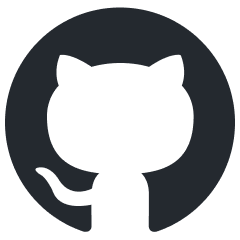
greenmask
PostgreSQL database anonymization and synthetic data generation tool
Stars: 1241
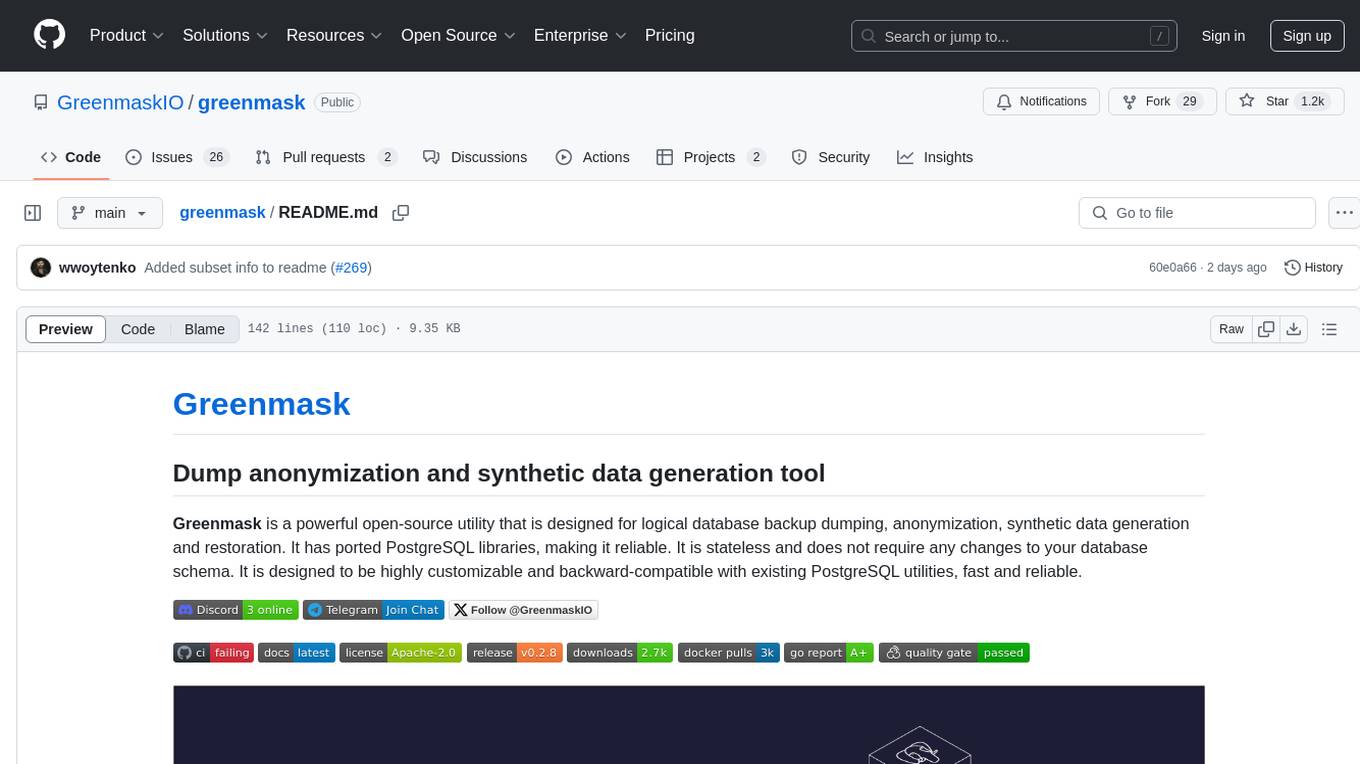
Greenmask is a powerful open-source utility designed for logical database backup dumping, anonymization, synthetic data generation, and restoration. It is highly customizable, stateless, and backward-compatible with existing PostgreSQL utilities. Greenmask supports advanced subset systems, deterministic transformers, dynamic parameters, transformation conditions, and more. It is cross-platform, database type safe, extensible, and supports parallel execution and various storage options. Ideal for backup and restoration tasks, anonymization, transformation, and data masking.
README:
Greenmask is a powerful open-source utility that is designed for logical database backup dumping, anonymization, synthetic data generation and restoration. It has ported PostgreSQL libraries, making it reliable. It is stateless and does not require any changes to your database schema. It is designed to be highly customizable and backward-compatible with existing PostgreSQL utilities, fast and reliable.
Greenmask has a Playground - it is a sandbox environment in Docker with sample databases included to help you try Greenmask without any additional actions
-
Clone the
greenmask
repository and navigate to its directory by running the following commands:git clone [email protected]:GreenmaskIO/greenmask.git && cd greenmask
-
Once you have cloned the repository, start the environment by running Docker Compose:
docker-compose run greenmask
- Database subset - One of the most advanced subset systems on the market. It supports virtual references, nullable columns, polymorphic references, and can subset even the most complex schemas with cyclic references.
-
Deterministic transformers — Uses hash functions to ensure consistent output for the same input. Most transformers support both
random
andhash
engines, offering flexibility for various use cases. - Dynamic parameters — most transformers support dynamic parameters, allowing them to adapt based on table column values. This feature helps manage dependencies between columns and meet constraints effectively.
- Transformation Condition — applies the transformation only when a specified condition is met, making it useful for targeting specific rows.
- Transformation validation and easy maintenance — Greenmask provides validation warnings, data transformation diffs, and schema diffs during configuration, enabling effective monitoring and maintenance of transformations. The schema diff feature helps prevent data leakage when the schema changes.
- Transformation inheritance — transformation inheritance for partitioned tables and tables with foreign keys. Define once and apply to all.
- Stateless — Greenmask operates as a logical dump and does not impact your existing database schema.
- Cross-platform — Can be easily built and executed on any platform, thanks to its Go-based architecture, which eliminates platform dependencies.
- Database type safe — Ensures data integrity by validating data and using the database driver for encoding and decoding operations, preserving accurate data formats.
-
Backward compatible — Fully supports the same features and protocols as standard PostgreSQL utilities. Dumps
created by Greenmask can be seamlessly restored using the
pg_restore
utility. - Extensible — Users have the flexibility to implement domain-based transformations in any programming language or use predefined templates.
- Parallel execution — Enables parallel dumping and restoration to significantly speed up results.
- Variety of storages — Supports both local and remote storage, including directories and S3-compatible solutions.
-
Pgzip support for faster compression — Speeds up dump and restoration processes with parallel compression
by setting
--pgzip
.
Greenmask is ideal for various scenarios, including:
- Backup and Restoration. Use Greenmask for your daily routines involving logical backup dumping and restoration. It seamlessly handles tasks like table restoration after truncation. Its functionality closely mirrors that of pg_dump and pg_restore, making it a straightforward replacement.
- Anonymization, Transformation, and Data Masking. Employ Greenmask for anonymizing, transforming, and masking backups, especially when setting up a staging environment or for analytical purposes. It simplifies the deployment of a pre-production environment with consistently anonymized data, facilitating faster time-to-market in the development lifecycle.
The best approach for logical backup dumping and restoration is to use core PostgreSQL utilities, specifically pg_dump
and pg_restore. Greenmask is designed to align with these native tools, ensuring full compatibility. It independently
manages data dumping while delegating schema dumping and restoration to pg_dump
and pg_restore
, ensuring smooth
integration with PostgreSQL’s standard workflow.
Greenmask utilizes the directory format of pg_dump
and pg_restore
, ideal for parallel execution and partial restoration.
This format includes metadata files to guide backup and restoration steps.
- s3 - Supports any S3-compatible storage system, including AWS S3, offering flexibility across different cloud storage solutions.
- directory - This is the default option, representing a standard filesystem directory for local storage.
Greenmask works with COPY lines, collects schema metadata using the Golang driver, and employs this driver in the encoding and decoding process. The validate command offers a way to assess the impact on both schema (validation warnings) and data (transformation and displaying differences). This command allows you to validate the schema and data transformations, ensuring the desired outcomes during the Anonymization process.
If your table schema relies on functional dependencies between columns, you can address this challenge using the Dynamic parameters. By setting dynamic parameters, you can resolve such as created_at and updated_at cases, where the updated_at must be greater or equal than the created_at.
If you need to implement custom logic imperatively use Cmd or TemplateRecord or Template transformers.
Greenmask is compatible with PostgreSQL versions 11 and higher.
- Utilized the Demo database, provided by PostgresPro, for integration testing purposes.
- Employed the adventureworks database created
by
morenoh149/postgresDBSamples
, in the Docker Compose playground.
For Tasks:
Click tags to check more tools for each tasksFor Jobs:
Alternative AI tools for greenmask
Similar Open Source Tools
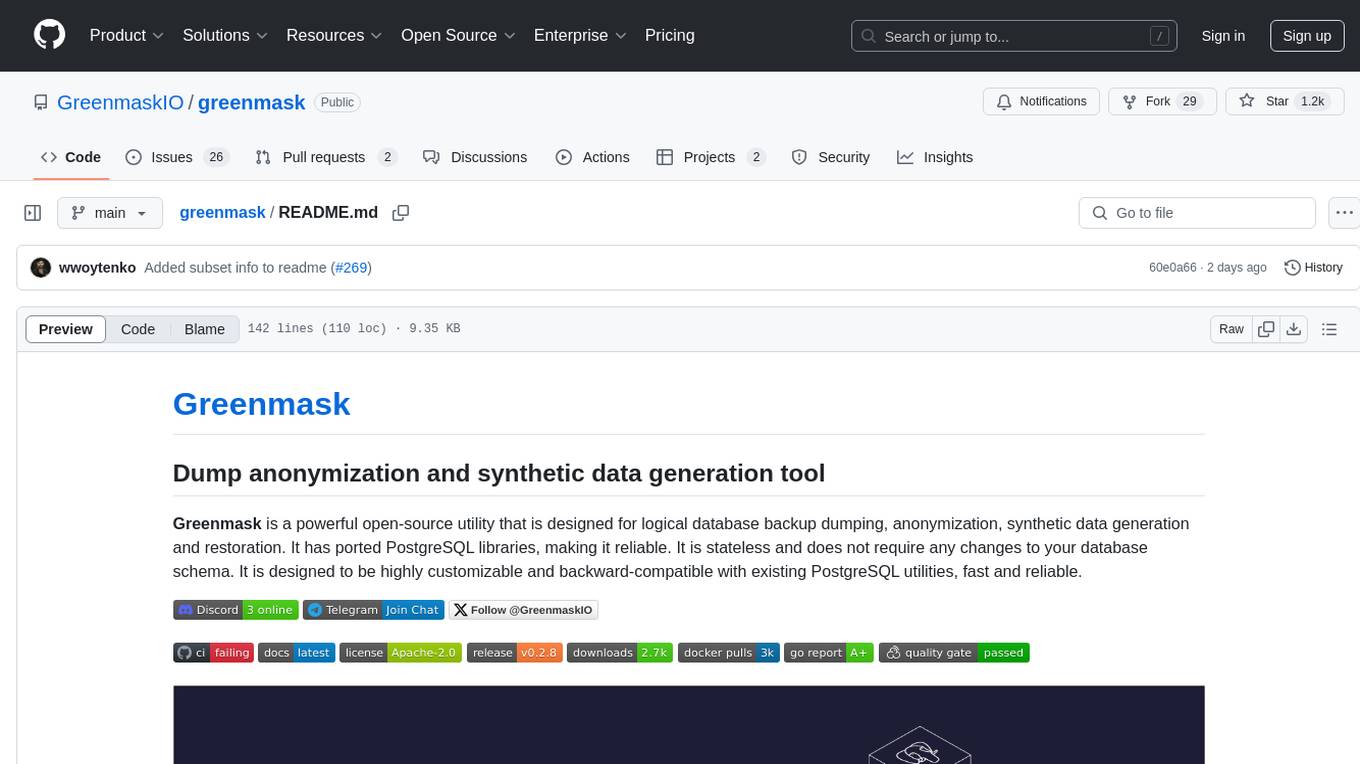
greenmask
Greenmask is a powerful open-source utility designed for logical database backup dumping, anonymization, synthetic data generation, and restoration. It is highly customizable, stateless, and backward-compatible with existing PostgreSQL utilities. Greenmask supports advanced subset systems, deterministic transformers, dynamic parameters, transformation conditions, and more. It is cross-platform, database type safe, extensible, and supports parallel execution and various storage options. Ideal for backup and restoration tasks, anonymization, transformation, and data masking.
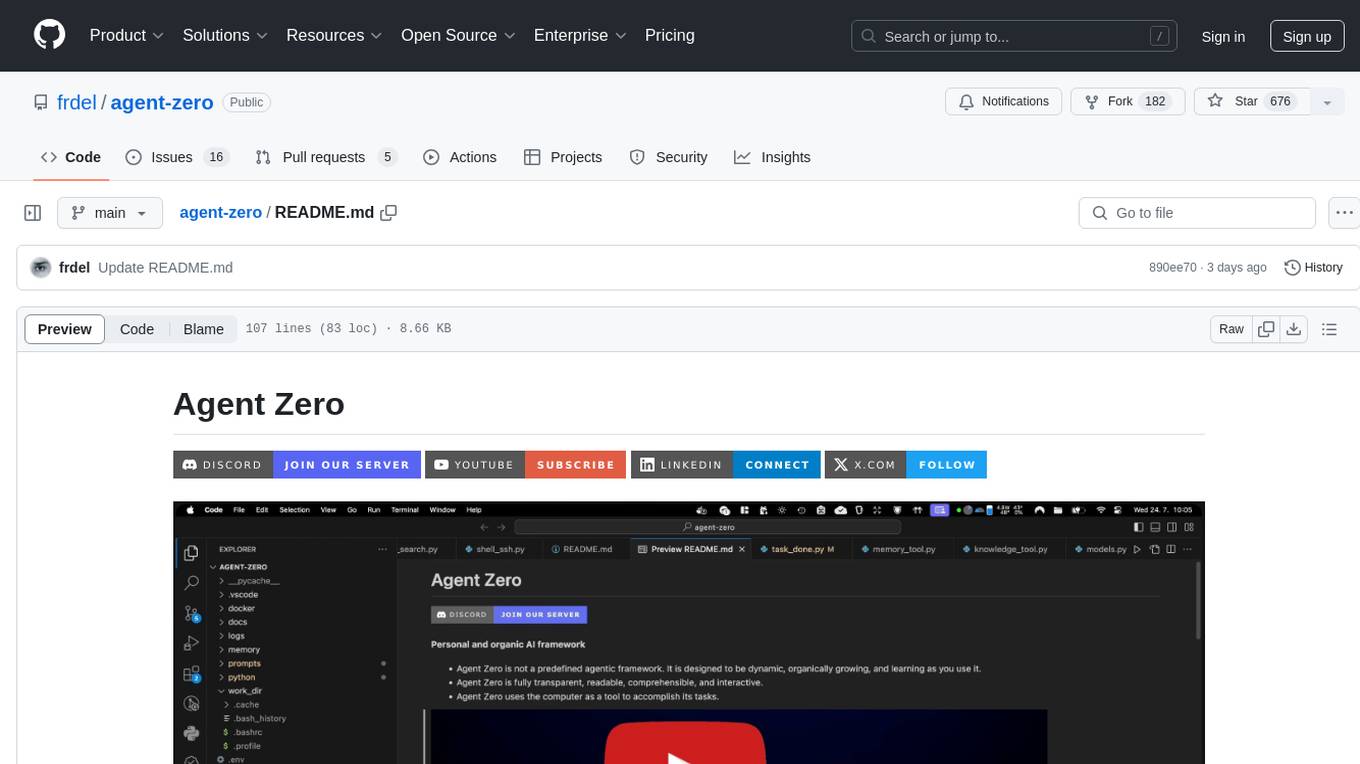
agent-zero
Agent Zero is a personal and organic AI framework designed to be dynamic, organically growing, and learning as you use it. It is fully transparent, readable, comprehensible, customizable, and interactive. The framework uses the computer as a tool to accomplish tasks, with no single-purpose tools pre-programmed. It emphasizes multi-agent cooperation, complete customization, and extensibility. Communication is key in this framework, allowing users to give proper system prompts and instructions to achieve desired outcomes. Agent Zero is capable of dangerous actions and should be run in an isolated environment. The framework is prompt-based, highly customizable, and requires a specific environment to run effectively.
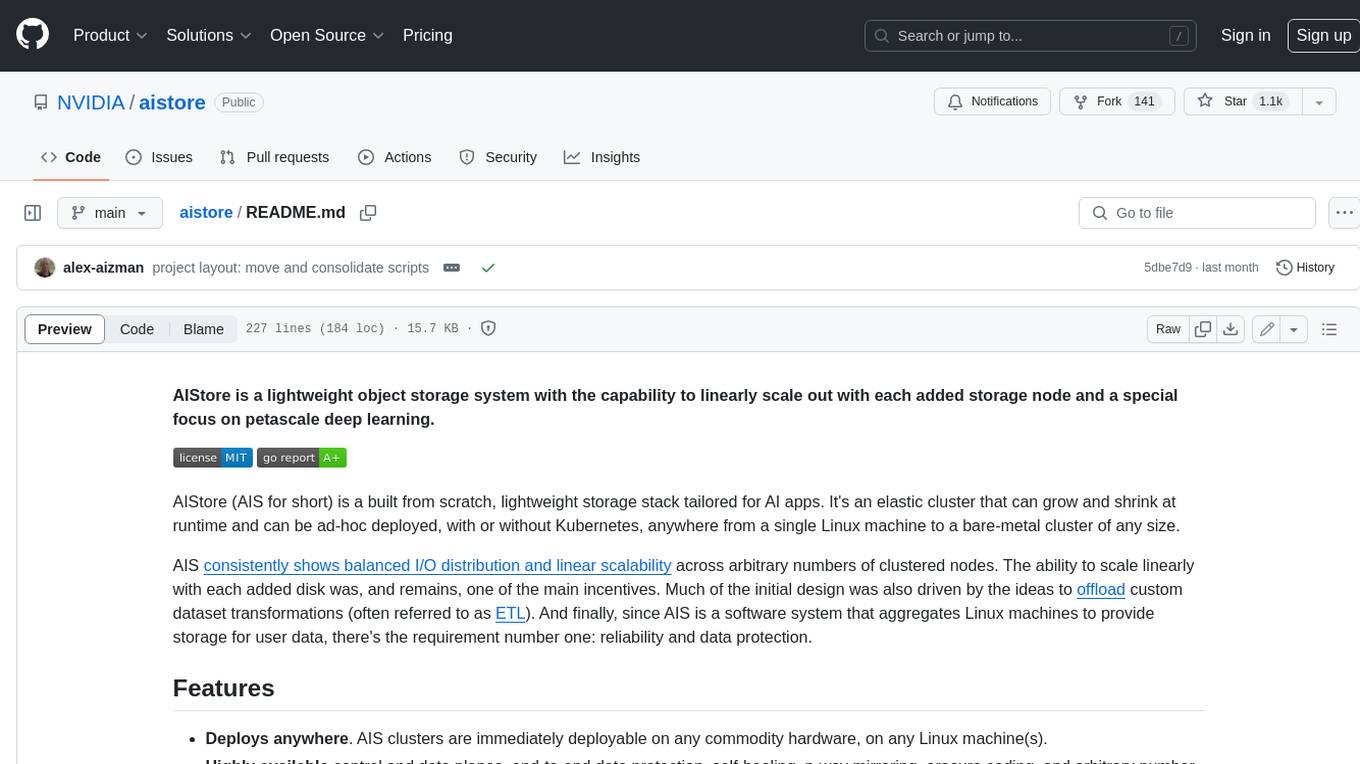
aistore
AIStore is a lightweight object storage system designed for AI applications. It is highly scalable, reliable, and easy to use. AIStore can be deployed on any commodity hardware, and it can be used to store and manage large datasets for deep learning and other AI applications.
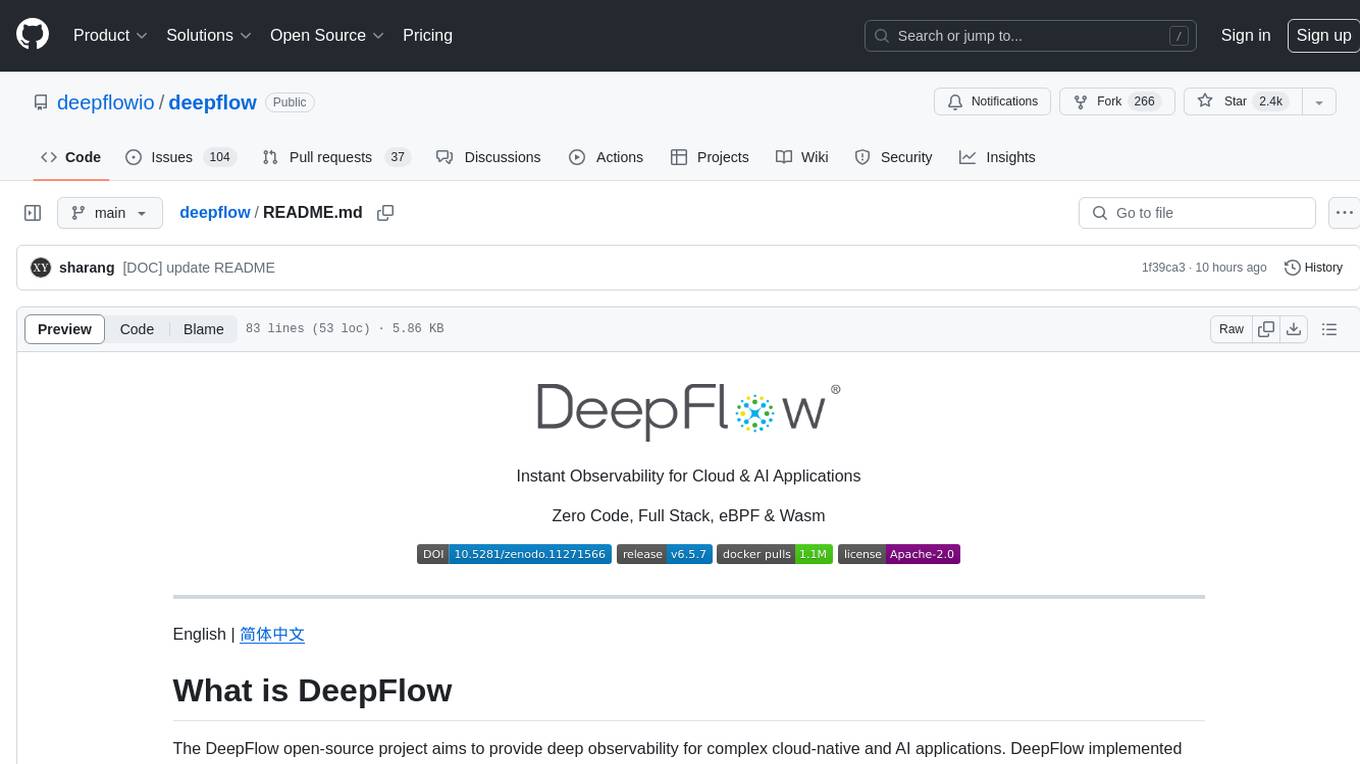
deepflow
DeepFlow is an open-source project that provides deep observability for complex cloud-native and AI applications. It offers Zero Code data collection with eBPF for metrics, distributed tracing, request logs, and function profiling. DeepFlow is integrated with SmartEncoding to achieve Full Stack correlation and efficient access to all observability data. With DeepFlow, cloud-native and AI applications automatically gain deep observability, removing the burden of developers continually instrumenting code and providing monitoring and diagnostic capabilities covering everything from code to infrastructure for DevOps/SRE teams.
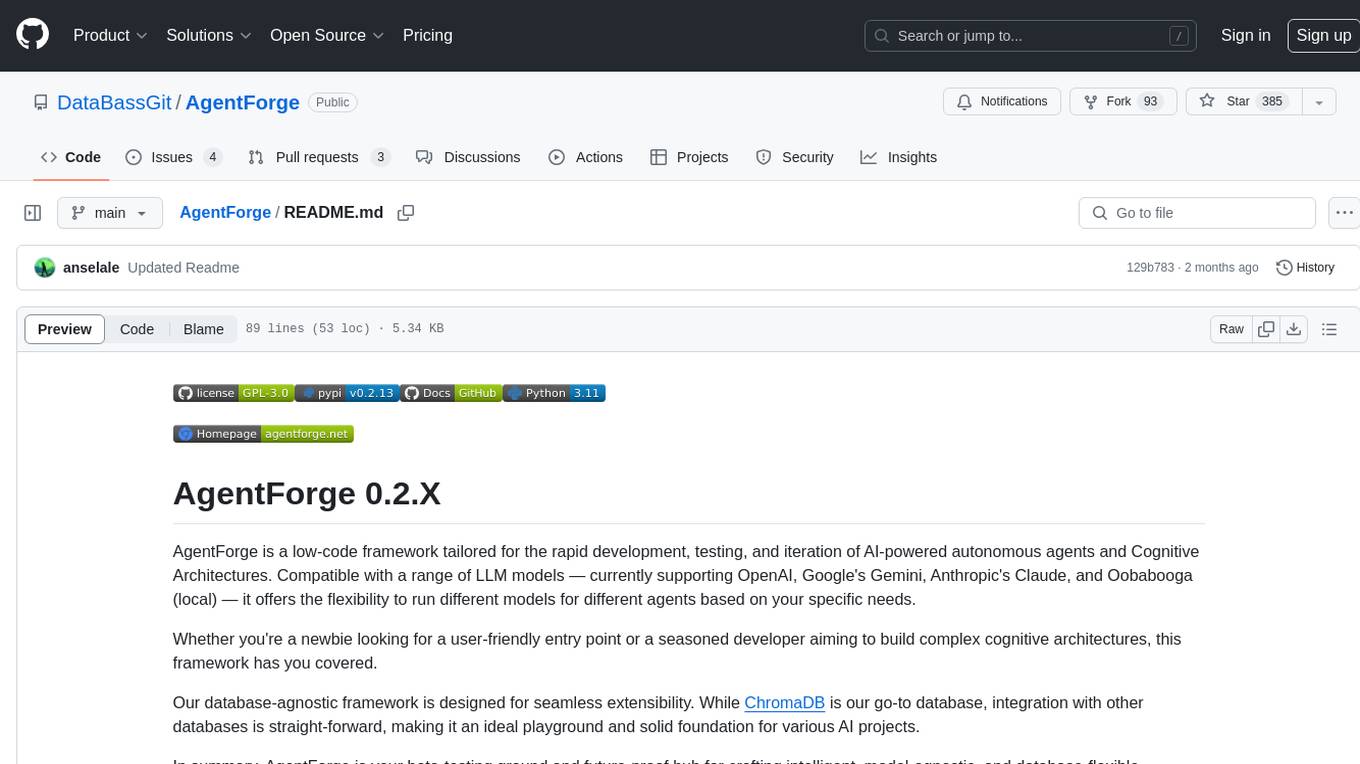
AgentForge
AgentForge is a low-code framework tailored for the rapid development, testing, and iteration of AI-powered autonomous agents and Cognitive Architectures. It is compatible with a range of LLM models and offers flexibility to run different models for different agents based on specific needs. The framework is designed for seamless extensibility and database-flexibility, making it an ideal playground for various AI projects. AgentForge is a beta-testing ground and future-proof hub for crafting intelligent, model-agnostic autonomous agents.
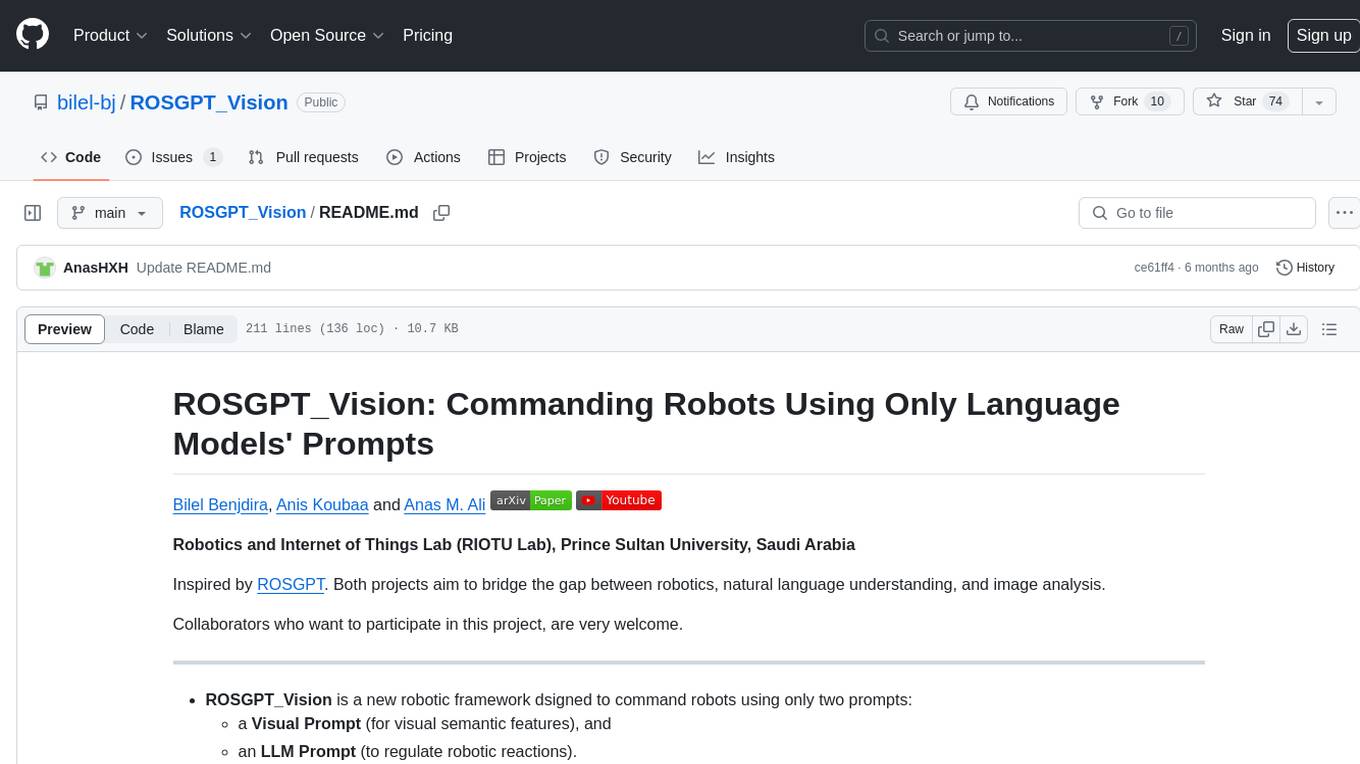
ROSGPT_Vision
ROSGPT_Vision is a new robotic framework designed to command robots using only two prompts: a Visual Prompt for visual semantic features and an LLM Prompt to regulate robotic reactions. It is based on the Prompting Robotic Modalities (PRM) design pattern and is used to develop CarMate, a robotic application for monitoring driver distractions and providing real-time vocal notifications. The framework leverages state-of-the-art language models to facilitate advanced reasoning about image data and offers a unified platform for robots to perceive, interpret, and interact with visual data through natural language. LangChain is used for easy customization of prompts, and the implementation includes the CarMate application for driver monitoring and assistance.
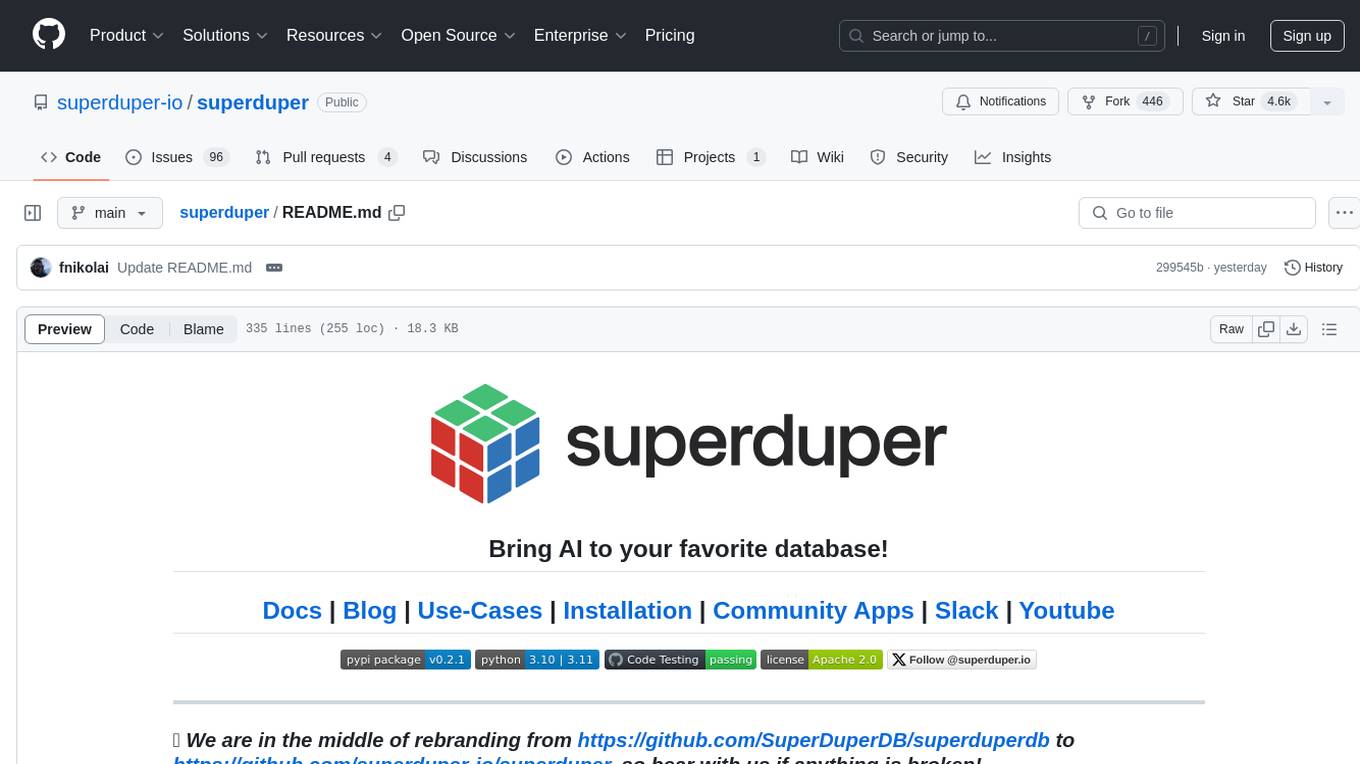
superduper
superduper.io is a Python framework that integrates AI models, APIs, and vector search engines directly with existing databases. It allows hosting of models, streaming inference, and scalable model training/fine-tuning. Key features include integration of AI with data infrastructure, inference via change-data-capture, scalable model training, model chaining, simple Python interface, Python-first approach, working with difficult data types, feature storing, and vector search capabilities. The tool enables users to turn their existing databases into centralized repositories for managing AI model inputs and outputs, as well as conducting vector searches without the need for specialized databases.
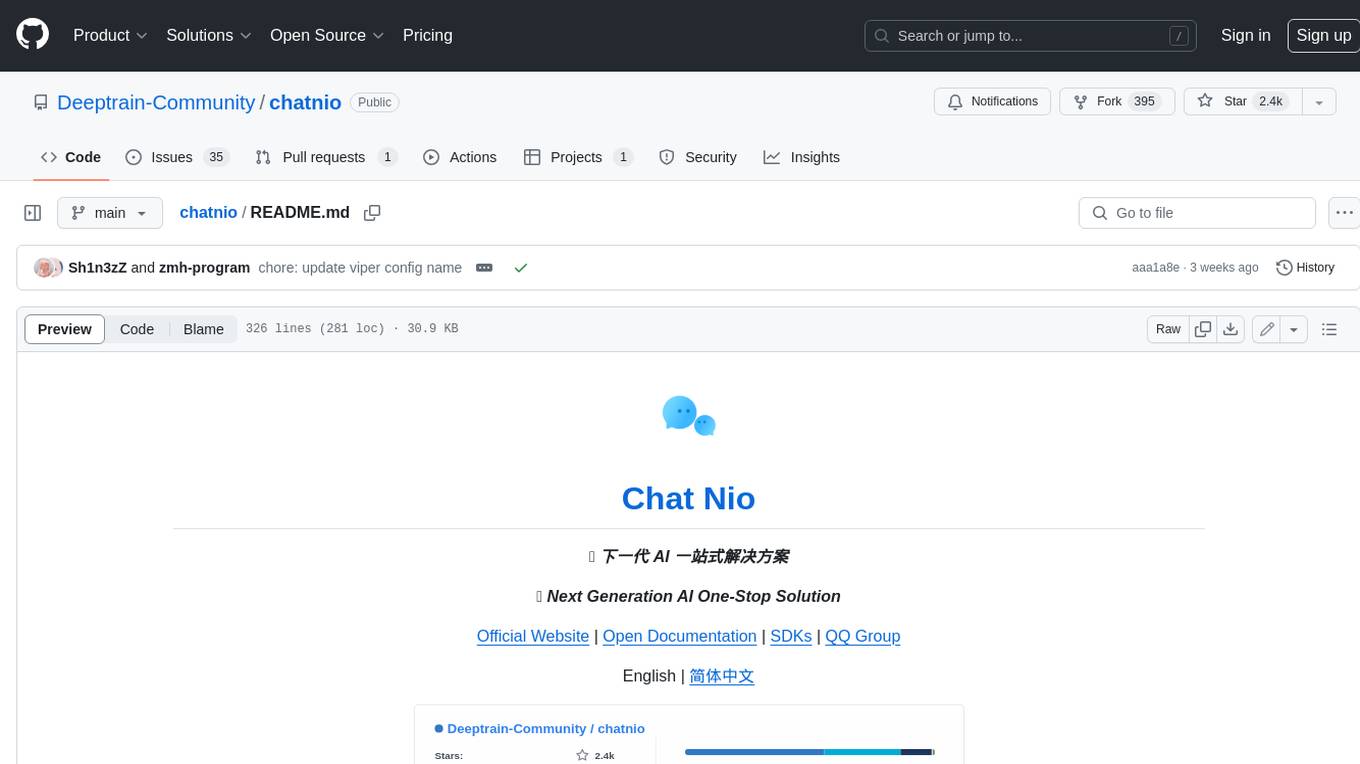
chatnio
Chat Nio is a next-generation AI one-stop solution that provides a rich and user-friendly interface for interacting with various AI models. It offers features such as AI chat conversation, rich format compatibility, markdown support, message menu support, multi-platform adaptation, dialogue memory, full-model file parsing, full-model DuckDuckGo online search, full-screen large text editing, model marketplace, preset support, site announcements, preference settings, internationalization support, and a rich admin system. Chat Nio also boasts a powerful channel management system that utilizes a self-developed channel distribution algorithm, supports multi-channel management, is compatible with multiple formats, allows for custom models, supports channel retries, enables balanced load within the same channel, and provides channel model mapping and user grouping. Additionally, Chat Nio offers forwarding API services that are compatible with multiple formats in the OpenAI universal format and support multiple model compatible layers. It also provides a custom build and install option for highly customizable deployments. Chat Nio is an open-source project licensed under the Apache License 2.0 and welcomes contributions from the community.
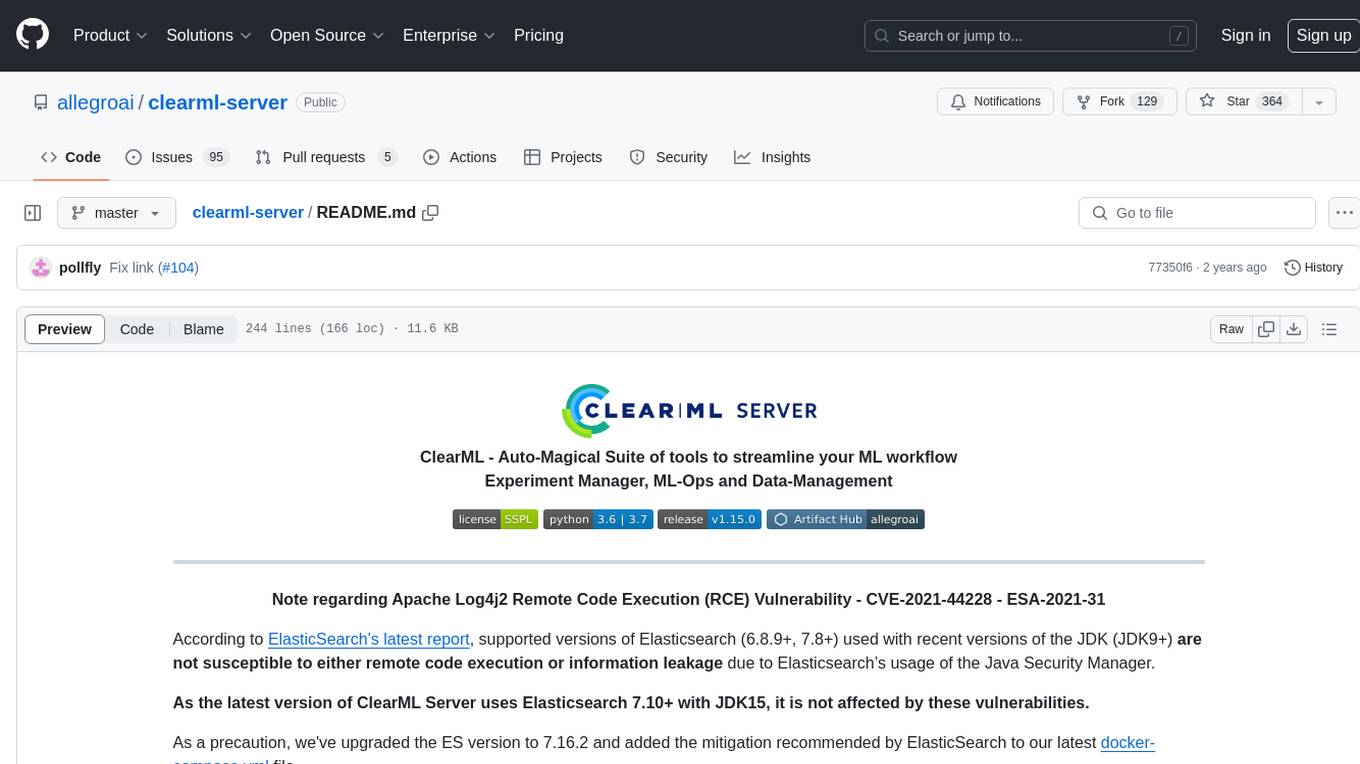
clearml-server
ClearML Server is a backend service infrastructure for ClearML, facilitating collaboration and experiment management. It includes a web app, RESTful API, and file server for storing images and models. Users can deploy ClearML Server using Docker, AWS EC2 AMI, or Kubernetes. The system design supports single IP or sub-domain configurations with specific open ports. ClearML-Agent Services container allows launching long-lasting jobs and various use cases like auto-scaler service, controllers, optimizer, and applications. Advanced functionality includes web login authentication and non-responsive experiments watchdog. Upgrading ClearML Server involves stopping containers, backing up data, downloading the latest docker-compose.yml file, configuring ClearML-Agent Services, and spinning up docker containers. Community support is available through ClearML FAQ, Stack Overflow, GitHub issues, and email contact.
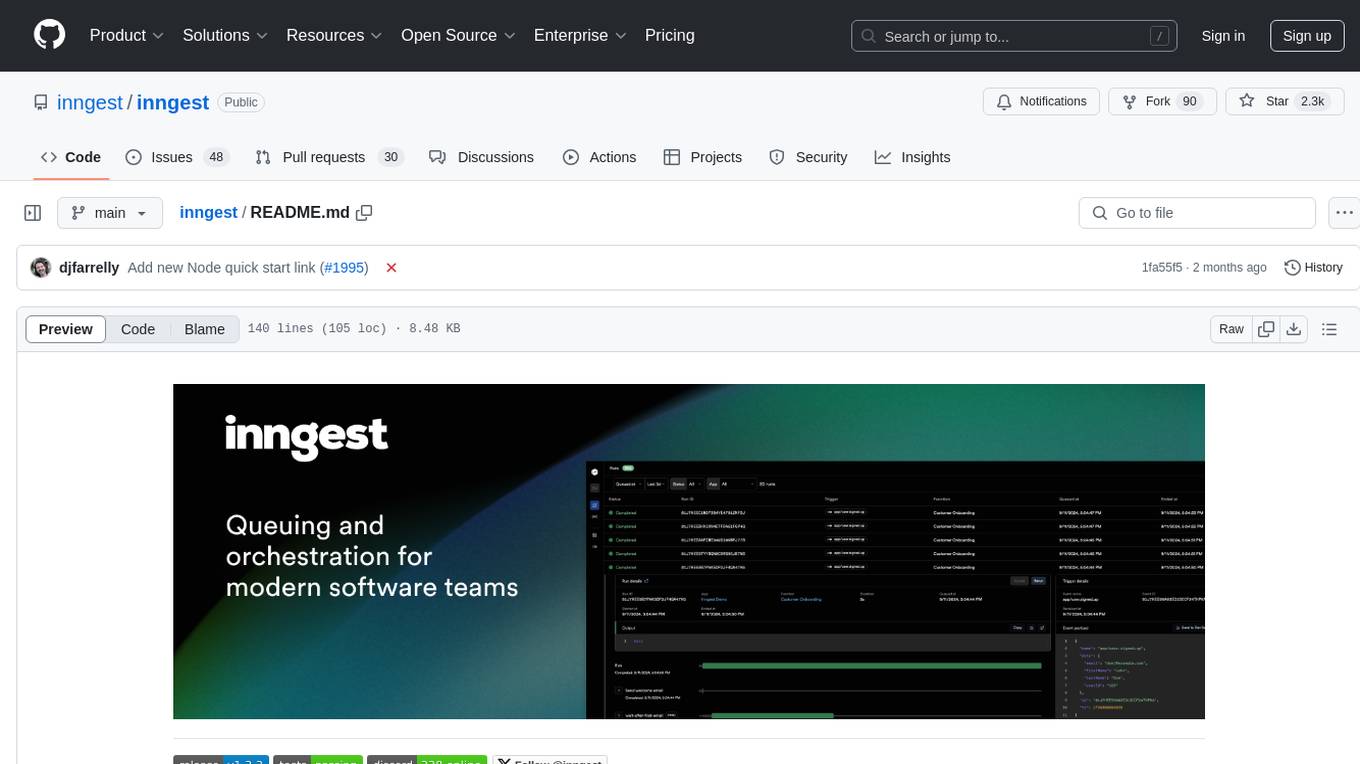
inngest
Inngest is a platform that offers durable functions to replace queues, state management, and scheduling for developers. It allows writing reliable step functions faster without dealing with infrastructure. Developers can create durable functions using various language SDKs, run a local development server, deploy functions to their infrastructure, sync functions with the Inngest Platform, and securely trigger functions via HTTPS. Inngest Functions support retrying, scheduling, and coordinating operations through triggers, flow control, and steps, enabling developers to build reliable workflows with robust support for various operations.
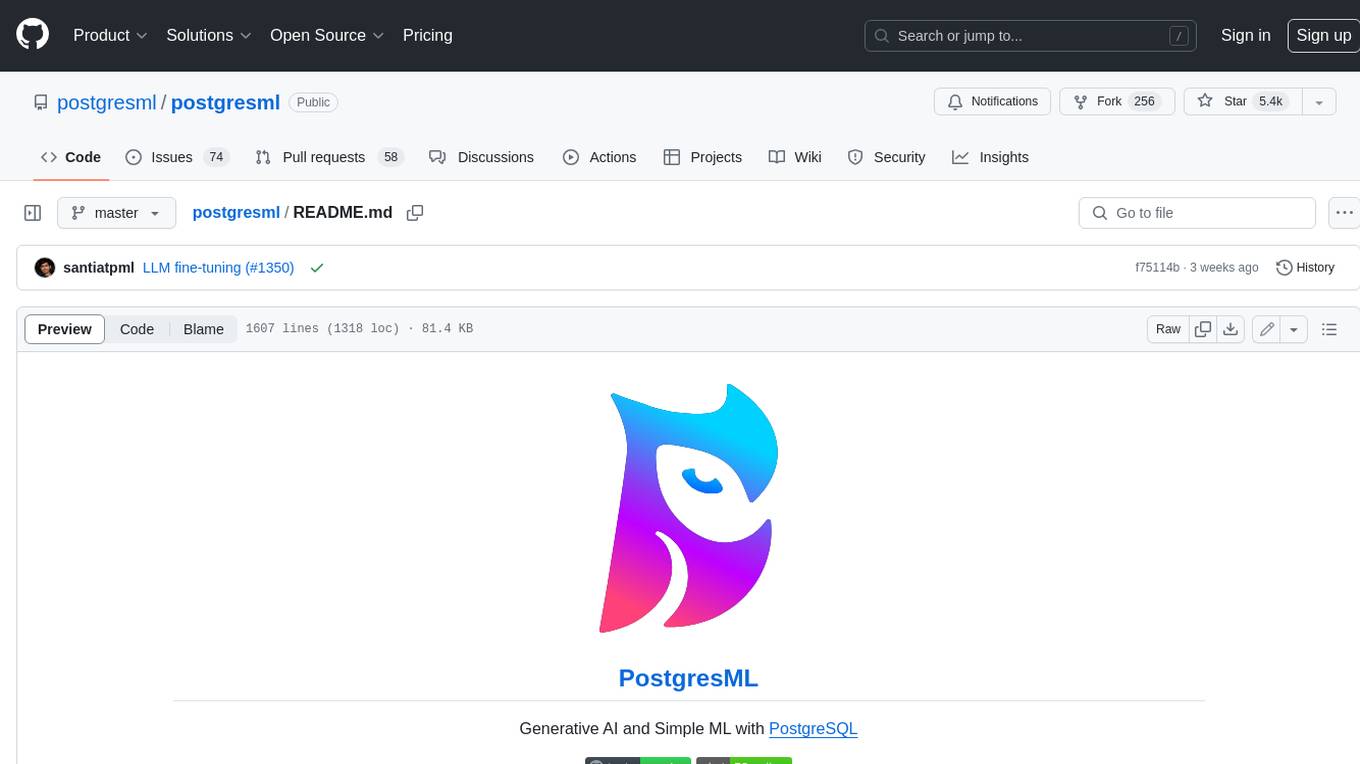
postgresml
PostgresML is a powerful Postgres extension that seamlessly combines data storage and machine learning inference within your database. It enables running machine learning and AI operations directly within PostgreSQL, leveraging GPU acceleration for faster computations, integrating state-of-the-art large language models, providing built-in functions for text processing, enabling efficient similarity search, offering diverse ML algorithms, ensuring high performance, scalability, and security, supporting a wide range of NLP tasks, and seamlessly integrating with existing PostgreSQL tools and client libraries.
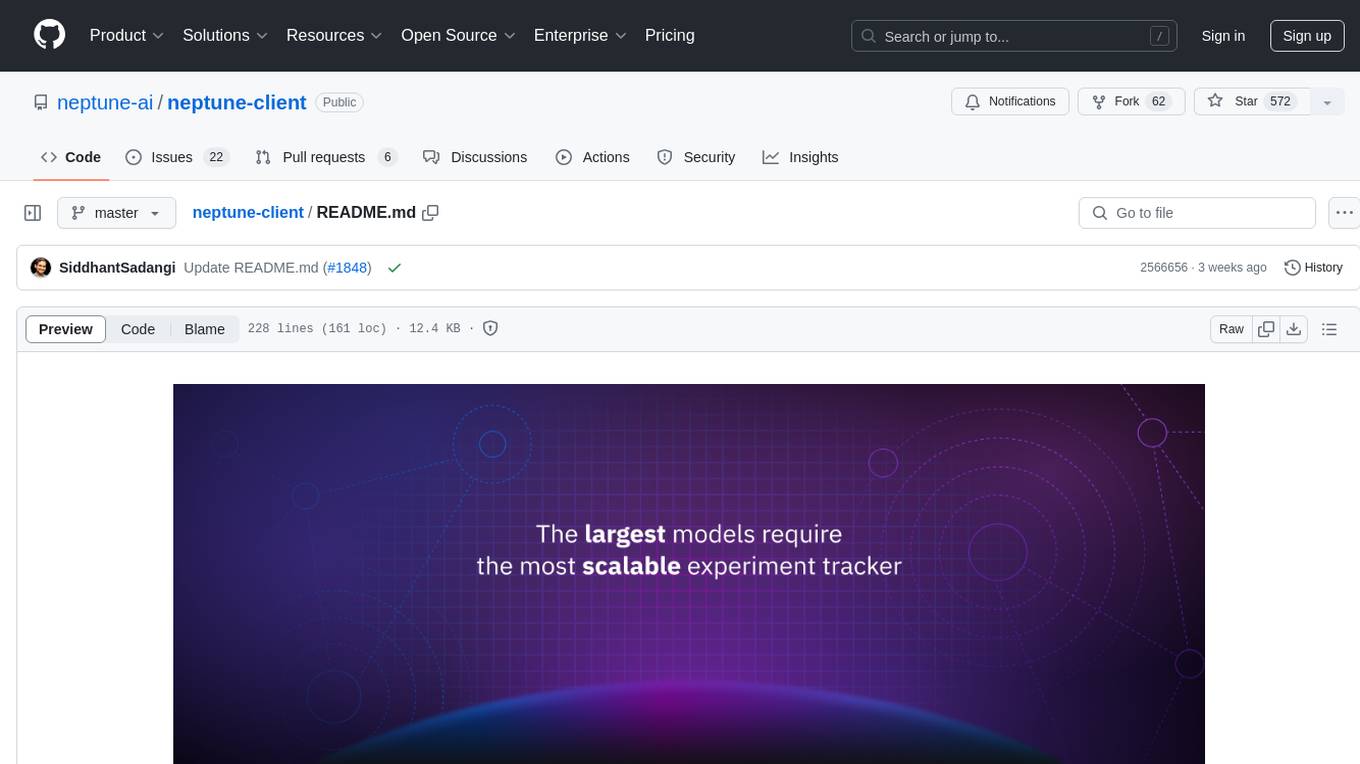
neptune-client
Neptune is a scalable experiment tracker for teams training foundation models. Log millions of runs, effortlessly monitor and visualize model training, and deploy on your infrastructure. Track 100% of metadata to accelerate AI breakthroughs. Log and display any framework and metadata type from any ML pipeline. Organize experiments with nested structures and custom dashboards. Compare results, visualize training, and optimize models quicker. Version models, review stages, and access production-ready models. Share results, manage users, and projects. Integrate with 25+ frameworks. Trusted by great companies to improve workflow.
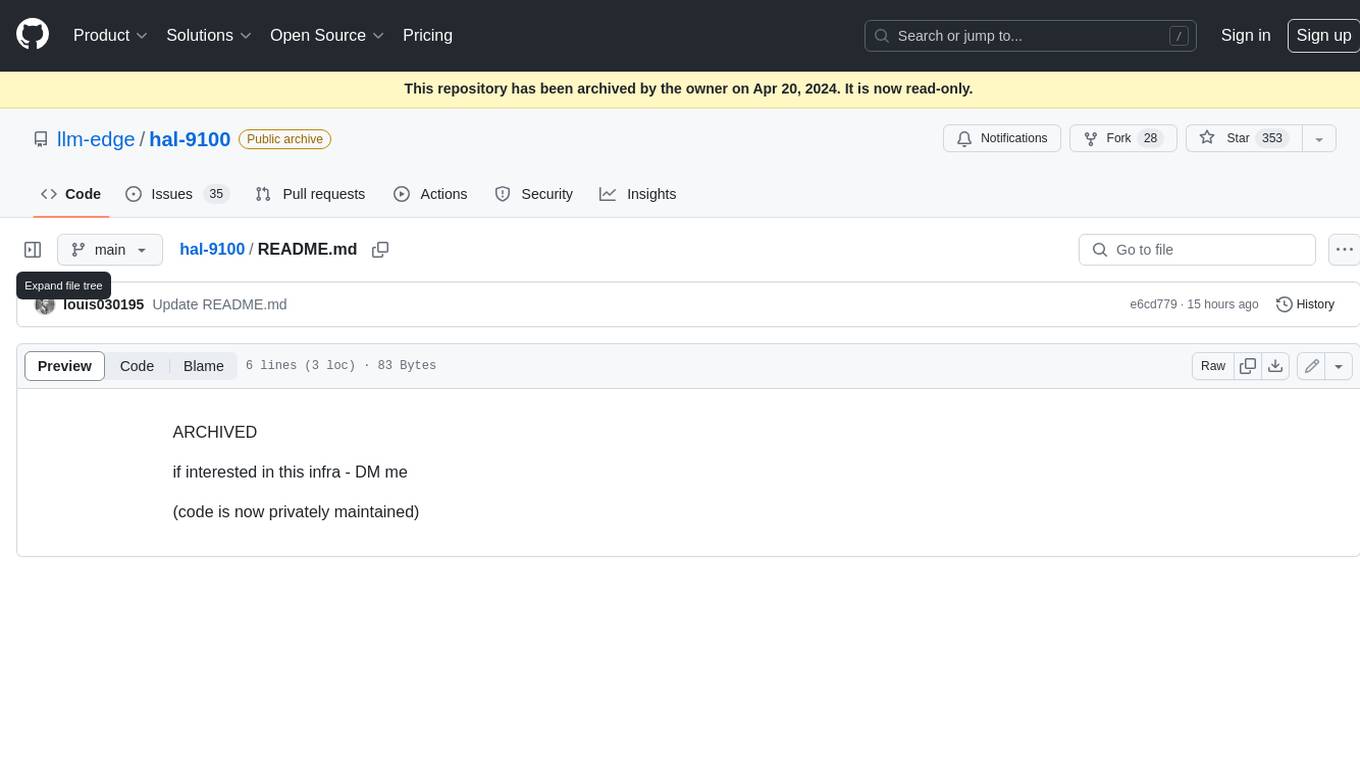
hal-9100
This repository is now archived and the code is privately maintained. If you are interested in this infrastructure, please contact the maintainer directly.
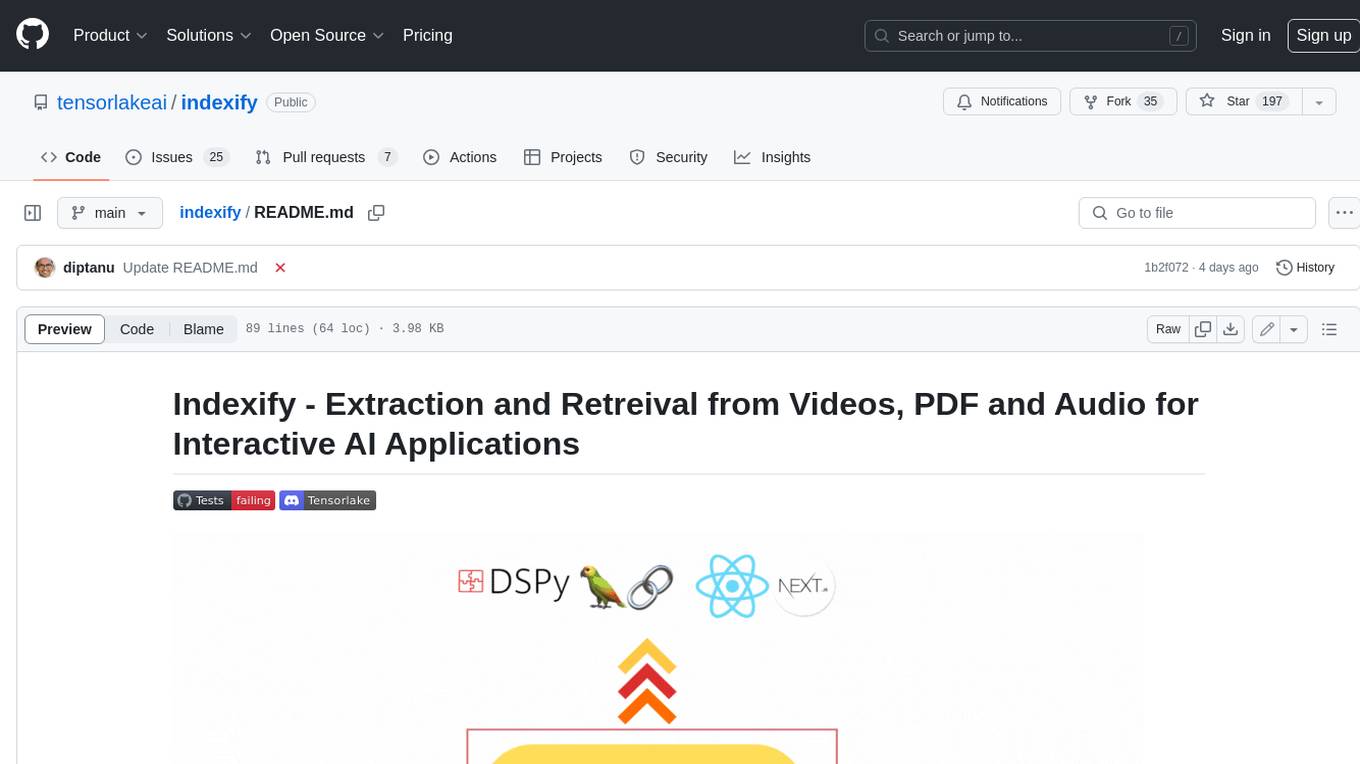
indexify
Indexify is an open-source engine for building fast data pipelines for unstructured data (video, audio, images, and documents) using reusable extractors for embedding, transformation, and feature extraction. LLM Applications can query transformed content friendly to LLMs by semantic search and SQL queries. Indexify keeps vector databases and structured databases (PostgreSQL) updated by automatically invoking the pipelines as new data is ingested into the system from external data sources. **Why use Indexify** * Makes Unstructured Data **Queryable** with **SQL** and **Semantic Search** * **Real-Time** Extraction Engine to keep indexes **automatically** updated as new data is ingested. * Create **Extraction Graph** to describe **data transformation** and extraction of **embedding** and **structured extraction**. * **Incremental Extraction** and **Selective Deletion** when content is deleted or updated. * **Extractor SDK** allows adding new extraction capabilities, and many readily available extractors for **PDF**, **Image**, and **Video** indexing and extraction. * Works with **any LLM Framework** including **Langchain**, **DSPy**, etc. * Runs on your laptop during **prototyping** and also scales to **1000s of machines** on the cloud. * Works with many **Blob Stores**, **Vector Stores**, and **Structured Databases** * We have even **Open Sourced Automation** to deploy to Kubernetes in production.
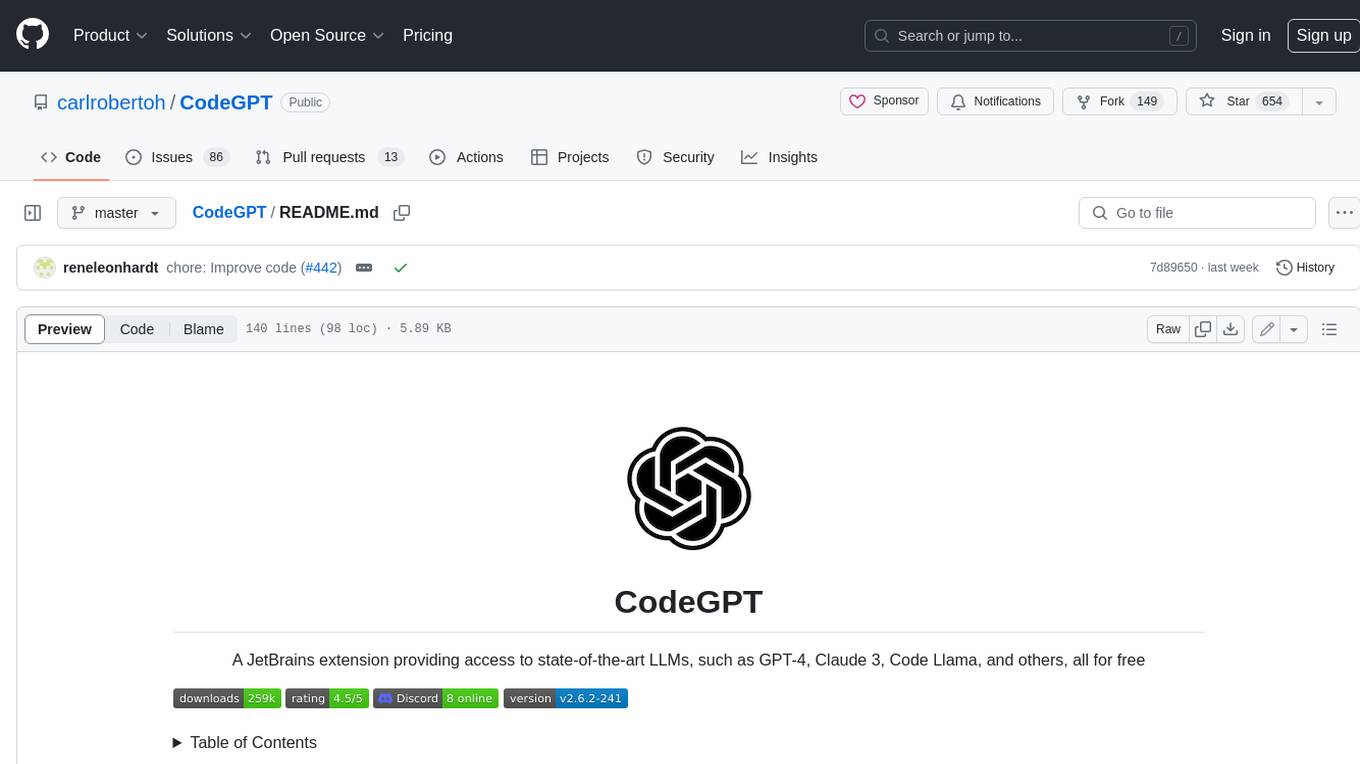
CodeGPT
CodeGPT is an extension for JetBrains IDEs that provides access to state-of-the-art large language models (LLMs) for coding assistance. It offers a range of features to enhance the coding experience, including code completions, a ChatGPT-like interface for instant coding advice, commit message generation, reference file support, name suggestions, and offline development support. CodeGPT is designed to keep privacy in mind, ensuring that user data remains secure and private.
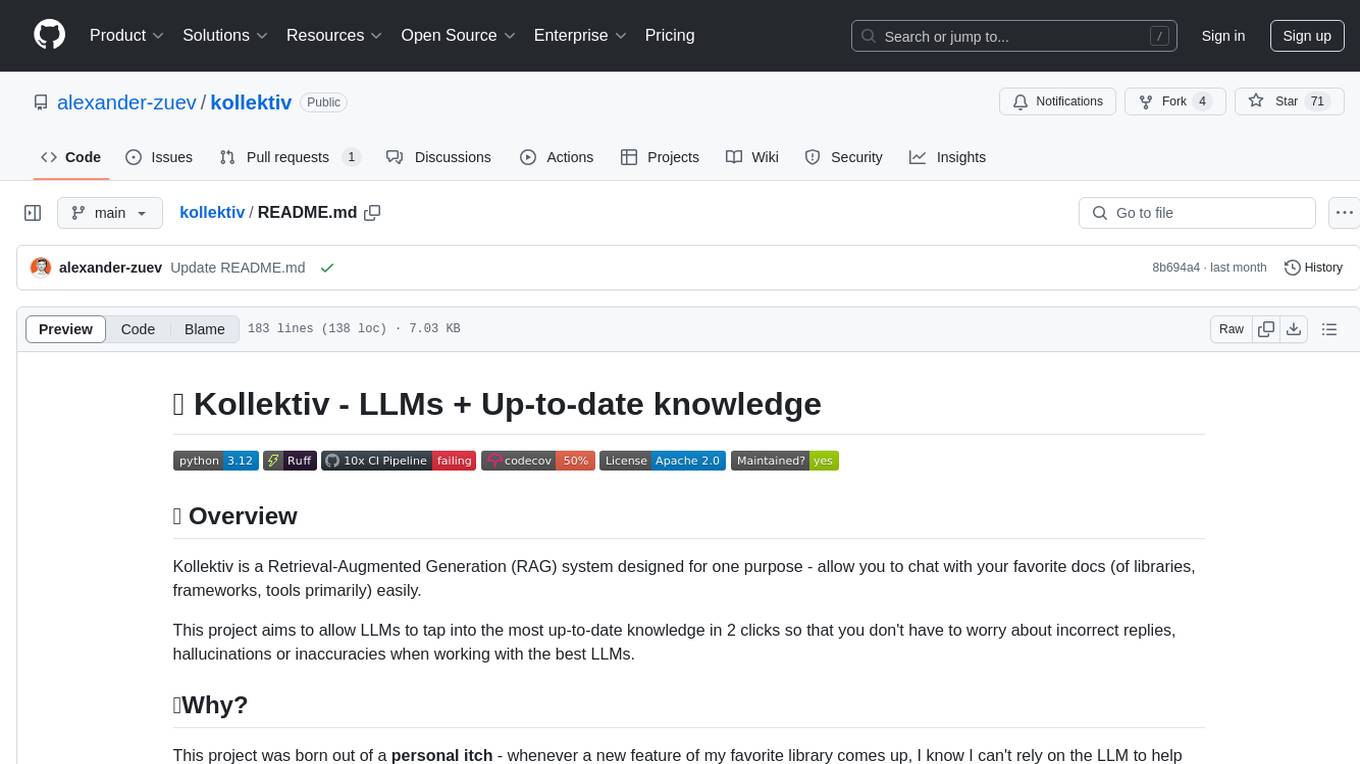
kollektiv
Kollektiv is a Retrieval-Augmented Generation (RAG) system designed to enable users to chat with their favorite documentation easily. It aims to provide LLMs with access to the most up-to-date knowledge, reducing inaccuracies and improving productivity. The system utilizes intelligent web crawling, advanced document processing, vector search, multi-query expansion, smart re-ranking, AI-powered responses, and dynamic system prompts. The technical stack includes Python/FastAPI for backend, Supabase, ChromaDB, and Redis for storage, OpenAI and Anthropic Claude 3.5 Sonnet for AI/ML, and Chainlit for UI. Kollektiv is licensed under a modified version of the Apache License 2.0, allowing free use for non-commercial purposes.
For similar tasks
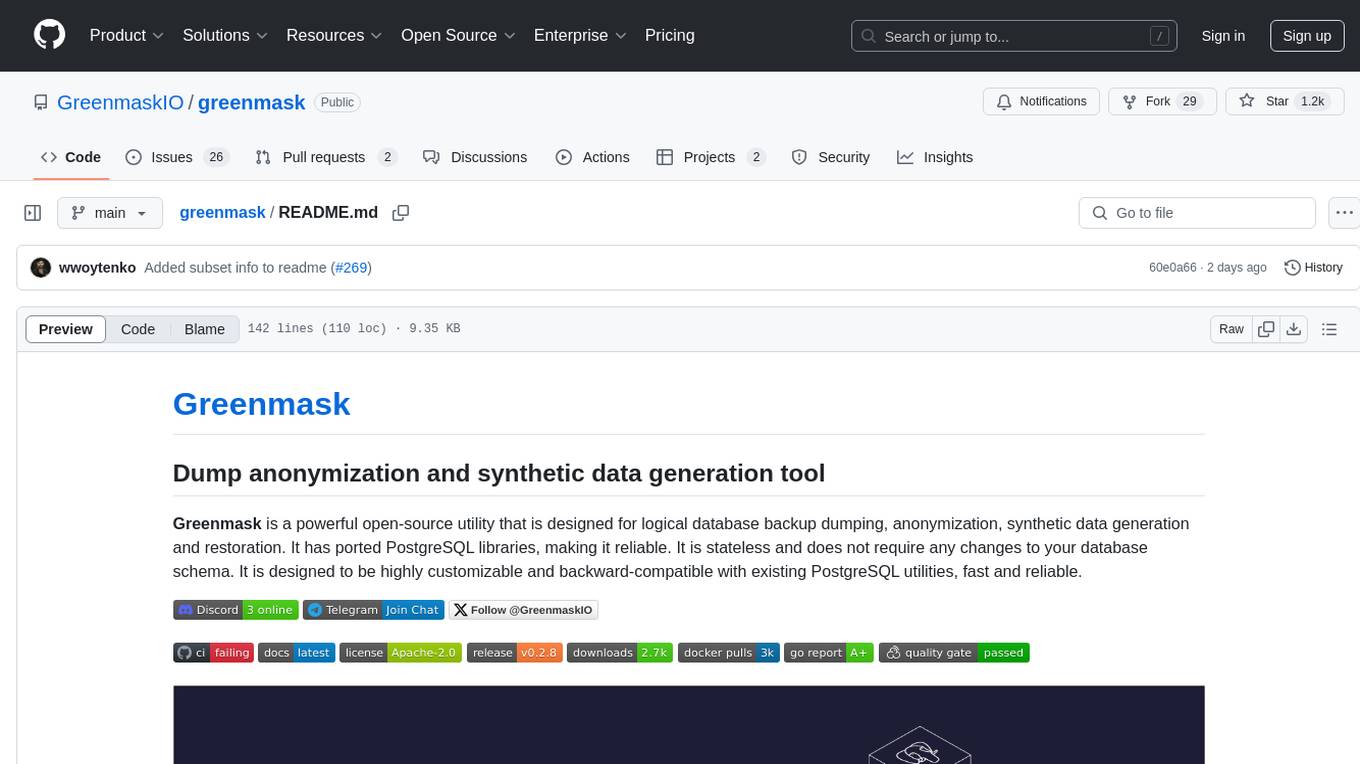
greenmask
Greenmask is a powerful open-source utility designed for logical database backup dumping, anonymization, synthetic data generation, and restoration. It is highly customizable, stateless, and backward-compatible with existing PostgreSQL utilities. Greenmask supports advanced subset systems, deterministic transformers, dynamic parameters, transformation conditions, and more. It is cross-platform, database type safe, extensible, and supports parallel execution and various storage options. Ideal for backup and restoration tasks, anonymization, transformation, and data masking.
For similar jobs
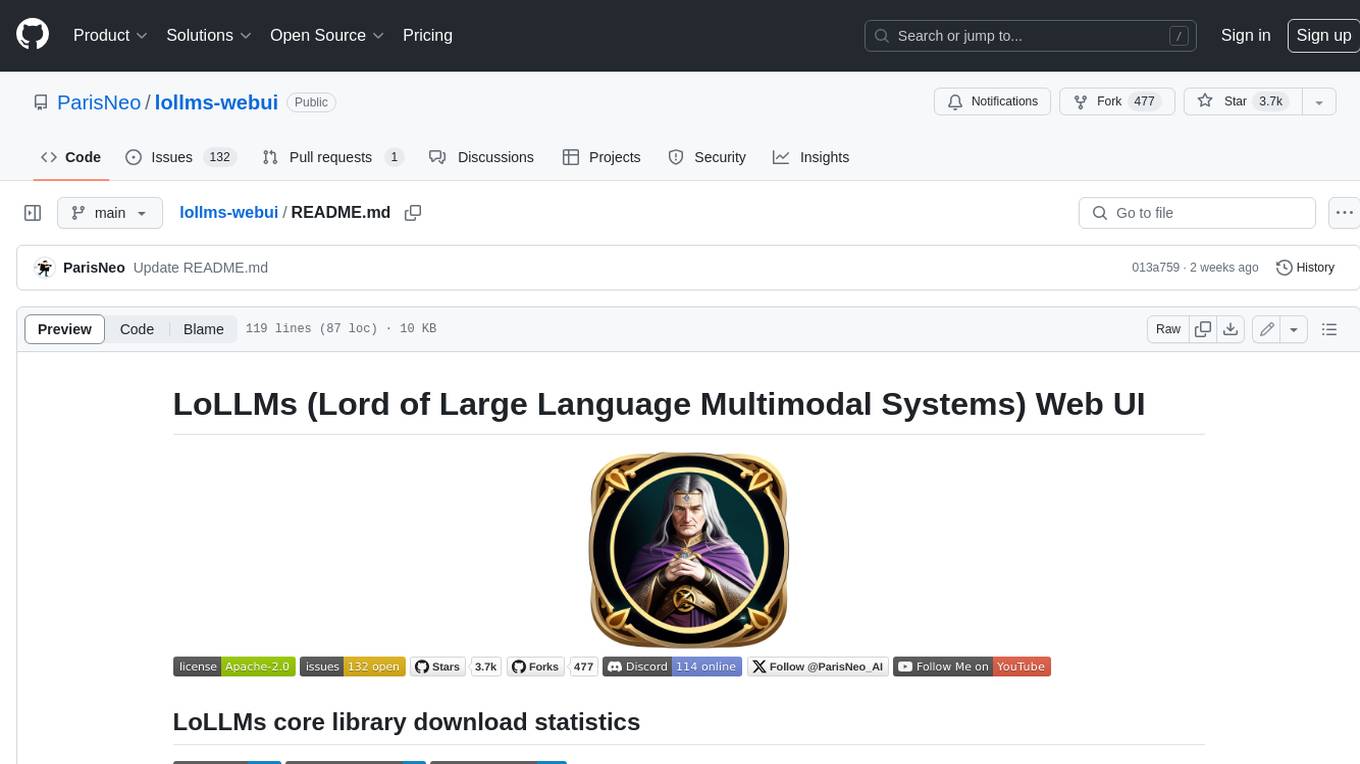
lollms-webui
LoLLMs WebUI (Lord of Large Language Multimodal Systems: One tool to rule them all) is a user-friendly interface to access and utilize various LLM (Large Language Models) and other AI models for a wide range of tasks. With over 500 AI expert conditionings across diverse domains and more than 2500 fine tuned models over multiple domains, LoLLMs WebUI provides an immediate resource for any problem, from car repair to coding assistance, legal matters, medical diagnosis, entertainment, and more. The easy-to-use UI with light and dark mode options, integration with GitHub repository, support for different personalities, and features like thumb up/down rating, copy, edit, and remove messages, local database storage, search, export, and delete multiple discussions, make LoLLMs WebUI a powerful and versatile tool.
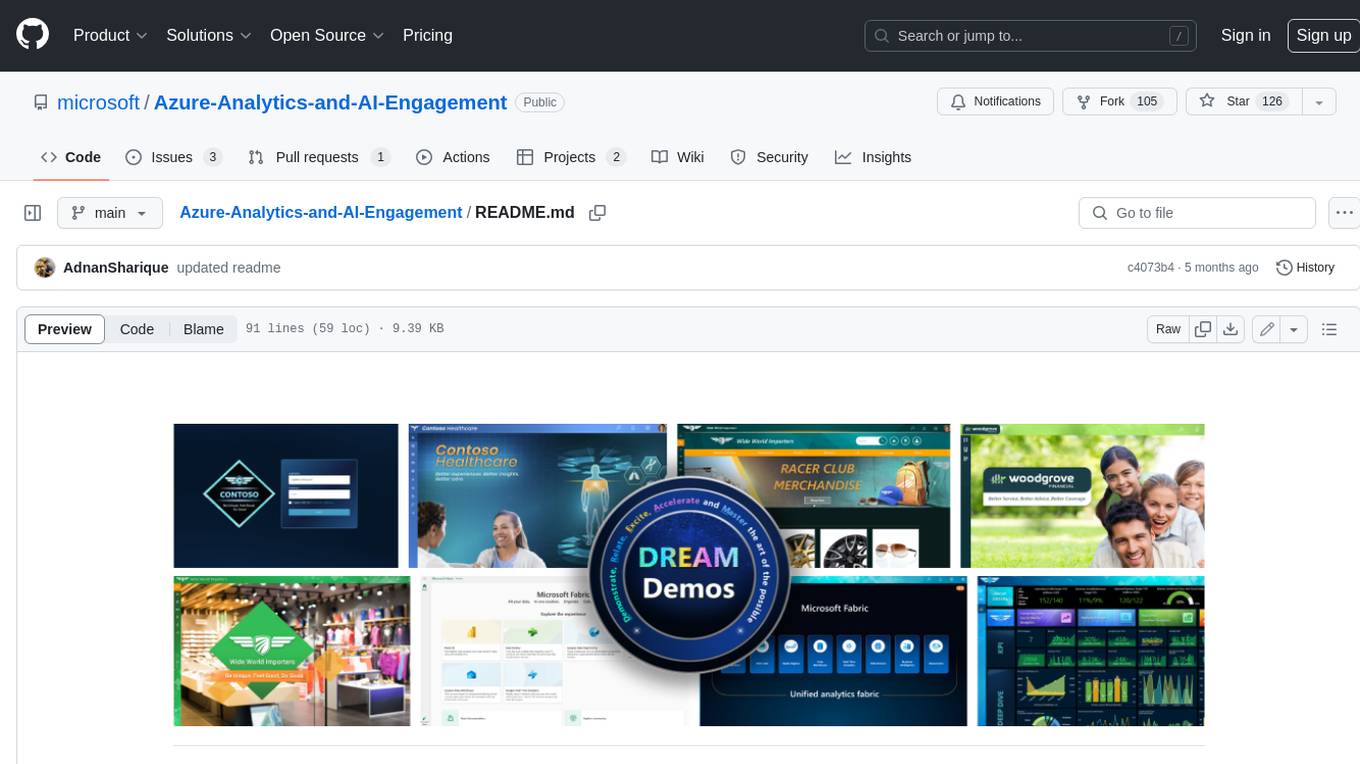
Azure-Analytics-and-AI-Engagement
The Azure-Analytics-and-AI-Engagement repository provides packaged Industry Scenario DREAM Demos with ARM templates (Containing a demo web application, Power BI reports, Synapse resources, AML Notebooks etc.) that can be deployed in a customer’s subscription using the CAPE tool within a matter of few hours. Partners can also deploy DREAM Demos in their own subscriptions using DPoC.
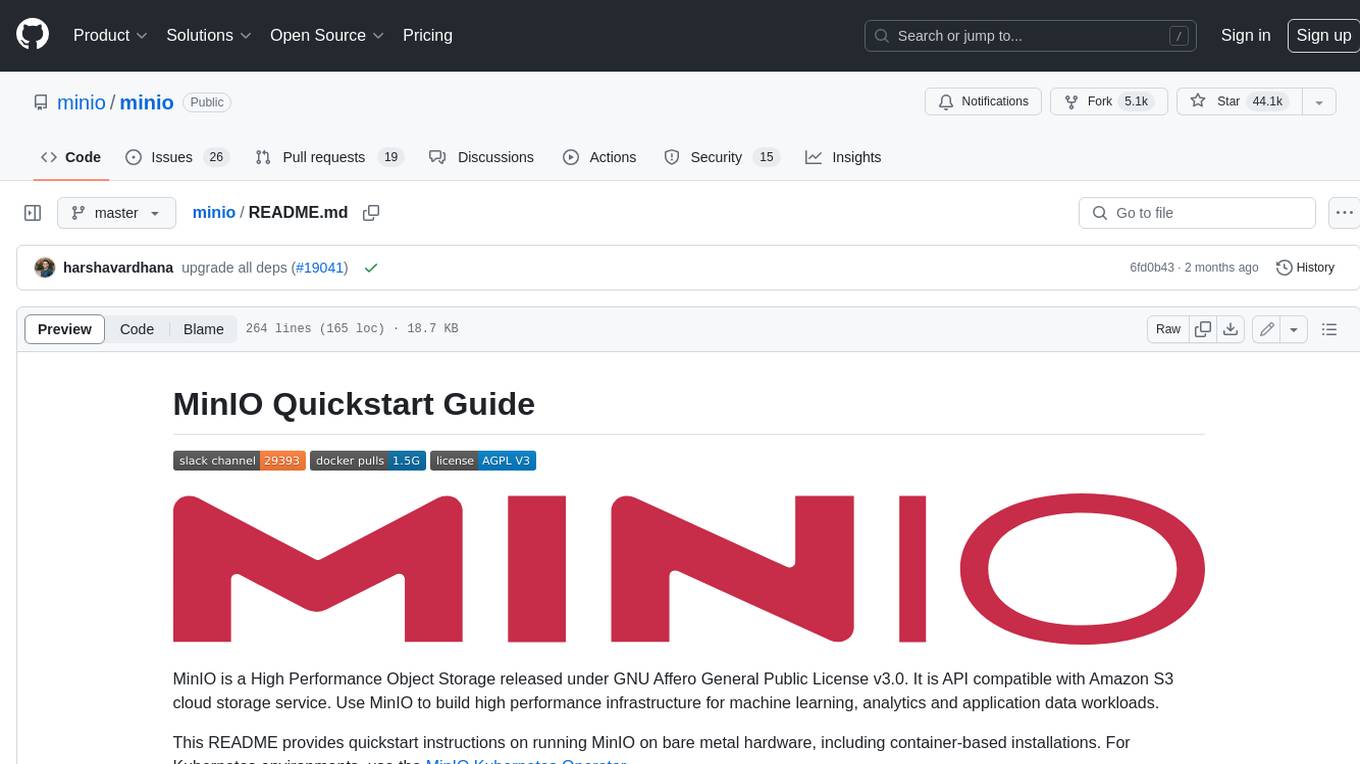
minio
MinIO is a High Performance Object Storage released under GNU Affero General Public License v3.0. It is API compatible with Amazon S3 cloud storage service. Use MinIO to build high performance infrastructure for machine learning, analytics and application data workloads.
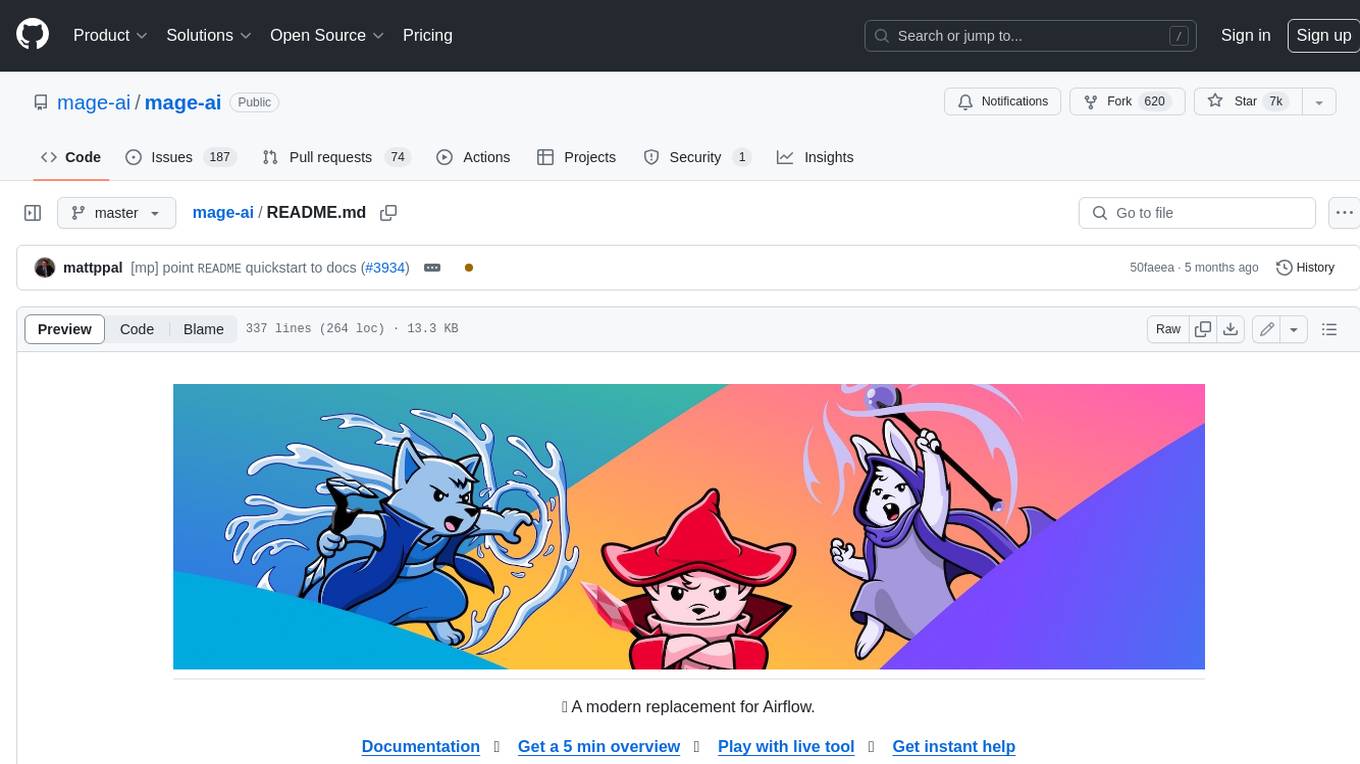
mage-ai
Mage is an open-source data pipeline tool for transforming and integrating data. It offers an easy developer experience, engineering best practices built-in, and data as a first-class citizen. Mage makes it easy to build, preview, and launch data pipelines, and provides observability and scaling capabilities. It supports data integrations, streaming pipelines, and dbt integration.
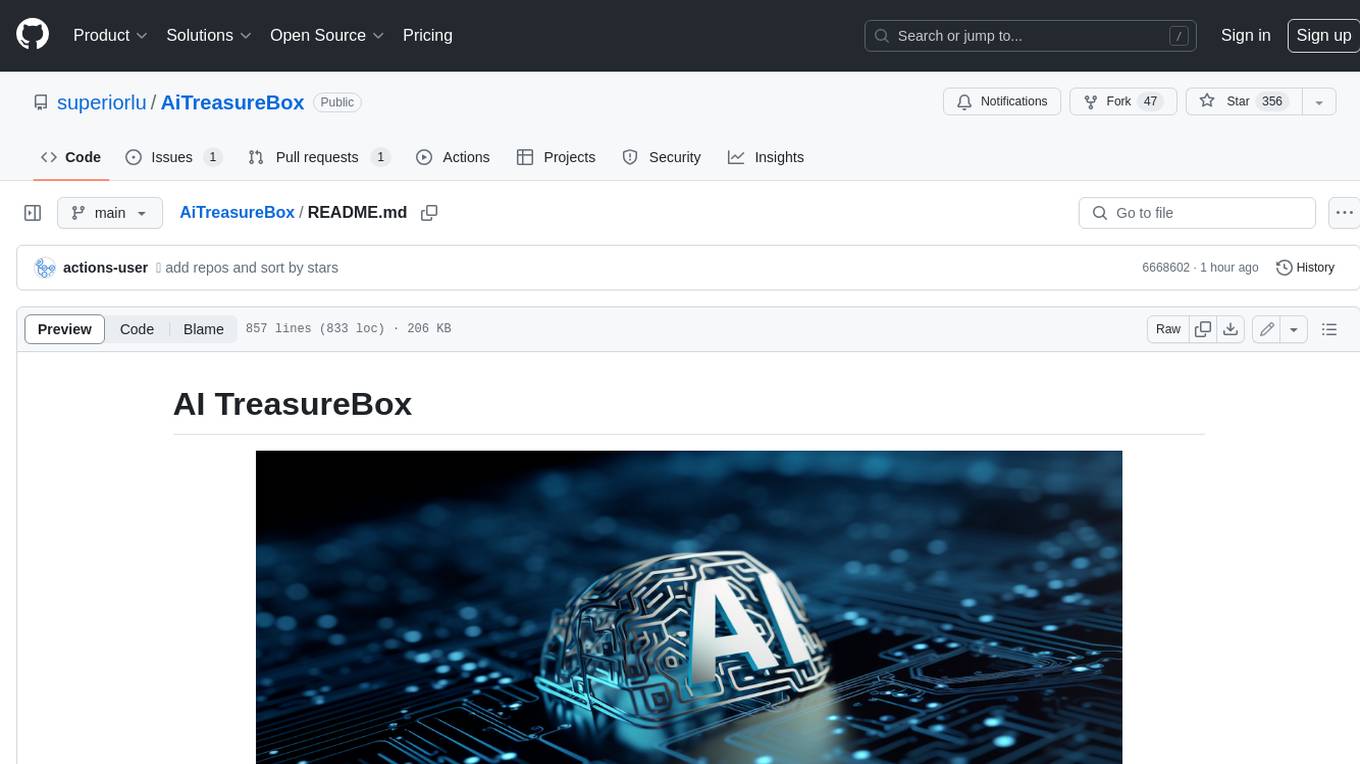
AiTreasureBox
AiTreasureBox is a versatile AI tool that provides a collection of pre-trained models and algorithms for various machine learning tasks. It simplifies the process of implementing AI solutions by offering ready-to-use components that can be easily integrated into projects. With AiTreasureBox, users can quickly prototype and deploy AI applications without the need for extensive knowledge in machine learning or deep learning. The tool covers a wide range of tasks such as image classification, text generation, sentiment analysis, object detection, and more. It is designed to be user-friendly and accessible to both beginners and experienced developers, making AI development more efficient and accessible to a wider audience.
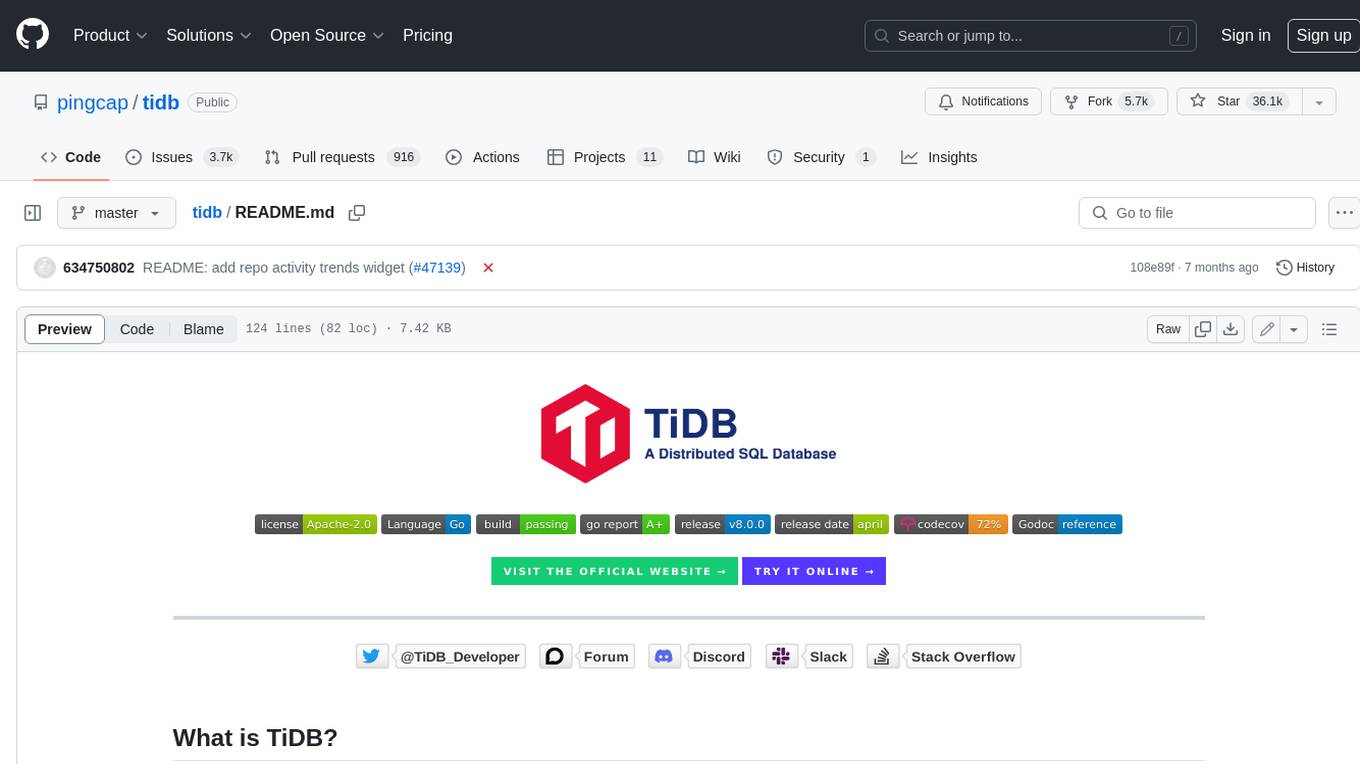
tidb
TiDB is an open-source distributed SQL database that supports Hybrid Transactional and Analytical Processing (HTAP) workloads. It is MySQL compatible and features horizontal scalability, strong consistency, and high availability.
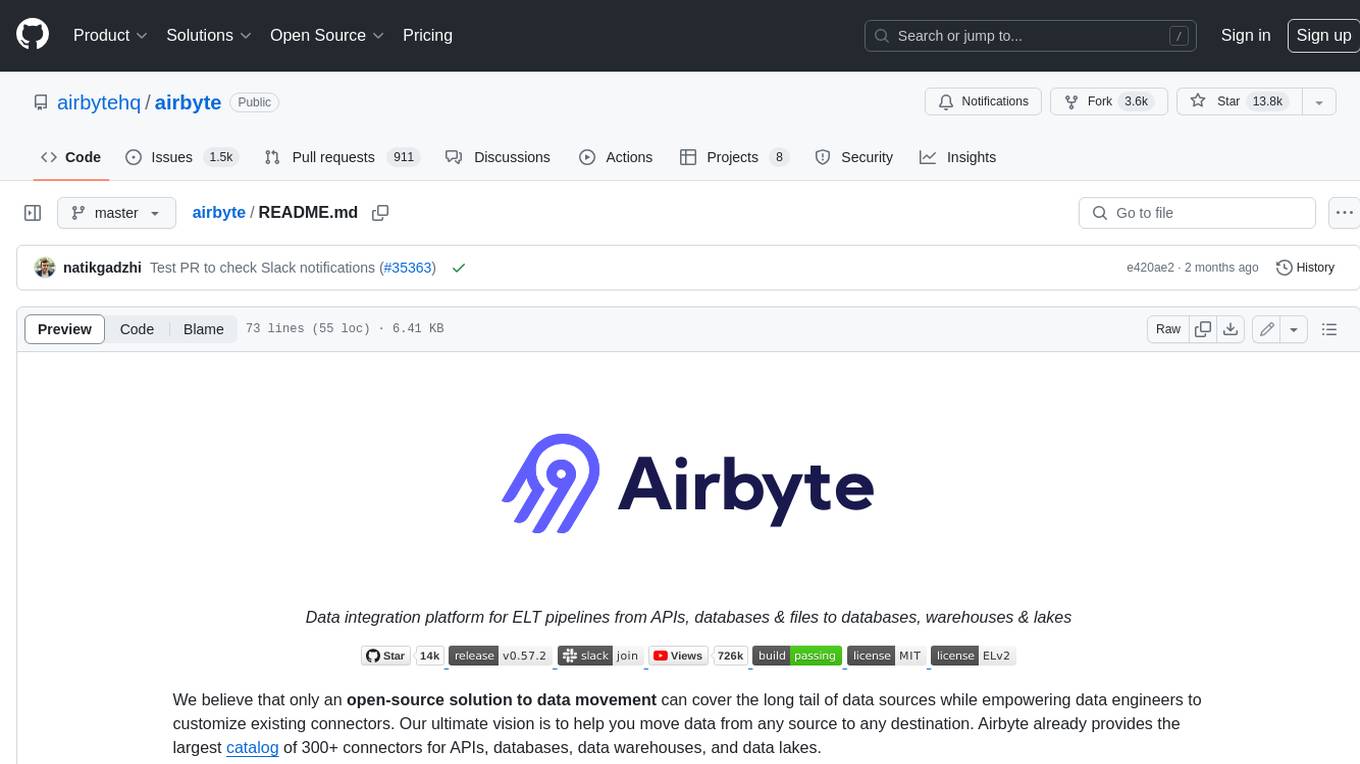
airbyte
Airbyte is an open-source data integration platform that makes it easy to move data from any source to any destination. With Airbyte, you can build and manage data pipelines without writing any code. Airbyte provides a library of pre-built connectors that make it easy to connect to popular data sources and destinations. You can also create your own connectors using Airbyte's no-code Connector Builder or low-code CDK. Airbyte is used by data engineers and analysts at companies of all sizes to build and manage their data pipelines.
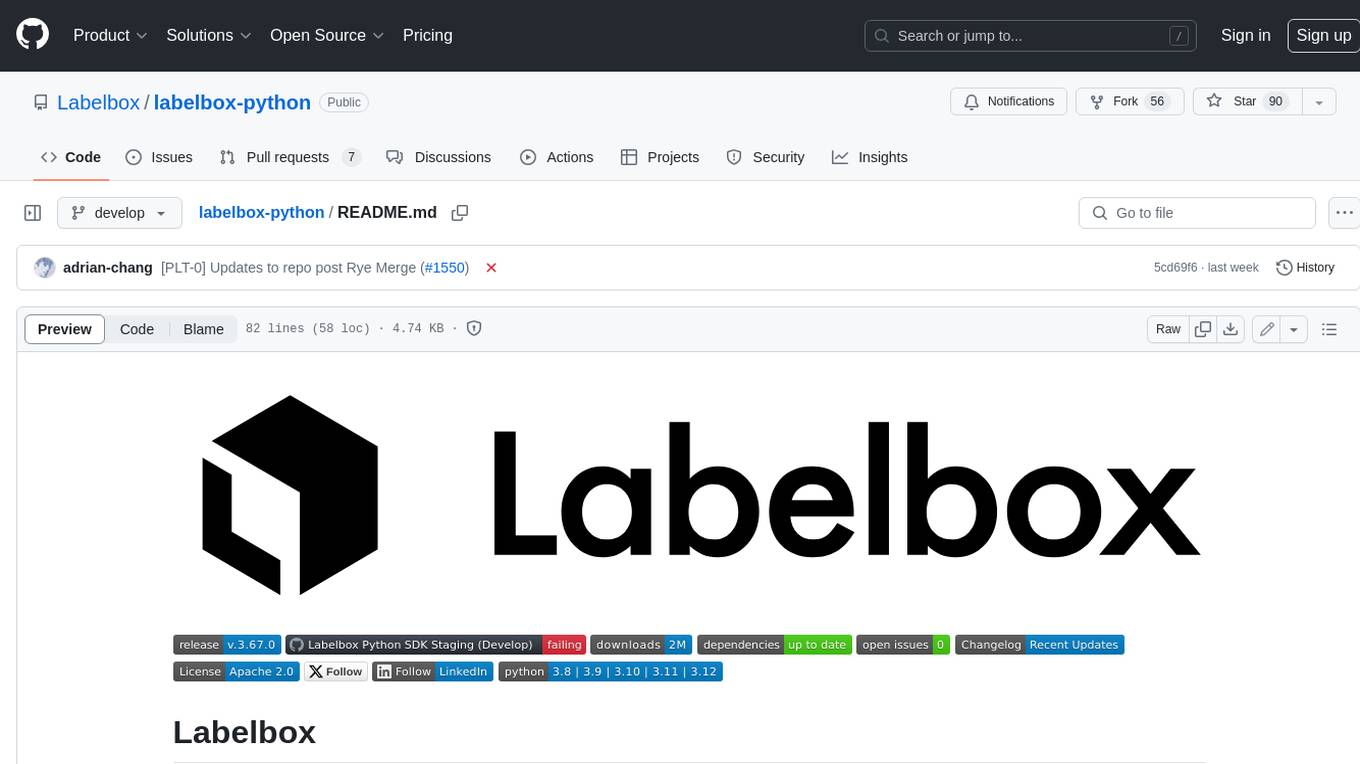
labelbox-python
Labelbox is a data-centric AI platform for enterprises to develop, optimize, and use AI to solve problems and power new products and services. Enterprises use Labelbox to curate data, generate high-quality human feedback data for computer vision and LLMs, evaluate model performance, and automate tasks by combining AI and human-centric workflows. The academic & research community uses Labelbox for cutting-edge AI research.