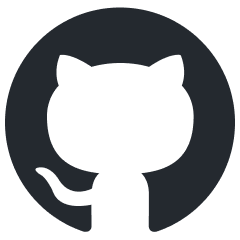
llm-awq
[MLSys 2024 Best Paper Award] AWQ: Activation-aware Weight Quantization for LLM Compression and Acceleration
Stars: 1871
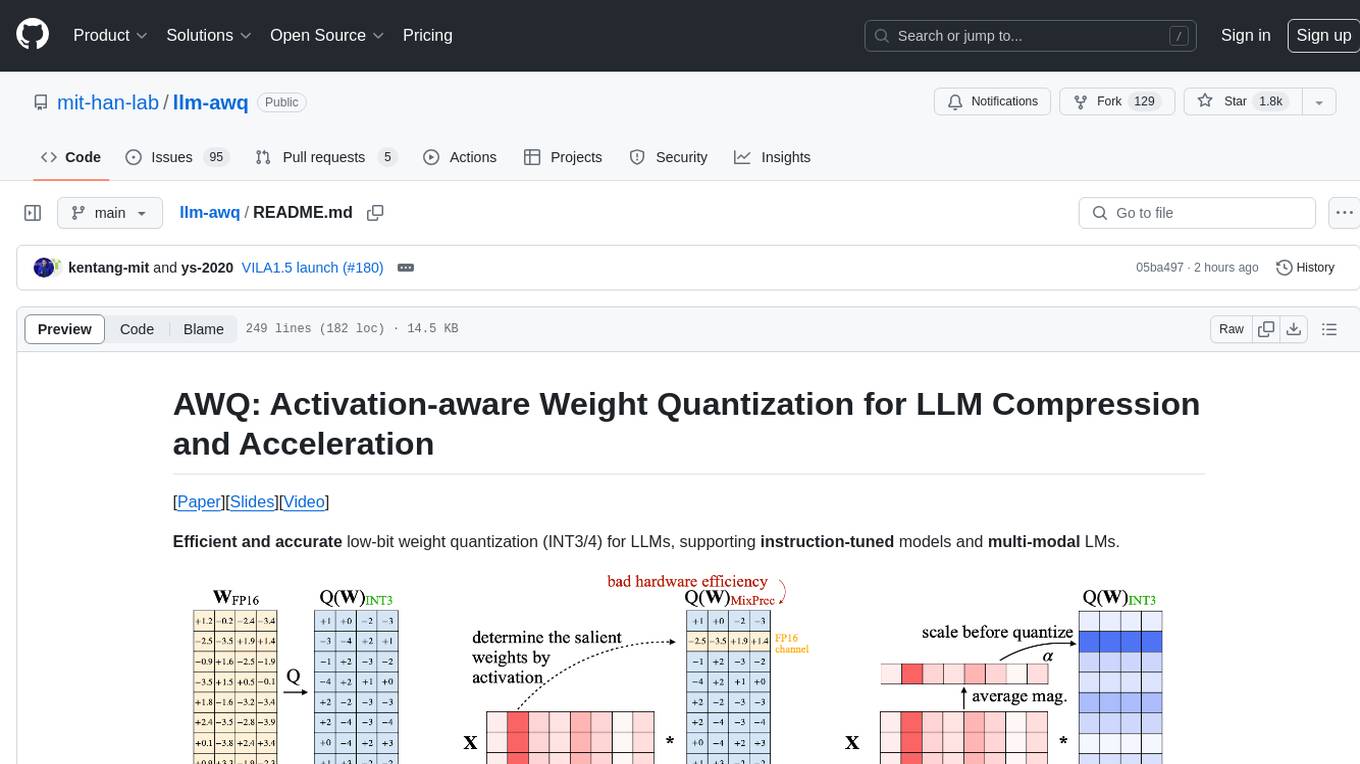
AWQ (Activation-aware Weight Quantization) is a tool designed for efficient and accurate low-bit weight quantization (INT3/4) for Large Language Models (LLMs). It supports instruction-tuned models and multi-modal LMs, providing features such as AWQ search for accurate quantization, pre-computed AWQ model zoo for various LLMs, memory-efficient 4-bit linear in PyTorch, and efficient CUDA kernel implementation for fast inference. The tool enables users to run large models on resource-constrained edge platforms, delivering more efficient responses with LLM/VLM chatbots through 4-bit inference.
README:
Efficient and accurate low-bit weight quantization (INT3/4) for LLMs, supporting instruction-tuned models and multi-modal LMs.
The current release supports:
- AWQ search for accurate quantization.
- Pre-computed AWQ model zoo for LLMs (Llama-1/2/3, OPT, CodeLlama, StarCoder, Vicuna, VILA, LLaVA; load to generate quantized weights).
- Memory-efficient 4-bit Linear in PyTorch.
- Efficient CUDA kernel implementation for fast inference (support context and decoding stage).
- Examples on 4-bit inference of an instruction-tuned model (Vicuna) and multi-modal LM (VILA).
Thanks to AWQ, TinyChat can deliver more efficient responses with LLM/VLM chatbots through 4-bit inference.
- TinyChat on RTX 4090 (3.4x faster than FP16):
- TinyChat on Jetson Orin (3.2x faster than FP16):
TinyChat also supports inference with vision language models (e.g., VILA, LLaVA). In the following examples, W4A16 quantized models from VILA family are launched with TinyChat.
- TinyChat with VILA-13B on RTX 4090 (multi-image inputs supported):
- TinyChat with VILA-7B/13B on Jetson Orin:
Check out TinyChat, which offers a turn-key solution for on-device inference of LLMs and VLMs on resource-constrained edge platforms. With TinyChat, it is now possible to efficiently run large models on small and low-power devices even without Internet connection!
- [2024/05] ๐ AWQ receives the Best Paper Award at MLSys 2024. ๐
- [2024/05] ๐ฅ The VILA-1.5 model family which features video understanding is now supported in AWQ and TinyChat. Check out out online demo powered by TinyChat here. Example is here.
- [2024/04] ๐ฅ We released AWQ and TinyChat support for The Llama-3 model family! Check out our example here.
- [2024/03] ๐ฅ AWQ has been widely adopted by the industry, such as NVIDIA, Google, Amazon, and Intel!
- [2024/02] ๐ฅ AWQ has been accepted to MLSys 2024!
- [2024/02] ๐ฅ We supported VILA Vision Languague Models in AWQ & TinyChat! Check our latest demos with multi-image inputs!
- [2024/02] ๐ฅ We released new version of quantized GEMM/GEMV kernels in TinyChat, leading to 38 tokens/second inference speed on NVIDIA Jetson Orin!
- [2023/11] ๐ฅ We added AWQ support and pre-computed search results for CodeLlama, StarCoder, StableCode models. Checkout our model zoo here!
- [2023/11] ๐ฅ AWQ is now integrated natively in Hugging Face transformers through
from_pretrained
. You can either load quantized models from the Hub or your own HF quantized models. - [2023/10] AWQ is integrated into NVIDIA TensorRT-LLM
- [2023/09] AWQ is integrated into FastChat, vLLM, HuggingFace TGI, and LMDeploy.
- [2023/09] โก Check out our latest TinyChat, which is ~2x faster than the first release on Orin!
- [2023/09] โก Check out AutoAWQ, a third-party implementation to make AWQ easier to expand to new models, improve inference speed, and integrate into Huggingface.
- [2023/07] ๐ฅ We released TinyChat, an efficient and lightweight chatbot interface based on AWQ. TinyChat enables efficient LLM inference on both cloud and edge GPUs. Llama-2-chat models are supported! Check out our implementation here.
- [2023/07] ๐ฅ We added AWQ support and pre-computed search results for Llama-2 models (7B & 13B). Checkout our model zoo here!
- [2023/07] We extended the support for more LLM models including MPT, Falcon, and BLOOM.
- Clone this repository and navigate to AWQ folder
git clone https://github.com/mit-han-lab/llm-awq
cd llm-awq
- Install Package
conda create -n awq python=3.10 -y
conda activate awq
pip install --upgrade pip # enable PEP 660 support
pip install -e .
-
For edge devices like Orin, before running the commands above, please:
- Modify pyproject.toml by commenting out this line.
- Set this line to transformers==4.32.0.
- Manually install precompiled PyTorch binaries (>=2.0.0) from NVIDIA.
- Set the appropriate Python version for conda environment (e.g.,
conda create -n awq python=3.8 -y
for JetPack 5).
- Install efficient W4A16 (4-bit weight, 16-bit activation) CUDA kernel and optimized FP16 kernels (e.g. layernorm, positional encodings).
cd awq/kernels
python setup.py install
- In order to run AWQ and TinyChat with VILA-1.5 model family, please install VILA:
git clone [email protected]:Efficient-Large-Model/VILA.git
cd VILA
pip install -e .
We provide pre-computed AWQ search results for multiple model families, including LLaMA, OPT, Vicuna, and LLaVA. To get the pre-computed AWQ search results, run:
# git lfs install # install git lfs if not already
git clone https://huggingface.co/datasets/mit-han-lab/awq-model-zoo awq_cache
The detailed support list:
Models | Sizes | INT4-g128 | INT3-g128 |
---|---|---|---|
VILA-1.5 | 3B/8B/13B/40B | โ | โ |
Llama3 | 8B/70B | โ | โ |
VILA | 7B/13B | โ | |
Llama2 | 7B/13B/70B | โ | โ |
LLaMA | 7B/13B/30B/65B | โ | โ |
OPT | 125m/1.3B/2.7B/6.7B/13B/30B | โ | โ |
CodeLlama | 7B/13B/34B | โ | โ |
StarCoder | 15.5B | โ | โ |
Vicuna-v1.1 | 7B/13B | โ | |
LLaVA-v0 | 13B | โ |
Note: We only list models that we have prepare the AWQ searching results in the table above. AWQ also supports models such as LLaVA-v1.5 7B, and you may need to run the AWQ search on your own to quantize these models.
AWQ can be easily applied to various LMs thanks to its good generalization, including instruction-tuned models and multi-modal LMs. It provides an easy-to-use tool to reduce the serving cost of LLMs.
Here we provide two examples of AWQ application: Vicuna-7B (chatbot) and LLaVA-13B (visual reasoning) under ./examples
directory. AWQ can easily reduce the GPU memory of model serving and speed up token generation. It provides accurate quantization, providing reasoning outputs. You should be able to observe memory savings when running the models with 4-bit weights.
Note that we perform AWQ using only textual calibration data, depsite we are running on multi-modal input. Please refer to ./examples
for details.
We provide several sample script to run AWQ (please refer to ./scripts
). We use Llama3-8B as an example.
- Perform AWQ search and save search results (we already did it for you):
python -m awq.entry --model_path /PATH/TO/LLAMA3/llama3-8b \
--w_bit 4 --q_group_size 128 \
--run_awq --dump_awq awq_cache/llama3-8b-w4-g128.pt
- Evaluate the AWQ quantized model on WikiText-2 (simulated pseudo quantization)
python -m awq.entry --model_path /PATH/TO/LLAMA3/llama3-8b \
--tasks wikitext \
--w_bit 4 --q_group_size 128 \
--load_awq awq_cache/llama3-8b-w4-g128.pt \
--q_backend fake
- Generate real quantized weights (INT4)
mkdir quant_cache
python -m awq.entry --model_path /PATH/TO/LLAMA3/llama3-8b \
--w_bit 4 --q_group_size 128 \
--load_awq awq_cache/llama3-8b-w4-g128.pt \
--q_backend real --dump_quant quant_cache/llama3-8b-w4-g128-awq.pt
- Load and evaluate the real quantized model (now you can see smaller gpu memory usage)
python -m awq.entry --model_path /PATH/TO/LLAMA3/llama3-8b \
--tasks wikitext \
--w_bit 4 --q_group_size 128 \
--load_quant quant_cache/llama3-8b-w4-g128-awq.pt
AWQ also seamlessly supports large multi-modal models (LMMs). We demonstrate the results on the recent VILA-1.5 model family.
VILA-1.5-3B | VQA-v2 | GQA | VizWiz | ScienceQA | TextVQA | POPE | MME | MMBench | MMBench-CN | SEED |
---|---|---|---|---|---|---|---|---|---|---|
FP16 | 80.4 | 61.5 | 53.5 | 69.0 | 60.4 | 85.9 | 1442.4 | 63.4 | 52.7 | 60.9 |
AWQ-INT4 | 80.0 | 61.1 | 53.8 | 67.8 | 60.4 | 85.9 | 1437.3 | 63.3 | 51.4 | 59.8 |
VILA-1.5-8B | VQA-v2 | GQA | VizWiz | ScienceQA | TextVQA | POPE | MME | MMBench | MMBench-CN | SEED |
---|---|---|---|---|---|---|---|---|---|---|
FP16 | 80.9 | 61.9 | 58.7 | 79.9 | 66.3 | 84.4 | 1577.01 | 72.3 | 66.2 | 64.2 |
AWQ-INT4 | 80.3 | 61.7 | 59.3 | 79.0 | 65.4 | 82.9 | 1593.65 | 71.0 | 64.9 | 64.0 |
VILA-1.5-13B | VQA-v2 | GQA | VizWiz | ScienceQA | TextVQA | POPE | MME | MMBench | MMBench-CN | SEED |
---|---|---|---|---|---|---|---|---|---|---|
FP16 | 82.8 | 64.3 | 62.6 | 80.1 | 65.0 | 86.3 | 1569.55 | 74.9 | 66.3 | 65.1 |
AWQ-INT4 | 82.7 | 64.5 | 63.3 | 79.7 | 64.7 | 86.7 | 1531.35 | 74.7 | 66.7 | 65.1 |
VILA-1.5-40B | VQA-v2 | GQA | VizWiz | ScienceQA | TextVQA | POPE | MME | MMBench | MMBench-CN | SEED |
---|---|---|---|---|---|---|---|---|---|---|
FP16 | 84.3 | 64.6 | 62.2 | 87.2 | 73.6 | 87.3 | 1726.82 | 82.4 | 80.2 | 69.1 |
AWQ-INT4 | 84.1 | 64.4 | 61.3 | 86.7 | 73.2 | 88.2 | 1714.79 | 83.2 | 79.6 | 68.9 |
$~~~~~~$ | Precision | A100 | 4090 | Orin |
---|---|---|---|---|
VILA1.5-3B | fp16 | 104.6 | 137.6 | 25.4 |
VILA1.5-3B-AWQ | int4 | 182.8 | 215.5 | 42.5 |
VILA1.5-3B-S2 | fp16 | 104.3 | 137.2 | 24.6 |
VILA1.5-3B-S2-AWQ | int4 | 180.2 | 219.3 | 40.1 |
Llama-3-VILA1.5-8B | fp16 | 74.9 | 57.4 | 10.2 |
Llama-3-VILA1.5-8B-AWQ | int4 | 168.9 | 150.2 | 28.7 |
VILA1.5-13B | fp16 | 50.9 | OOM | 6.1 |
VILA1.5-13B-AWQ | int4 | 115.9 | 105.7 | 20.6 |
VILA1.5-40B | fp16 | OOM | OOM | -- |
VILA1.5-40B-AWQ | int4 | 57.0 | OOM | -- |
If you find AWQ useful or relevant to your research, please kindly cite our paper:
@inproceedings{lin2023awq,
title={AWQ: Activation-aware Weight Quantization for LLM Compression and Acceleration},
author={Lin, Ji and Tang, Jiaming and Tang, Haotian and Yang, Shang and Chen, Wei-Ming and Wang, Wei-Chen and Xiao, Guangxuan and Dang, Xingyu and Gan, Chuang and Han, Song},
booktitle={MLSys},
year={2024}
}
SmoothQuant: Accurate and Efficient Post-Training Quantization for Large Language Models
GPTQ: Accurate Post-training Compression for Generative Pretrained Transformers
For Tasks:
Click tags to check more tools for each tasksFor Jobs:
Alternative AI tools for llm-awq
Similar Open Source Tools
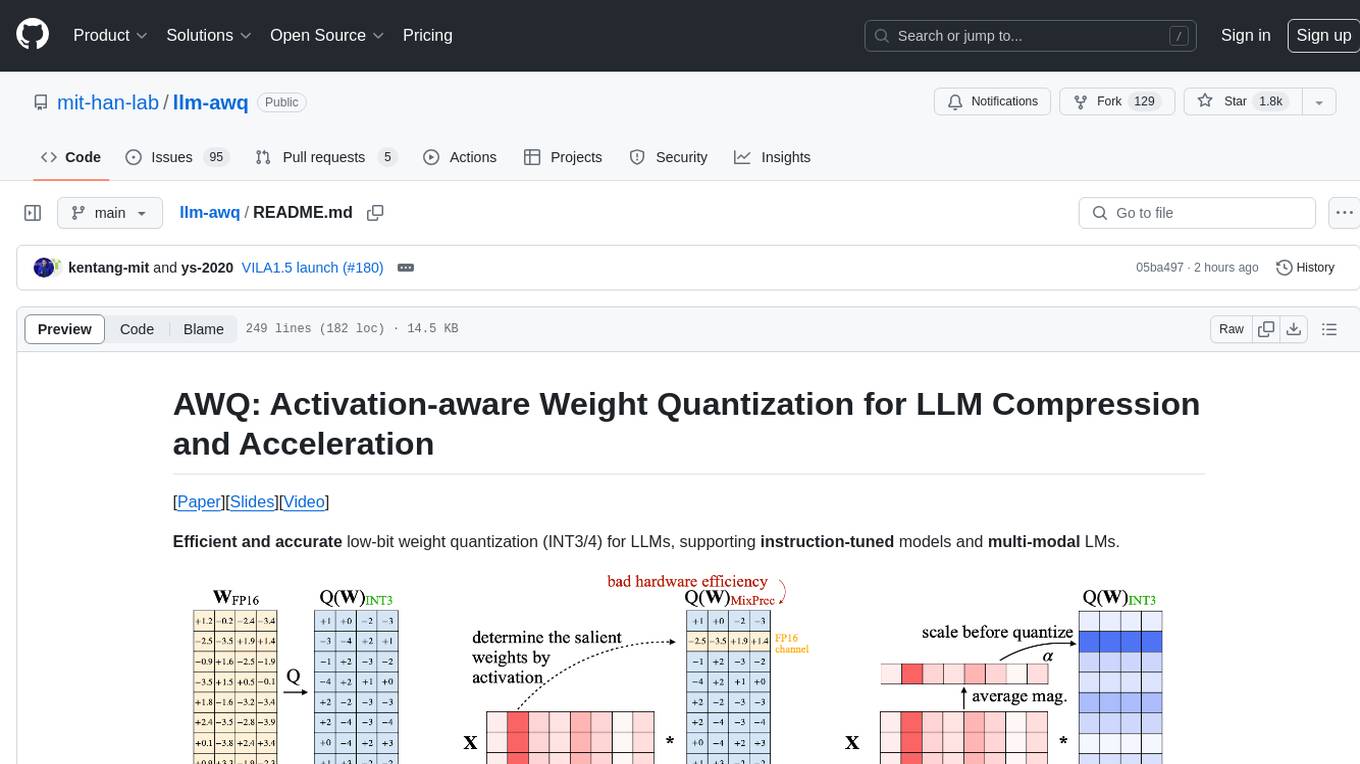
llm-awq
AWQ (Activation-aware Weight Quantization) is a tool designed for efficient and accurate low-bit weight quantization (INT3/4) for Large Language Models (LLMs). It supports instruction-tuned models and multi-modal LMs, providing features such as AWQ search for accurate quantization, pre-computed AWQ model zoo for various LLMs, memory-efficient 4-bit linear in PyTorch, and efficient CUDA kernel implementation for fast inference. The tool enables users to run large models on resource-constrained edge platforms, delivering more efficient responses with LLM/VLM chatbots through 4-bit inference.
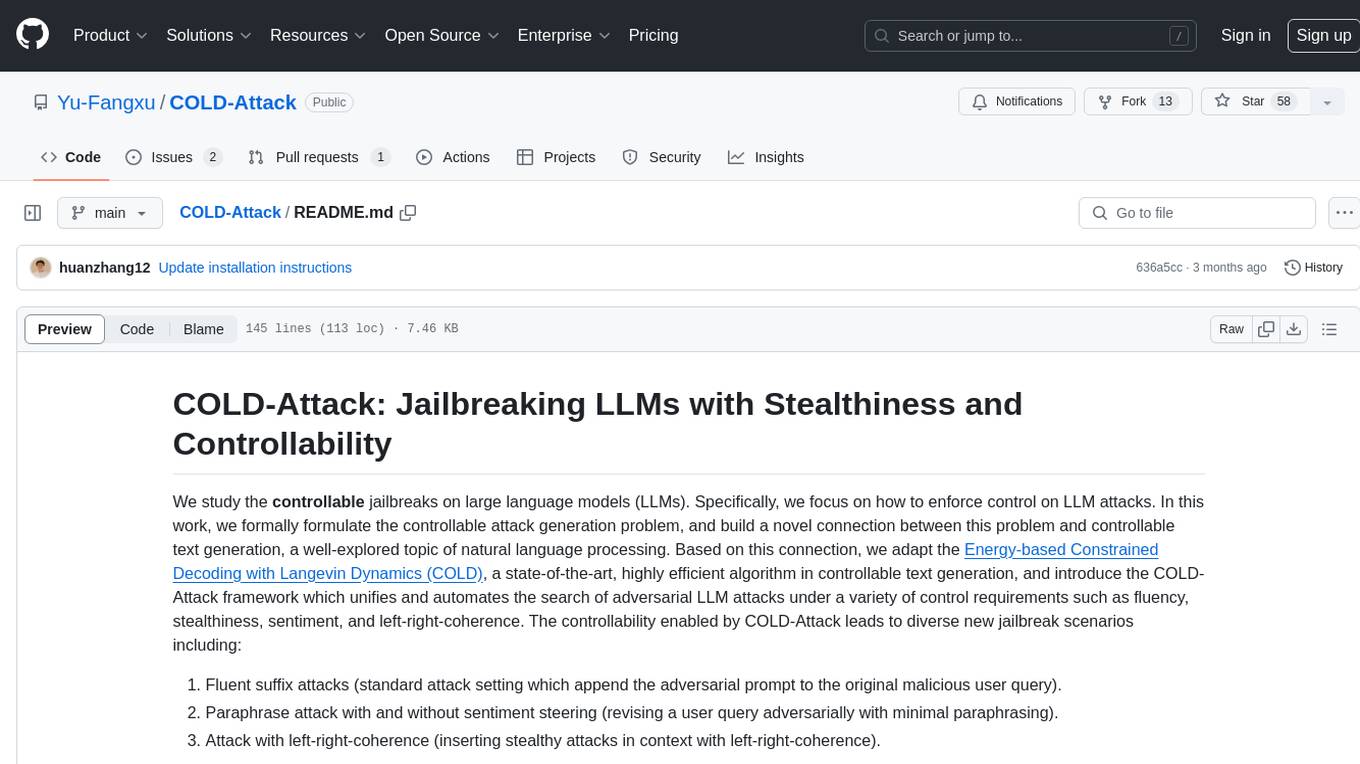
COLD-Attack
COLD-Attack is a framework designed for controllable jailbreaks on large language models (LLMs). It formulates the controllable attack generation problem and utilizes the Energy-based Constrained Decoding with Langevin Dynamics (COLD) algorithm to automate the search of adversarial LLM attacks with control over fluency, stealthiness, sentiment, and left-right-coherence. The framework includes steps for energy function formulation, Langevin dynamics sampling, and decoding process to generate discrete text attacks. It offers diverse jailbreak scenarios such as fluent suffix attacks, paraphrase attacks, and attacks with left-right-coherence.
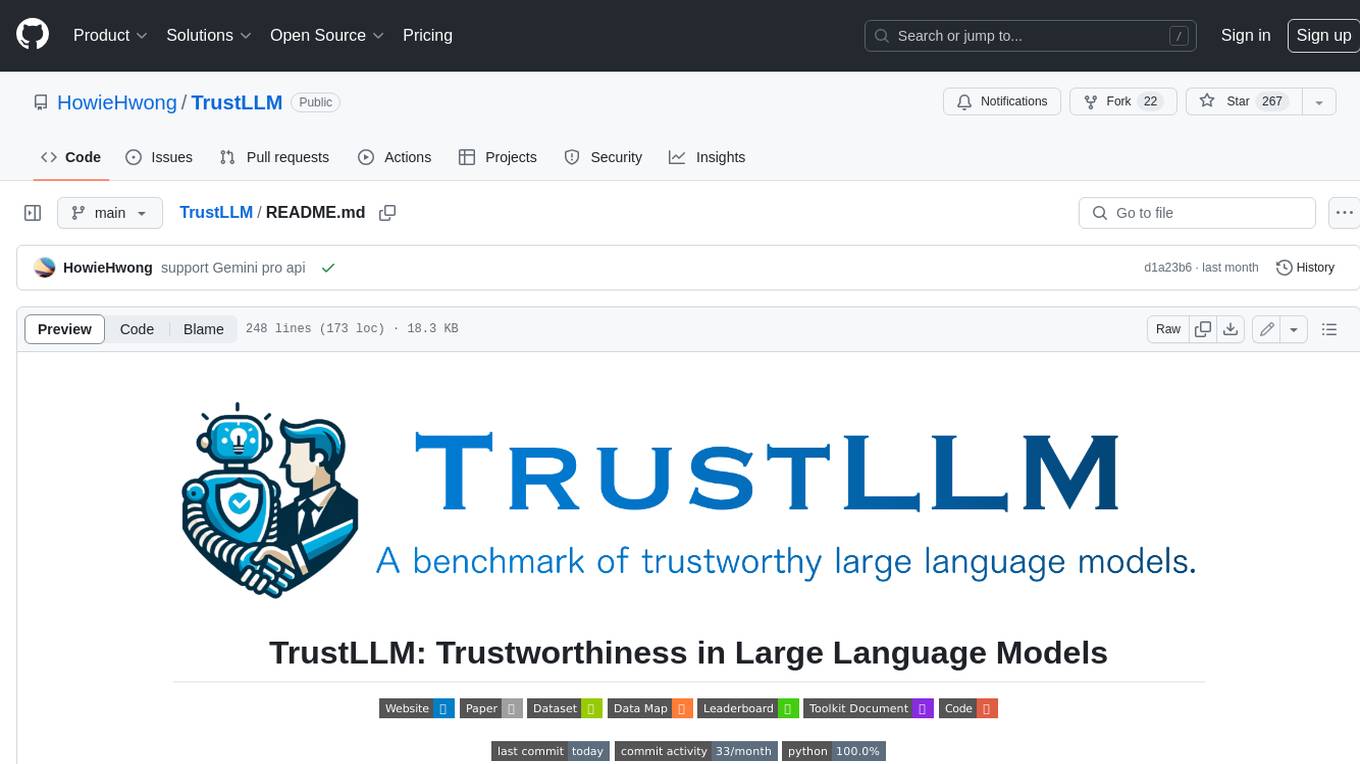
TrustLLM
TrustLLM is a comprehensive study of trustworthiness in LLMs, including principles for different dimensions of trustworthiness, established benchmark, evaluation, and analysis of trustworthiness for mainstream LLMs, and discussion of open challenges and future directions. Specifically, we first propose a set of principles for trustworthy LLMs that span eight different dimensions. Based on these principles, we further establish a benchmark across six dimensions including truthfulness, safety, fairness, robustness, privacy, and machine ethics. We then present a study evaluating 16 mainstream LLMs in TrustLLM, consisting of over 30 datasets. The document explains how to use the trustllm python package to help you assess the performance of your LLM in trustworthiness more quickly. For more details about TrustLLM, please refer to project website.
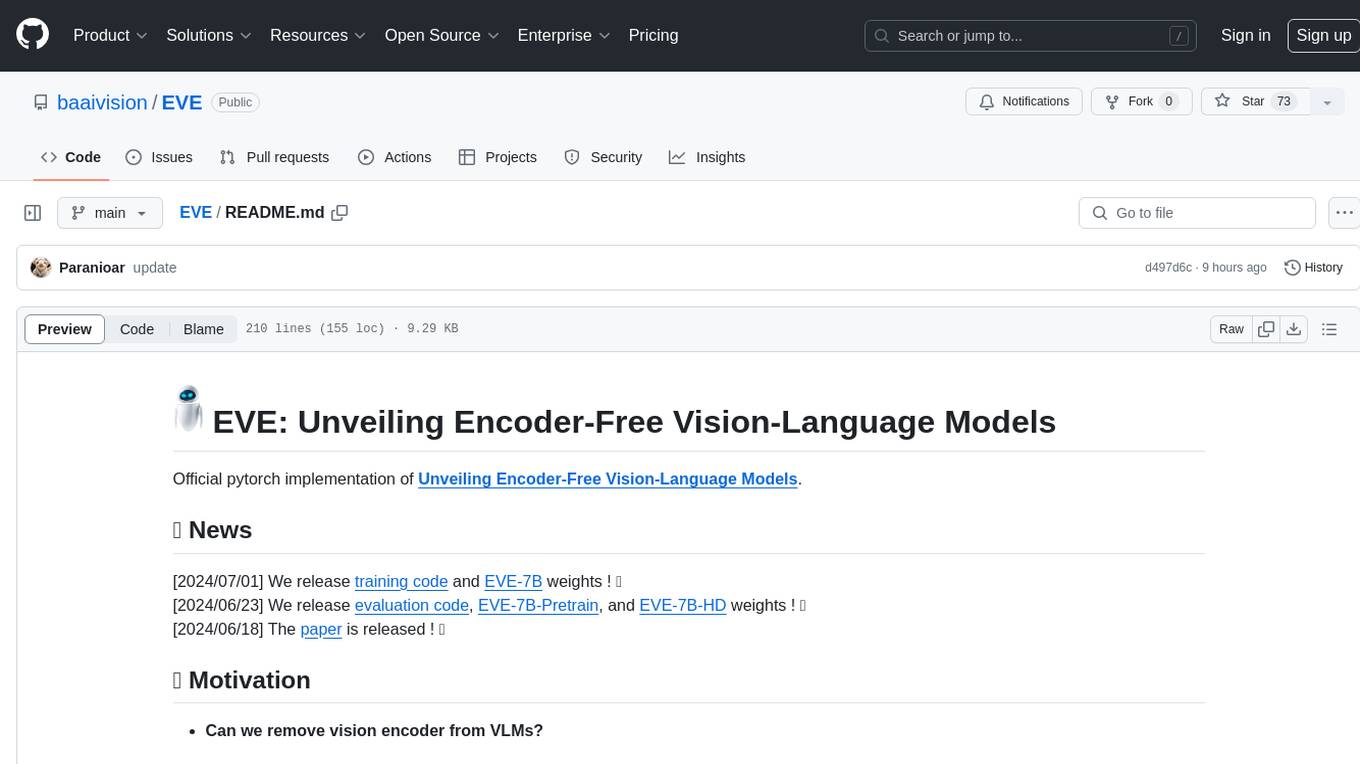
EVE
EVE is an official PyTorch implementation of Unveiling Encoder-Free Vision-Language Models. The project aims to explore the removal of vision encoders from Vision-Language Models (VLMs) and transfer LLMs to encoder-free VLMs efficiently. It also focuses on bridging the performance gap between encoder-free and encoder-based VLMs. EVE offers a superior capability with arbitrary image aspect ratio, data efficiency by utilizing publicly available data for pre-training, and training efficiency with a transparent and practical strategy for developing a pure decoder-only architecture across modalities.
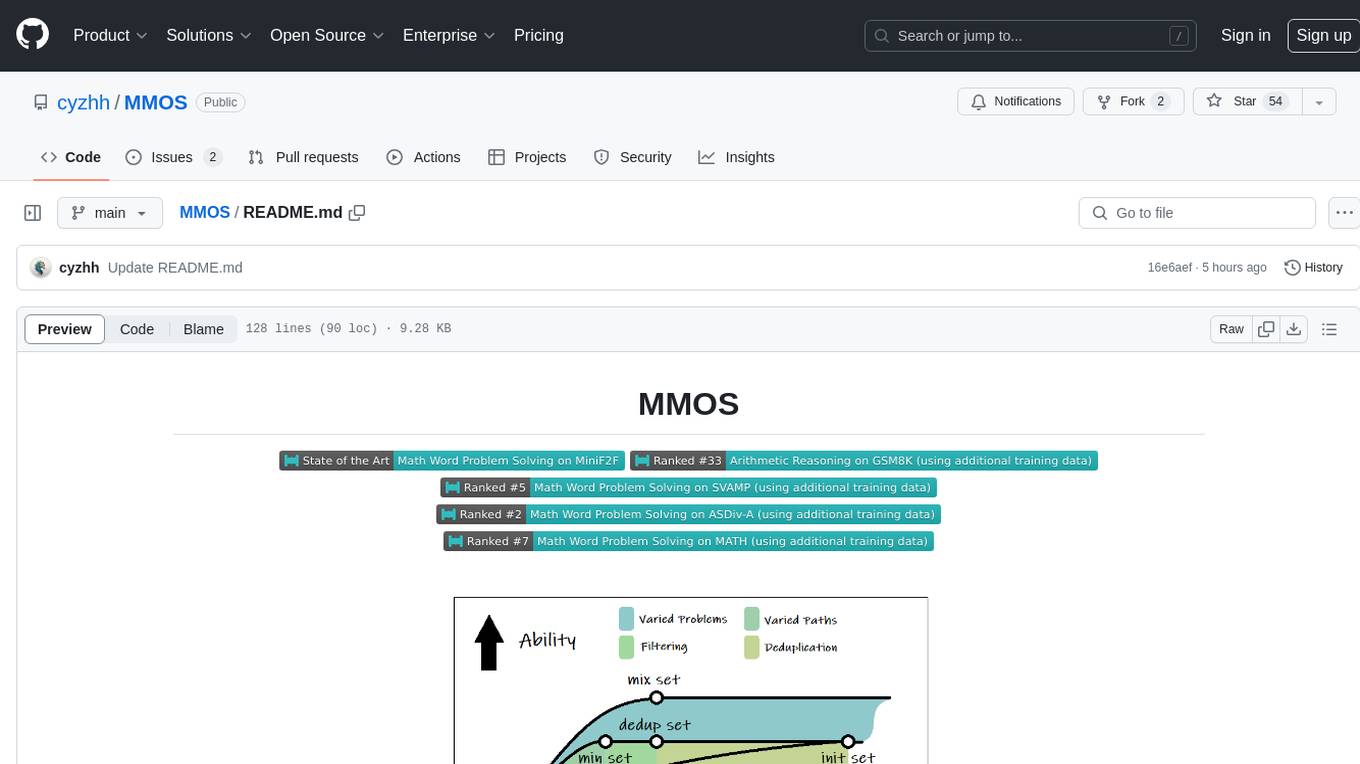
MMOS
MMOS (Mix of Minimal Optimal Sets) is a dataset designed for math reasoning tasks, offering higher performance and lower construction costs. It includes various models and data subsets for tasks like arithmetic reasoning and math word problem solving. The dataset is used to identify minimal optimal sets through reasoning paths and statistical analysis, with a focus on QA-pairs generated from open-source datasets. MMOS also provides an auto problem generator for testing model robustness and scripts for training and inference.
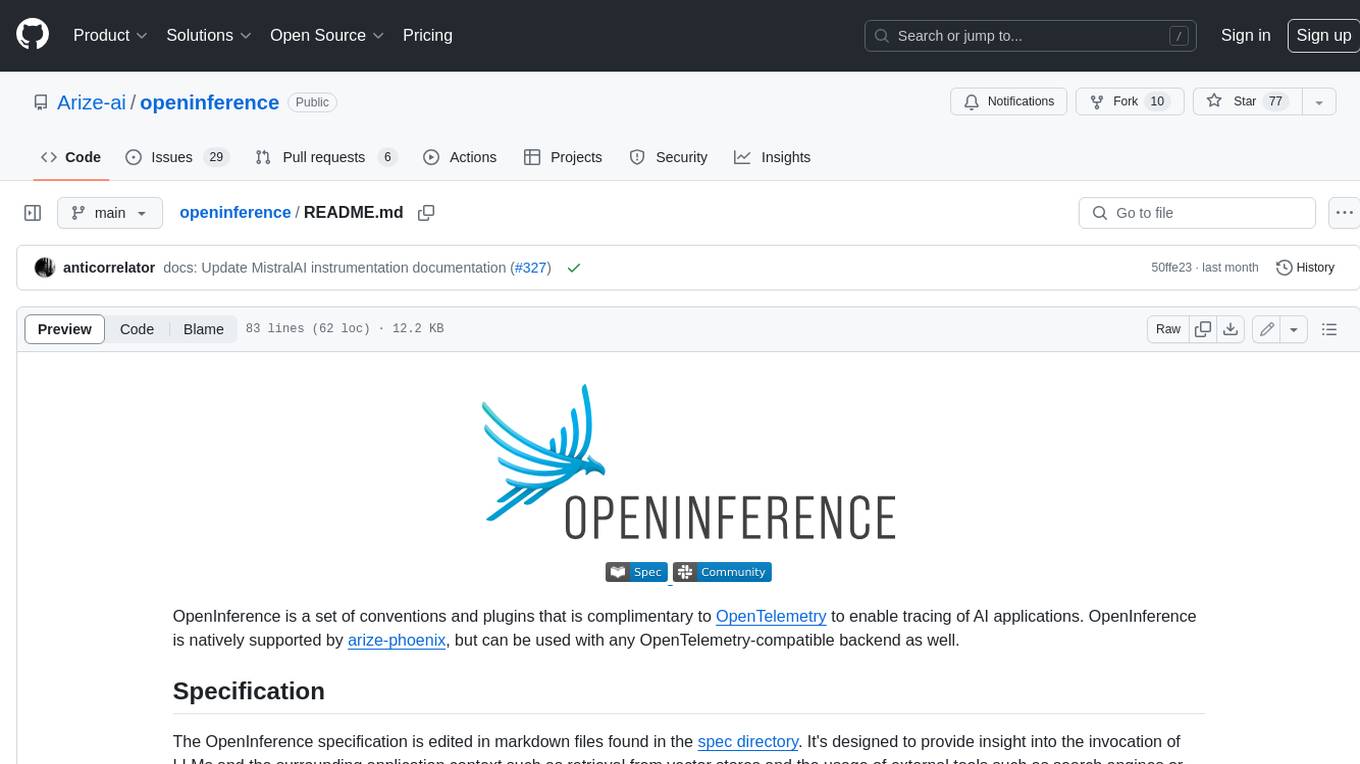
openinference
OpenInference is a set of conventions and plugins that complement OpenTelemetry to enable tracing of AI applications. It provides a way to capture and analyze the performance and behavior of AI models, including their interactions with other components of the application. OpenInference is designed to be language-agnostic and can be used with any OpenTelemetry-compatible backend. It includes a set of instrumentations for popular machine learning SDKs and frameworks, making it easy to add tracing to your AI applications.
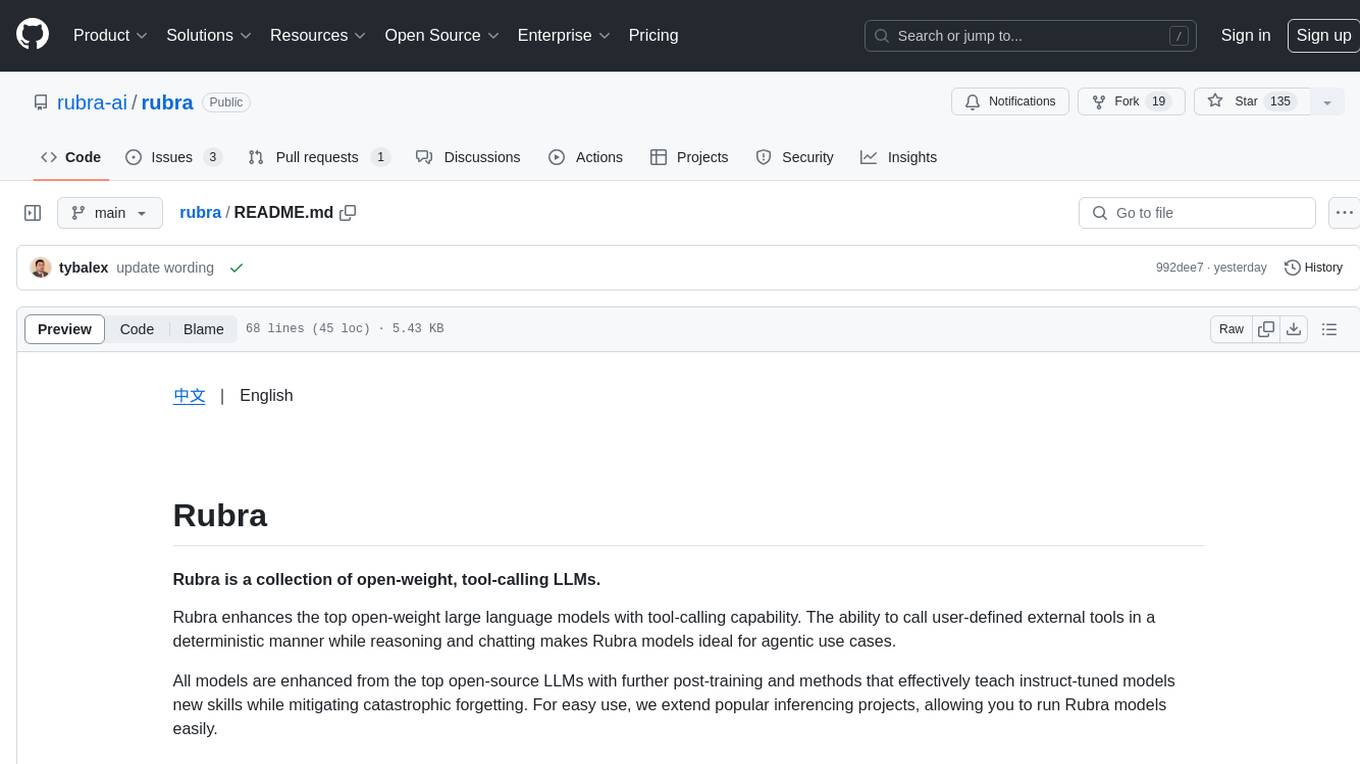
rubra
Rubra is a collection of open-weight large language models enhanced with tool-calling capability. It allows users to call user-defined external tools in a deterministic manner while reasoning and chatting, making it ideal for agentic use cases. The models are further post-trained to teach instruct-tuned models new skills and mitigate catastrophic forgetting. Rubra extends popular inferencing projects for easy use, enabling users to run the models easily.
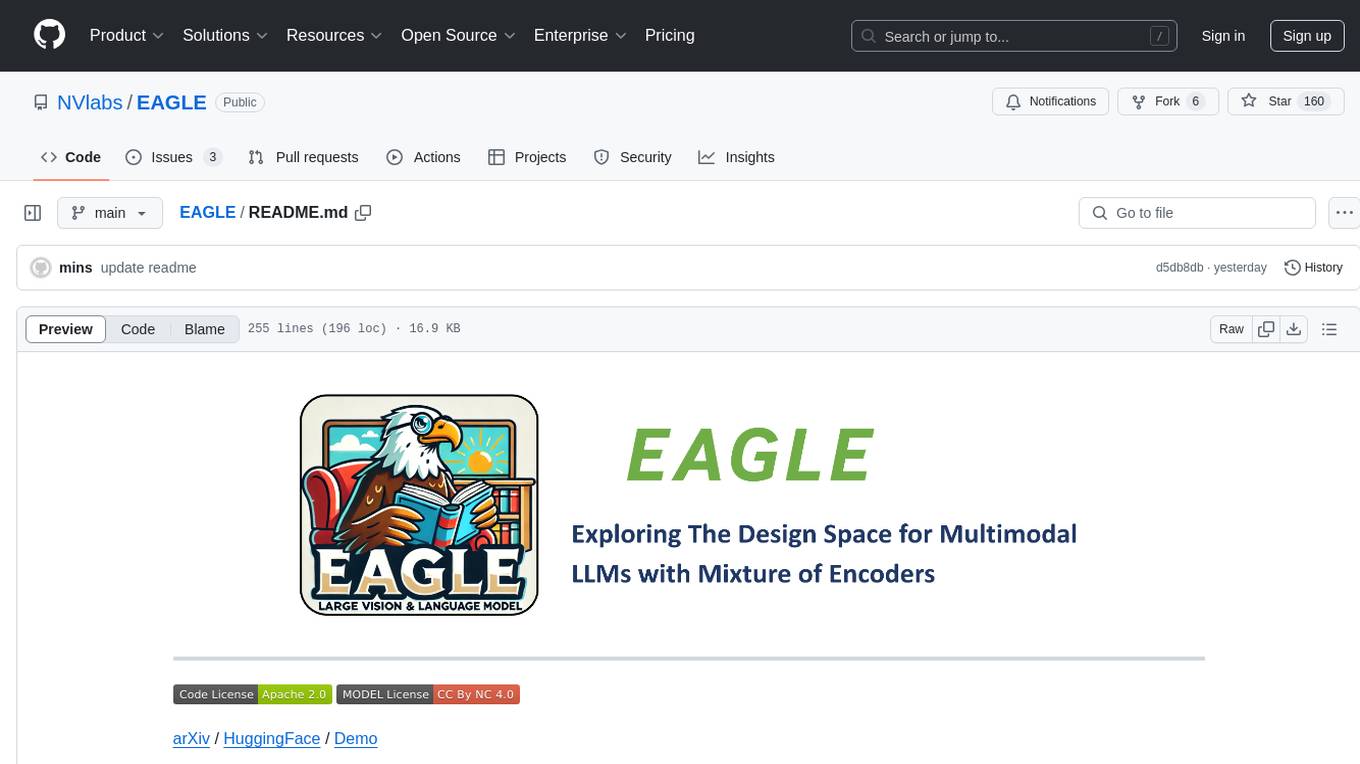
EAGLE
Eagle is a family of Vision-Centric High-Resolution Multimodal LLMs that enhance multimodal LLM perception using a mix of vision encoders and various input resolutions. The model features a channel-concatenation-based fusion for vision experts with different architectures and knowledge, supporting up to over 1K input resolution. It excels in resolution-sensitive tasks like optical character recognition and document understanding.
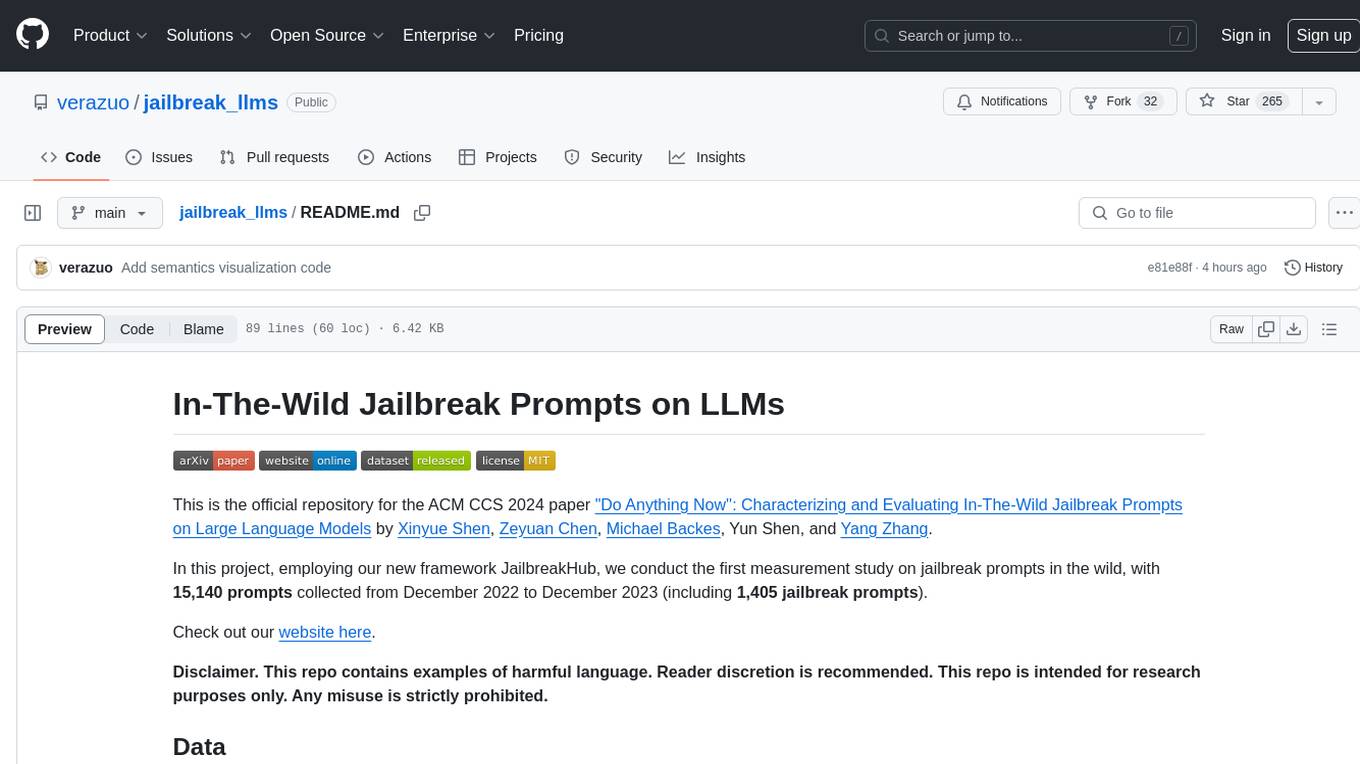
jailbreak_llms
This is the official repository for the ACM CCS 2024 paper 'Do Anything Now': Characterizing and Evaluating In-The-Wild Jailbreak Prompts on Large Language Models. The project employs a new framework called JailbreakHub to conduct the first measurement study on jailbreak prompts in the wild, collecting 15,140 prompts from December 2022 to December 2023, including 1,405 jailbreak prompts. The dataset serves as the largest collection of in-the-wild jailbreak prompts. The repository contains examples of harmful language and is intended for research purposes only.
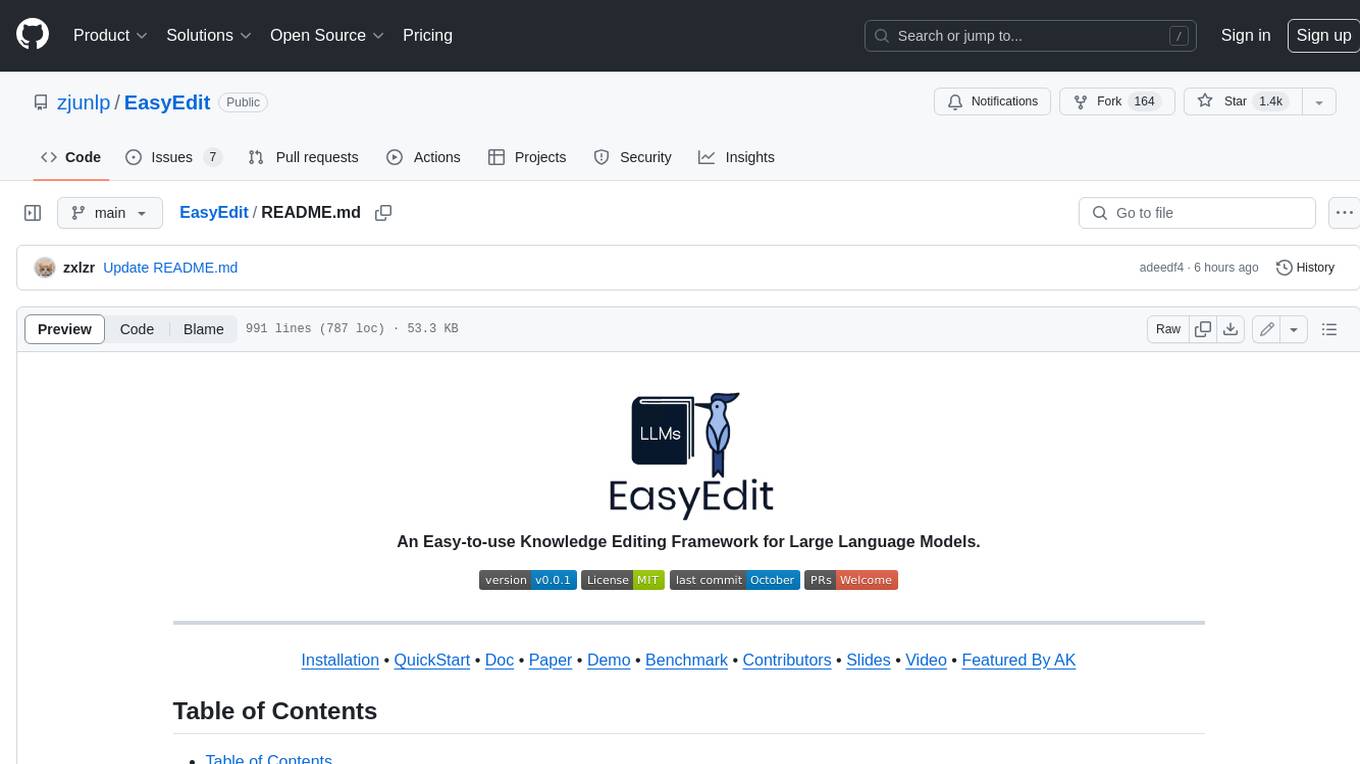
EasyEdit
EasyEdit is a Python package for edit Large Language Models (LLM) like `GPT-J`, `Llama`, `GPT-NEO`, `GPT2`, `T5`(support models from **1B** to **65B**), the objective of which is to alter the behavior of LLMs efficiently within a specific domain without negatively impacting performance across other inputs. It is designed to be easy to use and easy to extend.
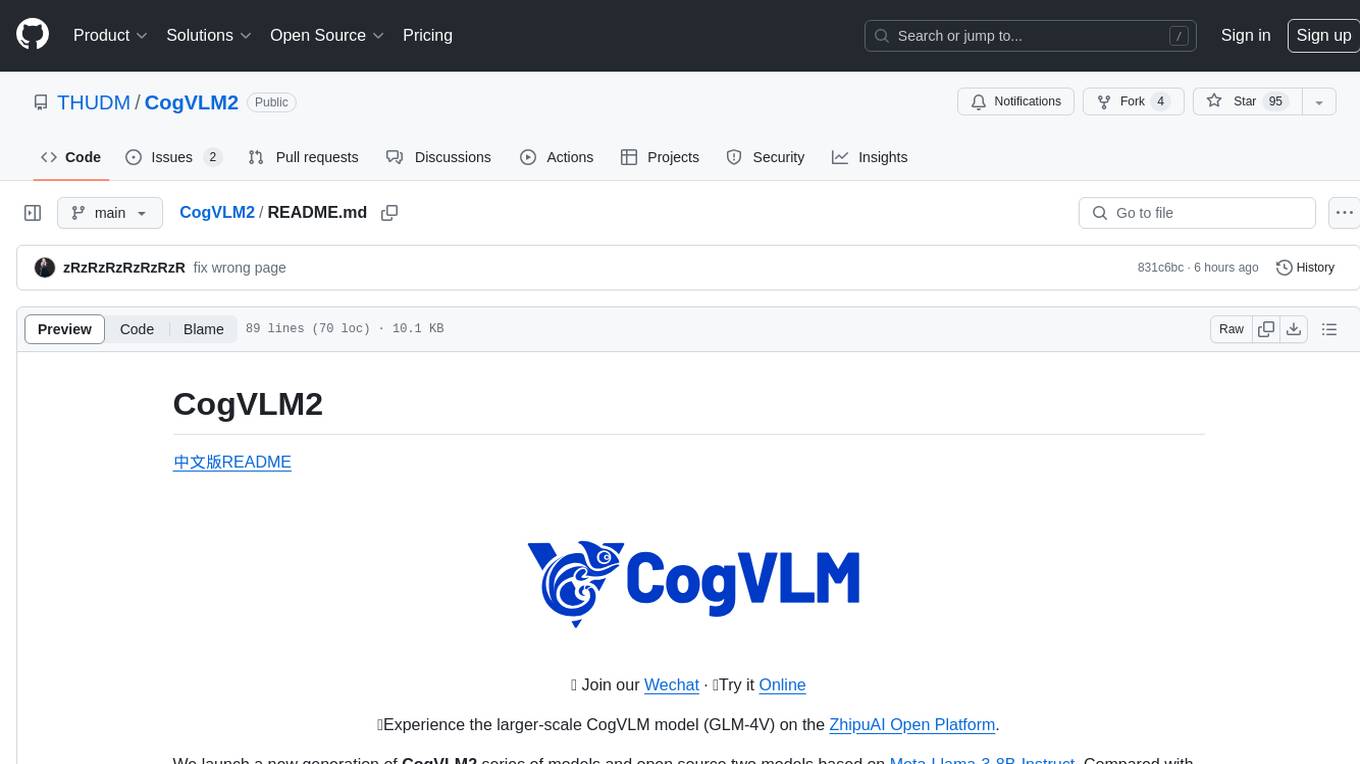
CogVLM2
CogVLM2 is a new generation of open source models that offer significant improvements in benchmarks such as TextVQA and DocVQA. It supports 8K content length, image resolution up to 1344 * 1344, and both Chinese and English languages. The project provides basic calling methods, fine-tuning examples, and OpenAI API format calling examples to help developers quickly get started with the model.
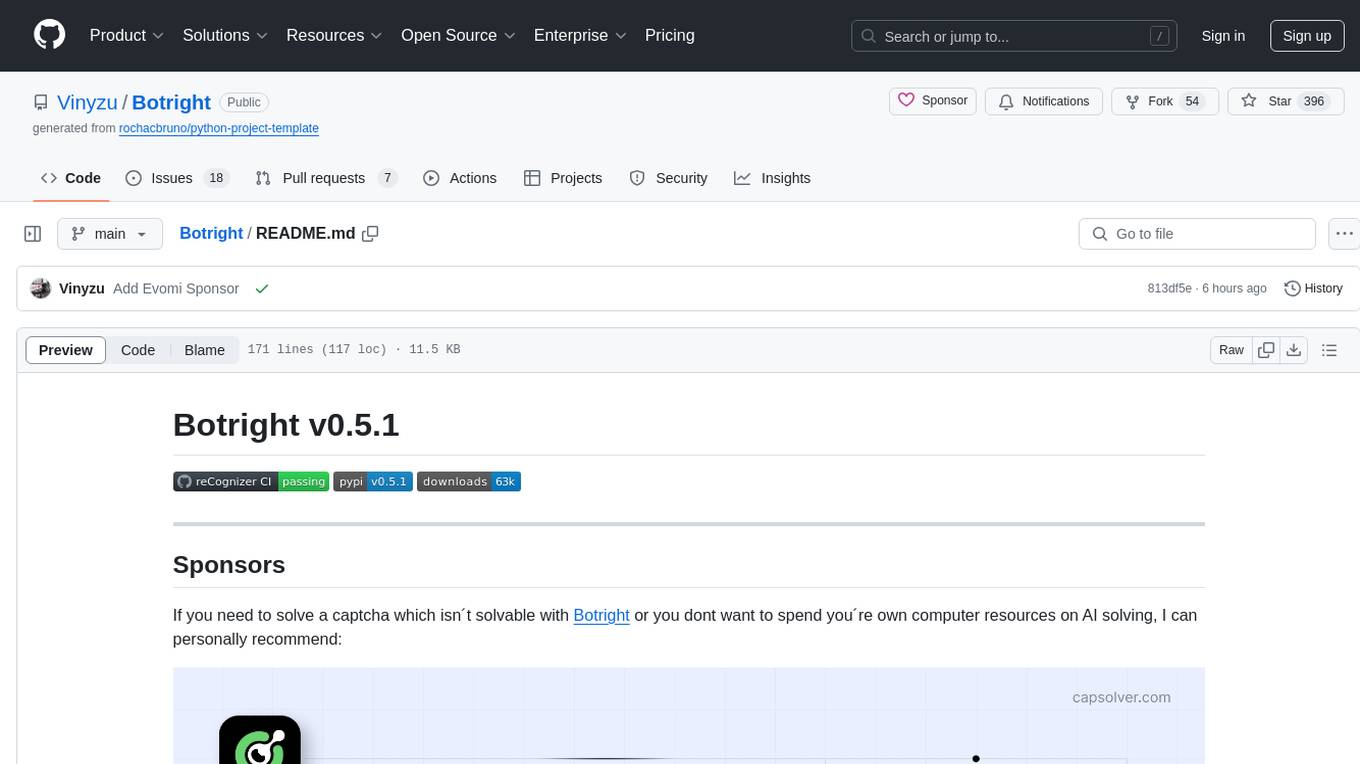
Botright
Botright is a tool designed for browser automation that focuses on stealth and captcha solving. It uses a real Chromium-based browser for enhanced stealth and offers features like browser fingerprinting and AI-powered captcha solving. The tool is suitable for developers looking to automate browser tasks while maintaining anonymity and bypassing captchas. Botright is available in async mode and can be easily integrated with existing Playwright code. It provides solutions for various captchas such as hCaptcha, reCaptcha, and GeeTest, with high success rates. Additionally, Botright offers browser stealth techniques and supports different browser functionalities for seamless automation.
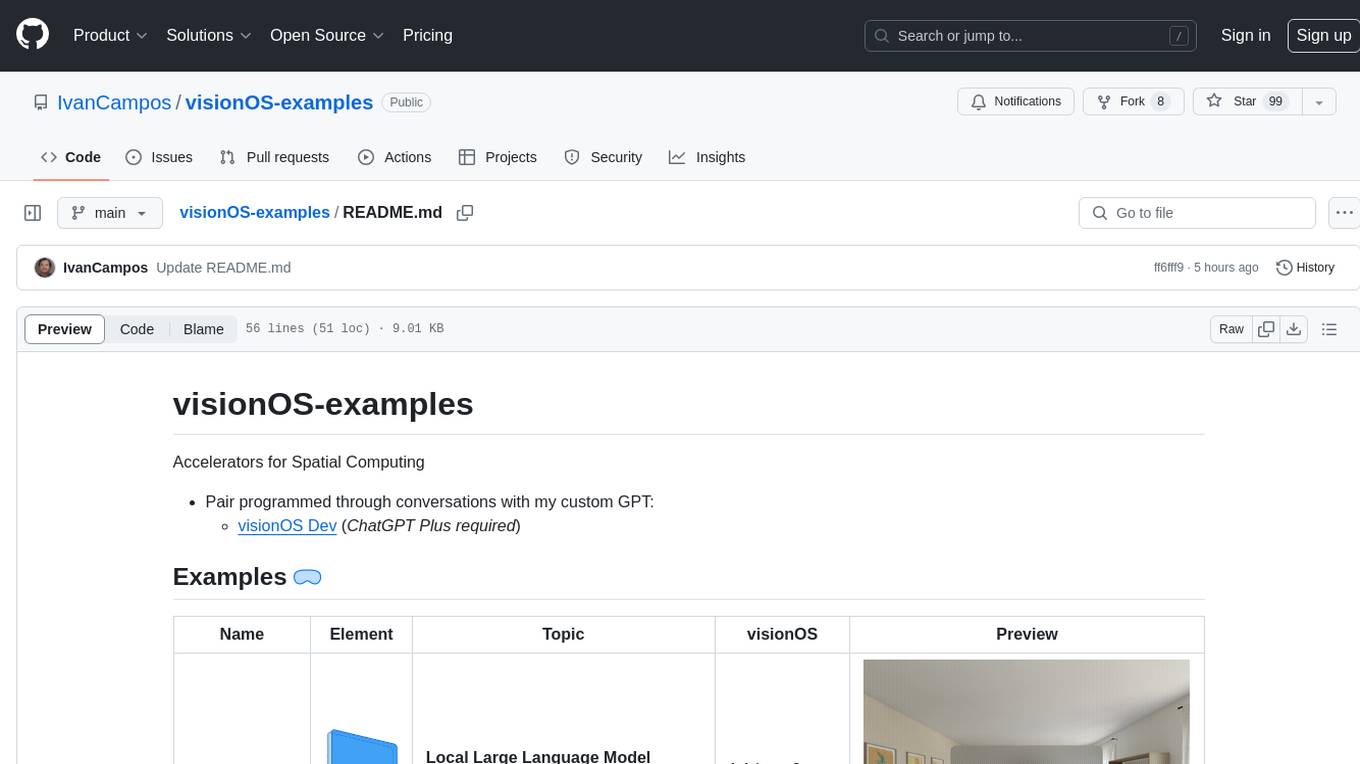
visionOS-examples
visionOS-examples is a repository containing accelerators for Spatial Computing. It includes examples such as Local Large Language Model, Chat Apple Vision Pro, WebSockets, Anchor To Head, Hand Tracking, Battery Life, Countdown, Plane Detection, Timer Vision, and PencilKit for visionOS. The repository showcases various functionalities and features for Apple Vision Pro, offering tools for developers to enhance their visionOS apps with capabilities like hand tracking, plane detection, and real-time cryptocurrency prices.
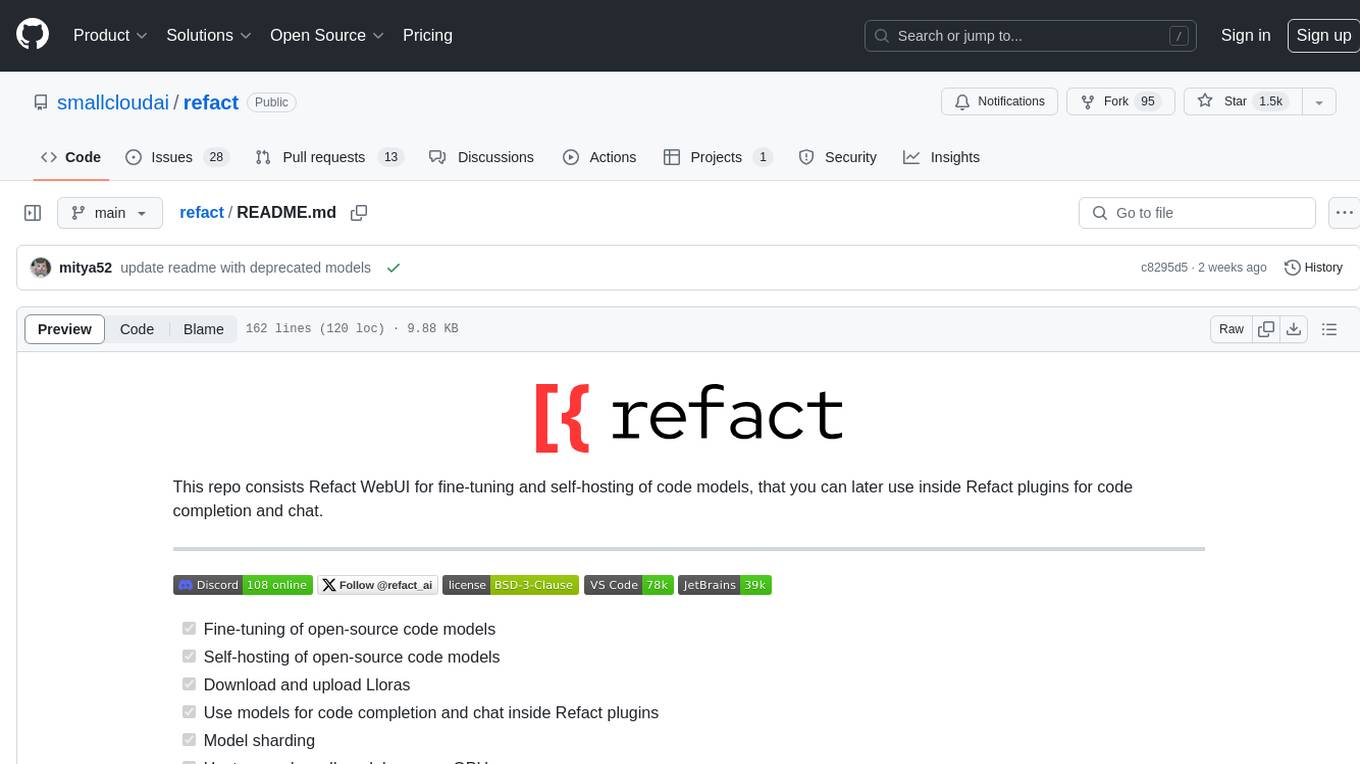
refact
This repository contains Refact WebUI for fine-tuning and self-hosting of code models, which can be used inside Refact plugins for code completion and chat. Users can fine-tune open-source code models, self-host them, download and upload Lloras, use models for code completion and chat inside Refact plugins, shard models, host multiple small models on one GPU, and connect GPT-models for chat using OpenAI and Anthropic keys. The repository provides a Docker container for running the self-hosted server and supports various models for completion, chat, and fine-tuning. Refact is free for individuals and small teams under the BSD-3-Clause license, with custom installation options available for GPU support. The community and support include contributing guidelines, GitHub issues for bugs, a community forum, Discord for chatting, and Twitter for product news and updates.
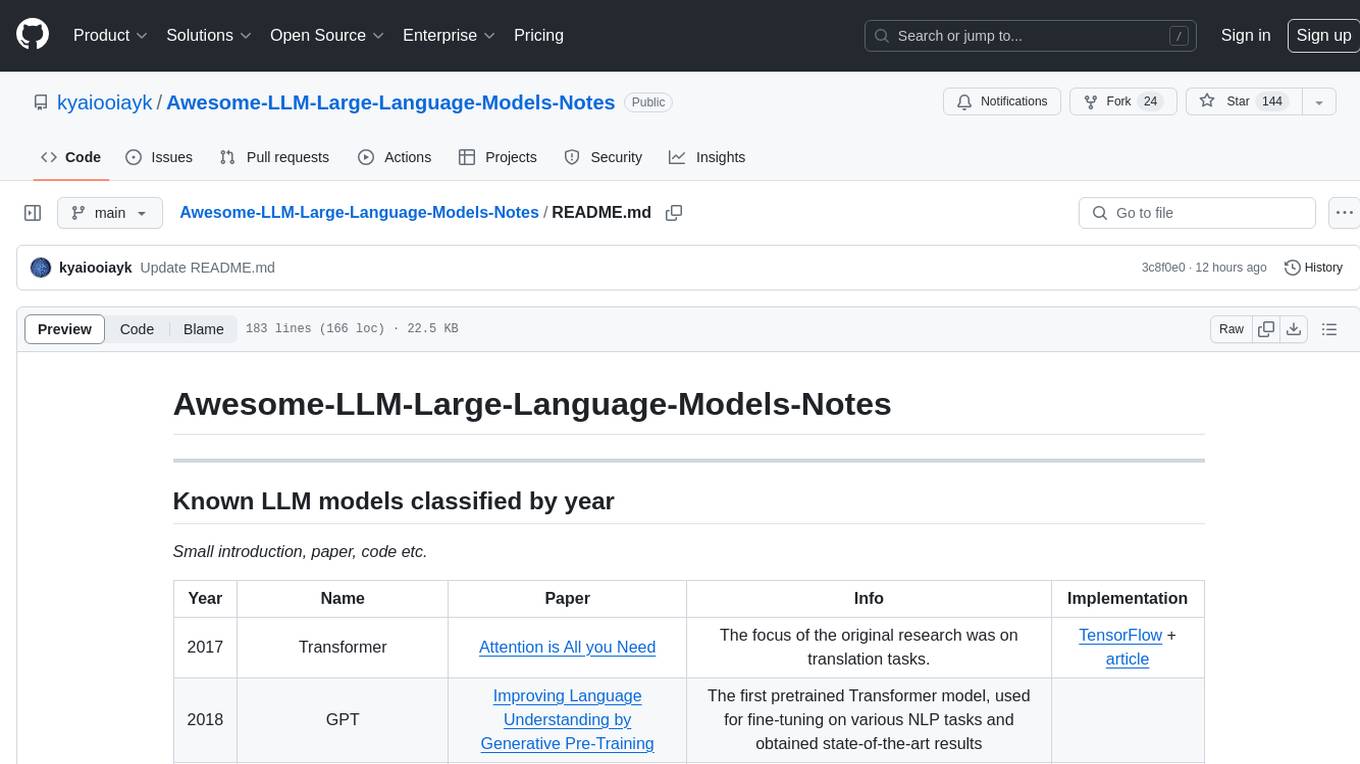
Awesome-LLM-Large-Language-Models-Notes
Awesome-LLM-Large-Language-Models-Notes is a repository that provides a comprehensive collection of information on various Large Language Models (LLMs) classified by year, size, and name. It includes details on known LLM models, their papers, implementations, and specific characteristics. The repository also covers LLM models classified by architecture, must-read papers, blog articles, tutorials, and implementations from scratch. It serves as a valuable resource for individuals interested in understanding and working with LLMs in the field of Natural Language Processing (NLP).
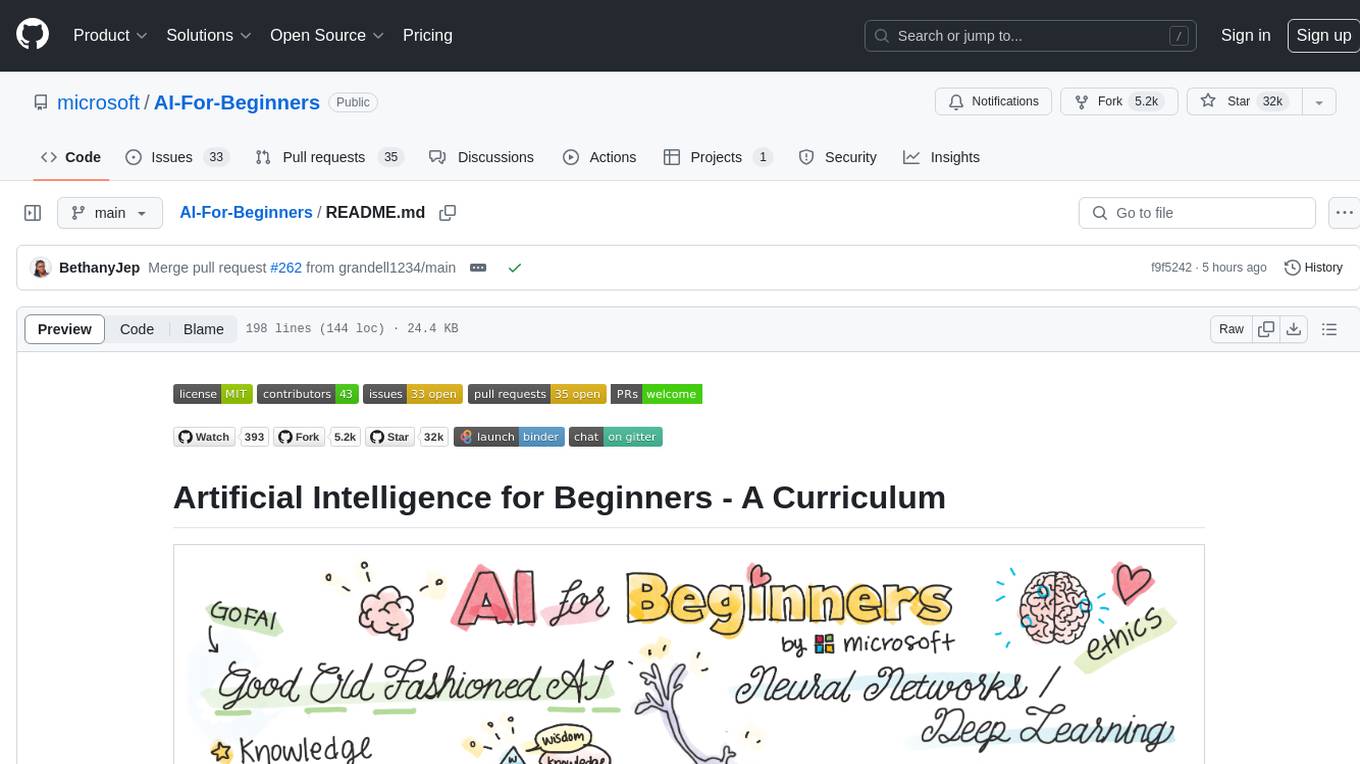
AI-For-Beginners
AI-For-Beginners is a comprehensive 12-week, 24-lesson curriculum designed by experts at Microsoft to introduce beginners to the world of Artificial Intelligence (AI). The curriculum covers various topics such as Symbolic AI, Neural Networks, Computer Vision, Natural Language Processing, Genetic Algorithms, and Multi-Agent Systems. It includes hands-on lessons, quizzes, and labs using popular frameworks like TensorFlow and PyTorch. The focus is on providing a foundational understanding of AI concepts and principles, making it an ideal starting point for individuals interested in AI.
For similar tasks
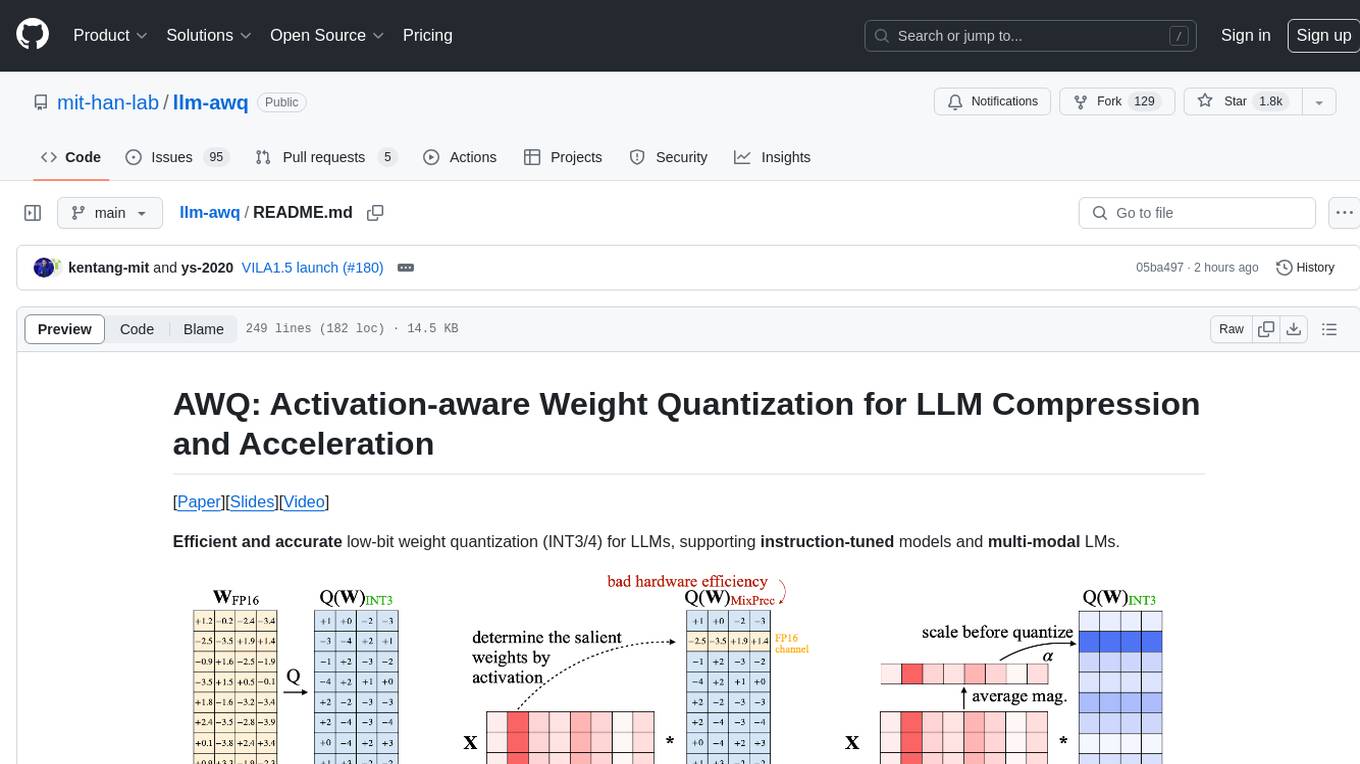
llm-awq
AWQ (Activation-aware Weight Quantization) is a tool designed for efficient and accurate low-bit weight quantization (INT3/4) for Large Language Models (LLMs). It supports instruction-tuned models and multi-modal LMs, providing features such as AWQ search for accurate quantization, pre-computed AWQ model zoo for various LLMs, memory-efficient 4-bit linear in PyTorch, and efficient CUDA kernel implementation for fast inference. The tool enables users to run large models on resource-constrained edge platforms, delivering more efficient responses with LLM/VLM chatbots through 4-bit inference.
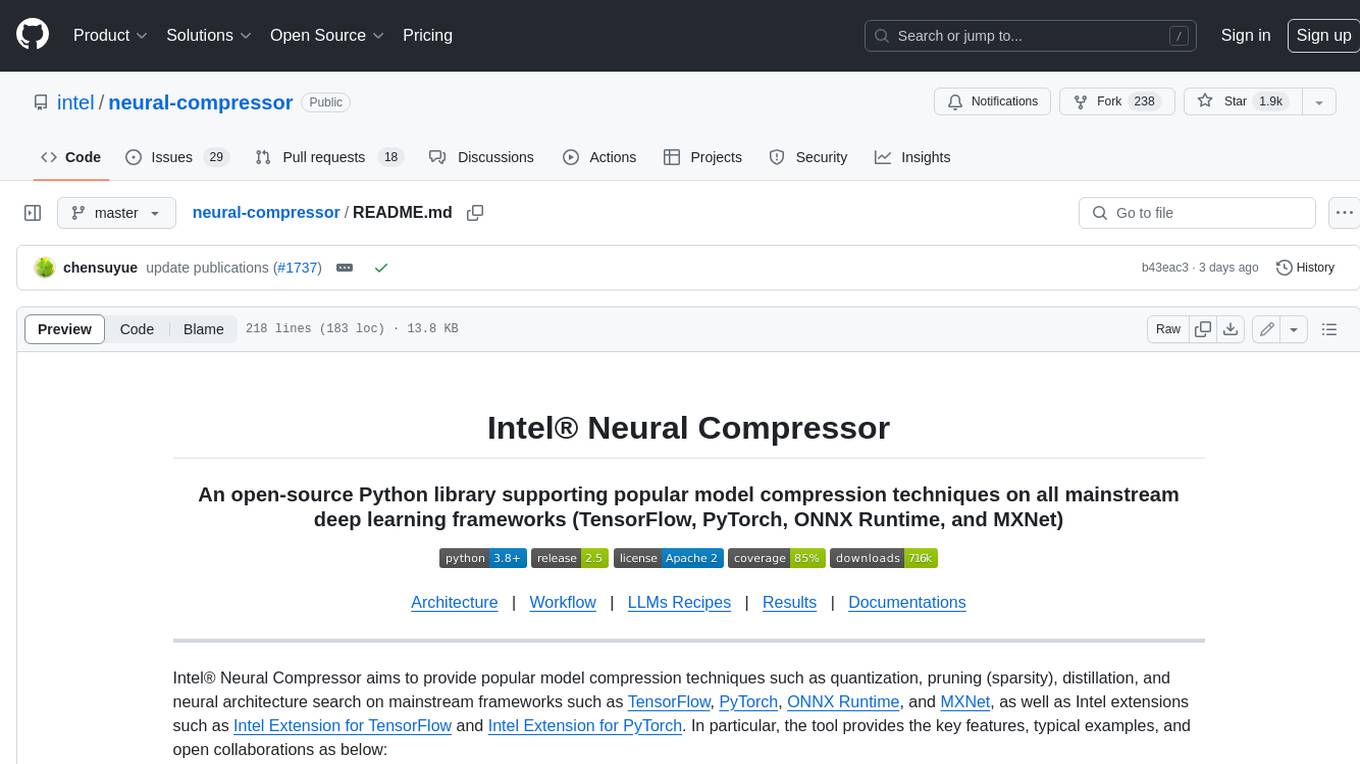
neural-compressor
Intelยฎ Neural Compressor is an open-source Python library that supports popular model compression techniques such as quantization, pruning (sparsity), distillation, and neural architecture search on mainstream frameworks such as TensorFlow, PyTorch, ONNX Runtime, and MXNet. It provides key features, typical examples, and open collaborations, including support for a wide range of Intel hardware, validation of popular LLMs, and collaboration with cloud marketplaces, software platforms, and open AI ecosystems.
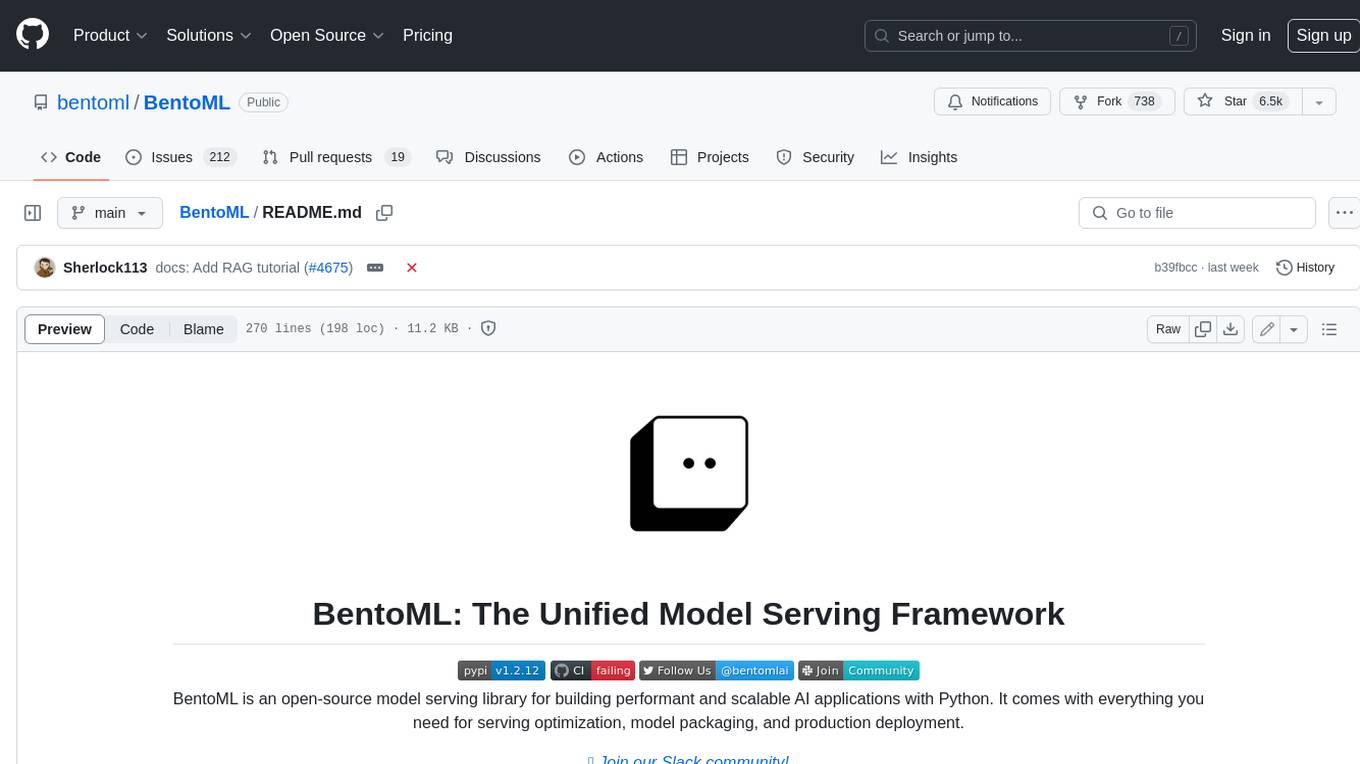
BentoML
BentoML is an open-source model serving library for building performant and scalable AI applications with Python. It comes with everything you need for serving optimization, model packaging, and production deployment.
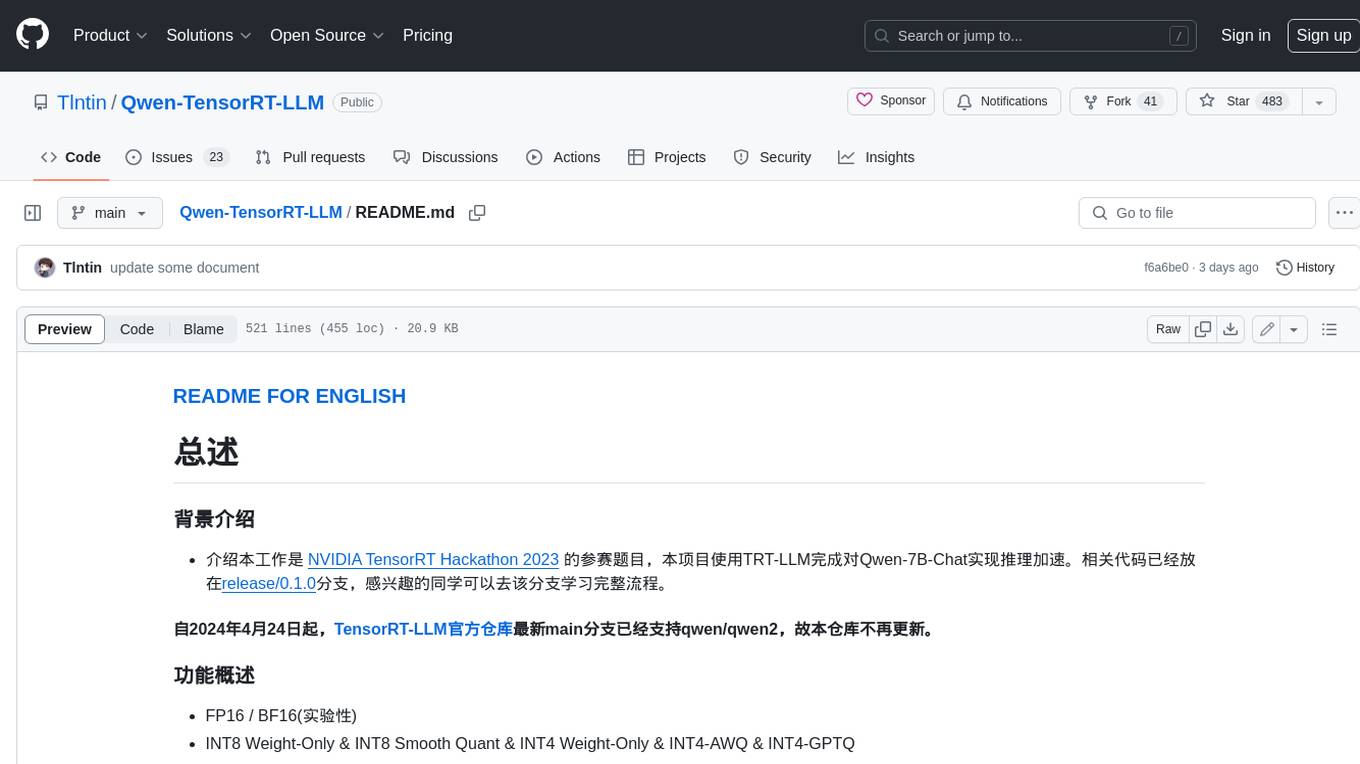
Qwen-TensorRT-LLM
Qwen-TensorRT-LLM is a project developed for the NVIDIA TensorRT Hackathon 2023, focusing on accelerating inference for the Qwen-7B-Chat model using TRT-LLM. The project offers various functionalities such as FP16/BF16 support, INT8 and INT4 quantization options, Tensor Parallel for multi-GPU parallelism, web demo setup with gradio, Triton API deployment for maximum throughput/concurrency, fastapi integration for openai requests, CLI interaction, and langchain support. It supports models like qwen2, qwen, and qwen-vl for both base and chat models. The project also provides tutorials on Bilibili and blogs for adapting Qwen models in NVIDIA TensorRT-LLM, along with hardware requirements and quick start guides for different model types and quantization methods.
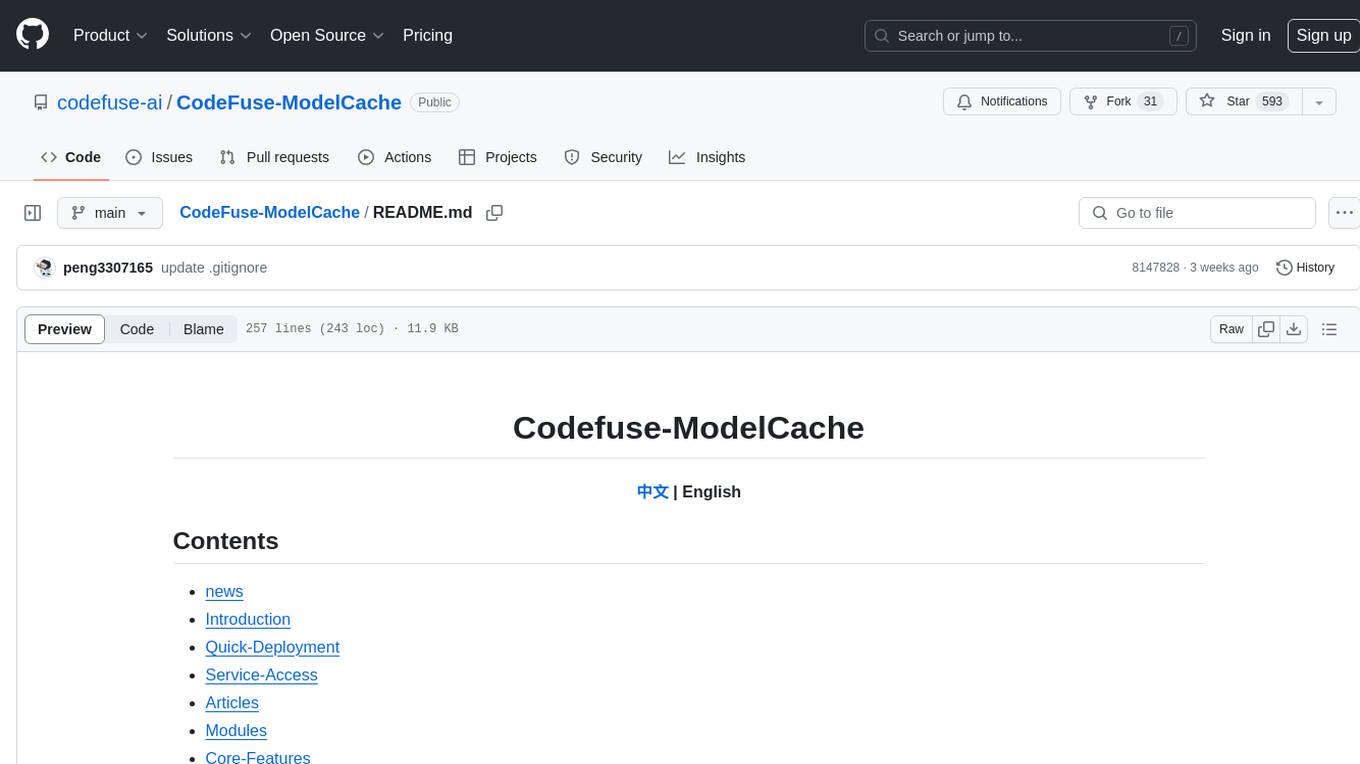
CodeFuse-ModelCache
Codefuse-ModelCache is a semantic cache for large language models (LLMs) that aims to optimize services by introducing a caching mechanism. It helps reduce the cost of inference deployment, improve model performance and efficiency, and provide scalable services for large models. The project caches pre-generated model results to reduce response time for similar requests and enhance user experience. It integrates various embedding frameworks and local storage options, offering functionalities like cache-writing, cache-querying, and cache-clearing through RESTful API. The tool supports multi-tenancy, system commands, and multi-turn dialogue, with features for data isolation, database management, and model loading schemes. Future developments include data isolation based on hyperparameters, enhanced system prompt partitioning storage, and more versatile embedding models and similarity evaluation algorithms.
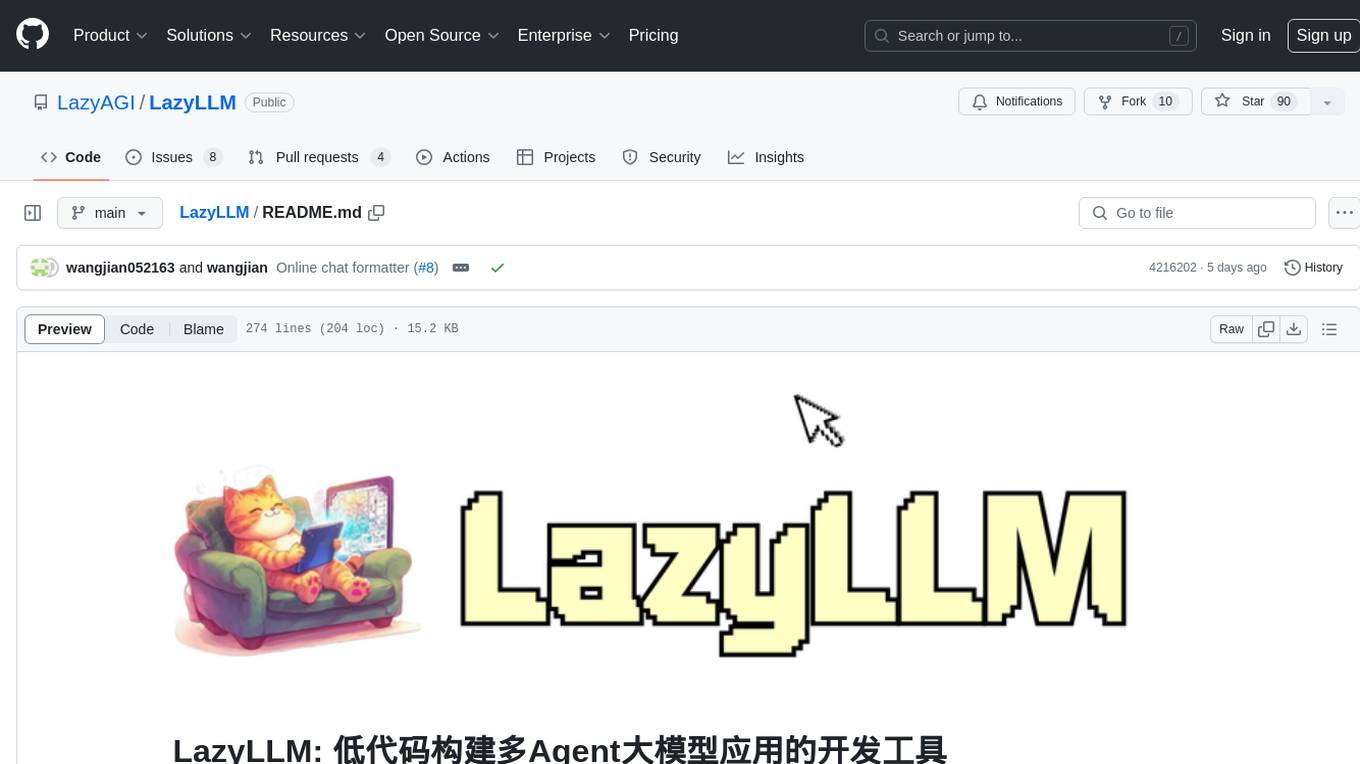
LazyLLM
LazyLLM is a low-code development tool for building complex AI applications with multiple agents. It assists developers in building AI applications at a low cost and continuously optimizing their performance. The tool provides a convenient workflow for application development and offers standard processes and tools for various stages of application development. Users can quickly prototype applications with LazyLLM, analyze bad cases with scenario task data, and iteratively optimize key components to enhance the overall application performance. LazyLLM aims to simplify the AI application development process and provide flexibility for both beginners and experts to create high-quality applications.
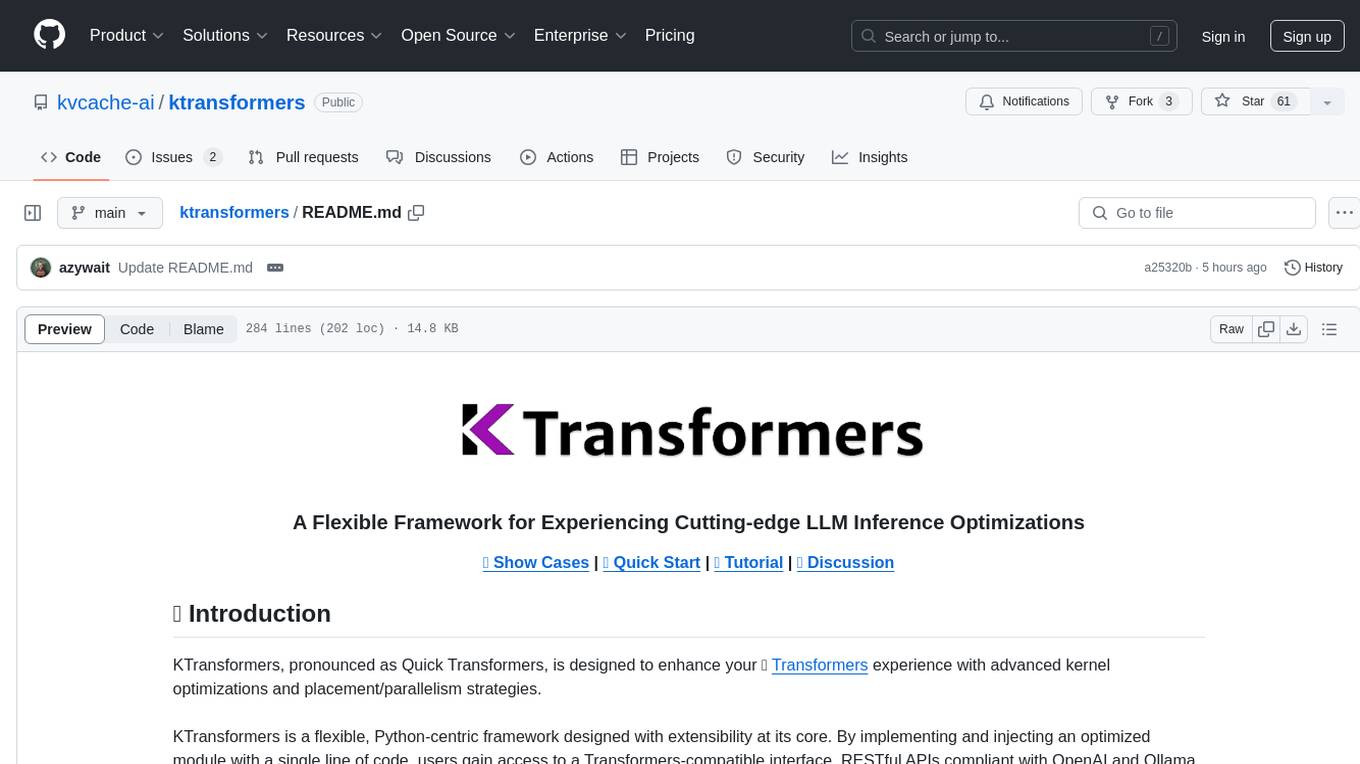
ktransformers
KTransformers is a flexible Python-centric framework designed to enhance the user's experience with advanced kernel optimizations and placement/parallelism strategies for Transformers. It provides a Transformers-compatible interface, RESTful APIs compliant with OpenAI and Ollama, and a simplified ChatGPT-like web UI. The framework aims to serve as a platform for experimenting with innovative LLM inference optimizations, focusing on local deployments constrained by limited resources and supporting heterogeneous computing opportunities like GPU/CPU offloading of quantized models.
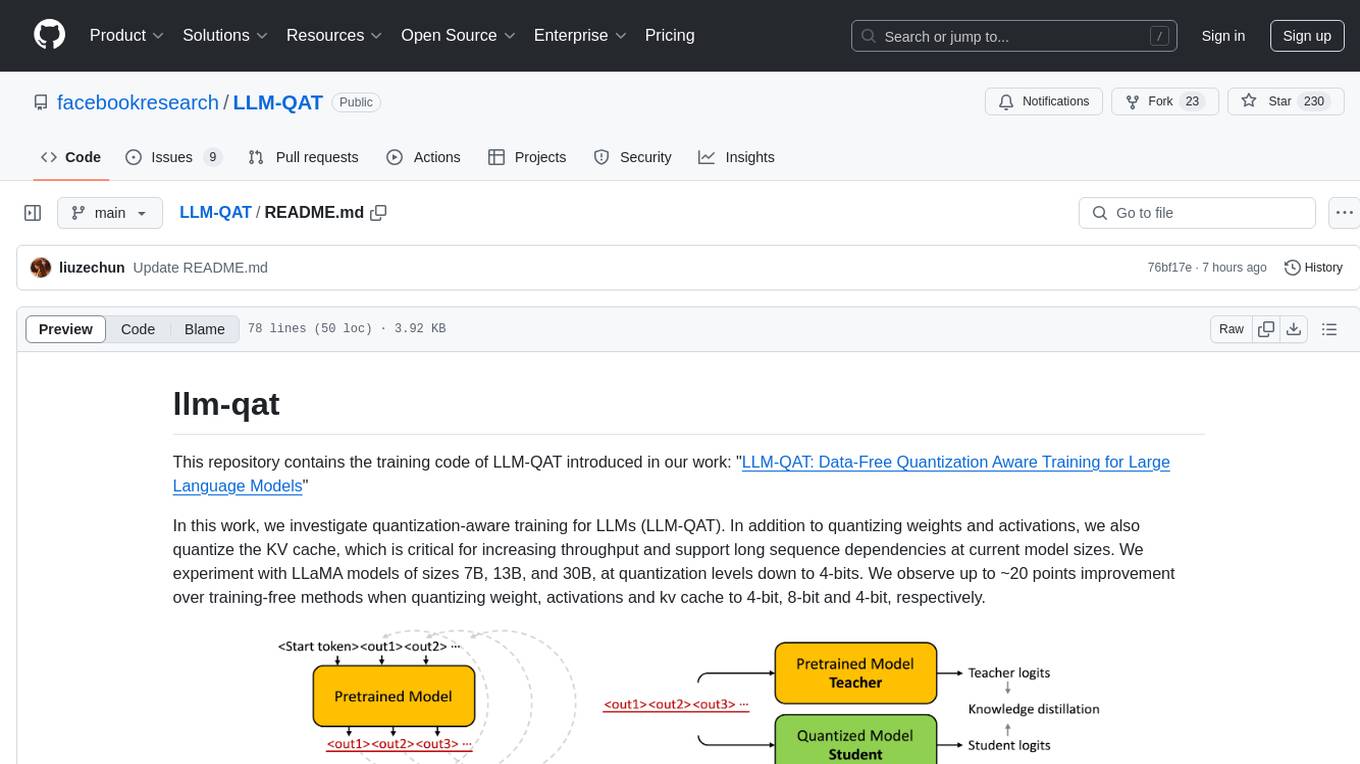
LLM-QAT
This repository contains the training code of LLM-QAT for large language models. The work investigates quantization-aware training for LLMs, including quantizing weights, activations, and the KV cache. Experiments were conducted on LLaMA models of sizes 7B, 13B, and 30B, at quantization levels down to 4-bits. Significant improvements were observed when quantizing weight, activations, and kv cache to 4-bit, 8-bit, and 4-bit, respectively.
For similar jobs
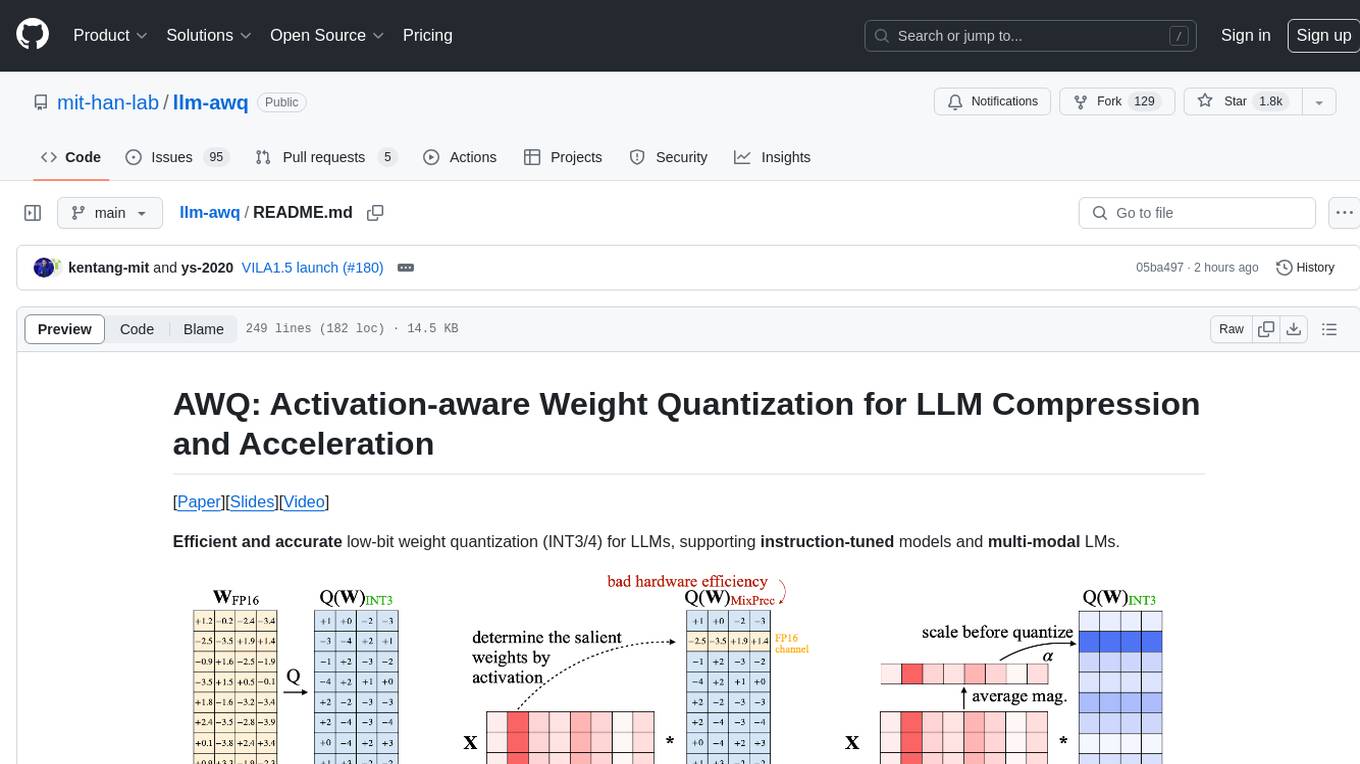
llm-awq
AWQ (Activation-aware Weight Quantization) is a tool designed for efficient and accurate low-bit weight quantization (INT3/4) for Large Language Models (LLMs). It supports instruction-tuned models and multi-modal LMs, providing features such as AWQ search for accurate quantization, pre-computed AWQ model zoo for various LLMs, memory-efficient 4-bit linear in PyTorch, and efficient CUDA kernel implementation for fast inference. The tool enables users to run large models on resource-constrained edge platforms, delivering more efficient responses with LLM/VLM chatbots through 4-bit inference.
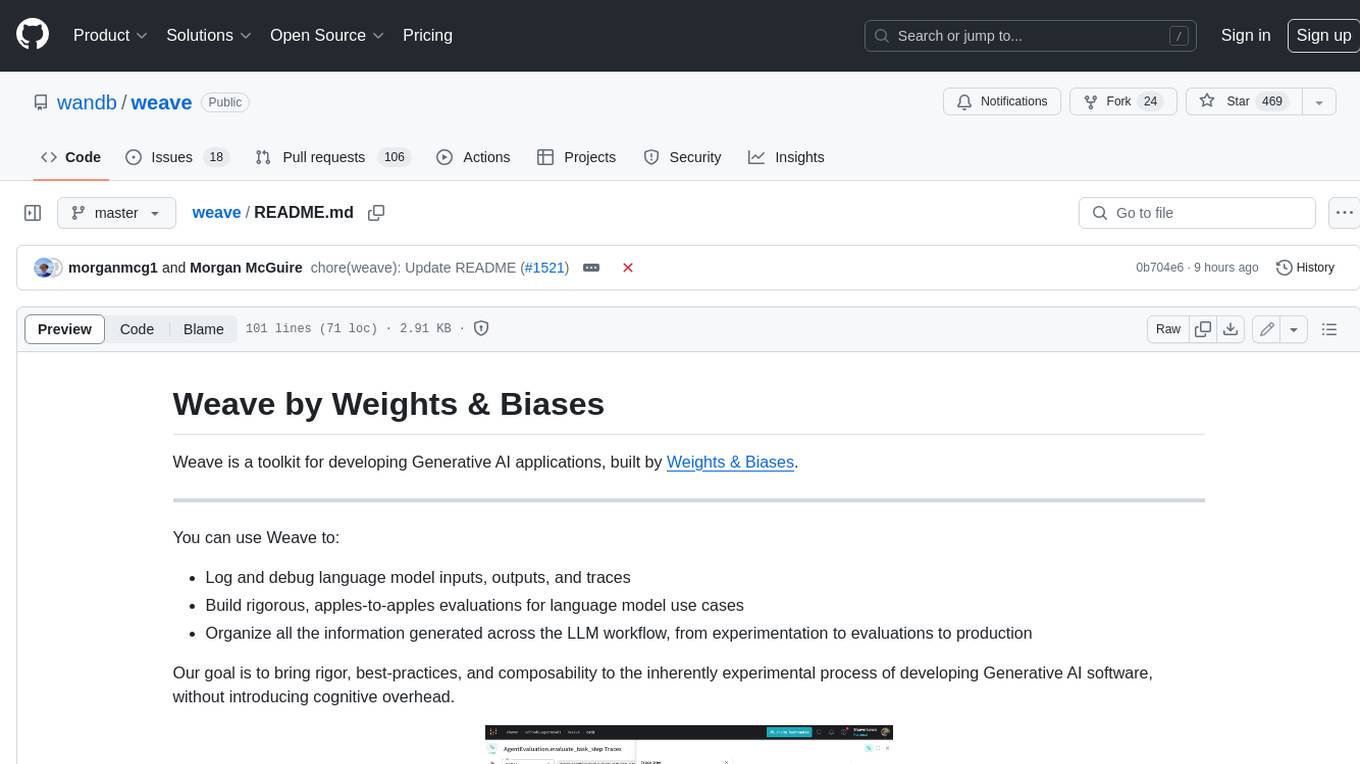
weave
Weave is a toolkit for developing Generative AI applications, built by Weights & Biases. With Weave, you can log and debug language model inputs, outputs, and traces; build rigorous, apples-to-apples evaluations for language model use cases; and organize all the information generated across the LLM workflow, from experimentation to evaluations to production. Weave aims to bring rigor, best-practices, and composability to the inherently experimental process of developing Generative AI software, without introducing cognitive overhead.
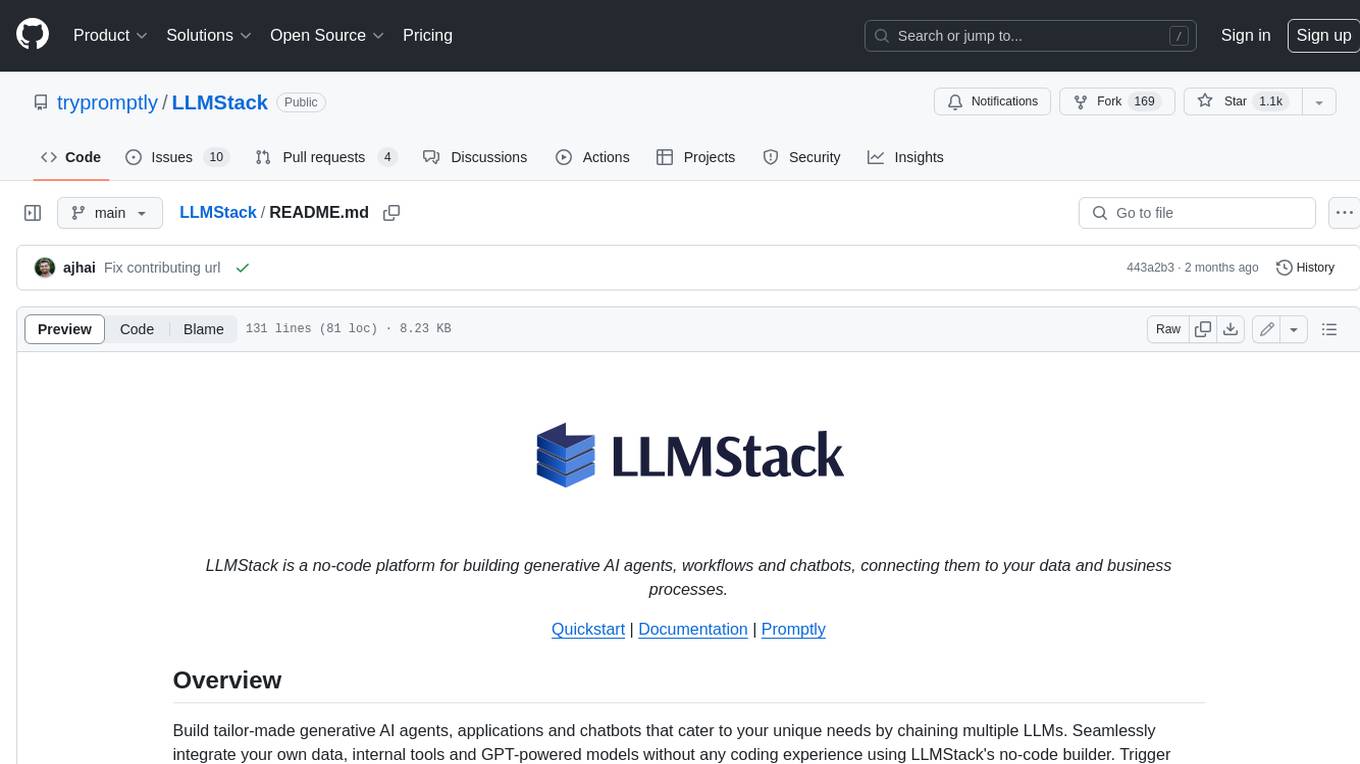
LLMStack
LLMStack is a no-code platform for building generative AI agents, workflows, and chatbots. It allows users to connect their own data, internal tools, and GPT-powered models without any coding experience. LLMStack can be deployed to the cloud or on-premise and can be accessed via HTTP API or triggered from Slack or Discord.
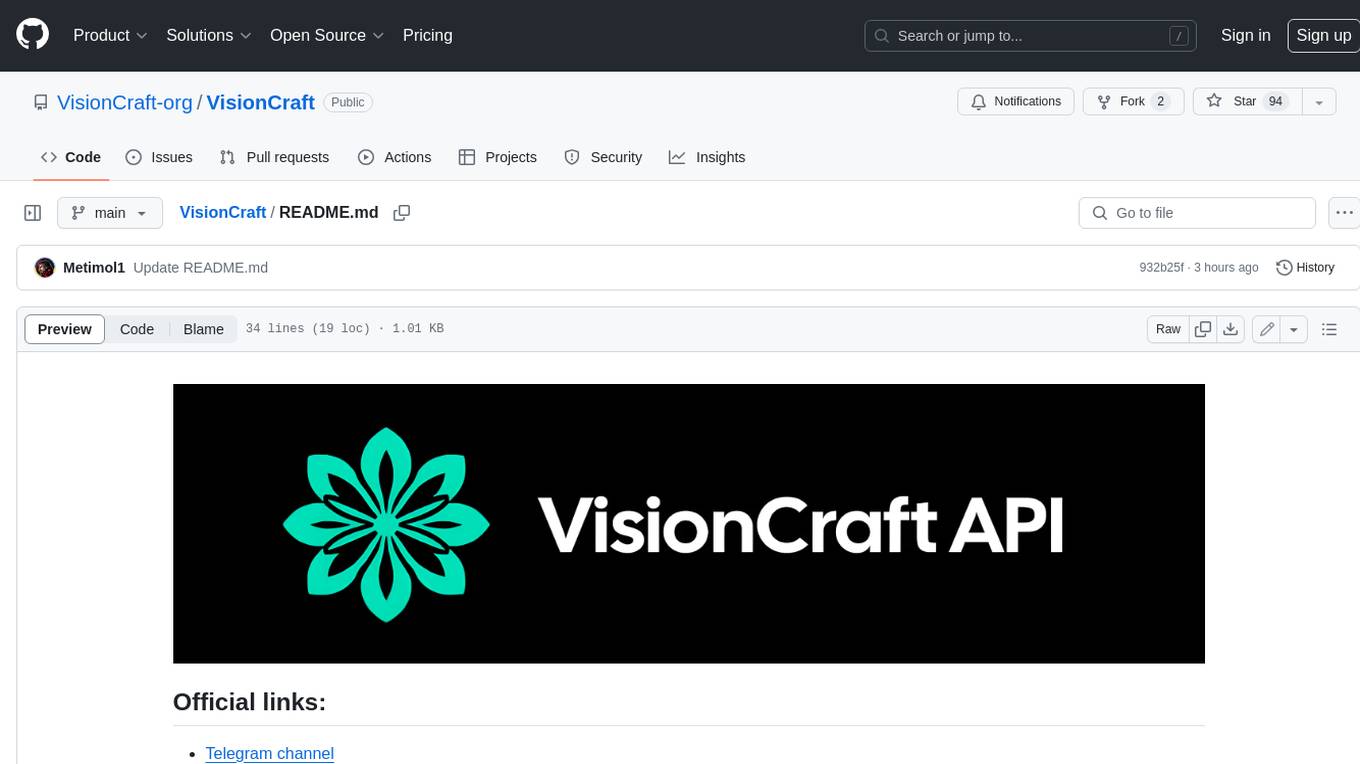
VisionCraft
The VisionCraft API is a free API for using over 100 different AI models. From images to sound.
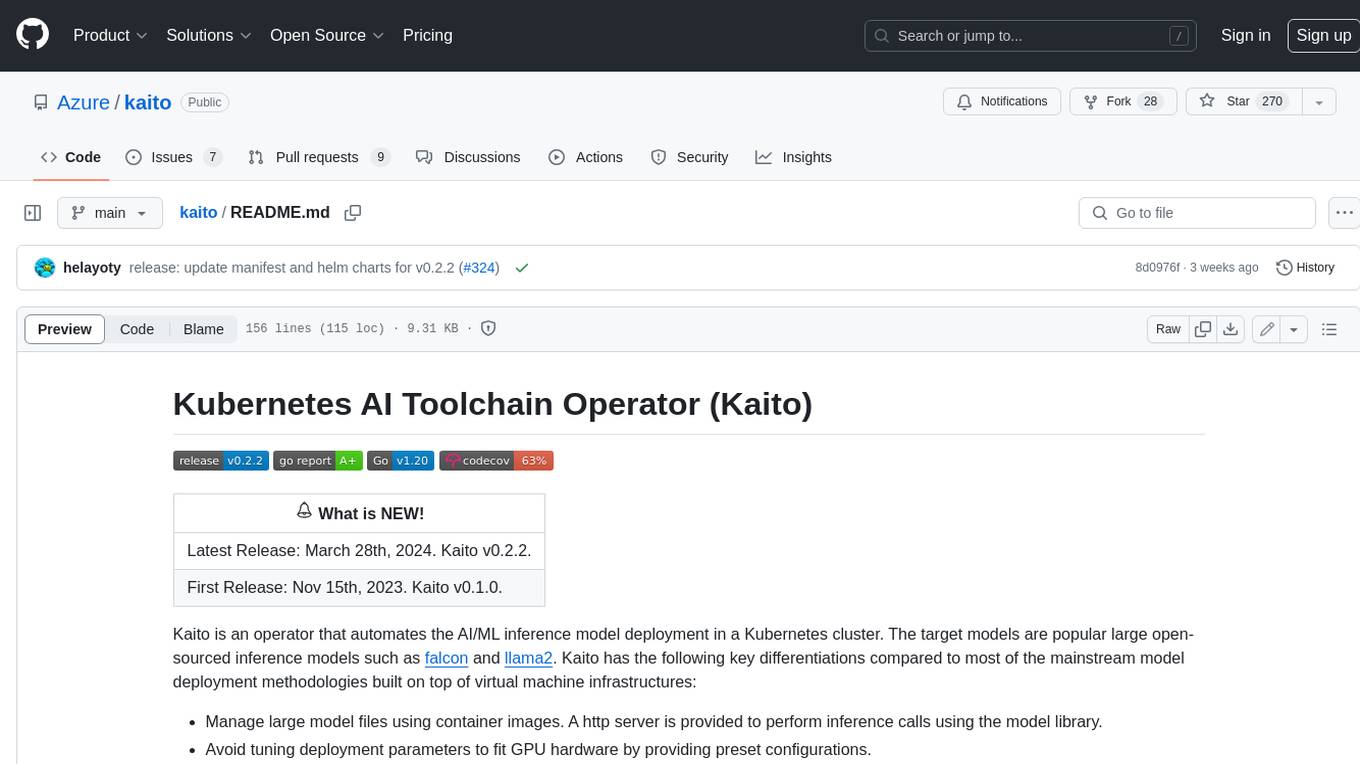
kaito
Kaito is an operator that automates the AI/ML inference model deployment in a Kubernetes cluster. It manages large model files using container images, avoids tuning deployment parameters to fit GPU hardware by providing preset configurations, auto-provisions GPU nodes based on model requirements, and hosts large model images in the public Microsoft Container Registry (MCR) if the license allows. Using Kaito, the workflow of onboarding large AI inference models in Kubernetes is largely simplified.
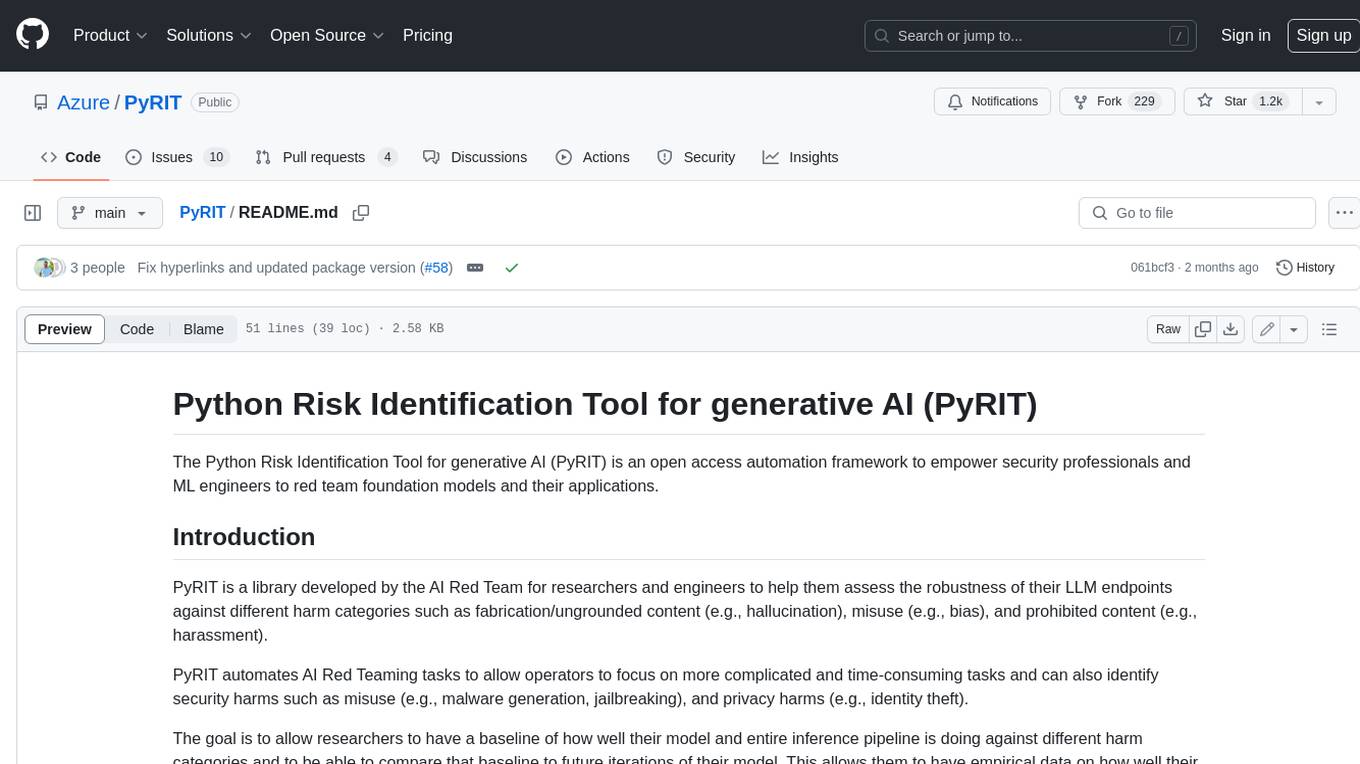
PyRIT
PyRIT is an open access automation framework designed to empower security professionals and ML engineers to red team foundation models and their applications. It automates AI Red Teaming tasks to allow operators to focus on more complicated and time-consuming tasks and can also identify security harms such as misuse (e.g., malware generation, jailbreaking), and privacy harms (e.g., identity theft). The goal is to allow researchers to have a baseline of how well their model and entire inference pipeline is doing against different harm categories and to be able to compare that baseline to future iterations of their model. This allows them to have empirical data on how well their model is doing today, and detect any degradation of performance based on future improvements.
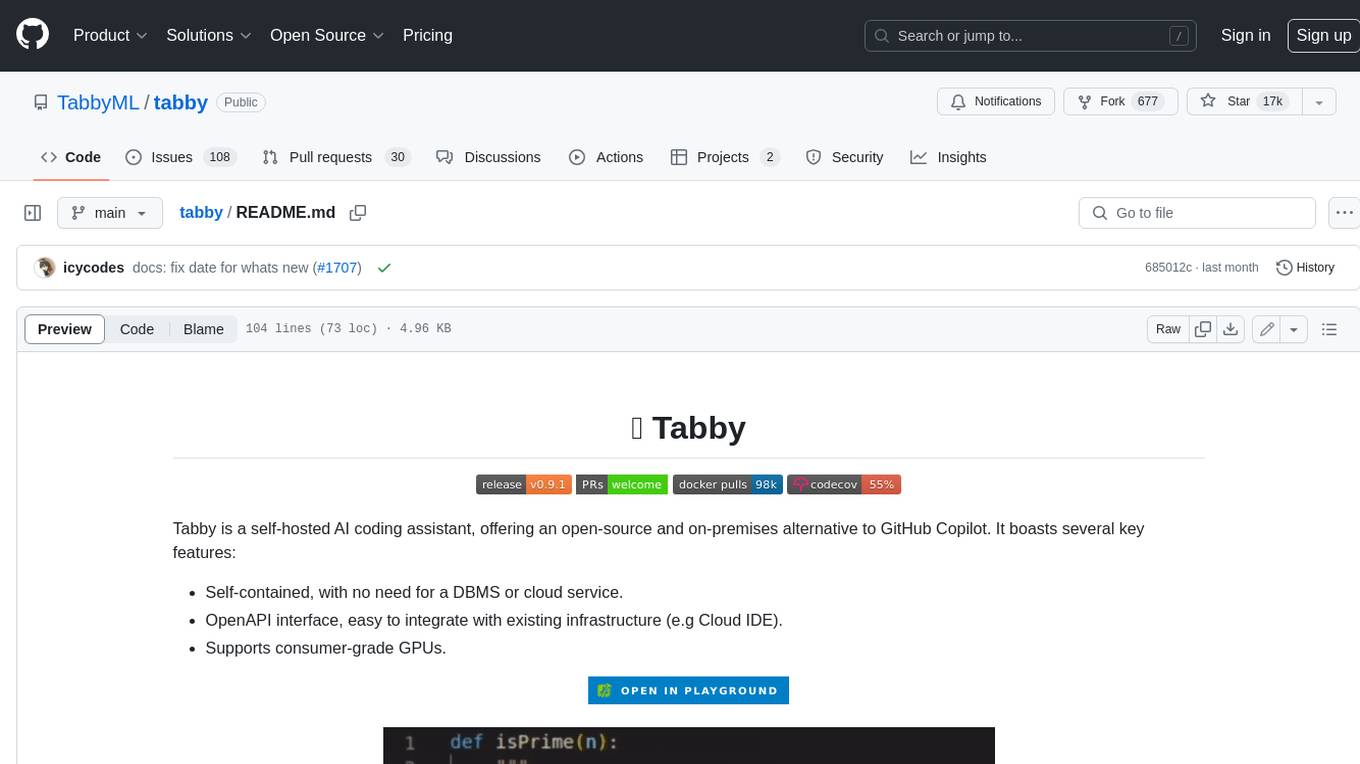
tabby
Tabby is a self-hosted AI coding assistant, offering an open-source and on-premises alternative to GitHub Copilot. It boasts several key features: * Self-contained, with no need for a DBMS or cloud service. * OpenAPI interface, easy to integrate with existing infrastructure (e.g Cloud IDE). * Supports consumer-grade GPUs.
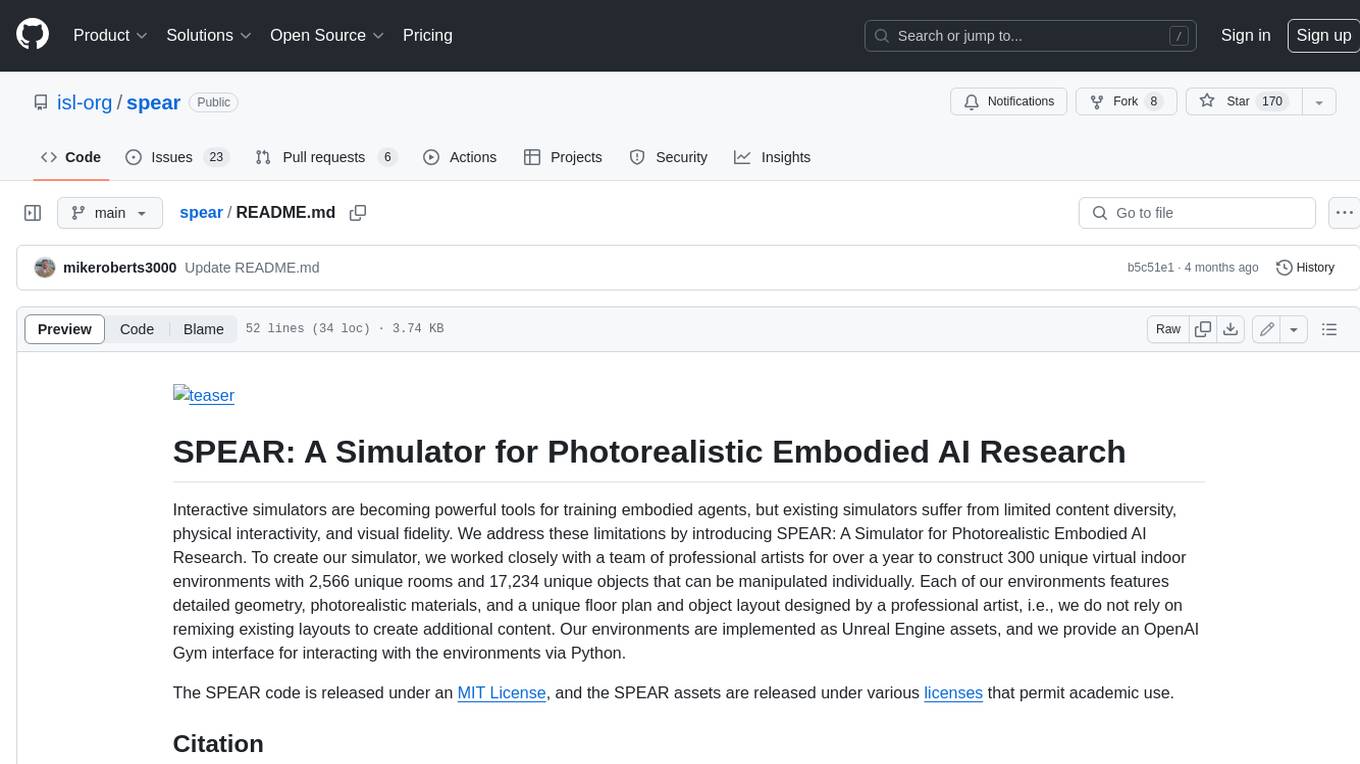
spear
SPEAR (Simulator for Photorealistic Embodied AI Research) is a powerful tool for training embodied agents. It features 300 unique virtual indoor environments with 2,566 unique rooms and 17,234 unique objects that can be manipulated individually. Each environment is designed by a professional artist and features detailed geometry, photorealistic materials, and a unique floor plan and object layout. SPEAR is implemented as Unreal Engine assets and provides an OpenAI Gym interface for interacting with the environments via Python.