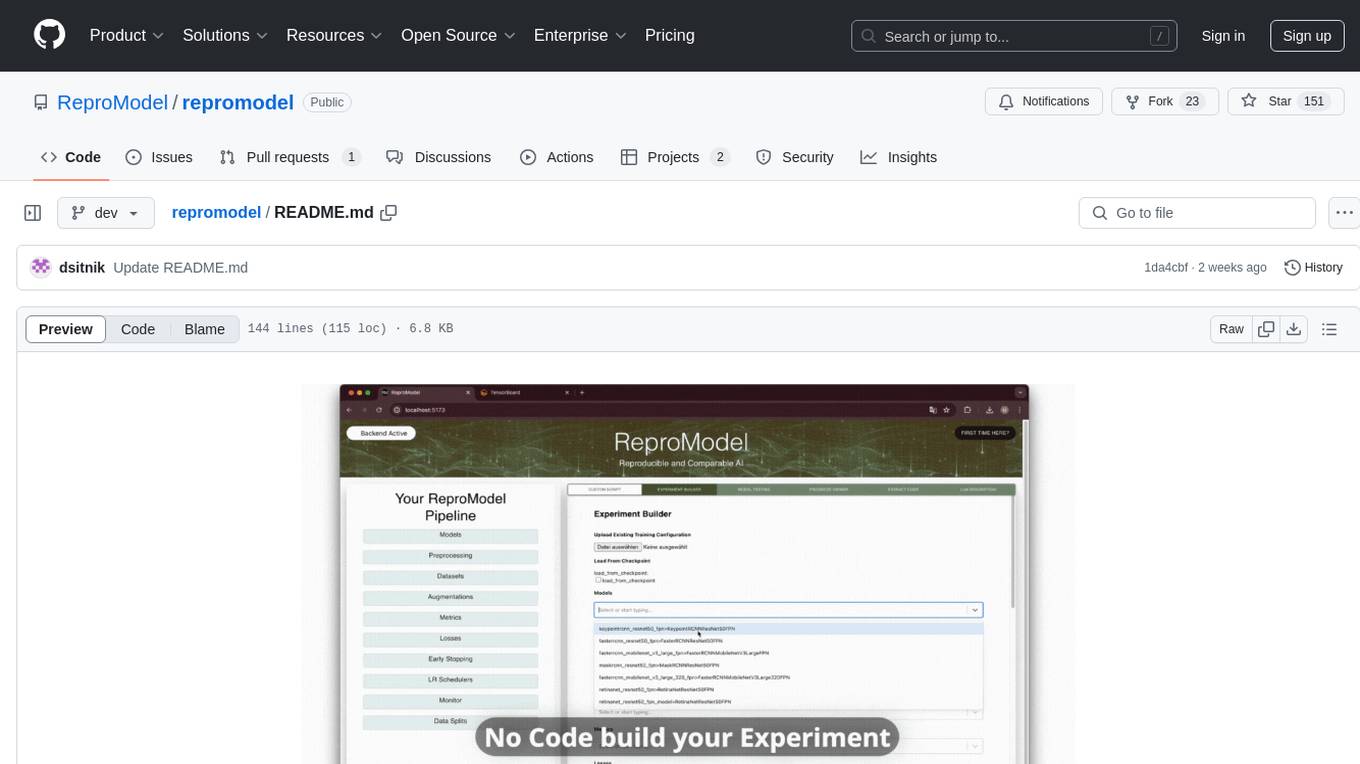
repromodel
ReproModel is an open-source toolbox designed to boost AI research efficiency by enabling researchers to reproduce, compare, train, and test AI models faster. It provides standardized models, dataloaders, and processing procedures, allowing researchers to focus on new datasets and model development. With a no-code solution, users can access benchmark and SOTA models and datasets, utilize training visualizations, extract code for publication, and leverage an LLM-powered automated methodology description writer. The toolbox helps researchers modularize development, compare pipeline performance reproducibly, and reduce time for model development, computation, and writing. Future versions aim to facilitate building upon state-of-the-art research by loading previously published study IDs with verified code, experiments, and results stored in the system.
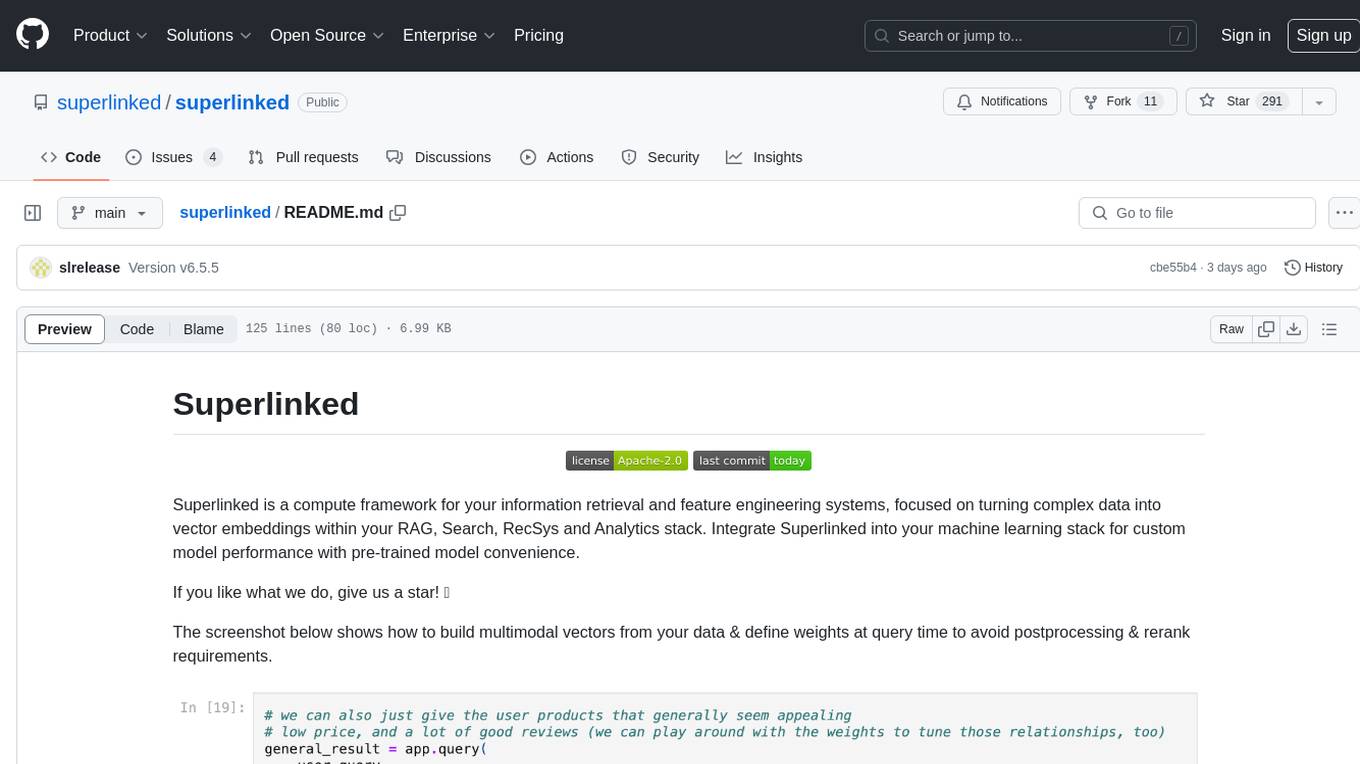
superlinked
Superlinked is a compute framework for information retrieval and feature engineering systems, focusing on converting complex data into vector embeddings for RAG, Search, RecSys, and Analytics stack integration. It enables custom model performance in machine learning with pre-trained model convenience. The tool allows users to build multimodal vectors, define weights at query time, and avoid postprocessing & rerank requirements. Users can explore the computational model through simple scripts and python notebooks, with a future release planned for production usage with built-in data infra and vector database integrations.
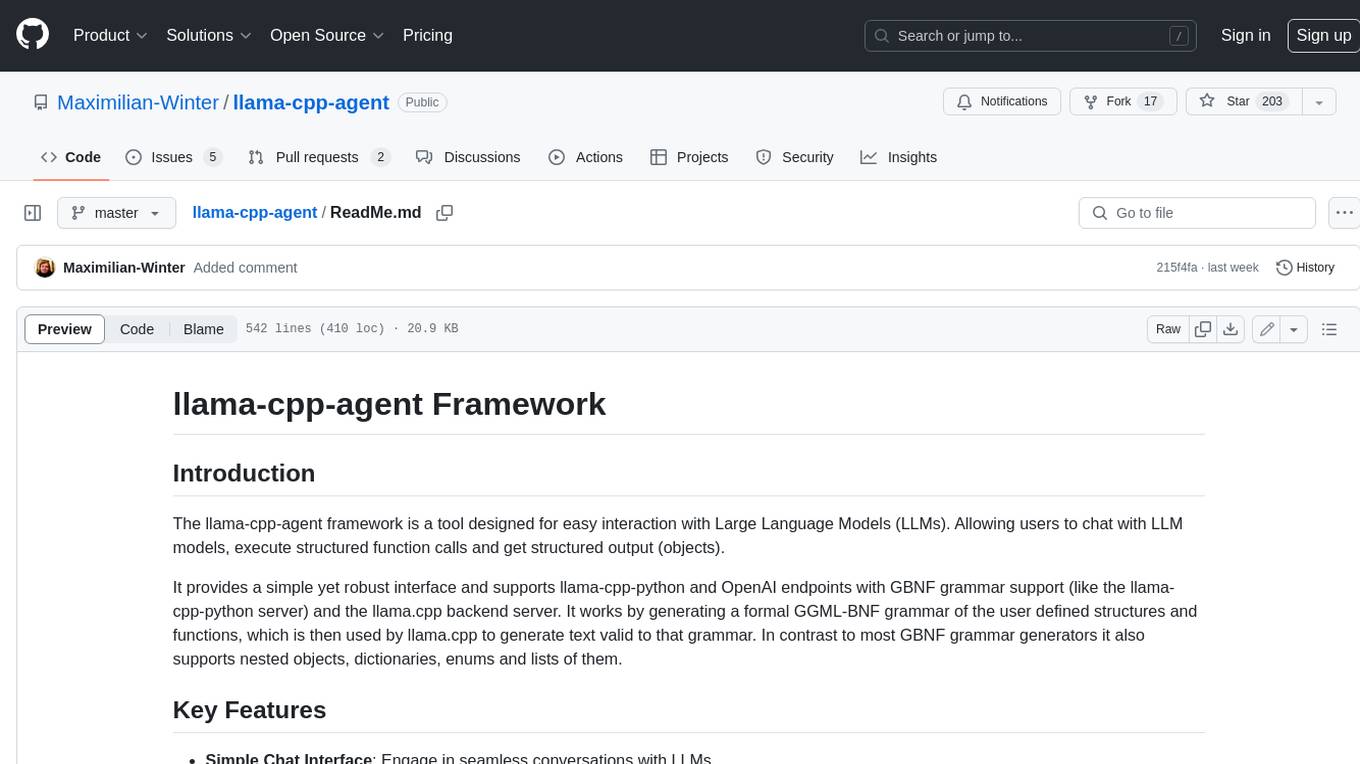
llama-cpp-agent
The llama-cpp-agent framework is a tool designed for easy interaction with Large Language Models (LLMs). Allowing users to chat with LLM models, execute structured function calls and get structured output (objects). It provides a simple yet robust interface and supports llama-cpp-python and OpenAI endpoints with GBNF grammar support (like the llama-cpp-python server) and the llama.cpp backend server. It works by generating a formal GGML-BNF grammar of the user defined structures and functions, which is then used by llama.cpp to generate text valid to that grammar. In contrast to most GBNF grammar generators it also supports nested objects, dictionaries, enums and lists of them.
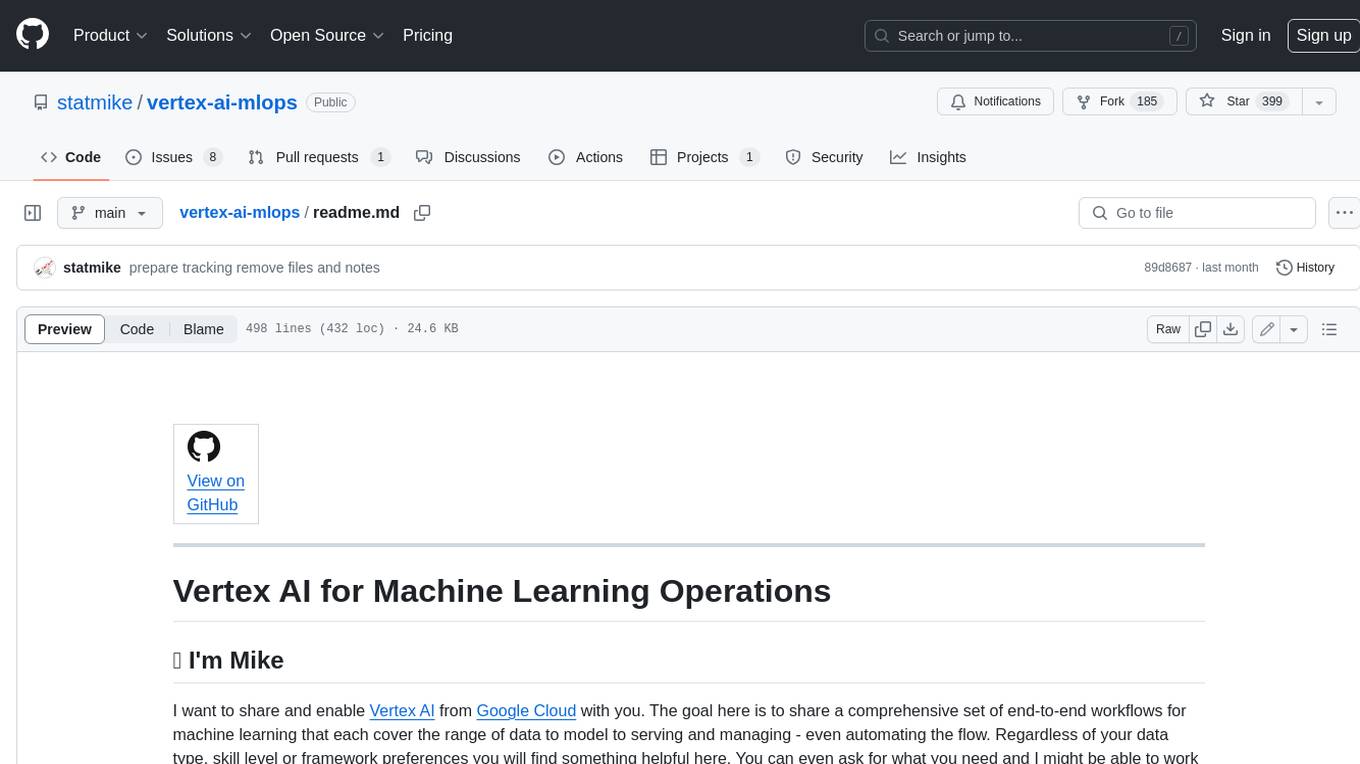
vertex-ai-mlops
Vertex AI is a platform for end-to-end model development. It consist of core components that make the processes of MLOps possible for design patterns of all types.
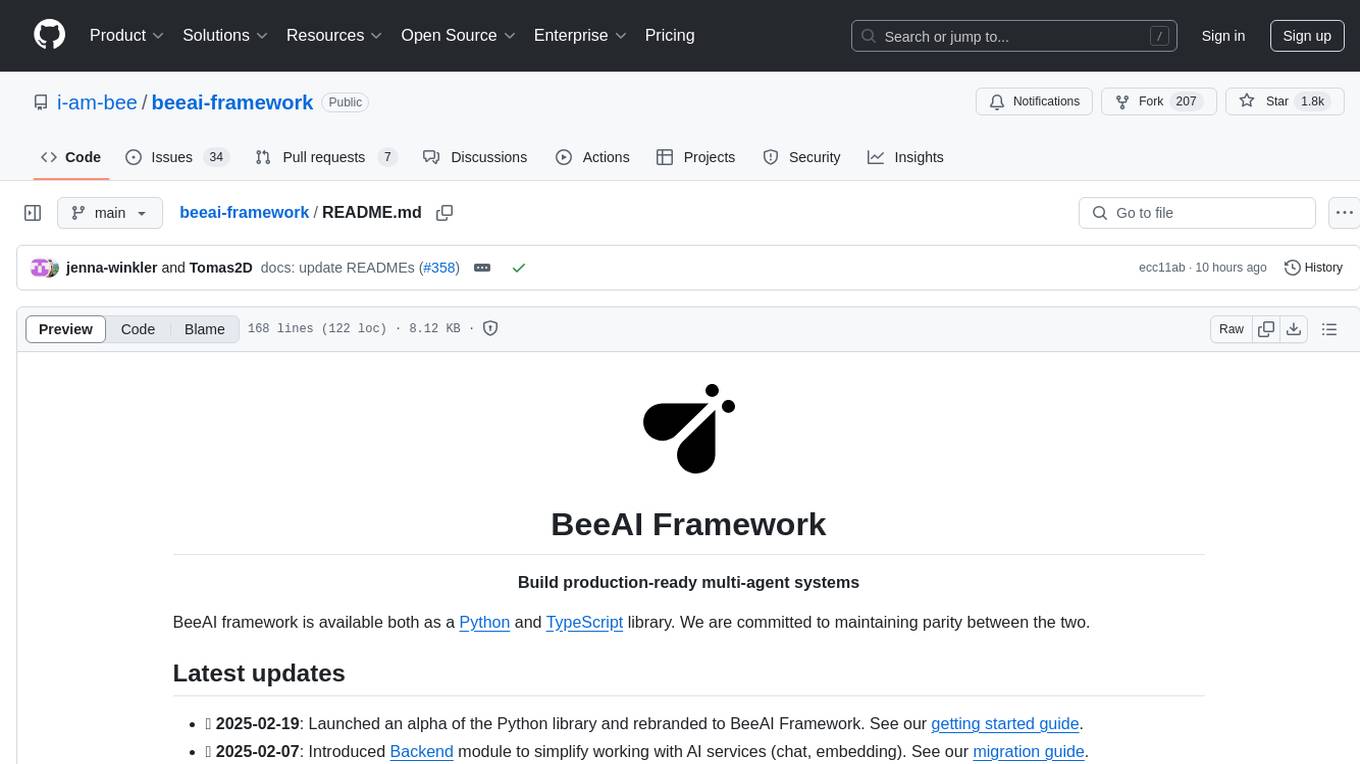
beeai-framework
BeeAI Framework is a versatile tool for building production-ready multi-agent systems. It offers flexibility in orchestrating agents, seamless integration with various models and tools, and production-grade controls for scaling. The framework supports Python and TypeScript libraries, enabling users to implement simple to complex multi-agent patterns, connect with AI services, and optimize token usage and resource management.
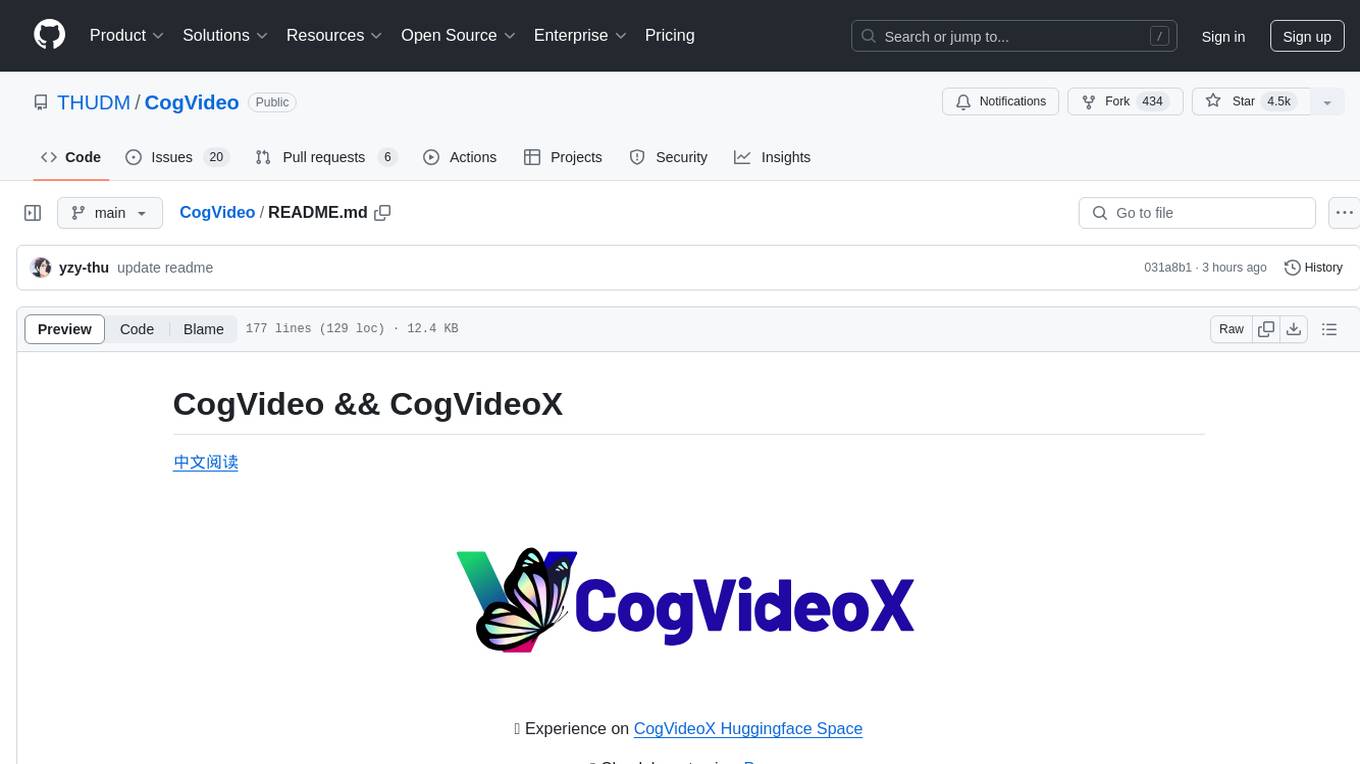
CogVideo
CogVideo is an open-source repository that provides pretrained text-to-video models for generating videos based on input text. It includes models like CogVideoX-2B and CogVideo, offering powerful video generation capabilities. The repository offers tools for inference, fine-tuning, and model conversion, along with demos showcasing the model's capabilities through CLI, web UI, and online experiences. CogVideo aims to facilitate the creation of high-quality videos from textual descriptions, catering to a wide range of applications.
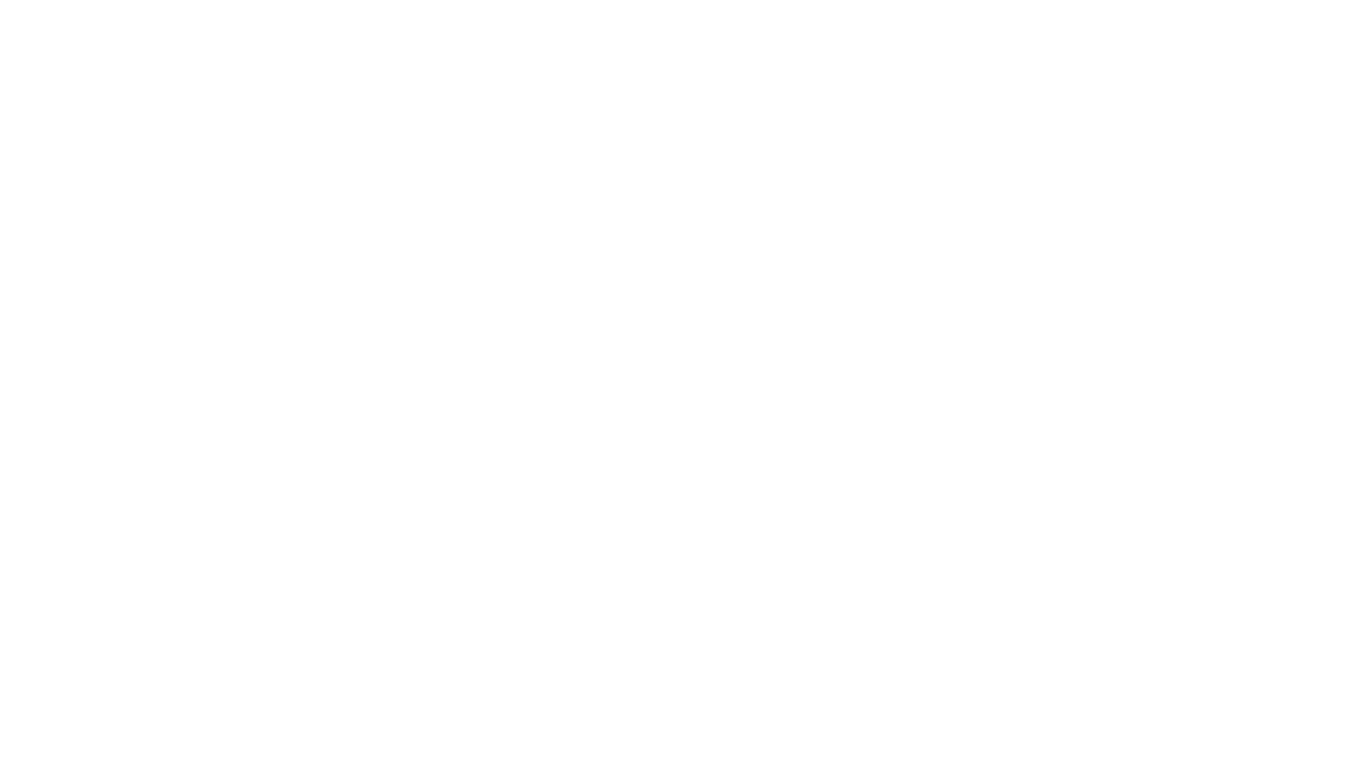
LinguaHaru
Next-generation AI translation tool that provides high-quality, precise translations for various common file formats with a single click. It is based on cutting-edge large language models, offering exceptional translation quality with minimal operation, supporting multiple document formats and languages. Features include multi-format compatibility, global language translation, one-click rapid translation, flexible translation engines, and LAN sharing for efficient collaborative work.
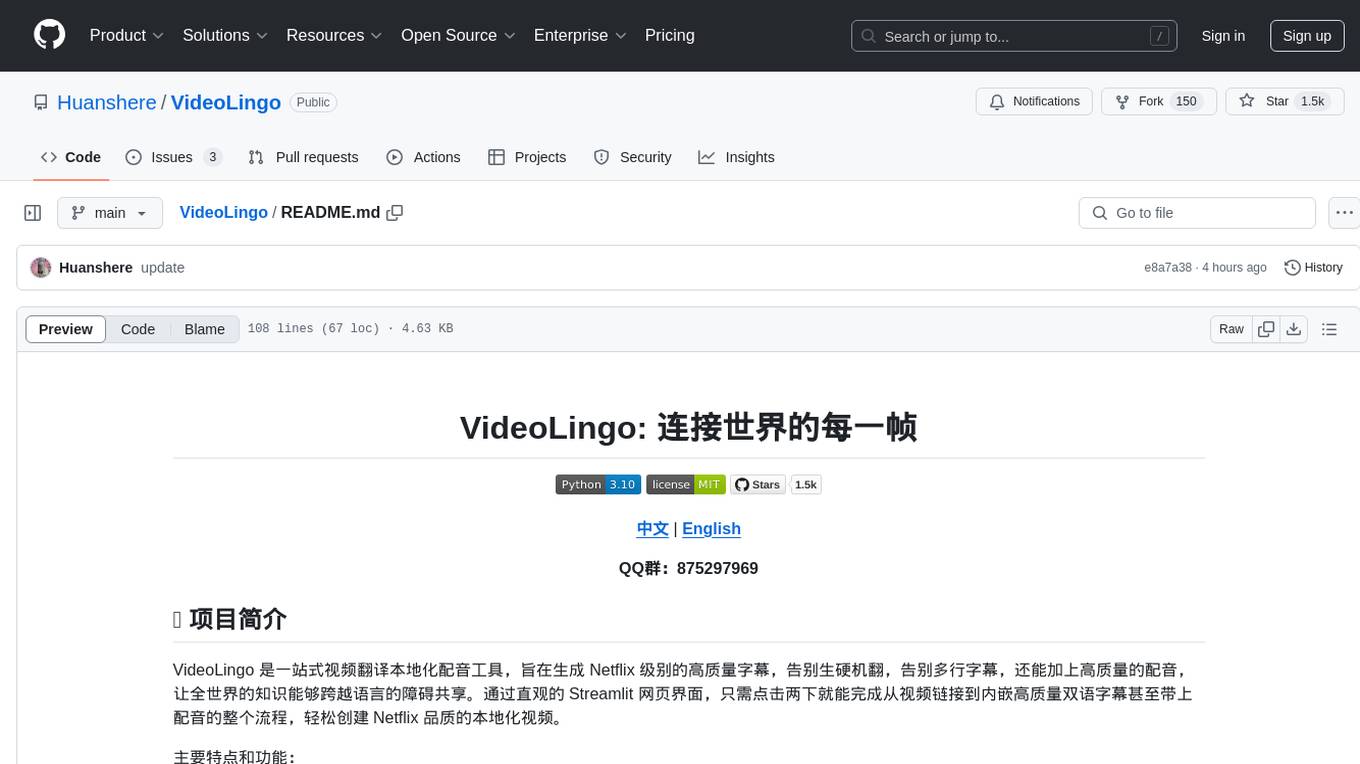
VideoLingo
VideoLingo is an all-in-one video translation and localization dubbing tool designed to generate Netflix-level high-quality subtitles. It aims to eliminate stiff machine translation, multiple lines of subtitles, and can even add high-quality dubbing, allowing knowledge from around the world to be shared across language barriers. Through an intuitive Streamlit web interface, the entire process from video link to embedded high-quality bilingual subtitles and even dubbing can be completed with just two clicks, easily creating Netflix-quality localized videos. Key features and functions include using yt-dlp to download videos from Youtube links, using WhisperX for word-level timeline subtitle recognition, using NLP and GPT for subtitle segmentation based on sentence meaning, summarizing intelligent term knowledge base with GPT for context-aware translation, three-step direct translation, reflection, and free translation to eliminate strange machine translation, checking single-line subtitle length and translation quality according to Netflix standards, using GPT-SoVITS for high-quality aligned dubbing, and integrating package for one-click startup and one-click output in streamlit.
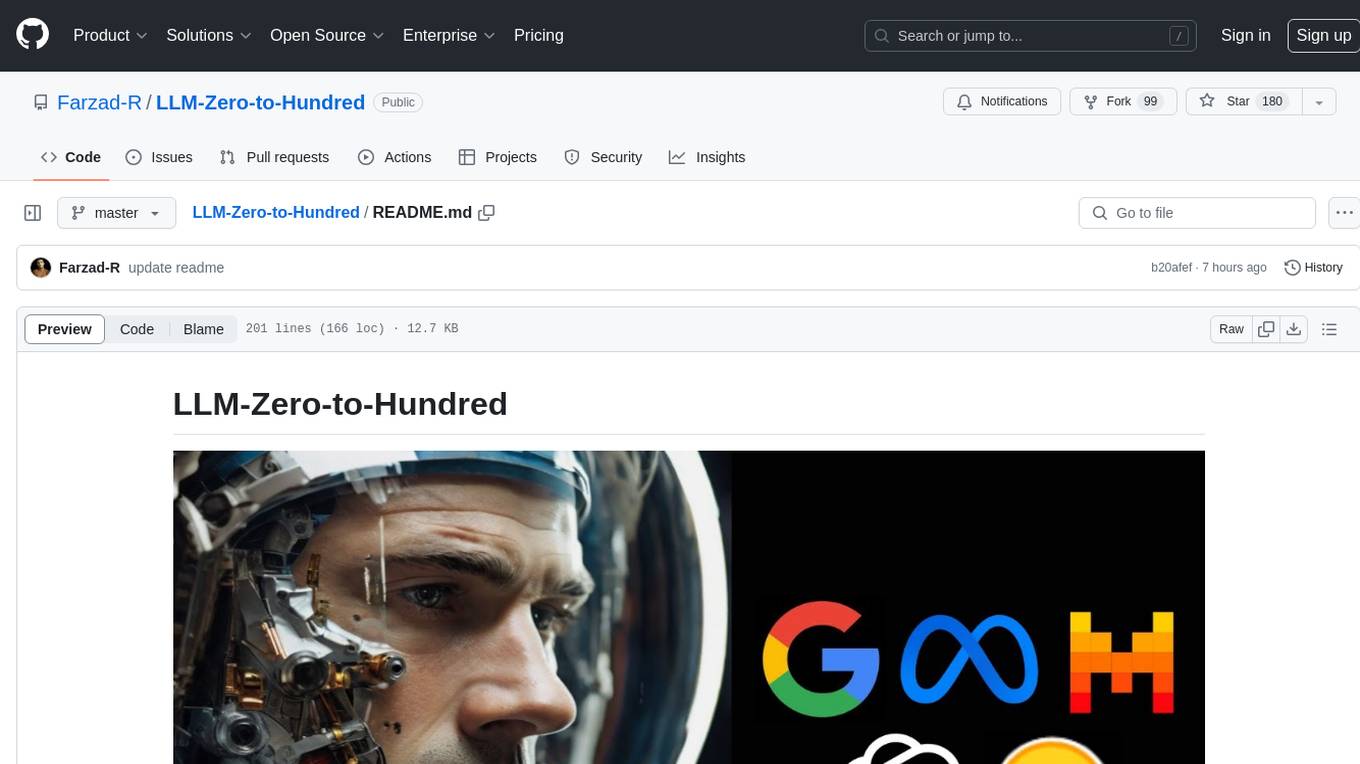
LLM-Zero-to-Hundred
LLM-Zero-to-Hundred is a repository showcasing various applications of LLM chatbots and providing insights into training and fine-tuning Language Models. It includes projects like WebGPT, RAG-GPT, WebRAGQuery, LLM Full Finetuning, RAG-Master LLamaindex vs Langchain, open-source-RAG-GEMMA, and HUMAIN: Advanced Multimodal, Multitask Chatbot. The projects cover features like ChatGPT-like interaction, RAG capabilities, image generation and understanding, DuckDuckGo integration, summarization, text and voice interaction, and memory access. Tutorials include LLM Function Calling and Visualizing Text Vectorization. The projects have a general structure with folders for README, HELPER, .env, configs, data, src, images, and utils.
For similar tasks
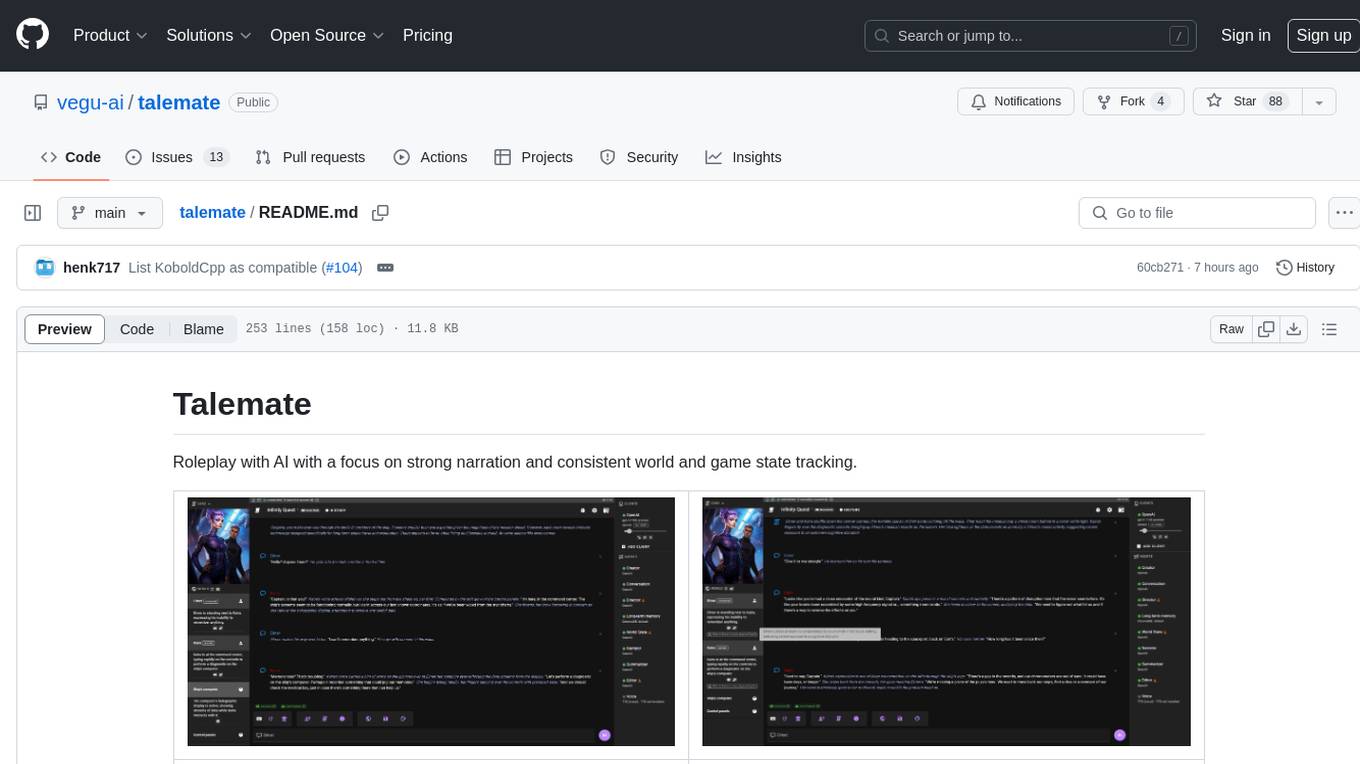
talemate
Talemate is a roleplay tool that allows users to interact with AI agents for dialogue, narration, summarization, direction, editing, world state management, character/scenario creation, text-to-speech, and visual generation. It supports multiple AI clients and APIs, offers long-term memory using ChromaDB, and provides tools for managing NPCs, AI-assisted character creation, and scenario creation. Users can customize prompts using Jinja2 templates and benefit from a modern, responsive UI. The tool also integrates with Runpod for enhanced functionality.
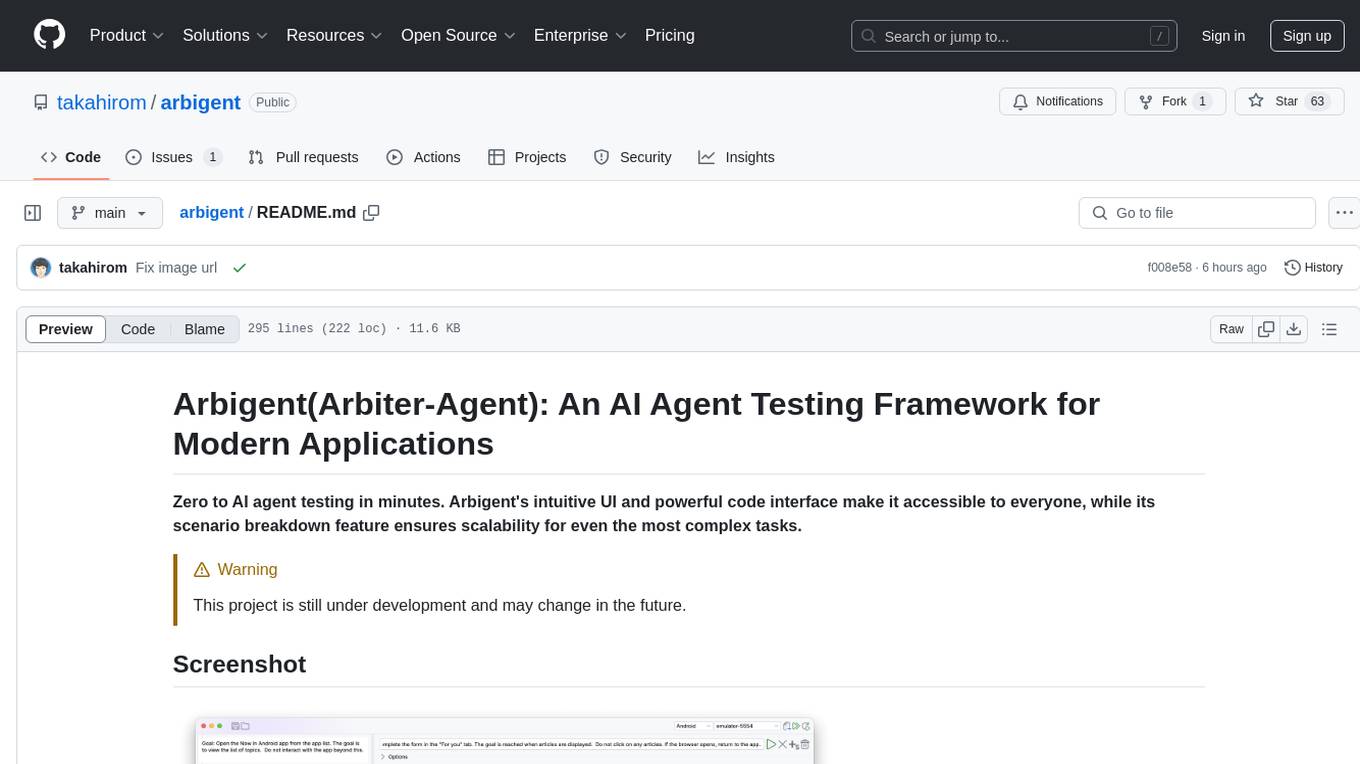
arbigent
Arbigent (Arbiter-Agent) is an AI agent testing framework designed to make AI agent testing practical for modern applications. It addresses challenges faced by traditional UI testing frameworks and AI agents by breaking down complex tasks into smaller, dependent scenarios. The framework is customizable for various AI providers, operating systems, and form factors, empowering users with extensive customization capabilities. Arbigent offers an intuitive UI for scenario creation and a powerful code interface for seamless test execution. It supports multiple form factors, optimizes UI for AI interaction, and is cost-effective by utilizing models like GPT-4o mini. With a flexible code interface and open-source nature, Arbigent aims to revolutionize AI agent testing in modern applications.
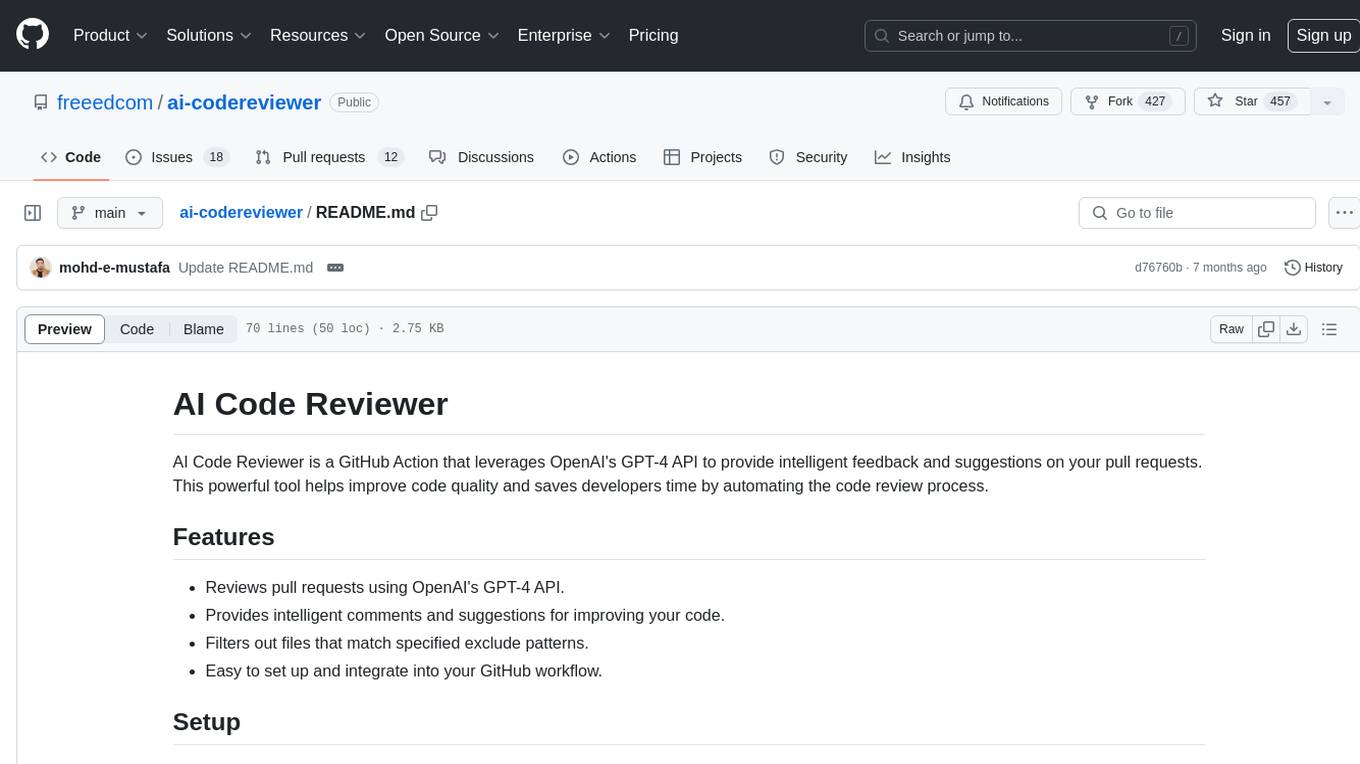
ai-codereviewer
AI Code Reviewer is a GitHub Action that utilizes OpenAI's GPT-4 API to provide intelligent feedback and suggestions on pull requests. It helps enhance code quality and streamline the code review process by offering insightful comments and filtering out specified files. The tool is easy to set up and integrate into GitHub workflows.
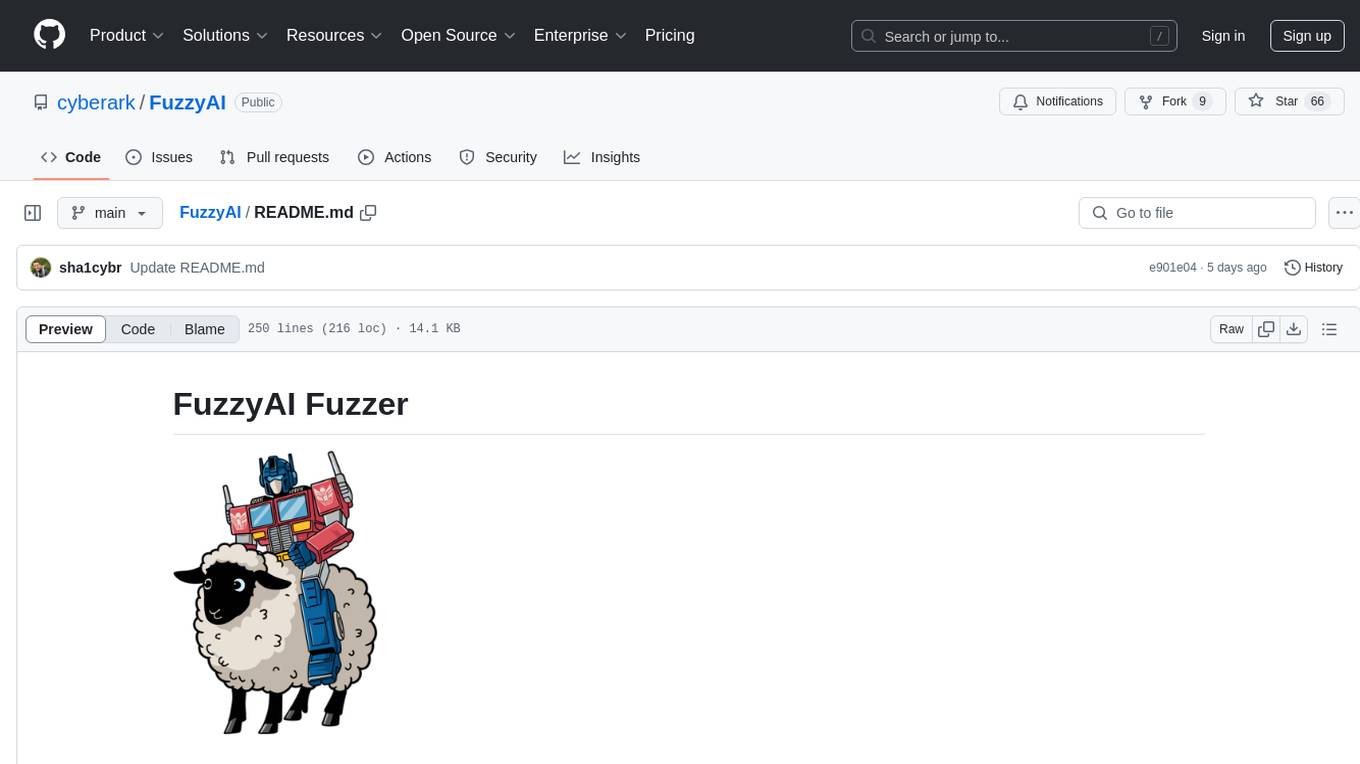
FuzzyAI
The FuzzyAI Fuzzer is a powerful tool for automated LLM fuzzing, designed to help developers and security researchers identify jailbreaks and mitigate potential security vulnerabilities in their LLM APIs. It supports various fuzzing techniques, provides input generation capabilities, can be easily integrated into existing workflows, and offers an extensible architecture for customization and extension. The tool includes attacks like ArtPrompt, Taxonomy-based paraphrasing, Many-shot jailbreaking, Genetic algorithm, Hallucinations, DAN (Do Anything Now), WordGame, Crescendo, ActorAttack, Back To The Past, Please, Thought Experiment, and Default. It supports models from providers like Anthropic, OpenAI, Gemini, Azure, Bedrock, AI21, and Ollama, with the ability to add support for newer models. The tool also supports various cloud APIs and datasets for testing and experimentation.
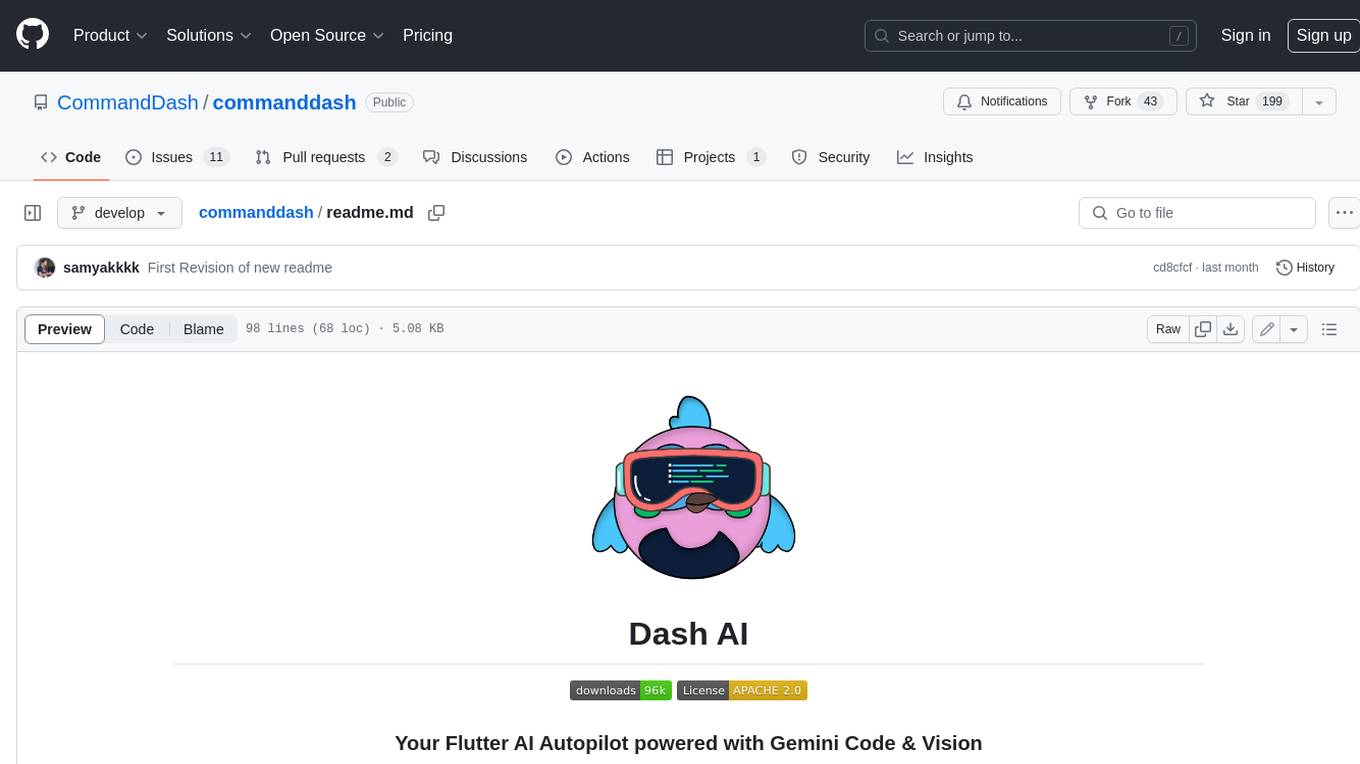
commanddash
Dash AI is an open-source coding assistant for Flutter developers. It is designed to not only write code but also run and debug it, allowing it to assist beyond code completion and automate routine tasks. Dash AI is powered by Gemini, integrated with the Dart Analyzer, and specifically tailored for Flutter engineers. The vision for Dash AI is to create a single-command assistant that can automate tedious development tasks, enabling developers to focus on creativity and innovation. It aims to assist with the entire process of engineering a feature for an app, from breaking down the task into steps to generating exploratory tests and iterating on the code until the feature is complete. To achieve this vision, Dash AI is working on providing LLMs with the same access and information that human developers have, including full contextual knowledge, the latest syntax and dependencies data, and the ability to write, run, and debug code. Dash AI welcomes contributions from the community, including feature requests, issue fixes, and participation in discussions. The project is committed to building a coding assistant that empowers all Flutter developers.
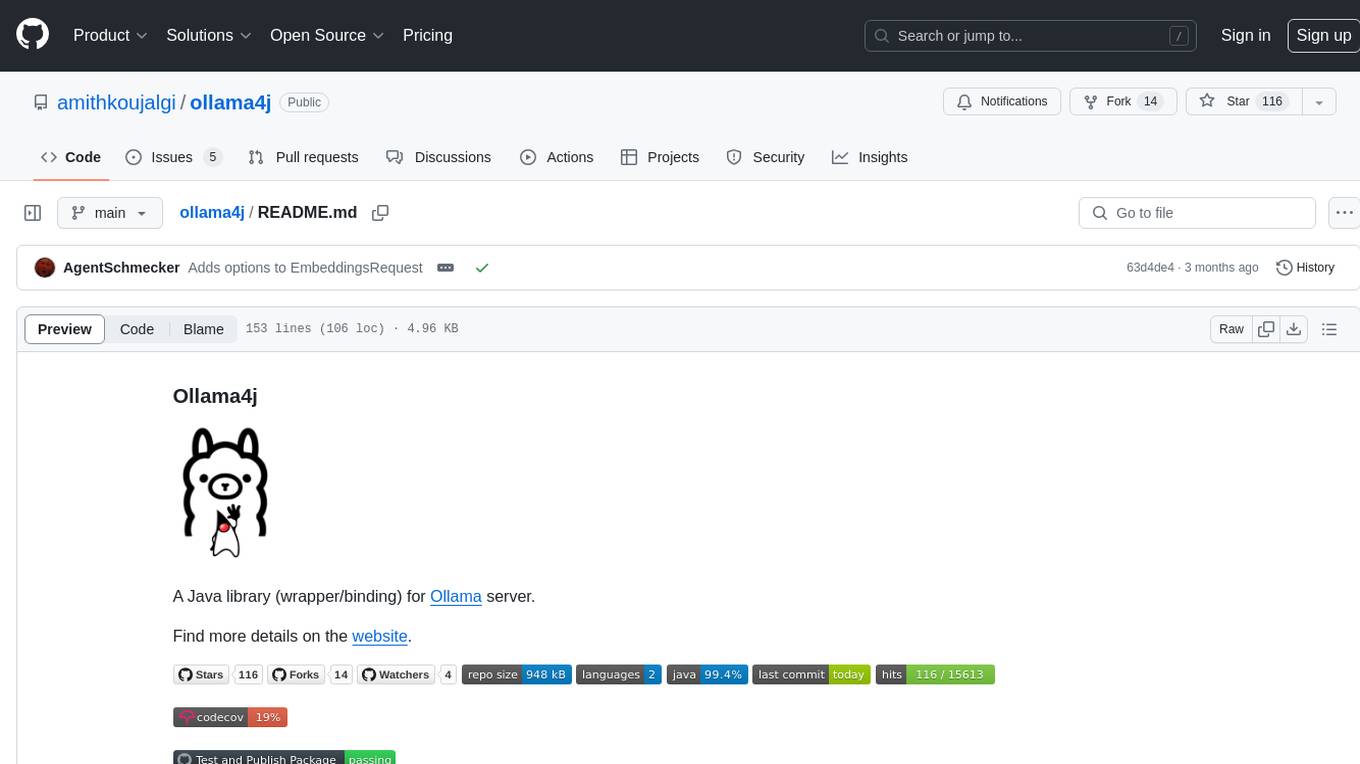
ollama4j
Ollama4j is a Java library that serves as a wrapper or binding for the Ollama server. It facilitates communication with the Ollama server and provides models for deployment. The tool requires Java 11 or higher and can be installed locally or via Docker. Users can integrate Ollama4j into Maven projects by adding the specified dependency. The tool offers API specifications and supports various development tasks such as building, running unit tests, and integration tests. Releases are automated through GitHub Actions CI workflow. Areas of improvement include adhering to Java naming conventions, updating deprecated code, implementing logging, using lombok, and enhancing request body creation. Contributions to the project are encouraged, whether reporting bugs, suggesting enhancements, or contributing code.
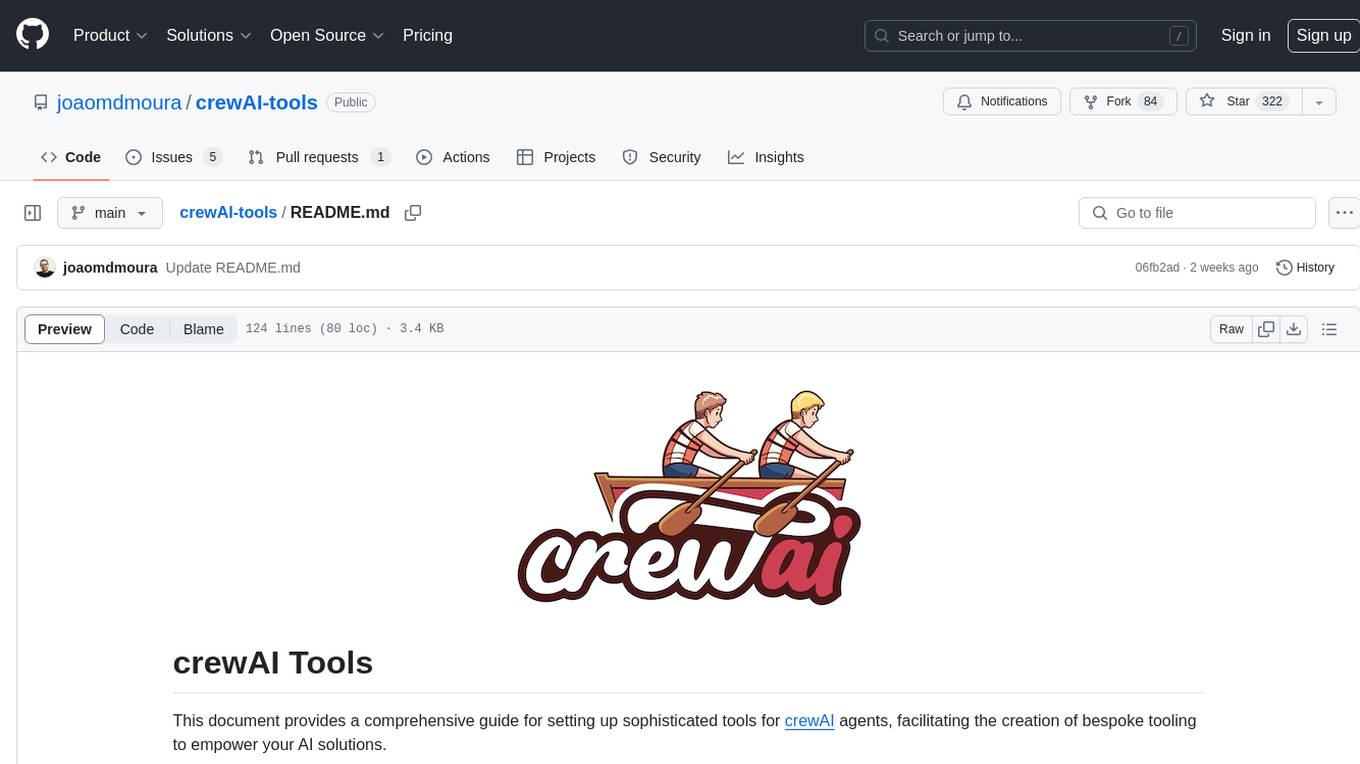
crewAI-tools
The crewAI Tools repository provides a guide for setting up tools for crewAI agents, enabling the creation of custom tools to enhance AI solutions. Tools play a crucial role in improving agent functionality. The guide explains how to equip agents with a range of tools and how to create new tools. Tools are designed to return strings for generating responses. There are two main methods for creating tools: subclassing BaseTool and using the tool decorator. Contributions to the toolset are encouraged, and the development setup includes steps for installing dependencies, activating the virtual environment, setting up pre-commit hooks, running tests, static type checking, packaging, and local installation. Enhance AI agent capabilities with advanced tooling.
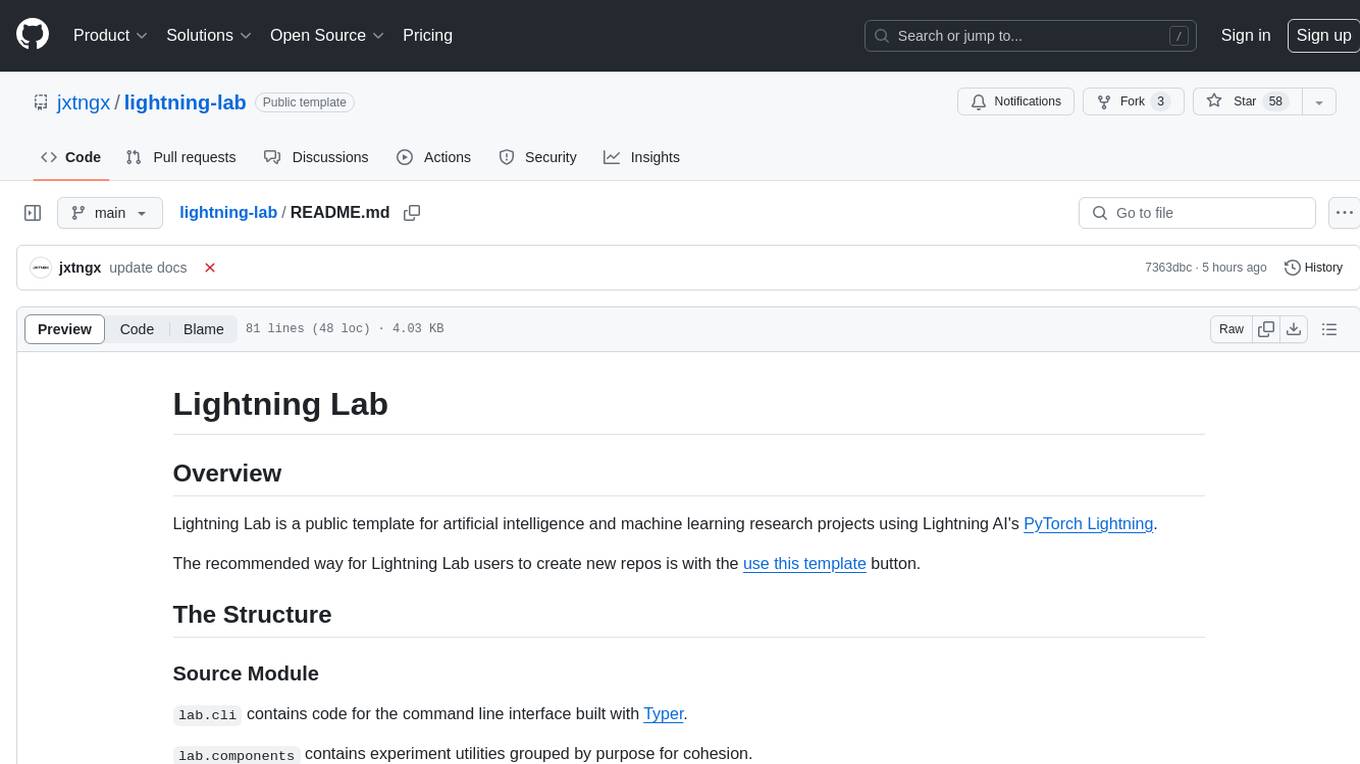
lightning-lab
Lightning Lab is a public template for artificial intelligence and machine learning research projects using Lightning AI's PyTorch Lightning. It provides a structured project layout with modules for command line interface, experiment utilities, Lightning Module and Trainer, data acquisition and preprocessing, model serving APIs, project configurations, training checkpoints, technical documentation, logs, notebooks for data analysis, requirements management, testing, and packaging. The template simplifies the setup of deep learning projects and offers extras for different domains like vision, text, audio, reinforcement learning, and forecasting.
For similar jobs
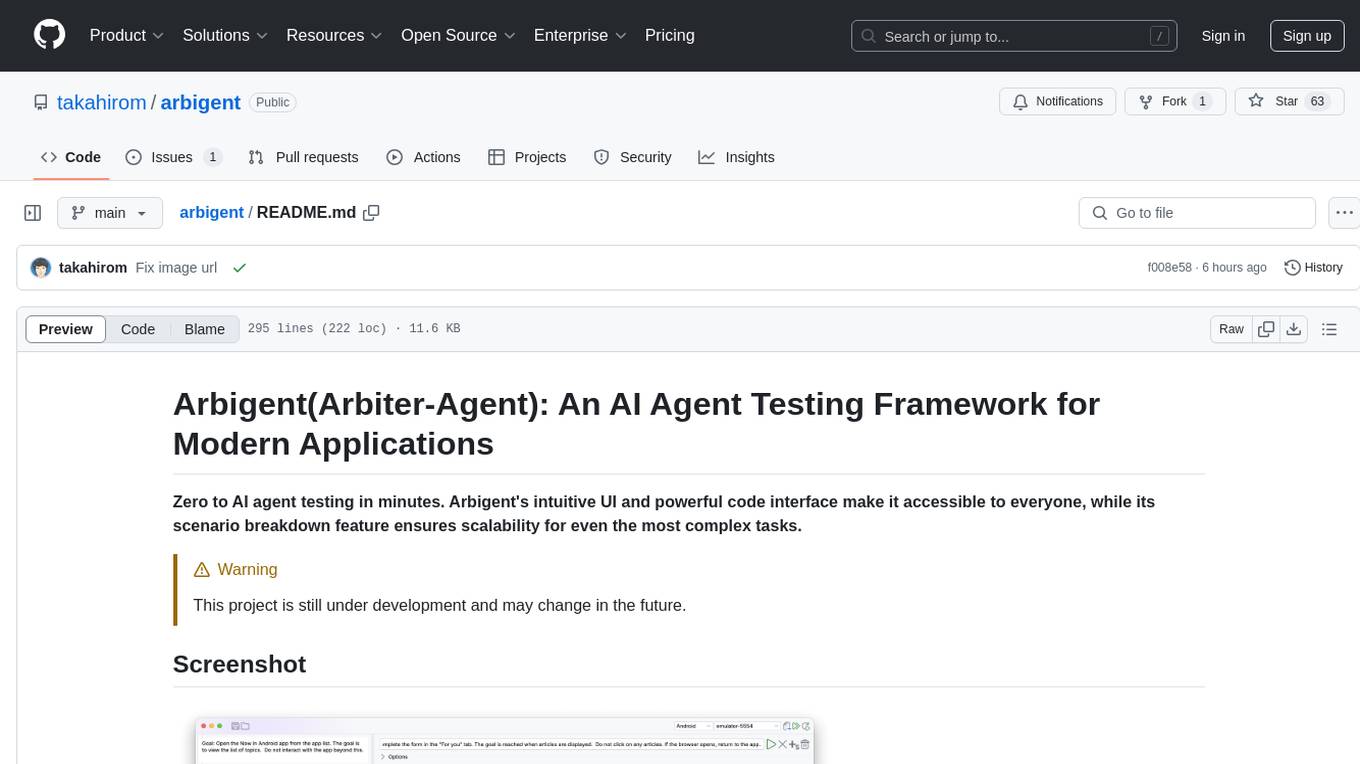
arbigent
Arbigent (Arbiter-Agent) is an AI agent testing framework designed to make AI agent testing practical for modern applications. It addresses challenges faced by traditional UI testing frameworks and AI agents by breaking down complex tasks into smaller, dependent scenarios. The framework is customizable for various AI providers, operating systems, and form factors, empowering users with extensive customization capabilities. Arbigent offers an intuitive UI for scenario creation and a powerful code interface for seamless test execution. It supports multiple form factors, optimizes UI for AI interaction, and is cost-effective by utilizing models like GPT-4o mini. With a flexible code interface and open-source nature, Arbigent aims to revolutionize AI agent testing in modern applications.
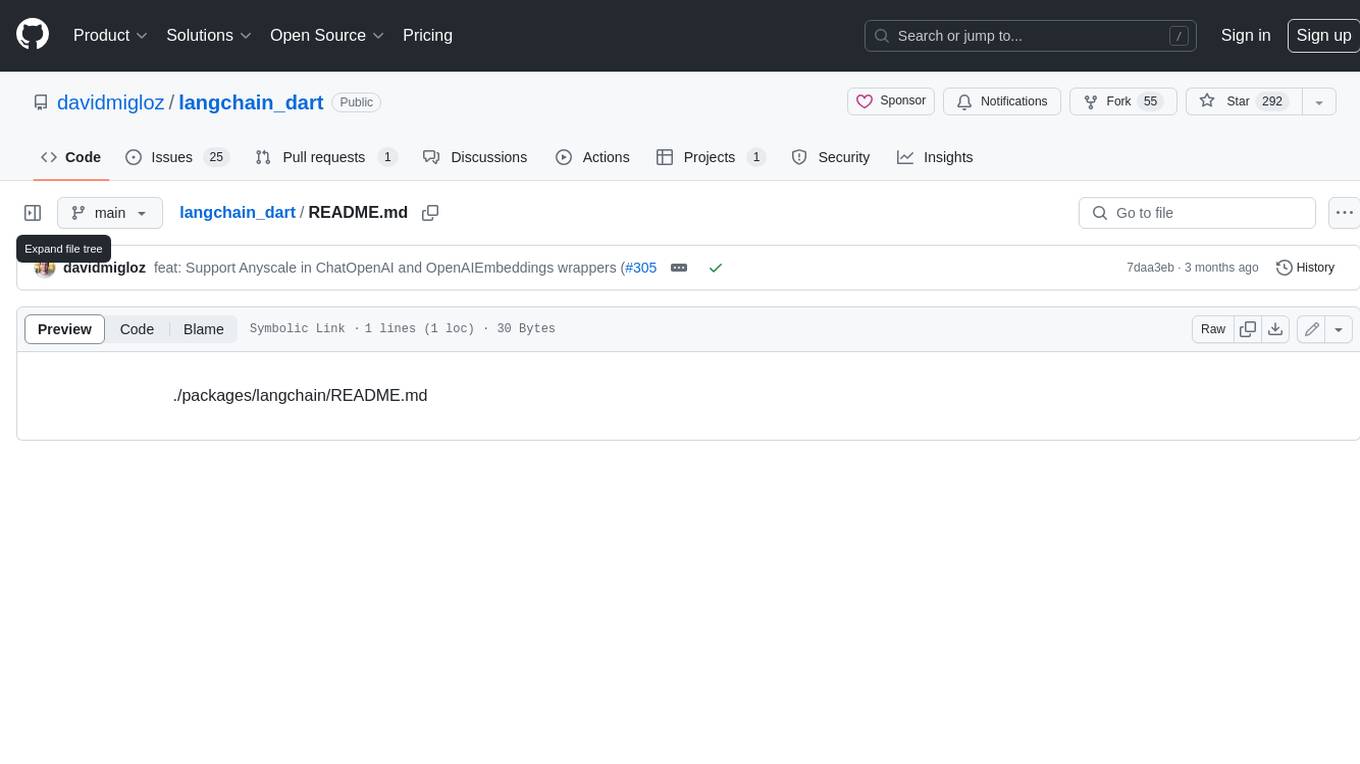
langchain_dart
LangChain.dart is a Dart port of the popular LangChain Python framework created by Harrison Chase. LangChain provides a set of ready-to-use components for working with language models and a standard interface for chaining them together to formulate more advanced use cases (e.g. chatbots, Q&A with RAG, agents, summarization, extraction, etc.). The components can be grouped into a few core modules: * **Model I/O:** LangChain offers a unified API for interacting with various LLM providers (e.g. OpenAI, Google, Mistral, Ollama, etc.), allowing developers to switch between them with ease. Additionally, it provides tools for managing model inputs (prompt templates and example selectors) and parsing the resulting model outputs (output parsers). * **Retrieval:** assists in loading user data (via document loaders), transforming it (with text splitters), extracting its meaning (using embedding models), storing (in vector stores) and retrieving it (through retrievers) so that it can be used to ground the model's responses (i.e. Retrieval-Augmented Generation or RAG). * **Agents:** "bots" that leverage LLMs to make informed decisions about which available tools (such as web search, calculators, database lookup, etc.) to use to accomplish the designated task. The different components can be composed together using the LangChain Expression Language (LCEL).
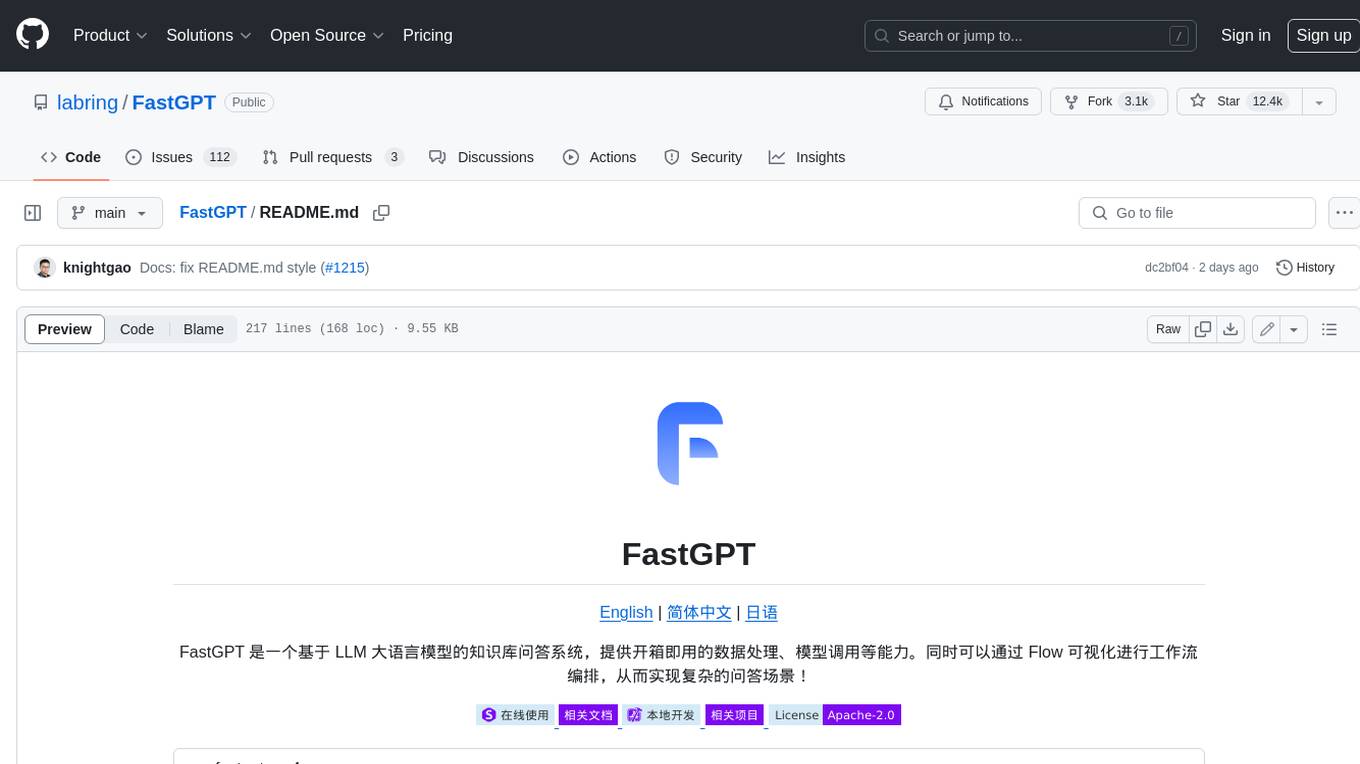
FastGPT
FastGPT is a knowledge base Q&A system based on the LLM large language model, providing out-of-the-box data processing, model calling and other capabilities. At the same time, you can use Flow to visually arrange workflows to achieve complex Q&A scenarios!
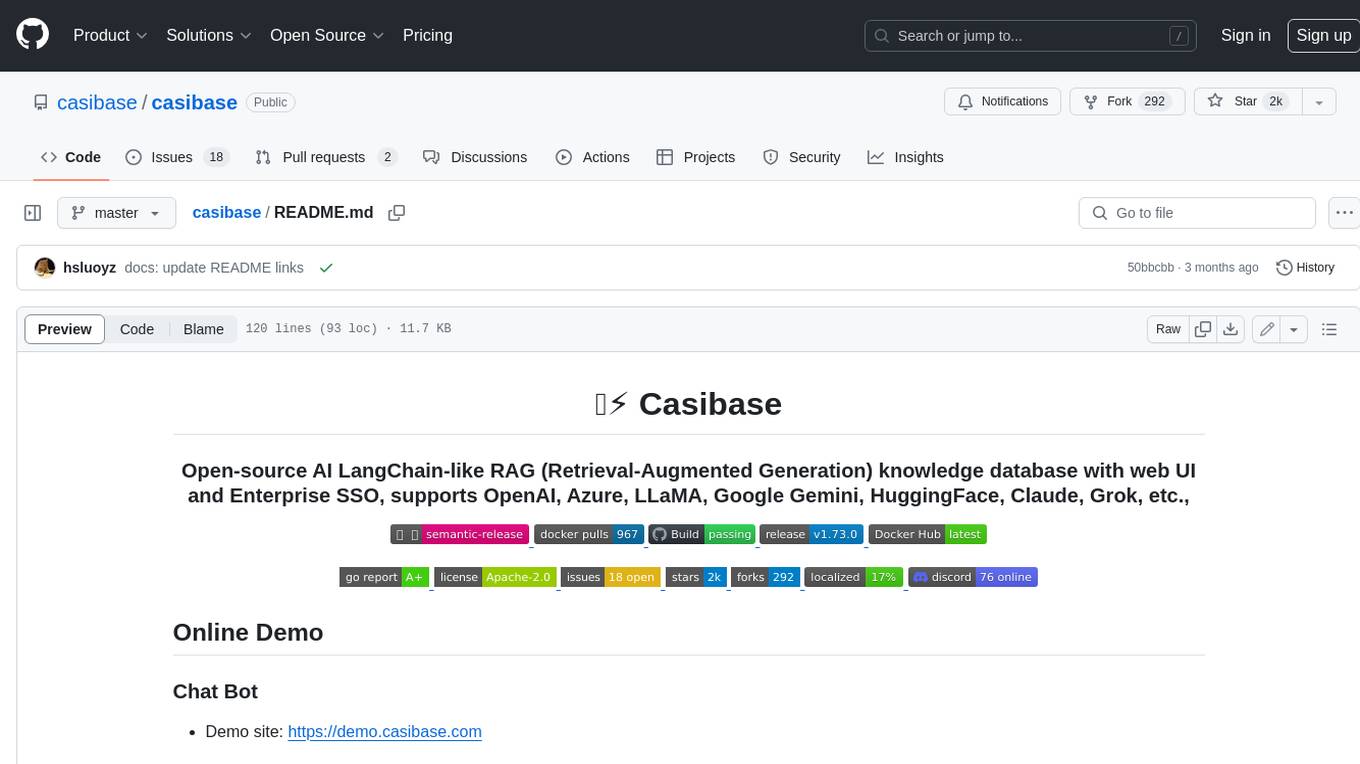
casibase
Casibase is an open-source AI LangChain-like RAG (Retrieval-Augmented Generation) knowledge database with web UI and Enterprise SSO, supports OpenAI, Azure, LLaMA, Google Gemini, HuggingFace, Claude, Grok, etc.
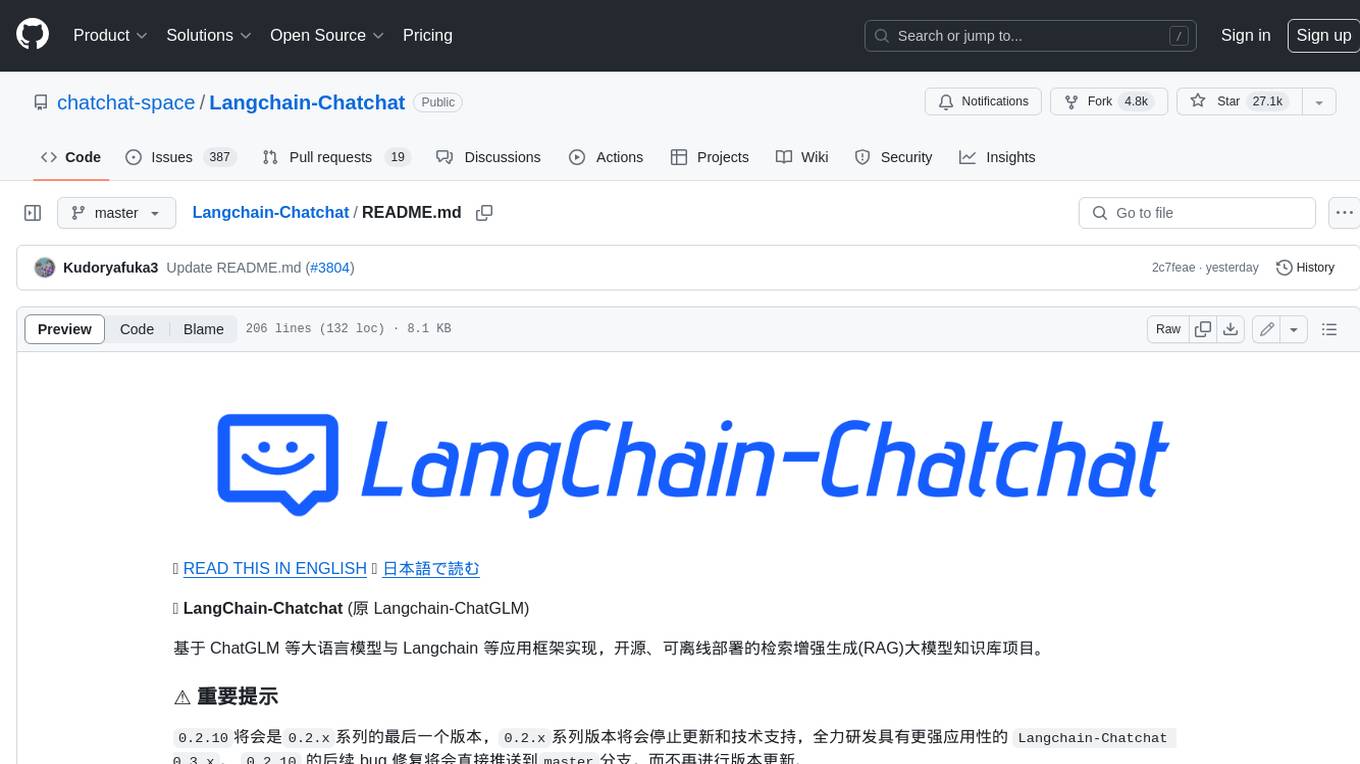
Langchain-Chatchat
LangChain-Chatchat is an open-source, offline-deployable retrieval-enhanced generation (RAG) large model knowledge base project based on large language models such as ChatGLM and application frameworks such as Langchain. It aims to establish a knowledge base Q&A solution that is friendly to Chinese scenarios, supports open-source models, and can run offline.
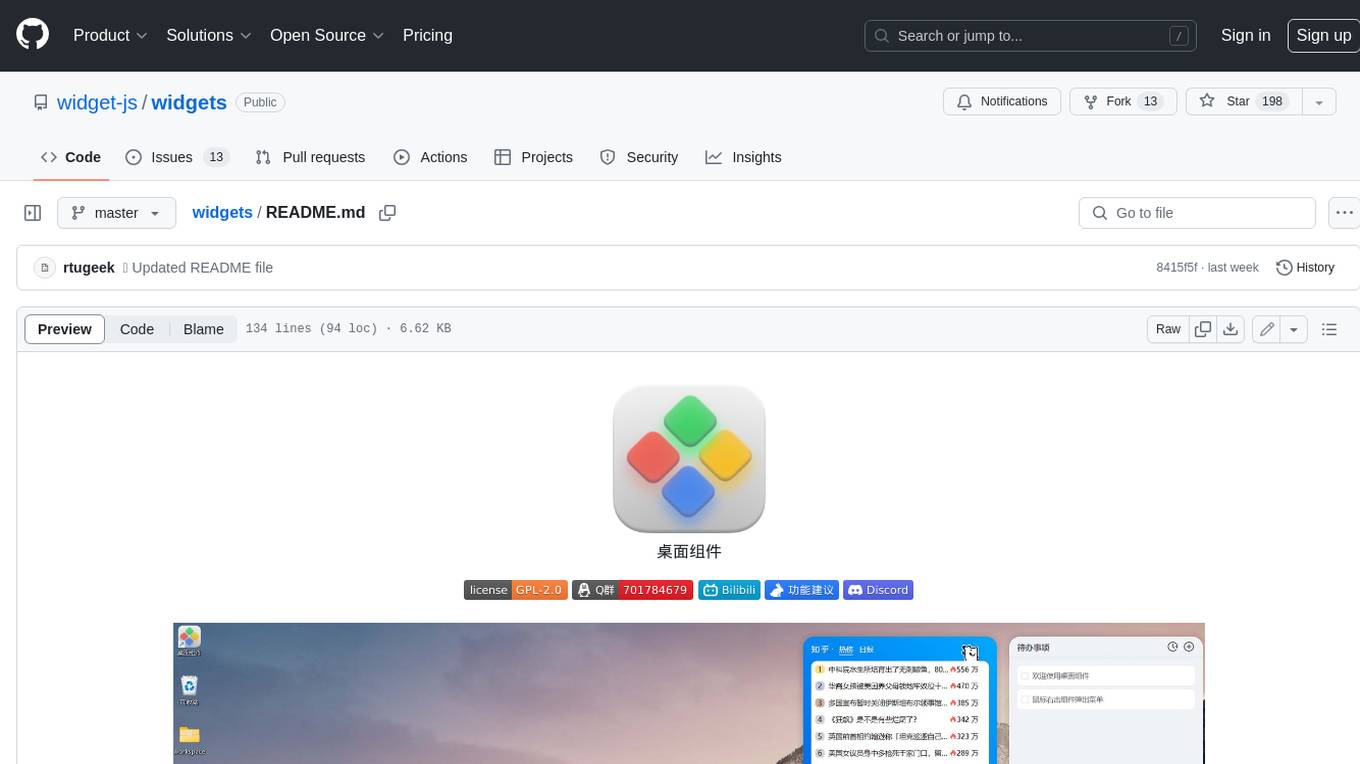
widgets
Widgets is a desktop component front-end open source component. The project is still being continuously improved. The desktop component client can be downloaded and run in two ways: 1. https://www.microsoft.com/store/productId/9NPR50GQ7T53 2. https://widgetjs.cn After cloning the code, you need to download the dependency in the project directory: `shell pnpm install` and run: `shell pnpm serve`
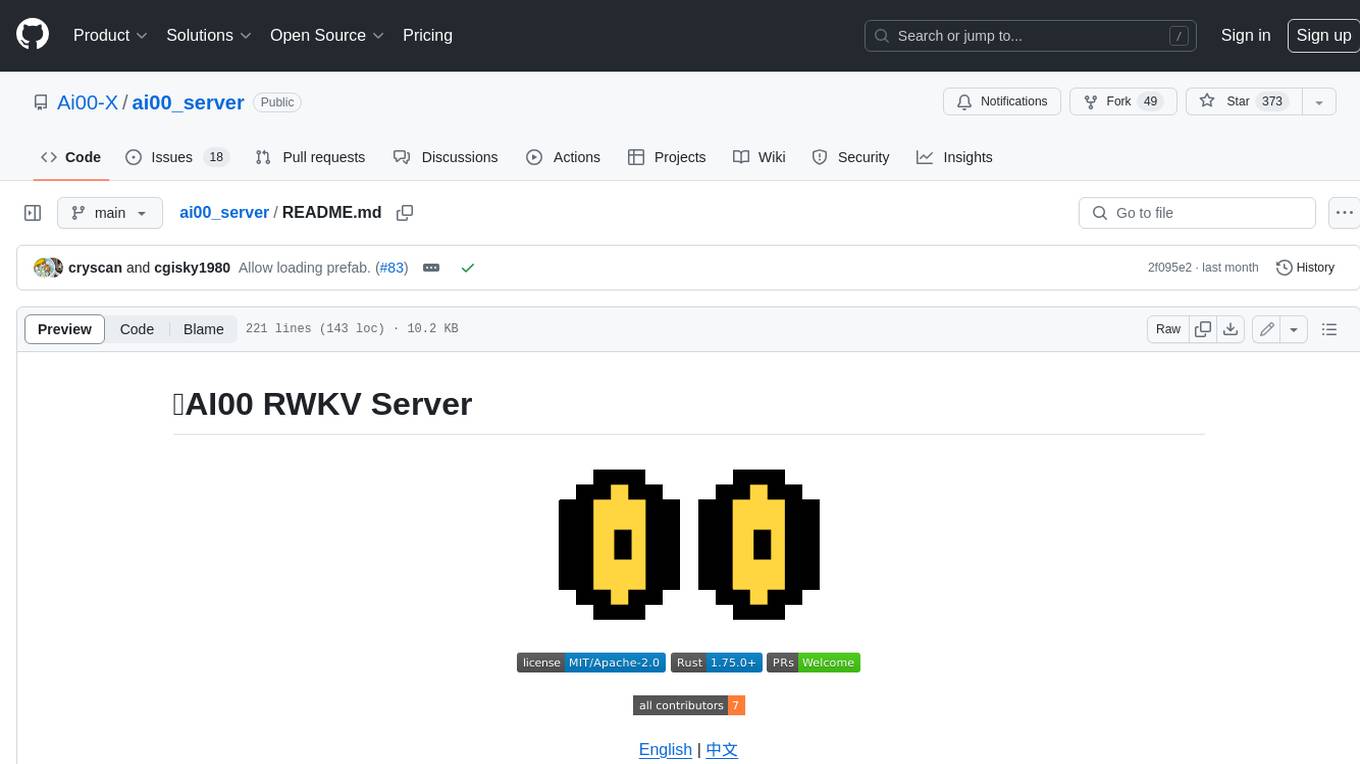
ai00_server
AI00 RWKV Server is an inference API server for the RWKV language model based upon the web-rwkv inference engine. It supports VULKAN parallel and concurrent batched inference and can run on all GPUs that support VULKAN. No need for Nvidia cards!!! AMD cards and even integrated graphics can be accelerated!!! No need for bulky pytorch, CUDA and other runtime environments, it's compact and ready to use out of the box! Compatible with OpenAI's ChatGPT API interface. 100% open source and commercially usable, under the MIT license. If you are looking for a fast, efficient, and easy-to-use LLM API server, then AI00 RWKV Server is your best choice. It can be used for various tasks, including chatbots, text generation, translation, and Q&A.
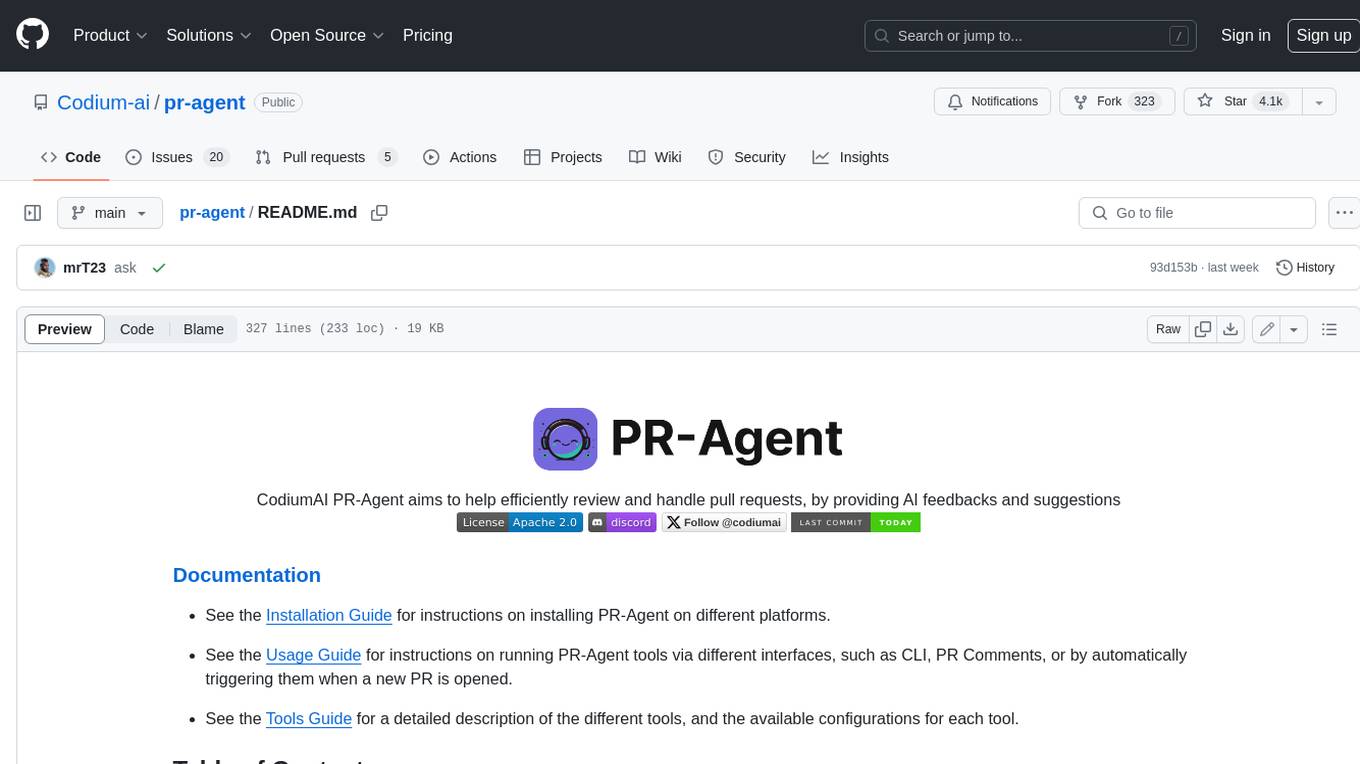
pr-agent
PR-Agent is a tool that helps to efficiently review and handle pull requests by providing AI feedbacks and suggestions. It supports various commands such as generating PR descriptions, providing code suggestions, answering questions about the PR, and updating the CHANGELOG.md file. PR-Agent can be used via CLI, GitHub Action, GitHub App, Docker, and supports multiple git providers and models. It emphasizes real-life practical usage, with each tool having a single GPT-4 call for quick and affordable responses. The PR Compression strategy enables effective handling of both short and long PRs, while the JSON prompting strategy allows for modular and customizable tools. PR-Agent Pro, the hosted version by CodiumAI, provides additional benefits such as full management, improved privacy, priority support, and extra features.