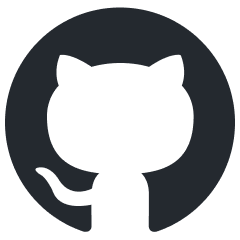
bittensor
Internet-scale Neural Networks
Stars: 886
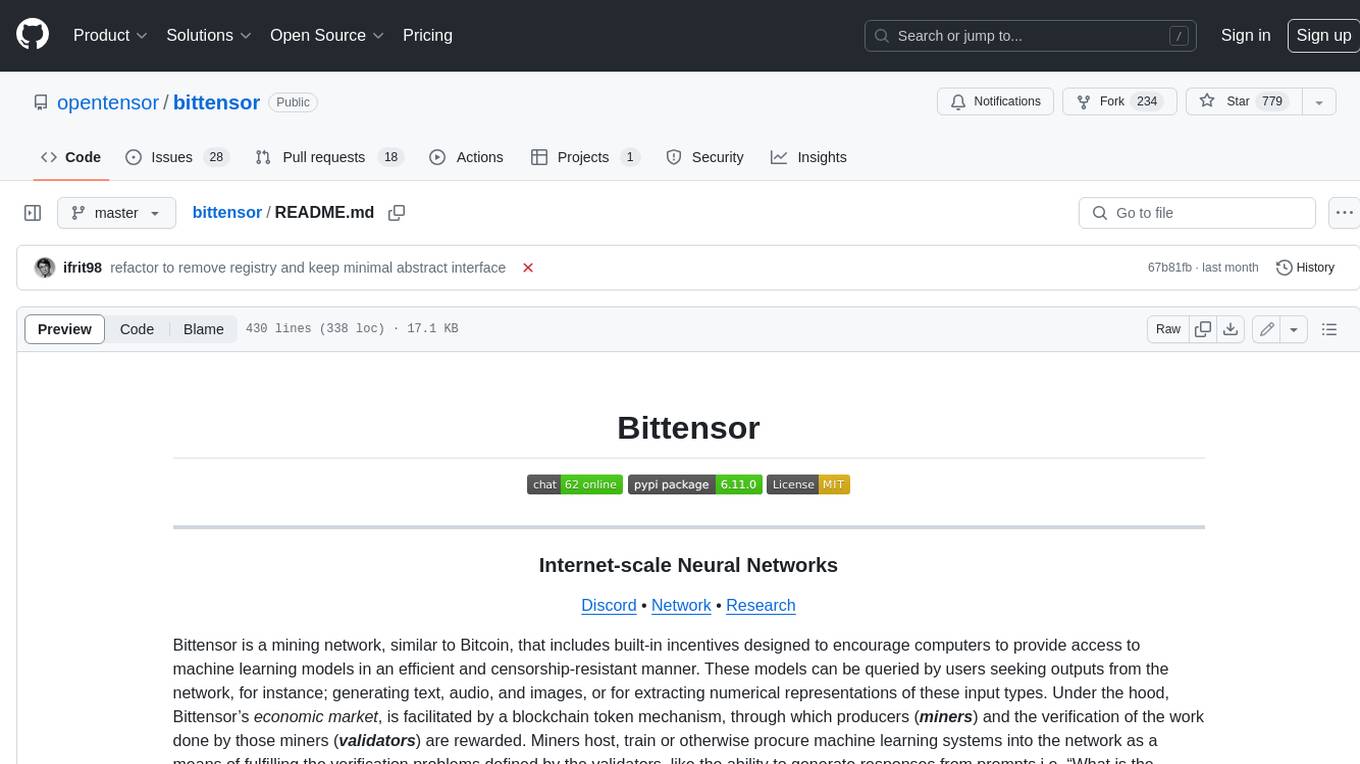
Bittensor is an internet-scale neural network that incentivizes computers to provide access to machine learning models in a decentralized and censorship-resistant manner. It operates through a token-based mechanism where miners host, train, and procure machine learning systems to fulfill verification problems defined by validators. The network rewards miners and validators for their contributions, ensuring continuous improvement in knowledge output. Bittensor allows anyone to participate, extract value, and govern the network without centralized control. It supports tasks such as generating text, audio, images, and extracting numerical representations.
README:
- Overview of Bittensor
- The Bittensor SDK
- Is Bittensor a blockchain or an AI platform?
- Subnets
- Subnet validators and subnet miners
- Yuma Consensus
- Release Notes
- Install Bittensor SDK
- Upgrade
- Install on macOS and Linux
- Release Guidelines
- Contributions
- License
- Acknowledgments
Welcome! Bittensor is an open source platform on which you can produce competitive digital commodities. These digital commodities can be machine intelligence, storage space, compute power, protein folding, financial markets prediction, and many more. You are rewarded in TAO when you produce best digital commodities.
The Opentensor Foundation (OTF) provides all the open source tools, including this Bittensor SDK, the codebase and the documentation, with step-by-step tutorials and guides, to enable you to participate in the Bittensor ecosystem.
- Developer documentation: https://docs.bittensor.com.
- A Beginner's Q and A on Bittensor: https://docs.bittensor.com/questions-and-answers.
- Bittensor whitepaper: https://bittensor.com/whitepaper.
This Bittensor SDK contains ready-to-use Python packages for interacting with the Bittensor ecosystem, writing subnet incentive mechanisms, subnet miners, subnet validators and querying the subtensor (the blockchain part of the Bittensor network).
In Bittensor there is one blockchain, and many platforms that are connected to this one blockchain. We call these platforms as subnets, and this one blockchain subtensor. So, a subnet can be AI-related or it can be something else. The Bittensor network has a number of distinct subnets. All these subnets interact with subtensor blockchain. If you are thinking, "So, subnets are not part of the blockchain but only interact with it?" then the answer is "yes, exactly."
Each category of the digital commodity is produced in a distinct subnet. Applications are built on these specific subnets. End-users of these applications would be served by these applications.
Subnets, which exist outside the blockchain and are connected to it, are off-chain competitions where only the best producers are rewarded. A subnet consists of off-chain subnet validators who initiate the competition for a specific digital commodity, and off-chain subnet miners who compete and respond by producing the best quality digital commodity.
Scores are assigned to the top-performing subnet miners and subnet validators. The on-chain Yuma Consensus determines the TAO rewards for these top performers. The Bittensor blockchain, the subtensor, runs on decentralized validation nodes, just like any blockchain.
This SDK repo is for Bittensor platform only This Bittensor SDK codebase is for the Bittensor platform only, designed to help developers create subnets and build tools on Bittensor. For subnets and applications, refer to subnet-specific websites, which are maintained by subnet owners.
See Bittensor SDK Release Notes.
Before you can start developing, you must install Bittensor SDK and then create Bittensor wallet.
If you already installed Bittensor SDK, make sure you upgrade to the latest version. Run the below command:
python3 -m pip install --upgrade bittensor
You can install Bittensor SDK on your local machine in either of the following ways. Make sure you verify your installation after you install:
This is the most straightforward method. It is recommended for a beginner as it will pre-install requirements like Python, if they are not already present on your machine. Copy and paste the following bash
command into your terminal:
/bin/bash -c "$(curl -fsSL https://raw.githubusercontent.com/opentensor/bittensor/master/scripts/install.sh)"
For Ubuntu-Linux users
If you are using Ubuntu-Linux, the script will prompt for sudo
access to install all required apt-get packages.
python3 -m venv bt_venv
source bt_venv/bin/activate
pip install bittensor
-
Create and activate a virtual environment
-
Create Python virtual environment. Follow this guide on python.org.
-
Activate the new environment. Follow this guide on python.org
-
-
Clone the Bittensor SDK repo
git clone https://github.com/opentensor/bittensor.git
- Install
You can install using any of the below options:
-
Install only SDK: Run the below command to install Bittensor SDK in the above virtual environment.
pip install bittensor
-
Install SDK with
btcli
: Install Bittensor SDK withbtcli
. Thebtcli
will be installed as an independent tool and its Python package isbittensor-cli
.pip install bittensor[btcli]
-
Install SDK with
torch
: Install Bittensor SDK withtorch
.pip install bittensor[torch]
-
Install SDK with
cubit
: Install Bittensor SDK withcubit
.- Install
cubit
first. See the Install section. Only Python 3.9 and 3.10 versions are supported. - Then install SDK with
pip install bittensor
.
- Install
To install and run Bittensor SDK on Windows you must install WSL 2 (Windows Subsystem for Linux) on Windows and select Ubuntu Linux distribution.
After you installed the above, follow the same installation steps described above in Install on macOS and Linux.
ALERT: Limited support on Windows While wallet transactions like delegating, transfer, registering, staking can be performed on a Windows machine using WSL 2, the mining and validating operations are not recommended and are not supported on Windows machines.
You can verify your installation in either of the below ways:
python3 -m bittensor
The above command will show you the version of the btsdk
you just installed.
-
Launch the Python interpreter on your terminal.
python3
-
Enter the following two lines in the Python interpreter.
import bittensor as bt print( bt.__version__ )
The Python interpreter output will look like below:
Python 3.11.6 (main, Oct 2 2023, 13:45:54) [Clang 15.0.0 (clang-1500.0.40.1)] on darwin Type "help", "copyright", "credits" or "license" for more information. >>> import bittensor as bt >>> print( bt.__version__ ) <version number>
You will see the version number you installed in place of <version number>
.
You can also verify the Bittensor SDK installation by listing the axon information for the neurons. Enter the following lines in the Python interpreter.
import bittensor
metagraph = bittensor.Metagraph(1)
metagraph.axons[:10]
The Python interpreter output will look like below.
[AxonInfo( /ipv4/3.139.80.241:11055, 5GqDsK6SAPyQtG243hbaKTsoeumjQQLhUu8GyrXikPTmxjn7, 5D7u5BTqF3j1XHnizp9oR67GFRr8fBEFhbdnuVQEx91vpfB5, 600 ), AxonInfo( /ipv4/8.222.132.190:5108, 5CwqDkDt1uk2Bngvf8avrapUshGmiUvYZjYa7bfA9Gv9kn1i, 5HQ9eTDorvovKTxBc9RUD22FZHZzpy1KRfaxCnRsT9QhuvR6, 600 ), AxonInfo( /ipv4/34.90.71.181:8091, 5HEo565WAy4Dbq3Sv271SAi7syBSofyfhhwRNjFNSM2gP9M2, 5ChuGqW2cxc5AZJ29z6vyTkTncg75L9ovfp8QN8eB8niSD75, 601 ), AxonInfo( /ipv4/64.247.206.79:8091, 5HK5tp6t2S59DywmHRWPBVJeJ86T61KjurYqeooqj8sREpeN, 5E7W9QXNoW7se7B11vWRMKRCSWkkAu9EYotG5Ci2f9cqV8jn, 601 ), AxonInfo( /ipv4/51.91.30.166:40203, 5EXYcaCdnvnMZbozeknFWbj6aKXojfBi9jUpJYHea68j4q1a, 5CsxoeDvWsQFZJnDCyzxaNKgA8pBJGUJyE1DThH8xU25qUMg, 601 ), AxonInfo( /ipv4/149.137.225.62:8091, 5F4tQyWrhfGVcNhoqeiNsR6KjD4wMZ2kfhLj4oHYuyHbZAc3, 5Ccmf1dJKzGtXX7h17eN72MVMRsFwvYjPVmkXPUaapczECf6, 600 ), AxonInfo( /ipv4/38.147.83.11:8091, 5Hddm3iBFD2GLT5ik7LZnT3XJUnRnN8PoeCFgGQgawUVKNm8, 5DCQw11aUW7bozAKkB8tB5bHqAjiu4F6mVLZBdgJnk8dzUoV, 610 ), AxonInfo( /ipv4/38.147.83.30:41422, 5HNQURvmjjYhTSksi8Wfsw676b4owGwfLR2BFAQzG7H3HhYf, 5EZUTdAbXyLmrs3oiPvfCM19nG6oRs4X7zpgxG5oL1iK4MAh, 610 ), AxonInfo( /ipv4/54.227.25.215:10022, 5DxrZuW8kmkZPKGKp1RBVovaP5zHtPLDHYc5Yu82Z1fWqK5u, 5FhXUSmSZ2ec7ozRSA8Bg3ywmGwrjoLLzsXjNcwmZme2GcSC, 601 ), AxonInfo( /ipv4/52.8.243.76:40033, 5EnZN591jjsKKbt3yBtfGKWHxhxRH9cJonqTKRT5yTRUyNon, 5ChzhHyGmWwEdHjuvAxoUifHEZ6xpUjR67fDd4a42UrPysyB, 601 )]
>>>
Instructions for the release manager: RELEASE_GUIDELINES.md document.
Ready to contribute? Read the contributing guide before making a pull request.
The MIT License (MIT) Copyright © 2024 The Opentensor Foundation
Permission is hereby granted, free of charge, to any person obtaining a copy of this software and associated documentation files (the “Software”), to deal in the Software without restriction, including without limitation the rights to use, copy, modify, merge, publish, distribute, sublicense, and/or sell copies of the Software, and to permit persons to whom the Software is furnished to do so, subject to the following conditions:
The above copyright notice and this permission notice shall be included in all copies or substantial portions of the Software.
THE SOFTWARE IS PROVIDED “AS IS”, WITHOUT WARRANTY OF ANY KIND, EXPRESS OR IMPLIED, INCLUDING BUT NOT LIMITED TO THE WARRANTIES OF MERCHANTABILITY, FITNESS FOR A PARTICULAR PURPOSE AND NONINFRINGEMENT. IN NO EVENT SHALL THE AUTHORS OR COPYRIGHT HOLDERS BE LIABLE FOR ANY CLAIM, DAMAGES OR OTHER LIABILITY, WHETHER IN AN ACTION OF CONTRACT, TORT OR OTHERWISE, ARISING FROM, OUT OF OR IN CONNECTION WITH THE SOFTWARE OR THE USE OR OTHER DEALINGS IN THE SOFTWARE.
learning-at-home/hivemind
For Tasks:
Click tags to check more tools for each tasksFor Jobs:
Alternative AI tools for bittensor
Similar Open Source Tools
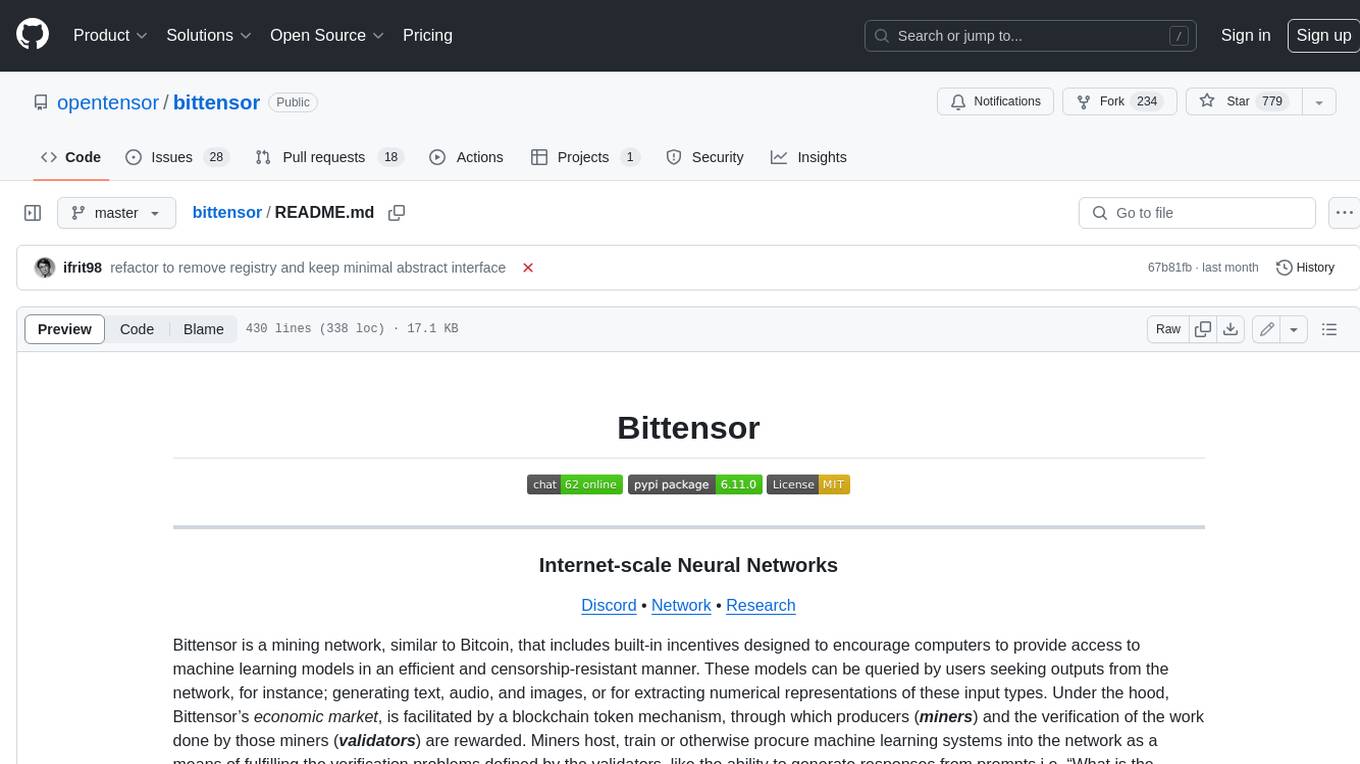
bittensor
Bittensor is an internet-scale neural network that incentivizes computers to provide access to machine learning models in a decentralized and censorship-resistant manner. It operates through a token-based mechanism where miners host, train, and procure machine learning systems to fulfill verification problems defined by validators. The network rewards miners and validators for their contributions, ensuring continuous improvement in knowledge output. Bittensor allows anyone to participate, extract value, and govern the network without centralized control. It supports tasks such as generating text, audio, images, and extracting numerical representations.
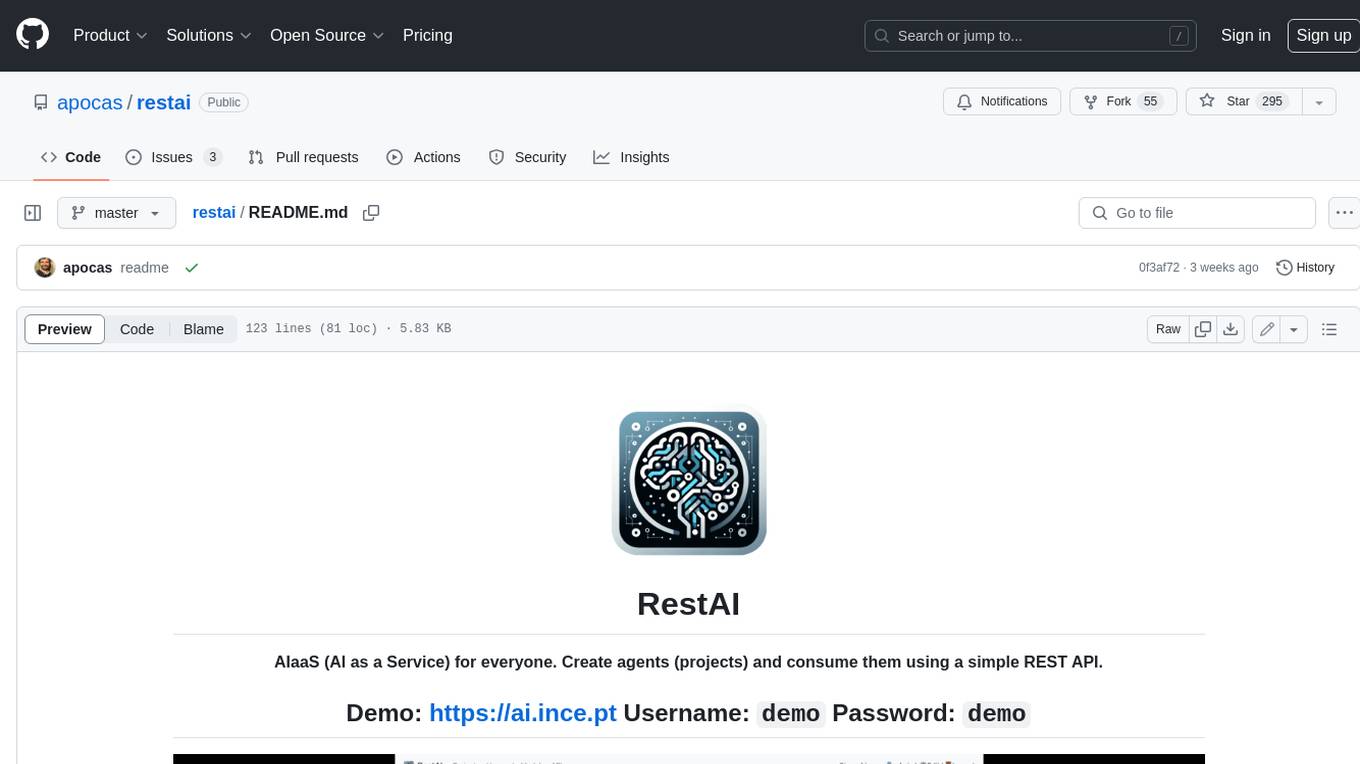
restai
RestAI is an AIaaS (AI as a Service) platform that allows users to create and consume AI agents (projects) using a simple REST API. It supports various types of agents, including RAG (Retrieval-Augmented Generation), RAGSQL (RAG for SQL), inference, vision, and router. RestAI features automatic VRAM management, support for any public LLM supported by LlamaIndex or any local LLM supported by Ollama, a user-friendly API with Swagger documentation, and a frontend for easy access. It also provides evaluation capabilities for RAG agents using deepeval.
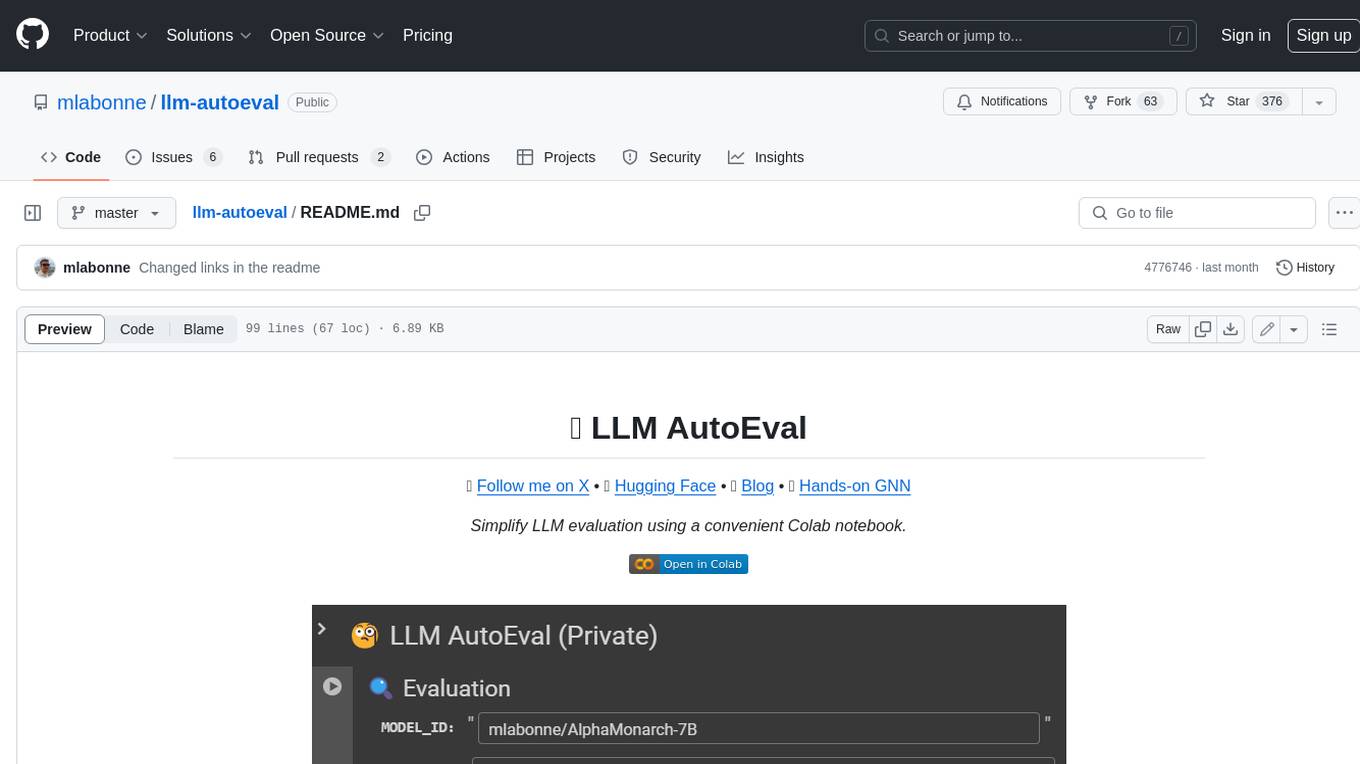
llm-autoeval
LLM AutoEval is a tool that simplifies the process of evaluating Large Language Models (LLMs) using a convenient Colab notebook. It automates the setup and execution of evaluations using RunPod, allowing users to customize evaluation parameters and generate summaries that can be uploaded to GitHub Gist for easy sharing and reference. LLM AutoEval supports various benchmark suites, including Nous, Lighteval, and Open LLM, enabling users to compare their results with existing models and leaderboards.
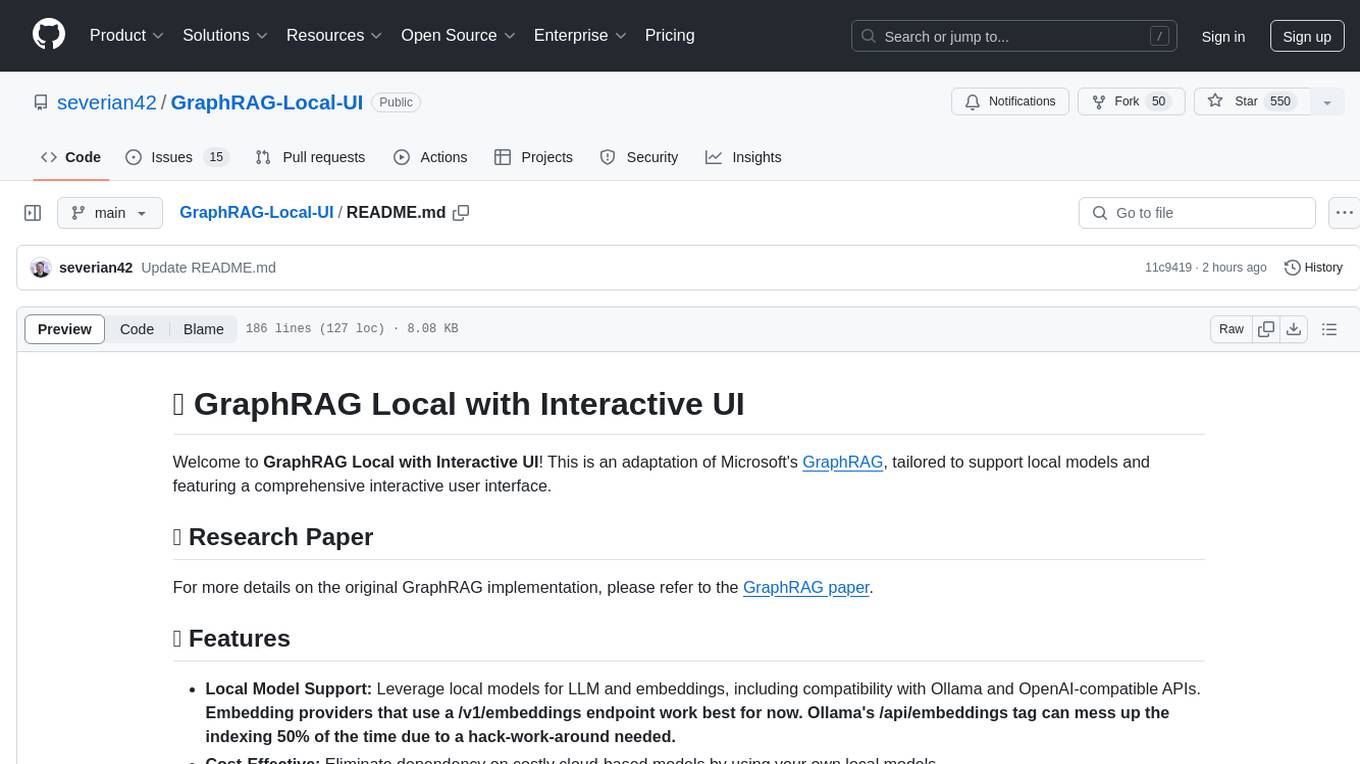
GraphRAG-Local-UI
GraphRAG Local with Interactive UI is an adaptation of Microsoft's GraphRAG, tailored to support local models and featuring a comprehensive interactive user interface. It allows users to leverage local models for LLM and embeddings, visualize knowledge graphs in 2D or 3D, manage files, settings, and queries, and explore indexing outputs. The tool aims to be cost-effective by eliminating dependency on costly cloud-based models and offers flexible querying options for global, local, and direct chat queries.
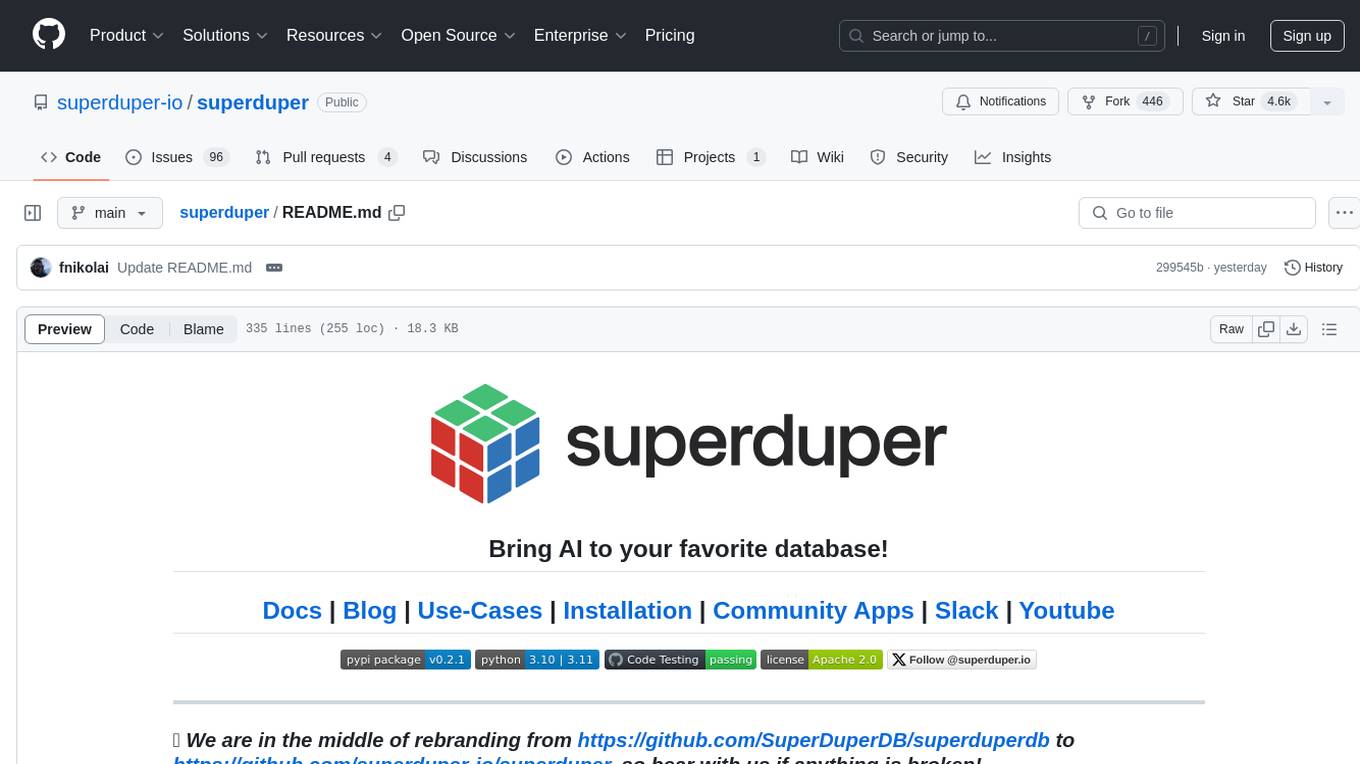
superduper
superduper.io is a Python framework that integrates AI models, APIs, and vector search engines directly with existing databases. It allows hosting of models, streaming inference, and scalable model training/fine-tuning. Key features include integration of AI with data infrastructure, inference via change-data-capture, scalable model training, model chaining, simple Python interface, Python-first approach, working with difficult data types, feature storing, and vector search capabilities. The tool enables users to turn their existing databases into centralized repositories for managing AI model inputs and outputs, as well as conducting vector searches without the need for specialized databases.
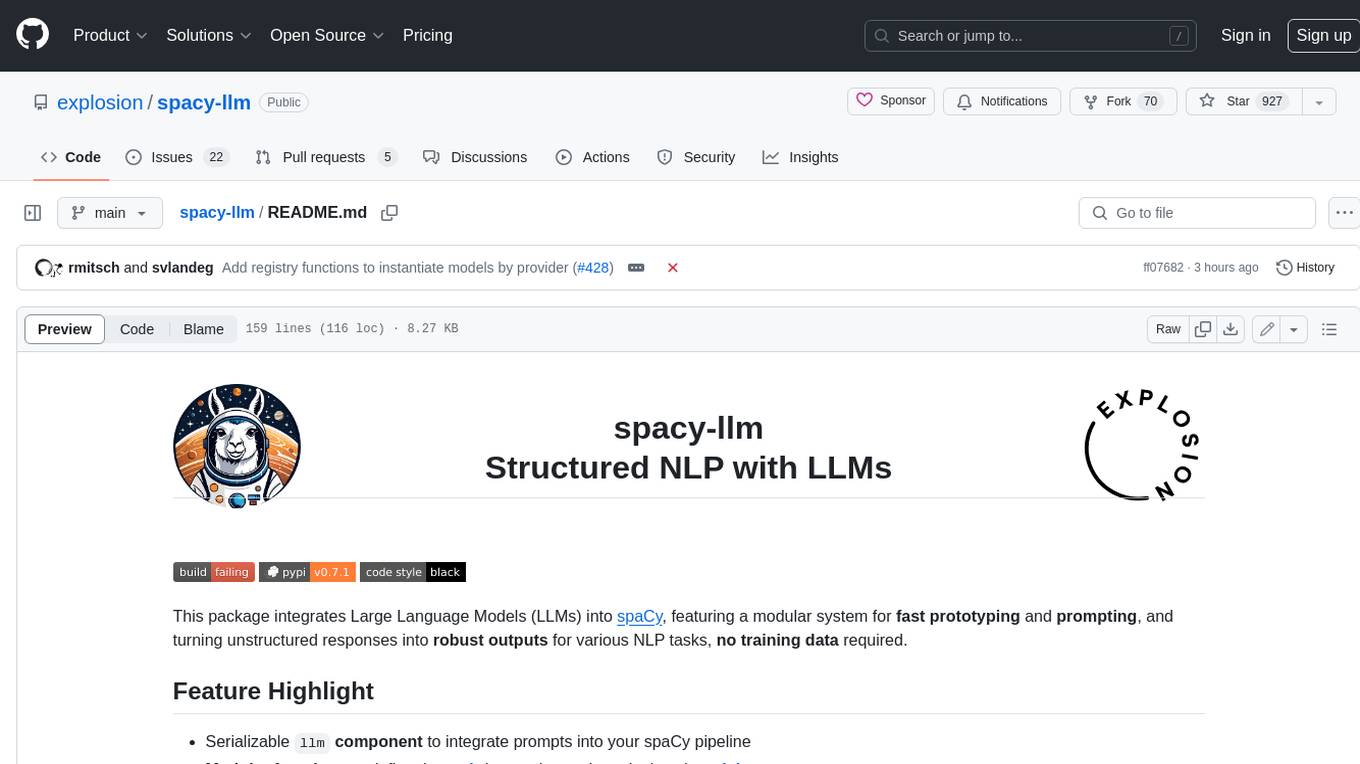
spacy-llm
This package integrates Large Language Models (LLMs) into spaCy, featuring a modular system for **fast prototyping** and **prompting** , and turning unstructured responses into **robust outputs** for various NLP tasks, **no training data** required. It supports open-source LLMs hosted on Hugging Face 🤗: Falcon, Dolly, Llama 2, OpenLLaMA, StableLM, Mistral. Integration with LangChain 🦜️🔗 - all `langchain` models and features can be used in `spacy-llm`. Tasks available out of the box: Named Entity Recognition, Text classification, Lemmatization, Relationship extraction, Sentiment analysis, Span categorization, Summarization, Entity linking, Translation, Raw prompt execution for maximum flexibility. Soon: Semantic role labeling. Easy implementation of **your own functions** via spaCy's registry for custom prompting, parsing and model integrations. For an example, see here. Map-reduce approach for splitting prompts too long for LLM's context window and fusing the results back together
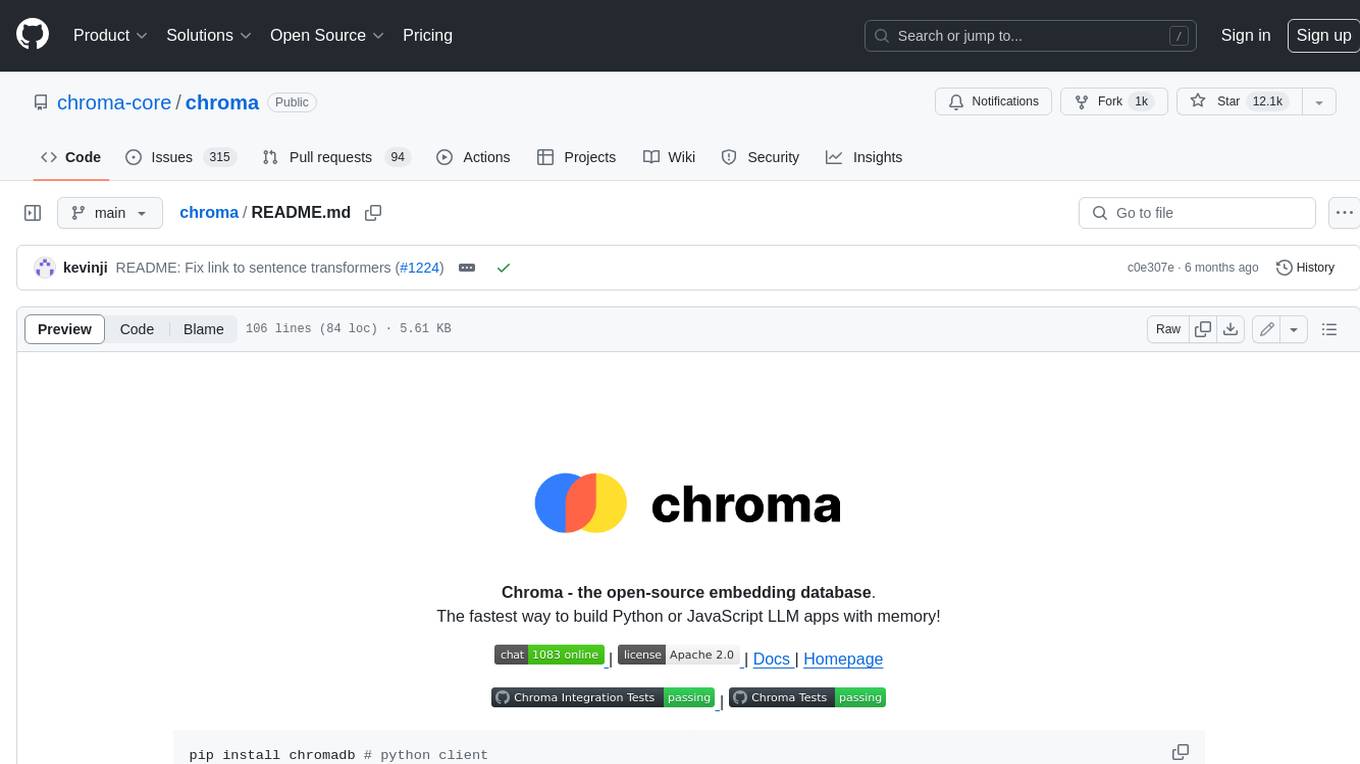
chroma
Chroma is an open-source embedding database that provides a simple, scalable, and feature-rich way to build Python or JavaScript LLM apps with memory. It offers a fully-typed, fully-tested, and fully-documented API that makes it easy to get started and scale your applications. Chroma also integrates with popular tools like LangChain and LlamaIndex, and supports a variety of embedding models, including Sentence Transformers, OpenAI embeddings, and Cohere embeddings. With Chroma, you can easily add documents to your database, query relevant documents with natural language, and compose documents into the context window of an LLM like GPT3 for additional summarization or analysis.
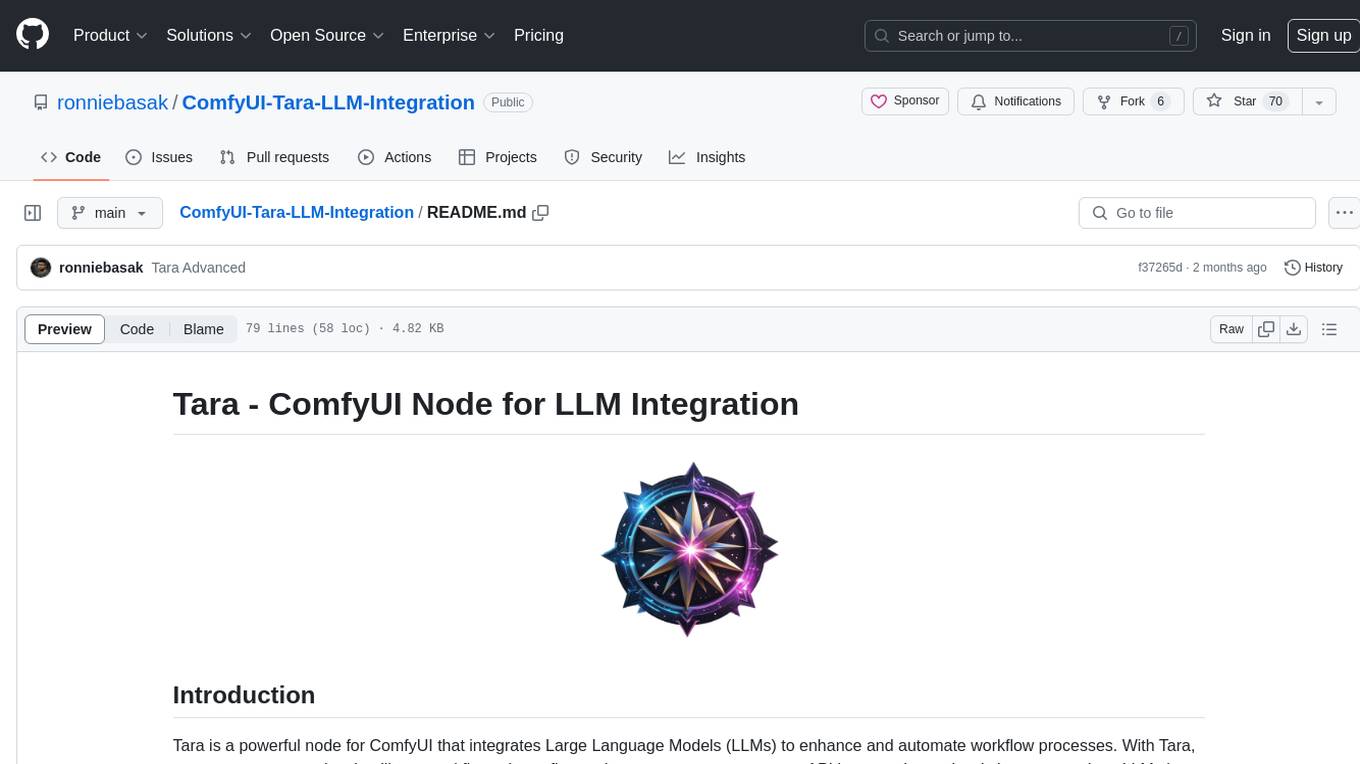
ComfyUI-Tara-LLM-Integration
Tara is a powerful node for ComfyUI that integrates Large Language Models (LLMs) to enhance and automate workflow processes. With Tara, you can create complex, intelligent workflows that refine and generate content, manage API keys, and seamlessly integrate various LLMs into your projects. It comprises nodes for handling OpenAI-compatible APIs, saving and loading API keys, composing multiple texts, and using predefined templates for OpenAI and Groq. Tara supports OpenAI and Grok models with plans to expand support to together.ai and Replicate. Users can install Tara via Git URL or ComfyUI Manager and utilize it for tasks like input guidance, saving and loading API keys, and generating text suitable for chaining in workflows.
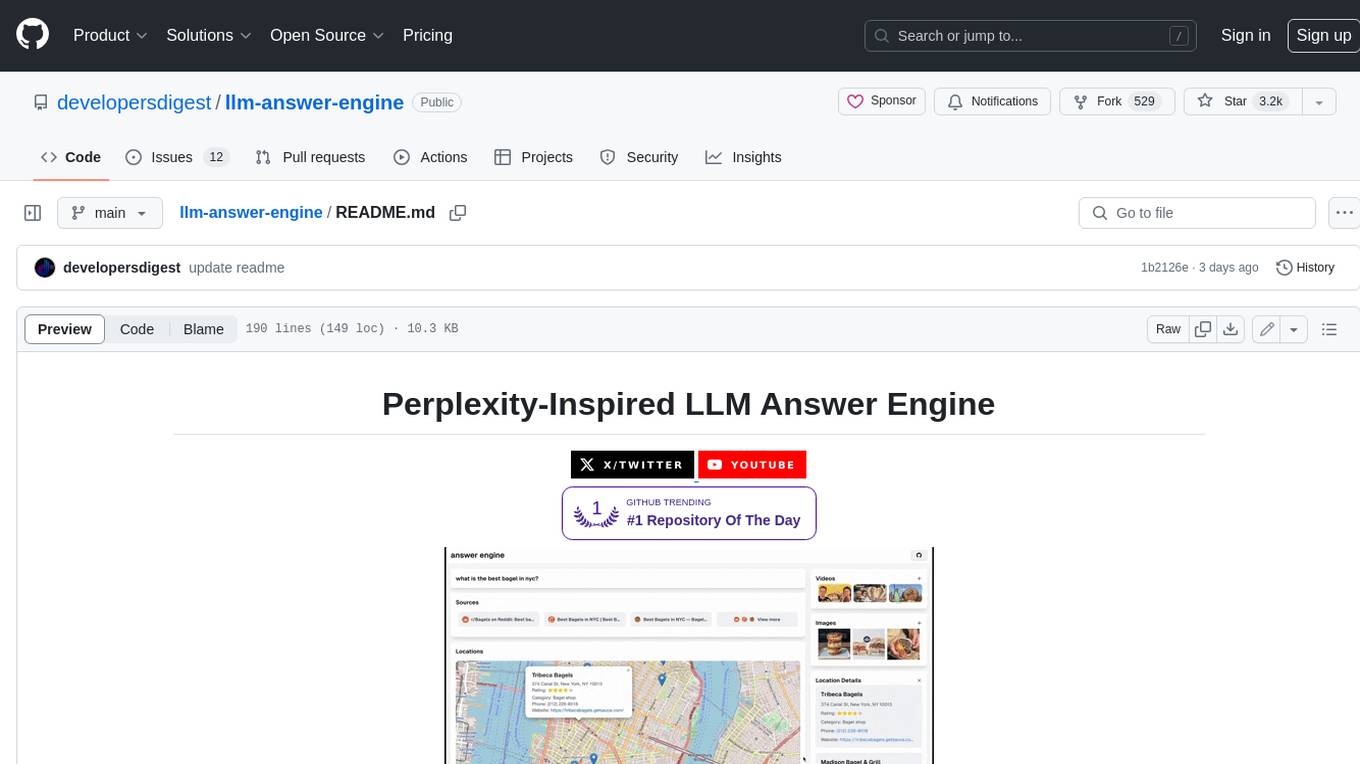
llm-answer-engine
This repository contains the code and instructions needed to build a sophisticated answer engine that leverages the capabilities of Groq, Mistral AI's Mixtral, Langchain.JS, Brave Search, Serper API, and OpenAI. Designed to efficiently return sources, answers, images, videos, and follow-up questions based on user queries, this project is an ideal starting point for developers interested in natural language processing and search technologies.
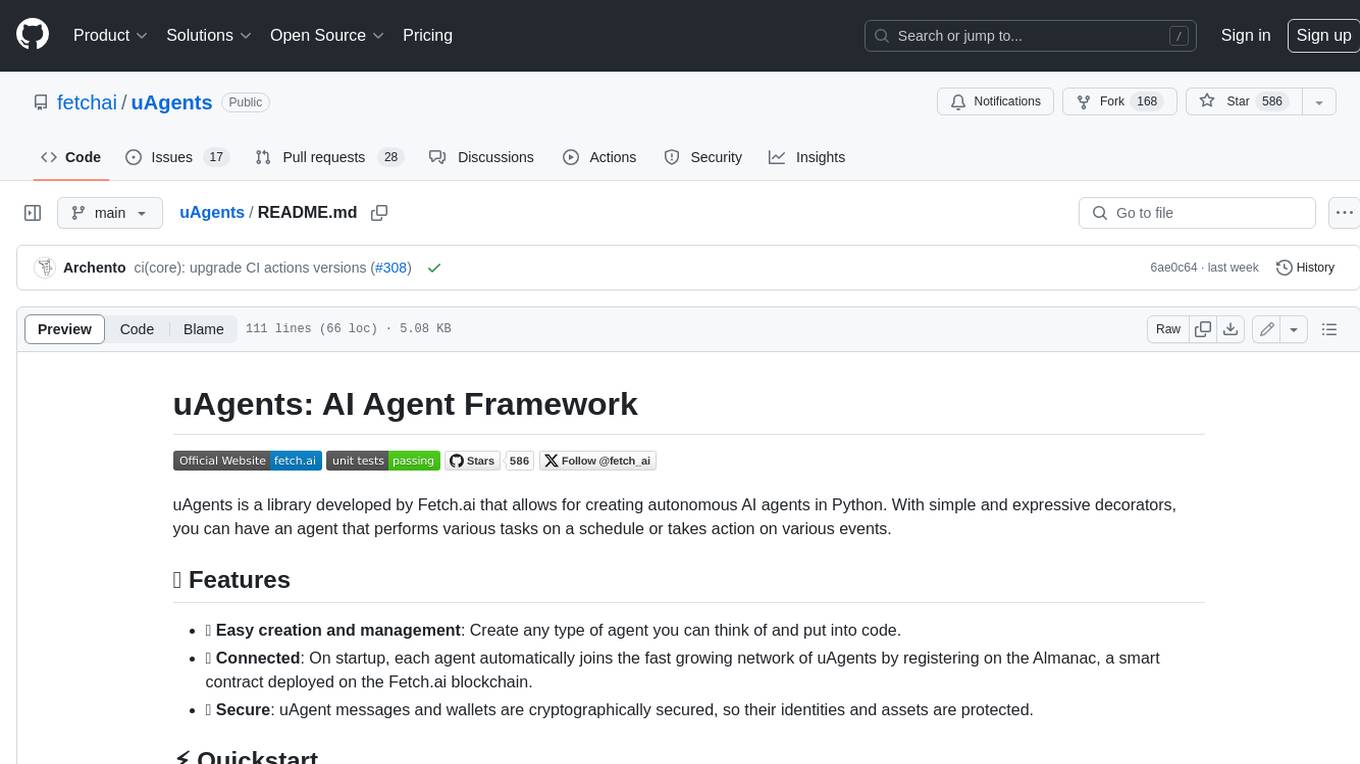
uAgents
uAgents is a Python library developed by Fetch.ai that allows for the creation of autonomous AI agents. These agents can perform various tasks on a schedule or take action on various events. uAgents are easy to create and manage, and they are connected to a fast-growing network of other uAgents. They are also secure, with cryptographically secured messages and wallets.
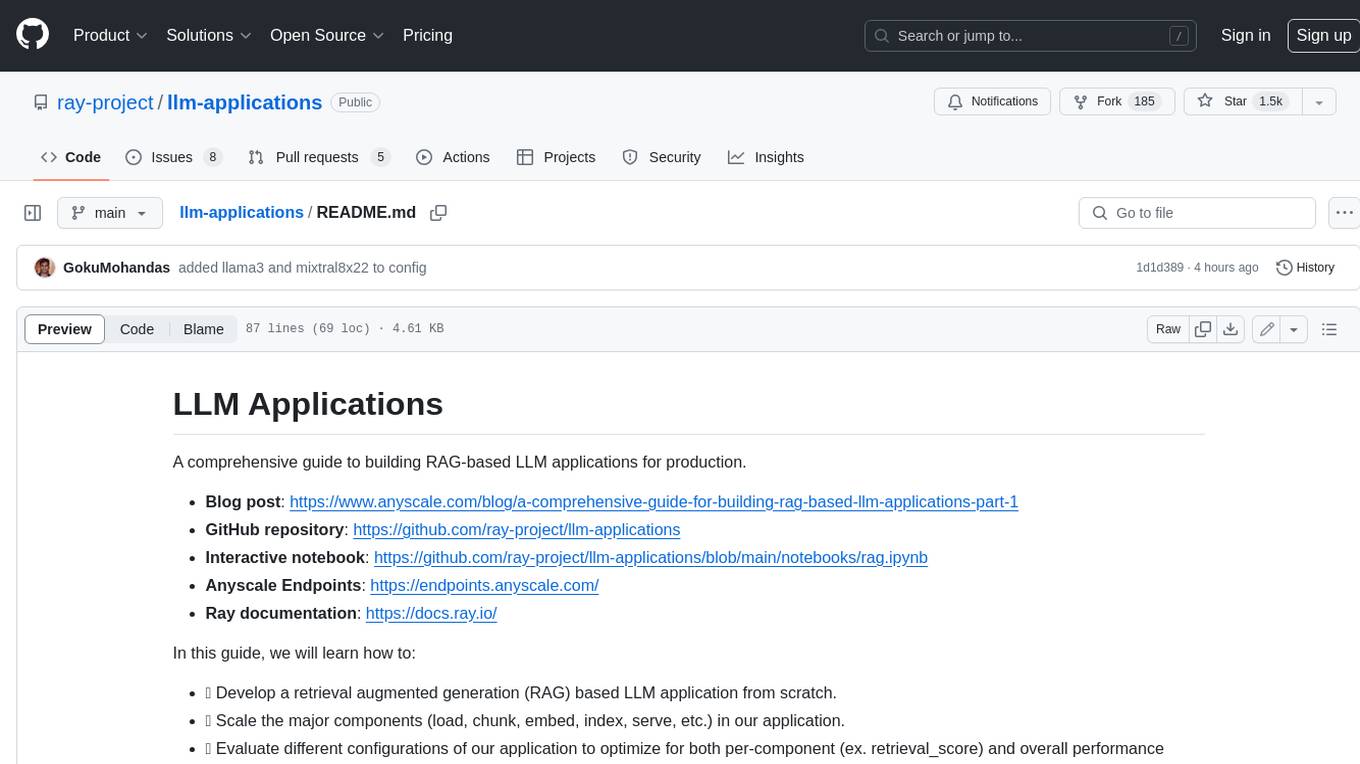
llm-applications
A comprehensive guide to building Retrieval Augmented Generation (RAG)-based LLM applications for production. This guide covers developing a RAG-based LLM application from scratch, scaling the major components, evaluating different configurations, implementing LLM hybrid routing, serving the application in a highly scalable and available manner, and sharing the impacts LLM applications have had on products.
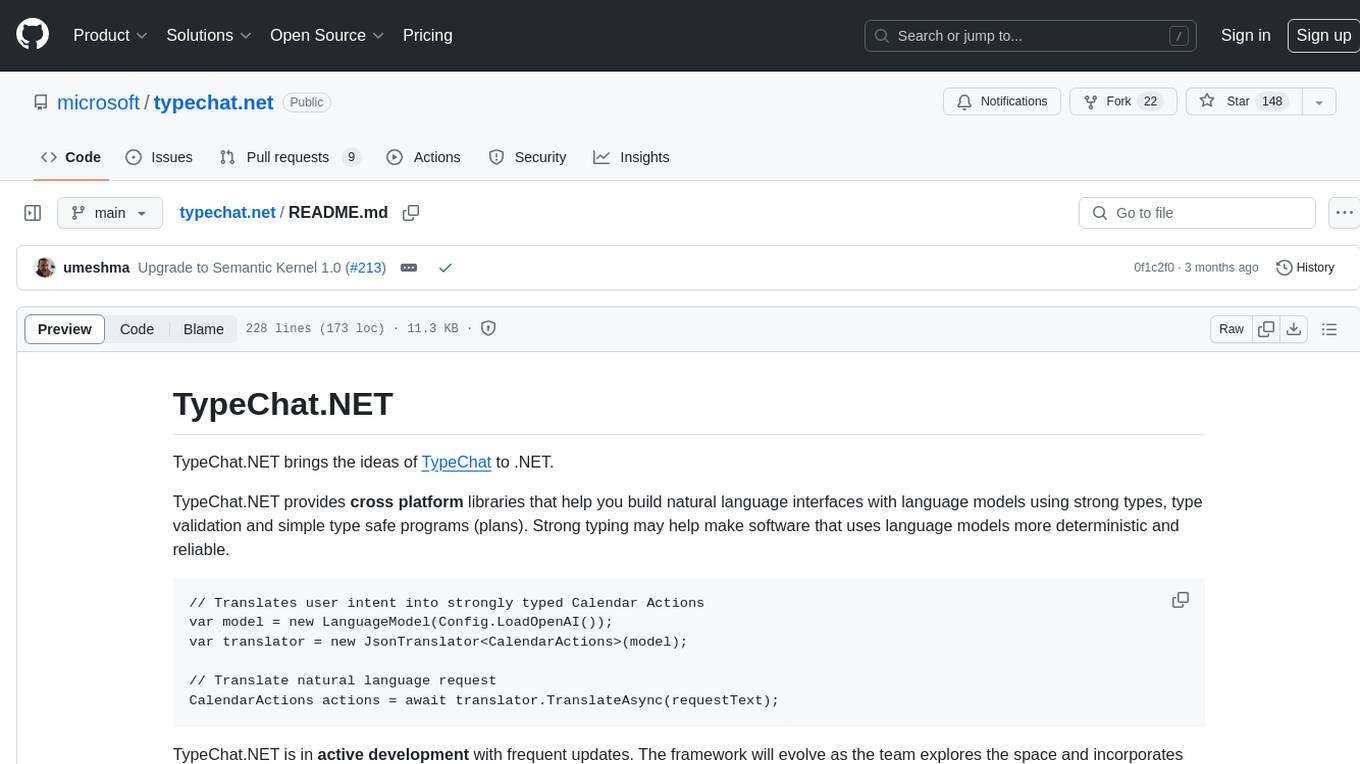
typechat.net
TypeChat.NET is a framework that provides cross-platform libraries for building natural language interfaces with language models using strong types, type validation, and simple type-safe programs. It translates user intent into strongly typed objects and JSON programs, with support for schema export, extensibility, and common scenarios. The framework is actively developed with frequent updates, evolving based on exploration and feedback. It consists of assemblies for translating user intent, synthesizing JSON programs, and integrating with Microsoft Semantic Kernel. TypeChat.NET requires familiarity with and access to OpenAI language models for its examples and scenarios.
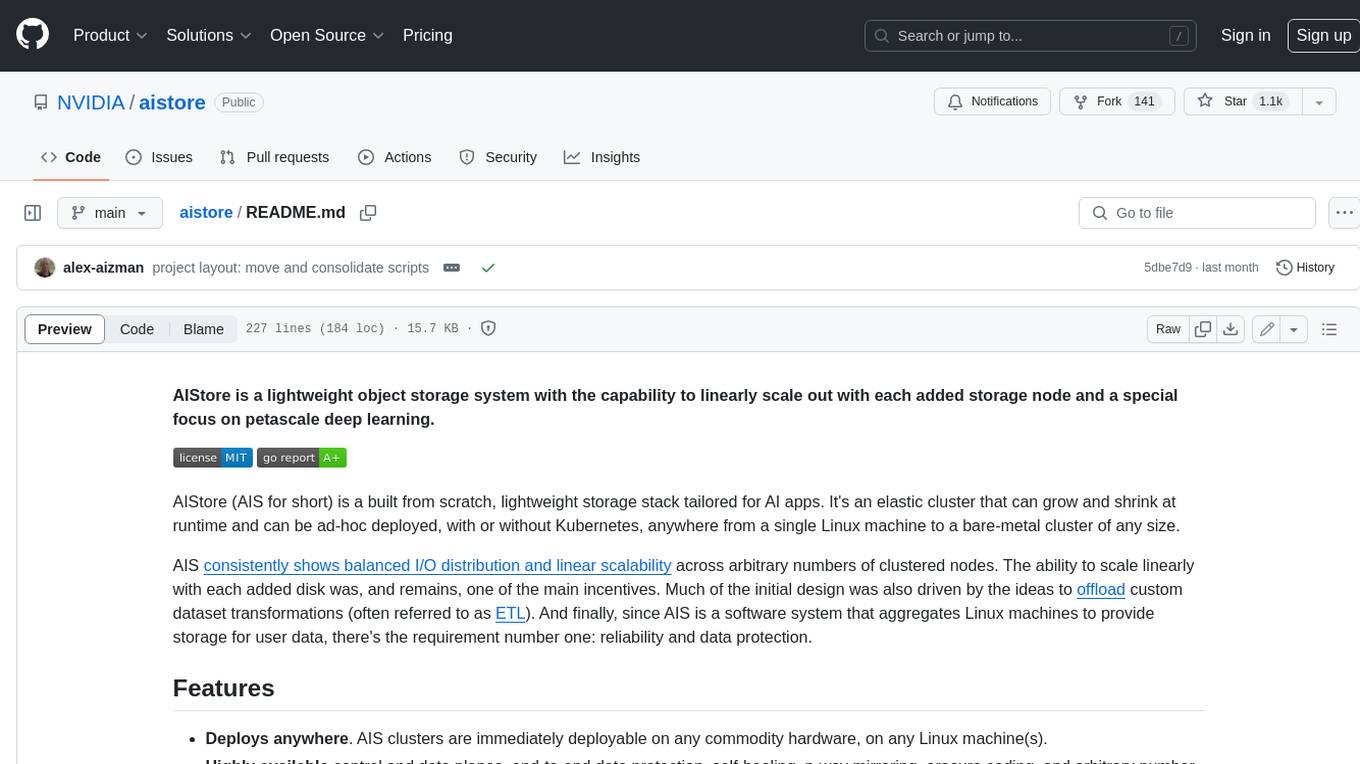
aistore
AIStore is a lightweight object storage system designed for AI applications. It is highly scalable, reliable, and easy to use. AIStore can be deployed on any commodity hardware, and it can be used to store and manage large datasets for deep learning and other AI applications.
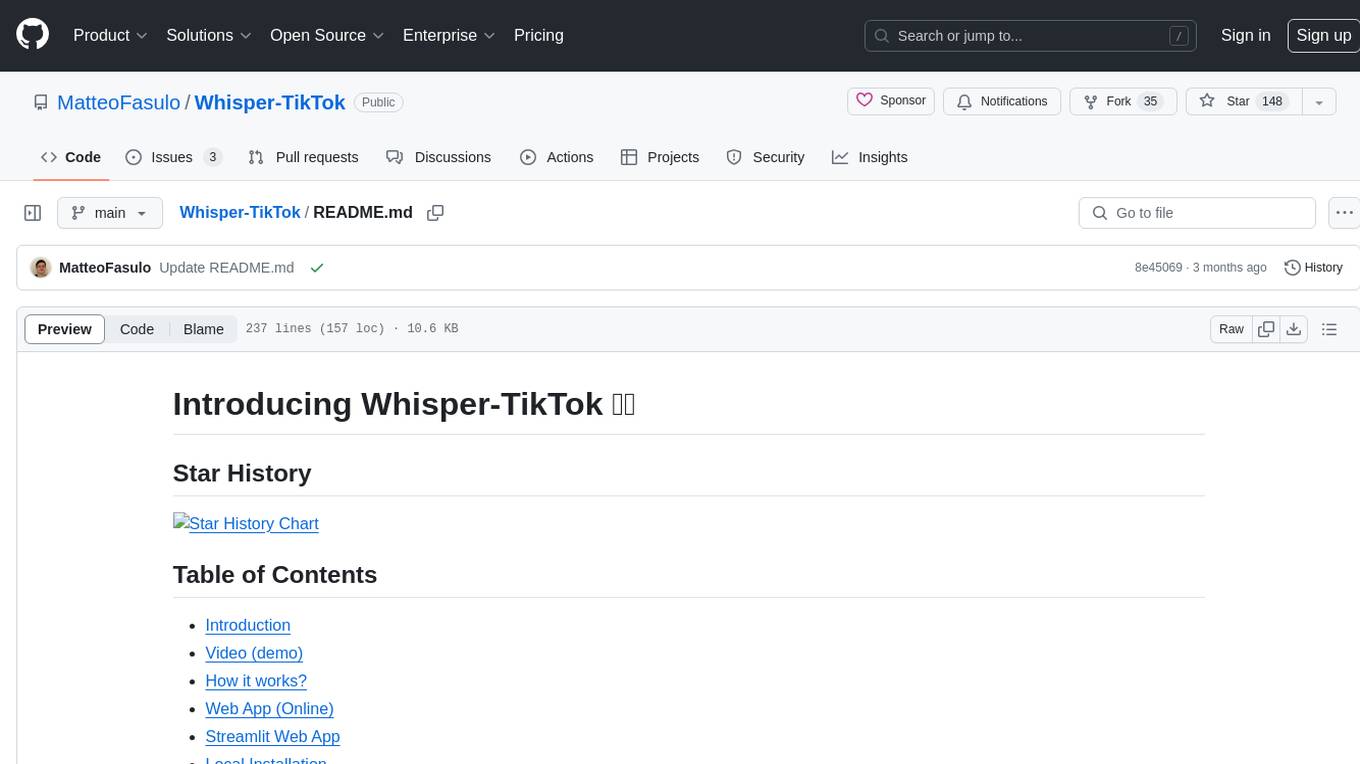
Whisper-TikTok
Discover Whisper-TikTok, an innovative AI-powered tool that leverages the prowess of Edge TTS, OpenAI-Whisper, and FFMPEG to craft captivating TikTok videos. Whisper-TikTok effortlessly generates accurate transcriptions from audio files and integrates Microsoft Edge Cloud Text-to-Speech API for vibrant voiceovers. The program orchestrates the synthesis of videos using a structured JSON dataset, generating mesmerizing TikTok content in minutes.
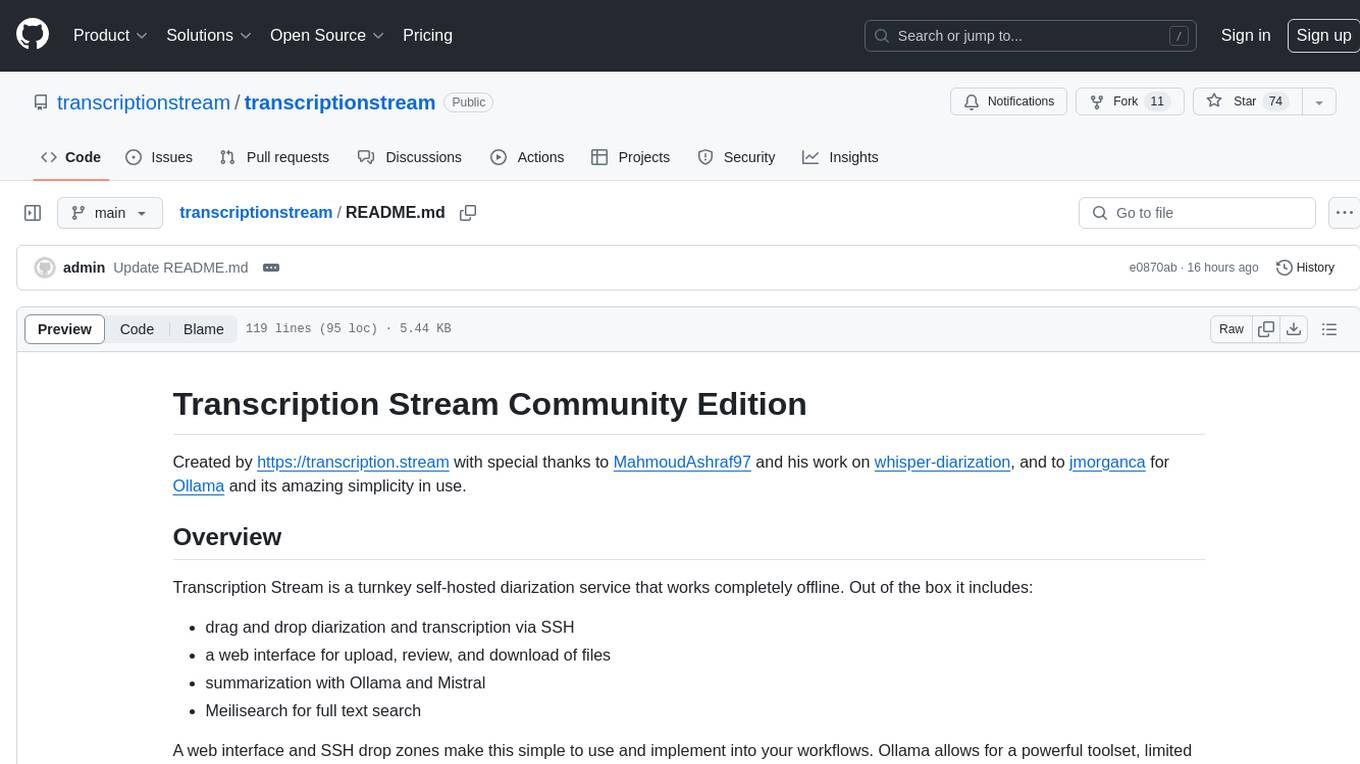
transcriptionstream
Transcription Stream is a self-hosted diarization service that works offline, allowing users to easily transcribe and summarize audio files. It includes a web interface for file management, Ollama for complex operations on transcriptions, and Meilisearch for fast full-text search. Users can upload files via SSH or web interface, with output stored in named folders. The tool requires a NVIDIA GPU and provides various scripts for installation and running. Ports for SSH, HTTP, Ollama, and Meilisearch are specified, along with access details for SSH server and web interface. Customization options and troubleshooting tips are provided in the documentation.
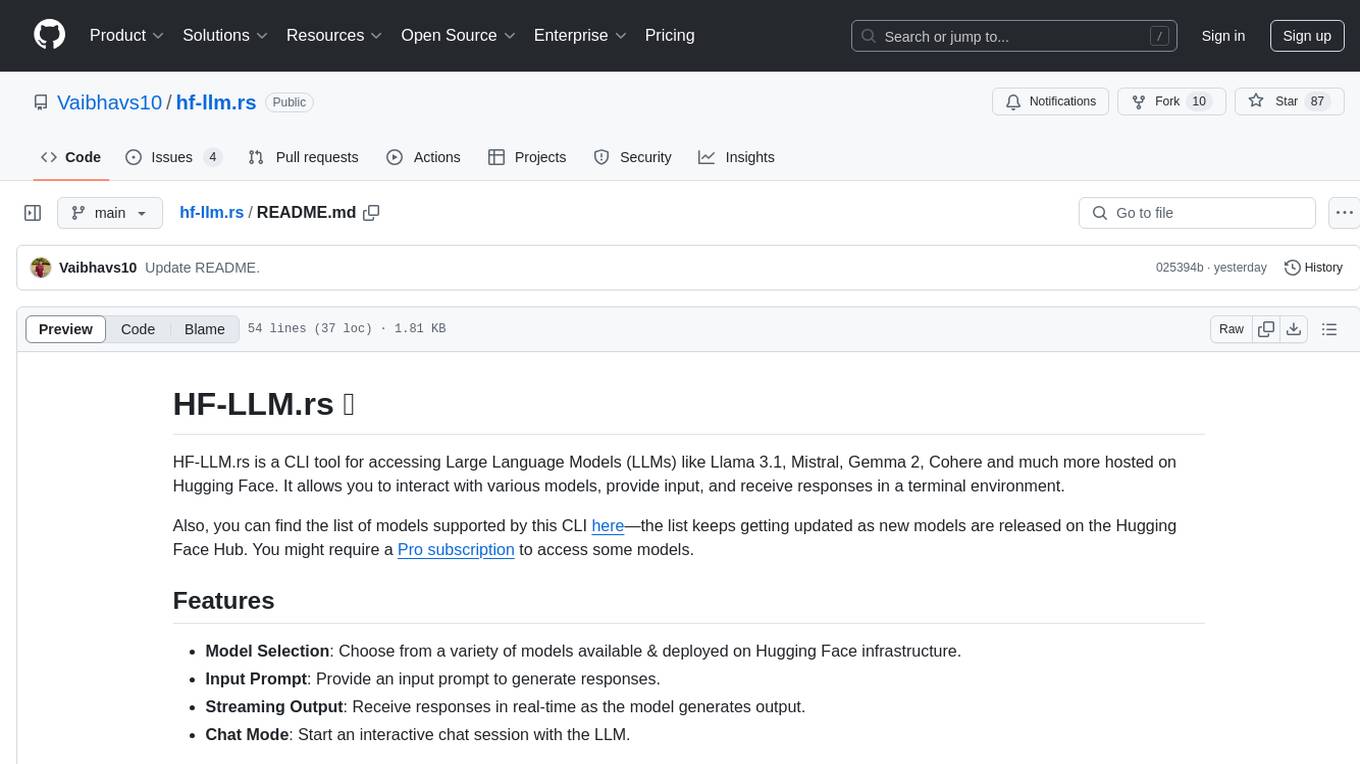
hf-llm.rs
HF-LLM.rs is a CLI tool for accessing Large Language Models (LLMs) like Llama 3.1, Mistral, Gemma 2, Cohere and more hosted on Hugging Face. It allows interaction with various models, providing input and receiving responses in a terminal environment. Users can select models, input prompts, receive streaming output, and engage in chat mode. The tool supports a variety of models available on Hugging Face infrastructure, with the list continuously updated. Some models may require a Pro subscription for access.
For similar tasks
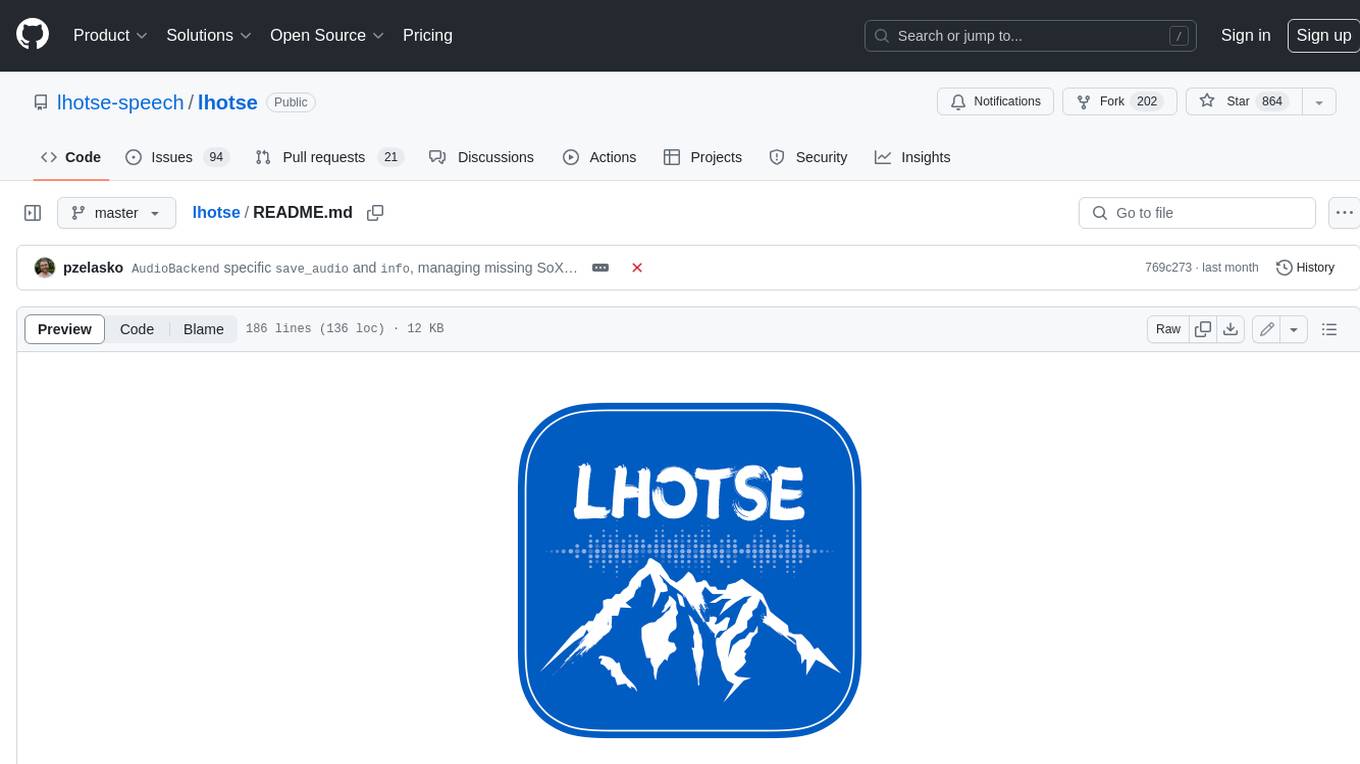
lhotse
Lhotse is a Python library designed to make speech and audio data preparation flexible and accessible. It aims to attract a wider community to speech processing tasks by providing a Python-centric design and an expressive command-line interface. Lhotse offers standard data preparation recipes, PyTorch Dataset classes for speech tasks, and efficient data preparation for model training with audio cuts. It supports data augmentation, feature extraction, and feature-space cut mixing. The tool extends Kaldi's data preparation recipes with seamless PyTorch integration, human-readable text manifests, and convenient Python classes.
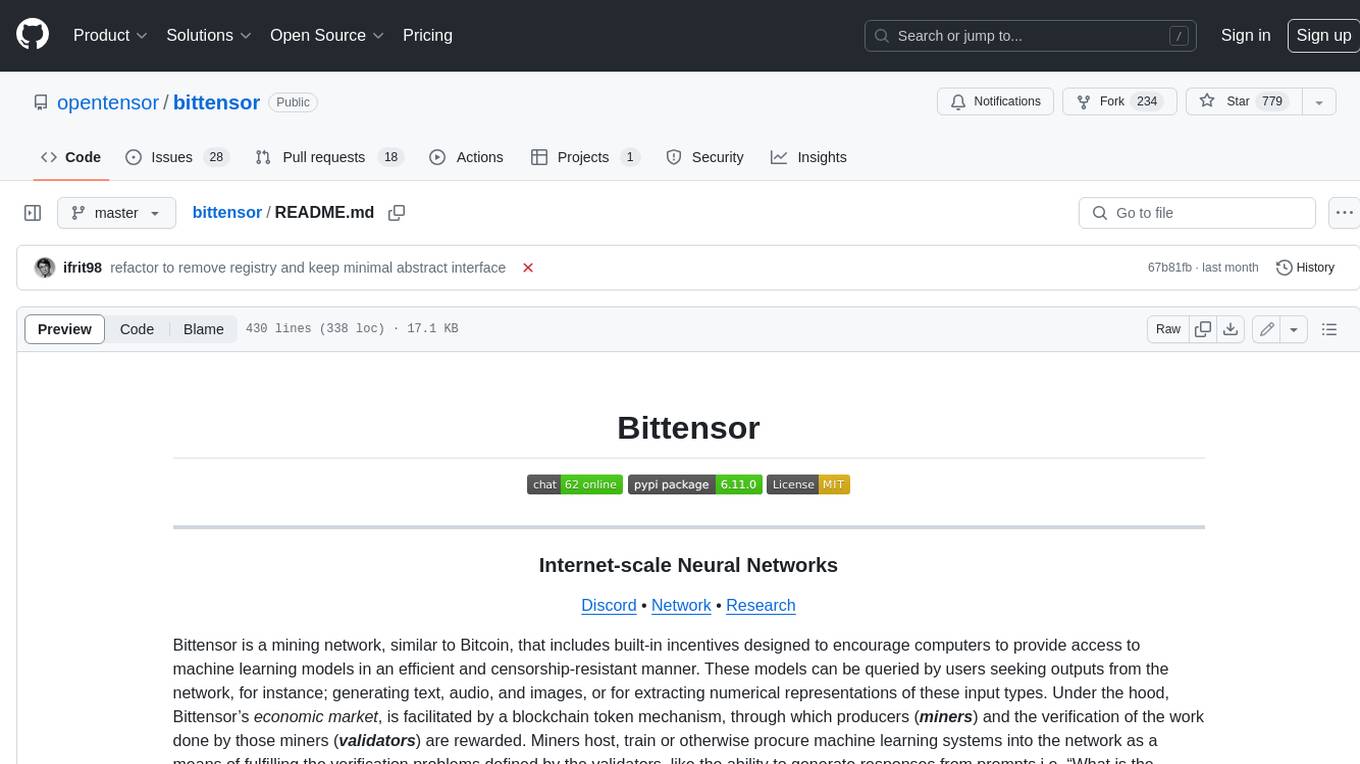
bittensor
Bittensor is an internet-scale neural network that incentivizes computers to provide access to machine learning models in a decentralized and censorship-resistant manner. It operates through a token-based mechanism where miners host, train, and procure machine learning systems to fulfill verification problems defined by validators. The network rewards miners and validators for their contributions, ensuring continuous improvement in knowledge output. Bittensor allows anyone to participate, extract value, and govern the network without centralized control. It supports tasks such as generating text, audio, images, and extracting numerical representations.
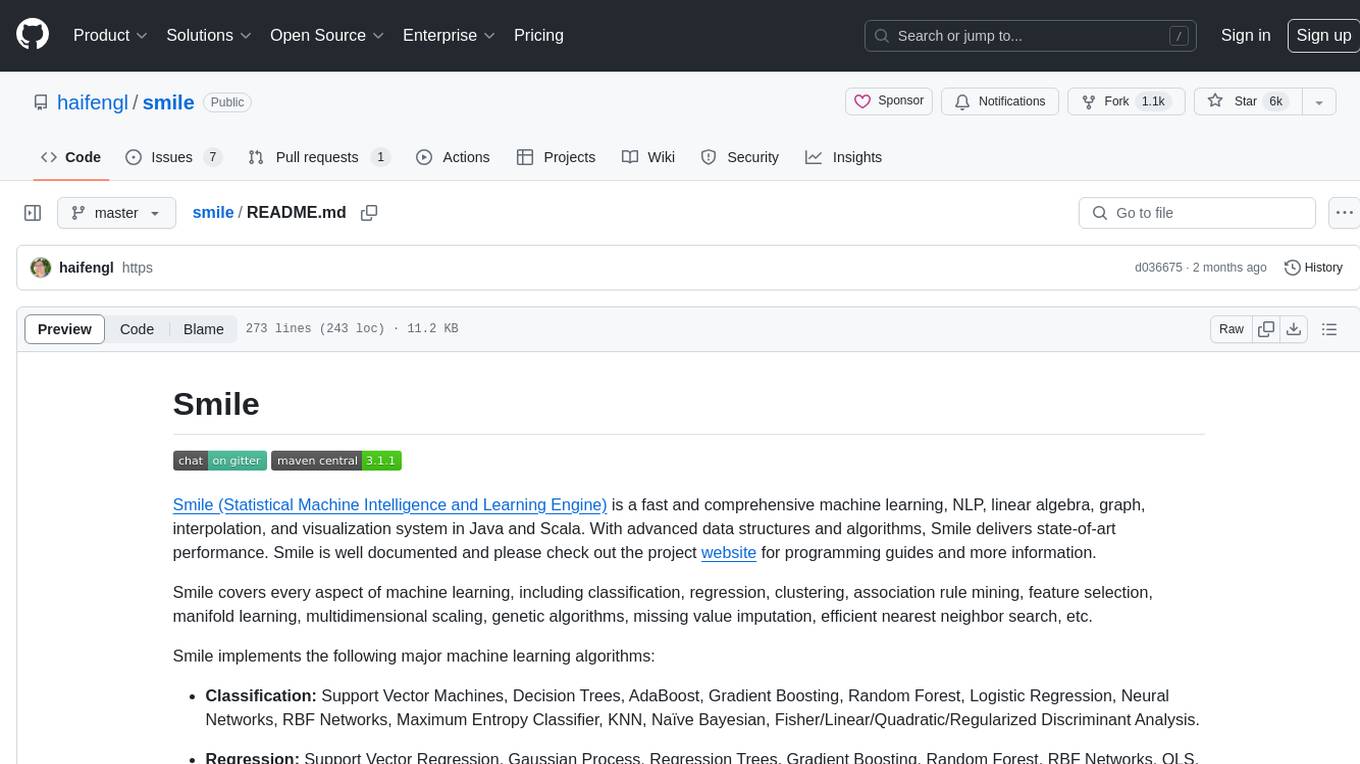
smile
Smile (Statistical Machine Intelligence and Learning Engine) is a comprehensive machine learning, NLP, linear algebra, graph, interpolation, and visualization system in Java and Scala. It covers every aspect of machine learning, including classification, regression, clustering, association rule mining, feature selection, manifold learning, multidimensional scaling, genetic algorithms, missing value imputation, efficient nearest neighbor search, etc. Smile implements major machine learning algorithms and provides interactive shells for Java, Scala, and Kotlin. It supports model serialization, data visualization using SmilePlot and declarative approach, and offers a gallery showcasing various algorithms and visualizations.
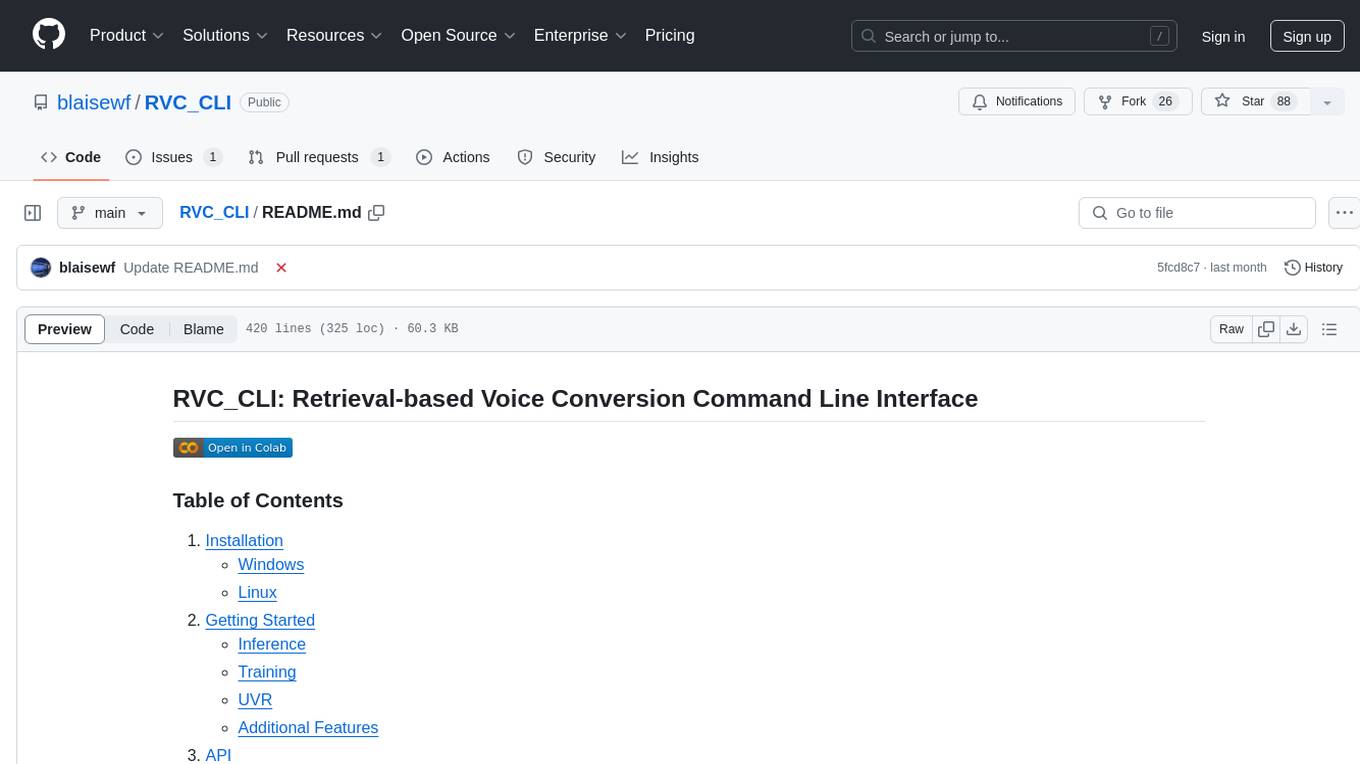
RVC_CLI
RVC_CLI is a command line interface tool for retrieval-based voice conversion. It provides functionalities for installation, getting started, inference, training, UVR, additional features, and API integration. Users can perform tasks like single inference, batch inference, TTS inference, preprocess dataset, extract features, start training, generate index file, model extract, model information, model blender, launch TensorBoard, download models, audio analyzer, and prerequisites download. The tool is built on various projects like ContentVec, HIFIGAN, audio-slicer, python-audio-separator, RMVPE, FCPE, VITS, So-Vits-SVC, Harmonify, and others.
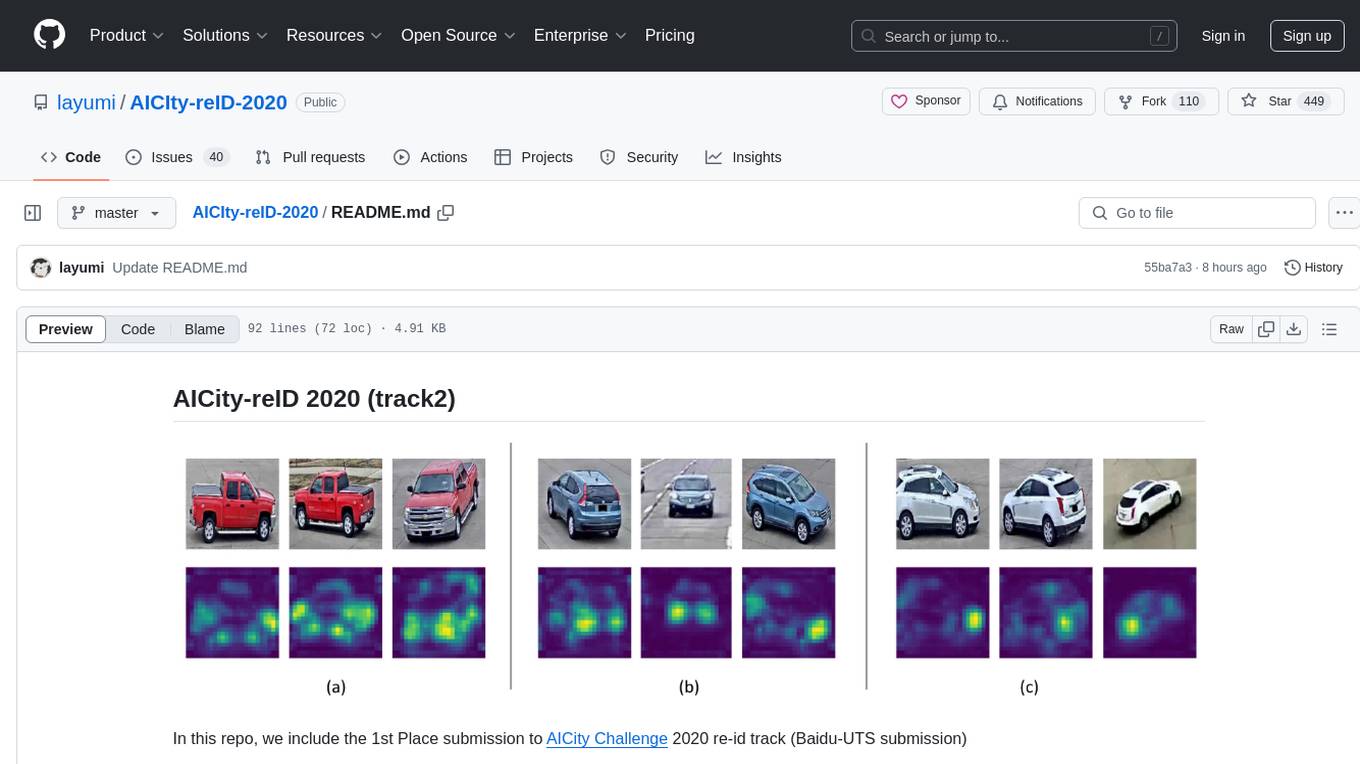
AICIty-reID-2020
AICIty-reID 2020 is a repository containing the 1st Place submission to AICity Challenge 2020 re-id track by Baidu-UTS. It includes models trained on Paddlepaddle and Pytorch, with performance metrics and trained models provided. Users can extract features, perform camera and direction prediction, and access related repositories for drone-based building re-id, vehicle re-ID, person re-ID baseline, and person/vehicle generation. Citations are also provided for research purposes.
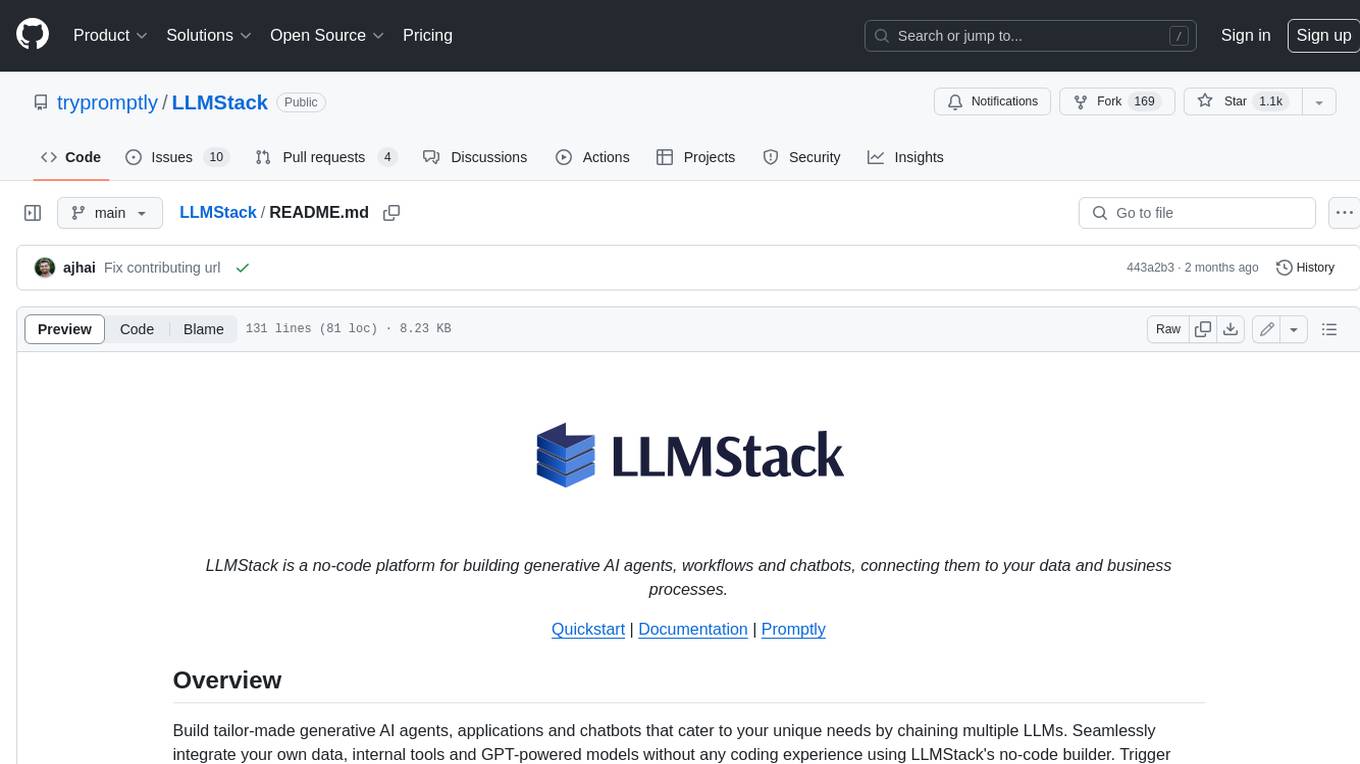
LLMStack
LLMStack is a no-code platform for building generative AI agents, workflows, and chatbots. It allows users to connect their own data, internal tools, and GPT-powered models without any coding experience. LLMStack can be deployed to the cloud or on-premise and can be accessed via HTTP API or triggered from Slack or Discord.
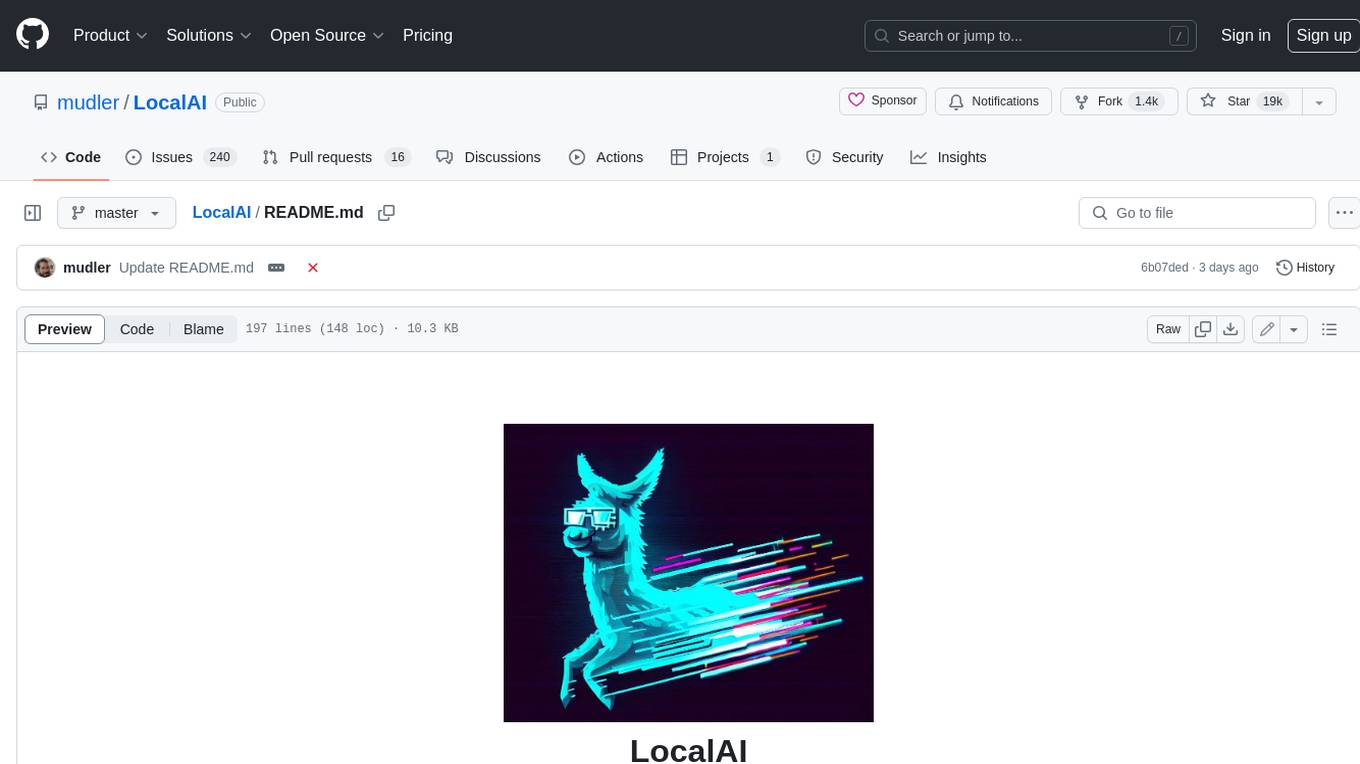
LocalAI
LocalAI is a free and open-source OpenAI alternative that acts as a drop-in replacement REST API compatible with OpenAI (Elevenlabs, Anthropic, etc.) API specifications for local AI inferencing. It allows users to run LLMs, generate images, audio, and more locally or on-premises with consumer-grade hardware, supporting multiple model families and not requiring a GPU. LocalAI offers features such as text generation with GPTs, text-to-audio, audio-to-text transcription, image generation with stable diffusion, OpenAI functions, embeddings generation for vector databases, constrained grammars, downloading models directly from Huggingface, and a Vision API. It provides a detailed step-by-step introduction in its Getting Started guide and supports community integrations such as custom containers, WebUIs, model galleries, and various bots for Discord, Slack, and Telegram. LocalAI also offers resources like an LLM fine-tuning guide, instructions for local building and Kubernetes installation, projects integrating LocalAI, and a how-tos section curated by the community. It encourages users to cite the repository when utilizing it in downstream projects and acknowledges the contributions of various software from the community.
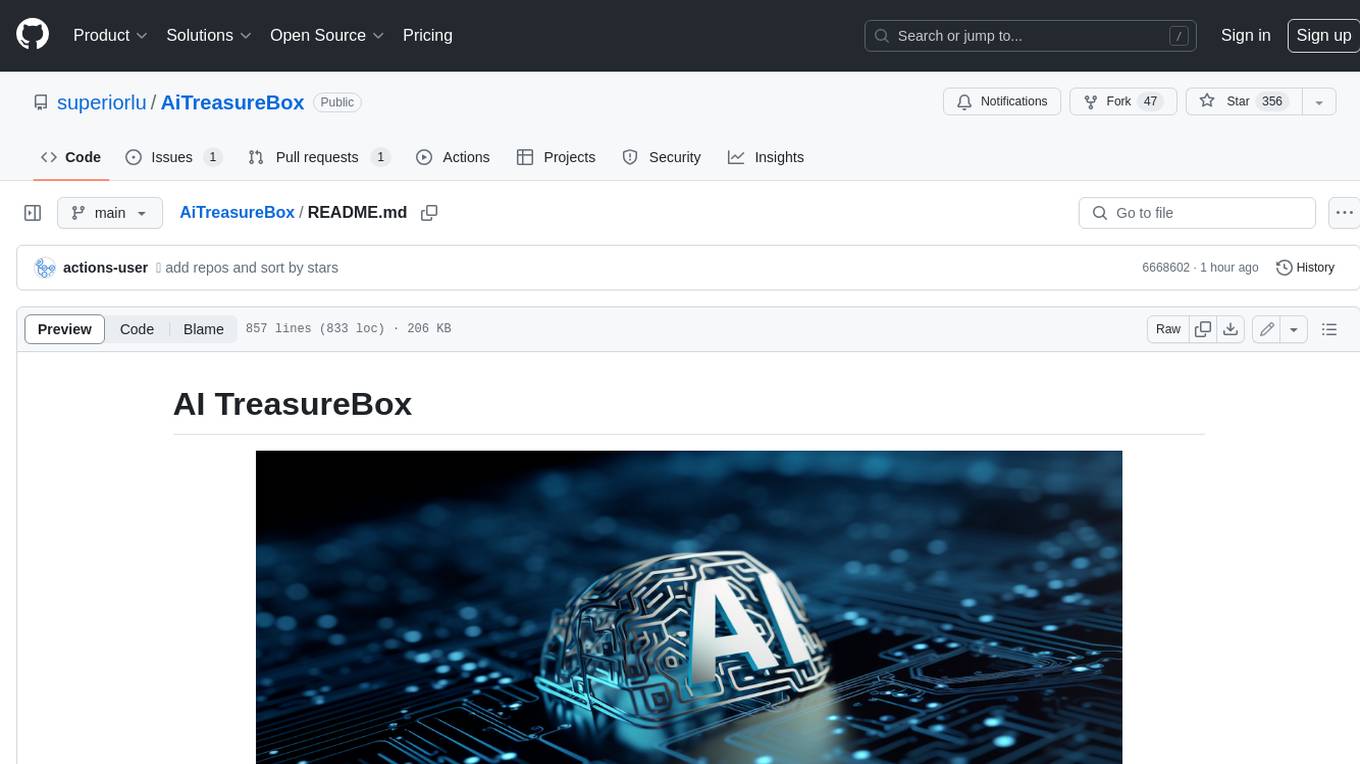
AiTreasureBox
AiTreasureBox is a versatile AI tool that provides a collection of pre-trained models and algorithms for various machine learning tasks. It simplifies the process of implementing AI solutions by offering ready-to-use components that can be easily integrated into projects. With AiTreasureBox, users can quickly prototype and deploy AI applications without the need for extensive knowledge in machine learning or deep learning. The tool covers a wide range of tasks such as image classification, text generation, sentiment analysis, object detection, and more. It is designed to be user-friendly and accessible to both beginners and experienced developers, making AI development more efficient and accessible to a wider audience.
For similar jobs
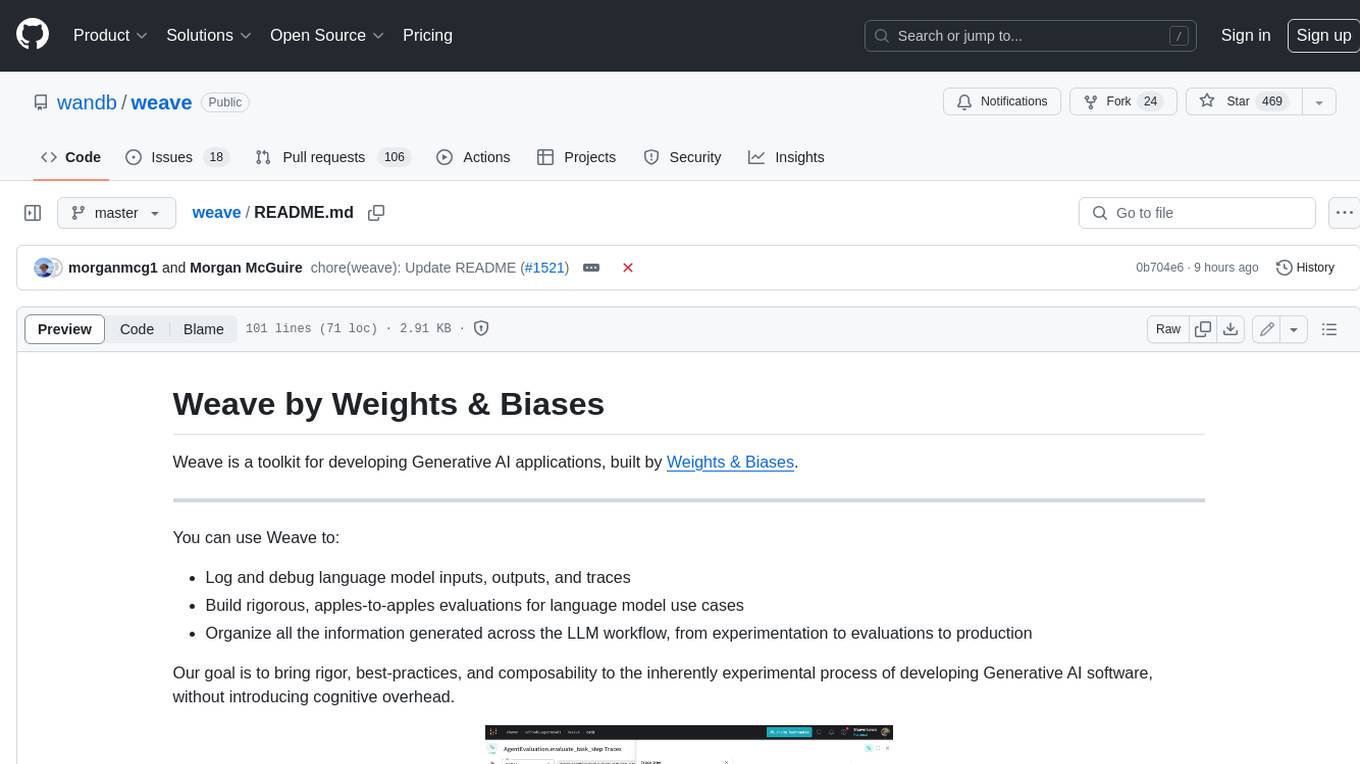
weave
Weave is a toolkit for developing Generative AI applications, built by Weights & Biases. With Weave, you can log and debug language model inputs, outputs, and traces; build rigorous, apples-to-apples evaluations for language model use cases; and organize all the information generated across the LLM workflow, from experimentation to evaluations to production. Weave aims to bring rigor, best-practices, and composability to the inherently experimental process of developing Generative AI software, without introducing cognitive overhead.
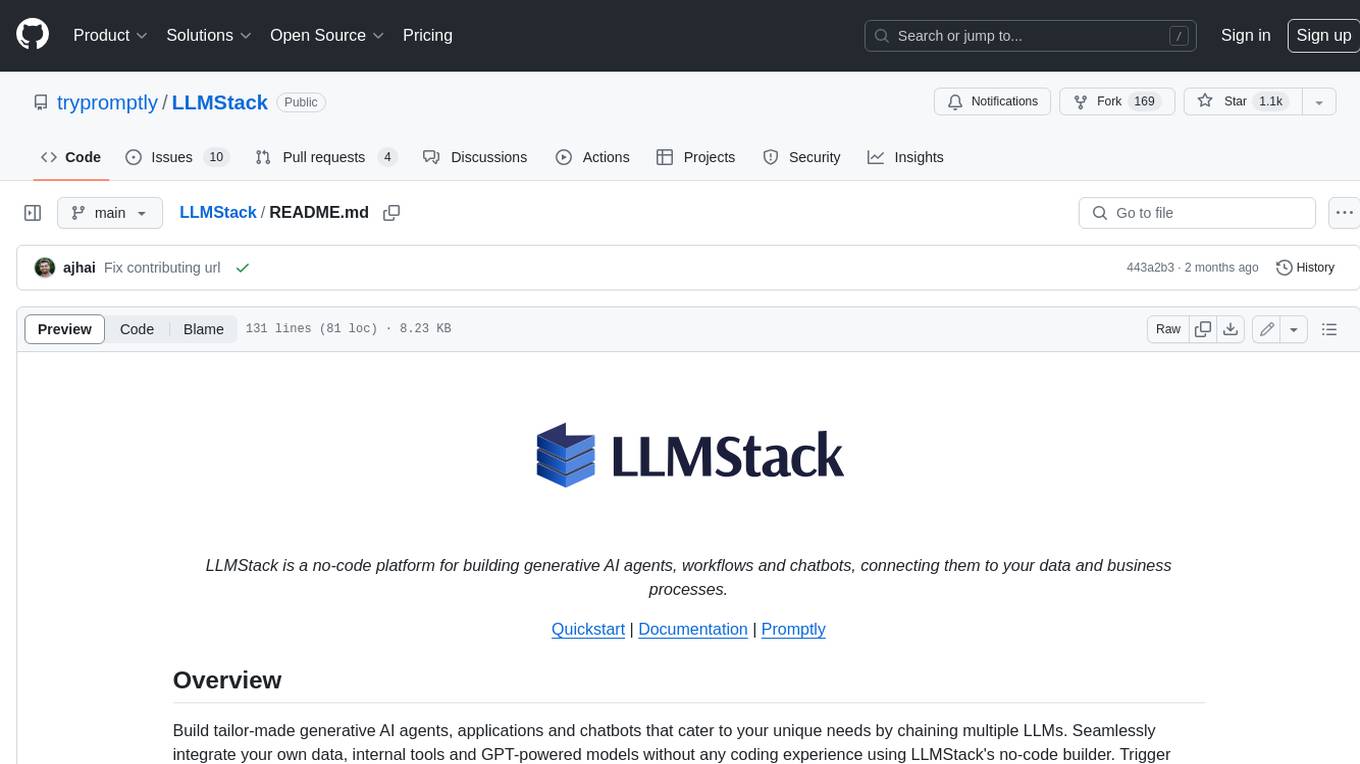
LLMStack
LLMStack is a no-code platform for building generative AI agents, workflows, and chatbots. It allows users to connect their own data, internal tools, and GPT-powered models without any coding experience. LLMStack can be deployed to the cloud or on-premise and can be accessed via HTTP API or triggered from Slack or Discord.
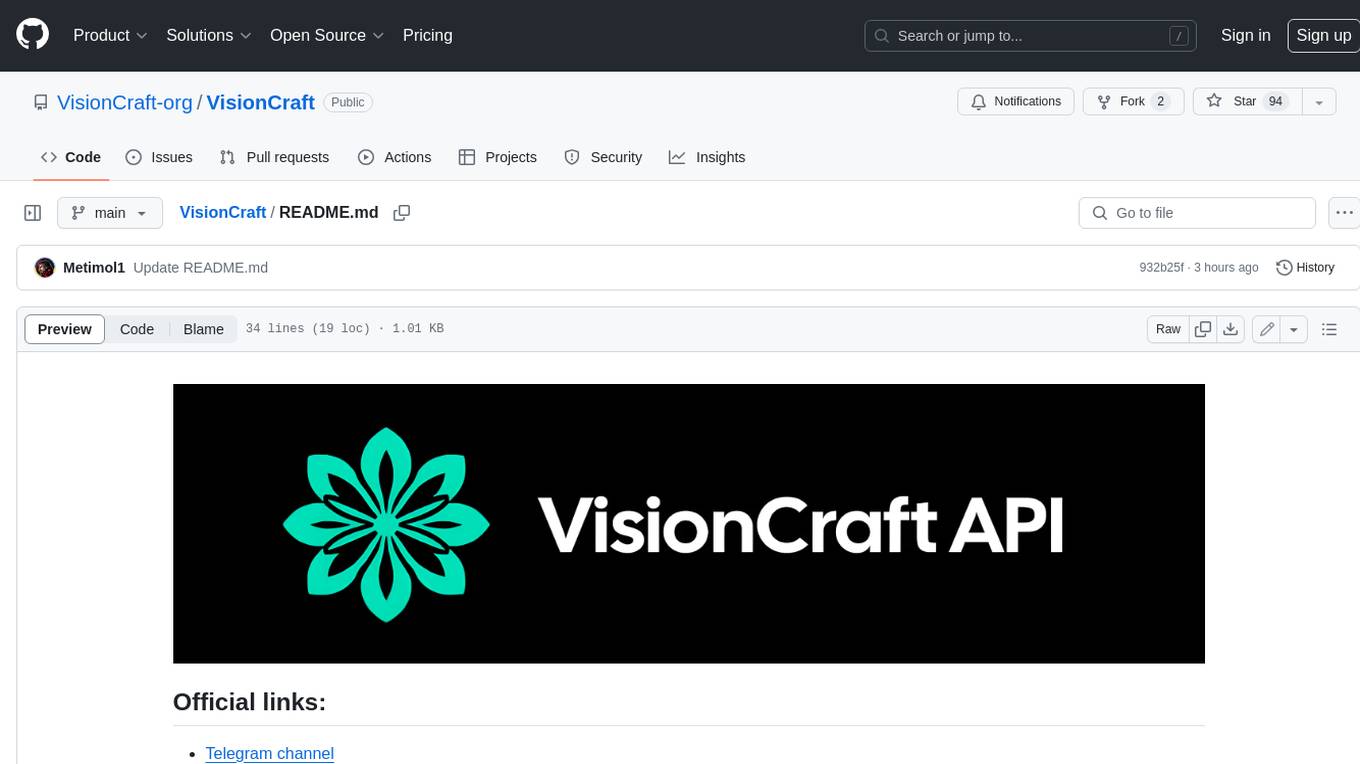
VisionCraft
The VisionCraft API is a free API for using over 100 different AI models. From images to sound.
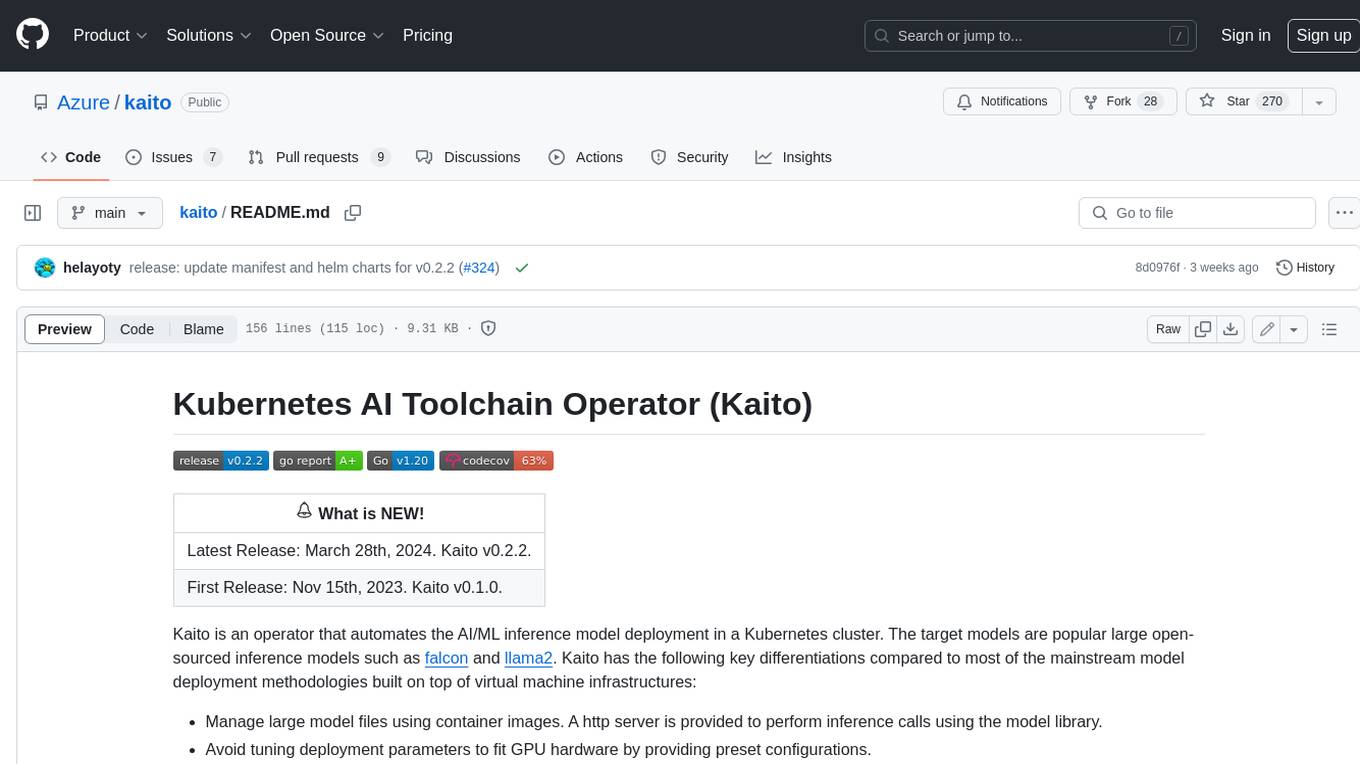
kaito
Kaito is an operator that automates the AI/ML inference model deployment in a Kubernetes cluster. It manages large model files using container images, avoids tuning deployment parameters to fit GPU hardware by providing preset configurations, auto-provisions GPU nodes based on model requirements, and hosts large model images in the public Microsoft Container Registry (MCR) if the license allows. Using Kaito, the workflow of onboarding large AI inference models in Kubernetes is largely simplified.
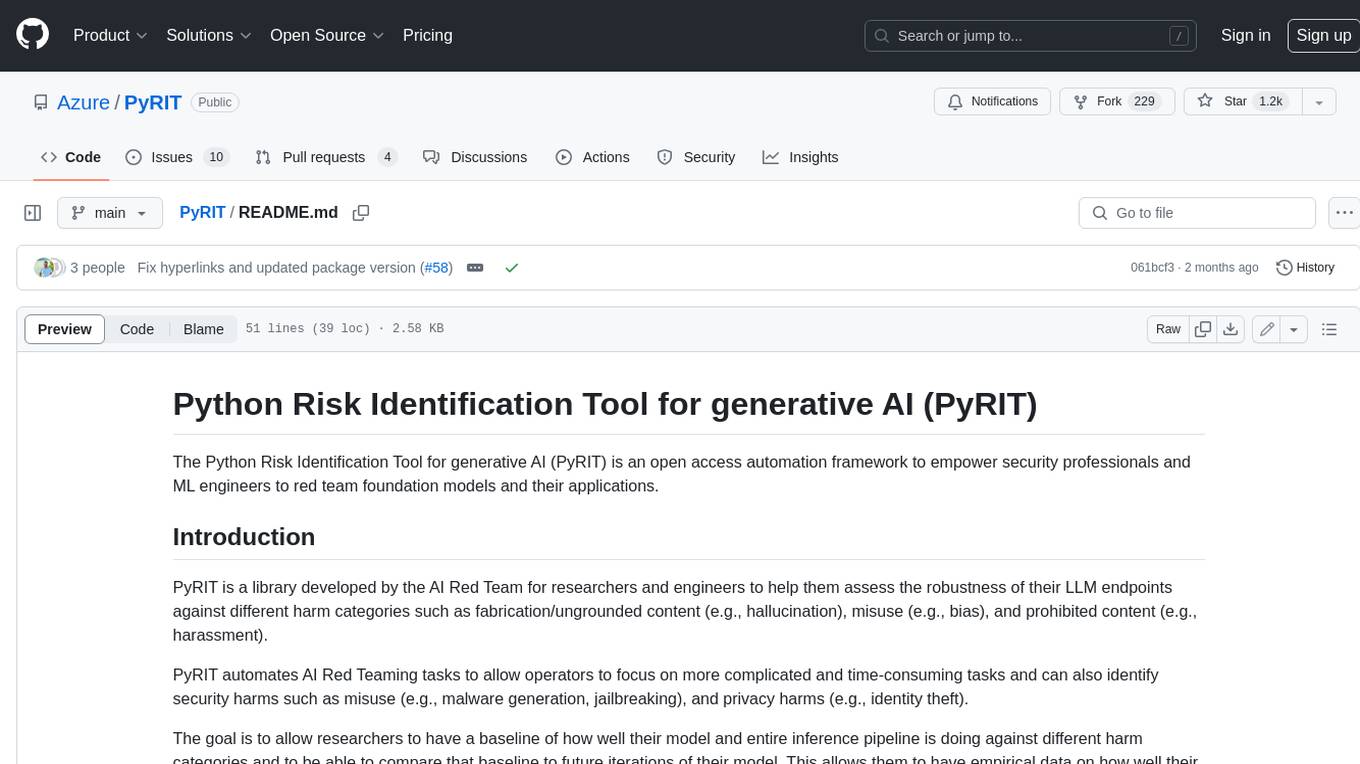
PyRIT
PyRIT is an open access automation framework designed to empower security professionals and ML engineers to red team foundation models and their applications. It automates AI Red Teaming tasks to allow operators to focus on more complicated and time-consuming tasks and can also identify security harms such as misuse (e.g., malware generation, jailbreaking), and privacy harms (e.g., identity theft). The goal is to allow researchers to have a baseline of how well their model and entire inference pipeline is doing against different harm categories and to be able to compare that baseline to future iterations of their model. This allows them to have empirical data on how well their model is doing today, and detect any degradation of performance based on future improvements.
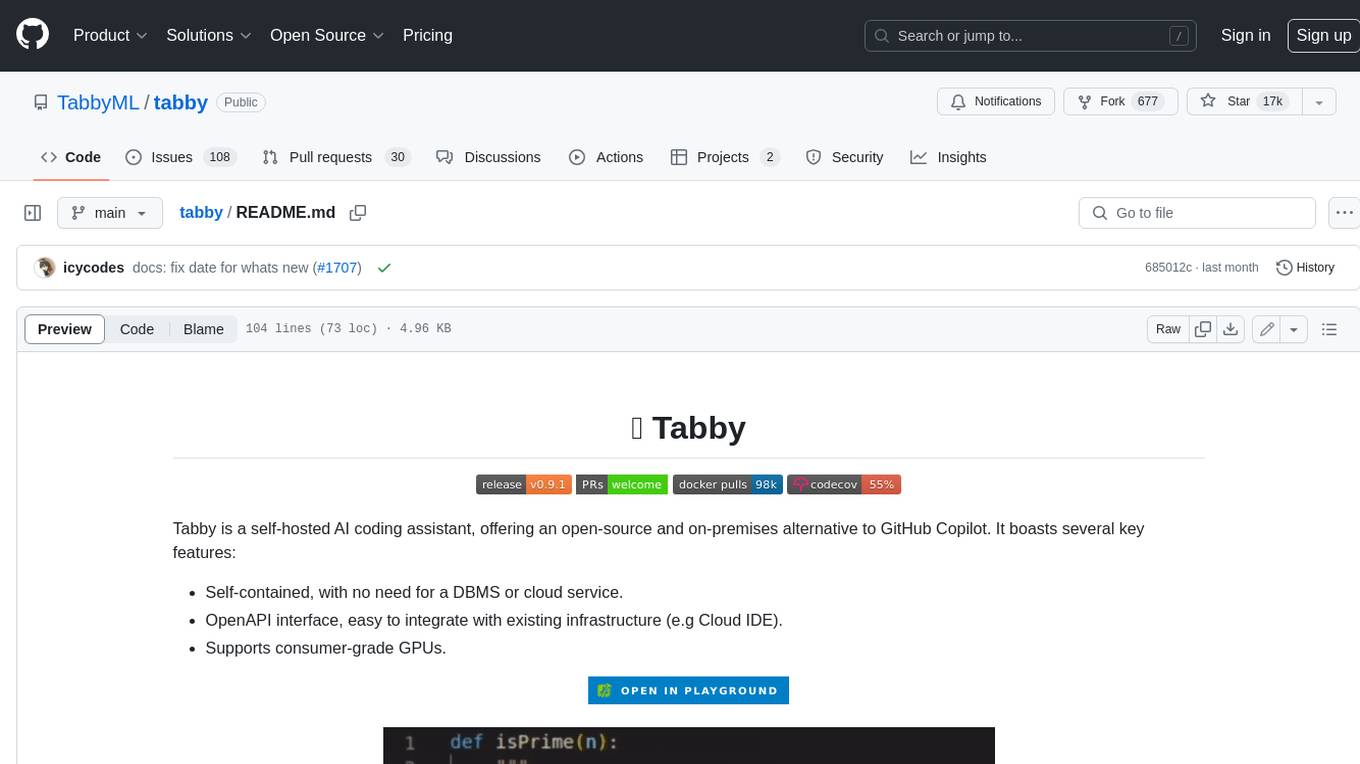
tabby
Tabby is a self-hosted AI coding assistant, offering an open-source and on-premises alternative to GitHub Copilot. It boasts several key features: * Self-contained, with no need for a DBMS or cloud service. * OpenAPI interface, easy to integrate with existing infrastructure (e.g Cloud IDE). * Supports consumer-grade GPUs.
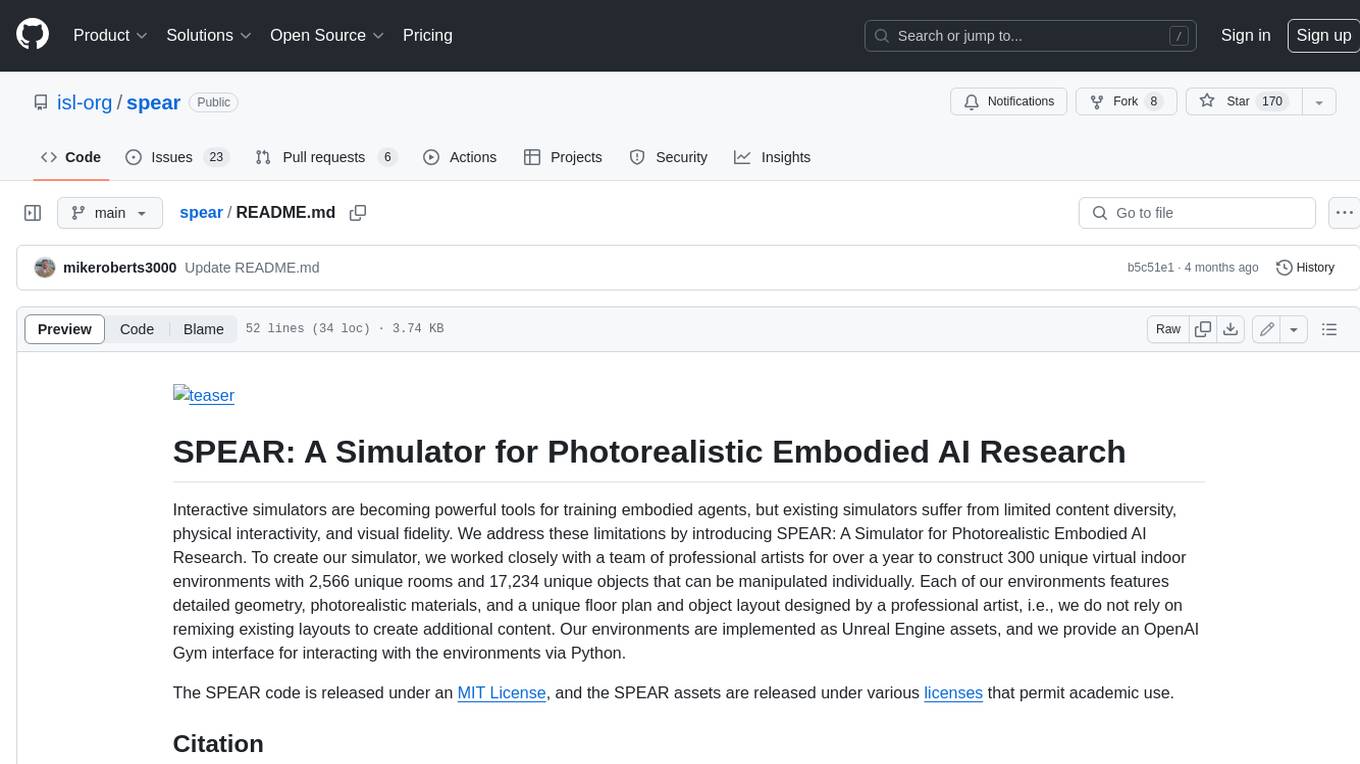
spear
SPEAR (Simulator for Photorealistic Embodied AI Research) is a powerful tool for training embodied agents. It features 300 unique virtual indoor environments with 2,566 unique rooms and 17,234 unique objects that can be manipulated individually. Each environment is designed by a professional artist and features detailed geometry, photorealistic materials, and a unique floor plan and object layout. SPEAR is implemented as Unreal Engine assets and provides an OpenAI Gym interface for interacting with the environments via Python.
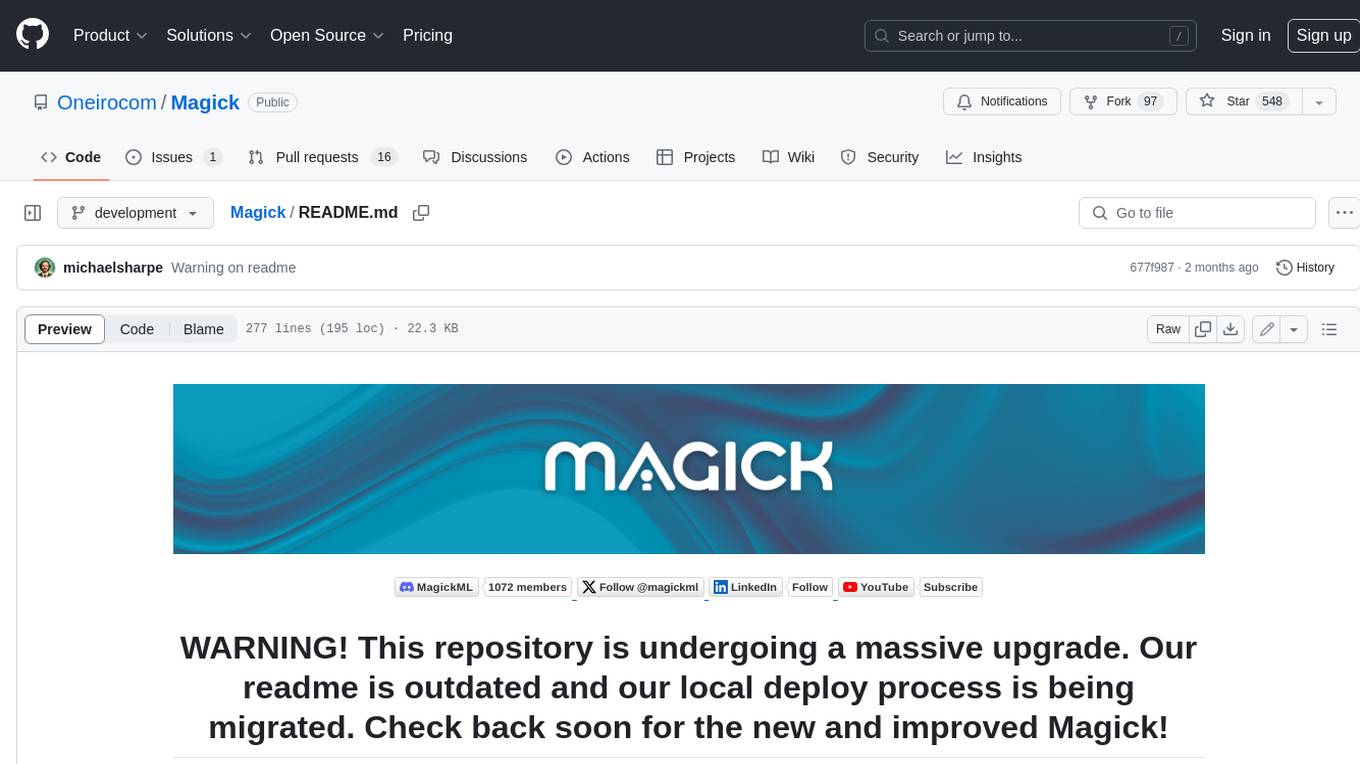
Magick
Magick is a groundbreaking visual AIDE (Artificial Intelligence Development Environment) for no-code data pipelines and multimodal agents. Magick can connect to other services and comes with nodes and templates well-suited for intelligent agents, chatbots, complex reasoning systems and realistic characters.