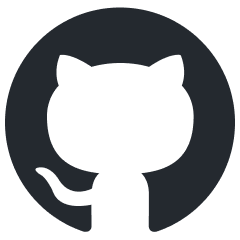
zipnn
A Lossless Compression Library for AI pipelines
Stars: 217
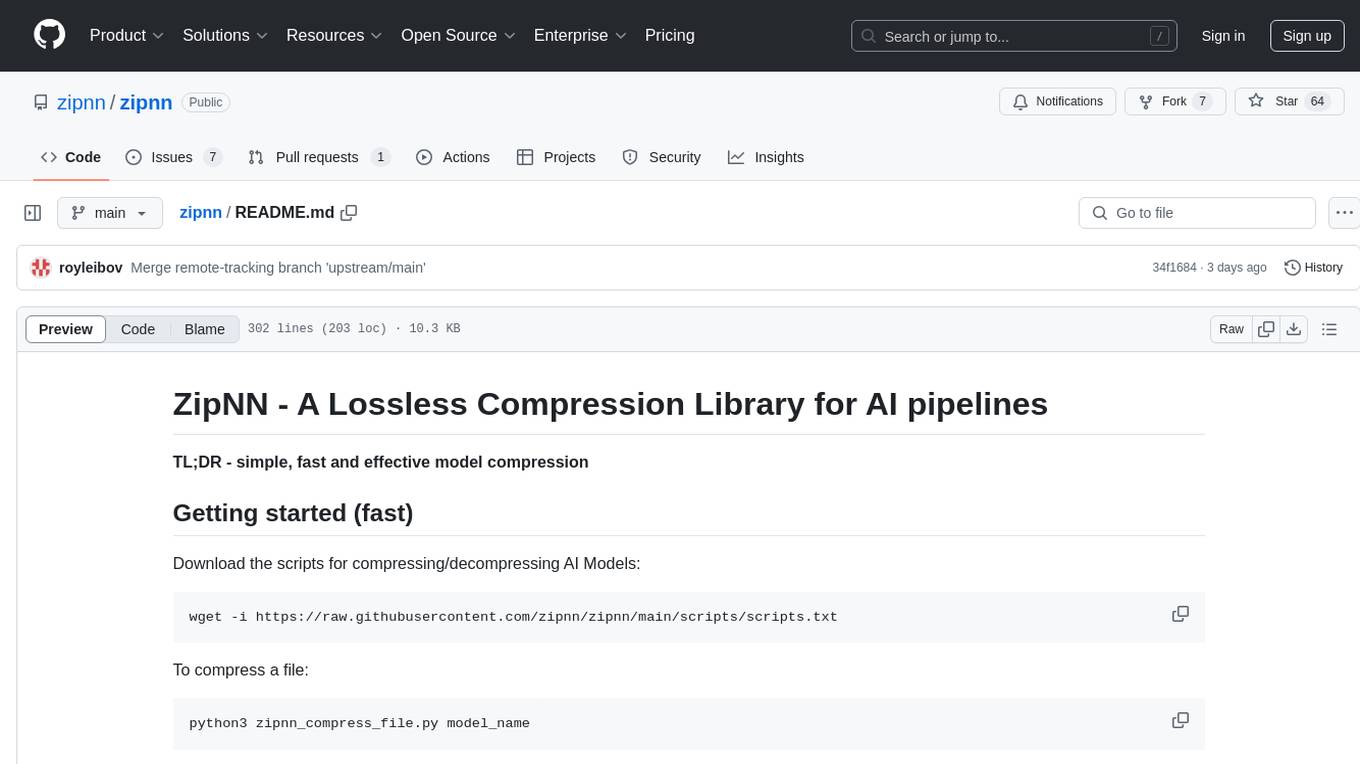
ZipNN is a lossless and near-lossless compression library optimized for numbers/tensors in the Foundation Models environment. It automatically prepares data for compression based on its type, allowing users to focus on core tasks without worrying about compression complexities. The library delivers effective compression techniques for different data types and structures, achieving high compression ratios and rates. ZipNN supports various compression methods like ZSTD, lz4, and snappy, and provides ready-made scripts for file compression/decompression. Users can also manually import the package to compress and decompress data. The library offers advanced configuration options for customization and validation tests for different input and compression types.
README:
TL;DR - simple, fast, and effective model compression.
arXiv Paper: "ZipNN: Lossless Compression for AI Models"
The default is set to the number of logical CPU threads.
Note: For compression, you might want to reduce the number of threads depending on your machine.
- NEW: HuggingFace Integration
- Getting Started
- Introduction
- Results
- Usage
- Examples
- Configuration
- Validation
- Support And Questions
- Contribution
- Citation
- Change Log
You can now choose to save the model compressed on your local storage by using the default plugin. When loading, the model includes a fast decompression phase on the CPU while remaining compressed on your storage.
What this means: Each time you load the model, less data is transferred to the GPU cluster, with decompression happening on the CPU.
zipnn_hf()
Alternatively, you can save the model uncompressed on your local storage. This way, future loads won’t require a decompression phase.
zipnn_hf(replace_local_file=True)
Click here to see full Hugging Face integration documentation, and to try state-of-the-art compressed models that are already present on HuggingFace, such as Roberta Base, Granite 3.0, Llama 3.2.
You can also try one of these python notebooks hosted on Kaggle: granite 3b, Llama 3.2, phi 3.5.
Download the scripts for compressing/decompressing AI Models:
wget -i https://raw.githubusercontent.com/zipnn/zipnn/main/scripts/scripts.txt
To compress a file:
python3 zipnn_compress_file.py model_name
To decompress a file:
python3 zipnn_decompress_file.py compressed_model_name.znn
In the realm of data compression, achieving a high compression/decompression ratio often requires careful consideration of the data types and the nature of the datasets being compressed. For instance, different strategies may be optimal for floating-point numbers compared to integers, and datasets in monotonic order may benefit from distinct preparations.
ZipNN (The NN stands for Neural Networks) is a lossless compression library optimized for numbers/tensors in the Foundation Models environment, designed to automatically prepare the data for compression according to its type. By simply calling zipnn.compress(data), users can rely on the package to apply the most effective compression technique under the hood.
Given a specific data set, ZipNN automatically rearranges the data according to it's type, and applies the most effective techniques for the given instance to improve compression ratios and speed. It is especially effective for BF16 models, typically saving 33% of the model size, whereas with models of type FP32 it usually reduces the model size by 17%.
Some of the techniques employed in ZipNN are described in our paper: Lossless and Near-Lossless Compression for Foundation Models A follow up version with a more complete description is under preparation.
Currently, ZipNN compression methods are implemented on CPUs, and GPU implementations are on the way.
Below is a comparison of compression results between ZipNN and several other methods on bfloat16 data.
Compressor name | Compression ratio / Output size | Compression Throughput | Decompression Throughput |
---|---|---|---|
ZipNN v0.2.0 | 1.51 / 66.3% | 1120MB/sec | 1660MB/sec |
ZSTD v1.56 | 1.27 / 78.3% | 785MB/sec | 950MB/sec |
LZ4 | 1 / 100% | --- | --- |
Snappy | 1 / 100% | --- | --- |
- Gzip, Zlib compression rate are similar to ZSTD, but much slower.
- The above results are for a single-threaded compression (Working with chunks size of 256KB).
- Similar results with other BF16 Models such as Mistral, Lamma-3, Lamma-3.1, Arcee-Nova and Jamba.
pip install zipnn
This project requires the numpy, zstandard and torch python packages.
You can integrate zipnn compression and decompression into your own projects by utilizing the scripts available in the scripts folder. This folder contains the following scripts:
-
zipnn_compress_file.py
: For compressing an individual file. -
zipnn_decompress_file.py
: For decompressing an individual file. -
zipnn_compress_path.py
: For compressing all files under a path. -
zipnn_decompress_path.py
: For decompressing all files under a path.
Compress one file:
python zipnn_compress_file.py model_name
Decompress one file:
python zipnn_decompress_file.py model_name.znn
For detailed information on how to use these scripts, please refer to the README.md file located in the scripts folder.
In this example, ZipNN compress and decompress 1GB of the Granite model and validate that the original file and the decompressed file are equal.
The script reads the file and compresses and decompresses in Byte format.
> python3 simple_example_granite.py
...
Are the original and decompressed byte strings the same [BYTE]? True
Similar examples demonstrating compression and decompression for Byte and Torch formats are included within the package.
> python3 simple_example_byte.py
...
Are the original and decompressed byte strings the same [BYTE]? True
> python3 simple_example_torch.py
...
Are the original and decompressed byte strings the same [TORCH]? True
The default configuration is ByteGrouping of 4 with vanilla ZSTD, and the input and outputs are "byte". For more advanced options, please consider the following parameters:
-
method
: Compression method, Supporting zstd, lz4, huffman and auto which chooses the best compression method automaticaly (default value = 'auto'). -
input_format
: The input data format, can be one of the following: torch, numpy, byte (default value = 'byte'). -
bytearray_dtype
: The data type of the byte array, if input_format is 'byte'. If input_format is torch or numpy, the dtype will be derived from the data automatically (default value = 'bfloat16'). -
threads
: The maximum threads for the compression and the bit manipulation. (default value = maximal amount of threads). -
compression_threshold
: Save original buffer if not compress above the threshold (default value = 0.95). -
check_th_after_percent
: Check the compression threshold after % from the number of chunk and stop compressing if not pass the compression_threshold. (default value = 10[%]). -
compression_chunk
: Chunk size for compression. (default value = 256KB). -
is_streaming
: A flag to compress the data using streaming. (default value = False). -
streaming_chunk
: Chunk size for streaming, only relevant if is_streaming is True. (default value = 1KB).
Run tests for Byte/File input types, Byte/File compression types, Byte/File decompression types.
python3 -m unittest discover -s tests/ -p test_suit.py
We are excited to hear your feedback! For issues and feature requests, please open a GitHub issue.
We welcome and value all contributions to the project! You can contact us in this email: [email protected]
If you use zipnn
in your research or projects, please cite the repository:
@misc{hershcovitch2024zipnnlosslesscompressionai,
title={ZipNN: Lossless Compression for AI Models},
author={Moshik Hershcovitch and Andrew Wood and Leshem Choshen and Guy Girmonsky and Roy Leibovitz and Ilias Ennmouri and Michal Malka and Peter Chin and Swaminathan Sundararaman and Danny Harnik},
year={2024},
eprint={2411.05239},
archivePrefix={arXiv},
primaryClass={cs.LG},
url={https://arxiv.org/abs/2411.05239},
}
- Add multithreading support on the CPU for both compression and decompression, with the default set to the number of logical CPU threads.
-
Update the Hugging Face plugin to support loading compressed files and add an option to save them uncompressed.
-
Fix the Hugging Face plugin to support different versions of Hugging Face Transformers.
- Fix bug that causes memory leaks in corner cases
- Add float32 to the C implementation with Huffman compression.
-
Plugin for Hugging Face transformers to allow using from_pretrained and decompressing the model after downloading it from Hugging Face.
-
Add Delta compression support in python -> save Xor between two models and compress them).
- Change ZipNN suffix from .zpn to .znn
-
Prepare dtype16 (BF16 and FP16) for multi-threading by changing its C logic. For each chunk, byte ordering, bit ordering, and compression are processed separately.
-
Integrate the Streaming support into zipnn python code.
-
Add support for Streaming when using outside scripts
-
Fix bug: Compression didn't work when compressing files larger than 3GB
-
Change the byte ordering implementation to C (for better performance).
-
Change the bfloat16/float16 implementation to a C implementation with Huffman encoding, running on chunks of 256KB each.
-
Float 32 using ZSTD compression as in v0.1.1
-
Add support with uint32 with ZSTD compression.
- Python implementation of compressing Models, float32, float15, bfloat16 with byte ordering and ZSTD.
For Tasks:
Click tags to check more tools for each tasksFor Jobs:
Alternative AI tools for zipnn
Similar Open Source Tools
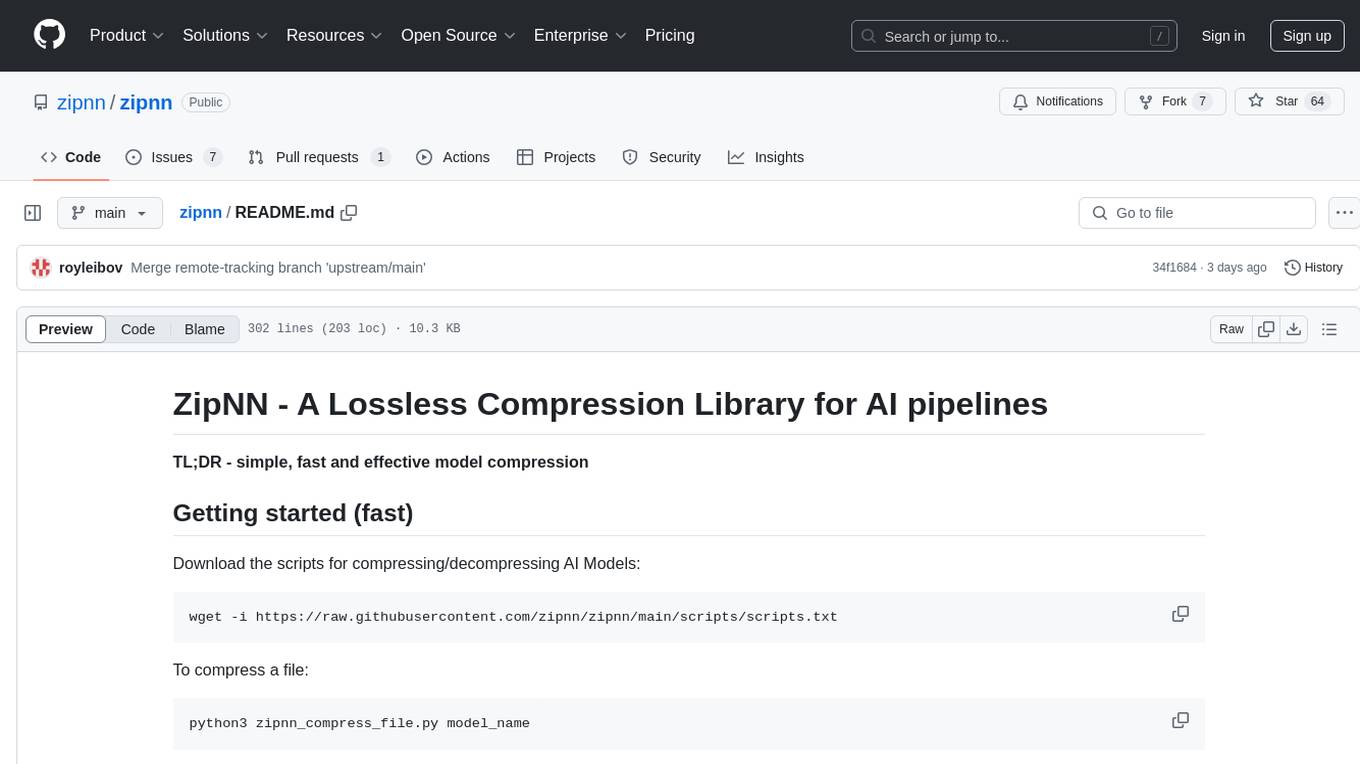
zipnn
ZipNN is a lossless and near-lossless compression library optimized for numbers/tensors in the Foundation Models environment. It automatically prepares data for compression based on its type, allowing users to focus on core tasks without worrying about compression complexities. The library delivers effective compression techniques for different data types and structures, achieving high compression ratios and rates. ZipNN supports various compression methods like ZSTD, lz4, and snappy, and provides ready-made scripts for file compression/decompression. Users can also manually import the package to compress and decompress data. The library offers advanced configuration options for customization and validation tests for different input and compression types.
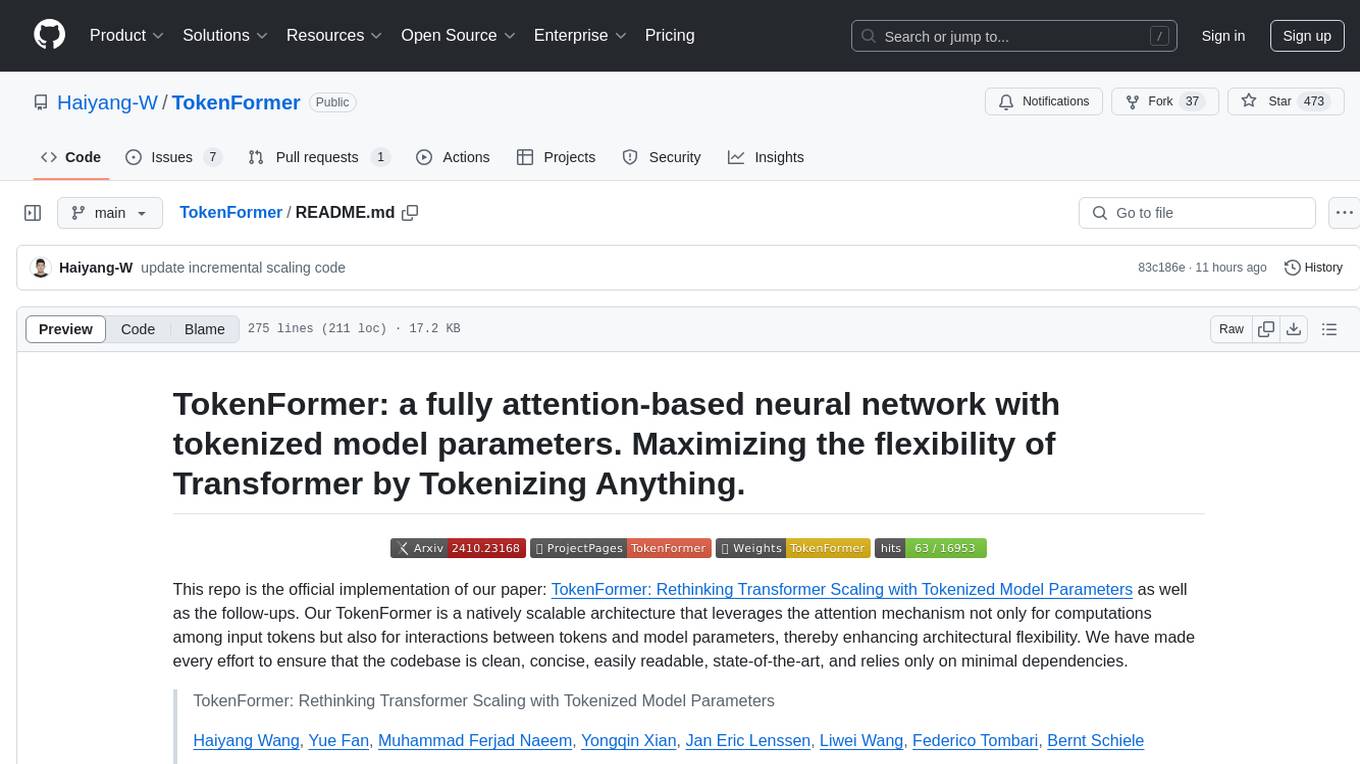
TokenFormer
TokenFormer is a fully attention-based neural network architecture that leverages tokenized model parameters to enhance architectural flexibility. It aims to maximize the flexibility of neural networks by unifying token-token and token-parameter interactions through the attention mechanism. The architecture allows for incremental model scaling and has shown promising results in language modeling and visual modeling tasks. The codebase is clean, concise, easily readable, state-of-the-art, and relies on minimal dependencies.
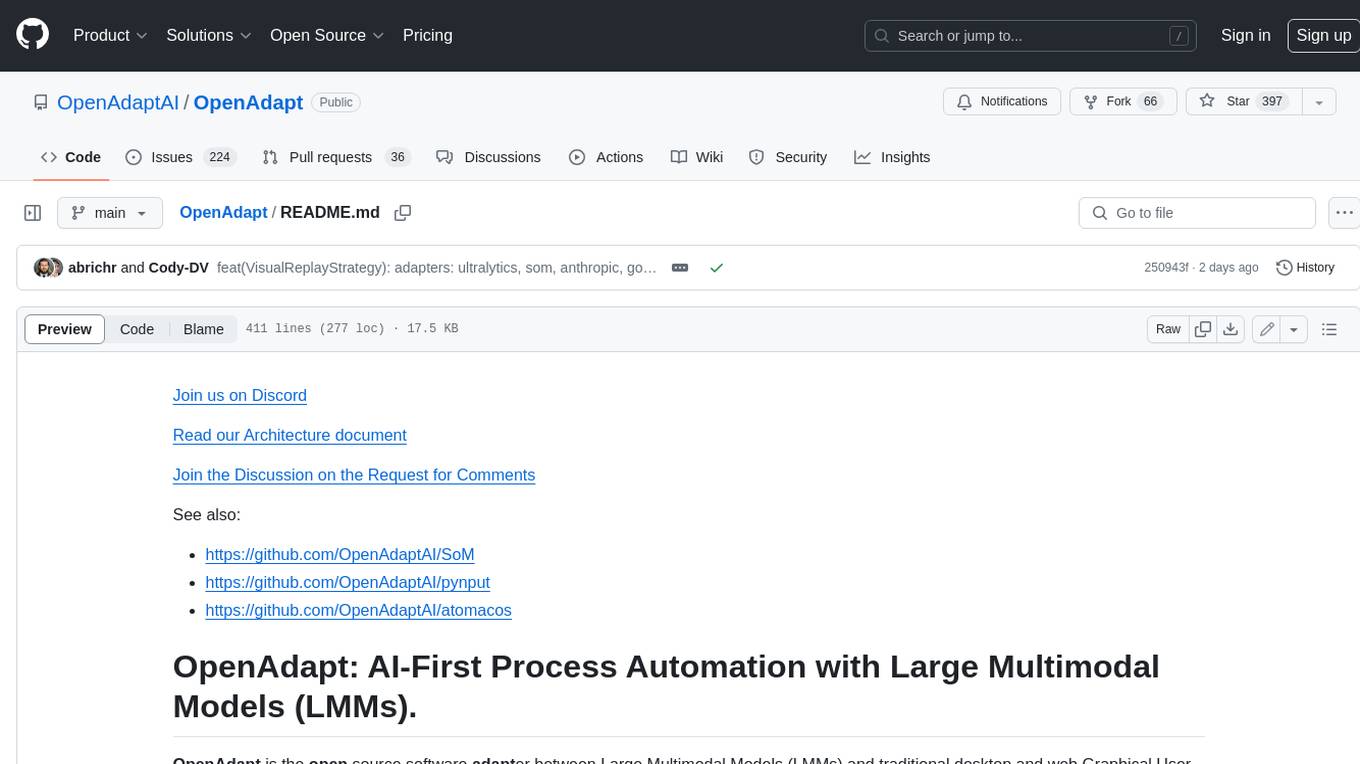
OpenAdapt
OpenAdapt is an open-source software adapter between Large Multimodal Models (LMMs) and traditional desktop and web Graphical User Interfaces (GUIs). It aims to automate repetitive GUI workflows by leveraging the power of LMMs. OpenAdapt records user input and screenshots, converts them into tokenized format, and generates synthetic input via transformer model completions. It also analyzes recordings to generate task trees and replay synthetic input to complete tasks. OpenAdapt is model agnostic and generates prompts automatically by learning from human demonstration, ensuring that agents are grounded in existing processes and mitigating hallucinations. It works with all types of desktop GUIs, including virtualized and web, and is open source under the MIT license.
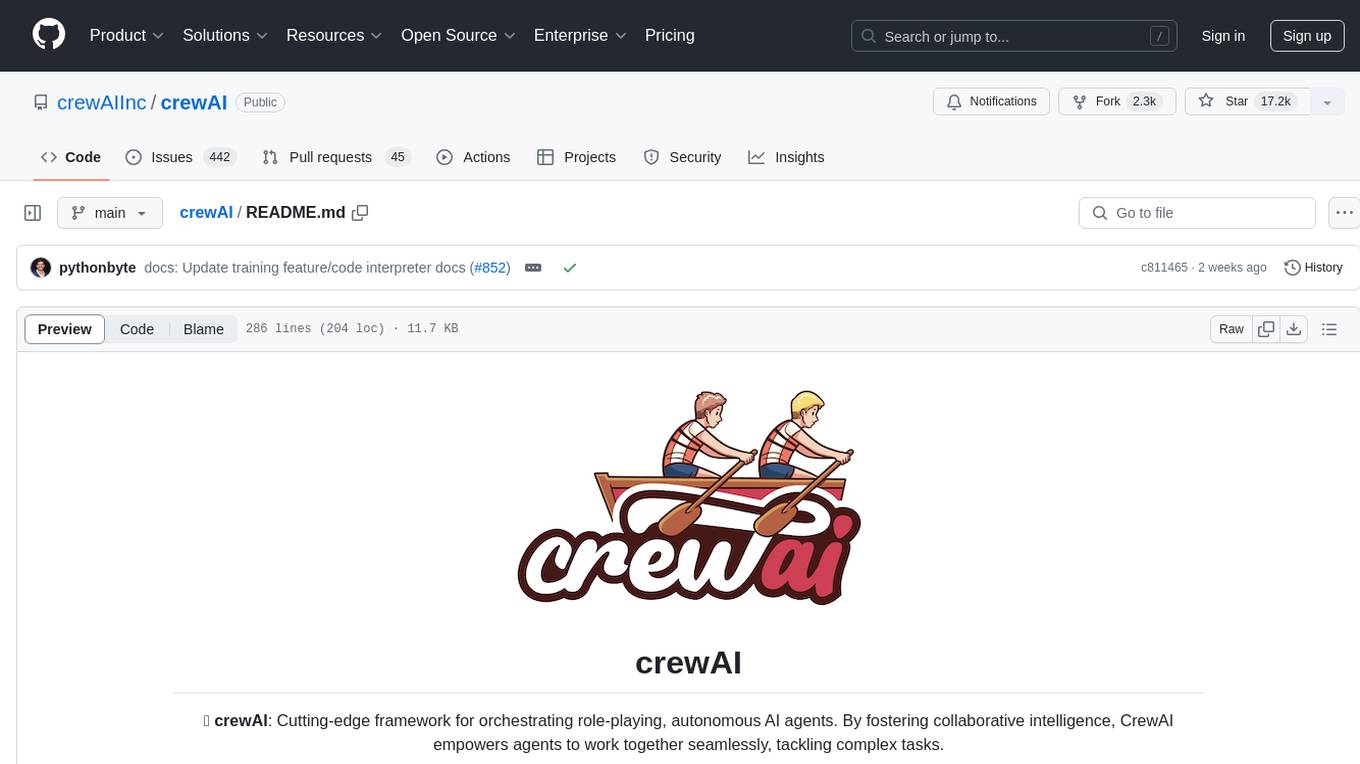
crewAI
CrewAI is a cutting-edge framework designed to orchestrate role-playing autonomous AI agents. By fostering collaborative intelligence, CrewAI empowers agents to work together seamlessly, tackling complex tasks. It enables AI agents to assume roles, share goals, and operate in a cohesive unit, much like a well-oiled crew. Whether you're building a smart assistant platform, an automated customer service ensemble, or a multi-agent research team, CrewAI provides the backbone for sophisticated multi-agent interactions. With features like role-based agent design, autonomous inter-agent delegation, flexible task management, and support for various LLMs, CrewAI offers a dynamic and adaptable solution for both development and production workflows.
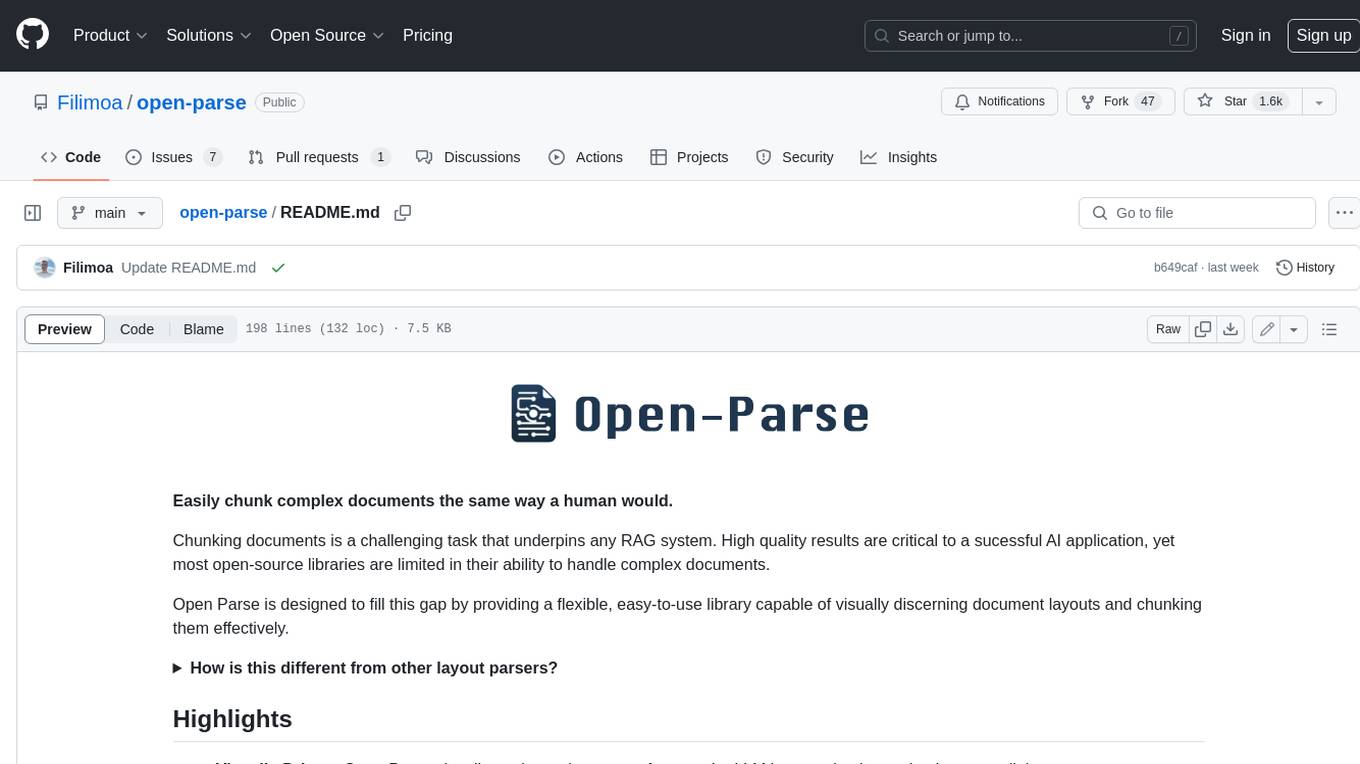
open-parse
Open Parse is a Python library for visually discerning document layouts and chunking them effectively. It is designed to fill the gap in open-source libraries for handling complex documents. Unlike text splitting, which converts a file to raw text and slices it up, Open Parse visually analyzes documents for superior LLM input. It also supports basic markdown for parsing headings, bold, and italics, and has high-precision table support, extracting tables into clean Markdown formats with accuracy that surpasses traditional tools. Open Parse is extensible, allowing users to easily implement their own post-processing steps. It is also intuitive, with great editor support and completion everywhere, making it easy to use and learn.
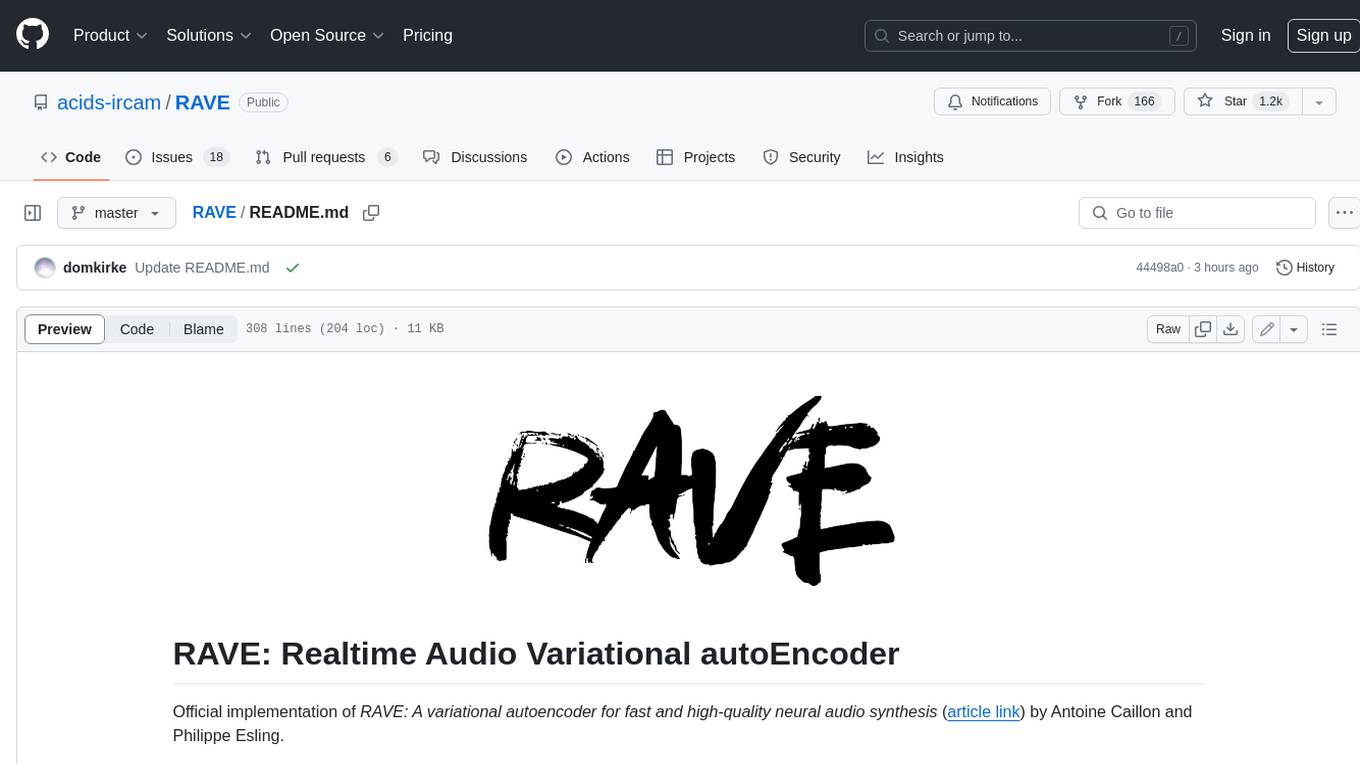
RAVE
RAVE is a variational autoencoder for fast and high-quality neural audio synthesis. It can be used to generate new audio samples from a given dataset, or to modify the style of existing audio samples. RAVE is easy to use and can be trained on a variety of audio datasets. It is also computationally efficient, making it suitable for real-time applications.
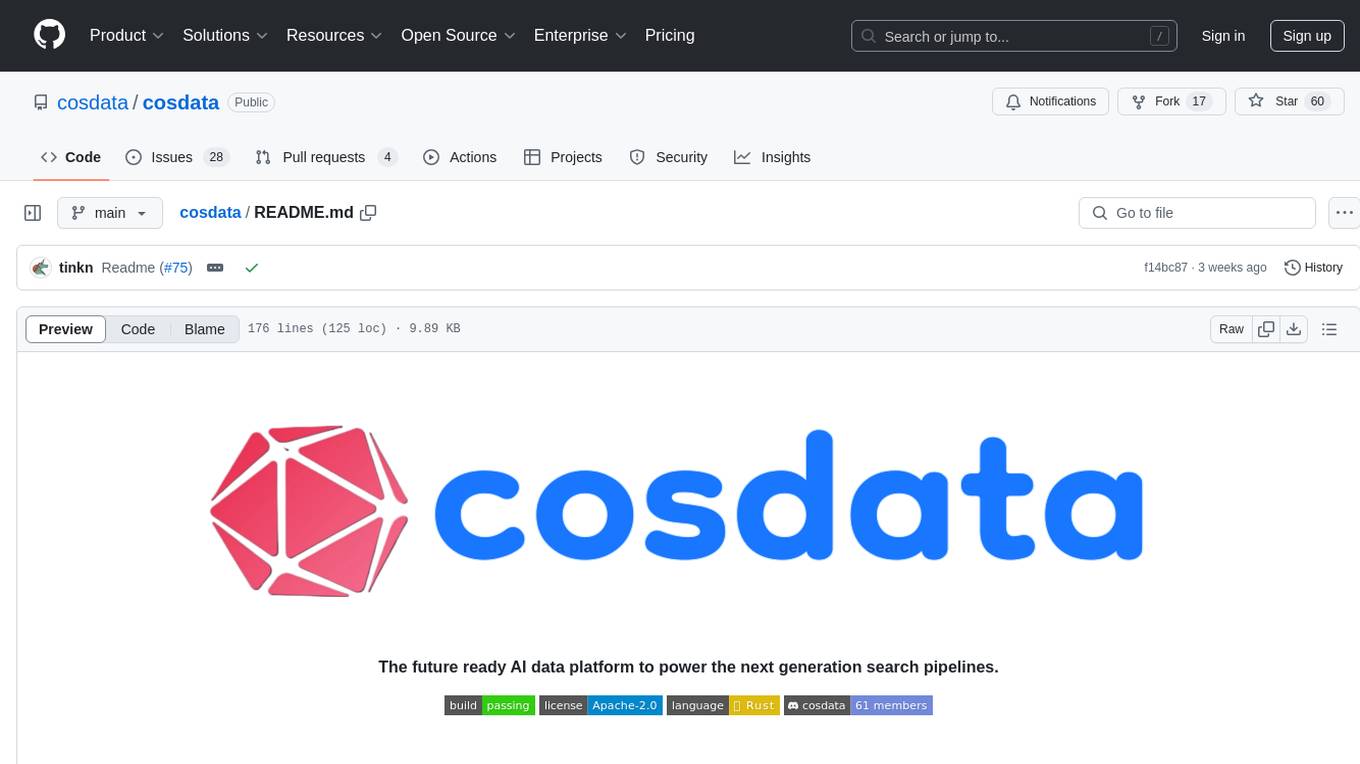
cosdata
Cosdata is a cutting-edge AI data platform designed to power the next generation search pipelines. It features immutability, version control, and excels in semantic search, structured knowledge graphs, hybrid search capabilities, real-time search at scale, and ML pipeline integration. The platform is customizable, scalable, efficient, enterprise-grade, easy to use, and can manage multi-modal data. It offers high performance, indexing, low latency, and high requests per second. Cosdata is designed to meet the demands of modern search applications, empowering businesses to harness the full potential of their data.
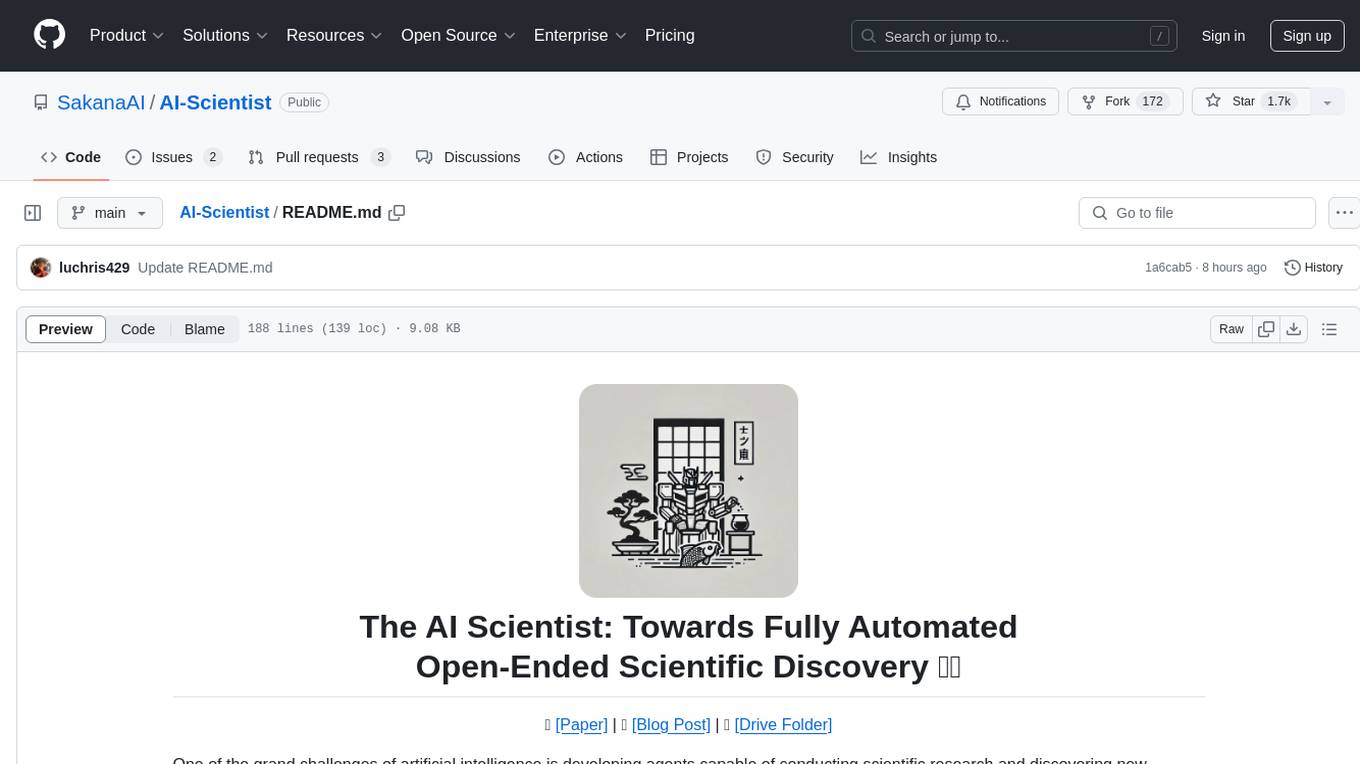
AI-Scientist
The AI Scientist is a comprehensive system for fully automatic scientific discovery, enabling Foundation Models to perform research independently. It aims to tackle the grand challenge of developing agents capable of conducting scientific research and discovering new knowledge. The tool generates papers on various topics using Large Language Models (LLMs) and provides a platform for exploring new research ideas. Users can create their own templates for specific areas of study and run experiments to generate papers. However, caution is advised as the codebase executes LLM-written code, which may pose risks such as the use of potentially dangerous packages and web access.
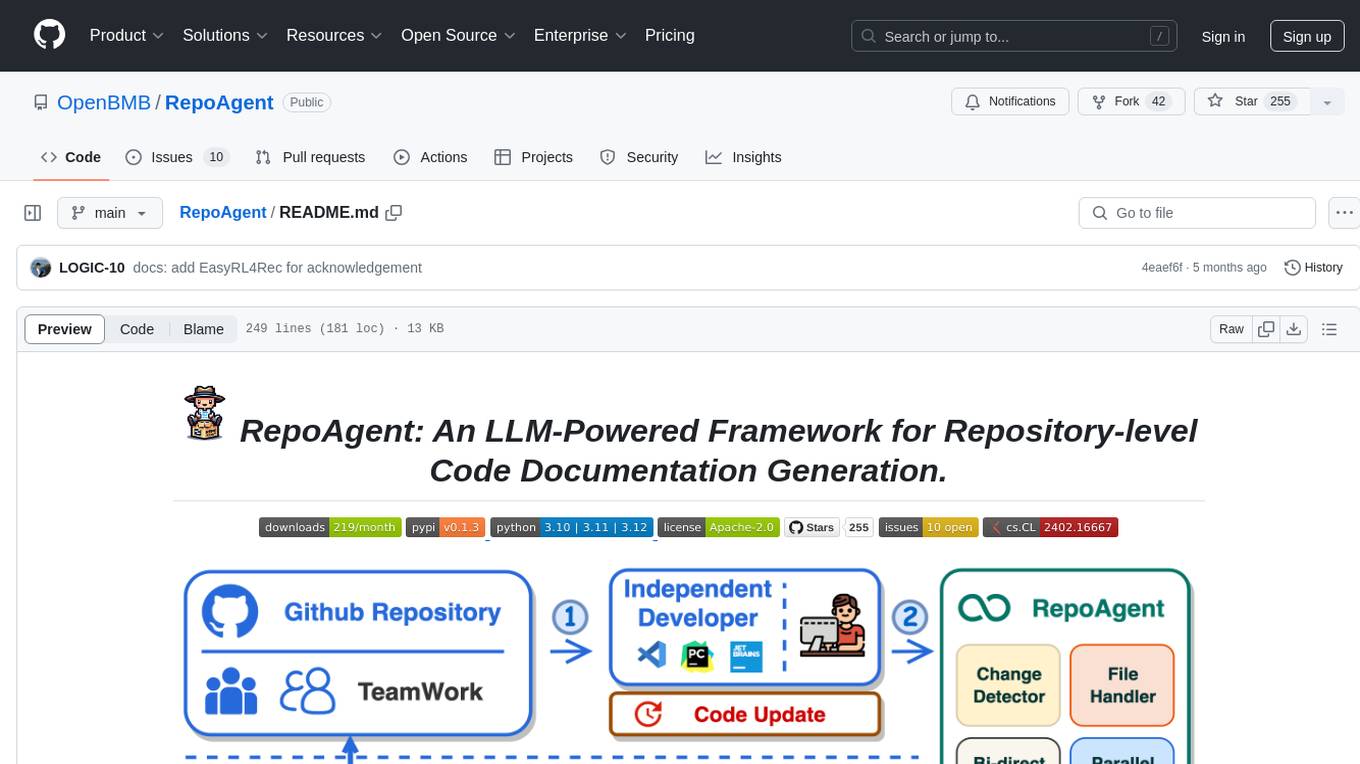
RepoAgent
RepoAgent is an LLM-powered framework designed for repository-level code documentation generation. It automates the process of detecting changes in Git repositories, analyzing code structure through AST, identifying inter-object relationships, replacing Markdown content, and executing multi-threaded operations. The tool aims to assist developers in understanding and maintaining codebases by providing comprehensive documentation, ultimately improving efficiency and saving time.
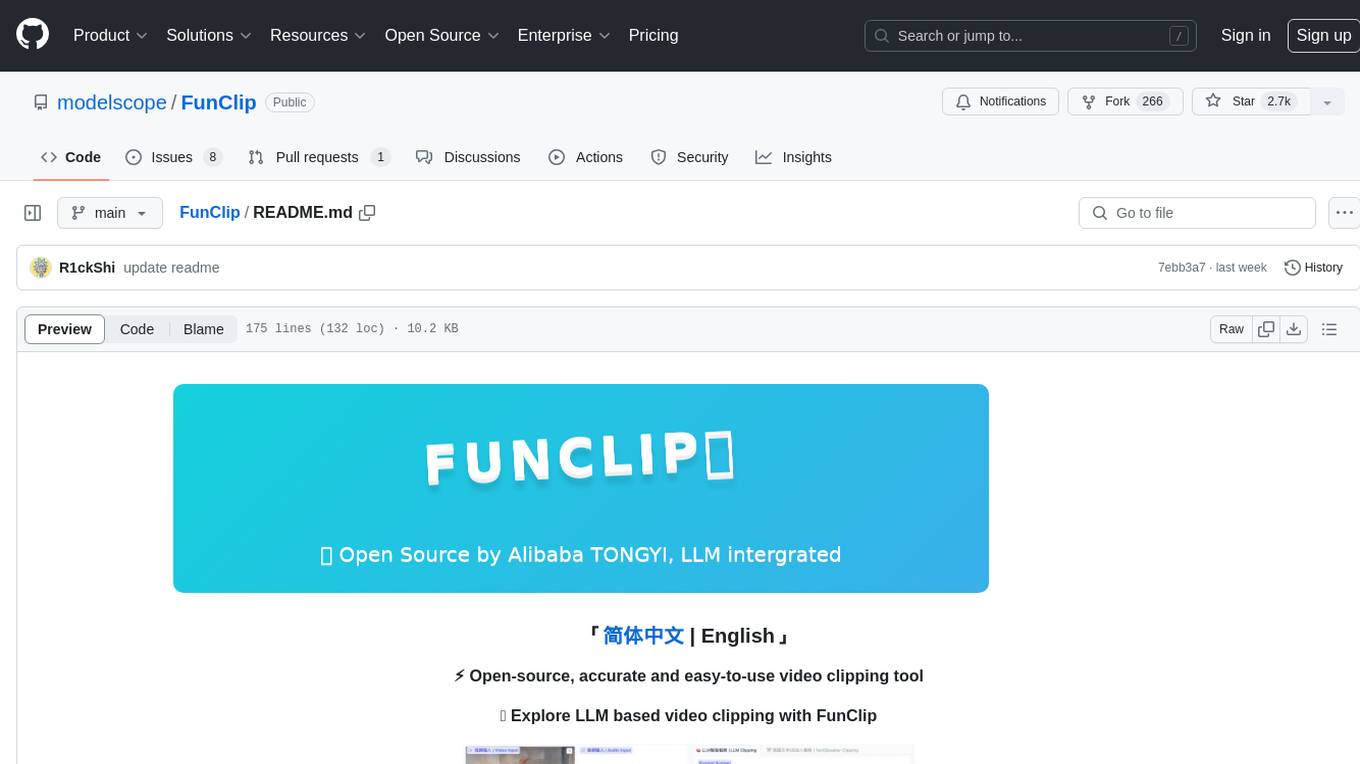
FunClip
FunClip is an open-source, locally deployed automated video clipping tool that leverages Alibaba TONGYI speech lab's FunASR Paraformer series models for speech recognition on videos. Users can select text segments or speakers from recognition results to obtain corresponding video clips. It integrates industrial-grade models for accurate predictions and offers hotword customization and speaker recognition features. The tool is user-friendly with Gradio interaction, supporting multi-segment clipping and providing full video and target segment subtitles. FunClip is suitable for users looking to automate video clipping tasks with advanced AI capabilities.
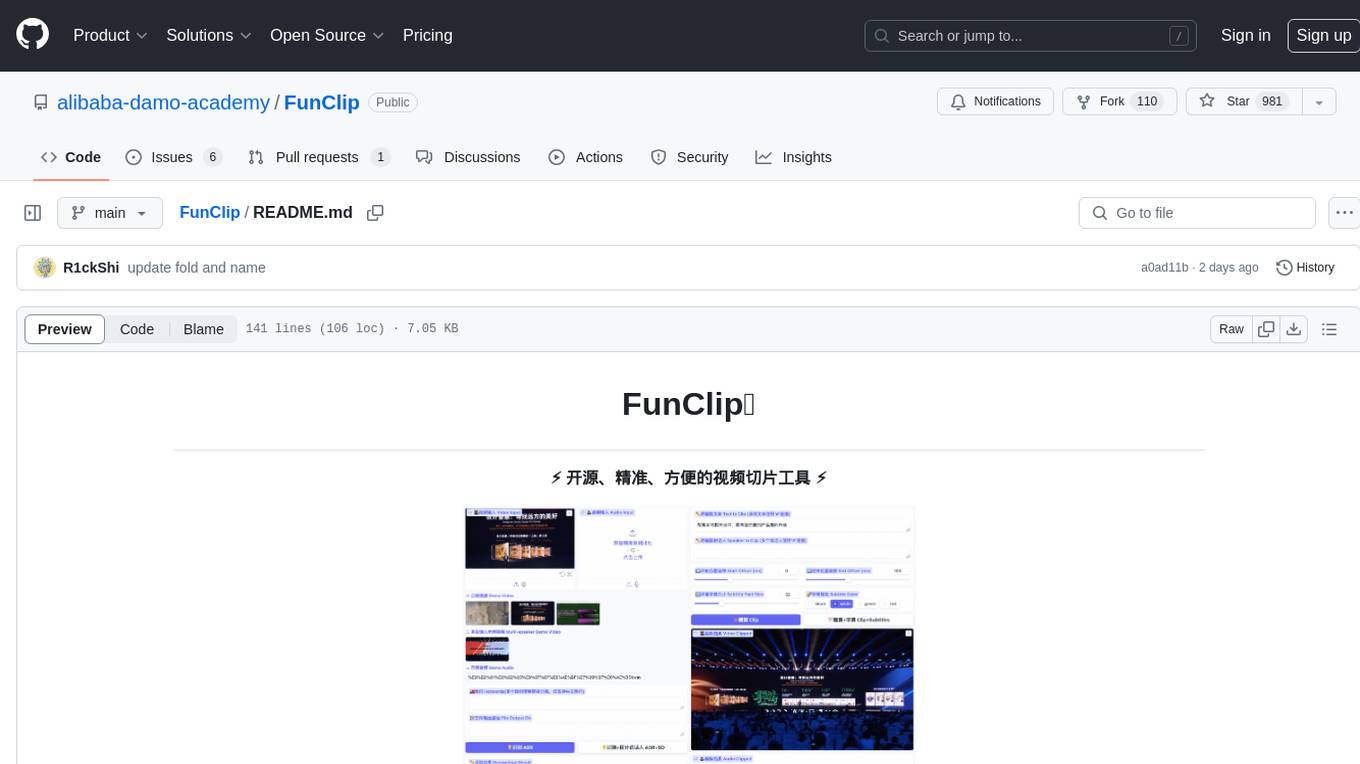
FunClip
FunClip is an open-source, locally deployable automated video editing tool that utilizes the FunASR Paraformer series models from Alibaba DAMO Academy for speech recognition in videos. Users can select text segments or speakers from the recognition results and click the clip button to obtain the corresponding video segments. FunClip integrates advanced features such as the Paraformer-Large model for accurate Chinese ASR, SeACo-Paraformer for customized hotword recognition, CAM++ speaker recognition model, Gradio interactive interface for easy usage, support for multiple free edits with automatic SRT subtitles generation, and segment-specific SRT subtitles.
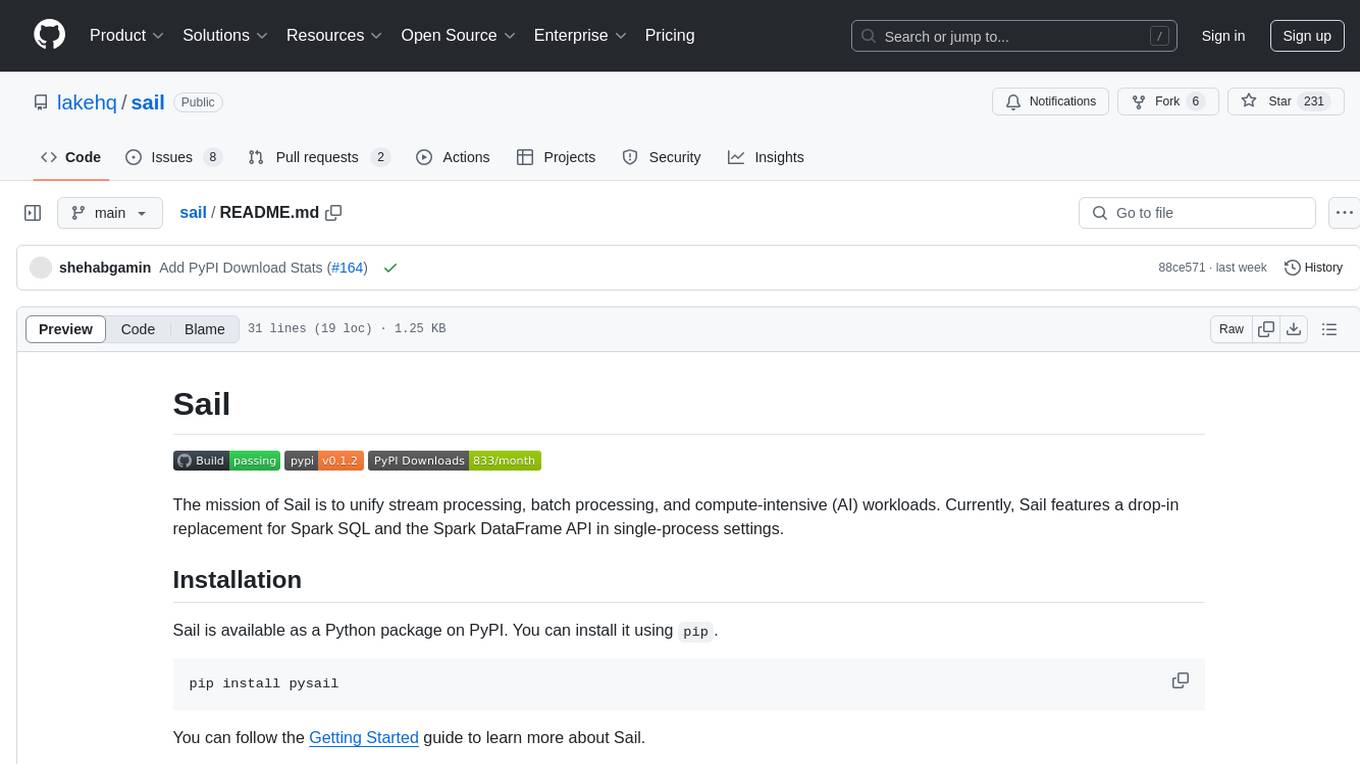
sail
Sail is a tool designed to unify stream processing, batch processing, and compute-intensive workloads, serving as a drop-in replacement for Spark SQL and the Spark DataFrame API in single-process settings. It aims to streamline data processing tasks and facilitate AI workloads.
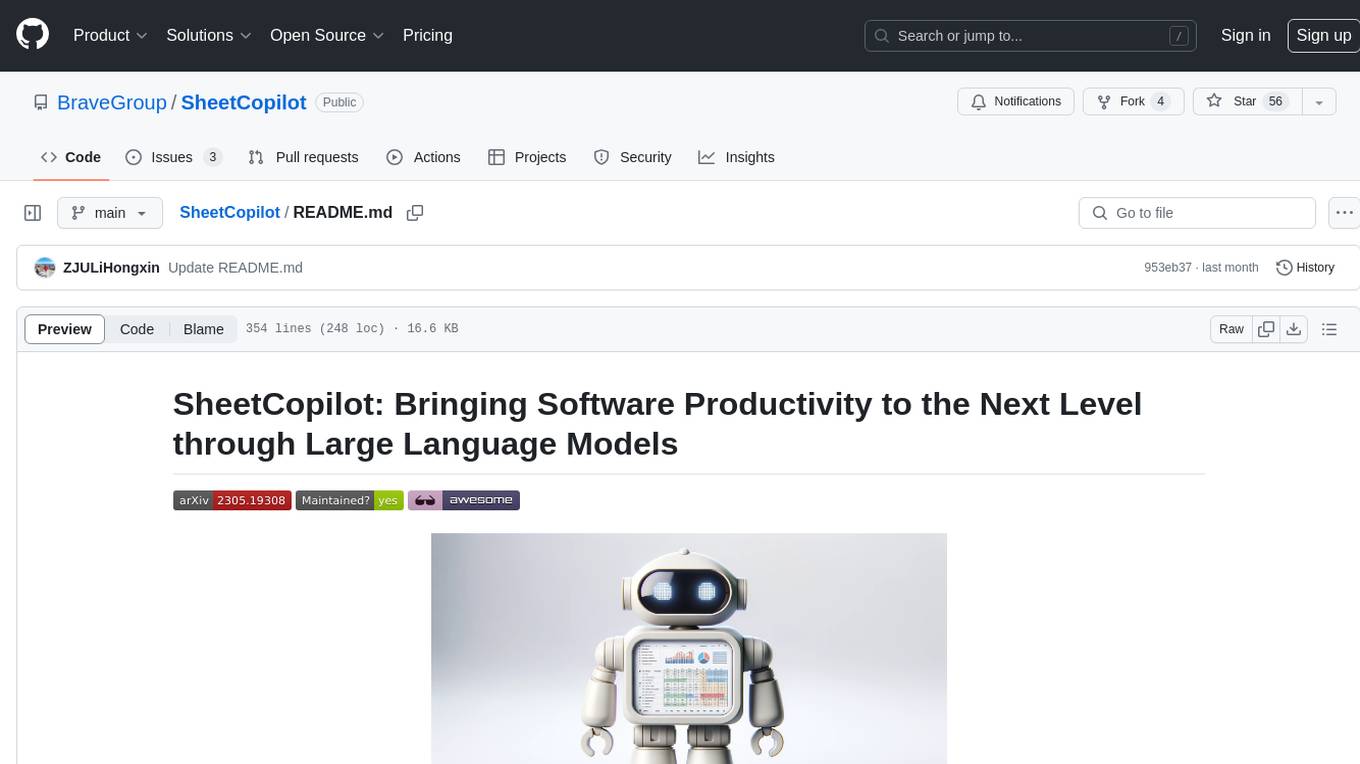
SheetCopilot
SheetCopilot is an assistant agent that manipulates spreadsheets by following user commands. It leverages Large Language Models (LLMs) to interact with spreadsheets like a human expert, enabling non-expert users to complete tasks on complex software such as Google Sheets and Excel via a language interface. The tool observes spreadsheet states, polishes generated solutions based on external action documents and error feedback, and aims to improve success rate and efficiency. SheetCopilot offers a dataset with diverse task categories and operations, supporting operations like entry & manipulation, management, formatting, charts, and pivot tables. Users can interact with SheetCopilot in Excel or Google Sheets, executing tasks like calculating revenue, creating pivot tables, and plotting charts. The tool's evaluation includes performance comparisons with leading LLMs and VBA-based methods on specific datasets, showcasing its capabilities in controlling various aspects of a spreadsheet.
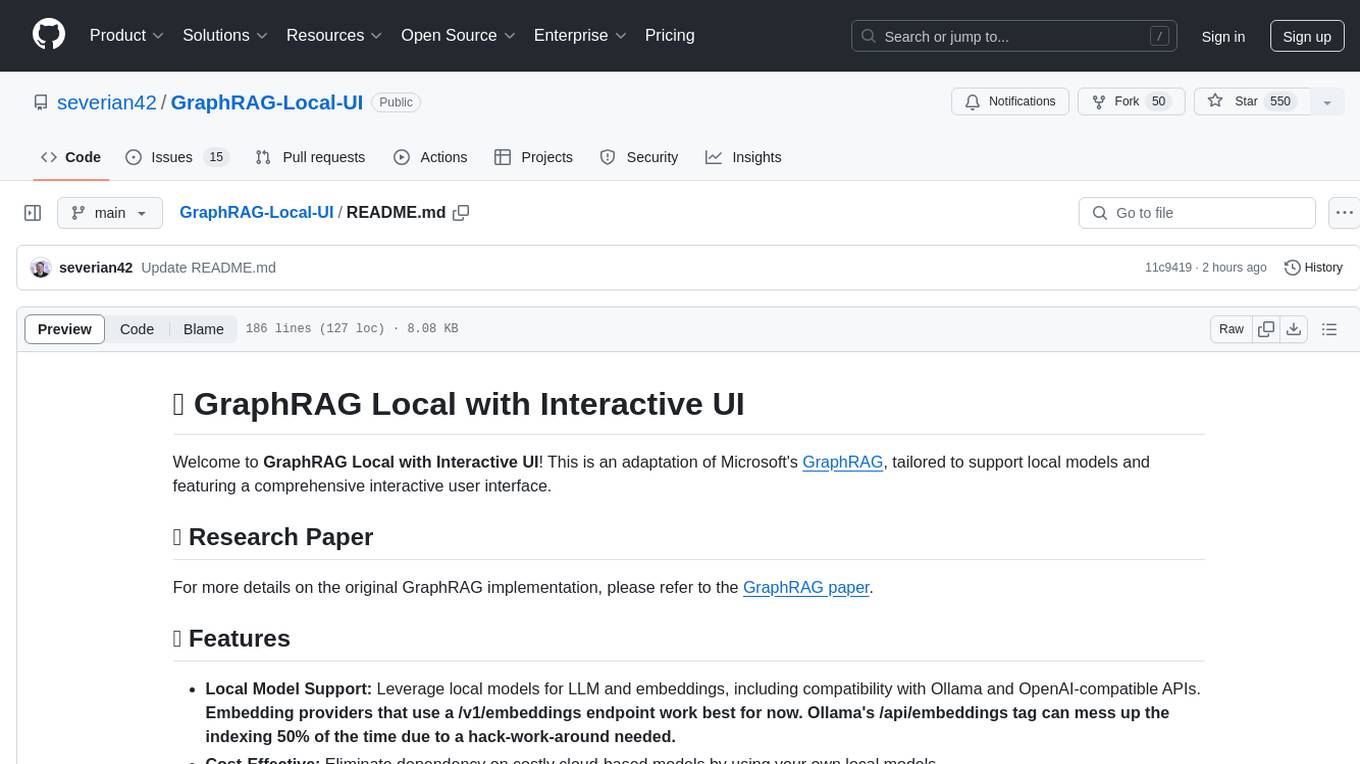
GraphRAG-Local-UI
GraphRAG Local with Interactive UI is an adaptation of Microsoft's GraphRAG, tailored to support local models and featuring a comprehensive interactive user interface. It allows users to leverage local models for LLM and embeddings, visualize knowledge graphs in 2D or 3D, manage files, settings, and queries, and explore indexing outputs. The tool aims to be cost-effective by eliminating dependency on costly cloud-based models and offers flexible querying options for global, local, and direct chat queries.
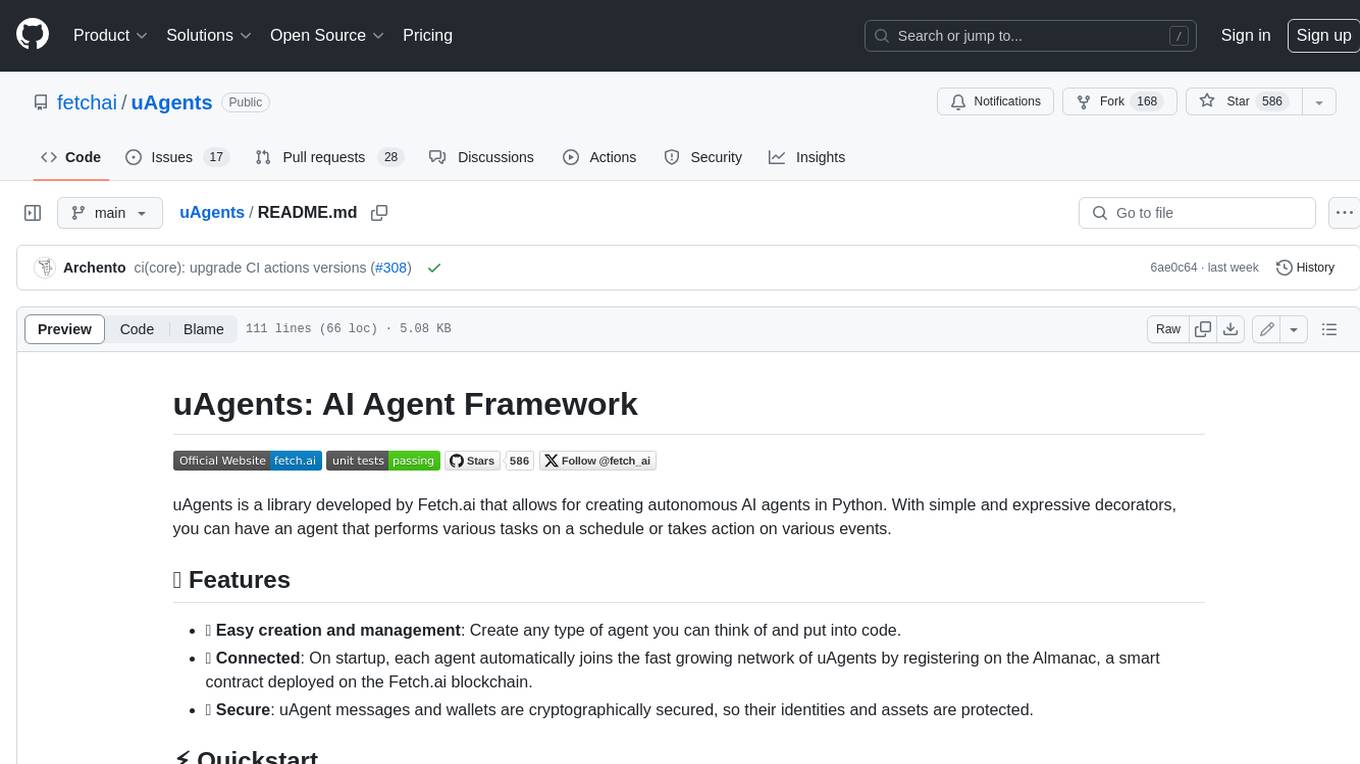
uAgents
uAgents is a Python library developed by Fetch.ai that allows for the creation of autonomous AI agents. These agents can perform various tasks on a schedule or take action on various events. uAgents are easy to create and manage, and they are connected to a fast-growing network of other uAgents. They are also secure, with cryptographically secured messages and wallets.
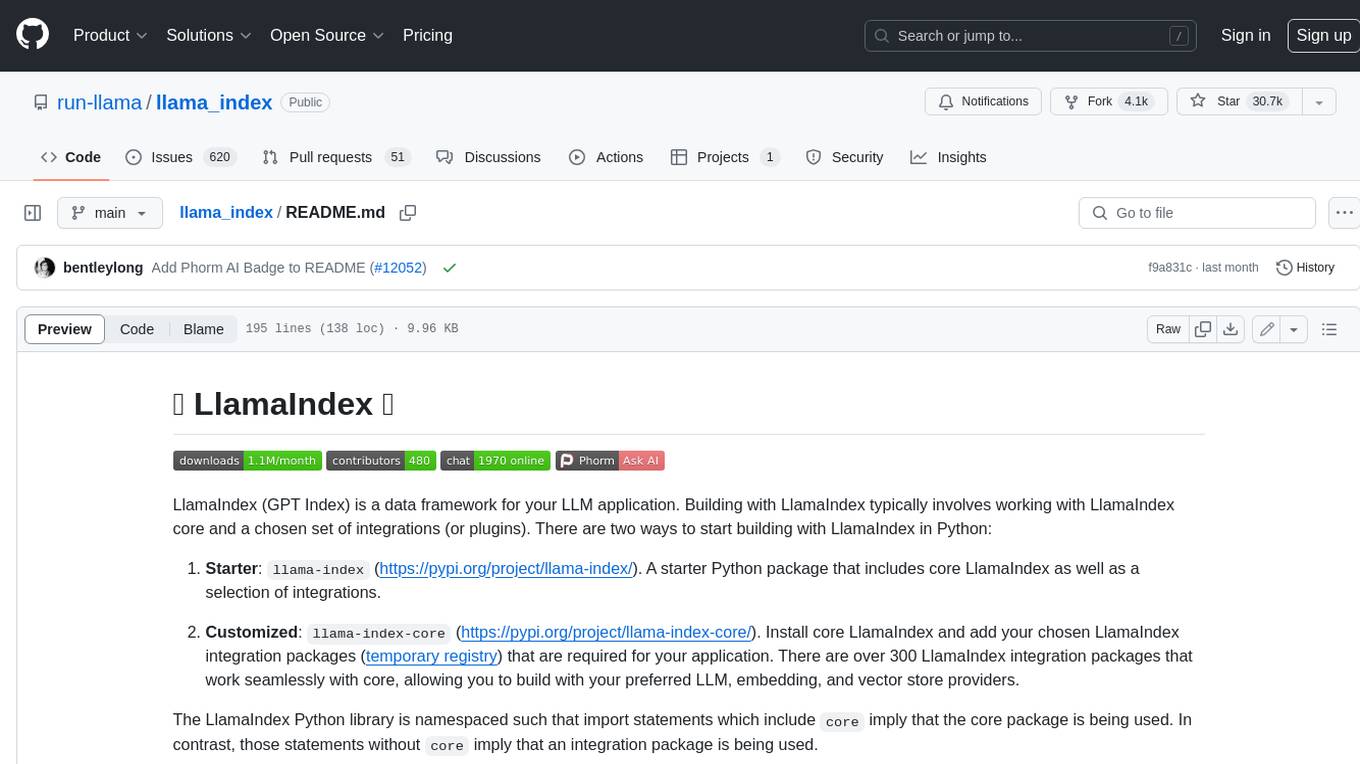
llama_index
LlamaIndex is a data framework for building LLM applications. It provides tools for ingesting, structuring, and querying data, as well as integrating with LLMs and other tools. LlamaIndex is designed to be easy to use for both beginner and advanced users, and it provides a comprehensive set of features for building LLM applications.
For similar tasks
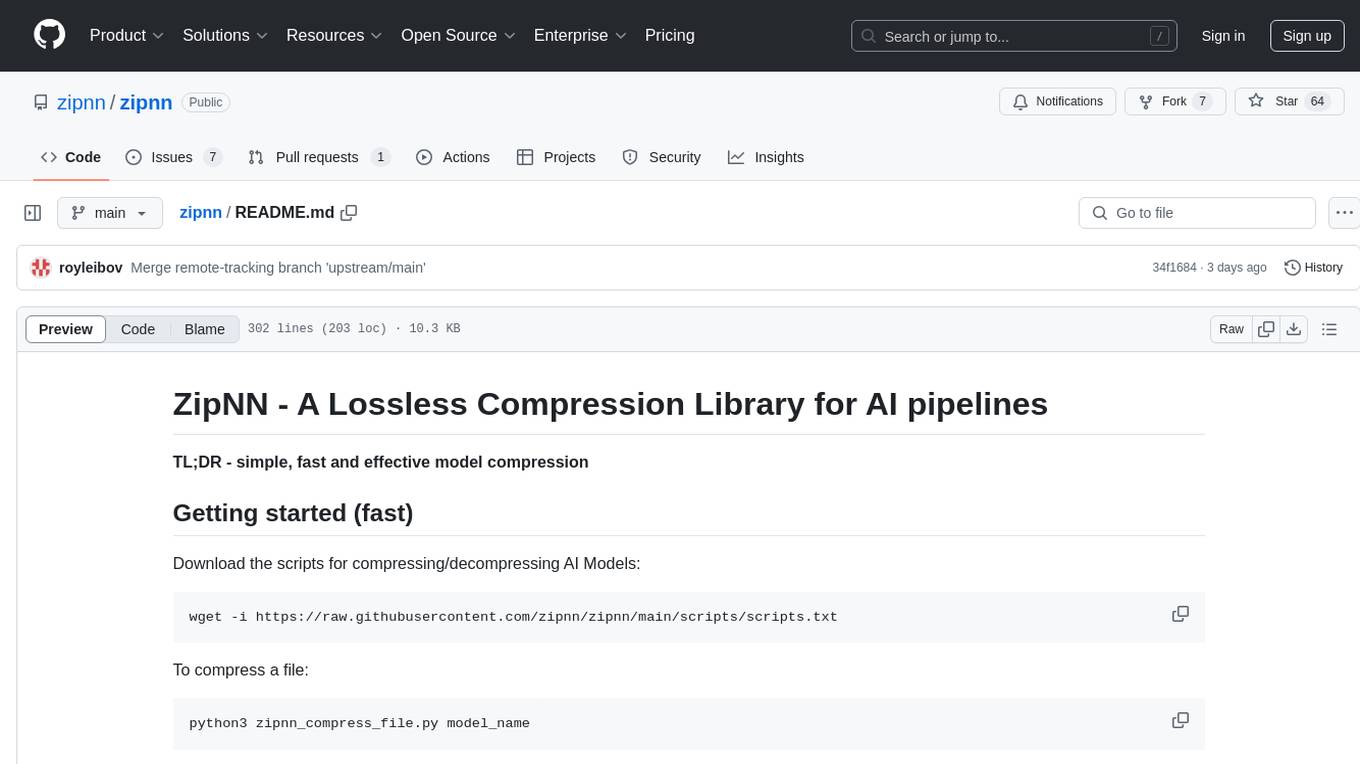
zipnn
ZipNN is a lossless and near-lossless compression library optimized for numbers/tensors in the Foundation Models environment. It automatically prepares data for compression based on its type, allowing users to focus on core tasks without worrying about compression complexities. The library delivers effective compression techniques for different data types and structures, achieving high compression ratios and rates. ZipNN supports various compression methods like ZSTD, lz4, and snappy, and provides ready-made scripts for file compression/decompression. Users can also manually import the package to compress and decompress data. The library offers advanced configuration options for customization and validation tests for different input and compression types.
For similar jobs
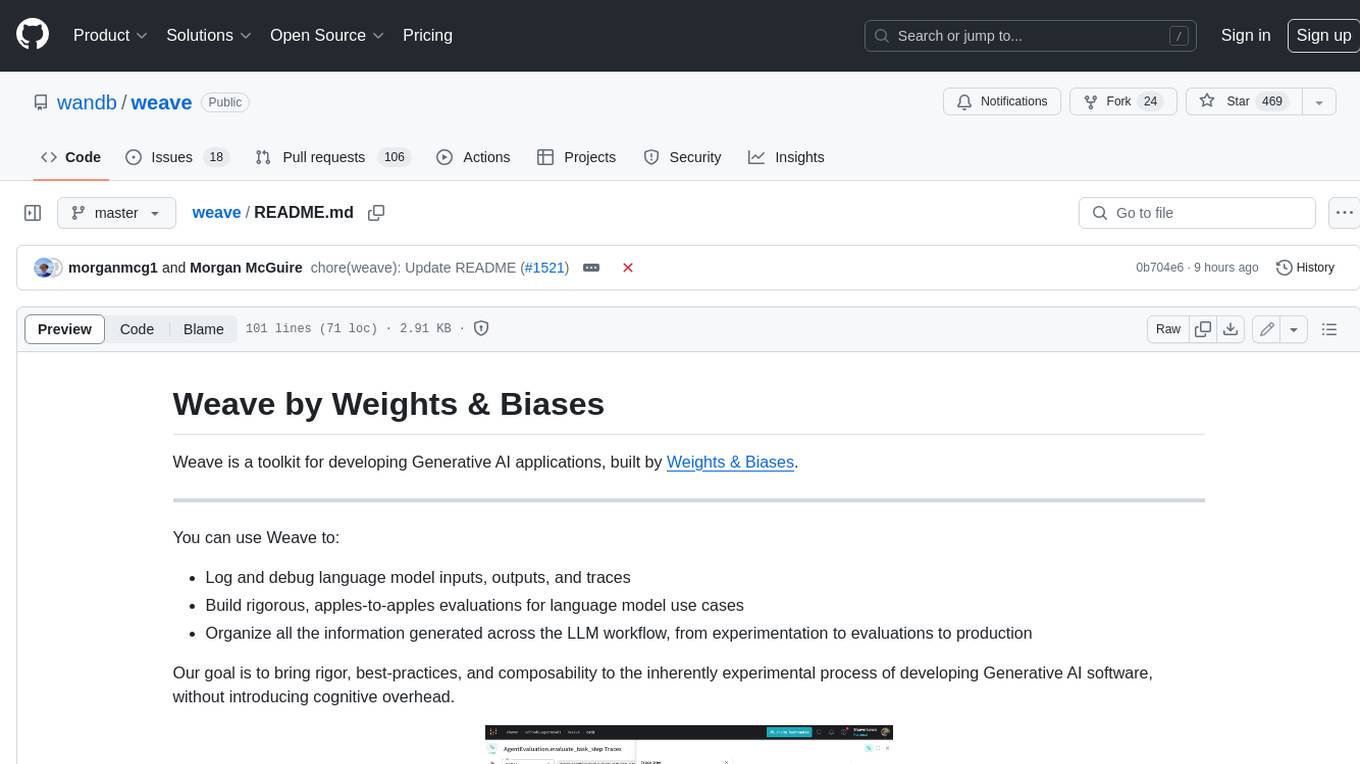
weave
Weave is a toolkit for developing Generative AI applications, built by Weights & Biases. With Weave, you can log and debug language model inputs, outputs, and traces; build rigorous, apples-to-apples evaluations for language model use cases; and organize all the information generated across the LLM workflow, from experimentation to evaluations to production. Weave aims to bring rigor, best-practices, and composability to the inherently experimental process of developing Generative AI software, without introducing cognitive overhead.
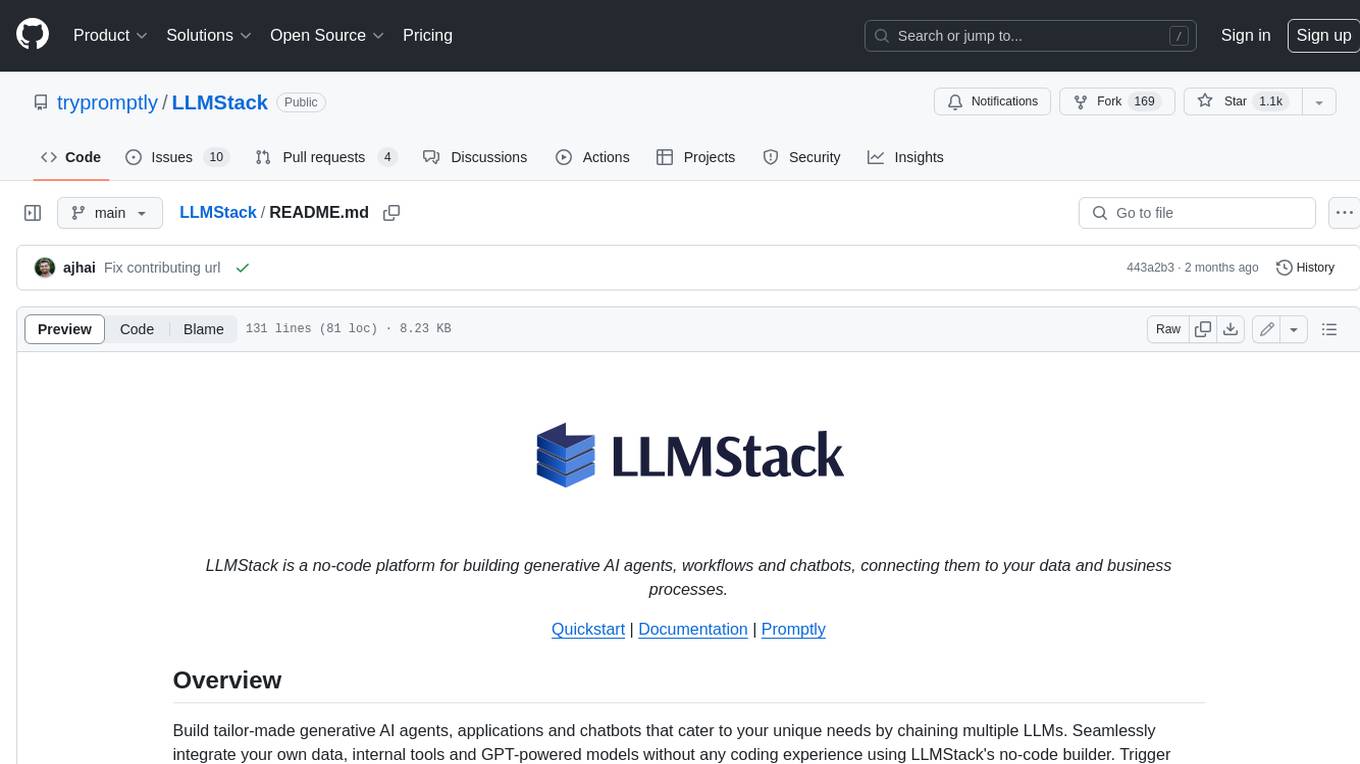
LLMStack
LLMStack is a no-code platform for building generative AI agents, workflows, and chatbots. It allows users to connect their own data, internal tools, and GPT-powered models without any coding experience. LLMStack can be deployed to the cloud or on-premise and can be accessed via HTTP API or triggered from Slack or Discord.
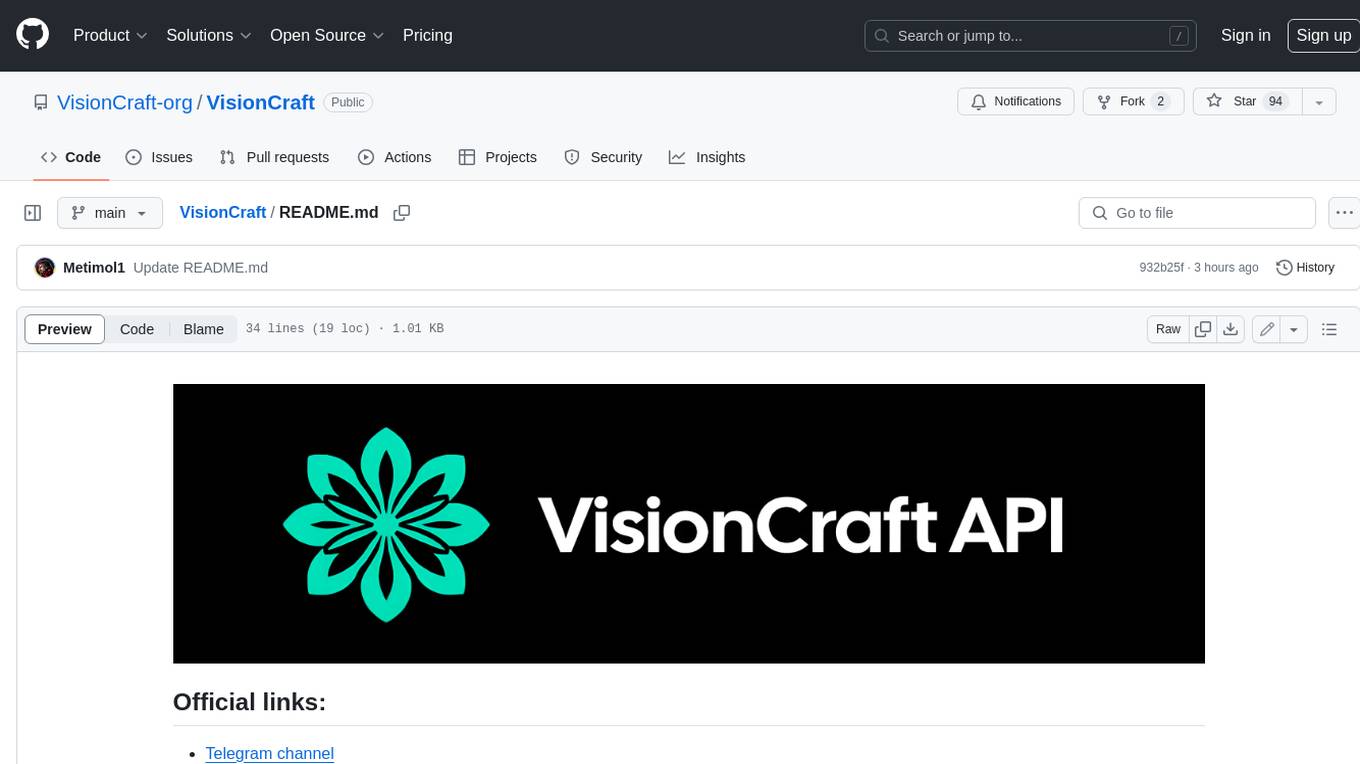
VisionCraft
The VisionCraft API is a free API for using over 100 different AI models. From images to sound.
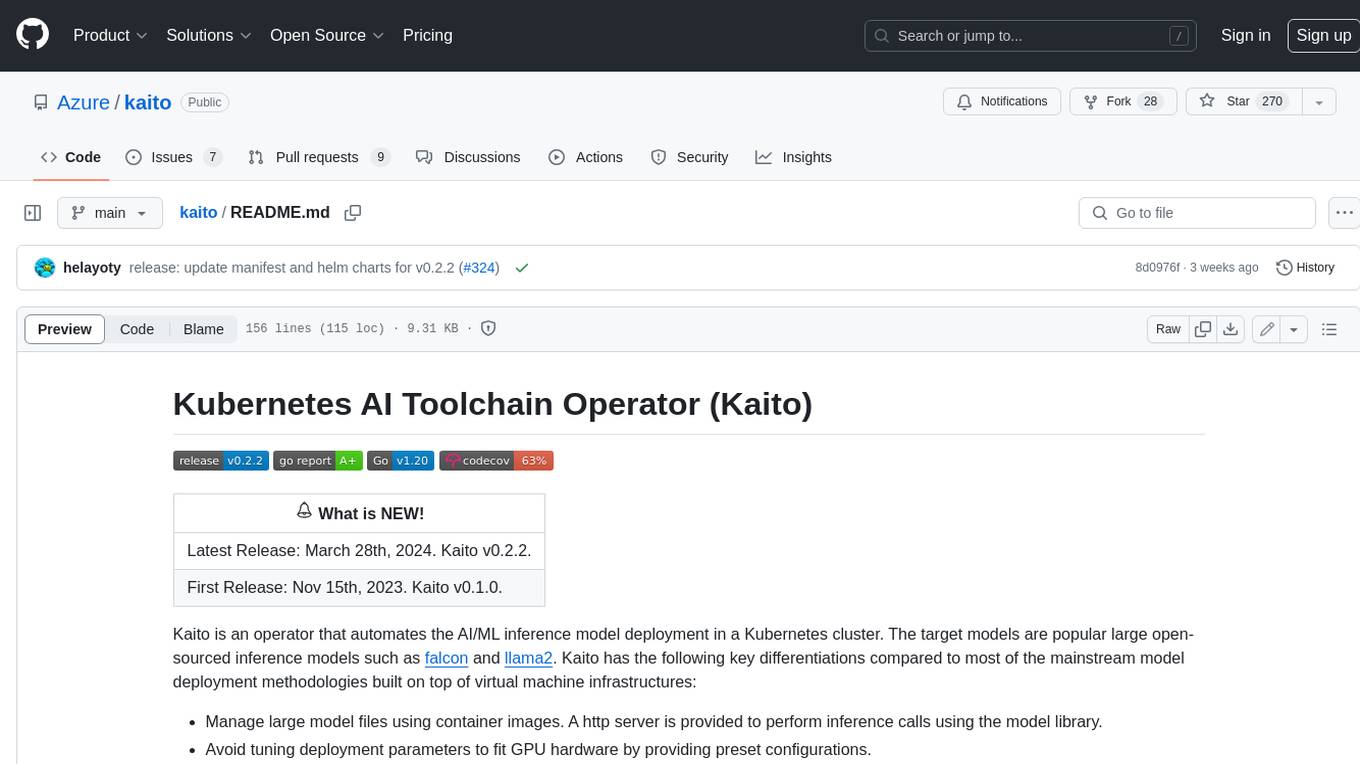
kaito
Kaito is an operator that automates the AI/ML inference model deployment in a Kubernetes cluster. It manages large model files using container images, avoids tuning deployment parameters to fit GPU hardware by providing preset configurations, auto-provisions GPU nodes based on model requirements, and hosts large model images in the public Microsoft Container Registry (MCR) if the license allows. Using Kaito, the workflow of onboarding large AI inference models in Kubernetes is largely simplified.
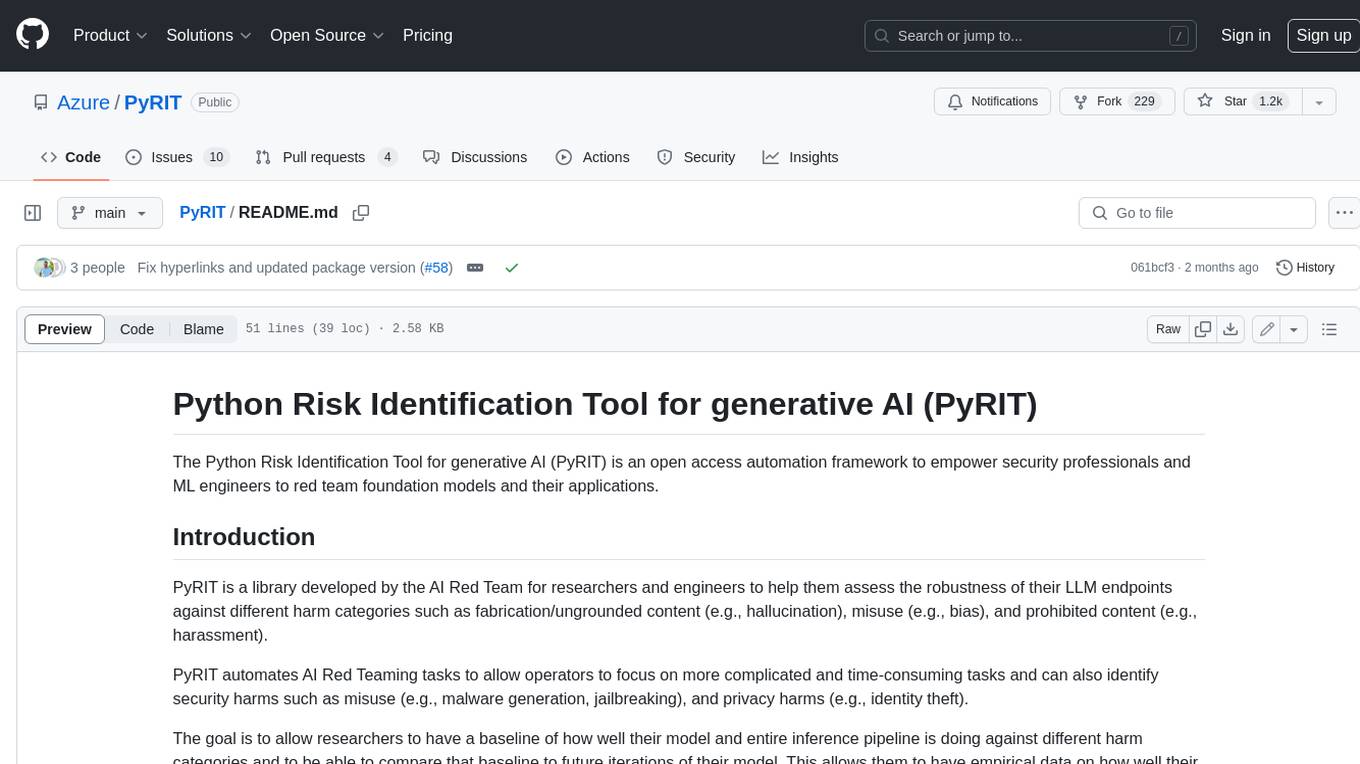
PyRIT
PyRIT is an open access automation framework designed to empower security professionals and ML engineers to red team foundation models and their applications. It automates AI Red Teaming tasks to allow operators to focus on more complicated and time-consuming tasks and can also identify security harms such as misuse (e.g., malware generation, jailbreaking), and privacy harms (e.g., identity theft). The goal is to allow researchers to have a baseline of how well their model and entire inference pipeline is doing against different harm categories and to be able to compare that baseline to future iterations of their model. This allows them to have empirical data on how well their model is doing today, and detect any degradation of performance based on future improvements.
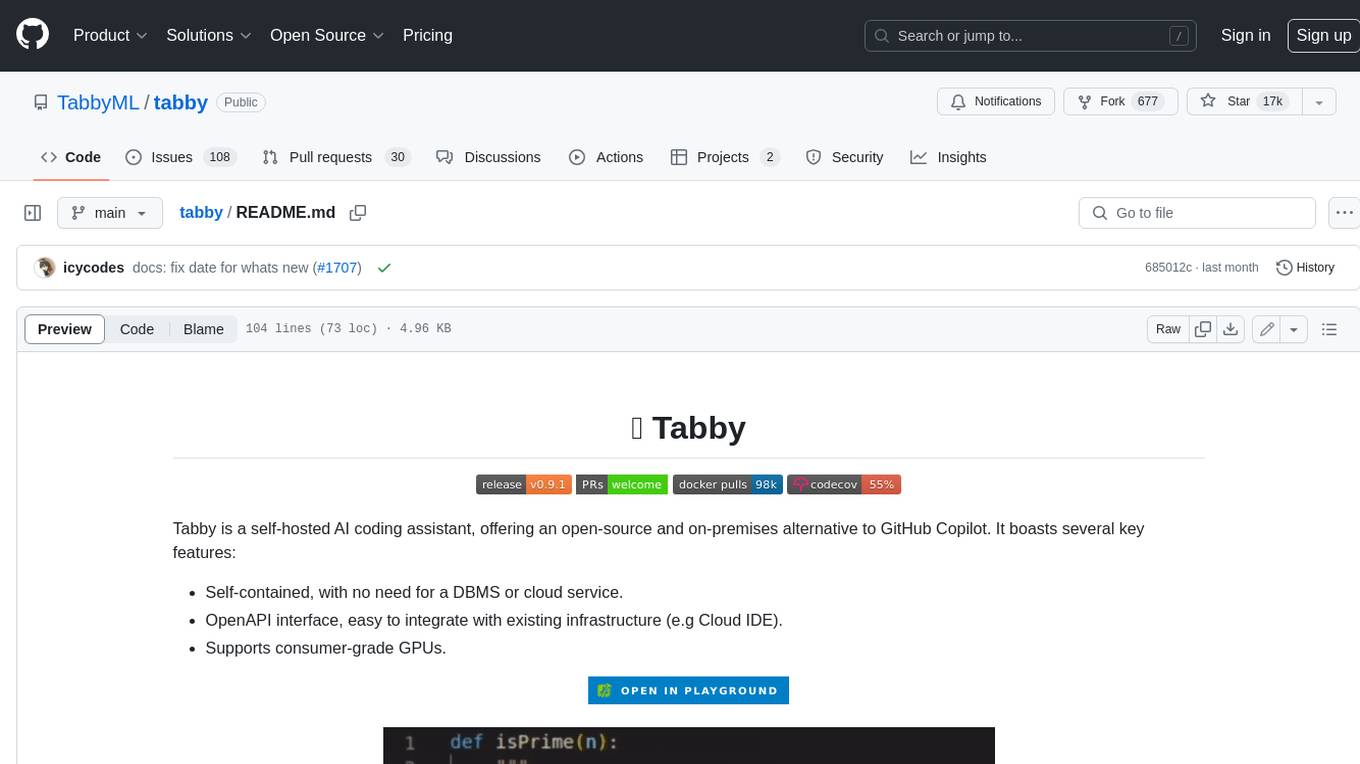
tabby
Tabby is a self-hosted AI coding assistant, offering an open-source and on-premises alternative to GitHub Copilot. It boasts several key features: * Self-contained, with no need for a DBMS or cloud service. * OpenAPI interface, easy to integrate with existing infrastructure (e.g Cloud IDE). * Supports consumer-grade GPUs.
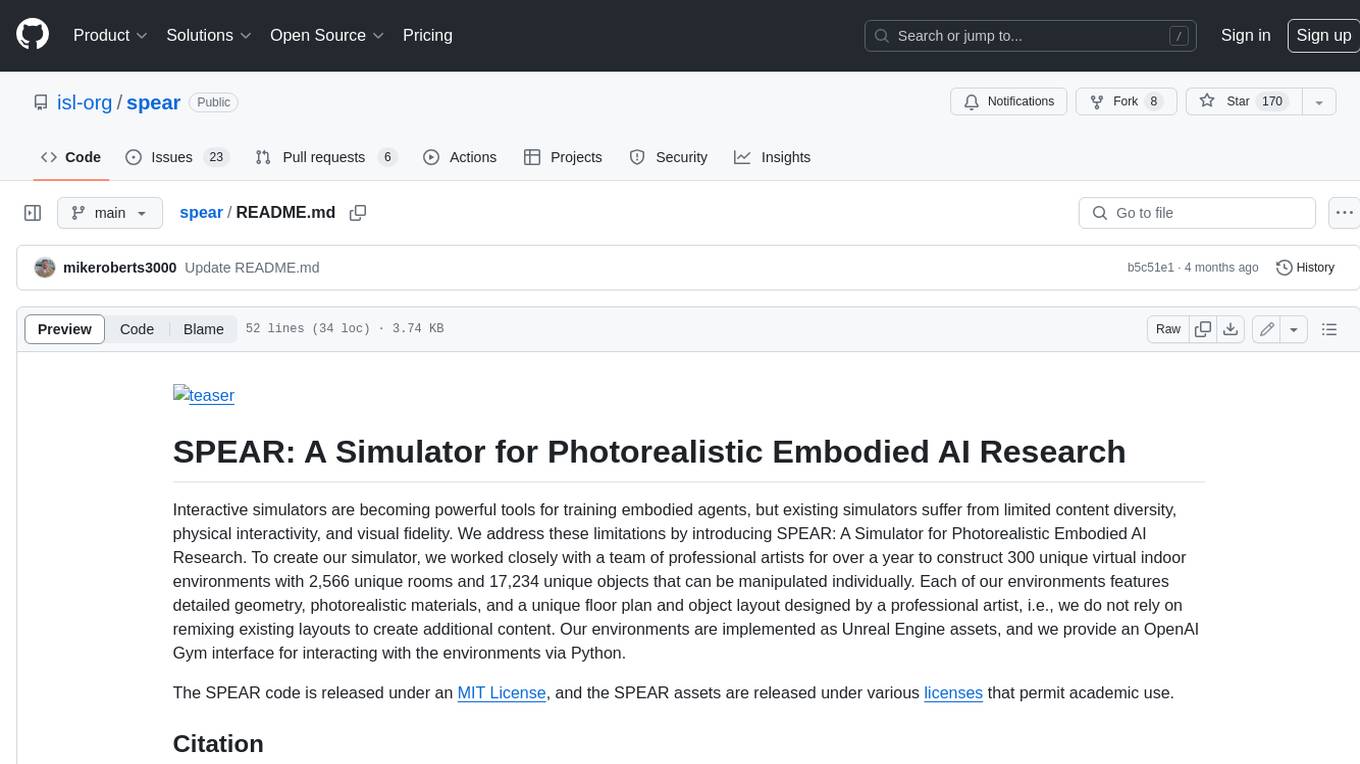
spear
SPEAR (Simulator for Photorealistic Embodied AI Research) is a powerful tool for training embodied agents. It features 300 unique virtual indoor environments with 2,566 unique rooms and 17,234 unique objects that can be manipulated individually. Each environment is designed by a professional artist and features detailed geometry, photorealistic materials, and a unique floor plan and object layout. SPEAR is implemented as Unreal Engine assets and provides an OpenAI Gym interface for interacting with the environments via Python.
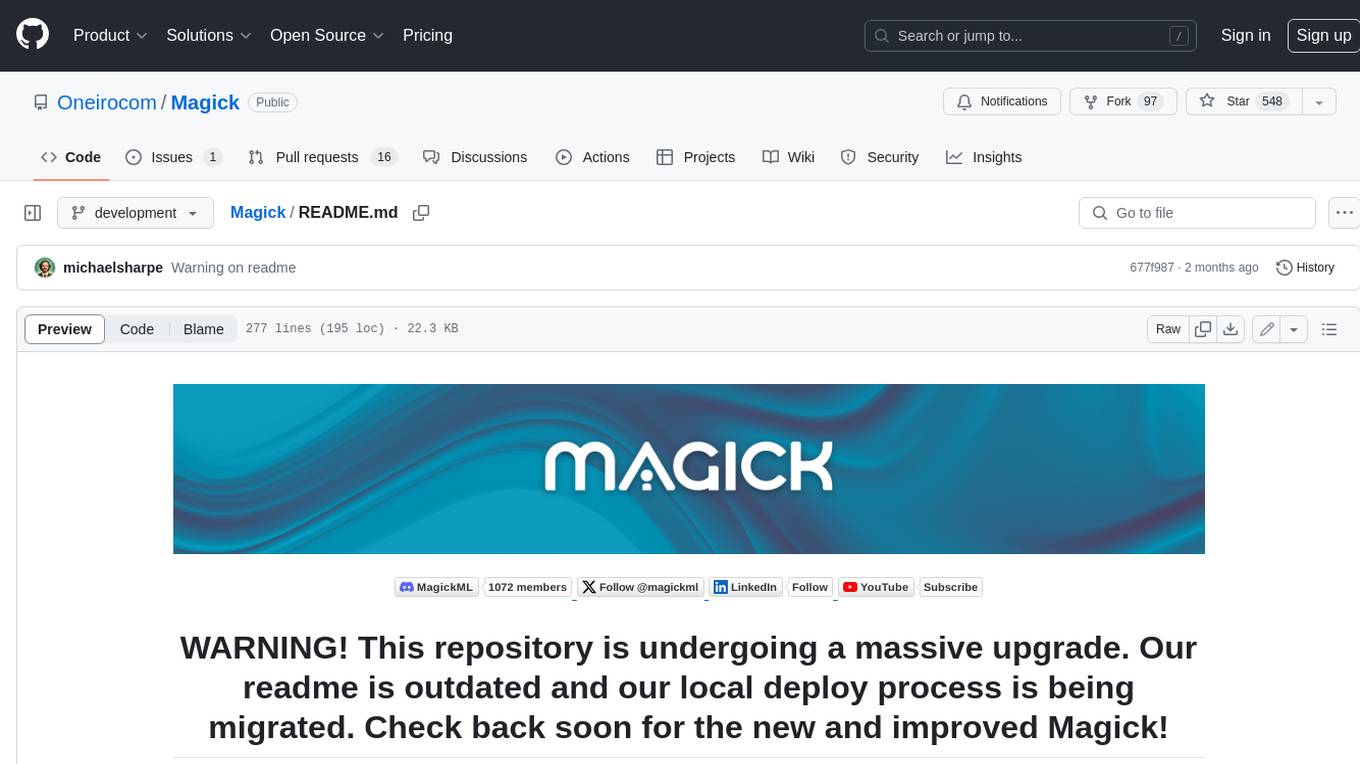
Magick
Magick is a groundbreaking visual AIDE (Artificial Intelligence Development Environment) for no-code data pipelines and multimodal agents. Magick can connect to other services and comes with nodes and templates well-suited for intelligent agents, chatbots, complex reasoning systems and realistic characters.