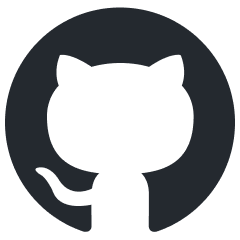
OlympicArena
This is the official repository of the paper "OlympicArena: Benchmarking Multi-discipline Cognitive Reasoning for Superintelligent AI"
Stars: 74
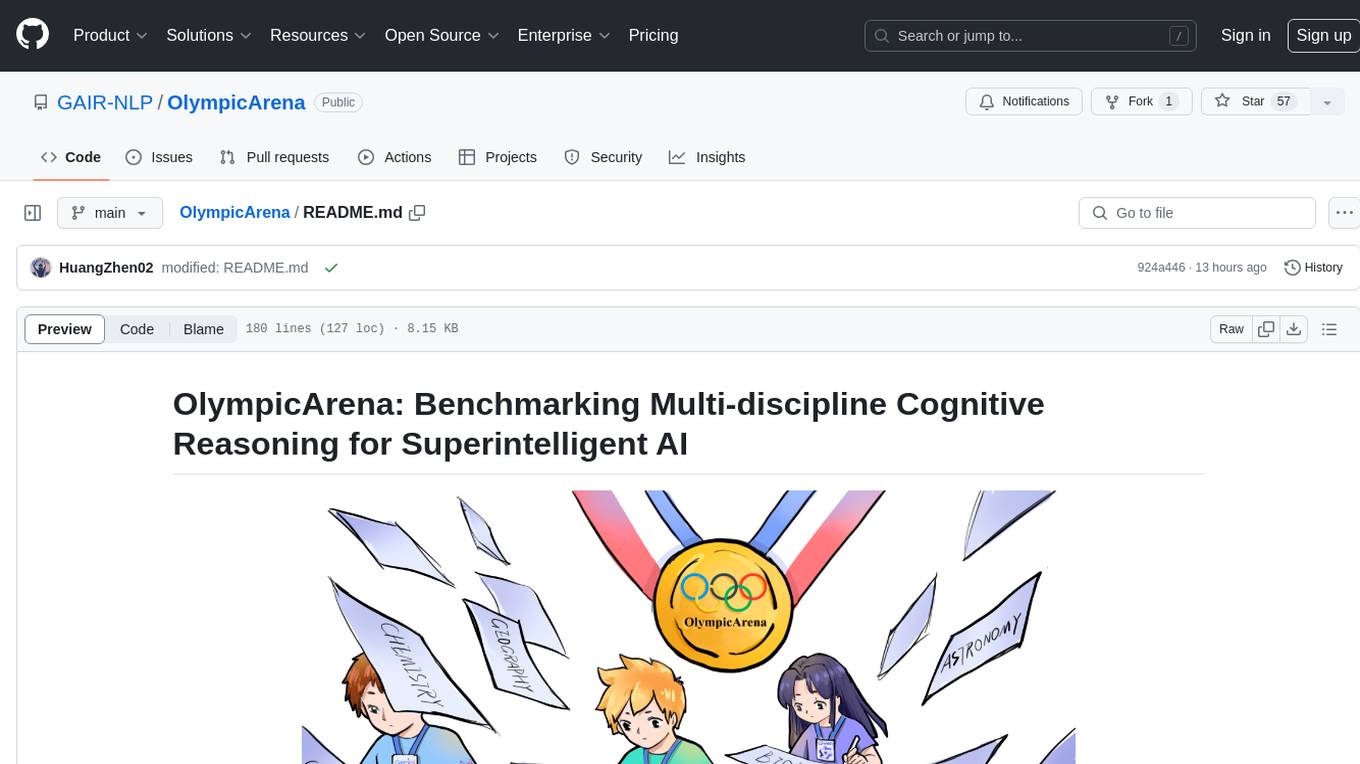
OlympicArena is a comprehensive benchmark designed to evaluate advanced AI capabilities across various disciplines. It aims to push AI towards superintelligence by tackling complex challenges in science and beyond. The repository provides detailed data for different disciplines, allows users to run inference and evaluation locally, and offers a submission platform for testing models on the test set. Additionally, it includes an annotation interface and encourages users to cite their paper if they find the code or dataset helpful.
README:
📄 Paper | 🤗 Hugging Face | 🌐 Website | 📤 Submit | 📘 机器之心
- [2024/07/12] We have updated our submission platform. Firstly, the entire submission process is now anonymous; only the user can see their submitted scores, and they will not be visible to others. Secondly, we now support submissions via the command line.
- [2024/06/27] We add the result of the Deepseek-Coder-V2 model to the leaderboard, and it demonstrates outstanding performance in Maths and CS!
- [2024/06/26] We test ByteDance's Doubao-pro-32k model and find that it achieves remarkably high scores on Chinese problems.
- [2024/06/24] A brand new technical report comparing the performance of Claude-3.5-Sonnet and GPT-4o on OlympicArena has been released at this link and 机器之心.
- [2024/06/22] We test the latest Gemini-1.5-Pro and Claude-3.5-Sonnet models and add them to the leaderboard.
- [2024/06/20] Our work is featured by 机器之心 on Wechat!
OlympicArena is a comprehensive, highly-challenging, and rigorously curated benchmark featuring a detailed, fine-grained evaluation mechanism designed to assess advanced AI capabilities across a broad spectrum of Olympic-level challenges. We aim to advance AI towards superintelligence, equipping it to address more complex challenges in science and beyond.
To begin using the OlympicArena benchmark, you need to install the required dependencies. You can do this by running the following command:
git clone https://github.com/GAIR-NLP/OlympicArena.git
pip install -r requirements.txt
If you need to define your own model for inference or evaluation, you will also need to install any additional packages required by your model (e.g., transformers).
We have released the data for seven disciplines on Hugging Face. Each discipline is divided into val and test splits. The val split includes the answers for small-scale testing, while the answers for the test split are not publicly available. You can submit your results to our platform for evaluation (refer to Submit your result).
Loading the data is very simple. You can use the following code snippet:
from datasets import load_dataset
# Load the dataset for a specific discipline, e.g., Math
dataset = load_dataset("GAIR/OlympicArena", "Math", split="val")
print(dataset[0])
Each data entry contains the following fields:
-
id
: The unique identifier for each problem -
problem
: The problem statement -
prompt
: The prompt used as input to the model (as used in the paper); we also encourage users to try their own prompts -
figure_urls
: Links to images that appear in the problem, in order -
answer
: The answer to the problem -
answer_type
: The type of the answer -
unit
: The unit corresponding to the answer -
answer_sequence
: The sequence in which the model should provide answers if multiple quantities are required -
type_sequence
: The sequence ofanswer_type
for each quantity if multiple quantities are required -
test_cases
: Test cases used for evaluation in CS code generation problems -
subject
: The subject of the problem -
language
: The language of the problem, where EN represents English and ZH represents Chinese -
modality
: The modality type of the problem statement, wheretext-only
indicates the problem statement does not contain images, andmulti-modal
indicates the problem statement contains images
If you only want to use a specific subset of our dataset (e.g., only English problems, or only text-only problems), you just need to modify the load_data code snippet (./code/utils.py):
def load_data(hf_data_path, split, language=None, modality=None):
subjects = ["Math", "Physics", "Chemistry", "Biology", "Geography", "Astronomy", "CS"]
datasets = []
for subject in subjects:
dataset = load_dataset(hf_data_path, subject, split=split)
if language:
dataset = dataset.filter(lambda x: x['language'] == language)
if modality:
dataset = dataset.filter(lambda x: x['modality'] == modality)
datasets.append(dataset)
return concatenate_datasets(datasets)
To run inference, first navigate to the code directory:
cd code
Then, execute the following command to run the inference script:
python inference.py \
--hf_data_path GAIR/OlympicArena \
--model_output_dir ./model_output/ \
--split val \
--model gpt-4o \
--batch 15 \
--api_key YOUR_API_KEY \
--base_url YOUR_BASE_URL \
--save_error
-
--hf_data_path
: Path to the Hugging Face dataset (default: "GAIR/OlympicArena") -
--model_output_dir
: Directory to save the model output (default: "./model_output/") -
--split
: Dataset split to use for inference, either "val" or "test" -
--model
: Model name to use for inference -
--batch
: Batch size for inference -
--api_key
: Your API key, if required -
--base_url
: Base URL for the API, if required -
--save_error
: Save errors as None (default: False). If set, any problem that fails or encounters an error during inference will be saved with an answer ofNone
.
After inference is complete, a JSON file containing the inference outputs will be generated in model_output_dir/model
, which can be used for evaluation.
If you want to use your own model for inference, you can enter the model
folder and define your model as a subclass of BaseModel
.
You can only run evaluation locally on the val set (because the answers for the test set are not publicly available). First, ensure that the corresponding JSON file representing the inference outputs is generated in the inference step.
Then you can execute the following script from the command line:
python evaluation.py \
--hf_data_path GAIR/OlympicArena \
--model_output_dir ./model_output/ \
--result_dir ./result/ \
--split val \
--model gpt-4o
Finally, we will print the overall accuracy, accuracy for different subjects, accuracy for different languages, and accuracy for different modalities.
If you want to test your own model's performance on the test set, you can use our submit platform.
Two different submission methods are supported:
- Direct Submission on the Platform:
-
Go to the submission platform.
-
Click "login in with Hugging Face" on the competition page then upload your result file.
- Command Line Submission:
-
First, install the required library:
pip install git+https://github.com/huggingface/competitions
-
Then use the following command:
competitions submit --competition_id GAIR/OlympicArenaSubmission --submission YOUR_RESULT_JSON_PATH --comment COMMENT --token YOUR_HF_READ_TOKEN
Detailed format instructions are available on the submission platform. We strongly recommend using the code framework provided in this repository for inference, as it will generate the required JSON file that can be directly submitted to the platform.
We also provide the data annotation interface used in this work for reference or use. You can check the details by navigating to the annotation
directory.
cd annotation
If you encounter any question about our work, please do not hesitate to submit an issue or directly contact us via email [email protected].
If you do find our code helpful or use our benchmark dataset, please citing our paper.
@article{huang2024olympicarena,
title={OlympicArena: Benchmarking Multi-discipline Cognitive Reasoning for Superintelligent AI},
author={Zhen Huang and Zengzhi Wang and Shijie Xia and Xuefeng Li and Haoyang Zou and Ruijie Xu and Run-Ze Fan and Lyumanshan Ye and Ethan Chern and Yixin Ye and Yikai Zhang and Yuqing Yang and Ting Wu and Binjie Wang and Shichao Sun and Yang Xiao and Yiyuan Li and Fan Zhou and Steffi Chern and Yiwei Qin and Yan Ma and Jiadi Su and Yixiu Liu and Yuxiang Zheng and Shaoting Zhang and Dahua Lin and Yu Qiao and Pengfei Liu},
year={2024},
journal={arXiv preprint arXiv:2406.12753},
url={https://arxiv.org/abs/2406.12753}
}
For Tasks:
Click tags to check more tools for each tasksFor Jobs:
Alternative AI tools for OlympicArena
Similar Open Source Tools
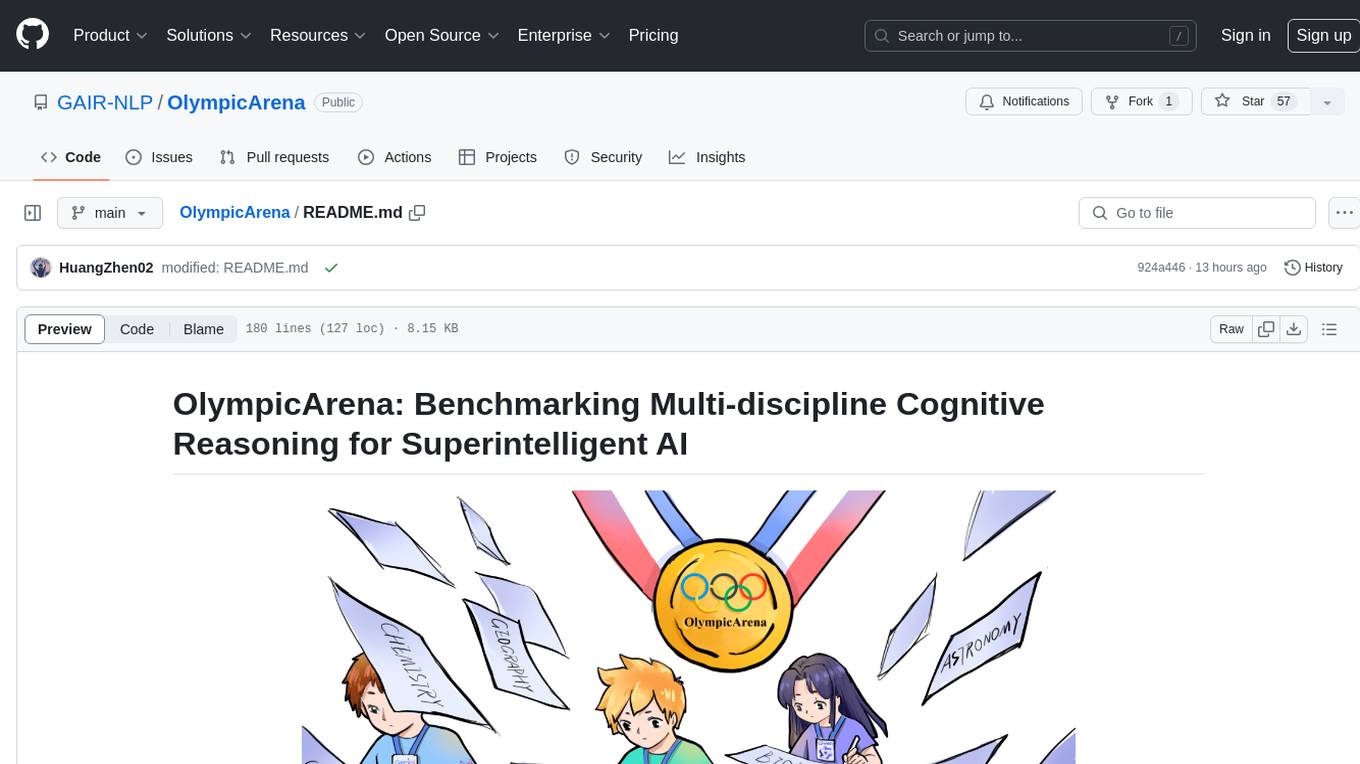
OlympicArena
OlympicArena is a comprehensive benchmark designed to evaluate advanced AI capabilities across various disciplines. It aims to push AI towards superintelligence by tackling complex challenges in science and beyond. The repository provides detailed data for different disciplines, allows users to run inference and evaluation locally, and offers a submission platform for testing models on the test set. Additionally, it includes an annotation interface and encourages users to cite their paper if they find the code or dataset helpful.
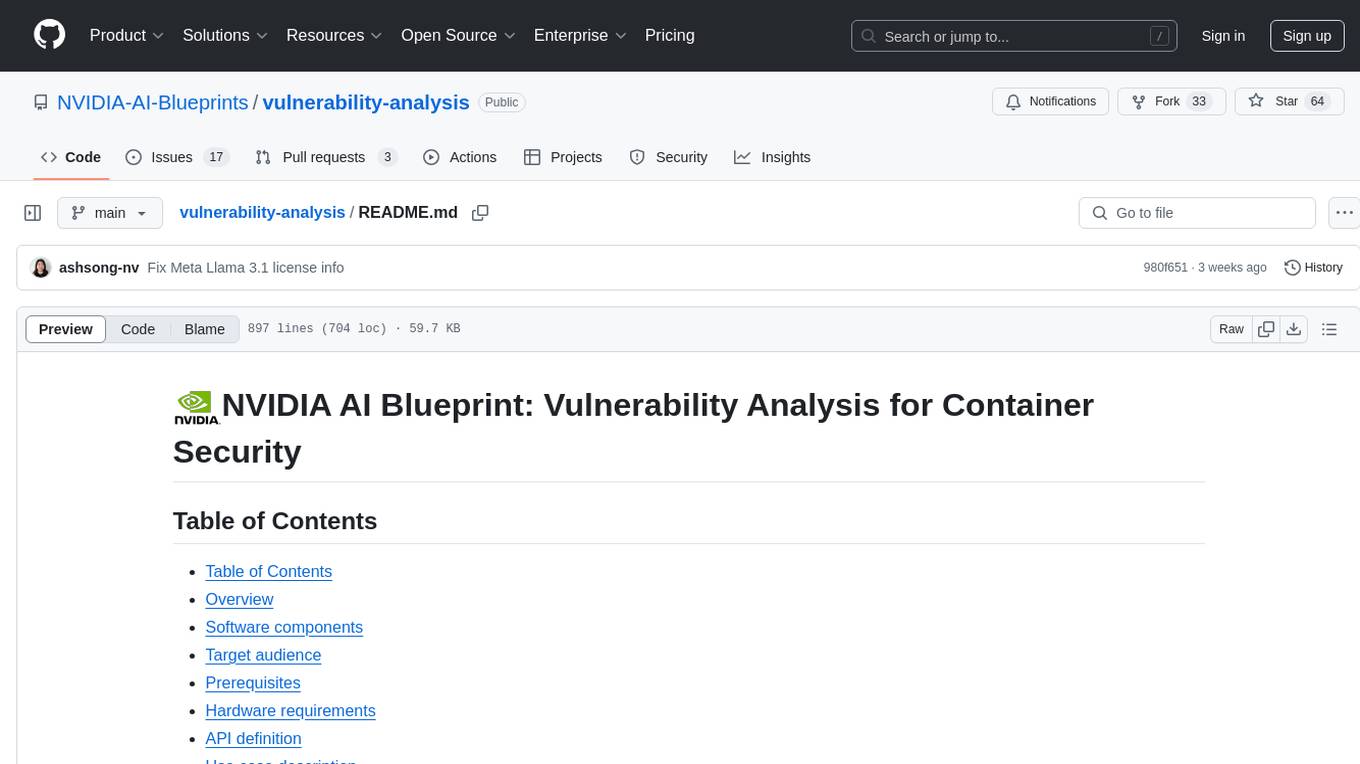
vulnerability-analysis
The NVIDIA AI Blueprint for Vulnerability Analysis for Container Security showcases accelerated analysis on common vulnerabilities and exposures (CVE) at an enterprise scale, reducing mitigation time from days to seconds. It enables security analysts to determine software package vulnerabilities using large language models (LLMs) and retrieval-augmented generation (RAG). The blueprint is designed for security analysts, IT engineers, and AI practitioners in cybersecurity. It requires NVAIE developer license and API keys for vulnerability databases, search engines, and LLM model services. Hardware requirements include L40 GPU for pipeline operation and optional LLM NIM and Embedding NIM. The workflow involves LLM pipeline for CVE impact analysis, utilizing LLM planner, agent, and summarization nodes. The blueprint uses NVIDIA NIM microservices and Morpheus Cybersecurity AI SDK for vulnerability analysis.
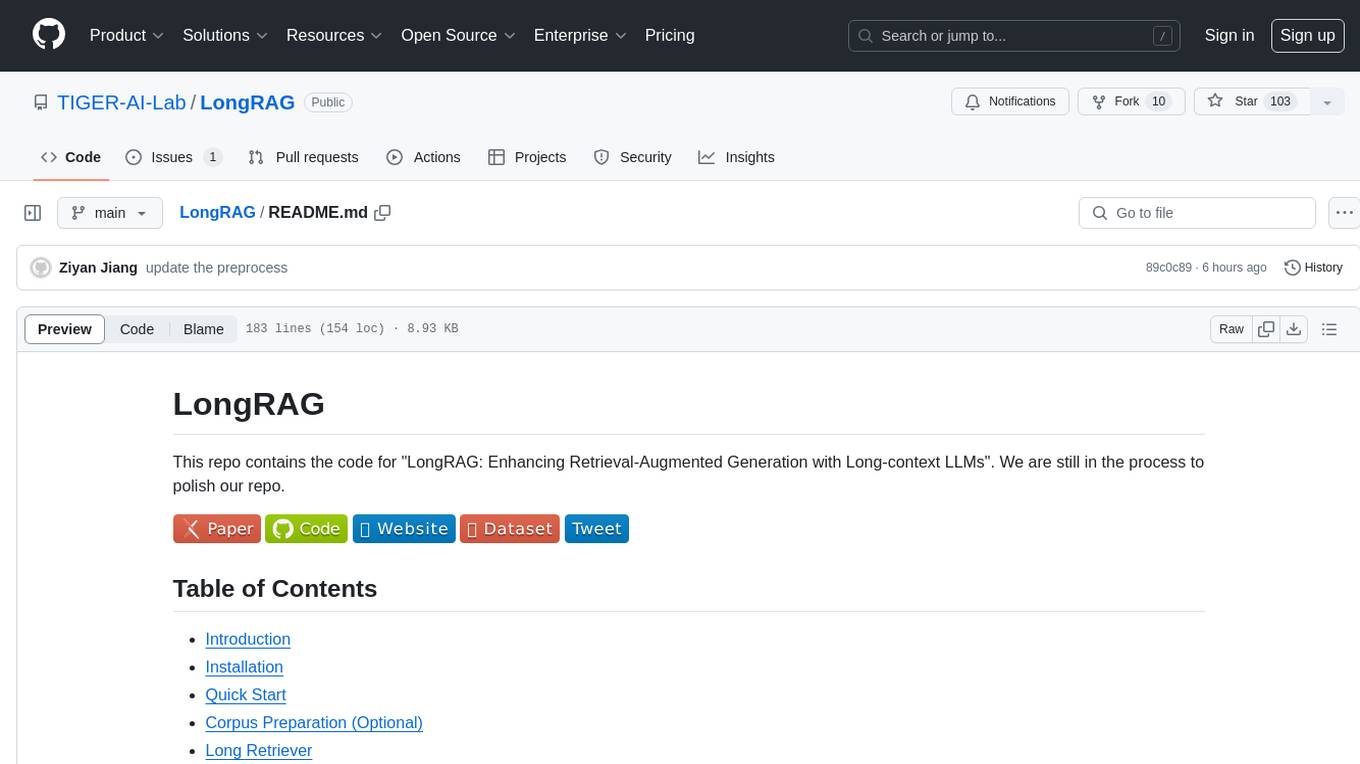
LongRAG
This repository contains the code for LongRAG, a framework that enhances retrieval-augmented generation with long-context LLMs. LongRAG introduces a 'long retriever' and a 'long reader' to improve performance by using a 4K-token retrieval unit, offering insights into combining RAG with long-context LLMs. The repo provides instructions for installation, quick start, corpus preparation, long retriever, and long reader.
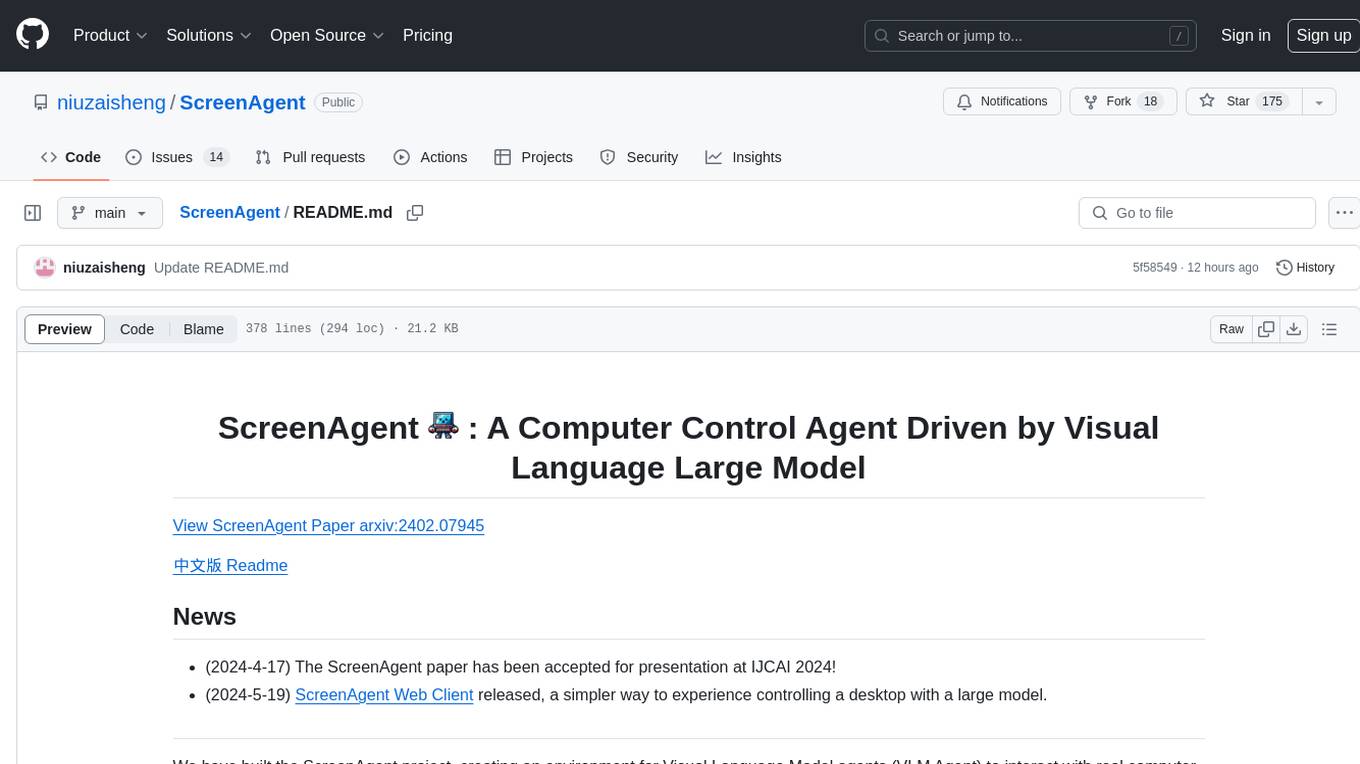
ScreenAgent
ScreenAgent is a project focused on creating an environment for Visual Language Model agents (VLM Agent) to interact with real computer screens. The project includes designing an automatic control process for agents to interact with the environment and complete multi-step tasks. It also involves building the ScreenAgent dataset, which collects screenshots and action sequences for various daily computer tasks. The project provides a controller client code, configuration files, and model training code to enable users to control a desktop with a large model.
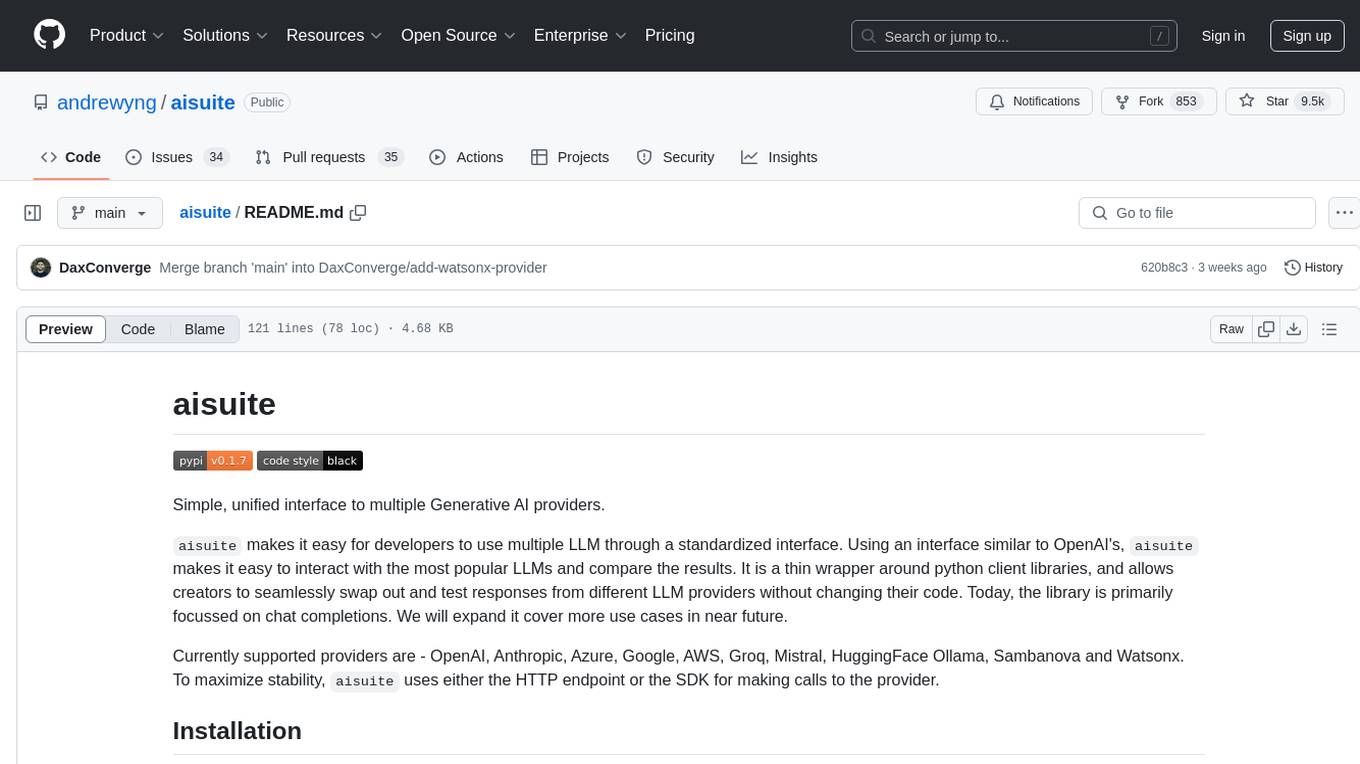
aisuite
Aisuite is a simple, unified interface to multiple Generative AI providers. It allows developers to easily interact with various Language Model (LLM) providers like OpenAI, Anthropic, Azure, Google, AWS, and more through a standardized interface. The library focuses on chat completions and provides a thin wrapper around python client libraries, enabling creators to test responses from different LLM providers without changing their code. Aisuite maximizes stability by using HTTP endpoints or SDKs for making calls to the providers. Users can install the base package or specific provider packages, set up API keys, and utilize the library to generate chat completion responses from different models.
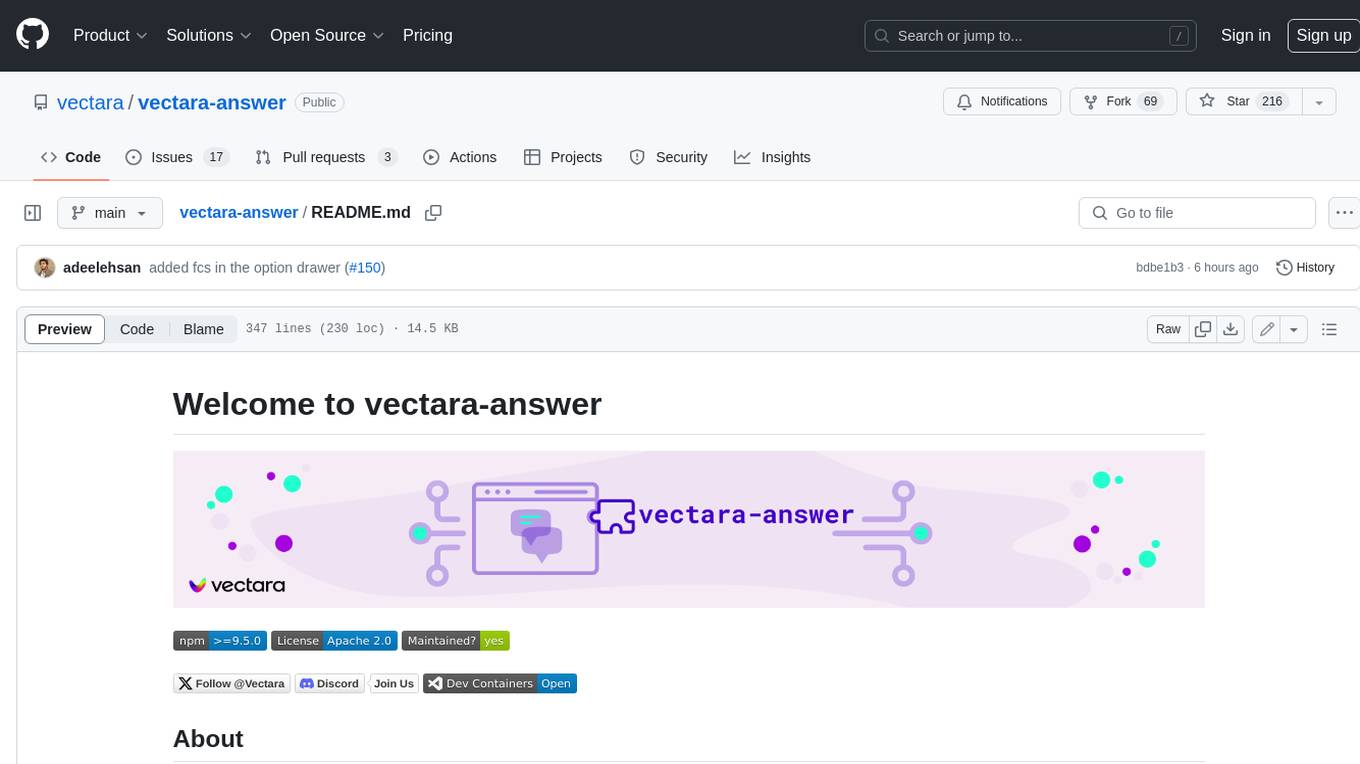
vectara-answer
Vectara Answer is a sample app for Vectara-powered Summarized Semantic Search (or question-answering) with advanced configuration options. For examples of what you can build with Vectara Answer, check out Ask News, LegalAid, or any of the other demo applications.
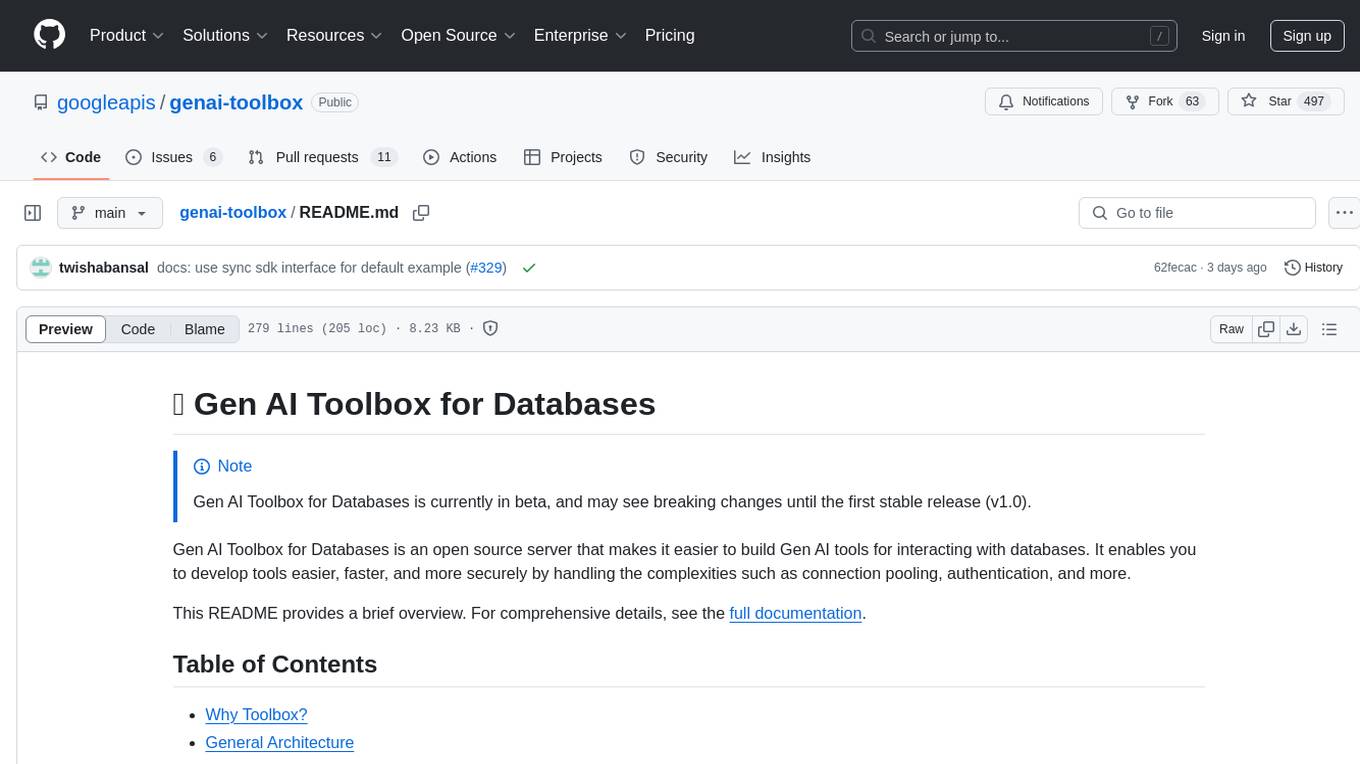
genai-toolbox
Gen AI Toolbox for Databases is an open source server that simplifies building Gen AI tools for interacting with databases. It handles complexities like connection pooling, authentication, and more, enabling easier, faster, and more secure tool development. The toolbox sits between the application's orchestration framework and the database, providing a control plane to modify, distribute, or invoke tools. It offers simplified development, better performance, enhanced security, and end-to-end observability. Users can install the toolbox as a binary, container image, or compile from source. Configuration is done through a 'tools.yaml' file, defining sources, tools, and toolsets. The project follows semantic versioning and welcomes contributions.
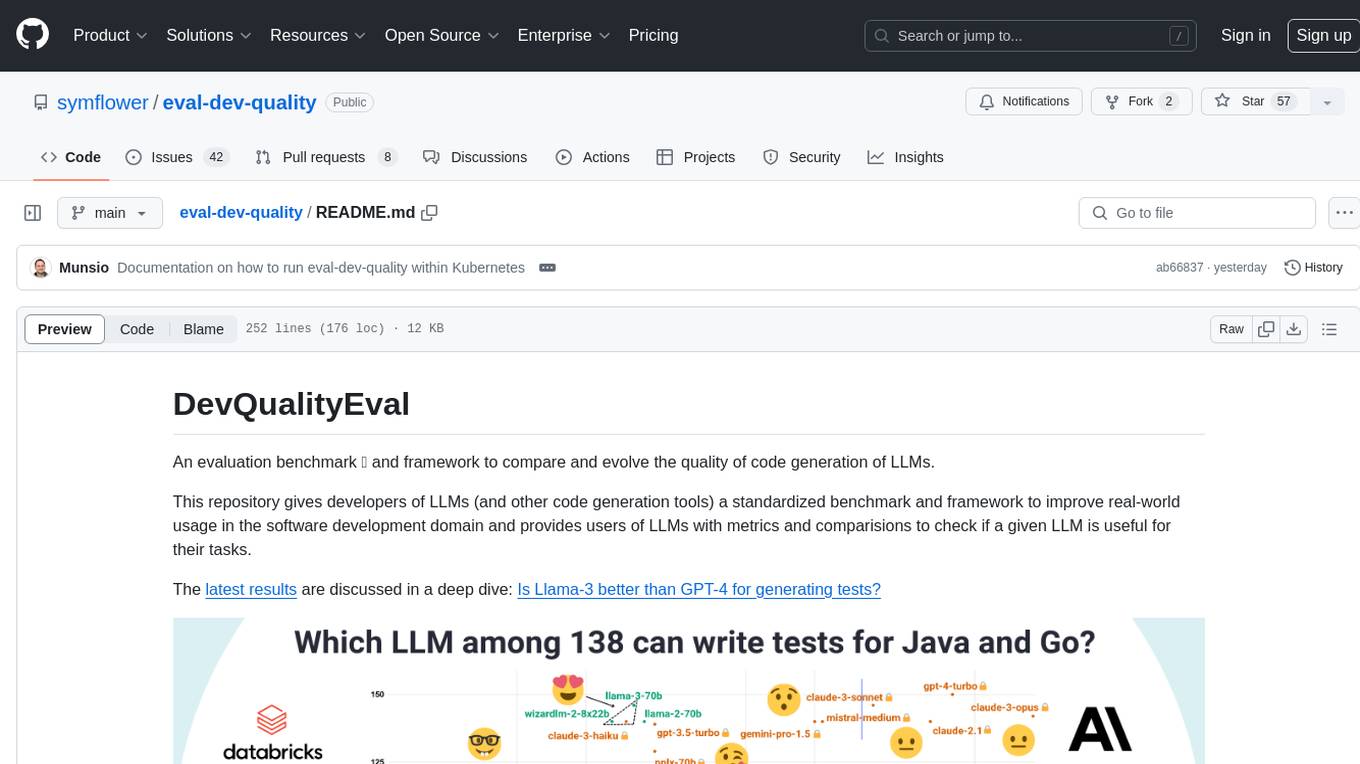
eval-dev-quality
DevQualityEval is an evaluation benchmark and framework designed to compare and improve the quality of code generation of Language Model Models (LLMs). It provides developers with a standardized benchmark to enhance real-world usage in software development and offers users metrics and comparisons to assess the usefulness of LLMs for their tasks. The tool evaluates LLMs' performance in solving software development tasks and measures the quality of their results through a point-based system. Users can run specific tasks, such as test generation, across different programming languages to evaluate LLMs' language understanding and code generation capabilities.
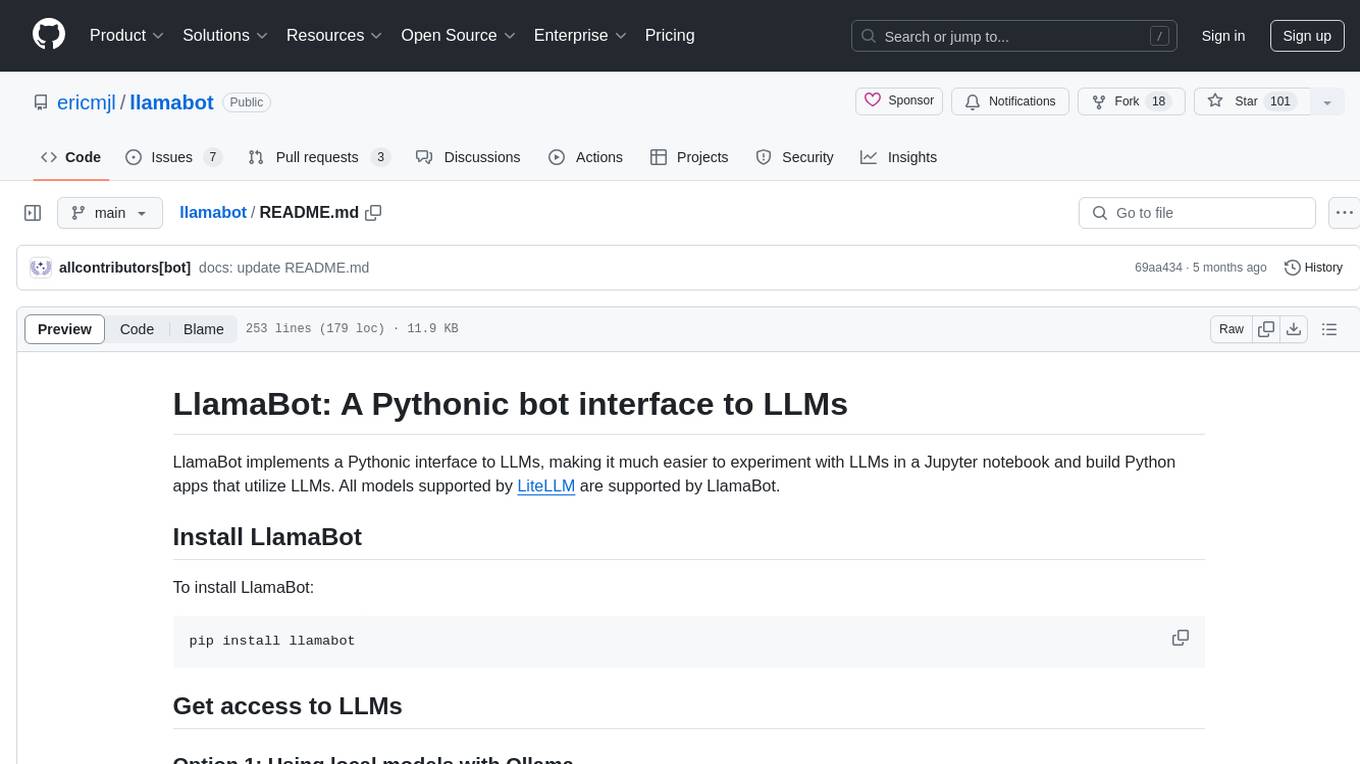
llamabot
LlamaBot is a Pythonic bot interface to Large Language Models (LLMs), providing an easy way to experiment with LLMs in Jupyter notebooks and build Python apps utilizing LLMs. It supports all models available in LiteLLM. Users can access LLMs either through local models with Ollama or by using API providers like OpenAI and Mistral. LlamaBot offers different bot interfaces like SimpleBot, ChatBot, QueryBot, and ImageBot for various tasks such as rephrasing text, maintaining chat history, querying documents, and generating images. The tool also includes CLI demos showcasing its capabilities and supports contributions for new features and bug reports from the community.
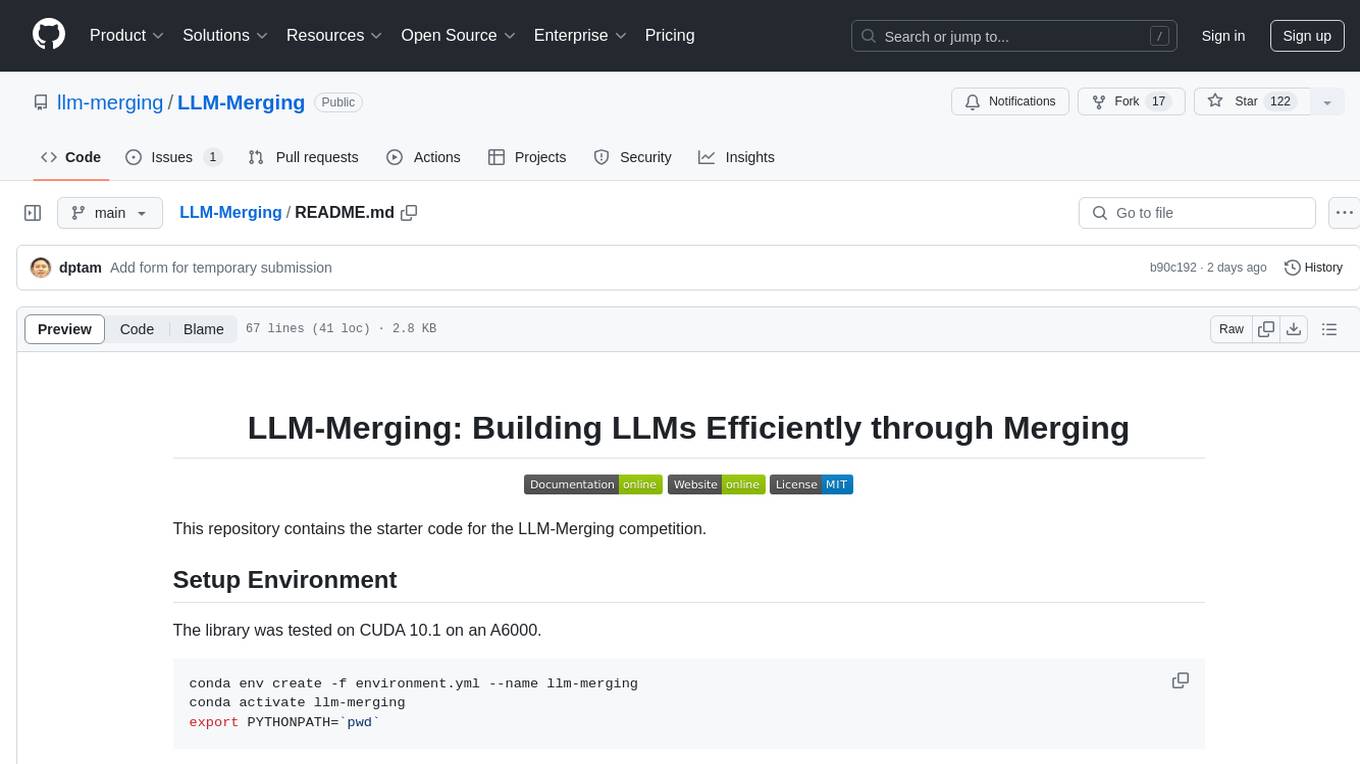
LLM-Merging
LLM-Merging is a repository containing starter code for the LLM-Merging competition. It provides a platform for efficiently building LLMs through merging methods. Users can develop new merging methods by creating new files in the specified directory and extending existing classes. The repository includes instructions for setting up the environment, developing new merging methods, testing the methods on specific datasets, and submitting solutions for evaluation. It aims to facilitate the development and evaluation of merging methods for LLMs.
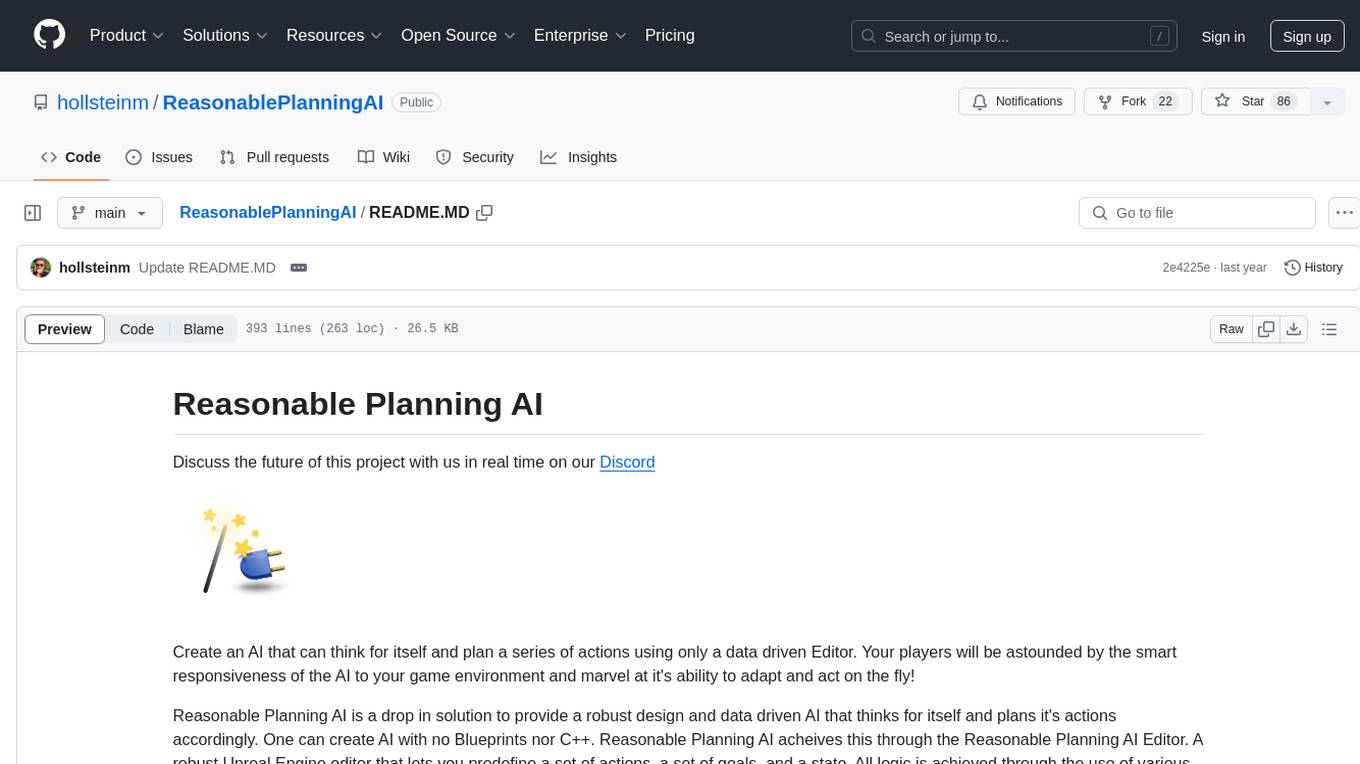
ReasonablePlanningAI
Reasonable Planning AI is a robust design and data-driven AI solution for game developers. It provides an AI Editor that allows creating AI without Blueprints or C++. The AI can think for itself, plan actions, adapt to the game environment, and act dynamically. It consists of Core components like RpaiGoalBase, RpaiActionBase, RpaiPlannerBase, RpaiReasonerBase, and RpaiBrainComponent, as well as Composer components for easier integration by Game Designers. The tool is extensible, cross-compatible with Behavior Trees, and offers debugging features like visual logging and heuristics testing. It follows a simple path of execution and supports versioning for stability and compatibility with Unreal Engine versions.
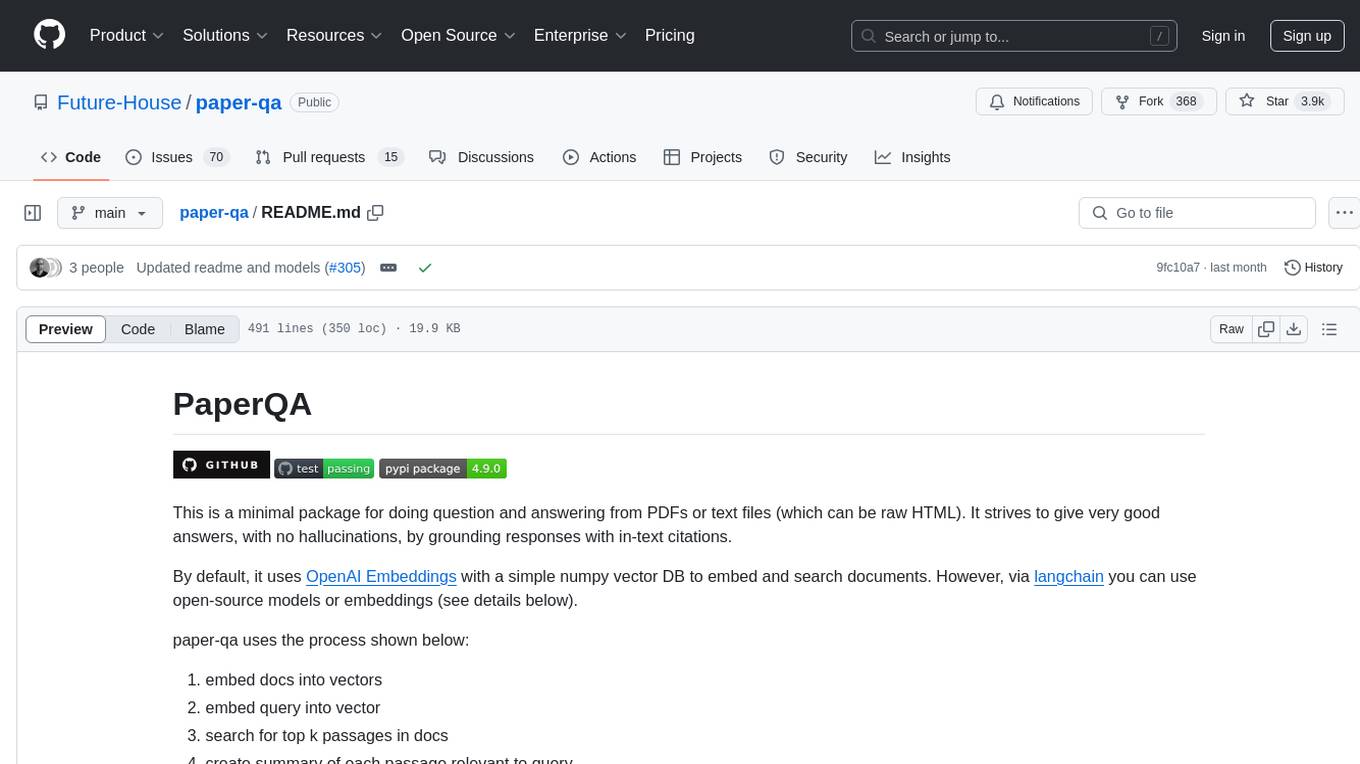
paper-qa
PaperQA is a minimal package for question and answering from PDFs or text files, providing very good answers with in-text citations. It uses OpenAI Embeddings to embed and search documents, and includes a process of embedding docs, queries, searching for top passages, creating summaries, using an LLM to re-score and select relevant summaries, putting summaries into prompt, and generating answers. The tool can be used to answer specific questions related to scientific research by leveraging citations and relevant passages from documents.
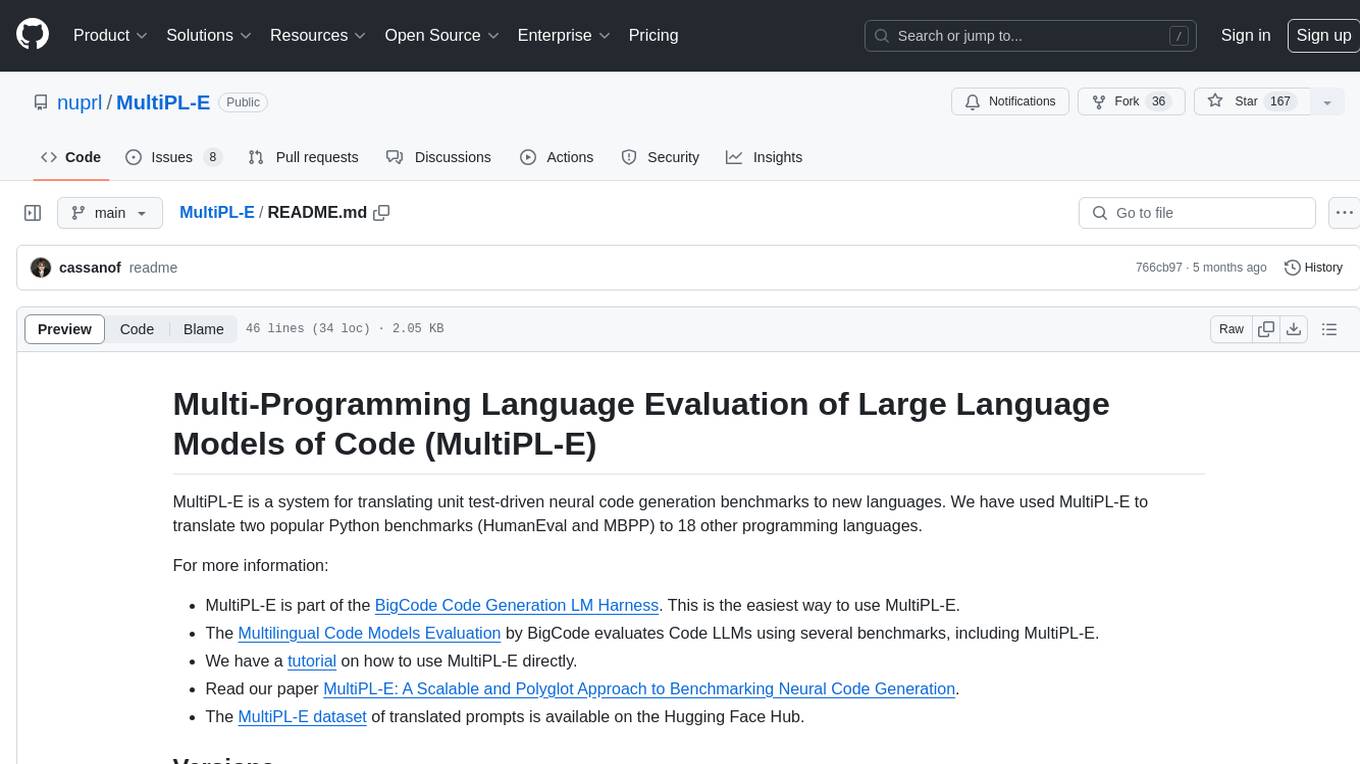
MultiPL-E
MultiPL-E is a system for translating unit test-driven neural code generation benchmarks to new languages. It is part of the BigCode Code Generation LM Harness and allows for evaluating Code LLMs using various benchmarks. The tool supports multiple versions with improvements and new language additions, providing a scalable and polyglot approach to benchmarking neural code generation. Users can access a tutorial for direct usage and explore the dataset of translated prompts on the Hugging Face Hub.
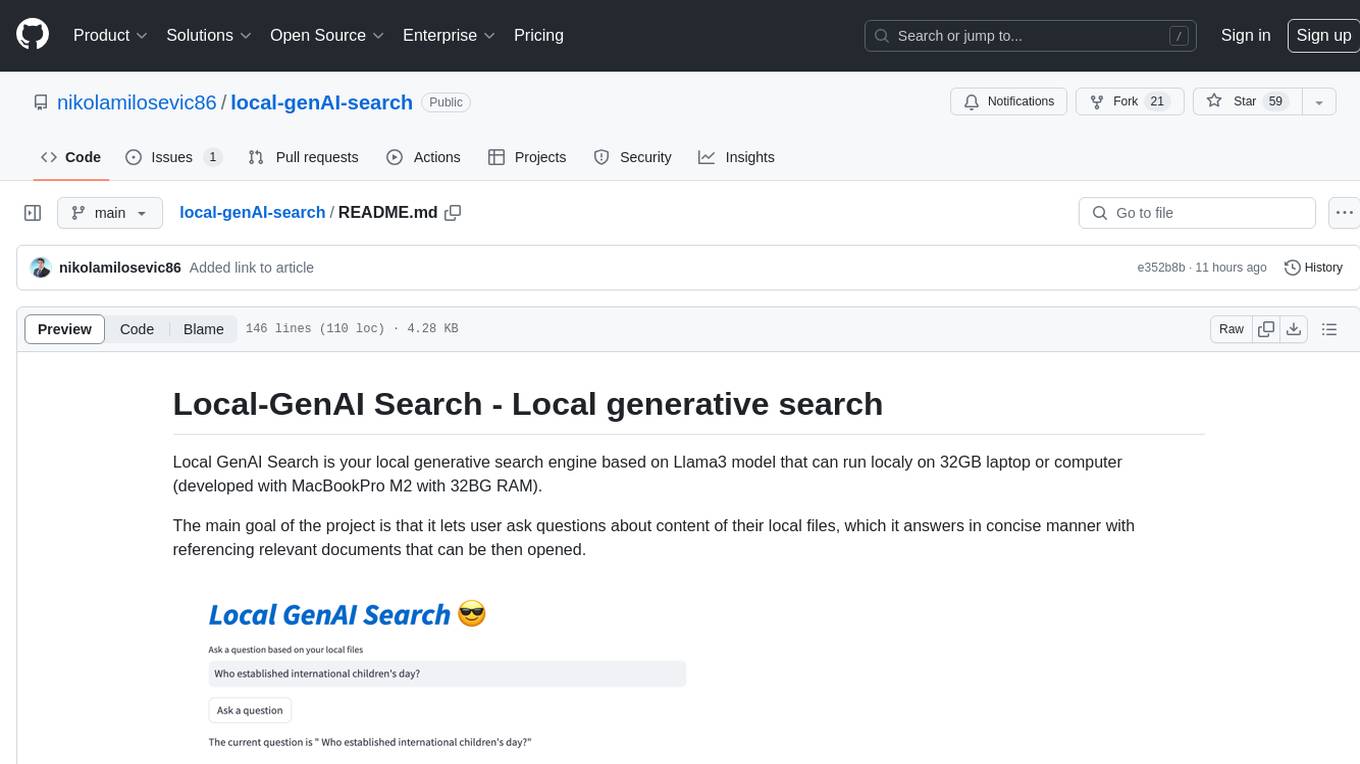
local-genAI-search
Local-GenAI Search is a local generative search engine powered by the Llama3 model, allowing users to ask questions about their local files and receive concise answers with relevant document references. It utilizes MS MARCO embeddings for semantic search and can run locally on a 32GB laptop or computer. The tool can be used to index local documents, search for information, and provide generative search services through a user interface.
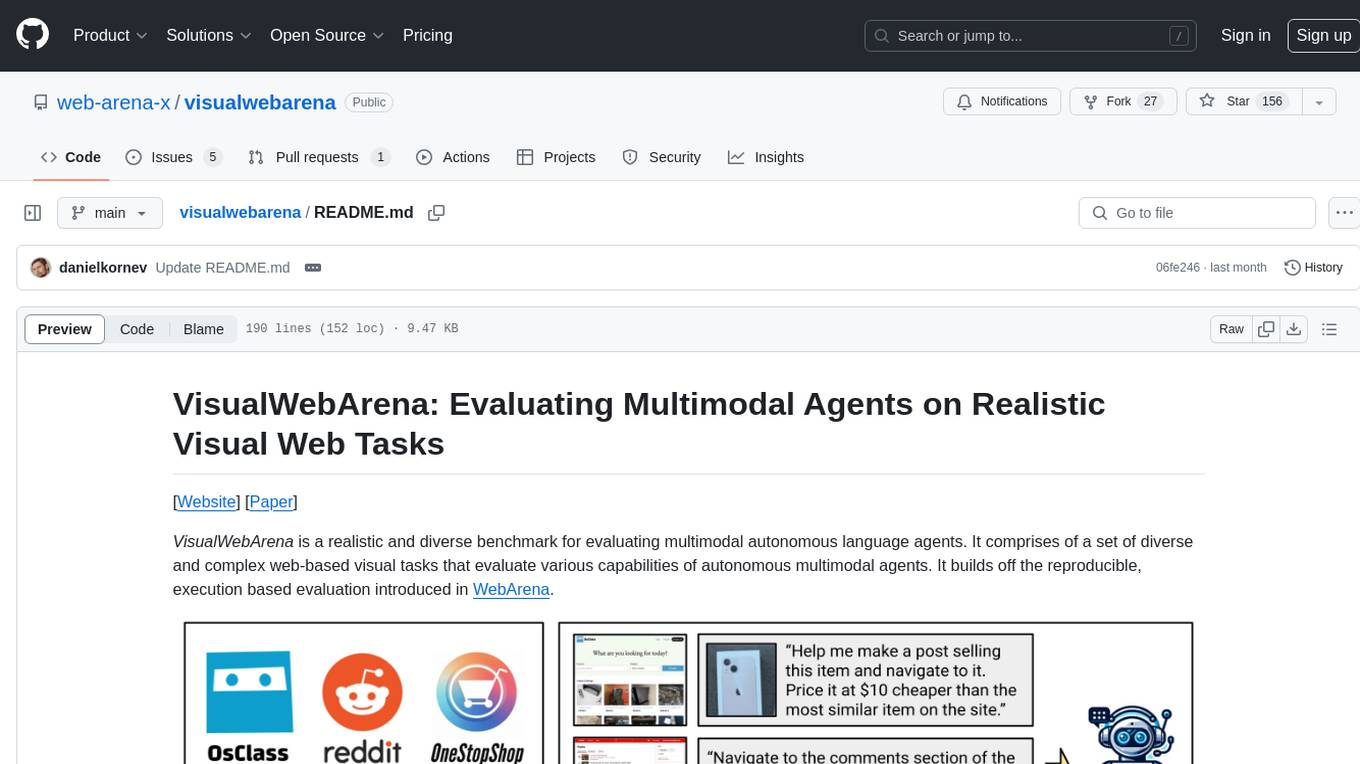
visualwebarena
VisualWebArena is a benchmark for evaluating multimodal autonomous language agents through diverse and complex web-based visual tasks. It builds on the reproducible evaluation introduced in WebArena. The repository provides scripts for end-to-end training, demos to run multimodal agents on webpages, and tools for setting up environments for evaluation. It includes trajectories of the GPT-4V + SoM agent on VWA tasks, along with human evaluations on 233 tasks. The environment supports OpenAI models and Gemini models for evaluation.
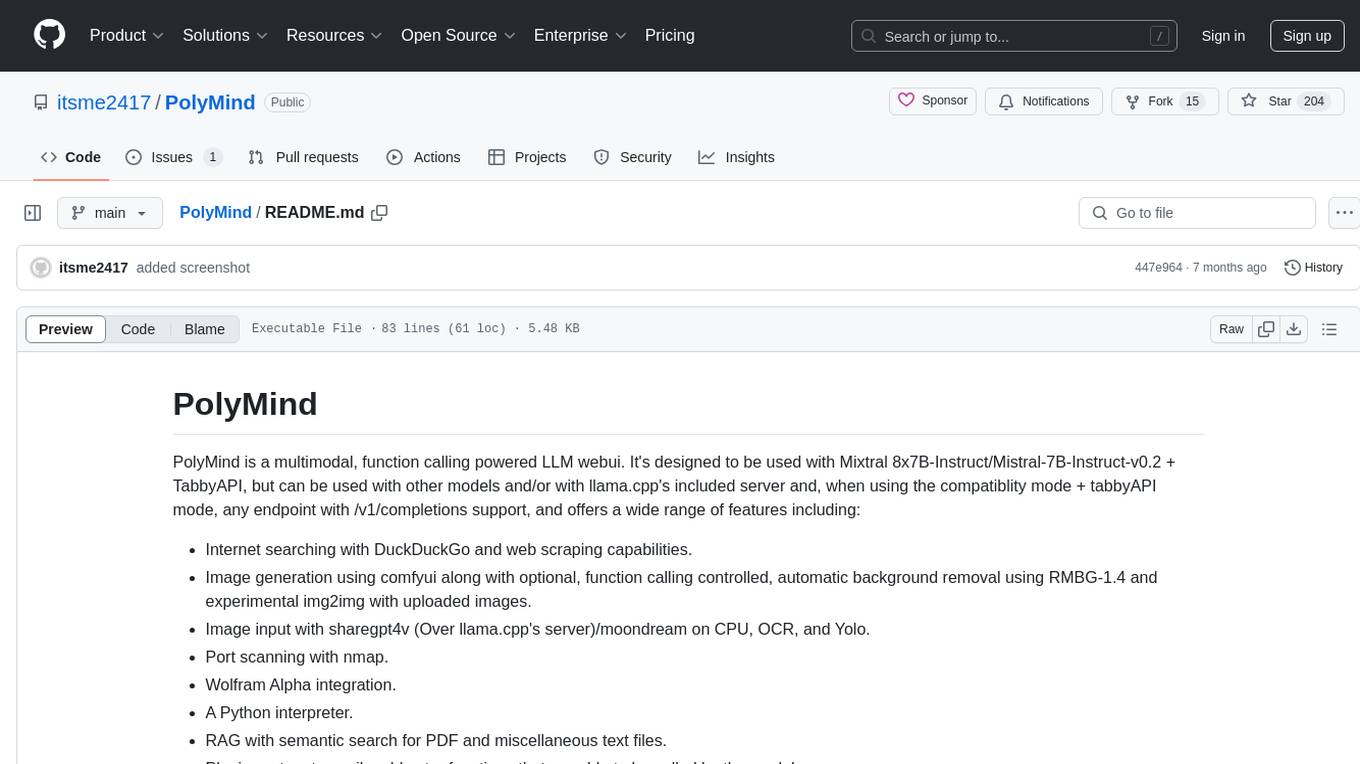
PolyMind
PolyMind is a multimodal, function calling powered LLM webui designed for various tasks such as internet searching, image generation, port scanning, Wolfram Alpha integration, Python interpretation, and semantic search. It offers a plugin system for adding extra functions and supports different models and endpoints. The tool allows users to interact via function calling and provides features like image input, image generation, and text file search. The application's configuration is stored in a `config.json` file with options for backend selection, compatibility mode, IP address settings, API key, and enabled features.
For similar tasks
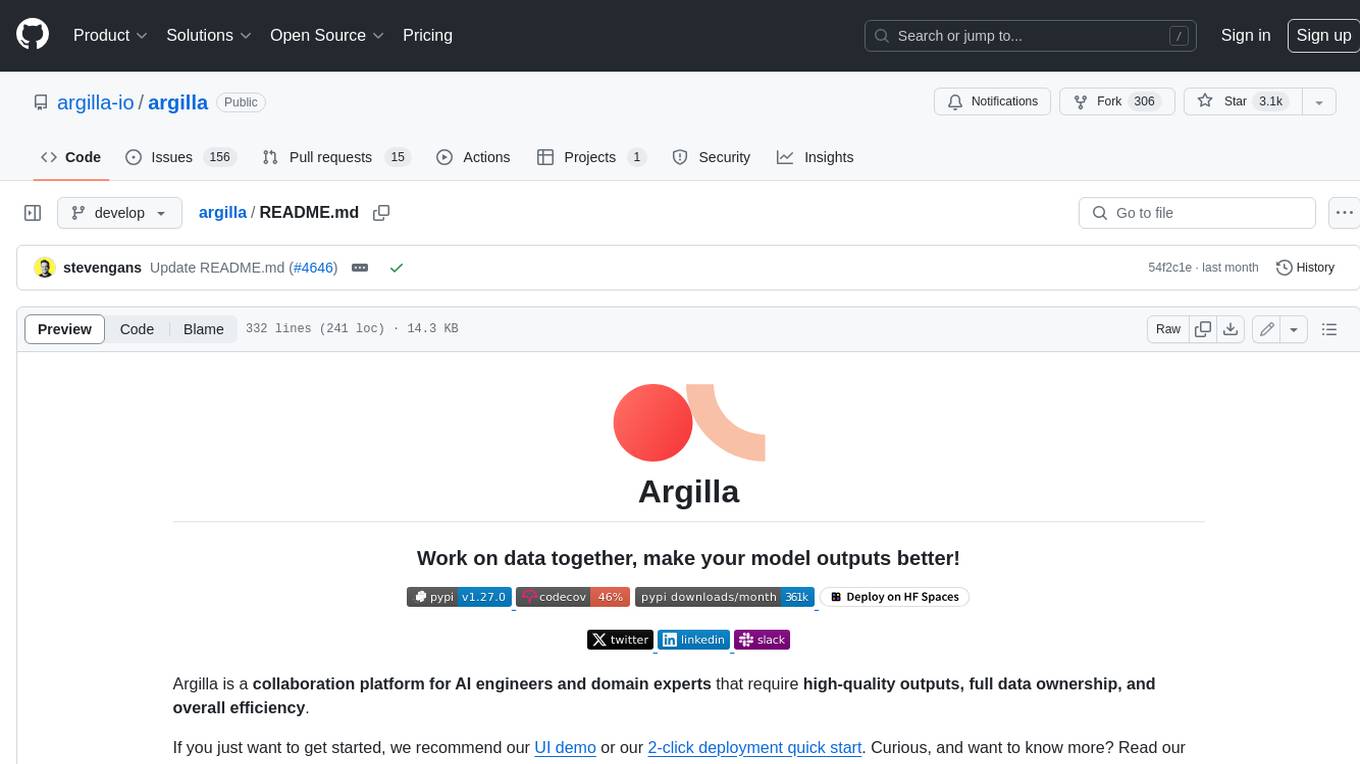
argilla
Argilla is a collaboration platform for AI engineers and domain experts that require high-quality outputs, full data ownership, and overall efficiency. It helps users improve AI output quality through data quality, take control of their data and models, and improve efficiency by quickly iterating on the right data and models. Argilla is an open-source community-driven project that provides tools for achieving and maintaining high-quality data standards, with a focus on NLP and LLMs. It is used by AI teams from companies like the Red Cross, Loris.ai, and Prolific to improve the quality and efficiency of AI projects.
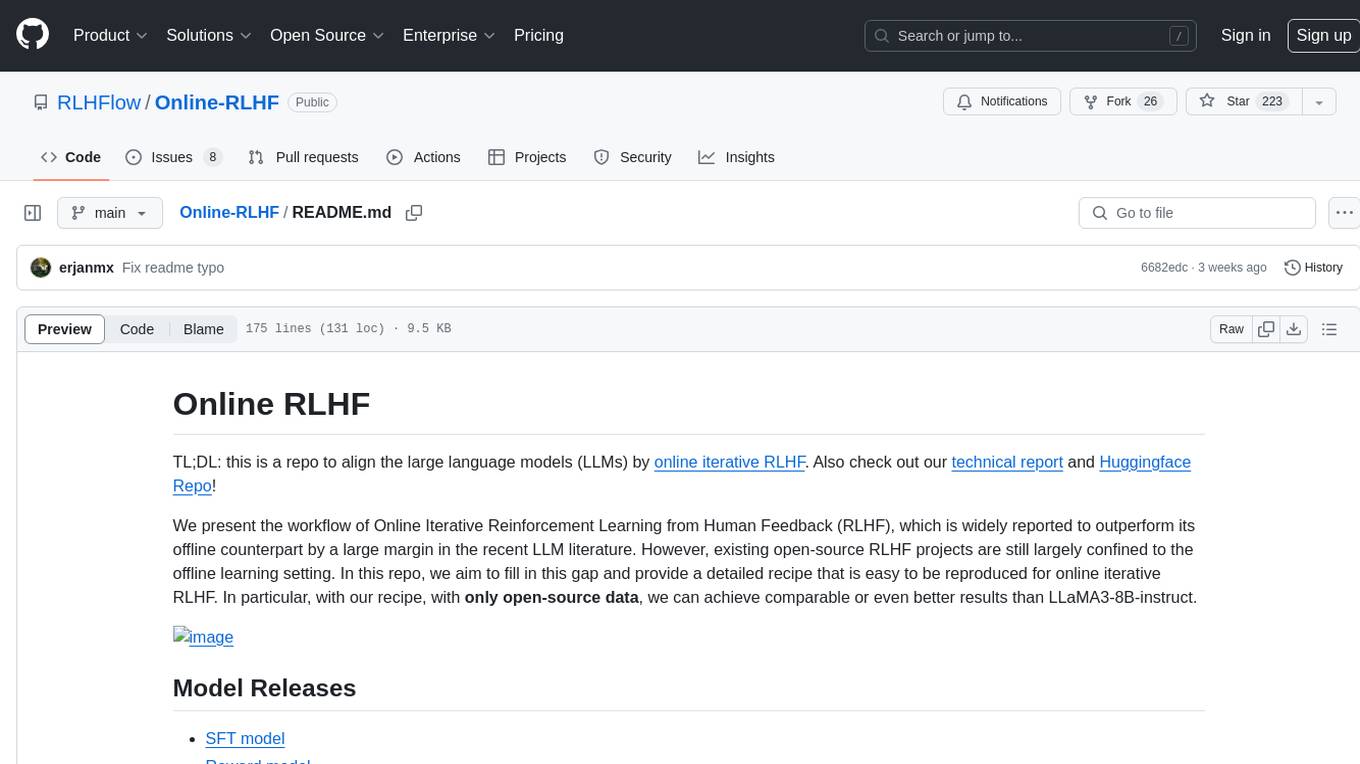
Online-RLHF
This repository, Online RLHF, focuses on aligning large language models (LLMs) through online iterative Reinforcement Learning from Human Feedback (RLHF). It aims to bridge the gap in existing open-source RLHF projects by providing a detailed recipe for online iterative RLHF. The workflow presented here has shown to outperform offline counterparts in recent LLM literature, achieving comparable or better results than LLaMA3-8B-instruct using only open-source data. The repository includes model releases for SFT, Reward model, and RLHF model, along with installation instructions for both inference and training environments. Users can follow step-by-step guidance for supervised fine-tuning, reward modeling, data generation, data annotation, and training, ultimately enabling iterative training to run automatically.
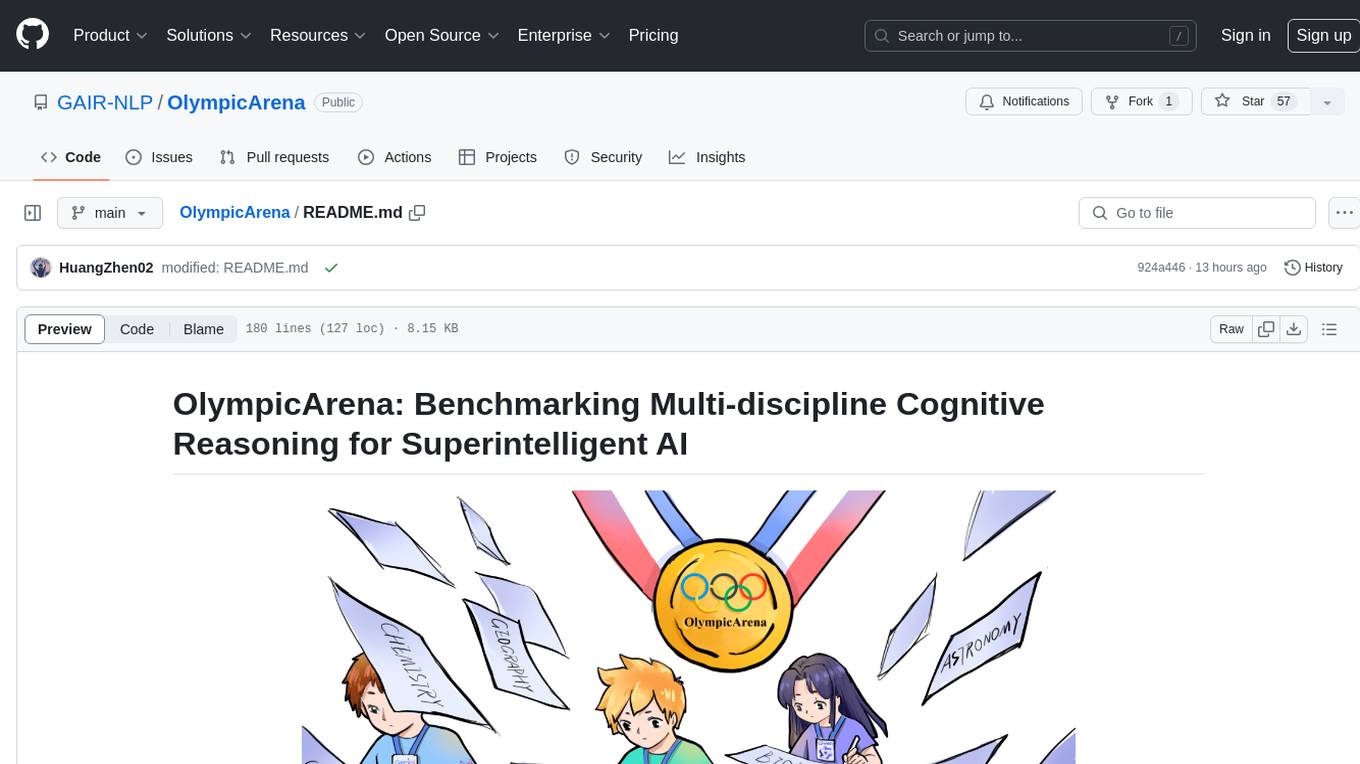
OlympicArena
OlympicArena is a comprehensive benchmark designed to evaluate advanced AI capabilities across various disciplines. It aims to push AI towards superintelligence by tackling complex challenges in science and beyond. The repository provides detailed data for different disciplines, allows users to run inference and evaluation locally, and offers a submission platform for testing models on the test set. Additionally, it includes an annotation interface and encourages users to cite their paper if they find the code or dataset helpful.
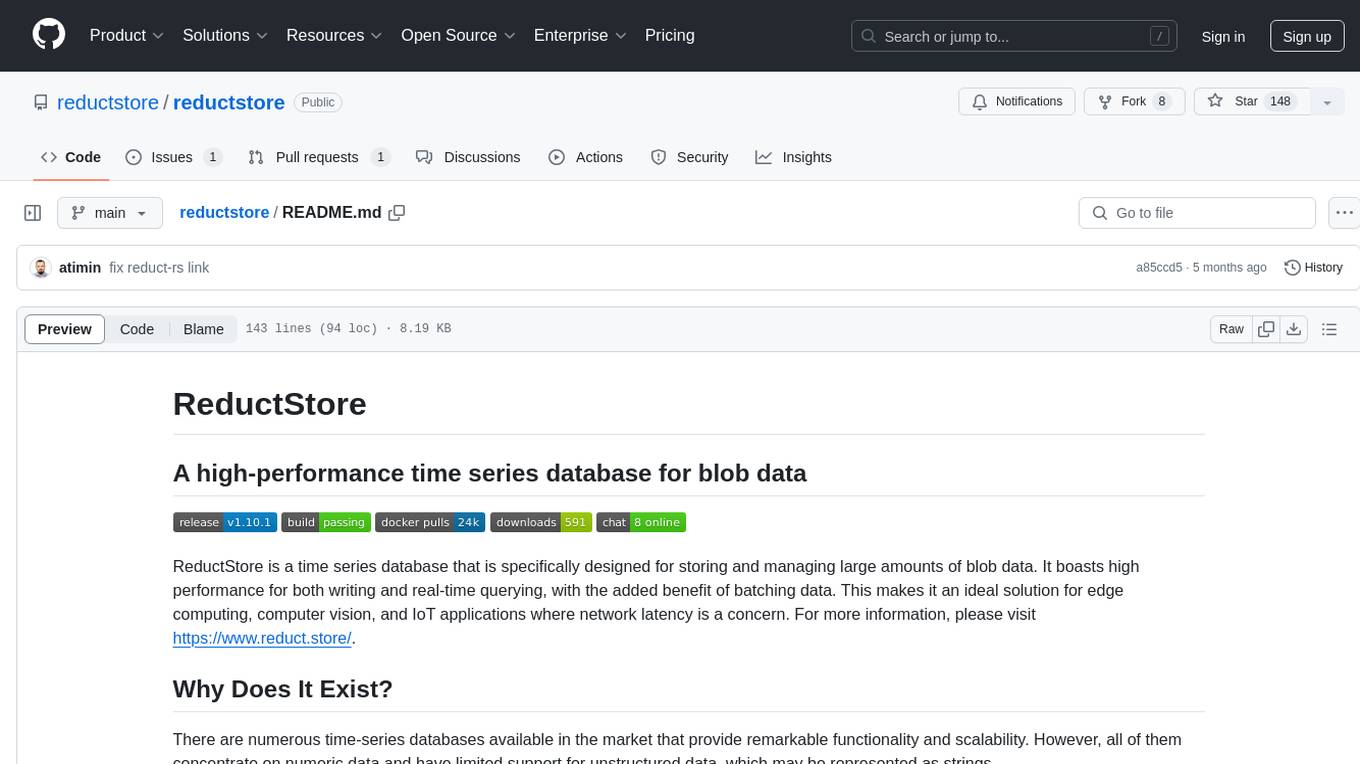
reductstore
ReductStore is a high-performance time series database designed for storing and managing large amounts of unstructured blob data. It offers features such as real-time querying, batching data, and HTTP(S) API for edge computing, computer vision, and IoT applications. The database ensures data integrity, implements retention policies, and provides efficient data access, making it a cost-effective solution for applications requiring unstructured data storage and access at specific time intervals.
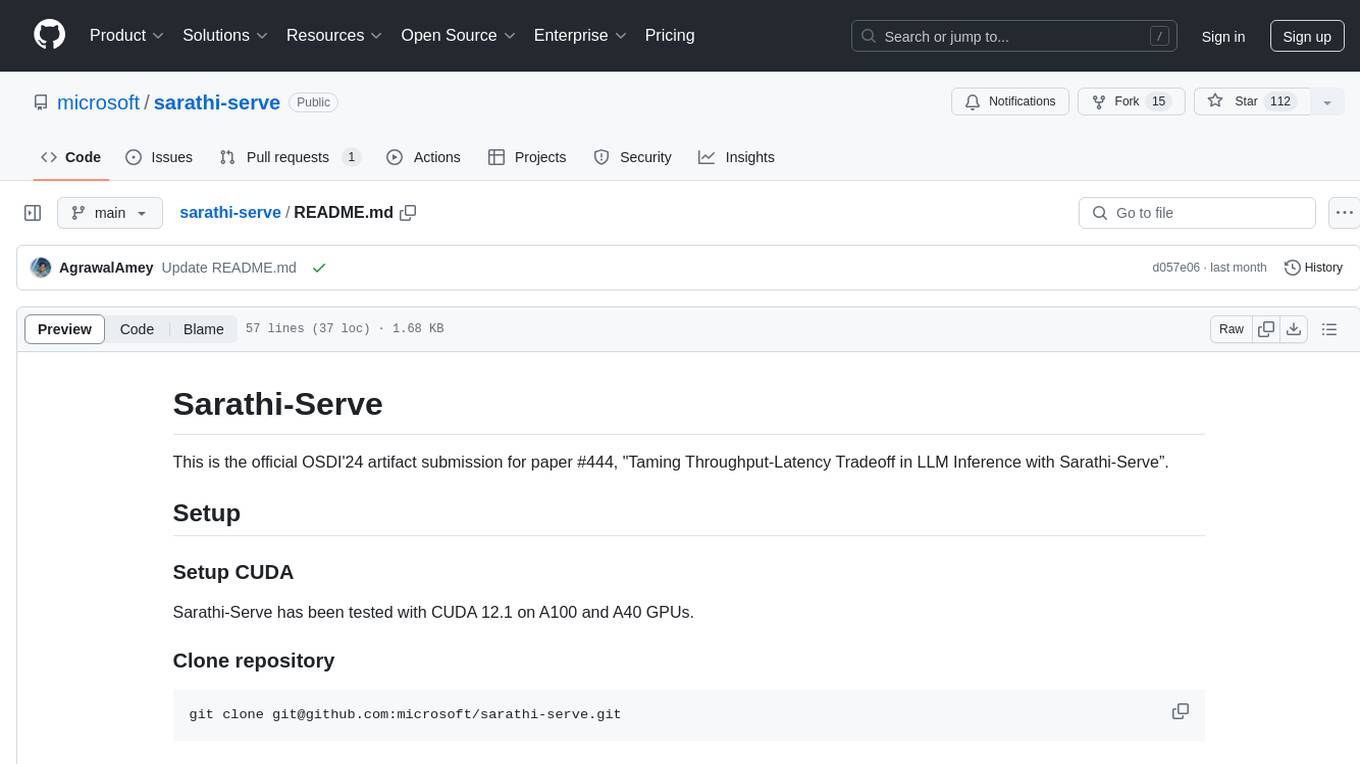
sarathi-serve
Sarathi-Serve is the official OSDI'24 artifact submission for paper #444, focusing on 'Taming Throughput-Latency Tradeoff in LLM Inference'. It is a research prototype built on top of CUDA 12.1, designed to optimize throughput-latency tradeoff in Large Language Models (LLM) inference. The tool provides a Python environment for users to install and reproduce results from the associated experiments. Users can refer to specific folders for individual figures and are encouraged to cite the paper if they use the tool in their work.
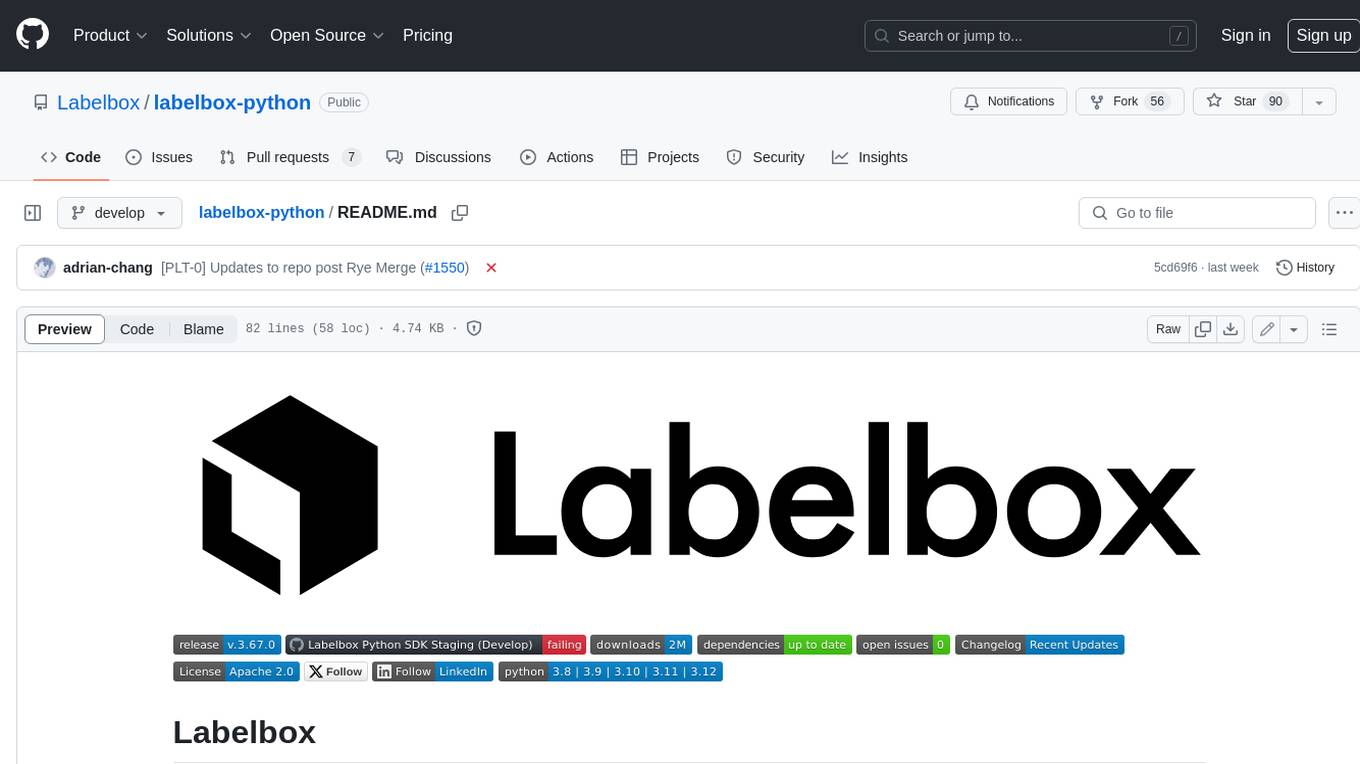
labelbox-python
Labelbox is a data-centric AI platform for enterprises to develop, optimize, and use AI to solve problems and power new products and services. Enterprises use Labelbox to curate data, generate high-quality human feedback data for computer vision and LLMs, evaluate model performance, and automate tasks by combining AI and human-centric workflows. The academic & research community uses Labelbox for cutting-edge AI research.
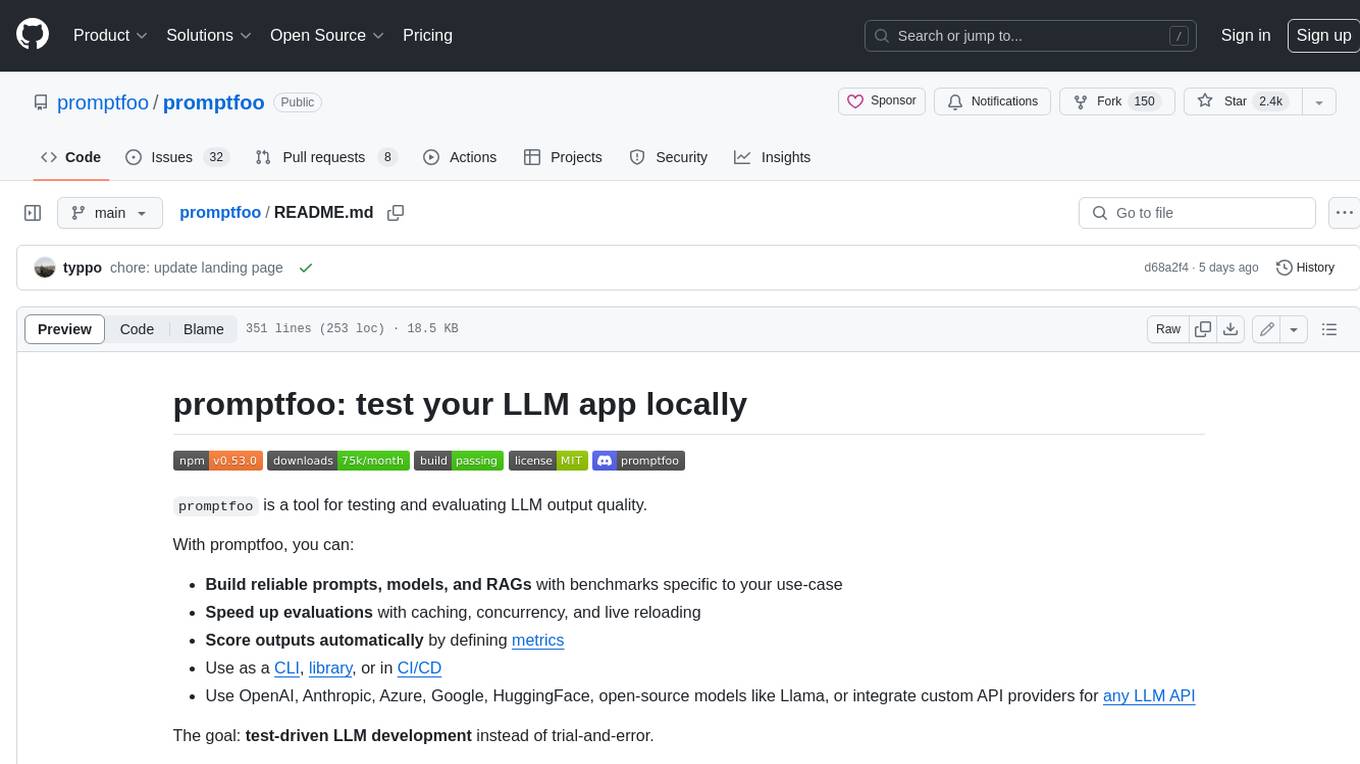
promptfoo
Promptfoo is a tool for testing and evaluating LLM output quality. With promptfoo, you can build reliable prompts, models, and RAGs with benchmarks specific to your use-case, speed up evaluations with caching, concurrency, and live reloading, score outputs automatically by defining metrics, use as a CLI, library, or in CI/CD, and use OpenAI, Anthropic, Azure, Google, HuggingFace, open-source models like Llama, or integrate custom API providers for any LLM API.
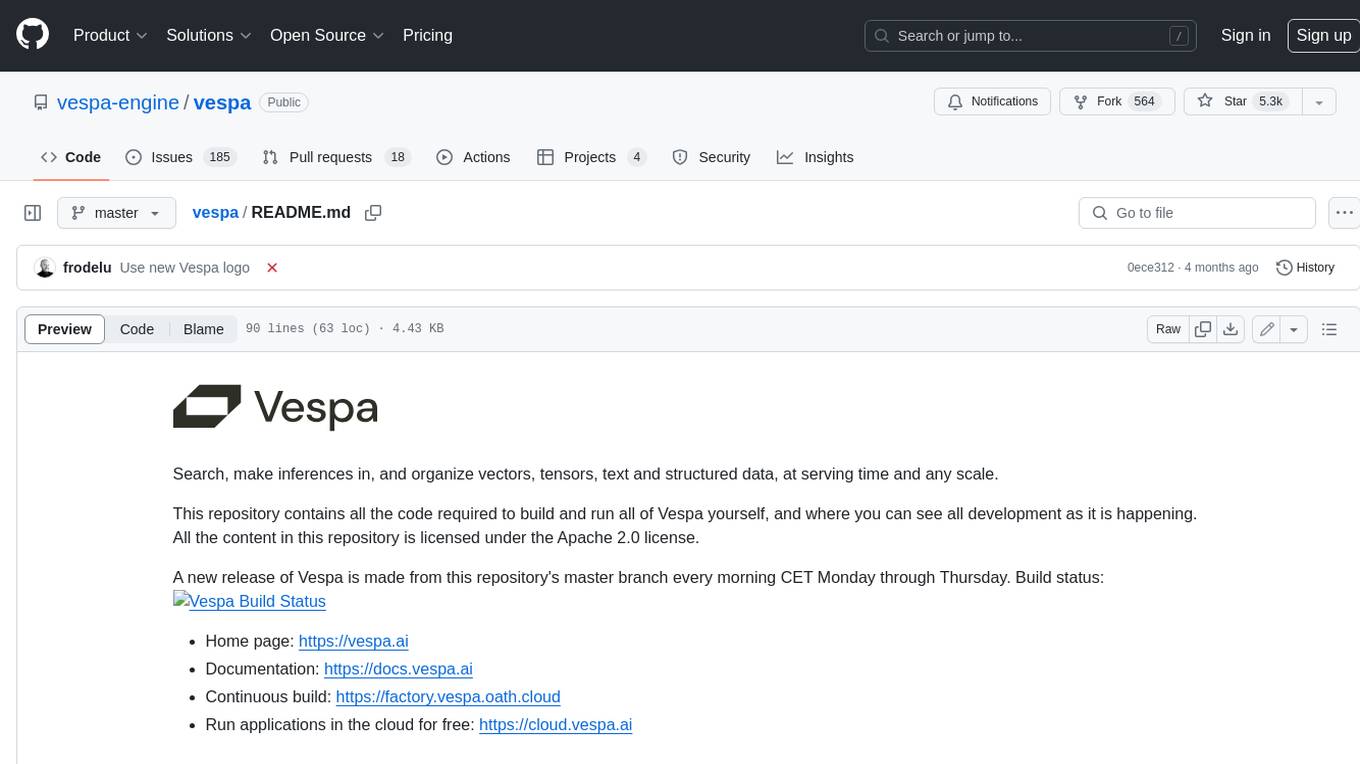
vespa
Vespa is a platform that performs operations such as selecting a subset of data in a large corpus, evaluating machine-learned models over the selected data, organizing and aggregating it, and returning it, typically in less than 100 milliseconds, all while the data corpus is continuously changing. It has been in development for many years and is used on a number of large internet services and apps which serve hundreds of thousands of queries from Vespa per second.
For similar jobs
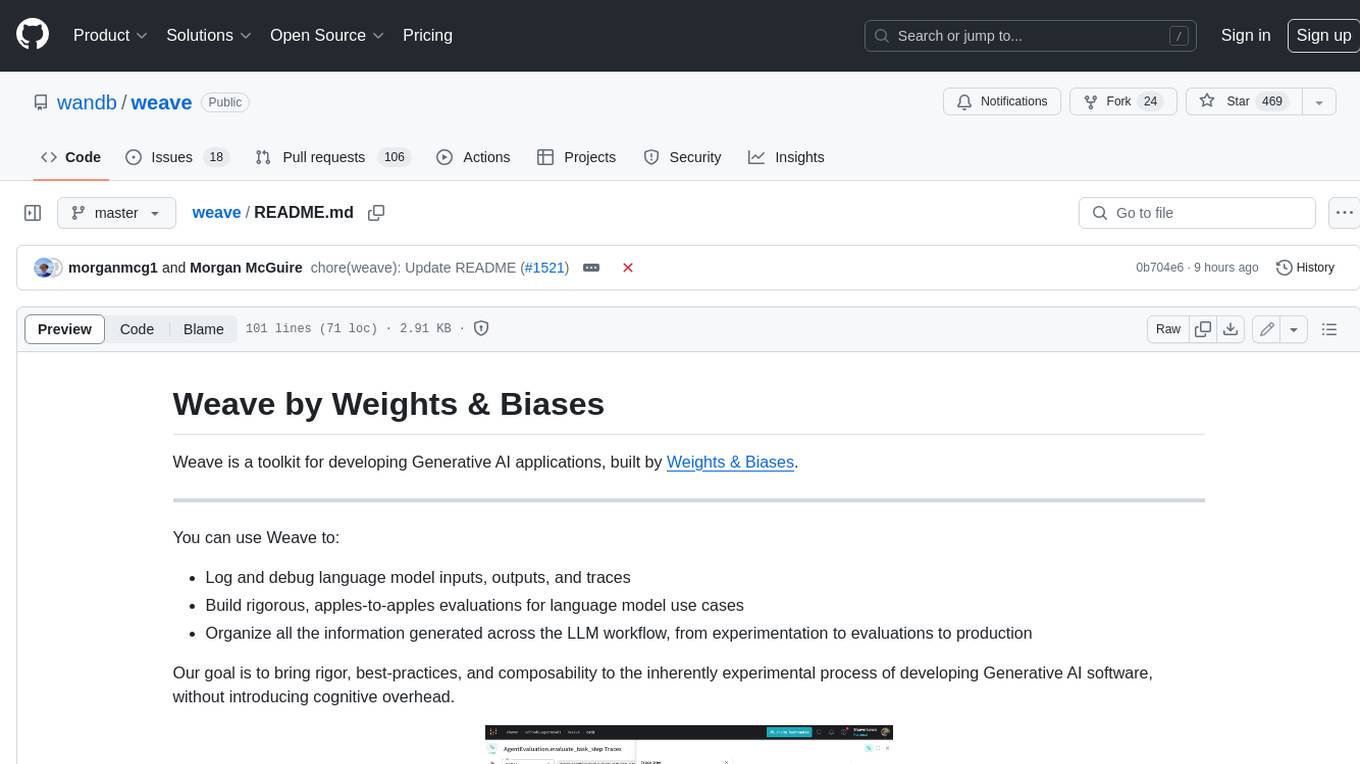
weave
Weave is a toolkit for developing Generative AI applications, built by Weights & Biases. With Weave, you can log and debug language model inputs, outputs, and traces; build rigorous, apples-to-apples evaluations for language model use cases; and organize all the information generated across the LLM workflow, from experimentation to evaluations to production. Weave aims to bring rigor, best-practices, and composability to the inherently experimental process of developing Generative AI software, without introducing cognitive overhead.
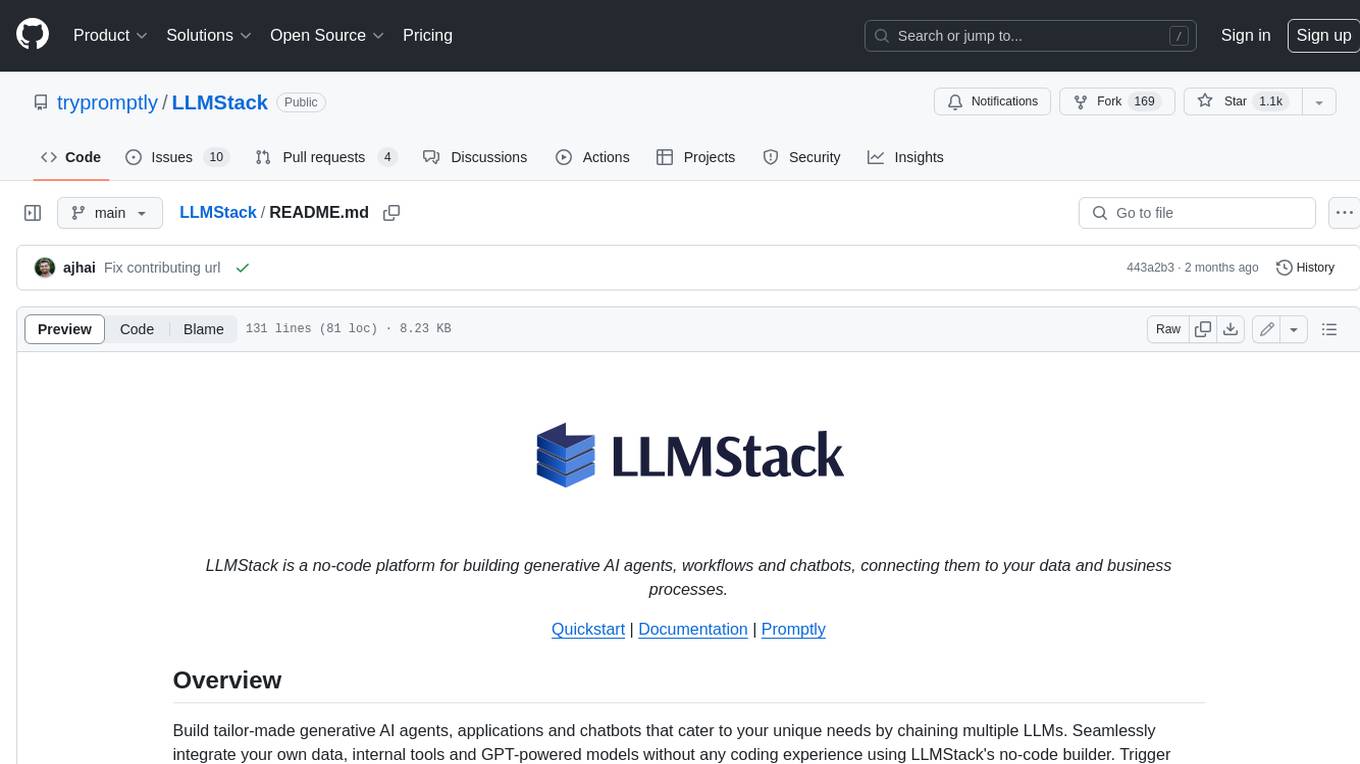
LLMStack
LLMStack is a no-code platform for building generative AI agents, workflows, and chatbots. It allows users to connect their own data, internal tools, and GPT-powered models without any coding experience. LLMStack can be deployed to the cloud or on-premise and can be accessed via HTTP API or triggered from Slack or Discord.
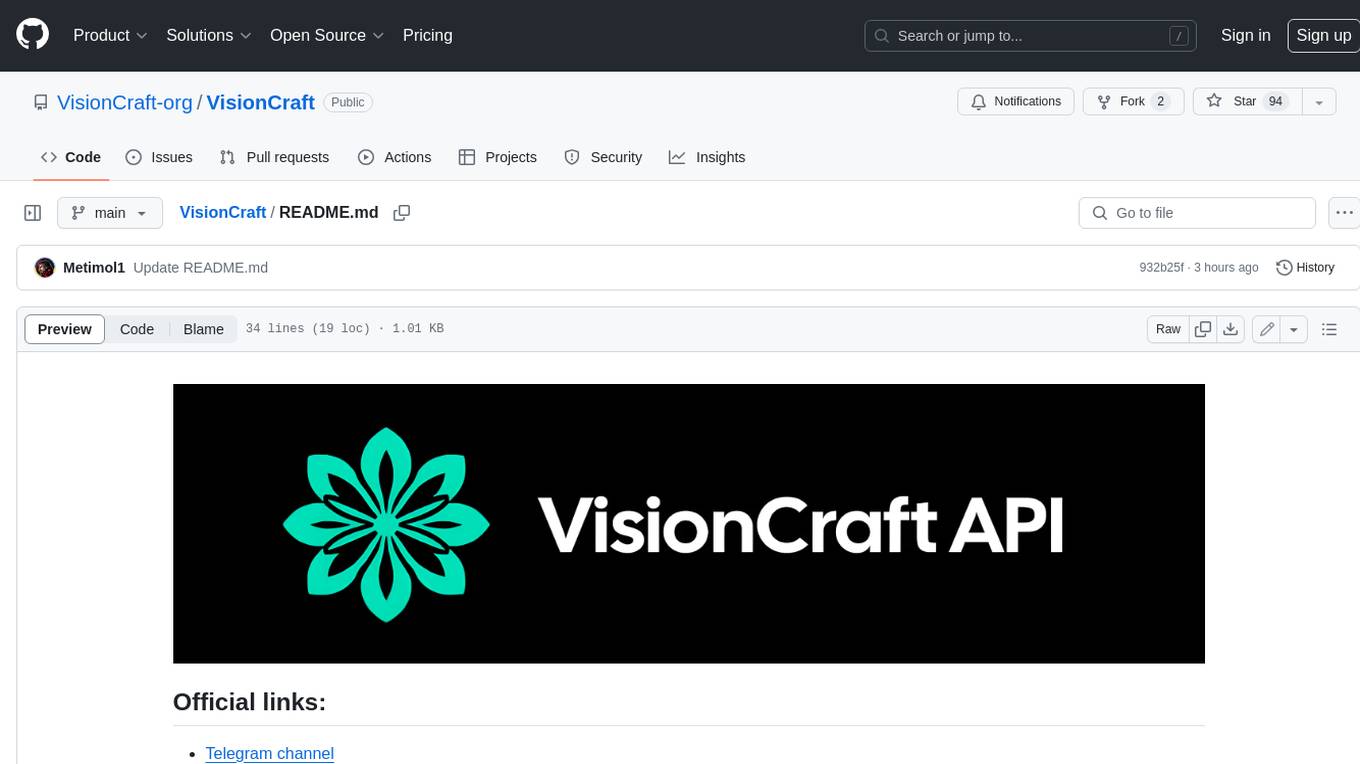
VisionCraft
The VisionCraft API is a free API for using over 100 different AI models. From images to sound.
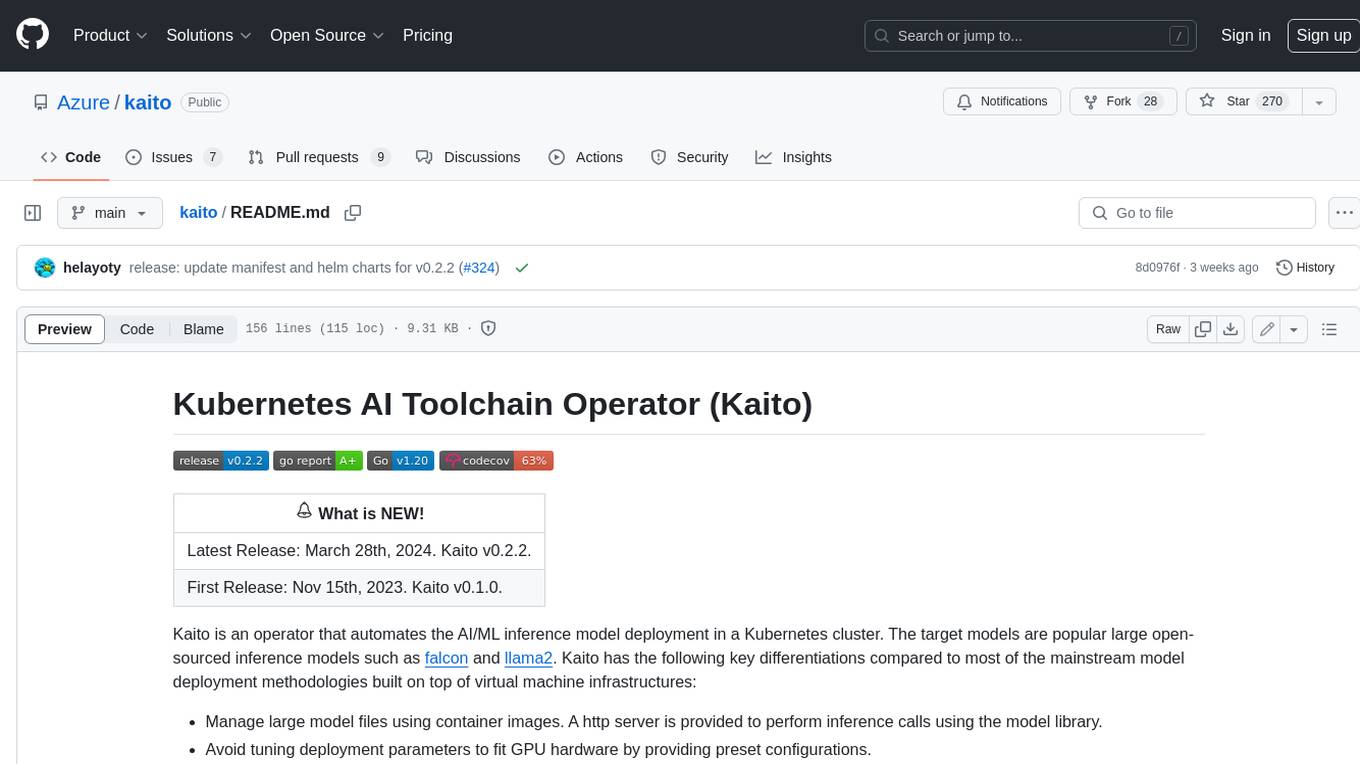
kaito
Kaito is an operator that automates the AI/ML inference model deployment in a Kubernetes cluster. It manages large model files using container images, avoids tuning deployment parameters to fit GPU hardware by providing preset configurations, auto-provisions GPU nodes based on model requirements, and hosts large model images in the public Microsoft Container Registry (MCR) if the license allows. Using Kaito, the workflow of onboarding large AI inference models in Kubernetes is largely simplified.
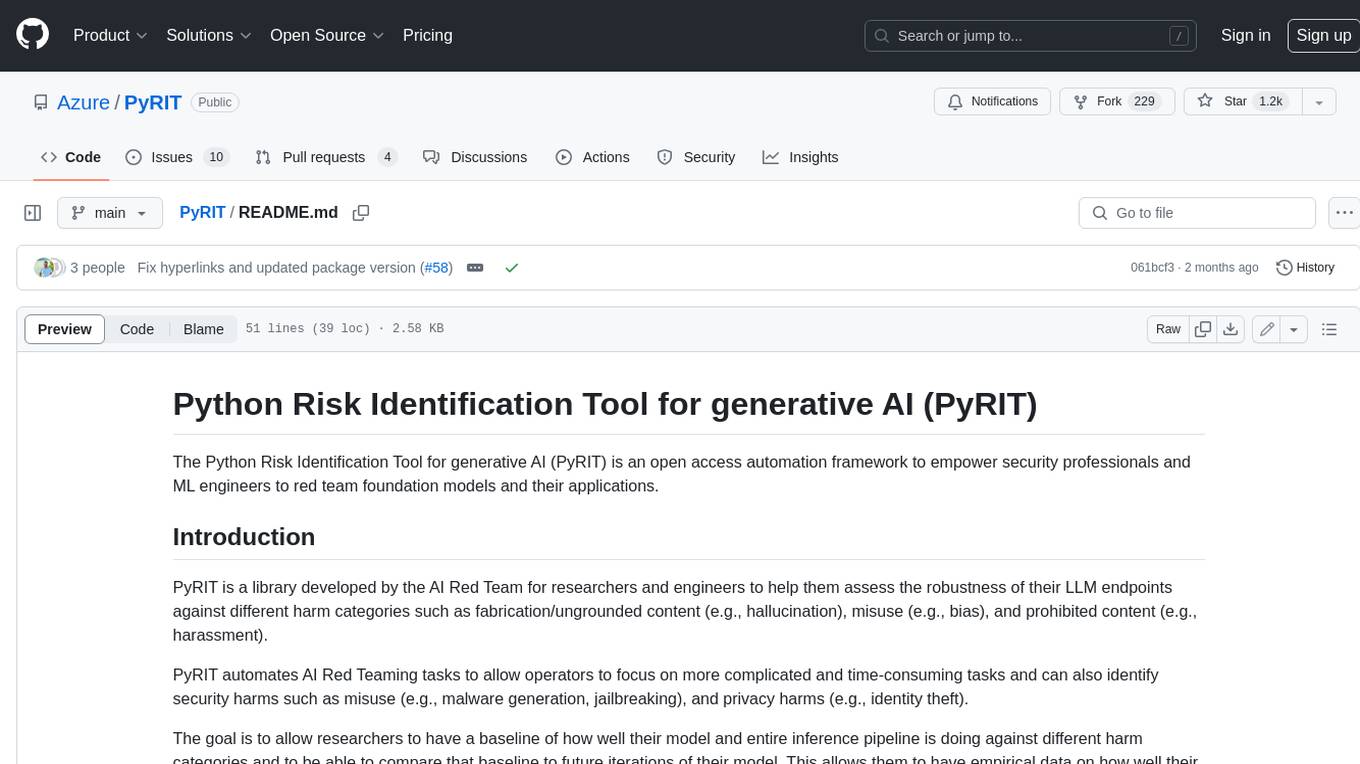
PyRIT
PyRIT is an open access automation framework designed to empower security professionals and ML engineers to red team foundation models and their applications. It automates AI Red Teaming tasks to allow operators to focus on more complicated and time-consuming tasks and can also identify security harms such as misuse (e.g., malware generation, jailbreaking), and privacy harms (e.g., identity theft). The goal is to allow researchers to have a baseline of how well their model and entire inference pipeline is doing against different harm categories and to be able to compare that baseline to future iterations of their model. This allows them to have empirical data on how well their model is doing today, and detect any degradation of performance based on future improvements.
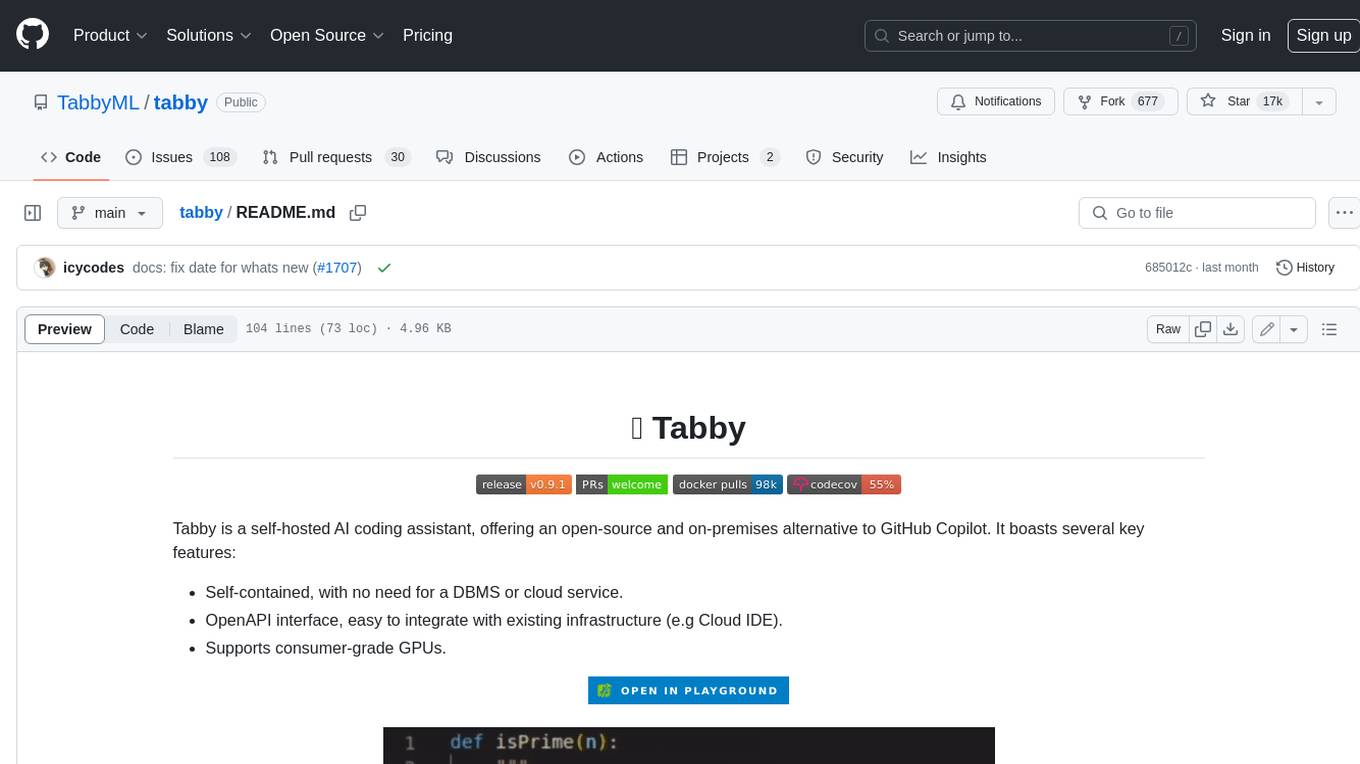
tabby
Tabby is a self-hosted AI coding assistant, offering an open-source and on-premises alternative to GitHub Copilot. It boasts several key features: * Self-contained, with no need for a DBMS or cloud service. * OpenAPI interface, easy to integrate with existing infrastructure (e.g Cloud IDE). * Supports consumer-grade GPUs.
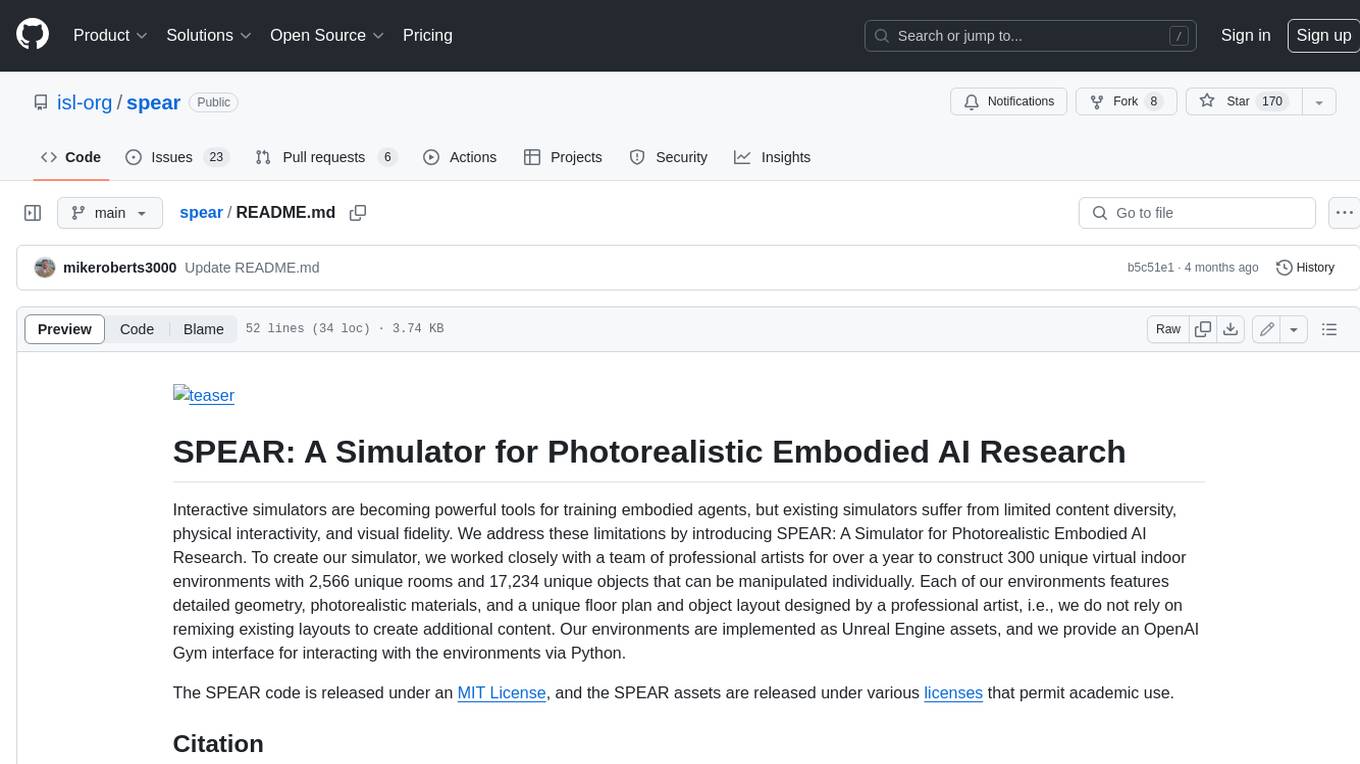
spear
SPEAR (Simulator for Photorealistic Embodied AI Research) is a powerful tool for training embodied agents. It features 300 unique virtual indoor environments with 2,566 unique rooms and 17,234 unique objects that can be manipulated individually. Each environment is designed by a professional artist and features detailed geometry, photorealistic materials, and a unique floor plan and object layout. SPEAR is implemented as Unreal Engine assets and provides an OpenAI Gym interface for interacting with the environments via Python.
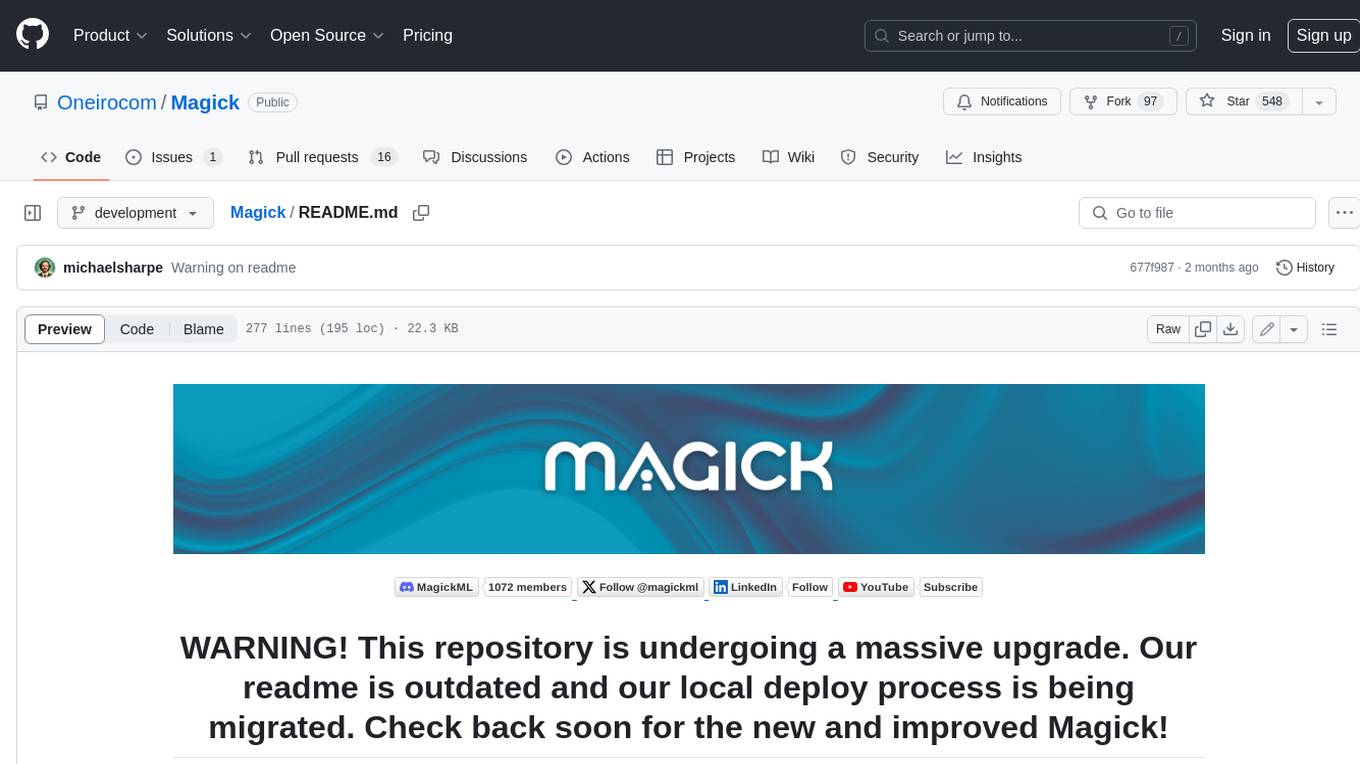
Magick
Magick is a groundbreaking visual AIDE (Artificial Intelligence Development Environment) for no-code data pipelines and multimodal agents. Magick can connect to other services and comes with nodes and templates well-suited for intelligent agents, chatbots, complex reasoning systems and realistic characters.