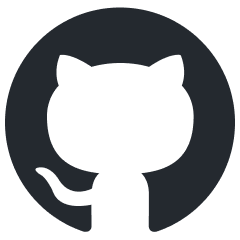
vectara-answer
LLM-powered Conversational AI experience using Vectara
Stars: 249
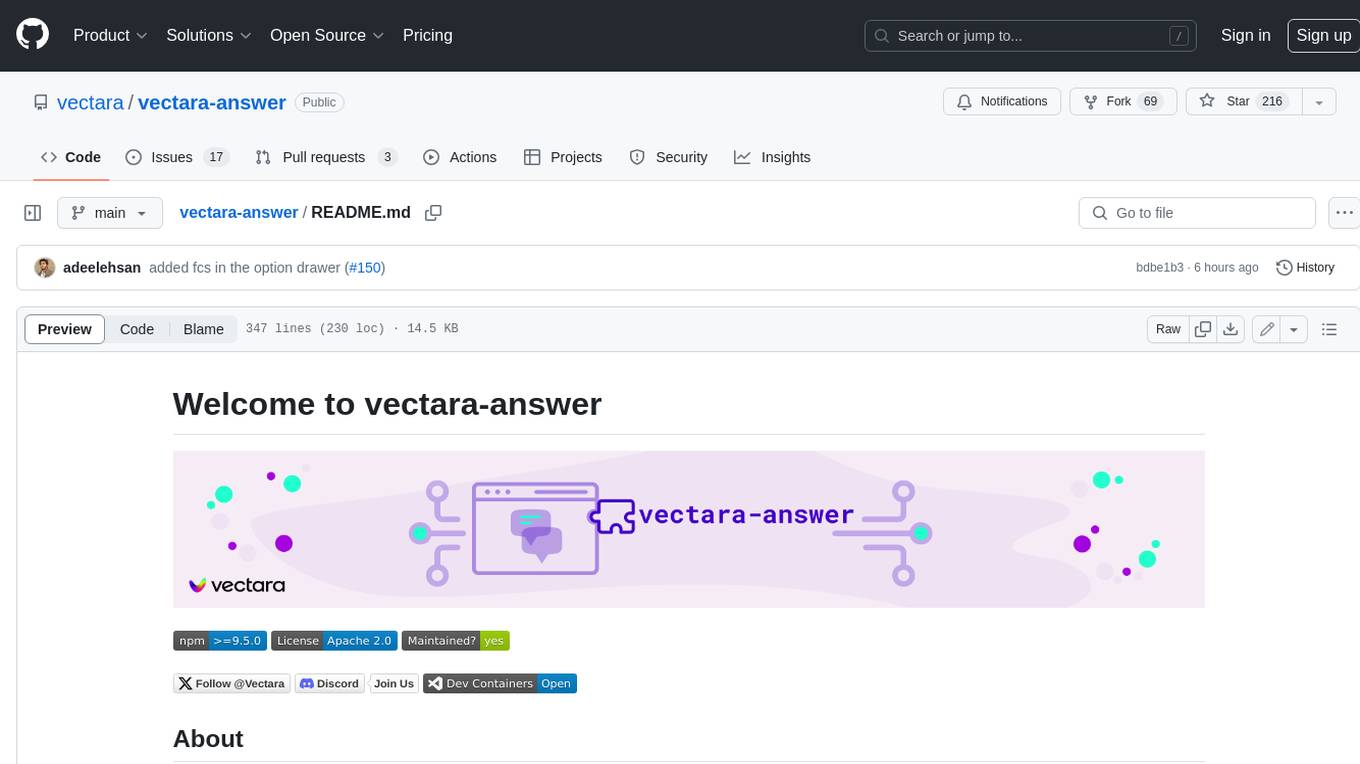
Vectara Answer is a sample app for Vectara-powered Summarized Semantic Search (or question-answering) with advanced configuration options. For examples of what you can build with Vectara Answer, check out Ask News, LegalAid, or any of the other demo applications.
README:
Vectara Answer is a sample app for Vectara-powered Summarized Semantic Search (or question-answering) with advanced configuration options. For examples of what you can build with Vectara Answer, check out Ask News, LegalAid, or any of the other demo applications.
Note: For more user interface options and a simpler codebase, use Create-UI.
[!TIP]
Looking for something else? Try another open-source project:
- React-Chatbot: Add a compact Vectara-powered chatbot widget chat to your React apps.
- Create-UI: The fastest way to generate a working React codebase for a range of generative and semantic search UIs.
- Vectara Ingest: Configurable crawlers for pulling data from many popular data sources and indexing into Vectara.
To get started, the minimum requirement is to install npm and node. That's it!
Vectara Answer comes packaged with preset configurations that allow you to spin up a sample application using Vectara's public datastores. To quickly get started, run the following command:
npm run bootstrap
When prompted for which application to create, select from one of three default apps:
-
Vectara Docs
- Answer questions about Vectara documentation -
Vectara.com
- Answer questions about the content of the Vectara company website -
AskFeynman
- Answer questions about Richard Feynman's lectures
After selecting which application to create, you'll have the app running in your browser at http://localhost:4444
.
Congratulations! You've just setup and run a sample app powered by Vectara!
The bootstrap
command installs dependencies, runs the configuration script to generate an .env
file
(which includes all the needed configuration parameters), and spins up the local application.
If you would like to run the setup steps individually, you can run:
-
npm install
: for installing dependencies -
npm run configure
: for running the configuration script -
npm run start
: for running the application locally
When building your own application, you will need to:
- Create a data store: Log into the Vectara Console and create a corpus.
- Add data to the corpus: You can use Vectara Ingest to crawl datasets and websites, upload files in the Vectara Console, or use our Indexing APIs directly.
[!TIP]
When ingesting data into your corpus you are free to add metadata to any indexed document. By convention, if you add the
url
field as metadata, Vectara Answer will use the value in that field to display a clickable link for each reference or citations it display on the results page.
When running npm run bootstrap
, if you choose [Create Your Own]
from the application selection prompt, you will be asked to provide:
- Your Vectara customer ID
- The ID of the corpus you created
- The API key of your selected Vectara corpus (NOTE: Depending on your set up, this may be visible to users. To ensure safe sharing, ensure that this key is set up to only have query access.)
- Any sample questions to display on the site, to get your users started.
Once provided, the values above will go into your own customized configuration (.env
file), and your site will be ready to go via npm start
.
By default, Vectara Answer runs locally on your machine using npm run start
. There is also an option to use Vectara Answer with Docker, which also makes it easy to deploy Vectara Answer to a cloud environment.
Please see these detailed instructions for more details on using Docker.
After the configuration process has created your .env
file (as part of the bootstrap process), you are free to make modifications to it to suit your development needs.
Note that the variables in the .env
file all have the REACT_APP
prefix, as is needed to be recognized by Vectara Answer code.
# These config vars are required for connecting to your Vectara data and issuing requests.
customer_id: 123456789
corpus_id: 5
corpus_key: vectara_docs_1
api_key: "zqt_abcdef..."
Notes:
- Vectara APIV2 uses
corpus_key
, but the oldercorpus_id
is also supported for backwards compatibility. We encourage you to usecorpus_key
as we may deprecate support for V1 in the future. -
corpus_key
(orcorpus_id
) can be a set of corpora in which case each query runs against all those corpora. In such a case, the format is a comma-separated list of corpus keys or corpus IDs, for example:
corpus_id: "123,234,345"
OR
corpus_key: "vectara_docs_1,vectara_website_3"
These configuration parameters enable you to configure the look and feel of the search header. The search header may include a logo, a title (text) and a description. Most commonly we just have to define a title and description.
Alternatively, the title can be disabled and instead you can use a logo instead, but note that currently the logo.png
file must be added to the codebase under the config_images
folder.
# Define the title of your application (renders next to the logo, if exists).
search_title: "My application"
# Define the description to render opposite the logo and title.
search_description: "Data that speaks for itself"
# Define the placeholder text inside the search box.
search_placeholder: "Ask me anything"
# Define the URL the browser will redirect to when the user clicks the logo above the search controls.
search_logo_link: "https://asknews.demo.vectara.com"
# Define the logo that appears in the search header. Any images you place in your `config_images` directory will be available.
search_logo_src: "config_images/logo.png"
# Describe the logo for improved accessibility.
search_logo_alt: "Vectara logo"
# Customize the height at which to render the logo. The width will scale proportionately.
search_logo_height: 20
The way summarization works can be configured as follows:
# Switches the mode of the ux to "summary" mode or "search" mode (if not specified defaults to "summary" mode). When set to "summary", a summary is shown along with references (citations) used in the summary. When set to "search", only search results are shown and no call is made to the summarization API.
ux: "summary"
# Default language for summary response (if not specified defaults to "auto").
# accepts two-letter code (en) or three-letter code (eng)
summary_default_language: "eng"
# Number of sentences before and after relevant text segment used for summarization.
summary_num_sentences: 3
# Number of results used for summarization.
summary_num_results: 10
# The name of the summarization prompt in Vectara.
# If unspecified, the default for the account is used.
# See https://docs.vectara.com/docs/learn/grounded-generation/select-a-summarizer for available summarization prompts. Note that some prompts are only available to Vectara scale customers.
summary_prompt_name: vectara-summary-ext-v1.2.0
# Filename for a local file (same folder as your config.yaml file) which includes the text for a custom prompt
# If specified, used to replace the prompt text used by the prompt_name. You can see an example prompt.txt file in the config/ask-feynman folder.
# Note this is a Scale only feature and is not yet enabled for streaming.
summary_prompt_text_filename: prompt.txt
# Whether to disable or enable factual consistency score as (score or badge) based on the HHEMv2 (based on https://huggingface.co/vectara/hallucination_evaluation_model).
# default value is disable. To enable it set it to score or badge.
summary_fcs_mode: score
# Add multiple prompts option for the summary.
summary_prompt_options: vectara-summary-ext-24-05-med-omni,vectara-summary-ext-24-05-sml
To stream the results, set the following parameter to True
.
# Streaming on/off for summarization
enable_stream_query: True
Hybrid search is a capability that combines the strength of neural (semantic) search with traditional keywords search. By default, Vectara Answer utilizes hybrid search with lambda=0.1 for short queries (num_words<=2) and lambda=0.0 (pure neural search) otherwise, but you can define other values here.
# hybrid search
hybrid_search_num_words: 2
hybrid_search_lambda_long: 0.0
hybrid_search_lambda_short: 0.1
Vectara Answer can display an application header and footer for branding purposes. These configuration parameters allow you to configure the look and feel of this header and footer.
# Hide or show the app header.
enable_app_header: False
# Hide or show the app footer.
enable_app_footer: False
# Define the title of your app to render in the browser tab.
app_title: "Your application title here"
# Define the URL the browser will redirect to when the user clicks the logo in the app header.
app_header_logo_link: "https://www.vectara.com"
# Define the logo that appears in the app header. Any images you place in your `config_images` directory will be available.
app_header_logo_src: "config_images/logo.png"
# Describe the logo for improved accessibility.
app_header_logo_alt: "Vectara logo"
# Customize the height at which to render the logo. The width will scale proportionately.
app_header_logo_height: 20
If your application uses more than one corpus, you can define source filters to enable the user to narrow their search to a specific corpus. This feature assumes the following:
- You have defined a
source
meta-data field on the Vectara corpus - During data ingestion, you've added the source text to each document appropriately (in the
source
metadata field)
The following parameters control how the sources feature works:
# Hide or show source filters.
enable_source_filters: True
# whether the "all source" button should be enabled or not (default True)
all_sources: True
# A comma-separated list of the sources on which users can filter.
sources: "BBC,NPR,FOX,CNBC,CNN"
The sources
parameters is a comma-separated list of source names that will be displayed underneath the search bar, and the user can select
if results returned should be from "all source" or one of the selected sources.
In this case you must specify corpus_id
(see above) to be the list of matching corpus IDs, also comma separated.
For example:
enable_source_filters: True
all_sources: True
sources: "BBC,NPR,FOX,CNBC,CNN"
corpus_id: "123,124,125,126,127"
If all_sources
is set to False, the application will only display the individual source but not the "All sources" button.
This means the user will only be able to select a specific source for each query.
Whether to use Vectara's reranking functionality. Note that reranking currently works for English language only, so if the documents in your corpus are in other languages, it's recommended to set this to "False".
# choose a reranker from the following choices, You can use single or multiple rerankers with coma separated. e.g `slingshot,mmr`
# if you are using multiple reranker please make sure slingshot should be first if you are using it. For more details [chain reranking](https://docs.vectara.com/docs/learn/chain-reranker)
reranker_name: normal | slingshot | mmr | userfn | slingshot,mmr,userfn
# number of results to use for reranking
rerank_num_results: 50
# enables users to define custom reranking functions using document-level metadata, part-level metadata.
user_function: "if (now() < iso_datetime_parse('2024-12-04T10:14:50Z')) 1 else 2"
To use Vectara's MMR (Maximum Marginal Relevance) functionality please set the reranker_name = mmr
, and add a mmr_diversity_bias
value.
# Diversity bias factor (0..1) for MMR reranker. The higher the value, the more MMR is preferred over relevance.
mmr_diversity_bias: 0.3
vectara-answer
supports Google SSO front-end authentication. Note this is not a fully secure SSO solution, and you should consult your security experts for in a full enterprise deployment.
# Configure your app to require the user to log in with Google SSO.
authenticate: True
google_client_id: "cb67dbce87wcc"
# Track user interaction with your app using Google Analytics.
google_analytics_tracking_code: "123456789"
# Track user interaction with your app using Google Tag Manager.
gtm_container_id: "GTM-1234567"
Customize the result display, this provides options to tailor the presentation of the results shown.
# Enable the "explore related content" link, which does a search for content related to the text in each results's matching snippet
related_content: True
# Track user experience with Full Story
full_story_org_id: "org1123"
In addition to customization via configuration, you can customize Vectara Answer further by modifying its code directly in your own fork of the repository.
The UI source code is all in the src/
directory. See the UI README.md to learn how to make changes to the UI source.
While npm run start
runs the application with a local client that accesses the Vectara API directly, running the app via Docker (see below) spins up a full-stack solution, using a proxy server to make Vectara API requests.
To modify the request handlers, make changes to /server/index.js
.
👤 Vectara
- Website: https://vectara.com
- Twitter: @vectara
- GitHub: @vectara
- LinkedIn: @vectara
- Discord: @vectara
Contributions, issues and feature requests are welcome!
Feel free to check issues page. You can also take a look at the contributing guide.
Give a ⭐️ if this project helped you!
Copyright © 2024 Vectara.
This project is Apache 2.0 licensed.
For Tasks:
Click tags to check more tools for each tasksFor Jobs:
Alternative AI tools for vectara-answer
Similar Open Source Tools
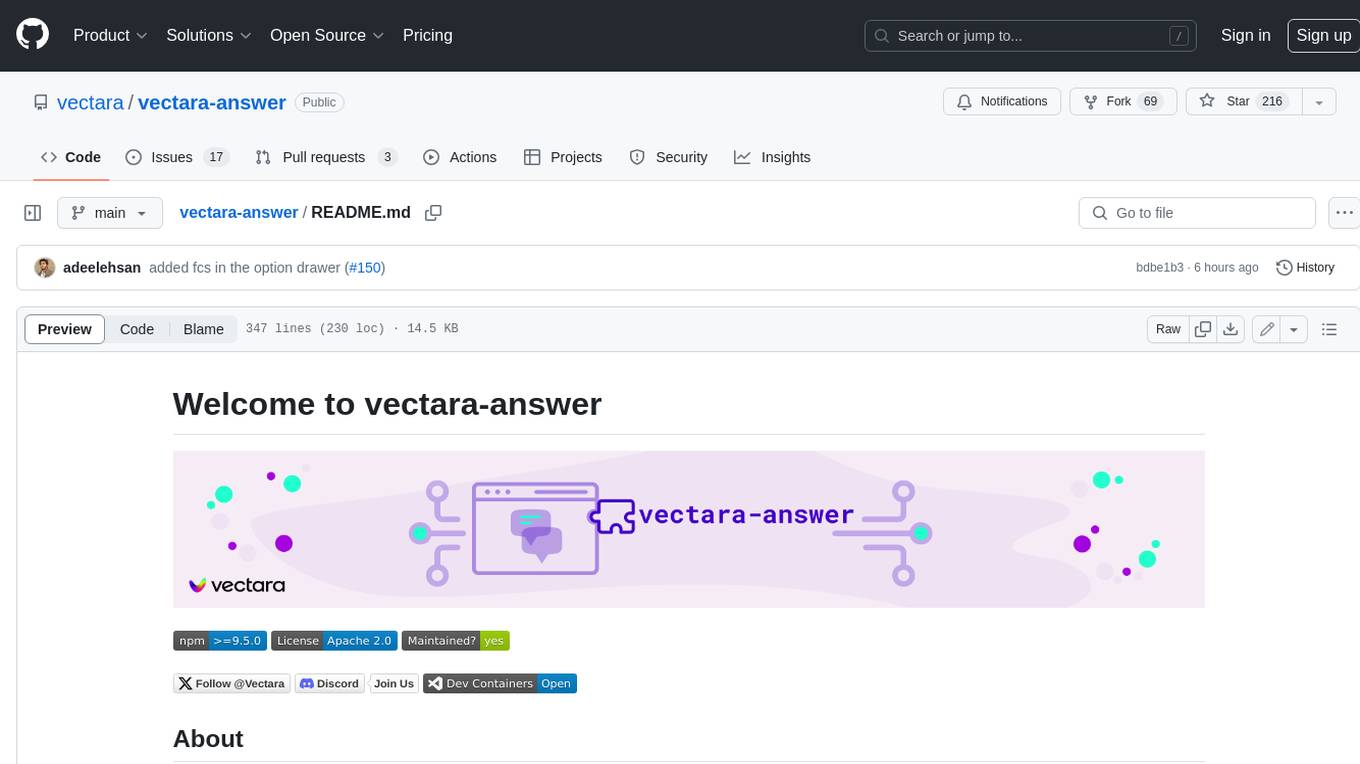
vectara-answer
Vectara Answer is a sample app for Vectara-powered Summarized Semantic Search (or question-answering) with advanced configuration options. For examples of what you can build with Vectara Answer, check out Ask News, LegalAid, or any of the other demo applications.
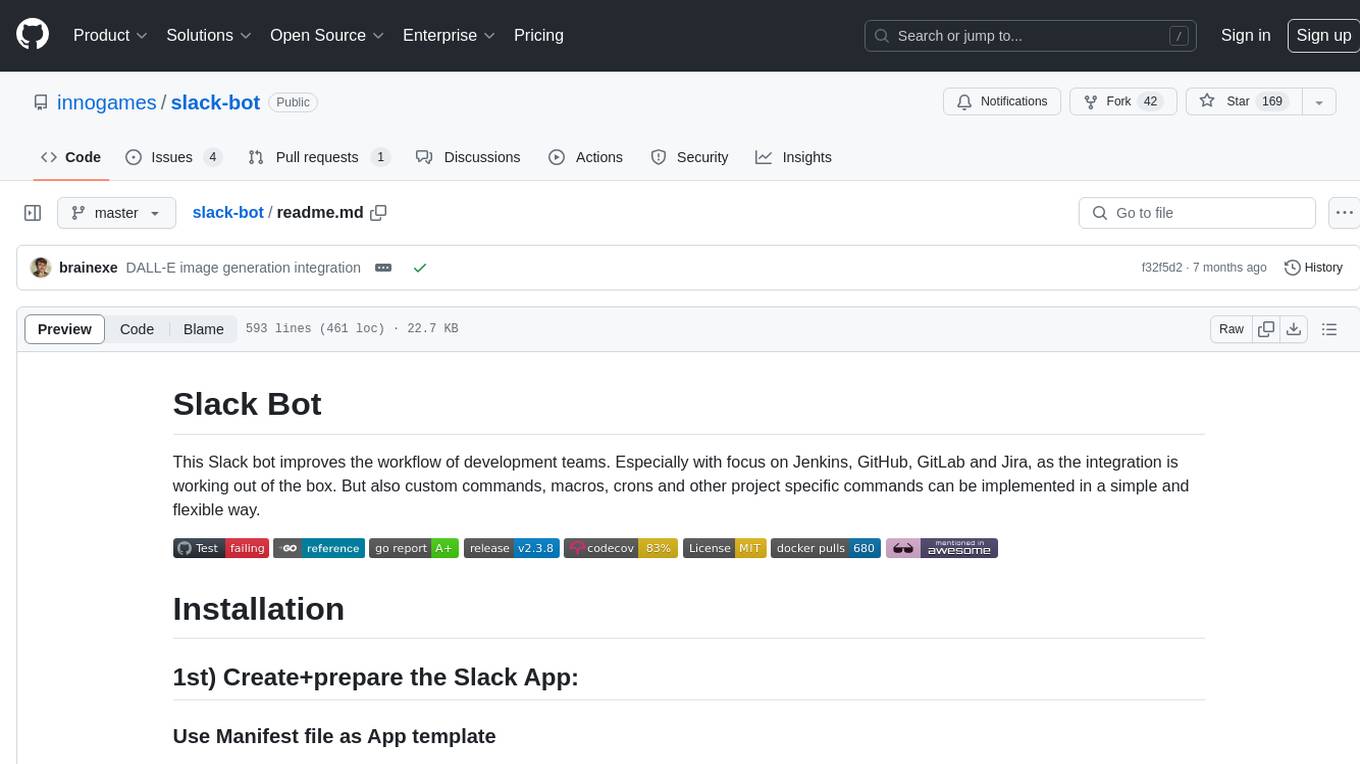
slack-bot
The Slack Bot is a tool designed to enhance the workflow of development teams by integrating with Jenkins, GitHub, GitLab, and Jira. It allows for custom commands, macros, crons, and project-specific commands to be implemented easily. Users can interact with the bot through Slack messages, execute commands, and monitor job progress. The bot supports features like starting and monitoring Jenkins jobs, tracking pull requests, querying Jira information, creating buttons for interactions, generating images with DALL-E, playing quiz games, checking weather, defining custom commands, and more. Configuration is managed via YAML files, allowing users to set up credentials for external services, define custom commands, schedule cron jobs, and configure VCS systems like Bitbucket for automated branch lookup in Jenkins triggers.
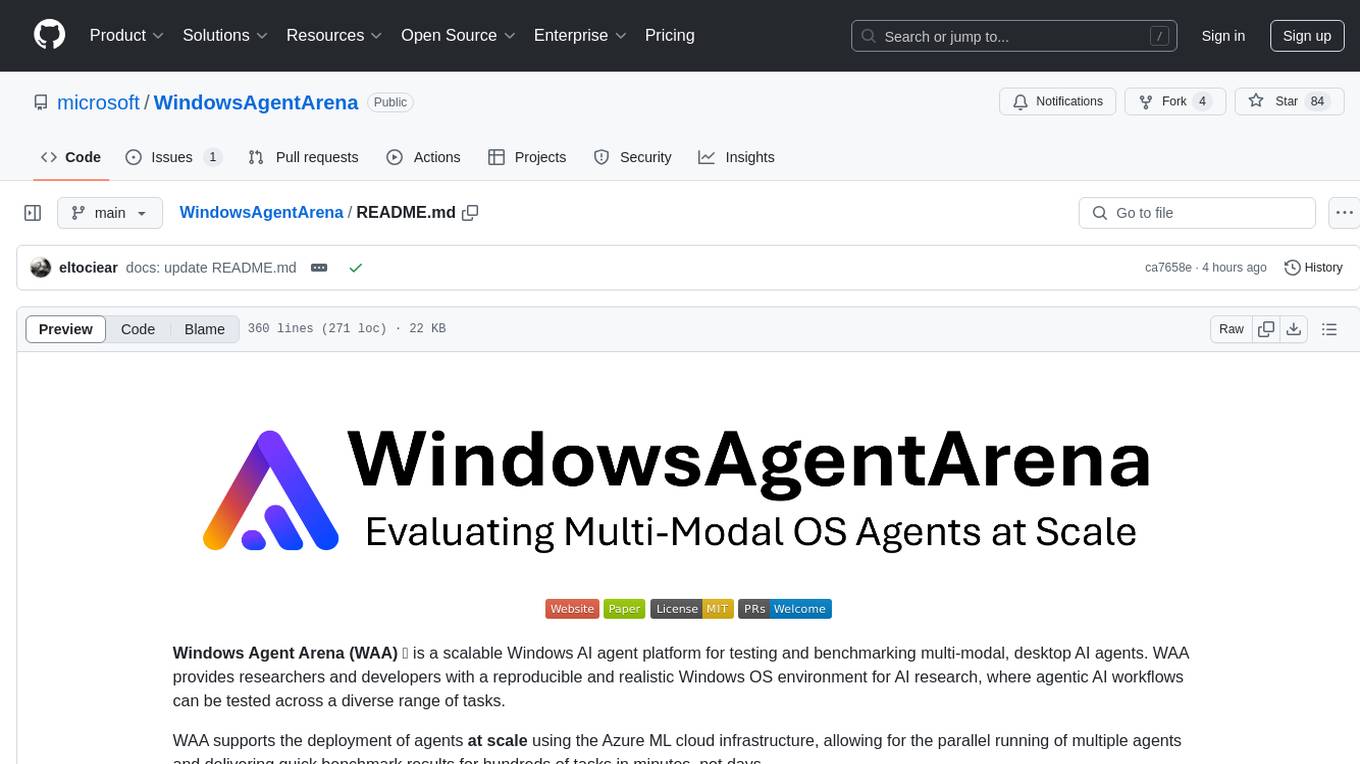
WindowsAgentArena
Windows Agent Arena (WAA) is a scalable Windows AI agent platform designed for testing and benchmarking multi-modal, desktop AI agents. It provides researchers and developers with a reproducible and realistic Windows OS environment for AI research, enabling testing of agentic AI workflows across various tasks. WAA supports deploying agents at scale using Azure ML cloud infrastructure, allowing parallel running of multiple agents and delivering quick benchmark results for hundreds of tasks in minutes.
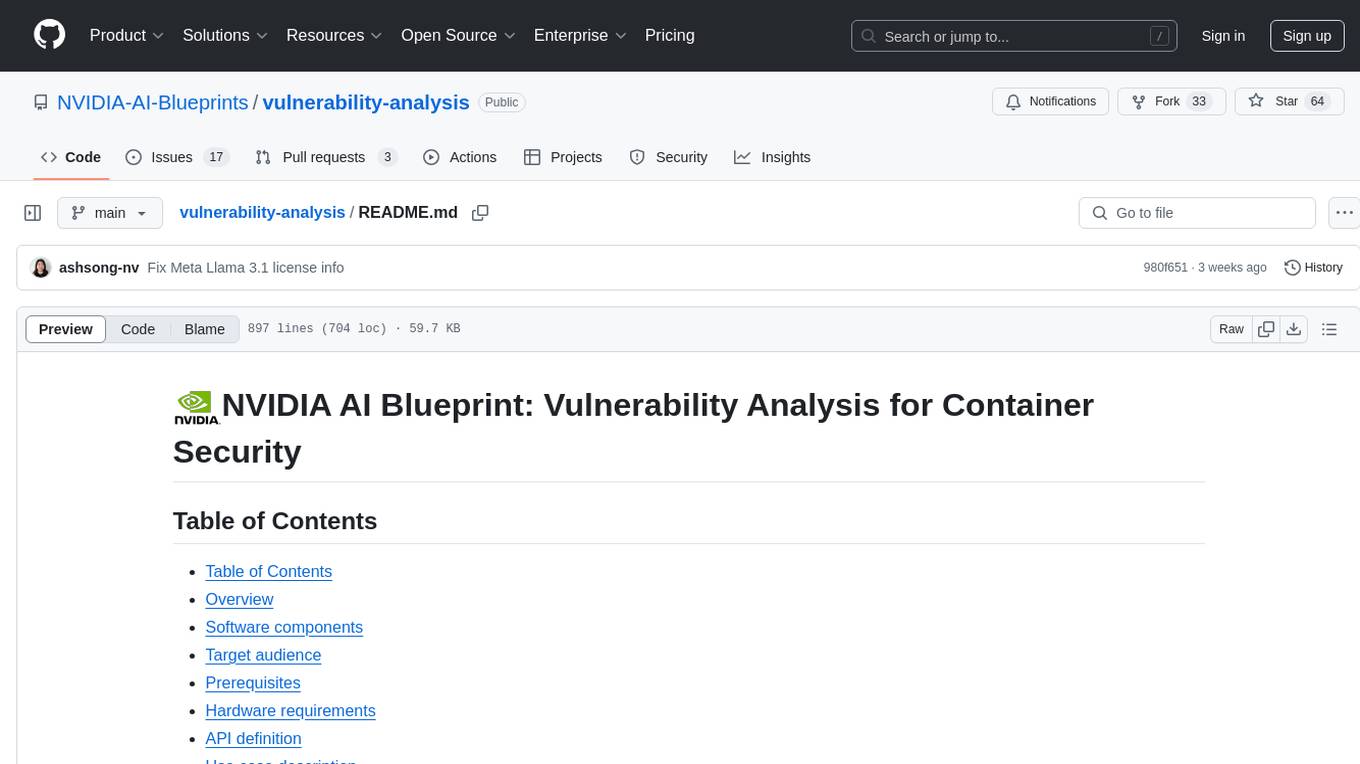
vulnerability-analysis
The NVIDIA AI Blueprint for Vulnerability Analysis for Container Security showcases accelerated analysis on common vulnerabilities and exposures (CVE) at an enterprise scale, reducing mitigation time from days to seconds. It enables security analysts to determine software package vulnerabilities using large language models (LLMs) and retrieval-augmented generation (RAG). The blueprint is designed for security analysts, IT engineers, and AI practitioners in cybersecurity. It requires NVAIE developer license and API keys for vulnerability databases, search engines, and LLM model services. Hardware requirements include L40 GPU for pipeline operation and optional LLM NIM and Embedding NIM. The workflow involves LLM pipeline for CVE impact analysis, utilizing LLM planner, agent, and summarization nodes. The blueprint uses NVIDIA NIM microservices and Morpheus Cybersecurity AI SDK for vulnerability analysis.
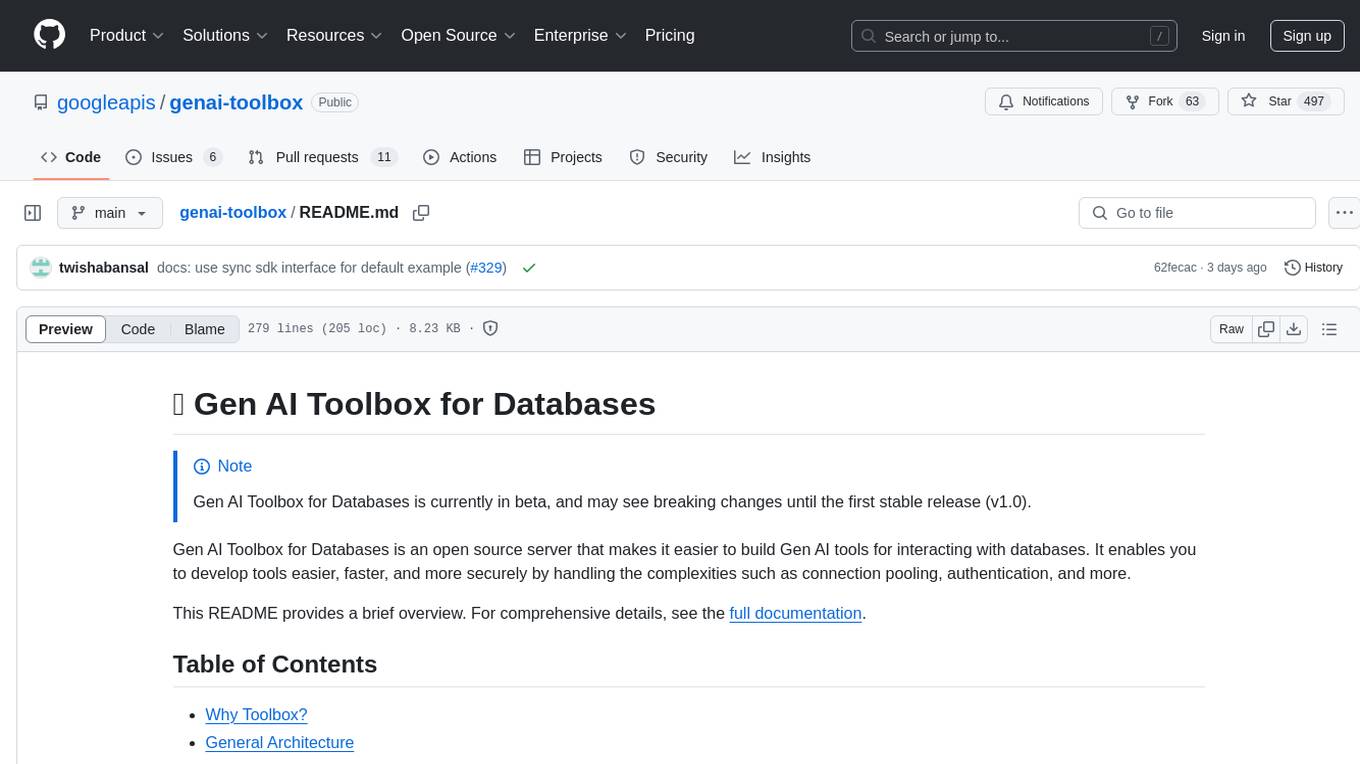
genai-toolbox
Gen AI Toolbox for Databases is an open source server that simplifies building Gen AI tools for interacting with databases. It handles complexities like connection pooling, authentication, and more, enabling easier, faster, and more secure tool development. The toolbox sits between the application's orchestration framework and the database, providing a control plane to modify, distribute, or invoke tools. It offers simplified development, better performance, enhanced security, and end-to-end observability. Users can install the toolbox as a binary, container image, or compile from source. Configuration is done through a 'tools.yaml' file, defining sources, tools, and toolsets. The project follows semantic versioning and welcomes contributions.
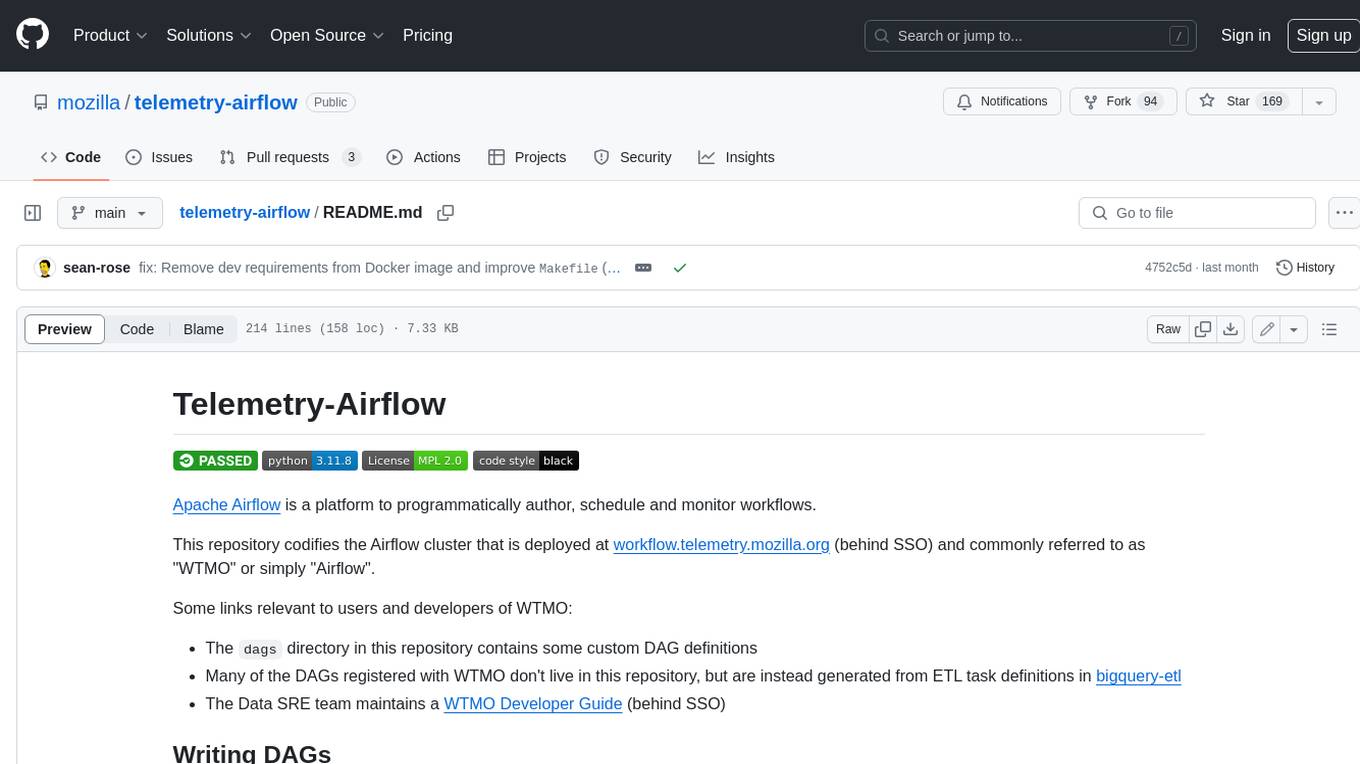
telemetry-airflow
This repository codifies the Airflow cluster that is deployed at workflow.telemetry.mozilla.org (behind SSO) and commonly referred to as "WTMO" or simply "Airflow". Some links relevant to users and developers of WTMO: * The `dags` directory in this repository contains some custom DAG definitions * Many of the DAGs registered with WTMO don't live in this repository, but are instead generated from ETL task definitions in bigquery-etl * The Data SRE team maintains a WTMO Developer Guide (behind SSO)
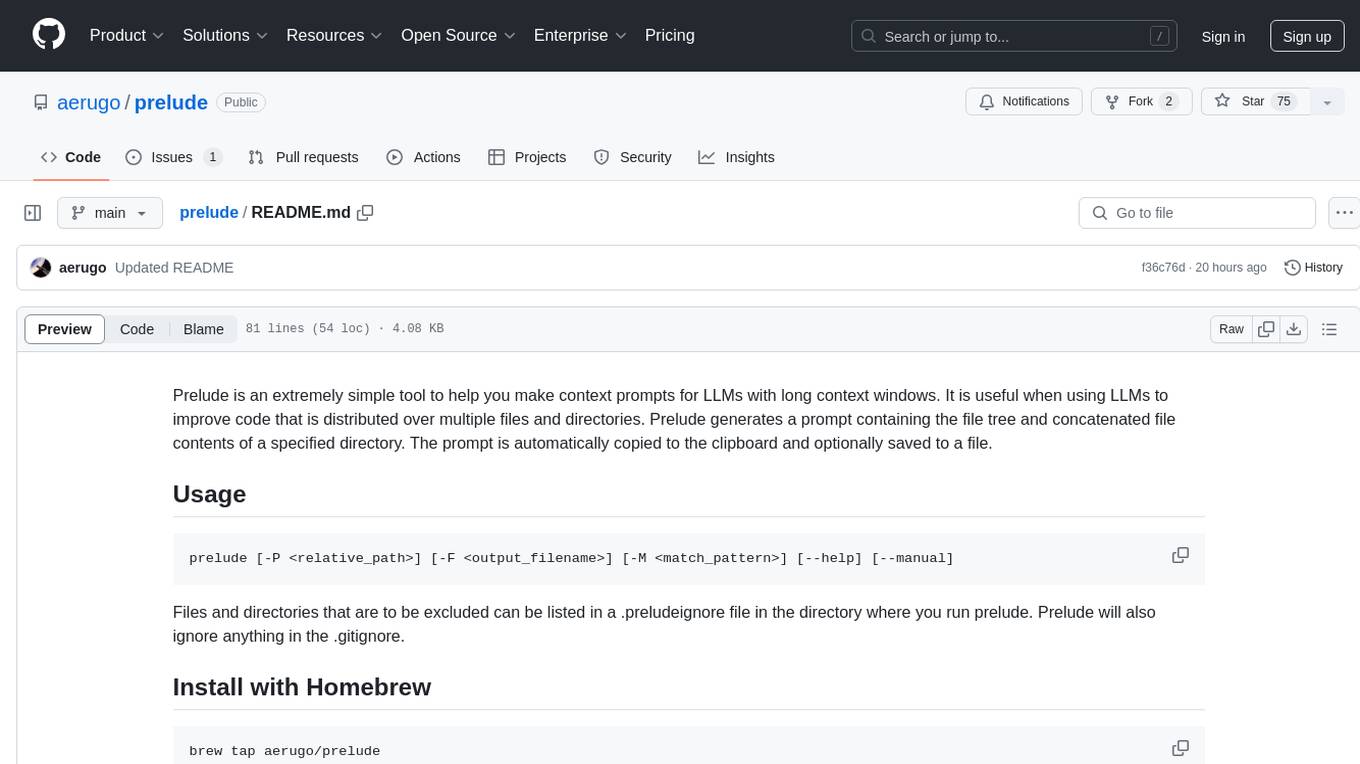
prelude
Prelude is a simple tool for creating context prompts for LLMs with long context windows. It helps improve code distributed over multiple files by generating prompts with file tree and concatenated file contents. The prompt is copied to clipboard and can be saved to a file. It excludes files listed in .preludeignore and .gitignore files. The tool requires the `tree` command to be installed on the system for functionality.
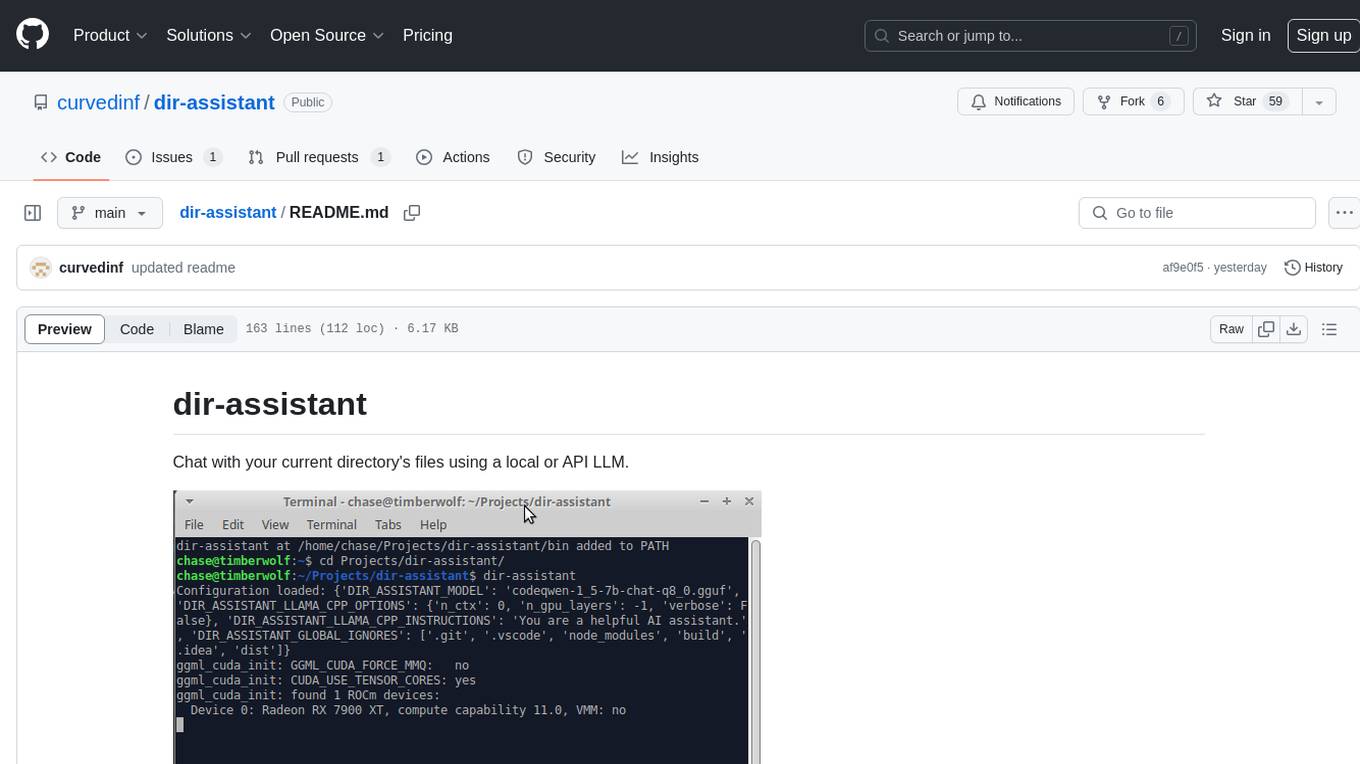
dir-assistant
Dir-assistant is a tool that allows users to interact with their current directory's files using local or API Language Models (LLMs). It supports various platforms and provides API support for major LLM APIs. Users can configure and customize their local LLMs and API LLMs using the tool. Dir-assistant also supports model downloads and configurations for efficient usage. It is designed to enhance file interaction and retrieval using advanced language models.
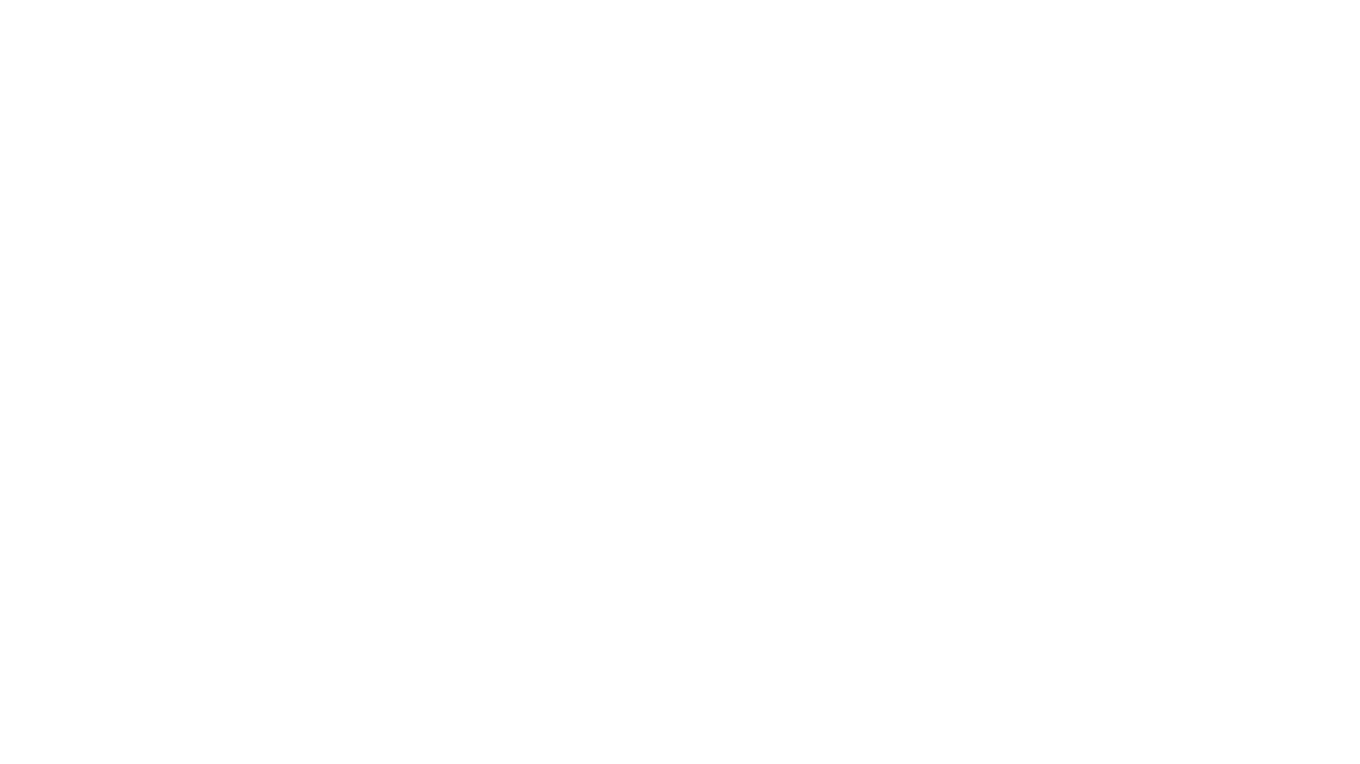
reader
Reader is a tool that converts any URL to an LLM-friendly input with a simple prefix `https://r.jina.ai/`. It improves the output for your agent and RAG systems at no cost. Reader supports image reading, captioning all images at the specified URL and adding `Image [idx]: [caption]` as an alt tag. This enables downstream LLMs to interact with the images in reasoning, summarizing, etc. Reader offers a streaming mode, useful when the standard mode provides an incomplete result. In streaming mode, Reader waits a bit longer until the page is fully rendered, providing more complete information. Reader also supports a JSON mode, which contains three fields: `url`, `title`, and `content`. Reader is backed by Jina AI and licensed under Apache-2.0.
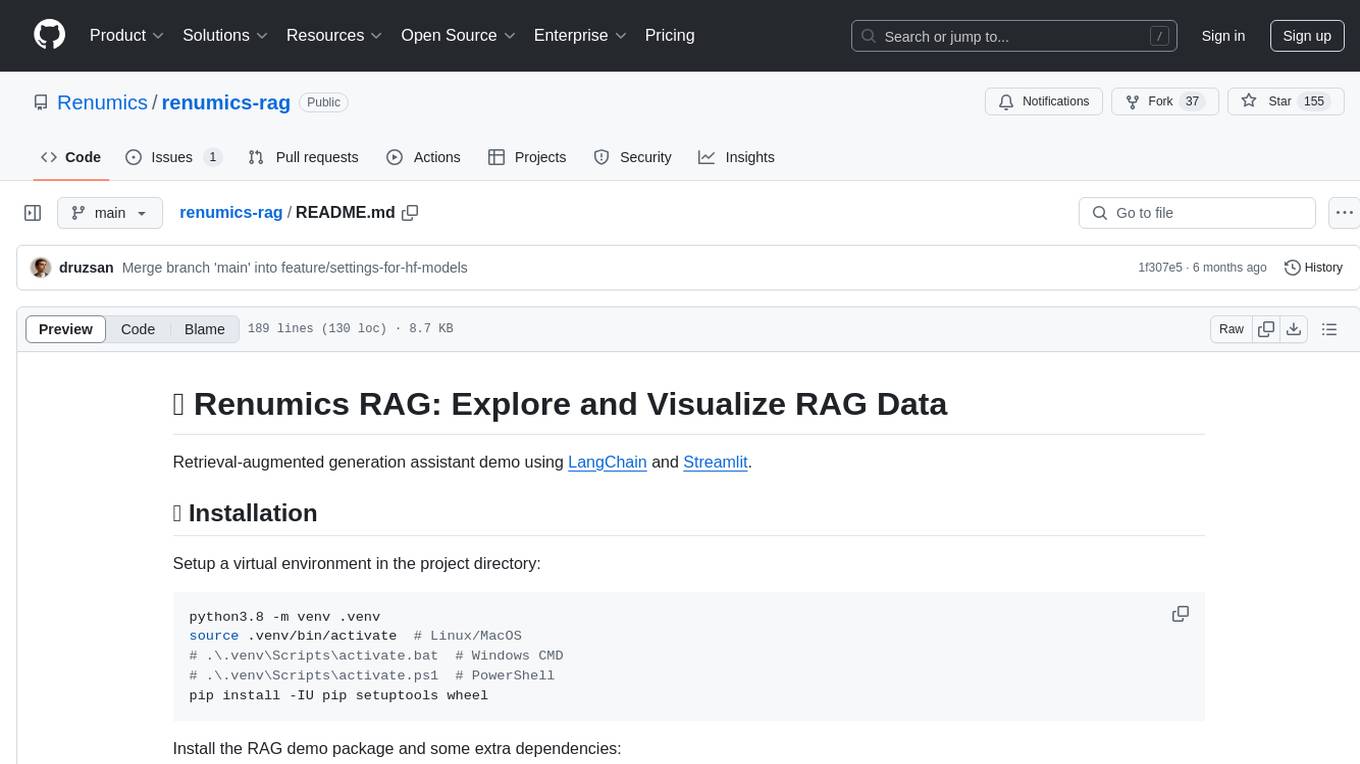
renumics-rag
Renumics RAG is a retrieval-augmented generation assistant demo that utilizes LangChain and Streamlit. It provides a tool for indexing documents and answering questions based on the indexed data. Users can explore and visualize RAG data, configure OpenAI and Hugging Face models, and interactively explore questions and document snippets. The tool supports GPU and CPU setups, offers a command-line interface for retrieving and answering questions, and includes a web application for easy access. It also allows users to customize retrieval settings, embeddings models, and database creation. Renumics RAG is designed to enhance the question-answering process by leveraging indexed documents and providing detailed answers with sources.
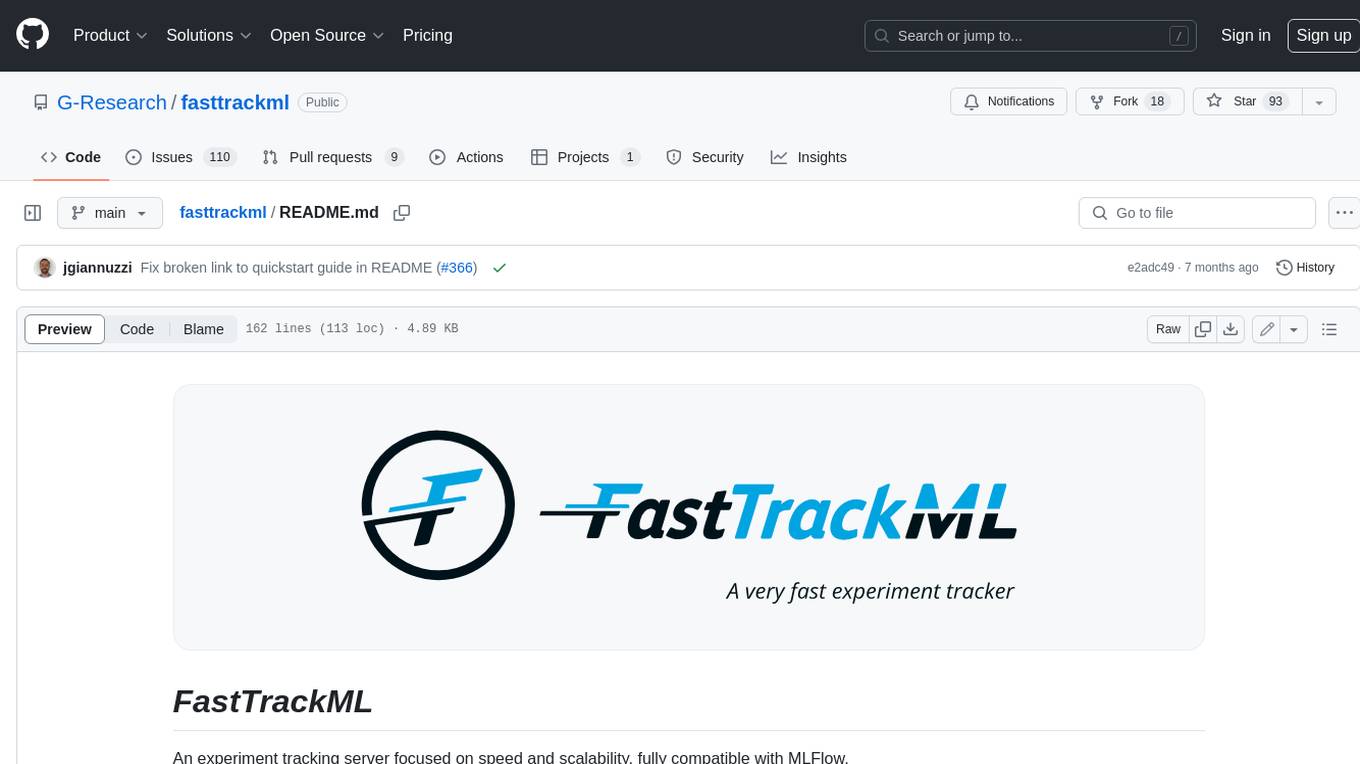
fasttrackml
FastTrackML is an experiment tracking server focused on speed and scalability, fully compatible with MLFlow. It provides a user-friendly interface to track and visualize your machine learning experiments, making it easy to compare different models and identify the best performing ones. FastTrackML is open source and can be easily installed and run with pip or Docker. It is also compatible with the MLFlow Python package, making it easy to integrate with your existing MLFlow workflows.
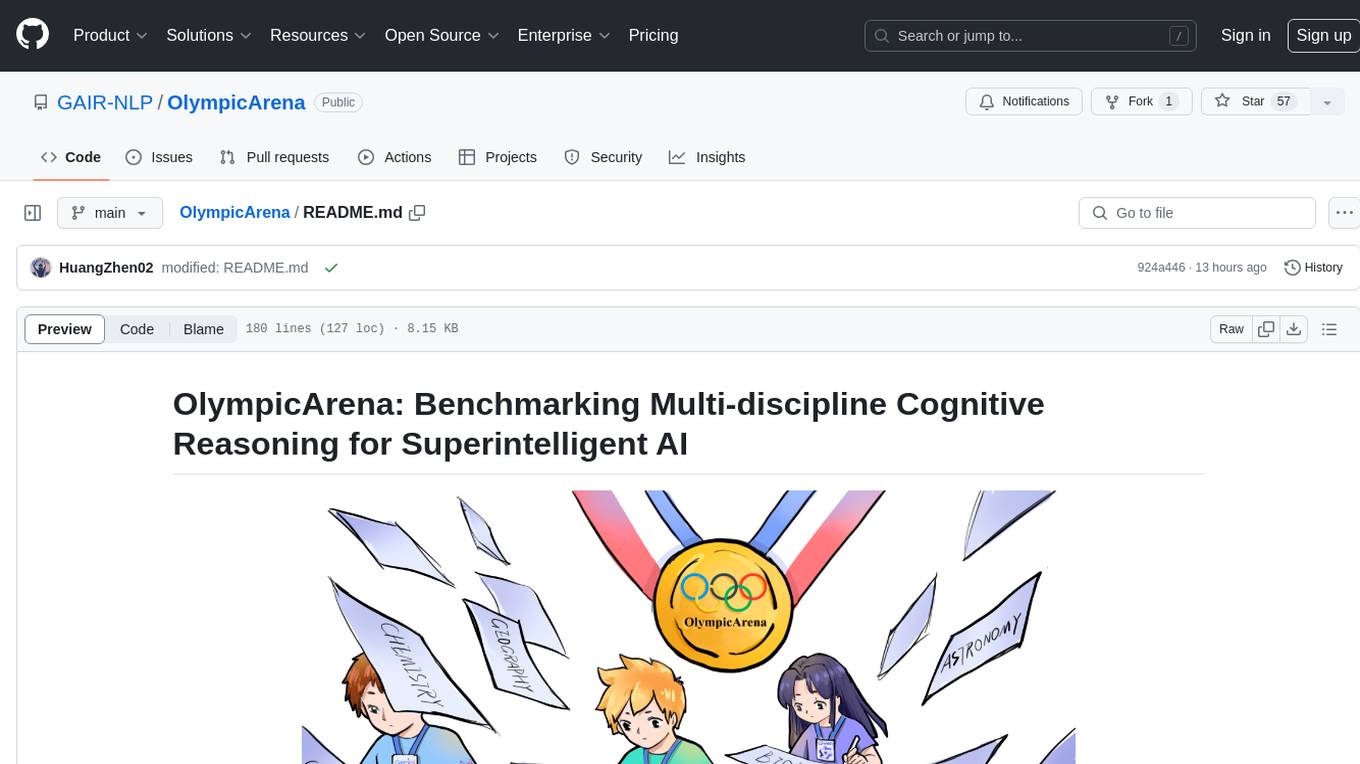
OlympicArena
OlympicArena is a comprehensive benchmark designed to evaluate advanced AI capabilities across various disciplines. It aims to push AI towards superintelligence by tackling complex challenges in science and beyond. The repository provides detailed data for different disciplines, allows users to run inference and evaluation locally, and offers a submission platform for testing models on the test set. Additionally, it includes an annotation interface and encourages users to cite their paper if they find the code or dataset helpful.
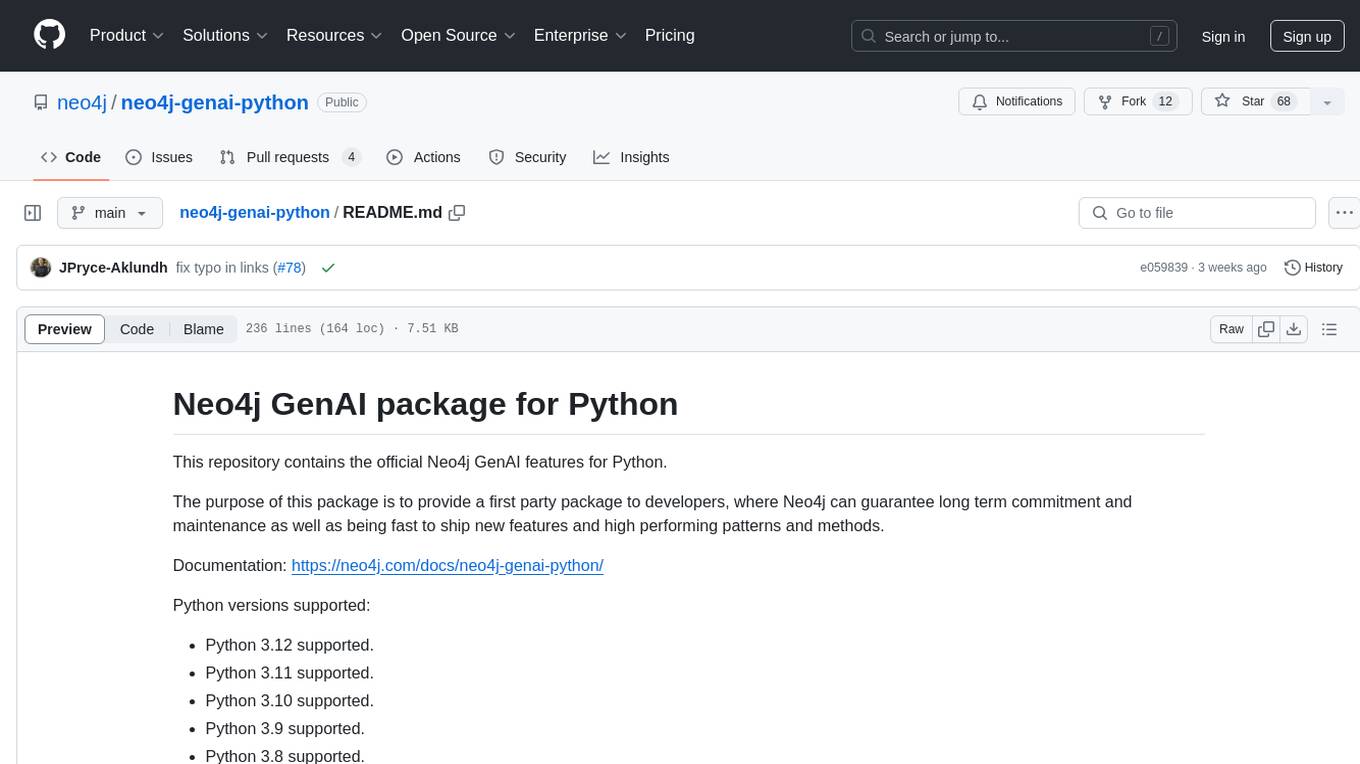
neo4j-genai-python
This repository contains the official Neo4j GenAI features for Python. The purpose of this package is to provide a first-party package to developers, where Neo4j can guarantee long-term commitment and maintenance as well as being fast to ship new features and high-performing patterns and methods.
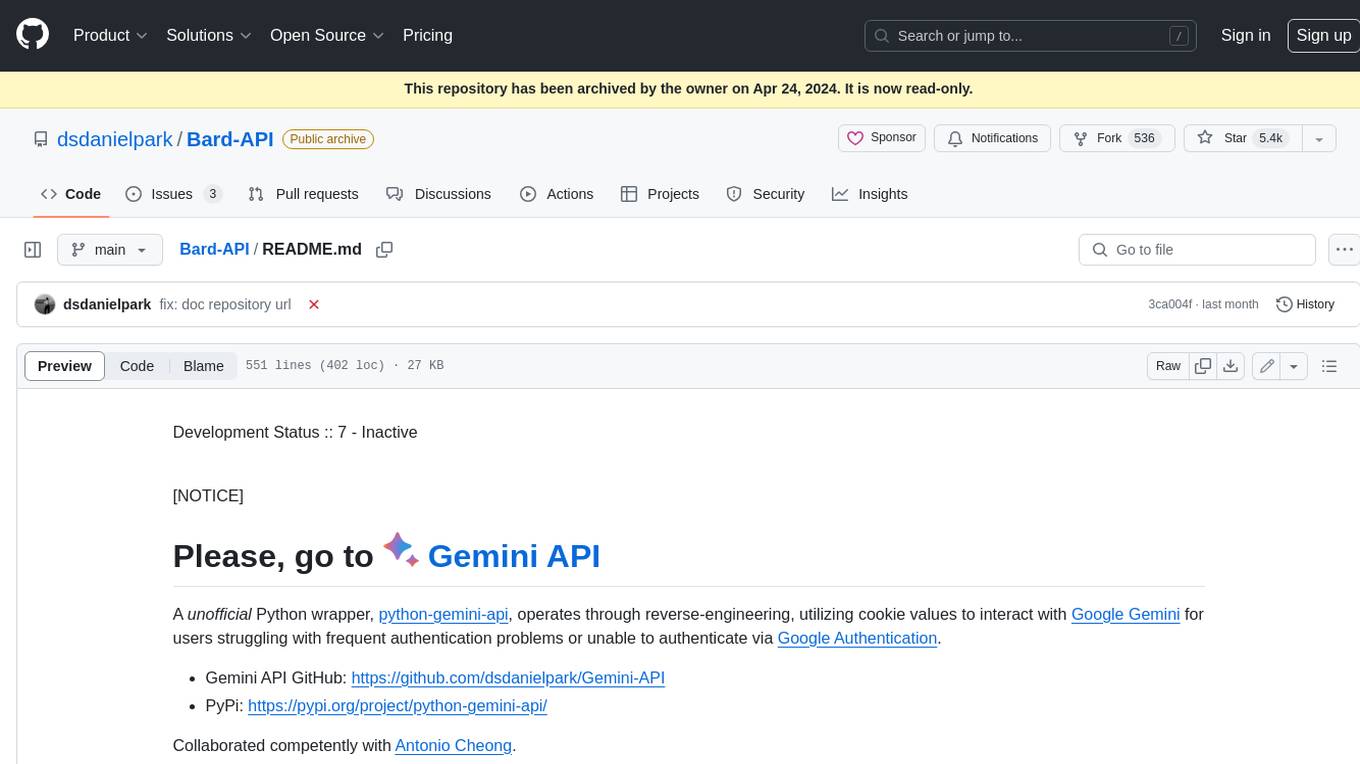
Bard-API
The Bard API is a Python package that returns responses from Google Bard through the value of a cookie. It is an unofficial API that operates through reverse-engineering, utilizing cookie values to interact with Google Bard for users struggling with frequent authentication problems or unable to authenticate via Google Authentication. The Bard API is not a free service, but rather a tool provided to assist developers with testing certain functionalities due to the delayed development and release of Google Bard's API. It has been designed with a lightweight structure that can easily adapt to the emergence of an official API. Therefore, using it for any other purposes is strongly discouraged. If you have access to a reliable official PaLM-2 API or Google Generative AI API, replace the provided response with the corresponding official code. Check out https://github.com/dsdanielpark/Bard-API/issues/262.
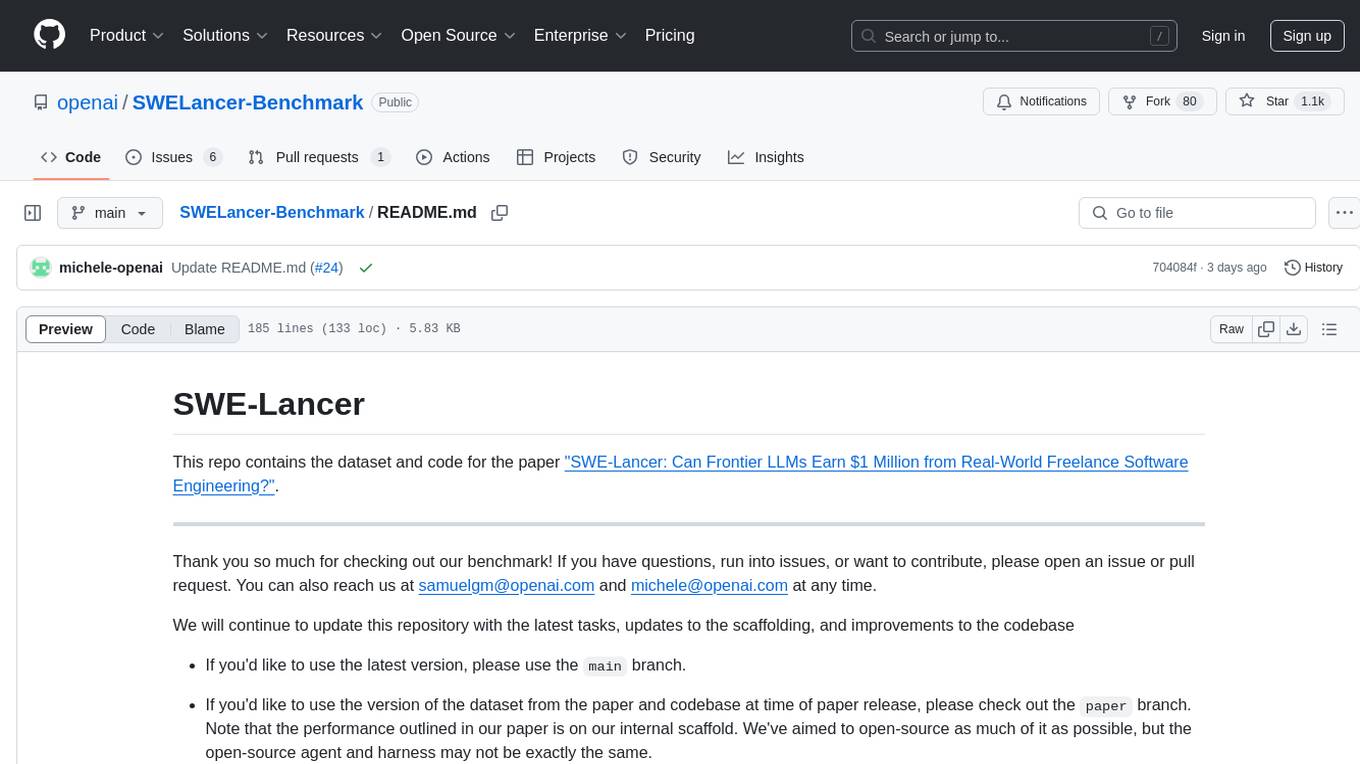
SWELancer-Benchmark
SWE-Lancer is a benchmark repository containing datasets and code for the paper 'SWE-Lancer: Can Frontier LLMs Earn $1 Million from Real-World Freelance Software Engineering?'. It provides instructions for package management, building Docker images, configuring environment variables, and running evaluations. Users can use this tool to assess the performance of language models in real-world freelance software engineering tasks.
For similar tasks
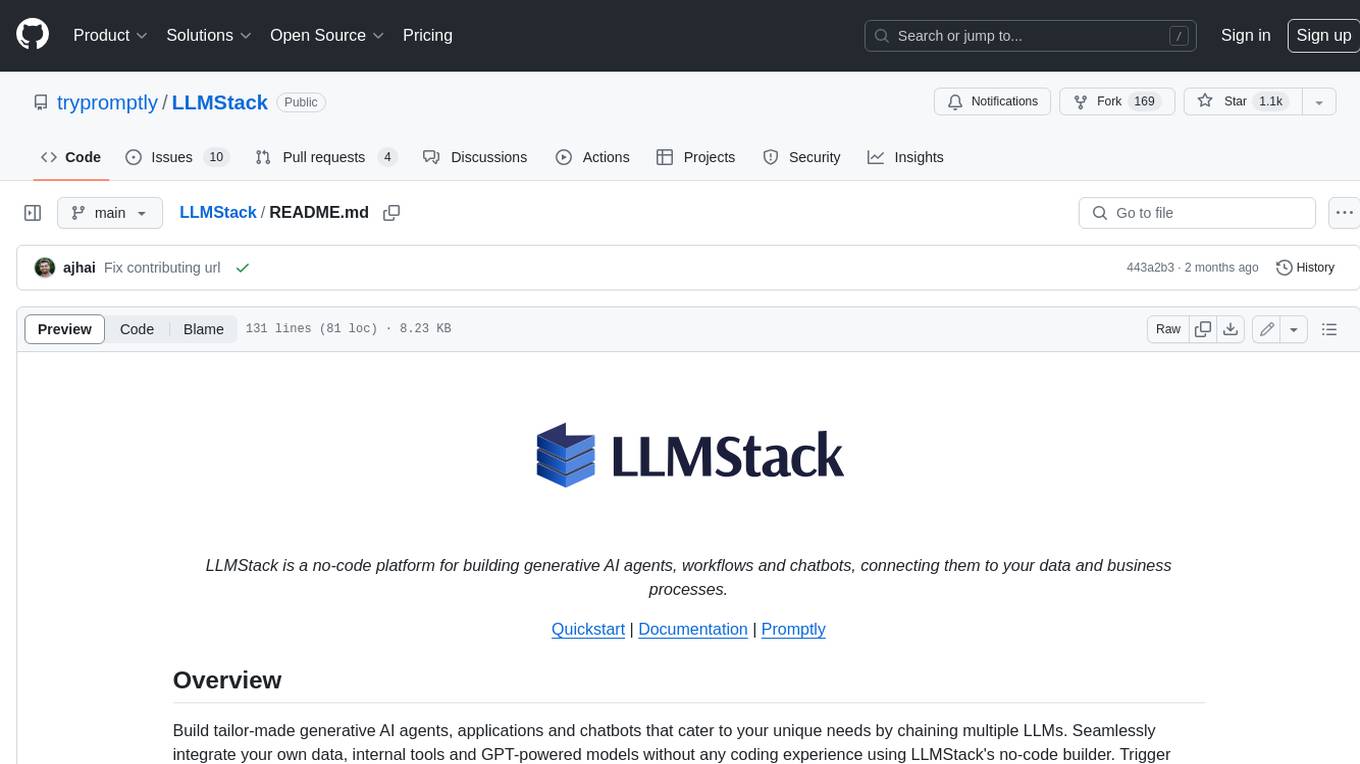
LLMStack
LLMStack is a no-code platform for building generative AI agents, workflows, and chatbots. It allows users to connect their own data, internal tools, and GPT-powered models without any coding experience. LLMStack can be deployed to the cloud or on-premise and can be accessed via HTTP API or triggered from Slack or Discord.
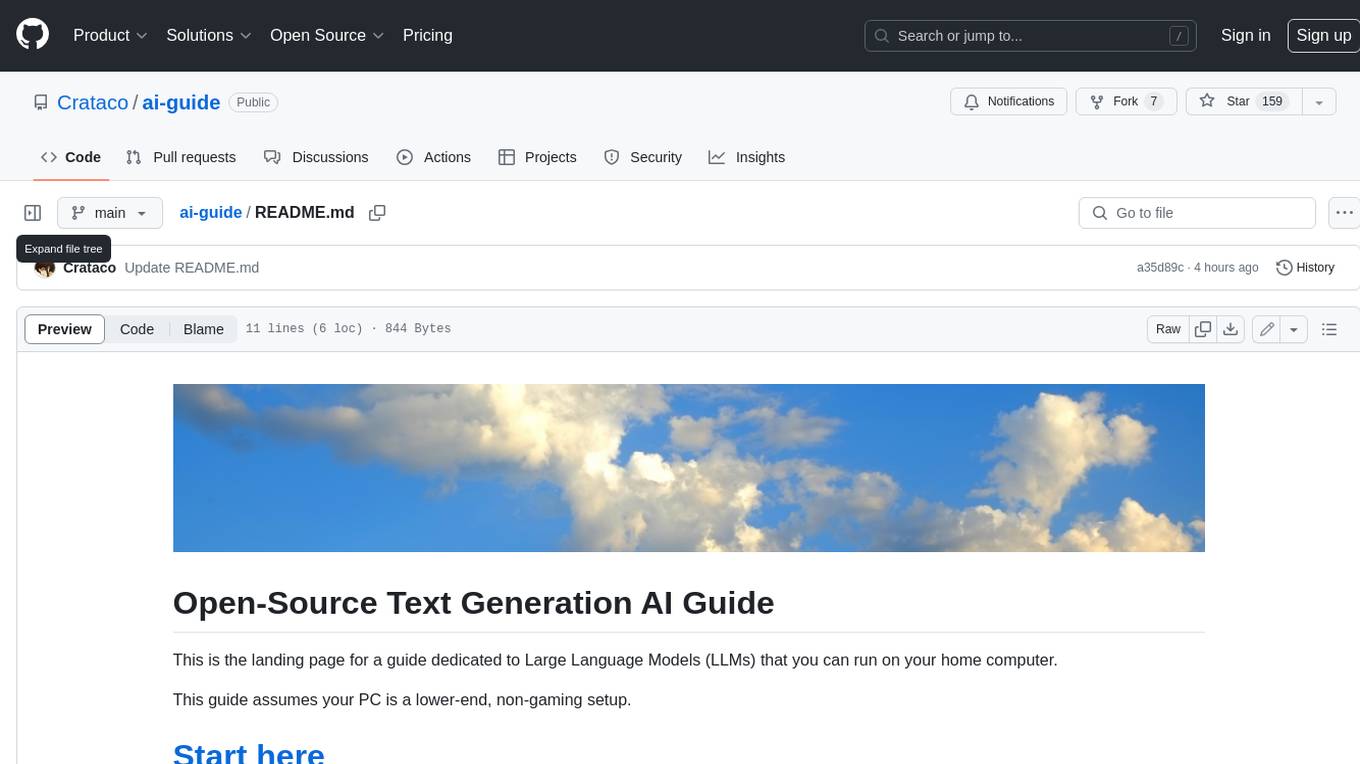
ai-guide
This guide is dedicated to Large Language Models (LLMs) that you can run on your home computer. It assumes your PC is a lower-end, non-gaming setup.
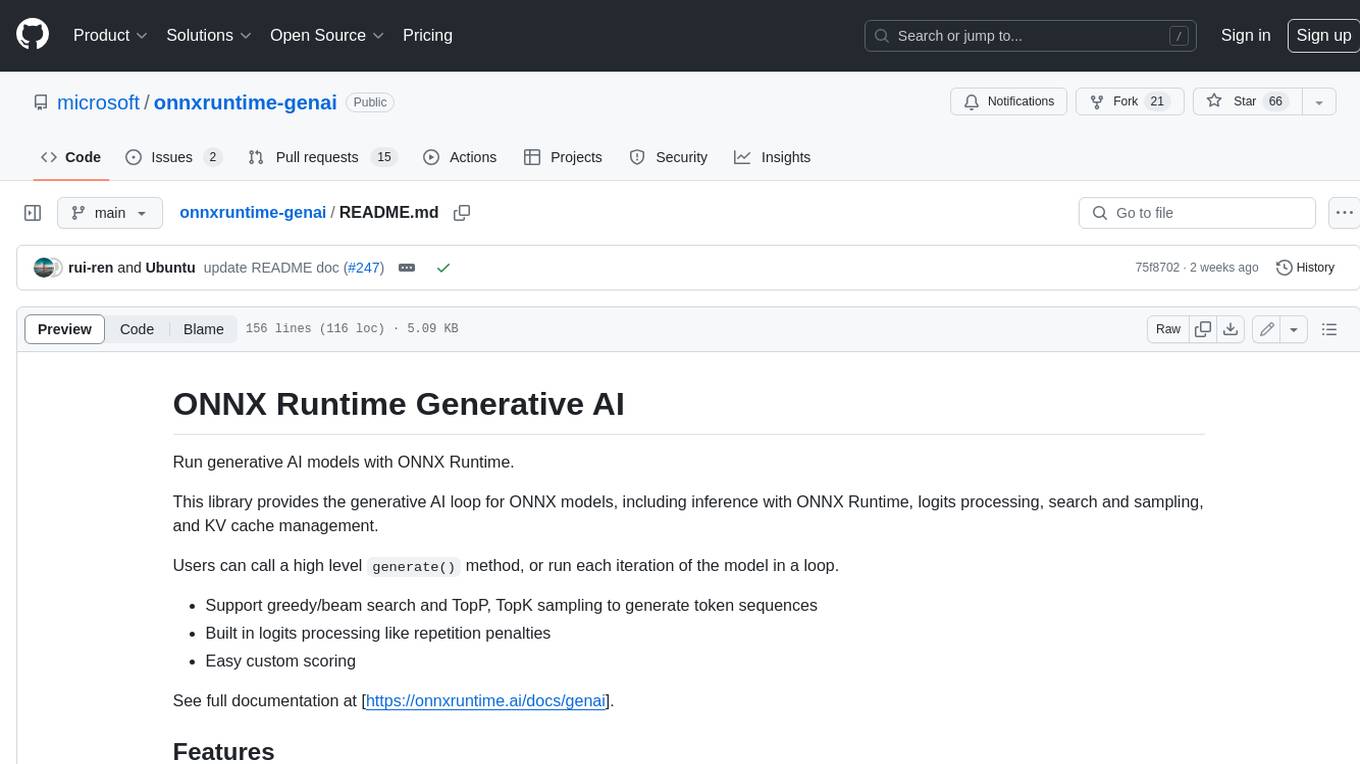
onnxruntime-genai
ONNX Runtime Generative AI is a library that provides the generative AI loop for ONNX models, including inference with ONNX Runtime, logits processing, search and sampling, and KV cache management. Users can call a high level `generate()` method, or run each iteration of the model in a loop. It supports greedy/beam search and TopP, TopK sampling to generate token sequences, has built in logits processing like repetition penalties, and allows for easy custom scoring.
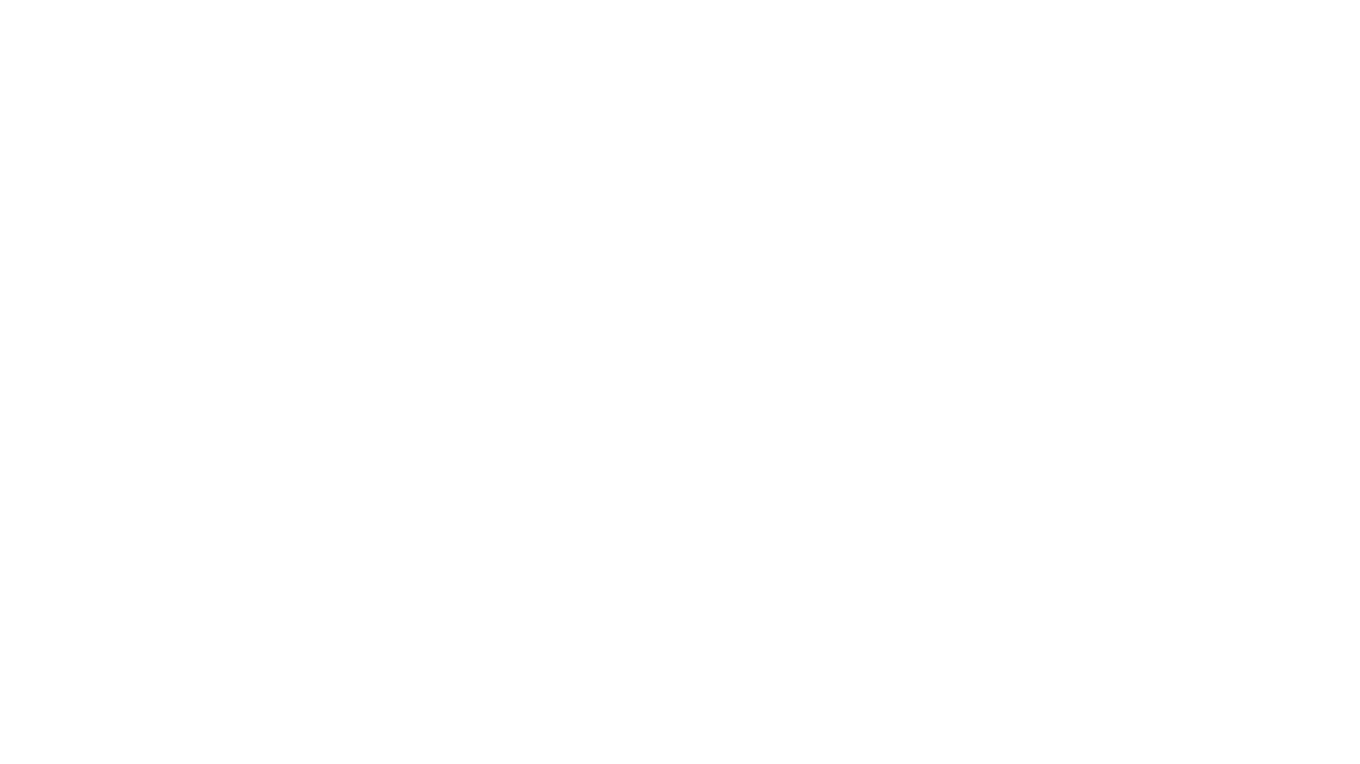
jupyter-ai
Jupyter AI connects generative AI with Jupyter notebooks. It provides a user-friendly and powerful way to explore generative AI models in notebooks and improve your productivity in JupyterLab and the Jupyter Notebook. Specifically, Jupyter AI offers: * An `%%ai` magic that turns the Jupyter notebook into a reproducible generative AI playground. This works anywhere the IPython kernel runs (JupyterLab, Jupyter Notebook, Google Colab, Kaggle, VSCode, etc.). * A native chat UI in JupyterLab that enables you to work with generative AI as a conversational assistant. * Support for a wide range of generative model providers, including AI21, Anthropic, AWS, Cohere, Gemini, Hugging Face, NVIDIA, and OpenAI. * Local model support through GPT4All, enabling use of generative AI models on consumer grade machines with ease and privacy.
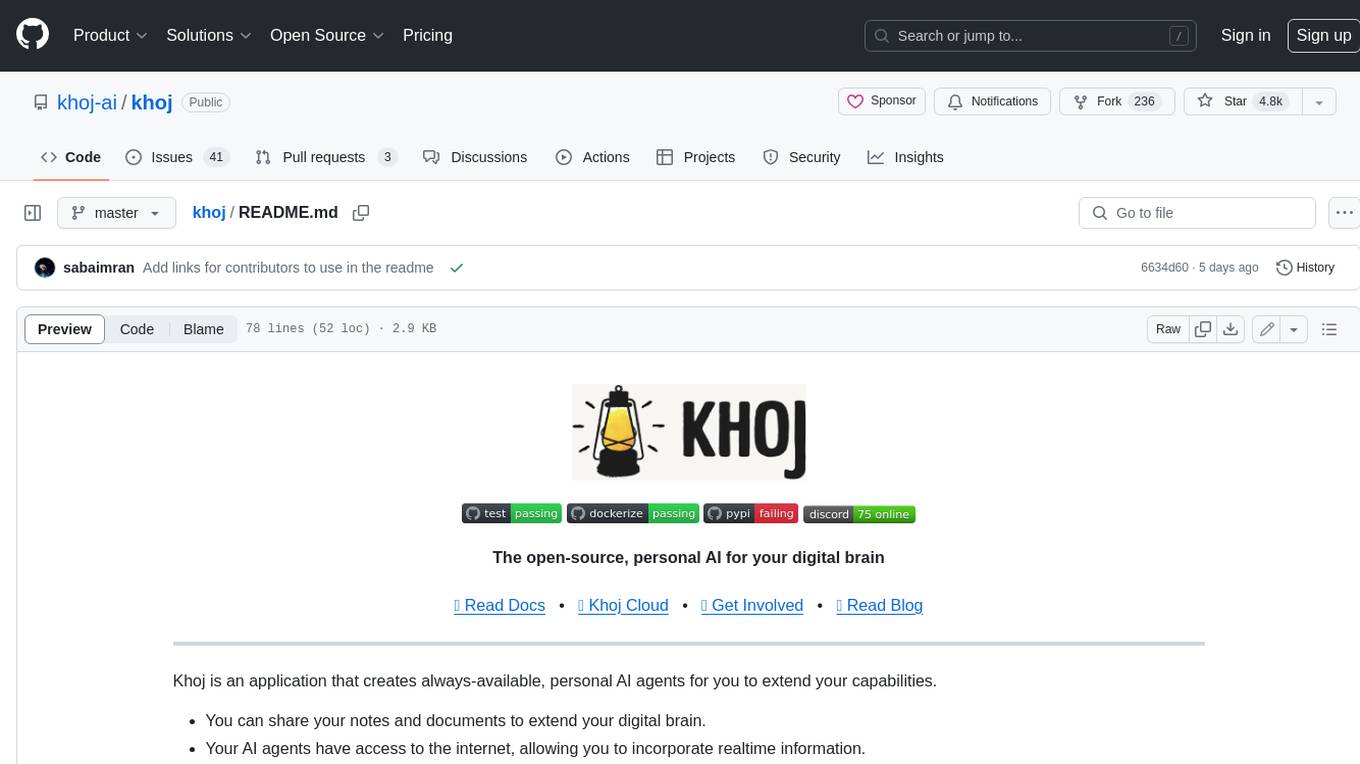
khoj
Khoj is an open-source, personal AI assistant that extends your capabilities by creating always-available AI agents. You can share your notes and documents to extend your digital brain, and your AI agents have access to the internet, allowing you to incorporate real-time information. Khoj is accessible on Desktop, Emacs, Obsidian, Web, and Whatsapp, and you can share PDF, markdown, org-mode, notion files, and GitHub repositories. You'll get fast, accurate semantic search on top of your docs, and your agents can create deeply personal images and understand your speech. Khoj is self-hostable and always will be.
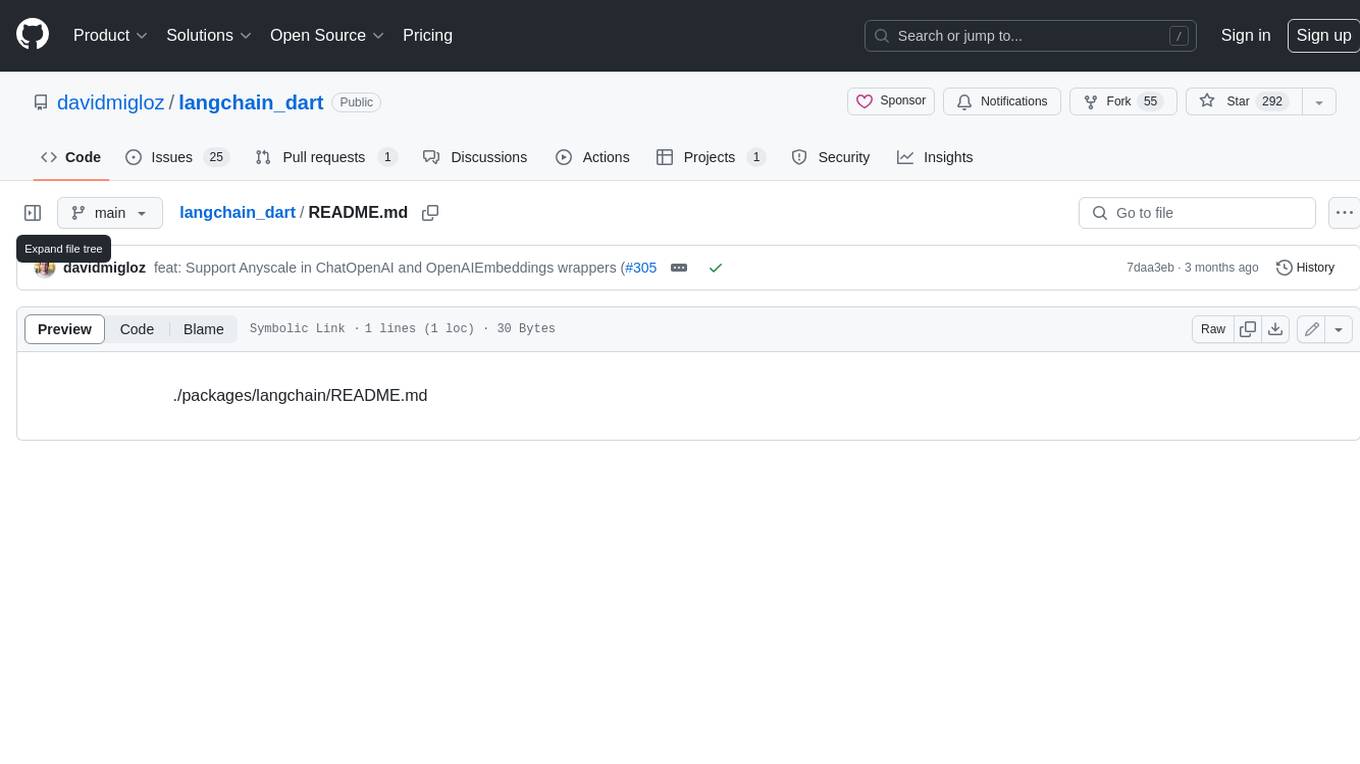
langchain_dart
LangChain.dart is a Dart port of the popular LangChain Python framework created by Harrison Chase. LangChain provides a set of ready-to-use components for working with language models and a standard interface for chaining them together to formulate more advanced use cases (e.g. chatbots, Q&A with RAG, agents, summarization, extraction, etc.). The components can be grouped into a few core modules: * **Model I/O:** LangChain offers a unified API for interacting with various LLM providers (e.g. OpenAI, Google, Mistral, Ollama, etc.), allowing developers to switch between them with ease. Additionally, it provides tools for managing model inputs (prompt templates and example selectors) and parsing the resulting model outputs (output parsers). * **Retrieval:** assists in loading user data (via document loaders), transforming it (with text splitters), extracting its meaning (using embedding models), storing (in vector stores) and retrieving it (through retrievers) so that it can be used to ground the model's responses (i.e. Retrieval-Augmented Generation or RAG). * **Agents:** "bots" that leverage LLMs to make informed decisions about which available tools (such as web search, calculators, database lookup, etc.) to use to accomplish the designated task. The different components can be composed together using the LangChain Expression Language (LCEL).
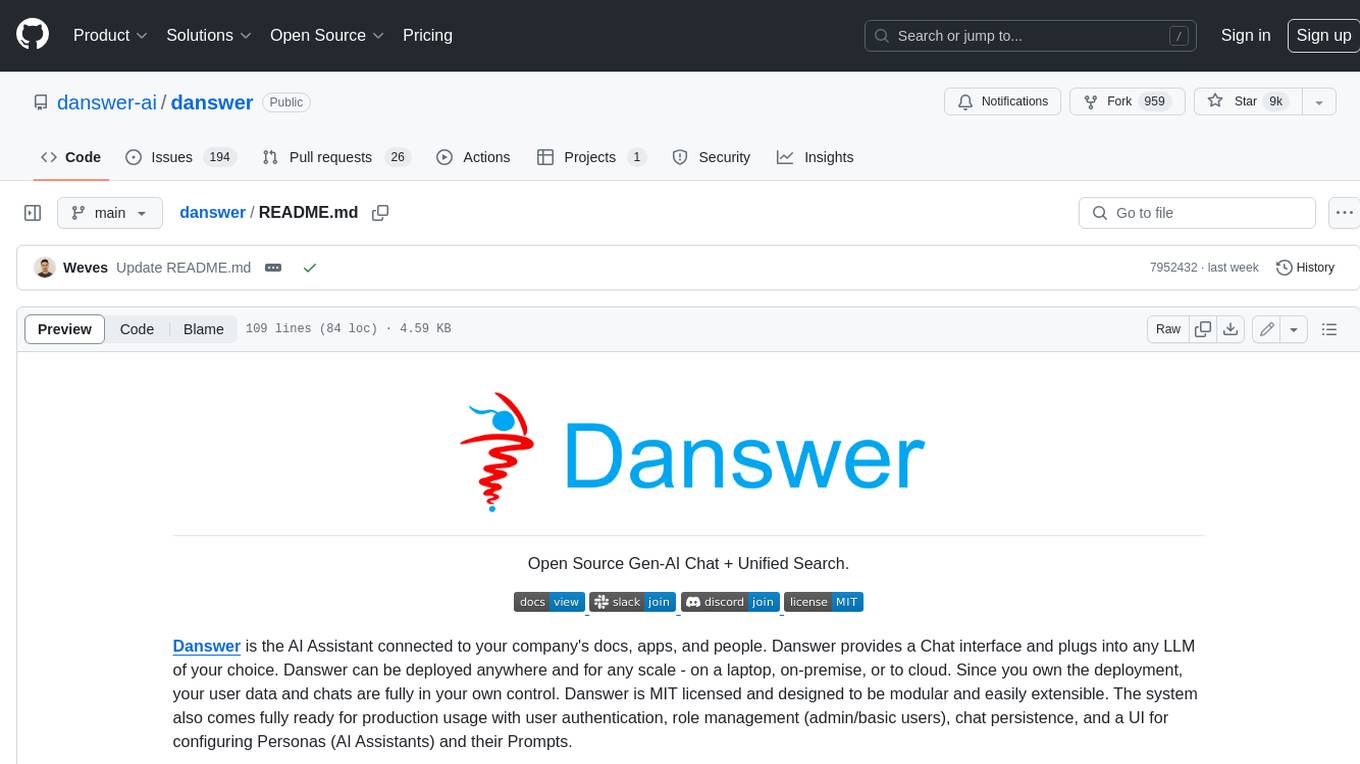
danswer
Danswer is an open-source Gen-AI Chat and Unified Search tool that connects to your company's docs, apps, and people. It provides a Chat interface and plugs into any LLM of your choice. Danswer can be deployed anywhere and for any scale - on a laptop, on-premise, or to cloud. Since you own the deployment, your user data and chats are fully in your own control. Danswer is MIT licensed and designed to be modular and easily extensible. The system also comes fully ready for production usage with user authentication, role management (admin/basic users), chat persistence, and a UI for configuring Personas (AI Assistants) and their Prompts. Danswer also serves as a Unified Search across all common workplace tools such as Slack, Google Drive, Confluence, etc. By combining LLMs and team specific knowledge, Danswer becomes a subject matter expert for the team. Imagine ChatGPT if it had access to your team's unique knowledge! It enables questions such as "A customer wants feature X, is this already supported?" or "Where's the pull request for feature Y?"
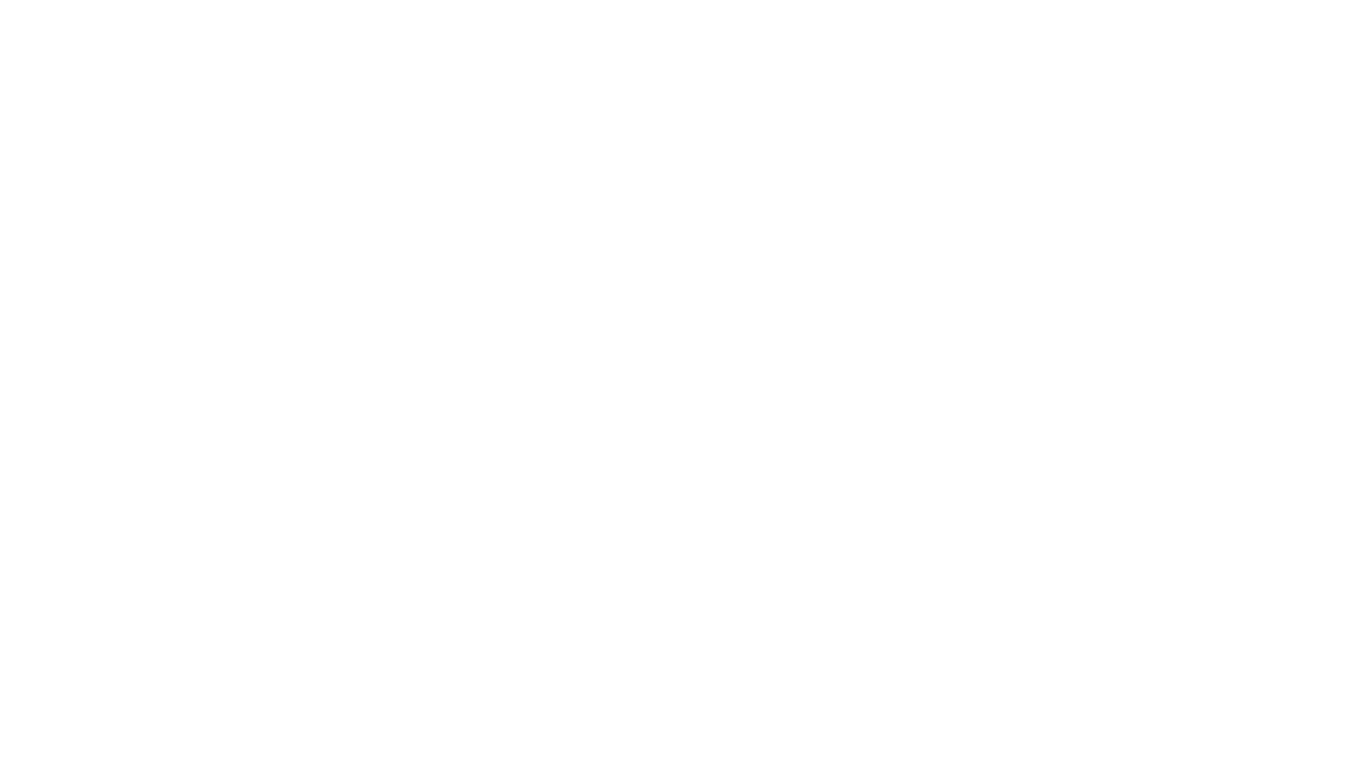
infinity
Infinity is an AI-native database designed for LLM applications, providing incredibly fast full-text and vector search capabilities. It supports a wide range of data types, including vectors, full-text, and structured data, and offers a fused search feature that combines multiple embeddings and full text. Infinity is easy to use, with an intuitive Python API and a single-binary architecture that simplifies deployment. It achieves high performance, with 0.1 milliseconds query latency on million-scale vector datasets and up to 15K QPS.
For similar jobs
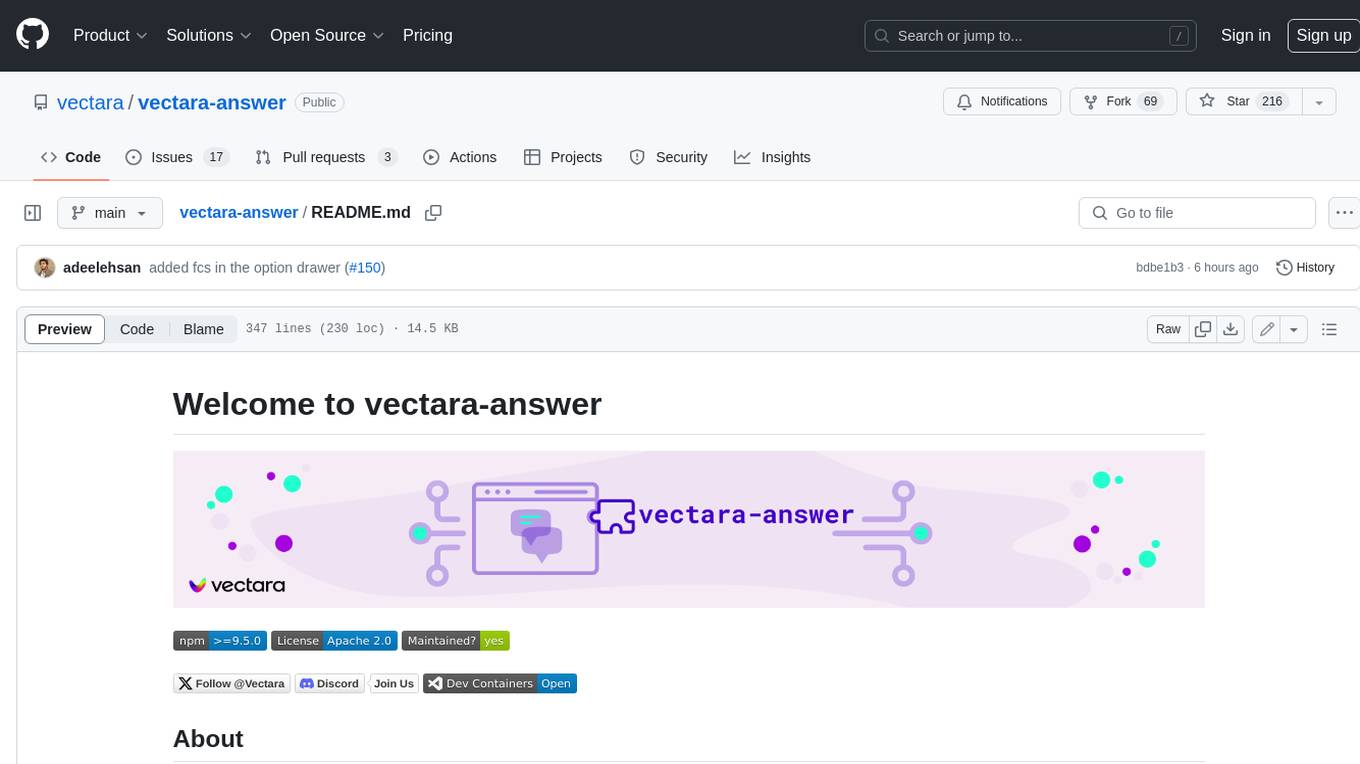
vectara-answer
Vectara Answer is a sample app for Vectara-powered Summarized Semantic Search (or question-answering) with advanced configuration options. For examples of what you can build with Vectara Answer, check out Ask News, LegalAid, or any of the other demo applications.
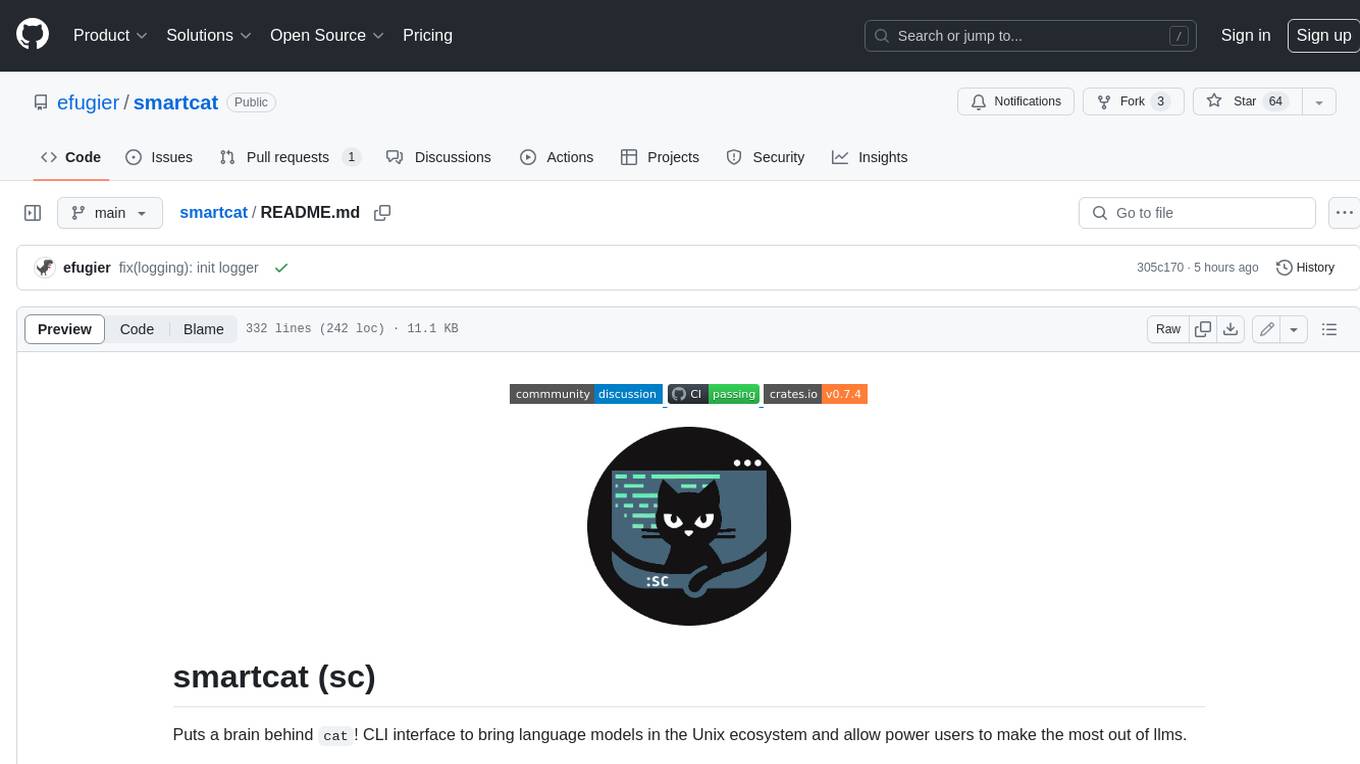
smartcat
Smartcat is a CLI interface that brings language models into the Unix ecosystem, allowing power users to leverage the capabilities of LLMs in their daily workflows. It features a minimalist design, seamless integration with terminal and editor workflows, and customizable prompts for specific tasks. Smartcat currently supports OpenAI, Mistral AI, and Anthropic APIs, providing access to a range of language models. With its ability to manipulate file and text streams, integrate with editors, and offer configurable settings, Smartcat empowers users to automate tasks, enhance code quality, and explore creative possibilities.
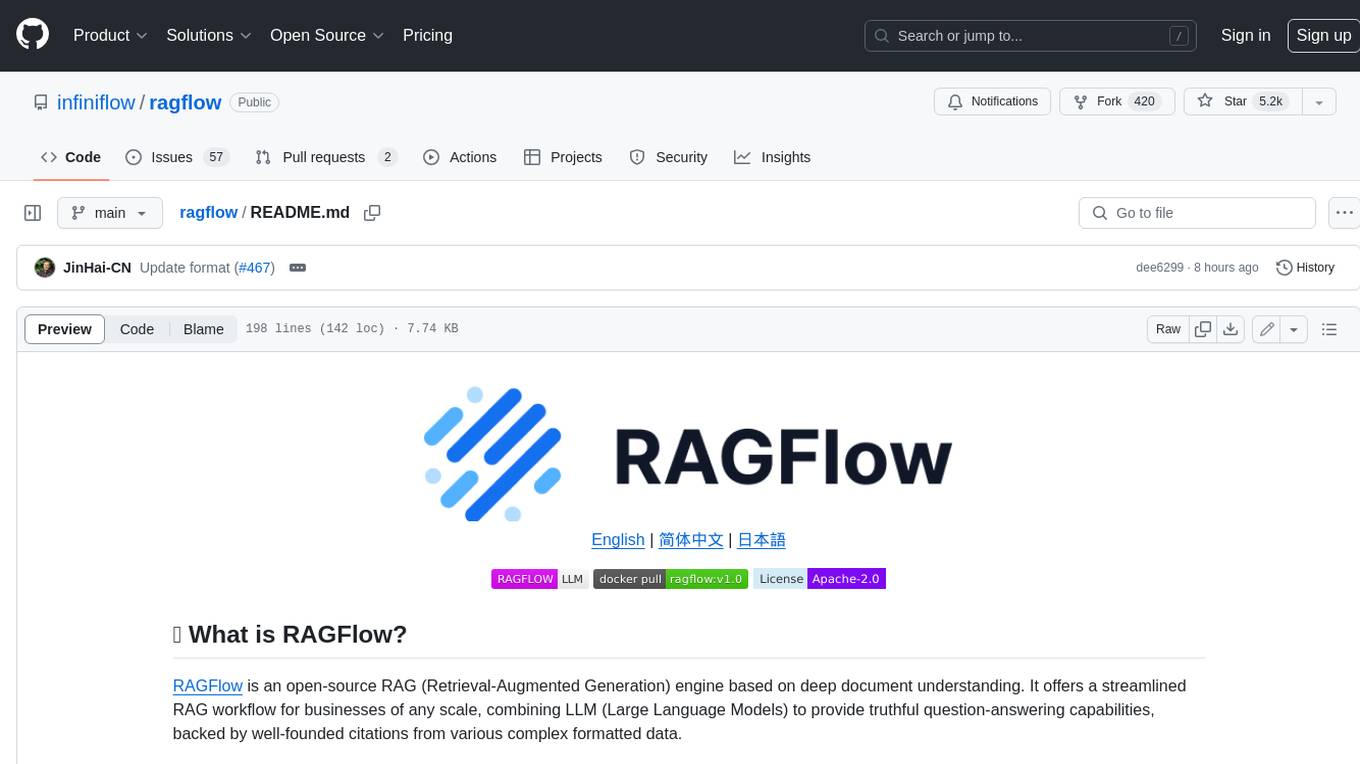
ragflow
RAGFlow is an open-source Retrieval-Augmented Generation (RAG) engine that combines deep document understanding with Large Language Models (LLMs) to provide accurate question-answering capabilities. It offers a streamlined RAG workflow for businesses of all sizes, enabling them to extract knowledge from unstructured data in various formats, including Word documents, slides, Excel files, images, and more. RAGFlow's key features include deep document understanding, template-based chunking, grounded citations with reduced hallucinations, compatibility with heterogeneous data sources, and an automated and effortless RAG workflow. It supports multiple recall paired with fused re-ranking, configurable LLMs and embedding models, and intuitive APIs for seamless integration with business applications.
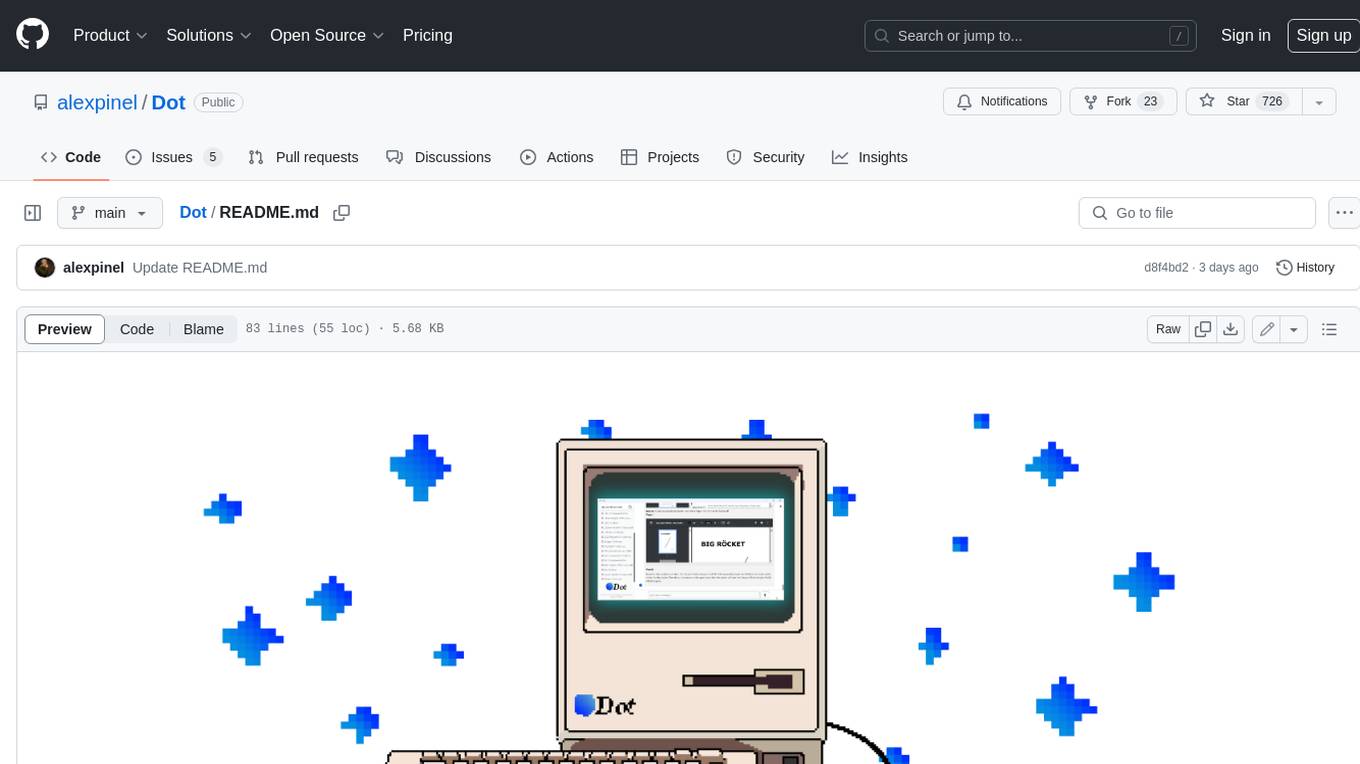
Dot
Dot is a standalone, open-source application designed for seamless interaction with documents and files using local LLMs and Retrieval Augmented Generation (RAG). It is inspired by solutions like Nvidia's Chat with RTX, providing a user-friendly interface for those without a programming background. Pre-packaged with Mistral 7B, Dot ensures accessibility and simplicity right out of the box. Dot allows you to load multiple documents into an LLM and interact with them in a fully local environment. Supported document types include PDF, DOCX, PPTX, XLSX, and Markdown. Users can also engage with Big Dot for inquiries not directly related to their documents, similar to interacting with ChatGPT. Built with Electron JS, Dot encapsulates a comprehensive Python environment that includes all necessary libraries. The application leverages libraries such as FAISS for creating local vector stores, Langchain, llama.cpp & Huggingface for setting up conversation chains, and additional tools for document management and interaction.
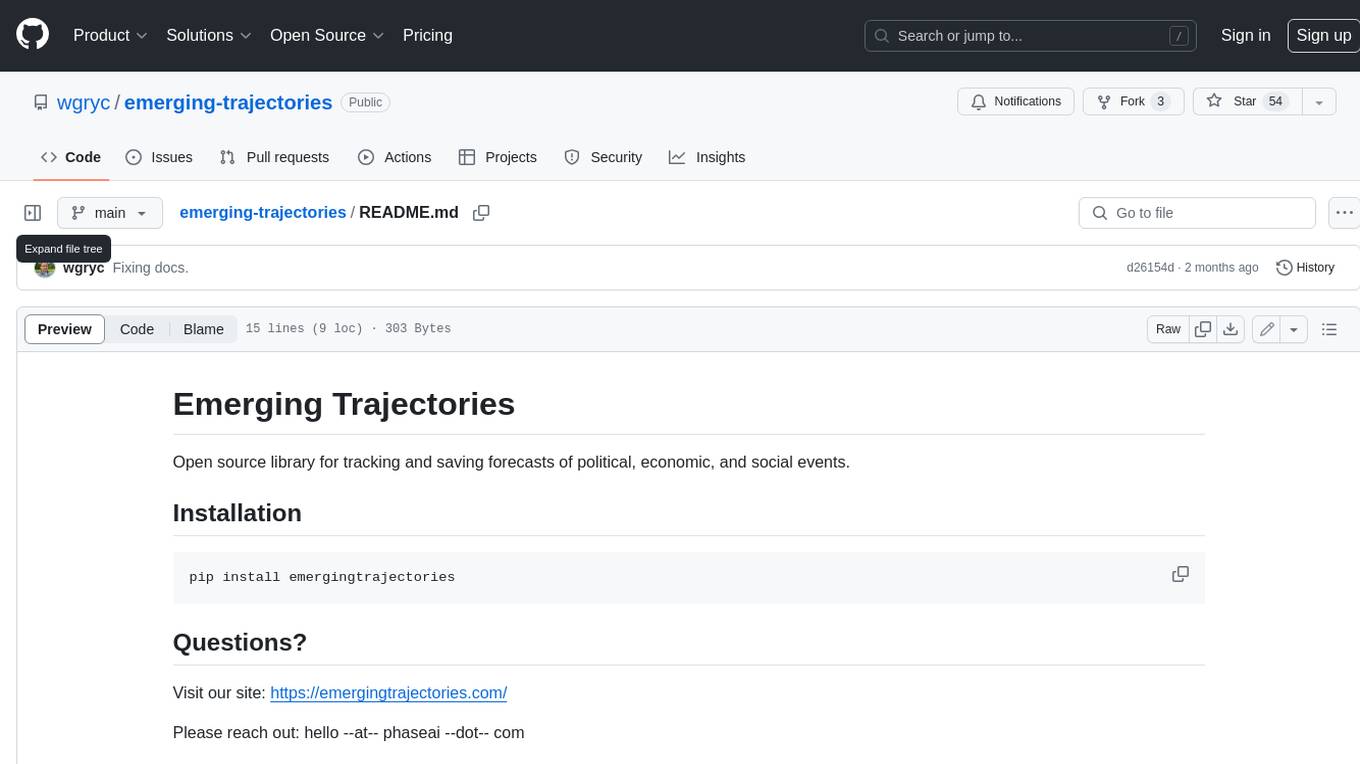
emerging-trajectories
Emerging Trajectories is an open source library for tracking and saving forecasts of political, economic, and social events. It provides a way to organize and store forecasts, as well as track their accuracy over time. This can be useful for researchers, analysts, and anyone else who wants to keep track of their predictions.
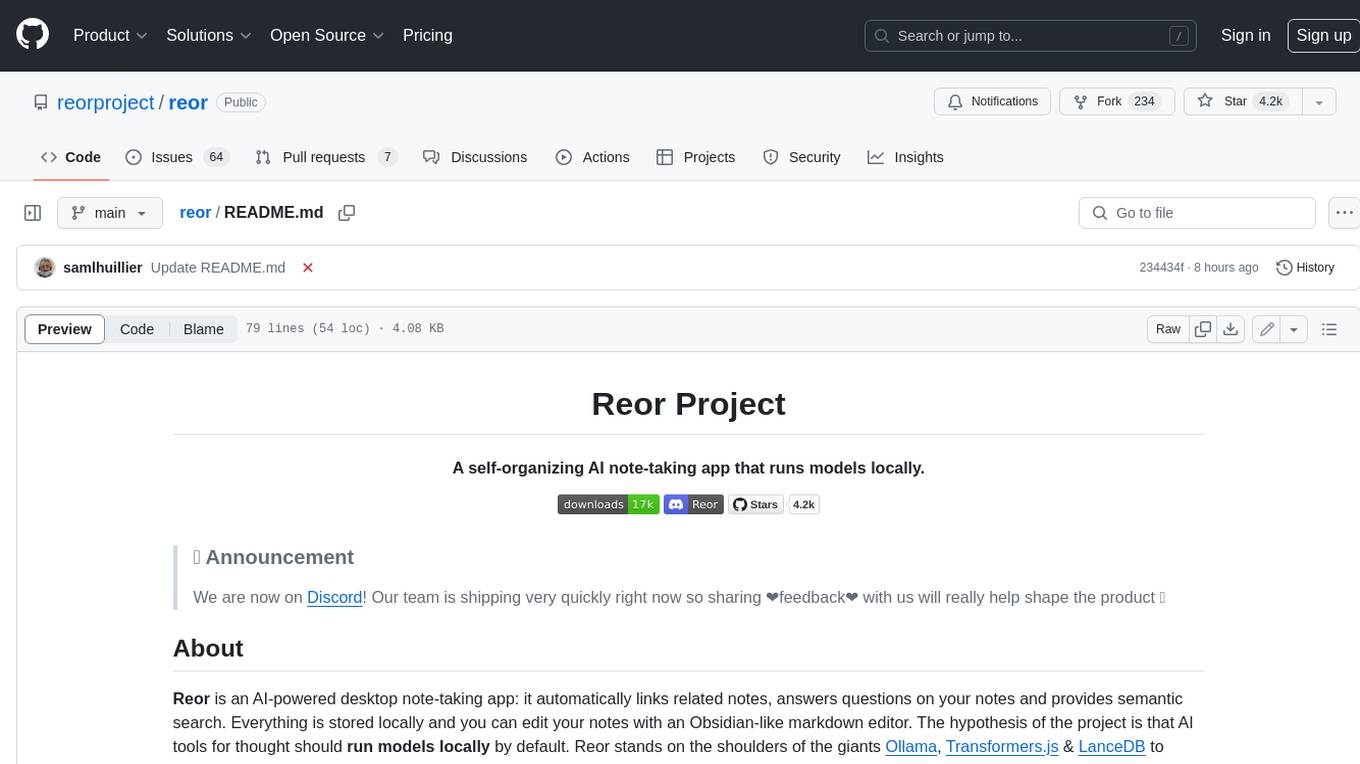
reor
Reor is an AI-powered desktop note-taking app that automatically links related notes, answers questions on your notes, and provides semantic search. Everything is stored locally and you can edit your notes with an Obsidian-like markdown editor. The hypothesis of the project is that AI tools for thought should run models locally by default. Reor stands on the shoulders of the giants Ollama, Transformers.js & LanceDB to enable both LLMs and embedding models to run locally. Connecting to OpenAI or OpenAI-compatible APIs like Oobabooga is also supported.
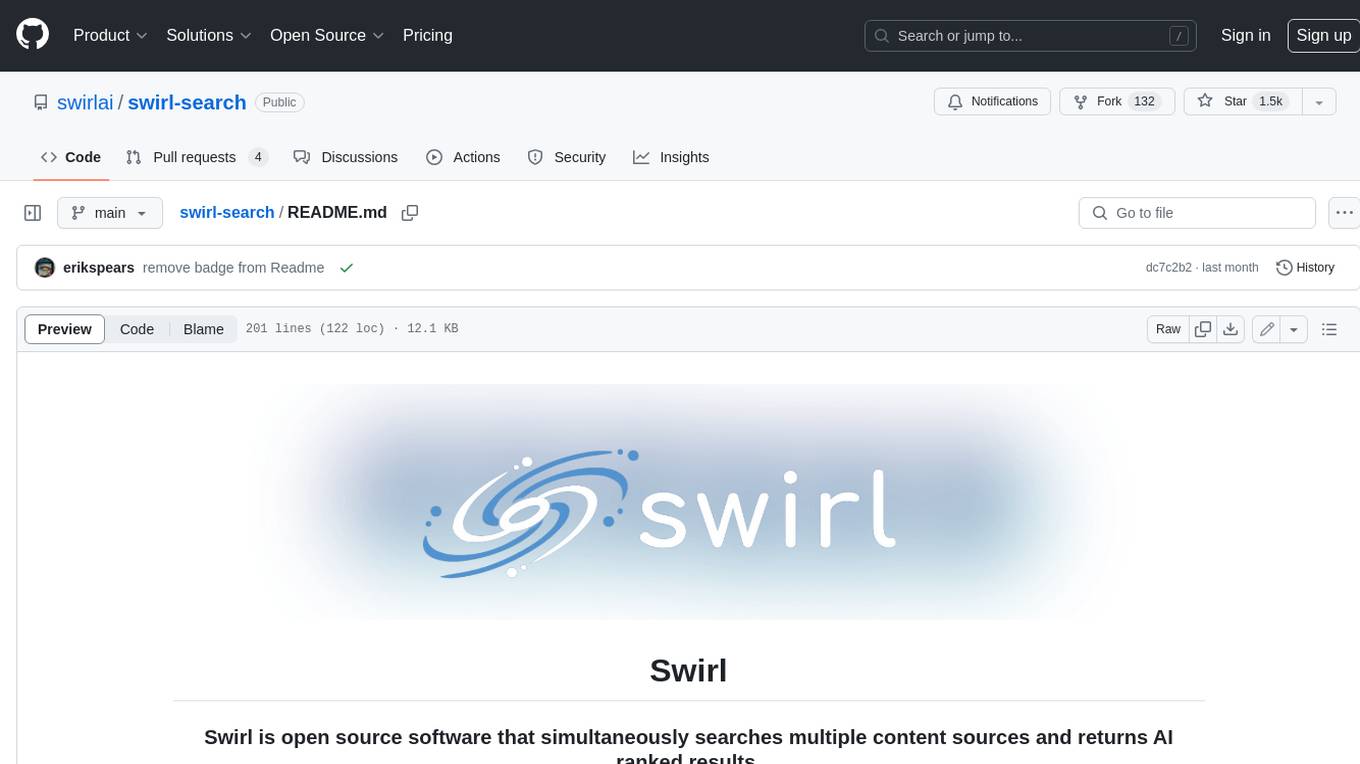
swirl-search
Swirl is an open-source software that allows users to simultaneously search multiple content sources and receive AI-ranked results. It connects to various data sources, including databases, public data services, and enterprise sources, and utilizes AI and LLMs to generate insights and answers based on the user's data. Swirl is easy to use, requiring only the download of a YML file, starting in Docker, and searching with Swirl. Users can add credentials to preloaded SearchProviders to access more sources. Swirl also offers integration with ChatGPT as a configured AI model. It adapts and distributes user queries to anything with a search API, re-ranking the unified results using Large Language Models without extracting or indexing anything. Swirl includes five Google Programmable Search Engines (PSEs) to get users up and running quickly. Key features of Swirl include Microsoft 365 integration, SearchProvider configurations, query adaptation, synchronous or asynchronous search federation, optional subscribe feature, pipelining of Processor stages, results stored in SQLite3 or PostgreSQL, built-in Query Transformation support, matching on word stems and handling of stopwords, duplicate detection, re-ranking of unified results using Cosine Vector Similarity, result mixers, page through all results requested, sample data sets, optional spell correction, optional search/result expiration service, easily extensible Connector and Mixer objects, and a welcoming community for collaboration and support.
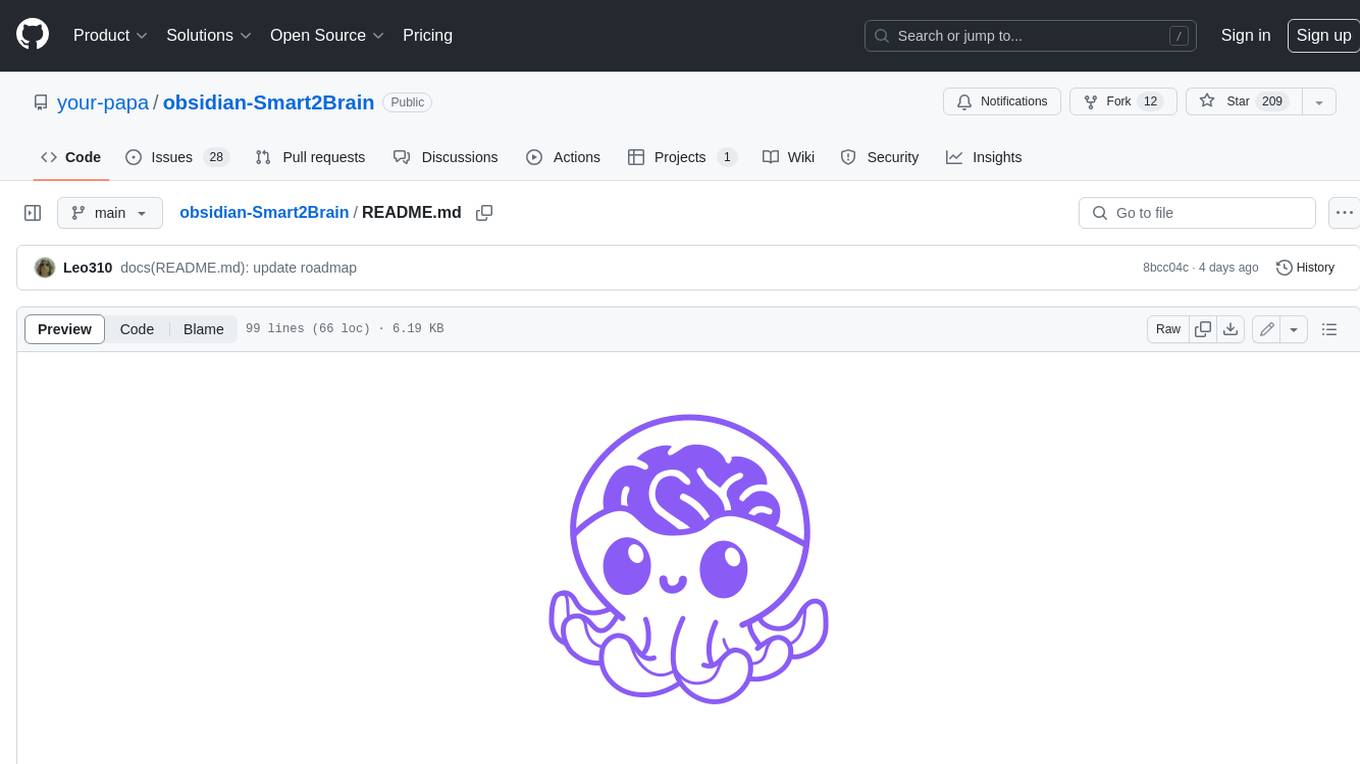
obsidian-Smart2Brain
Your Smart Second Brain is a free and open-source Obsidian plugin that serves as your personal assistant, powered by large language models like ChatGPT or Llama2. It can directly access and process your notes, eliminating the need for manual prompt editing, and it can operate completely offline, ensuring your data remains private and secure.