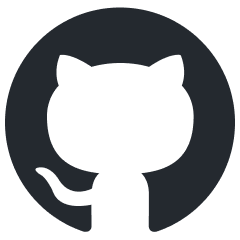
foundations-of-gen-ai
Transformer Architectures for Generative AI
Stars: 74
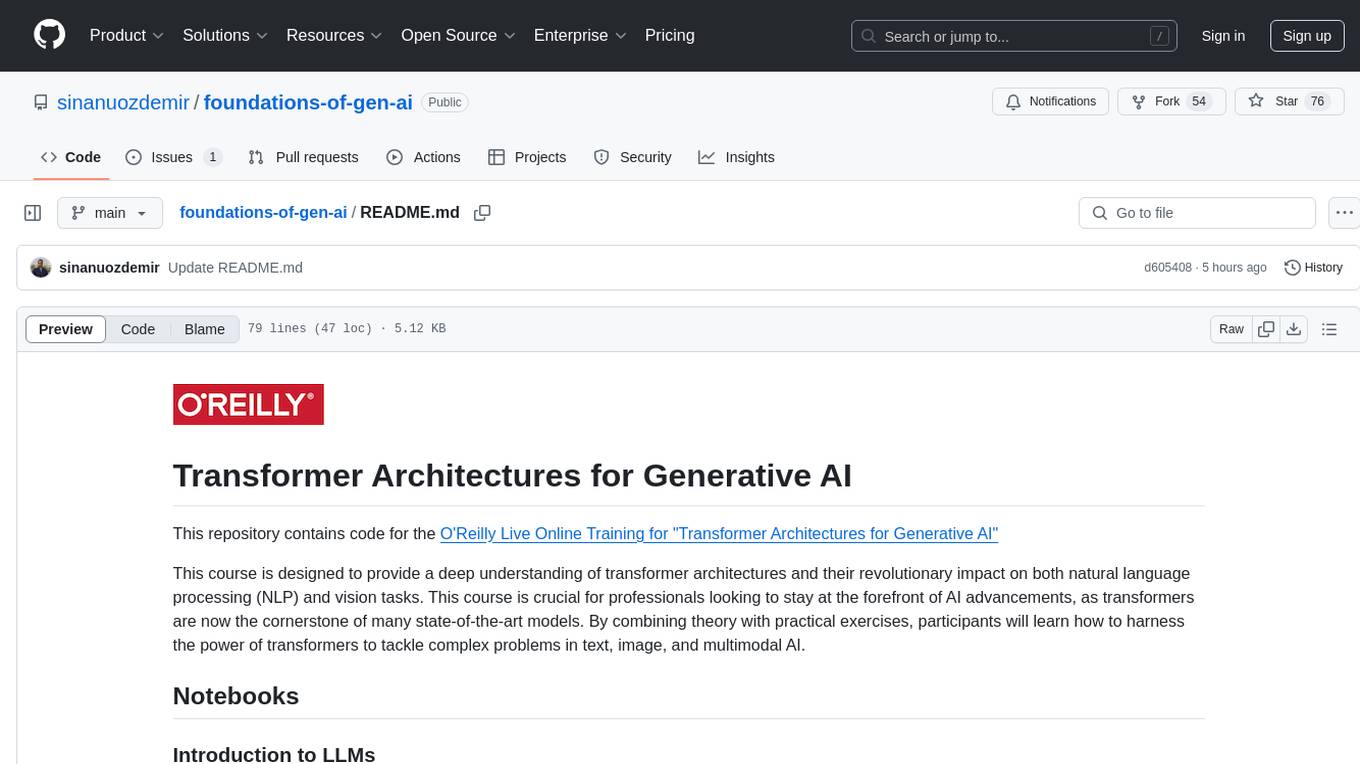
This repository contains code for the O'Reilly Live Online Training for 'Transformer Architectures for Generative AI'. The course provides a deep understanding of transformer architectures and their impact on natural language processing (NLP) and vision tasks. Participants learn to harness transformers to tackle problems in text, image, and multimodal AI through theory and practical exercises.
README:
This repository contains code for the O'Reilly Live Online Training for "Transformer Architectures for Generative AI"
This course is designed to provide a deep understanding of transformer architectures and their revolutionary impact on both natural language processing (NLP) and vision tasks. This course is crucial for professionals looking to stay at the forefront of AI advancements, as transformers are now the cornerstone of many state-of-the-art models. By combining theory with practical exercises, participants will learn how to harness the power of transformers to tackle complex problems in text, image, and multimodal AI.
- BERT - the beginnings of LLMs
- T5 - the beginnings of instructional alignment
- GPT - How LLMs learned to talk
- Multimodal LLMs
- [Inspecting LLM token embeddings](notebooks/LLM Embeddings.ipynb) - Explore how different attention mechanisms lead to different token embeddings
LLM Embedding
- Rivaling OpenAI embeddings with fine-tuning - Fine-tune Embeddings with Synthetic Data
LLM Classification
-
bert_app_review.ipynb
: Fine-tuning a BERT model for app review classification. -
openai_app_review_fine_tuning.ipynb
: Fine-tuning OpenAI models for app review classification.
Multimodal
-
Stock Image Search - Using a CLIP model to build an image search system
-
Visual Q/A
-
constructing_a_vqa_system.ipynb
: Step-by-step guide to constructing a Visual Question Answering (VQA) system using GPT-2 and Vision Transformer.-
using_our_vqa.ipynb
: Using the VQA system built in the previous notebook.
-
-
SAWYER - Instructional Fine-tuning
-
SAWYER_LLAMA_SFT.ipynb
: Fine-tuning the Llama-3 model to create the SAWYER bot. -
SAWYER_Reward_Model.ipynb
: Training a reward model from human preferences for the SAWYER bot. -
SAWYER_RLF.ipynb
: Applying Reinforcement Learning from Human Feedback (RLHF) to align the SAWYER bot. -
SAWYER_USE_SAWYER.ipynb
: Using the SAWYER bot.
Distillation / Speculative Decoding / Caching
-
Go Emotion Distillation: Exploring knowledge distillation techniques for transformer models.
-
Speculative Decoding - Using an assistant model to aid token decoding
-
Prompt Caching Llama 3 - Replicating prompt caching with HuggingFace tools
Agents / RAG
- RAG Retrieval: An introduction to vector databases, embeddings, and retrieval
- Evaluating Tool Selection - Calculating the accuracy of tool selection between different LLMs and quantifying the positional bias present in auto-regressive LLMs
Probing
-
There are over a dozen notebooks for the birth year/death year probing example so I will only share a few key ones here:
Sinan Ozdemir is founder and CTO of LoopGenius, where he uses state-of-the-art AI to help people create and run their businesses. He has lectured in data science at Johns Hopkins University and authored multiple books, videos and numerous online courses on data science, machine learning, and generative AI. He also founded the recently acquired Kylie.ai, an enterprise-grade conversational AI platform with RPA capabilities. Sinan most recently published Quick Guide to Large Language Models, and launched a podcast audio series, AI Unveiled. Ozdemir holds a masterβs degree in pure mathematics from Johns Hopkins University.
For Tasks:
Click tags to check more tools for each tasksFor Jobs:
Alternative AI tools for foundations-of-gen-ai
Similar Open Source Tools
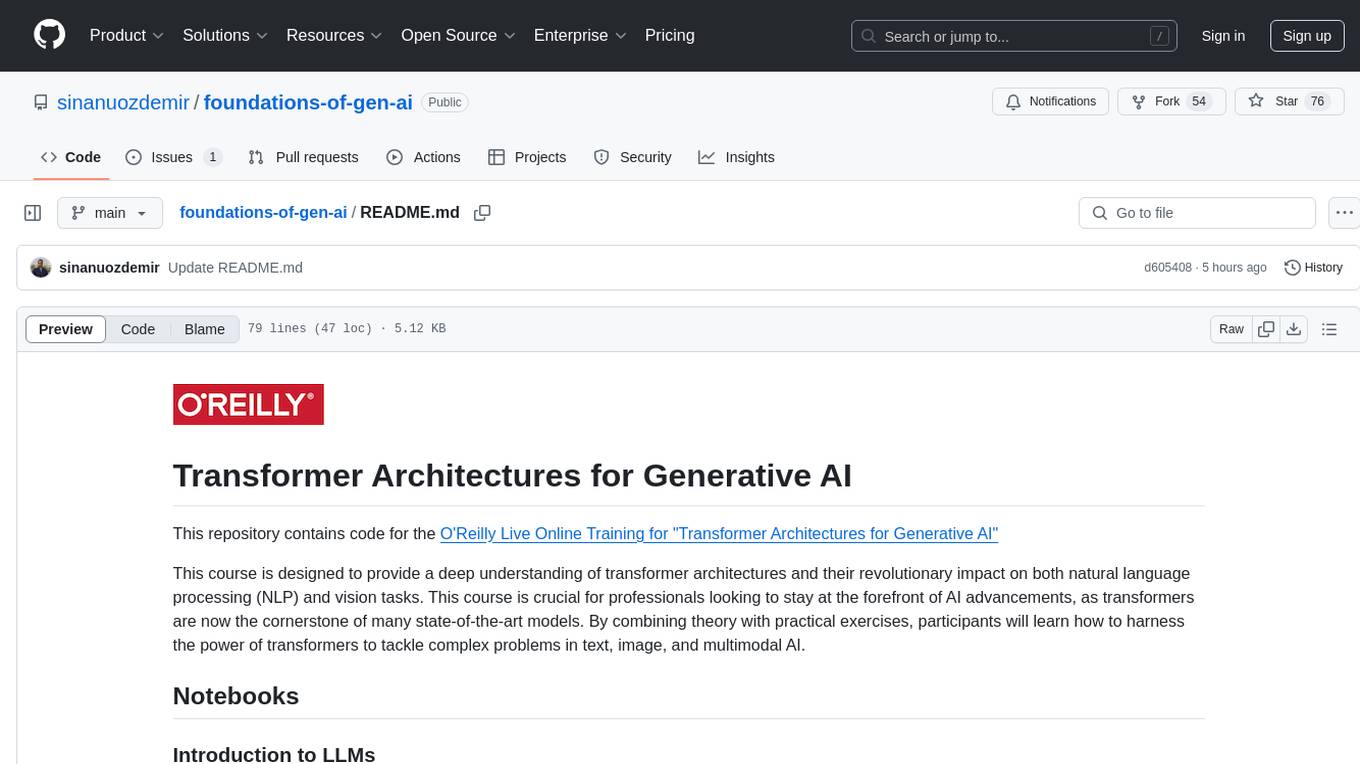
foundations-of-gen-ai
This repository contains code for the O'Reilly Live Online Training for 'Transformer Architectures for Generative AI'. The course provides a deep understanding of transformer architectures and their impact on natural language processing (NLP) and vision tasks. Participants learn to harness transformers to tackle problems in text, image, and multimodal AI through theory and practical exercises.
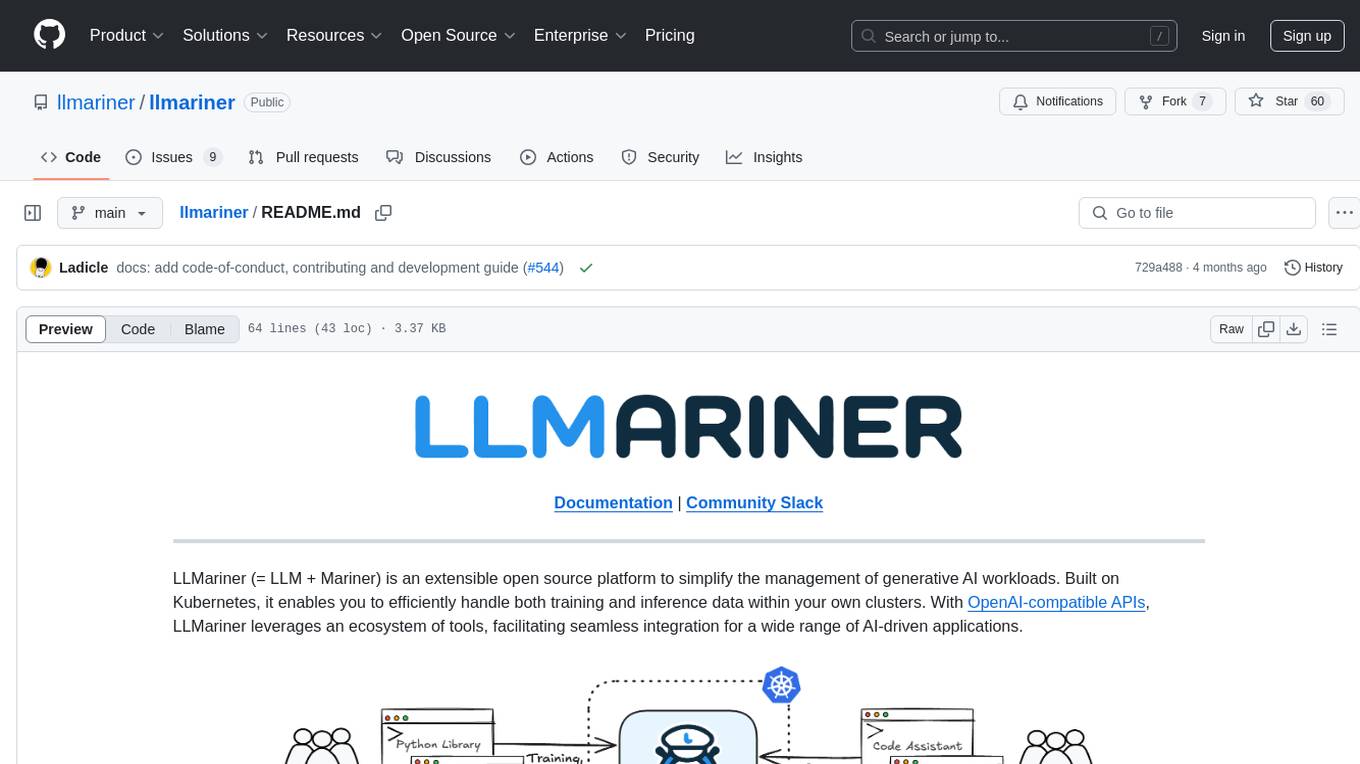
llmariner
LLMariner is an extensible open source platform built on Kubernetes to simplify the management of generative AI workloads. It enables efficient handling of training and inference data within clusters, with OpenAI-compatible APIs for seamless integration with a wide range of AI-driven applications.
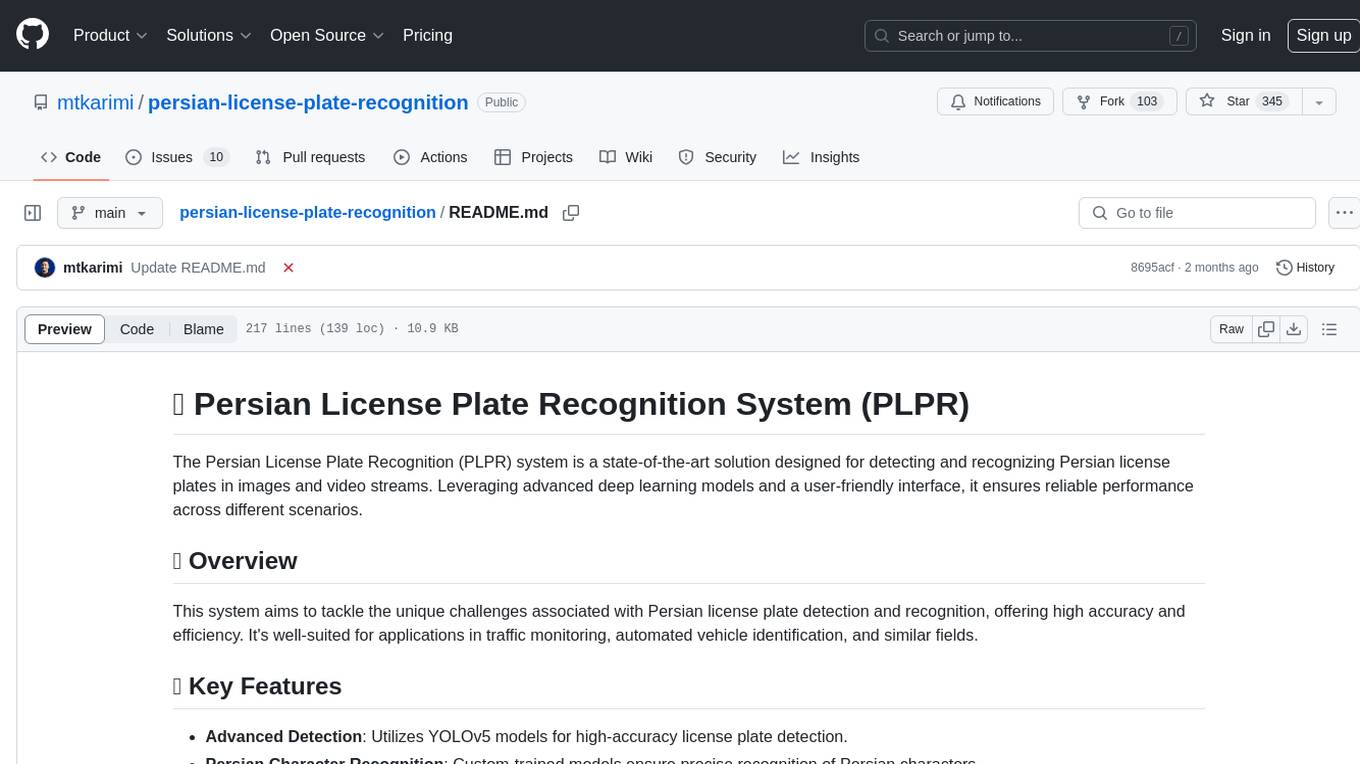
persian-license-plate-recognition
The Persian License Plate Recognition (PLPR) system is a state-of-the-art solution designed for detecting and recognizing Persian license plates in images and video streams. Leveraging advanced deep learning models and a user-friendly interface, it ensures reliable performance across different scenarios. The system offers advanced detection using YOLOv5 models, precise recognition of Persian characters, real-time processing capabilities, and a user-friendly GUI. It is well-suited for applications in traffic monitoring, automated vehicle identification, and similar fields. The system's architecture includes modules for resident management, entrance management, and a detailed flowchart explaining the process from system initialization to displaying results in the GUI. Hardware requirements include an Intel Core i5 processor, 8 GB RAM, a dedicated GPU with at least 4 GB VRAM, and an SSD with 20 GB of free space. The system can be installed by cloning the repository and installing required Python packages. Users can customize the video source for processing and run the application to upload and process images or video streams. The system's GUI allows for parameter adjustments to optimize performance, and the Wiki provides in-depth information on the system's architecture and model training.
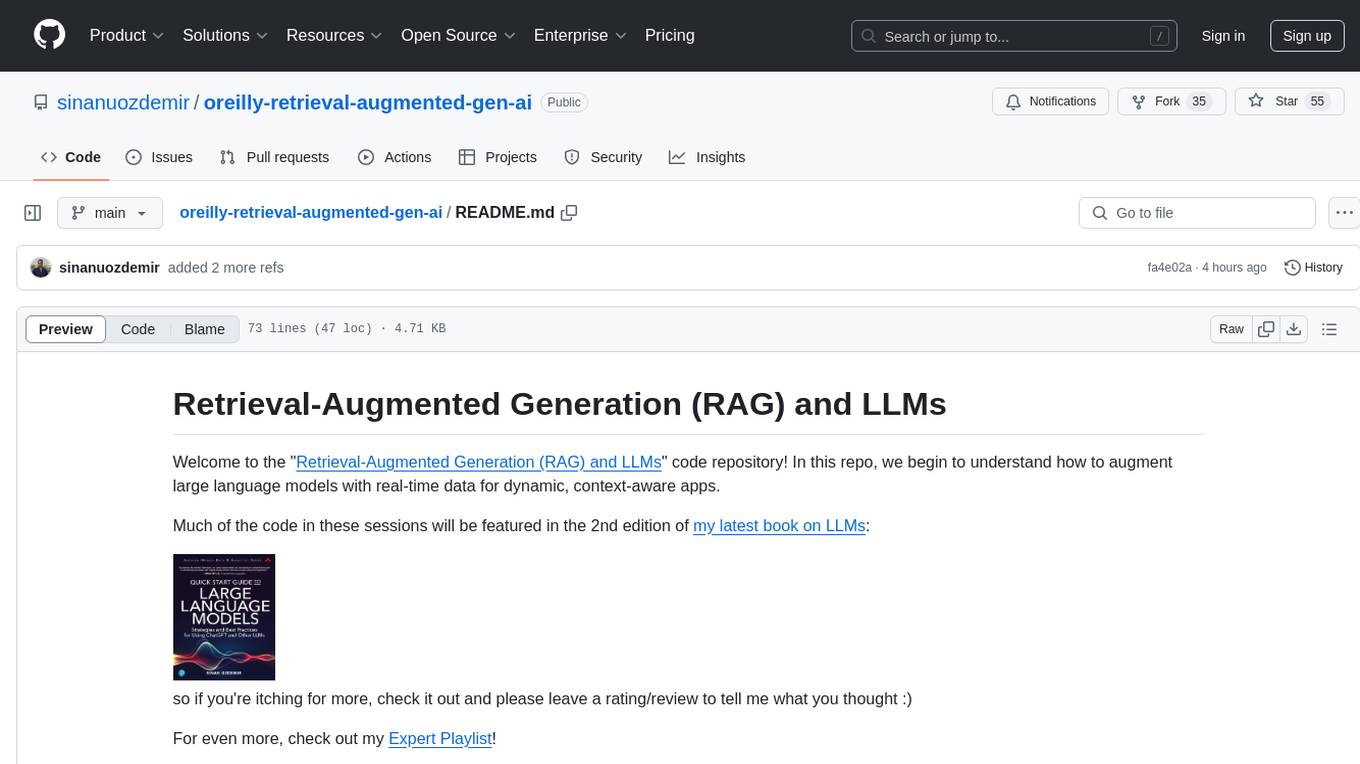
oreilly-retrieval-augmented-gen-ai
This repository focuses on Retrieval-Augmented Generation (RAG) and Large Language Models (LLMs). It provides code and resources to augment LLMs with real-time data for dynamic, context-aware applications. The content covers topics such as semantic search, fine-tuning embeddings, building RAG chatbots, evaluating LLMs, and using knowledge graphs in RAG. Prerequisites include Python skills, knowledge of machine learning and LLMs, and introductory experience with NLP and AI models.
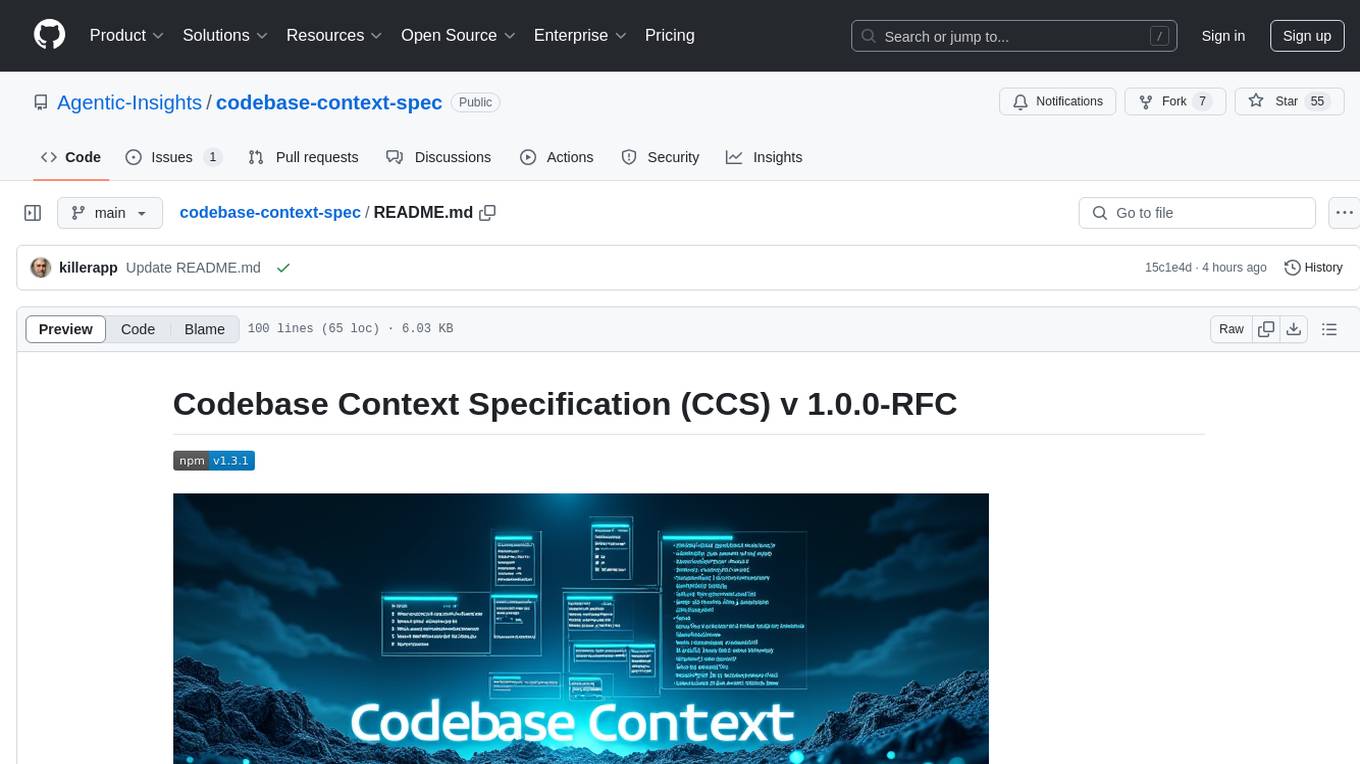
codebase-context-spec
The Codebase Context Specification (CCS) project aims to standardize embedding contextual information within codebases to enhance understanding for both AI and human developers. It introduces a convention similar to `.env` and `.editorconfig` files but focused on documenting code for both AI and humans. By providing structured contextual metadata, collaborative documentation guidelines, and standardized context files, developers can improve code comprehension, collaboration, and development efficiency. The project includes a linter for validating context files and provides guidelines for using the specification with AI assistants. Tooling recommendations suggest creating memory systems, IDE plugins, AI model integrations, and agents for context creation and utilization. Future directions include integration with existing documentation systems, dynamic context generation, and support for explicit context overriding.
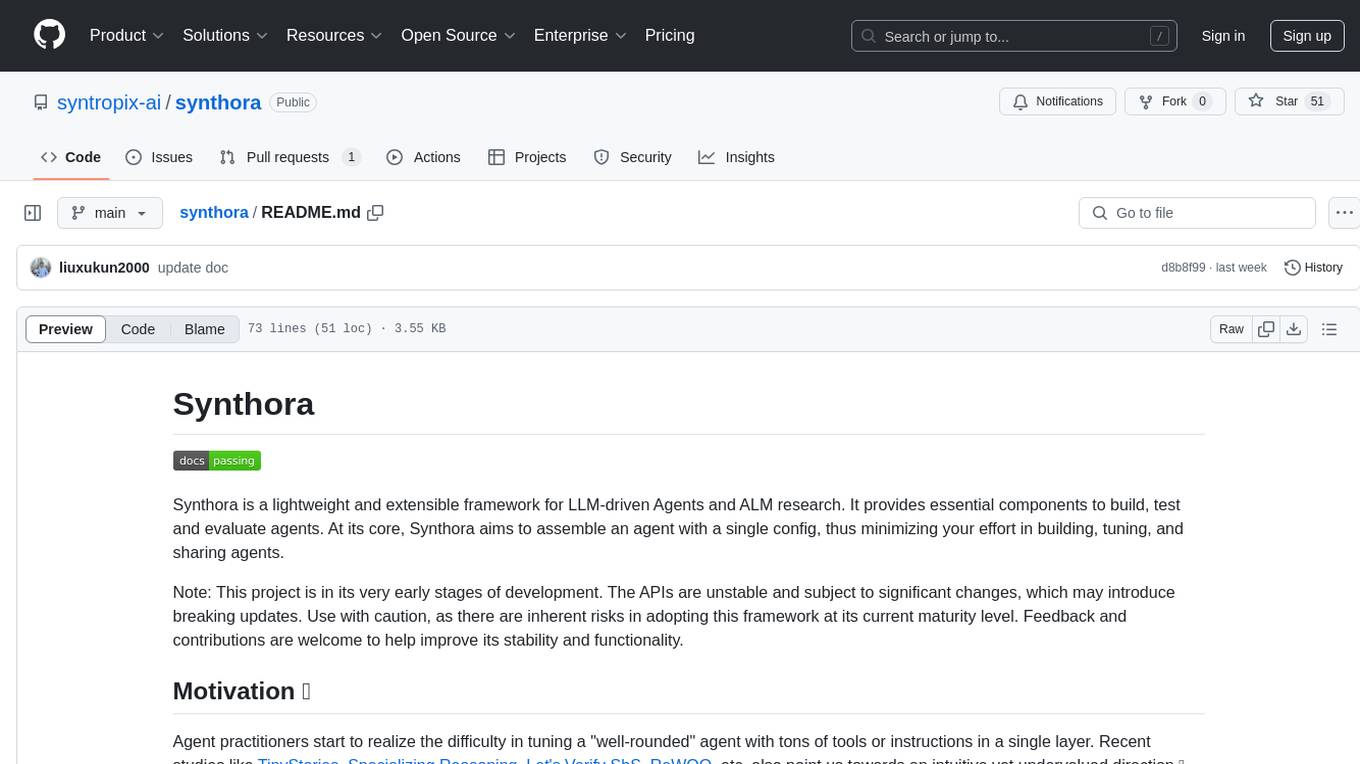
synthora
Synthora is a lightweight and extensible framework for LLM-driven Agents and ALM research. It aims to simplify the process of building, testing, and evaluating agents by providing essential components. The framework allows for easy agent assembly with a single config, reducing the effort required for tuning and sharing agents. Although in early development stages with unstable APIs, Synthora welcomes feedback and contributions to enhance its stability and functionality.
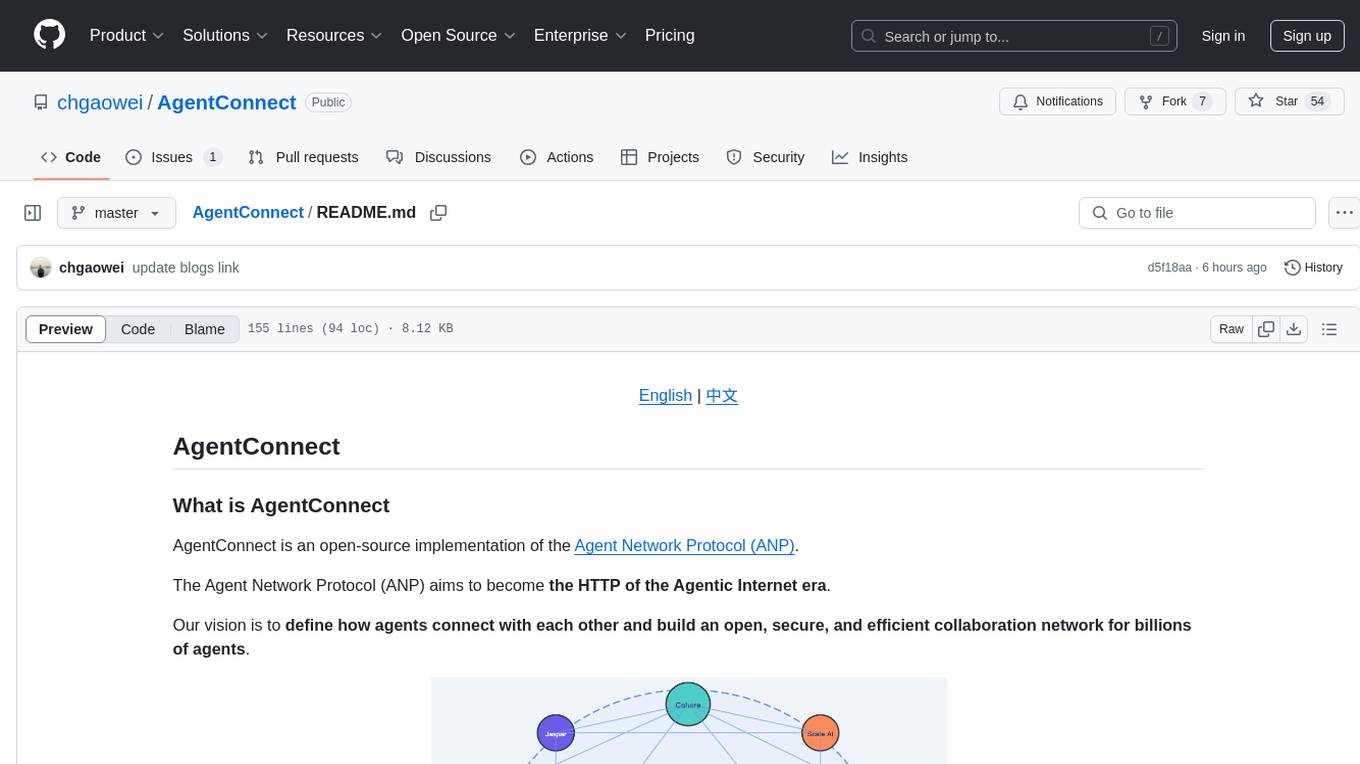
AgentConnect
AgentConnect is an open-source implementation of the Agent Network Protocol (ANP) aiming to define how agents connect with each other and build an open, secure, and efficient collaboration network for billions of agents. It addresses challenges like interconnectivity, native interfaces, and efficient collaboration. The architecture includes authentication, end-to-end encryption modules, meta-protocol module, and application layer protocol integration framework. AgentConnect focuses on performance and multi-platform support, with plans to rewrite core components in Rust and support mobile platforms and browsers. The project aims to establish ANP as an industry standard and form an ANP Standardization Committee. Installation is done via 'pip install agent-connect' and demos can be run after cloning the repository. Features include decentralized authentication based on did:wba and HTTP, and meta-protocol negotiation examples.
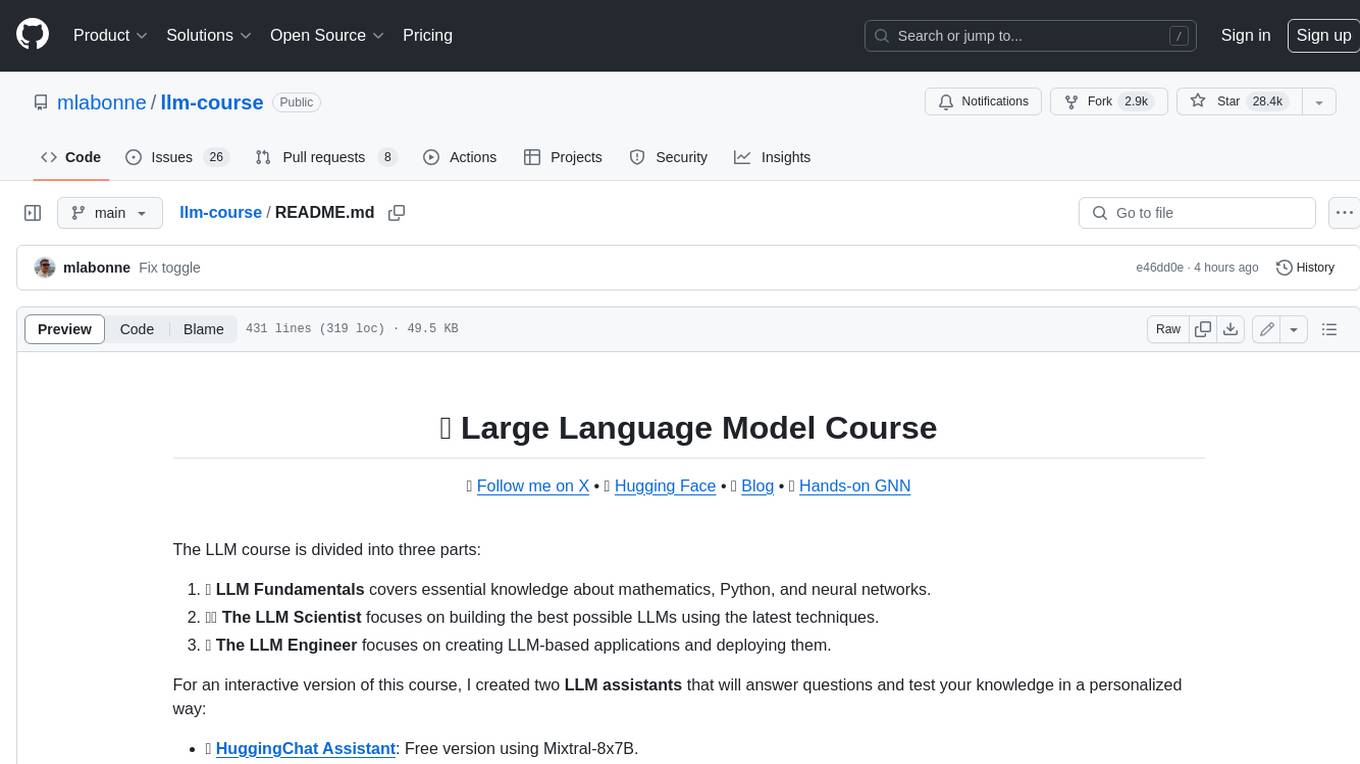
llm-course
The LLM course is divided into three parts: 1. π§© **LLM Fundamentals** covers essential knowledge about mathematics, Python, and neural networks. 2. π§βπ¬ **The LLM Scientist** focuses on building the best possible LLMs using the latest techniques. 3. π· **The LLM Engineer** focuses on creating LLM-based applications and deploying them. For an interactive version of this course, I created two **LLM assistants** that will answer questions and test your knowledge in a personalized way: * π€ **HuggingChat Assistant**: Free version using Mixtral-8x7B. * π€ **ChatGPT Assistant**: Requires a premium account. ## π Notebooks A list of notebooks and articles related to large language models. ### Tools | Notebook | Description | Notebook | |----------|-------------|----------| | π§ LLM AutoEval | Automatically evaluate your LLMs using RunPod |  | | π₯± LazyMergekit | Easily merge models using MergeKit in one click. |  | | π¦ LazyAxolotl | Fine-tune models in the cloud using Axolotl in one click. |  | | β‘ AutoQuant | Quantize LLMs in GGUF, GPTQ, EXL2, AWQ, and HQQ formats in one click. |  | | π³ Model Family Tree | Visualize the family tree of merged models. |  | | π ZeroSpace | Automatically create a Gradio chat interface using a free ZeroGPU. |  |
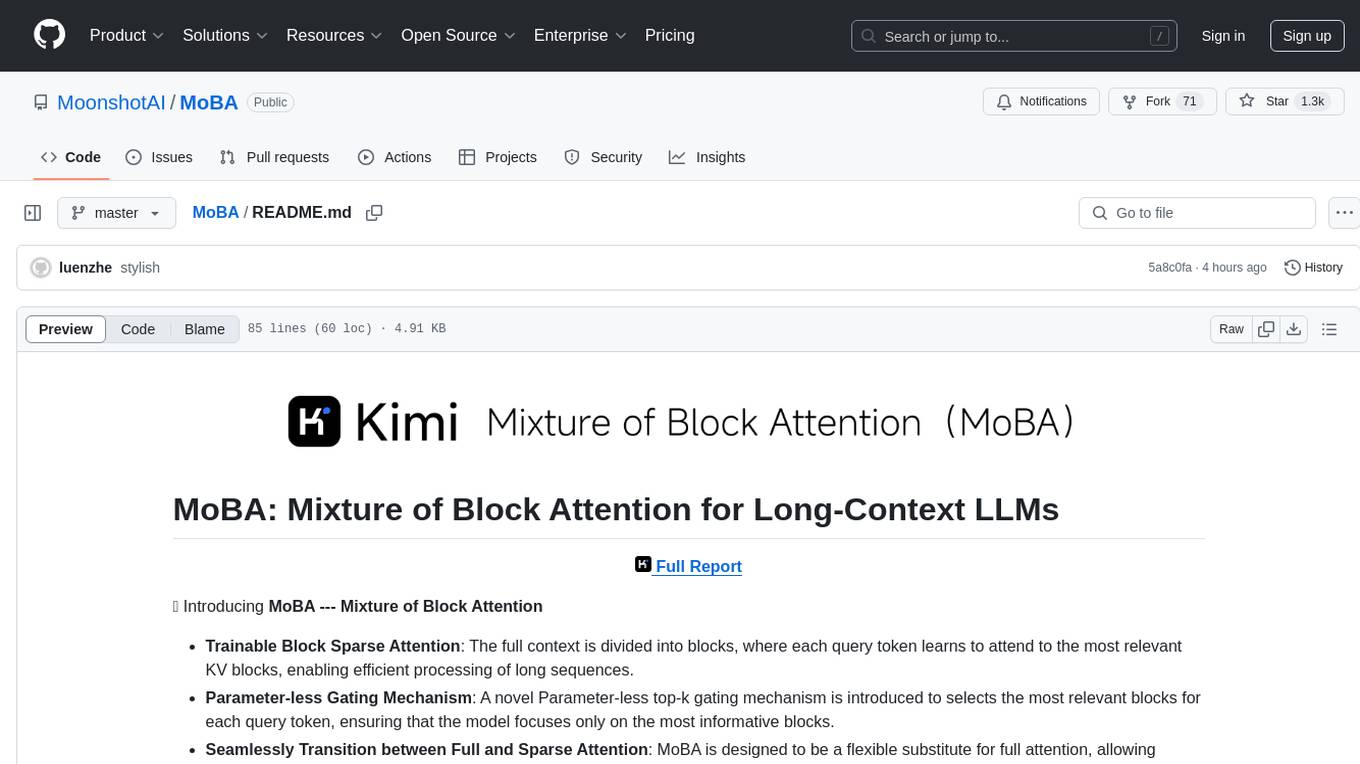
MoBA
MoBA (Mixture of Block Attention) is an innovative approach for long-context language models, enabling efficient processing of long sequences by dividing the full context into blocks and introducing a parameter-less gating mechanism. It allows seamless transitions between full and sparse attention modes, enhancing efficiency without compromising performance. MoBA has been deployed to support long-context requests and demonstrates significant advancements in efficient attention computation for large language models.
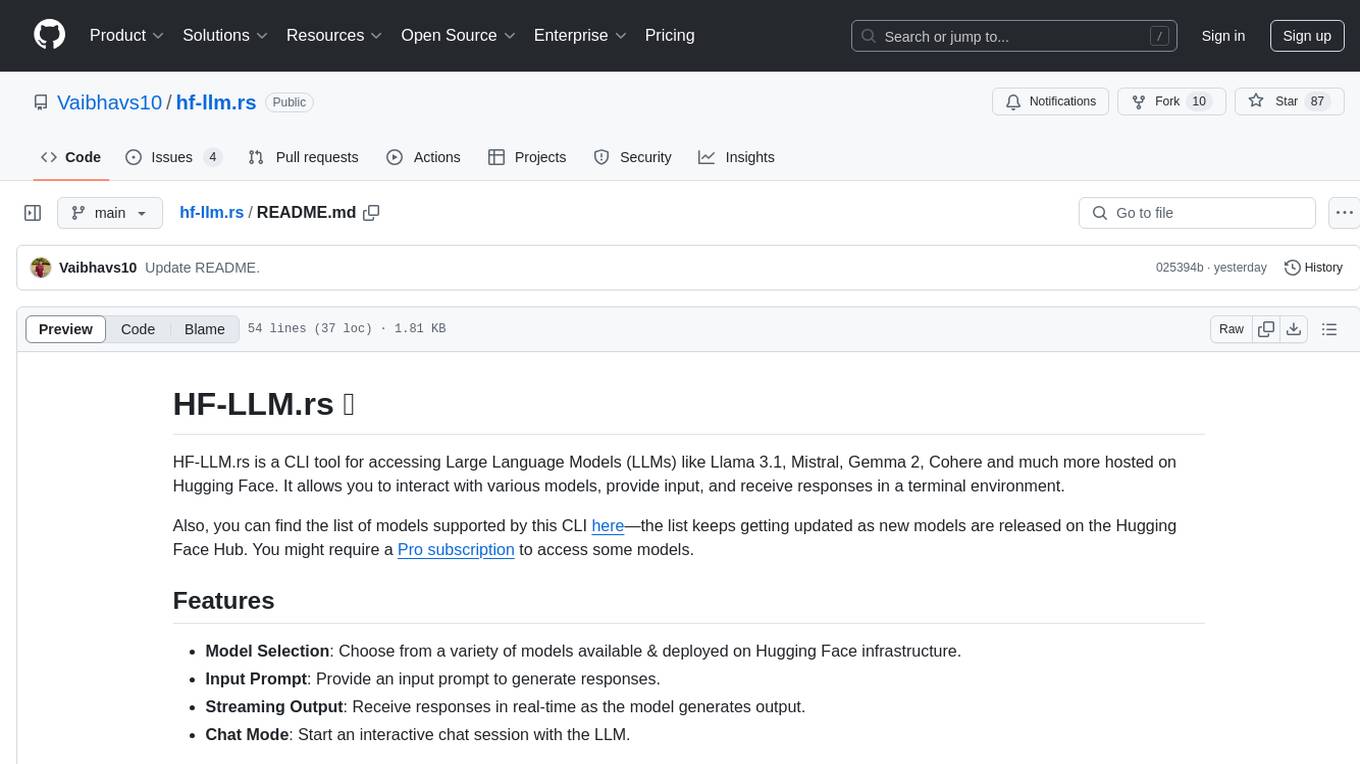
hf-llm.rs
HF-LLM.rs is a CLI tool for accessing Large Language Models (LLMs) like Llama 3.1, Mistral, Gemma 2, Cohere and more hosted on Hugging Face. It allows interaction with various models, providing input and receiving responses in a terminal environment. Users can select models, input prompts, receive streaming output, and engage in chat mode. The tool supports a variety of models available on Hugging Face infrastructure, with the list continuously updated. Some models may require a Pro subscription for access.
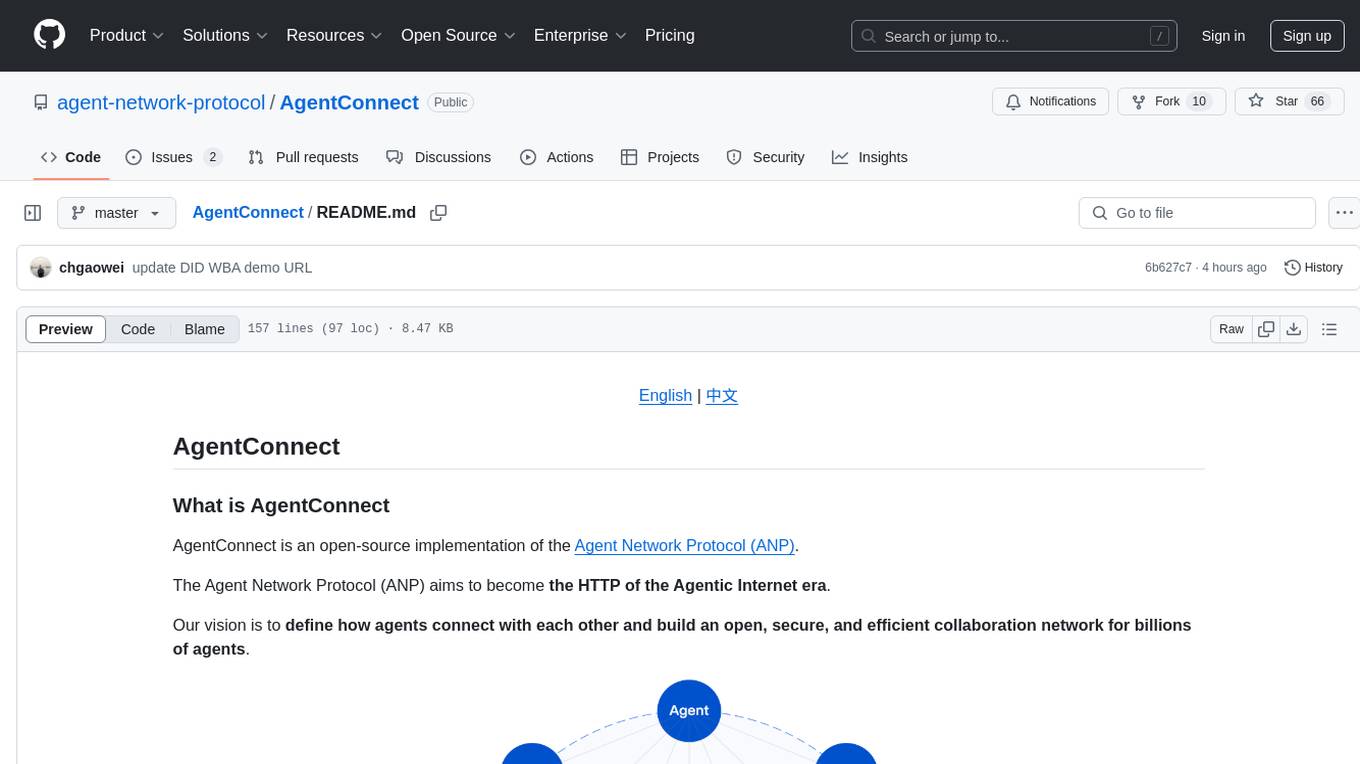
AgentConnect
AgentConnect is an open-source implementation of the Agent Network Protocol (ANP) aiming to define how agents connect with each other and build an open, secure, and efficient collaboration network for billions of agents. It addresses challenges like interconnectivity, native interfaces, and efficient collaboration by providing authentication, end-to-end encryption, meta-protocol handling, and application layer protocol integration. The project focuses on performance and multi-platform support, with plans to rewrite core components in Rust and support Mac, Linux, Windows, mobile platforms, and browsers. AgentConnect aims to establish ANP as an industry standard through protocol development and forming a standardization committee.
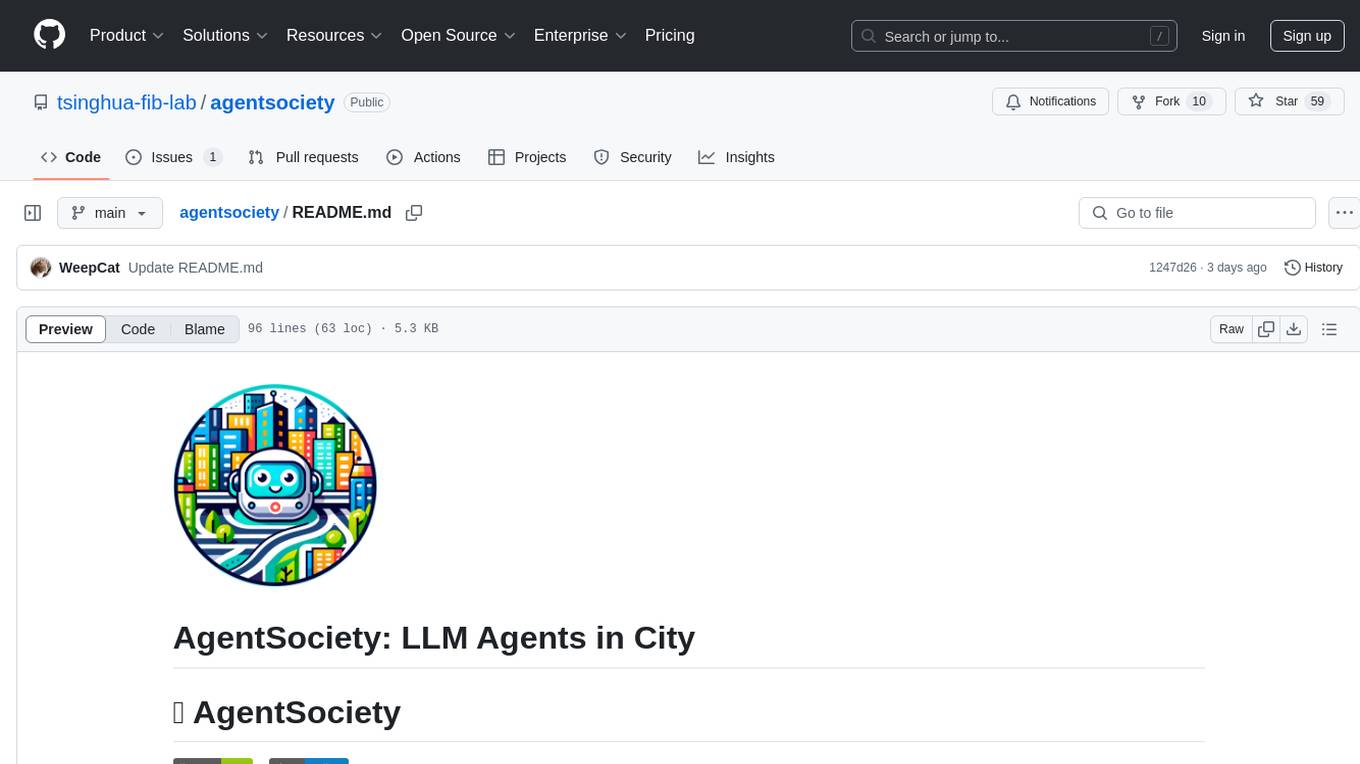
agentsociety
AgentSociety is an advanced framework designed for building agents in urban simulation environments. It integrates LLMs' planning, memory, and reasoning capabilities to generate realistic behaviors. The framework supports dataset-based, text-based, and rule-based environments with interactive visualization. It includes tools for interviews, surveys, interventions, and metric recording tailored for social experimentation.
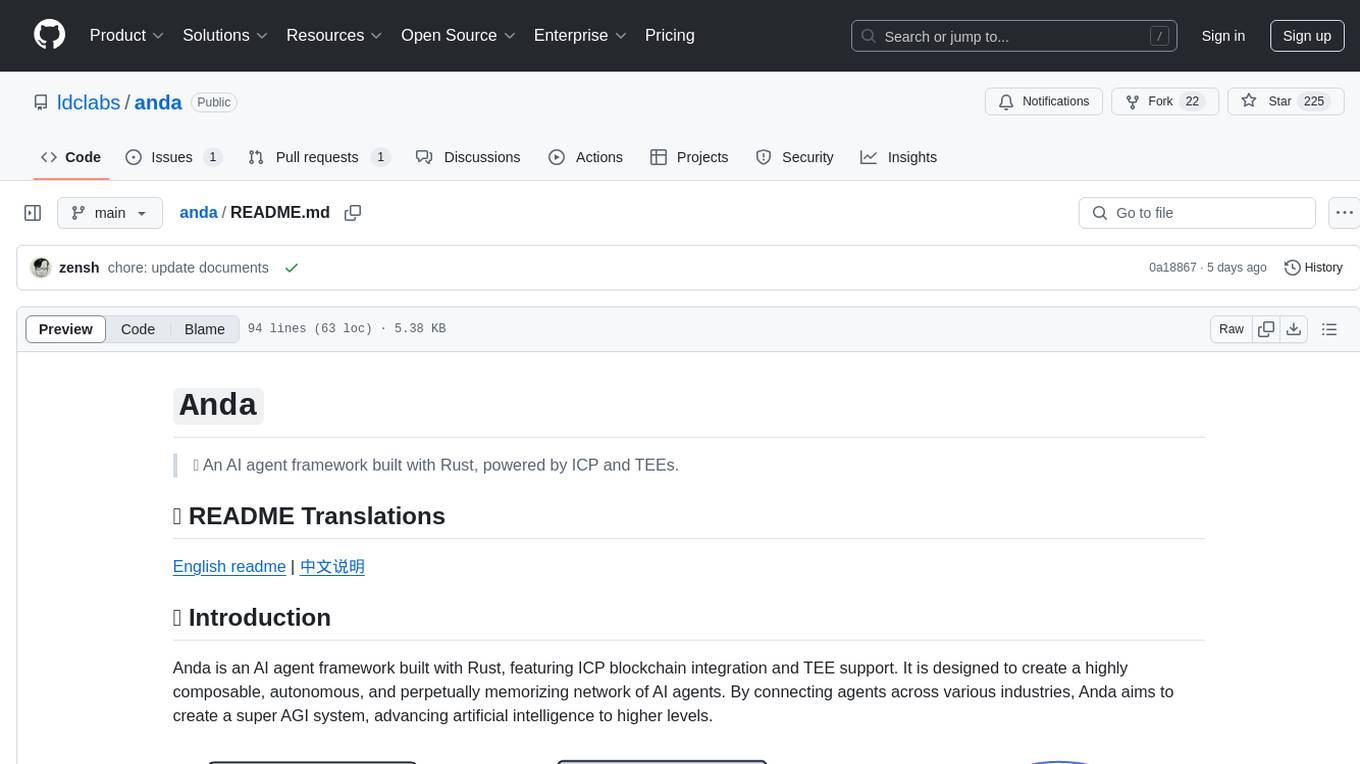
anda
Anda is an AI agent framework built with Rust, integrating ICP blockchain and TEE support. It aims to create a network of highly composable, autonomous AI agents across industries to advance artificial intelligence. Key features include composability, simplicity, trustworthiness, autonomy, and perpetual memory. Anda's vision is to build a collaborative network of agents leading to a super AGI system, revolutionizing AI technology applications and creating value for society.
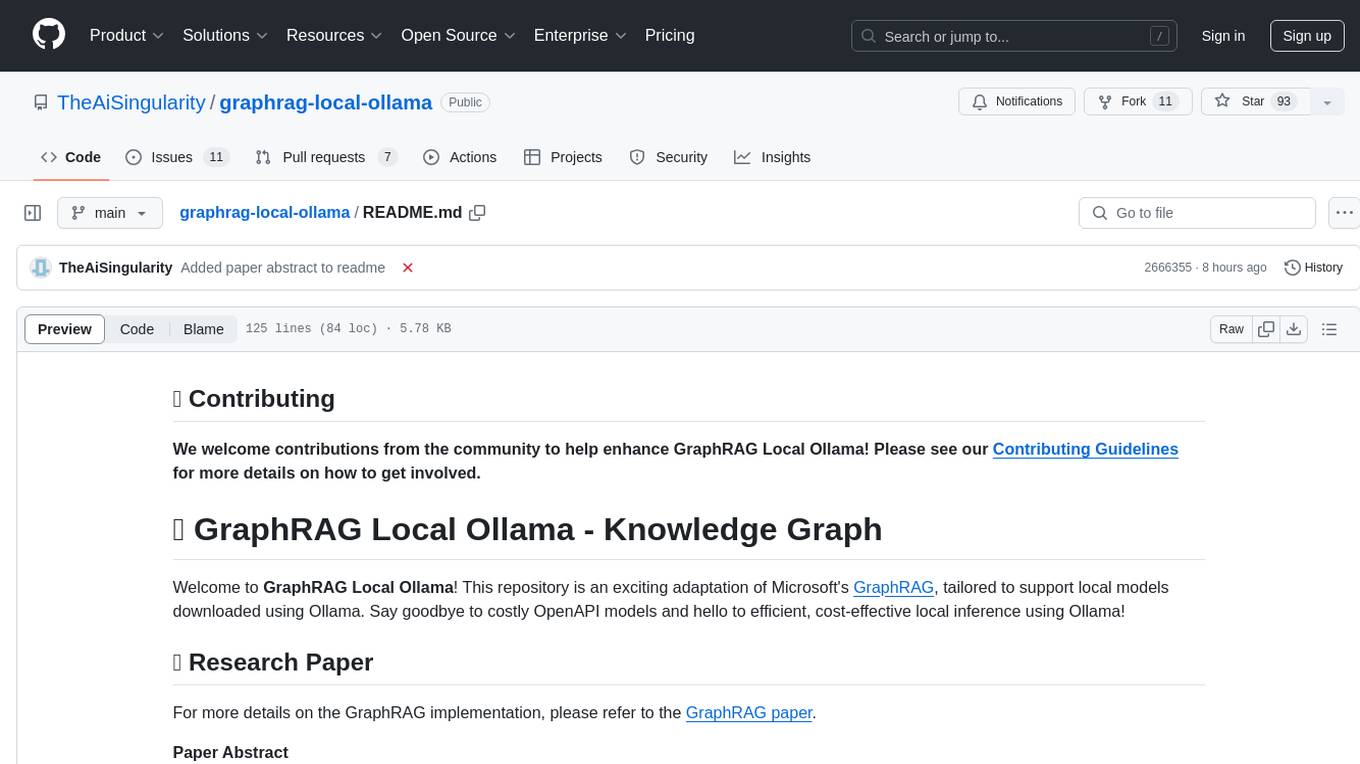
graphrag-local-ollama
GraphRAG Local Ollama is a repository that offers an adaptation of Microsoft's GraphRAG, customized to support local models downloaded using Ollama. It enables users to leverage local models with Ollama for large language models (LLMs) and embeddings, eliminating the need for costly OpenAPI models. The repository provides a simple setup process and allows users to perform question answering over private text corpora by building a graph-based text index and generating community summaries for closely-related entities. GraphRAG Local Ollama aims to improve the comprehensiveness and diversity of generated answers for global sensemaking questions over datasets.
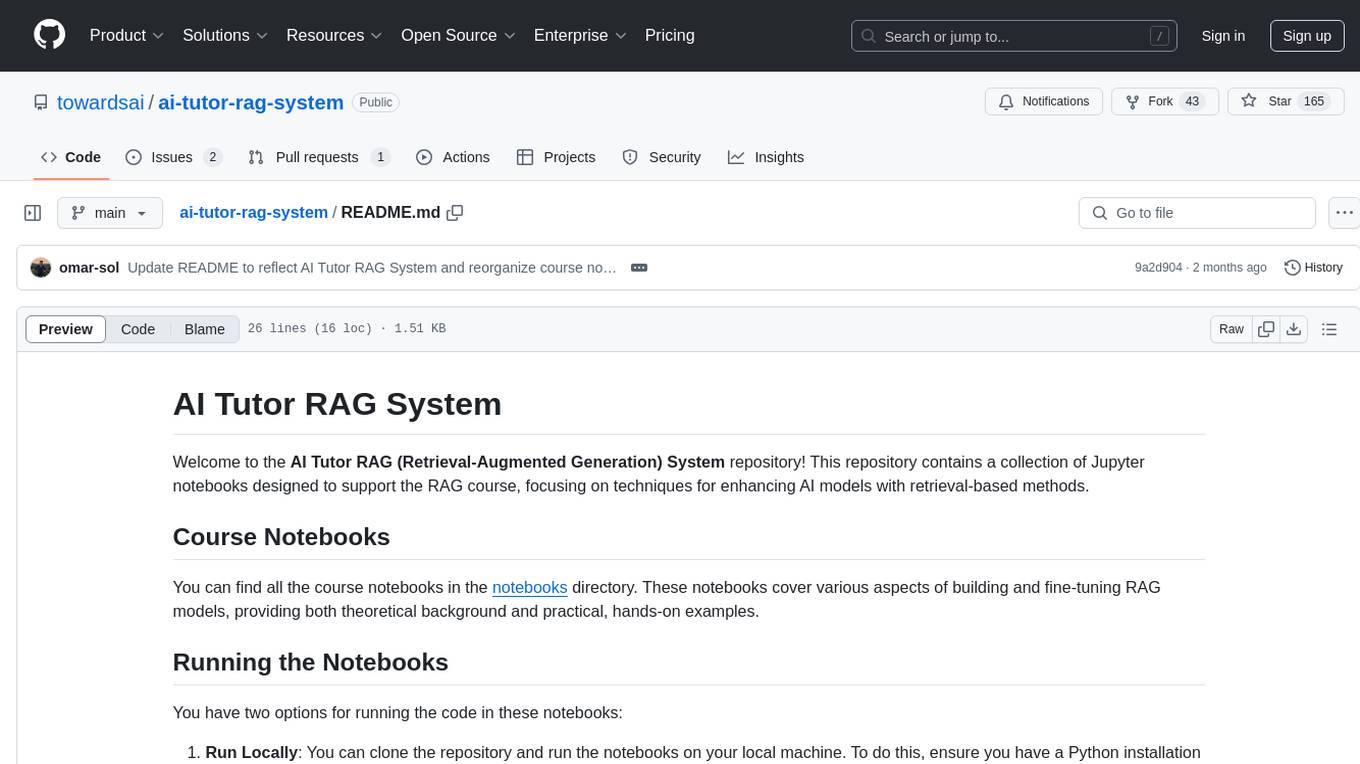
ai-tutor-rag-system
The AI Tutor RAG System repository contains Jupyter notebooks supporting the RAG course, focusing on enhancing AI models with retrieval-based methods. It covers foundational and advanced concepts in retrieval-augmented generation, including data retrieval techniques, model integration with retrieval systems, and practical applications of RAG in real-world scenarios.
For similar tasks
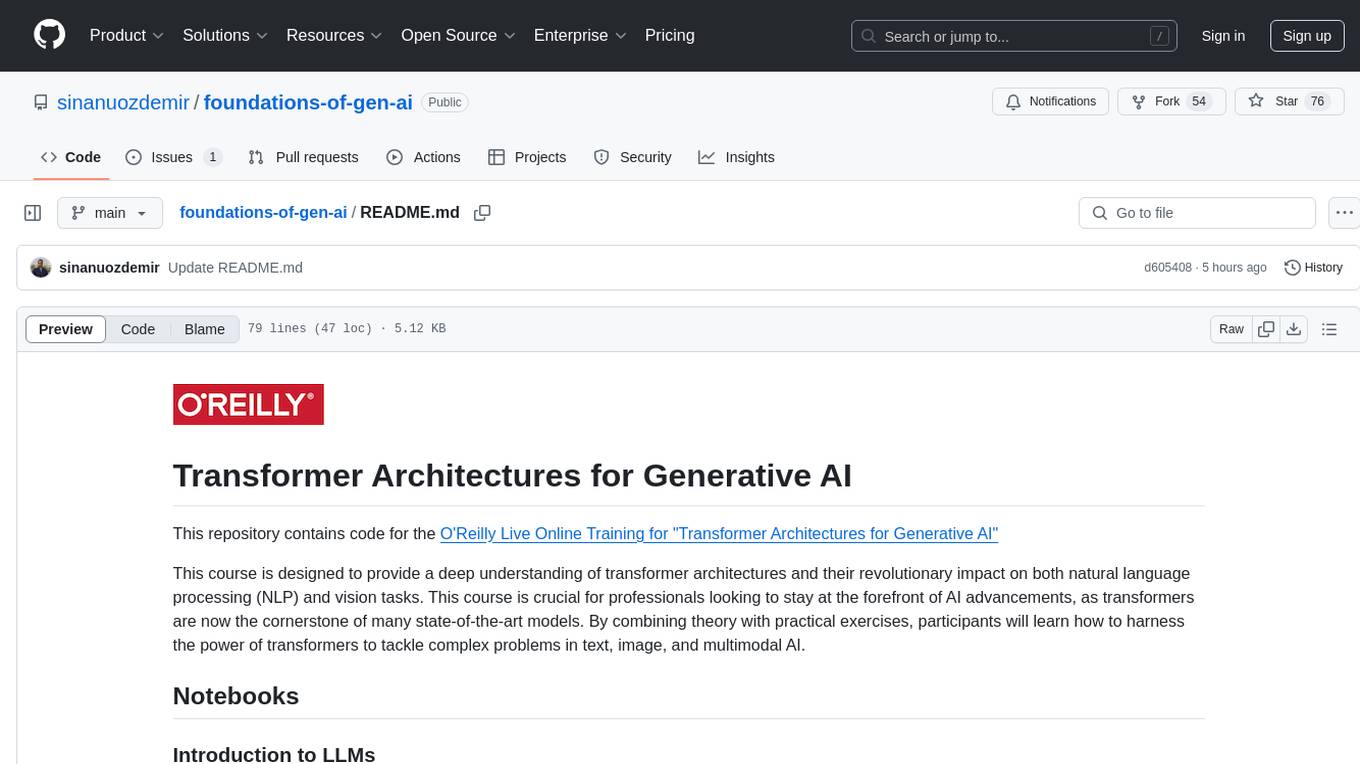
foundations-of-gen-ai
This repository contains code for the O'Reilly Live Online Training for 'Transformer Architectures for Generative AI'. The course provides a deep understanding of transformer architectures and their impact on natural language processing (NLP) and vision tasks. Participants learn to harness transformers to tackle problems in text, image, and multimodal AI through theory and practical exercises.
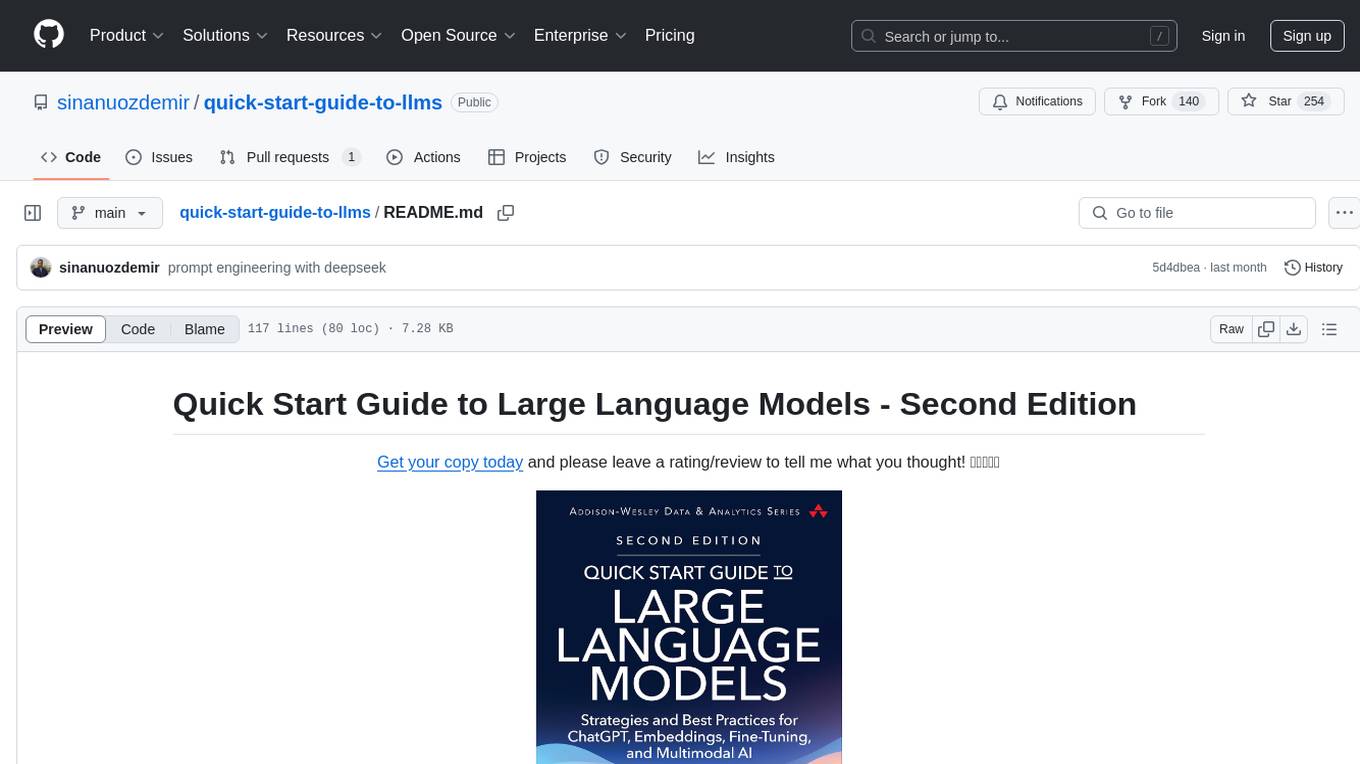
quick-start-guide-to-llms
This GitHub repository serves as the companion to the 'Quick Start Guide to Large Language Models - Second Edition' book. It contains code snippets and notebooks demonstrating various applications and advanced techniques in working with Transformer models and large language models (LLMs). The repository is structured into directories for notebooks, data, and images, with each notebook corresponding to a chapter in the book. Users can explore topics such as semantic search, prompt engineering, model fine-tuning, custom embeddings, advanced LLM usage, moving LLMs into production, and evaluating LLMs. The repository aims to provide practical examples and insights for working with LLMs in different contexts.
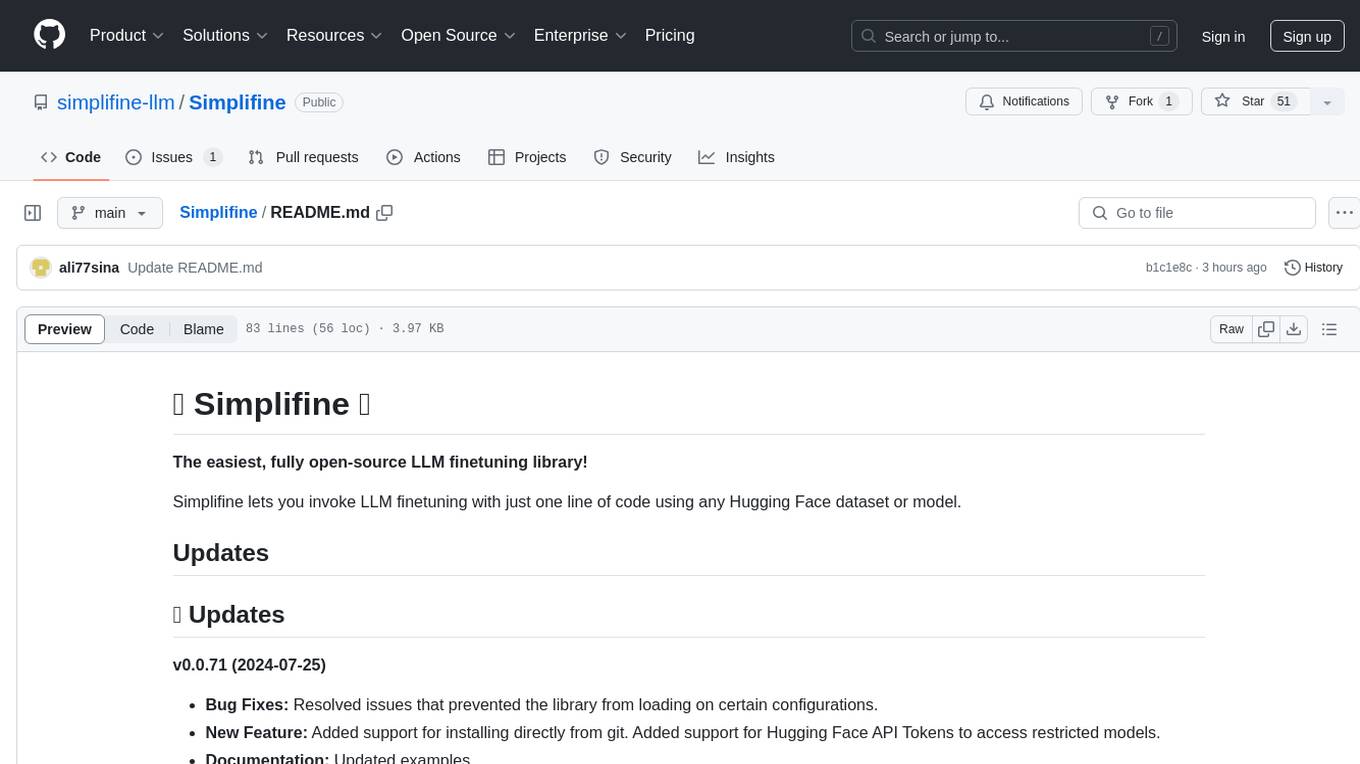
Simplifine
Simplifine is an open-source library designed for easy LLM finetuning, enabling users to perform tasks such as supervised fine tuning, question-answer finetuning, contrastive loss for embedding tasks, multi-label classification finetuning, and more. It provides features like WandB logging, in-built evaluation tools, automated finetuning parameters, and state-of-the-art optimization techniques. The library offers bug fixes, new features, and documentation updates in its latest version. Users can install Simplifine via pip or directly from GitHub. The project welcomes contributors and provides comprehensive documentation and support for users.
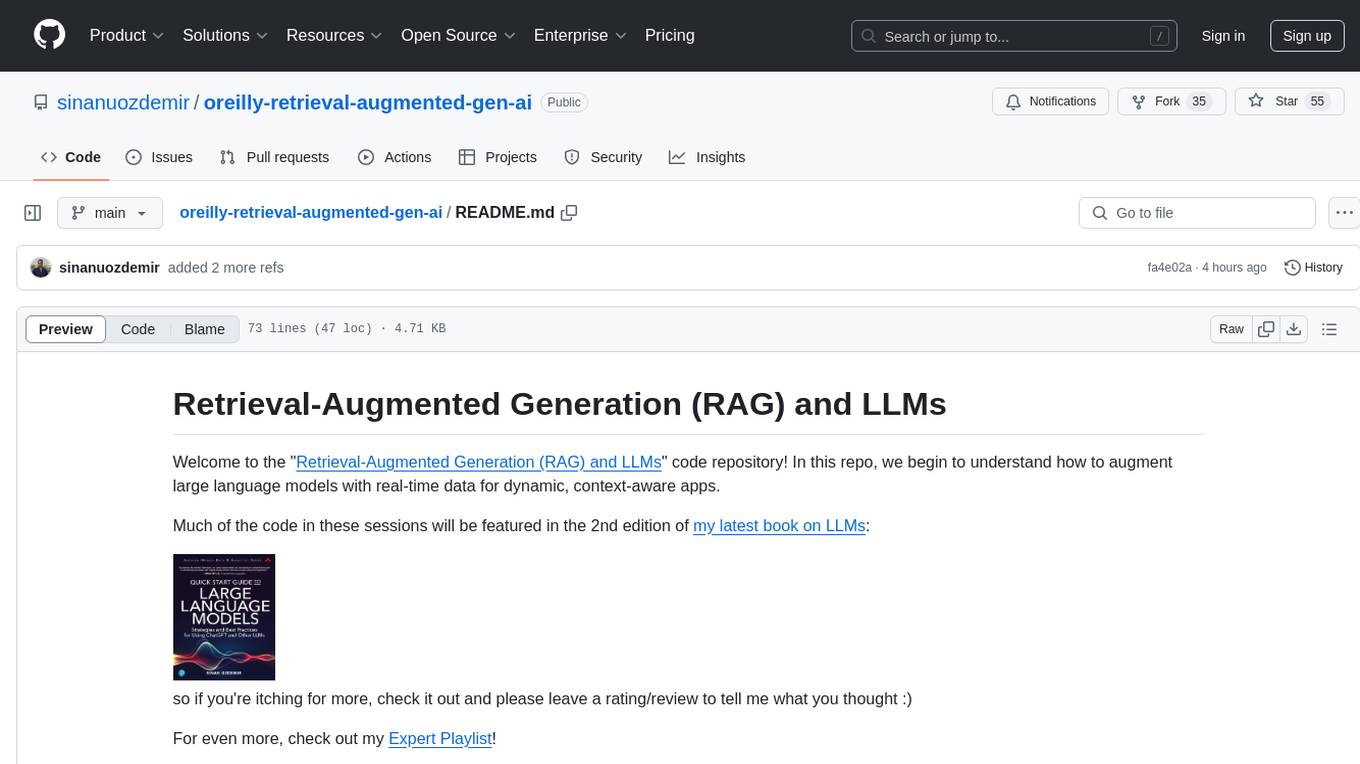
oreilly-retrieval-augmented-gen-ai
This repository focuses on Retrieval-Augmented Generation (RAG) and Large Language Models (LLMs). It provides code and resources to augment LLMs with real-time data for dynamic, context-aware applications. The content covers topics such as semantic search, fine-tuning embeddings, building RAG chatbots, evaluating LLMs, and using knowledge graphs in RAG. Prerequisites include Python skills, knowledge of machine learning and LLMs, and introductory experience with NLP and AI models.
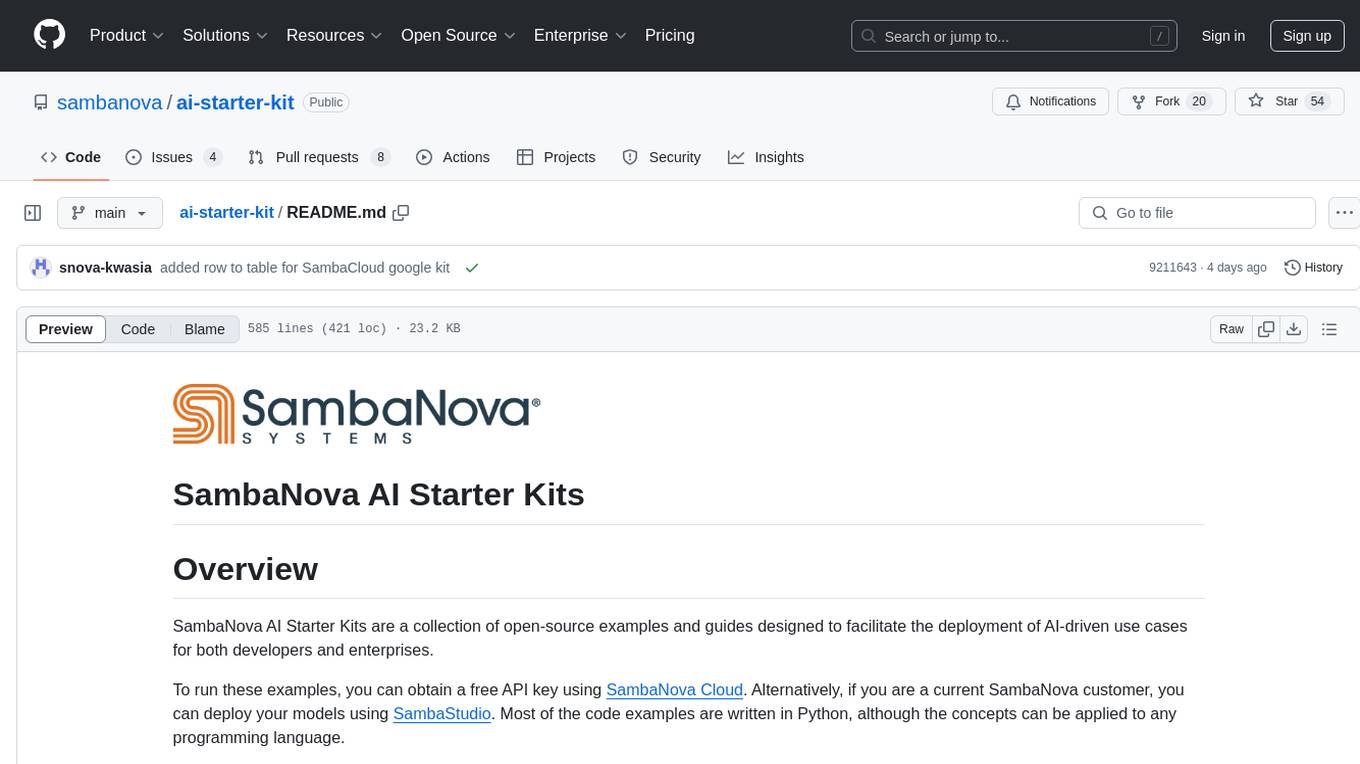
ai-starter-kit
SambaNova AI Starter Kits is a collection of open-source examples and guides designed to facilitate the deployment of AI-driven use cases for developers and enterprises. The kits cover various categories such as Data Ingestion & Preparation, Model Development & Optimization, Intelligent Information Retrieval, and Advanced AI Capabilities. Users can obtain a free API key using SambaNova Cloud or deploy models using SambaStudio. Most examples are written in Python but can be applied to any programming language. The kits provide resources for tasks like text extraction, fine-tuning embeddings, prompt engineering, question-answering, image search, post-call analysis, and more.
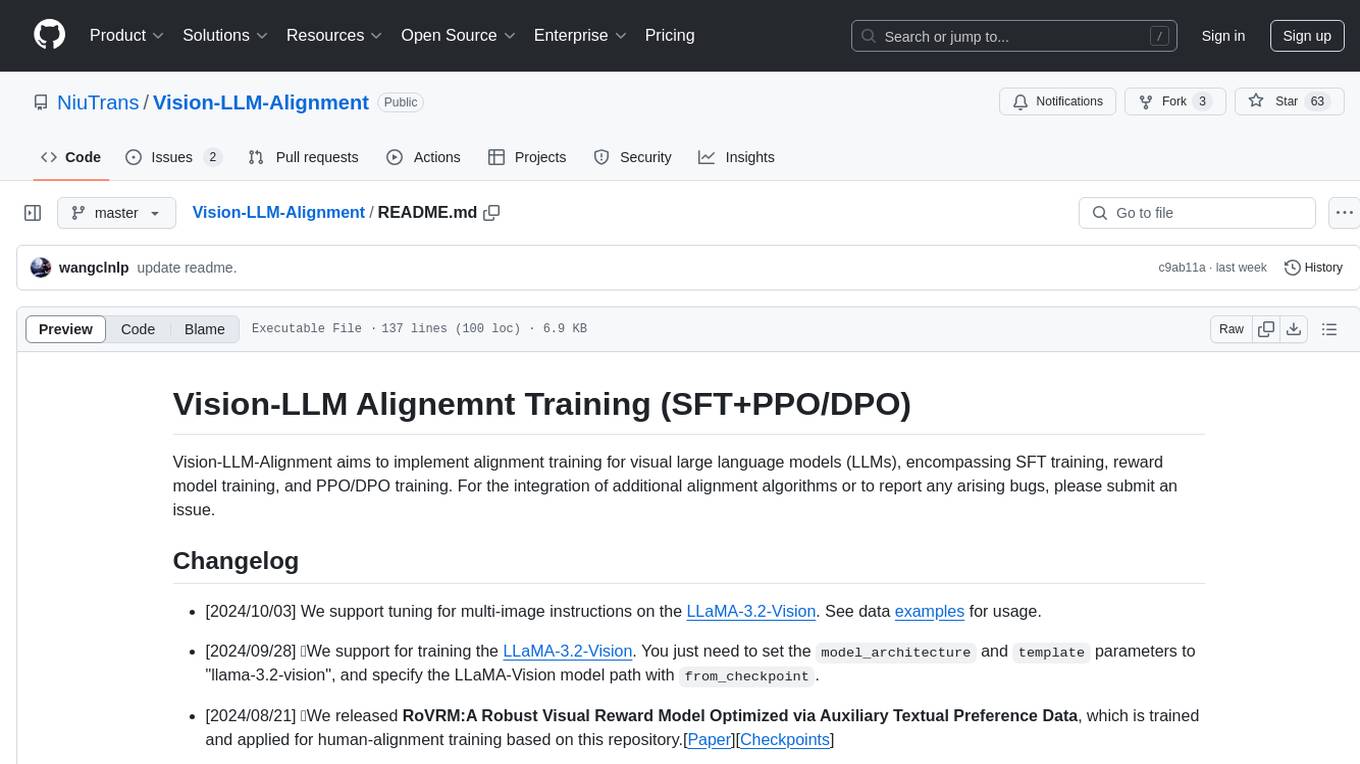
Vision-LLM-Alignment
Vision-LLM-Alignment is a repository focused on implementing alignment training for visual large language models (LLMs), including SFT training, reward model training, and PPO/DPO training. It supports various model architectures and provides datasets for training. The repository also offers benchmark results and installation instructions for users.
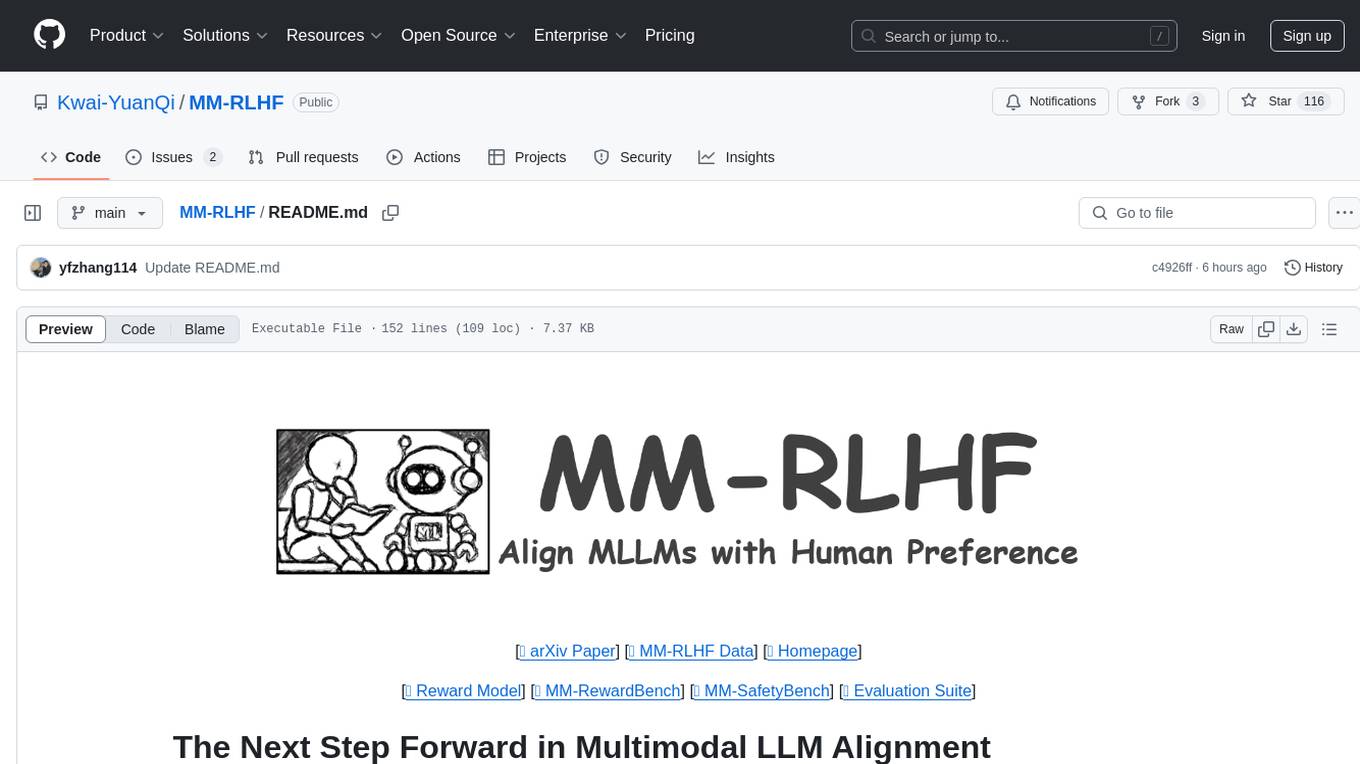
MM-RLHF
MM-RLHF is a comprehensive project for aligning Multimodal Large Language Models (MLLMs) with human preferences. It includes a high-quality MLLM alignment dataset, a Critique-Based MLLM reward model, a novel alignment algorithm MM-DPO, and benchmarks for reward models and multimodal safety. The dataset covers image understanding, video understanding, and safety-related tasks with model-generated responses and human-annotated scores. The reward model generates critiques of candidate texts before assigning scores for enhanced interpretability. MM-DPO is an alignment algorithm that achieves performance gains with simple adjustments to the DPO framework. The project enables consistent performance improvements across 10 dimensions and 27 benchmarks for open-source MLLMs.
For similar jobs
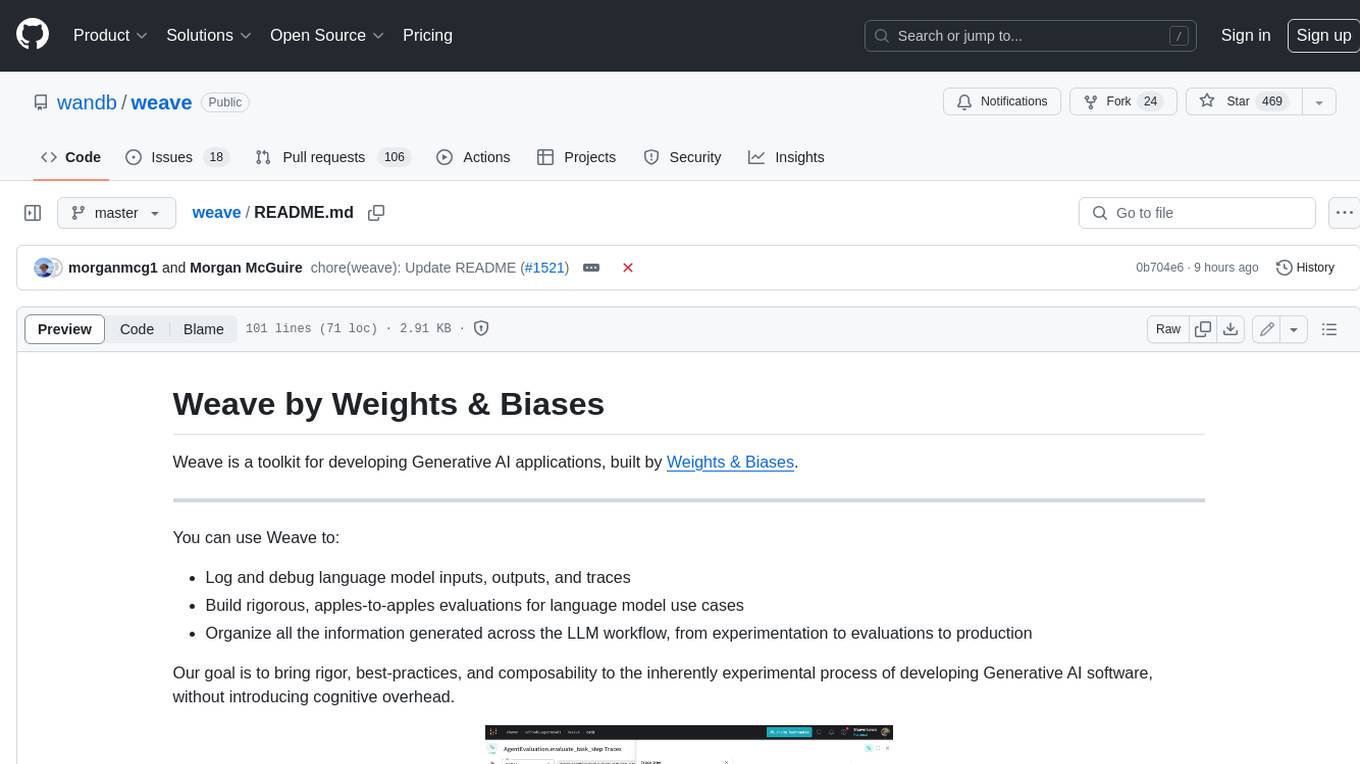
weave
Weave is a toolkit for developing Generative AI applications, built by Weights & Biases. With Weave, you can log and debug language model inputs, outputs, and traces; build rigorous, apples-to-apples evaluations for language model use cases; and organize all the information generated across the LLM workflow, from experimentation to evaluations to production. Weave aims to bring rigor, best-practices, and composability to the inherently experimental process of developing Generative AI software, without introducing cognitive overhead.
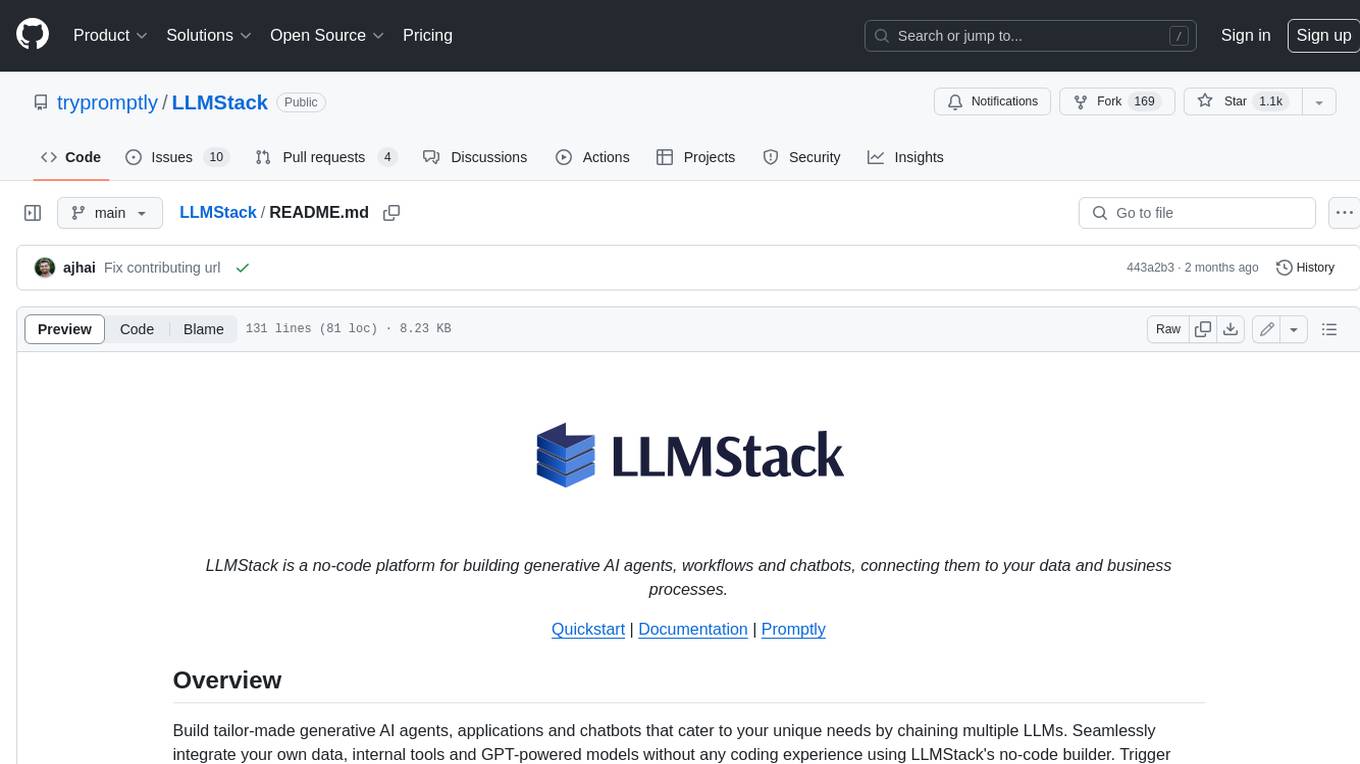
LLMStack
LLMStack is a no-code platform for building generative AI agents, workflows, and chatbots. It allows users to connect their own data, internal tools, and GPT-powered models without any coding experience. LLMStack can be deployed to the cloud or on-premise and can be accessed via HTTP API or triggered from Slack or Discord.
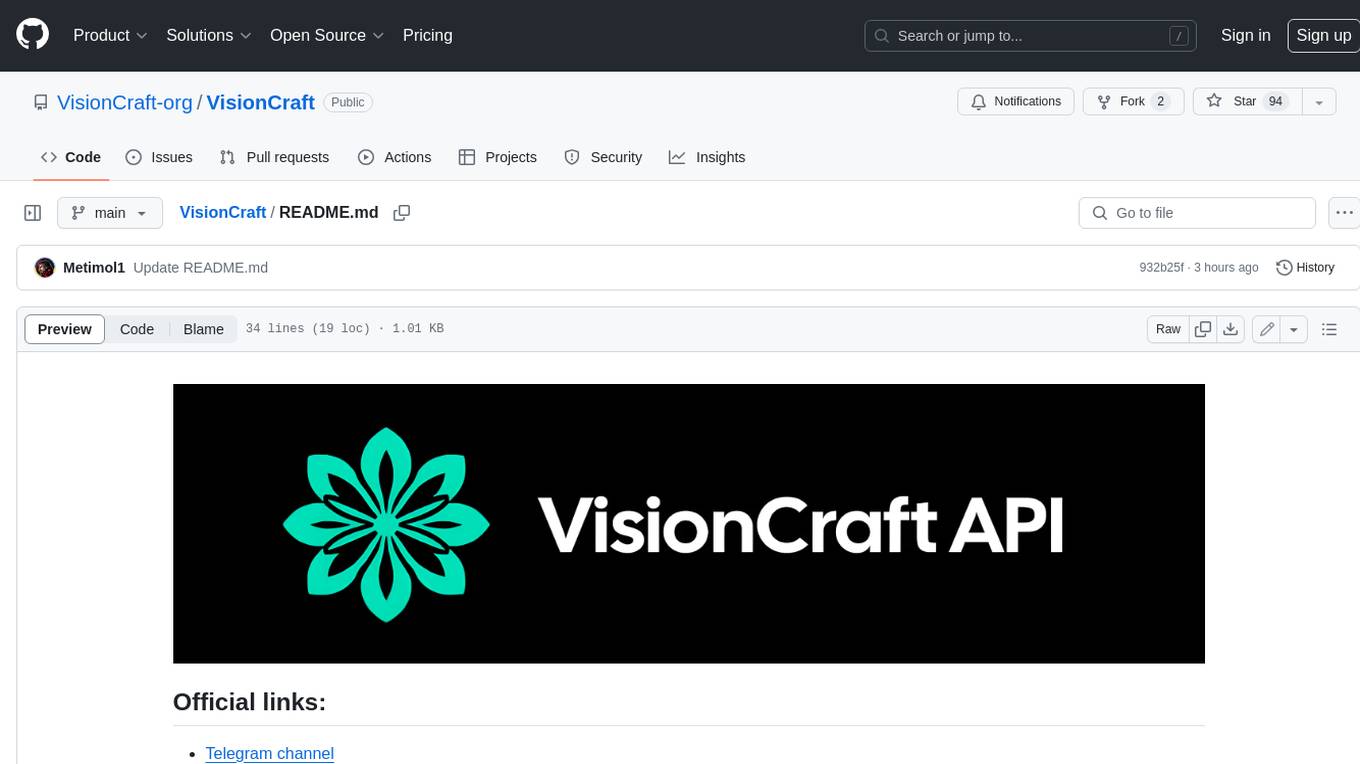
VisionCraft
The VisionCraft API is a free API for using over 100 different AI models. From images to sound.
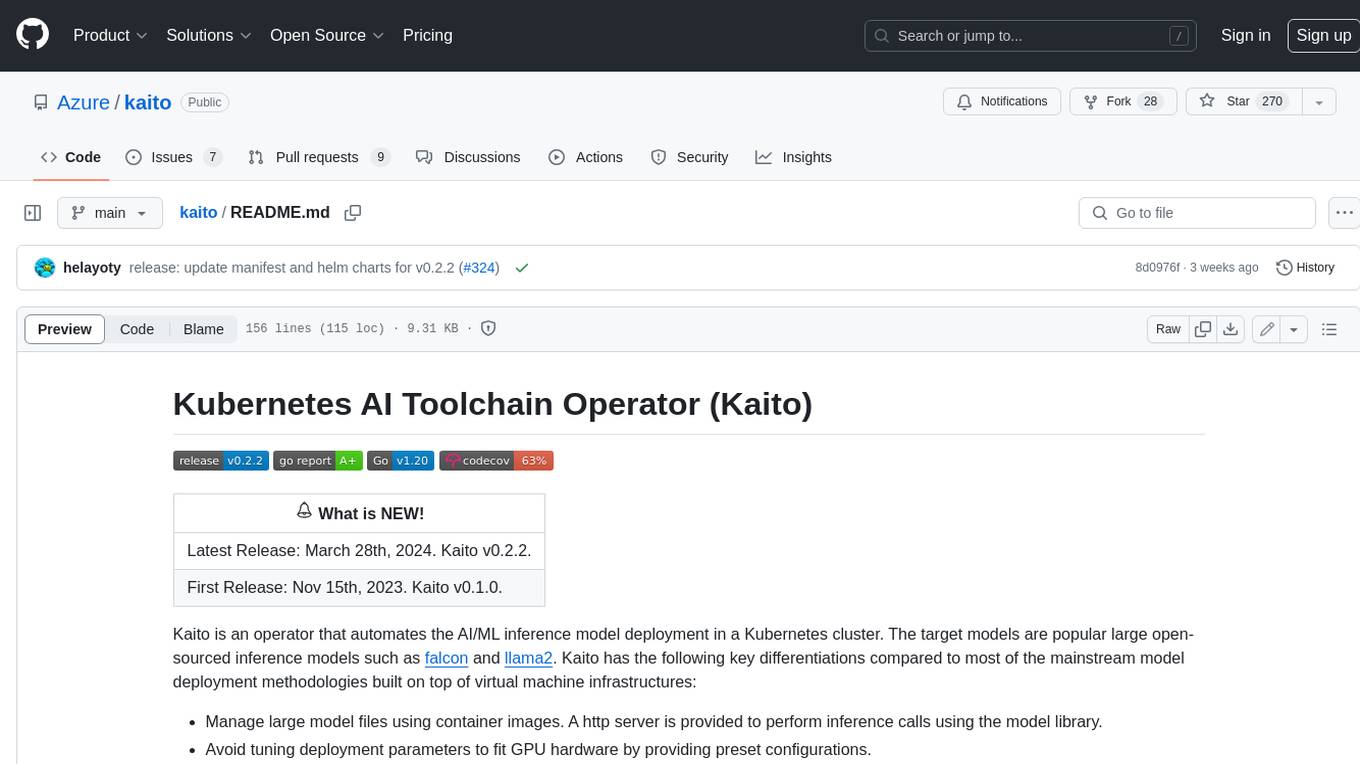
kaito
Kaito is an operator that automates the AI/ML inference model deployment in a Kubernetes cluster. It manages large model files using container images, avoids tuning deployment parameters to fit GPU hardware by providing preset configurations, auto-provisions GPU nodes based on model requirements, and hosts large model images in the public Microsoft Container Registry (MCR) if the license allows. Using Kaito, the workflow of onboarding large AI inference models in Kubernetes is largely simplified.
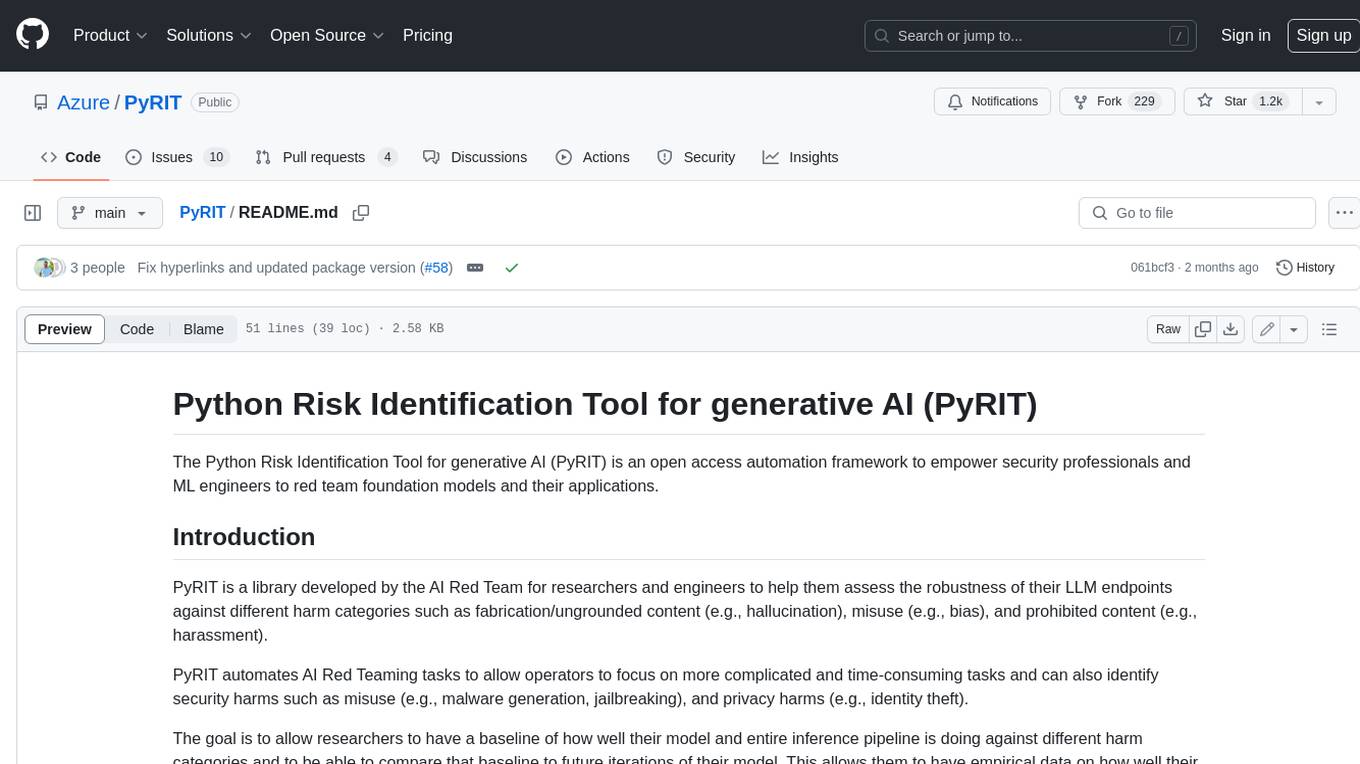
PyRIT
PyRIT is an open access automation framework designed to empower security professionals and ML engineers to red team foundation models and their applications. It automates AI Red Teaming tasks to allow operators to focus on more complicated and time-consuming tasks and can also identify security harms such as misuse (e.g., malware generation, jailbreaking), and privacy harms (e.g., identity theft). The goal is to allow researchers to have a baseline of how well their model and entire inference pipeline is doing against different harm categories and to be able to compare that baseline to future iterations of their model. This allows them to have empirical data on how well their model is doing today, and detect any degradation of performance based on future improvements.
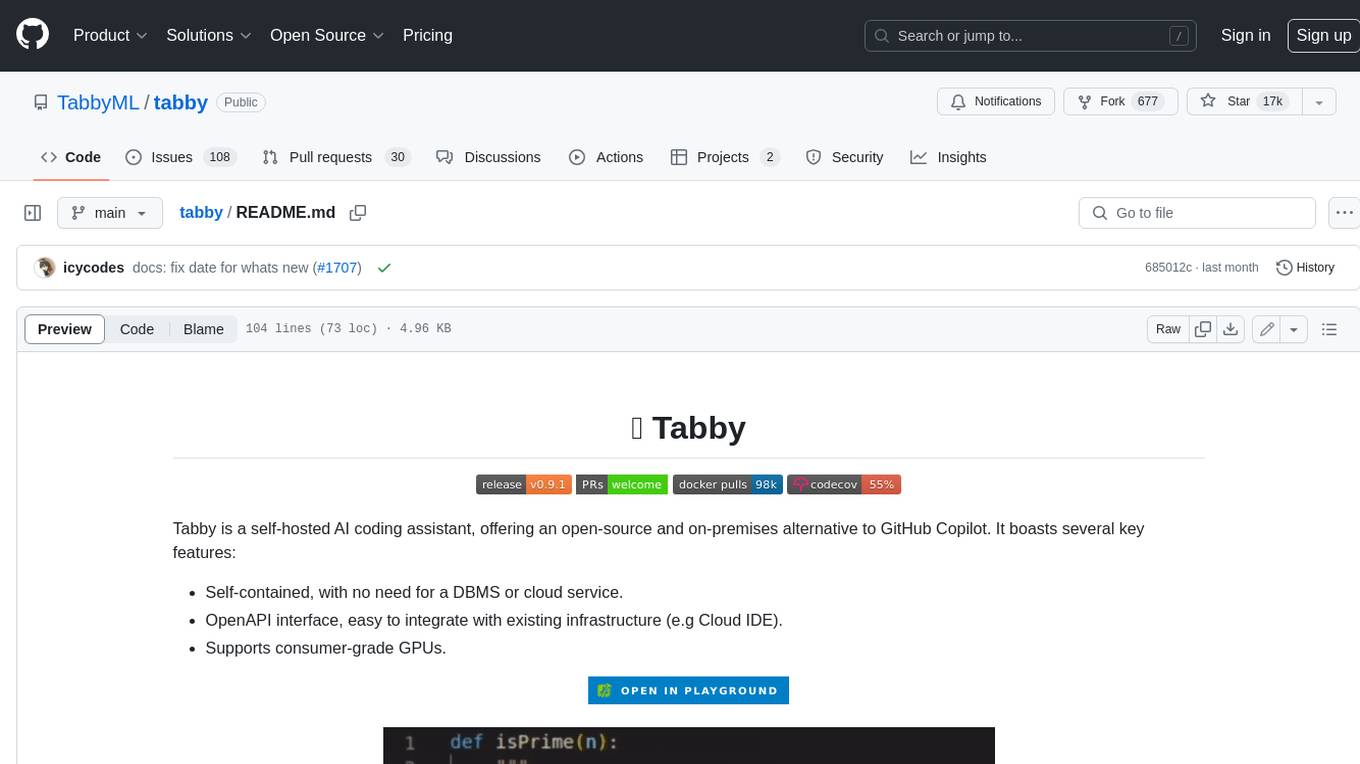
tabby
Tabby is a self-hosted AI coding assistant, offering an open-source and on-premises alternative to GitHub Copilot. It boasts several key features: * Self-contained, with no need for a DBMS or cloud service. * OpenAPI interface, easy to integrate with existing infrastructure (e.g Cloud IDE). * Supports consumer-grade GPUs.
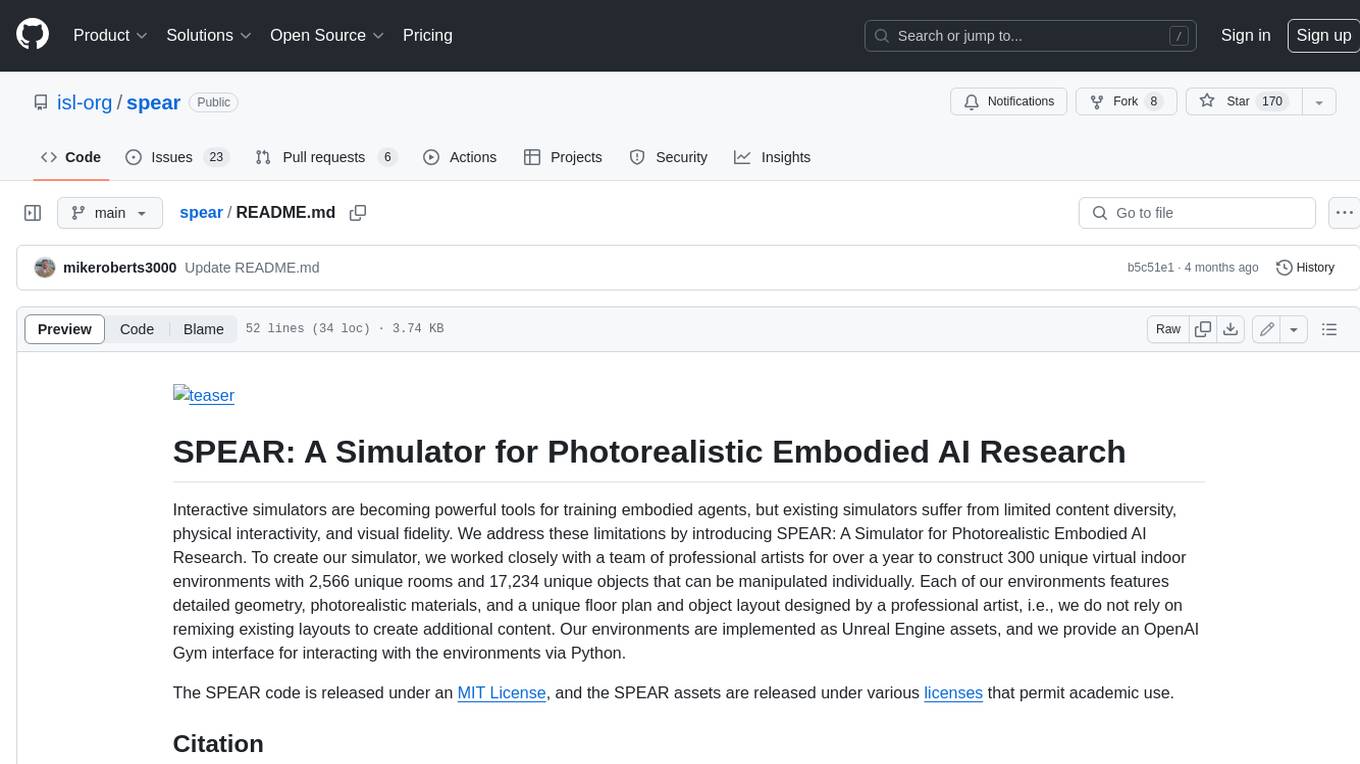
spear
SPEAR (Simulator for Photorealistic Embodied AI Research) is a powerful tool for training embodied agents. It features 300 unique virtual indoor environments with 2,566 unique rooms and 17,234 unique objects that can be manipulated individually. Each environment is designed by a professional artist and features detailed geometry, photorealistic materials, and a unique floor plan and object layout. SPEAR is implemented as Unreal Engine assets and provides an OpenAI Gym interface for interacting with the environments via Python.
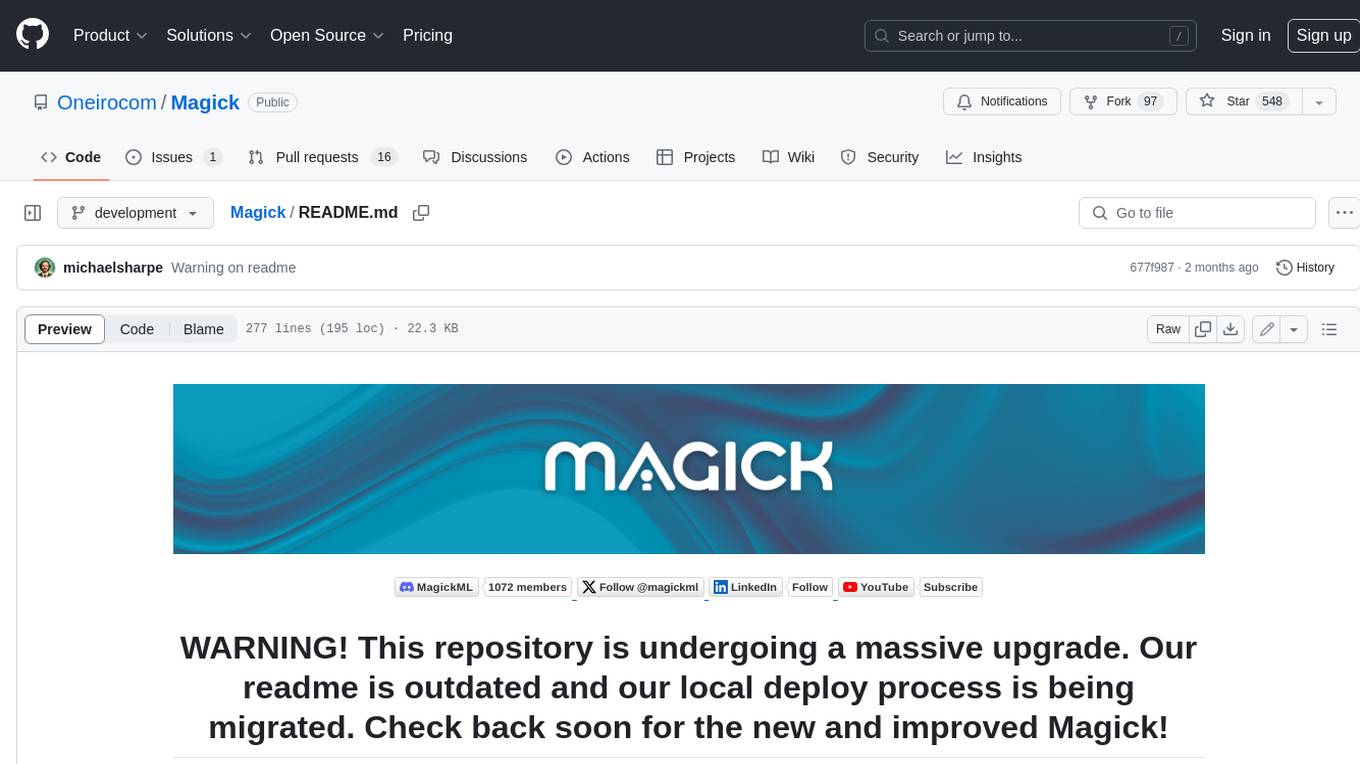
Magick
Magick is a groundbreaking visual AIDE (Artificial Intelligence Development Environment) for no-code data pipelines and multimodal agents. Magick can connect to other services and comes with nodes and templates well-suited for intelligent agents, chatbots, complex reasoning systems and realistic characters.