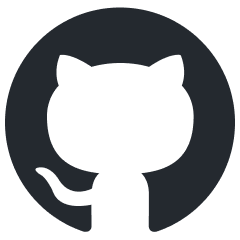
mindnlp
Easy-to-use and high-performance NLP and LLM framework based on MindSpore, compatible with models and datasets of 🤗Huggingface.
Stars: 849
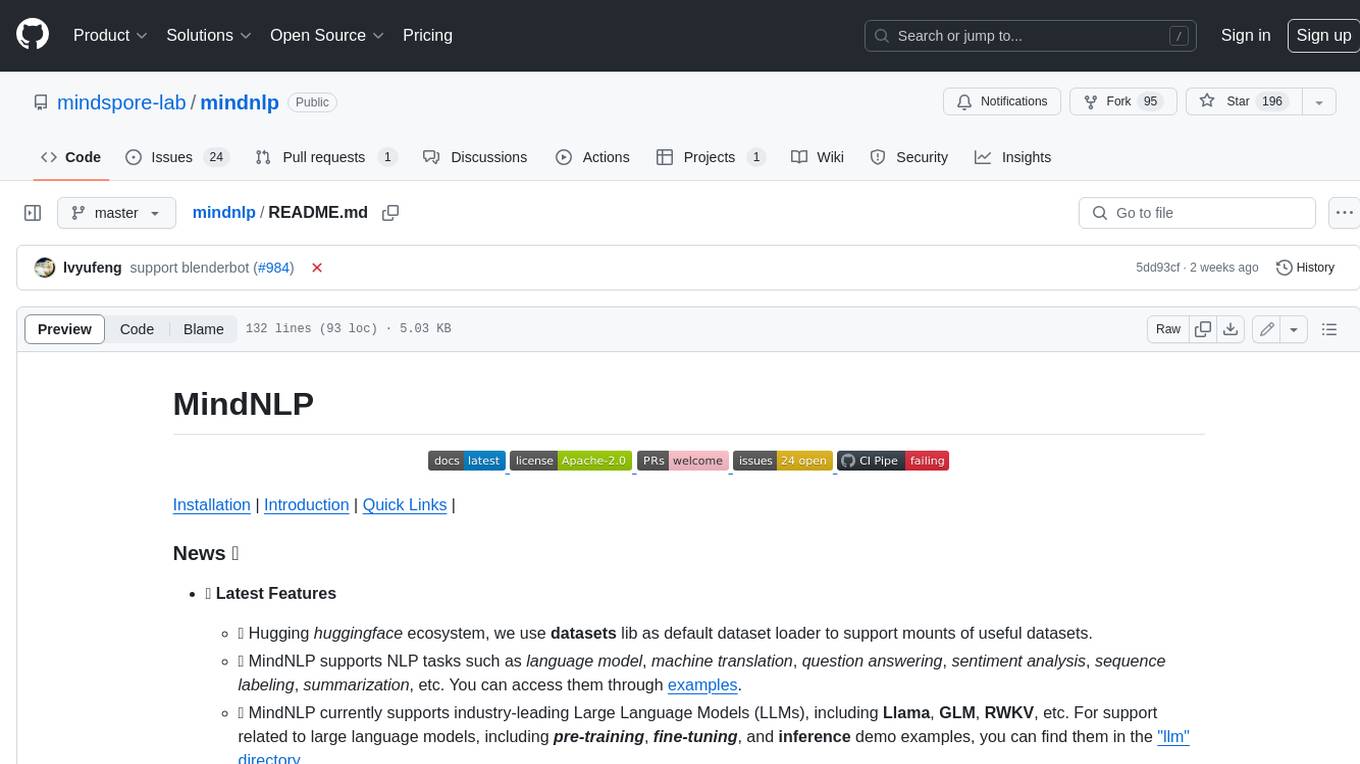
MindNLP is an open-source NLP library based on MindSpore. It provides a platform for solving natural language processing tasks, containing many common approaches in NLP. It can help researchers and developers to construct and train models more conveniently and rapidly. Key features of MindNLP include: * Comprehensive data processing: Several classical NLP datasets are packaged into a friendly module for easy use, such as Multi30k, SQuAD, CoNLL, etc. * Friendly NLP model toolset: MindNLP provides various configurable components. It is friendly to customize models using MindNLP. * Easy-to-use engine: MindNLP simplified complicated training process in MindSpore. It supports Trainer and Evaluator interfaces to train and evaluate models easily. MindNLP supports a wide range of NLP tasks, including: * Language modeling * Machine translation * Question answering * Sentiment analysis * Sequence labeling * Summarization MindNLP also supports industry-leading Large Language Models (LLMs), including Llama, GLM, RWKV, etc. For support related to large language models, including pre-training, fine-tuning, and inference demo examples, you can find them in the "llm" directory. To install MindNLP, you can either install it from Pypi, download the daily build wheel, or install it from source. The installation instructions are provided in the documentation. MindNLP is released under the Apache 2.0 license. If you find this project useful in your research, please consider citing the following paper: @misc{mindnlp2022, title={{MindNLP}: a MindSpore NLP library}, author={MindNLP Contributors}, howpublished = {\url{https://github.com/mindlab-ai/mindnlp}}, year={2022} }
README:
-
🔥 Latest Features
- 🤗 250+ Pretrained models support huggingface transformers-like apis.
You can use them easily by following code snippet:
from mindnlp.transformers import AutoModel model = AutoModel.from_pretrained('bert-base-cased')
-
Full Platform Support: Comprehensive support for
Ascend 910 series
,Ascend 310B (Orange Pi)
,GPU
, andCPU
. (Note: Currently the only AI development kit available on Orange Pi.) - Distributed Parallel Inference: Multi-device, multi-process parallel inference support for models exceeding 10B parameters.
- Quantization Algorithm Support: SmoothQuant available for Orange Pi; bitsandbytes-like int8 quantization supported on GPU.
- Sentence Transformer Support: Enables efficient RAG (Retrieval-Augmented Generation) development.
- Dynamic Graph Performance Optimization: Achieves PyTorch+GPU-level inference speeds for dynamic graphs on Ascend hardware (tested Llama performance at 85ms/token).
-
True Static and Dynamic Graph Unification: One-line switching to graph mode with
mindspore.jit
, fully compatible with Hugging Face code style for both ease of use and rapid performance improvement. Tested Llama performance on Ascend hardware reaches 2x dynamic graph speed (45ms/token), consistent with other MindSpore static graph-based suites. -
Extensive LLM Application Updates: Includes
Text information extraction
,Chatbots
,Speech recognition
,ChatPDF
,Music generation
,Code generation
,Voice clone
, etc. With increased model support, even more exciting applications await development!
- 🤗 250+ Pretrained models support huggingface transformers-like apis.
You can use them easily by following code snippet:
You can install the official version of MindNLP which is uploaded to pypi.
pip install mindnlp
You can download MindNLP daily wheel from here.
To install MindNLP from source, please run:
pip install git+https://github.com/mindspore-lab/mindnlp.git
# or
git clone https://github.com/mindspore-lab/mindnlp.git
cd mindnlp
bash scripts/build_and_reinstall.sh
MindNLP version | MindSpore version | Supported Python version |
---|---|---|
master | daily build | >=3.7.5, <=3.9 |
0.1.1 | >=1.8.1, <=2.0.0 | >=3.7.5, <=3.9 |
0.2.x | >=2.1.0 | >=3.8, <=3.9 |
0.3.x | >=2.1.0, <=2.3.1 | >=3.8, <=3.9 |
0.4.x | >=2.2.x | >=3.9, <=3.11 |
MindNLP is an open source NLP library based on MindSpore. It supports a platform for solving natural language processing tasks, containing many common approaches in NLP. It can help researchers and developers to construct and train models more conveniently and rapidly.
The master branch works with MindSpore master.
- Comprehensive data processing: Several classical NLP datasets are packaged into friendly module for easy use, such as Multi30k, SQuAD, CoNLL, etc.
- Friendly NLP model toolset: MindNLP provides various configurable components. It is friendly to customize models using MindNLP.
- Easy-to-use engine: MindNLP simplified the complicated training process in MindSpore. It supports Trainer and Evaluator interfaces to train and evaluate models easily.
Since there are too many supported models, please check here
This project is released under the Apache 2.0 license.
The dynamic version is still under development, if you find any issue or have an idea on new features, please don't hesitate to contact us via Github Issues.
MindSpore NLP SIG (Natural Language Processing Special Interest Group) is the main development team of the MindNLP framework. It aims to collaborate with developers from both industry and academia who are interested in research, application development, and the practical implementation of natural language processing. Our goal is to create the best NLP framework based on the domestic framework MindSpore. Additionally, we regularly hold NLP technology sharing sessions and offline events. Interested developers can join our SIG group using the QR code below.
MindSpore is an open source project that welcomes any contribution and feedback.
We wish that the toolbox and benchmark could serve the growing research
community by providing a flexible as well as standardized toolkit to re-implement existing methods
and develop their own new semantic segmentation methods.
If you find this project useful in your research, please consider citing:
@misc{mindnlp2022,
title={{MindNLP}: Easy-to-use and high-performance NLP and LLM framework based on MindSpore},
author={MindNLP Contributors},
howpublished = {\url{https://github.com/mindlab-ai/mindnlp}},
year={2022}
}
For Tasks:
Click tags to check more tools for each tasksFor Jobs:
Alternative AI tools for mindnlp
Similar Open Source Tools
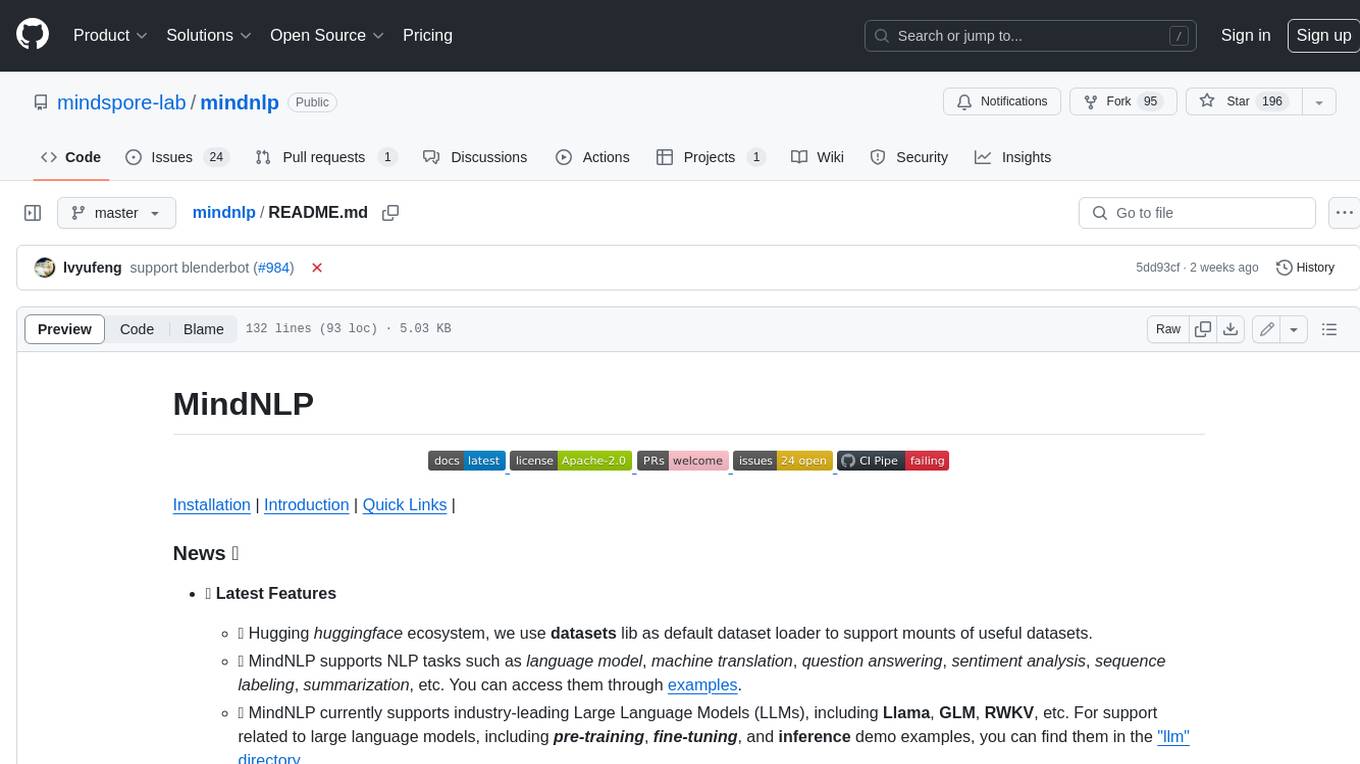
mindnlp
MindNLP is an open-source NLP library based on MindSpore. It provides a platform for solving natural language processing tasks, containing many common approaches in NLP. It can help researchers and developers to construct and train models more conveniently and rapidly. Key features of MindNLP include: * Comprehensive data processing: Several classical NLP datasets are packaged into a friendly module for easy use, such as Multi30k, SQuAD, CoNLL, etc. * Friendly NLP model toolset: MindNLP provides various configurable components. It is friendly to customize models using MindNLP. * Easy-to-use engine: MindNLP simplified complicated training process in MindSpore. It supports Trainer and Evaluator interfaces to train and evaluate models easily. MindNLP supports a wide range of NLP tasks, including: * Language modeling * Machine translation * Question answering * Sentiment analysis * Sequence labeling * Summarization MindNLP also supports industry-leading Large Language Models (LLMs), including Llama, GLM, RWKV, etc. For support related to large language models, including pre-training, fine-tuning, and inference demo examples, you can find them in the "llm" directory. To install MindNLP, you can either install it from Pypi, download the daily build wheel, or install it from source. The installation instructions are provided in the documentation. MindNLP is released under the Apache 2.0 license. If you find this project useful in your research, please consider citing the following paper: @misc{mindnlp2022, title={{MindNLP}: a MindSpore NLP library}, author={MindNLP Contributors}, howpublished = {\url{https://github.com/mindlab-ai/mindnlp}}, year={2022} }
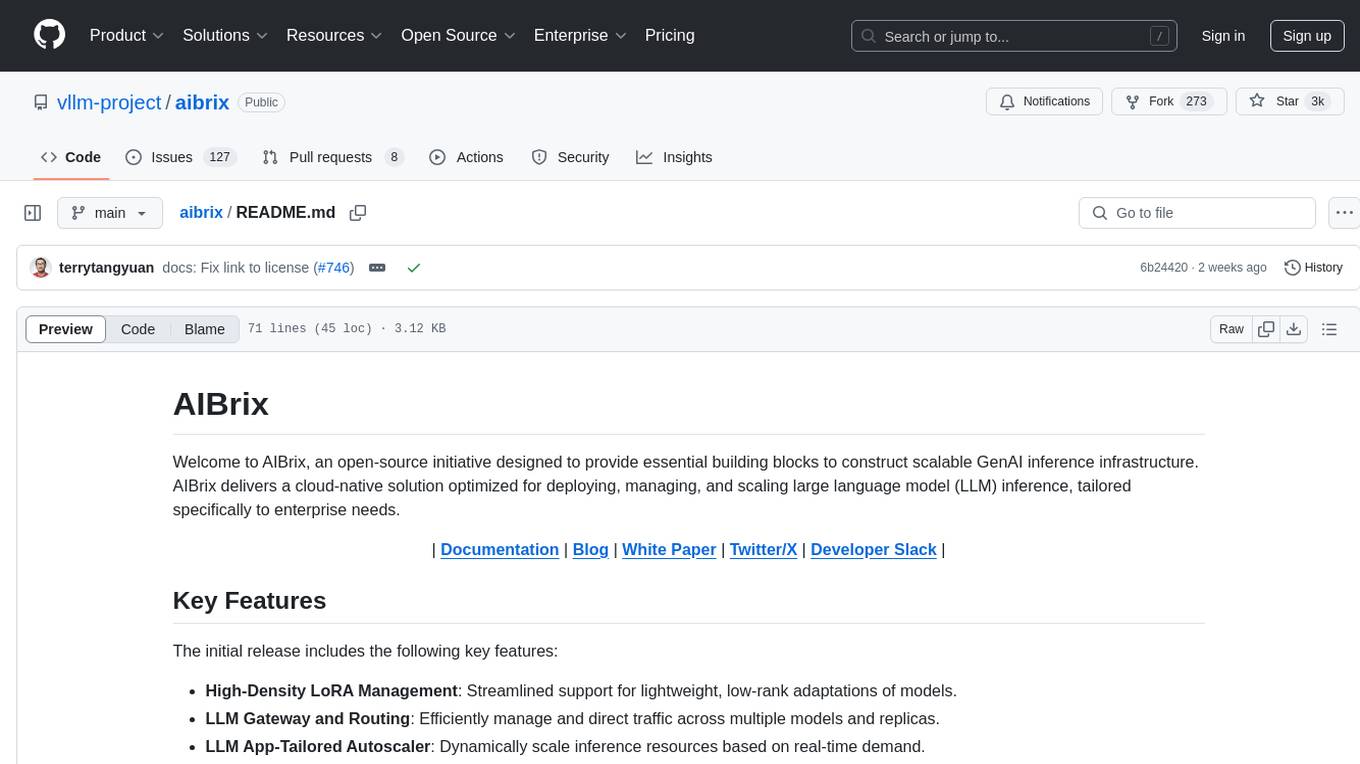
aibrix
AIBrix is an open-source initiative providing essential building blocks for scalable GenAI inference infrastructure. It delivers a cloud-native solution optimized for deploying, managing, and scaling large language model (LLM) inference, tailored to enterprise needs. Key features include High-Density LoRA Management, LLM Gateway and Routing, LLM App-Tailored Autoscaler, Unified AI Runtime, Distributed Inference, Distributed KV Cache, Cost-efficient Heterogeneous Serving, and GPU Hardware Failure Detection.
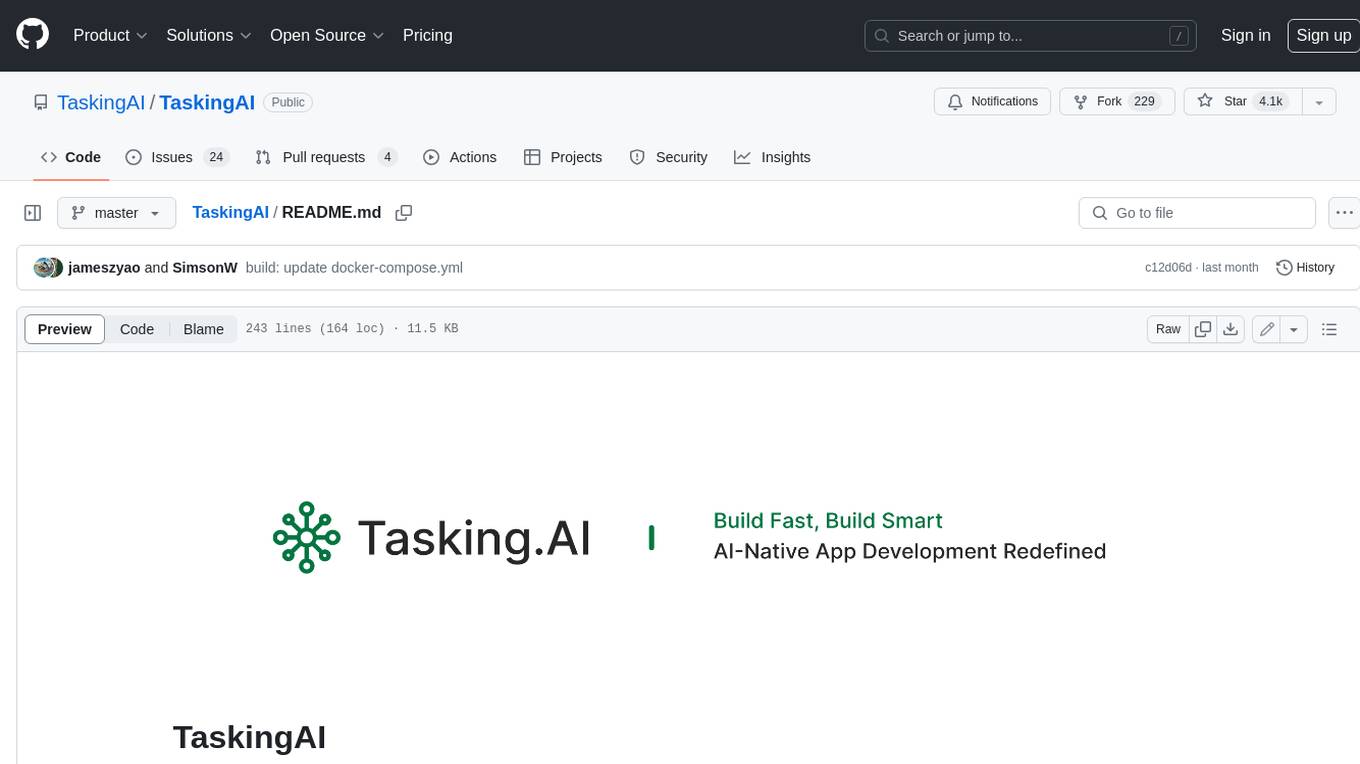
TaskingAI
TaskingAI brings Firebase's simplicity to **AI-native app development**. The platform enables the creation of GPTs-like multi-tenant applications using a wide range of LLMs from various providers. It features distinct, modular functions such as Inference, Retrieval, Assistant, and Tool, seamlessly integrated to enhance the development process. TaskingAI’s cohesive design ensures an efficient, intelligent, and user-friendly experience in AI application development.
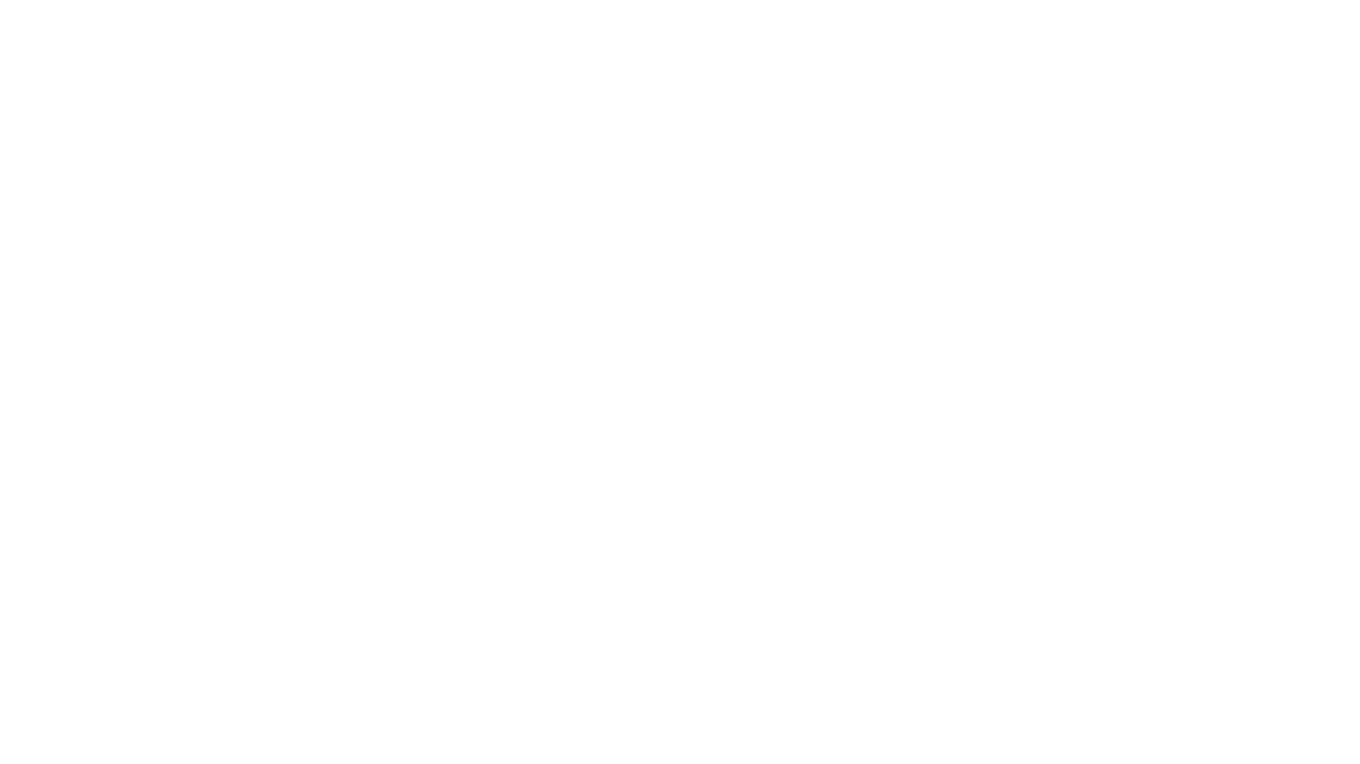
motia
Motia is an AI agent framework designed for software engineers to create, test, and deploy production-ready AI agents quickly. It provides a code-first approach, allowing developers to write agent logic in familiar languages and visualize execution in real-time. With Motia, developers can focus on business logic rather than infrastructure, offering zero infrastructure headaches, multi-language support, composable steps, built-in observability, instant APIs, and full control over AI logic. Ideal for building sophisticated agents and intelligent automations, Motia's event-driven architecture and modular steps enable the creation of GenAI-powered workflows, decision-making systems, and data processing pipelines.
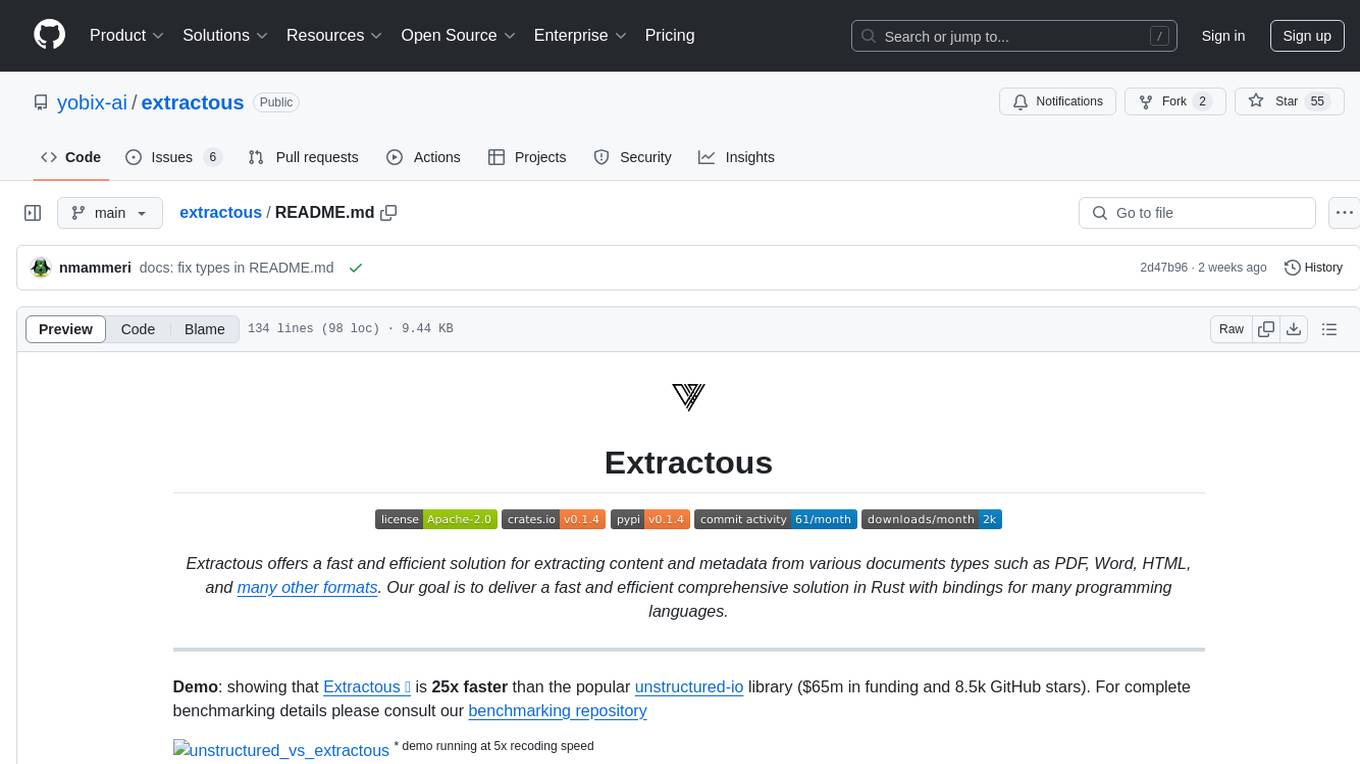
extractous
Extractous offers a fast and efficient solution for extracting content and metadata from various document types such as PDF, Word, HTML, and many other formats. It is built with Rust, providing high performance, memory safety, and multi-threading capabilities. The tool eliminates the need for external services or APIs, making data processing pipelines faster and more efficient. It supports multiple file formats, including Microsoft Office, OpenOffice, PDF, spreadsheets, web documents, e-books, text files, images, and email formats. Extractous provides a clear and simple API for extracting text and metadata content, with upcoming support for JavaScript/TypeScript. It is free for commercial use under the Apache 2.0 License.
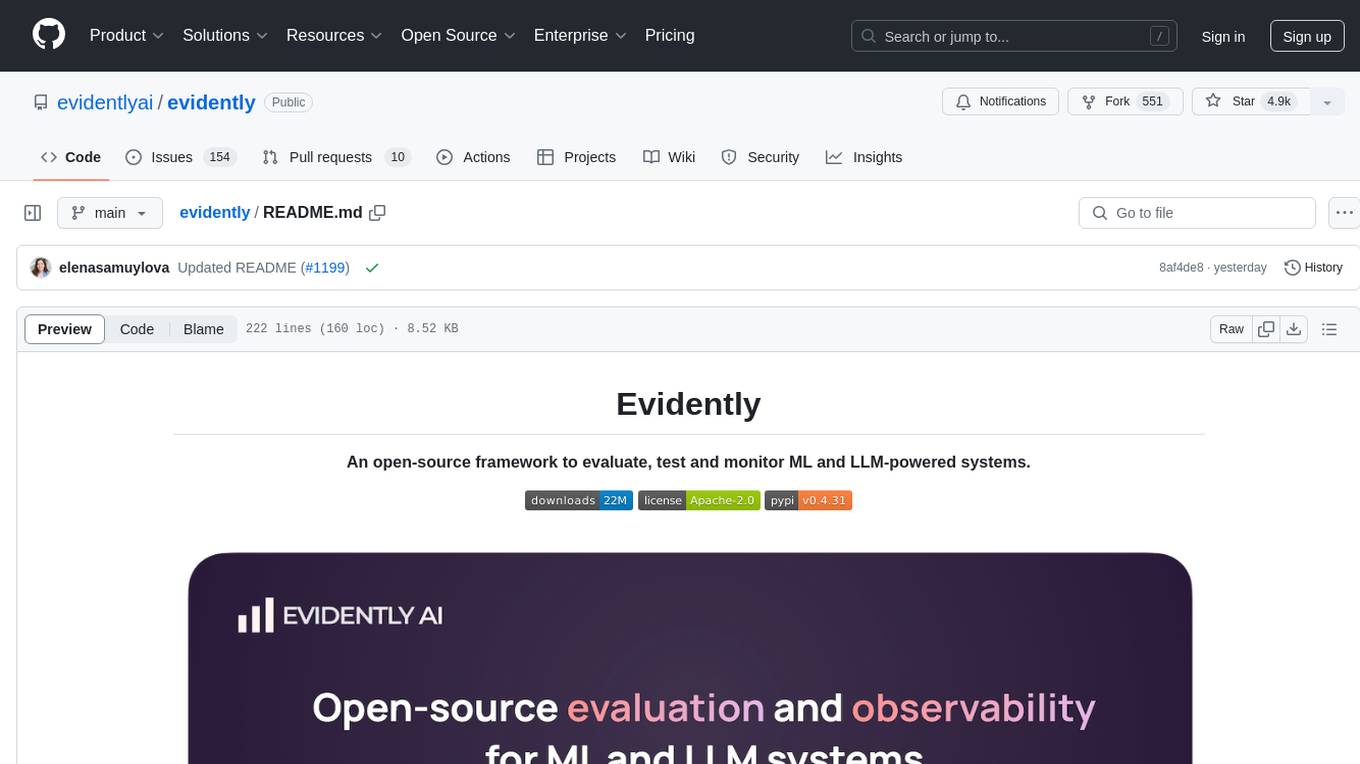
evidently
Evidently is an open-source Python library designed for evaluating, testing, and monitoring machine learning (ML) and large language model (LLM) powered systems. It offers a wide range of functionalities, including working with tabular, text data, and embeddings, supporting predictive and generative systems, providing over 100 built-in metrics for data drift detection and LLM evaluation, allowing for custom metrics and tests, enabling both offline evaluations and live monitoring, and offering an open architecture for easy data export and integration with existing tools. Users can utilize Evidently for one-off evaluations using Reports or Test Suites in Python, or opt for real-time monitoring through the Dashboard service.
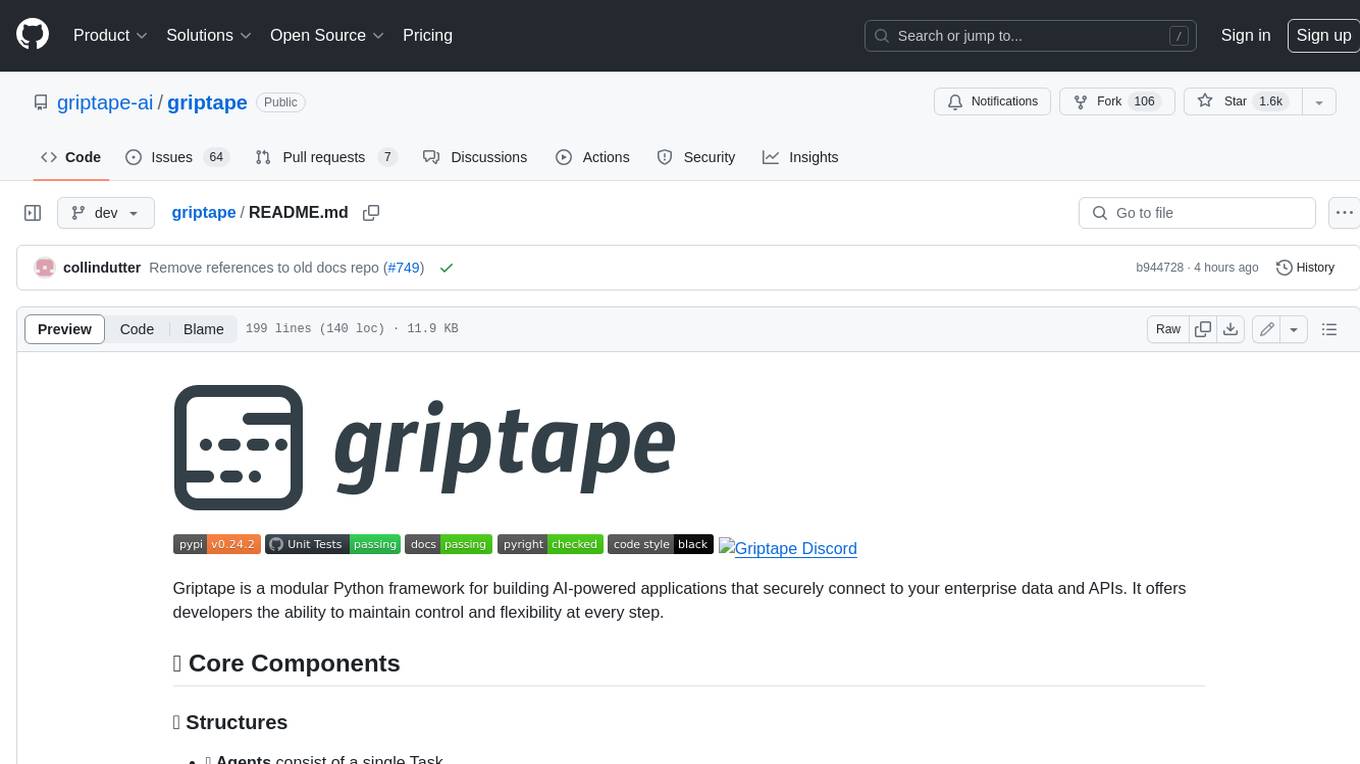
griptape
Griptape is a modular Python framework for building AI-powered applications that securely connect to your enterprise data and APIs. It offers developers the ability to maintain control and flexibility at every step. Griptape's core components include Structures (Agents, Pipelines, and Workflows), Tasks, Tools, Memory (Conversation Memory, Task Memory, and Meta Memory), Drivers (Prompt and Embedding Drivers, Vector Store Drivers, Image Generation Drivers, Image Query Drivers, SQL Drivers, Web Scraper Drivers, and Conversation Memory Drivers), Engines (Query Engines, Extraction Engines, Summary Engines, Image Generation Engines, and Image Query Engines), and additional components (Rulesets, Loaders, Artifacts, Chunkers, and Tokenizers). Griptape enables developers to create AI-powered applications with ease and efficiency.
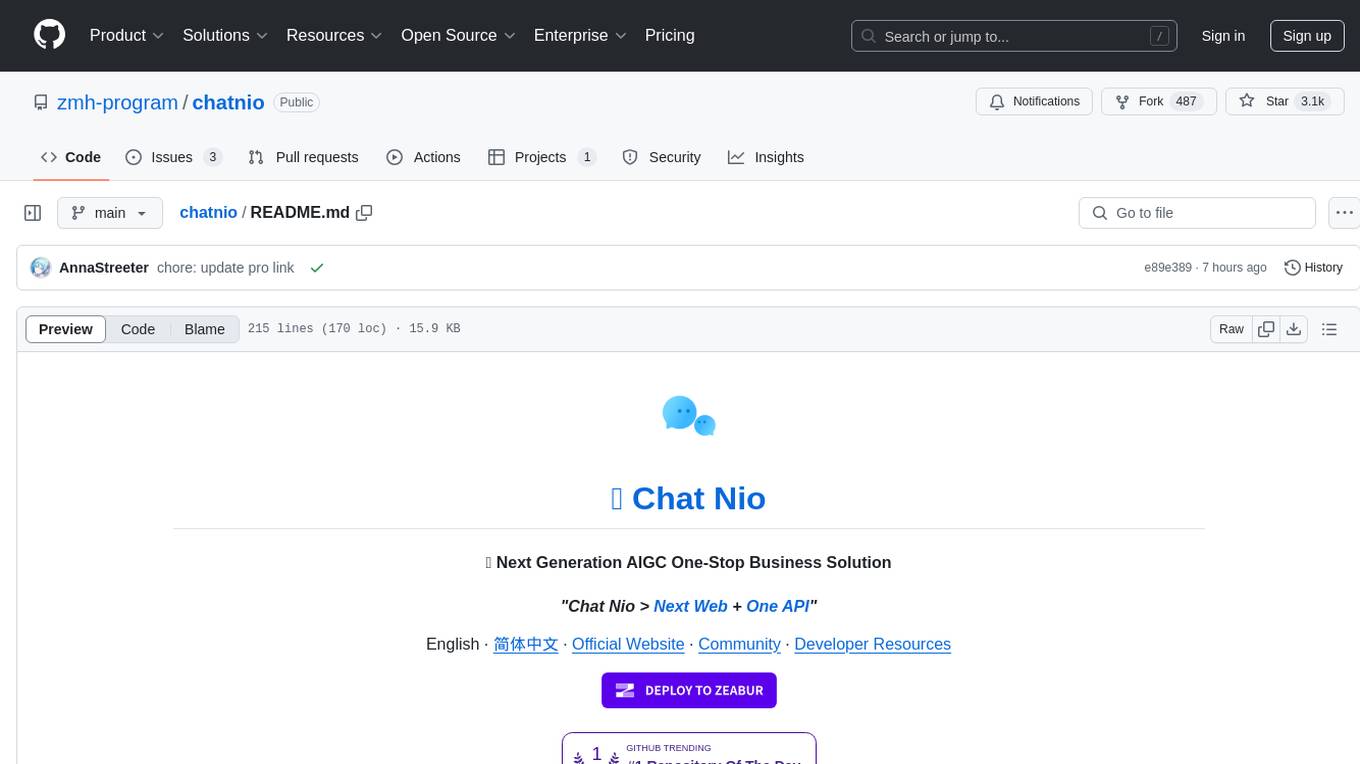
chatnio
Chat Nio is a next-generation AIGC one-stop business solution that combines the advantages of frontend-oriented lightweight deployment projects with powerful API distribution systems. It offers rich model support, beautiful UI design, complete Markdown support, multi-theme support, internationalization support, text-to-image support, powerful conversation sync, model market & preset system, rich file parsing, full model internet search, Progressive Web App (PWA) support, comprehensive backend management, multiple billing methods, innovative model caching, and additional features. The project aims to address limitations in conversation synchronization, billing, file parsing, conversation URL sharing, channel management, and API call support found in existing AIGC commercial sites, while also providing a user-friendly interface design and C-end features.
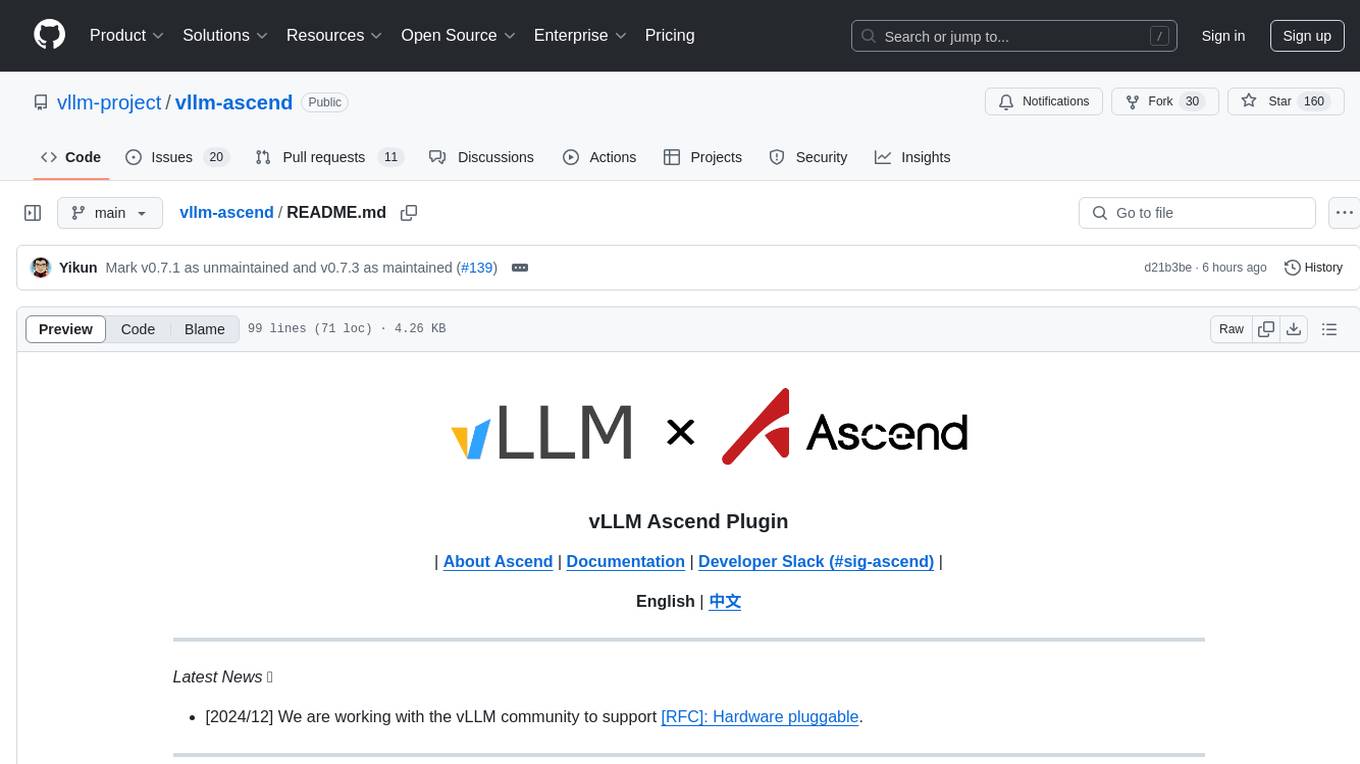
vllm-ascend
vLLM Ascend plugin is a backend plugin designed to run vLLM on the Ascend NPU. It provides a hardware-pluggable interface that allows popular open-source models to run seamlessly on the Ascend NPU. The plugin is recommended within the vLLM community and adheres to the principles of hardware pluggability outlined in the RFC. Users can set up their environment with specific hardware and software prerequisites to utilize this plugin effectively.
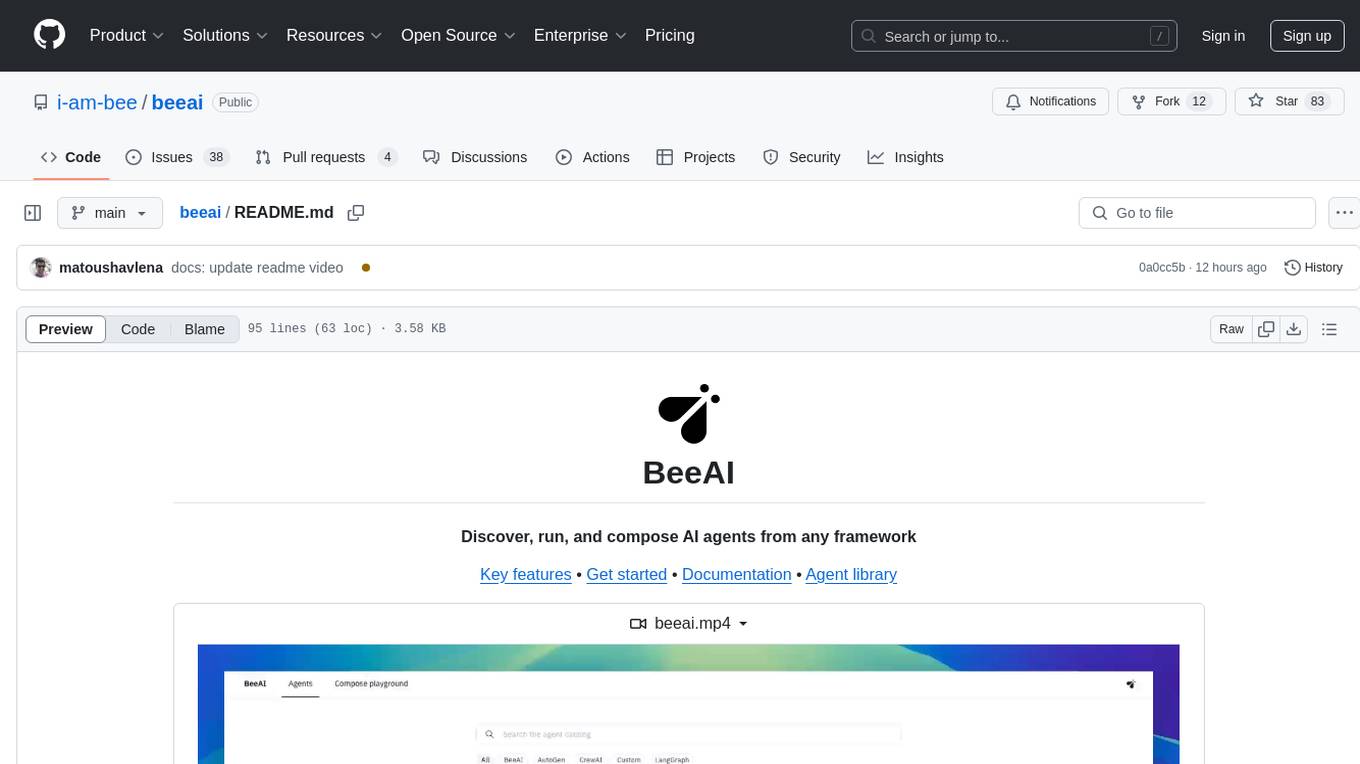
beeai
BeeAI is an open platform that helps users discover, run, and compose AI agents from any framework and language. It offers a framework-agnostic approach, allowing seamless integration of AI agents regardless of the language or platform. Users can build complex workflows using simple building blocks, explore a catalog of powerful agents with integrated search, and benefit from the BeeAI ecosystem with first-class support for Python and TypeScript agent developers.
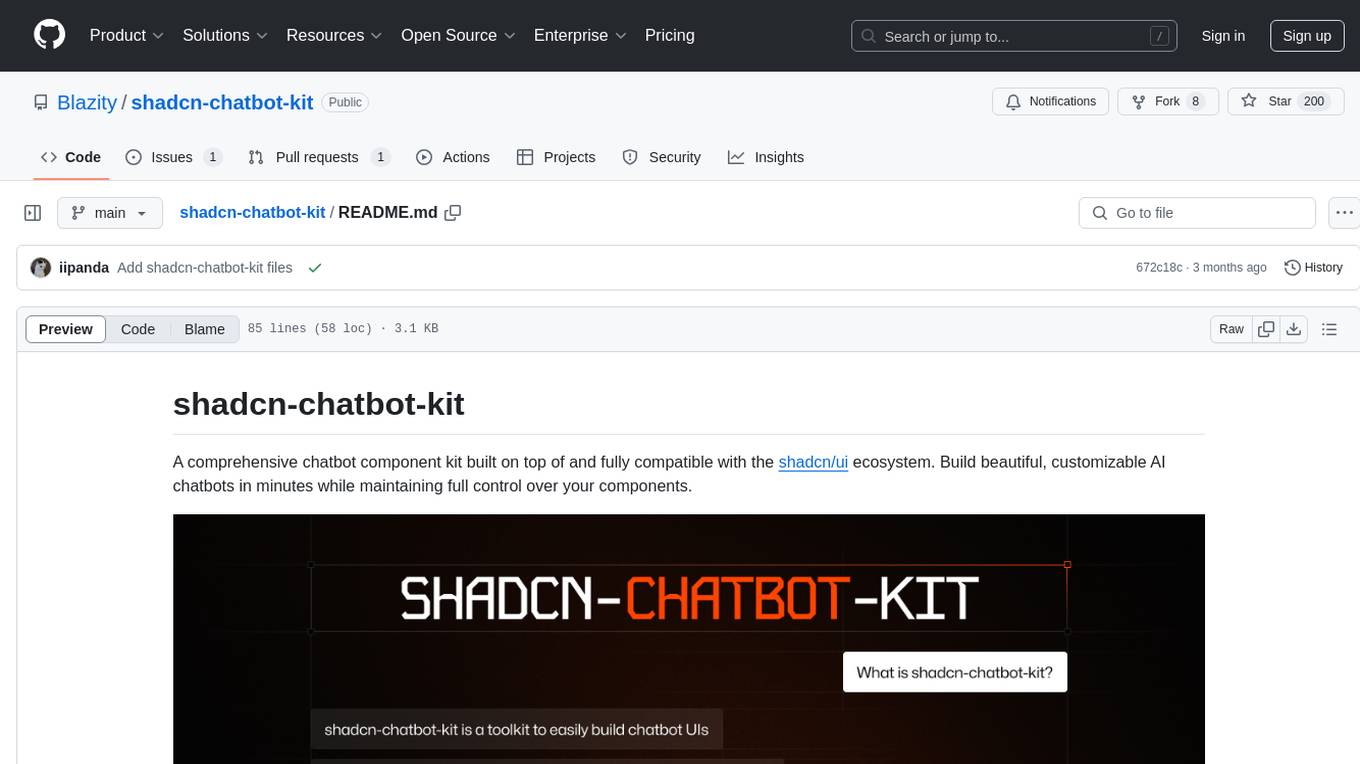
shadcn-chatbot-kit
A comprehensive chatbot component kit built on top of and fully compatible with the shadcn/ui ecosystem. Build beautiful, customizable AI chatbots in minutes while maintaining full control over your components. The kit includes pre-built chat components, auto-scroll message area, message input with auto-resize textarea and file upload support, prompt suggestions, message actions, loading states, and more. Fully themeable, highly customizable, and responsive design. Built with modern web standards and best practices. Installation instructions available with detailed documentation. Customizable using CSS variables.
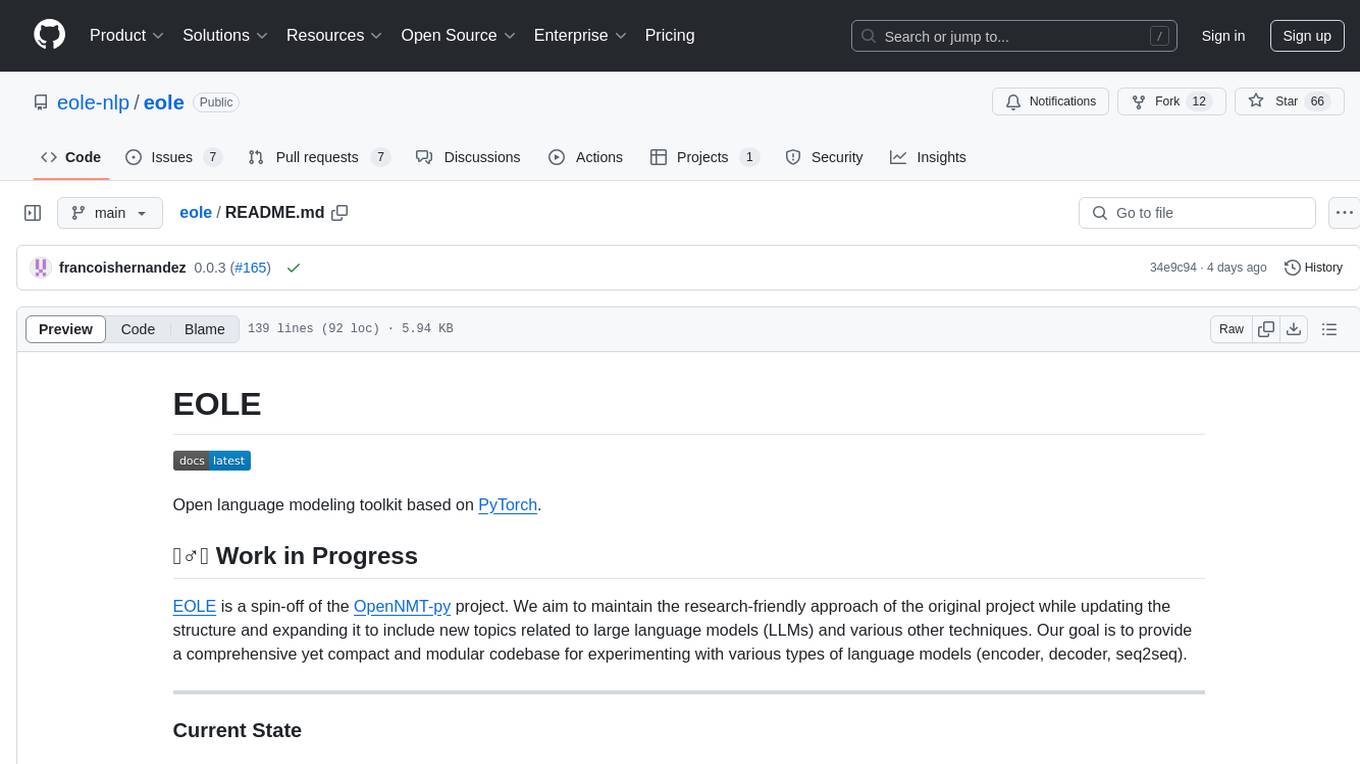
eole
EOLE is an open language modeling toolkit based on PyTorch. It aims to provide a research-friendly approach with a comprehensive yet compact and modular codebase for experimenting with various types of language models. The toolkit includes features such as versatile training and inference, dynamic data transforms, comprehensive large language model support, advanced quantization, efficient finetuning, flexible inference, and tensor parallelism. EOLE is a work in progress with ongoing enhancements in configuration management, command line entry points, reproducible recipes, core API simplification, and plans for further simplification, refactoring, inference server development, additional recipes, documentation enhancement, test coverage improvement, logging enhancements, and broader model support.
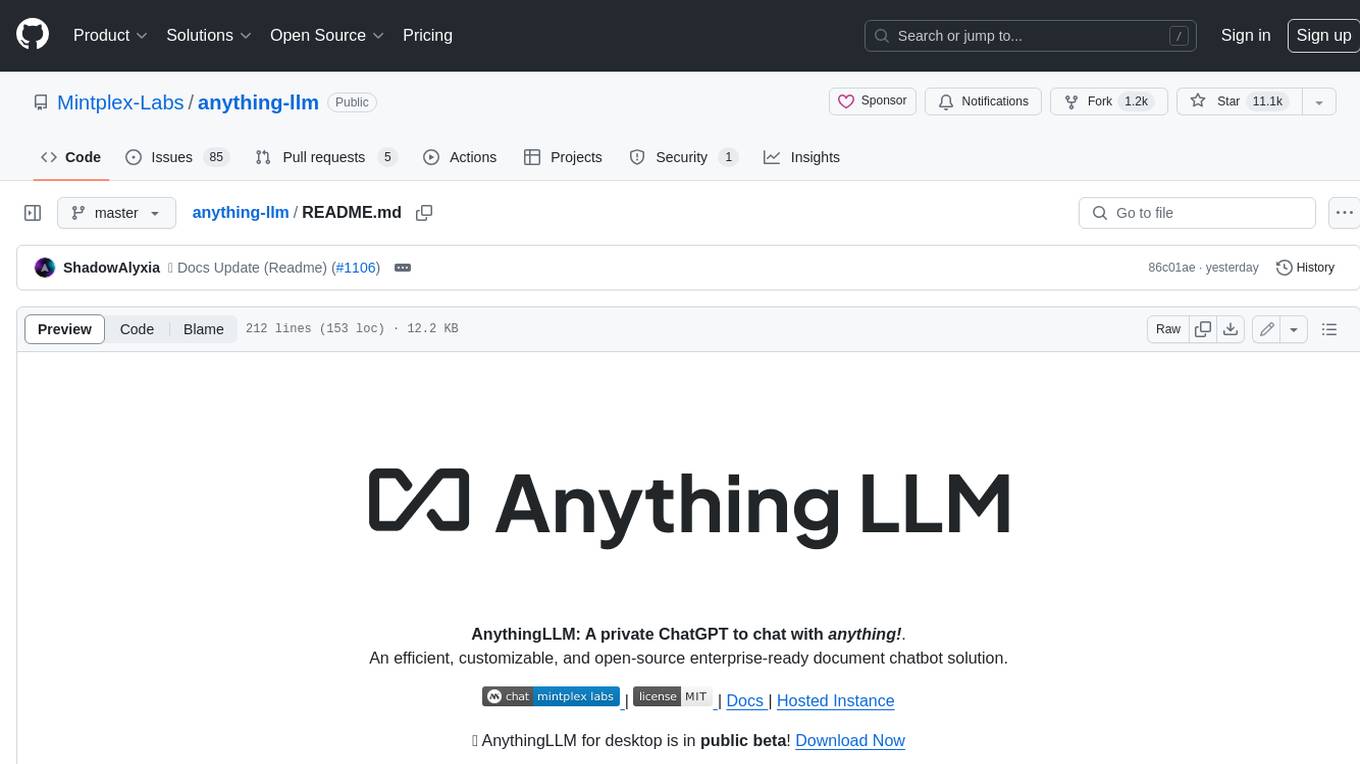
anything-llm
AnythingLLM is a full-stack application that enables you to turn any document, resource, or piece of content into context that any LLM can use as references during chatting. This application allows you to pick and choose which LLM or Vector Database you want to use as well as supporting multi-user management and permissions.
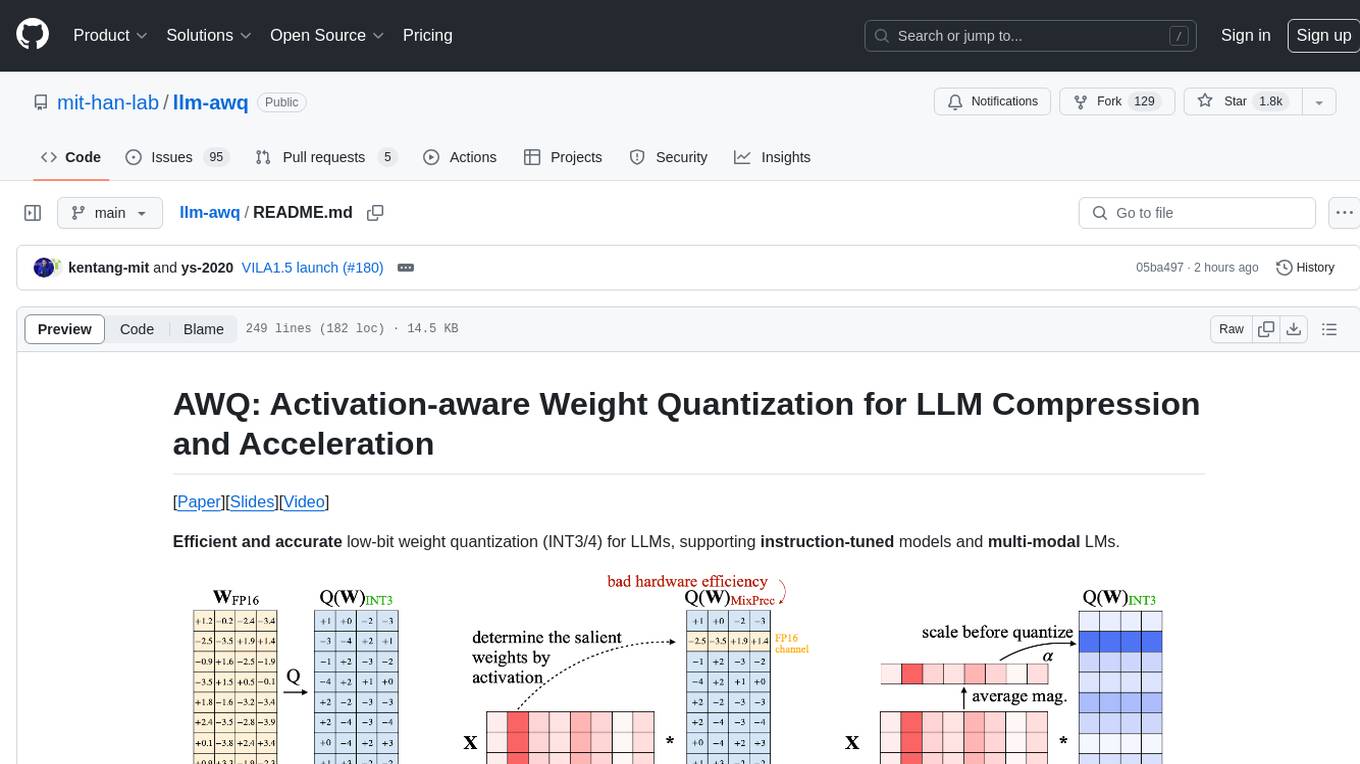
llm-awq
AWQ (Activation-aware Weight Quantization) is a tool designed for efficient and accurate low-bit weight quantization (INT3/4) for Large Language Models (LLMs). It supports instruction-tuned models and multi-modal LMs, providing features such as AWQ search for accurate quantization, pre-computed AWQ model zoo for various LLMs, memory-efficient 4-bit linear in PyTorch, and efficient CUDA kernel implementation for fast inference. The tool enables users to run large models on resource-constrained edge platforms, delivering more efficient responses with LLM/VLM chatbots through 4-bit inference.
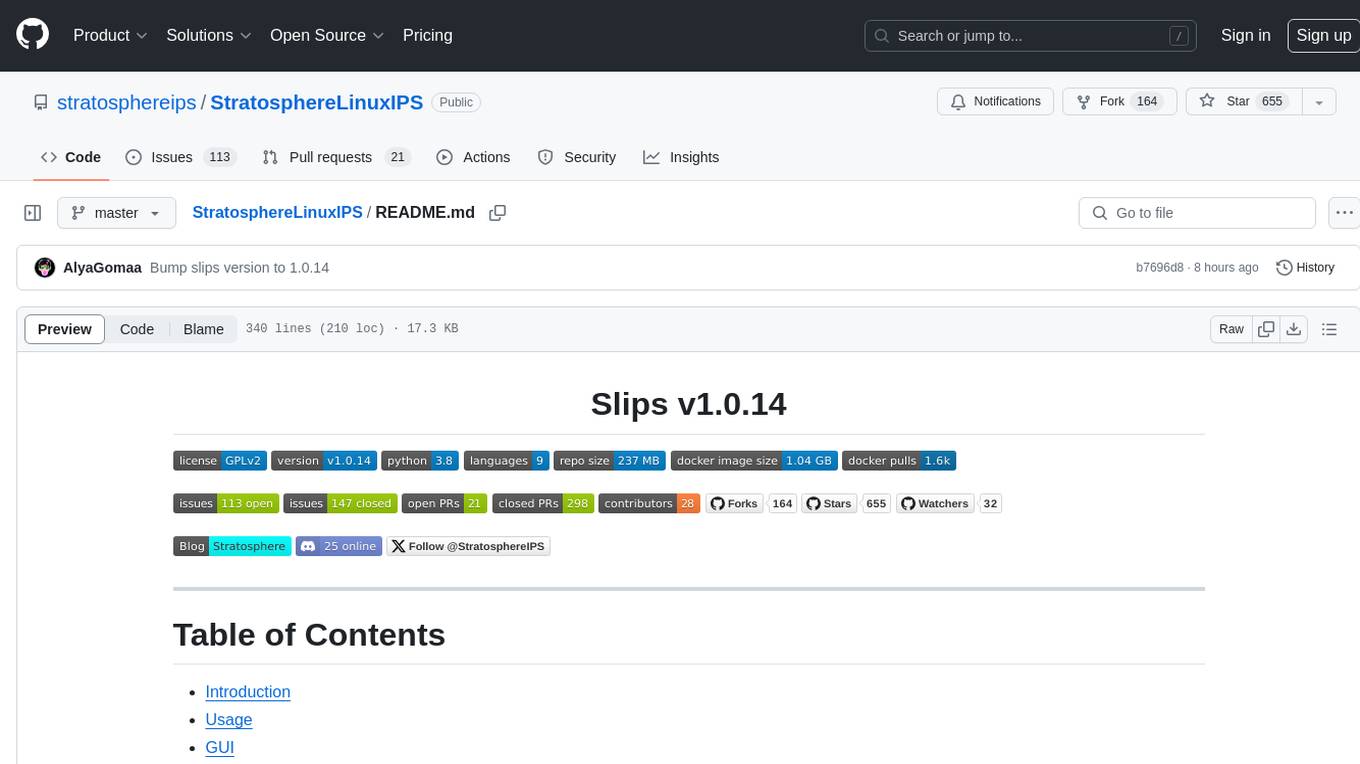
StratosphereLinuxIPS
Slips is a powerful endpoint behavioral intrusion prevention and detection system that uses machine learning to detect malicious behaviors in network traffic. It can work with network traffic in real-time, PCAP files, and network flows from tools like Suricata, Zeek/Bro, and Argus. Slips threat detection is based on machine learning models, threat intelligence feeds, and expert heuristics. It gathers evidence of malicious behavior and triggers alerts when enough evidence is accumulated. The tool is Python-based and supported on Linux and MacOS, with blocking features only on Linux. Slips relies on Zeek network analysis framework and Redis for interprocess communication. It offers a graphical user interface for easy monitoring and analysis.
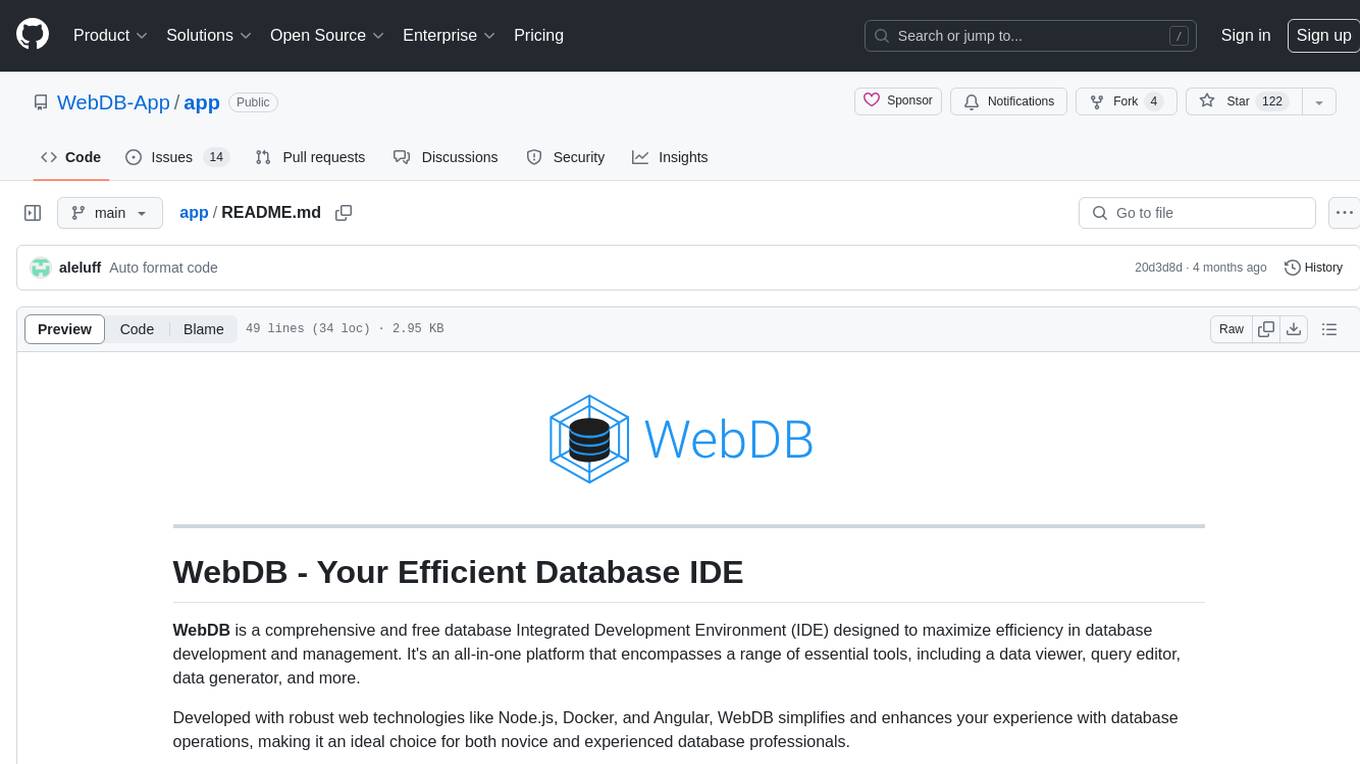
app
WebDB is a comprehensive and free database Integrated Development Environment (IDE) designed to maximize efficiency in database development and management. It simplifies and enhances database operations with features like DBMS discovery, query editor, time machine, NoSQL structure inferring, modern ERD visualization, and intelligent data generator. Developed with robust web technologies, WebDB is suitable for both novice and experienced database professionals.
For similar tasks
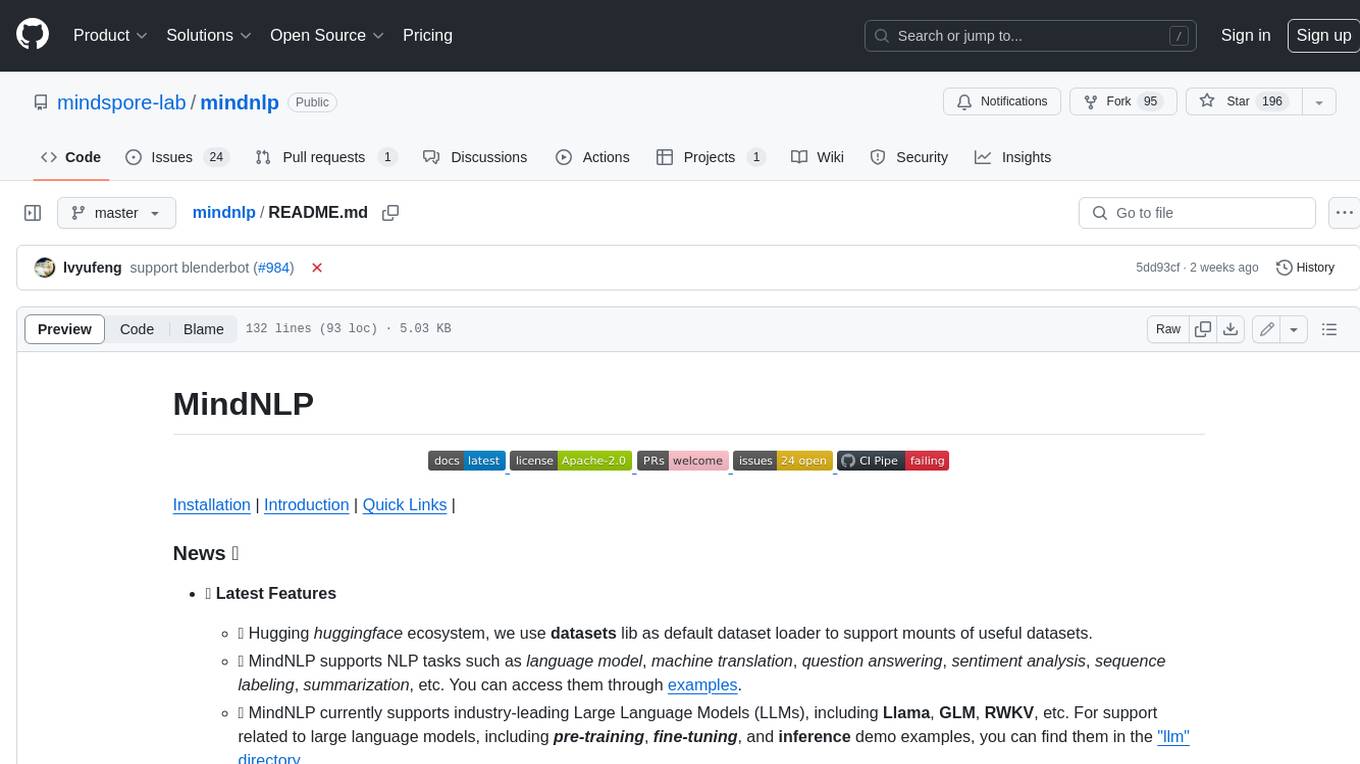
mindnlp
MindNLP is an open-source NLP library based on MindSpore. It provides a platform for solving natural language processing tasks, containing many common approaches in NLP. It can help researchers and developers to construct and train models more conveniently and rapidly. Key features of MindNLP include: * Comprehensive data processing: Several classical NLP datasets are packaged into a friendly module for easy use, such as Multi30k, SQuAD, CoNLL, etc. * Friendly NLP model toolset: MindNLP provides various configurable components. It is friendly to customize models using MindNLP. * Easy-to-use engine: MindNLP simplified complicated training process in MindSpore. It supports Trainer and Evaluator interfaces to train and evaluate models easily. MindNLP supports a wide range of NLP tasks, including: * Language modeling * Machine translation * Question answering * Sentiment analysis * Sequence labeling * Summarization MindNLP also supports industry-leading Large Language Models (LLMs), including Llama, GLM, RWKV, etc. For support related to large language models, including pre-training, fine-tuning, and inference demo examples, you can find them in the "llm" directory. To install MindNLP, you can either install it from Pypi, download the daily build wheel, or install it from source. The installation instructions are provided in the documentation. MindNLP is released under the Apache 2.0 license. If you find this project useful in your research, please consider citing the following paper: @misc{mindnlp2022, title={{MindNLP}: a MindSpore NLP library}, author={MindNLP Contributors}, howpublished = {\url{https://github.com/mindlab-ai/mindnlp}}, year={2022} }
For similar jobs
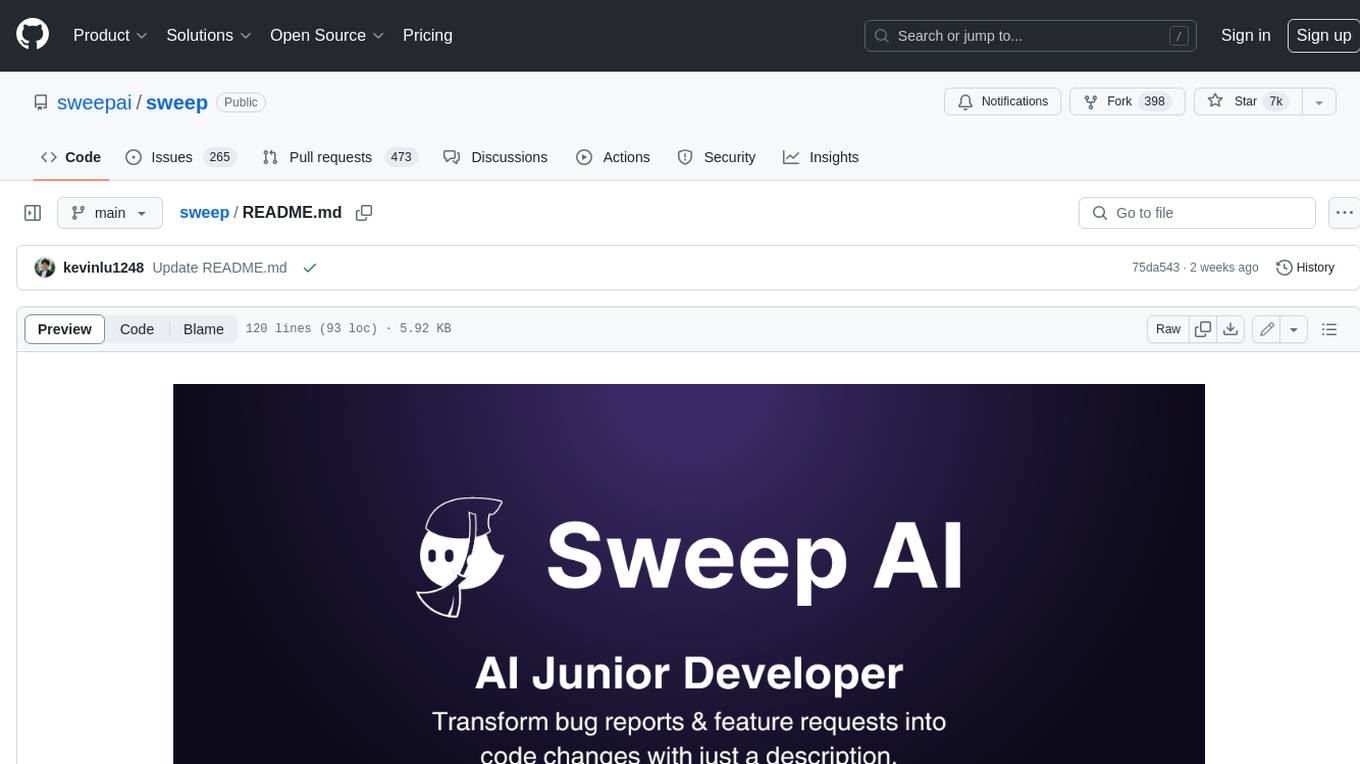
sweep
Sweep is an AI junior developer that turns bugs and feature requests into code changes. It automatically handles developer experience improvements like adding type hints and improving test coverage.
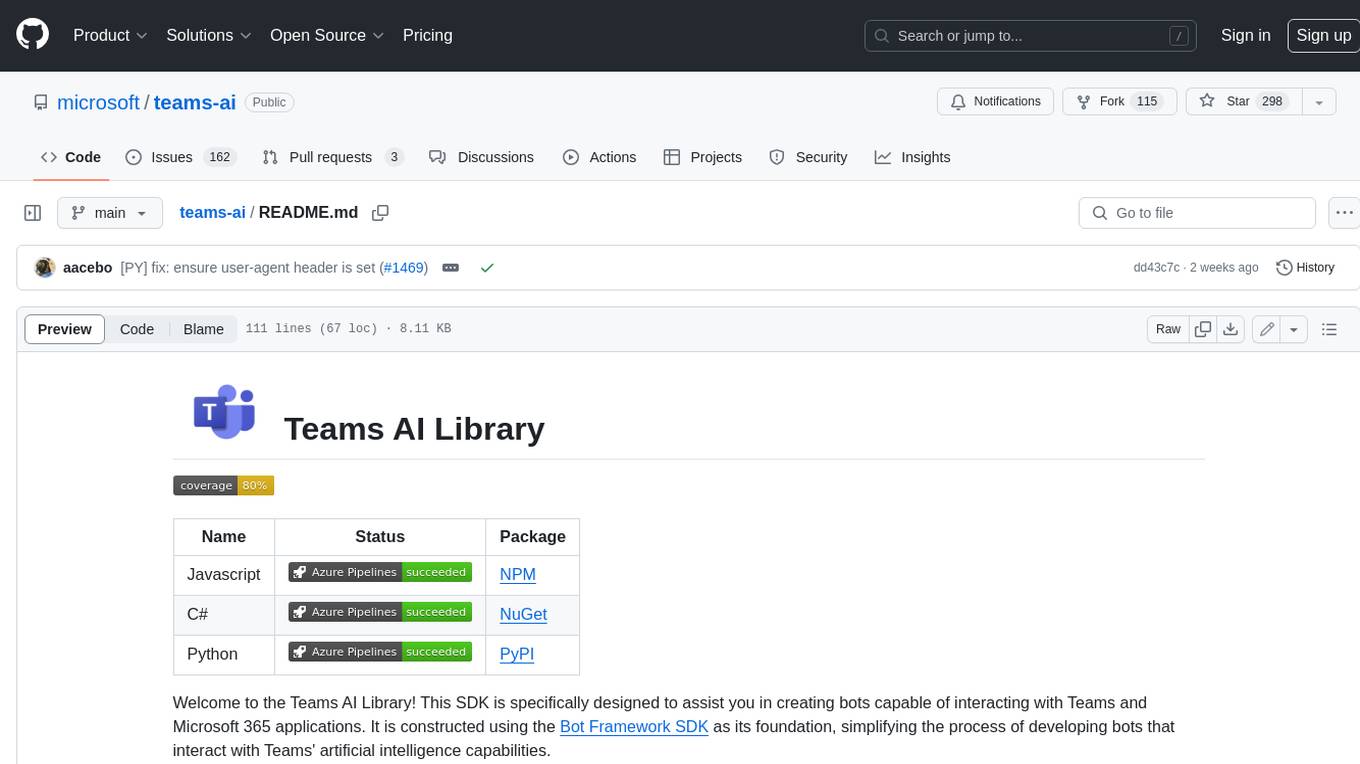
teams-ai
The Teams AI Library is a software development kit (SDK) that helps developers create bots that can interact with Teams and Microsoft 365 applications. It is built on top of the Bot Framework SDK and simplifies the process of developing bots that interact with Teams' artificial intelligence capabilities. The SDK is available for JavaScript/TypeScript, .NET, and Python.
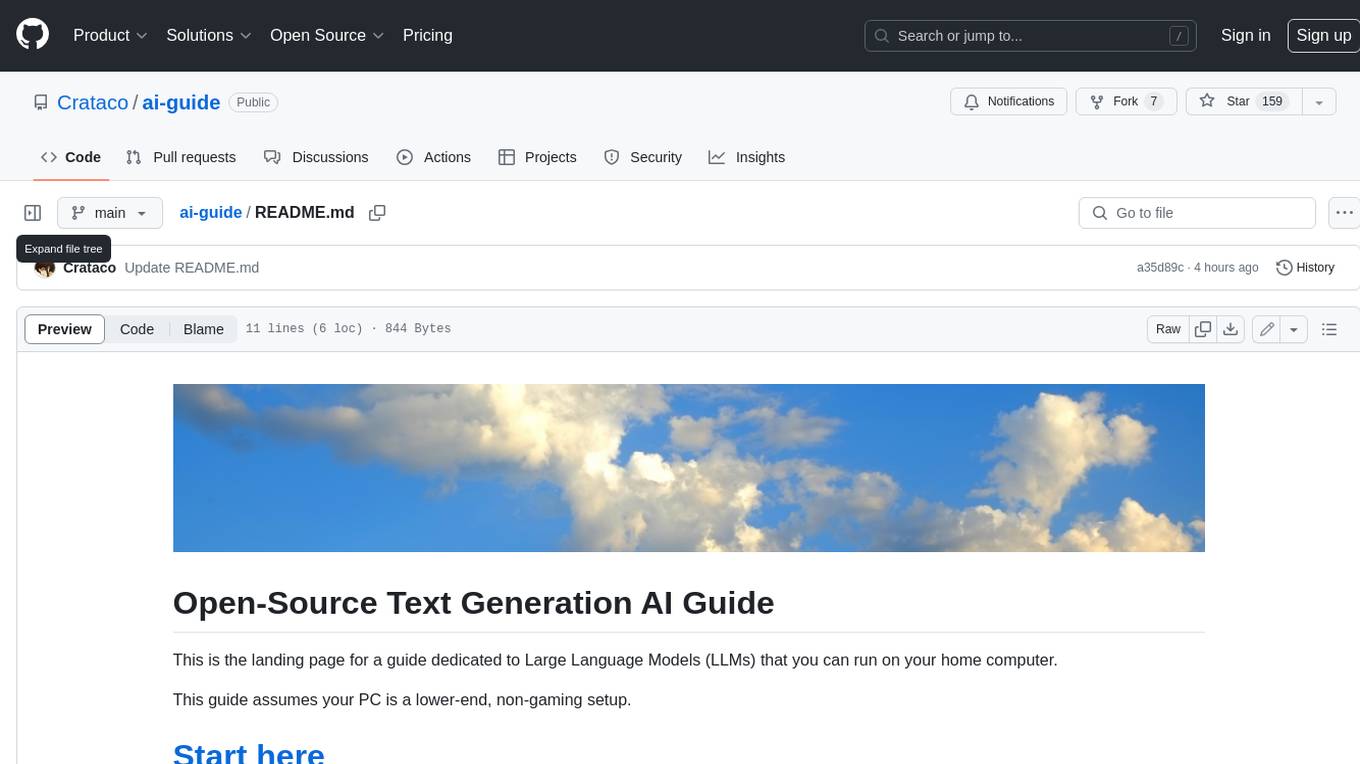
ai-guide
This guide is dedicated to Large Language Models (LLMs) that you can run on your home computer. It assumes your PC is a lower-end, non-gaming setup.
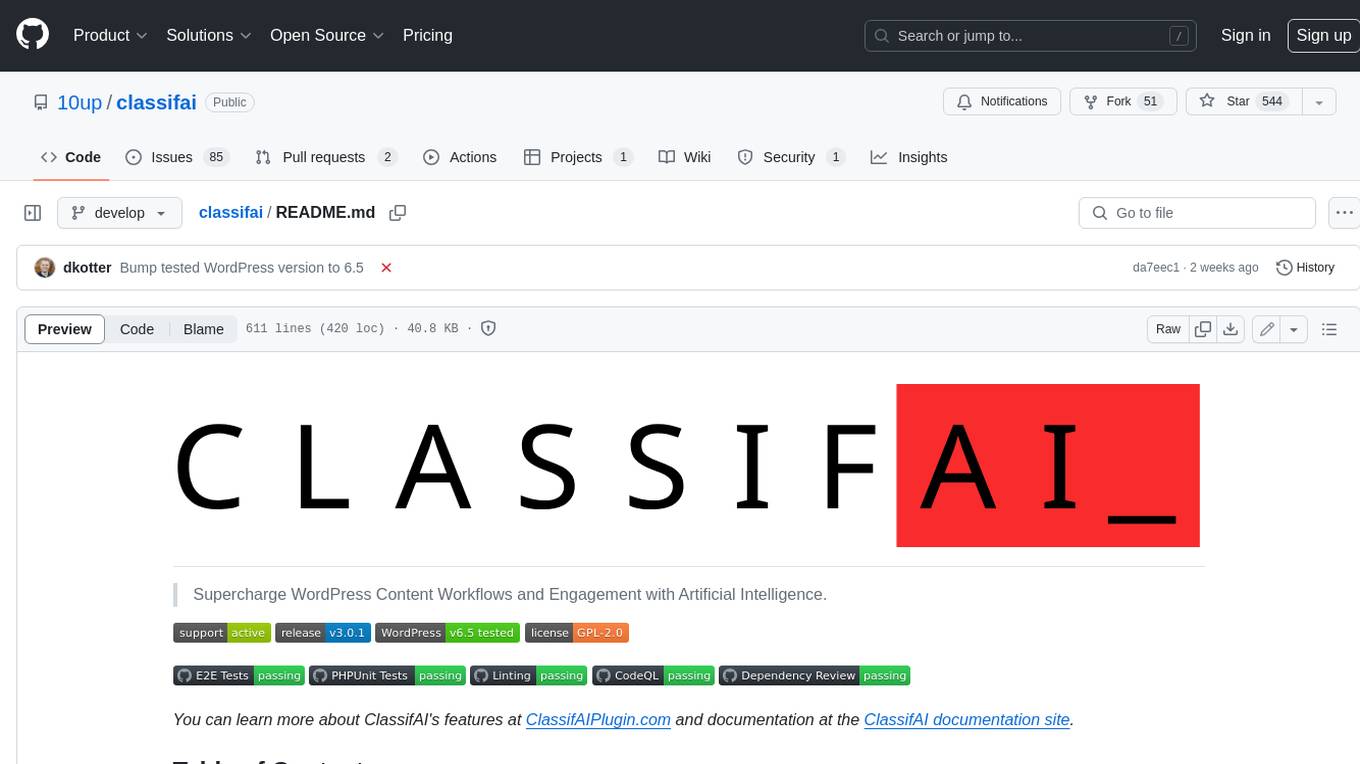
classifai
Supercharge WordPress Content Workflows and Engagement with Artificial Intelligence. Tap into leading cloud-based services like OpenAI, Microsoft Azure AI, Google Gemini and IBM Watson to augment your WordPress-powered websites. Publish content faster while improving SEO performance and increasing audience engagement. ClassifAI integrates Artificial Intelligence and Machine Learning technologies to lighten your workload and eliminate tedious tasks, giving you more time to create original content that matters.
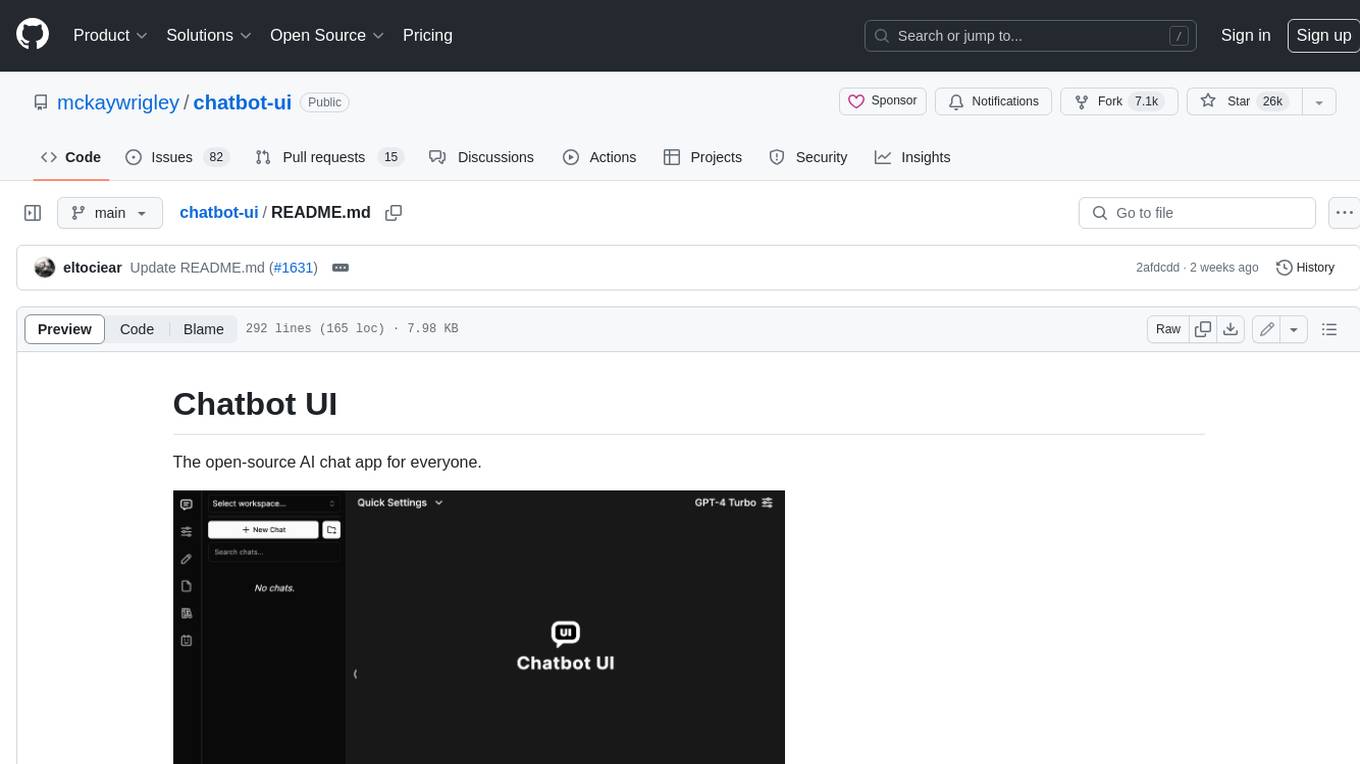
chatbot-ui
Chatbot UI is an open-source AI chat app that allows users to create and deploy their own AI chatbots. It is easy to use and can be customized to fit any need. Chatbot UI is perfect for businesses, developers, and anyone who wants to create a chatbot.
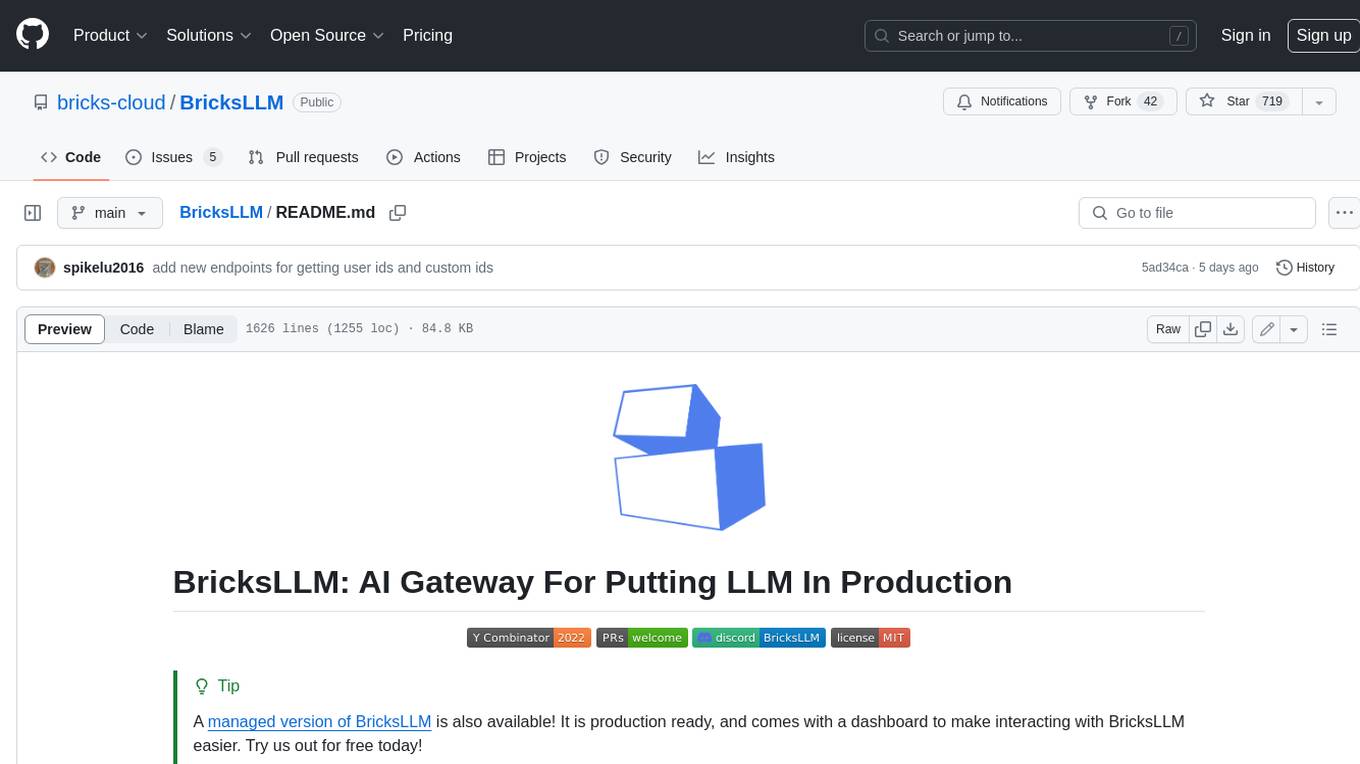
BricksLLM
BricksLLM is a cloud native AI gateway written in Go. Currently, it provides native support for OpenAI, Anthropic, Azure OpenAI and vLLM. BricksLLM aims to provide enterprise level infrastructure that can power any LLM production use cases. Here are some use cases for BricksLLM: * Set LLM usage limits for users on different pricing tiers * Track LLM usage on a per user and per organization basis * Block or redact requests containing PIIs * Improve LLM reliability with failovers, retries and caching * Distribute API keys with rate limits and cost limits for internal development/production use cases * Distribute API keys with rate limits and cost limits for students
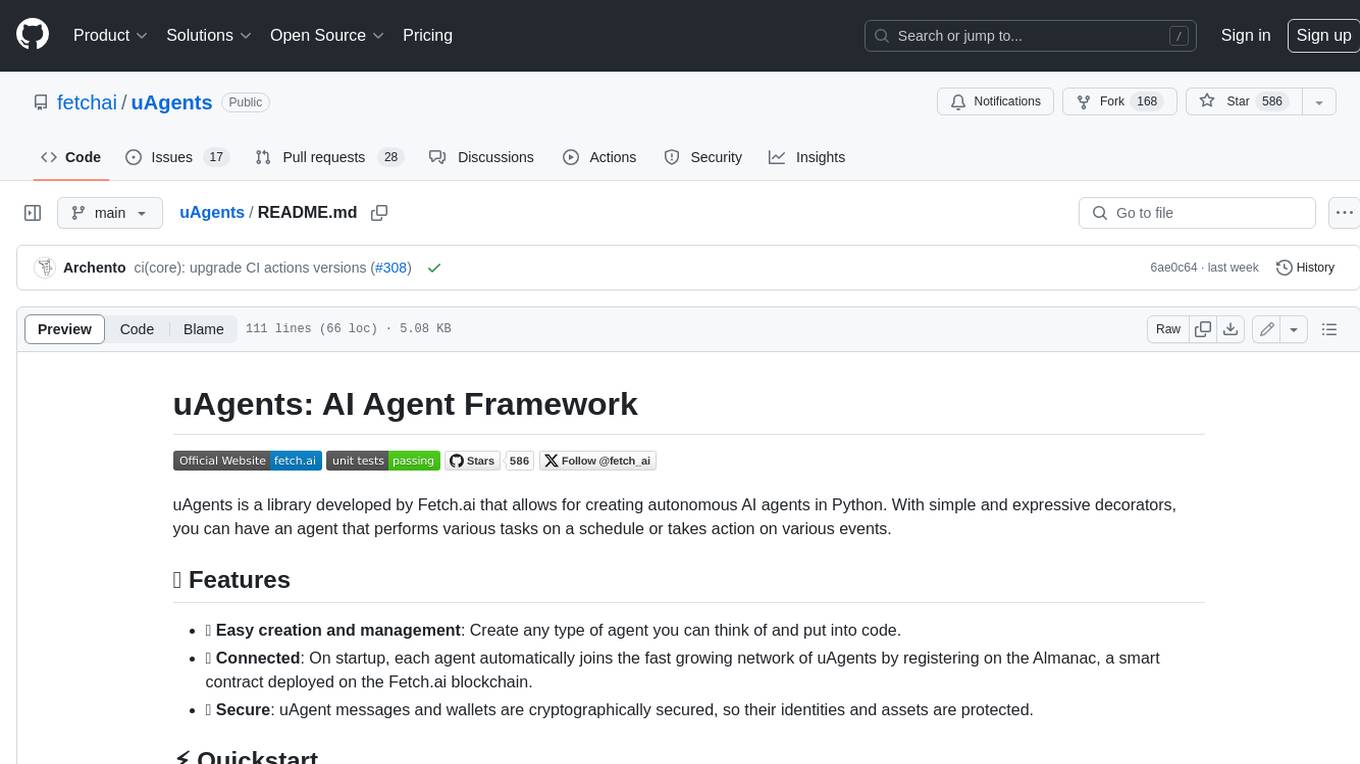
uAgents
uAgents is a Python library developed by Fetch.ai that allows for the creation of autonomous AI agents. These agents can perform various tasks on a schedule or take action on various events. uAgents are easy to create and manage, and they are connected to a fast-growing network of other uAgents. They are also secure, with cryptographically secured messages and wallets.
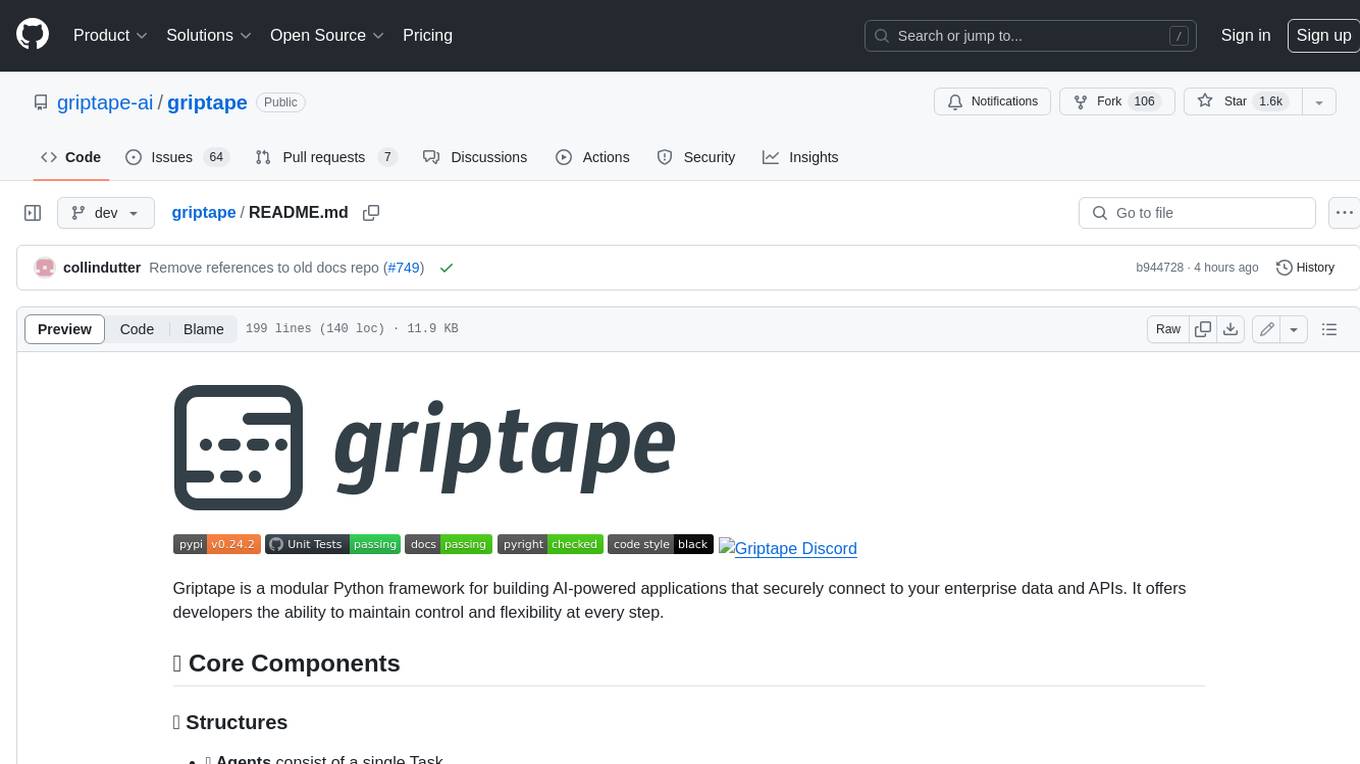
griptape
Griptape is a modular Python framework for building AI-powered applications that securely connect to your enterprise data and APIs. It offers developers the ability to maintain control and flexibility at every step. Griptape's core components include Structures (Agents, Pipelines, and Workflows), Tasks, Tools, Memory (Conversation Memory, Task Memory, and Meta Memory), Drivers (Prompt and Embedding Drivers, Vector Store Drivers, Image Generation Drivers, Image Query Drivers, SQL Drivers, Web Scraper Drivers, and Conversation Memory Drivers), Engines (Query Engines, Extraction Engines, Summary Engines, Image Generation Engines, and Image Query Engines), and additional components (Rulesets, Loaders, Artifacts, Chunkers, and Tokenizers). Griptape enables developers to create AI-powered applications with ease and efficiency.