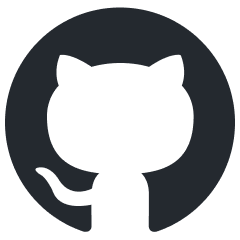
ragflow
RAGFlow is an open-source RAG (Retrieval-Augmented Generation) engine based on deep document understanding.
Stars: 28541
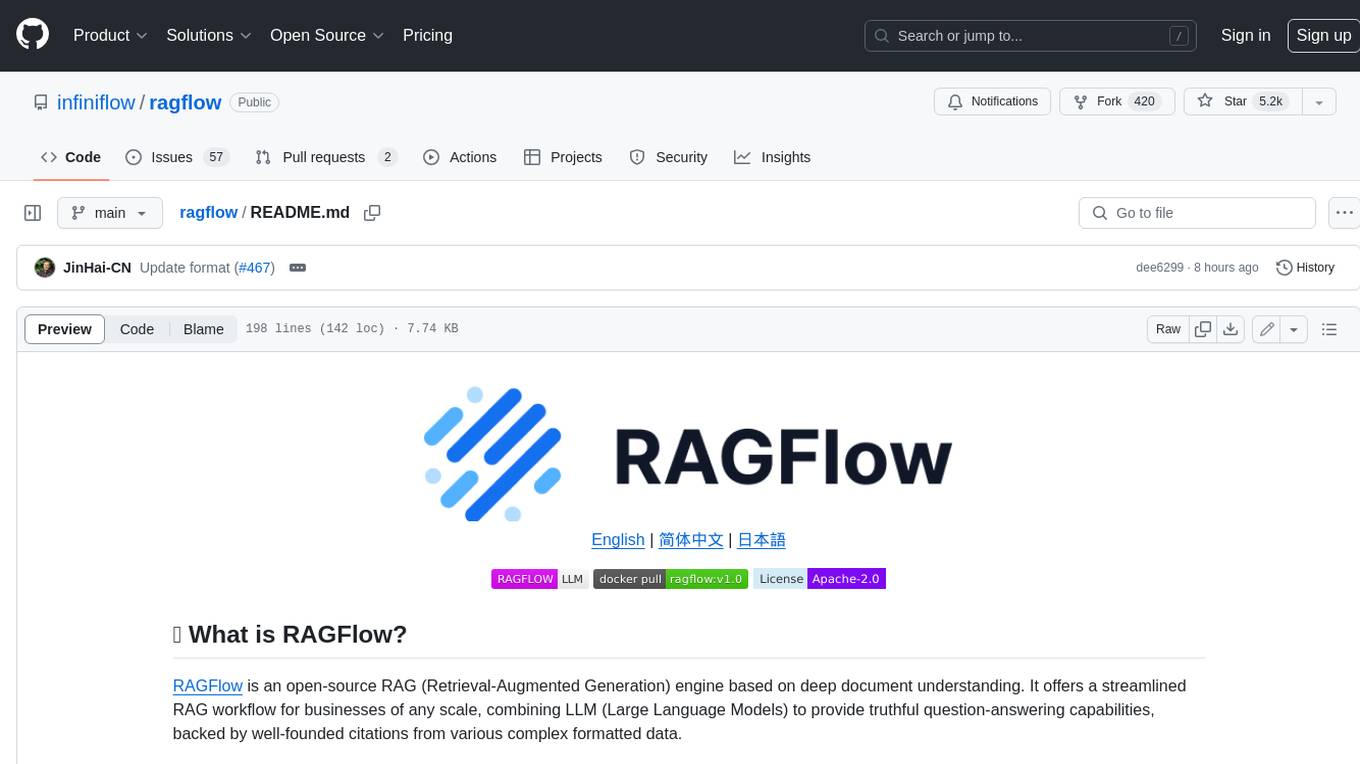
RAGFlow is an open-source Retrieval-Augmented Generation (RAG) engine that combines deep document understanding with Large Language Models (LLMs) to provide accurate question-answering capabilities. It offers a streamlined RAG workflow for businesses of all sizes, enabling them to extract knowledge from unstructured data in various formats, including Word documents, slides, Excel files, images, and more. RAGFlow's key features include deep document understanding, template-based chunking, grounded citations with reduced hallucinations, compatibility with heterogeneous data sources, and an automated and effortless RAG workflow. It supports multiple recall paired with fused re-ranking, configurable LLMs and embedding models, and intuitive APIs for seamless integration with business applications.
README:
English | 简体中文 | 日本語 | 한국어 | Bahasa Indonesia
📕 Table of Contents
- 💡 What is RAGFlow?
- 🎮 Demo
- 📌 Latest Updates
- 🌟 Key Features
- 🔎 System Architecture
- 🎬 Get Started
- 🔧 Configurations
- 🔧 Build a docker image without embedding models
- 🔧 Build a docker image including embedding models
- 🔨 Launch service from source for development
- 📚 Documentation
- 📜 Roadmap
- 🏄 Community
- 🙌 Contributing
RAGFlow is an open-source RAG (Retrieval-Augmented Generation) engine based on deep document understanding. It offers a streamlined RAG workflow for businesses of any scale, combining LLM (Large Language Models) to provide truthful question-answering capabilities, backed by well-founded citations from various complex formatted data.
Try our demo at https://demo.ragflow.io.
- 2024-12-18 Upgrades Document Layout Analysis model in Deepdoc.
- 2024-12-04 Adds support for pagerank score in knowledge base.
- 2024-11-22 Adds more variables to Agent.
- 2024-11-01 Adds keyword extraction and related question generation to the parsed chunks to improve the accuracy of retrieval.
- 2024-08-22 Support text to SQL statements through RAG.
- 2024-08-02 Supports GraphRAG inspired by graphrag and mind map.
⭐️ Star our repository to stay up-to-date with exciting new features and improvements! Get instant notifications for new releases! 🌟
- Deep document understanding-based knowledge extraction from unstructured data with complicated formats.
- Finds "needle in a data haystack" of literally unlimited tokens.
- Intelligent and explainable.
- Plenty of template options to choose from.
- Visualization of text chunking to allow human intervention.
- Quick view of the key references and traceable citations to support grounded answers.
- Supports Word, slides, excel, txt, images, scanned copies, structured data, web pages, and more.
- Streamlined RAG orchestration catered to both personal and large businesses.
- Configurable LLMs as well as embedding models.
- Multiple recall paired with fused re-ranking.
- Intuitive APIs for seamless integration with business.
- CPU >= 4 cores
- RAM >= 16 GB
- Disk >= 50 GB
- Docker >= 24.0.0 & Docker Compose >= v2.26.1
If you have not installed Docker on your local machine (Windows, Mac, or Linux), see Install Docker Engine.
-
Ensure
vm.max_map_count
>= 262144:To check the value of
vm.max_map_count
:$ sysctl vm.max_map_count
Reset
vm.max_map_count
to a value at least 262144 if it is not.# In this case, we set it to 262144: $ sudo sysctl -w vm.max_map_count=262144
This change will be reset after a system reboot. To ensure your change remains permanent, add or update the
vm.max_map_count
value in /etc/sysctl.conf accordingly:vm.max_map_count=262144
-
Clone the repo:
$ git clone https://github.com/infiniflow/ragflow.git
-
Start up the server using the pre-built Docker images:
The command below downloads the
v0.15.1-slim
edition of the RAGFlow Docker image. Refer to the following table for descriptions of different RAGFlow editions. To download an RAGFlow edition different fromv0.15.1-slim
, update theRAGFLOW_IMAGE
variable accordingly in docker/.env before usingdocker compose
to start the server. For example: setRAGFLOW_IMAGE=infiniflow/ragflow:v0.15.1
for the full editionv0.15.1
.$ cd ragflow $ docker compose -f docker/docker-compose.yml up -d
RAGFlow image tag Image size (GB) Has embedding models? Stable? v0.15.1 ≈9 ✔️ Stable release v0.15.1-slim ≈2 ❌ Stable release nightly ≈9 ✔️ Unstable nightly build nightly-slim ≈2 ❌ Unstable nightly build -
Check the server status after having the server up and running:
$ docker logs -f ragflow-server
The following output confirms a successful launch of the system:
____ ___ ______ ______ __ / __ \ / | / ____// ____// /____ _ __ / /_/ // /| | / / __ / /_ / // __ \| | /| / / / _, _// ___ |/ /_/ // __/ / // /_/ /| |/ |/ / /_/ |_|/_/ |_|\____//_/ /_/ \____/ |__/|__/ * Running on all addresses (0.0.0.0) * Running on http://127.0.0.1:9380 * Running on http://x.x.x.x:9380 INFO:werkzeug:Press CTRL+C to quit
If you skip this confirmation step and directly log in to RAGFlow, your browser may prompt a
network anormal
error because, at that moment, your RAGFlow may not be fully initialized. -
In your web browser, enter the IP address of your server and log in to RAGFlow.
With the default settings, you only need to enter
http://IP_OF_YOUR_MACHINE
(sans port number) as the default HTTP serving port80
can be omitted when using the default configurations. -
In service_conf.yaml.template, select the desired LLM factory in
user_default_llm
and update theAPI_KEY
field with the corresponding API key.See llm_api_key_setup for more information.
The show is on!
When it comes to system configurations, you will need to manage the following files:
-
.env: Keeps the fundamental setups for the system, such as
SVR_HTTP_PORT
,MYSQL_PASSWORD
, andMINIO_PASSWORD
. - service_conf.yaml.template: Configures the back-end services. The environment variables in this file will be automatically populated when the Docker container starts. Any environment variables set within the Docker container will be available for use, allowing you to customize service behavior based on the deployment environment.
- docker-compose.yml: The system relies on docker-compose.yml to start up.
The ./docker/README file provides a detailed description of the environment settings and service configurations which can be used as
${ENV_VARS}
in the service_conf.yaml.template file.
To update the default HTTP serving port (80), go to docker-compose.yml and change 80:80
to <YOUR_SERVING_PORT>:80
.
Updates to the above configurations require a reboot of all containers to take effect:
$ docker compose -f docker/docker-compose.yml up -d
RAGFlow uses Elasticsearch by default for storing full text and vectors. To switch to Infinity, follow these steps:
-
Stop all running containers:
$ docker compose -f docker/docker-compose.yml down -v
-
Set
DOC_ENGINE
in docker/.env toinfinity
. -
Start the containers:
$ docker compose -f docker/docker-compose.yml up -d
[!WARNING] Switching to Infinity on a Linux/arm64 machine is not yet officially supported.
This image is approximately 2 GB in size and relies on external LLM and embedding services.
git clone https://github.com/infiniflow/ragflow.git
cd ragflow/
docker build --build-arg LIGHTEN=1 -f Dockerfile -t infiniflow/ragflow:nightly-slim .
This image is approximately 9 GB in size. As it includes embedding models, it relies on external LLM services only.
git clone https://github.com/infiniflow/ragflow.git
cd ragflow/
docker build -f Dockerfile -t infiniflow/ragflow:nightly .
-
Install uv, or skip this step if it is already installed:
pipx install uv
-
Clone the source code and install Python dependencies:
git clone https://github.com/infiniflow/ragflow.git cd ragflow/ uv sync --python 3.10 --all-extras # install RAGFlow dependent python modules
-
Launch the dependent services (MinIO, Elasticsearch, Redis, and MySQL) using Docker Compose:
docker compose -f docker/docker-compose-base.yml up -d
Add the following line to
/etc/hosts
to resolve all hosts specified in docker/.env to127.0.0.1
:127.0.0.1 es01 infinity mysql minio redis
-
If you cannot access HuggingFace, set the
HF_ENDPOINT
environment variable to use a mirror site:export HF_ENDPOINT=https://hf-mirror.com
-
Launch backend service:
source .venv/bin/activate export PYTHONPATH=$(pwd) bash docker/launch_backend_service.sh
-
Install frontend dependencies:
cd web npm install
-
Launch frontend service:
npm run dev
The following output confirms a successful launch of the system:
See the RAGFlow Roadmap 2025
RAGFlow flourishes via open-source collaboration. In this spirit, we embrace diverse contributions from the community. If you would like to be a part, review our Contribution Guidelines first.
For Tasks:
Click tags to check more tools for each tasksFor Jobs:
Alternative AI tools for ragflow
Similar Open Source Tools
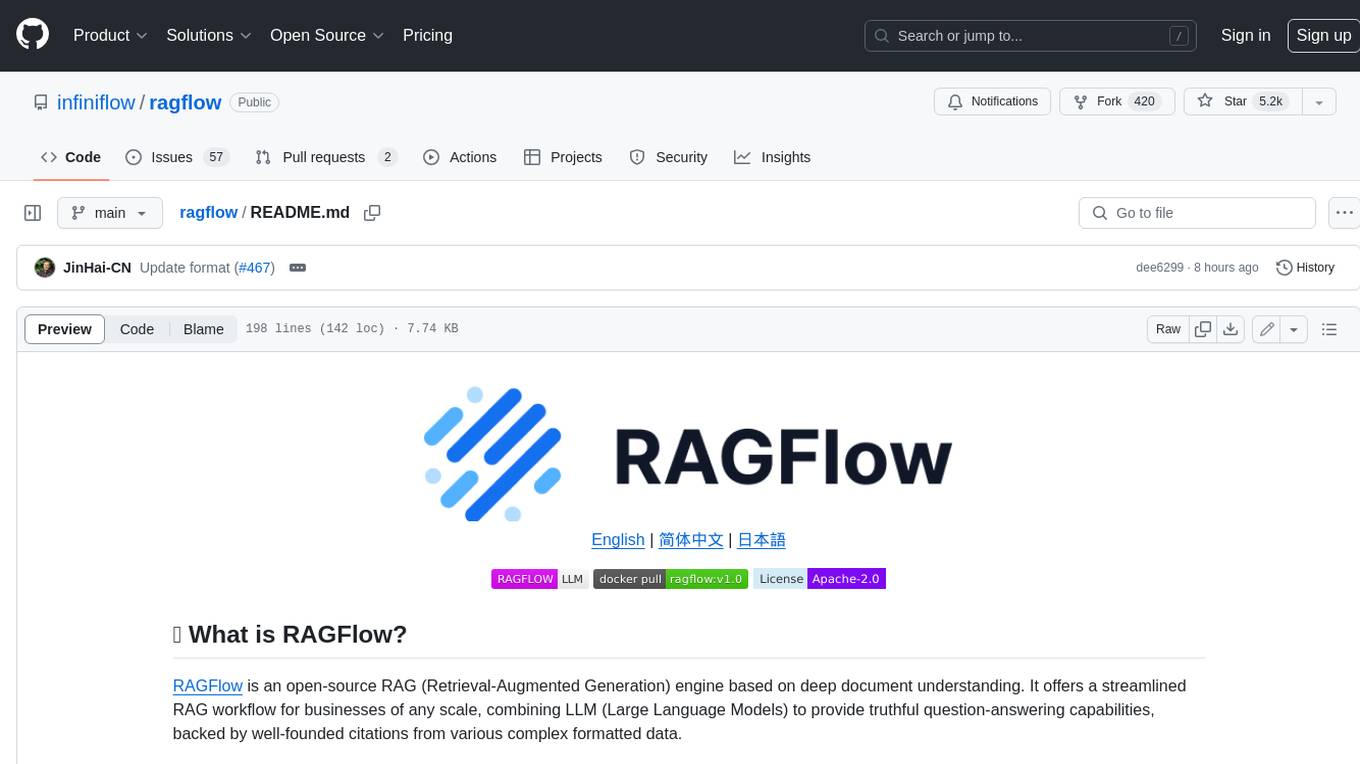
ragflow
RAGFlow is an open-source Retrieval-Augmented Generation (RAG) engine that combines deep document understanding with Large Language Models (LLMs) to provide accurate question-answering capabilities. It offers a streamlined RAG workflow for businesses of all sizes, enabling them to extract knowledge from unstructured data in various formats, including Word documents, slides, Excel files, images, and more. RAGFlow's key features include deep document understanding, template-based chunking, grounded citations with reduced hallucinations, compatibility with heterogeneous data sources, and an automated and effortless RAG workflow. It supports multiple recall paired with fused re-ranking, configurable LLMs and embedding models, and intuitive APIs for seamless integration with business applications.
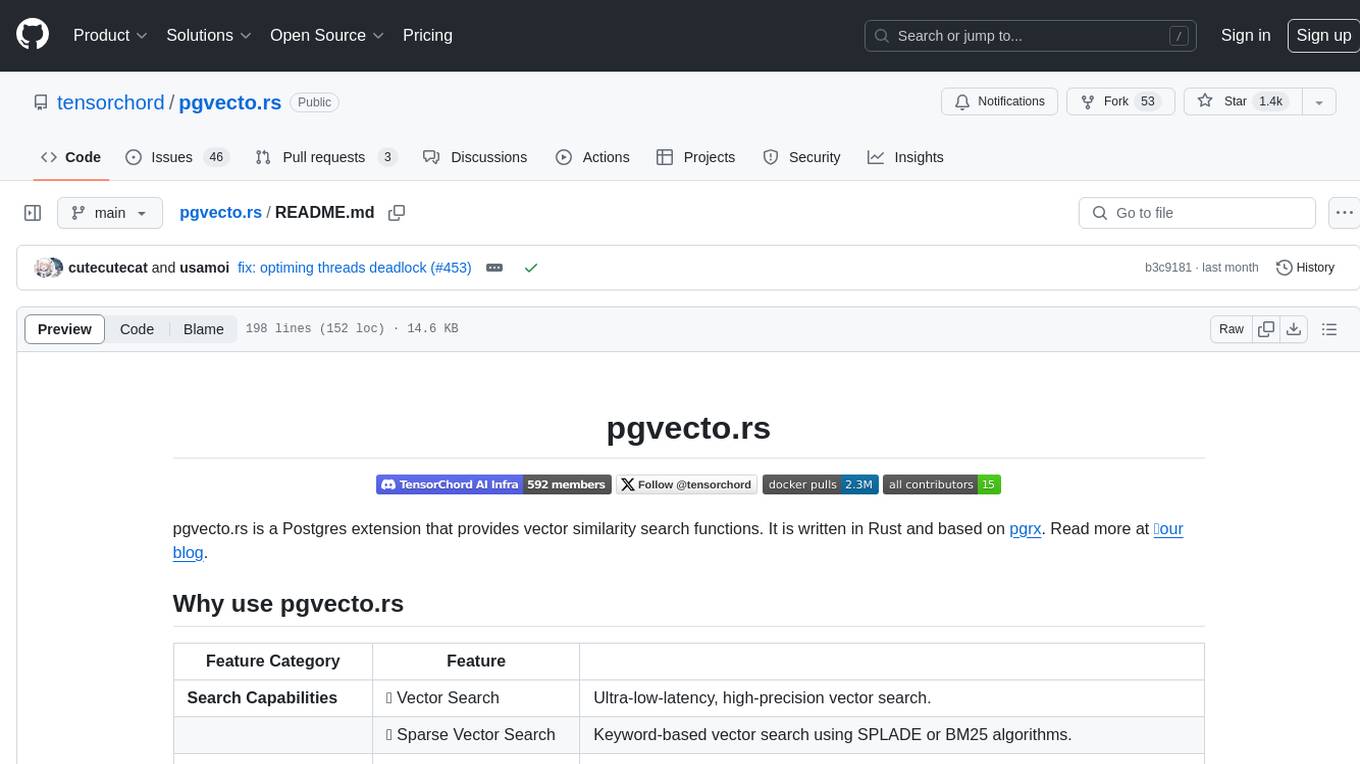
pgvecto.rs
pgvecto.rs is a Postgres extension written in Rust that provides vector similarity search functions. It offers ultra-low-latency, high-precision vector search capabilities, including sparse vector search and full-text search. With complete SQL support, async indexing, and easy data management, it simplifies data handling. The extension supports various data types like FP16/INT8, binary vectors, and Matryoshka embeddings. It ensures system performance with production-ready features, high availability, and resource efficiency. Security and permissions are managed through easy access control. The tool allows users to create tables with vector columns, insert vector data, and calculate distances between vectors using different operators. It also supports half-precision floating-point numbers for better performance and memory usage optimization.
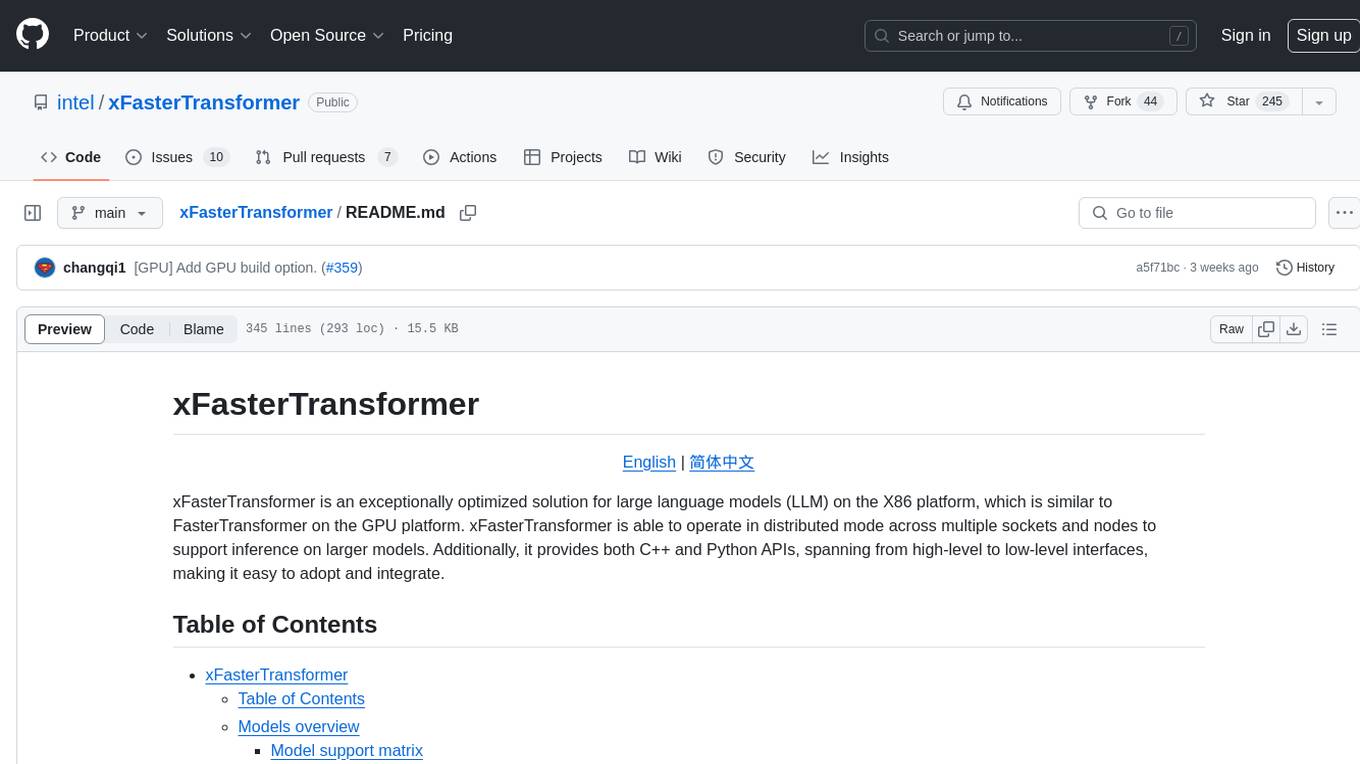
xFasterTransformer
xFasterTransformer is an optimized solution for Large Language Models (LLMs) on the X86 platform, providing high performance and scalability for inference on mainstream LLM models. It offers C++ and Python APIs for easy integration, along with example codes and benchmark scripts. Users can prepare models in a different format, convert them, and use the APIs for tasks like encoding input prompts, generating token ids, and serving inference requests. The tool supports various data types and models, and can run in single or multi-rank modes using MPI. A web demo based on Gradio is available for popular LLM models like ChatGLM and Llama2. Benchmark scripts help evaluate model inference performance quickly, and MLServer enables serving with REST and gRPC interfaces.
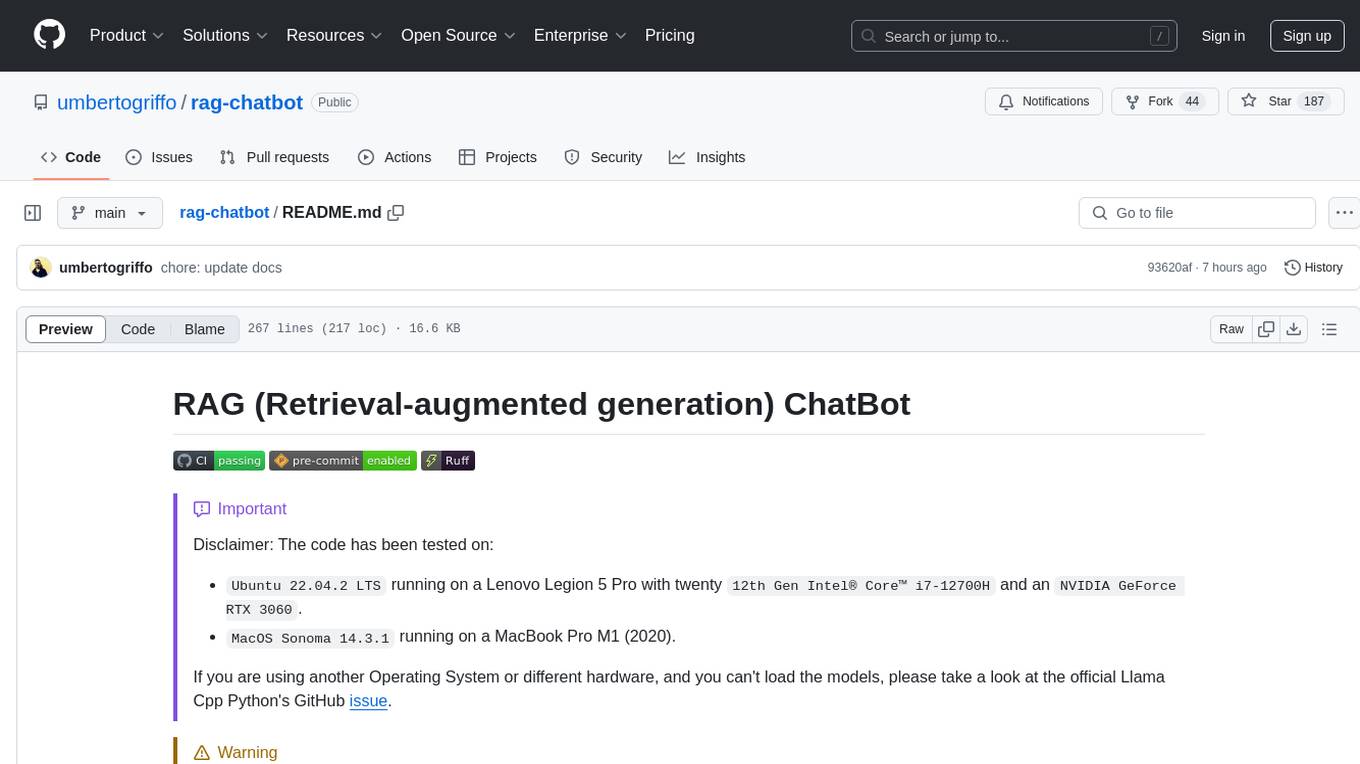
rag-chatbot
The RAG ChatBot project combines Lama.cpp, Chroma, and Streamlit to build a Conversation-aware Chatbot and a Retrieval-augmented generation (RAG) ChatBot. The RAG Chatbot works by taking a collection of Markdown files as input and provides answers based on the context provided by those files. It utilizes a Memory Builder component to load Markdown pages, divide them into sections, calculate embeddings, and save them in an embedding database. The chatbot retrieves relevant sections from the database, rewrites questions for optimal retrieval, and generates answers using a local language model. It also remembers previous interactions for more accurate responses. Various strategies are implemented to deal with context overflows, including creating and refining context, hierarchical summarization, and async hierarchical summarization.
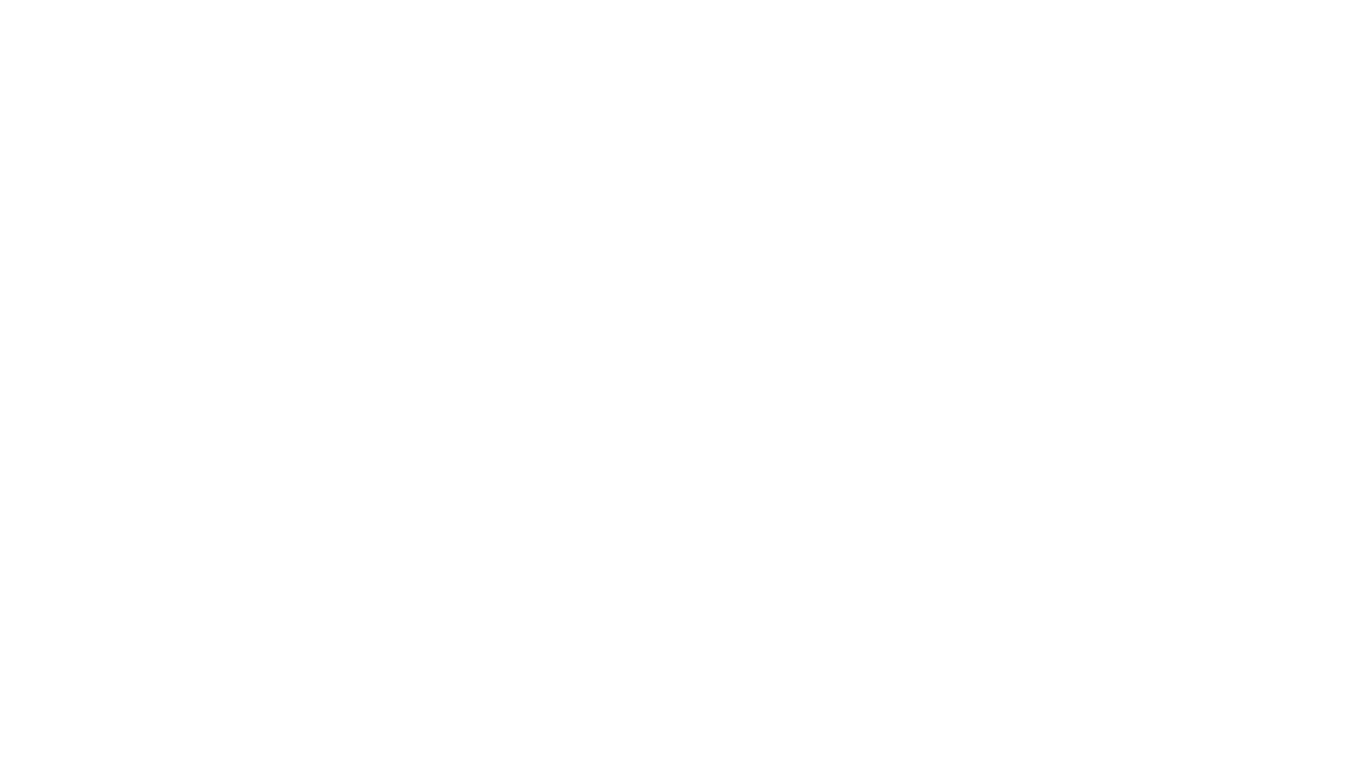
QodeAssist
QodeAssist is an AI-powered coding assistant plugin for Qt Creator, offering intelligent code completion and suggestions for C++ and QML. It leverages large language models like Ollama to enhance coding productivity with context-aware AI assistance directly in the Qt development environment. The plugin supports multiple LLM providers, extensive model-specific templates, and easy configuration for enhanced coding experience.
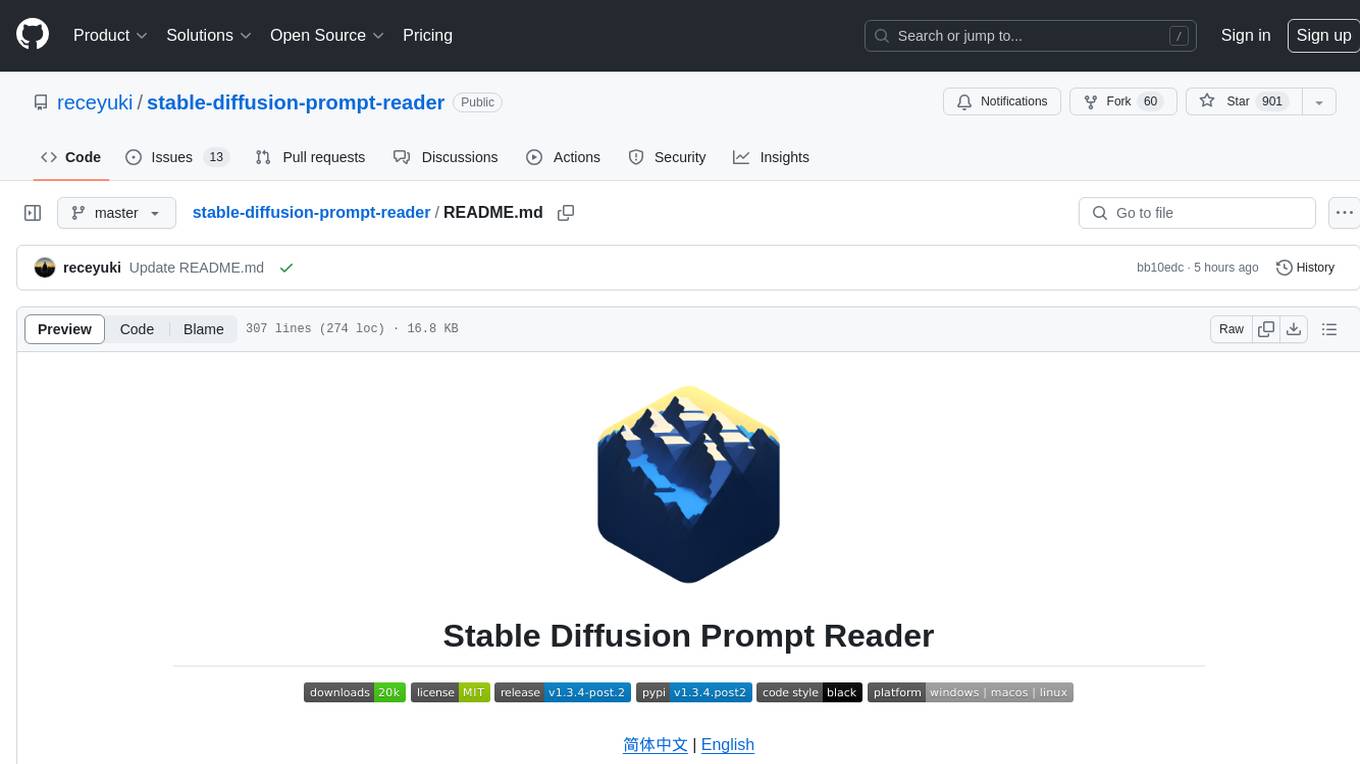
stable-diffusion-prompt-reader
A simple standalone viewer for reading prompt from Stable Diffusion generated image outside the webui. The tool supports macOS, Windows, and Linux, providing both GUI and CLI functionalities. Users can interact with the tool through drag and drop, copy prompt to clipboard, remove prompt from image, export prompt to text file, edit or import prompt to images, and more. It supports multiple formats including PNG, JPEG, WEBP, TXT, and various tools like A1111's webUI, Easy Diffusion, StableSwarmUI, Fooocus-MRE, NovelAI, InvokeAI, ComfyUI, Draw Things, and Naifu(4chan). Users can download the tool for different platforms and install it via Homebrew Cask or pip. The tool can be used to read, export, remove, and edit prompts from images, providing various modes and options for different tasks.
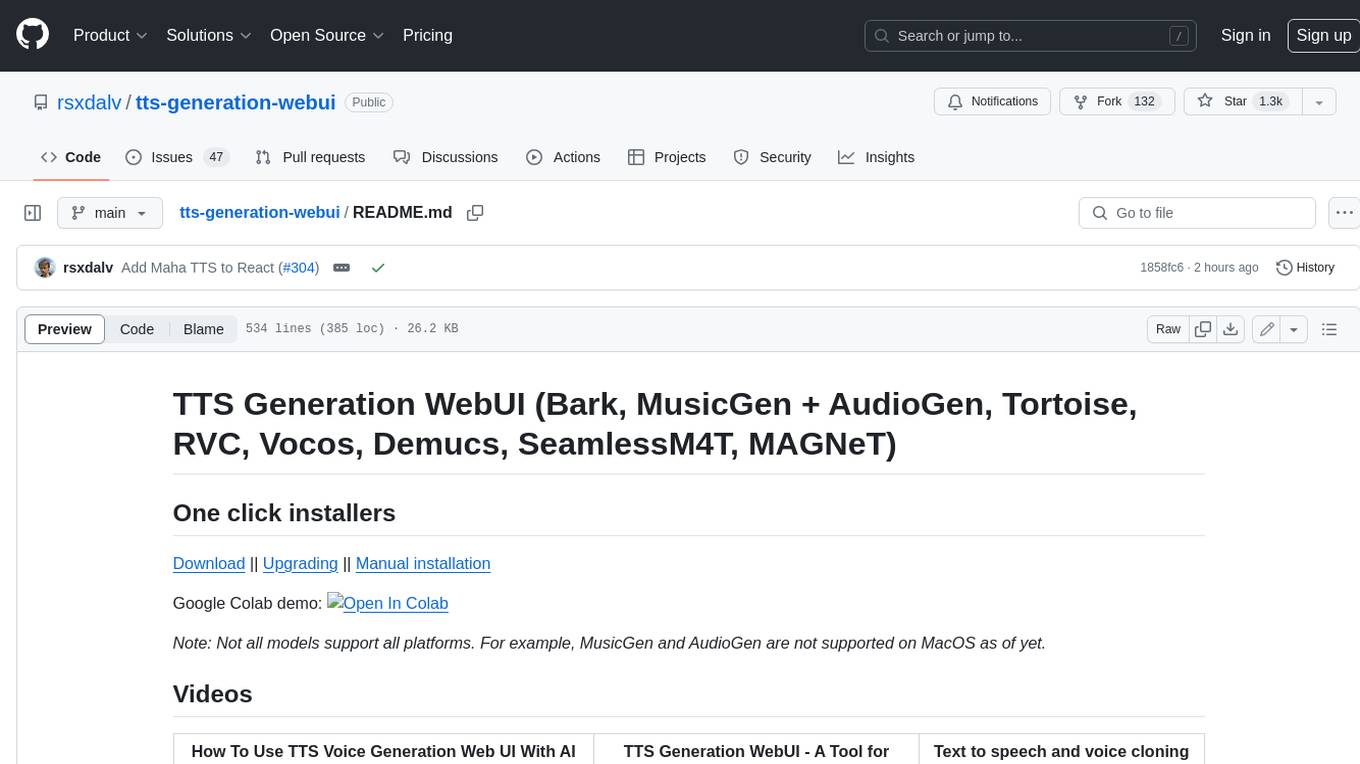
tts-generation-webui
TTS Generation WebUI is a comprehensive tool that provides a user-friendly interface for text-to-speech and voice cloning tasks. It integrates various AI models such as Bark, MusicGen, AudioGen, Tortoise, RVC, Vocos, Demucs, SeamlessM4T, and MAGNeT. The tool offers one-click installers, Google Colab demo, videos for guidance, and extra voices for Bark. Users can generate audio outputs, manage models, caches, and system space for AI projects. The project is open-source and emphasizes ethical and responsible use of AI technology.
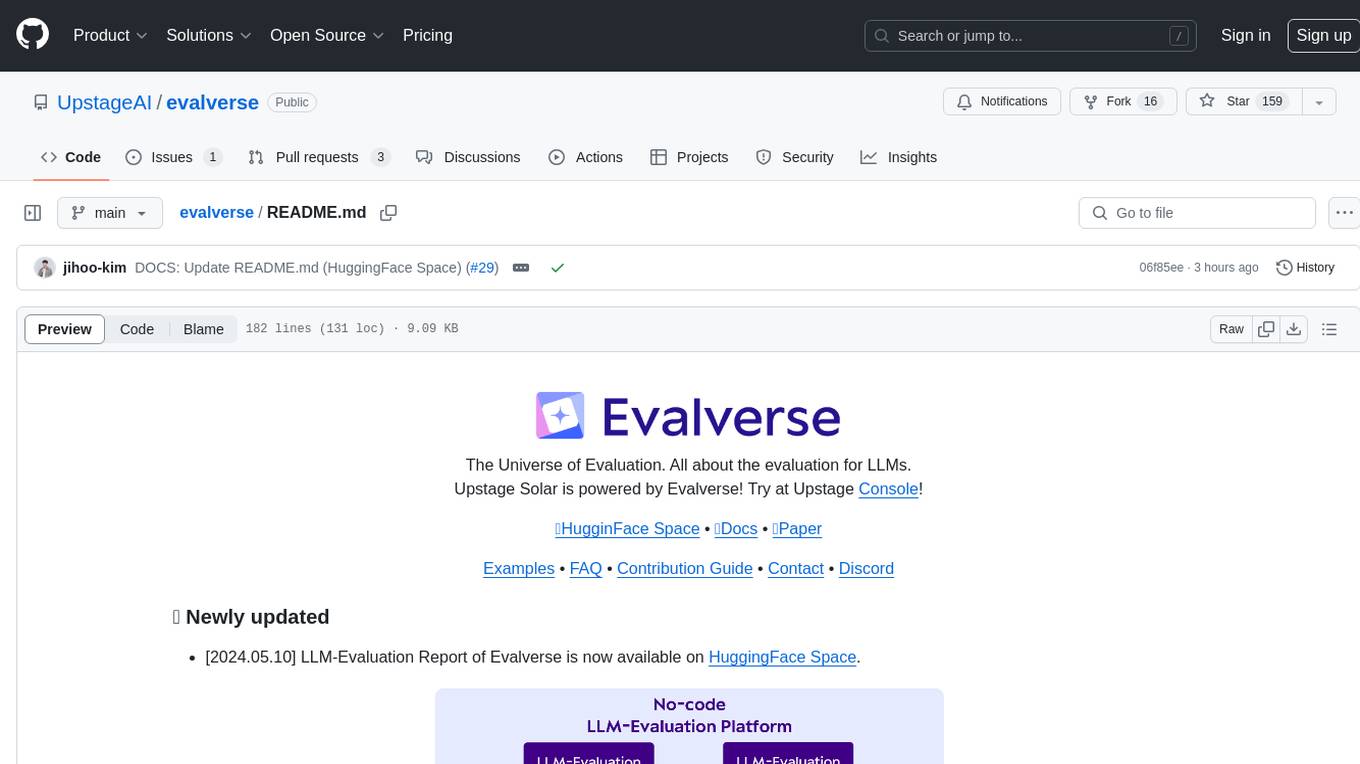
evalverse
Evalverse is an open-source project designed to support Large Language Model (LLM) evaluation needs. It provides a standardized and user-friendly solution for processing and managing LLM evaluations, catering to AI research engineers and scientists. Evalverse supports various evaluation methods, insightful reports, and no-code evaluation processes. Users can access unified evaluation with submodules, request evaluations without code via Slack bot, and obtain comprehensive reports with scores, rankings, and visuals. The tool allows for easy comparison of scores across different models and swift addition of new evaluation tools.
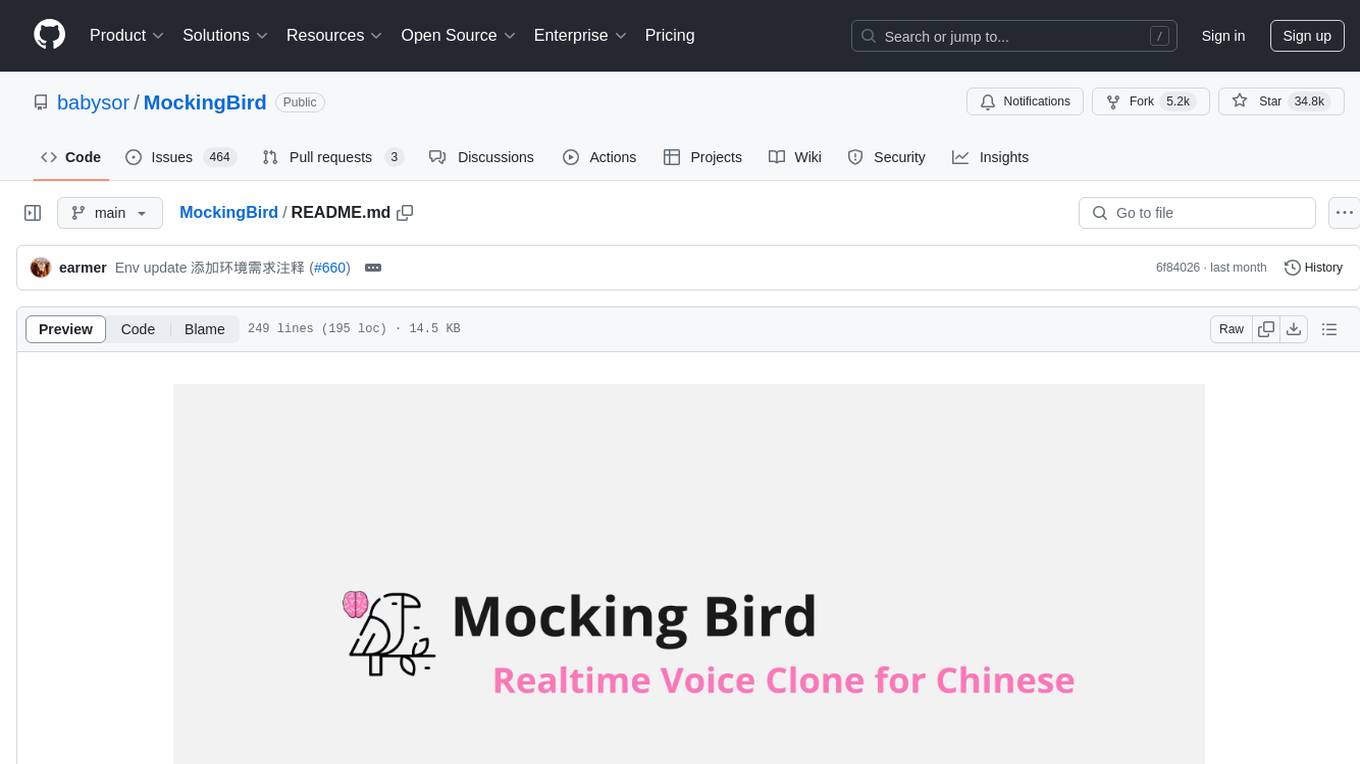
MockingBird
MockingBird is a toolbox designed for Mandarin speech synthesis using PyTorch. It supports multiple datasets such as aidatatang_200zh, magicdata, aishell3, and data_aishell. The toolbox can run on Windows, Linux, and M1 MacOS, providing easy and effective speech synthesis with pretrained encoder/vocoder models. It is webserver ready for remote calling. Users can train their own models or use existing ones for the encoder, synthesizer, and vocoder. The toolbox offers a demo video and detailed setup instructions for installation and model training.
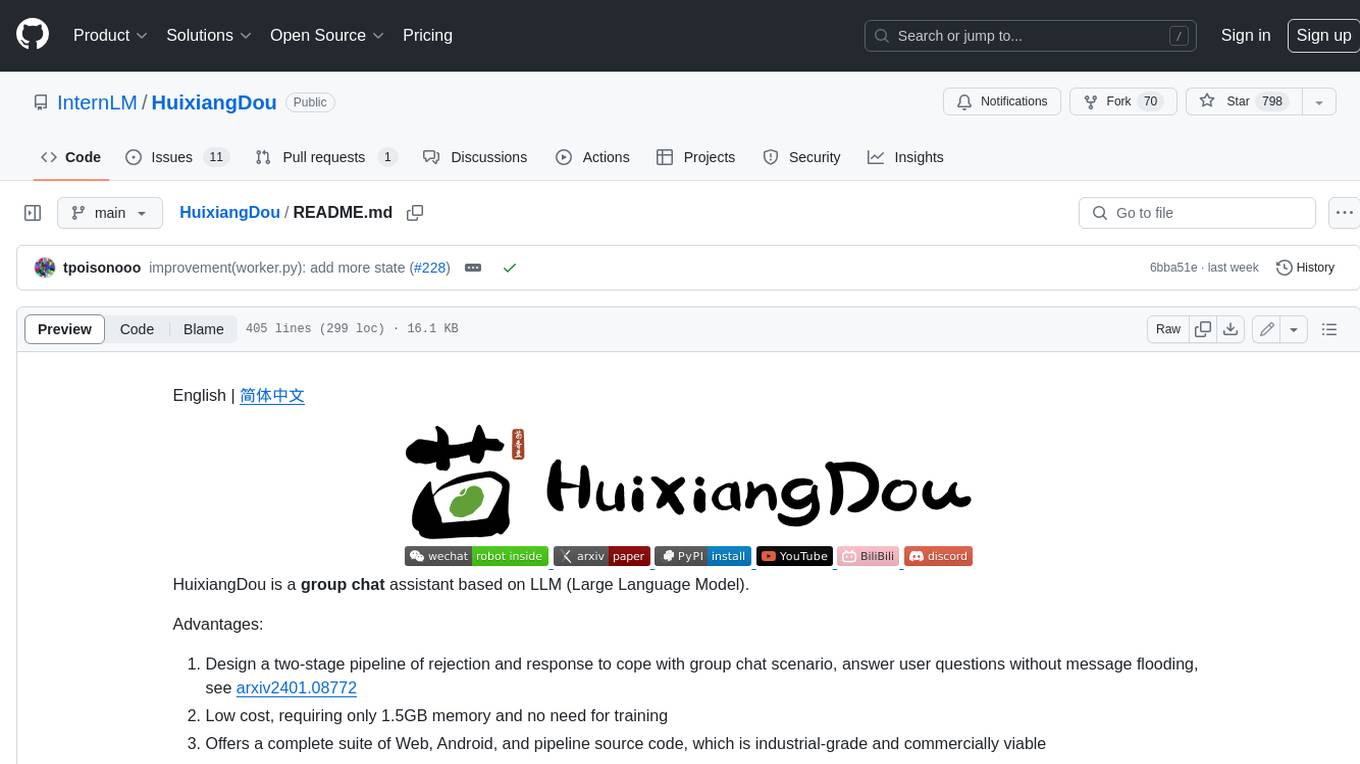
HuixiangDou
HuixiangDou is a **group chat** assistant based on LLM (Large Language Model). Advantages: 1. Design a two-stage pipeline of rejection and response to cope with group chat scenario, answer user questions without message flooding, see arxiv2401.08772 2. Low cost, requiring only 1.5GB memory and no need for training 3. Offers a complete suite of Web, Android, and pipeline source code, which is industrial-grade and commercially viable Check out the scenes in which HuixiangDou are running and join WeChat Group to try AI assistant inside. If this helps you, please give it a star ⭐
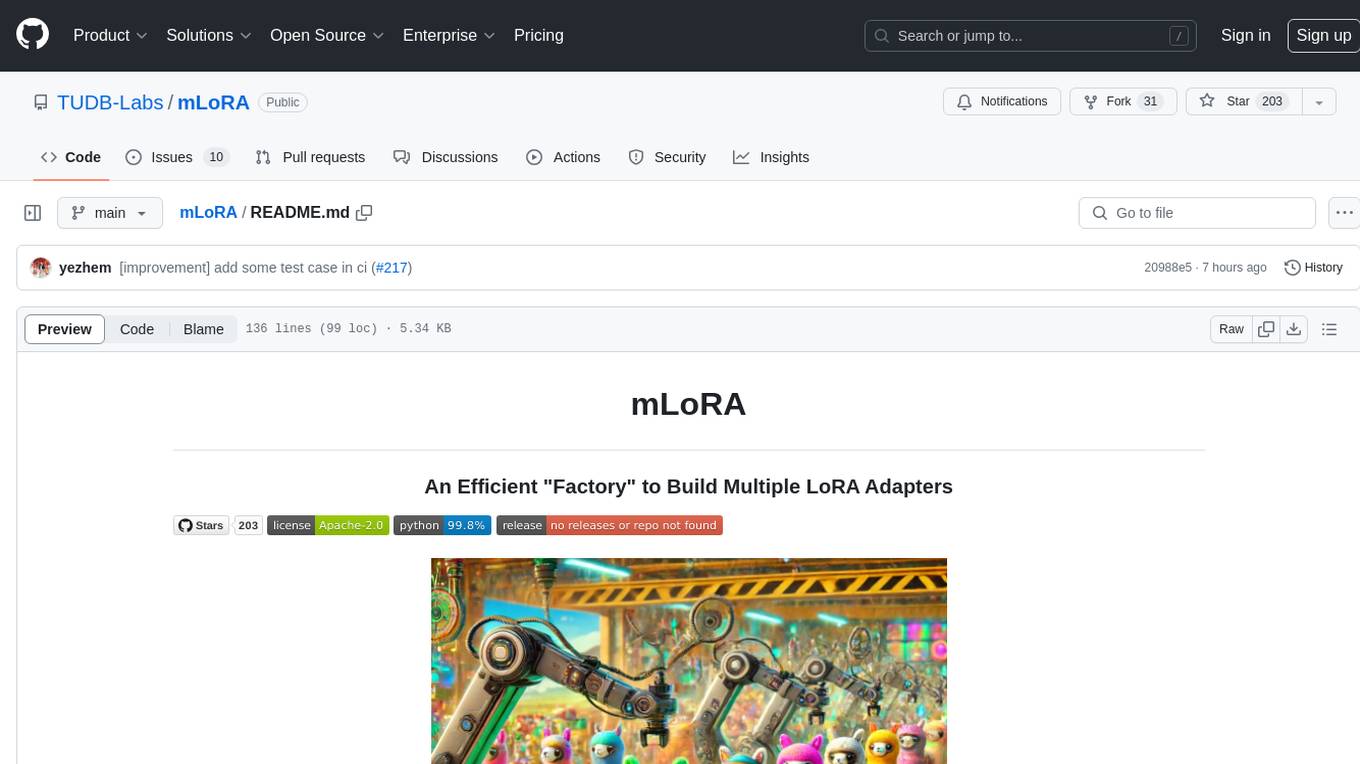
mLoRA
mLoRA (Multi-LoRA Fine-Tune) is an open-source framework for efficient fine-tuning of multiple Large Language Models (LLMs) using LoRA and its variants. It allows concurrent fine-tuning of multiple LoRA adapters with a shared base model, efficient pipeline parallelism algorithm, support for various LoRA variant algorithms, and reinforcement learning preference alignment algorithms. mLoRA helps save computational and memory resources when training multiple adapters simultaneously, achieving high performance on consumer hardware.
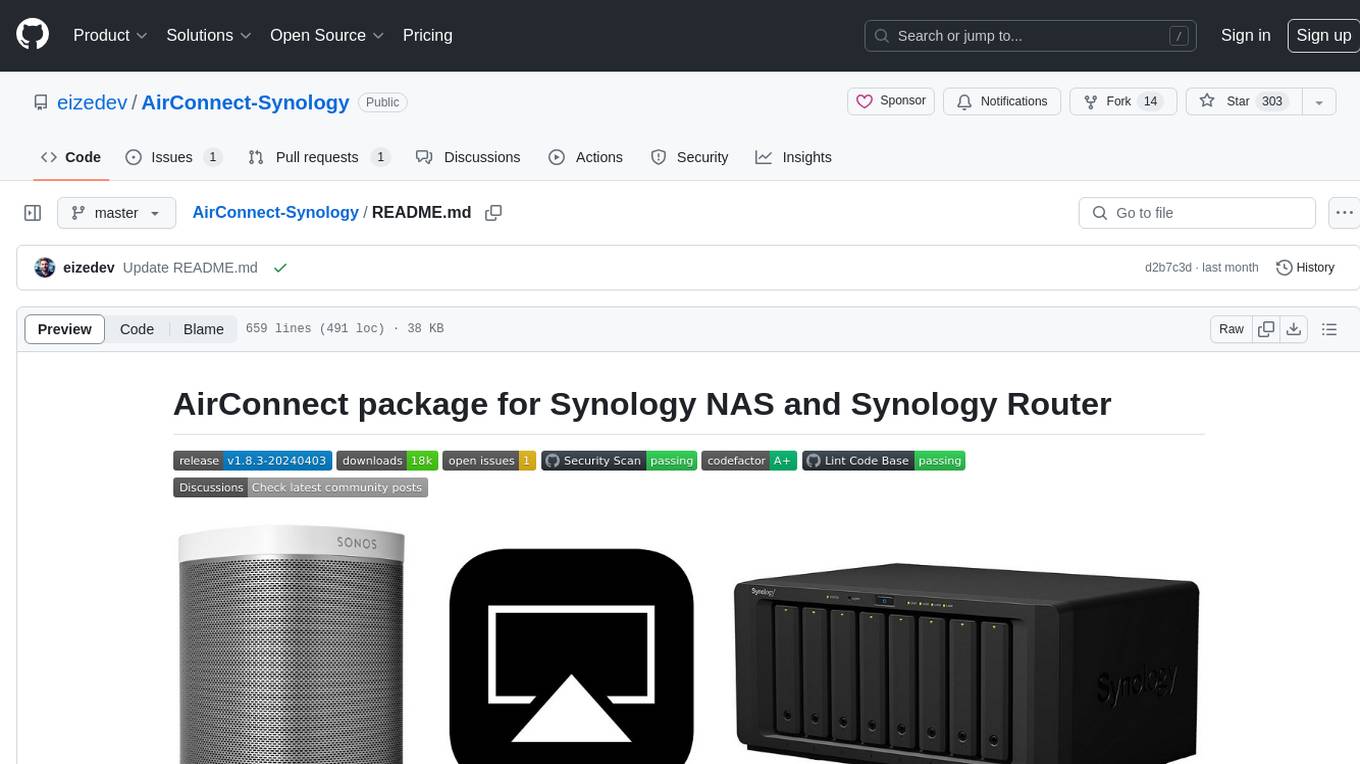
AirConnect-Synology
AirConnect-Synology is a minimal Synology package that allows users to use AirPlay to stream to UPnP/Sonos & Chromecast devices that do not natively support AirPlay. It is compatible with DSM 7.0 and DSM 7.1, and provides detailed information on installation, configuration, supported devices, troubleshooting, and more. The package automates the installation and usage of AirConnect on Synology devices, ensuring compatibility with various architectures and firmware versions. Users can customize the configuration using the airconnect.conf file and adjust settings for specific speakers like Sonos, Bose SoundTouch, and Pioneer/Phorus/Play-Fi.
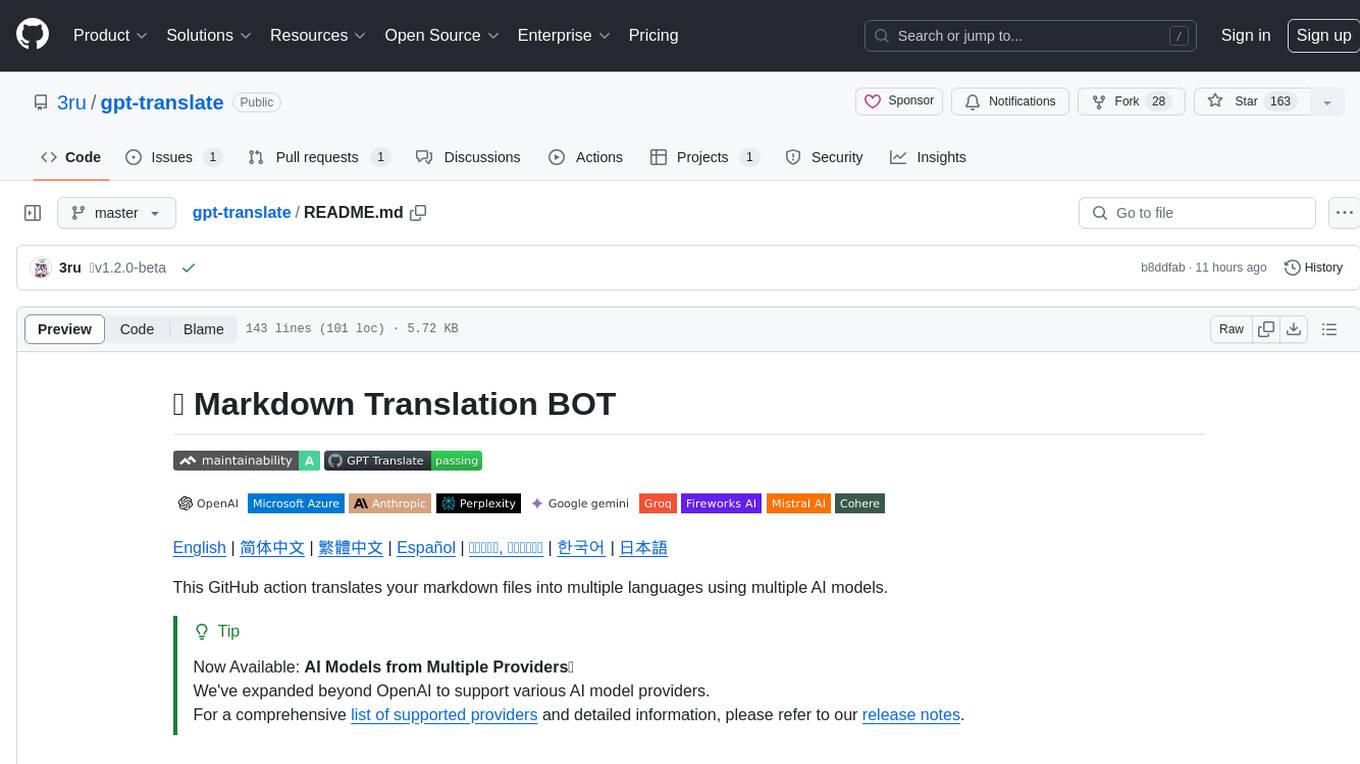
gpt-translate
Markdown Translation BOT is a GitHub action that translates markdown files into multiple languages using various AI models. It supports markdown, markdown-jsx, and json files only. The action can be executed by individuals with write permissions to the repository, preventing API abuse by non-trusted parties. Users can set up the action by providing their API key and configuring the workflow settings. The tool allows users to create comments with specific commands to trigger translations and automatically generate pull requests or add translated files to existing pull requests. It supports multiple file translations and can interpret any language supported by GPT-4 or GPT-3.5.
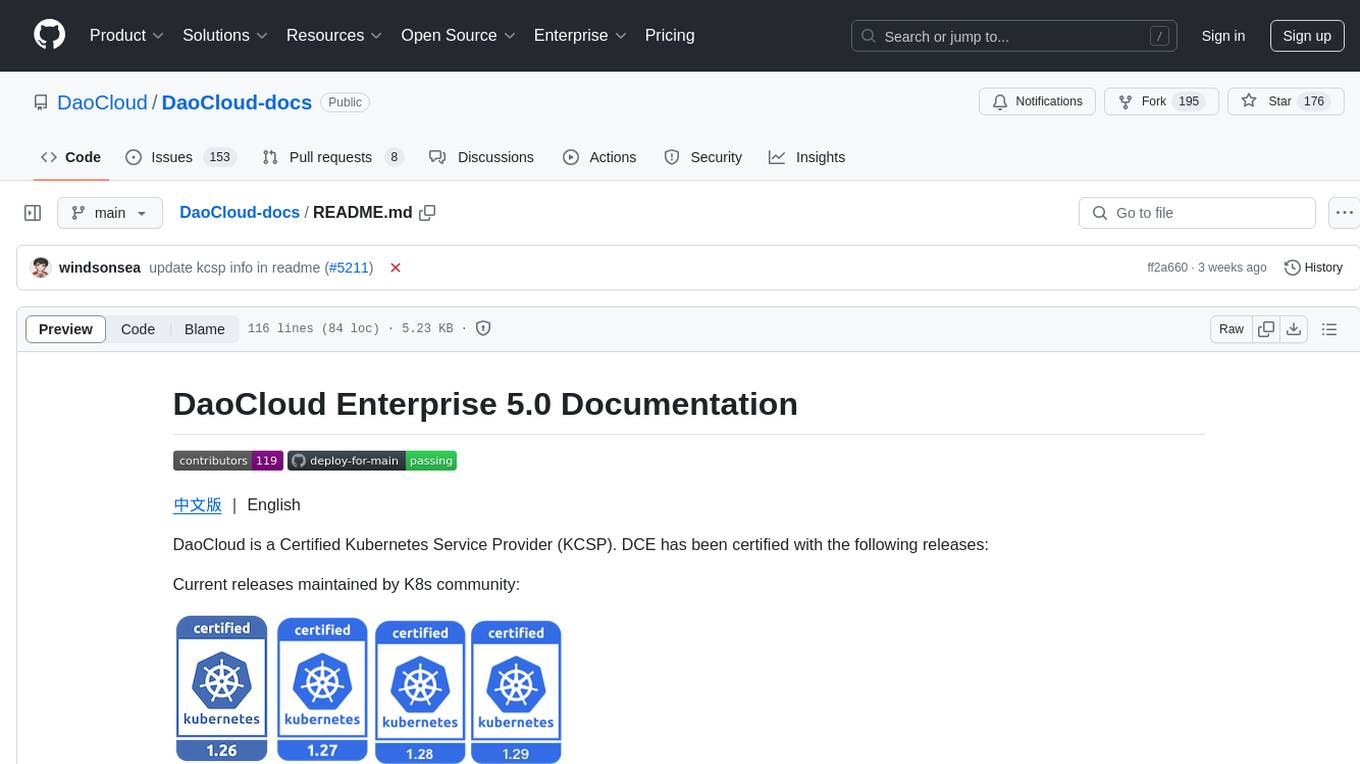
DaoCloud-docs
DaoCloud Enterprise 5.0 Documentation provides detailed information on using DaoCloud, a Certified Kubernetes Service Provider. The documentation covers current and legacy versions, workflow control using GitOps, and instructions for opening a PR and previewing changes locally. It also includes naming conventions, writing tips, references, and acknowledgments to contributors. Users can find guidelines on writing, contributing, and translating pages, along with using tools like MkDocs, Docker, and Poetry for managing the documentation.
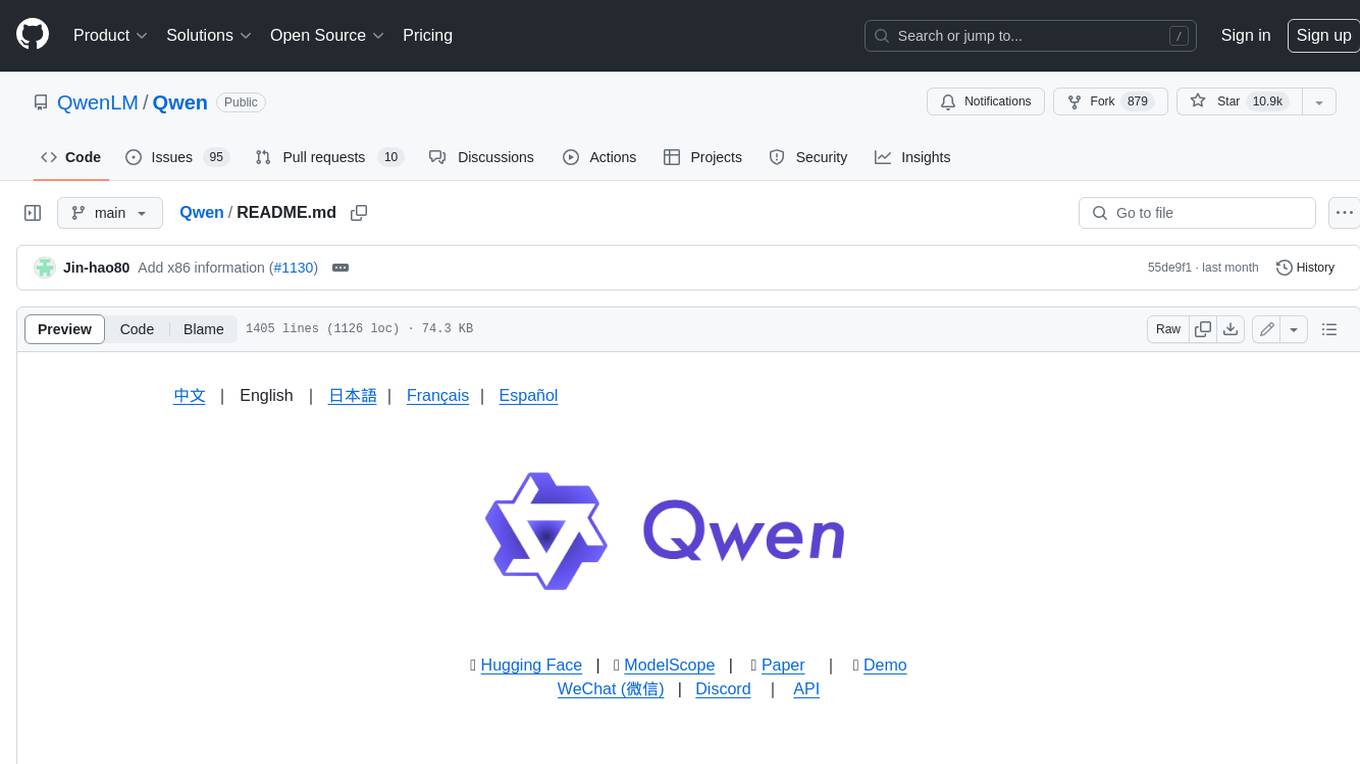
Qwen
Qwen is a series of large language models developed by Alibaba DAMO Academy. It outperforms the baseline models of similar model sizes on a series of benchmark datasets, e.g., MMLU, C-Eval, GSM8K, MATH, HumanEval, MBPP, BBH, etc., which evaluate the models’ capabilities on natural language understanding, mathematic problem solving, coding, etc. Qwen models outperform the baseline models of similar model sizes on a series of benchmark datasets, e.g., MMLU, C-Eval, GSM8K, MATH, HumanEval, MBPP, BBH, etc., which evaluate the models’ capabilities on natural language understanding, mathematic problem solving, coding, etc. Qwen-72B achieves better performance than LLaMA2-70B on all tasks and outperforms GPT-3.5 on 7 out of 10 tasks.
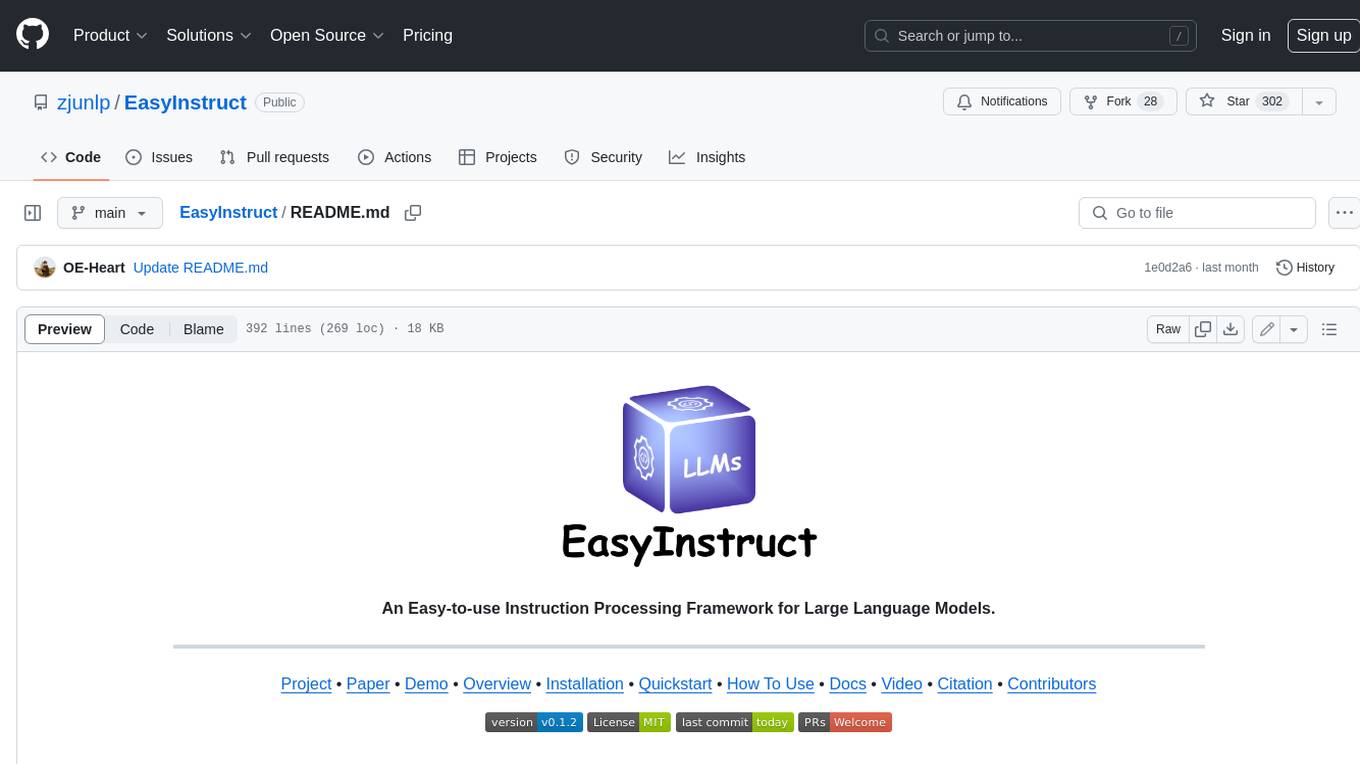
EasyInstruct
EasyInstruct is a Python package proposed as an easy-to-use instruction processing framework for Large Language Models (LLMs) like GPT-4, LLaMA, ChatGLM in your research experiments. EasyInstruct modularizes instruction generation, selection, and prompting, while also considering their combination and interaction.
For similar tasks
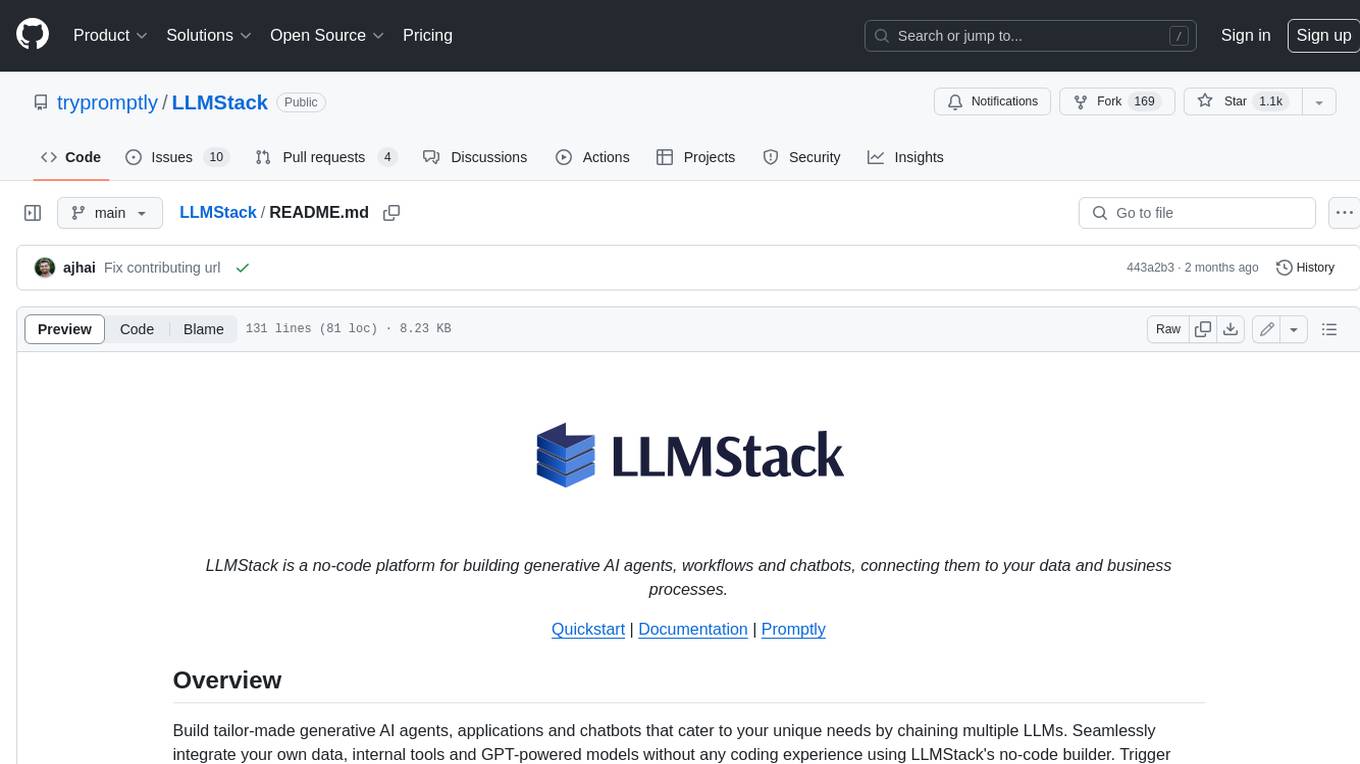
LLMStack
LLMStack is a no-code platform for building generative AI agents, workflows, and chatbots. It allows users to connect their own data, internal tools, and GPT-powered models without any coding experience. LLMStack can be deployed to the cloud or on-premise and can be accessed via HTTP API or triggered from Slack or Discord.
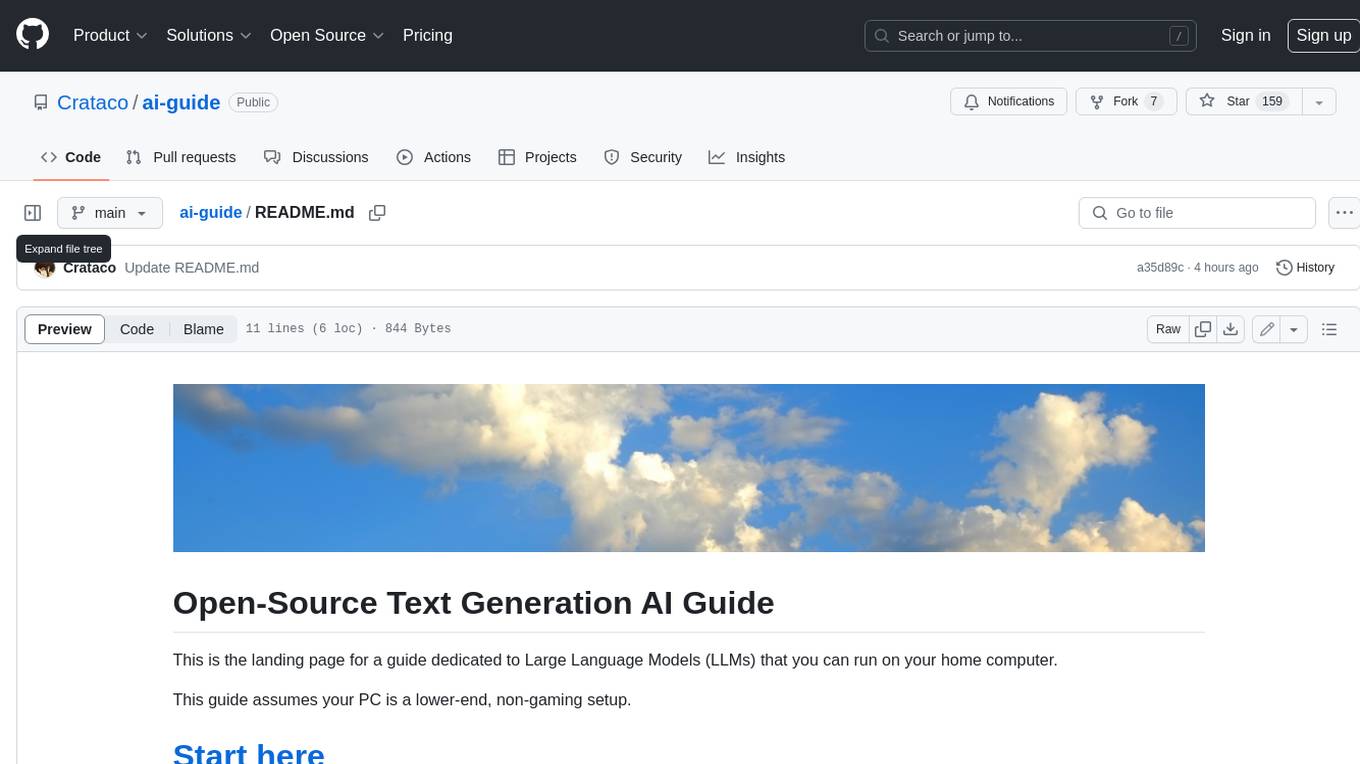
ai-guide
This guide is dedicated to Large Language Models (LLMs) that you can run on your home computer. It assumes your PC is a lower-end, non-gaming setup.
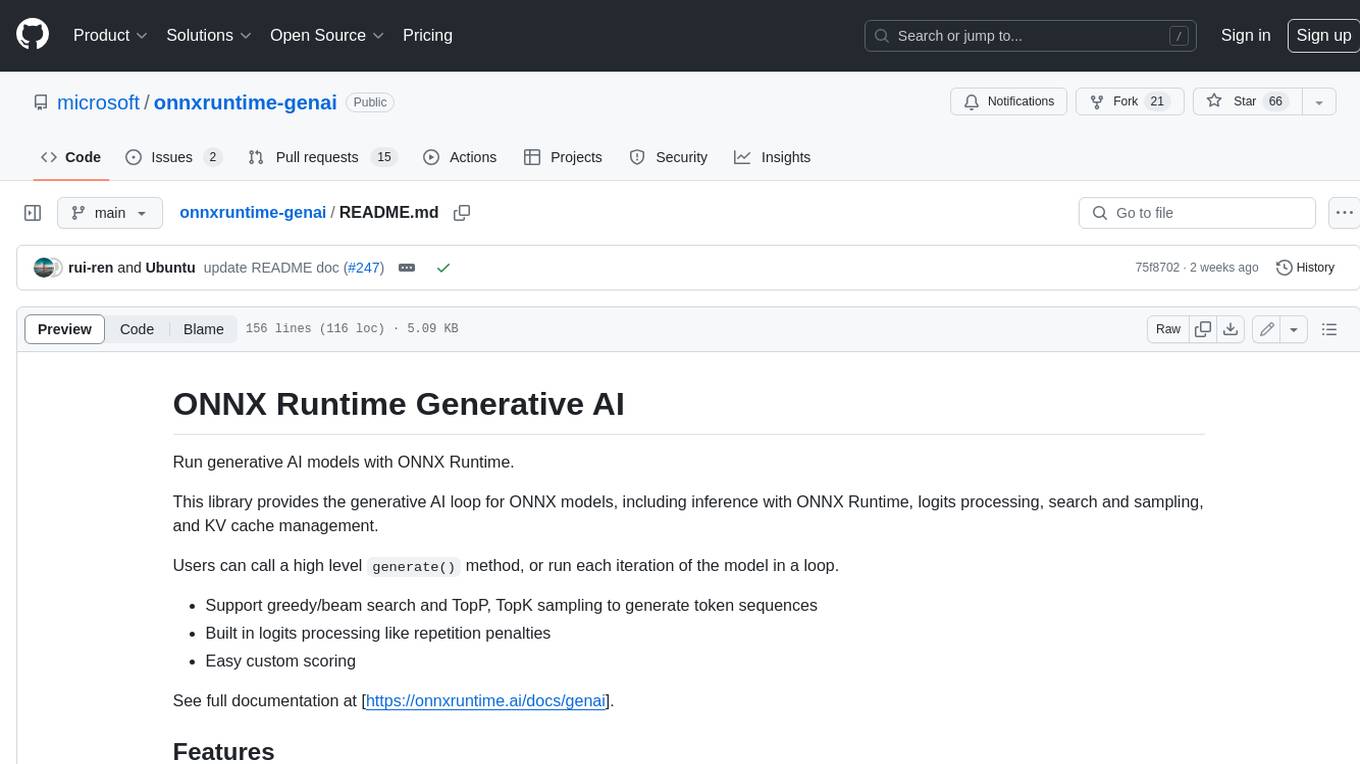
onnxruntime-genai
ONNX Runtime Generative AI is a library that provides the generative AI loop for ONNX models, including inference with ONNX Runtime, logits processing, search and sampling, and KV cache management. Users can call a high level `generate()` method, or run each iteration of the model in a loop. It supports greedy/beam search and TopP, TopK sampling to generate token sequences, has built in logits processing like repetition penalties, and allows for easy custom scoring.
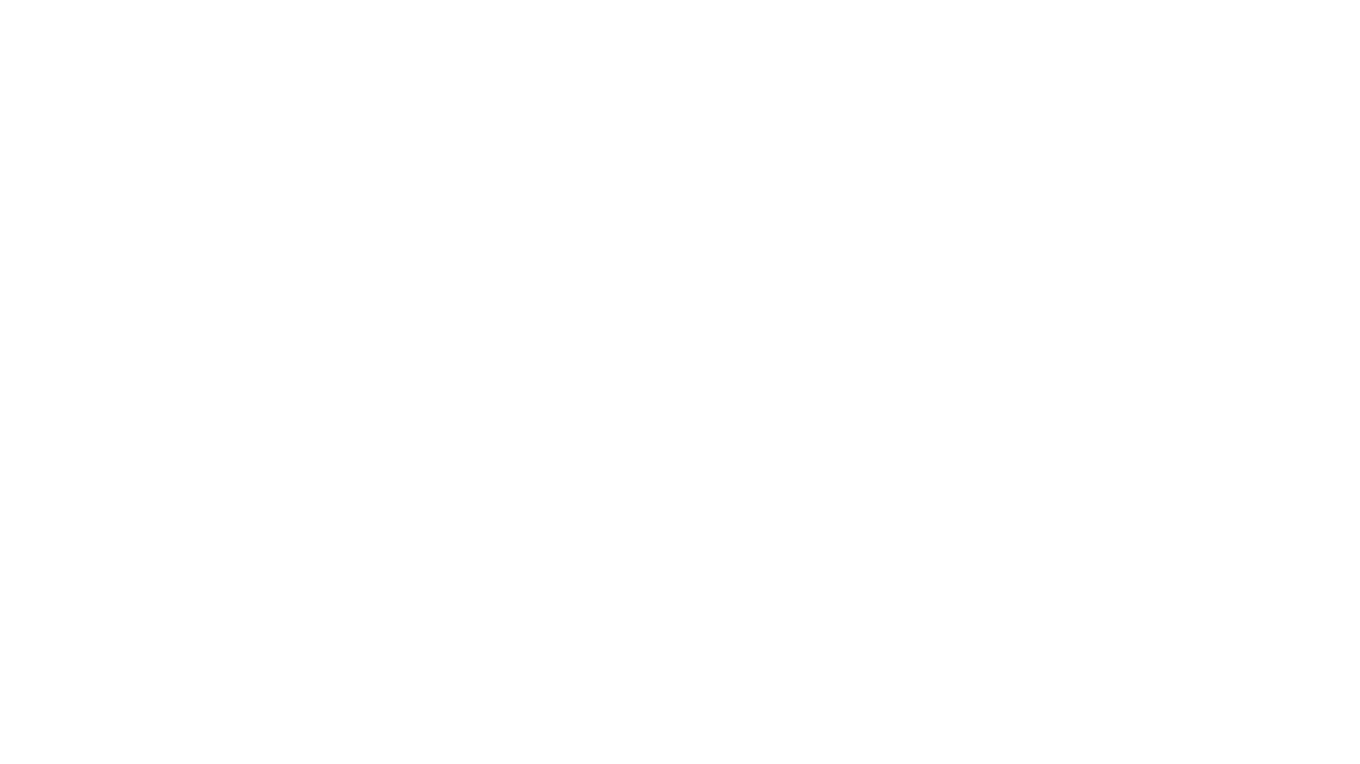
jupyter-ai
Jupyter AI connects generative AI with Jupyter notebooks. It provides a user-friendly and powerful way to explore generative AI models in notebooks and improve your productivity in JupyterLab and the Jupyter Notebook. Specifically, Jupyter AI offers: * An `%%ai` magic that turns the Jupyter notebook into a reproducible generative AI playground. This works anywhere the IPython kernel runs (JupyterLab, Jupyter Notebook, Google Colab, Kaggle, VSCode, etc.). * A native chat UI in JupyterLab that enables you to work with generative AI as a conversational assistant. * Support for a wide range of generative model providers, including AI21, Anthropic, AWS, Cohere, Gemini, Hugging Face, NVIDIA, and OpenAI. * Local model support through GPT4All, enabling use of generative AI models on consumer grade machines with ease and privacy.
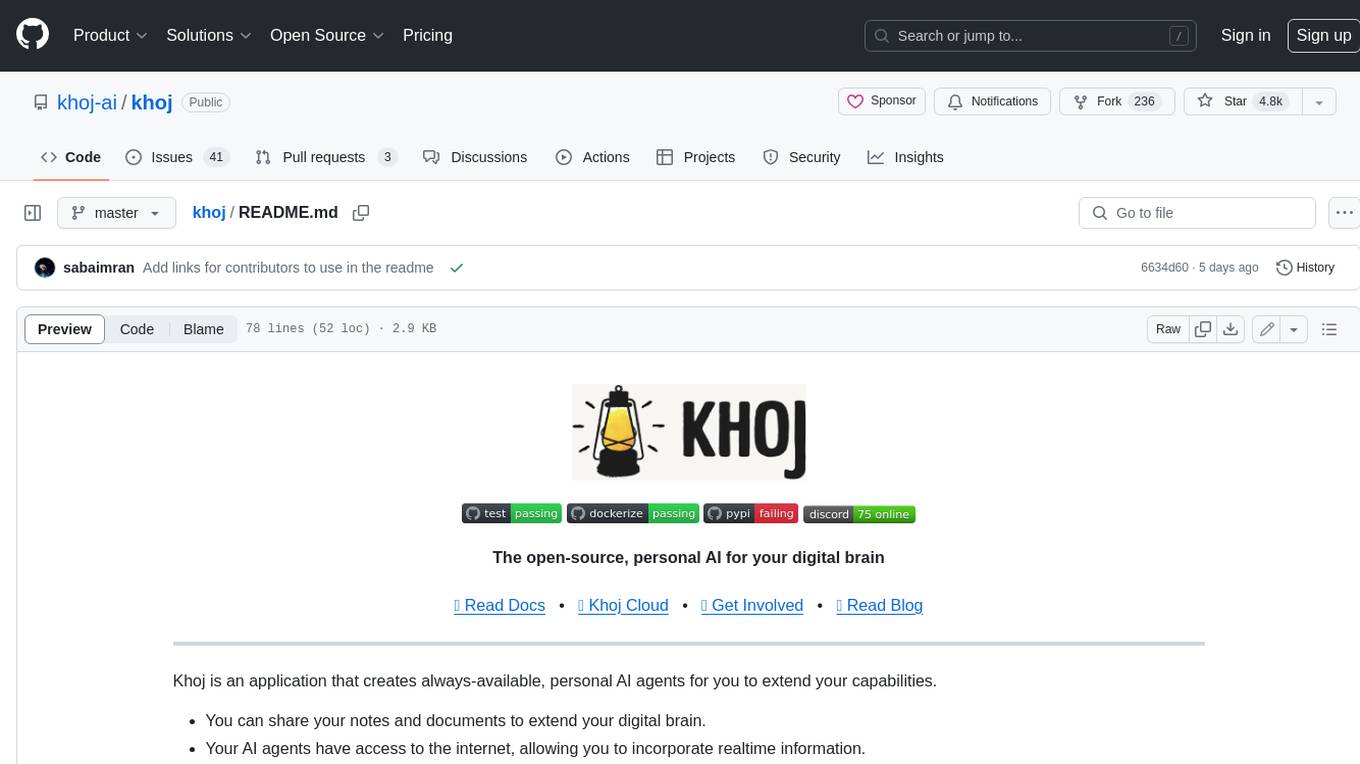
khoj
Khoj is an open-source, personal AI assistant that extends your capabilities by creating always-available AI agents. You can share your notes and documents to extend your digital brain, and your AI agents have access to the internet, allowing you to incorporate real-time information. Khoj is accessible on Desktop, Emacs, Obsidian, Web, and Whatsapp, and you can share PDF, markdown, org-mode, notion files, and GitHub repositories. You'll get fast, accurate semantic search on top of your docs, and your agents can create deeply personal images and understand your speech. Khoj is self-hostable and always will be.
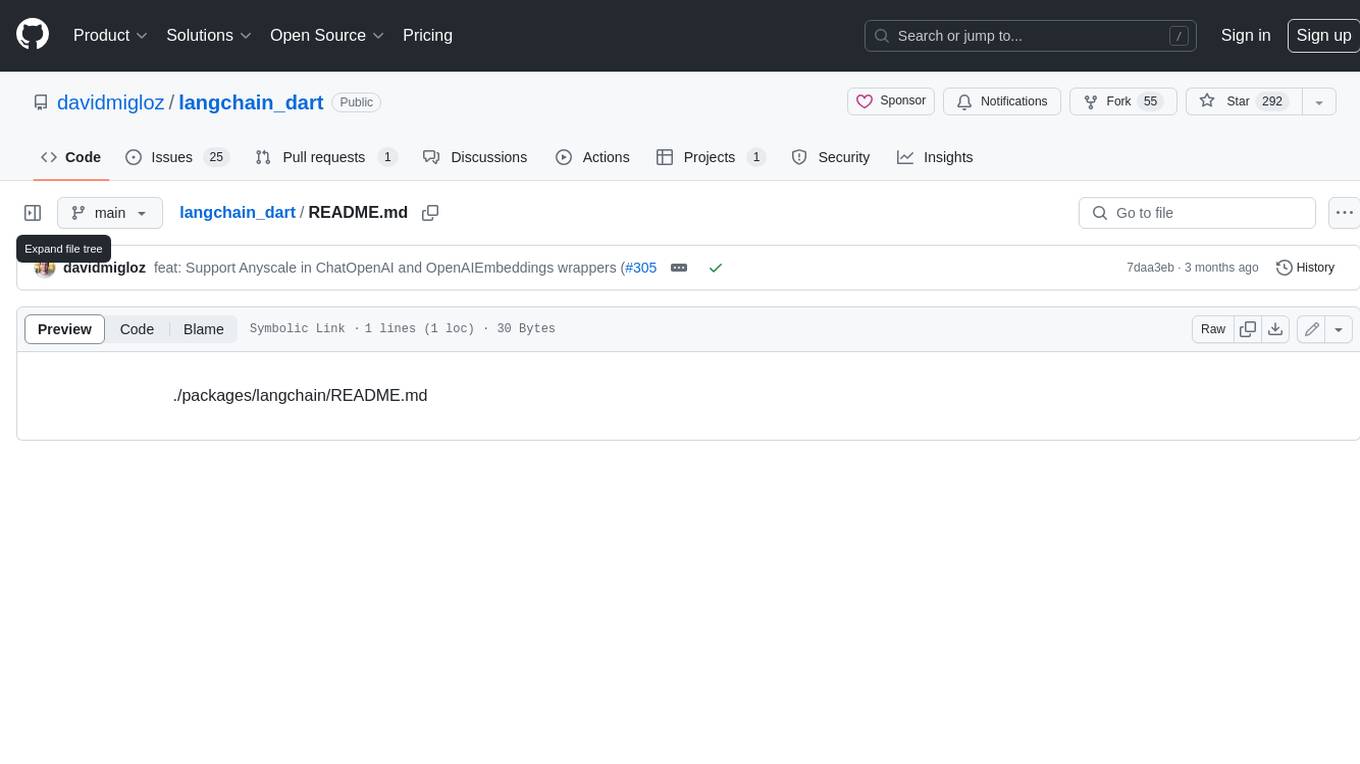
langchain_dart
LangChain.dart is a Dart port of the popular LangChain Python framework created by Harrison Chase. LangChain provides a set of ready-to-use components for working with language models and a standard interface for chaining them together to formulate more advanced use cases (e.g. chatbots, Q&A with RAG, agents, summarization, extraction, etc.). The components can be grouped into a few core modules: * **Model I/O:** LangChain offers a unified API for interacting with various LLM providers (e.g. OpenAI, Google, Mistral, Ollama, etc.), allowing developers to switch between them with ease. Additionally, it provides tools for managing model inputs (prompt templates and example selectors) and parsing the resulting model outputs (output parsers). * **Retrieval:** assists in loading user data (via document loaders), transforming it (with text splitters), extracting its meaning (using embedding models), storing (in vector stores) and retrieving it (through retrievers) so that it can be used to ground the model's responses (i.e. Retrieval-Augmented Generation or RAG). * **Agents:** "bots" that leverage LLMs to make informed decisions about which available tools (such as web search, calculators, database lookup, etc.) to use to accomplish the designated task. The different components can be composed together using the LangChain Expression Language (LCEL).
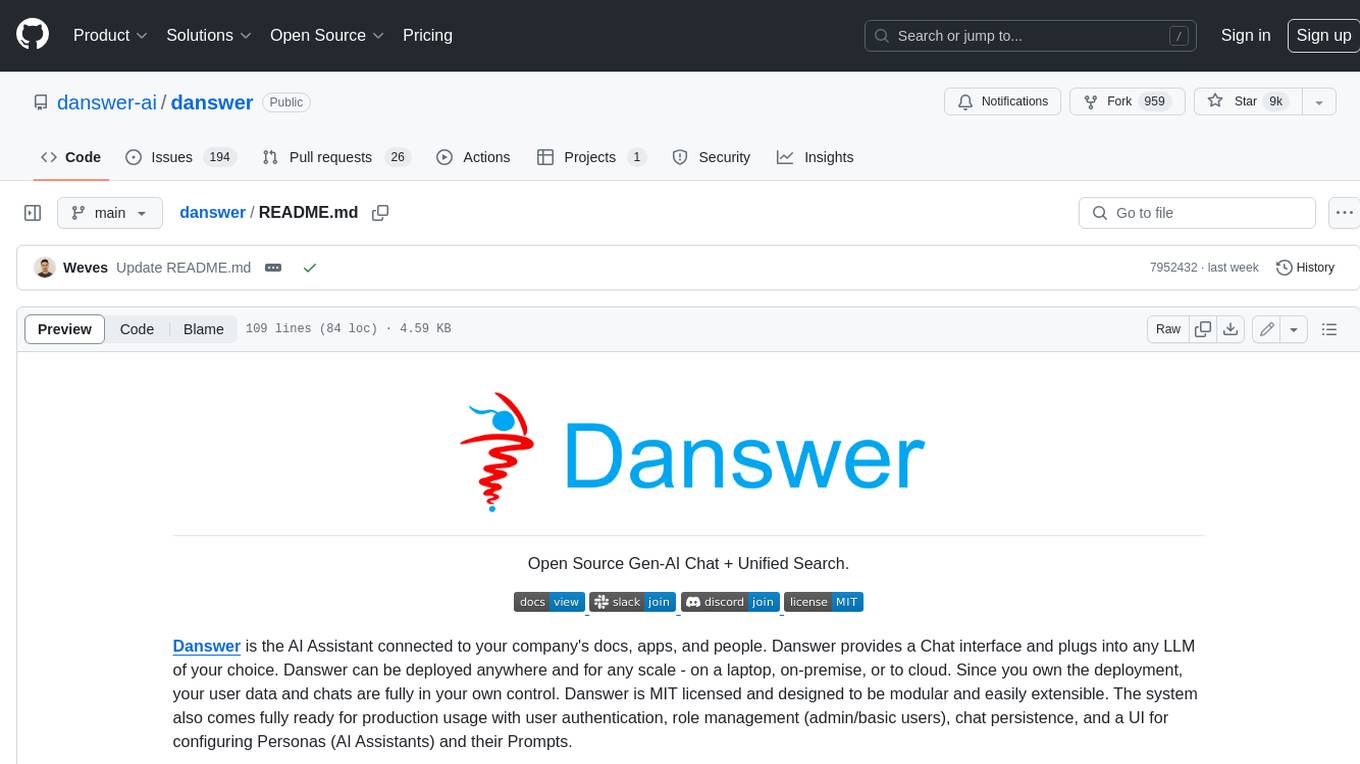
danswer
Danswer is an open-source Gen-AI Chat and Unified Search tool that connects to your company's docs, apps, and people. It provides a Chat interface and plugs into any LLM of your choice. Danswer can be deployed anywhere and for any scale - on a laptop, on-premise, or to cloud. Since you own the deployment, your user data and chats are fully in your own control. Danswer is MIT licensed and designed to be modular and easily extensible. The system also comes fully ready for production usage with user authentication, role management (admin/basic users), chat persistence, and a UI for configuring Personas (AI Assistants) and their Prompts. Danswer also serves as a Unified Search across all common workplace tools such as Slack, Google Drive, Confluence, etc. By combining LLMs and team specific knowledge, Danswer becomes a subject matter expert for the team. Imagine ChatGPT if it had access to your team's unique knowledge! It enables questions such as "A customer wants feature X, is this already supported?" or "Where's the pull request for feature Y?"
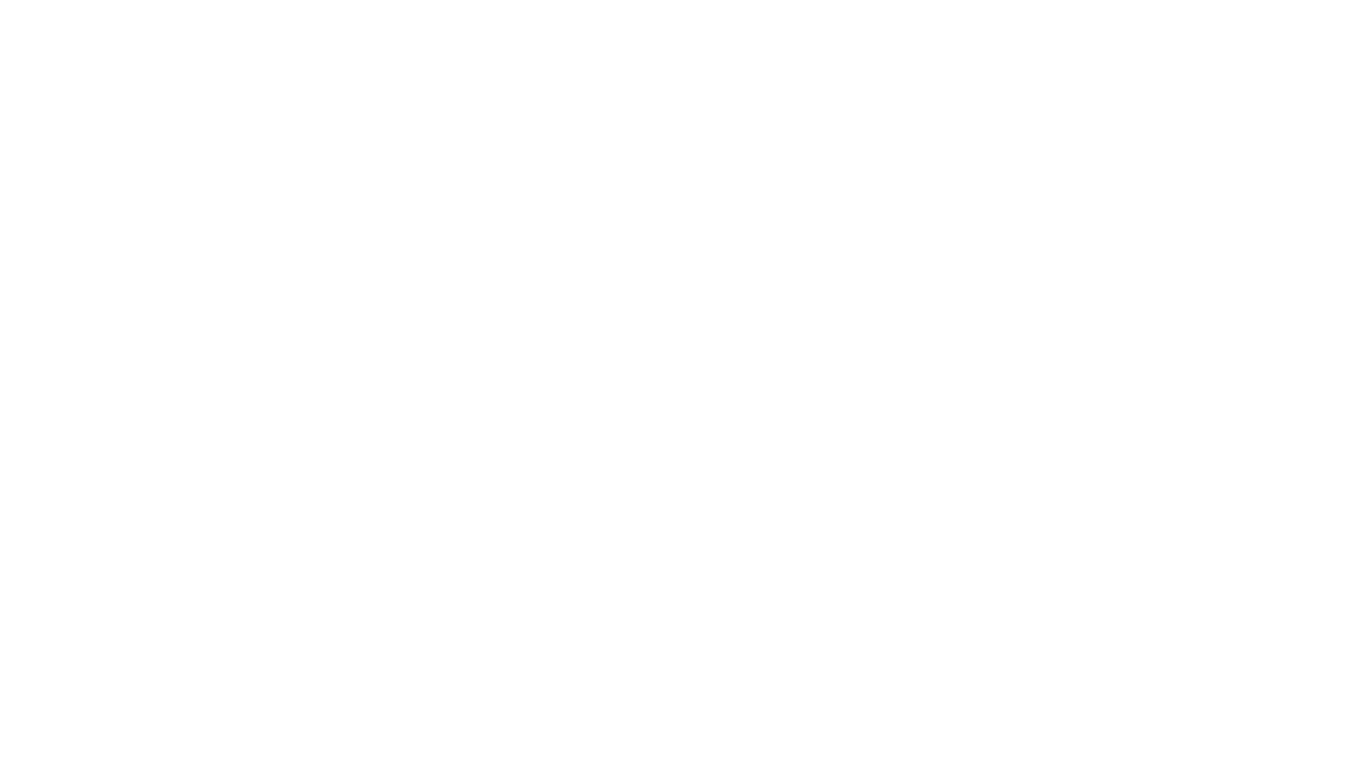
infinity
Infinity is an AI-native database designed for LLM applications, providing incredibly fast full-text and vector search capabilities. It supports a wide range of data types, including vectors, full-text, and structured data, and offers a fused search feature that combines multiple embeddings and full text. Infinity is easy to use, with an intuitive Python API and a single-binary architecture that simplifies deployment. It achieves high performance, with 0.1 milliseconds query latency on million-scale vector datasets and up to 15K QPS.
For similar jobs
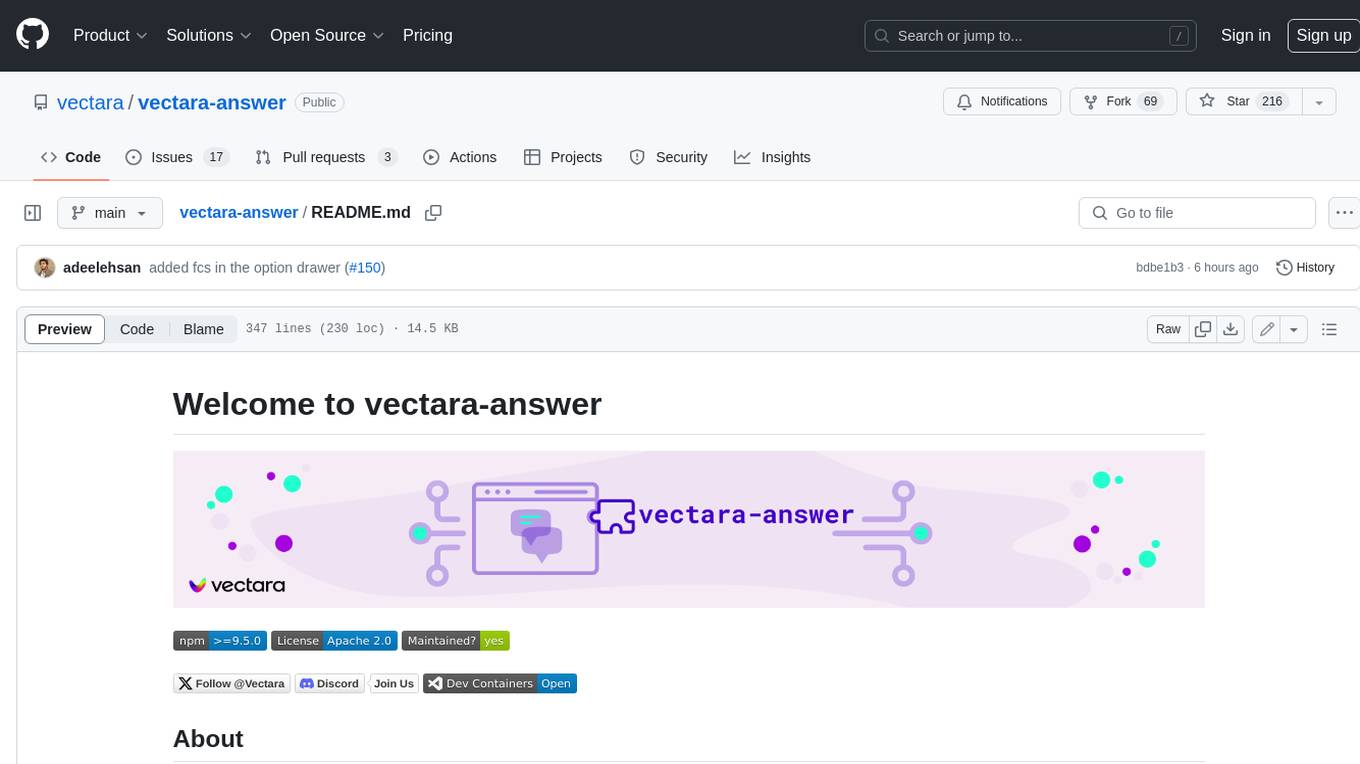
vectara-answer
Vectara Answer is a sample app for Vectara-powered Summarized Semantic Search (or question-answering) with advanced configuration options. For examples of what you can build with Vectara Answer, check out Ask News, LegalAid, or any of the other demo applications.
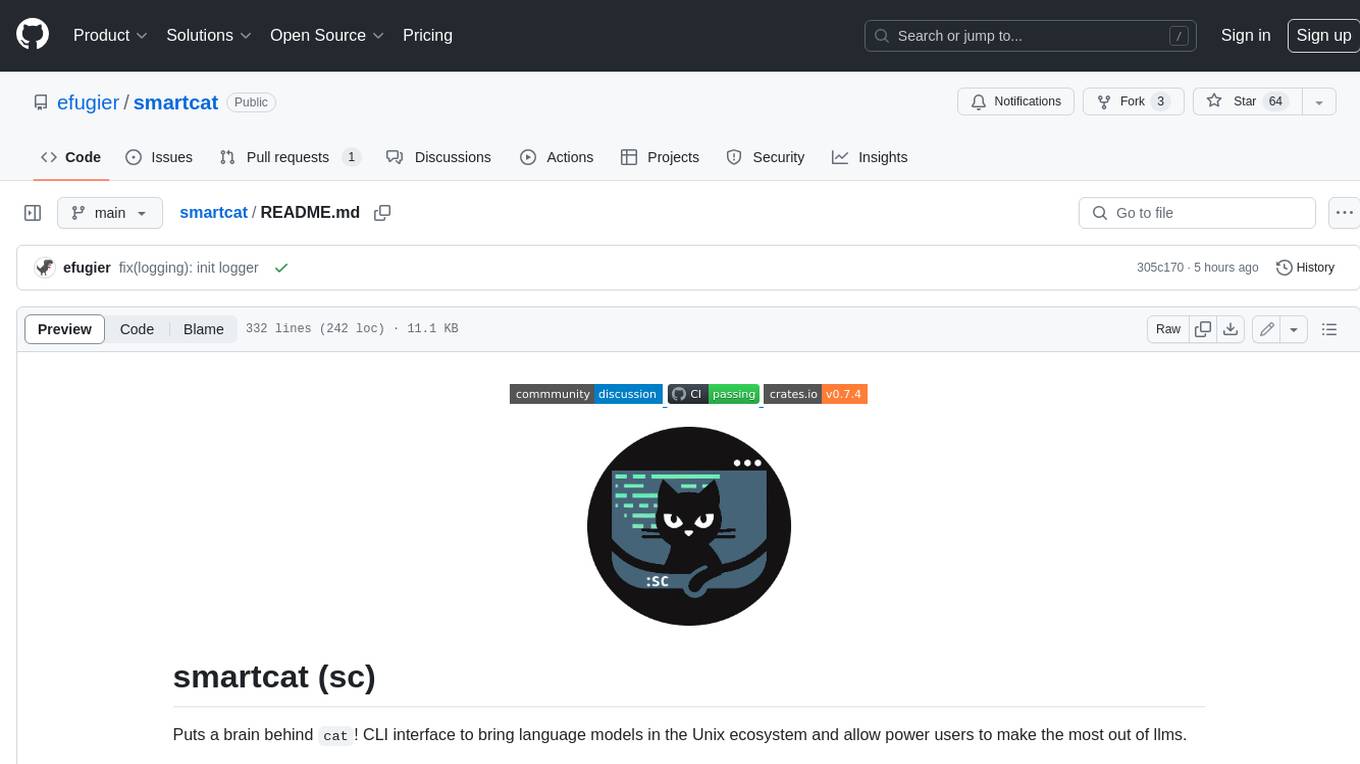
smartcat
Smartcat is a CLI interface that brings language models into the Unix ecosystem, allowing power users to leverage the capabilities of LLMs in their daily workflows. It features a minimalist design, seamless integration with terminal and editor workflows, and customizable prompts for specific tasks. Smartcat currently supports OpenAI, Mistral AI, and Anthropic APIs, providing access to a range of language models. With its ability to manipulate file and text streams, integrate with editors, and offer configurable settings, Smartcat empowers users to automate tasks, enhance code quality, and explore creative possibilities.
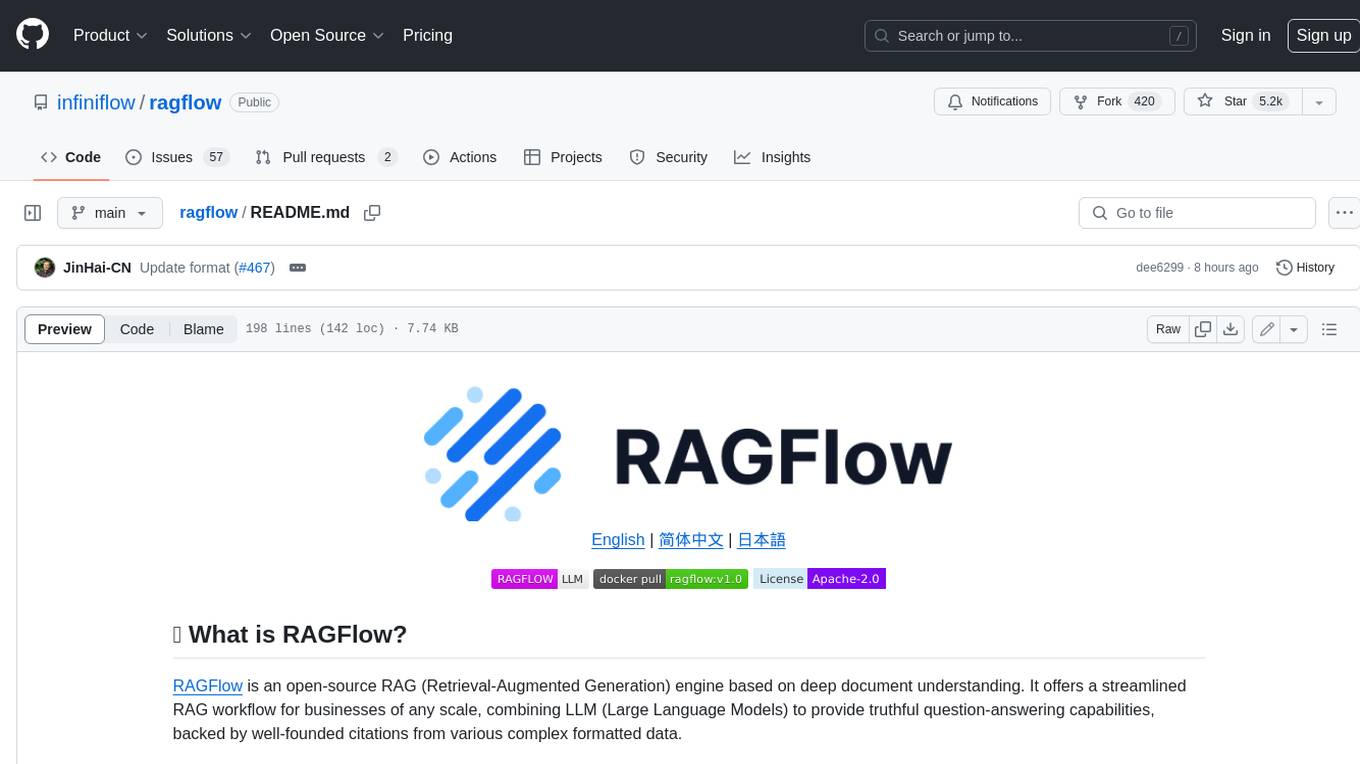
ragflow
RAGFlow is an open-source Retrieval-Augmented Generation (RAG) engine that combines deep document understanding with Large Language Models (LLMs) to provide accurate question-answering capabilities. It offers a streamlined RAG workflow for businesses of all sizes, enabling them to extract knowledge from unstructured data in various formats, including Word documents, slides, Excel files, images, and more. RAGFlow's key features include deep document understanding, template-based chunking, grounded citations with reduced hallucinations, compatibility with heterogeneous data sources, and an automated and effortless RAG workflow. It supports multiple recall paired with fused re-ranking, configurable LLMs and embedding models, and intuitive APIs for seamless integration with business applications.
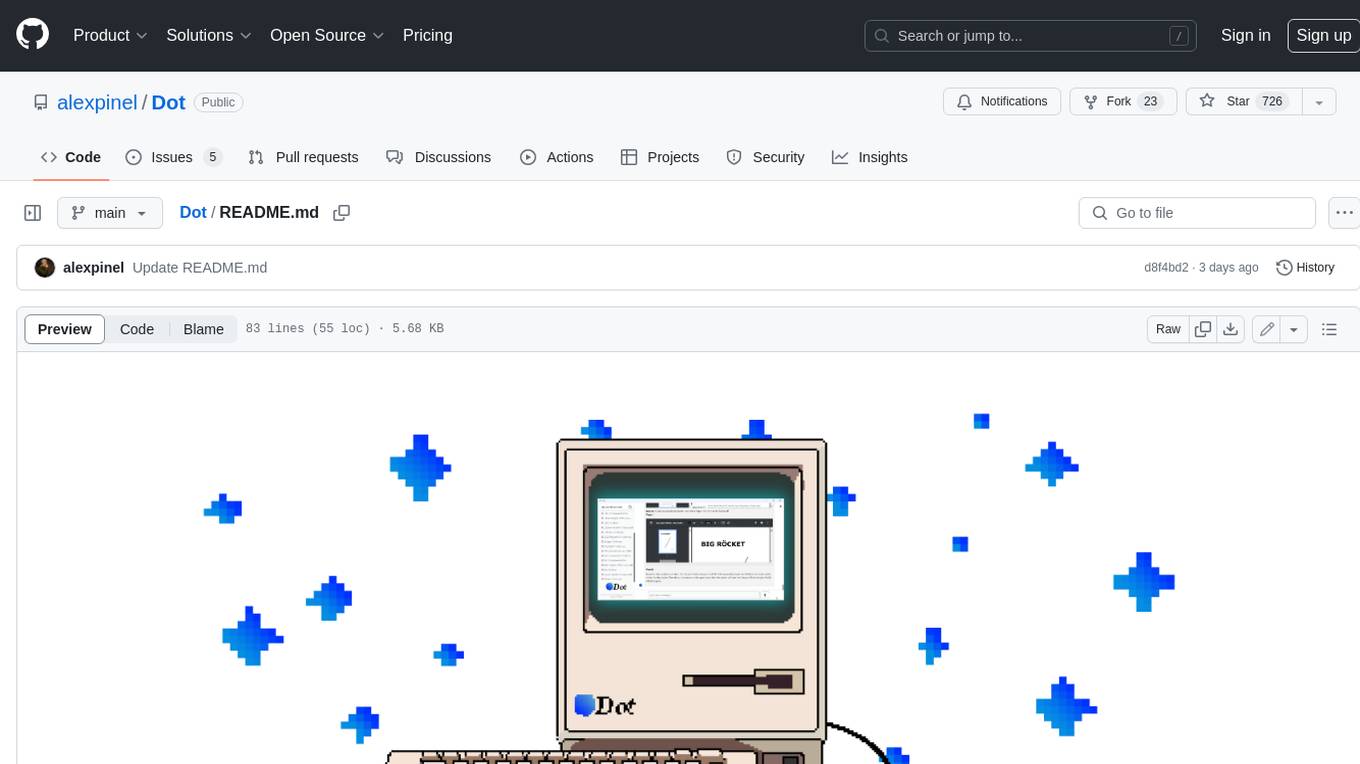
Dot
Dot is a standalone, open-source application designed for seamless interaction with documents and files using local LLMs and Retrieval Augmented Generation (RAG). It is inspired by solutions like Nvidia's Chat with RTX, providing a user-friendly interface for those without a programming background. Pre-packaged with Mistral 7B, Dot ensures accessibility and simplicity right out of the box. Dot allows you to load multiple documents into an LLM and interact with them in a fully local environment. Supported document types include PDF, DOCX, PPTX, XLSX, and Markdown. Users can also engage with Big Dot for inquiries not directly related to their documents, similar to interacting with ChatGPT. Built with Electron JS, Dot encapsulates a comprehensive Python environment that includes all necessary libraries. The application leverages libraries such as FAISS for creating local vector stores, Langchain, llama.cpp & Huggingface for setting up conversation chains, and additional tools for document management and interaction.
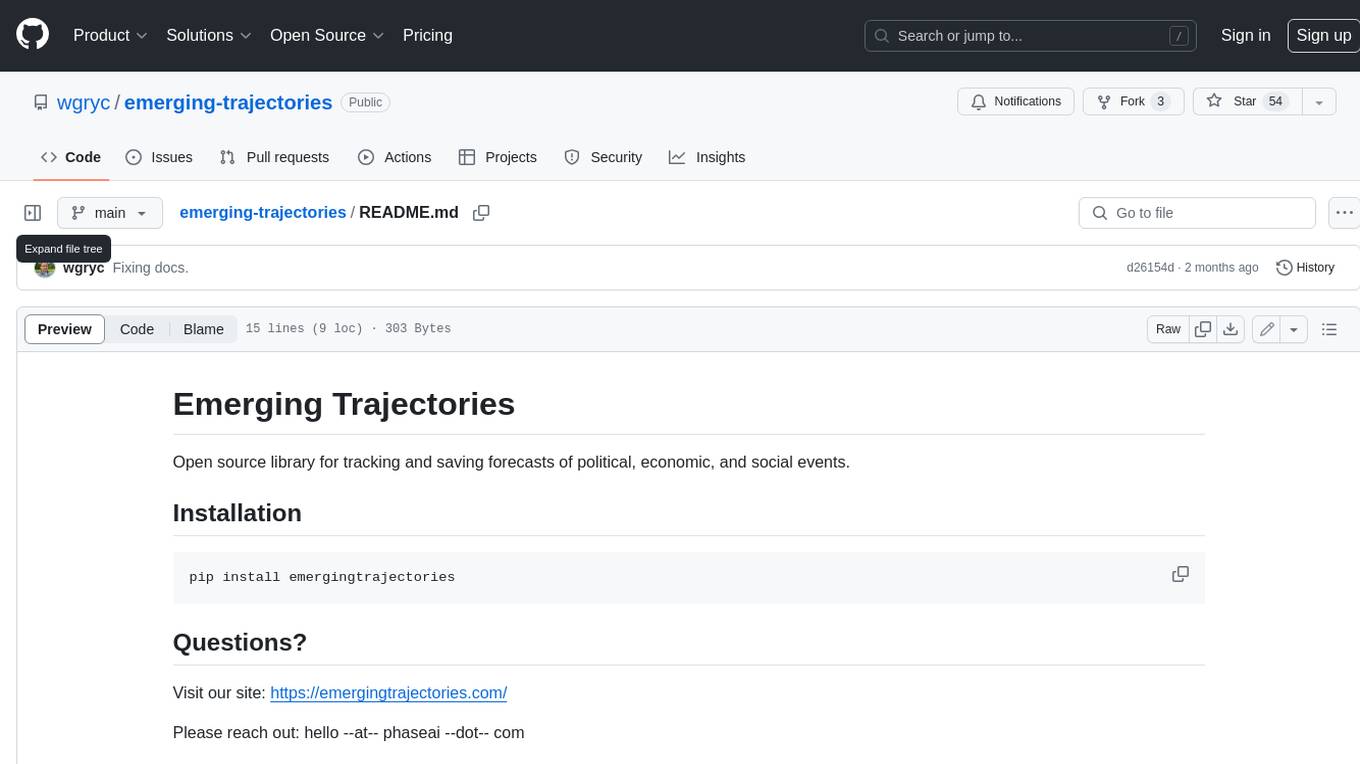
emerging-trajectories
Emerging Trajectories is an open source library for tracking and saving forecasts of political, economic, and social events. It provides a way to organize and store forecasts, as well as track their accuracy over time. This can be useful for researchers, analysts, and anyone else who wants to keep track of their predictions.
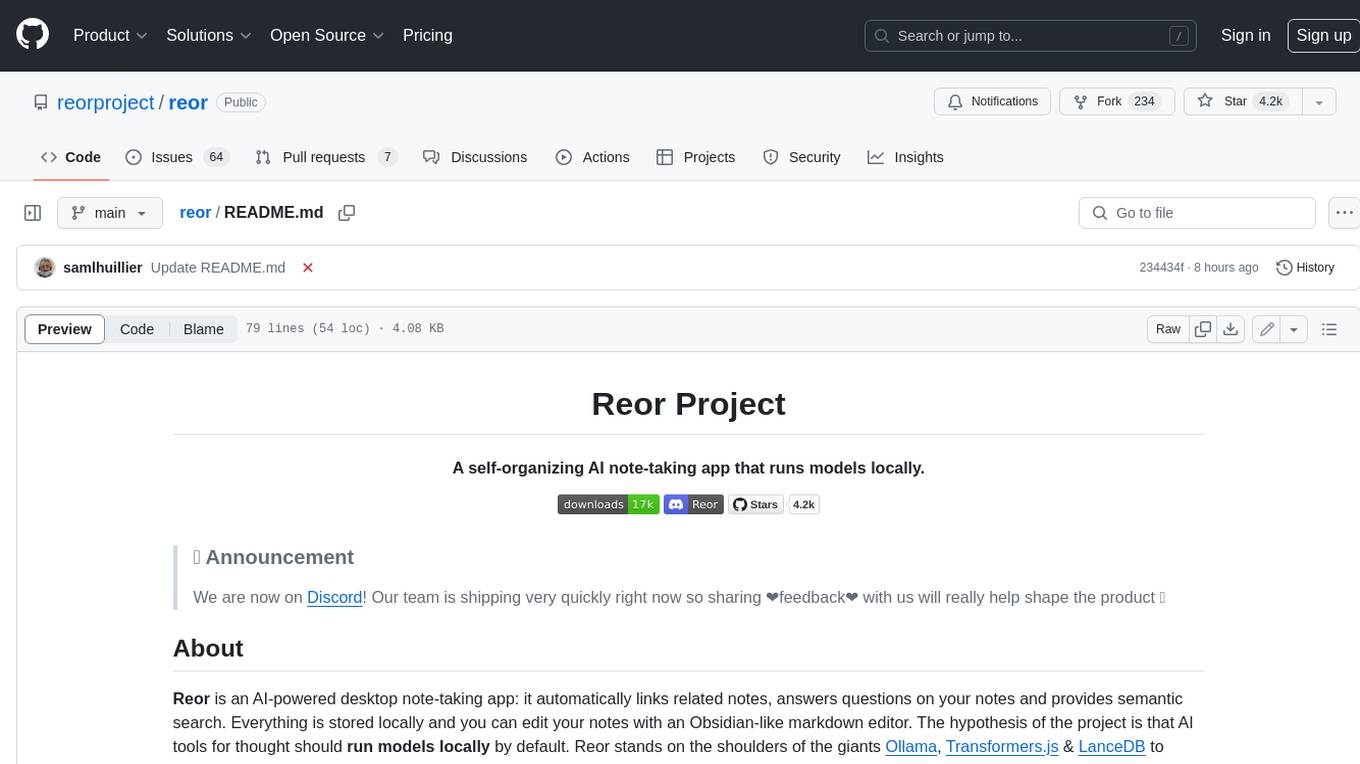
reor
Reor is an AI-powered desktop note-taking app that automatically links related notes, answers questions on your notes, and provides semantic search. Everything is stored locally and you can edit your notes with an Obsidian-like markdown editor. The hypothesis of the project is that AI tools for thought should run models locally by default. Reor stands on the shoulders of the giants Ollama, Transformers.js & LanceDB to enable both LLMs and embedding models to run locally. Connecting to OpenAI or OpenAI-compatible APIs like Oobabooga is also supported.
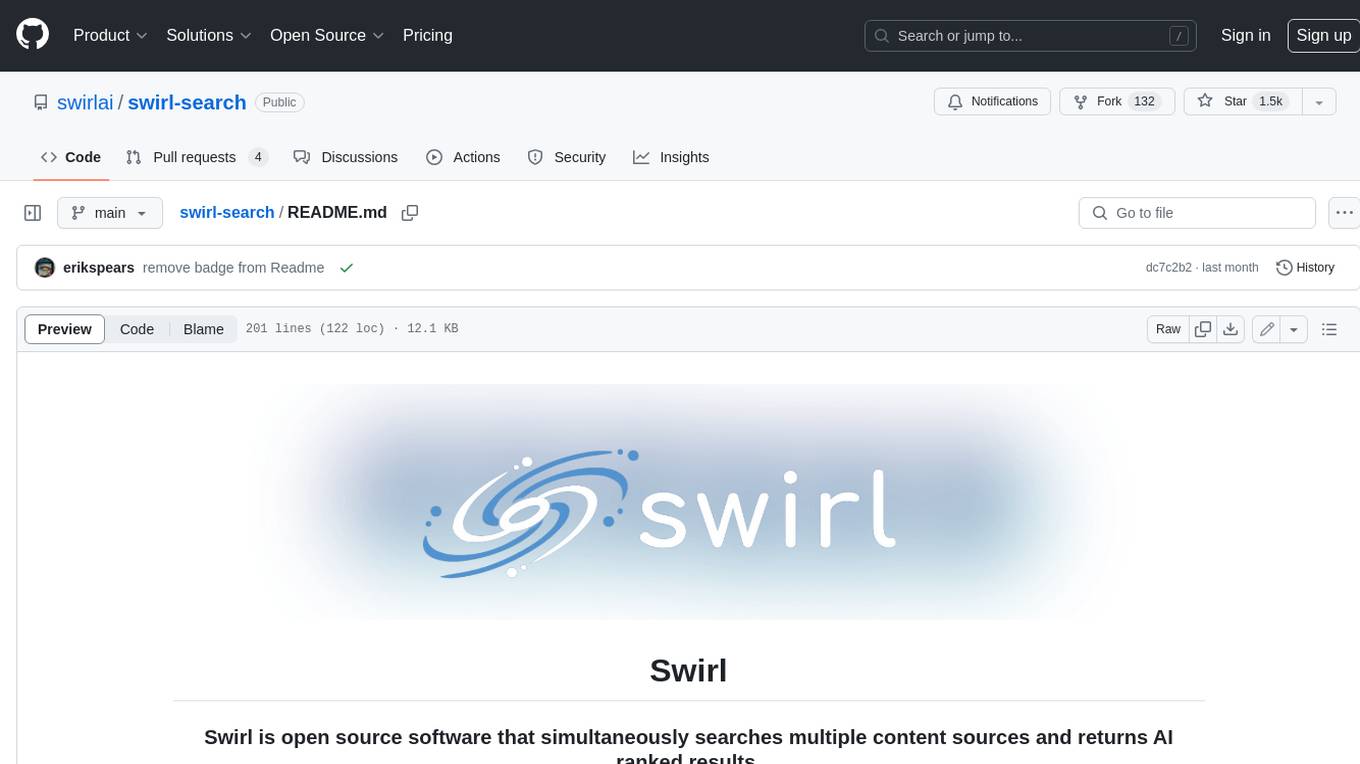
swirl-search
Swirl is an open-source software that allows users to simultaneously search multiple content sources and receive AI-ranked results. It connects to various data sources, including databases, public data services, and enterprise sources, and utilizes AI and LLMs to generate insights and answers based on the user's data. Swirl is easy to use, requiring only the download of a YML file, starting in Docker, and searching with Swirl. Users can add credentials to preloaded SearchProviders to access more sources. Swirl also offers integration with ChatGPT as a configured AI model. It adapts and distributes user queries to anything with a search API, re-ranking the unified results using Large Language Models without extracting or indexing anything. Swirl includes five Google Programmable Search Engines (PSEs) to get users up and running quickly. Key features of Swirl include Microsoft 365 integration, SearchProvider configurations, query adaptation, synchronous or asynchronous search federation, optional subscribe feature, pipelining of Processor stages, results stored in SQLite3 or PostgreSQL, built-in Query Transformation support, matching on word stems and handling of stopwords, duplicate detection, re-ranking of unified results using Cosine Vector Similarity, result mixers, page through all results requested, sample data sets, optional spell correction, optional search/result expiration service, easily extensible Connector and Mixer objects, and a welcoming community for collaboration and support.
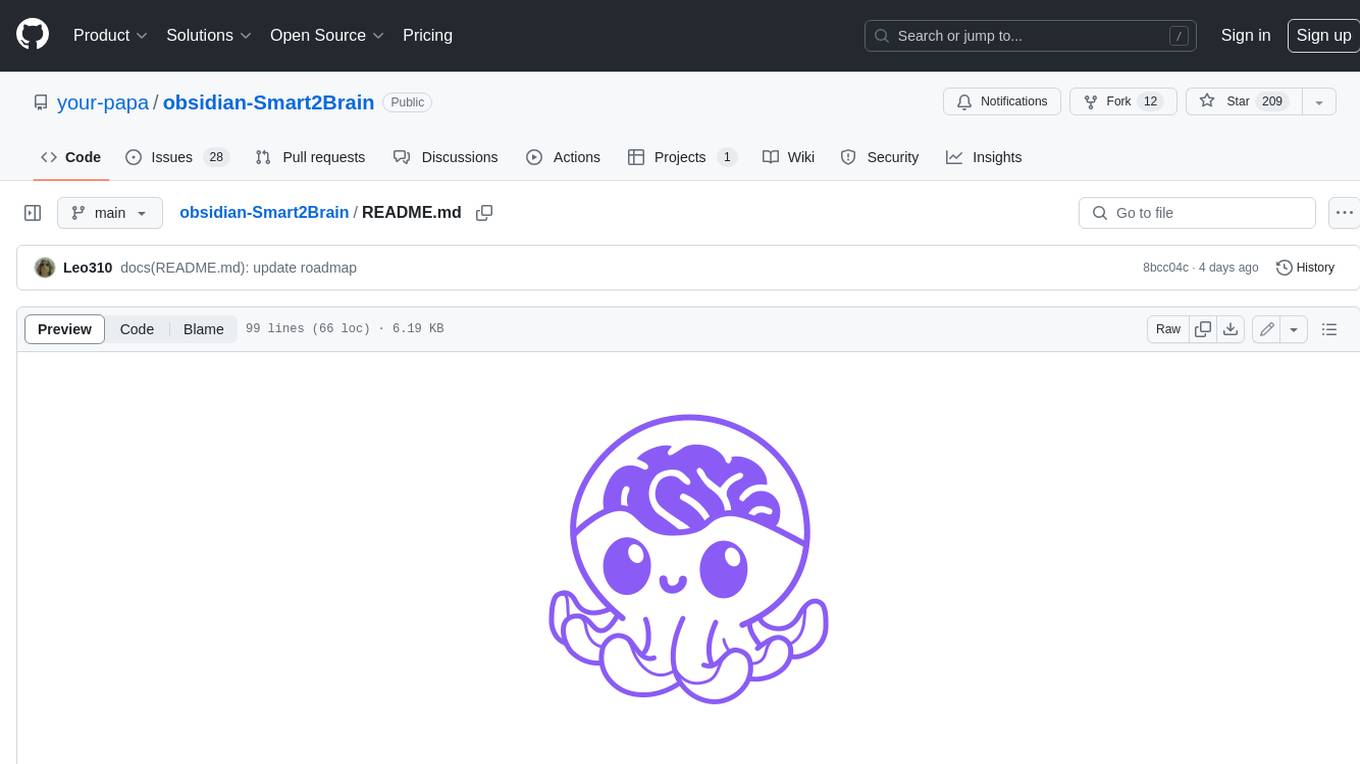
obsidian-Smart2Brain
Your Smart Second Brain is a free and open-source Obsidian plugin that serves as your personal assistant, powered by large language models like ChatGPT or Llama2. It can directly access and process your notes, eliminating the need for manual prompt editing, and it can operate completely offline, ensuring your data remains private and secure.