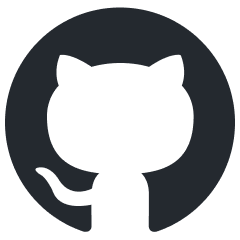
TokenFormer
[ICLR2025] Official Implementation of TokenFormer: Rethinking Transformer Scaling with Tokenized Model Parameters
Stars: 481
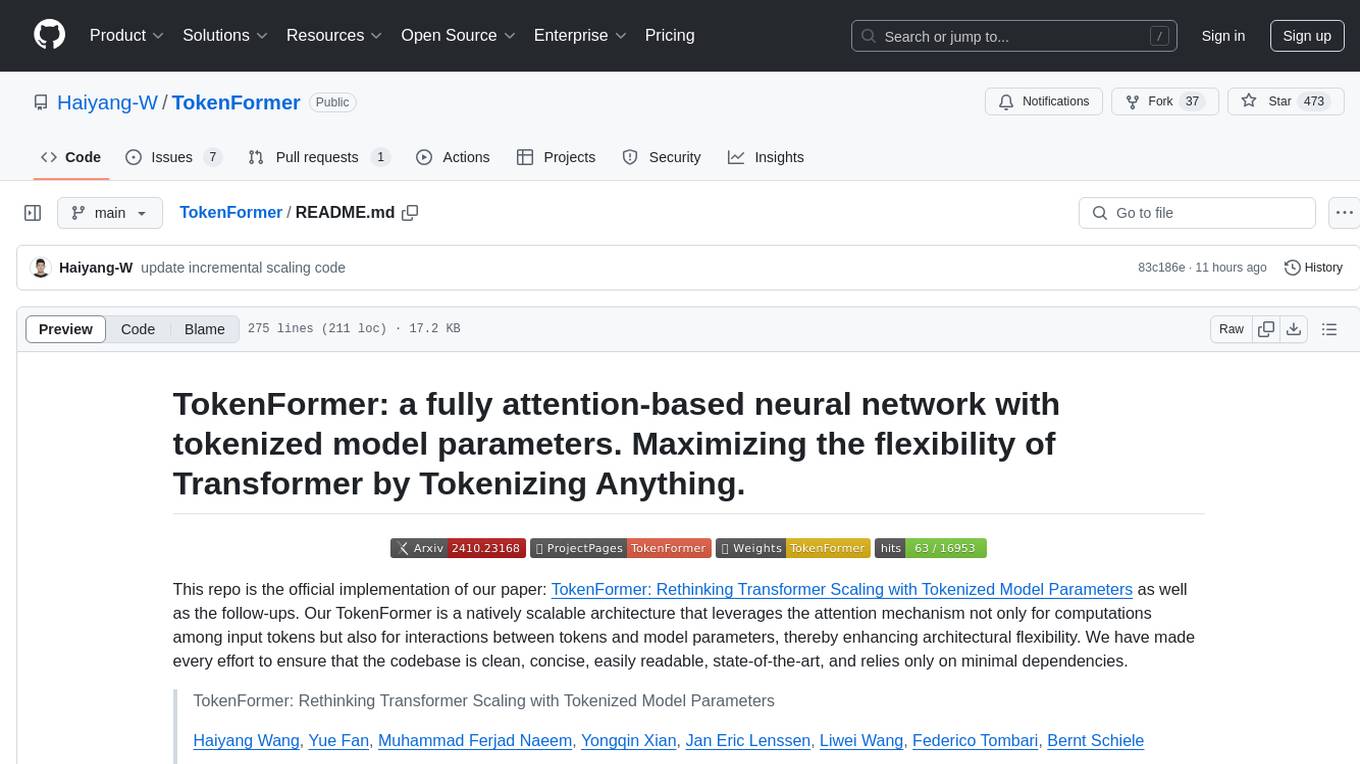
TokenFormer is a fully attention-based neural network architecture that leverages tokenized model parameters to enhance architectural flexibility. It aims to maximize the flexibility of neural networks by unifying token-token and token-parameter interactions through the attention mechanism. The architecture allows for incremental model scaling and has shown promising results in language modeling and visual modeling tasks. The codebase is clean, concise, easily readable, state-of-the-art, and relies on minimal dependencies.
README:
TokenFormer: a fully attention-based neural network with tokenized model parameters. Maximizing the flexibility of Transformer by Tokenizing Anything.
This repo is the official implementation of our paper: TokenFormer: Rethinking Transformer Scaling with Tokenized Model Parameters as well as the follow-ups. Our TokenFormer is a natively scalable architecture that leverages the attention mechanism not only for computations among input tokens but also for interactions between tokens and model parameters, thereby enhancing architectural flexibility. We have made every effort to ensure that the codebase is clean, concise, easily readable, state-of-the-art, and relies only on minimal dependencies.
TokenFormer: Rethinking Transformer Scaling with Tokenized Model Parameters
Haiyang Wang*, Yue Fan*, Muhammad Ferjad Naeem, Yongqin Xian, Jan Eric Lenssen, Liwei Wang, Federico Tombari, Bernt Schiele
- Primary contact: Haiyang Wang
([email protected])([email protected]), Bernt Schiele ([email protected])
- [25-01-22] π₯ TokenFormer is accepted by ICLR2025.
- [25-01-12] Jax code on TPU (GCP-Cloud) is released, please see here.
- [24-11-08] π Training code with pytorch is released.
- [24-11-02] Please feel free to email me if I've missed any relevant papers. I will do my best to include all related papers in future versions.
- [24-10-31] π Inference code with pytorch is released.
- [24-10-31] π TokenFormer is released on arXiv.
- We aim to offer a new perspective to models, applicable to any computation graph in the future. In theory, by using data tokens, parameter tokens, and memory tokens, and through dot-product interactions, it's possible to flexibly construct any network. There are many design possibilities here. For example, introducing memory tokens can build RNN-like networks similar to Mamba. Merging parameter tokens with memory tokens creates something akin to a TTT network. Parameter tokens can also attend to input data in reverse, making the network parameters dynamically data-dependent, updating layer by layer.
- π« What we want to do
- π Main Results
- π Model Zoo
- π οΈ Quick Start
- π Todo
- π Citation
We introduce Tokenformer, a fully attention-based architecture that unifies the computations of token-token and token-parameter interactions by entirely employing the attention mechanism, maximizes the flexibility of neural network. The advantage makes it can handle a variable number of parameters, inherently enhances the model's scalability, facilitating progressively efficient scaling.
We not only tokenizes data but also model parameters, replacing the model concept with interaction flows between data and parameter tokens, further advancing the network architecture towards unification.
Hope that this architecture can offer greater flexibility than traditional Transformers, will further contribute to the development of foundation models, sparse inference (MoE), parameter efficient tuning, device-cloud collaboration, vision-language, model interpretability, and so on.
# Pattention Implementations with given inputs
query, key, value = inputs, key_param_tokens, value_param_tokens
attn_weight = query @ key.transpose(-2, -1) * scale_factor
attn_weight *= attn_masks
# modified softmax, softmax is equal to exp + L1 norm
attn_weight = nonlinear_norm_func(attn_weight, self.norm_activation_type, dim=-1)
output = attn_weight @ value
Traditionally, large transformer architectures are trained from scratch without reusing previous smaller-scale models. In this paper, we propose a novel fully attention-based architecture that allows scaling model incrementally, thus greatly reducing the overall cost of training large transformer architectures.
(Zero-shot Evaluations.) The best performance for each model size is highlighted in bold. Our comparisons are made with publicly available transformer-based LMs with various tokenizers. Following Pythia, our model is trained for up to 300B tokens on pile dataset.
(Image Classification.) Comparison of standard vision transformer on ImageNet-1K.
Pretrained models are uploaded to huggingface TokenFormer-150M
, TokenFormer-450M
, TokenFormer-900M
and TokenFormer-1-5B
, trained on 300B tokens on the Pile.
These models were trained on the Pile, and follow the standard model dimensions of Transformer, and evaluated on standard zero-shot benchmark described by mamba:
Model | Params | Layers | Model dim. | ckpt | config | log |
---|---|---|---|---|---|---|
TokenFormer-150M | 150M | 12 | 768 | ckpt | config | log |
TokenFormer-450M | 450M | 24 | 1024 | ckpt | config | log |
TokenFormer-900M | 900M | 32 | 1280 | ckpt | config | log |
TokenFormer-1-5B | 1-5B | 40 | 1536 | ckpt | config | log |
Note: these are base models trained only for 300B tokens, without any form of downstream modification (instruction tuning, etc.). Performance is expected to be comparable or better than other architectures trained on similar data, but not to match larger or fine-tuned models.
Will be released later.
First make sure you are in an environment with Python 3.8 and CUDA 12 with an appropriate version of PyTorch 1.8 or later installed. Note: our TokenFormer is based on the GPT-NeoX, some of the libraries that GPT-NeoX depends on have not been updated to be compatible with Python 3.10+. Python 3.9 appears to work, but this codebase has been developed and tested for Python 3.8.
To install the remaining basic dependencies, run:
conda create -n TokenFormer python=3.8
git clone https://github.com/Haiyang-W/TokenFormer.git
pip install torch==2.2.1 torchvision==0.17.1 torchaudio==2.2.1 --index-url https://download.pytorch.org/whl/cu121
### raven module load gcc/10
### If you face cargo problem when run pip install -r requirements/requirements.txt, please follow the bellow command
# curl https://sh.rustup.rs -sSf | sh
# export PATH="$HOME/.cargo/bin:$PATH"
# source ~/.profile
# source ~/.cargo/env
### if you face mpi4py problem when run pip install -r requirements/requirements.txt, please:
# conda install -c conda-forge mpi4py=3.0.3
pip install -r requirements/requirements.txt
pip install -r requirements/requirements-flashattention.txt # need gcc > 9
pip install -r requirements/requirements-wandb.txt # optional, if logging using WandB
pip install -r requirements/requirements-tensorboard.txt # optional, if logging via tensorboard
pip install -r requirements/requirements-comet.txt # optional, if logging via Comet
# install apex
pip install -r requirements/requirements-apex-pip.txt # pip > 23.1
git clone https://github.com/NVIDIA/apex
cd apex
pip install -v --disable-pip-version-check --no-cache-dir --no-build-isolation --config-settings "--build-option=--cpp_ext" --config-settings "--build-option=--cuda_ext" ./
from the repository root.
To run zero-shot evaluations of models (corresponding to Table 1 of the paper), we use the lm-evaluation-harness library.
First you should download the pre-trained weights from huggingface to your local directory. For example, the relative path is ./TokenFormer-150M/pytorch_model.bin
from the repository root.
# single-gpu evaluation (currently only tested on single-gpu.)
cd ./TokenFormer
python ./deepy.py eval.py -d configs tokenformer/150M_eval.yml --eval_tasks lambada_openai hellaswag piqa arc_challenge arc_easy winogrande
Several preconfigured datasets are available, including most components from openwebtext and Pile.
e.g. to download and tokenize the openwebtext2 dataset with GPT-NeoX 20B Tokenizer. You can try this small dataset first.
python prepare_data.py -d ./data -t HFTokenizer --vocab-file tokenizer.json openwebtext2
The preprocessed data will be located in ./data/openwebtext2
.
For the Pile 300B (unofficial and uncopyied version):
python prepare_data.py -d ./data -t HFTokenizer --vocab-file tokenizer.json pile
The preprocessed data will be located in ./data/pile
.
The tokenized data will be saved out to two files: [data-dir]/[dataset-name]/[dataset-name]_text_document.bin
and [data-dir]/[dataset-name]/[dataset-name]_text_document.idx
. You will need to add the prefix that both these files share to your training configuration file under the data-path field. E.G:
"data-path": "./data/pile/pile_0.87_deduped_text_document",
If you just want to get it running easily, you can try enwik8
.
Note that this is for single node. Applicable if you can already SSH into an 8-GPU machine and run programs directly.
CUDA_VISIBLE_DEVICES=0,1,2,3,4,5,6,7 python deepy.py train.py configs/tokenformer/150M_train_pile.yml
Please refer multi-node-launching. I use slurm and give some guidance as follows.
First, modify your training config
{
"launcher": "slurm",
"deepspeed_slurm": true,
}
Then I provide my slurm script with 16 GPUs as an example.
#!/bin/bash
#SBATCH --job-name="150M_16gpus"
#SBATCH --constraint="gpu"
#SBATCH --nodes=2
#SBATCH --ntasks-per-node=8
#SBATCH --gres=gpu:8
#SBATCH --cpus-per-task=4 # using 4 cores each.
#SBATCH --time=24:00:00
#SBATCH -o /tmp/150M_%A_%a.out
conda activate TokenFormer
# Some potentially useful distributed environment variables
export HOSTNAMES=`scontrol show hostnames "$SLURM_JOB_NODELIST"`
export MASTER_ADDR=$(scontrol show hostnames "$SLURM_JOB_NODELIST" | head -n 1)
export MASTER_PORT=12856
export COUNT_NODE=`scontrol show hostnames "$SLURM_JOB_NODELIST" | wc -l`
# Your hostfile creation script from above
bash ./write_hostfile.sh
# Tell DeepSpeed where to find our generated hostfile via DLTS_HOSTFILE, you can customize any path.
export DLTS_HOSTFILE=/tmp/hosts_$SLURM_JOBID
python3 deepy.py train.py ./configs/tokenformer/150M_train_pile.yml
All paths here can be customized; you can replace /tmp
in the above script and write_hostfile.sh
with any path you want. Then run the scripts
sbatch scripts.sh
Go to your checkpoint directory, E.G., 150M
cd ./work_dirs/150M_TokenFormer_Pile/checkpoints
python zero_to_fp32.py . pytorch_model.bin
Then use that path to replace the eval_ckpt in 150M_eval.yml
.
cd ./TokenFormer
python ./deepy.py eval.py -d configs tokenformer/150M_eval.yml --eval_tasks lambada_openai hellaswag piqa arc_challenge arc_easy winogrande
NOTE:
Iβve only run the training code for the first 1000 iterations to check the loss, and it looks fine, so Iβm releasing it for everyone to use for now. I canβt guarantee there are no issues. If youβd prefer to wait, I can do a final check, but it might take some time.
Please download the pretrained 354M TokenFormer on openwebtext2 dataset from huggingface:354M_TokenFormer_Openwebtext2.zip and unzip it to your local directory. For example, the relative path is ./354M_TokenFormer_Openwebtext2/
from the repository root. Then make the language dataset and pretrained checkpoints well with your local path. The pretrain checkpoints here are the results of training TokenFormer 354M on OpenWebText2 for 600k steps. And the corresponing config is here
Please follow here to prepare the openwebtext2 dataset.
cd ./TokenFormer
python deepy.py train.py configs/incremental_scaling_openwebtext2/354M_to_757M_train_openwebtext2_60k.yml
The model's performance is as follows:
Model | strategy | Layers | Model dim. | iterations | val ppl | config |
---|---|---|---|---|---|---|
TokenFormer-354M | scratch | 24 | 1024 | 600k | 11.9 | config |
TransFormer-757M | scratch | 24 | 1536 | 60k | 12.0 | - |
TransFormer-757M | scratch | 24 | 1536 | 120k | 11.3 | - |
TransFormer-757M | scratch | 24 | 1536 | 600k | 10.5 | - |
TokenFormer-757M | incremental | 24 | 1024 | 60k | 10.9 | config |
TokenFormer-757M | incremental | 24 | 1024 | 120k | 10.7 | config |
- [x] Release the arXiv version.
- [x] Release inference code and model weights of LLM.
- [x] Release training code of LLM.
- [x] Release incremental scaling training code of LLM.
- [ ] Release training code of Image Classification.
- [ ] Release model weights of CLIP trained on DataComp-1B.
- [ ] Release some initial results of Vision Language Modeling on LLaVA benchmark.
Please consider citing our work as follows if it is helpful.
@article{wang2024tokenformer,
title={TokenFormer: Rethinking Transformer Scaling with Tokenized Model Parameters},
author={Wang, Haiyang and Fan, Yue and Naeem, Muhammad Ferjad and Xian, Yongqin and Lenssen, Jan Eric and Wang, Liwei and Tombari, Federico and Schiele, Bernt},
journal={arXiv preprint arXiv:2410.23168},
year={2024}
}
For Tasks:
Click tags to check more tools for each tasksFor Jobs:
Alternative AI tools for TokenFormer
Similar Open Source Tools
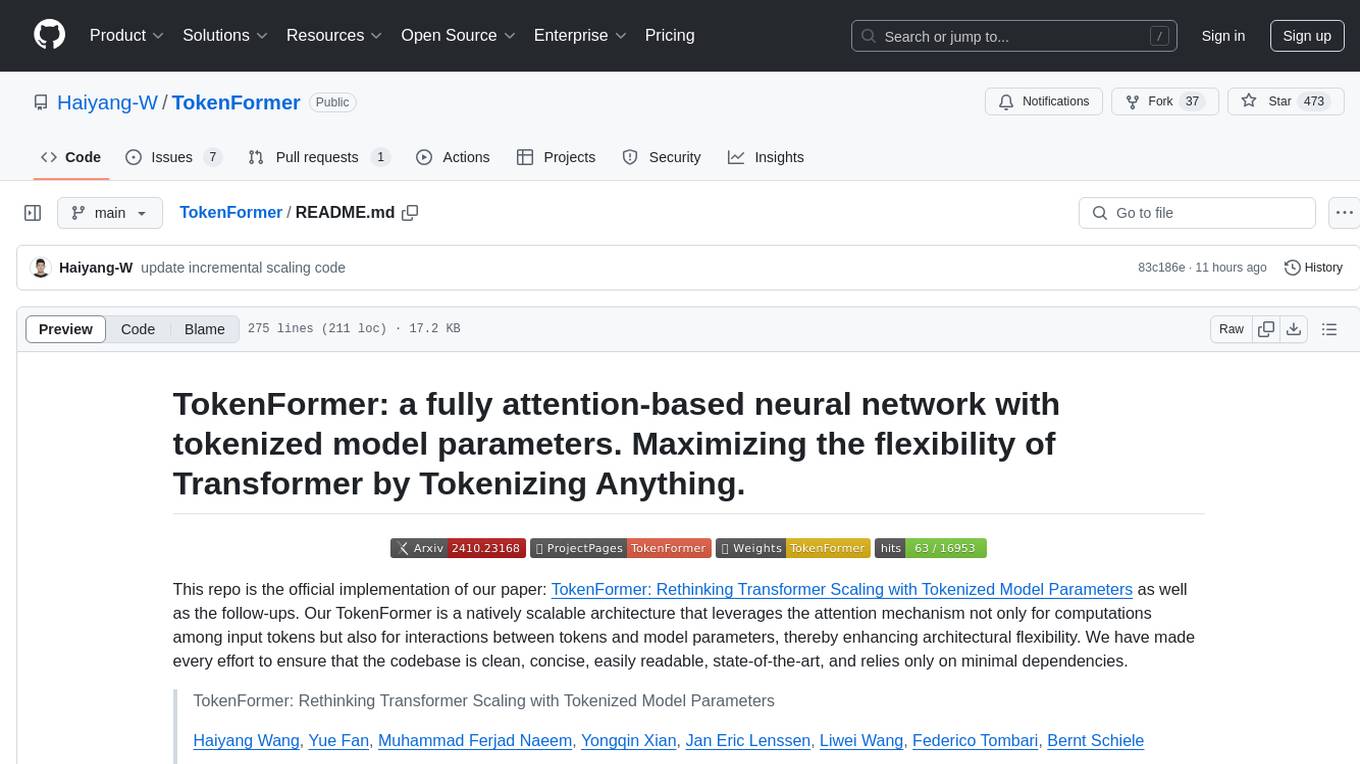
TokenFormer
TokenFormer is a fully attention-based neural network architecture that leverages tokenized model parameters to enhance architectural flexibility. It aims to maximize the flexibility of neural networks by unifying token-token and token-parameter interactions through the attention mechanism. The architecture allows for incremental model scaling and has shown promising results in language modeling and visual modeling tasks. The codebase is clean, concise, easily readable, state-of-the-art, and relies on minimal dependencies.
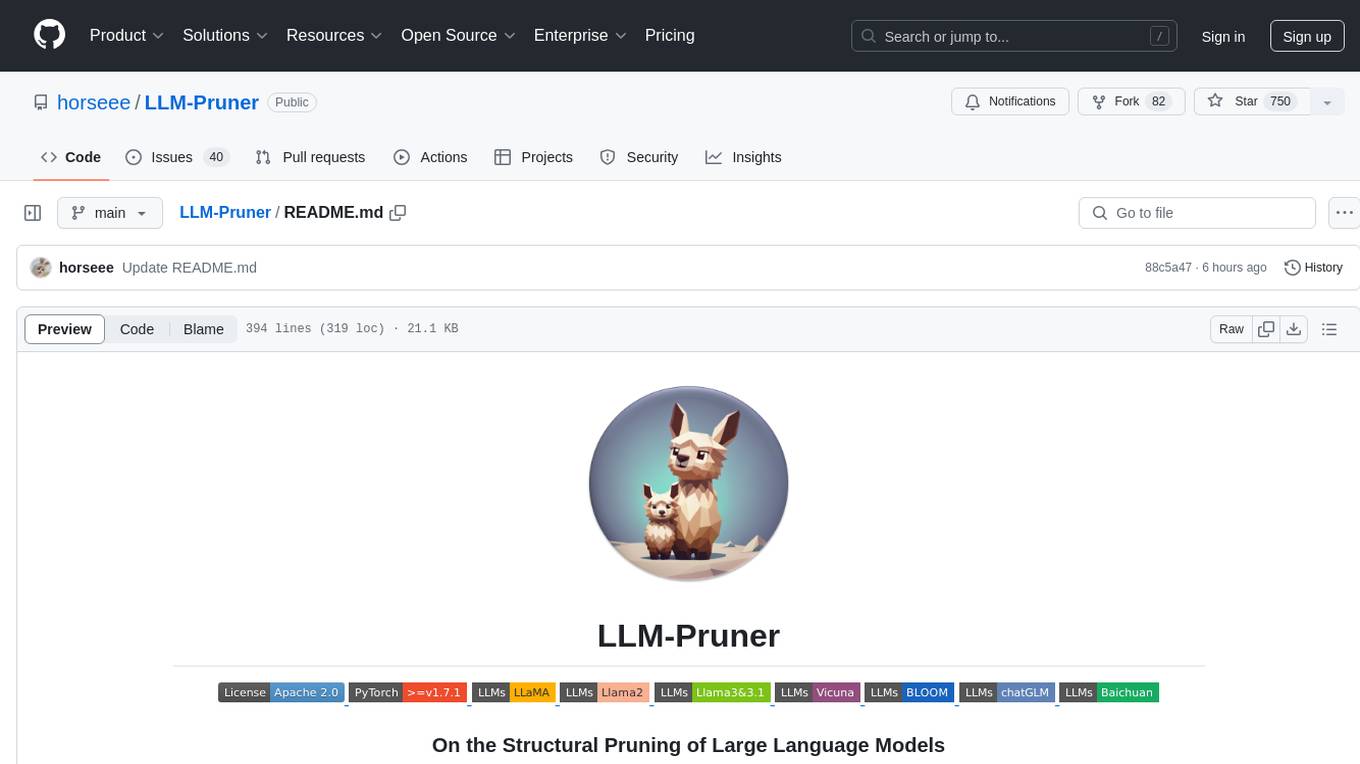
LLM-Pruner
LLM-Pruner is a tool for structural pruning of large language models, allowing task-agnostic compression while retaining multi-task solving ability. It supports automatic structural pruning of various LLMs with minimal human effort. The tool is efficient, requiring only 3 minutes for pruning and 3 hours for post-training. Supported LLMs include Llama-3.1, Llama-3, Llama-2, LLaMA, BLOOM, Vicuna, and Baichuan. Updates include support for new LLMs like GQA and BLOOM, as well as fine-tuning results achieving high accuracy. The tool provides step-by-step instructions for pruning, post-training, and evaluation, along with a Gradio interface for text generation. Limitations include issues with generating repetitive or nonsensical tokens in compressed models and manual operations for certain models.
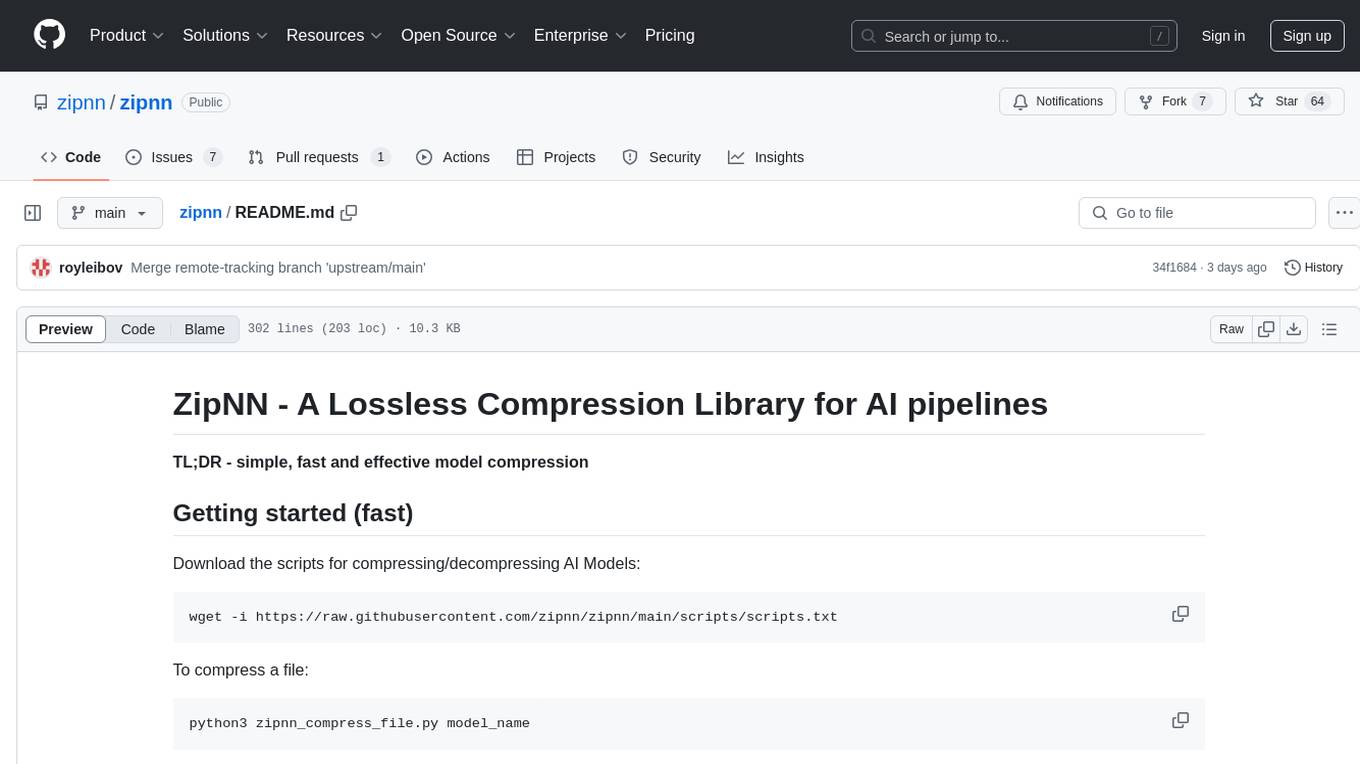
zipnn
ZipNN is a lossless and near-lossless compression library optimized for numbers/tensors in the Foundation Models environment. It automatically prepares data for compression based on its type, allowing users to focus on core tasks without worrying about compression complexities. The library delivers effective compression techniques for different data types and structures, achieving high compression ratios and rates. ZipNN supports various compression methods like ZSTD, lz4, and snappy, and provides ready-made scripts for file compression/decompression. Users can also manually import the package to compress and decompress data. The library offers advanced configuration options for customization and validation tests for different input and compression types.
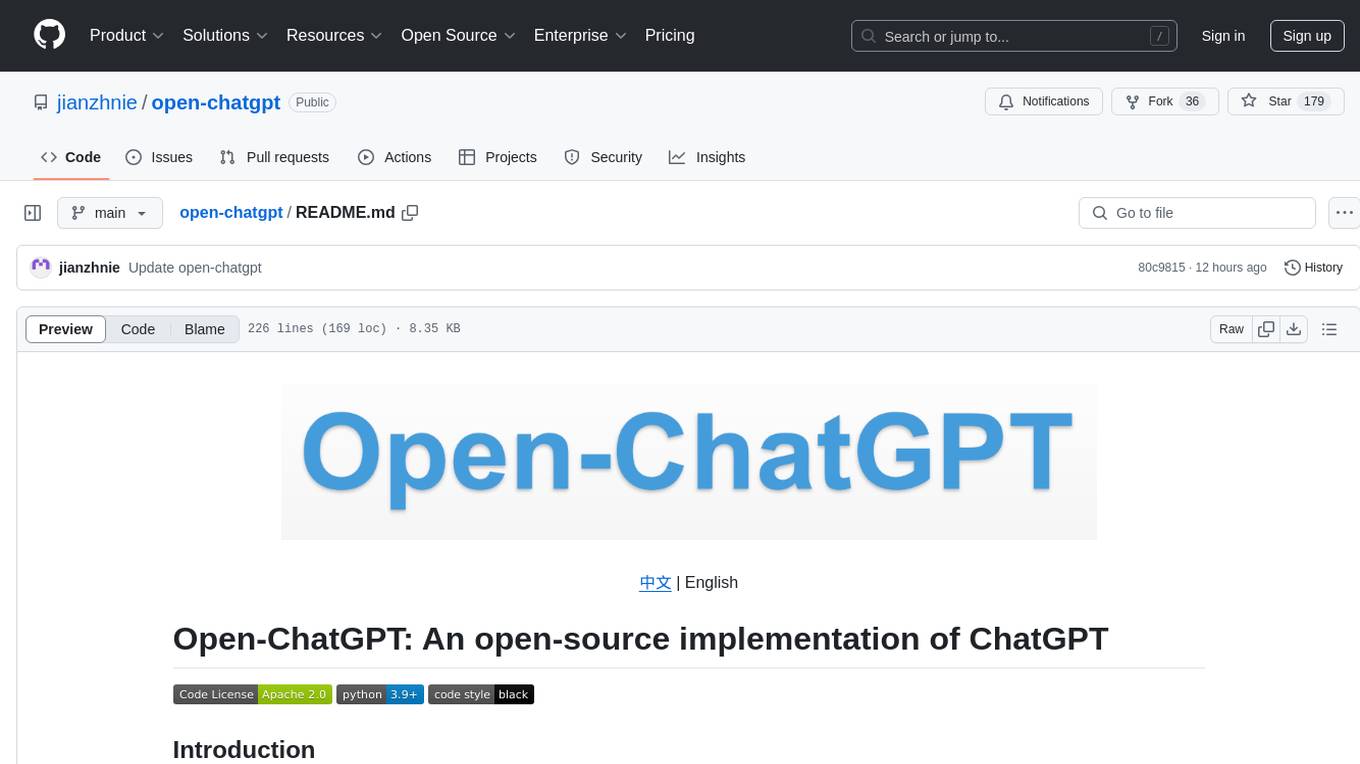
open-chatgpt
Open-ChatGPT is an open-source library that enables users to train a hyper-personalized ChatGPT-like AI model using their own data with minimal computational resources. It provides an end-to-end training framework for ChatGPT-like models, supporting distributed training and offloading for extremely large models. The project implements RLHF (Reinforcement Learning with Human Feedback) powered by transformer library and DeepSpeed, allowing users to create high-quality ChatGPT-style models. Open-ChatGPT is designed to be user-friendly and efficient, aiming to empower users to develop their own conversational AI models easily.
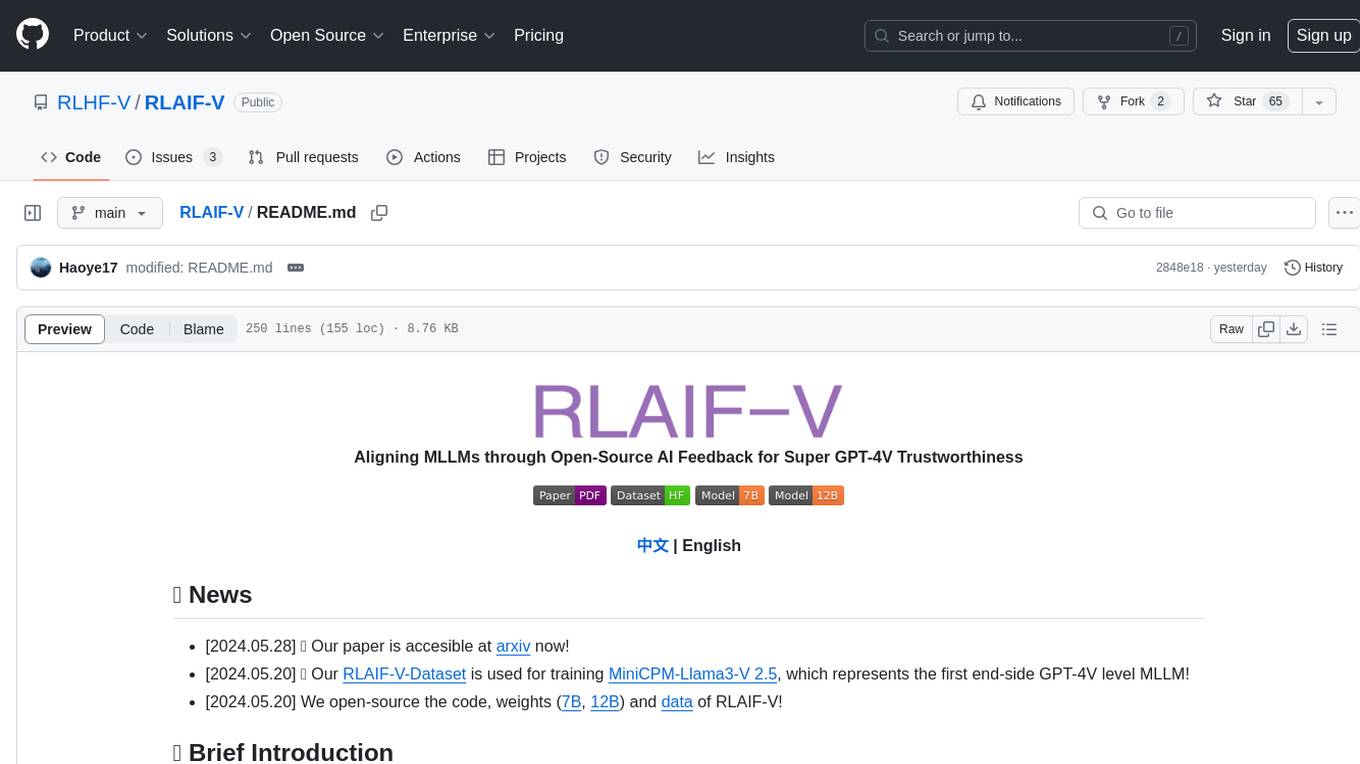
RLAIF-V
RLAIF-V is a novel framework that aligns MLLMs in a fully open-source paradigm for super GPT-4V trustworthiness. It maximally exploits open-source feedback from high-quality feedback data and online feedback learning algorithm. Notable features include achieving super GPT-4V trustworthiness in both generative and discriminative tasks, using high-quality generalizable feedback data to reduce hallucination of different MLLMs, and exhibiting better learning efficiency and higher performance through iterative alignment.
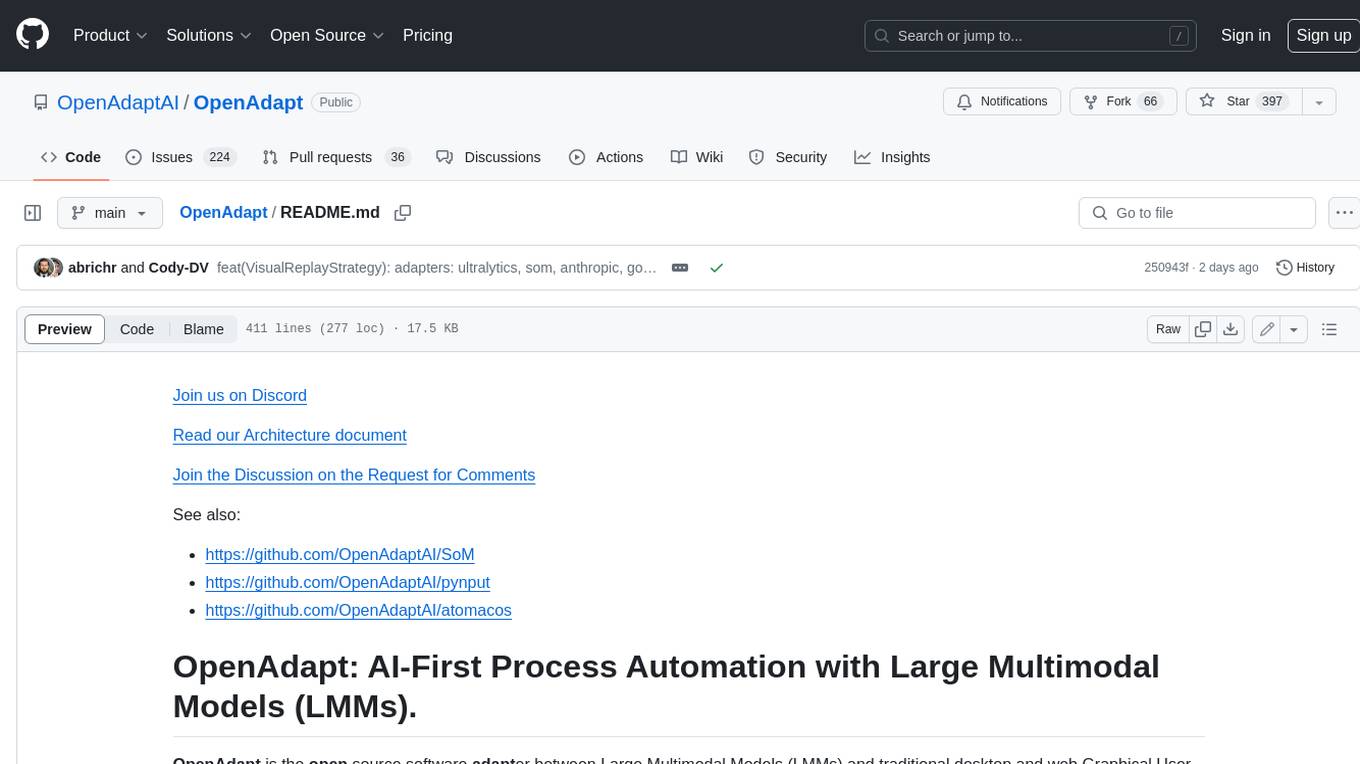
OpenAdapt
OpenAdapt is an open-source software adapter between Large Multimodal Models (LMMs) and traditional desktop and web Graphical User Interfaces (GUIs). It aims to automate repetitive GUI workflows by leveraging the power of LMMs. OpenAdapt records user input and screenshots, converts them into tokenized format, and generates synthetic input via transformer model completions. It also analyzes recordings to generate task trees and replay synthetic input to complete tasks. OpenAdapt is model agnostic and generates prompts automatically by learning from human demonstration, ensuring that agents are grounded in existing processes and mitigating hallucinations. It works with all types of desktop GUIs, including virtualized and web, and is open source under the MIT license.
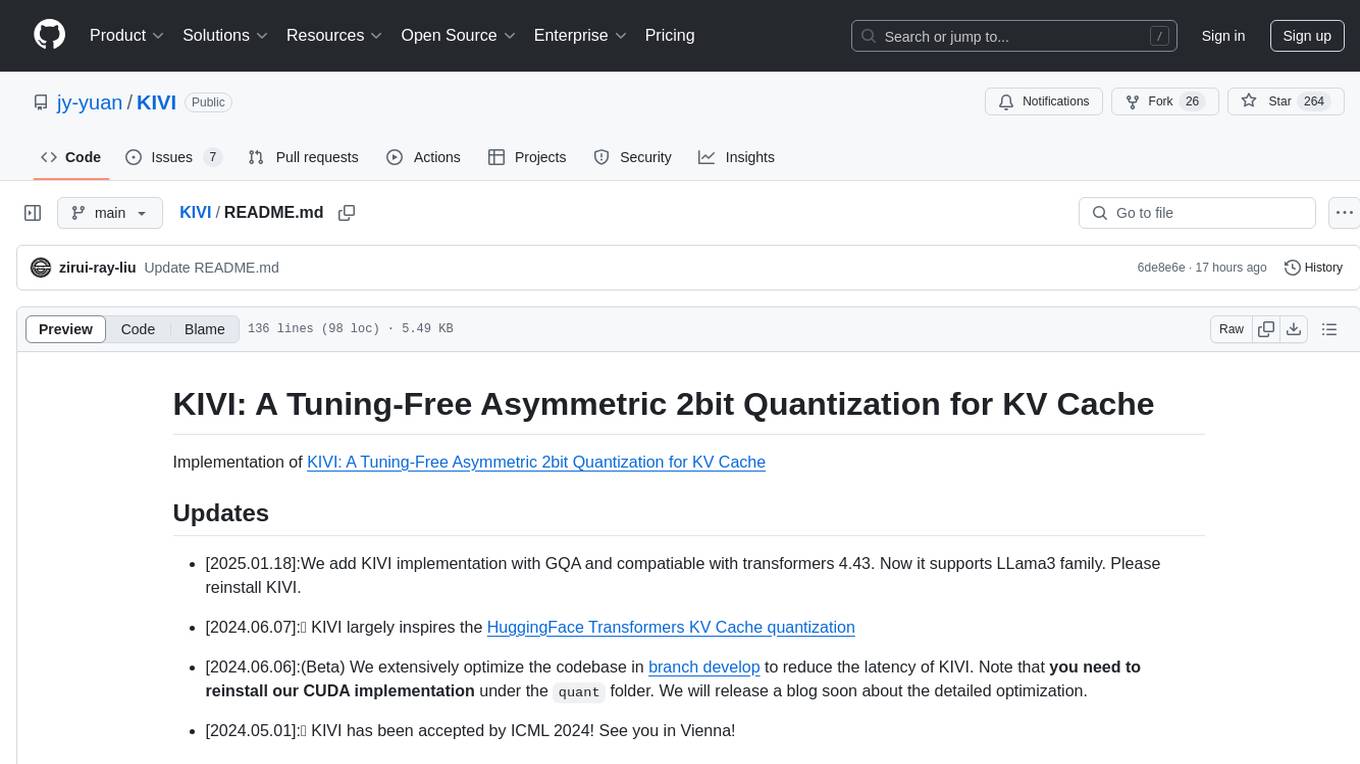
KIVI
KIVI is a plug-and-play 2bit KV cache quantization algorithm optimizing memory usage by quantizing key cache per-channel and value cache per-token to 2bit. It enables LLMs to maintain quality while reducing memory usage, allowing larger batch sizes and increasing throughput in real LLM inference workloads.
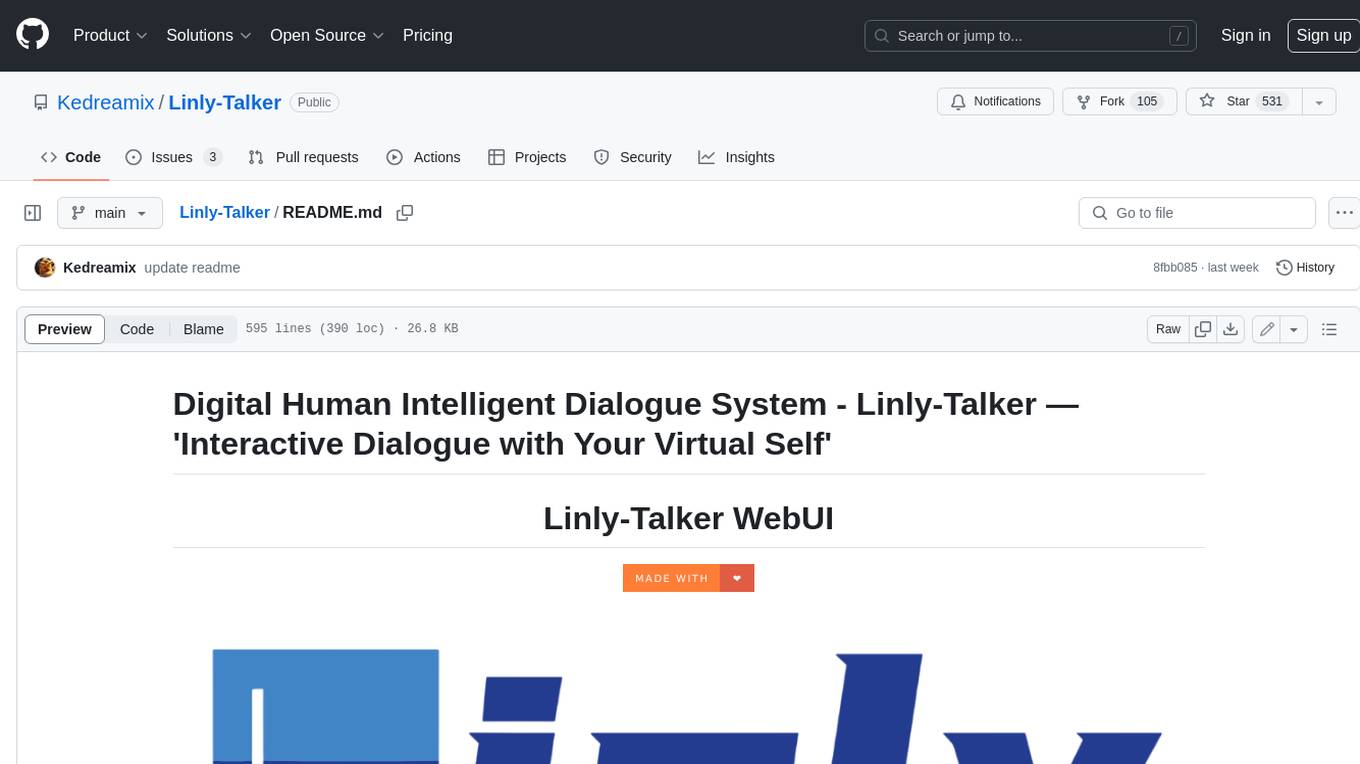
Linly-Talker
Linly-Talker is an innovative digital human conversation system that integrates the latest artificial intelligence technologies, including Large Language Models (LLM) π€, Automatic Speech Recognition (ASR) ποΈ, Text-to-Speech (TTS) π£οΈ, and voice cloning technology π€. This system offers an interactive web interface through the Gradio platform π, allowing users to upload images π· and engage in personalized dialogues with AI π¬.
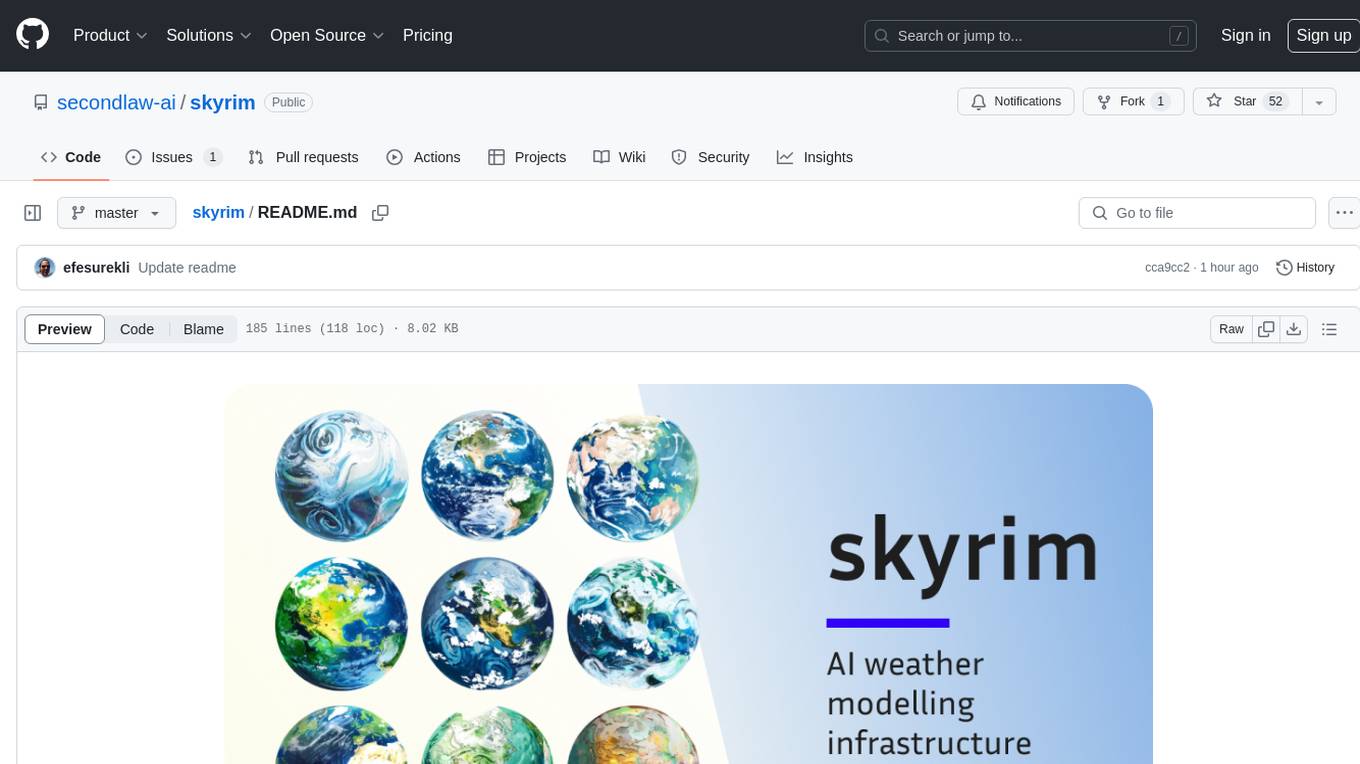
skyrim
Skyrim is a weather forecasting tool that enables users to run large weather models using consumer-grade GPUs. It provides access to state-of-the-art foundational weather models through a well-maintained infrastructure. Users can forecast weather conditions, such as wind speed and direction, by running simulations on their own GPUs or using modal volume or cloud services like s3 buckets. Skyrim supports various large weather models like Graphcast, Pangu, Fourcastnet, and DLWP, with plans for future enhancements like ensemble prediction and model quantization.
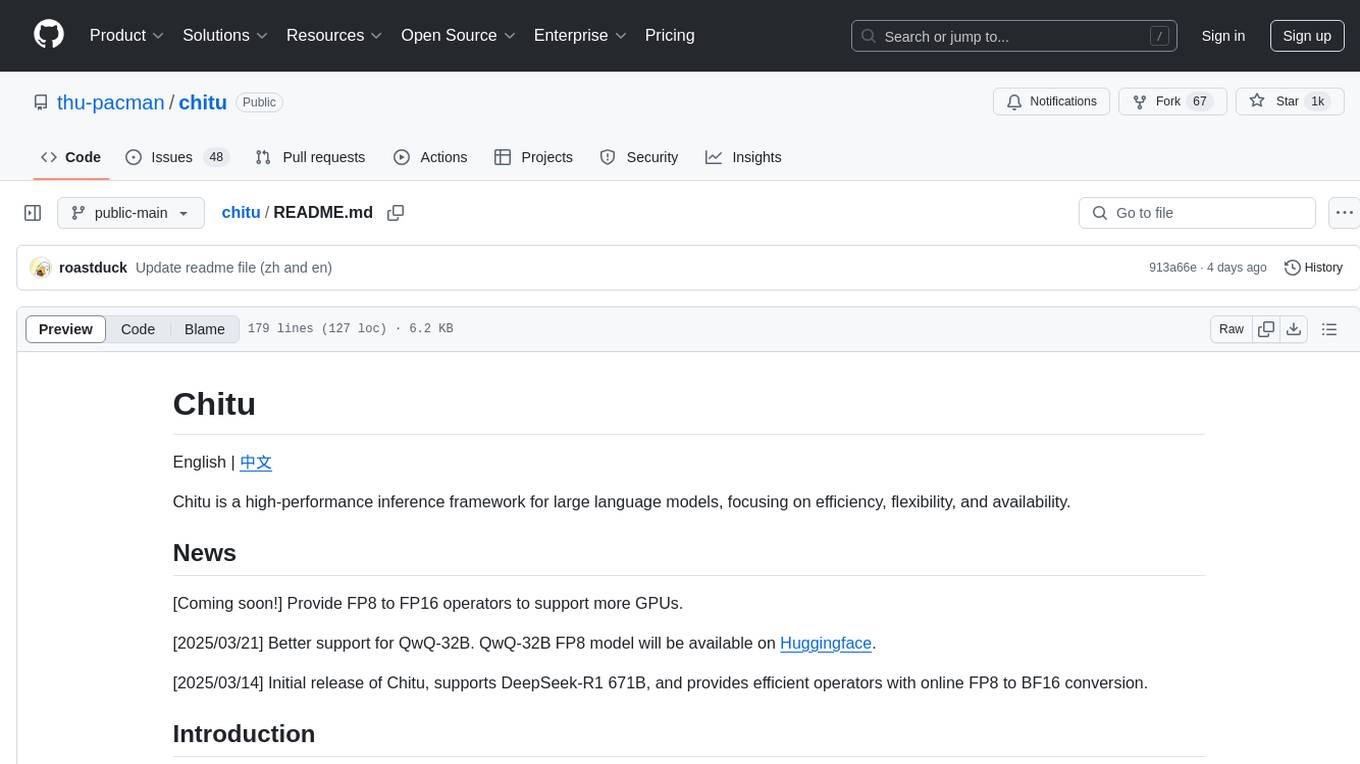
chitu
Chitu is a high-performance inference framework for large language models, focusing on efficiency, flexibility, and availability. It supports various mainstream large language models, including DeepSeek, LLaMA series, Mixtral, and more. Chitu integrates latest optimizations for large language models, provides efficient operators with online FP8 to BF16 conversion, and is deployed for real-world production. The framework is versatile, supporting various hardware environments beyond NVIDIA GPUs. Chitu aims to enhance output speed per unit computing power, especially in decoding processes dependent on memory bandwidth.
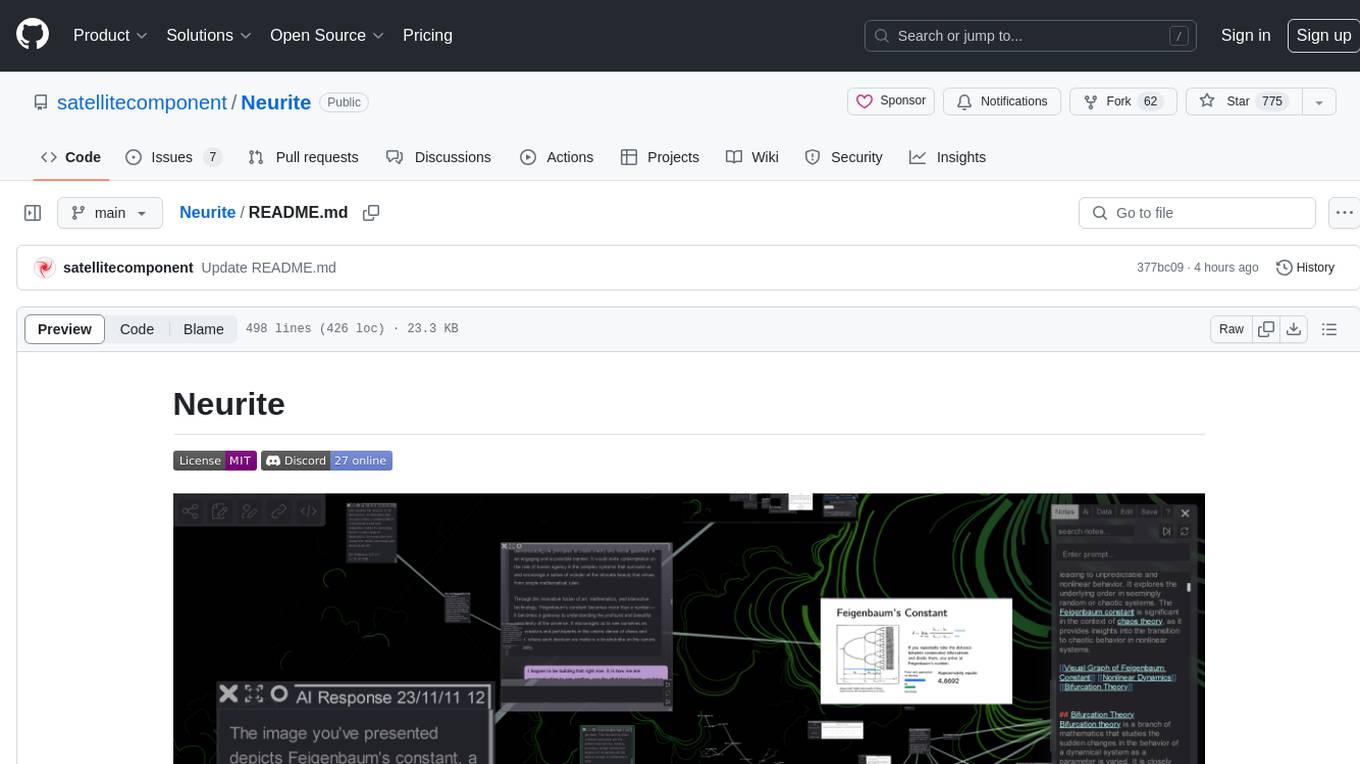
Neurite
Neurite is an innovative project that combines chaos theory and graph theory to create a digital interface that explores hidden patterns and connections for creative thinking. It offers a unique workspace blending fractals with mind mapping techniques, allowing users to navigate the Mandelbrot set in real-time. Nodes in Neurite represent various content types like text, images, videos, code, and AI agents, enabling users to create personalized microcosms of thoughts and inspirations. The tool supports synchronized knowledge management through bi-directional synchronization between mind-mapping and text-based hyperlinking. Neurite also features FractalGPT for modular conversation with AI, local AI capabilities for multi-agent chat networks, and a Neural API for executing code and sequencing animations. The project is actively developed with plans for deeper fractal zoom, advanced control over node placement, and experimental features.
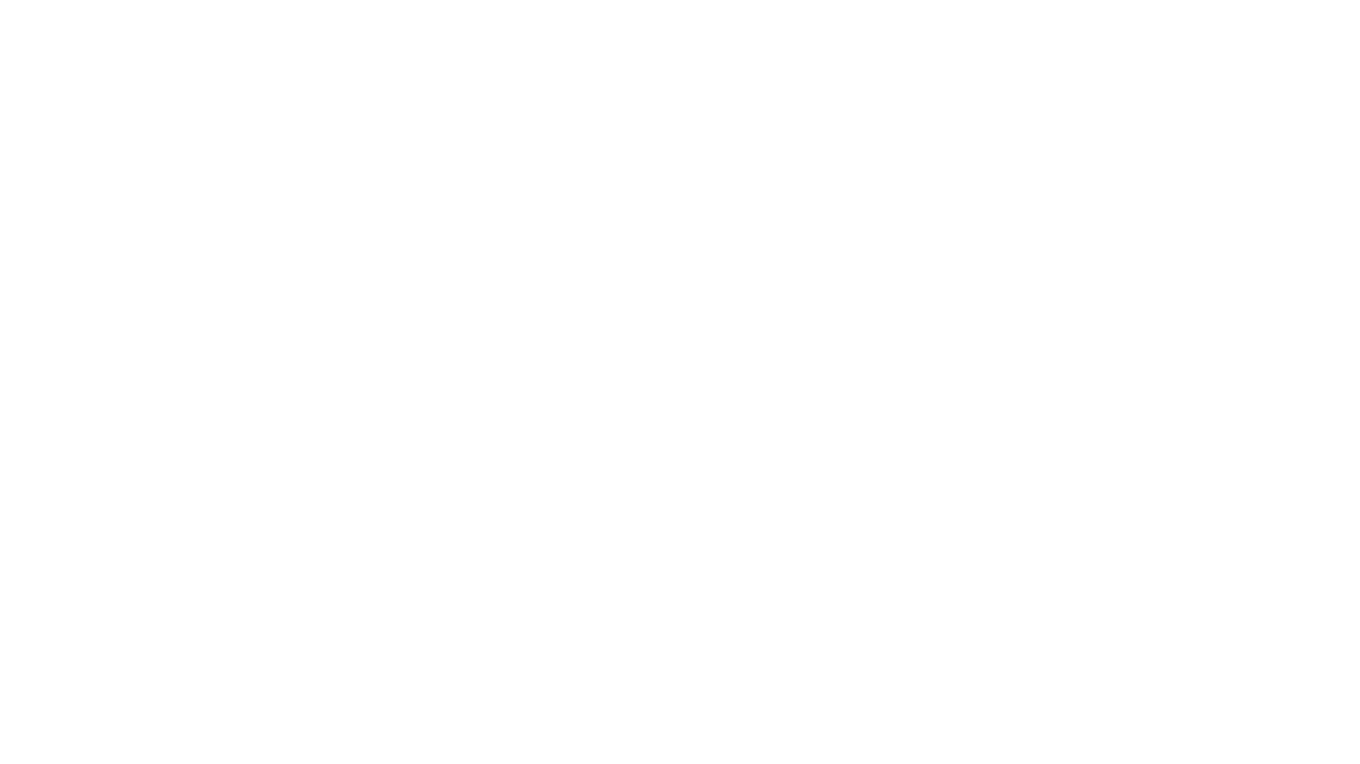
HolmesVAD
Holmes-VAD is a framework for unbiased and explainable Video Anomaly Detection using multimodal instructions. It addresses biased detection in challenging events by leveraging precise temporal supervision and rich multimodal instructions. The framework includes a largescale VAD instruction-tuning benchmark, VAD-Instruct50k, created with single-frame annotations and a robust video captioner. It offers accurate anomaly localization and comprehensive explanations through a customized solution for interpretable video anomaly detection.
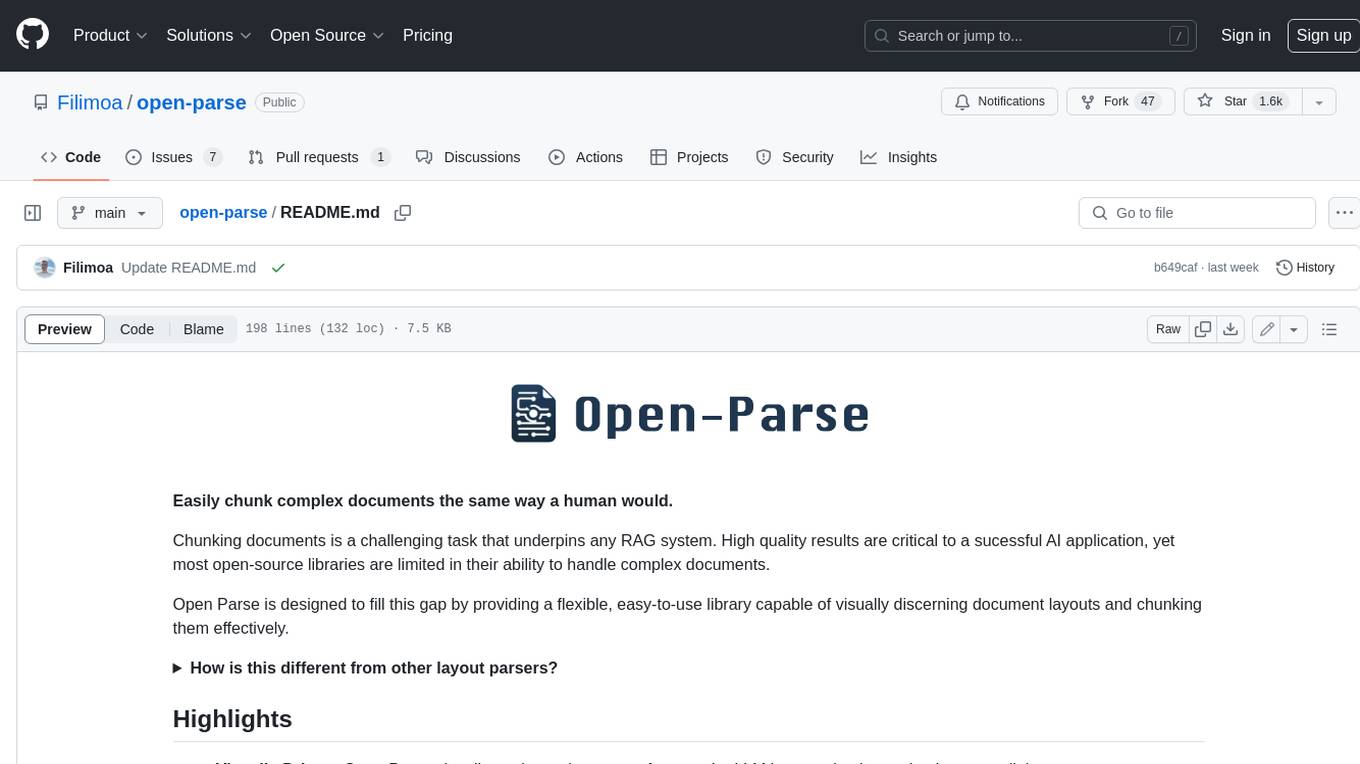
open-parse
Open Parse is a Python library for visually discerning document layouts and chunking them effectively. It is designed to fill the gap in open-source libraries for handling complex documents. Unlike text splitting, which converts a file to raw text and slices it up, Open Parse visually analyzes documents for superior LLM input. It also supports basic markdown for parsing headings, bold, and italics, and has high-precision table support, extracting tables into clean Markdown formats with accuracy that surpasses traditional tools. Open Parse is extensible, allowing users to easily implement their own post-processing steps. It is also intuitive, with great editor support and completion everywhere, making it easy to use and learn.
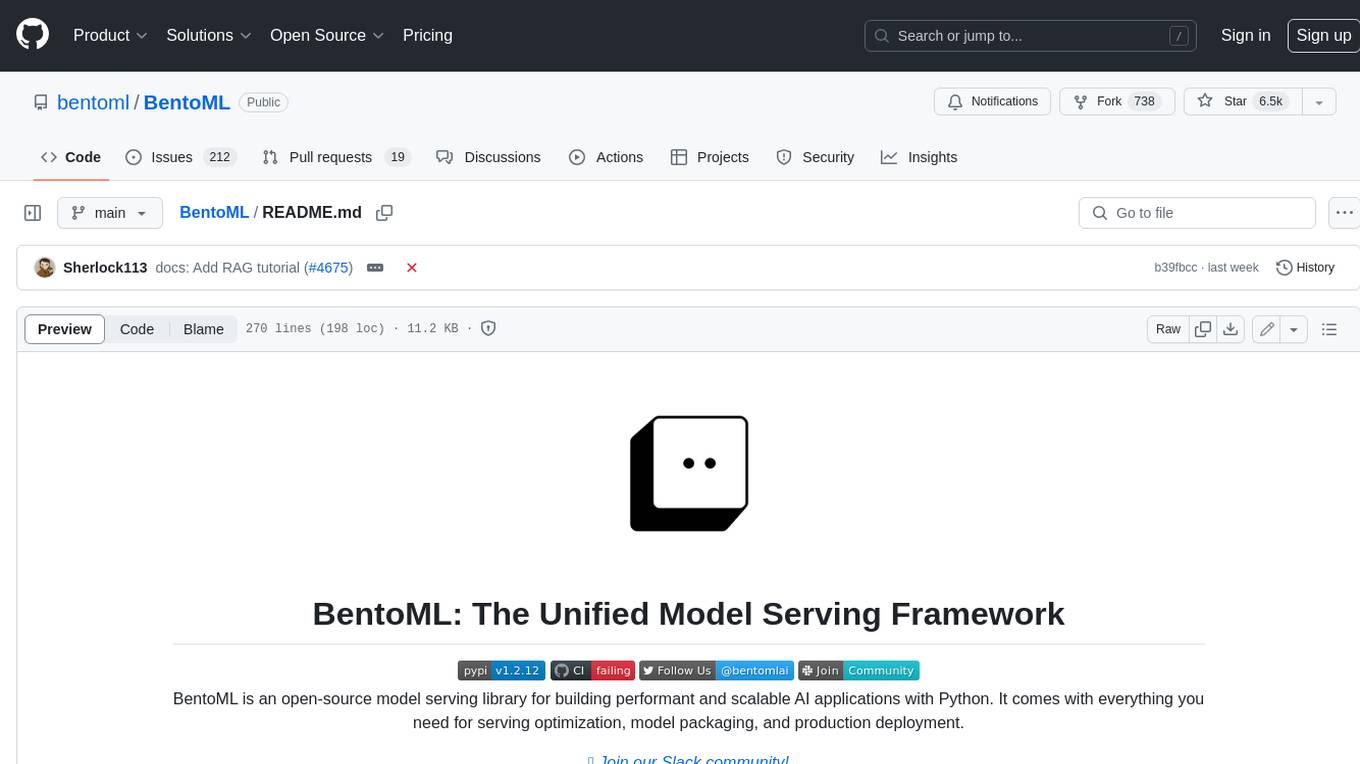
BentoML
BentoML is an open-source model serving library for building performant and scalable AI applications with Python. It comes with everything you need for serving optimization, model packaging, and production deployment.
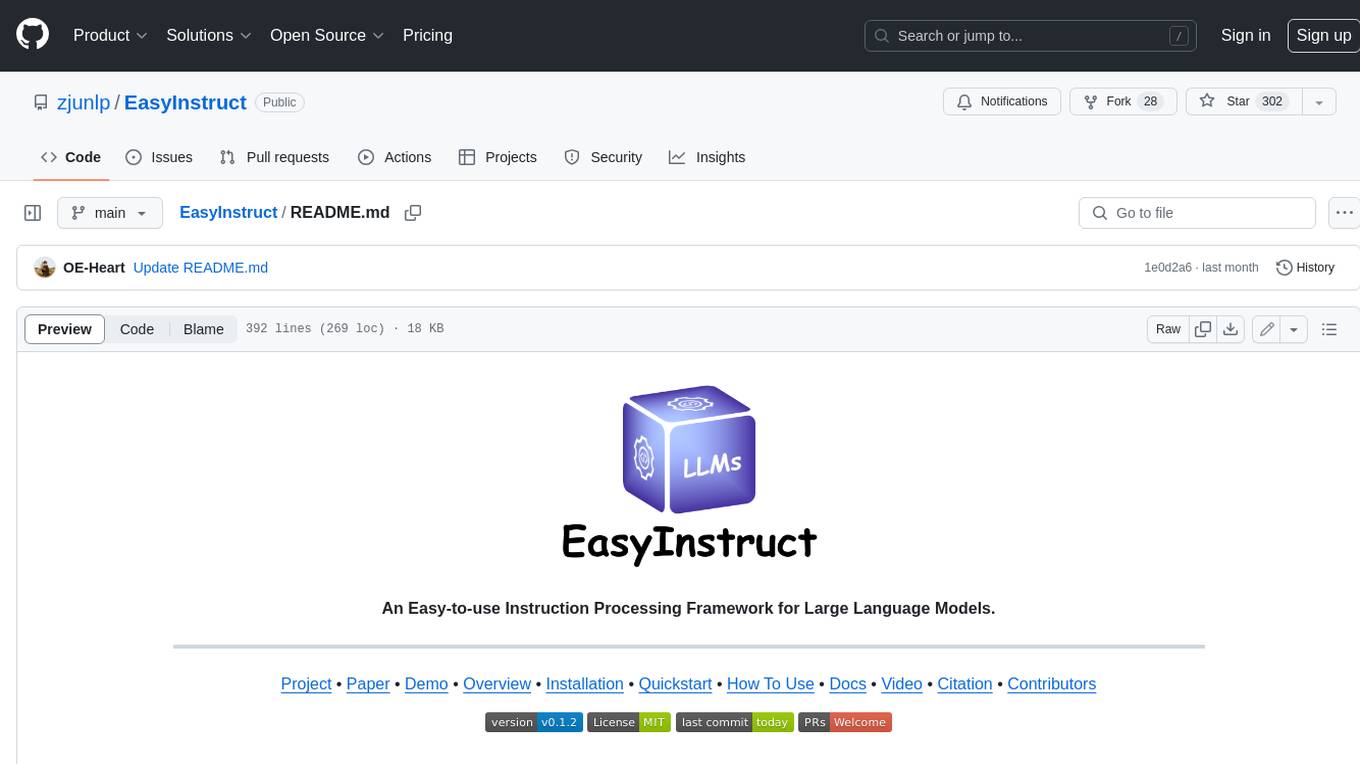
EasyInstruct
EasyInstruct is a Python package proposed as an easy-to-use instruction processing framework for Large Language Models (LLMs) like GPT-4, LLaMA, ChatGLM in your research experiments. EasyInstruct modularizes instruction generation, selection, and prompting, while also considering their combination and interaction.
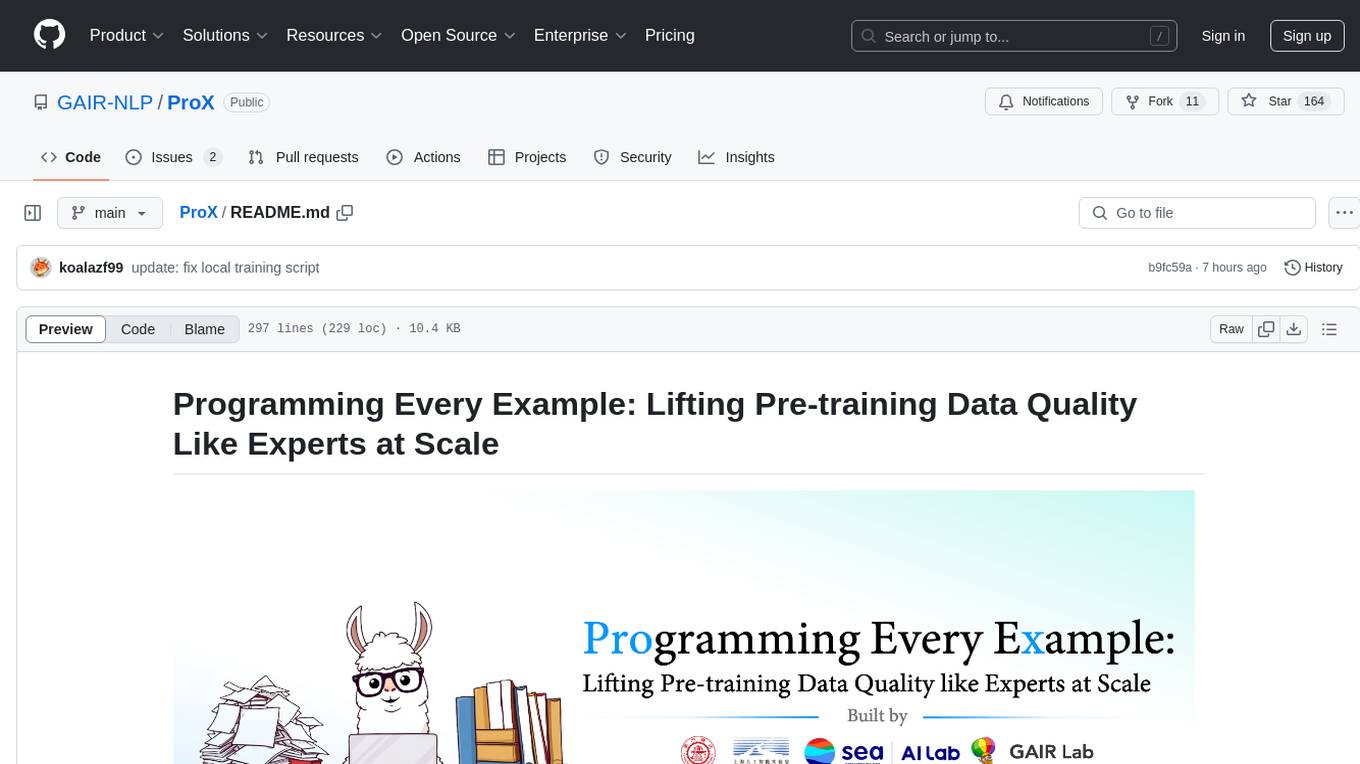
ProX
ProX is a lm-based data refinement framework that automates the process of cleaning and improving data used in pre-training large language models. It offers better performance, domain flexibility, efficiency, and cost-effectiveness compared to traditional methods. The framework has been shown to improve model performance by over 2% and boost accuracy by up to 20% in tasks like math. ProX is designed to refine data at scale without the need for manual adjustments, making it a valuable tool for data preprocessing in natural language processing tasks.
For similar tasks
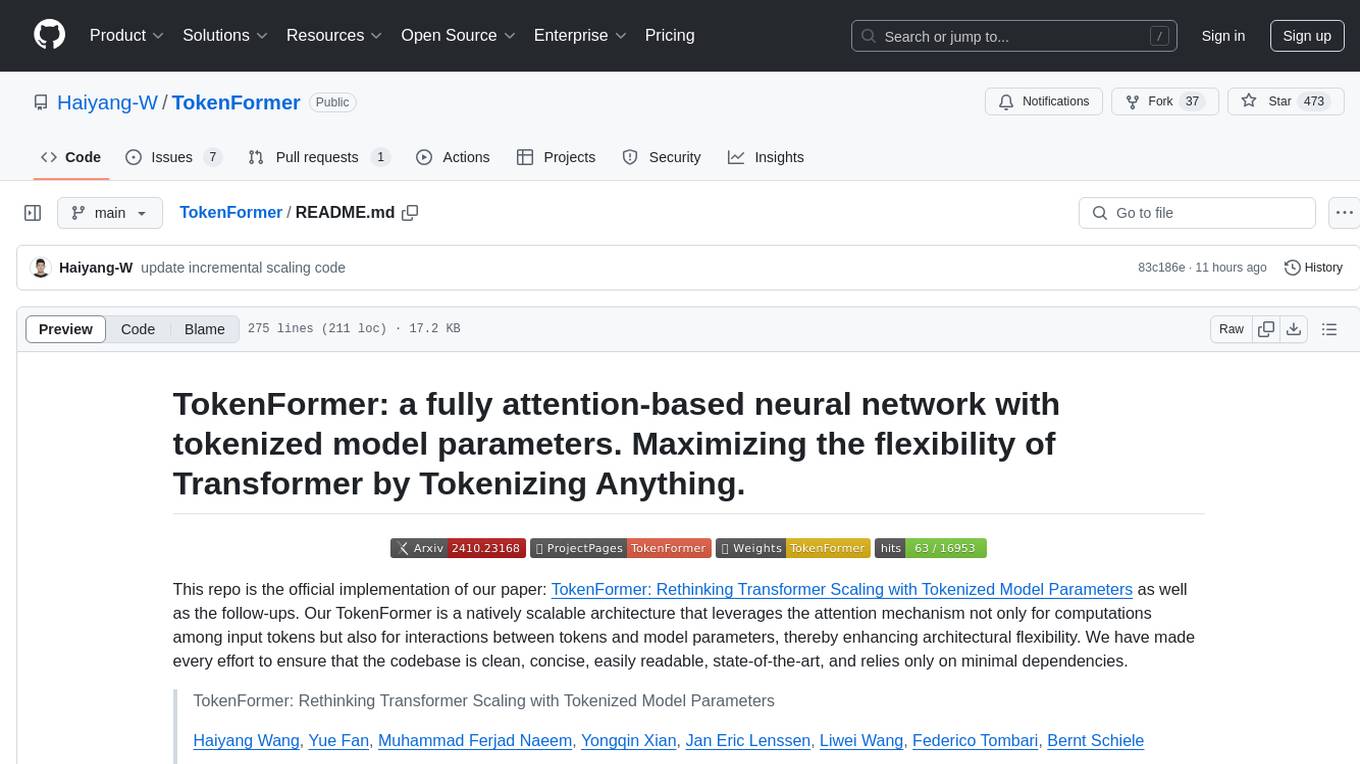
TokenFormer
TokenFormer is a fully attention-based neural network architecture that leverages tokenized model parameters to enhance architectural flexibility. It aims to maximize the flexibility of neural networks by unifying token-token and token-parameter interactions through the attention mechanism. The architecture allows for incremental model scaling and has shown promising results in language modeling and visual modeling tasks. The codebase is clean, concise, easily readable, state-of-the-art, and relies on minimal dependencies.
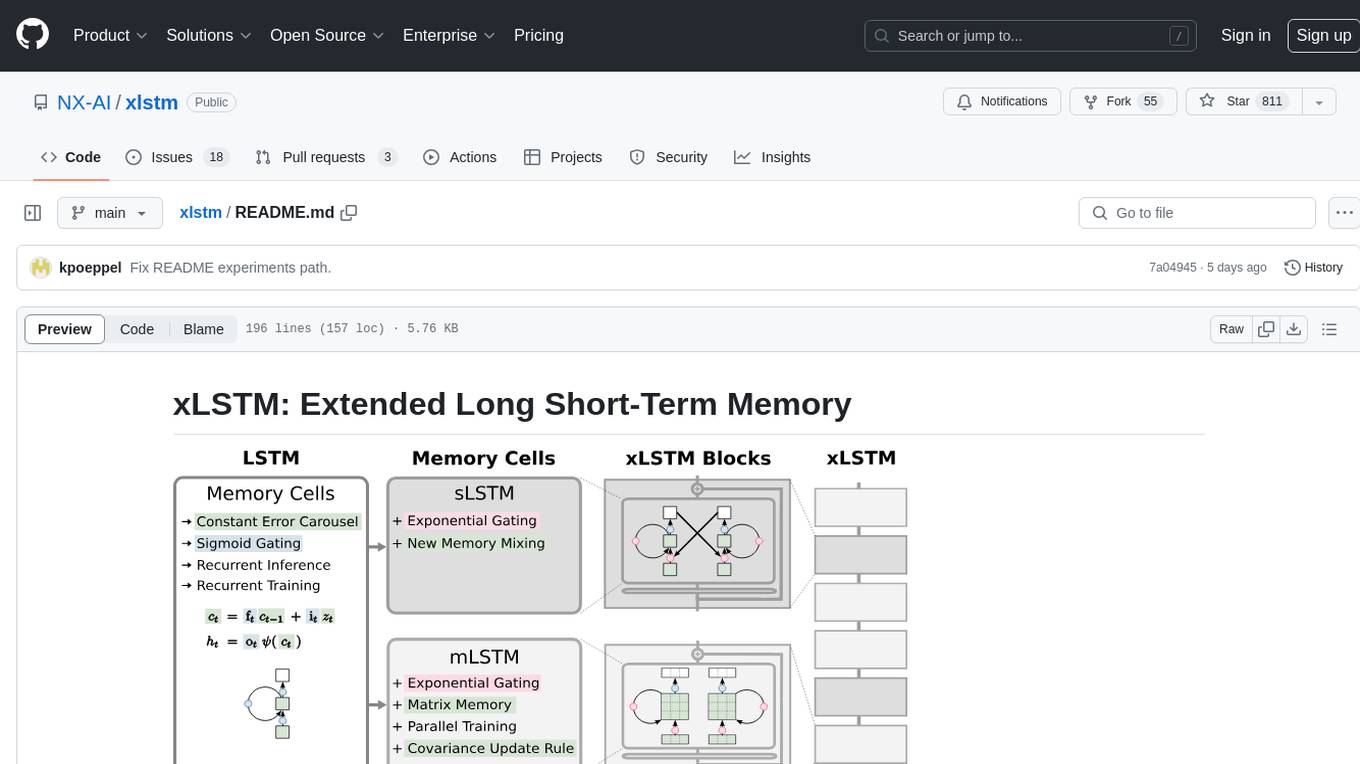
xlstm
xLSTM is a new Recurrent Neural Network architecture based on ideas of the original LSTM. Through Exponential Gating with appropriate normalization and stabilization techniques and a new Matrix Memory it overcomes the limitations of the original LSTM and shows promising performance on Language Modeling when compared to Transformers or State Space Models. The package is based on PyTorch and was tested for versions >=1.8. For the CUDA version of xLSTM, you need Compute Capability >= 8.0. The xLSTM tool provides two main components: xLSTMBlockStack for non-language applications or integrating in other architectures, and xLSTMLMModel for language modeling or other token-based applications.
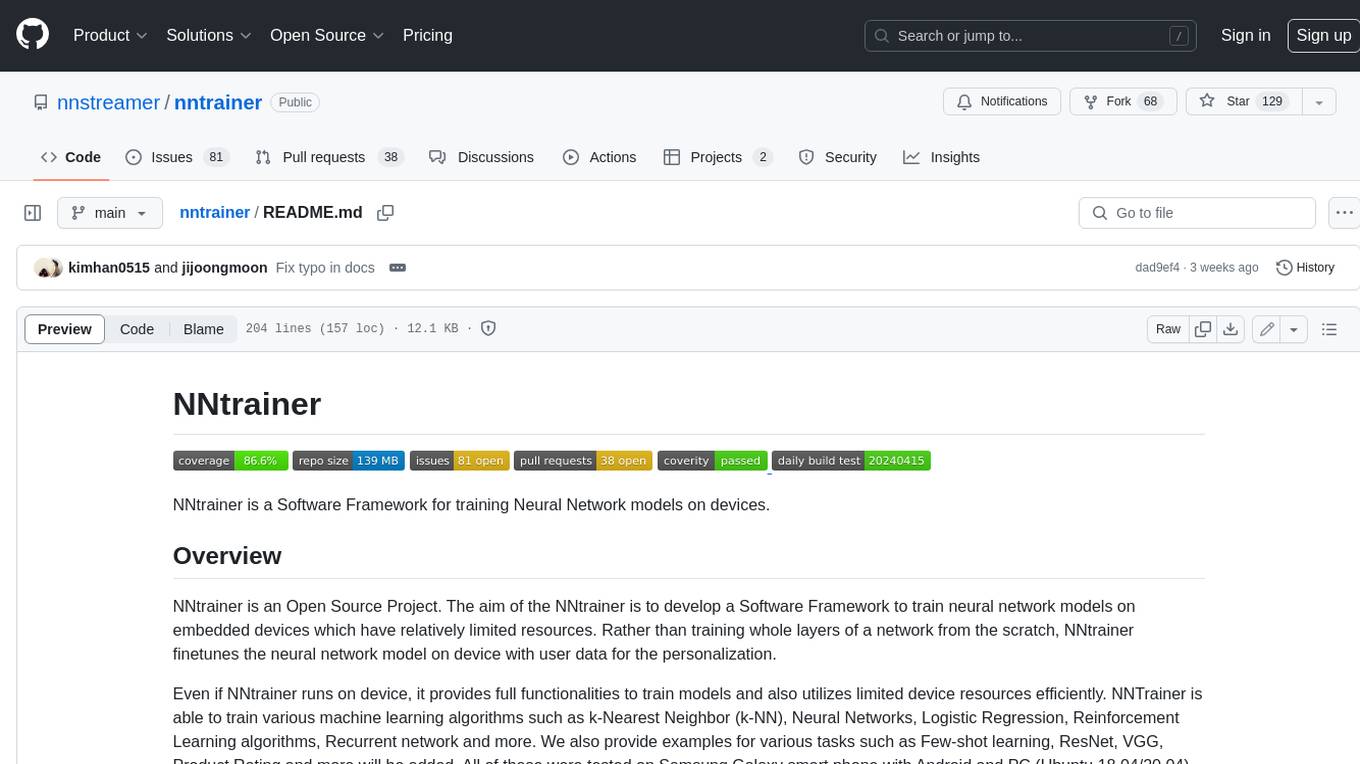
nntrainer
NNtrainer is a software framework for training neural network models on devices with limited resources. It enables on-device fine-tuning of neural networks using user data for personalization. NNtrainer supports various machine learning algorithms and provides examples for tasks such as few-shot learning, ResNet, VGG, and product rating. It is optimized for embedded devices and utilizes CBLAS and CUBLAS for accelerated calculations. NNtrainer is open source and released under the Apache License version 2.0.
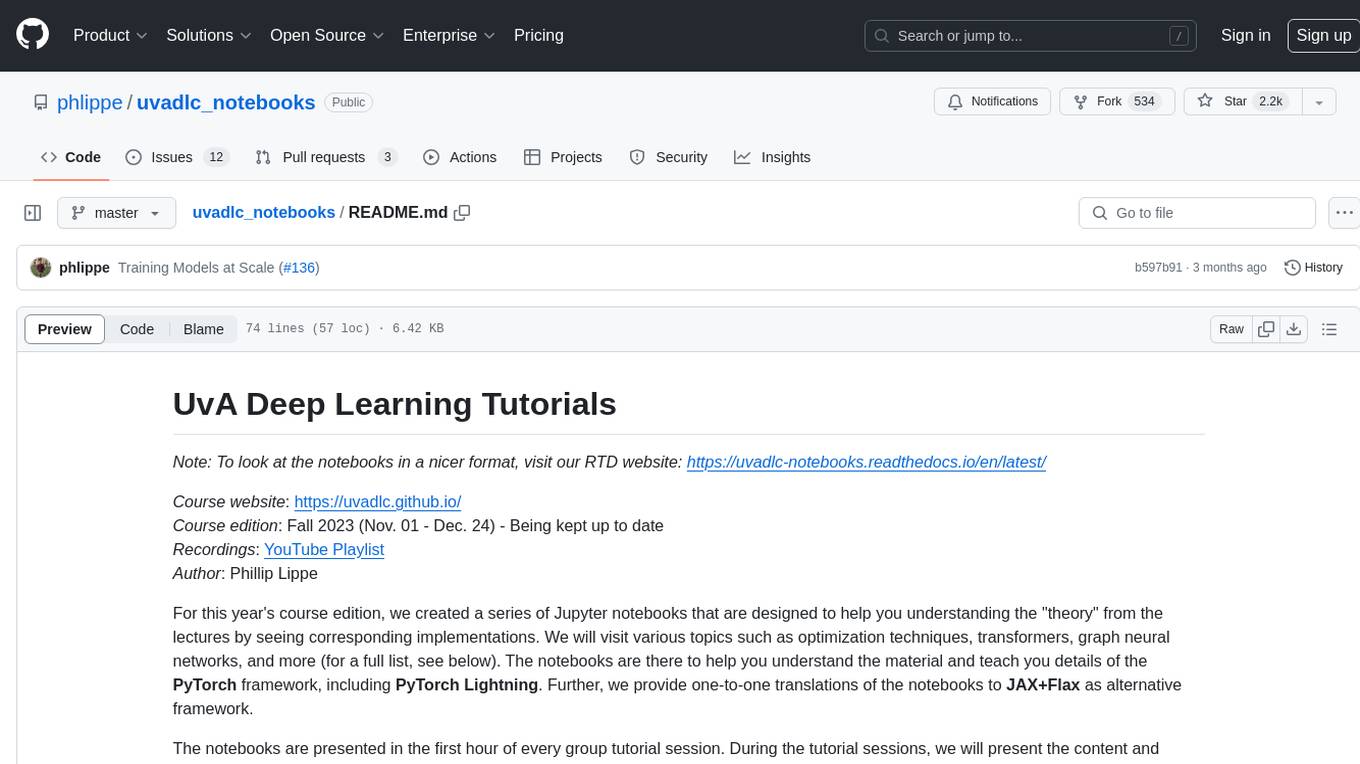
uvadlc_notebooks
The UvA Deep Learning Tutorials repository contains a series of Jupyter notebooks designed to help understand theoretical concepts from lectures by providing corresponding implementations. The notebooks cover topics such as optimization techniques, transformers, graph neural networks, and more. They aim to teach details of the PyTorch framework, including PyTorch Lightning, with alternative translations to JAX+Flax. The tutorials are integrated as official tutorials of PyTorch Lightning and are relevant for graded assignments and exams.
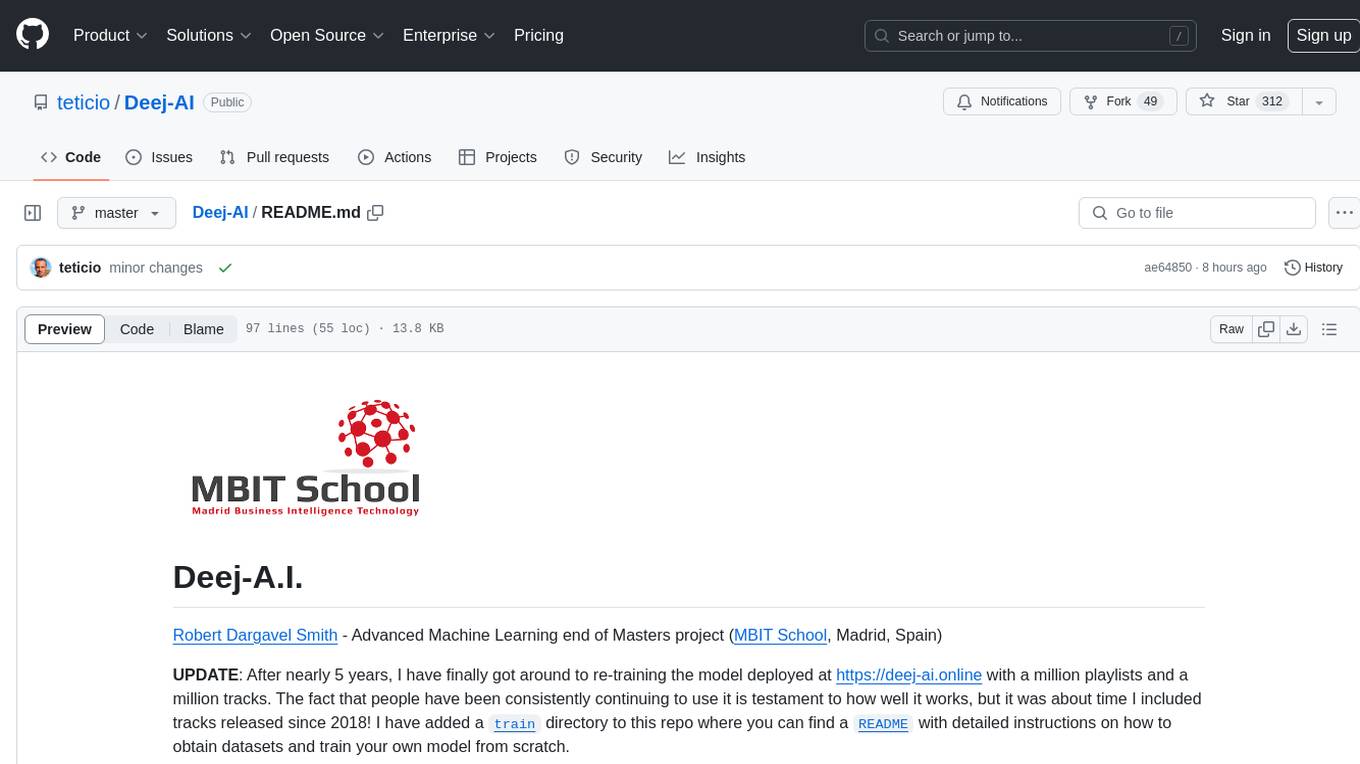
Deej-AI
Deej-A.I. is an advanced machine learning project that aims to revolutionize music recommendation systems by using artificial intelligence to analyze and recommend songs based on their content and characteristics. The project involves scraping playlists from Spotify, creating embeddings of songs, training neural networks to analyze spectrograms, and generating recommendations based on similarities in music features. Deej-A.I. offers a unique approach to music curation, focusing on the 'what' rather than the 'how' of DJing, and providing users with personalized and creative music suggestions.
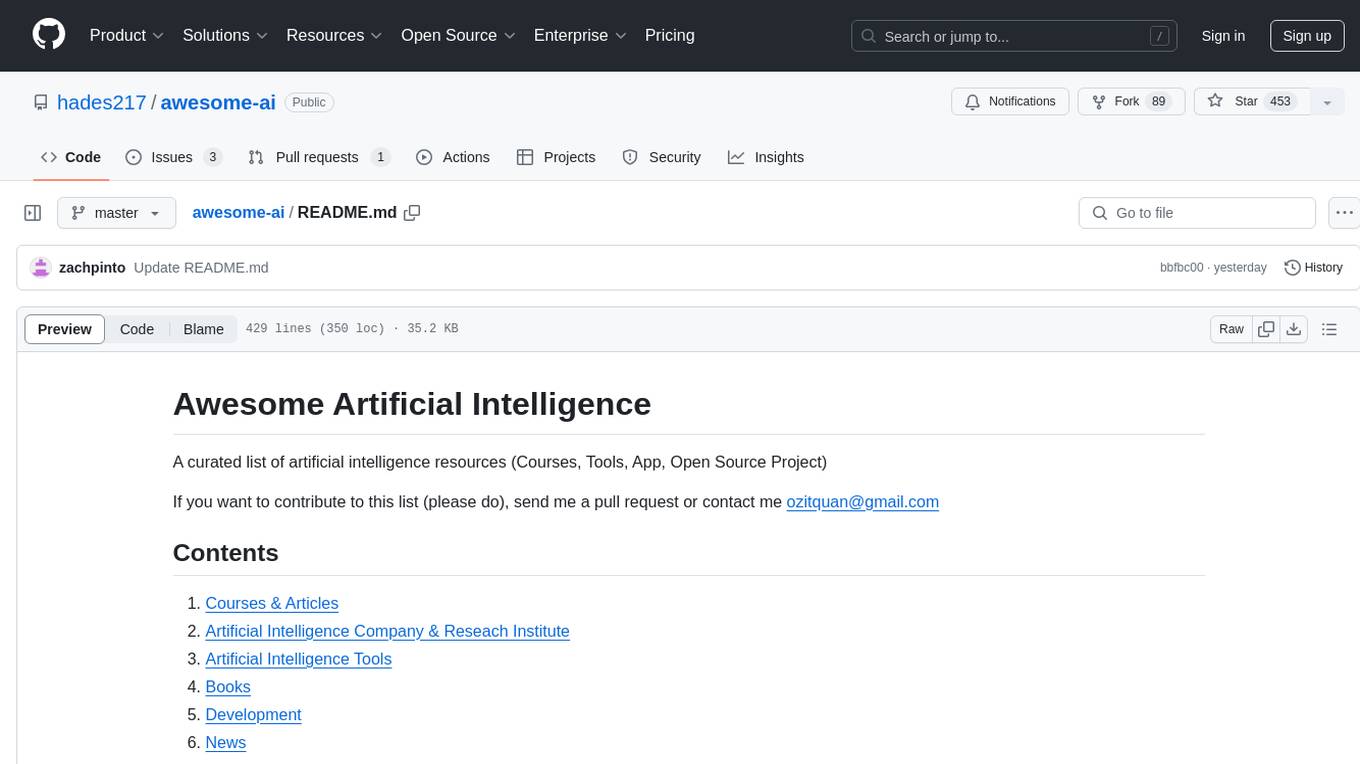
awesome-ai
Awesome AI is a curated list of artificial intelligence resources including courses, tools, apps, and open-source projects. It covers a wide range of topics such as machine learning, deep learning, natural language processing, robotics, conversational interfaces, data science, and more. The repository serves as a comprehensive guide for individuals interested in exploring the field of artificial intelligence and its applications across various domains.
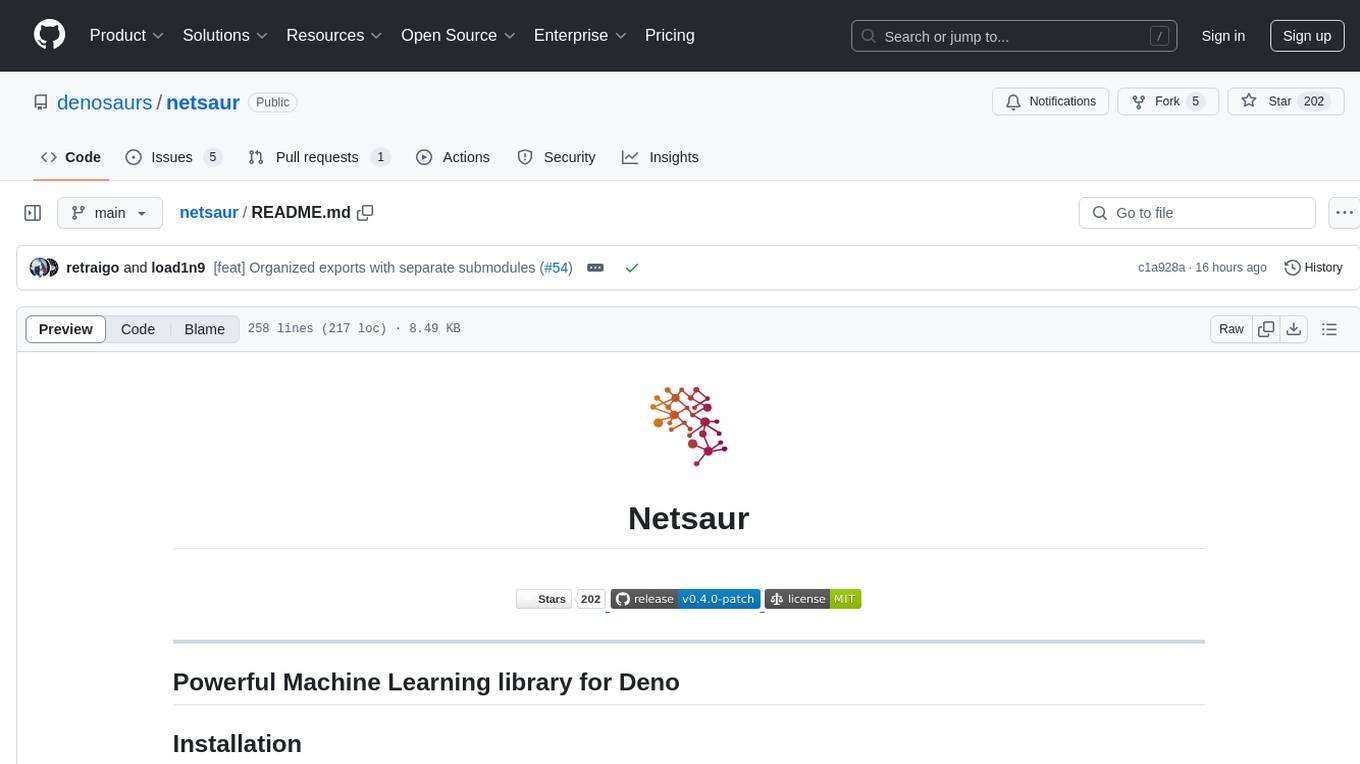
netsaur
Netsaur is a powerful machine learning library for Deno, offering a lightweight and easy-to-use neural network solution. It is blazingly fast and efficient, providing a simple API for creating and training neural networks. Netsaur can run on both CPU and GPU, making it suitable for serverless environments. With Netsaur, users can quickly build and deploy machine learning models for various applications with minimal dependencies. This library is perfect for both beginners and experienced machine learning practitioners.
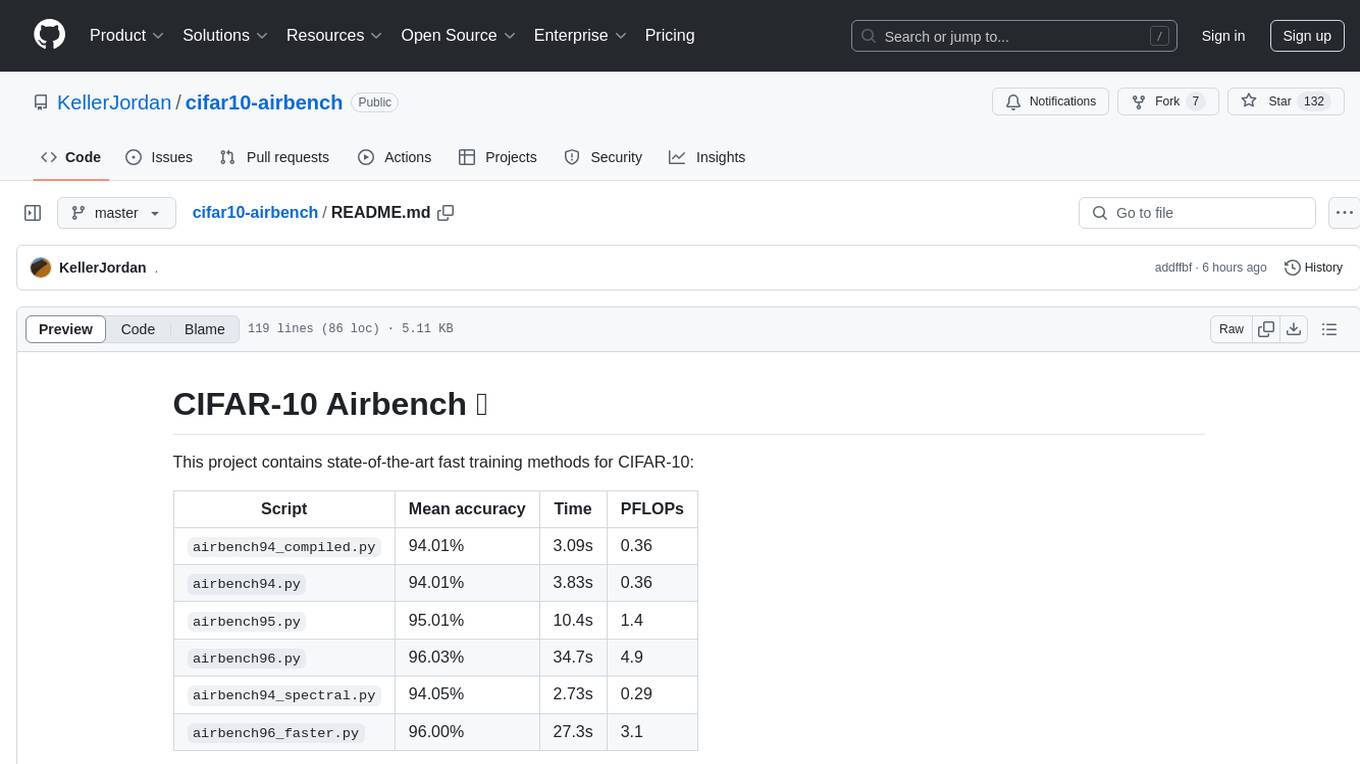
cifar10-airbench
CIFAR-10 Airbench is a project offering fast and stable training baselines for CIFAR-10 dataset, facilitating machine learning research. It provides easily runnable PyTorch scripts for training neural networks with high accuracy levels. The methods used in this project aim to accelerate research on fundamental properties of deep learning. The project includes GPU-accelerated dataloader for custom experiments and trainings, and can be used for data selection and active learning experiments. The training methods provided are faster than standard ResNet training, offering improved performance for research projects.
For similar jobs
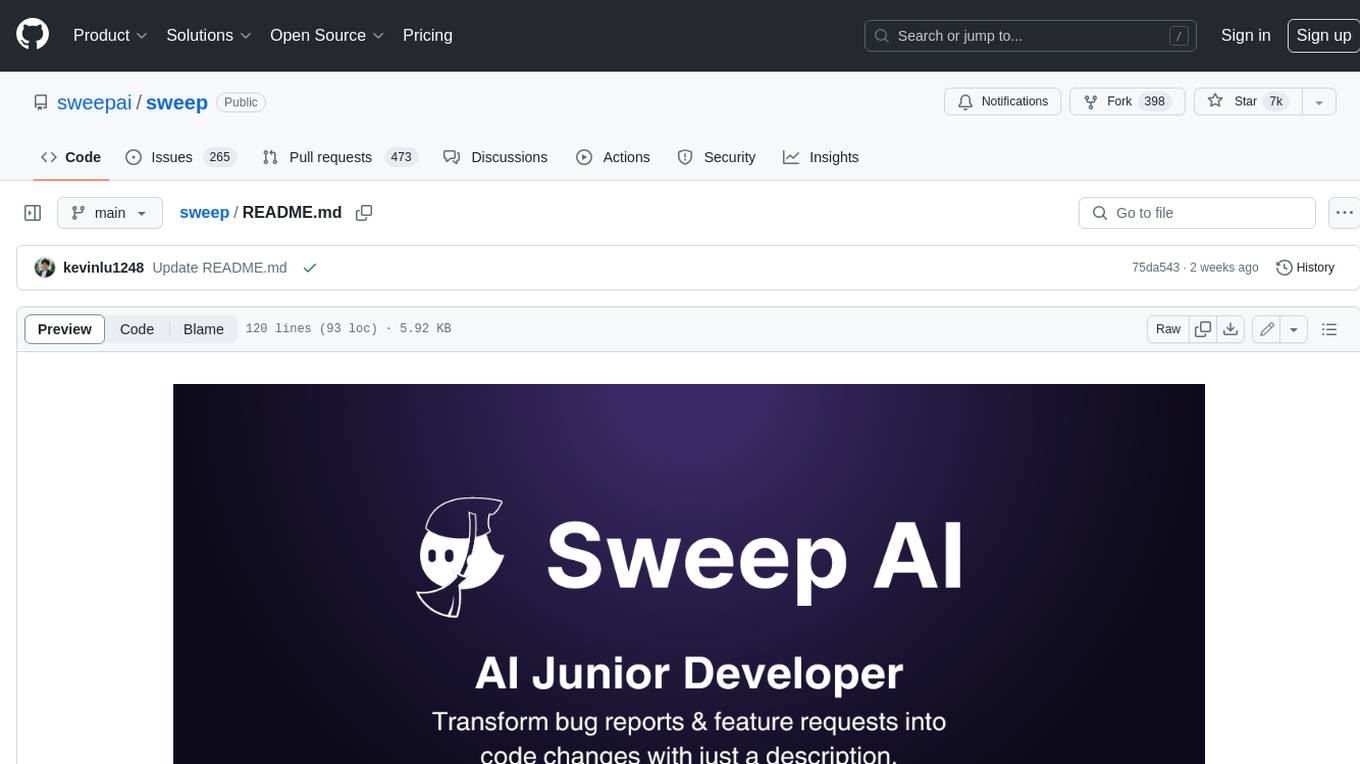
sweep
Sweep is an AI junior developer that turns bugs and feature requests into code changes. It automatically handles developer experience improvements like adding type hints and improving test coverage.
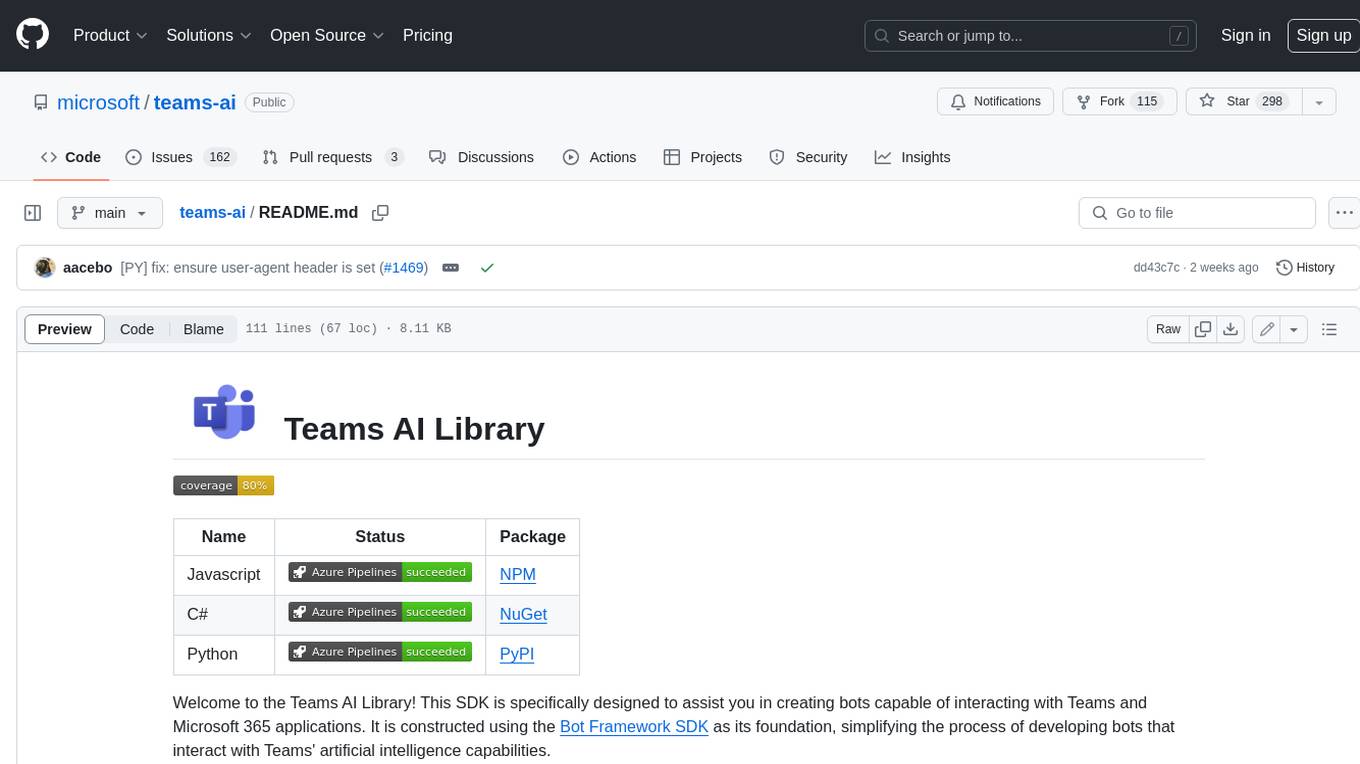
teams-ai
The Teams AI Library is a software development kit (SDK) that helps developers create bots that can interact with Teams and Microsoft 365 applications. It is built on top of the Bot Framework SDK and simplifies the process of developing bots that interact with Teams' artificial intelligence capabilities. The SDK is available for JavaScript/TypeScript, .NET, and Python.
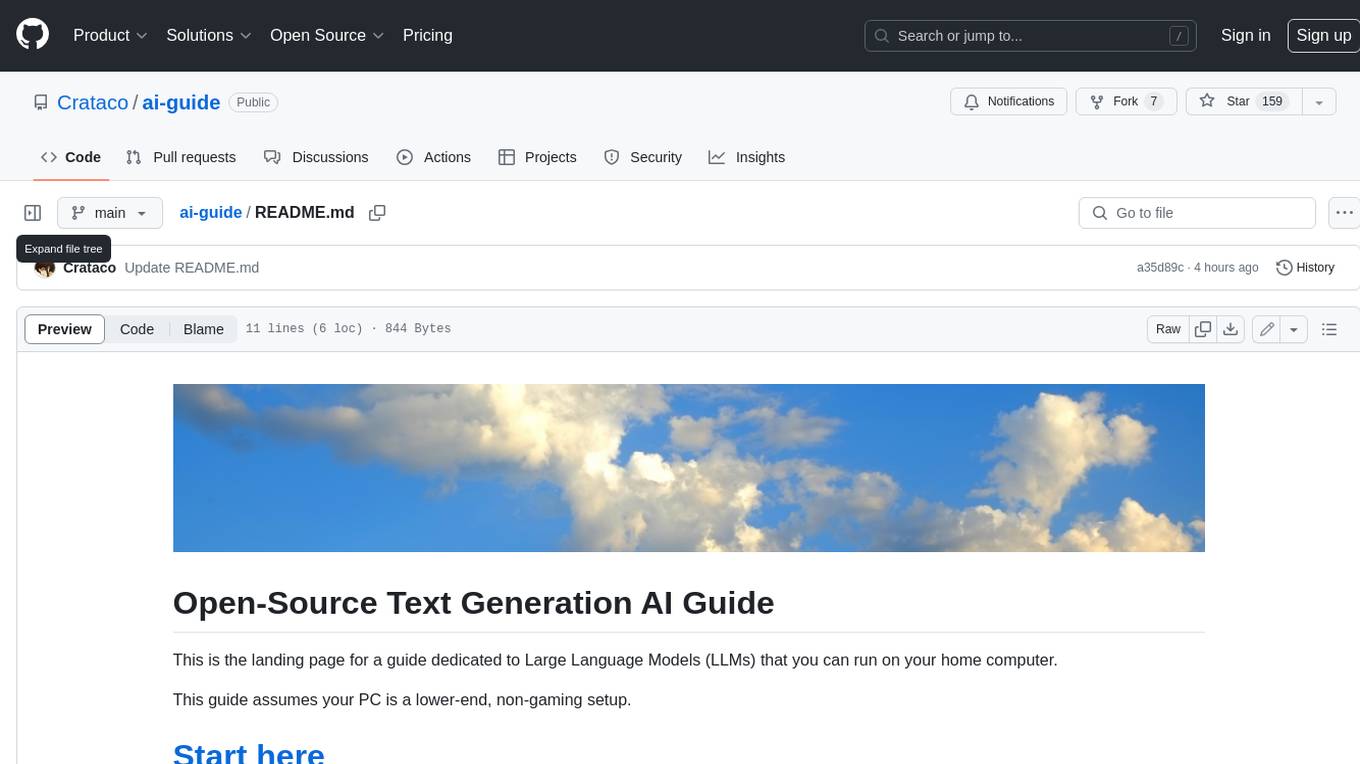
ai-guide
This guide is dedicated to Large Language Models (LLMs) that you can run on your home computer. It assumes your PC is a lower-end, non-gaming setup.
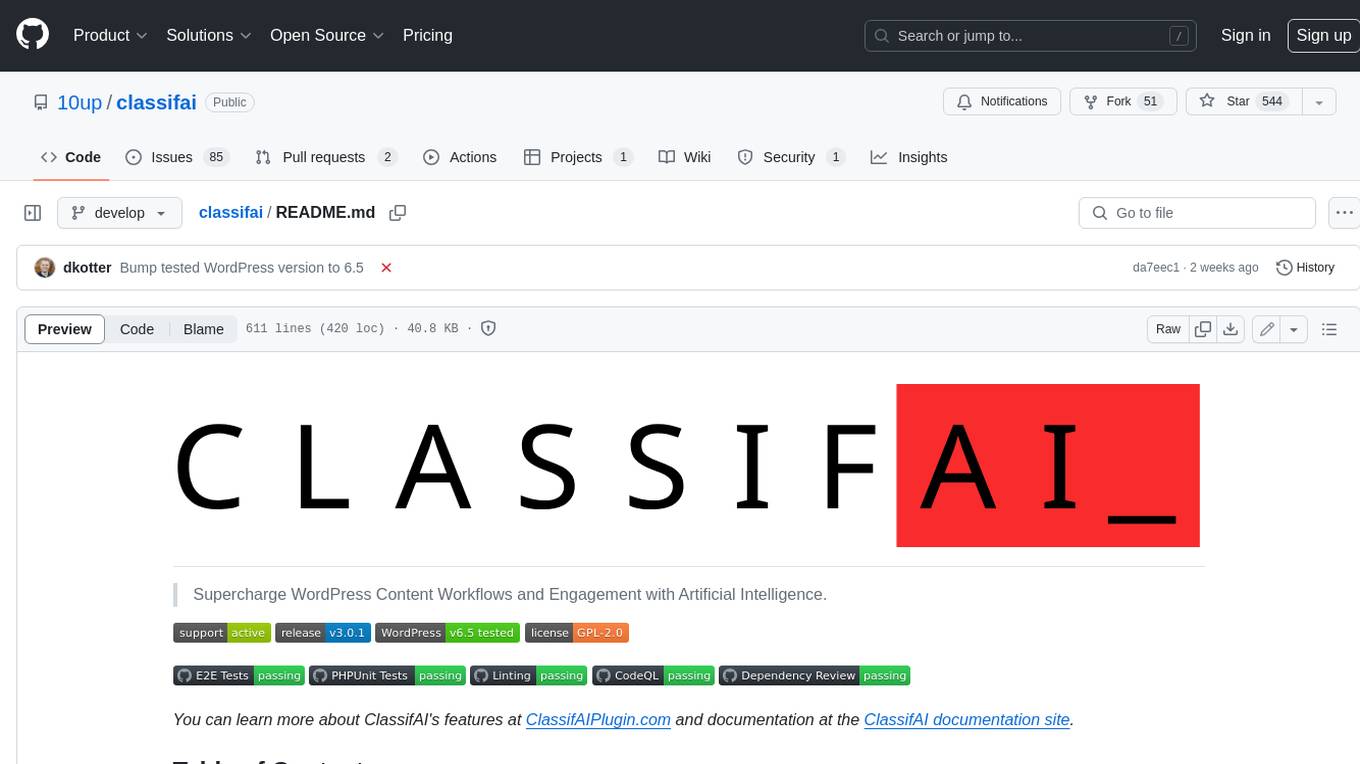
classifai
Supercharge WordPress Content Workflows and Engagement with Artificial Intelligence. Tap into leading cloud-based services like OpenAI, Microsoft Azure AI, Google Gemini and IBM Watson to augment your WordPress-powered websites. Publish content faster while improving SEO performance and increasing audience engagement. ClassifAI integrates Artificial Intelligence and Machine Learning technologies to lighten your workload and eliminate tedious tasks, giving you more time to create original content that matters.
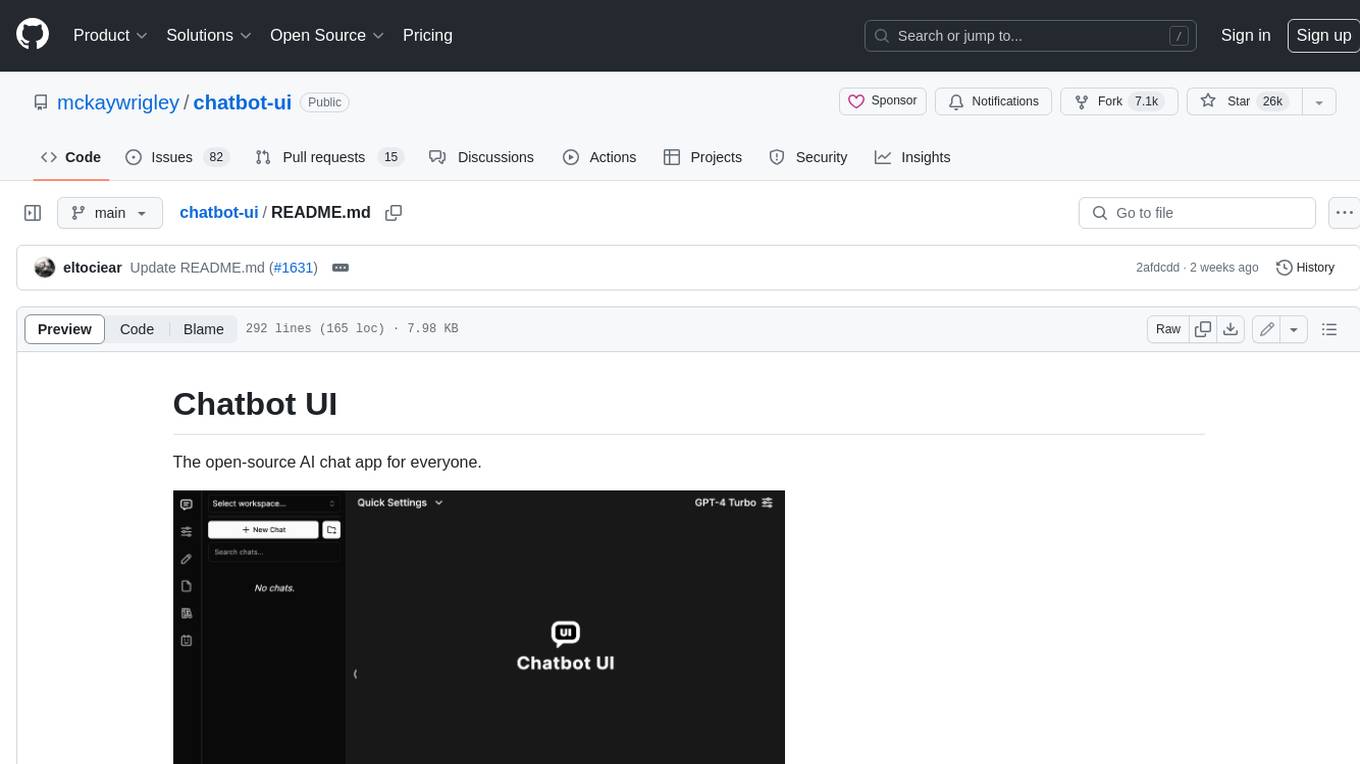
chatbot-ui
Chatbot UI is an open-source AI chat app that allows users to create and deploy their own AI chatbots. It is easy to use and can be customized to fit any need. Chatbot UI is perfect for businesses, developers, and anyone who wants to create a chatbot.
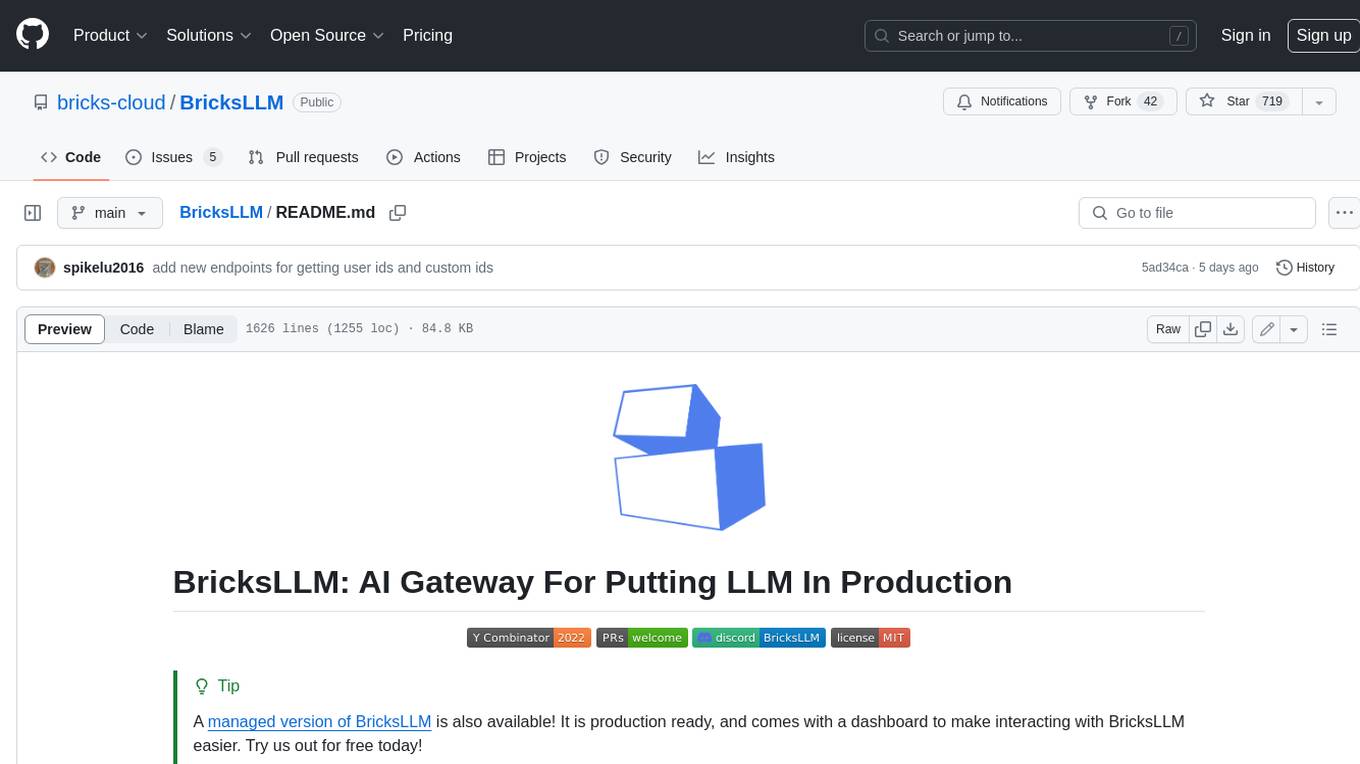
BricksLLM
BricksLLM is a cloud native AI gateway written in Go. Currently, it provides native support for OpenAI, Anthropic, Azure OpenAI and vLLM. BricksLLM aims to provide enterprise level infrastructure that can power any LLM production use cases. Here are some use cases for BricksLLM: * Set LLM usage limits for users on different pricing tiers * Track LLM usage on a per user and per organization basis * Block or redact requests containing PIIs * Improve LLM reliability with failovers, retries and caching * Distribute API keys with rate limits and cost limits for internal development/production use cases * Distribute API keys with rate limits and cost limits for students
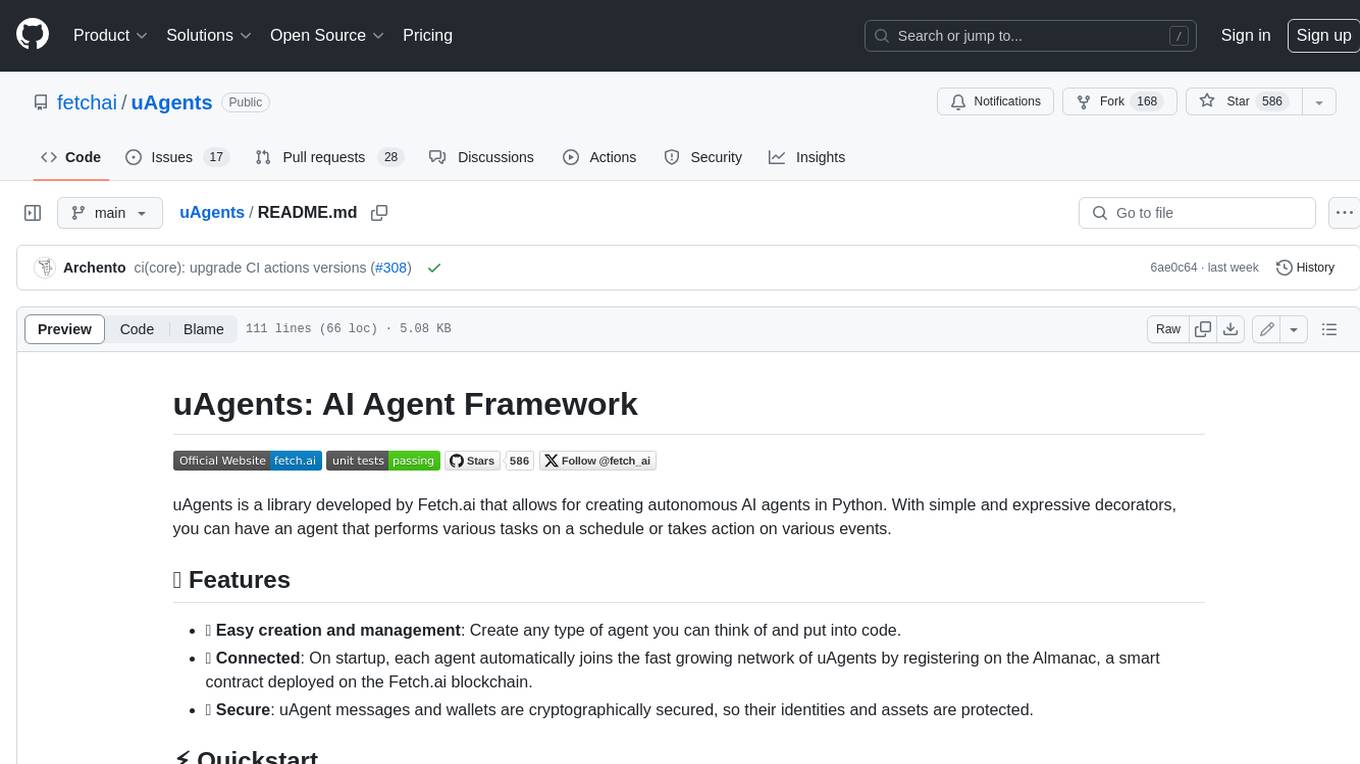
uAgents
uAgents is a Python library developed by Fetch.ai that allows for the creation of autonomous AI agents. These agents can perform various tasks on a schedule or take action on various events. uAgents are easy to create and manage, and they are connected to a fast-growing network of other uAgents. They are also secure, with cryptographically secured messages and wallets.
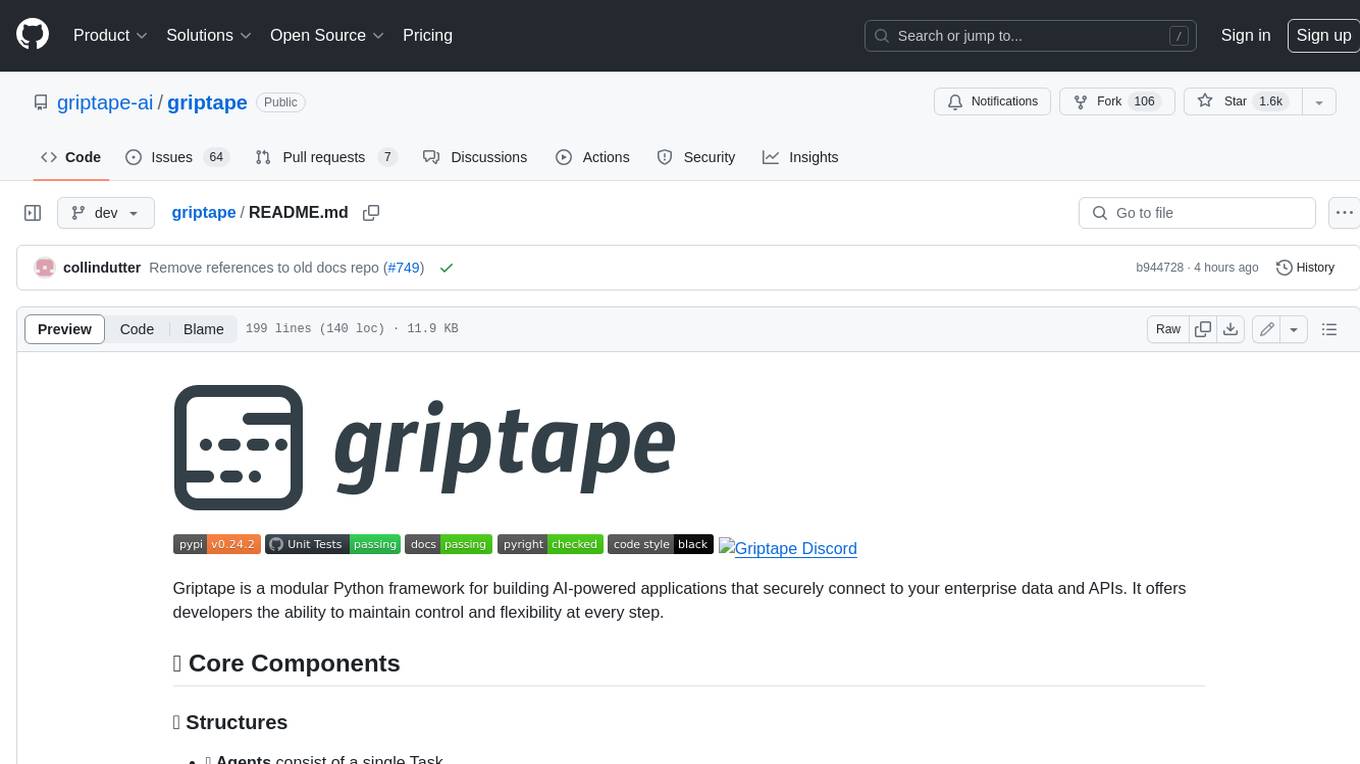
griptape
Griptape is a modular Python framework for building AI-powered applications that securely connect to your enterprise data and APIs. It offers developers the ability to maintain control and flexibility at every step. Griptape's core components include Structures (Agents, Pipelines, and Workflows), Tasks, Tools, Memory (Conversation Memory, Task Memory, and Meta Memory), Drivers (Prompt and Embedding Drivers, Vector Store Drivers, Image Generation Drivers, Image Query Drivers, SQL Drivers, Web Scraper Drivers, and Conversation Memory Drivers), Engines (Query Engines, Extraction Engines, Summary Engines, Image Generation Engines, and Image Query Engines), and additional components (Rulesets, Loaders, Artifacts, Chunkers, and Tokenizers). Griptape enables developers to create AI-powered applications with ease and efficiency.