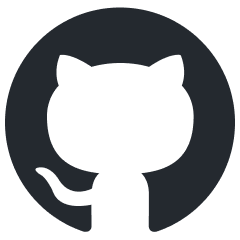
tonic_validate
Metrics to evaluate the quality of responses of your Retrieval Augmented Generation (RAG) applications.
Stars: 233
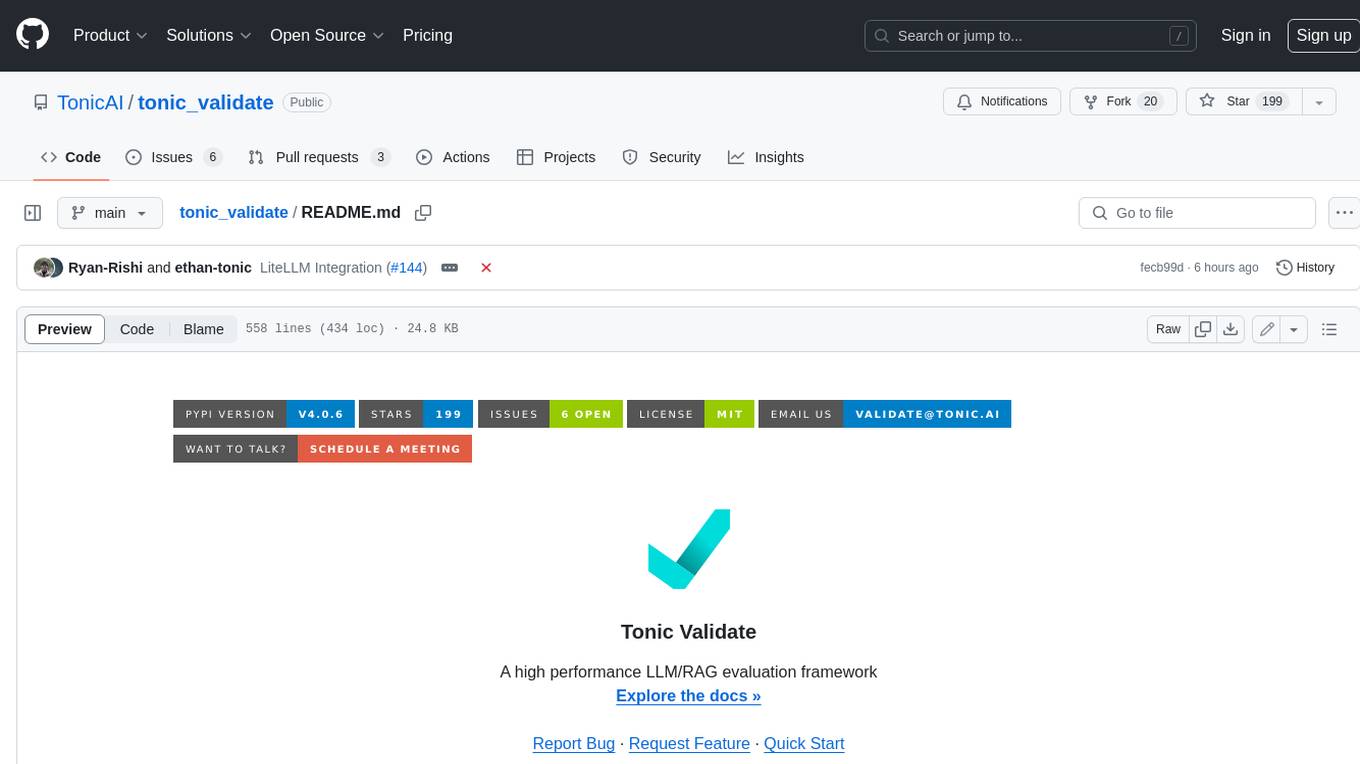
Tonic Validate is a framework for the evaluation of LLM outputs, such as Retrieval Augmented Generation (RAG) pipelines. Validate makes it easy to evaluate, track, and monitor your LLM and RAG applications. Validate allows you to evaluate your LLM outputs through the use of our provided metrics which measure everything from answer correctness to LLM hallucination. Additionally, Validate has an optional UI to visualize your evaluation results for easy tracking and monitoring.
README:
A high performance LLM/RAG evaluation framework
Explore the docs »
Enhance your data for RAG »
Report Bug
·
Request Feature
·
Quick Start
Table of Contents
Tonic Validate is a framework for the evaluation of LLM outputs, such as Retrieval Augmented Generation (RAG) pipelines. Validate makes it easy to evaluate, track, and monitor your LLM and RAG applications. Validate allows you to evaluate your LLM outputs through the use of our provided metrics which measure everything from answer correctness to LLM hallucination. Additionally, Validate has an optional UI to visualize your evaluation results for easy tracking and monitoring.
The foundation of a high-performing RAG systems is quality, secure data. High-quality data ensures that the information retrieved and generated is accurate, relevant, and reliable, which enhances the system's overall performance and user trust. Security, on the other hand, protects this valuable data from breaches, ensuring that sensitive information remains confidential and tamper-proof. Together, they form the bedrock of a robust RAG system, facilitating efficient, trustworthy retrieval and generation.
We are excited to introduce Tonic Textual, a powerful companion to Tonic Validate that can help improve your RAG system's performance. We created Tonic Textual to simplify and augment the data pre-processing for RAG systems. In just a few minutes, you can build automated unstructured data pipelines that extract text from unstructured data, detect and de-identify sensitive information, and transform the data into a format optimized for RAG systems. We also enrich your data with document metadata and contextual entity tags to build semantic entity graphs that ground your RAG system in truth, preventing hallucinations and improving the overall quality of the generated outputs.
You can learn more and try the tool completely free here. We'd love to hear what you think!
This is an example of how you may give instructions on setting up your project locally. To get a local copy up and running follow these simple example steps.
-
Install Tonic Validate
pip install tonic-validate
-
Use the following code snippet to get started.
from tonic_validate import ValidateScorer, Benchmark import os os.environ["OPENAI_API_KEY"] = "your-openai-key" # Function to simulate getting a response and context from your LLM # Replace this with your actual function call def get_llm_response(question): return { "llm_answer": "Paris", "llm_context_list": ["Paris is the capital of France."] } benchmark = Benchmark(questions=["What is the capital of France?"], answers=["Paris"]) # Score the responses for each question and answer pair scorer = ValidateScorer() run = scorer.score(benchmark, get_llm_response)
This code snippet, creates a benchmark with one question and reference answer and then scores the answer. Providing a reference answer is not required for most metrics (see below Metrics table).
Many users find value in running evaluations during the code review/pull request process. You can create your own automation here using the snippet above and knowledge found in our documentation and this readme OR you can take advantage of our absolutely free Github Action in the Github Marketplace. The listing is here. It's easy to setup but if you have any questions, just create an issue in the corresponding repository.
Metrics are used to score your LLM's performance. Validate ships with many different metrics which are applicable to most RAG systems. You can create your own metrics as well by providing your own implementation of metric.py. To compute a metric, you must provide it data from your RAG application. The table below shows a few of the many metrics we offer with Tonic Validate. For more detail explanations of our metrics refer to our documentation.
Metric Name | Inputs | Score Range | What does it measure? |
---|---|---|---|
Answer similarity score |
Question Reference answer LLM answer
|
0 to 5 | How well the reference answer matches the LLM answer. |
Retrieval precision |
Question Retrieved Context
|
0 to 1 | Whether the context retrieved is relevant to answer the given question. |
Augmentation precision |
Question Retrieved Context LLM answer
|
0 to 1 | Whether the relevant context is in the LLM answer. |
Augmentation accuracy |
Retrieved Context LLM answer
|
0 to 1 | Whether all the context is in the LLM answer. |
Answer consistency |
Retrieved Context LLM answer
|
0 to 1 | Whether there is information in the LLM answer that does not come from the context. |
Latency | Run Time |
0 or 1 | Measures how long it takes for the LLM to complete a request. |
Contains Text | LLM Answer |
0 or 1 | Checks whether or not response contains the given text. |
Metric inputs in Tonic Validate are used to provide the metrics with the information they need to calculate performance. Below, we explain each input type and how to pass them into Tonic Validate's SDK.
What is it: The question asked
How to use: You can provide the questions by passing them into the Benchmark
via the questions
argument.
from tonic_validate import Benchmark
benchmark = Benchmark(
questions=["What is the capital of France?", "What is the capital of Germany?"]
)
What is it: A prewritten answer that serves as the ground truth for how the RAG application should answer the question.
How to use: You can provide the reference answers by passing it into the Benchmark
via the answers
argument. Each reference answer must correspond to a given question. So if the reference answer is for the third question in the questions
list, then the reference answer must also be the third item in the answers
list. The only metric that requires a reference answer is the Answer Similarity Score
from tonic_validate import Benchmark
benchmark = Benchmark(
questions=["What is the capital of France?", "What is the capital of Germany?"]
answers=["Paris", "Berlin"]
)
What is it: The answer the RAG application / LLM gives to the question.
How to use: You can provide the LLM answer via the callback you provide to the Validate scorer. The answer is the first item in the tuple response.
# Function to simulate getting a response and context from your LLM
# Replace this with your actual function call
def get_rag_response(question):
return {
"llm_answer": "Paris",
"llm_context_list": ["Paris is the capital of France."]
}
# Score the responses
scorer = ValidateScorer()
run = scorer.score(benchmark, ask_rag)
If you are manually logging the answers without using the callback, then you can provide the LLM answer via llm_answer
when creating the LLMResponse
.
from tonic_validate import LLMResponse
# Save the responses into an array for scoring
responses = []
for item in benchmark:
# llm_answer is the answer that LLM gives
llm_response = LLMResponse(
llm_answer="Paris",
benchmark_item=item
)
responses.append(llm_response)
# Score the responses
scorer = ValidateScorer()
run = scorer.score_responses(responses)
What is it: The context that your RAG application retrieves when answering a given question. This context is what's put in the prompt by the RAG application to help the LLM answer the question.
How to use: You can provide the LLM's retrieved context list via the callback you provide to the Validate scorer. The answer is the second item in the tuple response. The retrieved context is always a list
# Function to simulate getting a response and context from your LLM
# Replace this with your actual function call
def get_rag_response(question):
return {
"llm_answer": "Paris",
"llm_context_list": ["Paris is the capital of France."]
}
# Score the responses
scorer = ValidateScorer()
run = scorer.score(benchmark, ask_rag)
If you are manually logging the answers, then you can provide the LLM context via llm_context_list
when creating the LLMResponse
.
from tonic_validate import LLMResponse
# Save the responses into an array for scoring
responses = []
for item in benchmark:
# llm_answer is the answer that LLM gives
# llm_context_list is a list of the context that the LLM used to answer the question
llm_response = LLMResponse(
llm_answer="Paris",
llm_context_list=["Paris is the capital of France."],
benchmark_item=item
)
responses.append(llm_response)
# Score the responses
scorer = ValidateScorer()
run = scorer.score_responses(responses)
What is it: Used for the latency metric to measure how long it took the LLM to respond.
How to use: If you are using the Validate scorer callback, then this metric is automatically calculated for you. If you are manually creating the LLM responses, then you need to provide how long the LLM took yourself via the run_time
argument.
from tonic_validate import LLMResponse
# Save the responses into an array for scoring
responses = []
for item in benchmark:
run_time = # Float representing how many seconds the LLM took to respond
# llm_answer is the answer that LLM gives
# llm_context_list is a list of the context that the LLM used to answer the question
llm_response = LLMResponse(
llm_answer="Paris",
llm_context_list=["Paris is the capital of France."],
benchmark_item=item
run_time=run_time
)
responses.append(llm_response)
# Score the responses
scorer = ValidateScorer()
run = scorer.score_responses(responses)
Most metrics are scored with the assistance of a LLM. Validate supports OpenAI and Azure OpenAI but other LLMs can easily be integrated (just file an github issue against this repository).
In order to use OpenAI you must provide an OpenAI API Key.
import os
os.environ["OPENAI_API_KEY"] = "put-your-openai-api-key-here"
If you already have the OPENAI_API_KEY
set in your system's environment variables then you can skip this step. Otherwise, please set the environment variable before proceeding.
If you are using Azure, instead of setting the OPENAI_API_KEY
environment variable, you instead need to set AZURE_OPENAI_API_KEY
and AZURE_OPENAI_ENDPOINT
. AZURE_OPENAI_ENDPOINT
is the endpoint url for your Azure OpenAI deployment and AZURE_OPENAI_API_KEY
is your API key.
import os
os.environ["AZURE_OPENAI_API_KEY"] = "put-your-azure-openai-api-key-here"
os.environ["AZURE_OPENAI_ENDPOINT"] = "put-your-azure-endpoint-here"
If you already have the GEMINI_API_KEY
set in your system's environment variables then you can skip this step. Otherwise, please set the environment variable before proceeding.
import os
os.environ["GEMINI_API_KEY"] = "put-your-gemini-api-key-here"
Note that to use gemini, your Python version must be 3.9 or higher.
If you already have the ANTHROPIC_API_KEY
set in your system's environment variables then you can skip this step. Otherwise, please set the environment variable before proceeding.
import os
os.environ["ANTHROPIC_API_KEY"] = "put-your-anthropic-api-key-here"
If you already have the MISTRAL_API_KEY
set in your system's environment variables then you can skip this step. Otherwise, please set the environment variable before proceeding.
import os
os.environ["MISTRAL_API_KEY"] = "put-your-mistral-api-key-here"
If you already have the COHERE_API_KEY
set in your system's environment variables then you can skip this step. Otherwise, please set the environment variable before proceeding.
import os
os.environ["COHERE_API_KEY"] = "put-your-cohere-api-key-here"
If you already have the TOGETHERAI_API_KEY
set in your system's environment variables then you can skip this step. Otherwise, please set the environment variable before proceeding.
import os
os.environ["TOGETHERAI_API_KEY"] = "put-your-togetherai-api-key-here"
If you already have the "AWS_SECRET_ACCESS_KEY
, AWS_ACCESS_KEY_ID
, and AWS_REGION_NAME
set in your system's environment variables then you can skip this step. Otherwise, please set the environment variables before proceeding.
import os
os.environ["AWS_ACCESS_KEY_ID"]="put-your-aws-access-key-id-here"
os.environ["AWS_SECRET_ACCESS_KEY"]="put-your-aws-secret-access-key-here"
os.environ["AWS_REGION_NAME"]="put-your-aws-region-name-here"
To use metrics, instantiate an instance of ValidateScorer.
from tonic_validate import ValidateScorer
scorer = ValidateScorer()
The default model used for scoring metrics is GPT 4 Turbo. To change the OpenAI model, pass the OpenAI model name into the model_evaluator
argument for ValidateScorer
. You can also pass in custom metrics via an array of metrics.
from tonic_validate import ValidateScorer
from tonic_validate.metrics import AnswerConsistencyMetric, AnswerSimilarityMetric
scorer = ValidateScorer([
AnswerConsistencyMetric(),
AugmentationAccuracyMetric()
], model_evaluator="gpt-3.5-turbo")
You can also pass in other models like Google Gemini, Claude, Mistral, Cohere, Together AI, and AWS Bedrock by setting the model_evaluator
argument to the model name like so
scorer = ValidateScorer(model_evaluator="gemini/gemini-1.5-pro-latest")
scorer = ValidateScorer(model_evaluator="claude-3")
scorer = ValidateScorer(model_evaluator="mistral/mistral-tiny")
scorer = ValidateScorer(model_evaluator="command-r")
scorer = ValidateScorer(model_evaluator="together_ai/togethercomputer/Llama-2-7B-32K-Instruct")
scorer = ValidateScorer(model_evaluator="bedrock/your-bedrock-model")
If an error occurs while scoring an item's metric, the score for that metric will be set to None
. If you instead wish to have Tonic Validate throw an exception when there's an error scoring, then set fail_on_error
to True
in the constructor
scorer = ValidateScorer(fail_on_error=True)
If you are using Azure, you MUST set the model_evaluator
argument to your deployment name like so
scorer = ValidateScorer(model_evaluator="your-deployment-name")
If you are using AWS Bedrock, you MUST set the model_evaluator
argument to your endpoint name and the model_id
argument to your model name like so
scorer = ValidateScorer(model_evaluator="your-endpoint-name", model_id="your-model-name")
After you instantiate the ValidateScorer
with your desired metrics, you can then score the metrics using the callback you defined earlier.
from tonic_validate import ValidateScorer, ValidateApi
# Function to simulate getting a response and context from your LLM
# Replace this with your actual function call
def get_rag_response(question):
return {
"llm_answer": "Paris",
"llm_context_list": ["Paris is the capital of France."]
}
# Score the responses
scorer = ValidateScorer()
run = scorer.score(benchmark, ask_rag)
If you don't want to use the callback, you can instead log your answers manually by iterating over the benchmark and then score the answers.
from tonic_validate import ValidateScorer, LLMResponse
# Save the responses into an array for scoring
responses = []
for item in benchmark:
llm_response = LLMResponse(
llm_answer="Paris",
llm_context_list=["Paris is the capital of France"],
benchmark_item=item
)
responses.append(llm_response)
# Score the responses
scorer = ValidateScorer()
run = scorer.score_responses(responses)
There are two ways to view the results of a run.
You can manually print out the results via python like so
print("Overall Scores")
print(run.overall_scores)
print("------")
for item in run.run_data:
print("Question: ", item.reference_question)
print("Answer: ", item.reference_answer)
print("LLM Answer: ", item.llm_answer)
print("LLM Context: ", item.llm_context)
print("Scores: ", item.scores)
print("------")
which outputs the following
Overall Scores
{'answer_consistency': 1.0, 'augmentation_accuracy': 1.0}
------
Question: What is the capital of France?
Answer: Paris
LLM Answer: Paris
LLM Context: ['Paris is the capital of France.']
Scores: {'answer_consistency': 1.0, 'augmentation_accuracy': 1.0}
------
Question: What is the capital of Spain?
Answer: Madrid
LLM Answer: Paris
LLM Context: ['Paris is the capital of France.']
Scores: {'answer_consistency': 1.0, 'augmentation_accuracy': 1.0}
------
You can easily view your run results by uploading them to our free to use UI. The main advantage of this method is the Tonic Validate UI provides graphing for your results along with additional visualization features. To sign up for the UI, go to here.
Once you sign up for the UI, you will go through an onboarding to create an API Key and Project.
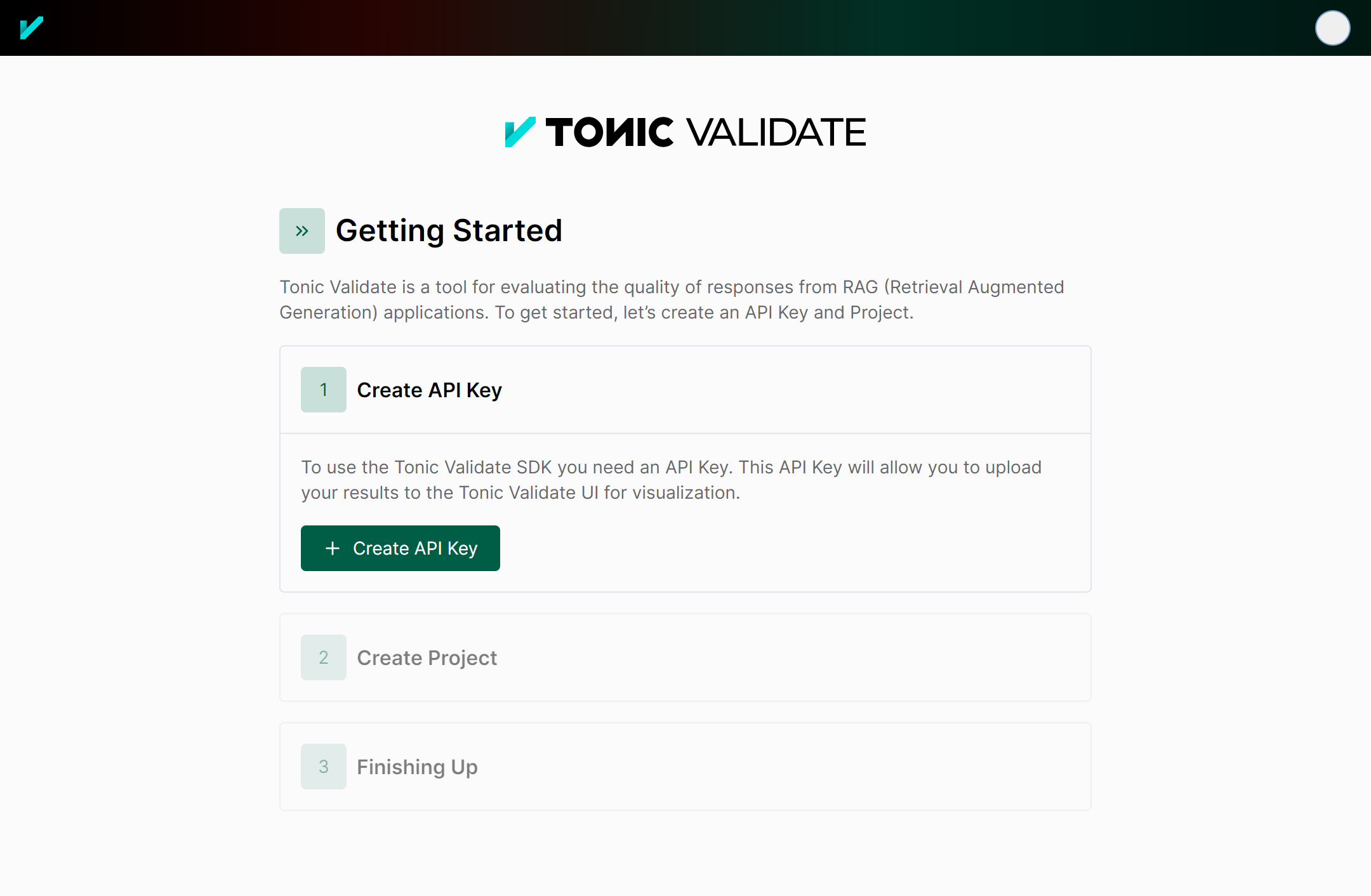
Copy both the API Key and Project ID from the onboarding and insert it into the following code
from tonic_validate import ValidateApi
validate_api = ValidateApi("your-api-key")
validate_api.upload_run("your-project-id", run)
This will upload your run to the Tonic Validate UI where you can view the results. On the home page (as seen below) you can view the change in scores across runs over time.
You can also view the results of an individual run in the UI as well.
Tonic Validate collects minimal telemetry to help us figure out what users want and how they're using the product. We do not use any existing telemetry framework and instead created our own privacy focused setup. Only the following information is tracked
- What metrics were used for a run
- Number of questions in a run
- Time taken for a run to be evaluated
- Number of questions in a benchmark
- SDK Version being used
We do NOT track things such as the contents of the questions / answers, your scores, or any other sensitive information. For detecting CI/CD, we only check for common environment variables in different CI/CD environments. We do not log the values of these environment variables.
We also generate a random UUID to help us figure out how many users are using the product. This UUID is linked to your Validate account only to help track who is using the SDK and UI at once and to get user counts. If you want to see how we implemented telemetry, you can do so in the tonic_validate/utils/telemetry.py
file
If you wish to opt out of telemetry, you only need to set the TONIC_VALIDATE_DO_NOT_TRACK
environment variable to True
.
We currently allow the family of chat completion models from Open AI, Google, Anthropic, and more. We are always looking to add more models to our evaluator. If you have a model you would like to see added, please file an issue against this repository.
We'd like to add more models as choices for the LLM evaluator without adding to the complexity of the package too much.
The default model used for scoring metrics is GPT 4 Turbo. To change the model, pass the model name into the model
argument for ValidateScorer
scorer = ValidateScorer([
AnswerConsistencyMetric(),
AugmentationAccuracyMetric()
], model_evaluator="gpt-3.5-turbo")
Contributions are what make the open source community such an amazing place to learn, inspire, and create. Any contributions you make are greatly appreciated.
If you have a suggestion that would make this better, please fork the repo and create a pull request. You can also simply open an issue with the tag "enhancement". Don't forget to give the project a star! Thanks again!
- Fork the Project
- Create your Feature Branch (
git checkout -b feature/AmazingFeature
) - Commit your Changes (
git commit -m 'Add some AmazingFeature'
) - Push to the Branch (
git push origin feature/AmazingFeature
) - Open a Pull Request
Distributed under the MIT License. See LICENSE.txt
for more information.
For Tasks:
Click tags to check more tools for each tasksFor Jobs:
Alternative AI tools for tonic_validate
Similar Open Source Tools
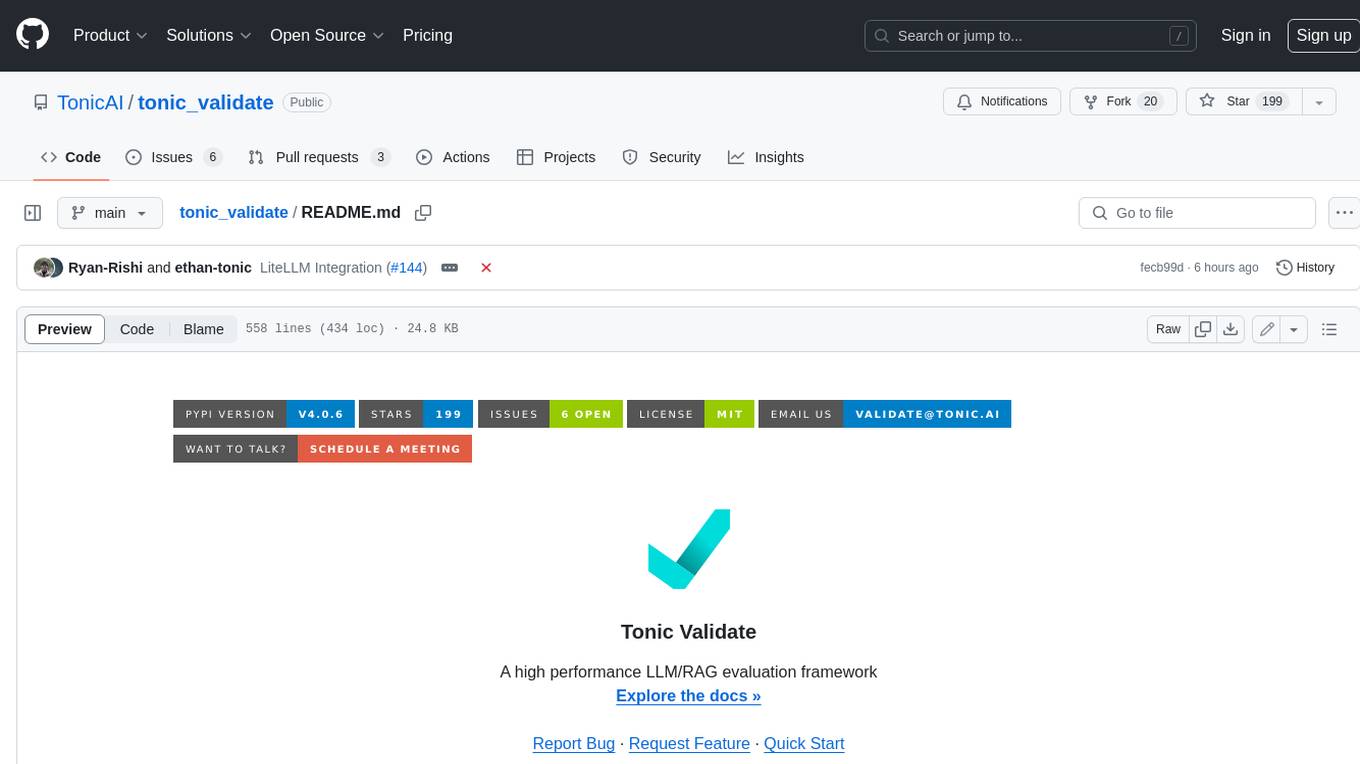
tonic_validate
Tonic Validate is a framework for the evaluation of LLM outputs, such as Retrieval Augmented Generation (RAG) pipelines. Validate makes it easy to evaluate, track, and monitor your LLM and RAG applications. Validate allows you to evaluate your LLM outputs through the use of our provided metrics which measure everything from answer correctness to LLM hallucination. Additionally, Validate has an optional UI to visualize your evaluation results for easy tracking and monitoring.
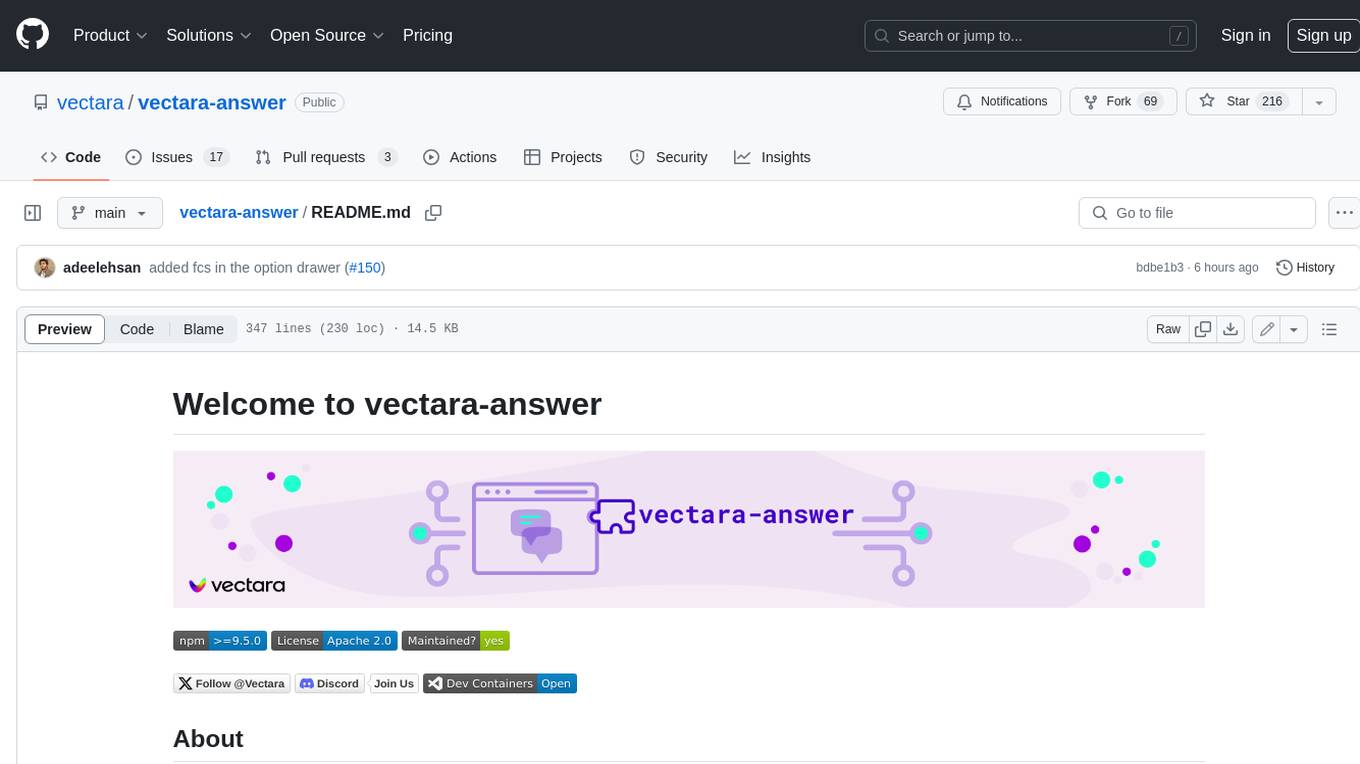
vectara-answer
Vectara Answer is a sample app for Vectara-powered Summarized Semantic Search (or question-answering) with advanced configuration options. For examples of what you can build with Vectara Answer, check out Ask News, LegalAid, or any of the other demo applications.
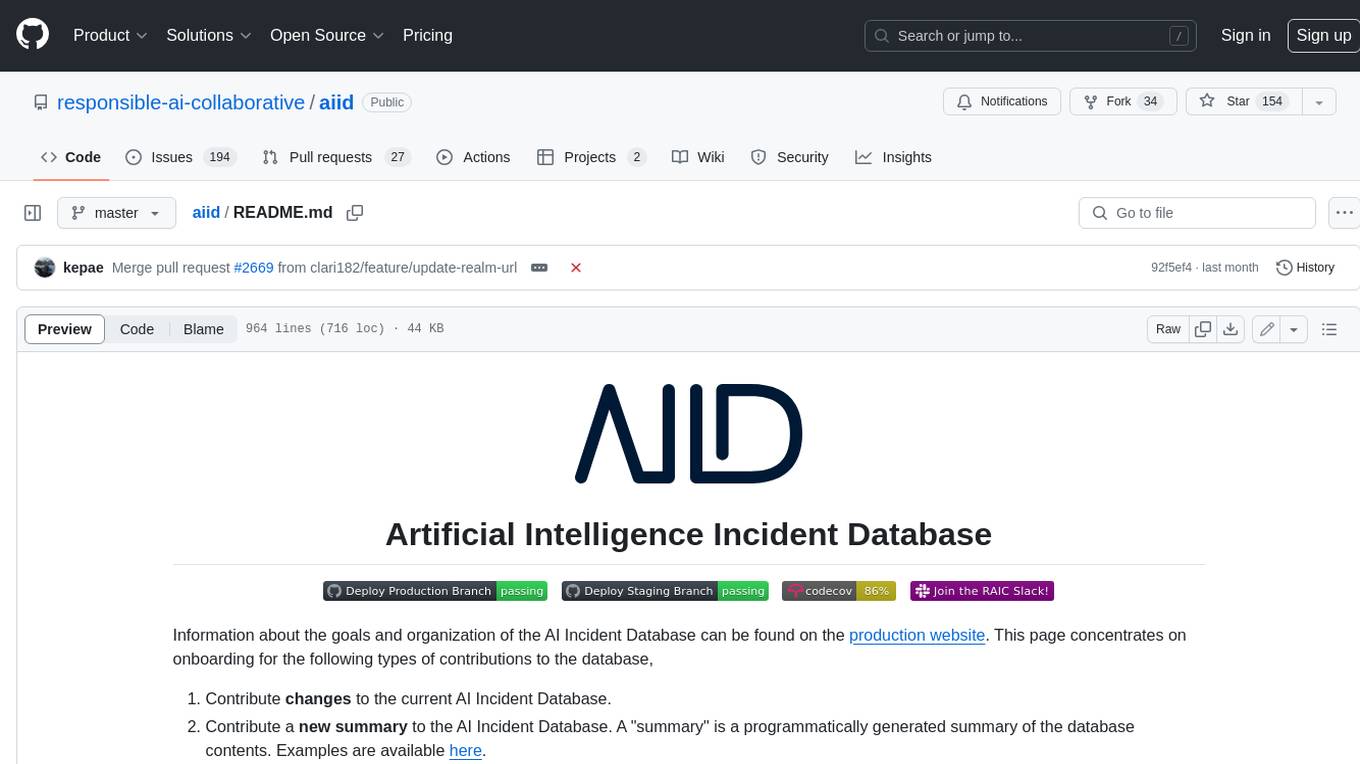
aiid
The Artificial Intelligence Incident Database (AIID) is a collection of incidents involving the development and use of artificial intelligence (AI). The database is designed to help researchers, policymakers, and the public understand the potential risks and benefits of AI, and to inform the development of policies and practices to mitigate the risks and promote the benefits of AI. The AIID is a collaborative project involving researchers from the University of California, Berkeley, the University of Washington, and the University of Toronto.
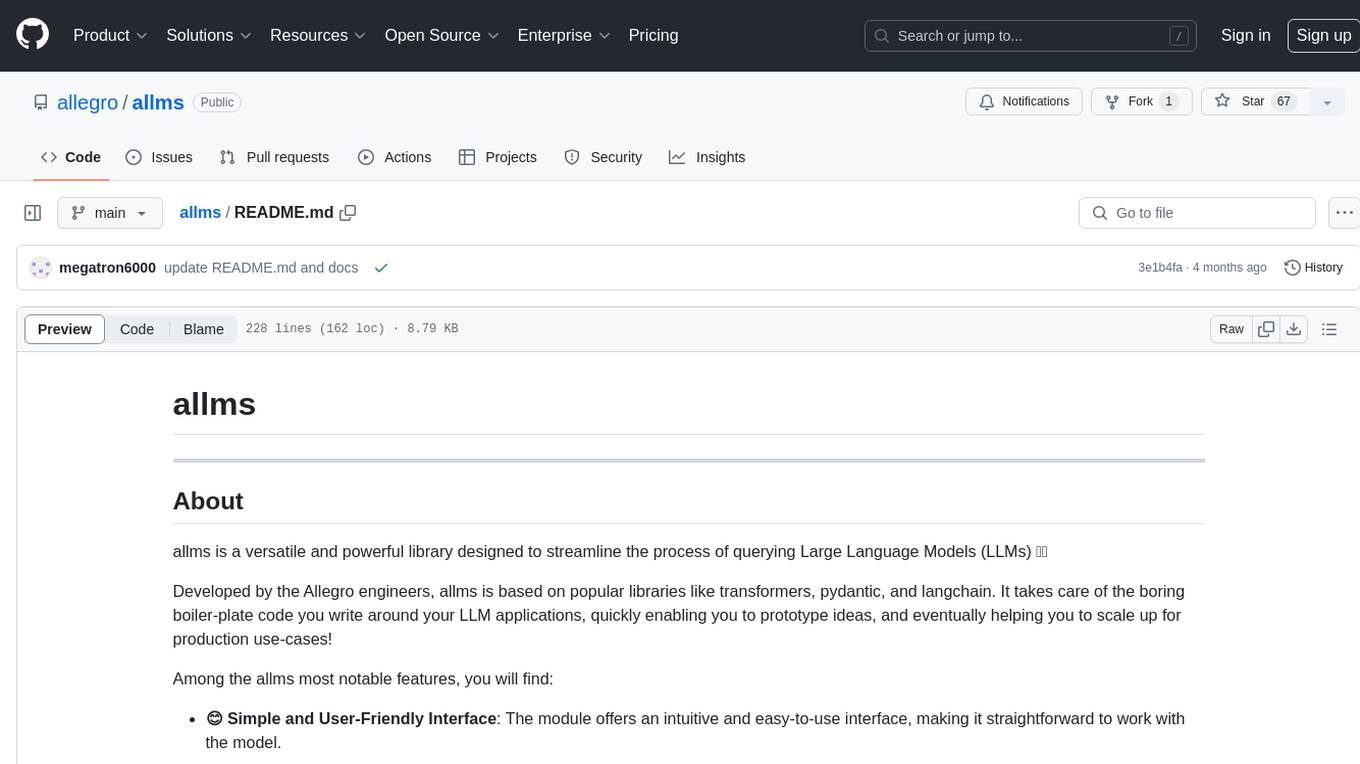
allms
allms is a versatile and powerful library designed to streamline the process of querying Large Language Models (LLMs). Developed by Allegro engineers, it simplifies working with LLM applications by providing a user-friendly interface, asynchronous querying, automatic retrying mechanism, error handling, and output parsing. It supports various LLM families hosted on different platforms like OpenAI, Google, Azure, and GCP. The library offers features for configuring endpoint credentials, batch querying with symbolic variables, and forcing structured output format. It also provides documentation, quickstart guides, and instructions for local development, testing, updating documentation, and making new releases.
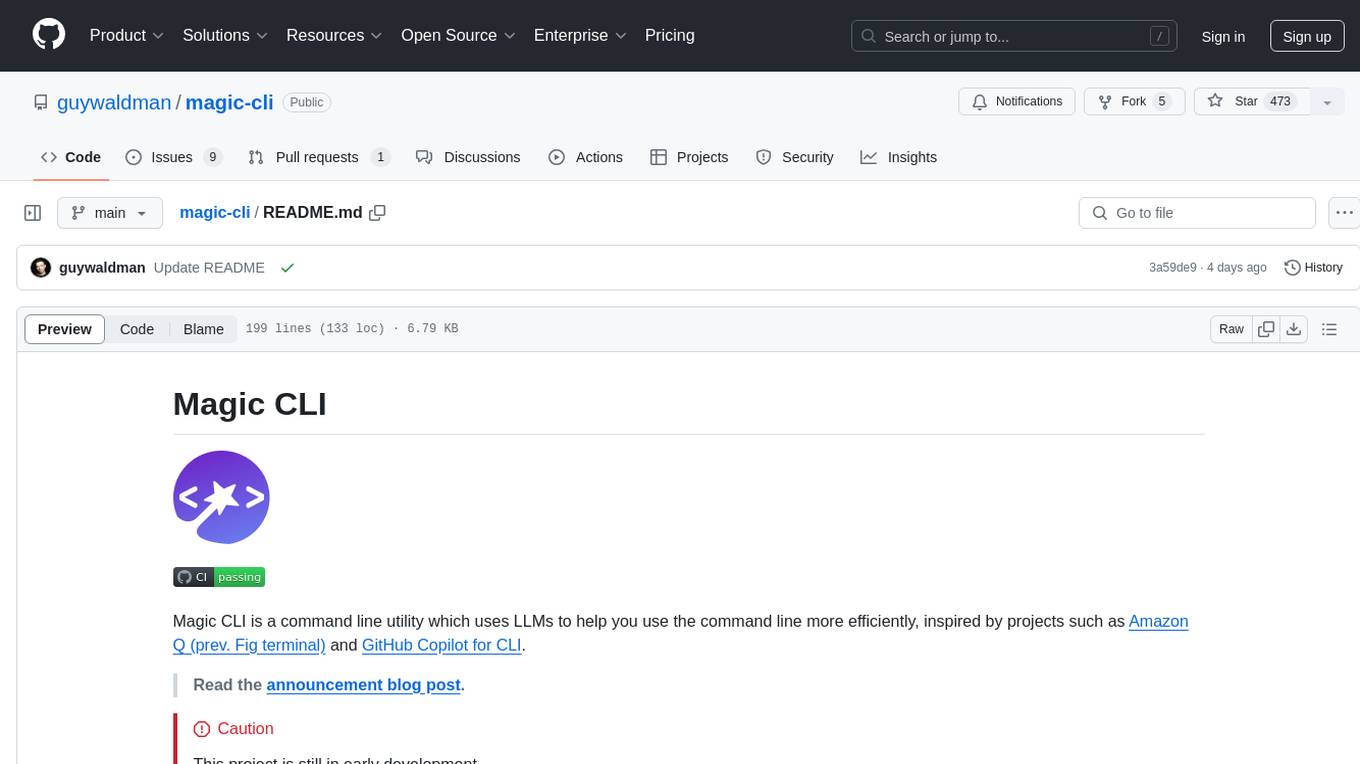
magic-cli
Magic CLI is a command line utility that leverages Large Language Models (LLMs) to enhance command line efficiency. It is inspired by projects like Amazon Q and GitHub Copilot for CLI. The tool allows users to suggest commands, search across command history, and generate commands for specific tasks using local or remote LLM providers. Magic CLI also provides configuration options for LLM selection and response generation. The project is still in early development, so users should expect breaking changes and bugs.
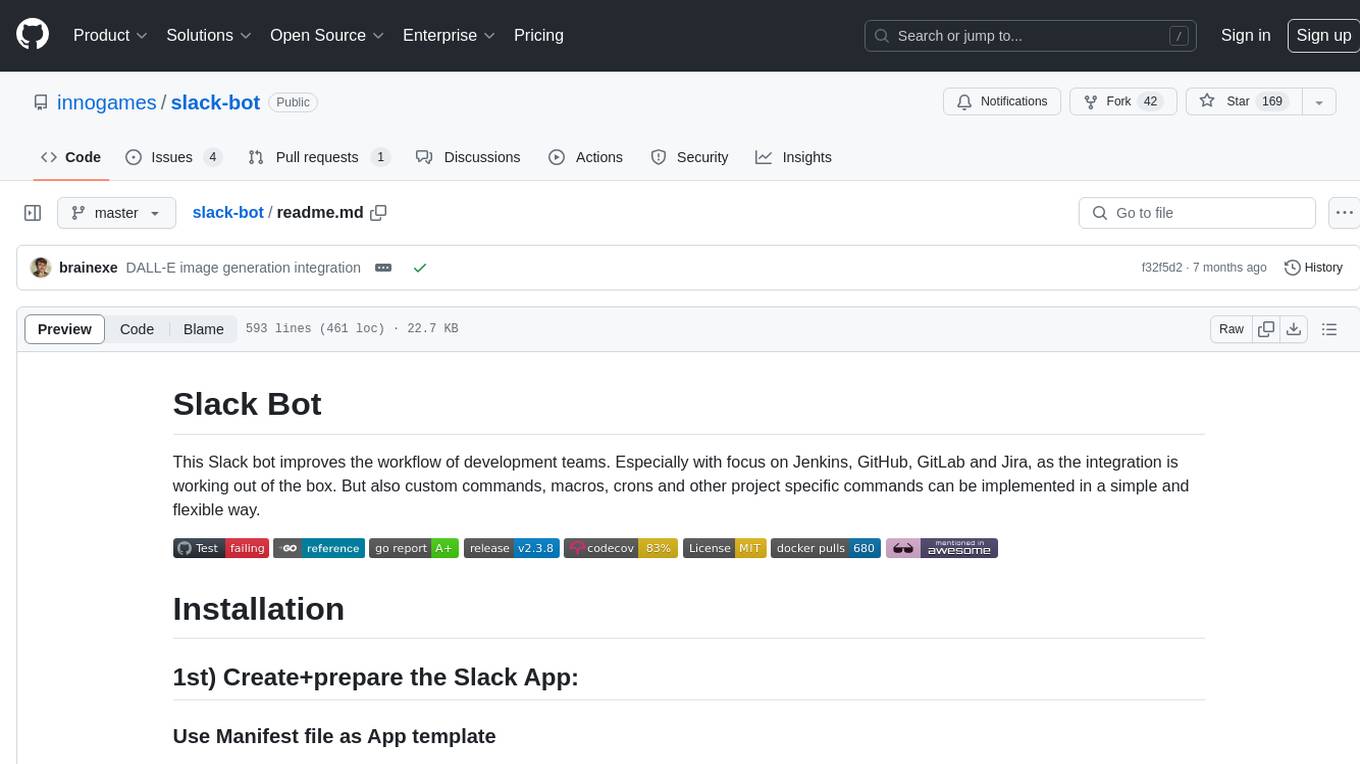
slack-bot
The Slack Bot is a tool designed to enhance the workflow of development teams by integrating with Jenkins, GitHub, GitLab, and Jira. It allows for custom commands, macros, crons, and project-specific commands to be implemented easily. Users can interact with the bot through Slack messages, execute commands, and monitor job progress. The bot supports features like starting and monitoring Jenkins jobs, tracking pull requests, querying Jira information, creating buttons for interactions, generating images with DALL-E, playing quiz games, checking weather, defining custom commands, and more. Configuration is managed via YAML files, allowing users to set up credentials for external services, define custom commands, schedule cron jobs, and configure VCS systems like Bitbucket for automated branch lookup in Jenkins triggers.
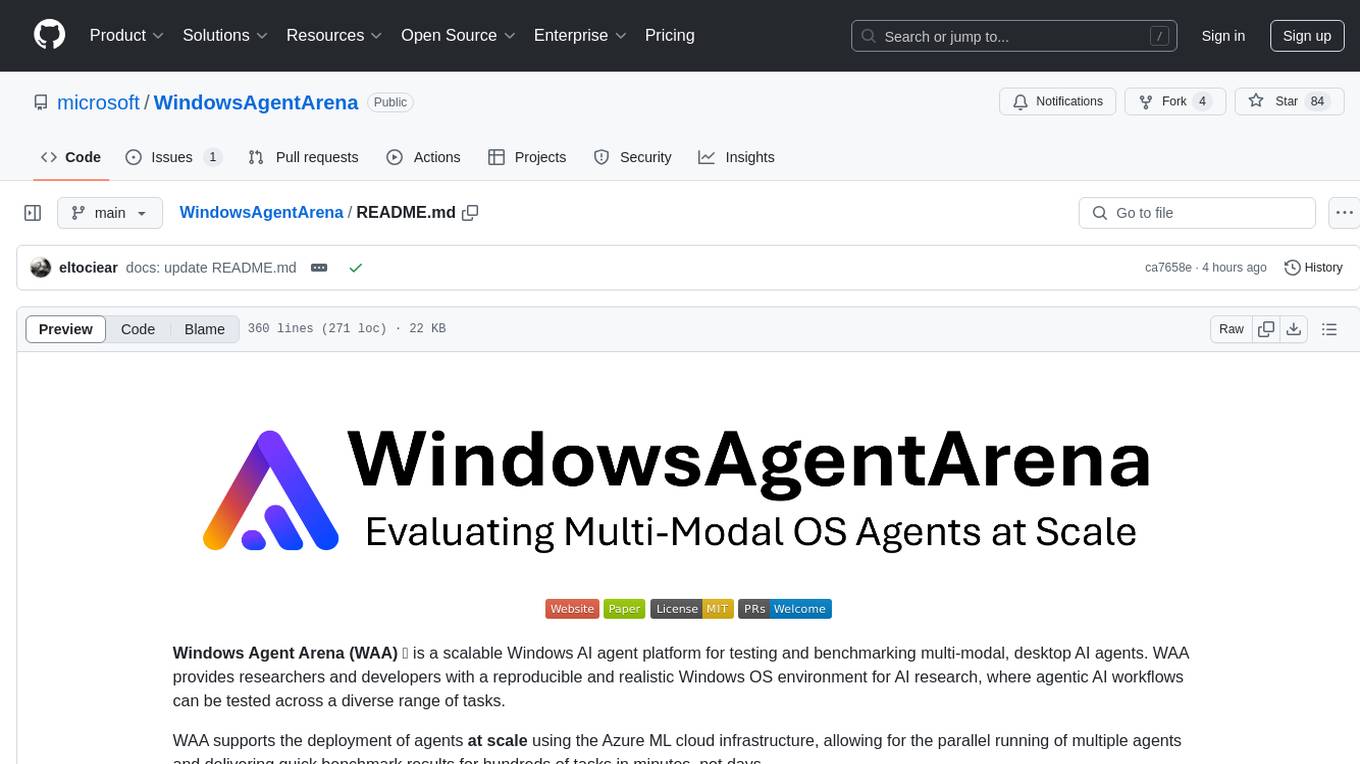
WindowsAgentArena
Windows Agent Arena (WAA) is a scalable Windows AI agent platform designed for testing and benchmarking multi-modal, desktop AI agents. It provides researchers and developers with a reproducible and realistic Windows OS environment for AI research, enabling testing of agentic AI workflows across various tasks. WAA supports deploying agents at scale using Azure ML cloud infrastructure, allowing parallel running of multiple agents and delivering quick benchmark results for hundreds of tasks in minutes.
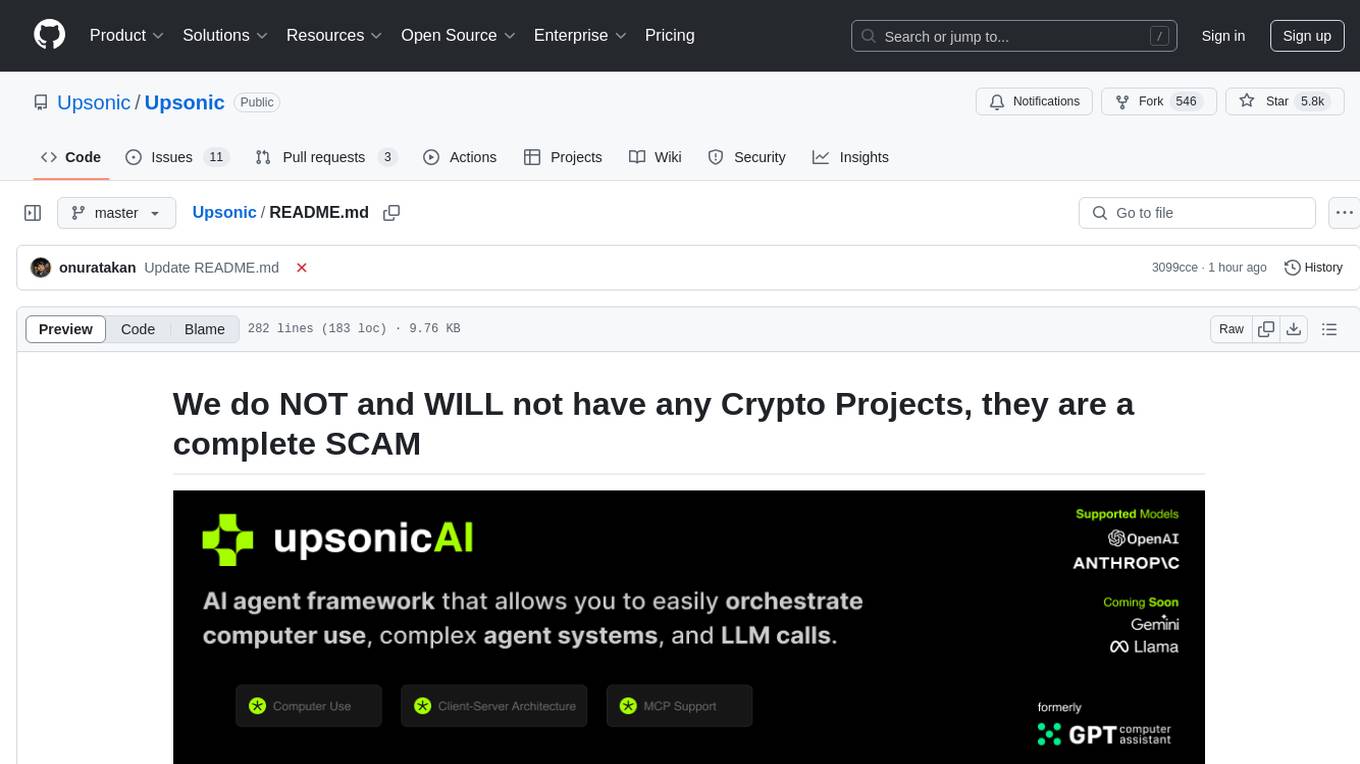
Upsonic
Upsonic offers a cutting-edge enterprise-ready framework for orchestrating LLM calls, agents, and computer use to complete tasks cost-effectively. It provides reliable systems, scalability, and a task-oriented structure for real-world cases. Key features include production-ready scalability, task-centric design, MCP server support, tool-calling server, computer use integration, and easy addition of custom tools. The framework supports client-server architecture and allows seamless deployment on AWS, GCP, or locally using Docker.
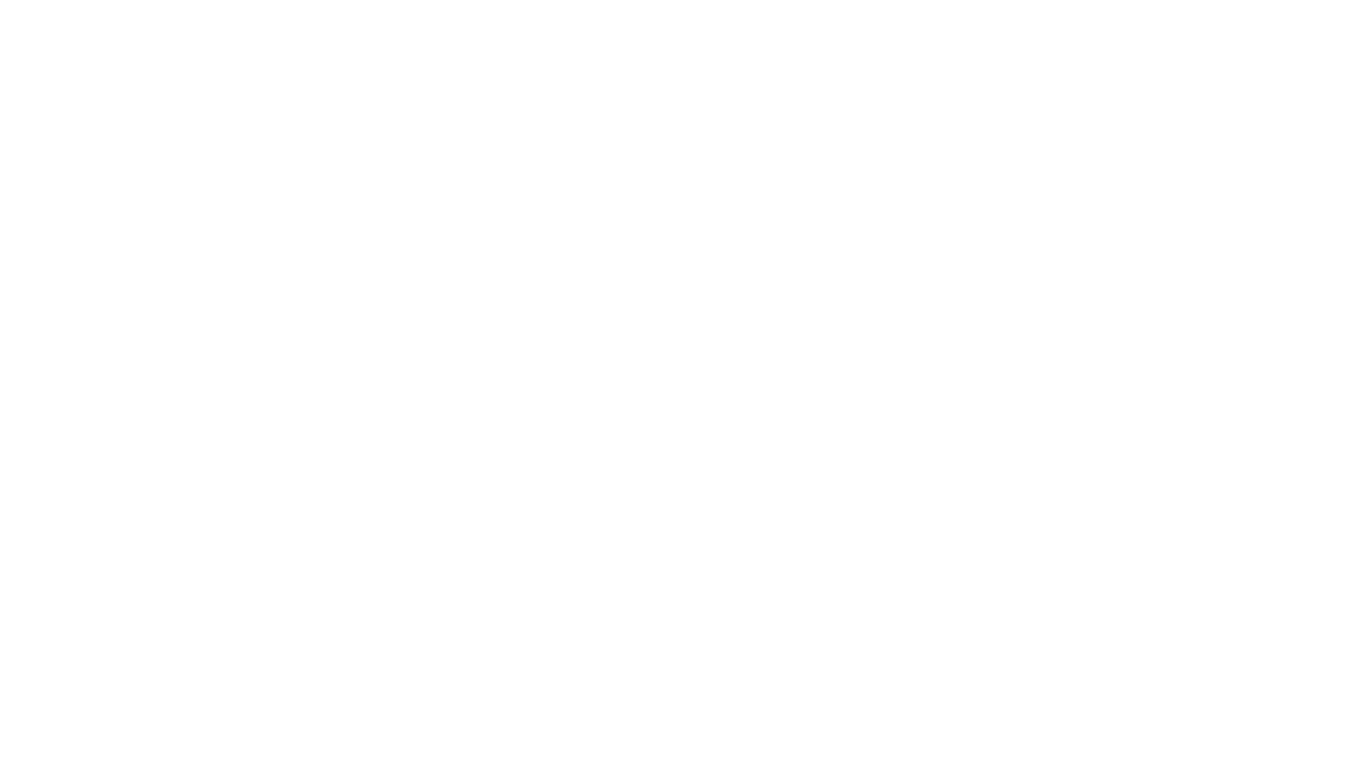
pytest-evals
pytest-evals is a minimalistic pytest plugin designed to help evaluate the performance of Language Model (LLM) outputs against test cases. It allows users to test and evaluate LLM prompts against multiple cases, track metrics, and integrate easily with pytest, Jupyter notebooks, and CI/CD pipelines. Users can scale up by running tests in parallel with pytest-xdist and asynchronously with pytest-asyncio. The tool focuses on simplifying evaluation processes without the need for complex frameworks, keeping tests and evaluations together, and emphasizing logic over infrastructure.
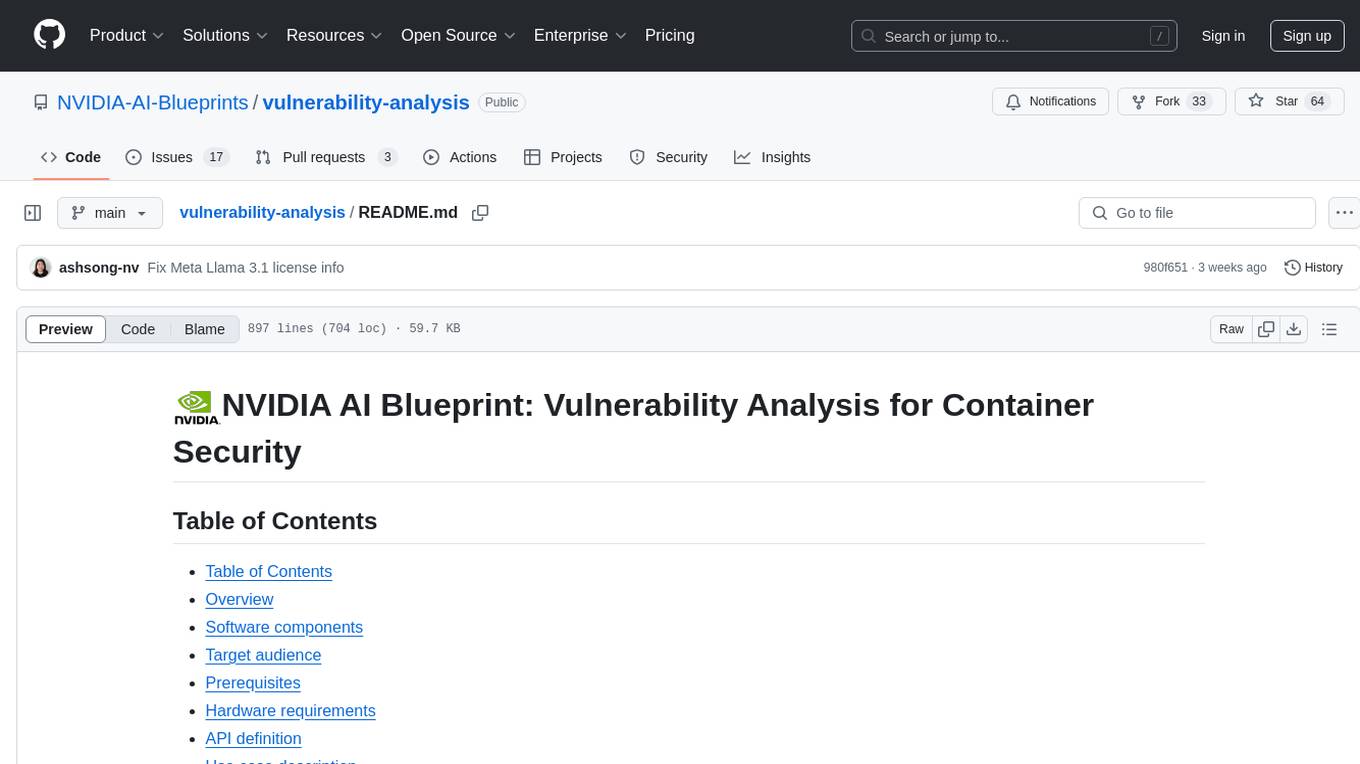
vulnerability-analysis
The NVIDIA AI Blueprint for Vulnerability Analysis for Container Security showcases accelerated analysis on common vulnerabilities and exposures (CVE) at an enterprise scale, reducing mitigation time from days to seconds. It enables security analysts to determine software package vulnerabilities using large language models (LLMs) and retrieval-augmented generation (RAG). The blueprint is designed for security analysts, IT engineers, and AI practitioners in cybersecurity. It requires NVAIE developer license and API keys for vulnerability databases, search engines, and LLM model services. Hardware requirements include L40 GPU for pipeline operation and optional LLM NIM and Embedding NIM. The workflow involves LLM pipeline for CVE impact analysis, utilizing LLM planner, agent, and summarization nodes. The blueprint uses NVIDIA NIM microservices and Morpheus Cybersecurity AI SDK for vulnerability analysis.
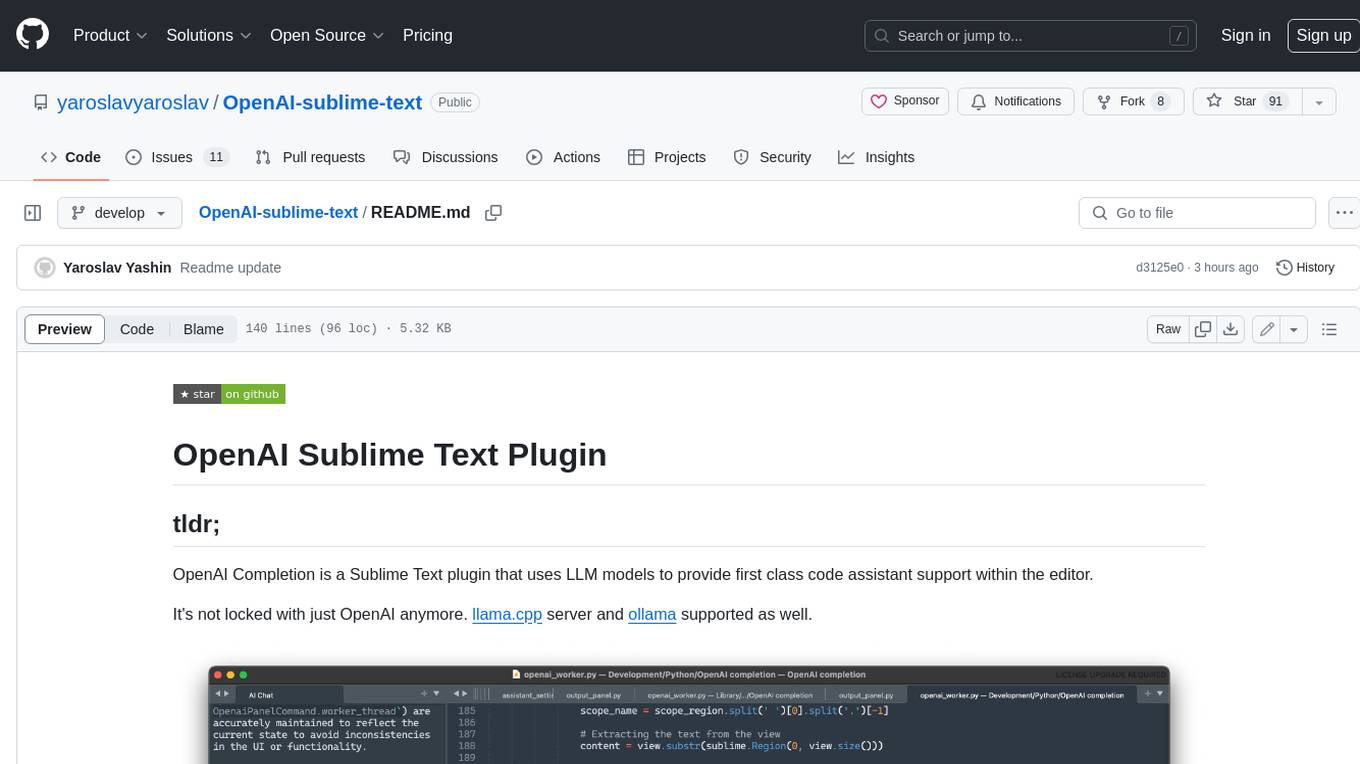
OpenAI-sublime-text
The OpenAI Completion plugin for Sublime Text provides first-class code assistant support within the editor. It utilizes LLM models to manipulate code, engage in chat mode, and perform various tasks. The plugin supports OpenAI, llama.cpp, and ollama models, allowing users to customize their AI assistant experience. It offers separated chat histories and assistant settings for different projects, enabling context-specific interactions. Additionally, the plugin supports Markdown syntax with code language syntax highlighting, server-side streaming for faster response times, and proxy support for secure connections. Users can configure the plugin's settings to set their OpenAI API key, adjust assistant modes, and manage chat history. Overall, the OpenAI Completion plugin enhances the Sublime Text editor with powerful AI capabilities, streamlining coding workflows and fostering collaboration with AI assistants.
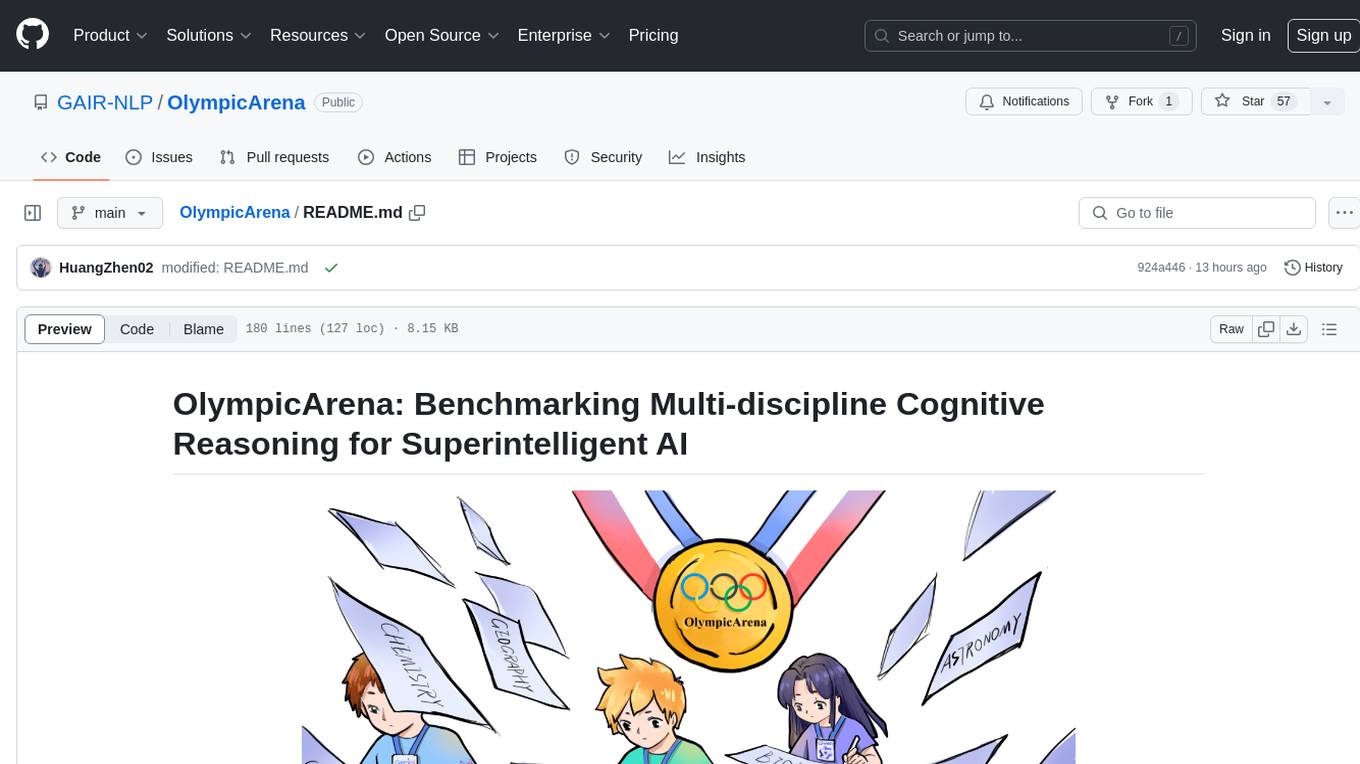
OlympicArena
OlympicArena is a comprehensive benchmark designed to evaluate advanced AI capabilities across various disciplines. It aims to push AI towards superintelligence by tackling complex challenges in science and beyond. The repository provides detailed data for different disciplines, allows users to run inference and evaluation locally, and offers a submission platform for testing models on the test set. Additionally, it includes an annotation interface and encourages users to cite their paper if they find the code or dataset helpful.
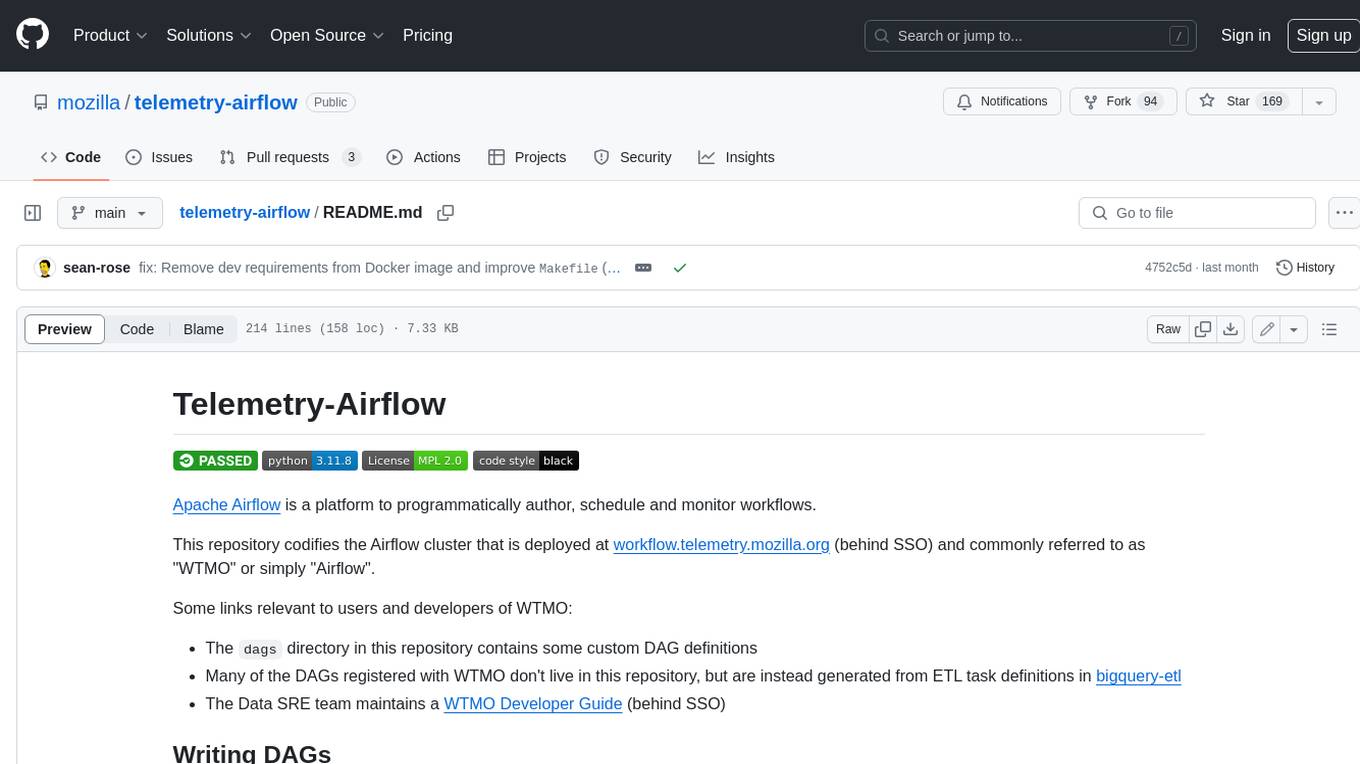
telemetry-airflow
This repository codifies the Airflow cluster that is deployed at workflow.telemetry.mozilla.org (behind SSO) and commonly referred to as "WTMO" or simply "Airflow". Some links relevant to users and developers of WTMO: * The `dags` directory in this repository contains some custom DAG definitions * Many of the DAGs registered with WTMO don't live in this repository, but are instead generated from ETL task definitions in bigquery-etl * The Data SRE team maintains a WTMO Developer Guide (behind SSO)
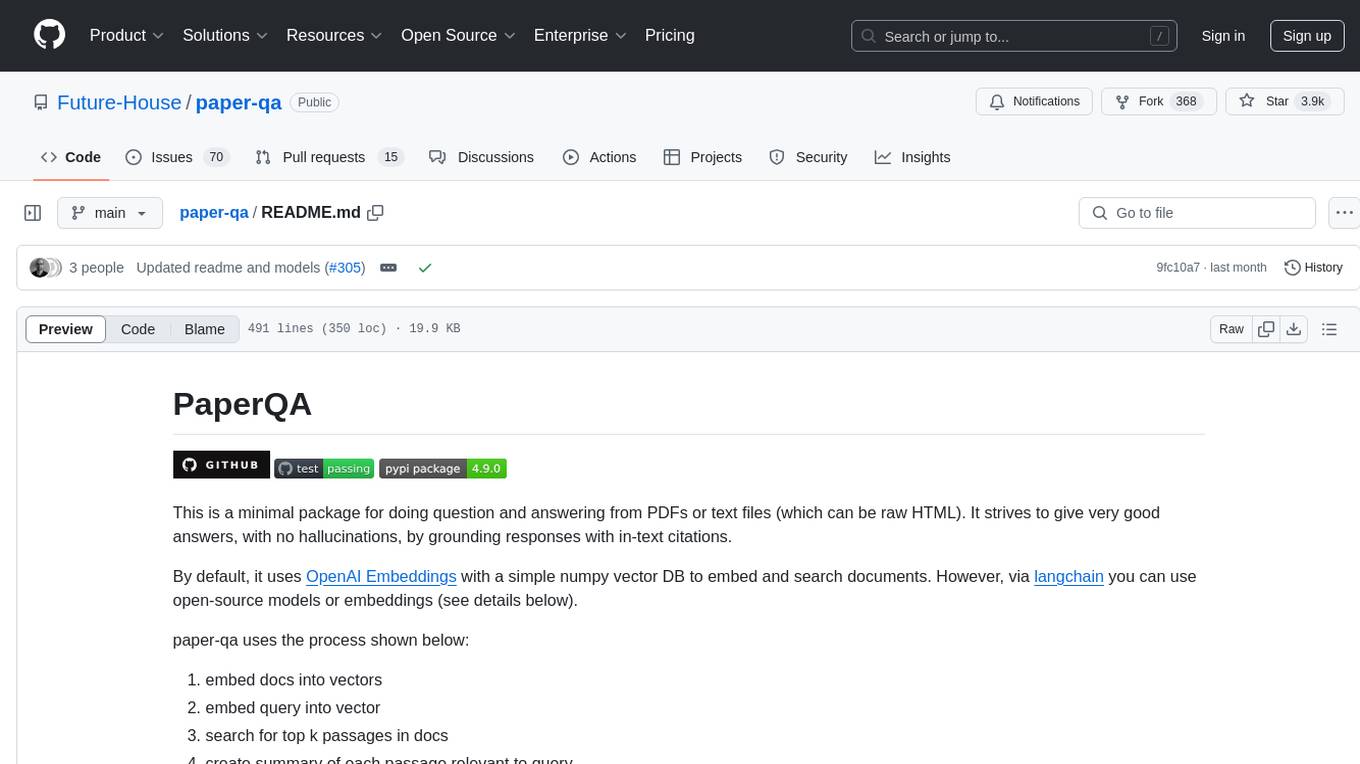
paper-qa
PaperQA is a minimal package for question and answering from PDFs or text files, providing very good answers with in-text citations. It uses OpenAI Embeddings to embed and search documents, and includes a process of embedding docs, queries, searching for top passages, creating summaries, using an LLM to re-score and select relevant summaries, putting summaries into prompt, and generating answers. The tool can be used to answer specific questions related to scientific research by leveraging citations and relevant passages from documents.
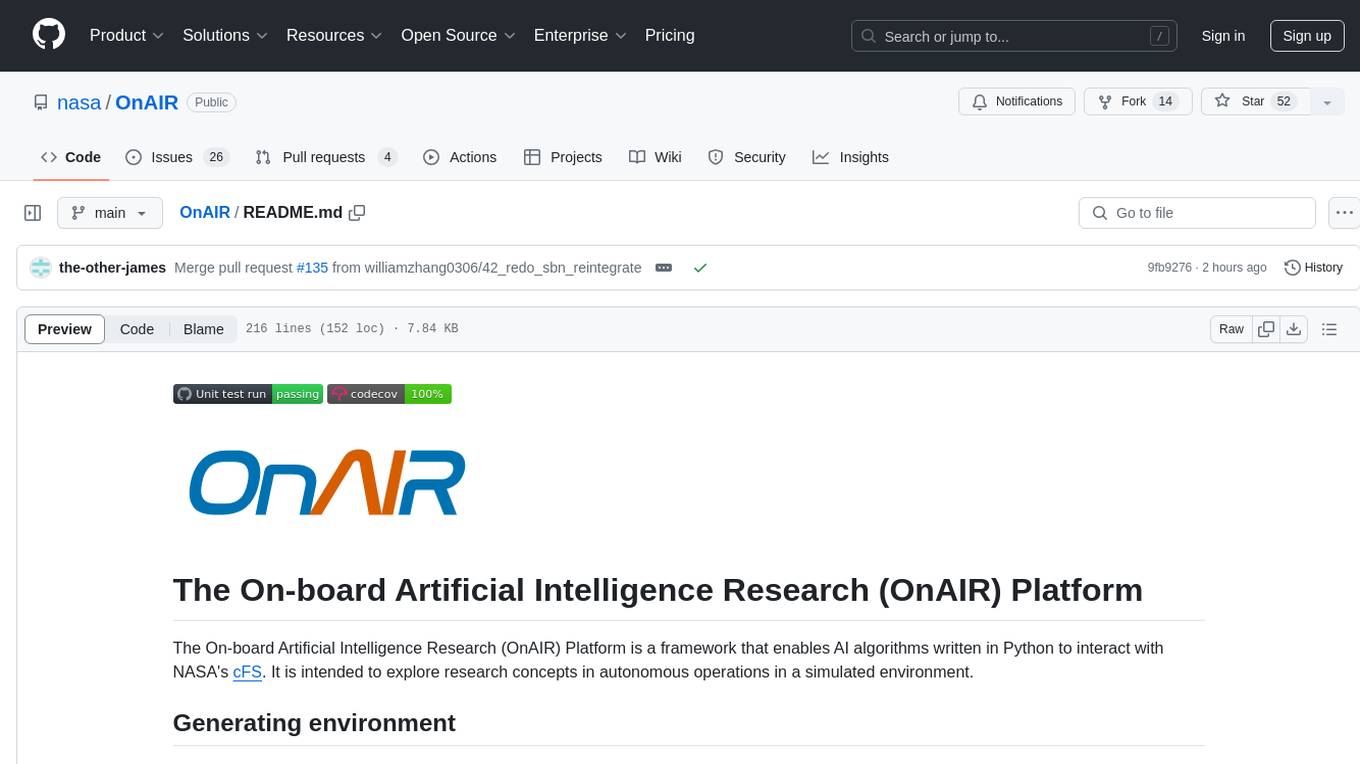
OnAIR
The On-board Artificial Intelligence Research (OnAIR) Platform is a framework that enables AI algorithms written in Python to interact with NASA's cFS. It is intended to explore research concepts in autonomous operations in a simulated environment. The platform provides tools for generating environments, handling telemetry data through Redis, running unit tests, and contributing to the repository. Users can set up a conda environment, configure telemetry and Redis examples, run simulations, and conduct unit tests to ensure the functionality of their AI algorithms. The platform also includes guidelines for licensing, copyright, and contributions to the repository.
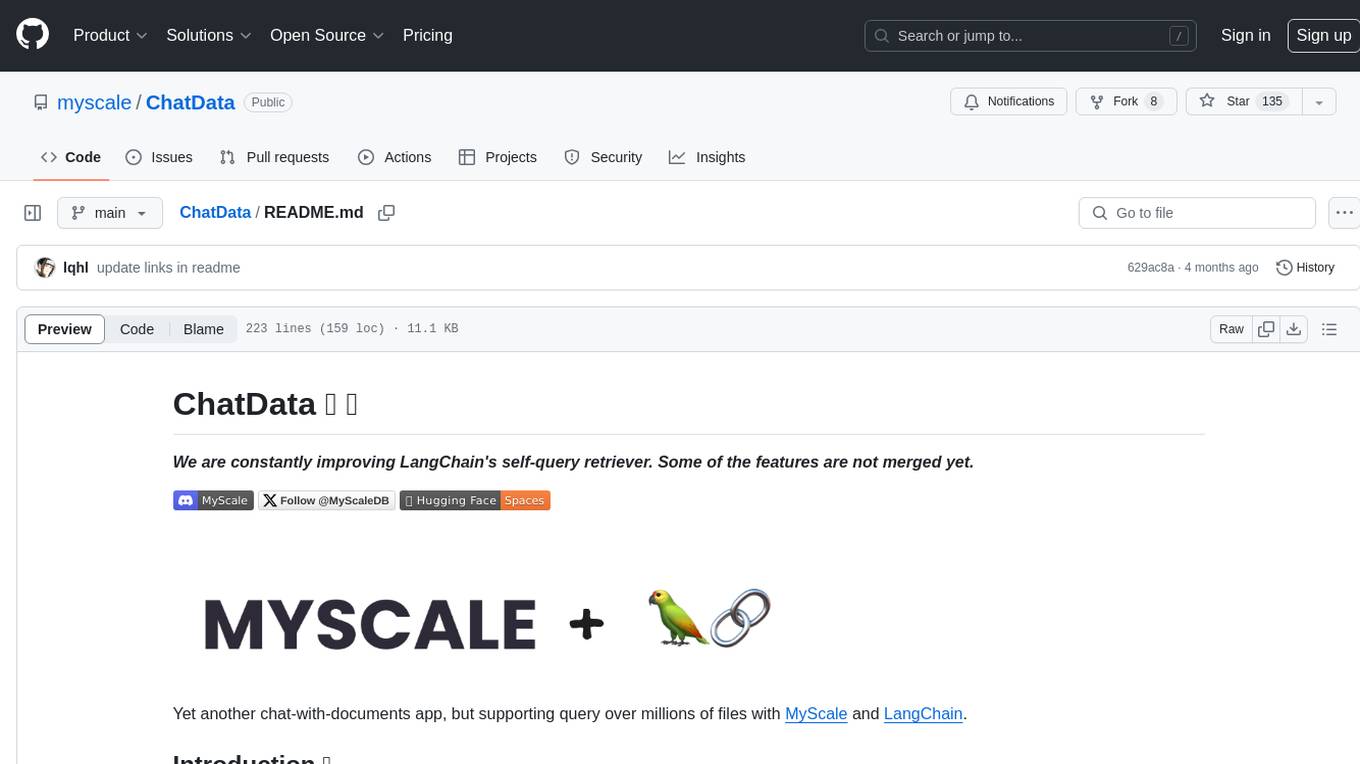
ChatData
ChatData is a robust chat-with-documents application designed to extract information and provide answers by querying the MyScale free knowledge base or uploaded documents. It leverages the Retrieval Augmented Generation (RAG) framework, millions of Wikipedia pages, and arXiv papers. Features include self-querying retriever, VectorSQL, session management, and building a personalized knowledge base. Users can effortlessly navigate vast data, explore academic papers, and research documents. ChatData empowers researchers, students, and knowledge enthusiasts to unlock the true potential of information retrieval.
For similar tasks
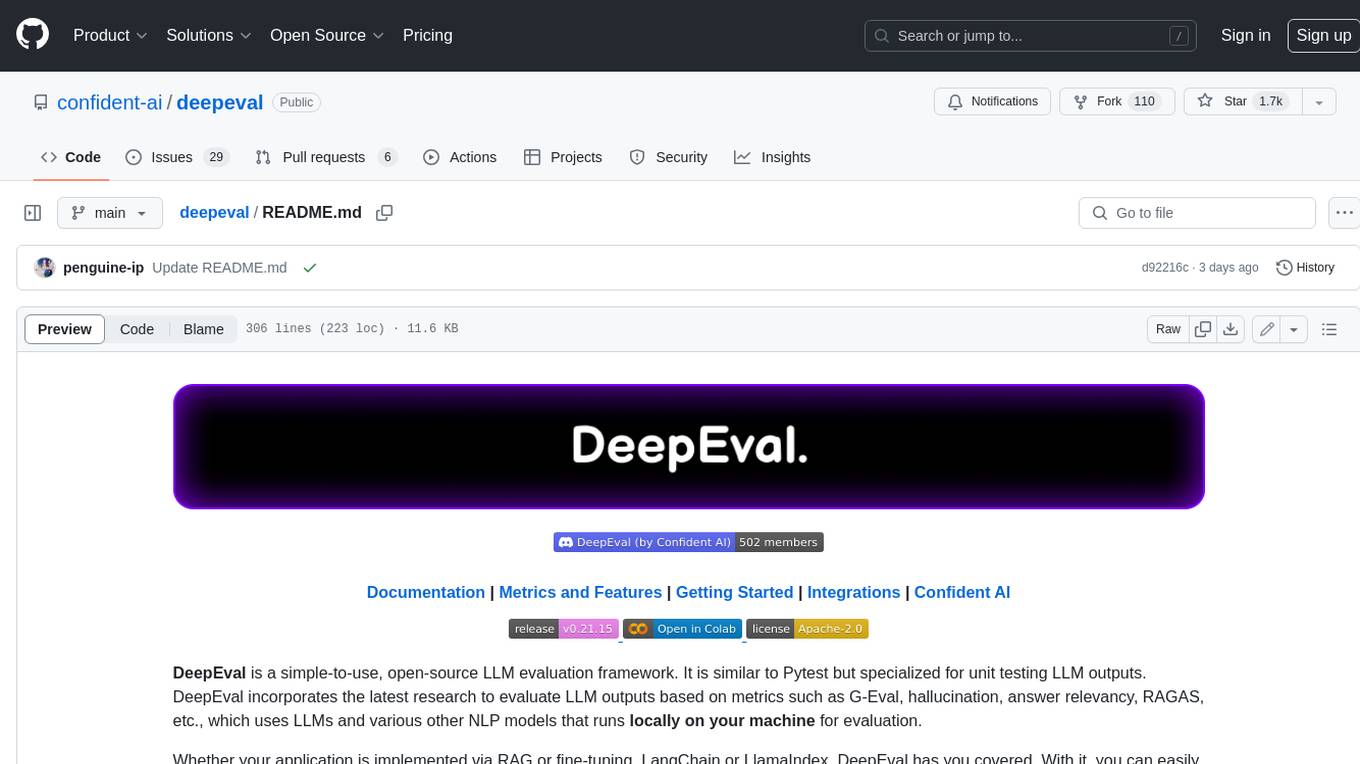
deepeval
DeepEval is a simple-to-use, open-source LLM evaluation framework specialized for unit testing LLM outputs. It incorporates various metrics such as G-Eval, hallucination, answer relevancy, RAGAS, etc., and runs locally on your machine for evaluation. It provides a wide range of ready-to-use evaluation metrics, allows for creating custom metrics, integrates with any CI/CD environment, and enables benchmarking LLMs on popular benchmarks. DeepEval is designed for evaluating RAG and fine-tuning applications, helping users optimize hyperparameters, prevent prompt drifting, and transition from OpenAI to hosting their own Llama2 with confidence.
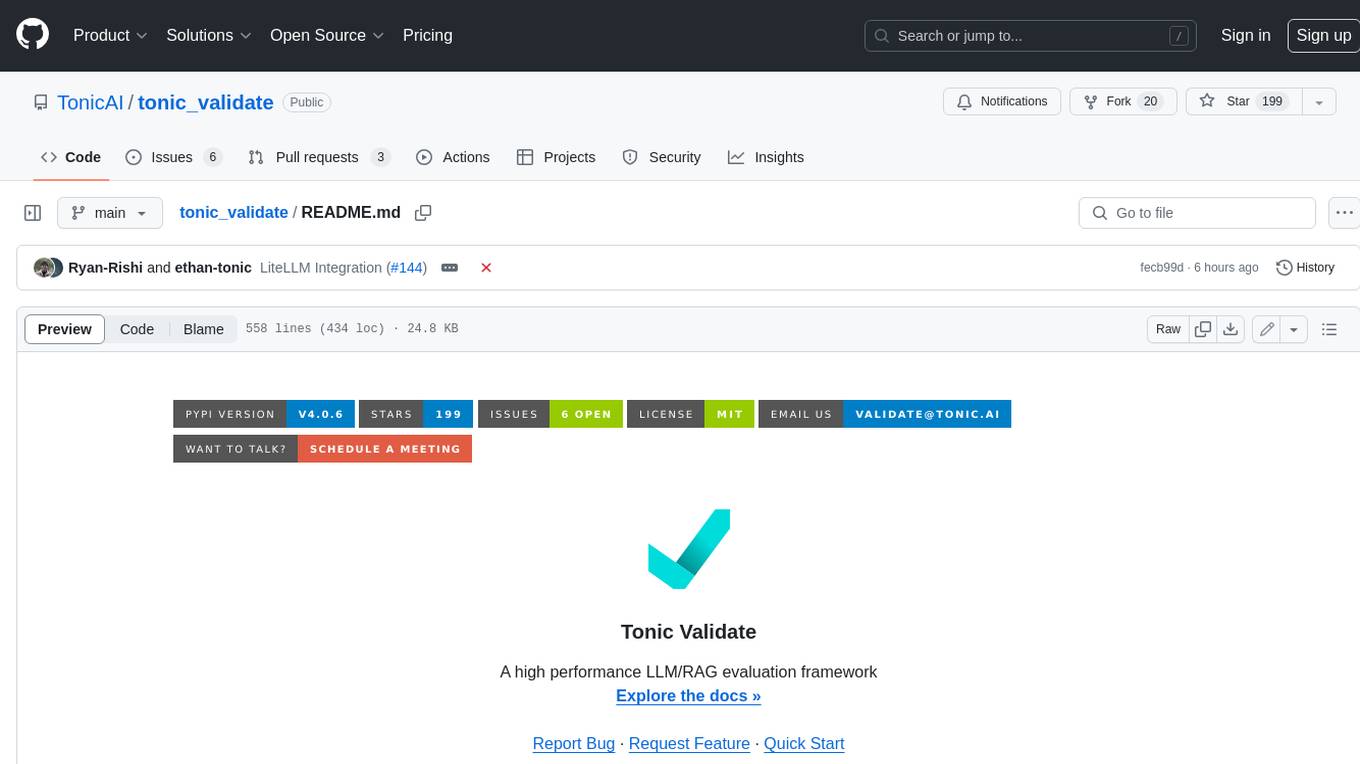
tonic_validate
Tonic Validate is a framework for the evaluation of LLM outputs, such as Retrieval Augmented Generation (RAG) pipelines. Validate makes it easy to evaluate, track, and monitor your LLM and RAG applications. Validate allows you to evaluate your LLM outputs through the use of our provided metrics which measure everything from answer correctness to LLM hallucination. Additionally, Validate has an optional UI to visualize your evaluation results for easy tracking and monitoring.
For similar jobs
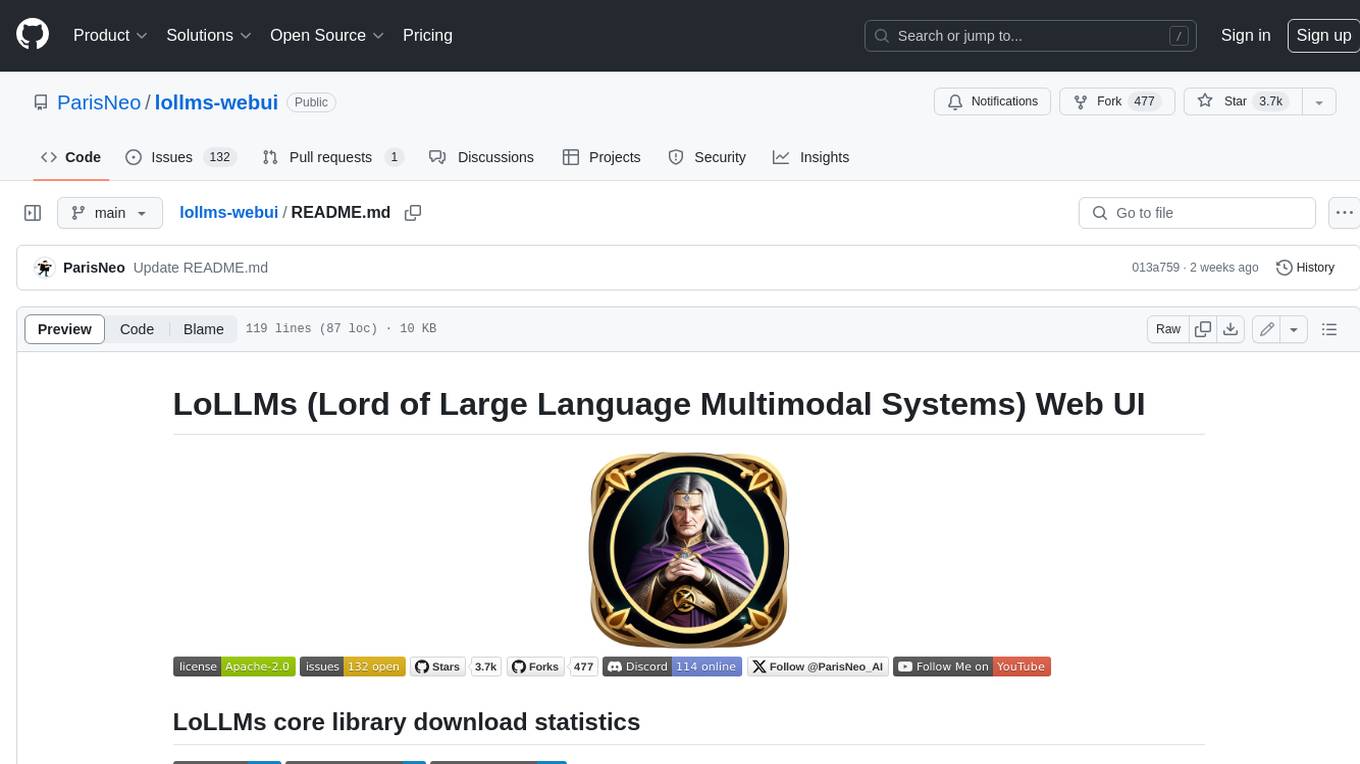
lollms-webui
LoLLMs WebUI (Lord of Large Language Multimodal Systems: One tool to rule them all) is a user-friendly interface to access and utilize various LLM (Large Language Models) and other AI models for a wide range of tasks. With over 500 AI expert conditionings across diverse domains and more than 2500 fine tuned models over multiple domains, LoLLMs WebUI provides an immediate resource for any problem, from car repair to coding assistance, legal matters, medical diagnosis, entertainment, and more. The easy-to-use UI with light and dark mode options, integration with GitHub repository, support for different personalities, and features like thumb up/down rating, copy, edit, and remove messages, local database storage, search, export, and delete multiple discussions, make LoLLMs WebUI a powerful and versatile tool.
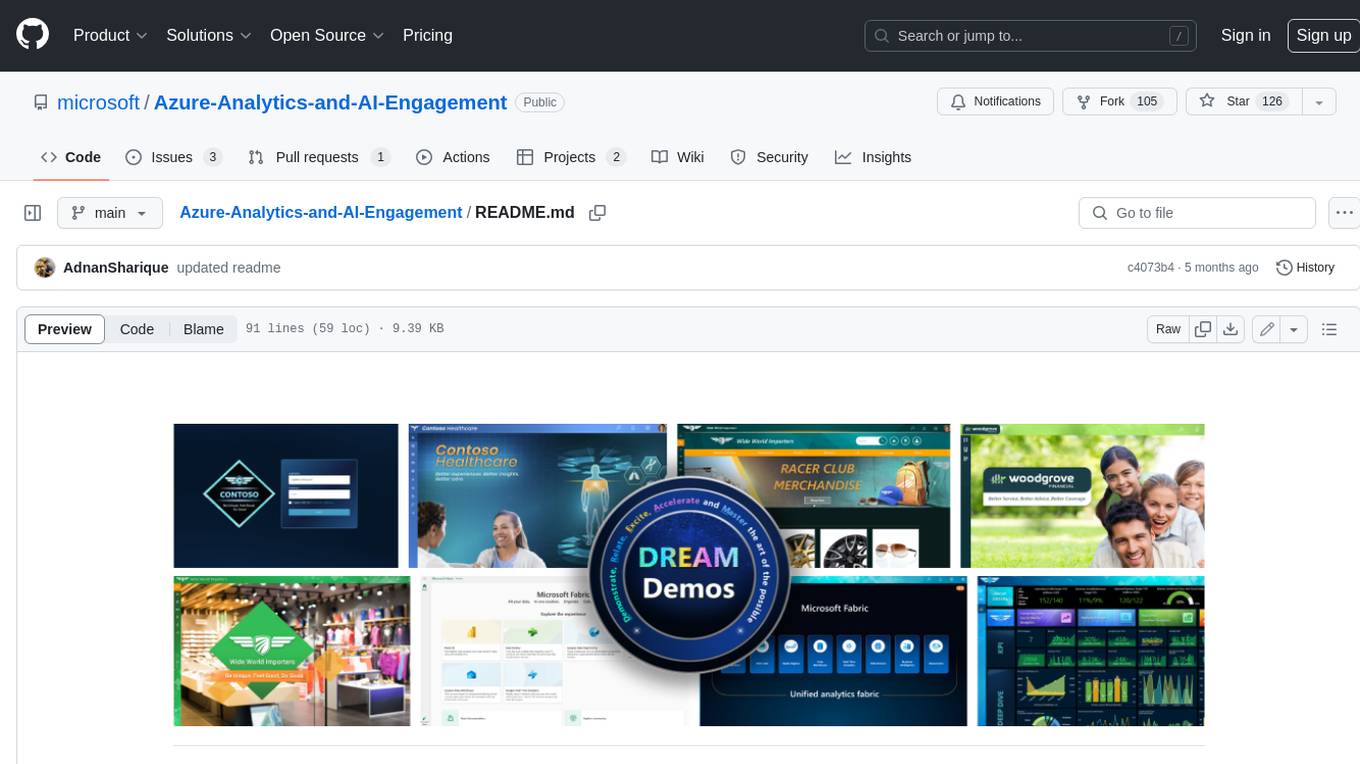
Azure-Analytics-and-AI-Engagement
The Azure-Analytics-and-AI-Engagement repository provides packaged Industry Scenario DREAM Demos with ARM templates (Containing a demo web application, Power BI reports, Synapse resources, AML Notebooks etc.) that can be deployed in a customer’s subscription using the CAPE tool within a matter of few hours. Partners can also deploy DREAM Demos in their own subscriptions using DPoC.
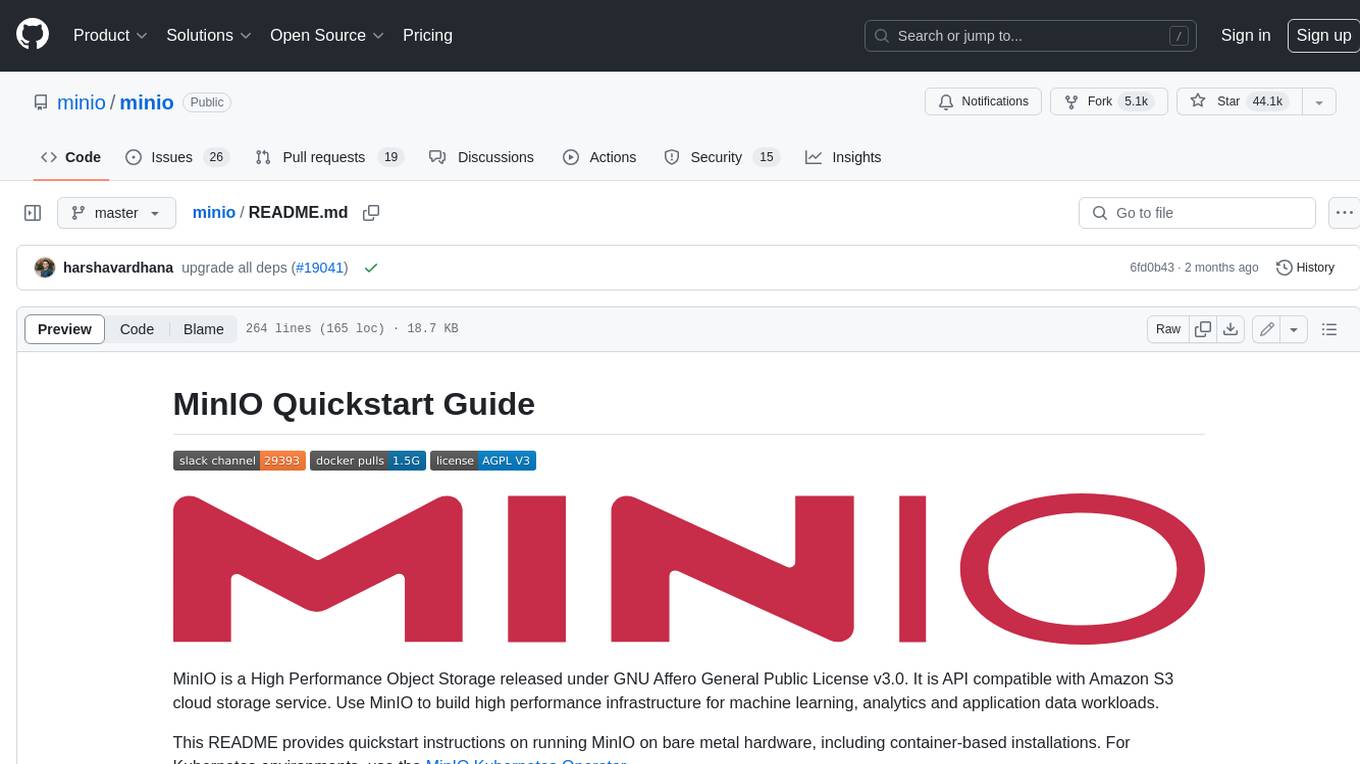
minio
MinIO is a High Performance Object Storage released under GNU Affero General Public License v3.0. It is API compatible with Amazon S3 cloud storage service. Use MinIO to build high performance infrastructure for machine learning, analytics and application data workloads.
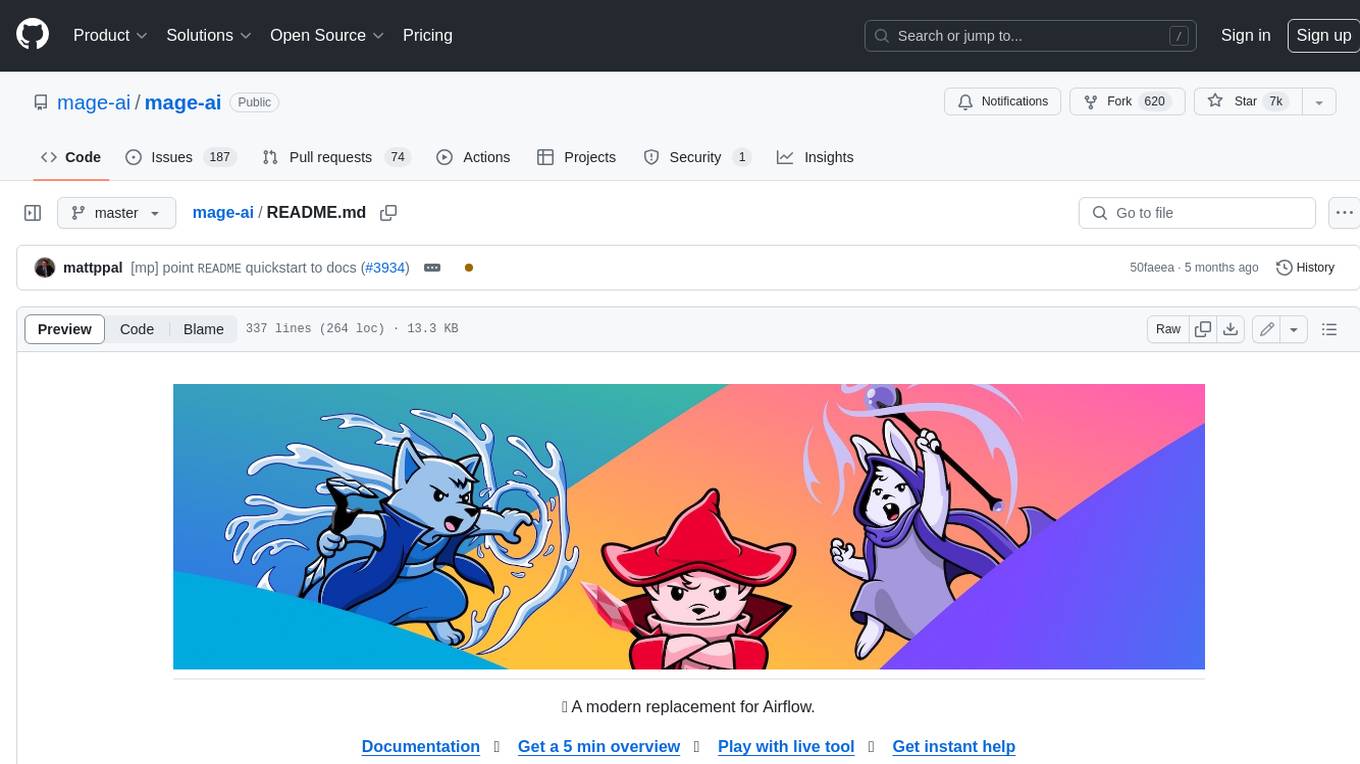
mage-ai
Mage is an open-source data pipeline tool for transforming and integrating data. It offers an easy developer experience, engineering best practices built-in, and data as a first-class citizen. Mage makes it easy to build, preview, and launch data pipelines, and provides observability and scaling capabilities. It supports data integrations, streaming pipelines, and dbt integration.
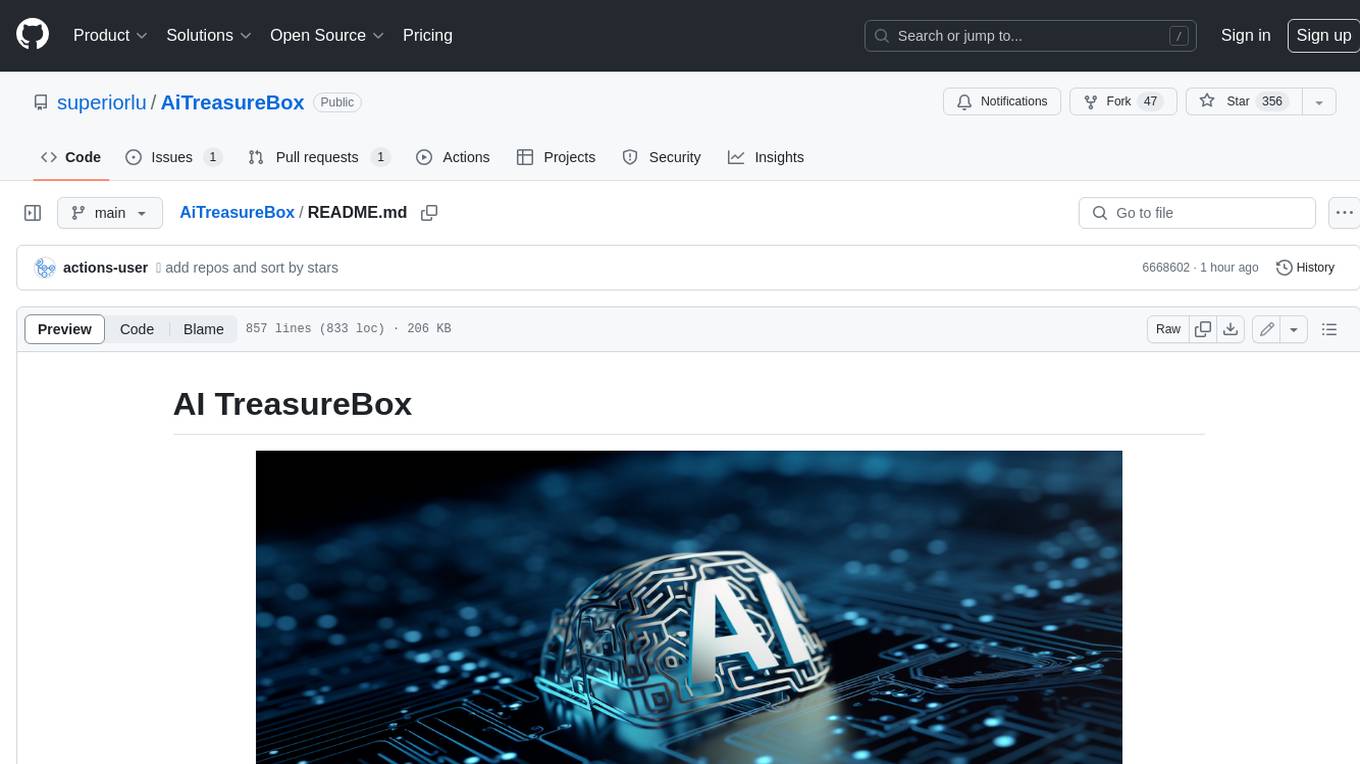
AiTreasureBox
AiTreasureBox is a versatile AI tool that provides a collection of pre-trained models and algorithms for various machine learning tasks. It simplifies the process of implementing AI solutions by offering ready-to-use components that can be easily integrated into projects. With AiTreasureBox, users can quickly prototype and deploy AI applications without the need for extensive knowledge in machine learning or deep learning. The tool covers a wide range of tasks such as image classification, text generation, sentiment analysis, object detection, and more. It is designed to be user-friendly and accessible to both beginners and experienced developers, making AI development more efficient and accessible to a wider audience.
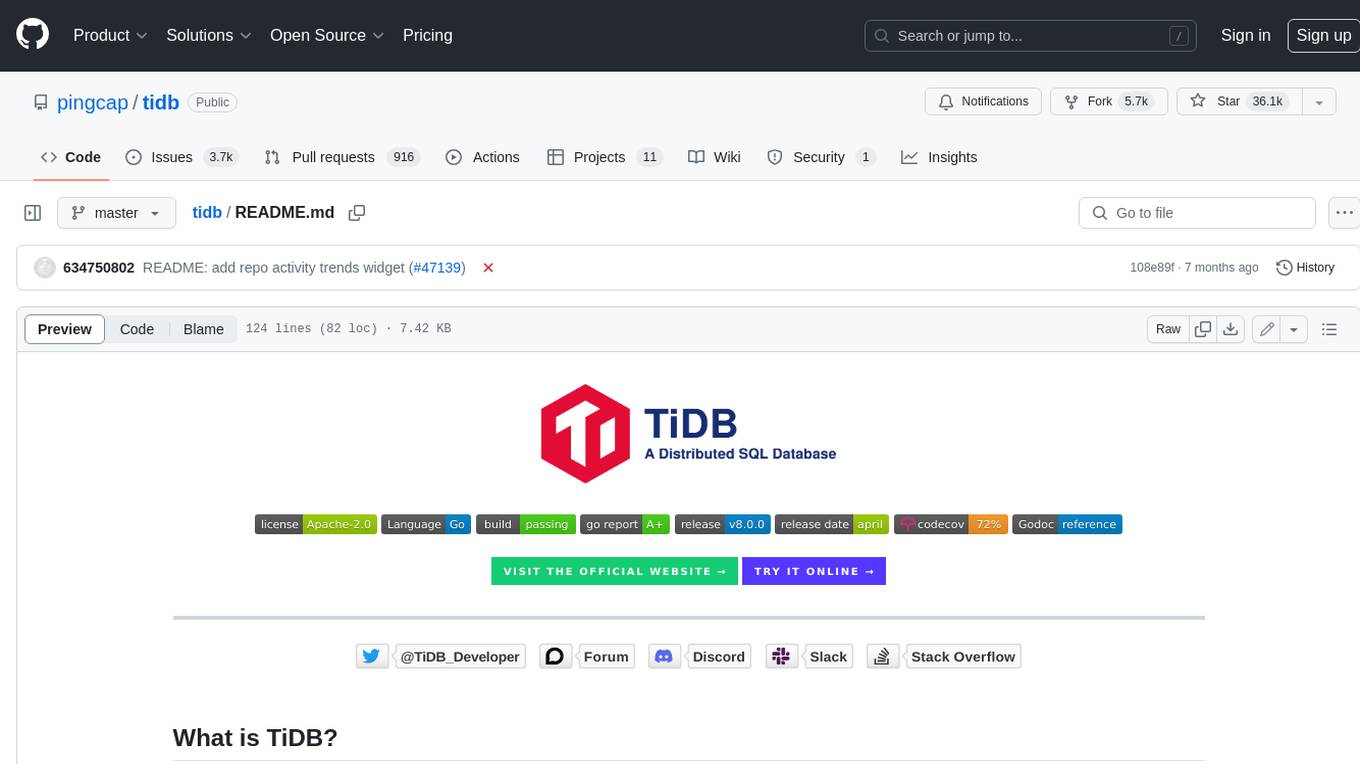
tidb
TiDB is an open-source distributed SQL database that supports Hybrid Transactional and Analytical Processing (HTAP) workloads. It is MySQL compatible and features horizontal scalability, strong consistency, and high availability.
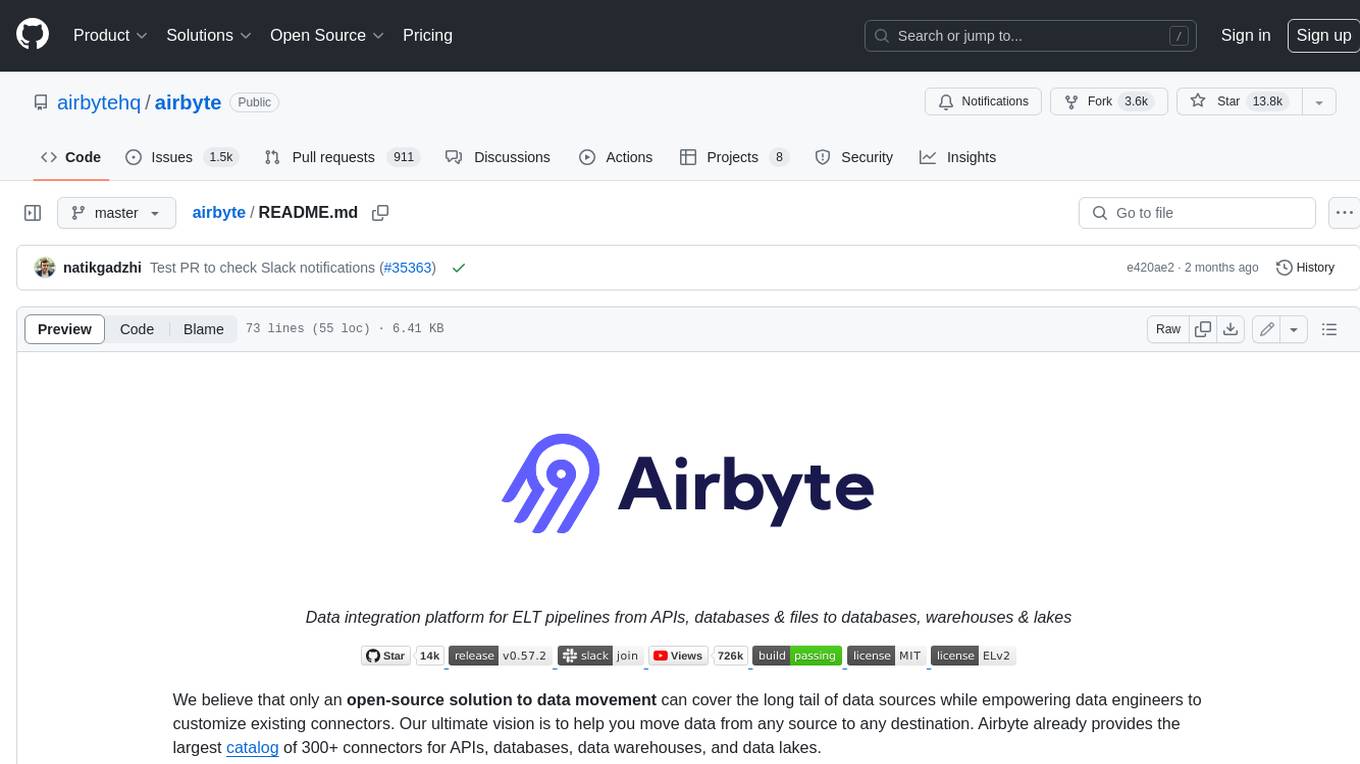
airbyte
Airbyte is an open-source data integration platform that makes it easy to move data from any source to any destination. With Airbyte, you can build and manage data pipelines without writing any code. Airbyte provides a library of pre-built connectors that make it easy to connect to popular data sources and destinations. You can also create your own connectors using Airbyte's no-code Connector Builder or low-code CDK. Airbyte is used by data engineers and analysts at companies of all sizes to build and manage their data pipelines.
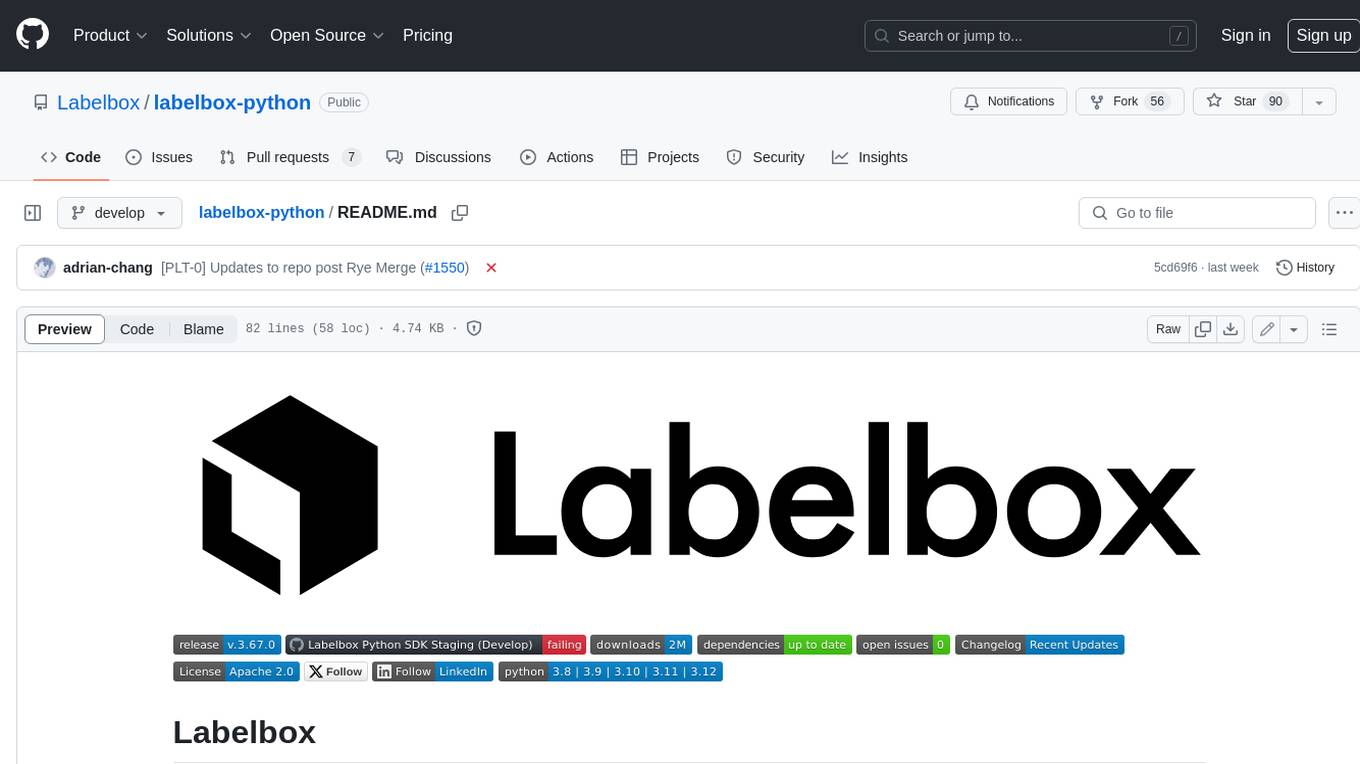
labelbox-python
Labelbox is a data-centric AI platform for enterprises to develop, optimize, and use AI to solve problems and power new products and services. Enterprises use Labelbox to curate data, generate high-quality human feedback data for computer vision and LLMs, evaluate model performance, and automate tasks by combining AI and human-centric workflows. The academic & research community uses Labelbox for cutting-edge AI research.