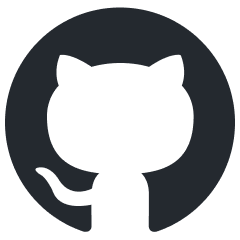
reductstore
High Performance Storage and Streaming Solution for Data Acquisition Systems
Stars: 199
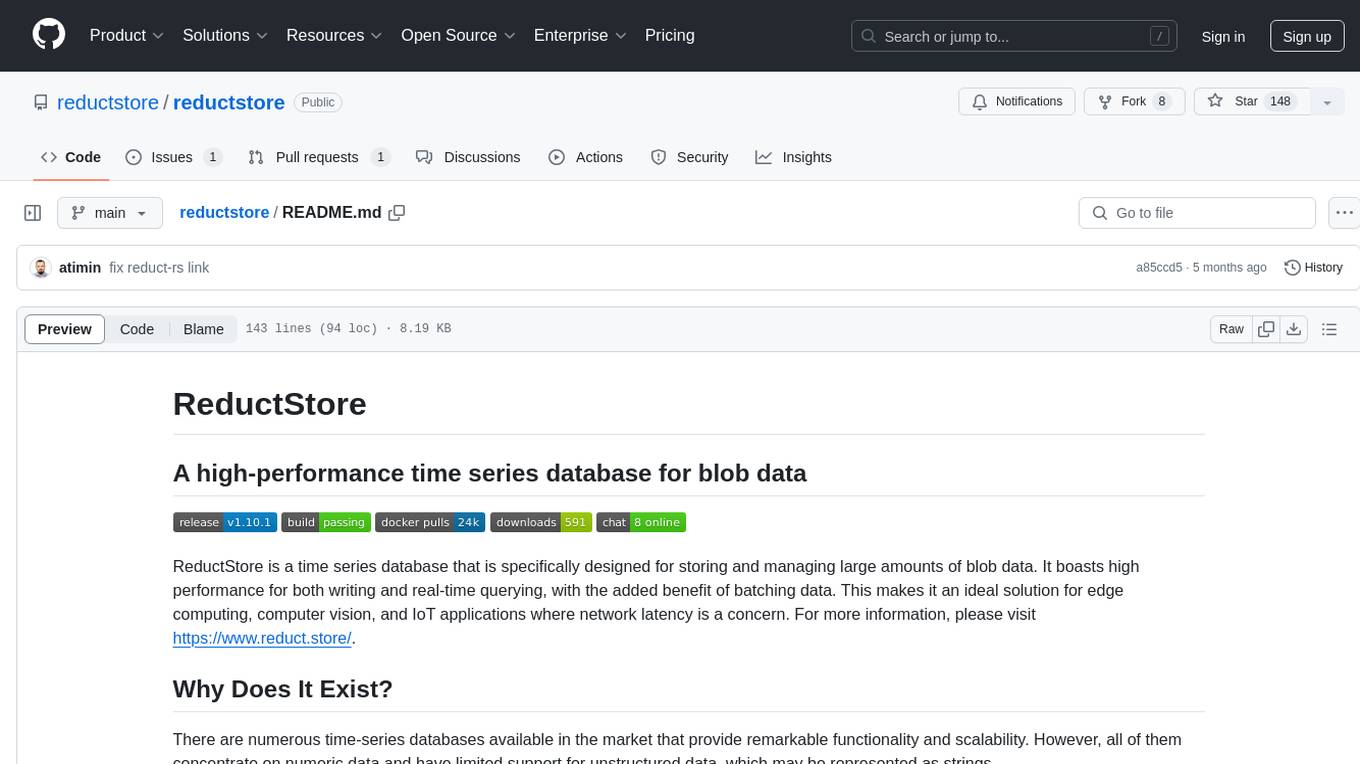
ReductStore is a high-performance time series database designed for storing and managing large amounts of unstructured blob data. It offers features such as real-time querying, batching data, and HTTP(S) API for edge computing, computer vision, and IoT applications. The database ensures data integrity, implements retention policies, and provides efficient data access, making it a cost-effective solution for applications requiring unstructured data storage and access at specific time intervals.
README:
ReductStore is a high-performance, time-series object storage and streaming solution for ELT-based data acquisition (DAQ) systems in robotics and industrial IoT (IIoT). It's designed to handle large volumes of unstructured data - images, sensor readings, logs, files, ROS bags - captured in raw form and stored with a precise time index (timestamp) and optional labels (e.g. device status, AI inference). This enables fast, efficient retrieval based on when the data was collected and how it's categorized, while also allowing control over data reduction strategies by replicating (streaming) only selected data from the edge to the cloud.
For more information, please visit https://www.reduct.store/.
There are numerous time-series databases available in the market that provide remarkable functionality and scalability. However, all of them concentrate on numeric data and have limited support for unstructured data, which may be represented as strings.
On the other hand, S3-like object storage solutions could be the best place to keep blob objects, but they don't provide an API to work with data in the time domain.
There are many kinds of applications where we need to collect unstructured data such as images, high-frequency sensor data, binary packages, or huge text documents and provide access to their history. Many companies build a storage solution for these applications based on a combination of TSDB and Blob storage in-house. It might be a working solution; however, it is a challenging development task to keep data integrity in both databases, implement retention policies, and provide data access with good performance.
The ReductStore project aims to solve the problem of providing a complete solution for applications that require unstructured data to be stored and accessed at specific time intervals. It guarantees that your data will not overflow your hard disk and batches records to reduce the number of critical HTTP requests for networks with high latency.
All of these features make the database the right choice for edge computing and IoT applications if you want to avoid development costs for your in-house solution.
- HTTP(S) API
- Storing and accessing unstructured data as time series
- No limit for maximum size of objects
- Labeling data for annotation and filtering
- JSON-based query language for filtering data
- Append-only data replication
- Batching records in an HTTP response for write and read operations
- Real-time FIFO bucket quota based on size to avoid disk space shortage
- Embedded Web Console
- Token authorization for managing data access
The quickest way to get up and running is with our Docker image:
docker run -p 8383:8383 -v ${PWD}/data:/data reduct/store:latest
Alternatively, you can opt for Cargo:
curl --proto '=https' --tlsv1.2 -sSf https://sh.rustup.rs | sh # Install the latest Rust
apt install protobuf-compiler
cargo install reductstore
RS_DATA_PATH=./data reductstore
For a more in-depth guide, visit the Getting Started and Download sections.
After initializing the instance, dive in with one of our Client SDKs to write or retrieve data. To illustrate, here's a Python sample:
from reduct import Client, BucketSettings, QuotaType
async def main():
# 1. Create a ReductStore client
async with Client("http://localhost:8383", api_token="my-token") as client:
# 2. Get or create a bucket with 1Gb quota
bucket = await client.create_bucket(
"my-bucket",
BucketSettings(quota_type=QuotaType.FIFO, quota_size=1_000_000_000),
exist_ok=True,
)
# 3. Write some data with timestamps and labels to the 'entry-1' entry
await bucket.write("sensor-1", b"<Blob data>", timestamp="2024-01-01T10:00:00Z",
labels={"score": 10})
await bucket.write("sensor-1", b"<Blob data>", timestamp="2024-01-01T10:00:01Z",
labels={"score": 20})
# 4. Query the data by time range and condition
async for record in bucket.query("sensor-1",
start="2024-01-01T10:00:00Z",
stop="2024-01-01T10:00:02Z",
when={"&score": {"$gt": 20}}):
print(f"Record timestamp: {record.timestamp}")
print(f"Record size: {record.size}")
print(await record.read_all())
# 5. Run the main function
if __name__ == "__main__":
import asyncio
asyncio.run(main())
ReductStore is built with adaptability in mind. While it comes with a straightforward HTTP API that can be integrated into virtually any environment, we understand that not everyone wants to interact with the API directly. To streamline your development process and make integrations smoother, we've developed a series of client SDKs tailored for different programming languages and environments. These SDKs wrap around the core API, offering a more intuitive and language-native way to interact with ReductStore, thus accelerating your development cycle. Here are the client SDKs available:
ReductStore is not just about data storage; it's about simplifying and enhancing your data management experience. Along with its robust core features, ReductStore offers a suite of tools to streamline administration, monitoring, and optimization. Here are the key tools you can leverage:
- CLI Client - a command-line interface for direct interactions with ReductStore
- Web Console - a web interface to administrate a ReductStore instance
Your input is invaluable to us! 🌟 If you've found a bug, have suggestions for improvements, or want to contribute directly to the codebase, here's how you can help:
- Questions and Ideas: Join our Discourse community to ask questions, share ideas, and collaborate with fellow ReductStore users.
- Bug Reports: Open an issue on our GitHub repository. Please provide as much detail as possible so we can address it effectively.
We believe in the power of community and collaboration. If you've built something amazing with ReductStore, we'd love to hear about it! Share your projects, experiences, and insights on our Discourse community.
If you find ReductStore beneficial, give us a ⭐ on our GitHub repository.
Your support fuels our passion and drives us to keep improving.
Together, let's redefine the future of blob data storage! 🚀
Q1: What sets ReductStore apart from other time-series databases?
A1: ReductStore is specially designed for storing and managing large amounts of blob data, optimized for both high performance and real-time querying. Unlike other databases that focus primarily on numeric data, ReductStore excels in handling unstructured data, making it ideal for various applications like edge computing and IoT.
Q2: How do I get started with ReductStore?
A2: You can easily set up ReductStore using our Docker image or by using cargo. Detailed instructions are provided in the Getting Started section.
Q3: Is there any size limitation for the blob data?
A3: While ReductStore is optimized for small objects (less than 1 MB), there's no hard limit for the maximum size of a blob.
Q4: Can I integrate ReductStore with my current infrastructure?
A4: Absolutely! With our variety of client SDKs and its adaptable HTTP API, ReductStore can be integrated into almost any environment.
Q5: I'm facing issues with the installation. Where can I get help?
A5: We recommend checking out our documentation. If you still face issues, feel free to join our Discord community or raise an issue on our GitHub repository.
For Tasks:
Click tags to check more tools for each tasksFor Jobs:
Alternative AI tools for reductstore
Similar Open Source Tools
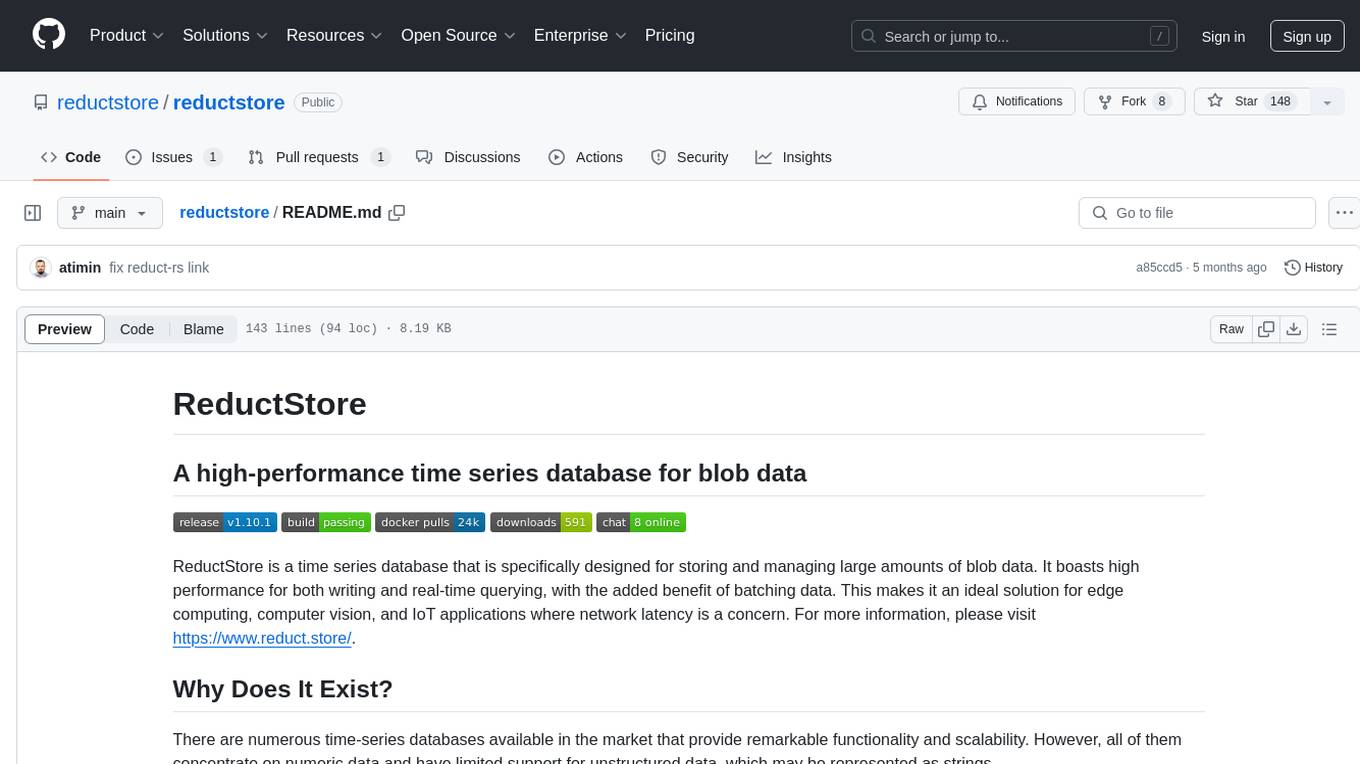
reductstore
ReductStore is a high-performance time series database designed for storing and managing large amounts of unstructured blob data. It offers features such as real-time querying, batching data, and HTTP(S) API for edge computing, computer vision, and IoT applications. The database ensures data integrity, implements retention policies, and provides efficient data access, making it a cost-effective solution for applications requiring unstructured data storage and access at specific time intervals.
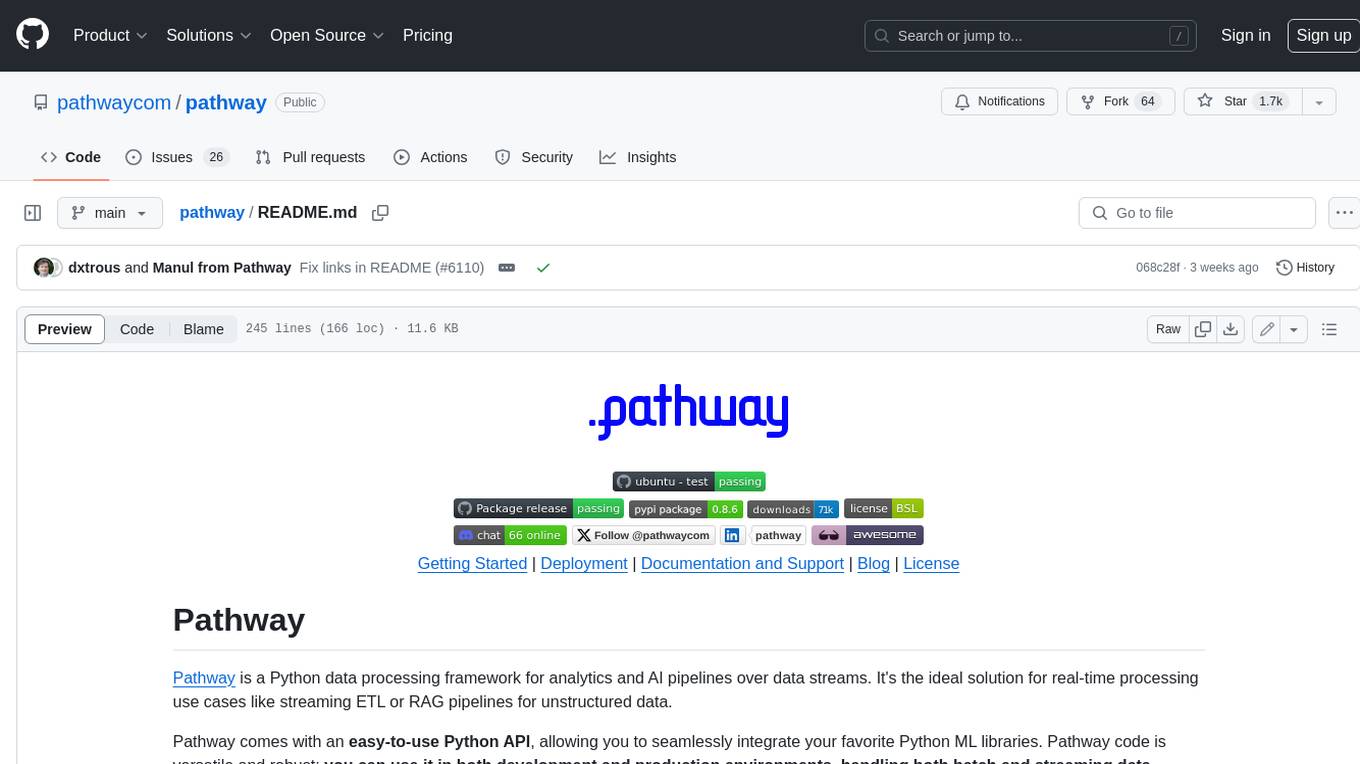
pathway
Pathway is a Python data processing framework for analytics and AI pipelines over data streams. It's the ideal solution for real-time processing use cases like streaming ETL or RAG pipelines for unstructured data. Pathway comes with an **easy-to-use Python API** , allowing you to seamlessly integrate your favorite Python ML libraries. Pathway code is versatile and robust: **you can use it in both development and production environments, handling both batch and streaming data effectively**. The same code can be used for local development, CI/CD tests, running batch jobs, handling stream replays, and processing data streams. Pathway is powered by a **scalable Rust engine** based on Differential Dataflow and performs incremental computation. Your Pathway code, despite being written in Python, is run by the Rust engine, enabling multithreading, multiprocessing, and distributed computations. All the pipeline is kept in memory and can be easily deployed with **Docker and Kubernetes**. You can install Pathway with pip: `pip install -U pathway` For any questions, you will find the community and team behind the project on Discord.
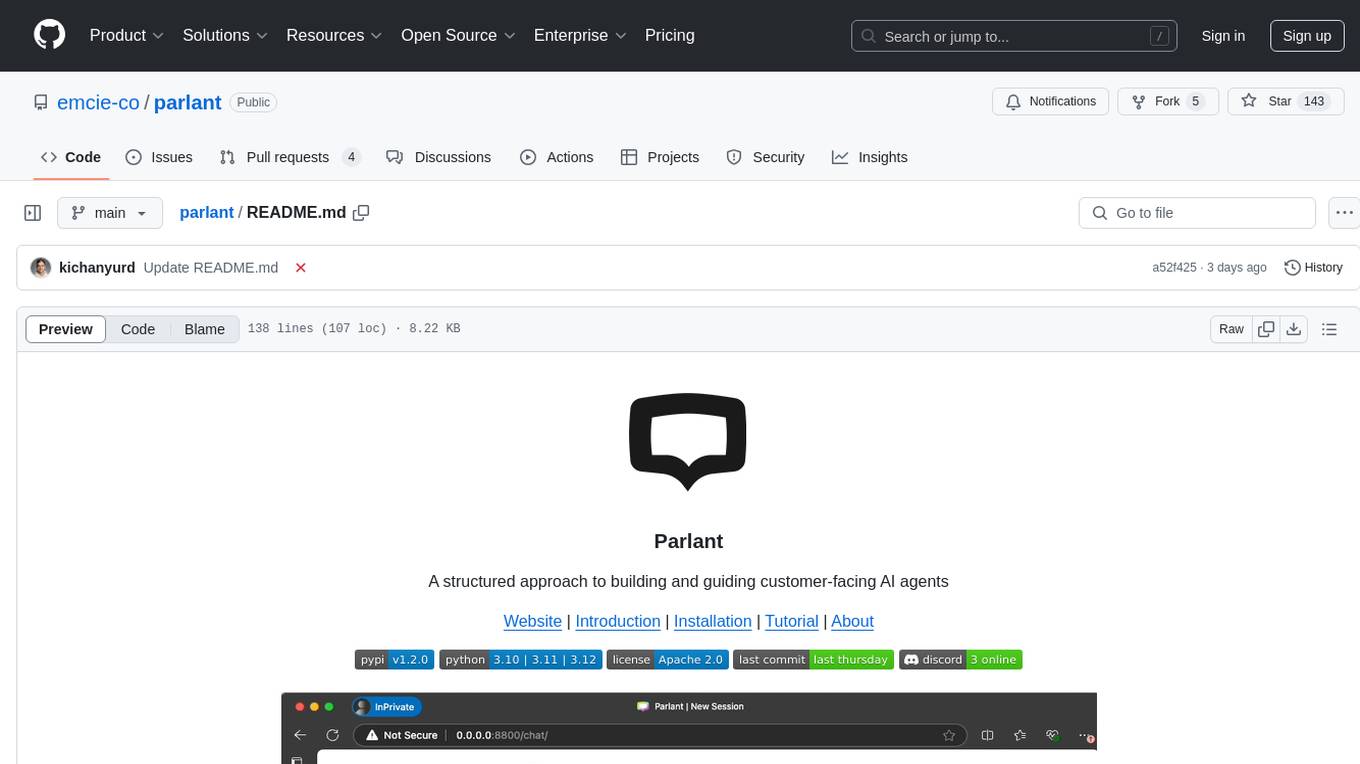
parlant
Parlant is a structured approach to building and guiding customer-facing AI agents. It allows developers to create and manage robust AI agents, providing specific feedback on agent behavior and helping understand user intentions better. With features like guidelines, glossary, coherence checks, dynamic context, and guided tool use, Parlant offers control over agent responses and behavior. Developer-friendly aspects include instant changes, Git integration, clean architecture, and type safety. It enables confident deployment with scalability, effective debugging, and validation before deployment. Parlant works with major LLM providers and offers client SDKs for Python and TypeScript. The tool facilitates natural customer interactions through asynchronous communication and provides a chat UI for testing new behaviors before deployment.
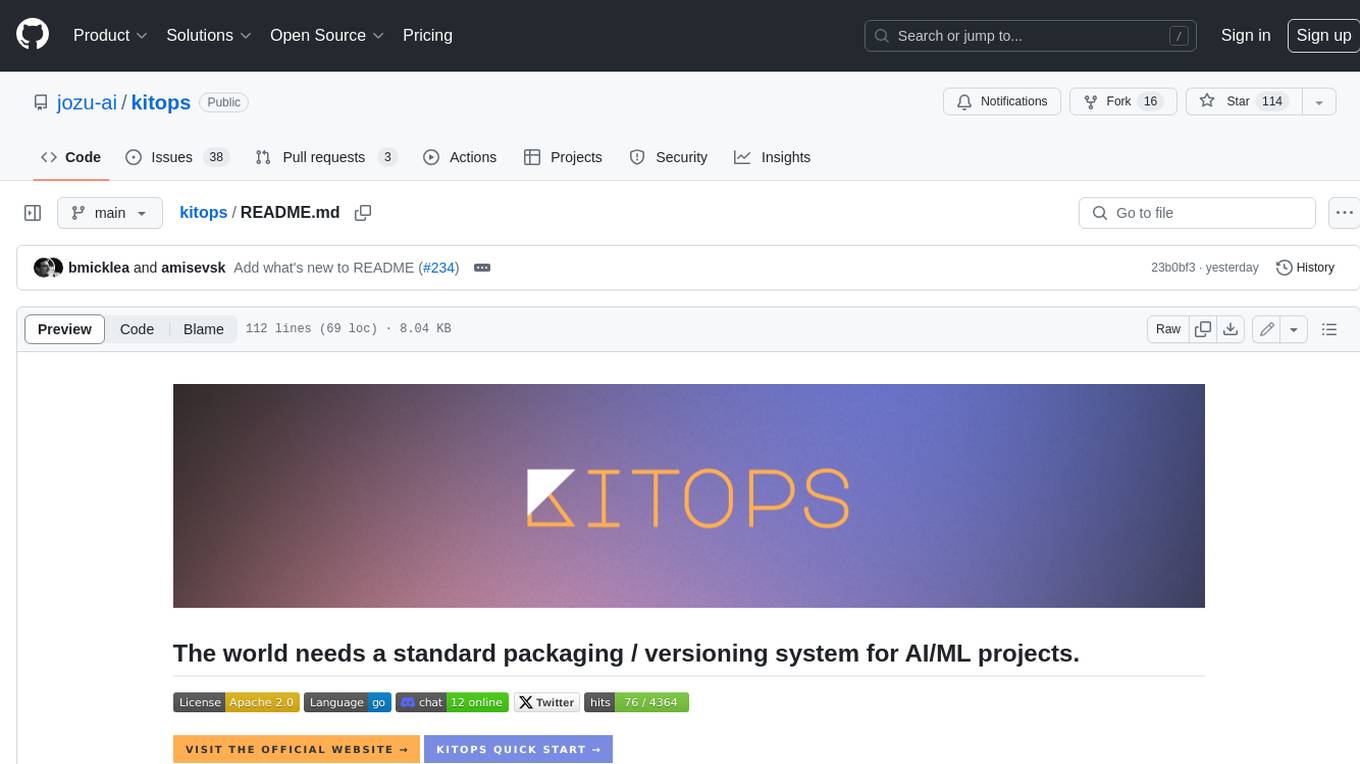
kitops
KitOps is a packaging and versioning system for AI/ML projects that uses open standards so it works with the AI/ML, development, and DevOps tools you are already using. KitOps simplifies the handoffs between data scientists, application developers, and SREs working with LLMs and other AI/ML models. KitOps' ModelKits are a standards-based package for models, their dependencies, configurations, and codebases. ModelKits are portable, reproducible, and work with the tools you already use.
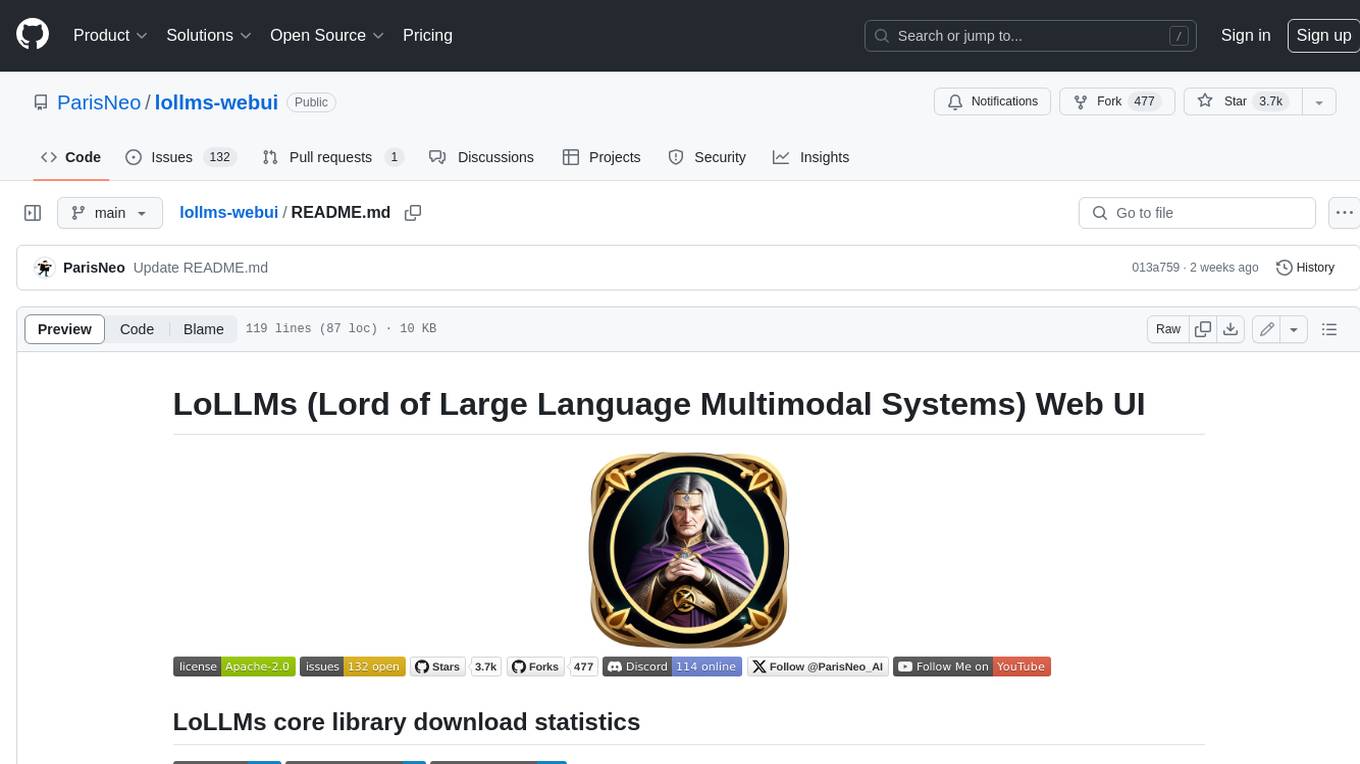
lollms-webui
LoLLMs WebUI (Lord of Large Language Multimodal Systems: One tool to rule them all) is a user-friendly interface to access and utilize various LLM (Large Language Models) and other AI models for a wide range of tasks. With over 500 AI expert conditionings across diverse domains and more than 2500 fine tuned models over multiple domains, LoLLMs WebUI provides an immediate resource for any problem, from car repair to coding assistance, legal matters, medical diagnosis, entertainment, and more. The easy-to-use UI with light and dark mode options, integration with GitHub repository, support for different personalities, and features like thumb up/down rating, copy, edit, and remove messages, local database storage, search, export, and delete multiple discussions, make LoLLMs WebUI a powerful and versatile tool.
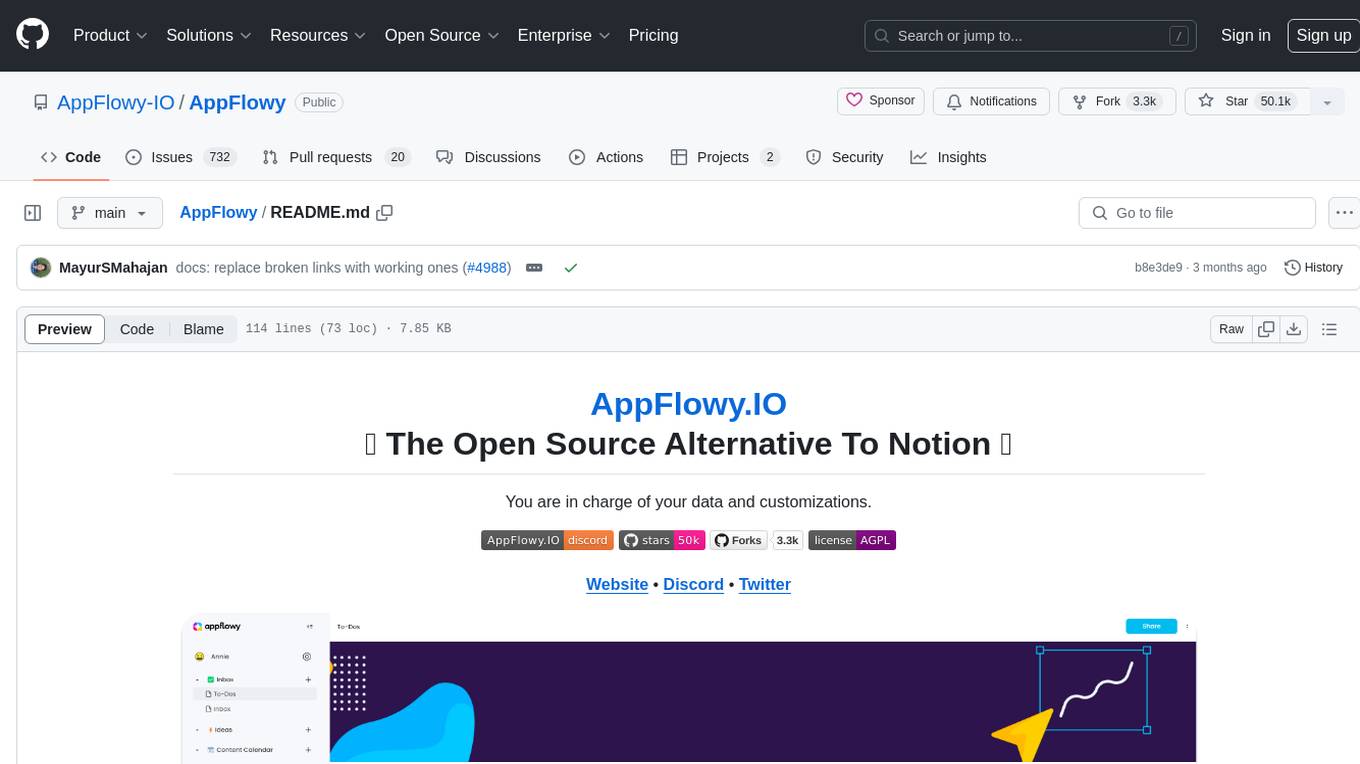
AppFlowy
AppFlowy.IO is an open-source alternative to Notion, providing users with control over their data and customizations. It aims to offer functionality, data security, and cross-platform native experience to individuals, as well as building blocks and collaboration infra services to enterprises and hackers. The tool is built with Flutter and Rust, supporting multiple platforms and emphasizing long-term maintainability. AppFlowy prioritizes data privacy, reliable native experience, and community-driven extensibility, aiming to democratize the creation of complex workplace management tools.
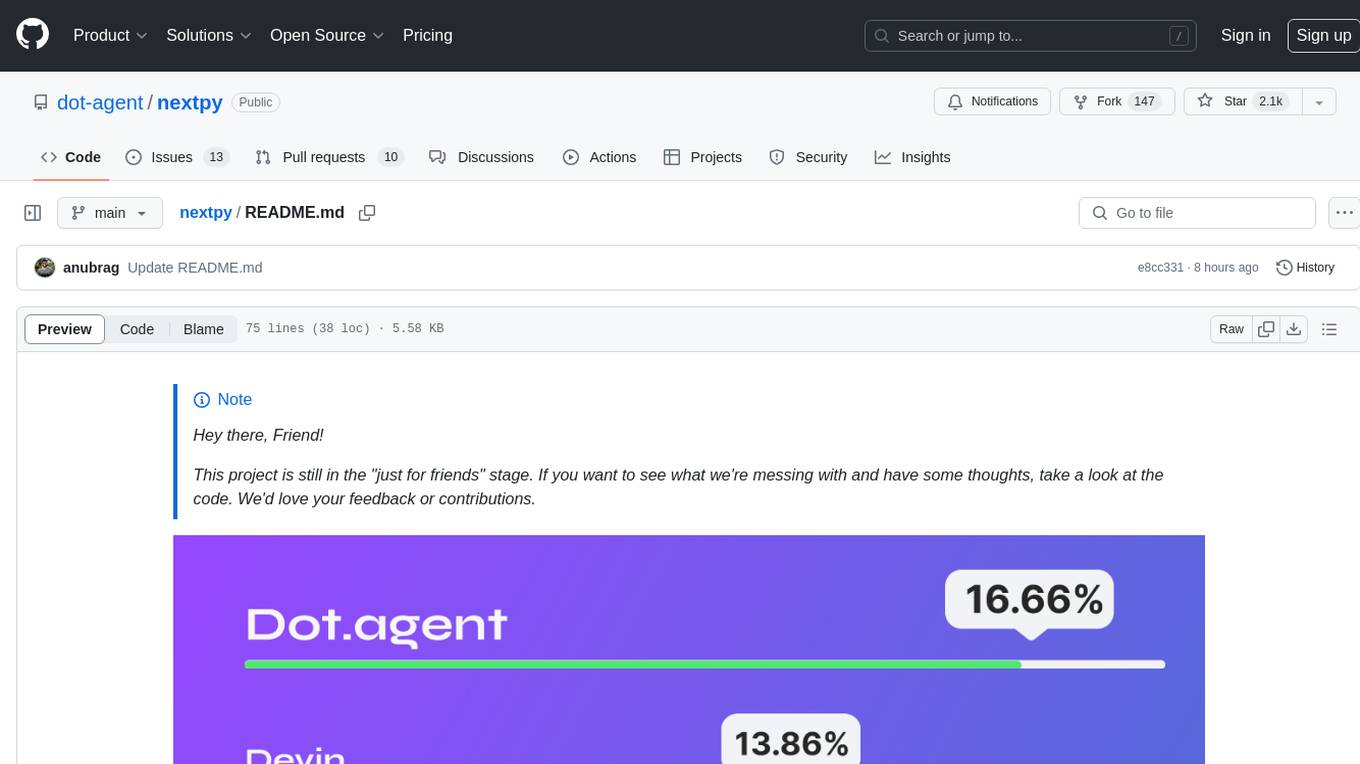
nextpy
Nextpy is a cutting-edge software development framework optimized for AI-based code generation. It provides guardrails for defining AI system boundaries, structured outputs for prompt engineering, a powerful prompt engine for efficient processing, better AI generations with precise output control, modularity for multiplatform and extensible usage, developer-first approach for transferable knowledge, and containerized & scalable deployment options. It offers 4-10x faster performance compared to Streamlit apps, with a focus on cooperation within the open-source community and integration of key components from various projects.
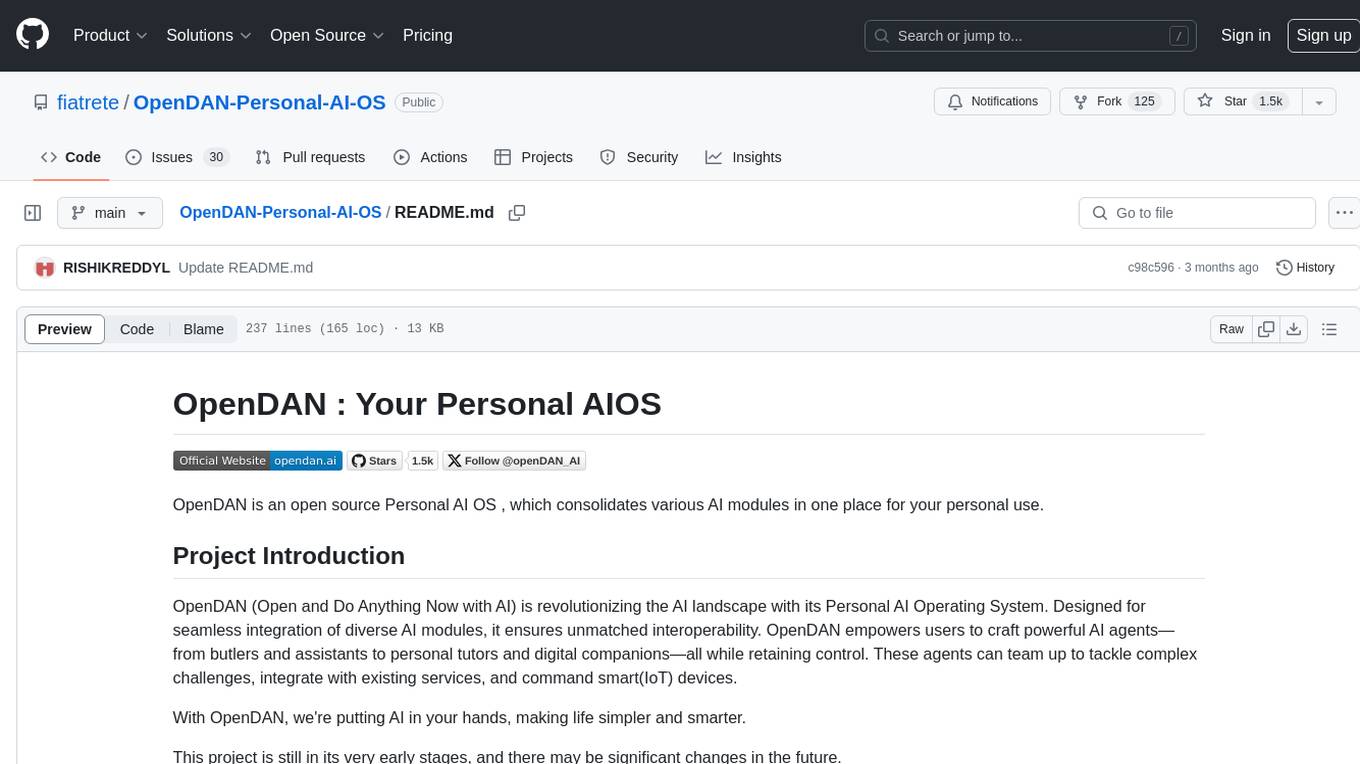
OpenDAN-Personal-AI-OS
OpenDAN is an open source Personal AI OS that consolidates various AI modules for personal use. It empowers users to create powerful AI agents like assistants, tutors, and companions. The OS allows agents to collaborate, integrate with services, and control smart devices. OpenDAN offers features like rapid installation, AI agent customization, connectivity via Telegram/Email, building a local knowledge base, distributed AI computing, and more. It aims to simplify life by putting AI in users' hands. The project is in early stages with ongoing development and future plans for user and kernel mode separation, home IoT device control, and an official OpenDAN SDK release.
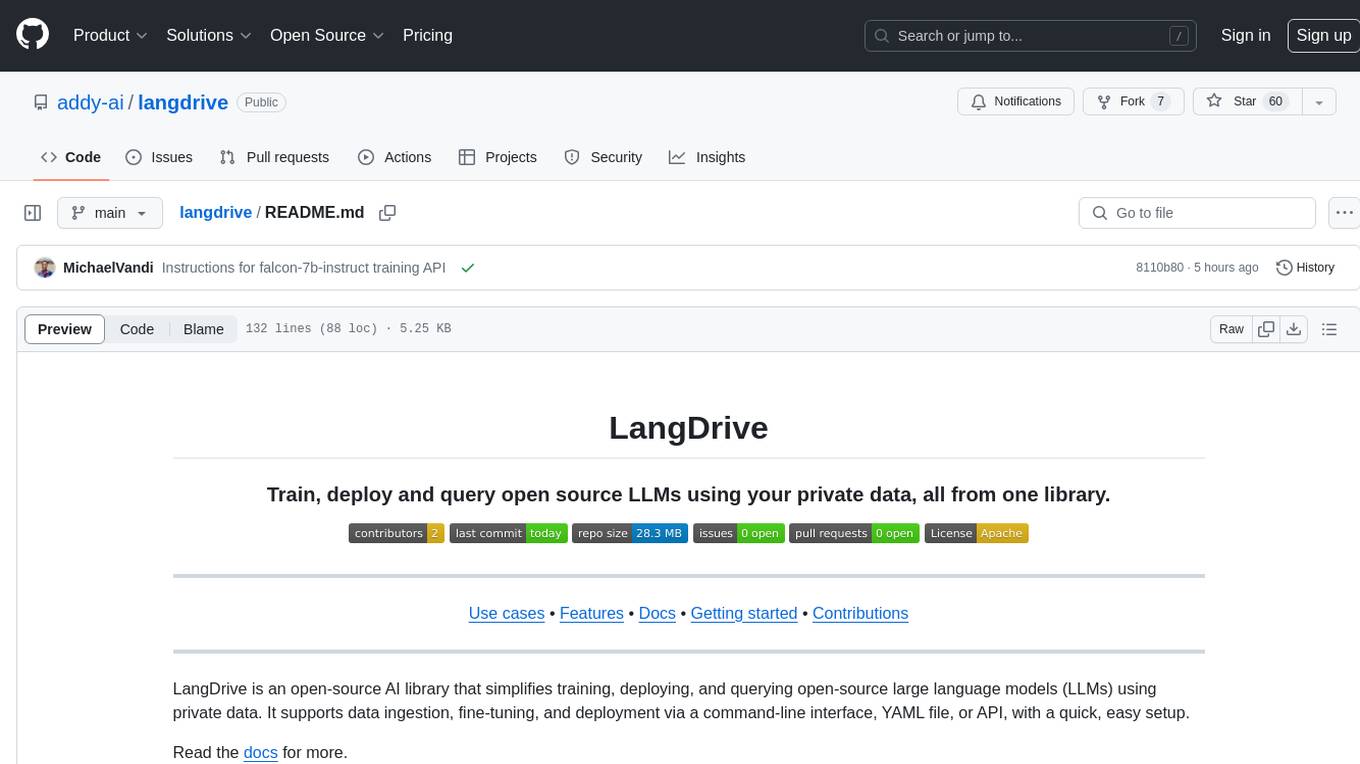
langdrive
LangDrive is an open-source AI library that simplifies training, deploying, and querying open-source large language models (LLMs) using private data. It supports data ingestion, fine-tuning, and deployment via a command-line interface, YAML file, or API, with a quick, easy setup. Users can build AI applications such as question/answering systems, chatbots, AI agents, and content generators. The library provides features like data connectors for ingestion, fine-tuning of LLMs, deployment to Hugging Face hub, inference querying, data utilities for CRUD operations, and APIs for model access. LangDrive is designed to streamline the process of working with LLMs and making AI development more accessible.
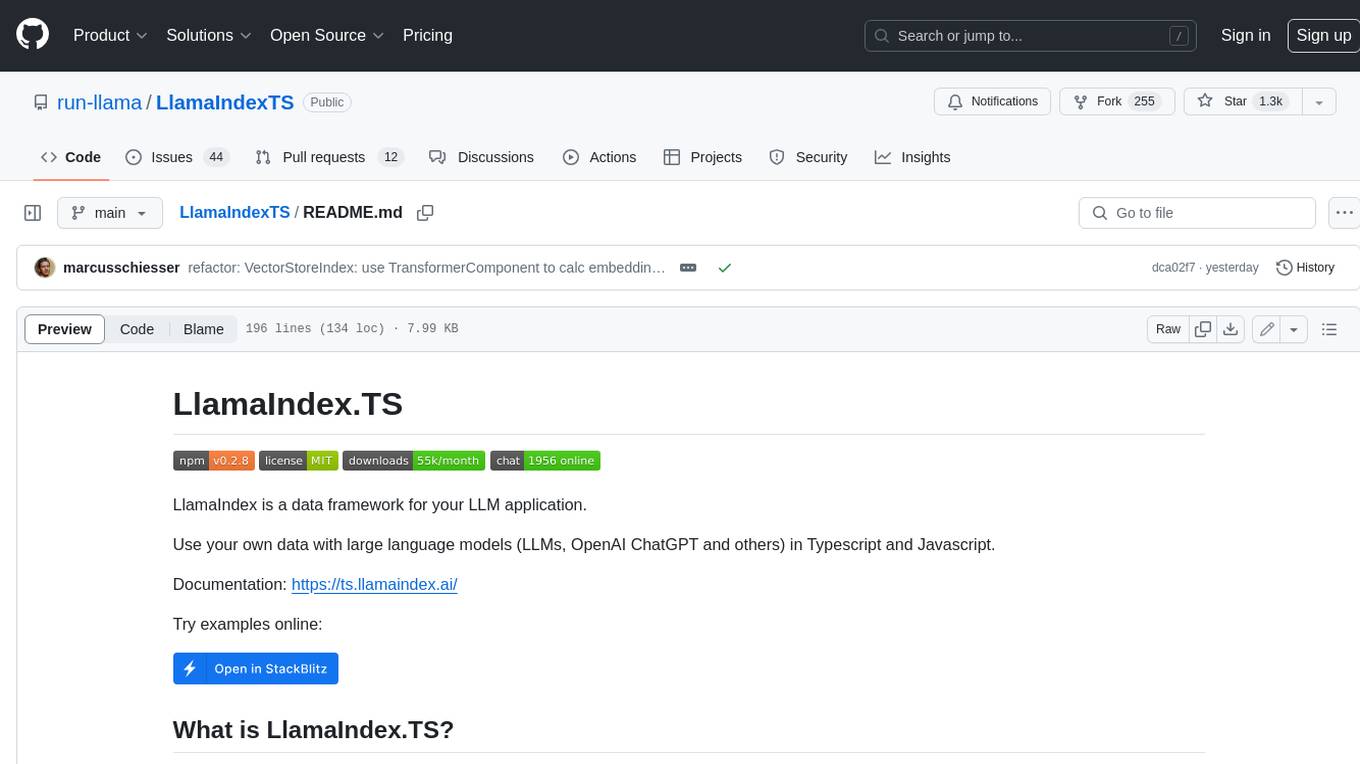
LlamaIndexTS
LlamaIndex.TS is a data framework for your LLM application. Use your own data with large language models (LLMs, OpenAI ChatGPT and others) in Typescript and Javascript.
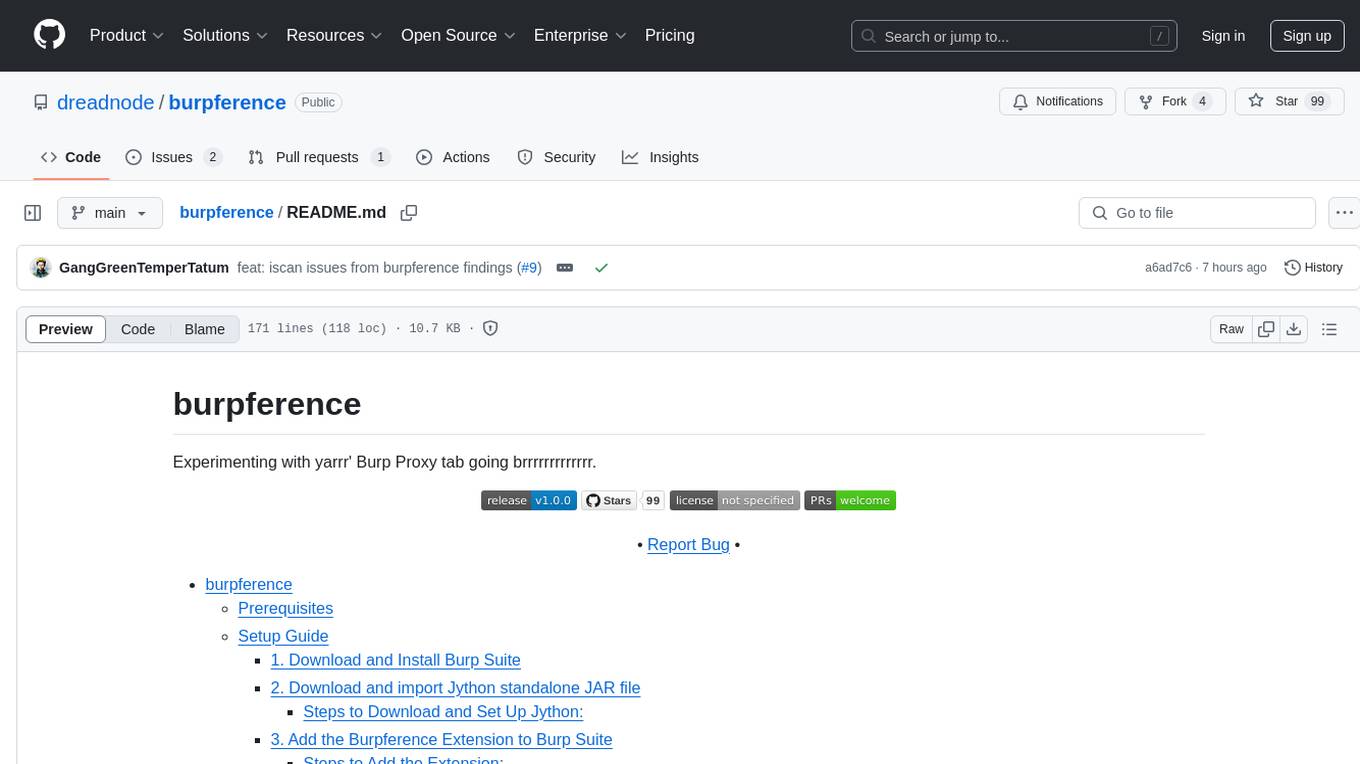
burpference
Burpference is an open-source extension designed to capture in-scope HTTP requests and responses from Burp's proxy history and send them to a remote LLM API in JSON format. It automates response capture, integrates with APIs, optimizes resource usage, provides color-coded findings visualization, offers comprehensive logging, supports native Burp reporting, and allows flexible configuration. Users can customize system prompts, API keys, and remote hosts, and host models locally to prevent high inference costs. The tool is ideal for offensive web application engagements to surface findings and vulnerabilities.
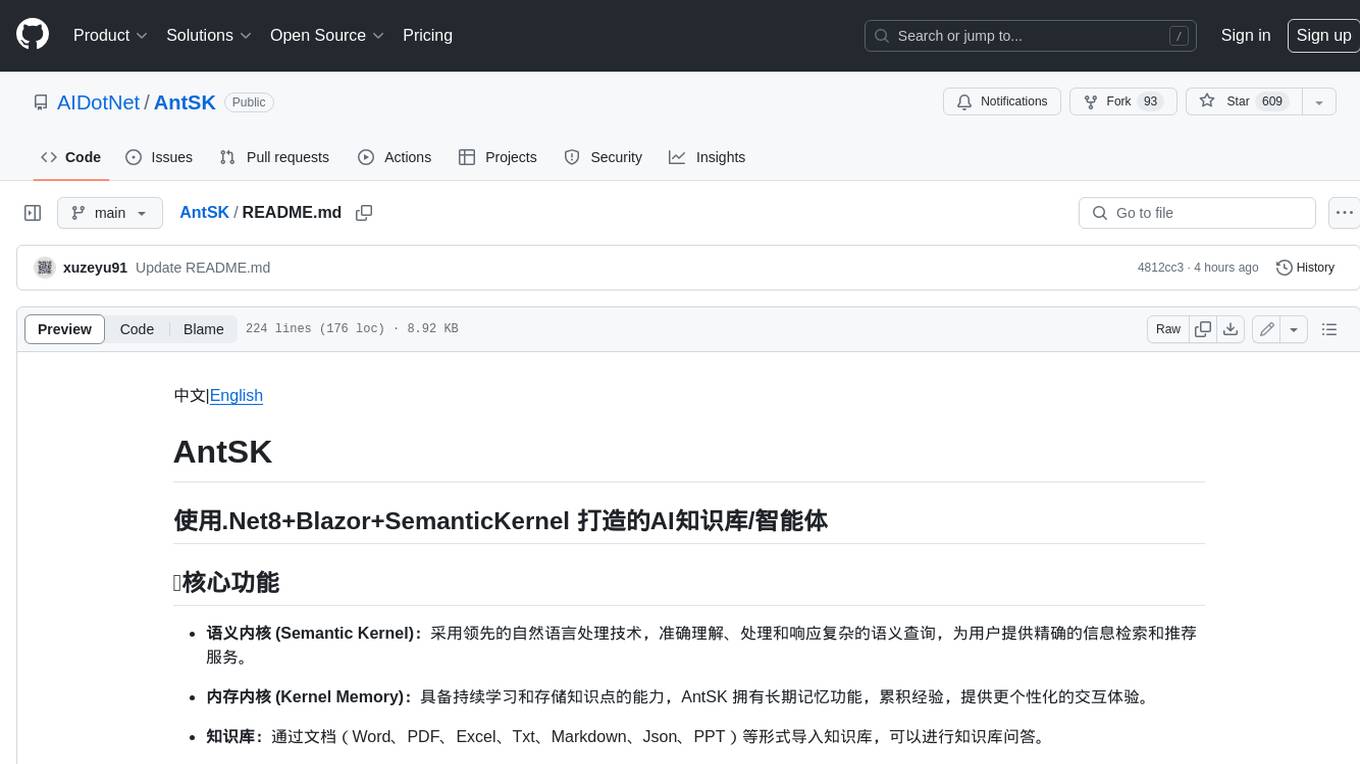
AntSK
AntSK is an AI knowledge base/agent built with .Net8+Blazor+SemanticKernel. It features a semantic kernel for accurate natural language processing, a memory kernel for continuous learning and knowledge storage, a knowledge base for importing and querying knowledge from various document formats, a text-to-image generator integrated with StableDiffusion, GPTs generation for creating personalized GPT models, API interfaces for integrating AntSK into other applications, an open API plugin system for extending functionality, a .Net plugin system for integrating business functions, real-time information retrieval from the internet, model management for adapting and managing different models from different vendors, support for domestic models and databases for operation in a trusted environment, and planned model fine-tuning based on llamafactory.
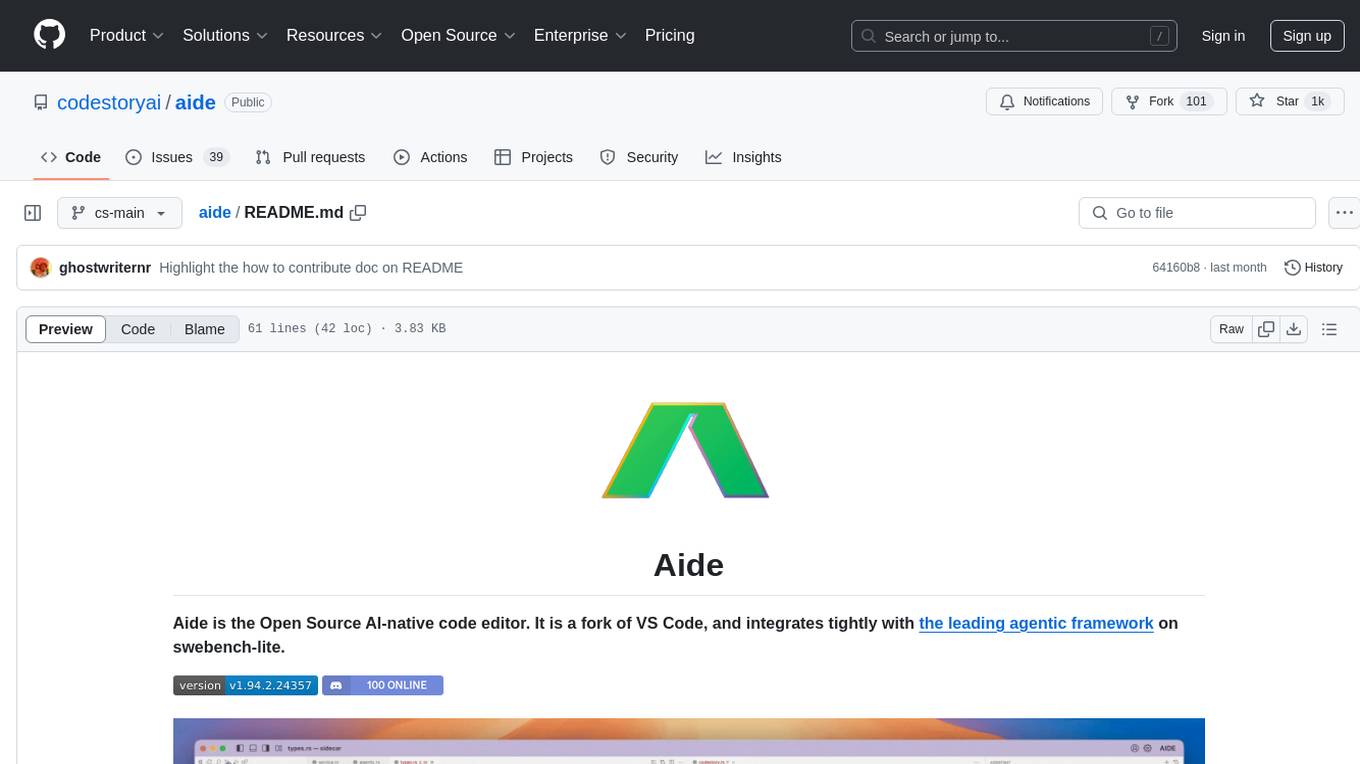
aide
Aide is an Open Source AI-native code editor that combines the powerful features of VS Code with advanced AI capabilities. It provides a combined chat + edit flow, proactive agents for fixing errors, inline editing widget, intelligent code completion, and AST navigation. Aide is designed to be an intelligent coding companion, helping users write better code faster while maintaining control over the development process.
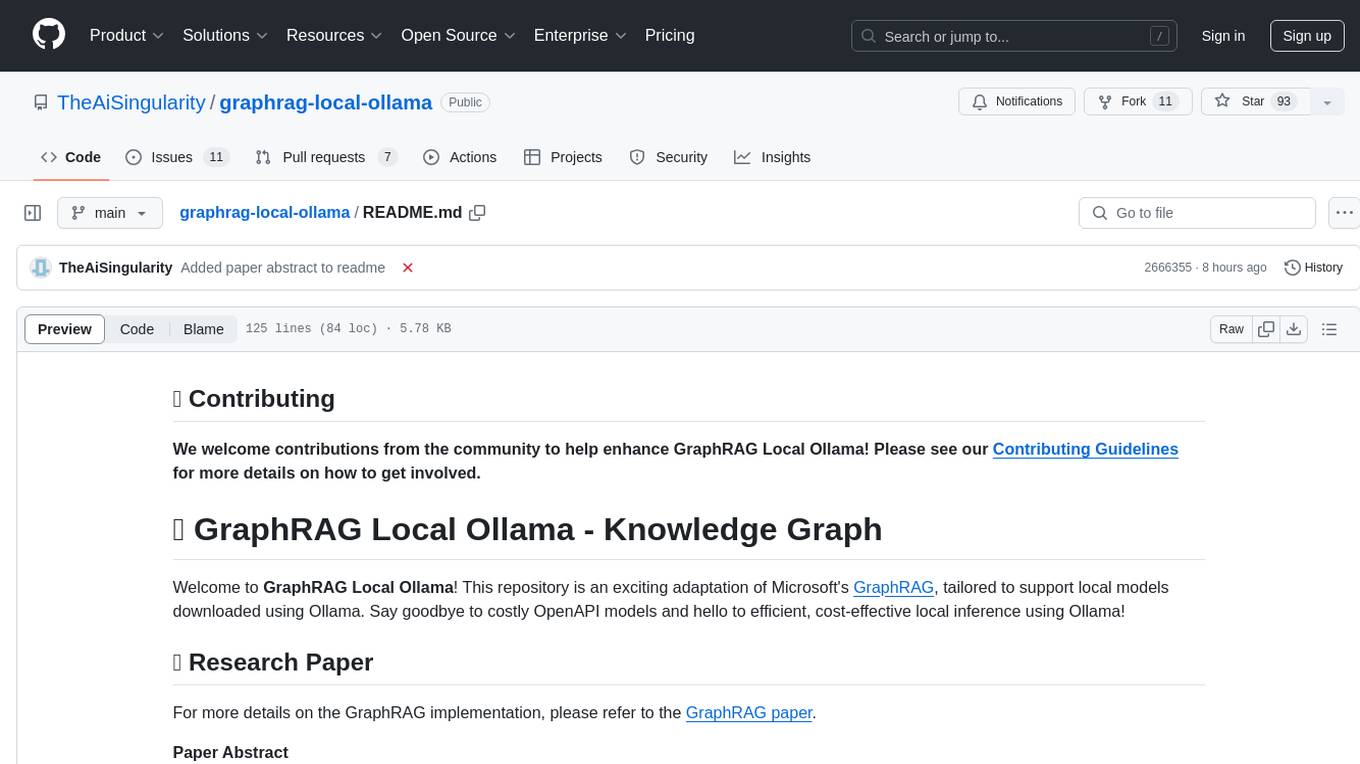
graphrag-local-ollama
GraphRAG Local Ollama is a repository that offers an adaptation of Microsoft's GraphRAG, customized to support local models downloaded using Ollama. It enables users to leverage local models with Ollama for large language models (LLMs) and embeddings, eliminating the need for costly OpenAPI models. The repository provides a simple setup process and allows users to perform question answering over private text corpora by building a graph-based text index and generating community summaries for closely-related entities. GraphRAG Local Ollama aims to improve the comprehensiveness and diversity of generated answers for global sensemaking questions over datasets.
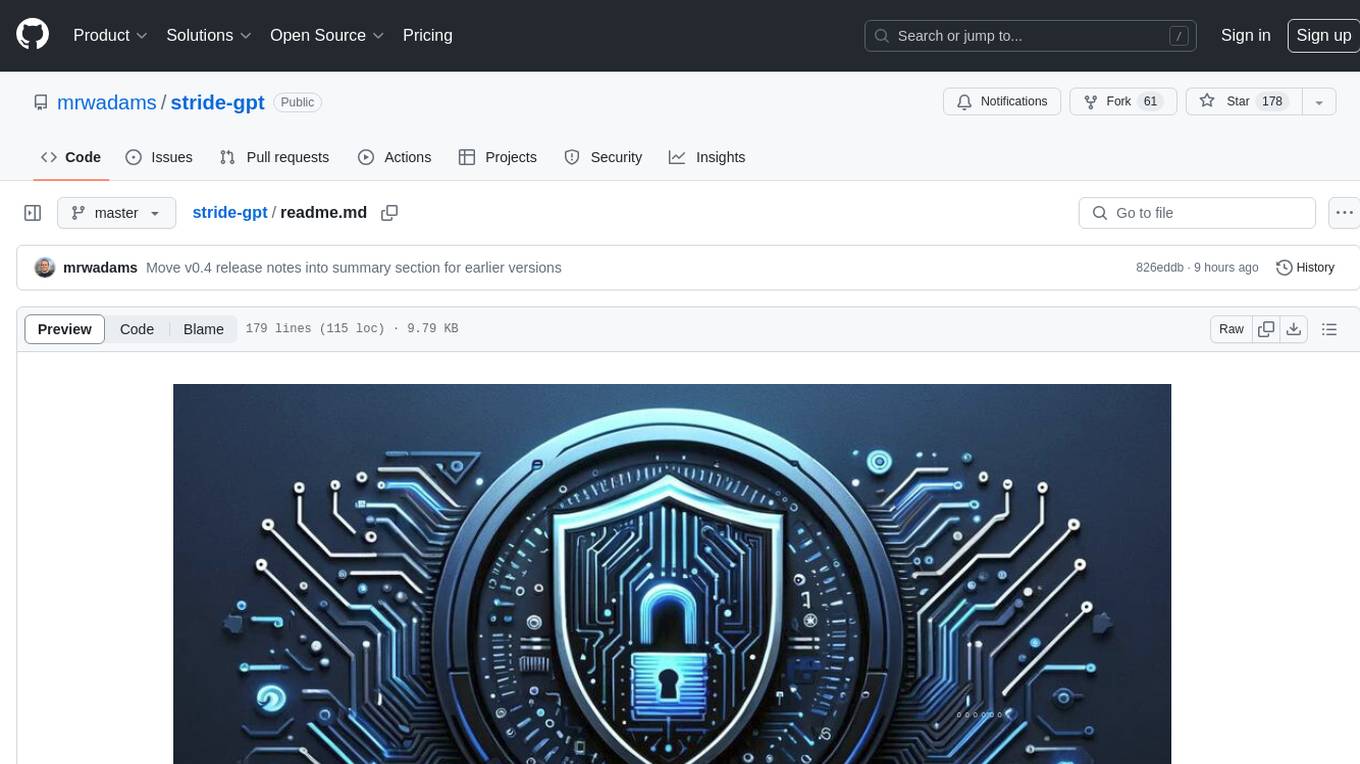
stride-gpt
STRIDE GPT is an AI-powered threat modelling tool that leverages Large Language Models (LLMs) to generate threat models and attack trees for a given application based on the STRIDE methodology. Users provide application details, such as the application type, authentication methods, and whether the application is internet-facing or processes sensitive data. The model then generates its output based on the provided information. It features a simple and user-friendly interface, supports multi-modal threat modelling, generates attack trees, suggests possible mitigations for identified threats, and does not store application details. STRIDE GPT can be accessed via OpenAI API, Azure OpenAI Service, Google AI API, or Mistral API. It is available as a Docker container image for easy deployment.
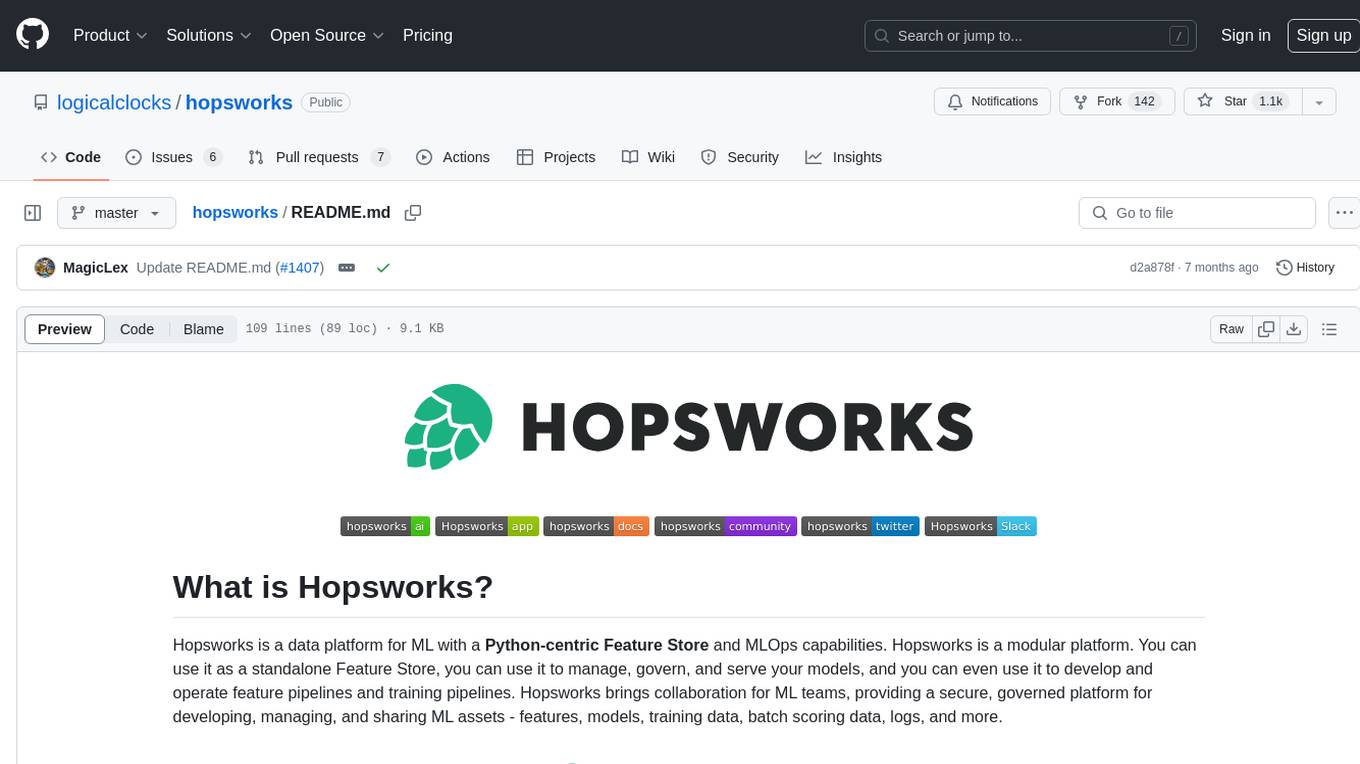
hopsworks
Hopsworks is a data platform for ML with a Python-centric Feature Store and MLOps capabilities. It provides collaboration for ML teams, offering a secure, governed platform for developing, managing, and sharing ML assets. Hopsworks supports project-based multi-tenancy, team collaboration, development tools for Data Science, and is available on any platform including managed cloud services and on-premise installations. The platform enables end-to-end responsibility from raw data to managed features and models, supports versioning, lineage, and provenance, and facilitates the complete MLOps life cycle.
For similar tasks
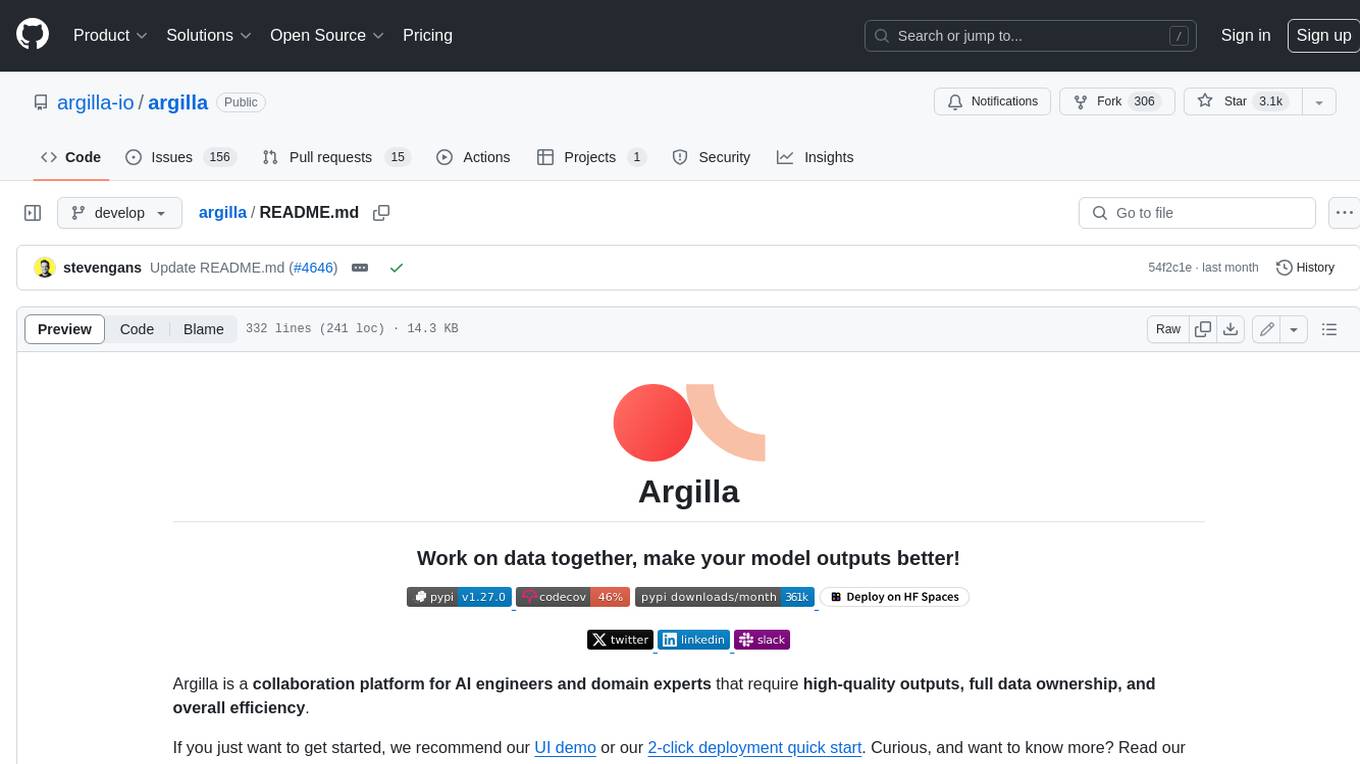
argilla
Argilla is a collaboration platform for AI engineers and domain experts that require high-quality outputs, full data ownership, and overall efficiency. It helps users improve AI output quality through data quality, take control of their data and models, and improve efficiency by quickly iterating on the right data and models. Argilla is an open-source community-driven project that provides tools for achieving and maintaining high-quality data standards, with a focus on NLP and LLMs. It is used by AI teams from companies like the Red Cross, Loris.ai, and Prolific to improve the quality and efficiency of AI projects.
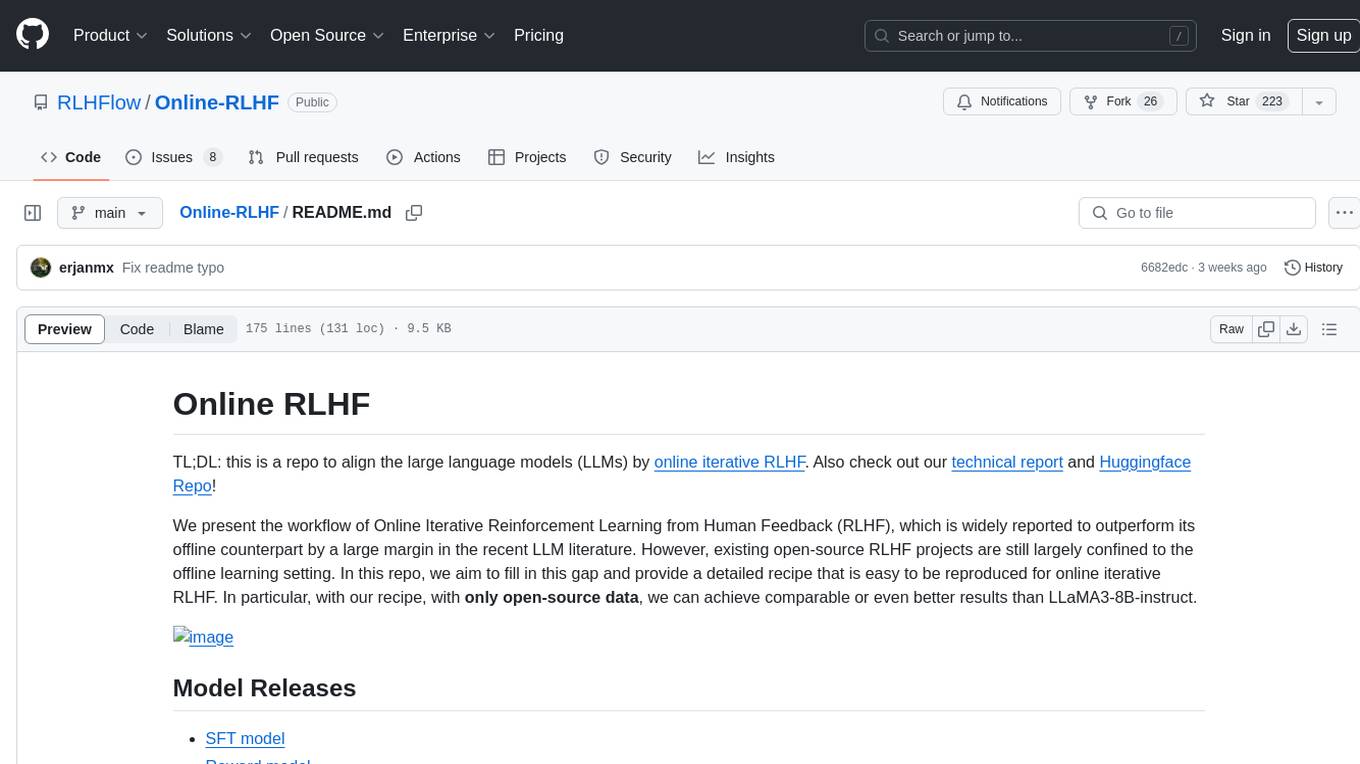
Online-RLHF
This repository, Online RLHF, focuses on aligning large language models (LLMs) through online iterative Reinforcement Learning from Human Feedback (RLHF). It aims to bridge the gap in existing open-source RLHF projects by providing a detailed recipe for online iterative RLHF. The workflow presented here has shown to outperform offline counterparts in recent LLM literature, achieving comparable or better results than LLaMA3-8B-instruct using only open-source data. The repository includes model releases for SFT, Reward model, and RLHF model, along with installation instructions for both inference and training environments. Users can follow step-by-step guidance for supervised fine-tuning, reward modeling, data generation, data annotation, and training, ultimately enabling iterative training to run automatically.
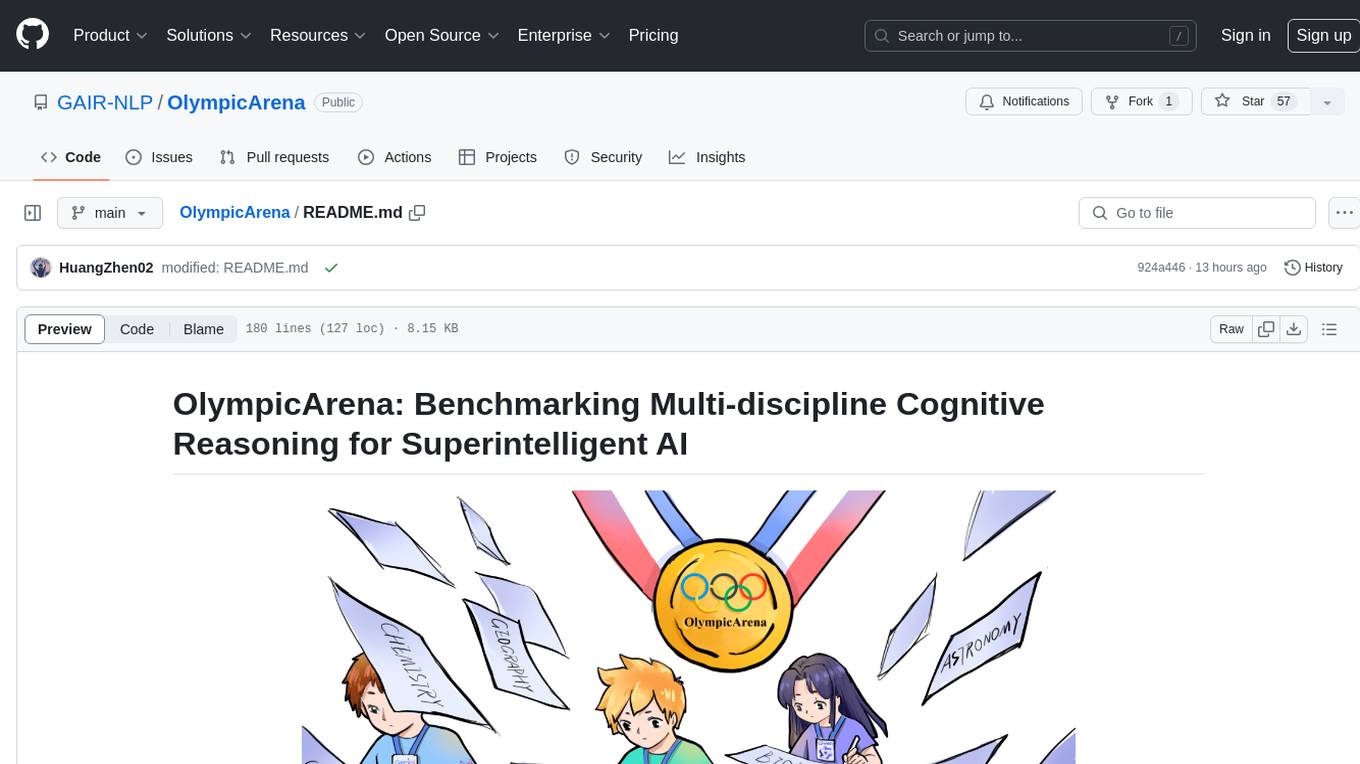
OlympicArena
OlympicArena is a comprehensive benchmark designed to evaluate advanced AI capabilities across various disciplines. It aims to push AI towards superintelligence by tackling complex challenges in science and beyond. The repository provides detailed data for different disciplines, allows users to run inference and evaluation locally, and offers a submission platform for testing models on the test set. Additionally, it includes an annotation interface and encourages users to cite their paper if they find the code or dataset helpful.
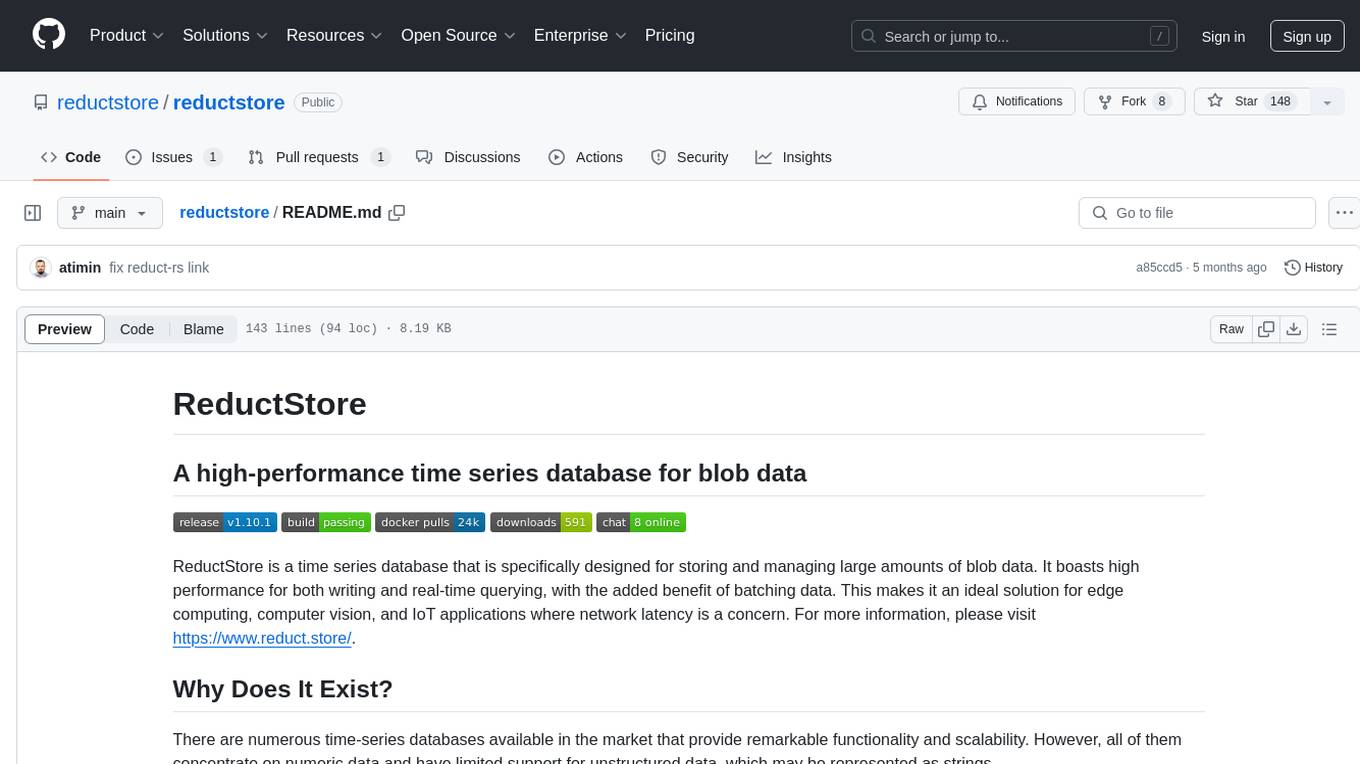
reductstore
ReductStore is a high-performance time series database designed for storing and managing large amounts of unstructured blob data. It offers features such as real-time querying, batching data, and HTTP(S) API for edge computing, computer vision, and IoT applications. The database ensures data integrity, implements retention policies, and provides efficient data access, making it a cost-effective solution for applications requiring unstructured data storage and access at specific time intervals.
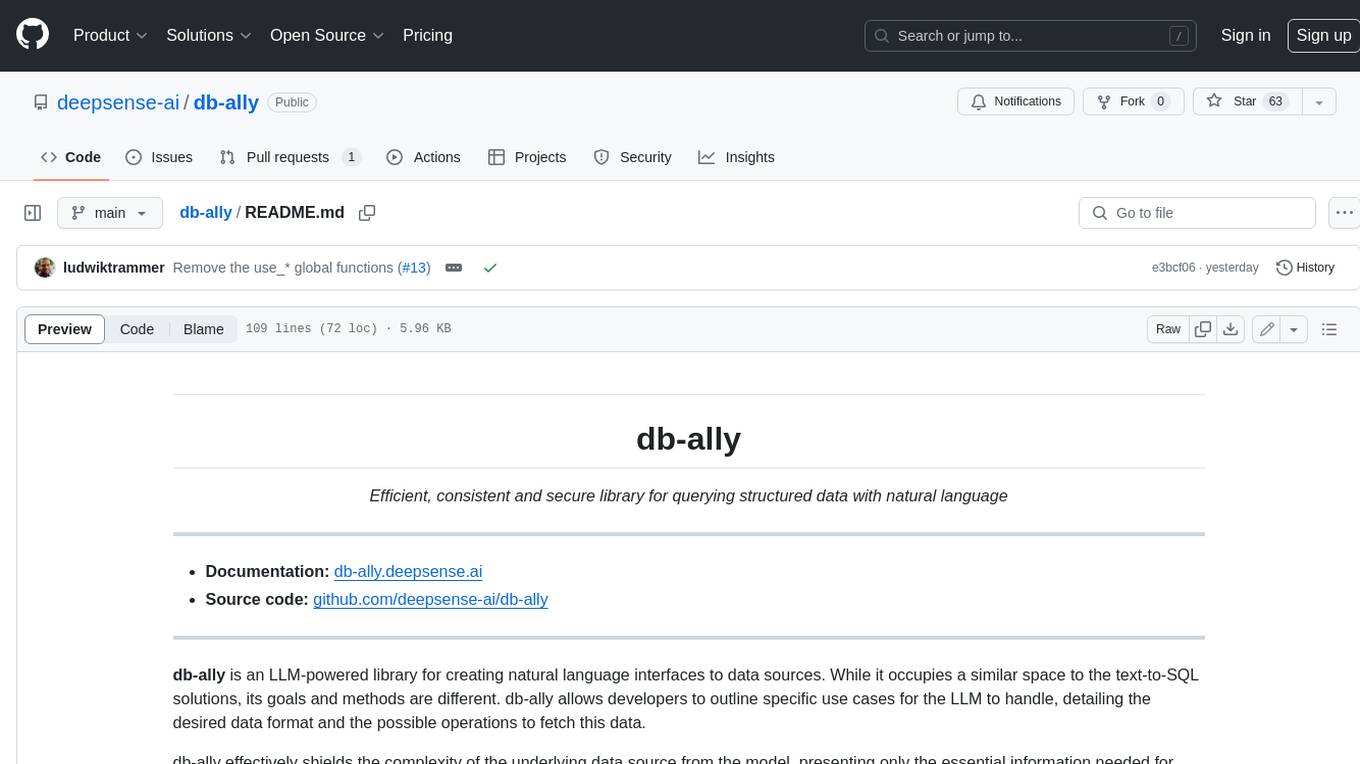
db-ally
db-ally is a library for creating natural language interfaces to data sources. It allows developers to outline specific use cases for a large language model (LLM) to handle, detailing the desired data format and the possible operations to fetch this data. db-ally effectively shields the complexity of the underlying data source from the model, presenting only the essential information needed for solving the specific use cases. Instead of generating arbitrary SQL, the model is asked to generate responses in a simplified query language.
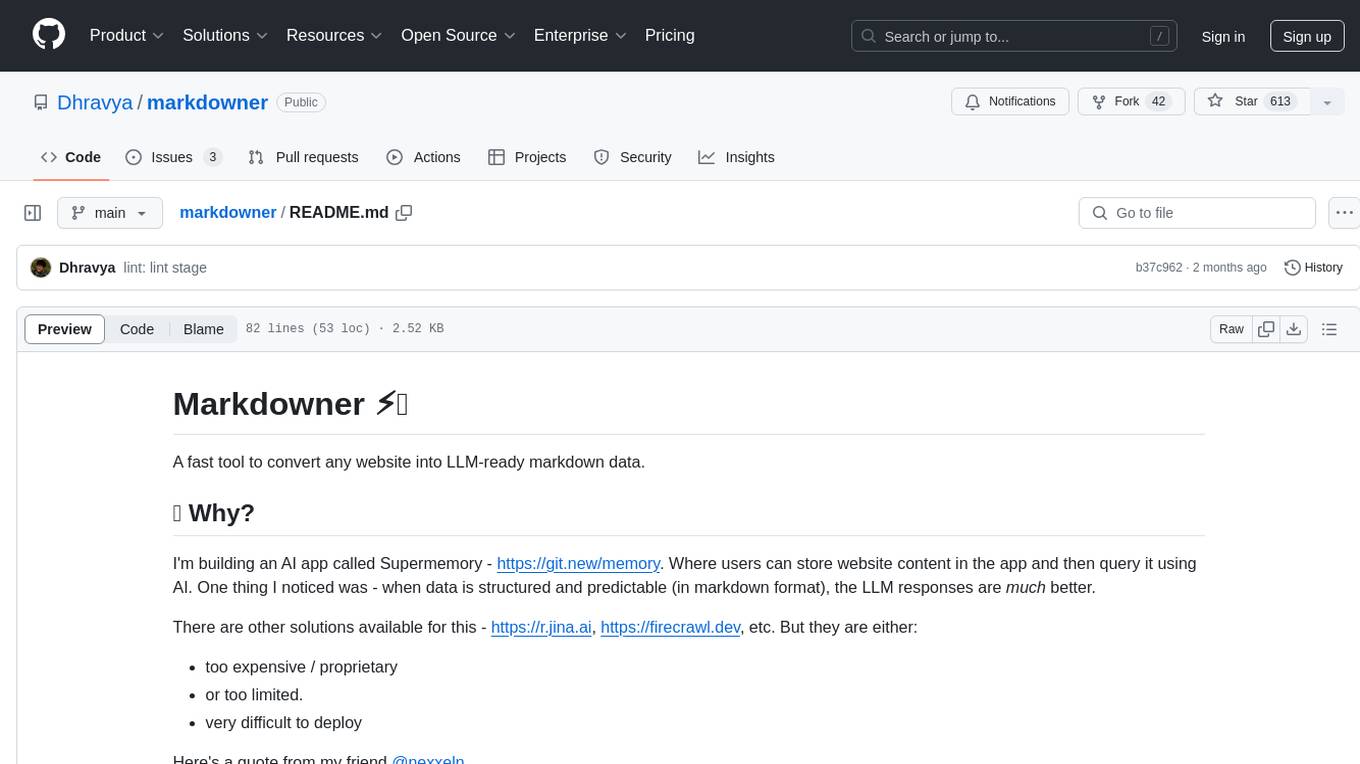
markdowner
Markdowner is a fast tool designed to convert any website into LLM-ready markdown data. It aims to improve the quality of responses in the AI app Supermemory by structuring and predicting data in markdown format. The tool offers features such as website conversion, LLM filtering, detailed markdown mode, auto crawler, text and JSON responses, and easy self-hosting. Markdowner utilizes Cloudflare's Browser rendering and Durable objects for browser instance creation and markdown conversion. Users can self-host the project with the Workers paid plan, following simple steps. Support the project by starring the repository.
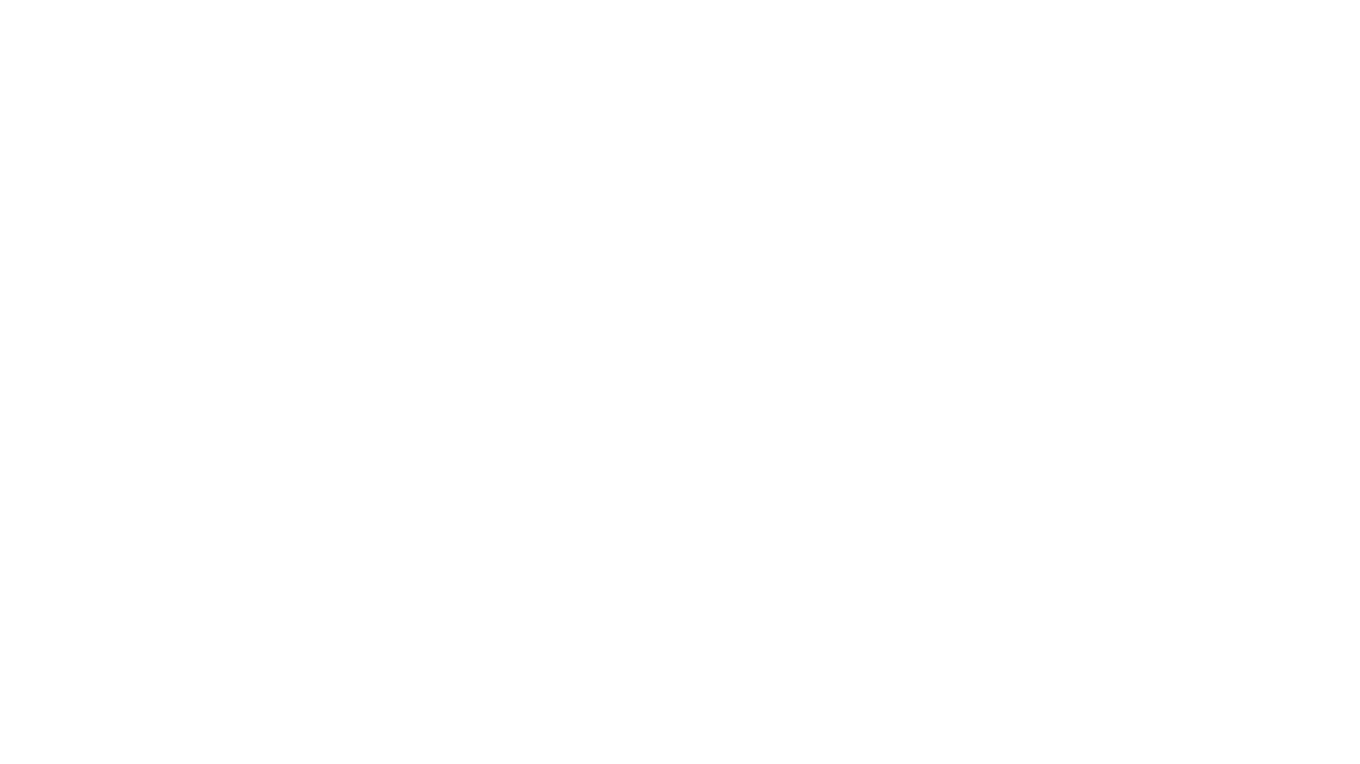
letsql
LETSQL is a data processing library built on top of Ibis and DataFusion to write multi-engine data workflows. It is currently in development and does not have a stable release. Users can install LETSQL from PyPI and use it to connect to data sources, read data, filter, group, and aggregate data for analysis. Contributions to the project are welcome, and the library is actively maintained with support available for any issues. LETSQL heavily relies on Ibis and DataFusion for its functionality.
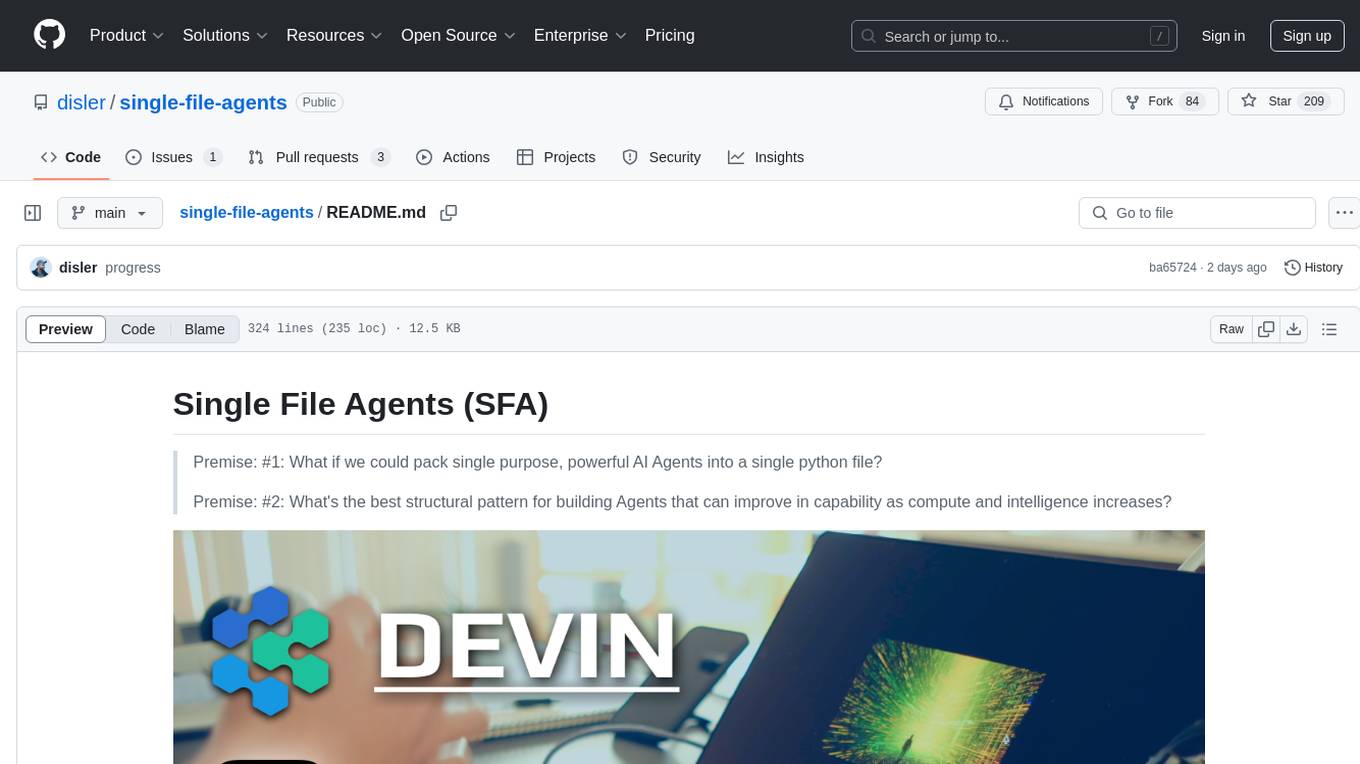
single-file-agents
Single File Agents (SFA) is a collection of powerful single-file agents built on top of uv, a modern Python package installer and resolver. These agents aim to perform specific tasks efficiently, demonstrating precise prompt engineering and GenAI patterns. The repository contains agents built across major GenAI providers like Gemini, OpenAI, and Anthropic. Each agent is self-contained, minimal, and built on modern Python for fast and reliable dependency management. Users can run these scripts from their server or directly from a gist. The agents are patternful, emphasizing the importance of setting up effective prompts, tools, and processes for reusability.
For similar jobs
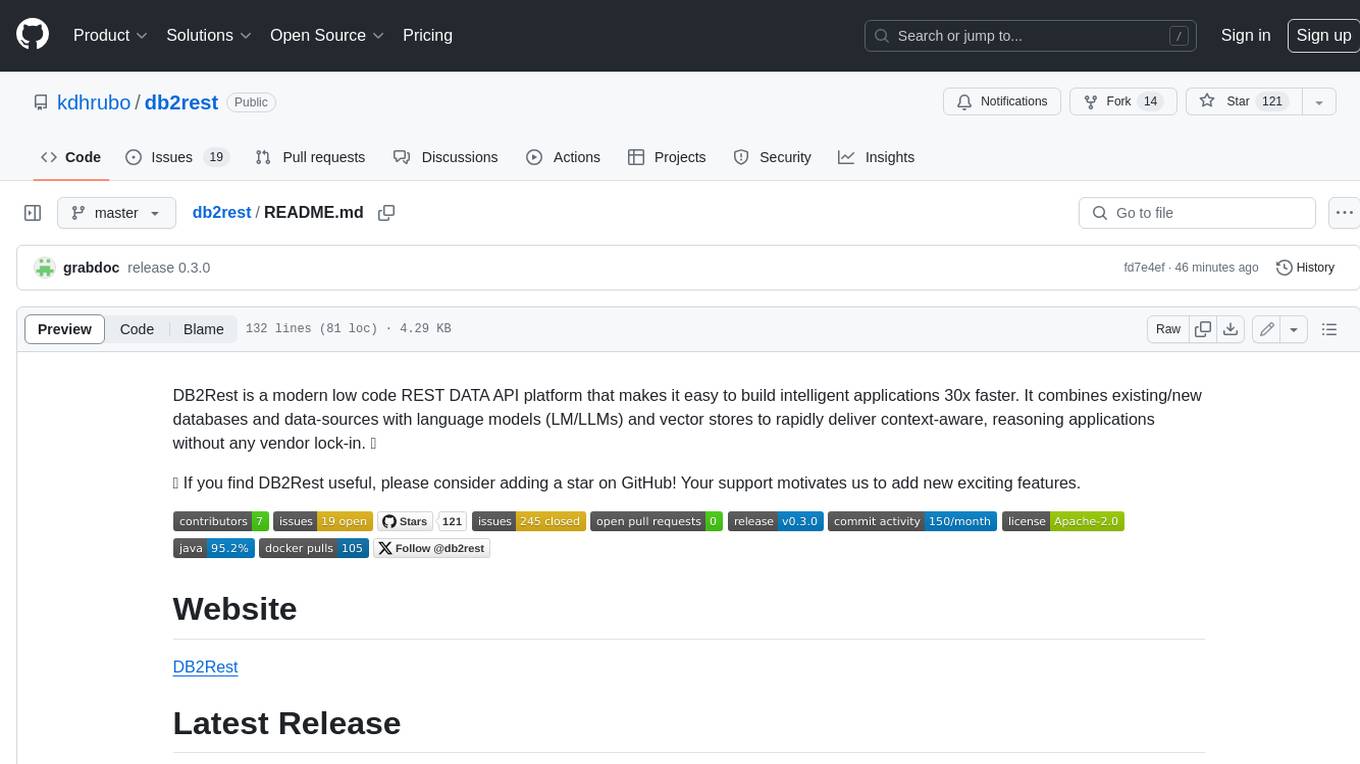
db2rest
DB2Rest is a modern low-code REST DATA API platform that simplifies the development of intelligent applications. It seamlessly integrates existing and new databases with language models (LMs/LLMs) and vector stores, enabling the rapid delivery of context-aware, reasoning applications without vendor lock-in.
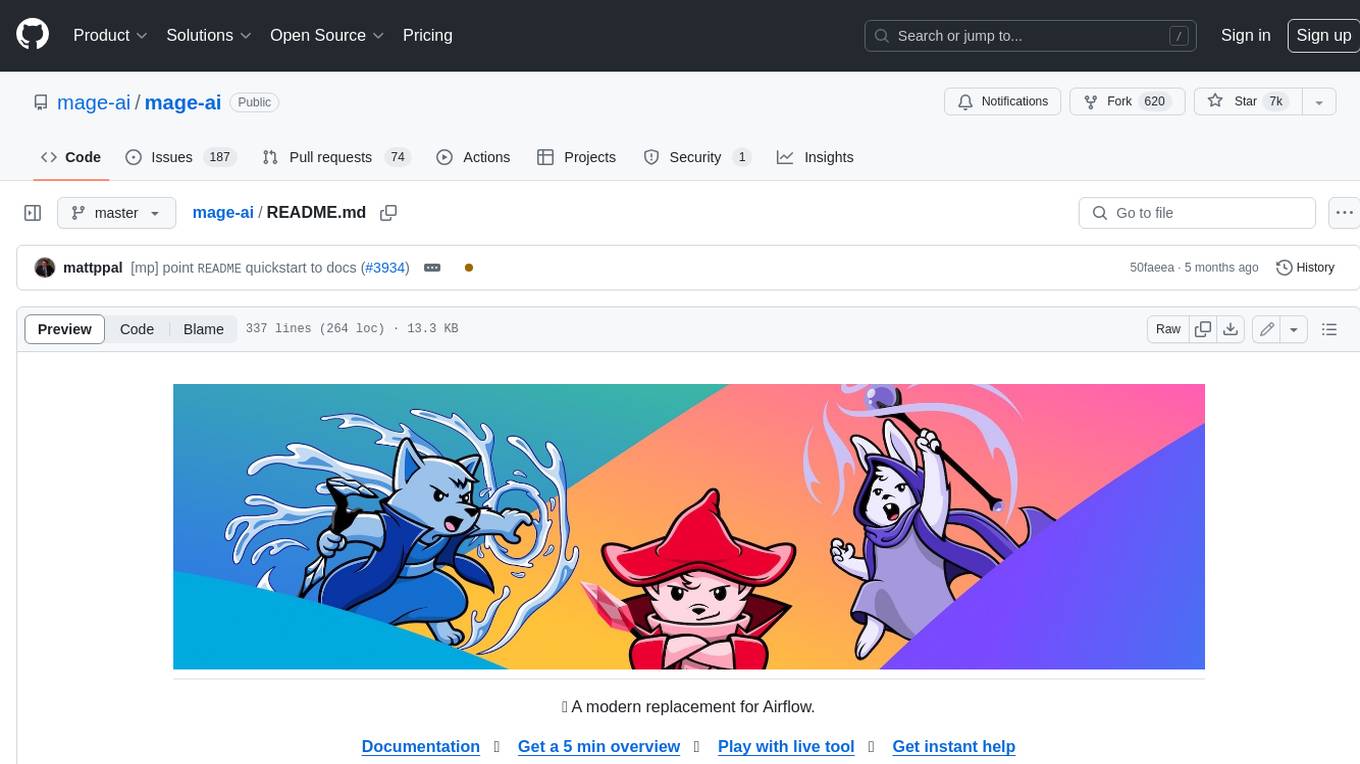
mage-ai
Mage is an open-source data pipeline tool for transforming and integrating data. It offers an easy developer experience, engineering best practices built-in, and data as a first-class citizen. Mage makes it easy to build, preview, and launch data pipelines, and provides observability and scaling capabilities. It supports data integrations, streaming pipelines, and dbt integration.
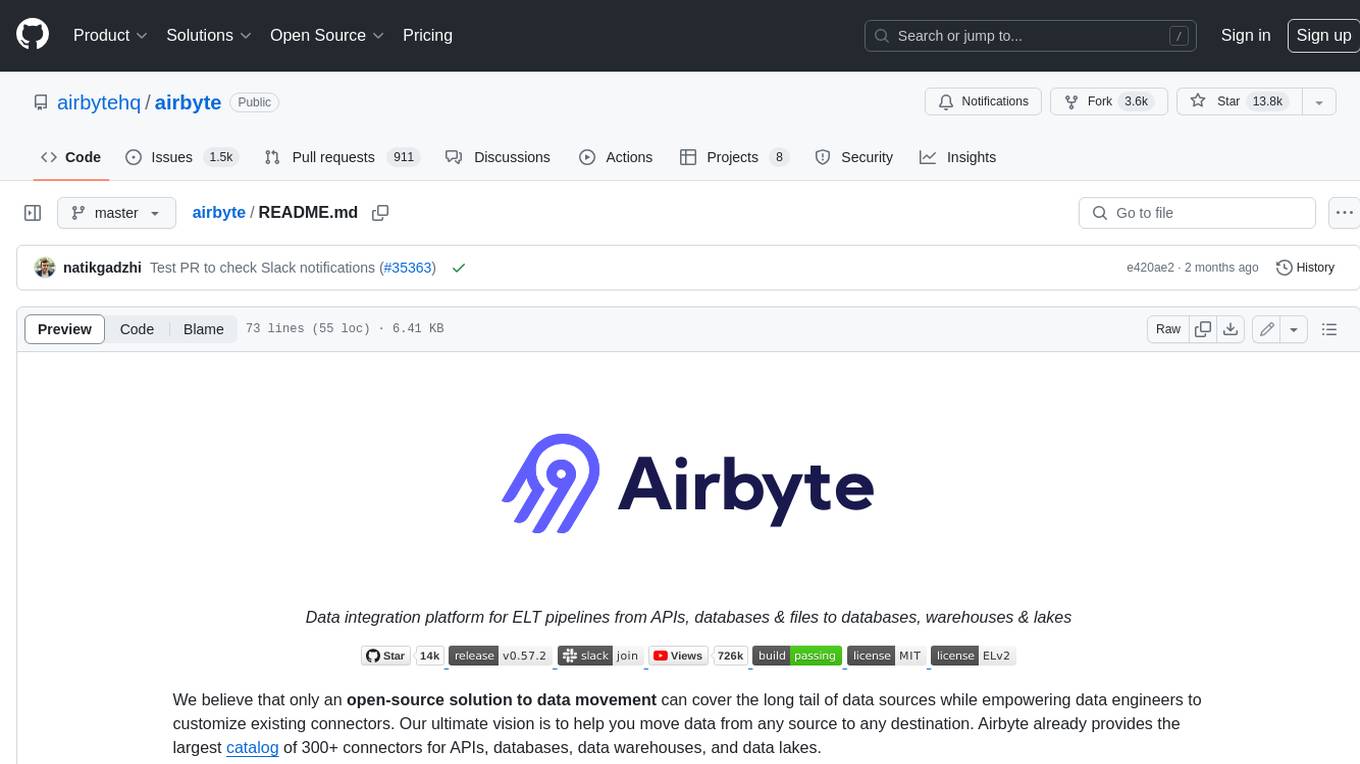
airbyte
Airbyte is an open-source data integration platform that makes it easy to move data from any source to any destination. With Airbyte, you can build and manage data pipelines without writing any code. Airbyte provides a library of pre-built connectors that make it easy to connect to popular data sources and destinations. You can also create your own connectors using Airbyte's no-code Connector Builder or low-code CDK. Airbyte is used by data engineers and analysts at companies of all sizes to build and manage their data pipelines.
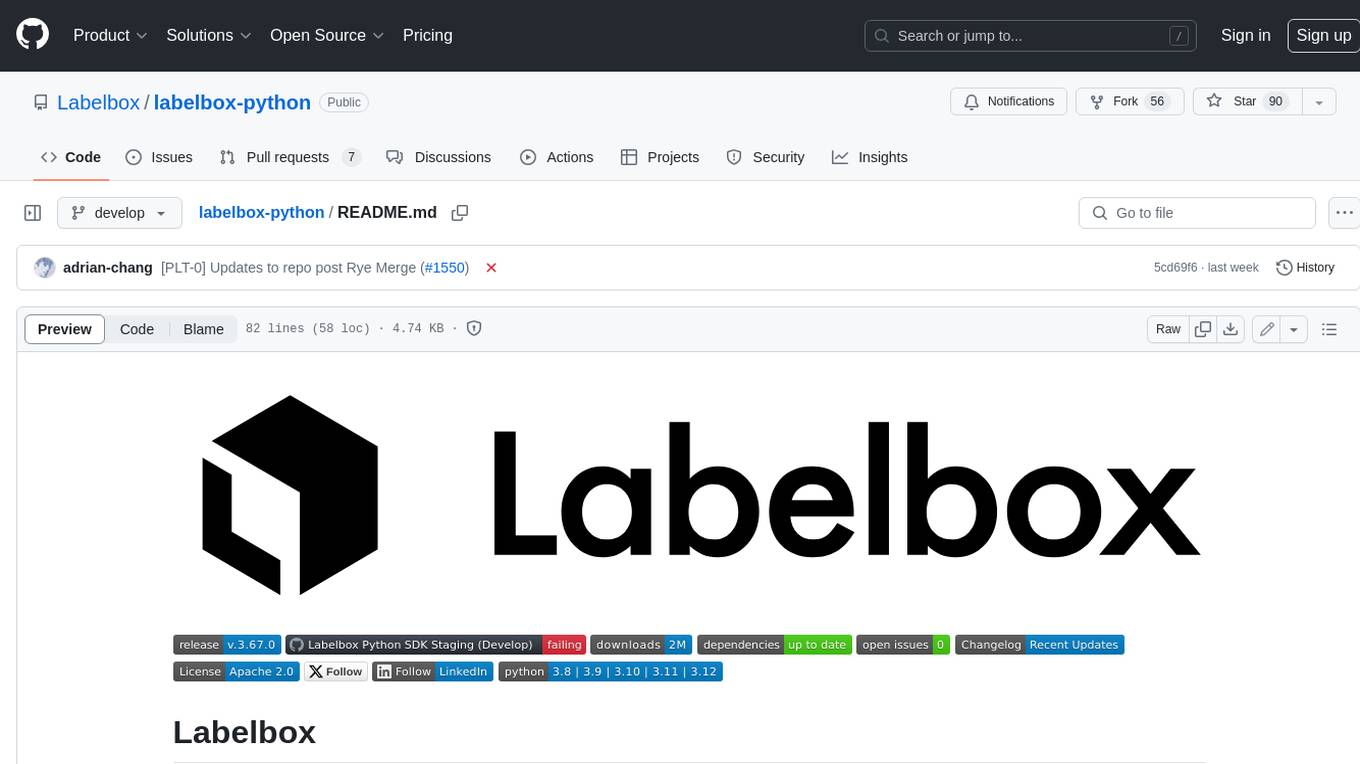
labelbox-python
Labelbox is a data-centric AI platform for enterprises to develop, optimize, and use AI to solve problems and power new products and services. Enterprises use Labelbox to curate data, generate high-quality human feedback data for computer vision and LLMs, evaluate model performance, and automate tasks by combining AI and human-centric workflows. The academic & research community uses Labelbox for cutting-edge AI research.
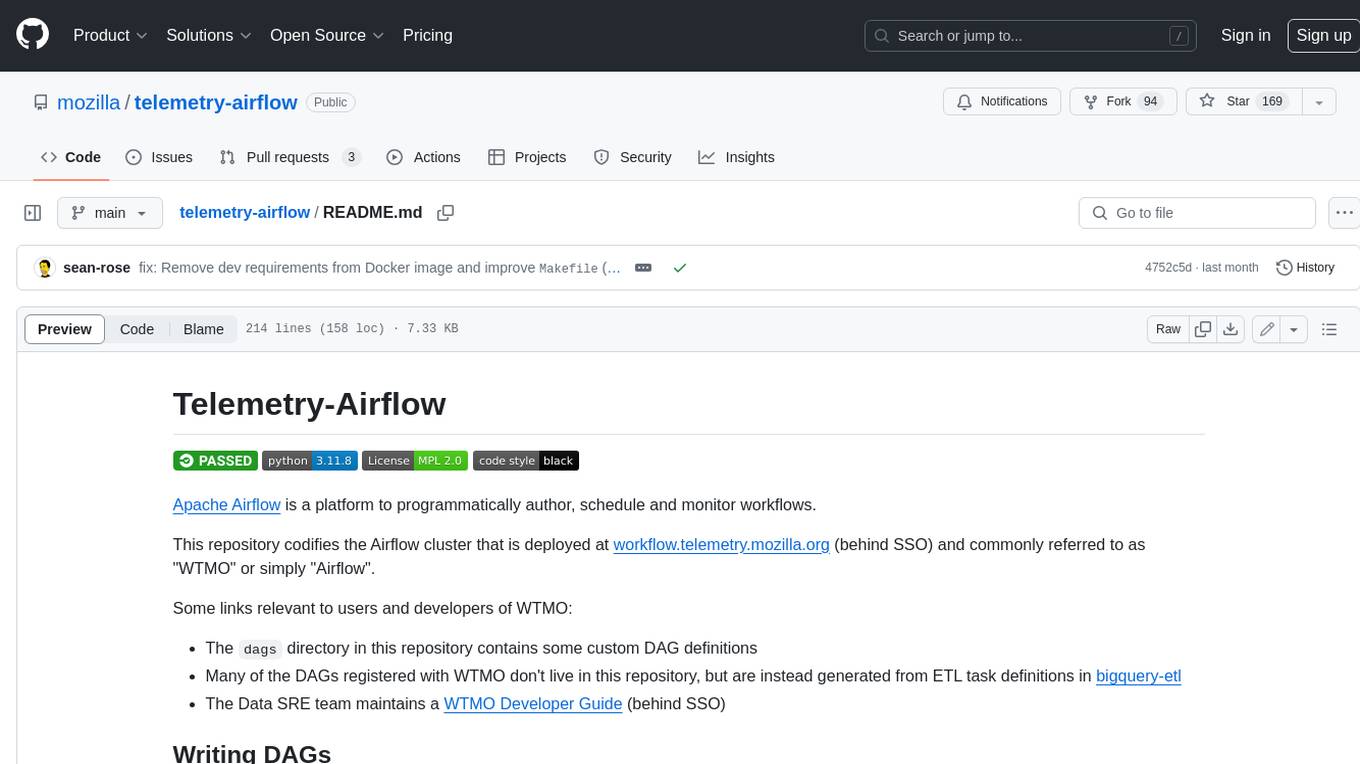
telemetry-airflow
This repository codifies the Airflow cluster that is deployed at workflow.telemetry.mozilla.org (behind SSO) and commonly referred to as "WTMO" or simply "Airflow". Some links relevant to users and developers of WTMO: * The `dags` directory in this repository contains some custom DAG definitions * Many of the DAGs registered with WTMO don't live in this repository, but are instead generated from ETL task definitions in bigquery-etl * The Data SRE team maintains a WTMO Developer Guide (behind SSO)
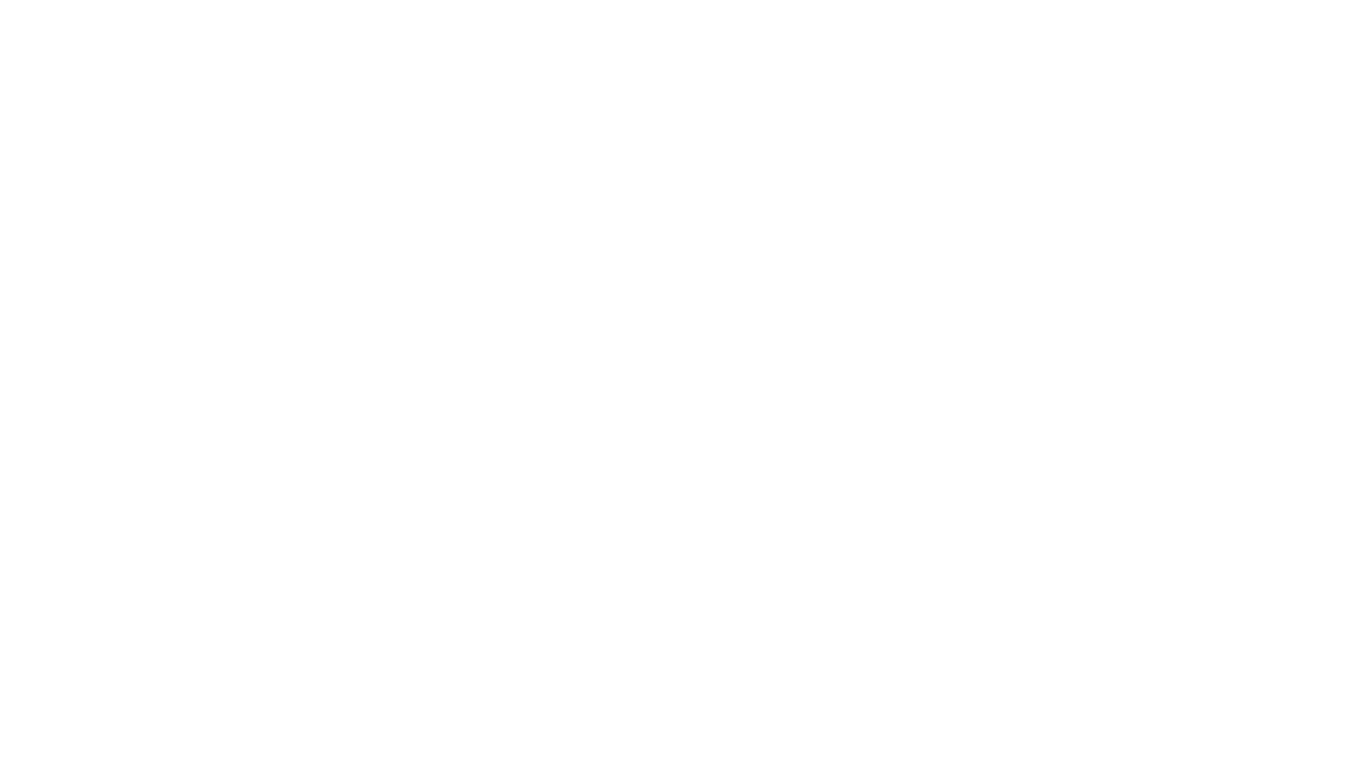
airflow
Apache Airflow (or simply Airflow) is a platform to programmatically author, schedule, and monitor workflows. When workflows are defined as code, they become more maintainable, versionable, testable, and collaborative. Use Airflow to author workflows as directed acyclic graphs (DAGs) of tasks. The Airflow scheduler executes your tasks on an array of workers while following the specified dependencies. Rich command line utilities make performing complex surgeries on DAGs a snap. The rich user interface makes it easy to visualize pipelines running in production, monitor progress, and troubleshoot issues when needed.
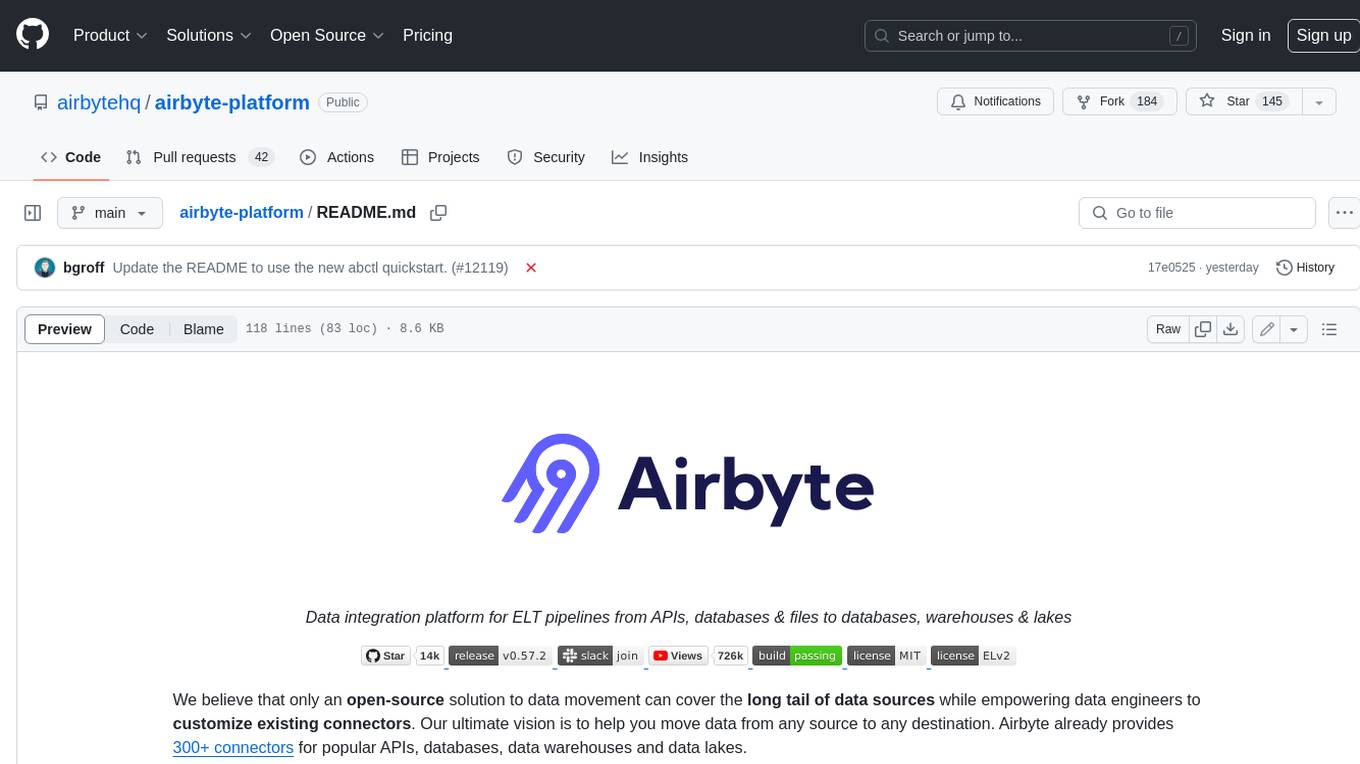
airbyte-platform
Airbyte is an open-source data integration platform that makes it easy to move data from any source to any destination. With Airbyte, you can build and manage data pipelines without writing any code. Airbyte provides a library of pre-built connectors that make it easy to connect to popular data sources and destinations. You can also create your own connectors using Airbyte's low-code Connector Development Kit (CDK). Airbyte is used by data engineers and analysts at companies of all sizes to move data for a variety of purposes, including data warehousing, data analysis, and machine learning.
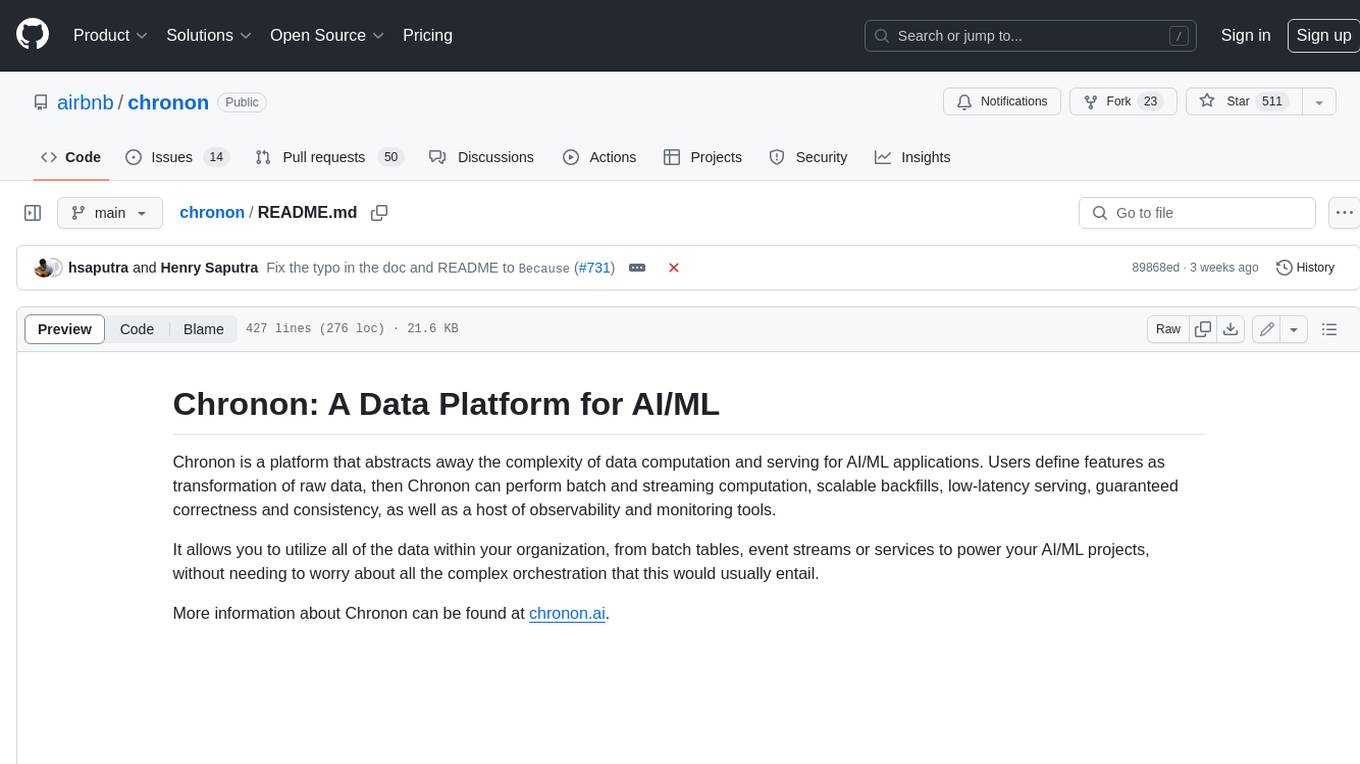
chronon
Chronon is a platform that simplifies and improves ML workflows by providing a central place to define features, ensuring point-in-time correctness for backfills, simplifying orchestration for batch and streaming pipelines, offering easy endpoints for feature fetching, and guaranteeing and measuring consistency. It offers benefits over other approaches by enabling the use of a broad set of data for training, handling large aggregations and other computationally intensive transformations, and abstracting away the infrastructure complexity of data plumbing.