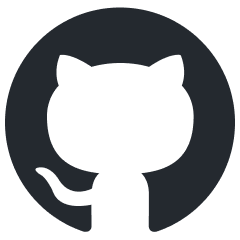
Bard-API
The unofficial python package that returns response of Google Bard through cookie value.
Stars: 5386
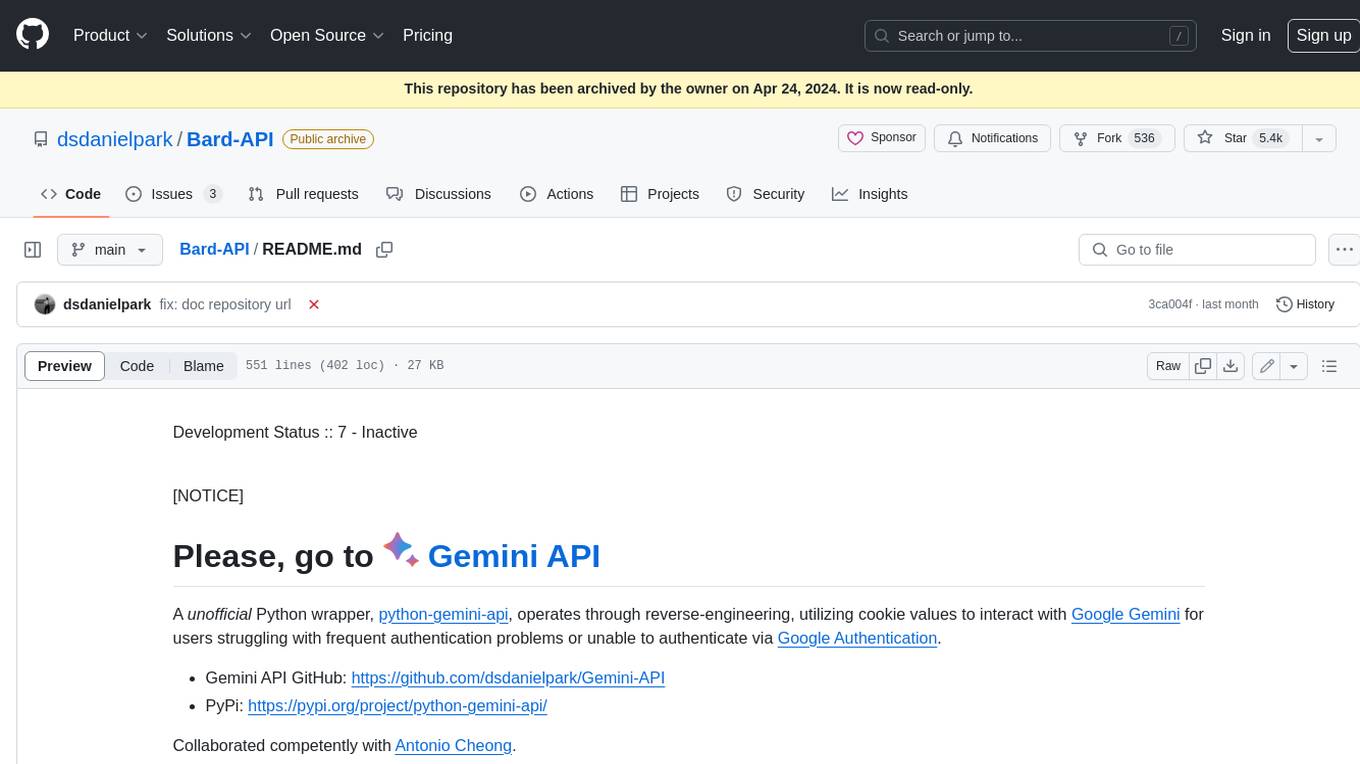
The Bard API is a Python package that returns responses from Google Bard through the value of a cookie. It is an unofficial API that operates through reverse-engineering, utilizing cookie values to interact with Google Bard for users struggling with frequent authentication problems or unable to authenticate via Google Authentication. The Bard API is not a free service, but rather a tool provided to assist developers with testing certain functionalities due to the delayed development and release of Google Bard's API. It has been designed with a lightweight structure that can easily adapt to the emergence of an official API. Therefore, using it for any other purposes is strongly discouraged. If you have access to a reliable official PaLM-2 API or Google Generative AI API, replace the provided response with the corresponding official code. Check out https://github.com/dsdanielpark/Bard-API/issues/262.
README:
Development Status :: 7 - Inactive
[NOTICE]
Please, go to
Gemini API
A unofficial Python wrapper, python-gemini-api, operates through reverse-engineering, utilizing cookie values to interact with Google Gemini for users struggling with frequent authentication problems or unable to authenticate via Google Authentication.
- Gemini API GitHub: https://github.com/dsdanielpark/Gemini-API
- PyPi: https://pypi.org/project/python-gemini-api/
Collaborated competently with Antonio Cheong.
pip install python-gemini-api
pip install git+https://github.com/dsdanielpark/Gemini-API.git
For the updated version, use as follows:
pip install -q -U python-gemini-api
Reflection on the Bard API Project https://github.com/dsdanielpark/Bard-API/issues/289
The python package that returns response of Google Bard through value of cookie.
Please exercise caution and use this package responsibly. This python package is UNOFFICIAL.
I referred to this github repository(github.com/acheong08/Bard) where inference process of Bard was reverse engineered. Using __Secure-1PSID
, you can ask questions and get answers from Google Bard. Please note that the bardapi is not a free service, but rather a tool provided to assist developers with testing certain functionalities due to the delayed development and release of Google Bard's API. It has been designed with a lightweight structure that can easily adapt to the emergence of an official API. Therefore, I strongly discourage using it for any other purposes. If you have access to reliable official PaLM-2 API or Google Generative AI API, replace the provided response with the corresponding official code. Check out https://github.com/dsdanielpark/Bard-API/issues/262.
- Google Bard API
What is Google Bard?
Bard is a conversational generative artificial intelligence chatbot developed by Google, based initially on the LaMDA family of LLMs(Large Language Models) and later the PaLM LLM. Please check official documents for updates on Bard, including available regions and languages.
$ pip install bardapi
$ pip install git+https://github.com/dsdanielpark/Bard-API.git
Due to certain dependency packages that are not compatible with 64bit windows(OS), we are releasing a lightweight alpha release of bard that only returns responses for simple requests. This release is a continuation of the pypi 0.1.18
version, which was maintained with lightweight and simple functionality. See alpha-release github branch for more details.
$ pip install bardapi==0.1.23a
Warning Do not expose the
__Secure-1PSID
. For testing purposes only; avoid direct application use. Cookie values change periodically (every 15-20 minutes). Frequent session changes may briefly block access; headless mode is challenging. Rate limiting applies and changes often. If the cookie changes, log out of your Google account, close the browser, and enter the new cookie value. Or manually reset the cookie for a new value. See FAQ and issue pages for details.
- Visit https://gemini.google.com/
- F12 for console
- Session: Application → Cookies → Copy the value of
__Secure-1PSID
cookie. Or try to useSIDCC
as token.
Note that while I referred to __Secure-1PSID
or SIDCC
value as an API key for convenience, it is not an officially provided API key.
Cookie value subject to frequent changes. Verify the value again if an error occurs. Most errors occur when an invalid cookie value is entered.
If you need to set multiple cookie values:
- Multi-cookie Bard - After confirming that multiple cookie values are required to receive responses reliably in certain countries, I will deploy it for testing purposes. Please debug and create a pull request.
Simple Usage
from bardapi import Bard
token = 'xxxxxxx'
bard = Bard(token=token)
bard.get_answer("나와 내 동년배들이 좋아하는 뉴진스에 대해서 알려줘")['content']
Or you can use this
from bardapi import Bard
import os
os.environ['_BARD_API_KEY'] = "xxxxxxx"
Bard().get_answer("나와 내 동년배들이 좋아하는 뉴진스에 대해서 알려줘")['content']
To get reponse dictionary
import bardapi
# set your __Secure-1PSID value to key
token = 'xxxxxxx'
# set your input text
input_text = "나와 내 동년배들이 좋아하는 뉴진스에 대해서 알려줘"
# Send an API request and get a response.
response = bardapi.core.Bard(token).get_answer(input_text)
Addressing errors caused by delayed responses in environments like Google Colab and containers. If an error occurs despite following the proper procedure, utilize the timeout argument.
from bardapi import Bard
import os
os.environ['_BARD_API_KEY']="xxxxxxx"
bard = Bard(timeout=30) # Set timeout in seconds
bard.get_answer("나와 내 동년배들이 좋아하는 뉴진스에 대해서 알려줘")['content']
If you are working behind a proxy, use the following.
from bardapi import Bard
# Change 'http://proxy.example.com:8080' to your http proxy
# timeout in seconds
proxies = {
'http': 'http://proxy.example.com:8080',
'https': 'https://proxy.example.com:8080'
}
bard = Bard(token='xxxxxxx', proxies=proxies, timeout=30)
bard.get_answer("나와 내 동년배들이 좋아하는 뉴진스에 대해서 알려줘")['content']
If you want to avoid blocked requests and bans, then use Smart Proxy by Crawlbase. It forwards your connection requests to a randomly rotating IP address in a pool of proxies before reaching the target website. The combination of AI and ML make it more effective to avoid CAPTCHAs and blocks.
from bardapi import Bard
import requests
# Get your proxy url at crawlbase https://crawlbase.com/docs/smart-proxy/get/
proxy_url = "http://xxxxxxxxxxxxxx:@smartproxy.crawlbase.com:8012"
proxies = {"http": proxy_url, "https": proxy_url}
bard = Bard(token='xxxxxxx', proxies=proxies, timeout=30)
bard.get_answer("나와 내 동년배들이 좋아하는 뉴진스에 대해서 알려줘")['content']
You can continue the conversation using a reusable session. However, this feature is limited, and it is difficult for a package-level feature to perfectly maintain conversation_id and context. You can try to maintain the consistency of conversations same way as other LLM services, such as passing some sort of summary of past conversations to the DB.
from bardapi import Bard
import requests
# import os
# os.environ['_BARD_API_KEY'] = 'xxxxxxx'
token = 'xxxxxxx'
session = requests.Session()
session.headers = {
"Host": "gemini.google.com",
"X-Same-Domain": "1",
"User-Agent": "Mozilla/5.0 (Windows NT 10.0; WOW64) AppleWebKit/537.36 (KHTML, like Gecko) Chrome/91.0.4472.114 Safari/537.36",
"Content-Type": "application/x-www-form-urlencoded;charset=UTF-8",
"Origin": "https://gemini.google.com",
"Referer": "https://gemini.google.com/",
}
# session.cookies.set("__Secure-1PSID", os.getenv("_BARD_API_KEY"))
session.cookies.set("__Secure-1PSID", token)
bard = Bard(token=token, session=session, timeout=30)
bard.get_answer("나와 내 동년배들이 좋아하는 뉴진스에 대해서 알려줘")['content']
# Continued conversation without set new session
bard.get_answer("What is my last prompt??")['content']
Async Bard Code (Click to expand)
from httpx import AsyncClient
from bardapi import BardAsync
import os
# Uncomment and set your API key as needed
# os.environ['_BARD_API_KEY'] = 'xxxxxxx'
token = 'xxxxxxx' # Replace with your actual token
SESSION_HEADERS = {
"Host": "gemini.google.com",
"X-Same-Domain": "1",
"User-Agent": "Mozilla/5.0 (Windows NT 10.0; WOW64) AppleWebKit/537.36 (KHTML, like Gecko) Chrome/91.0.4472.114 Safari/537.36",
"Content-Type": "application/x-www-form-urlencoded;charset=UTF-8",
"Origin": "https://gemini.google.com",
"Referer": "https://gemini.google.com/",
}
timeout = 30 # Example timeout
proxies = {} # Replace with your proxies if needed
client = AsyncClient(
http2=True,
headers=SESSION_HEADERS,
cookies={"__Secure-1PSID": token},
timeout=timeout,
proxies=proxies,
)
bard_async = BardAsync(token=token, client=client)
# Asynchronous function to get the answer
async def get_bard_answer(question):
await bard_async.async_setup() # Ensure async setup is done
return await bard_async.get_answer(question)
response = await get_bard_answer("나와 내 동년배들이 좋아하는 뉴진스에 대해서 알려줘")
print(response['content'])
Using browser_cookie3 we extract the __Secure-1PSID
cookie from all browsers, and then we can use the API without passing the token. However, there are still incomplete dependency packages and various variables, so please seek assistance in the following GitHub Issues or adjust your browser's version.
- Visit https://gemini.google.com/ in your browser and execute the following command while in the chat-enabled state. Refer to browser_cookie3 for details on how it works. If any issues arise, please restart the browser or log in to your Google account again. Recommended to keep the browser open.
from bardapi import Bard
bard = Bard(token_from_browser=True)
response = bard.get_answer("Do you like cookies?")
print(response['content'])
It may not work as it is only available for certain accounts, regions, and other restrictions. As an experimental feature, it is possible to ask questions with an image. However, this functionality is only available for accounts with image upload capability in Bard's web UI.
from bardapi import Bard
bard = Bard(token='xxxxxxx')
image = open('image.jpg', 'rb').read() # (jpeg, png, webp) are supported.
bard_answer = bard.ask_about_image('What is in the image?', image)
print(bard_answer['content'])
Text To Speech(TTS) from Bard
Business users and high traffic volume may be subject to account restrictions according to Google's policies. Please use the Official Google Cloud API for any other purpose. The user is solely responsible for all code, and it is imperative to consult Google's official services and policies. Furthermore, the code in this repository is provided under the MIT license, and it disclaims any liability, including explicit or implied legal responsibilities.
from bardapi import Bard
bard = Bard(token='xxxxxxx')
audio = bard.speech('Hello, I am Bard! How can I help you today?')
with open("speech.ogg", "wb") as f:
f.write(bytes(audio['audio']))
Starting from version 0.1.18
, the GitHub version of BardAPI will be synchronized with the PyPI version and released simultaneously. However, the version undergoing QA can still be used from the GitHub repository.
$ pip install git+https://github.com/dsdanielpark/Bard-API.git
- Multi-cookie Bard
- Auto Cookie Bard
- TTS from Bard
- Multi-language Bard API
- Get image links
- ChatBard
- Export Conversation
- Export Code to Repl.it
- Executing Python code received as a response from Bard
- Using Bard Asynchronously
- Bard Cookies
- Fix Coversation ID (Fix Context)
- Max_token, Max_sentences
- Translation to another programming language
Amazing Bard Prompts Is All You Need!
- Helpful prompts for Google Bard
If you want to comfortably use the open-source LLM models in your native language, which are released under the Apache License (allowing free commercial use)
in various languages
, you can try using the hf-transllm package. hf-transllm also supports multilingual inference for various LLMs stored in hugging face repository.
Example code of hf-transllm
In case the Google package is no longer available due to policy restrictions, here's a simple example code for using open-source language models (LLMs) in both English and multiple languages.
For the decoder models provided by Hugging Face, you can easily use them by either following a simple approach or overriding the inference method. You can explore various open-source language models at this link. Through the ranking information from Open LLM Leader Board Report repository, you can find information about good models.
from transllm import LLMtranslator
open_llama3b_kor = LLMtranslator('openlm-research/open_llama_3b', target_lang='ko', translator='google') # Korean
trnaslated_answer = open_llama3b_kor.generate("나와 내 동년배들이 좋아하는 뉴진스에 대해서 알려줘")
print(trnaslated_answer)
Refer https://github.com/openlm-research/open_llama or using like this:
from transllm import LLMtranslator
open_llama3b = LLMtranslator('openlm-research/open_llama_3b)
answer = open_llama3b.generate("Tell me about the Korean girl group Newjeans.")
print(answer)
What is Google Gemini?
Gemini or formerly knowns as Bard is an advanced, multimodal AI model by Google DeepMind, capable of understanding and integrating various information types like text, code, audio, images, and video.
- Paper: https://storage.googleapis.com/deepmind-media/gemini/gemini_1_report.pdf
- Web: https://blog.google/technology/ai/google-gemini-ai/#capabilities
- Code Guide: https://ai.google.dev/tutorials/python_quickstart
- Official API On Google AI Studio.
Google AI Studio creates a new Google Cloud project for each new API key. You also can create an API key in an existing Google Cloud project. All projects are subject to the Google Cloud Platform Terms of Service.
- Web: https://makersuite.google.com/app/apikey
- Note: The Gemini API is currently in public preview. Production applications are not supported yet.
The Bard API, sourcing responses from Google BardGemini's official website, allows you to receive the same responses as the website. So, if Gemini answers are available on the web, you can also accessed Gemini through the Bard API. However, it's important to note that responses might also come from other models, not exclusively Gemini Pro or Ultra.
- There is no official Bard API or early access/waiting list for Gemini, although the PaLM2 API is available.
- Google's PaLM2 API differs from Bard, with some aspects of Bard being superior.
- It's speculated that after expert review, Bard Advanced lineup will likely provide an official API in 2024.
- Gemini and previous generative AI model responses are provided randomly on Bard Web.
- The Bard API, with its imperfect extension features(e.g,
ask_about_image
), occasionally demonstrates Gemini's capabilities. This behavior may vary by region, language, or Google account. - More information in the FAQ.
For more on Gemini:
- Official API
- Introducing Gemini: our largest and most capable AI model
- How it's made: multimodal prompting
- YouTube Demo
Try demo at https://makersuite.google.com/app/prompts/new_text.
who are you?
>> I am powered by PaLM 2, which stands for Pathways Language Model 2, a large language model from Google AI.
Google Generative AI
- Official Page: https://blog.google/technology/ai/google-palm-2-ai-large-language-model/
- GitHub: https://github.com/GoogleCloudPlatform/generative-ai
- Try Demo: https://makersuite.google.com/app/prompts/new_text.
- Official Library: https://makersuite.google.com/app/library
- Get API Key: https://makersuite.google.com/app/apikey
- Quick Start Tutorial: https://developers.generativeai.google/tutorials/text_quickstart
$ pip install -q google-generativeai
import pprint
import google.generativeai as palm
palm.configure(api_key='YOUR_API_KEY')
models = [m for m in palm.list_models() if 'generateText' in m.supported_generation_methods]
model = models[0].name
print(model)
prompt = "Who are you?"
completion = palm.generate_text(
model=model,
prompt=prompt,
temperature=0,
# The maximum length of the response
max_output_tokens=800,
)
print(completion.result)
Use data scraping to train your AI models.
- Easy to use API to crawl and scrape millions of websites
- Use crawlbase for efficient data extraction for your LLMs
- Average success rate: 98%
- Uptime guarantee: 99.9%
- Simple docs to get started in minutes
- Asynchronous Crawling API if you need massive amounts of data
- GDPR and CCPA compliant
Used by 70k+ developers.
Please check the FAQ and open issues for similar questions before creating a new issue. Repeated questions will be kept as open issues. Too many requests can trigger a temporary account block (HTTP 429). Maintain proper intervals, using functions like sleep to avoid rate limits. Policies may vary by country and language, so all users could face temporary or permanent errors via the API.
In the scripts folder, I have released a script to help you compare OpenAI-ChatGPT, Microsoft-EdgeGPT and Google-Bard. I hope they will help more developers.
We would like to express our sincere gratitude to all the contributors.
MIT
We hold no legal responsibility; for more information, please refer to the bottom of the readme file. We just want you to give me and them a star. This project is a personal initiative and is not affiliated with or endorsed by Google. It is recommended to use Google's official API.
The MIT License (MIT)
Copyright (c) 2023 Minwoo Park
Permission is hereby granted, free of charge, to any person obtaining a copy
of this software and associated documentation files (the "Software"), to deal
in the Software without restriction, including without limitation the rights
to use, copy, modify, merge, publish, distribute, sublicense, and/or sell
copies of the Software, and to permit persons to whom the Software is
furnished to do so, subject to the following conditions:
The above copyright notice and this permission notice shall be included in all
copies or substantial portions of the Software.
THE SOFTWARE IS PROVIDED "AS IS", WITHOUT WARRANTY OF ANY KIND, EXPRESS OR
IMPLIED, INCLUDING BUT NOT LIMITED TO THE WARRANTIES OF MERCHANTABILITY,
FITNESS FOR A PARTICULAR PURPOSE AND NONINFRINGEMENT. IN NO EVENT SHALL THE
AUTHORS OR COPYRIGHT HOLDERS BE LIABLE FOR ANY CLAIM, DAMAGES OR OTHER
LIABILITY, WHETHER IN AN ACTION OF CONTRACT, TORT OR OTHERWISE, ARISING FROM,
OUT OF OR IN CONNECTION WITH THE SOFTWARE OR THE USE OR OTHER DEALINGS IN THE
SOFTWARE.
Bard's service status and Google's API interfaces are in constant flux. The number of replies is currently limited, but certain users, such as those utilizing VPNs or proxy servers, have reported slightly higher message caps. Adaptability is crucial in navigating these dynamic service policies. Please note that the cookie values used in this package are not official API values.
Sincerely grateful for any reports on new features or bugs. Your valuable feedback on the code is highly appreciated.
- Core maintainer:
[1] https://github.com/acheong08/Bard
Warning Important Notice The user assumes all legal responsibilities associated with using the BardAPI package. This Python package merely facilitates easy access to Google Bard for developers. Users are solely responsible for managing data and using the package appropriately. For further information, please consult the Google Bard Official Document.
Warning Caution This Python package is not an official Google package or API service. It is not affiliated with Google and uses Google account cookies, which means that excessive or commercial usage may result in restrictions on your Google account. The package was created to support developers in testing functionalities due to delays in the official Google package. However, it should not be misused or abused. Please be cautious and refer to the Readme for more information.
Copyright (c) 2023 MinWoo Park, South Korea
For Tasks:
Click tags to check more tools for each tasksFor Jobs:
Alternative AI tools for Bard-API
Similar Open Source Tools
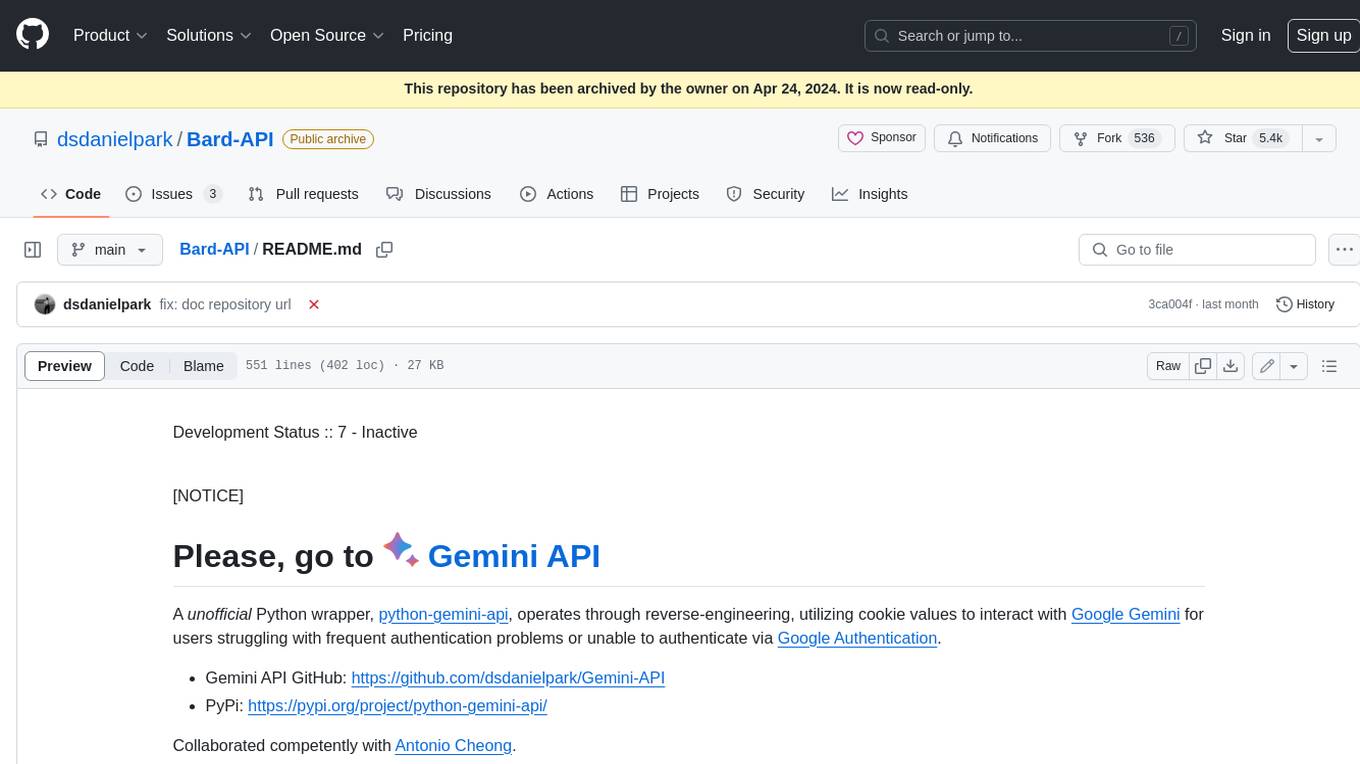
Bard-API
The Bard API is a Python package that returns responses from Google Bard through the value of a cookie. It is an unofficial API that operates through reverse-engineering, utilizing cookie values to interact with Google Bard for users struggling with frequent authentication problems or unable to authenticate via Google Authentication. The Bard API is not a free service, but rather a tool provided to assist developers with testing certain functionalities due to the delayed development and release of Google Bard's API. It has been designed with a lightweight structure that can easily adapt to the emergence of an official API. Therefore, using it for any other purposes is strongly discouraged. If you have access to a reliable official PaLM-2 API or Google Generative AI API, replace the provided response with the corresponding official code. Check out https://github.com/dsdanielpark/Bard-API/issues/262.
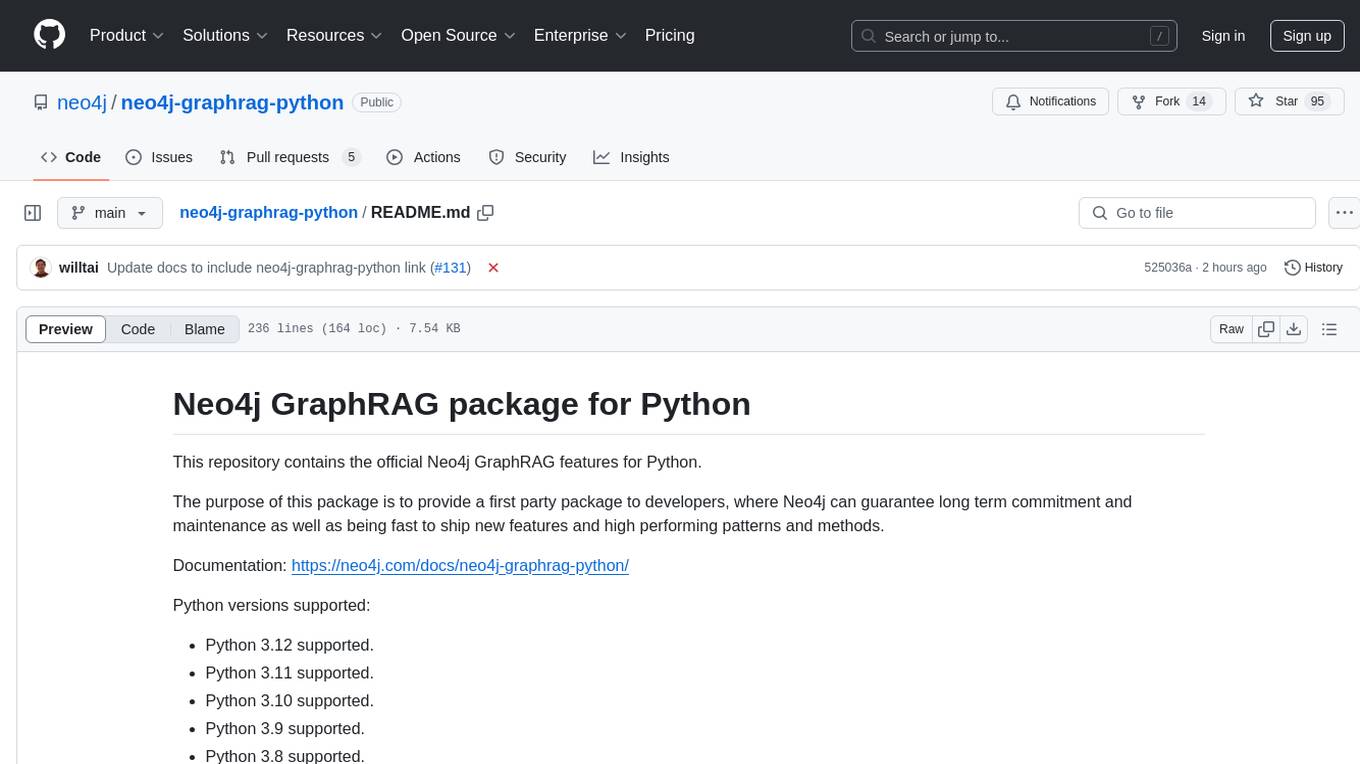
neo4j-graphrag-python
The Neo4j GraphRAG package for Python is an official repository that provides features for creating and managing vector indexes in Neo4j databases. It aims to offer developers a reliable package with long-term commitment, maintenance, and fast feature updates. The package supports various Python versions and includes functionalities for creating vector indexes, populating them, and performing similarity searches. It also provides guidelines for installation, examples, and development processes such as installing dependencies, making changes, and running tests.
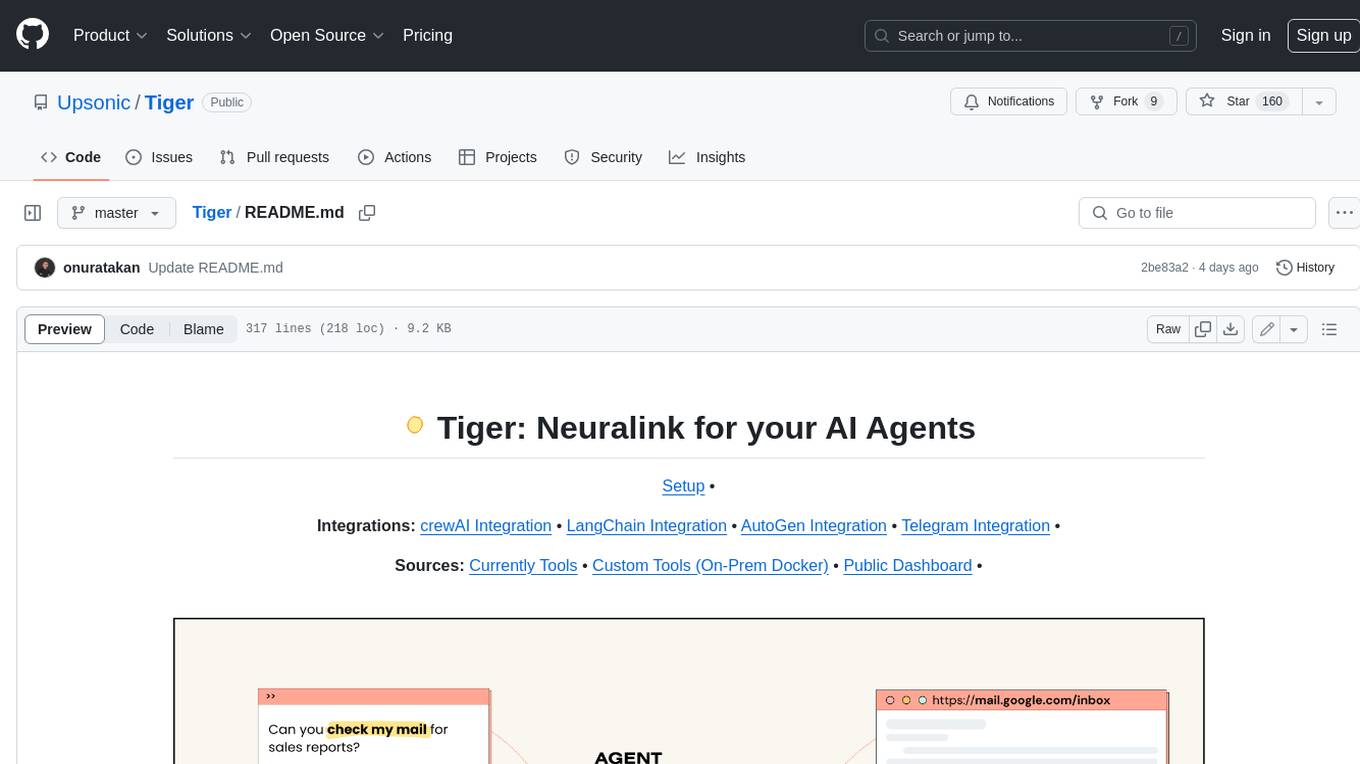
Tiger
Tiger is a community-driven project developing a reusable and integrated tool ecosystem for LLM Agent Revolution. It utilizes Upsonic for isolated tool storage, profiling, and automatic document generation. With Tiger, you can create a customized environment for your agents or leverage the robust and publicly maintained Tiger curated by the community itself.
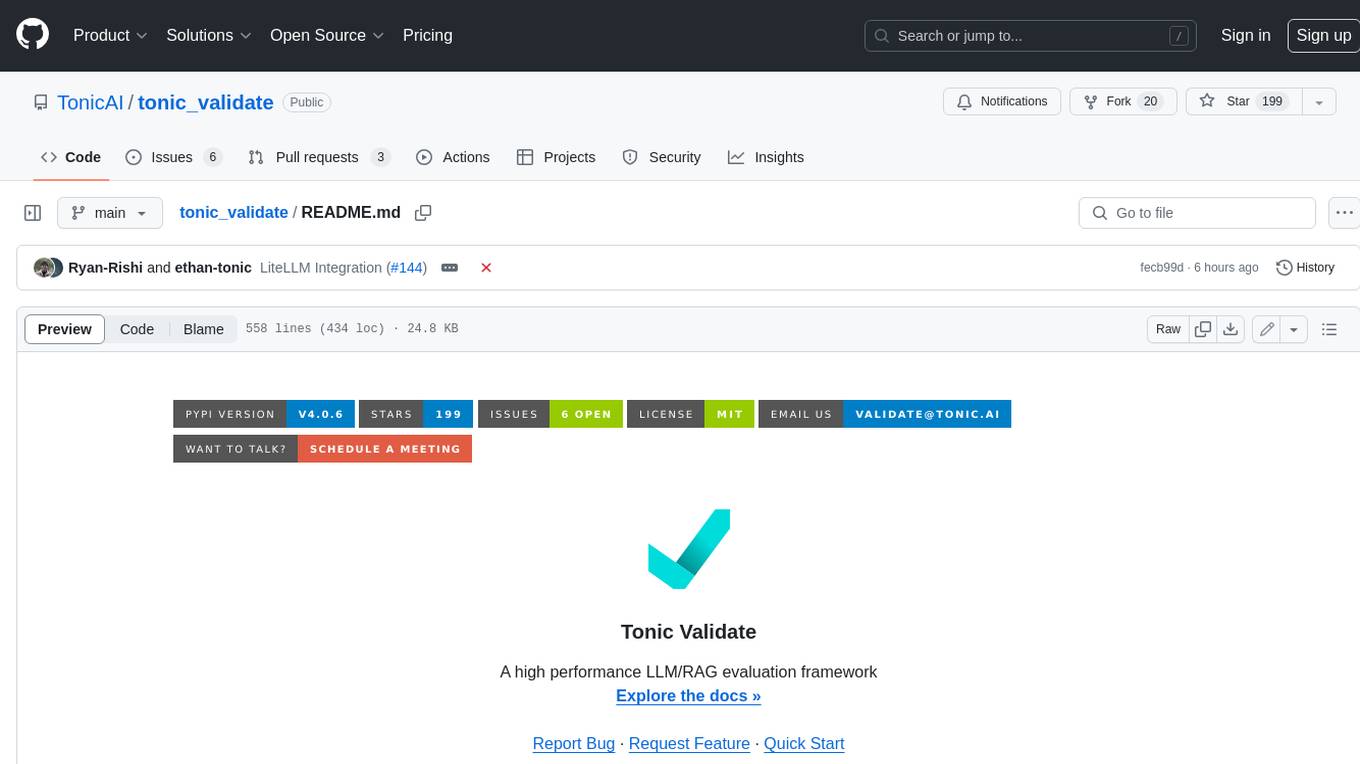
tonic_validate
Tonic Validate is a framework for the evaluation of LLM outputs, such as Retrieval Augmented Generation (RAG) pipelines. Validate makes it easy to evaluate, track, and monitor your LLM and RAG applications. Validate allows you to evaluate your LLM outputs through the use of our provided metrics which measure everything from answer correctness to LLM hallucination. Additionally, Validate has an optional UI to visualize your evaluation results for easy tracking and monitoring.
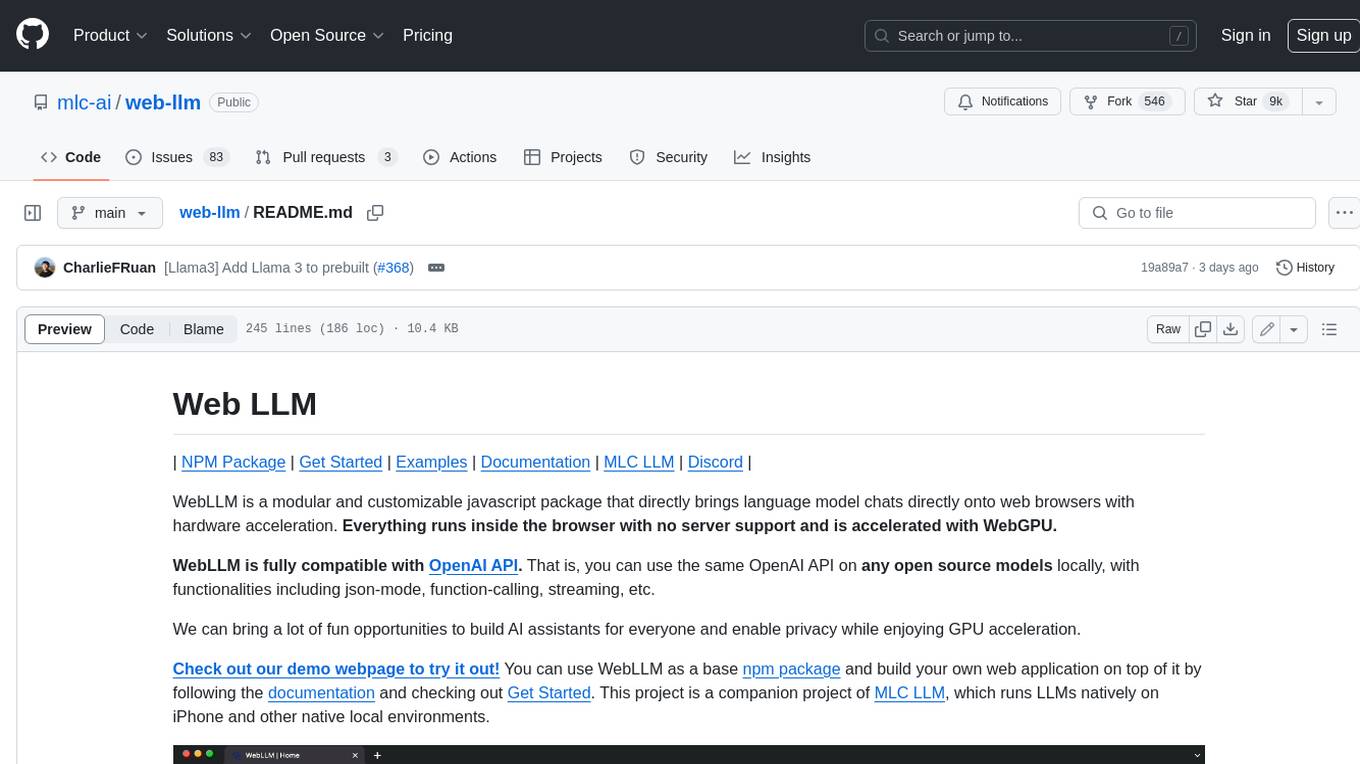
web-llm
WebLLM is a modular and customizable javascript package that directly brings language model chats directly onto web browsers with hardware acceleration. Everything runs inside the browser with no server support and is accelerated with WebGPU. WebLLM is fully compatible with OpenAI API. That is, you can use the same OpenAI API on any open source models locally, with functionalities including json-mode, function-calling, streaming, etc. We can bring a lot of fun opportunities to build AI assistants for everyone and enable privacy while enjoying GPU acceleration.
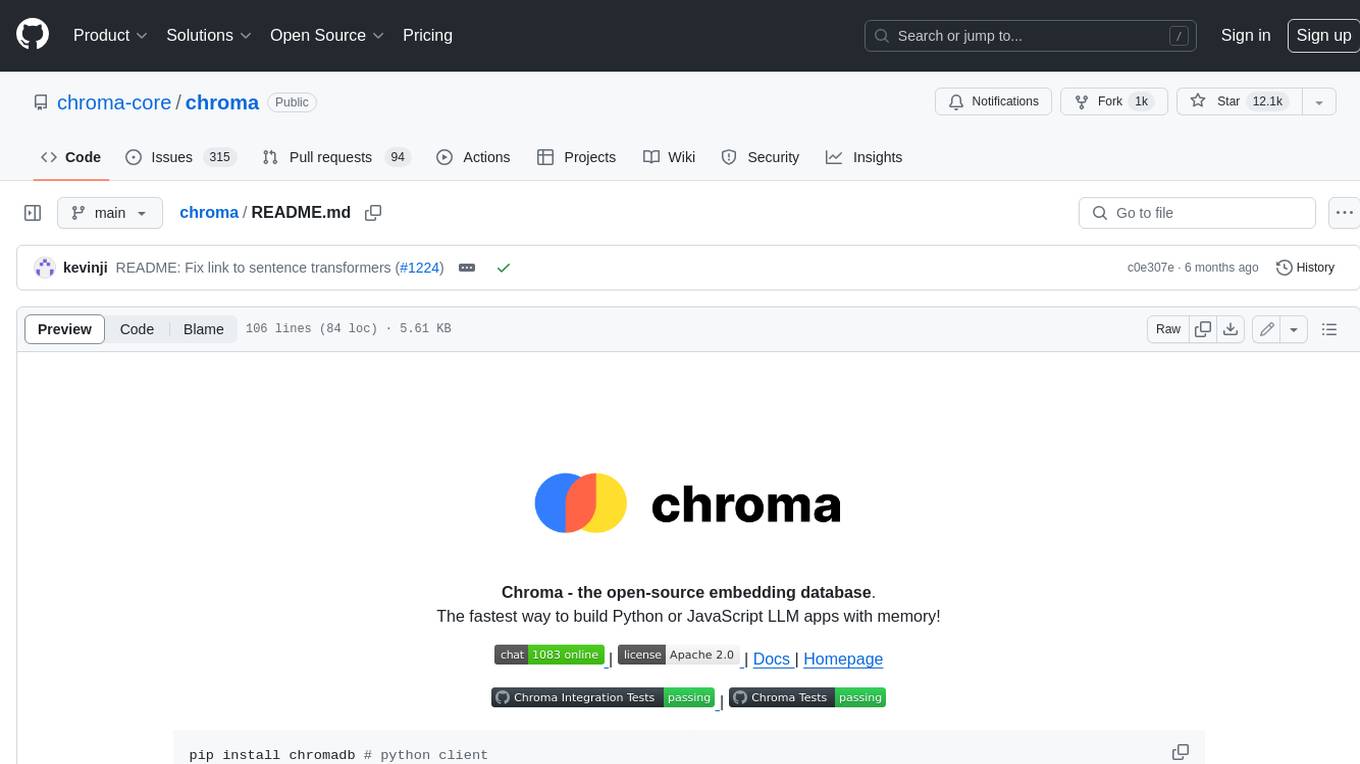
chroma
Chroma is an open-source embedding database that provides a simple, scalable, and feature-rich way to build Python or JavaScript LLM apps with memory. It offers a fully-typed, fully-tested, and fully-documented API that makes it easy to get started and scale your applications. Chroma also integrates with popular tools like LangChain and LlamaIndex, and supports a variety of embedding models, including Sentence Transformers, OpenAI embeddings, and Cohere embeddings. With Chroma, you can easily add documents to your database, query relevant documents with natural language, and compose documents into the context window of an LLM like GPT3 for additional summarization or analysis.
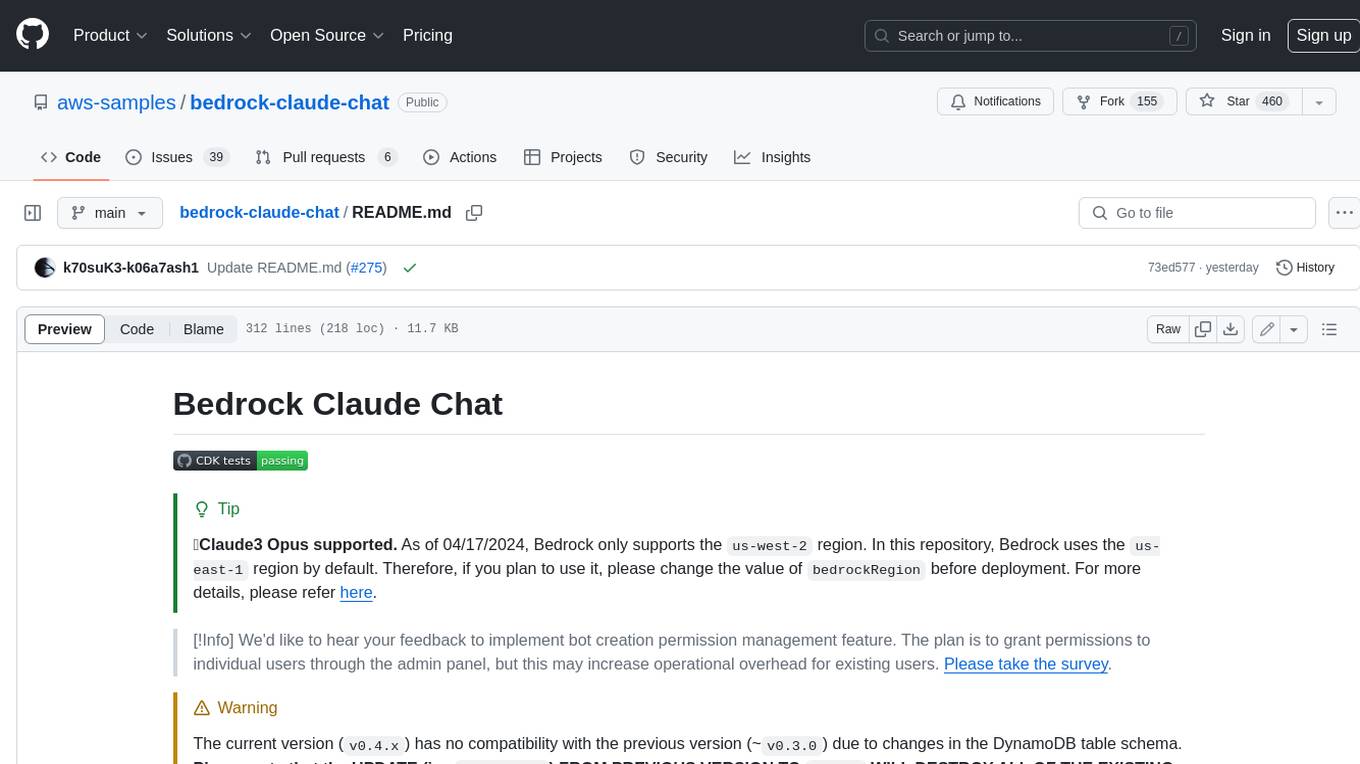
bedrock-claude-chat
This repository is a sample chatbot using the Anthropic company's LLM Claude, one of the foundational models provided by Amazon Bedrock for generative AI. It allows users to have basic conversations with the chatbot, personalize it with their own instructions and external knowledge, and analyze usage for each user/bot on the administrator dashboard. The chatbot supports various languages, including English, Japanese, Korean, Chinese, French, German, and Spanish. Deployment is straightforward and can be done via the command line or by using AWS CDK. The architecture is built on AWS managed services, eliminating the need for infrastructure management and ensuring scalability, reliability, and security.
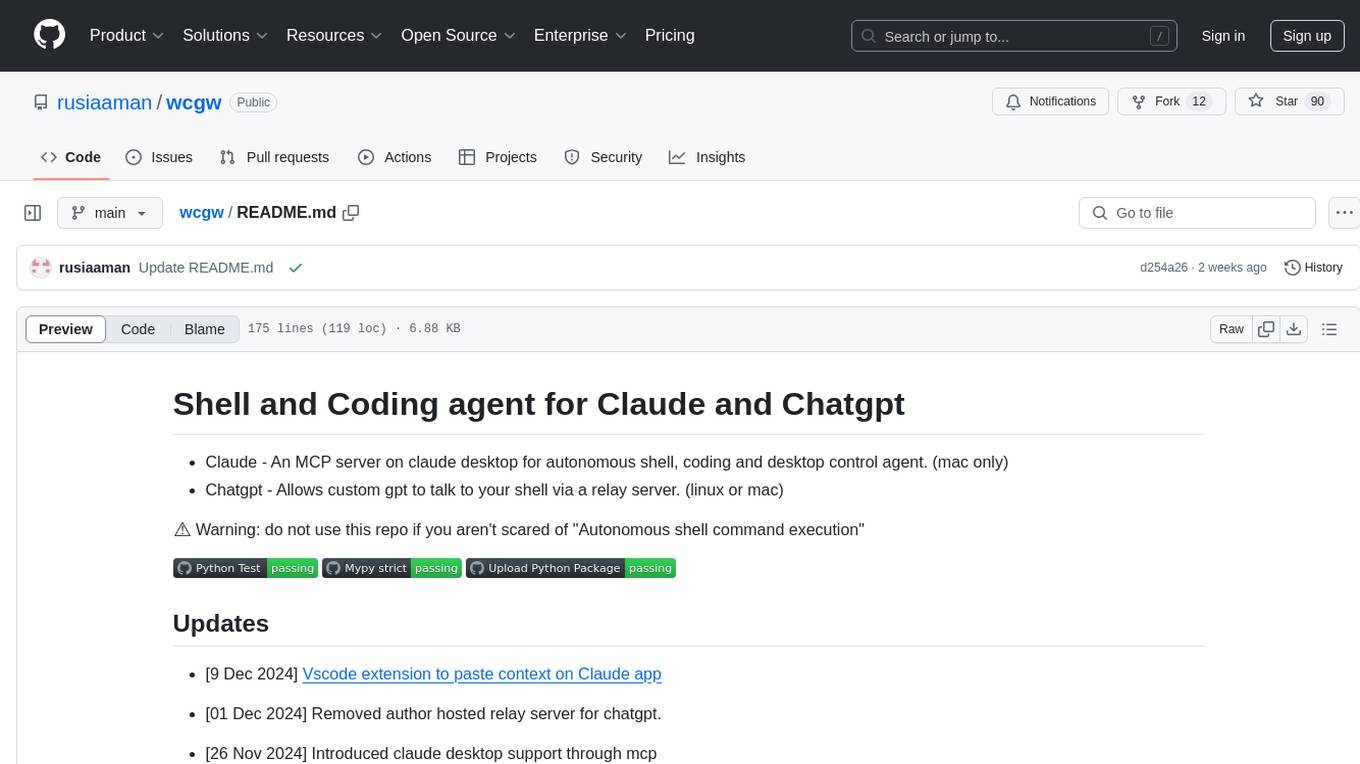
wcgw
wcgw is a shell and coding agent designed for Claude and Chatgpt. It provides full shell access with no restrictions, desktop control on Claude for screen capture and control, interactive command handling, large file editing, and REPL support. Users can use wcgw to create, execute, and iterate on tasks, such as solving problems with Python, finding code instances, setting up projects, creating web apps, editing large files, and running server commands. Additionally, wcgw supports computer use on Docker containers for desktop control. The tool can be extended with a VS Code extension for pasting context on Claude app and integrates with Chatgpt for custom GPT interactions.
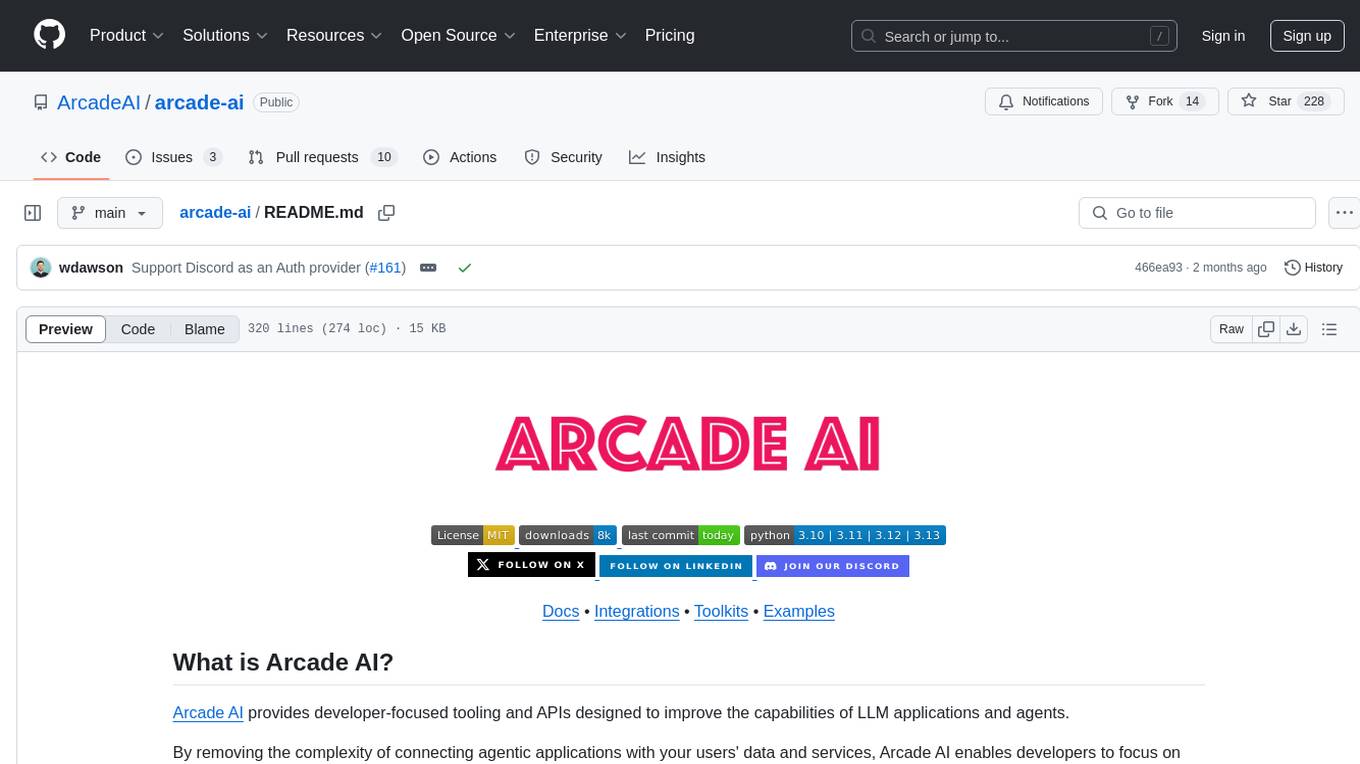
arcade-ai
Arcade AI is a developer-focused tooling and API platform designed to enhance the capabilities of LLM applications and agents. It simplifies the process of connecting agentic applications with user data and services, allowing developers to concentrate on building their applications. The platform offers prebuilt toolkits for interacting with various services, supports multiple authentication providers, and provides access to different language models. Users can also create custom toolkits and evaluate their tools using Arcade AI. Contributions are welcome, and self-hosting is possible with the provided documentation.
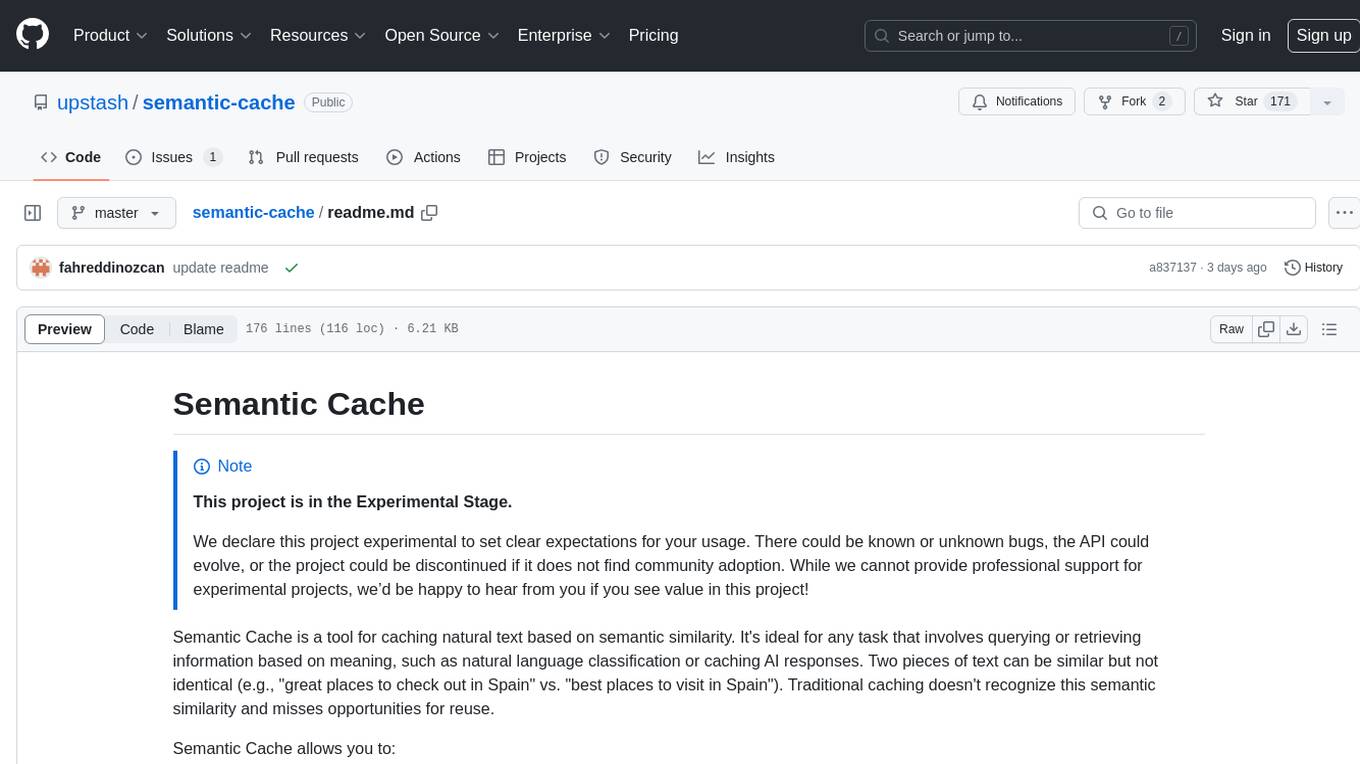
semantic-cache
Semantic Cache is a tool for caching natural text based on semantic similarity. It allows for classifying text into categories, caching AI responses, and reducing API latency by responding to similar queries with cached values. The tool stores cache entries by meaning, handles synonyms, supports multiple languages, understands complex queries, and offers easy integration with Node.js applications. Users can set a custom proximity threshold for filtering results. The tool is ideal for tasks involving querying or retrieving information based on meaning, such as natural language classification or caching AI responses.
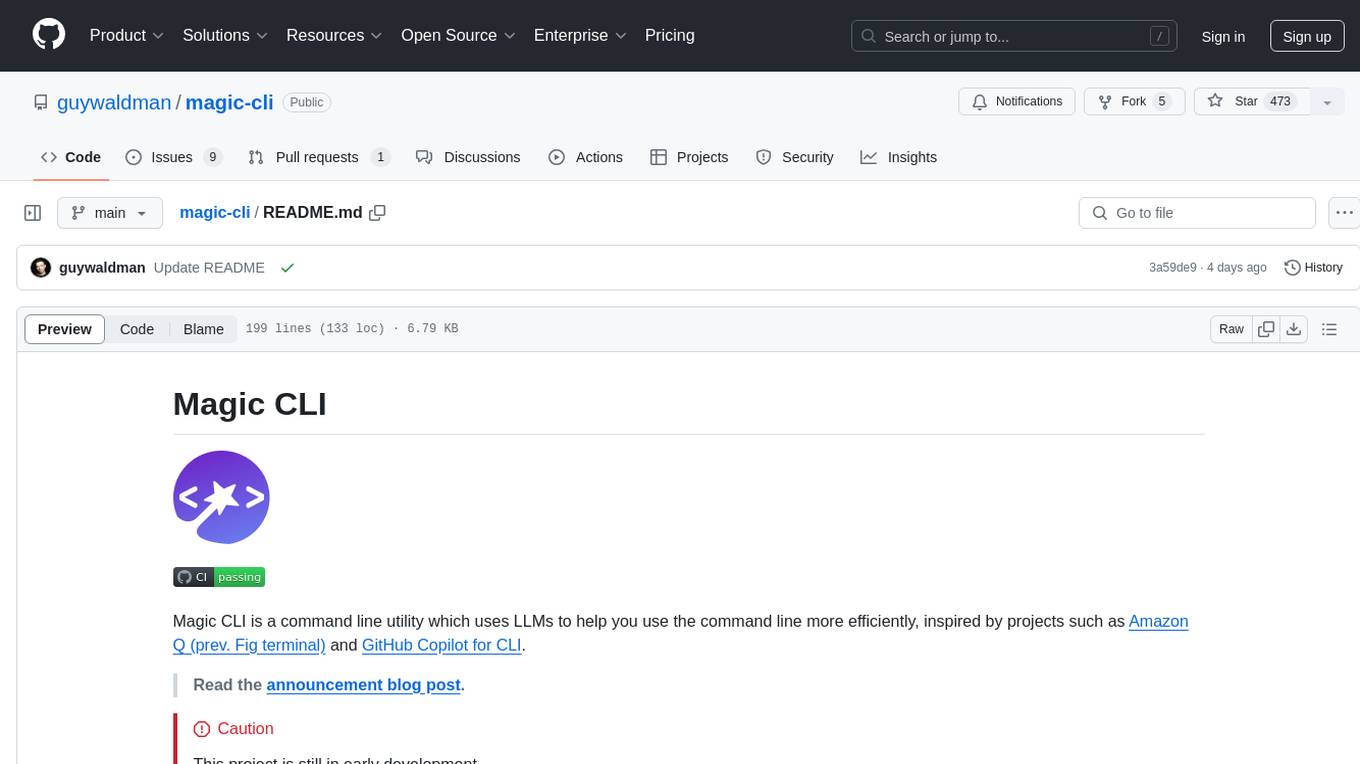
magic-cli
Magic CLI is a command line utility that leverages Large Language Models (LLMs) to enhance command line efficiency. It is inspired by projects like Amazon Q and GitHub Copilot for CLI. The tool allows users to suggest commands, search across command history, and generate commands for specific tasks using local or remote LLM providers. Magic CLI also provides configuration options for LLM selection and response generation. The project is still in early development, so users should expect breaking changes and bugs.
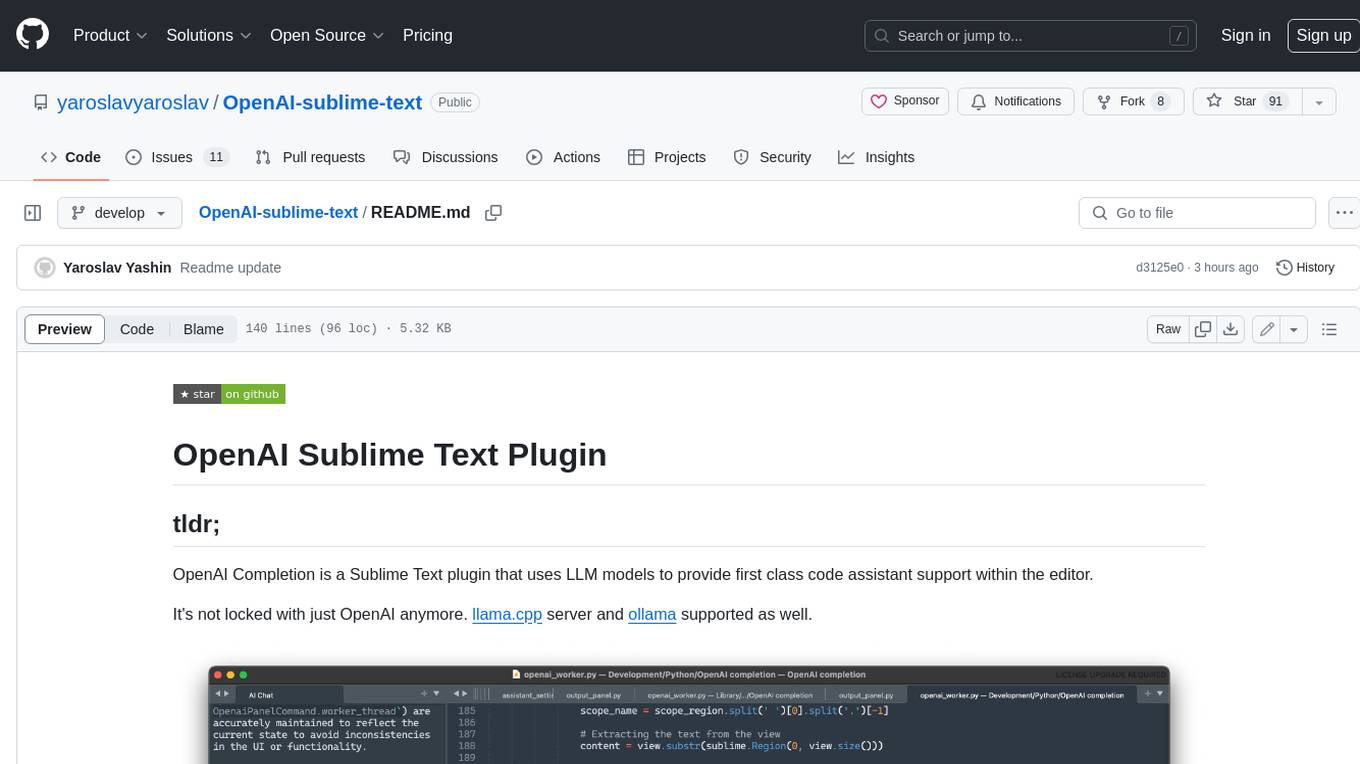
OpenAI-sublime-text
The OpenAI Completion plugin for Sublime Text provides first-class code assistant support within the editor. It utilizes LLM models to manipulate code, engage in chat mode, and perform various tasks. The plugin supports OpenAI, llama.cpp, and ollama models, allowing users to customize their AI assistant experience. It offers separated chat histories and assistant settings for different projects, enabling context-specific interactions. Additionally, the plugin supports Markdown syntax with code language syntax highlighting, server-side streaming for faster response times, and proxy support for secure connections. Users can configure the plugin's settings to set their OpenAI API key, adjust assistant modes, and manage chat history. Overall, the OpenAI Completion plugin enhances the Sublime Text editor with powerful AI capabilities, streamlining coding workflows and fostering collaboration with AI assistants.
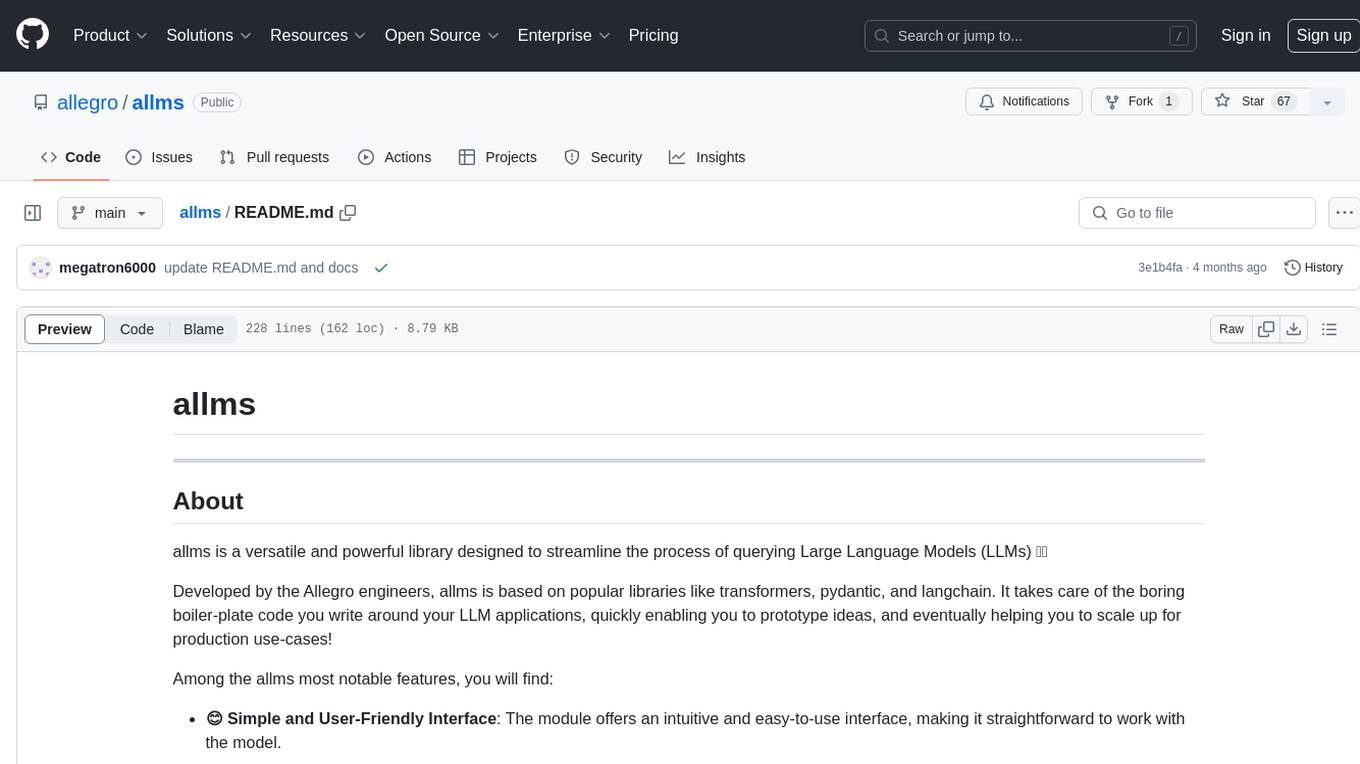
allms
allms is a versatile and powerful library designed to streamline the process of querying Large Language Models (LLMs). Developed by Allegro engineers, it simplifies working with LLM applications by providing a user-friendly interface, asynchronous querying, automatic retrying mechanism, error handling, and output parsing. It supports various LLM families hosted on different platforms like OpenAI, Google, Azure, and GCP. The library offers features for configuring endpoint credentials, batch querying with symbolic variables, and forcing structured output format. It also provides documentation, quickstart guides, and instructions for local development, testing, updating documentation, and making new releases.
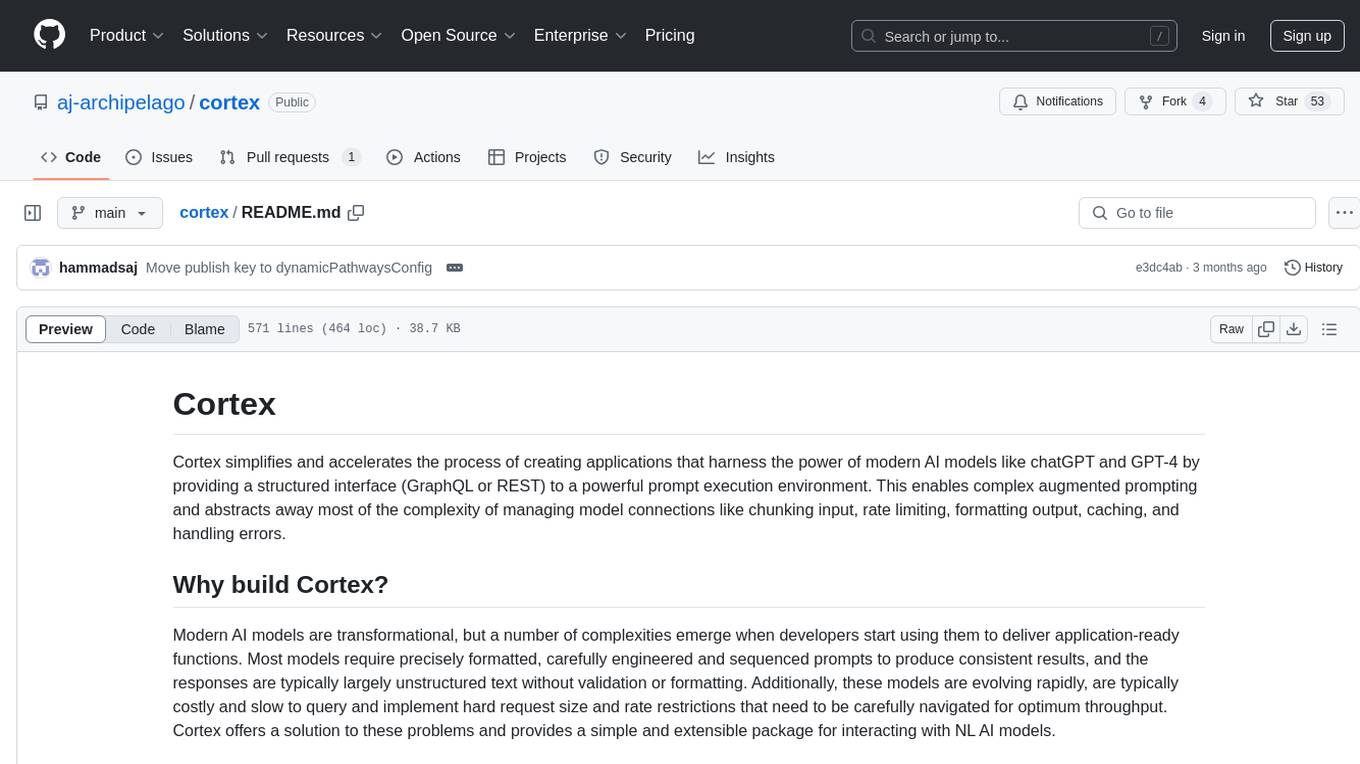
cortex
Cortex is a tool that simplifies and accelerates the process of creating applications utilizing modern AI models like chatGPT and GPT-4. It provides a structured interface (GraphQL or REST) to a prompt execution environment, enabling complex augmented prompting and abstracting away model connection complexities like input chunking, rate limiting, output formatting, caching, and error handling. Cortex offers a solution to challenges faced when using AI models, providing a simple package for interacting with NL AI models.
For similar tasks
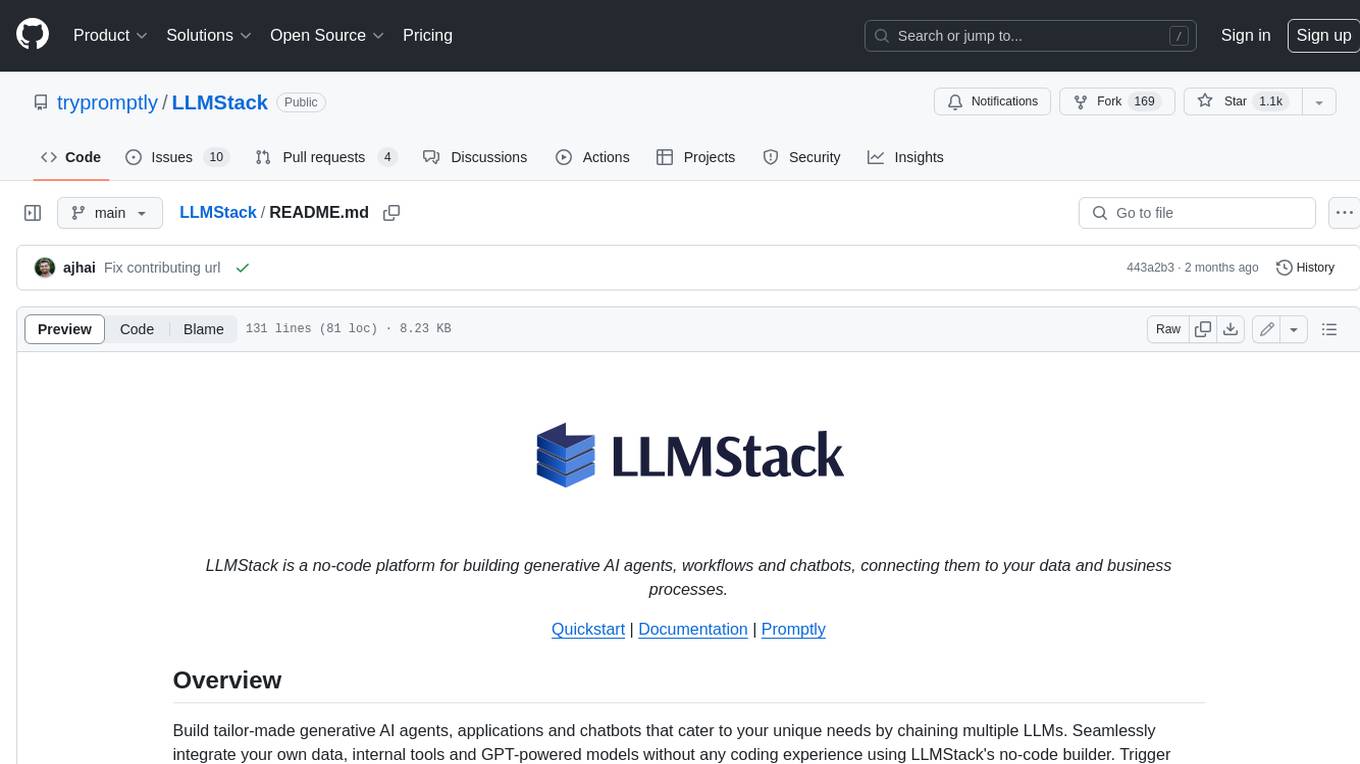
LLMStack
LLMStack is a no-code platform for building generative AI agents, workflows, and chatbots. It allows users to connect their own data, internal tools, and GPT-powered models without any coding experience. LLMStack can be deployed to the cloud or on-premise and can be accessed via HTTP API or triggered from Slack or Discord.
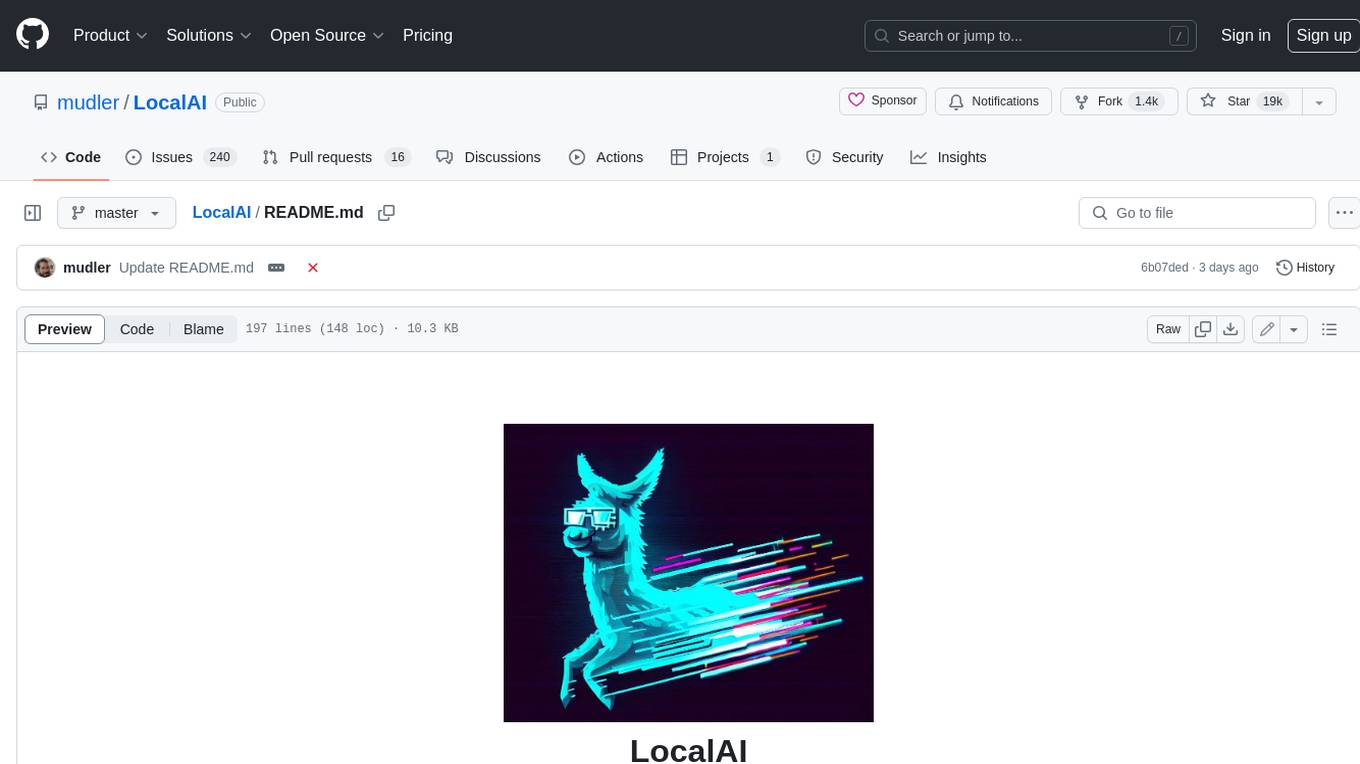
LocalAI
LocalAI is a free and open-source OpenAI alternative that acts as a drop-in replacement REST API compatible with OpenAI (Elevenlabs, Anthropic, etc.) API specifications for local AI inferencing. It allows users to run LLMs, generate images, audio, and more locally or on-premises with consumer-grade hardware, supporting multiple model families and not requiring a GPU. LocalAI offers features such as text generation with GPTs, text-to-audio, audio-to-text transcription, image generation with stable diffusion, OpenAI functions, embeddings generation for vector databases, constrained grammars, downloading models directly from Huggingface, and a Vision API. It provides a detailed step-by-step introduction in its Getting Started guide and supports community integrations such as custom containers, WebUIs, model galleries, and various bots for Discord, Slack, and Telegram. LocalAI also offers resources like an LLM fine-tuning guide, instructions for local building and Kubernetes installation, projects integrating LocalAI, and a how-tos section curated by the community. It encourages users to cite the repository when utilizing it in downstream projects and acknowledges the contributions of various software from the community.
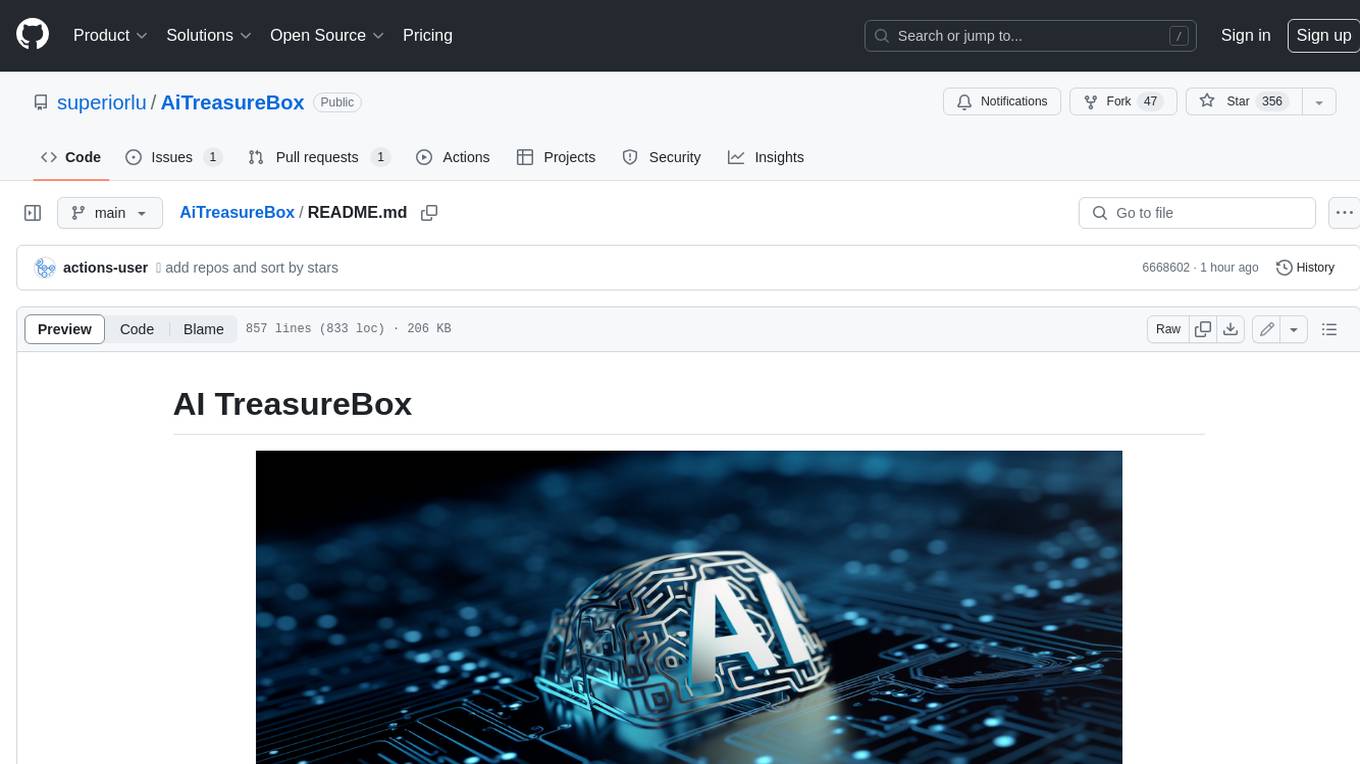
AiTreasureBox
AiTreasureBox is a versatile AI tool that provides a collection of pre-trained models and algorithms for various machine learning tasks. It simplifies the process of implementing AI solutions by offering ready-to-use components that can be easily integrated into projects. With AiTreasureBox, users can quickly prototype and deploy AI applications without the need for extensive knowledge in machine learning or deep learning. The tool covers a wide range of tasks such as image classification, text generation, sentiment analysis, object detection, and more. It is designed to be user-friendly and accessible to both beginners and experienced developers, making AI development more efficient and accessible to a wider audience.
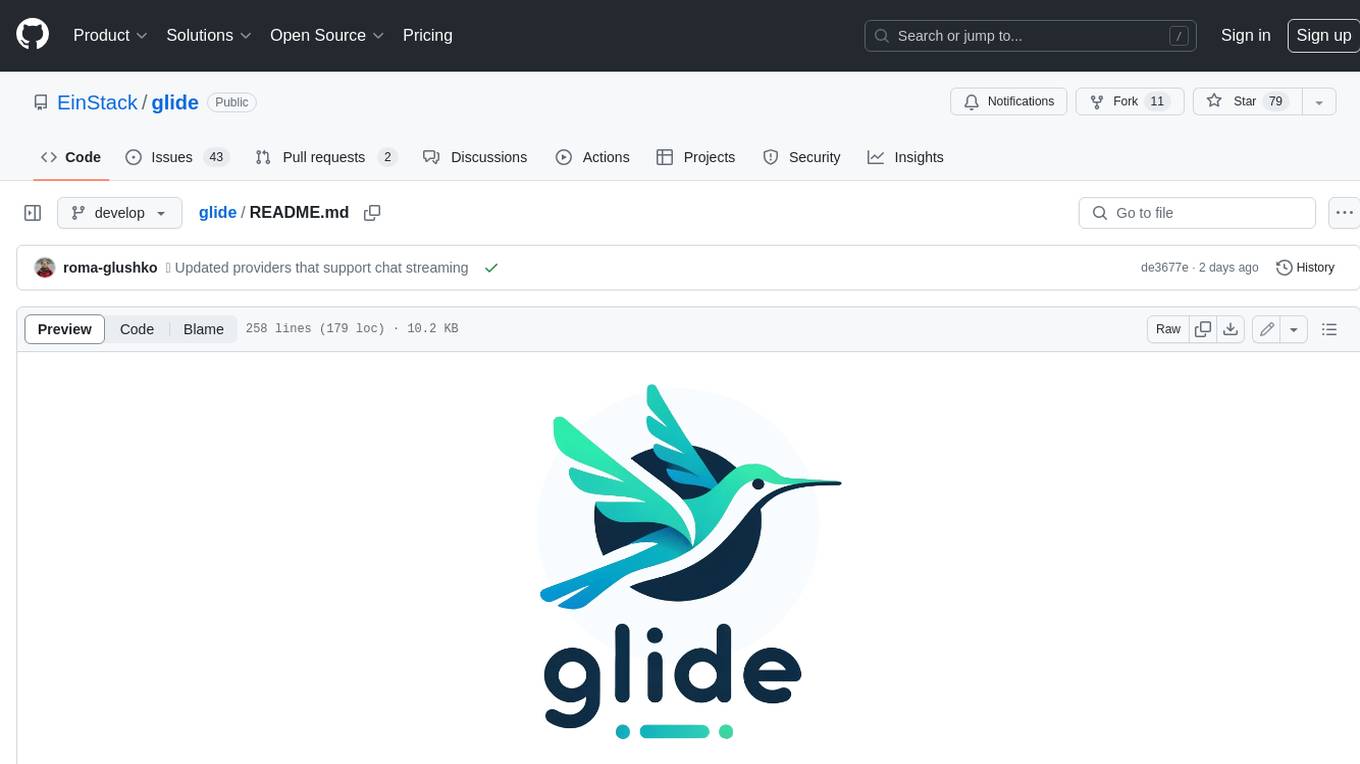
glide
Glide is a cloud-native LLM gateway that provides a unified REST API for accessing various large language models (LLMs) from different providers. It handles LLMOps tasks such as model failover, caching, key management, and more, making it easy to integrate LLMs into applications. Glide supports popular LLM providers like OpenAI, Anthropic, Azure OpenAI, AWS Bedrock (Titan), Cohere, Google Gemini, OctoML, and Ollama. It offers high availability, performance, and observability, and provides SDKs for Python and NodeJS to simplify integration.
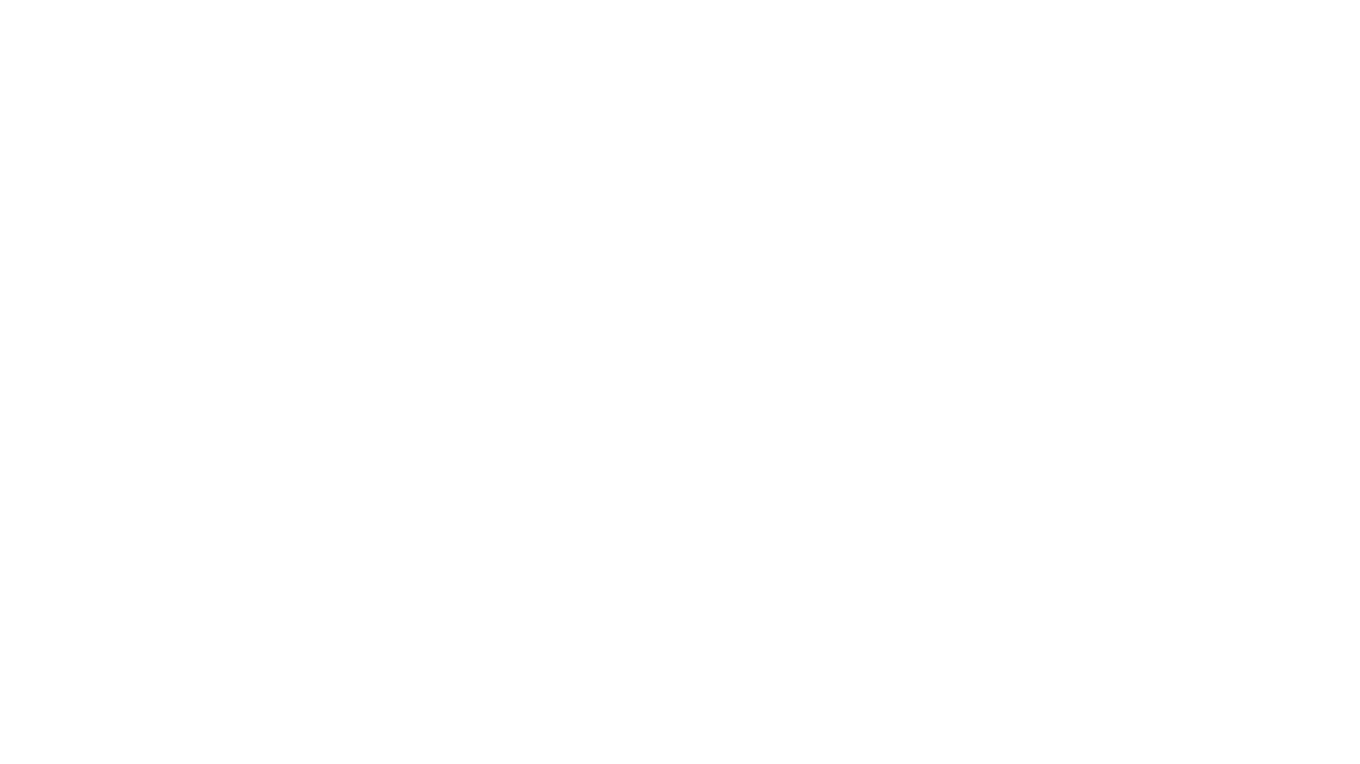
jupyter-ai
Jupyter AI connects generative AI with Jupyter notebooks. It provides a user-friendly and powerful way to explore generative AI models in notebooks and improve your productivity in JupyterLab and the Jupyter Notebook. Specifically, Jupyter AI offers: * An `%%ai` magic that turns the Jupyter notebook into a reproducible generative AI playground. This works anywhere the IPython kernel runs (JupyterLab, Jupyter Notebook, Google Colab, Kaggle, VSCode, etc.). * A native chat UI in JupyterLab that enables you to work with generative AI as a conversational assistant. * Support for a wide range of generative model providers, including AI21, Anthropic, AWS, Cohere, Gemini, Hugging Face, NVIDIA, and OpenAI. * Local model support through GPT4All, enabling use of generative AI models on consumer grade machines with ease and privacy.
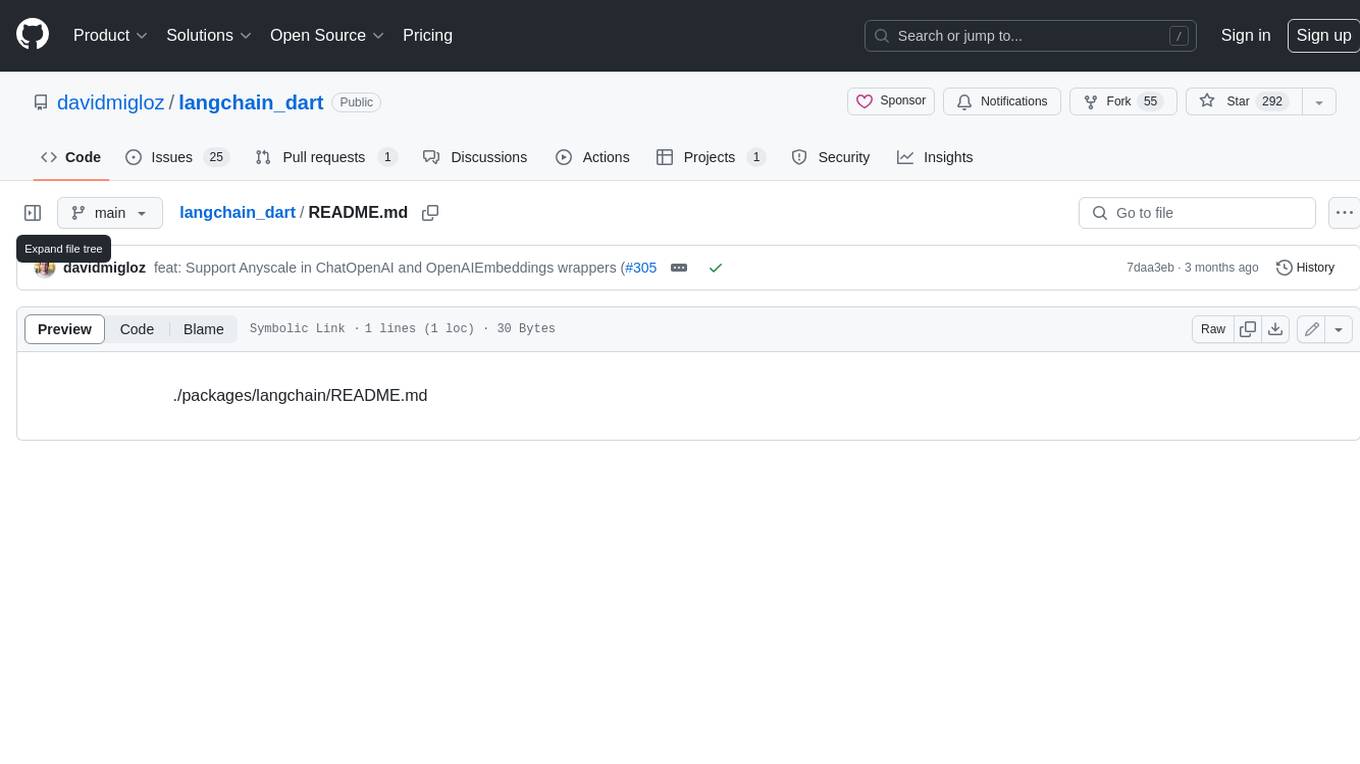
langchain_dart
LangChain.dart is a Dart port of the popular LangChain Python framework created by Harrison Chase. LangChain provides a set of ready-to-use components for working with language models and a standard interface for chaining them together to formulate more advanced use cases (e.g. chatbots, Q&A with RAG, agents, summarization, extraction, etc.). The components can be grouped into a few core modules: * **Model I/O:** LangChain offers a unified API for interacting with various LLM providers (e.g. OpenAI, Google, Mistral, Ollama, etc.), allowing developers to switch between them with ease. Additionally, it provides tools for managing model inputs (prompt templates and example selectors) and parsing the resulting model outputs (output parsers). * **Retrieval:** assists in loading user data (via document loaders), transforming it (with text splitters), extracting its meaning (using embedding models), storing (in vector stores) and retrieving it (through retrievers) so that it can be used to ground the model's responses (i.e. Retrieval-Augmented Generation or RAG). * **Agents:** "bots" that leverage LLMs to make informed decisions about which available tools (such as web search, calculators, database lookup, etc.) to use to accomplish the designated task. The different components can be composed together using the LangChain Expression Language (LCEL).
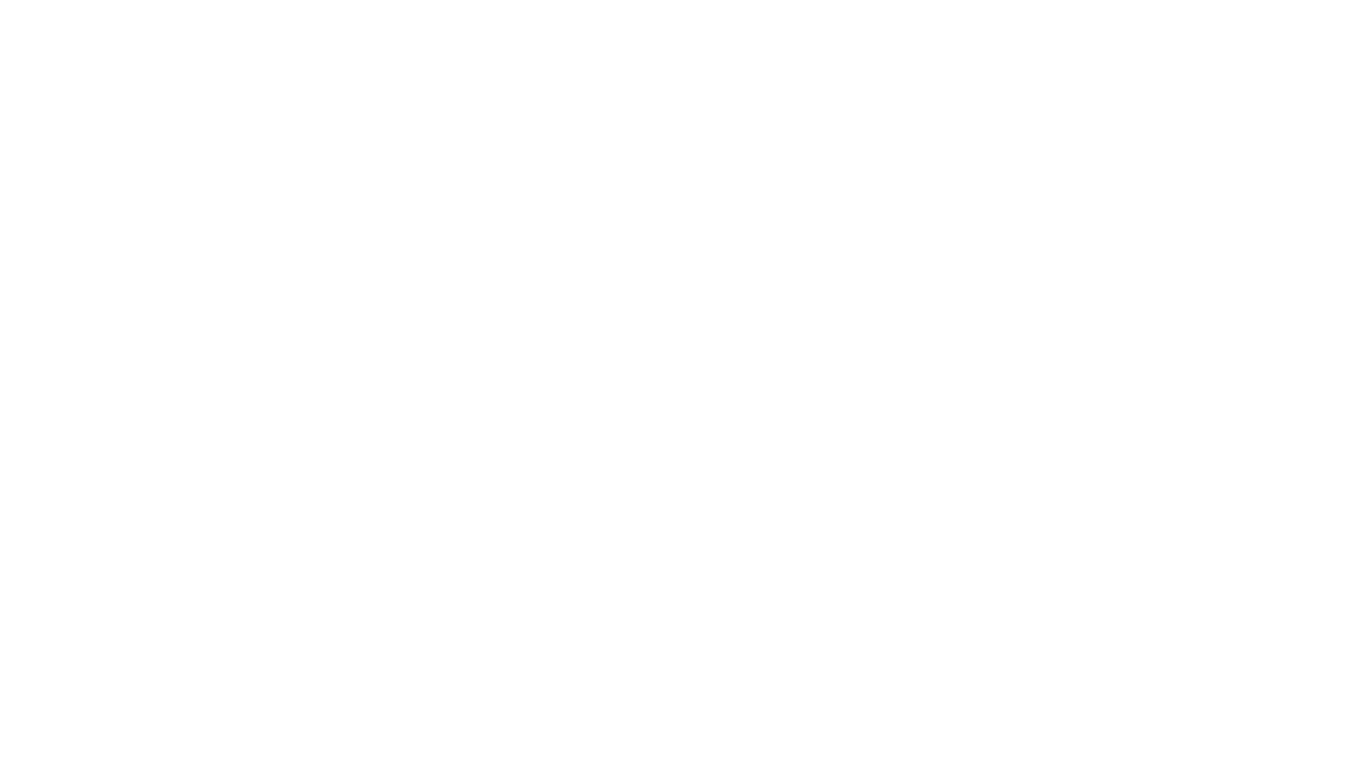
infinity
Infinity is an AI-native database designed for LLM applications, providing incredibly fast full-text and vector search capabilities. It supports a wide range of data types, including vectors, full-text, and structured data, and offers a fused search feature that combines multiple embeddings and full text. Infinity is easy to use, with an intuitive Python API and a single-binary architecture that simplifies deployment. It achieves high performance, with 0.1 milliseconds query latency on million-scale vector datasets and up to 15K QPS.
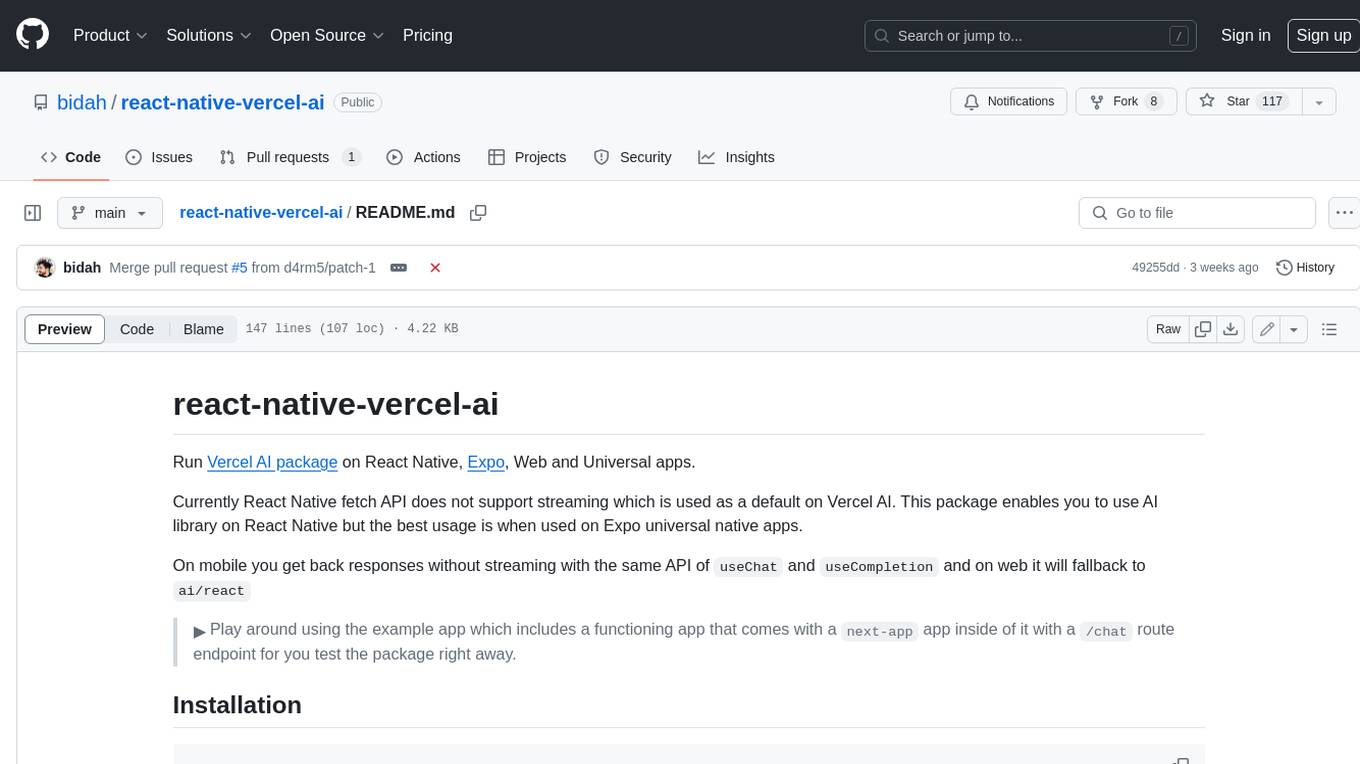
react-native-vercel-ai
Run Vercel AI package on React Native, Expo, Web and Universal apps. Currently React Native fetch API does not support streaming which is used as a default on Vercel AI. This package enables you to use AI library on React Native but the best usage is when used on Expo universal native apps. On mobile you get back responses without streaming with the same API of `useChat` and `useCompletion` and on web it will fallback to `ai/react`
For similar jobs
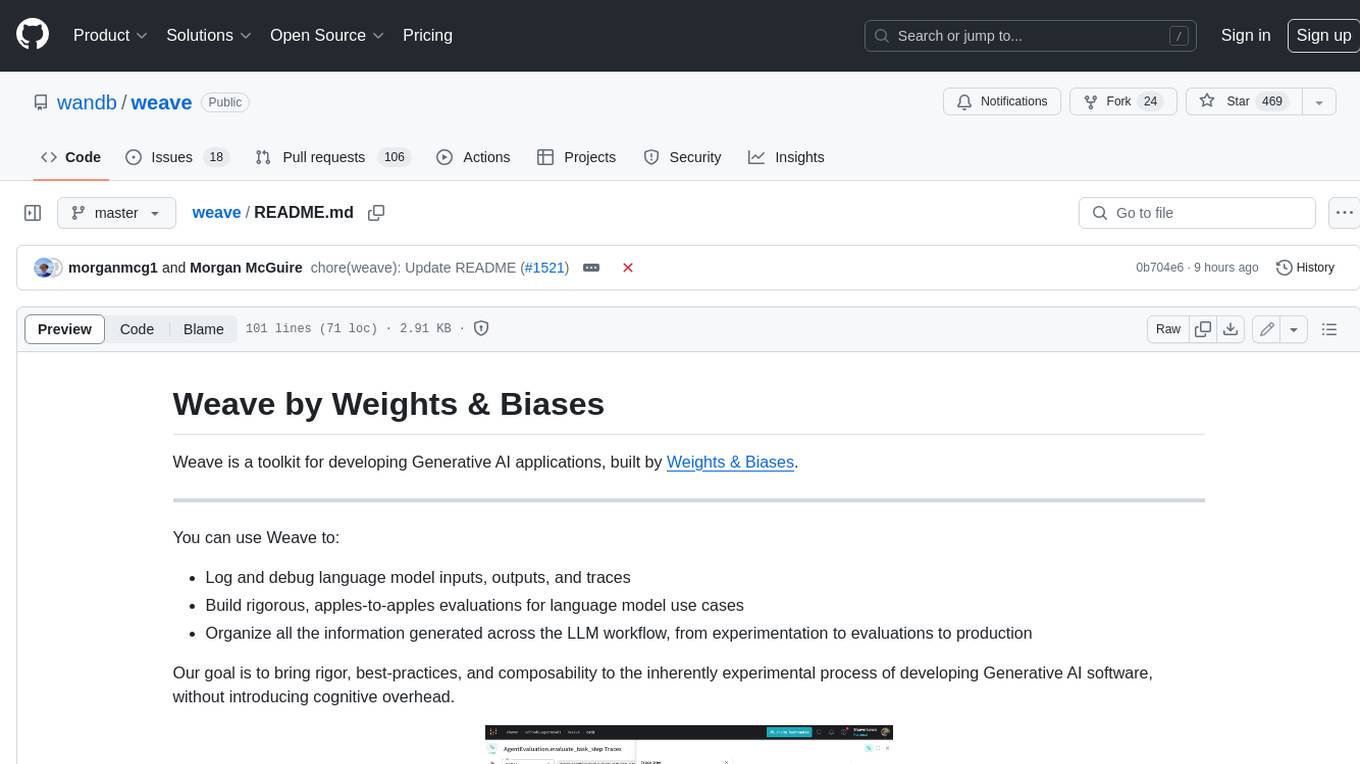
weave
Weave is a toolkit for developing Generative AI applications, built by Weights & Biases. With Weave, you can log and debug language model inputs, outputs, and traces; build rigorous, apples-to-apples evaluations for language model use cases; and organize all the information generated across the LLM workflow, from experimentation to evaluations to production. Weave aims to bring rigor, best-practices, and composability to the inherently experimental process of developing Generative AI software, without introducing cognitive overhead.
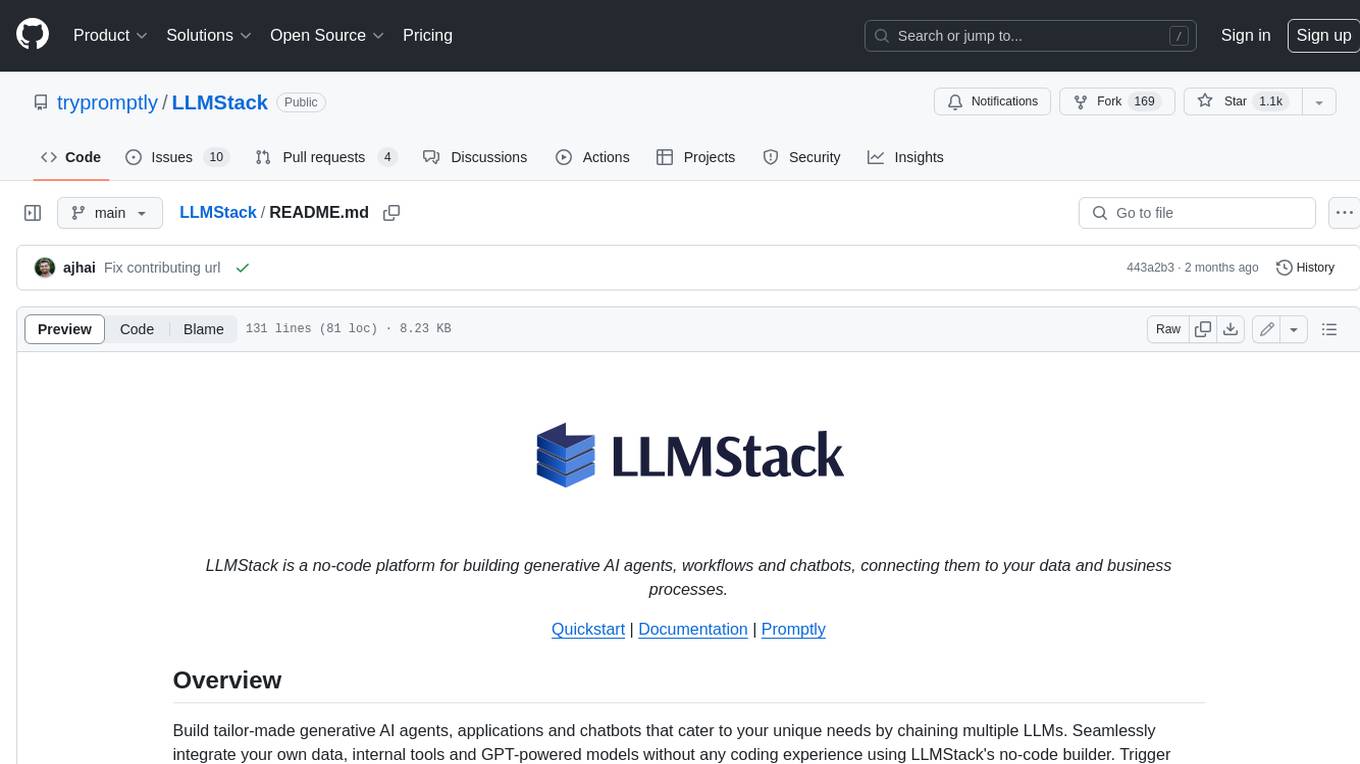
LLMStack
LLMStack is a no-code platform for building generative AI agents, workflows, and chatbots. It allows users to connect their own data, internal tools, and GPT-powered models without any coding experience. LLMStack can be deployed to the cloud or on-premise and can be accessed via HTTP API or triggered from Slack or Discord.
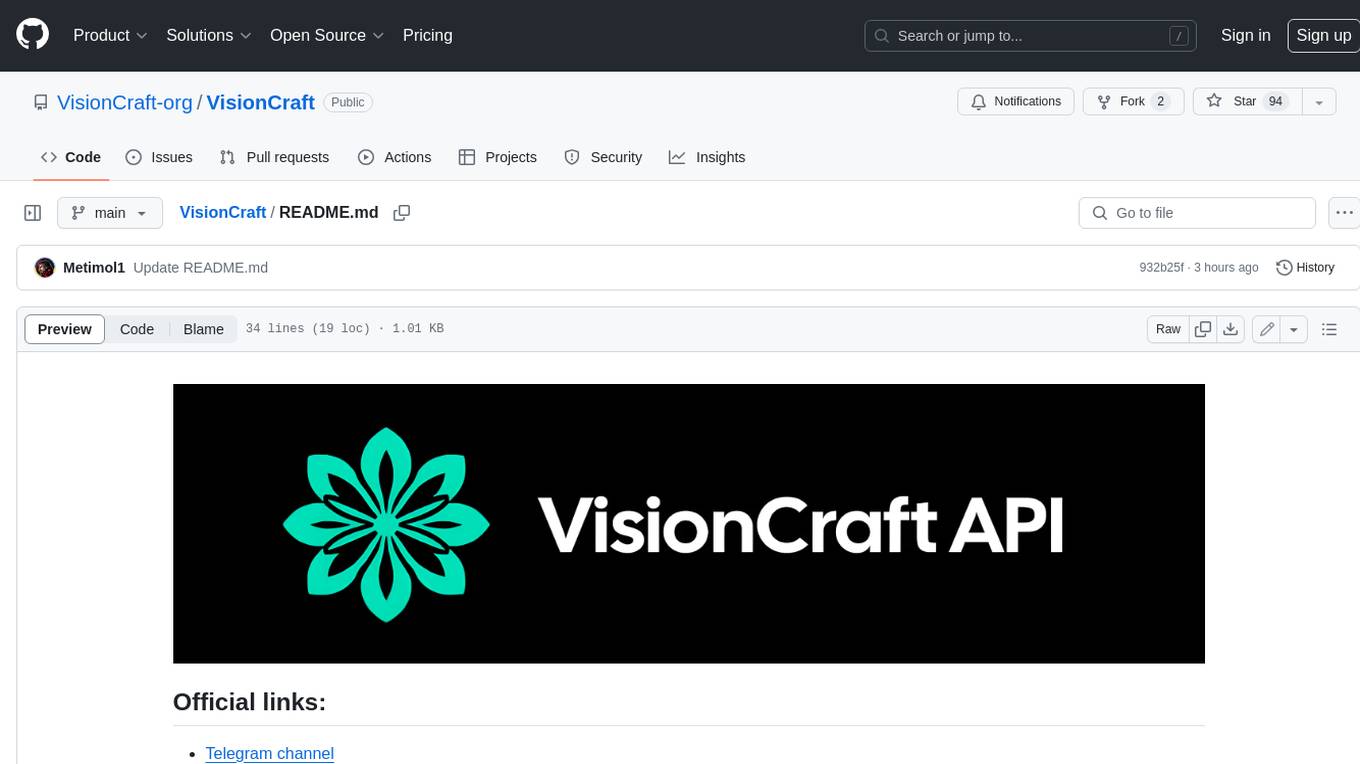
VisionCraft
The VisionCraft API is a free API for using over 100 different AI models. From images to sound.
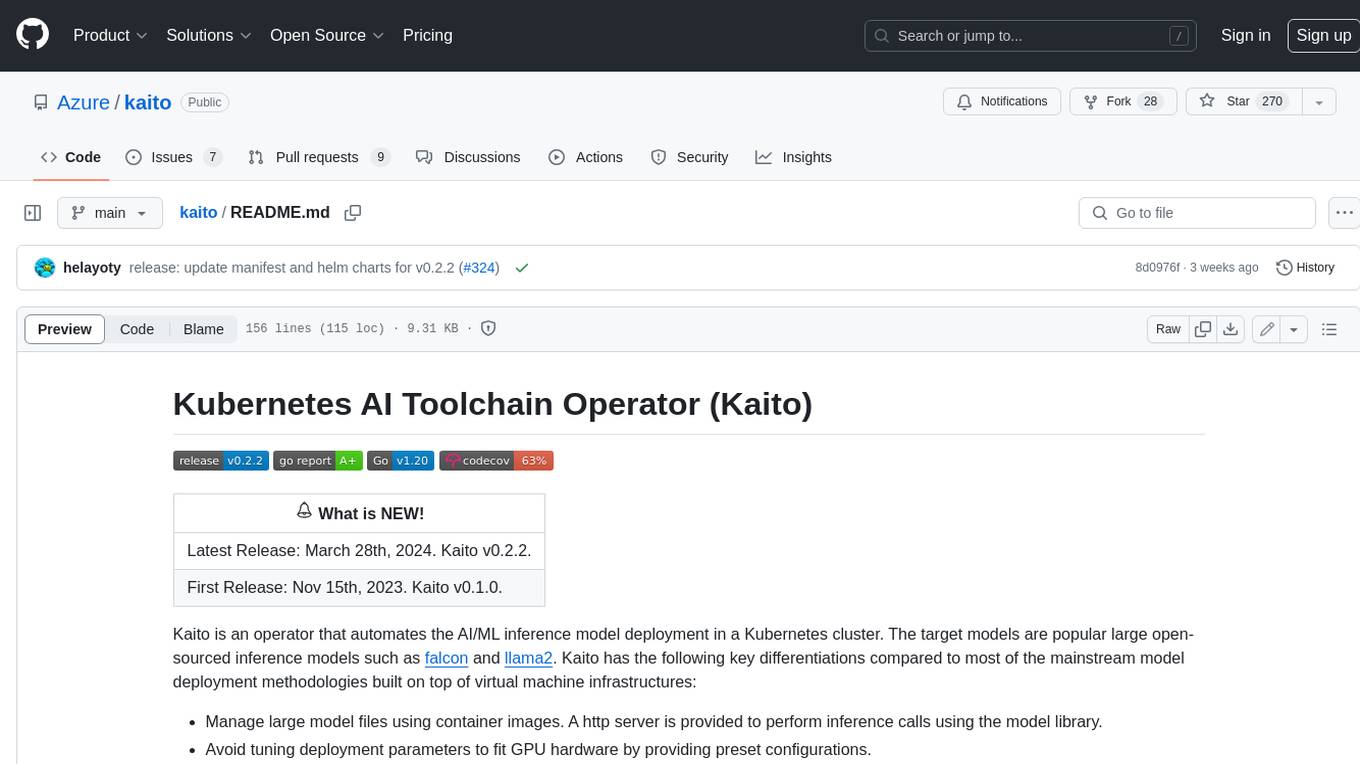
kaito
Kaito is an operator that automates the AI/ML inference model deployment in a Kubernetes cluster. It manages large model files using container images, avoids tuning deployment parameters to fit GPU hardware by providing preset configurations, auto-provisions GPU nodes based on model requirements, and hosts large model images in the public Microsoft Container Registry (MCR) if the license allows. Using Kaito, the workflow of onboarding large AI inference models in Kubernetes is largely simplified.
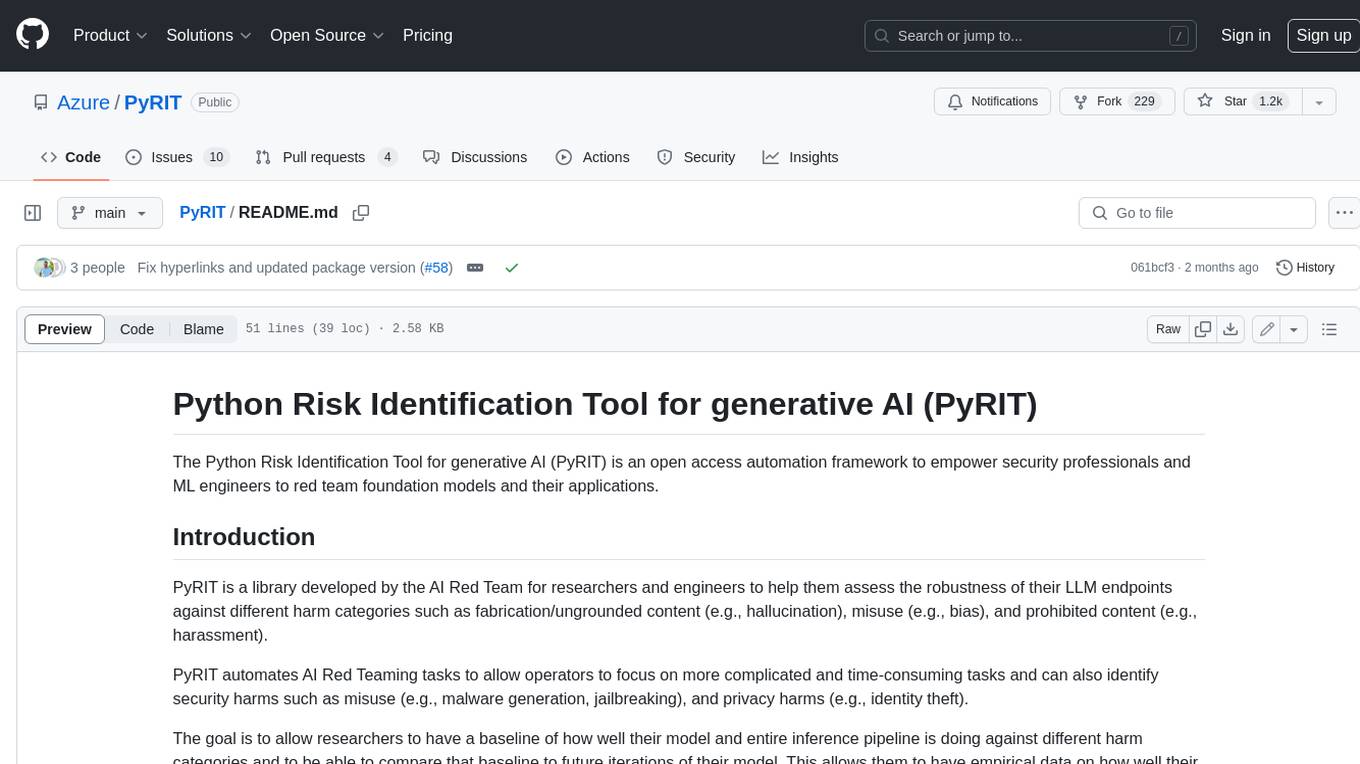
PyRIT
PyRIT is an open access automation framework designed to empower security professionals and ML engineers to red team foundation models and their applications. It automates AI Red Teaming tasks to allow operators to focus on more complicated and time-consuming tasks and can also identify security harms such as misuse (e.g., malware generation, jailbreaking), and privacy harms (e.g., identity theft). The goal is to allow researchers to have a baseline of how well their model and entire inference pipeline is doing against different harm categories and to be able to compare that baseline to future iterations of their model. This allows them to have empirical data on how well their model is doing today, and detect any degradation of performance based on future improvements.
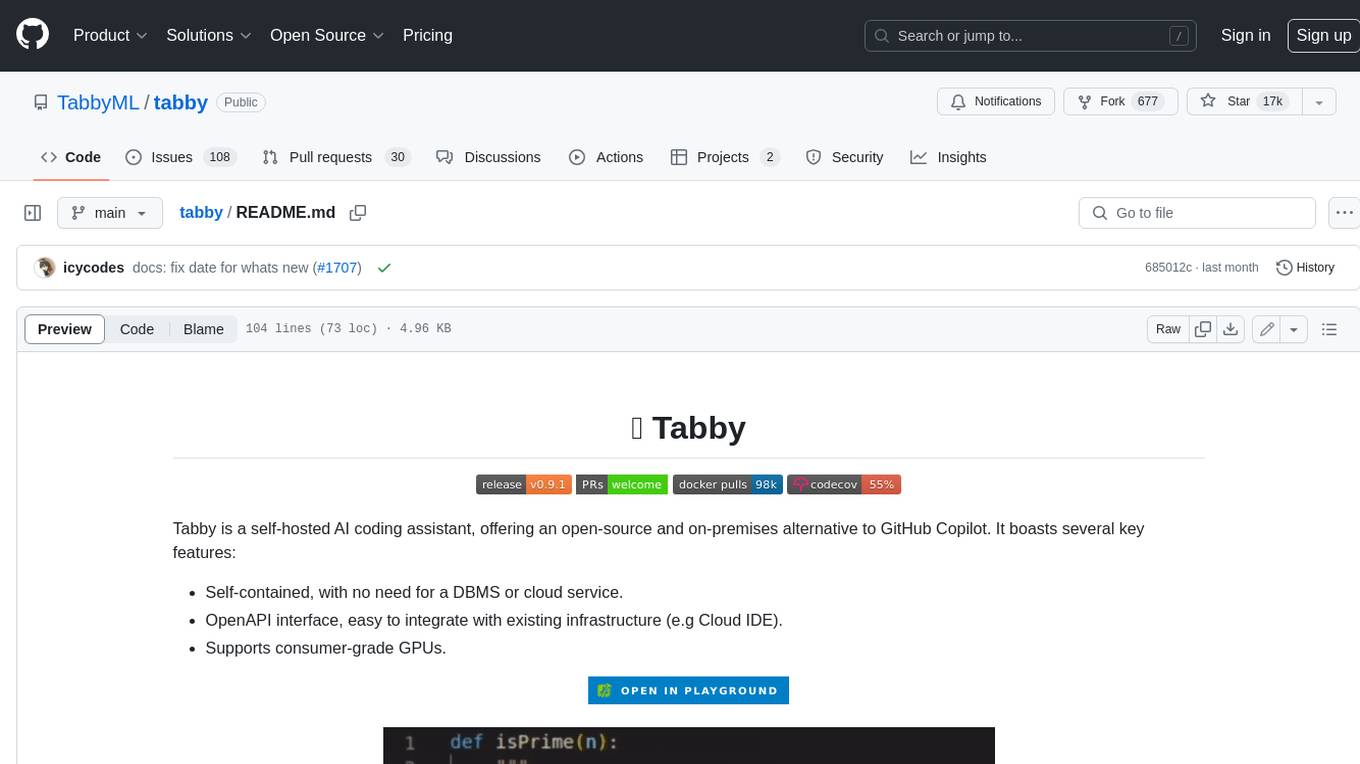
tabby
Tabby is a self-hosted AI coding assistant, offering an open-source and on-premises alternative to GitHub Copilot. It boasts several key features: * Self-contained, with no need for a DBMS or cloud service. * OpenAPI interface, easy to integrate with existing infrastructure (e.g Cloud IDE). * Supports consumer-grade GPUs.
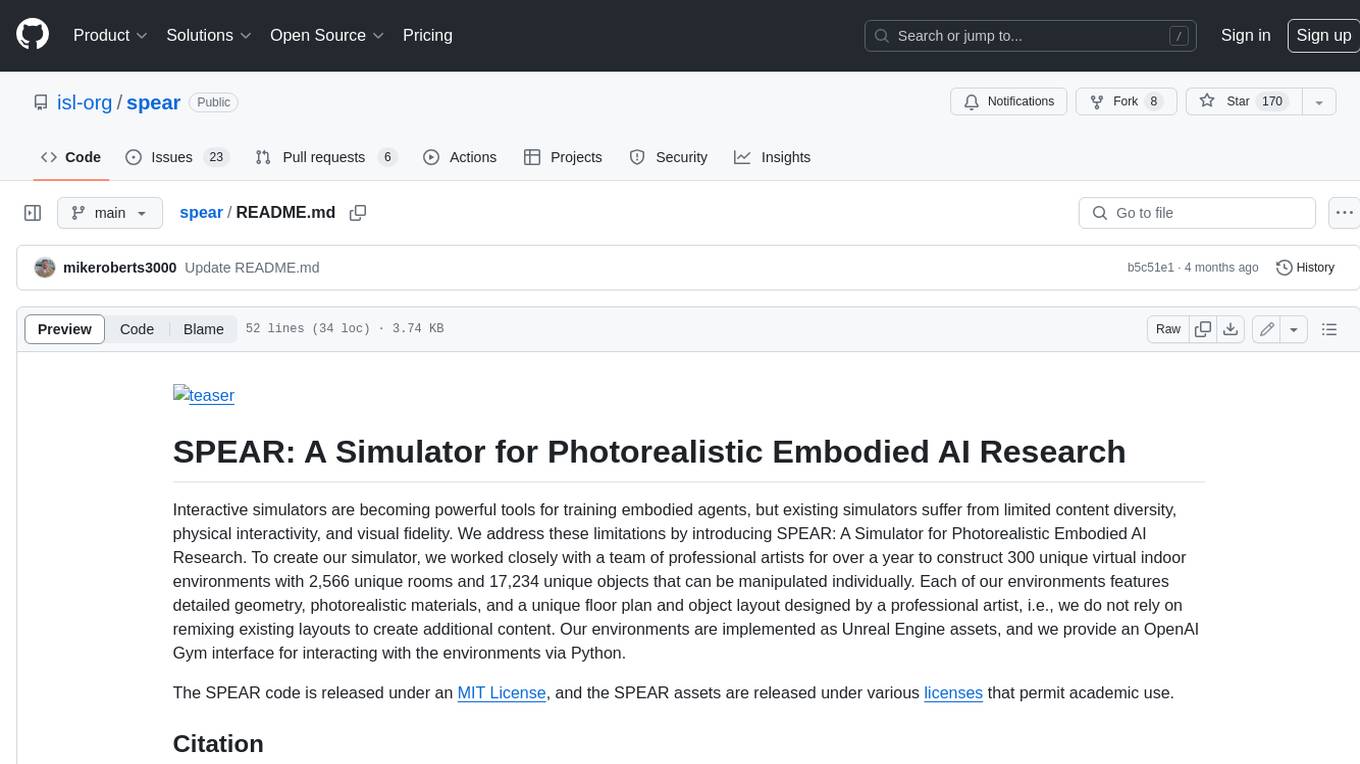
spear
SPEAR (Simulator for Photorealistic Embodied AI Research) is a powerful tool for training embodied agents. It features 300 unique virtual indoor environments with 2,566 unique rooms and 17,234 unique objects that can be manipulated individually. Each environment is designed by a professional artist and features detailed geometry, photorealistic materials, and a unique floor plan and object layout. SPEAR is implemented as Unreal Engine assets and provides an OpenAI Gym interface for interacting with the environments via Python.
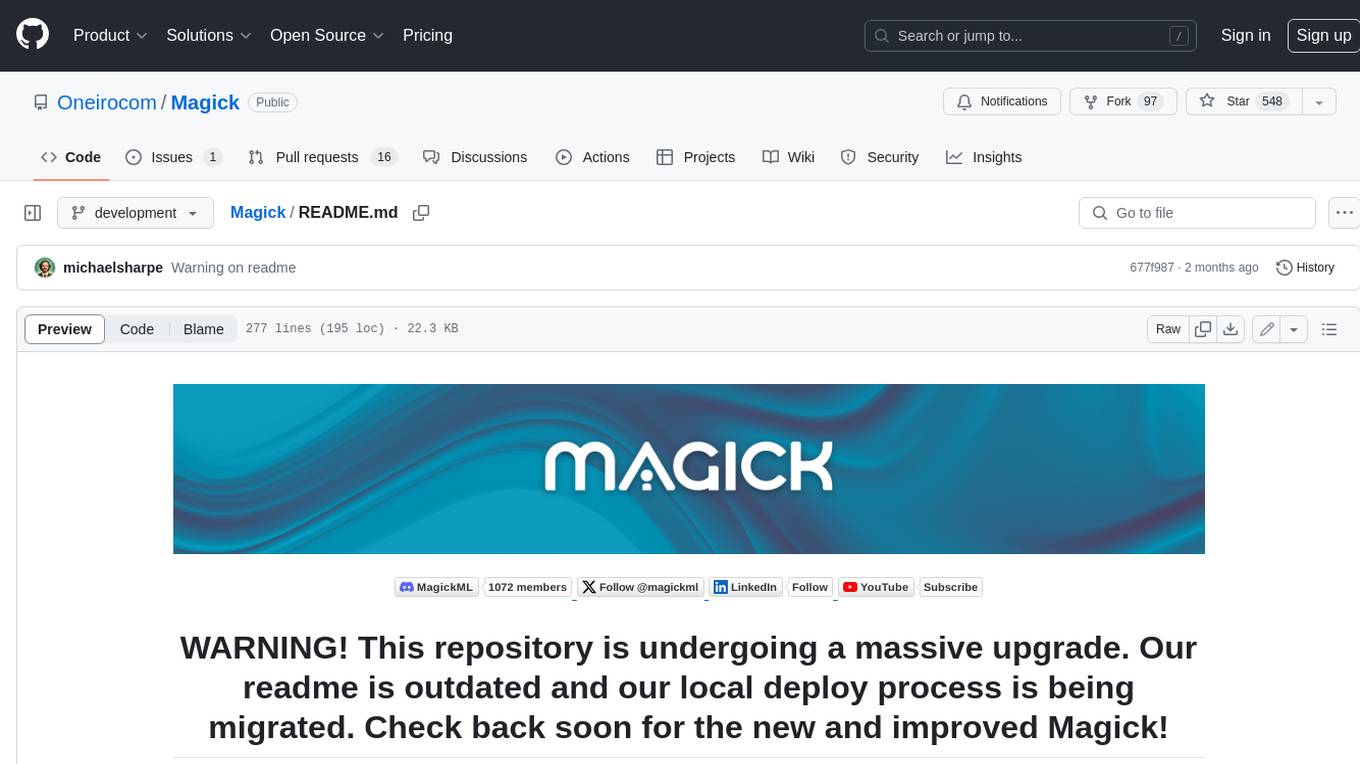
Magick
Magick is a groundbreaking visual AIDE (Artificial Intelligence Development Environment) for no-code data pipelines and multimodal agents. Magick can connect to other services and comes with nodes and templates well-suited for intelligent agents, chatbots, complex reasoning systems and realistic characters.