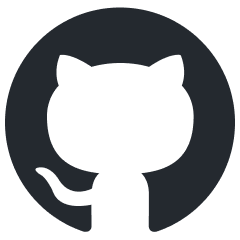
skyrim
π π€ AI weather models united
Stars: 150
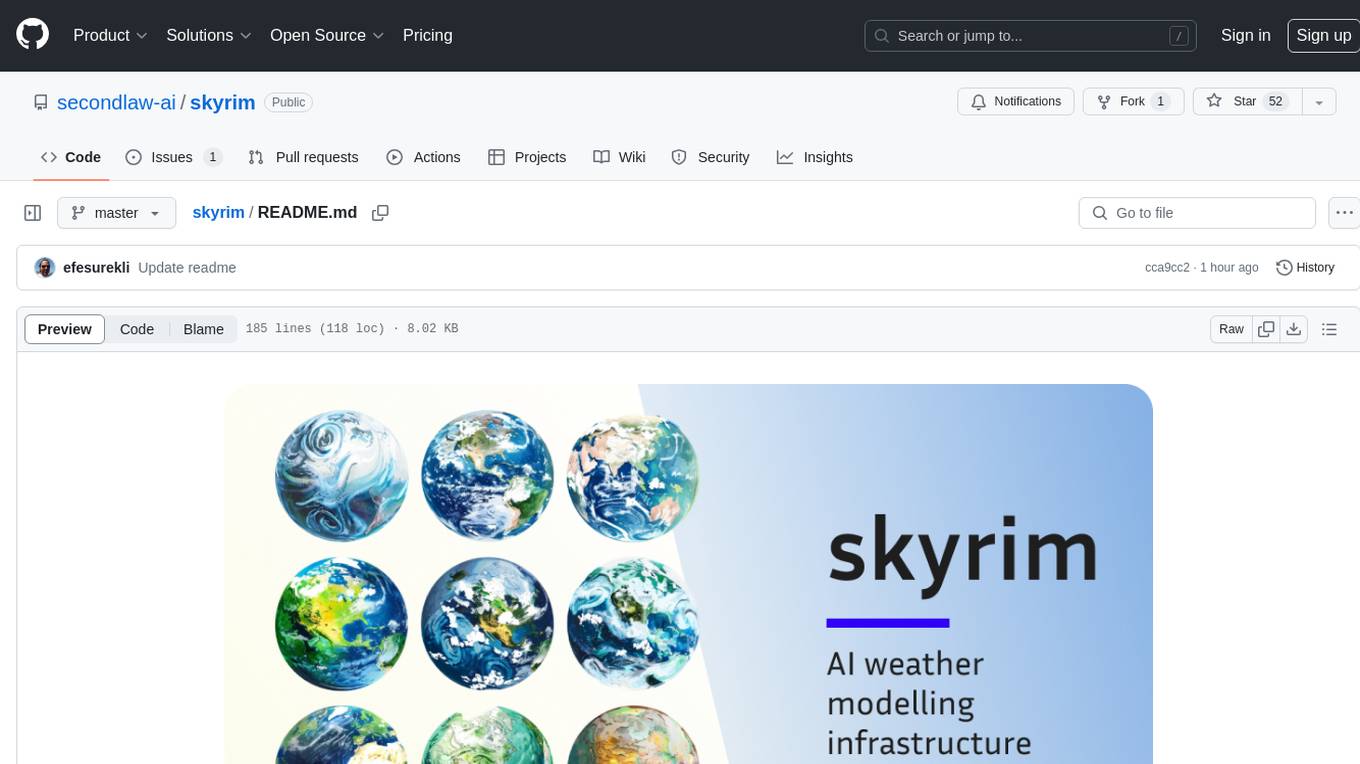
Skyrim is a weather forecasting tool that enables users to run large weather models using consumer-grade GPUs. It provides access to state-of-the-art foundational weather models through a well-maintained infrastructure. Users can forecast weather conditions, such as wind speed and direction, by running simulations on their own GPUs or using modal volume or cloud services like s3 buckets. Skyrim supports various large weather models like Graphcast, Pangu, Fourcastnet, and DLWP, with plans for future enhancements like ensemble prediction and model quantization.
README:
π₯ Run state-of-the-art large weather models in less than 2 minutes.
πͺοΈ Ensemble and fine-tune (soon) to push the limits on forecasting.
π Simulate extreme weather events!
Skyrim allows you to run any large weather model with a consumer grade GPU.
Until very recently, weather forecasts were run in 100K+ CPU HPC clusters, solving massive numerical weather models (NWP). Within last 2 years, open-source foundation models trained on weather simulation datasets surpassed the skill level of these numerical models.
Our goal is to make these models accessible by providing a well maintained infrastructure.
Clone the repo, set an env (either conda or venv) and then run
git clone https://github.com/your-repo/skyrim.git
cd skyrim
pip install .
Depending on your use-case (i.e. AWS storage needs or CDS initial conditions), you may need to fill in a .env
by cp .env.example .env
.
Skyrim currently supports either running on on modal, on a container βfor instance vast.ai or bare metal(you will need an NVIDIA GPU with at least 24GB and installation can be long).
Modal is the fastest option, it will run forecasts "serverless" so you don't have to worry about the infrastructure.
You will need a modal key. Run modal setup
and set it up (<1 min).
Modal comes with $30 free credits and a single forecast costs about 2 cents as of May 2024.
Once you are all good to go, then run:
modal run skyrim/modal/forecast.py
This by default uses pangu
model to forecast for the next 6 hours, starting from yesterday. It gets initial conditions from NOAA GFS and writes the forecast to a modal volume. You can choose different dates and weather models as shown in here.
After you have your forecast, you can explore it by running a notebook (without GPU, so cheap) in modal:
modal run skyrim/modal/forecast.py::run_analysis
This will output a jupyter notebook link that you can follow and access the forecast. For instance, to read the forecast you can run from the notebook the following:
import xarray as xr
forecast = xr.open_dataset('/skyrim/outputs/[forecast_id]/[filename], engine='scipy')
Once you are done, best is to delete the volume as a daily forecast is about 2GB:
modal volume rm forecasts /[forecast_id] -r
If you don't want to use modal volume, and want to aggregate results in a bucket (currently only s3), you just have to run:
modal run skyrim/modal/forecast.py --output_dir s3://skyrim-dev
where skyrim-dev
is the bucket that you want to aggregate the forecasts. By default, zarr
format is used to store in AWS/GCP so you can read and move only the parts of the forecasts that you need.
See examples section for more.βοΈ
If you are running on your own GPUs, installed either via bare metal or via containers such as vast.ai then you can directly get forecasts as such:
from skyrim.core import Skyrim
model = Skyrim("pangu")
final_pred, pred_paths = model.predict(
date="20240507", # format: YYYYMMDD, start date of the forecast
time="0000", # format: HHMM, start time of the forecast
lead_time=24 * 7, # in hours, next week
save=True,
)
To visualise the forecast:
from skyrim.libs.plotting import visualize_rollout
visualize_rollout(output_paths=pred_paths, channels=["u10m", "v10m"], output_dir=".")
or you can still use the command line:
forecast -m graphcast --lead_time 24 --initial_conditions cds --date 20240330`
See examples section for more.βοΈ
- Find a machine you like RTX3090 or above with at least 24GB memory. Make sure you have good bandwith (+500MB/s).
- Select the instance template from here.
- Then clone the repo and
pip install . && pip install -r requirements.txt
- You will need a NVIDIA GPU with at least 24GB. We are working on quantization as well so that in the future it would be possible to run simulations with much less compute. Have an environment set with Python == 3.10, Pytorch => 2.2.2 and CUDA +12.x. Or if easier start with the docker image:
nvcr.io/nvidia/pytorch:24.01-py3
. - Install conda (miniconda for instance). Then run in that environment:
conda create -y -n skyenv python=3.10
conda activate skyenv
conda install eccodes python-eccodes -c conda-forge
pip install . && pip install -r requirements.txt
For each run, you will first pull the initial conditions of your interest (most recent one by default), then the model will run for the desired time step. Initial conditions are pulled from GFS, ECMWF IFS (Operational) or CDS (ERA5 Reanalysis Dataset).
If you are using CDS initial conditions, then you will need a CDS API key in your .env
βcp .env.example
and paste.
All examples can be run using forecast
or modal run skyrim/modal/forecast.py
. You just have to make snake case options kebab-case -i.e. model_name
to model-name
.
Forecast using graphcast
model, with ECMWF IFS initial conditions, starting from 2024-04-30T00:00:00 and with a lead time of a week (forecast for the next week, i.e. 168 hours):
forecast --model_name graphcast --initial_conditions ifs --date 20240403 -output_dir s3://skyrim-dev --lead_time 168
or in modal:
modal run skyrim/modal/forecast.py --model-name graphcast --initial-conditions ifs --date 20240403 --output-dir s3://skyrim-dev --lead-time 168
Say you re interested in wind at 37.0344Β° N, 27.4305 E to see if we can kite tomorrow. If we need wind speed, we need to pull wind vectors at about surface level, these are u10m and v10m components of wind. Here is how you go about it:
modal run skyrim/modal/forecast.py --output-dir s3://[your_bucket]/[optional_path] --lead-time 24
Then you can read the forecast as below:
import xarray as xr
import pandas as pd
zarr_store_path = "s3://[your_bucket]/[forecast_id]"
forecast = xr.open_dataset(zarr_store_path, engine='zarr') # reads the metadata
df = forecast.sel(lat=37.0344, lon=27.4305, channel=['u10m', 'v10m']).to_pandas()
Normally each day is about 2GB but using zarr_store you will only fetch what you need.βοΈ
Assuming you have a local gpu set up ready to roll:
from skyrim.core import Skyrim
model = Skyrim("pangu")
final_pred, pred_paths = model.predict(
date="20240501", # format: YYYYMMDD, start date of the forecast
time="0000", # format: HHMM, start time of the forecast
lead_time=12, # in hours
save=True,
)
akyaka_coords = {"lat": 37.0557, "lon": 28.3242}
wind_speed = final_pred.wind_speed(**akyaka_coords) * 1.94384 # m/s to knots
print(f"Wind speed at Akyaka: {wind_speed:.2f} knots")
- NOAA GFS
- ECMWF IFS
- ERA5 Re-analysis Dataset
Currently supported models are:
- [x] Graphcast
- [x] Pangu
- [x] Fourcastnet (v1 & v2)
- [x] DLWP
- [x] (NWP) ECMWF IFS (HRES) -- notebook
- [x] (NWP) NOAA GFS -- notebook
- [x] (NWP) ECMWF ENS
- [ ] (NWP) ICON
- [ ] Aurora
- [X] FuXi
- [X] FengWu
- [ ] Nano MetNet
For detailed information regarding licensing, please refer to the license details provided on each model's main homepage, which we link to from each of the corresponding components within our repository.
- Pangu Weather : Original, ECMWF, NVIDIA
- FourcastNet : Original, ECMWF,NVIDIA
- Graphcast : Original, ECMWF, NVIDIA
- Fuxi: Original
- FengWu Original
- [x] ensemble prediction
- [x] interface to fetch real-time NWP-based predictions, e.g. via ECMWF API.
- [ ] global model performance comparison across various regions and parameters.
- [ ] finetuning api that trains a downstream model on top of features coming from a global/foundation model, that is optimized wrt to a specific criteria and region
- [ ] model quantization and its effect on model efficiency and accuracy.
This README will be updated regularly to reflect the progress and integration of new models or features into the library. It serves as a guide for internal development efforts and aids in prioritizing tasks and milestones.
All in here βοΈ
Skyrim is built on top of NVIDIA's earth2mip, earth2studio, and ECMWF's ai-models. Definitely check them out!
For Tasks:
Click tags to check more tools for each tasksFor Jobs:
Alternative AI tools for skyrim
Similar Open Source Tools
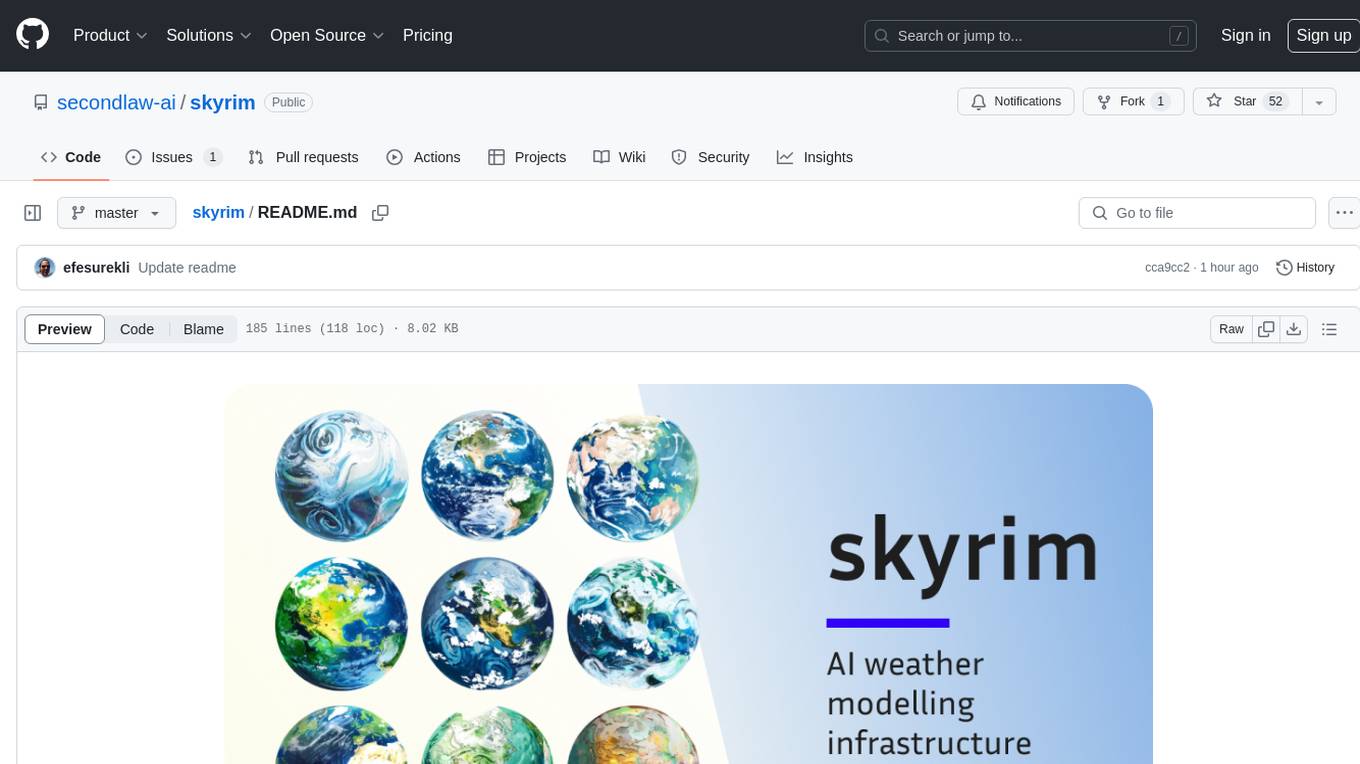
skyrim
Skyrim is a weather forecasting tool that enables users to run large weather models using consumer-grade GPUs. It provides access to state-of-the-art foundational weather models through a well-maintained infrastructure. Users can forecast weather conditions, such as wind speed and direction, by running simulations on their own GPUs or using modal volume or cloud services like s3 buckets. Skyrim supports various large weather models like Graphcast, Pangu, Fourcastnet, and DLWP, with plans for future enhancements like ensemble prediction and model quantization.
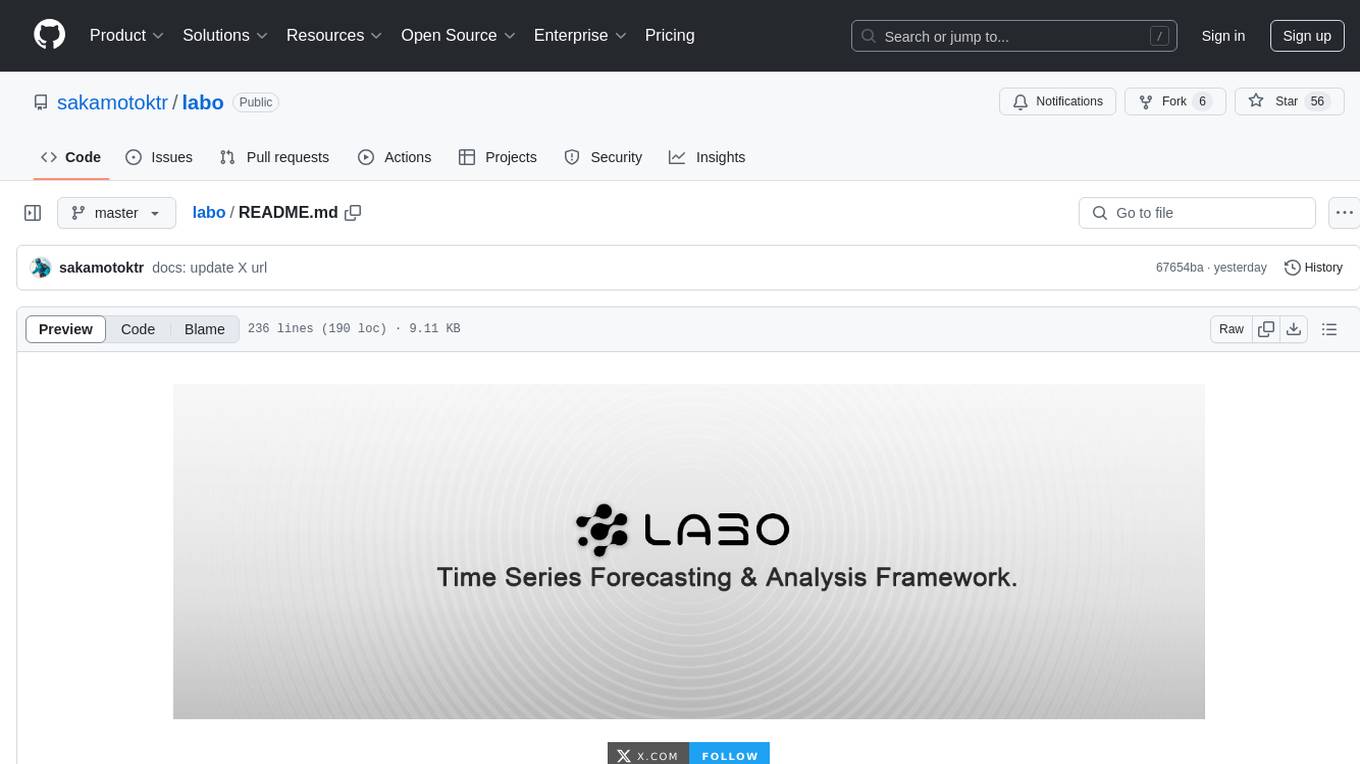
labo
LABO is a time series forecasting and analysis framework that integrates pre-trained and fine-tuned LLMs with multi-domain agent-based systems. It allows users to create and tune agents easily for various scenarios, such as stock market trend prediction and web public opinion analysis. LABO requires a specific runtime environment setup, including system requirements, Python environment, dependency installations, and configurations. Users can fine-tune their own models using LABO's Low-Rank Adaptation (LoRA) for computational efficiency and continuous model updates. Additionally, LABO provides a Python library for building model training pipelines and customizing agents for specific tasks.
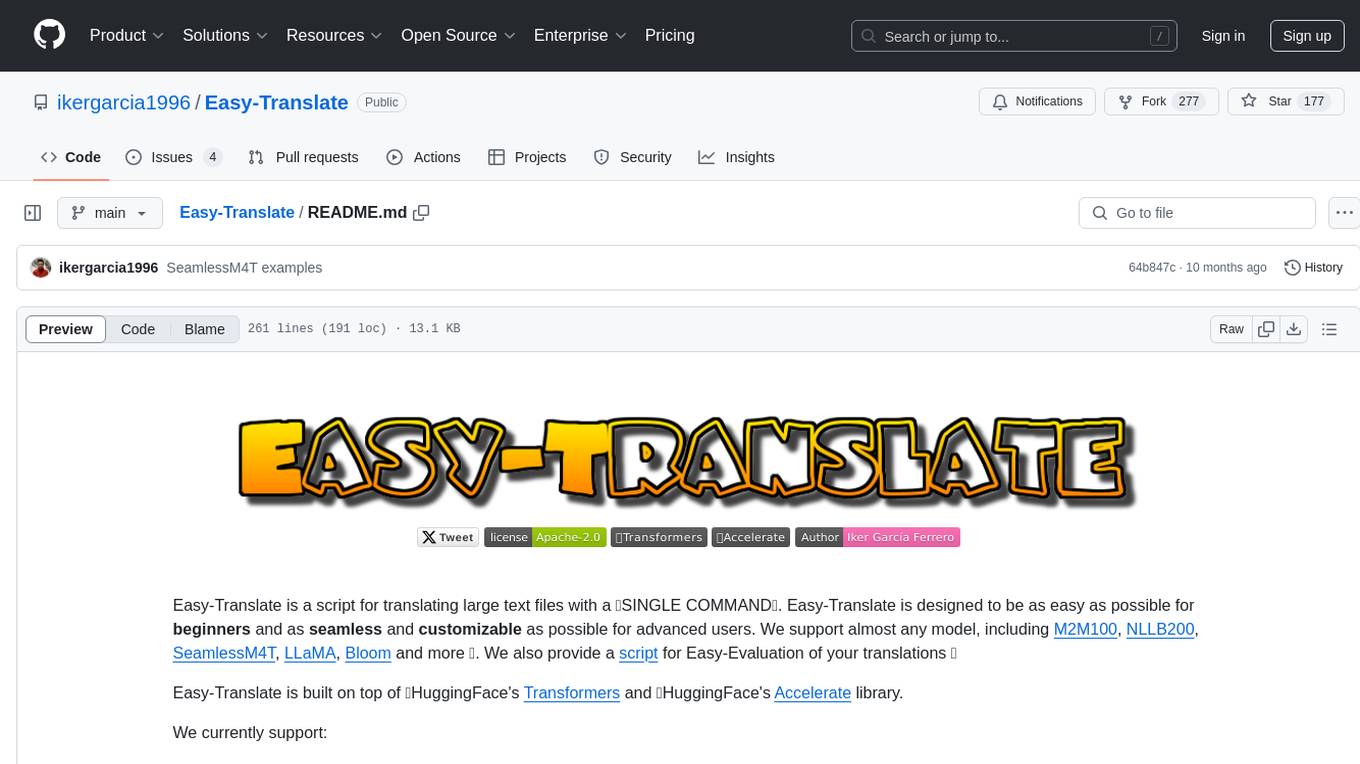
Easy-Translate
Easy-Translate is a script designed for translating large text files with a single command. It supports various models like M2M100, NLLB200, SeamlessM4T, LLaMA, and Bloom. The tool is beginner-friendly and offers seamless and customizable features for advanced users. It allows acceleration on CPU, multi-CPU, GPU, multi-GPU, and TPU, with support for different precisions and decoding strategies. Easy-Translate also provides an evaluation script for translations. Built on HuggingFace's Transformers and Accelerate library, it supports prompt usage and loading huge models efficiently.
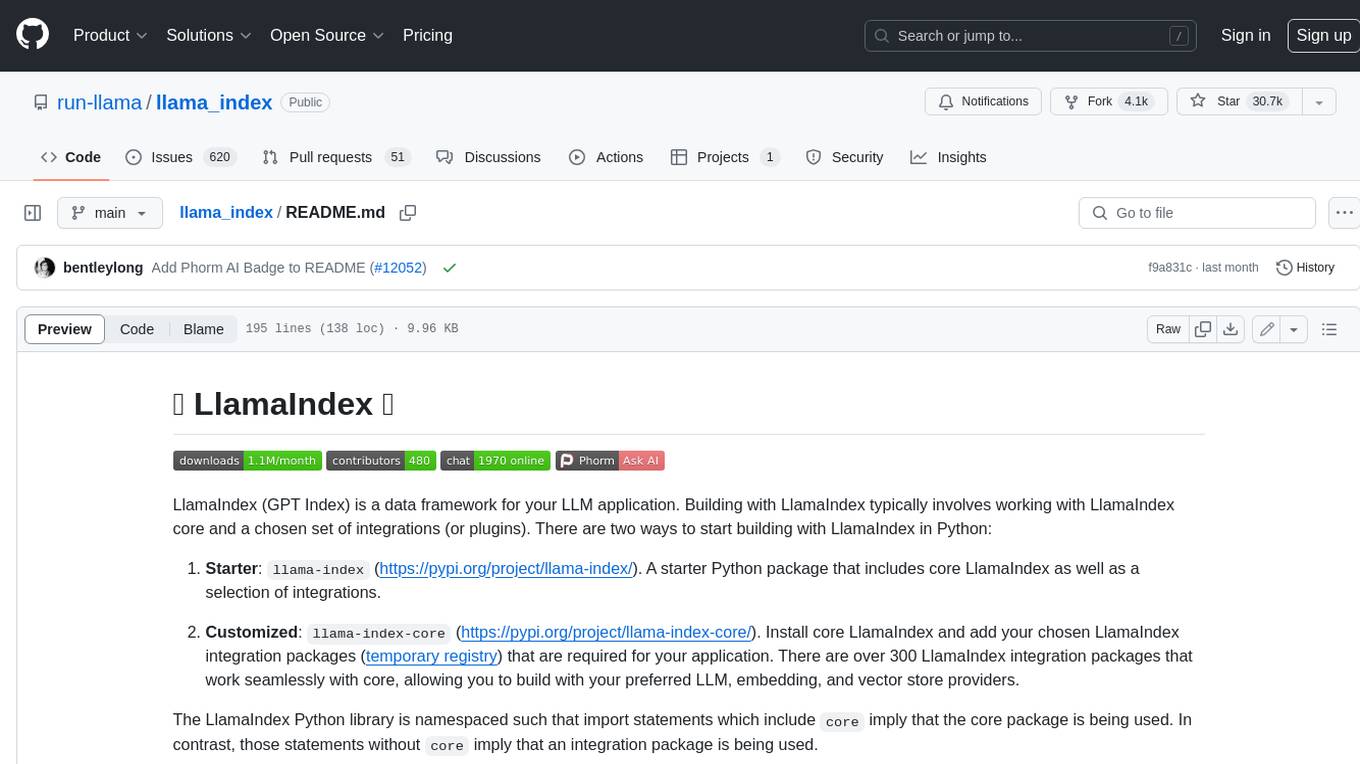
llama_index
LlamaIndex is a data framework for building LLM applications. It provides tools for ingesting, structuring, and querying data, as well as integrating with LLMs and other tools. LlamaIndex is designed to be easy to use for both beginner and advanced users, and it provides a comprehensive set of features for building LLM applications.
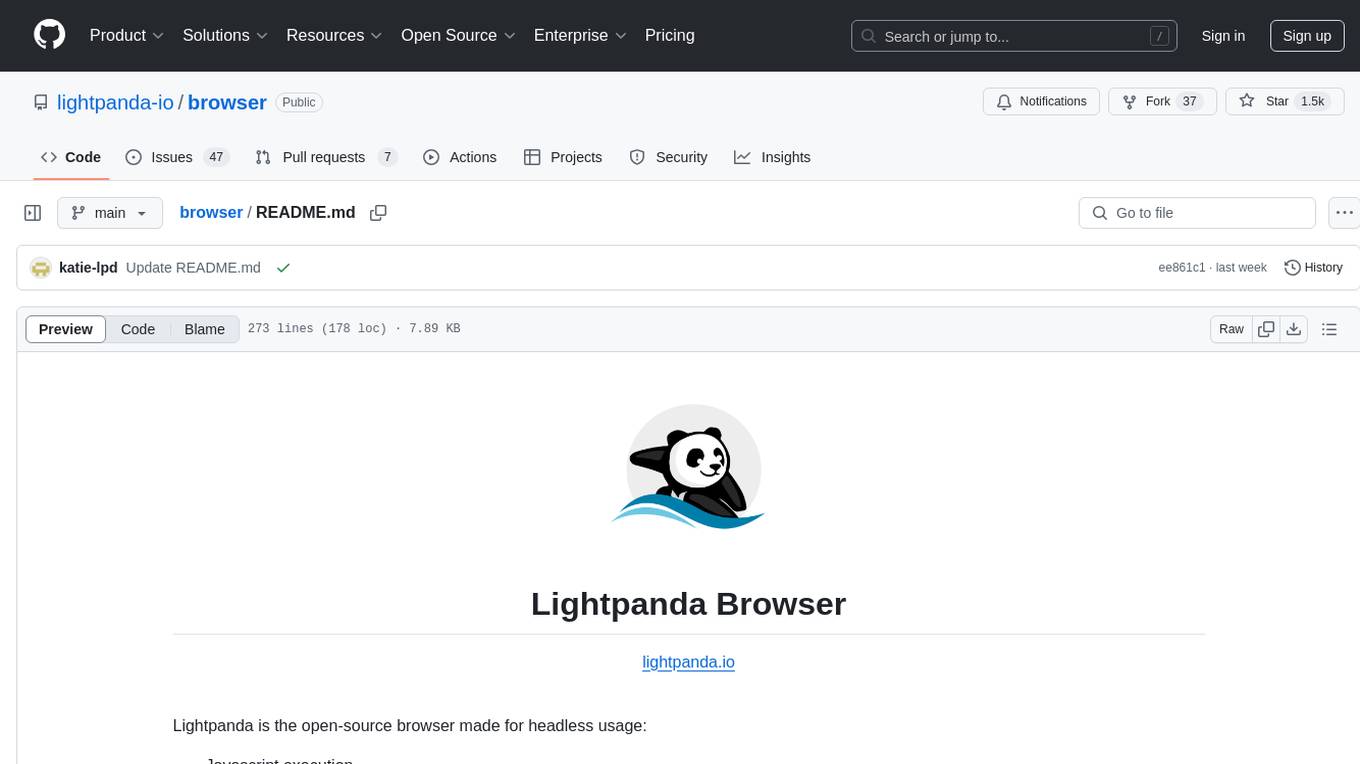
browser
Lightpanda Browser is an open-source headless browser designed for fast web automation, AI agents, LLM training, scraping, and testing. It features ultra-low memory footprint, exceptionally fast execution, and compatibility with Playwright and Puppeteer through CDP. Built for performance, Lightpanda offers Javascript execution, support for Web APIs, and is optimized for minimal memory usage. It is a modern solution for web scraping and automation tasks, providing a lightweight alternative to traditional browsers like Chrome.
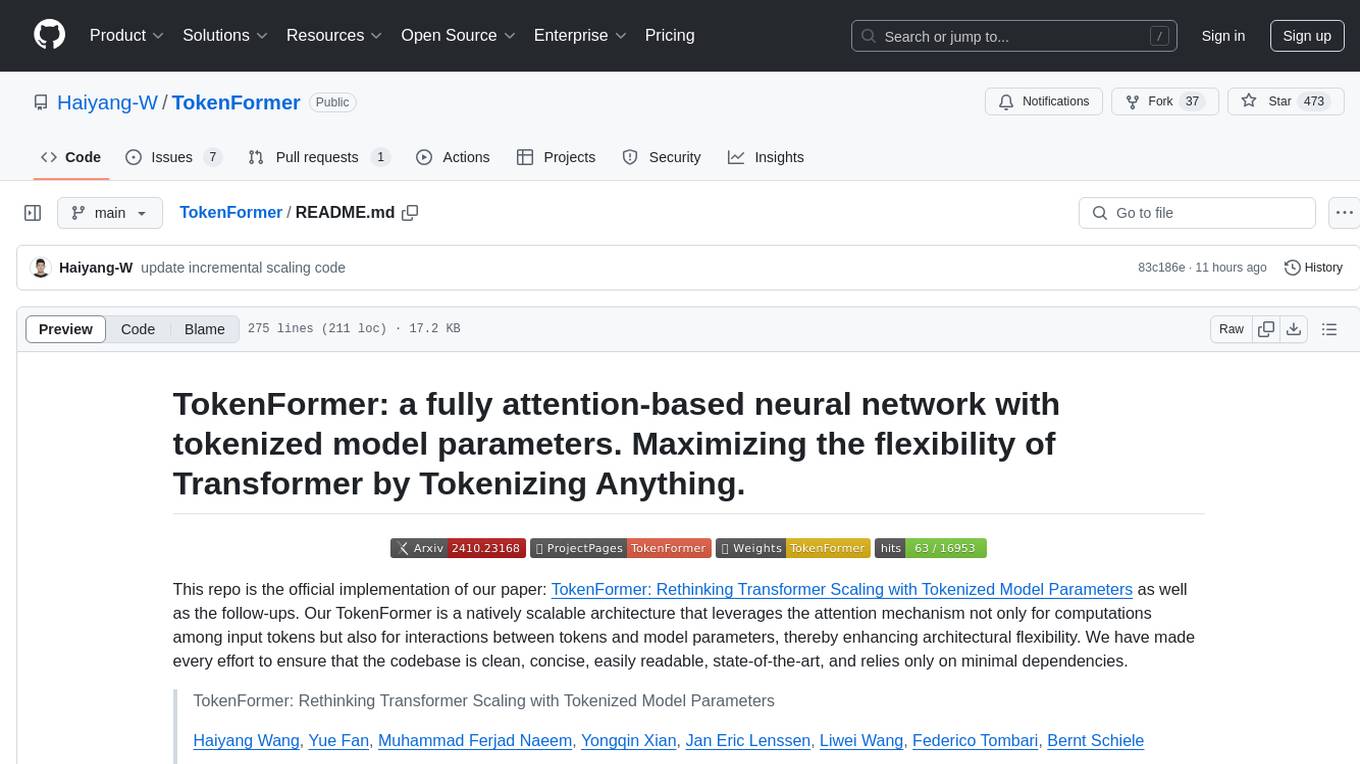
TokenFormer
TokenFormer is a fully attention-based neural network architecture that leverages tokenized model parameters to enhance architectural flexibility. It aims to maximize the flexibility of neural networks by unifying token-token and token-parameter interactions through the attention mechanism. The architecture allows for incremental model scaling and has shown promising results in language modeling and visual modeling tasks. The codebase is clean, concise, easily readable, state-of-the-art, and relies on minimal dependencies.
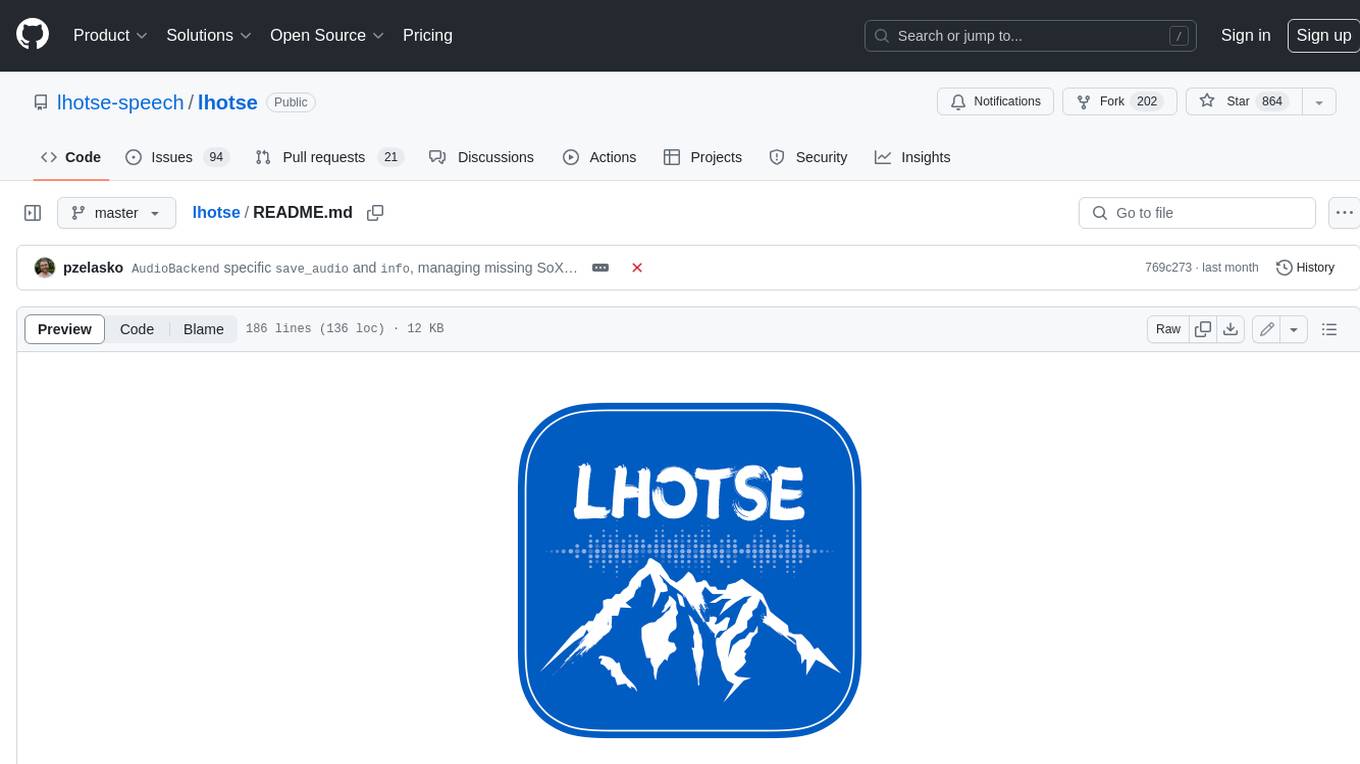
lhotse
Lhotse is a Python library designed to make speech and audio data preparation flexible and accessible. It aims to attract a wider community to speech processing tasks by providing a Python-centric design and an expressive command-line interface. Lhotse offers standard data preparation recipes, PyTorch Dataset classes for speech tasks, and efficient data preparation for model training with audio cuts. It supports data augmentation, feature extraction, and feature-space cut mixing. The tool extends Kaldi's data preparation recipes with seamless PyTorch integration, human-readable text manifests, and convenient Python classes.
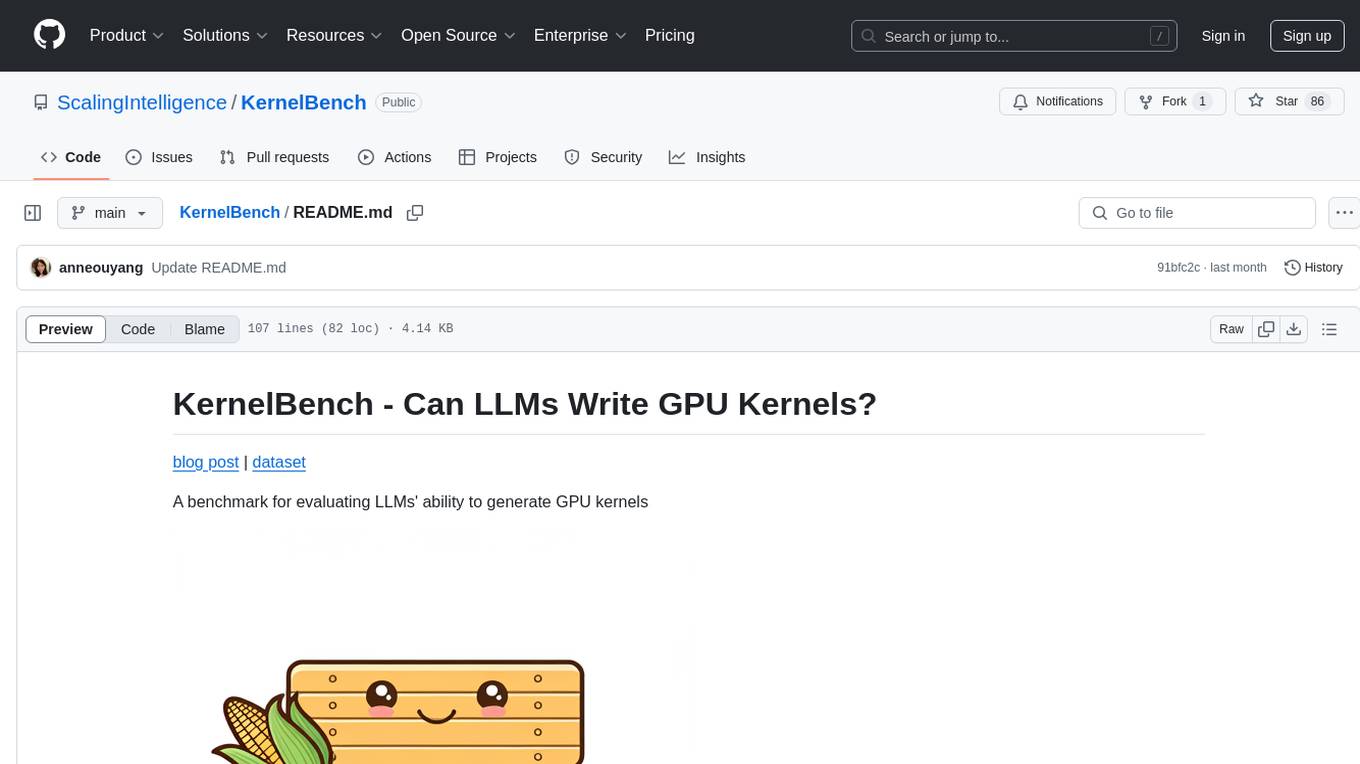
KernelBench
KernelBench is a benchmark tool designed to evaluate Large Language Models' (LLMs) ability to generate GPU kernels. It focuses on transpiling operators from PyTorch to CUDA kernels at different levels of granularity. The tool categorizes problems into four levels, ranging from single-kernel operators to full model architectures, and assesses solutions based on compilation, correctness, and speed. The repository provides a structured directory layout, setup instructions, usage examples for running single or multiple problems, and upcoming roadmap features like additional GPU platform support and integration with other frameworks.
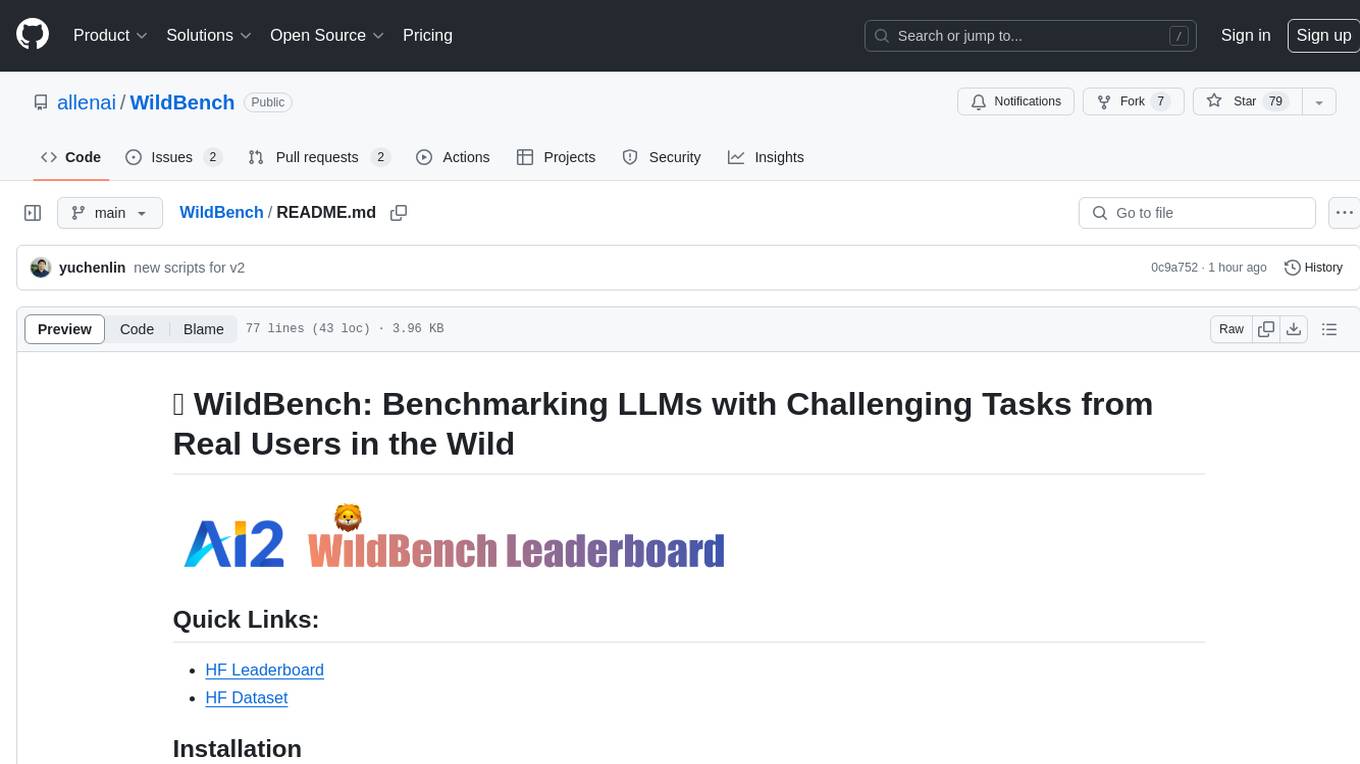
WildBench
WildBench is a tool designed for benchmarking Large Language Models (LLMs) with challenging tasks sourced from real users in the wild. It provides a platform for evaluating the performance of various models on a range of tasks. Users can easily add new models to the benchmark by following the provided guidelines. The tool supports models from Hugging Face and other APIs, allowing for comprehensive evaluation and comparison. WildBench facilitates running inference and evaluation scripts, enabling users to contribute to the benchmark and collaborate on improving model performance.
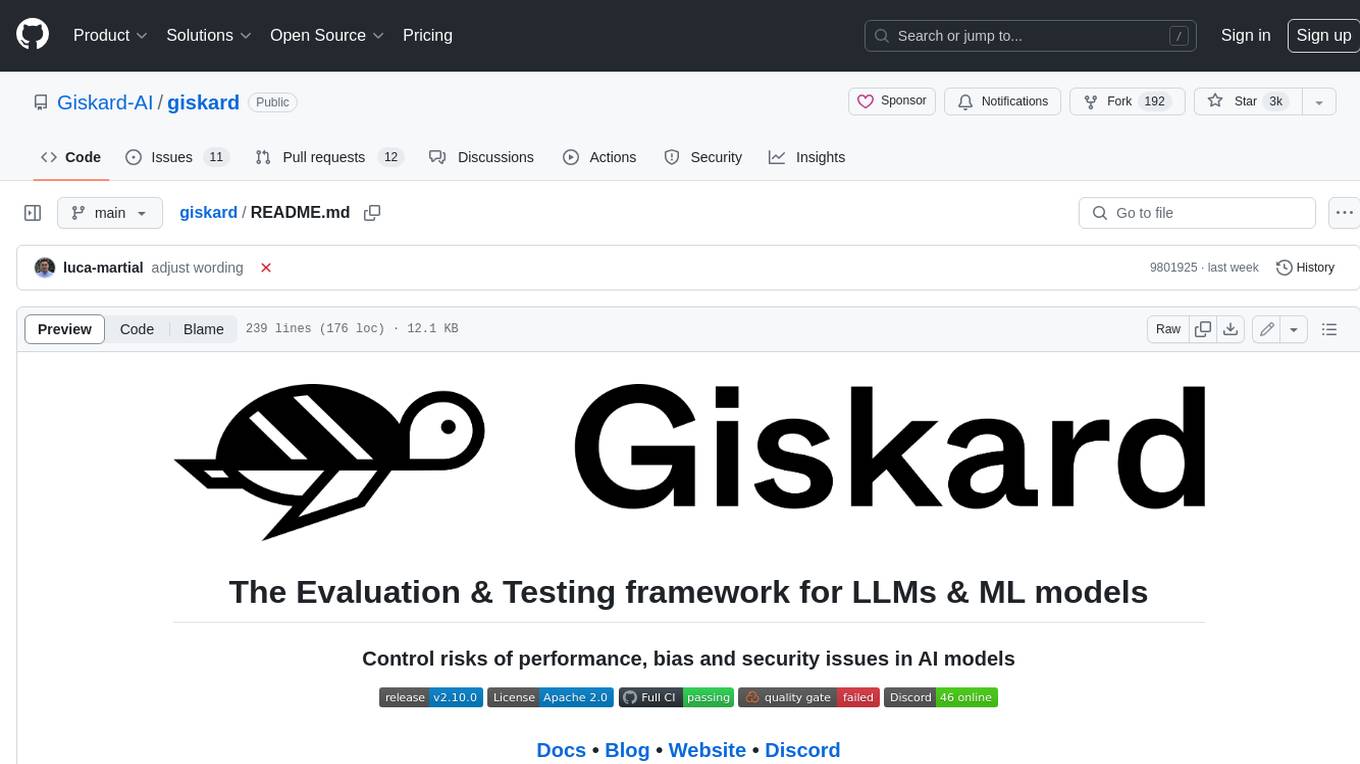
giskard
Giskard is an open-source Python library that automatically detects performance, bias & security issues in AI applications. The library covers LLM-based applications such as RAG agents, all the way to traditional ML models for tabular data.
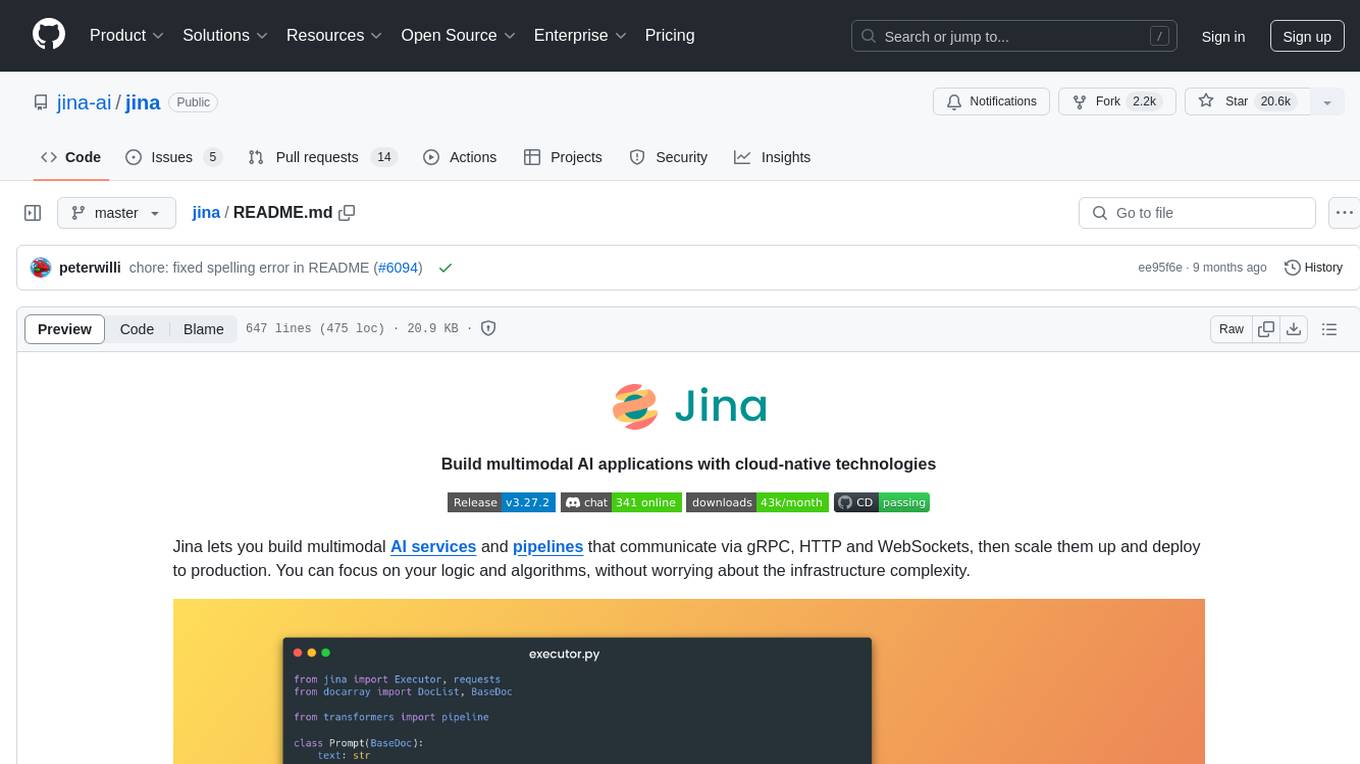
jina
Jina is a tool that allows users to build multimodal AI services and pipelines using cloud-native technologies. It provides a Pythonic experience for serving ML models and transitioning from local deployment to advanced orchestration frameworks like Docker-Compose, Kubernetes, or Jina AI Cloud. Users can build and serve models for any data type and deep learning framework, design high-performance services with easy scaling, serve LLM models while streaming their output, integrate with Docker containers via Executor Hub, and host on CPU/GPU using Jina AI Cloud. Jina also offers advanced orchestration and scaling capabilities, a smooth transition to the cloud, and easy scalability and concurrency features for applications. Users can deploy to their own cloud or system with Kubernetes and Docker Compose integration, and even deploy to JCloud for autoscaling and monitoring.
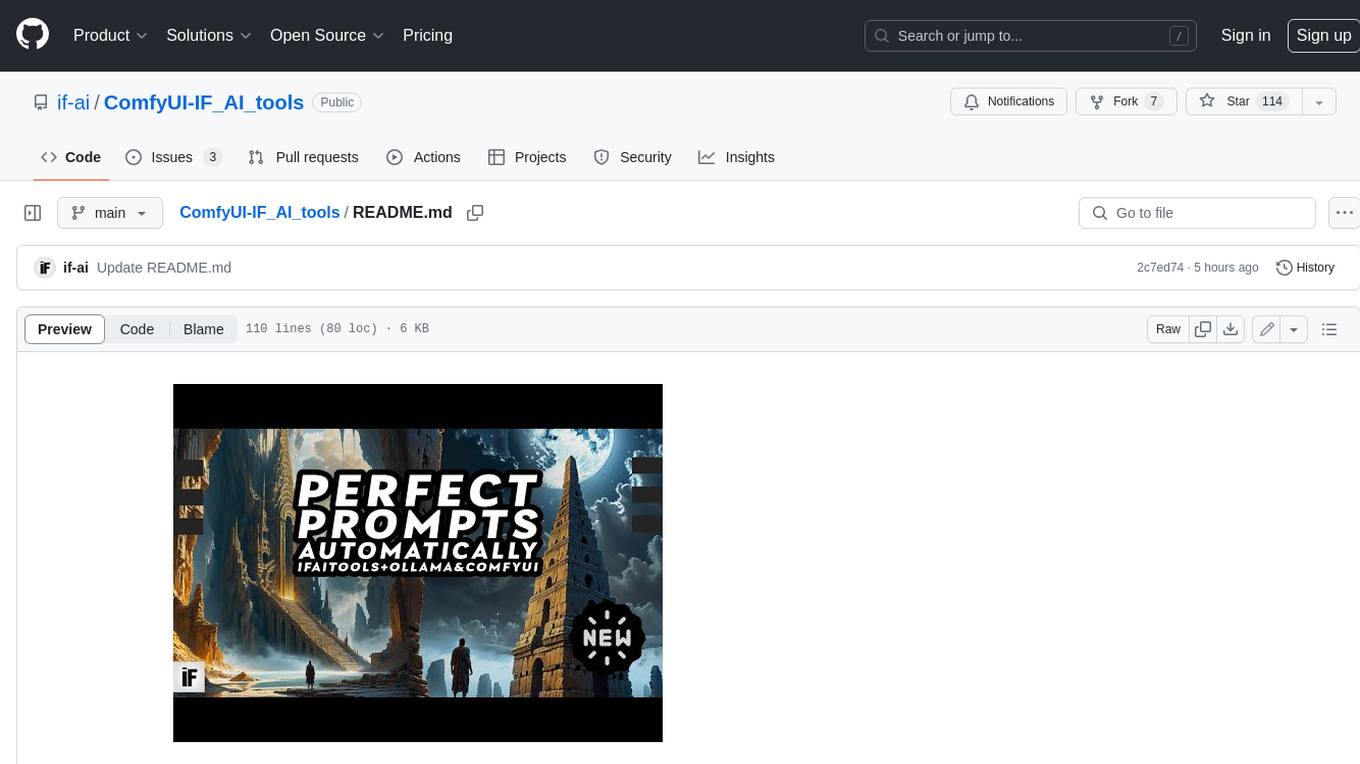
ComfyUI-IF_AI_tools
ComfyUI-IF_AI_tools is a set of custom nodes for ComfyUI that allows you to generate prompts using a local Large Language Model (LLM) via Ollama. This tool enables you to enhance your image generation workflow by leveraging the power of language models.
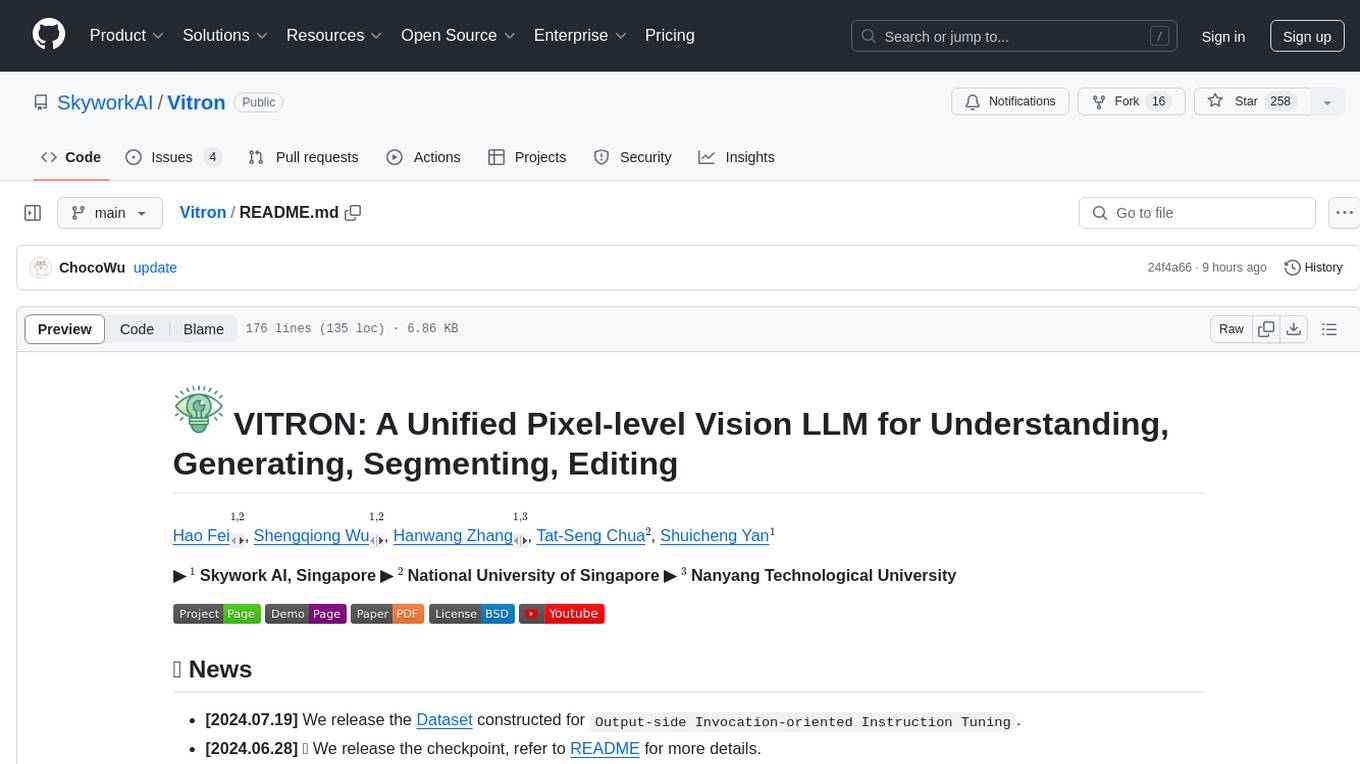
Vitron
Vitron is a unified pixel-level vision LLM designed for comprehensive understanding, generating, segmenting, and editing static images and dynamic videos. It addresses challenges in existing vision LLMs such as superficial instance-level understanding, lack of unified support for images and videos, and insufficient coverage across various vision tasks. The tool requires Python >= 3.8, Pytorch == 2.1.0, and CUDA Version >= 11.8 for installation. Users can deploy Gradio demo locally and fine-tune their models for specific tasks.
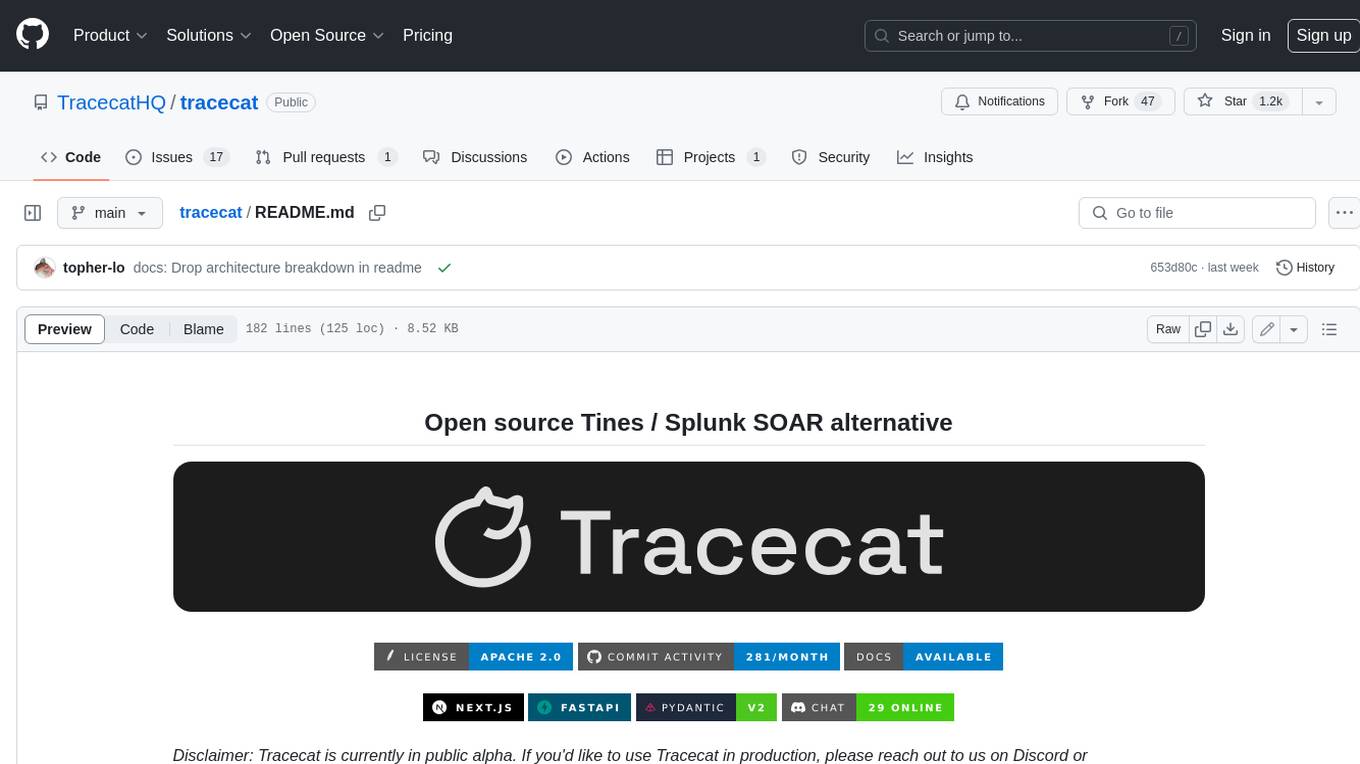
tracecat
Tracecat is an open-source automation platform for security teams. It's designed to be simple but powerful, with a focus on AI features and a practitioner-obsessed UI/UX. Tracecat can be used to automate a variety of tasks, including phishing email investigation, evidence collection, and remediation plan generation.
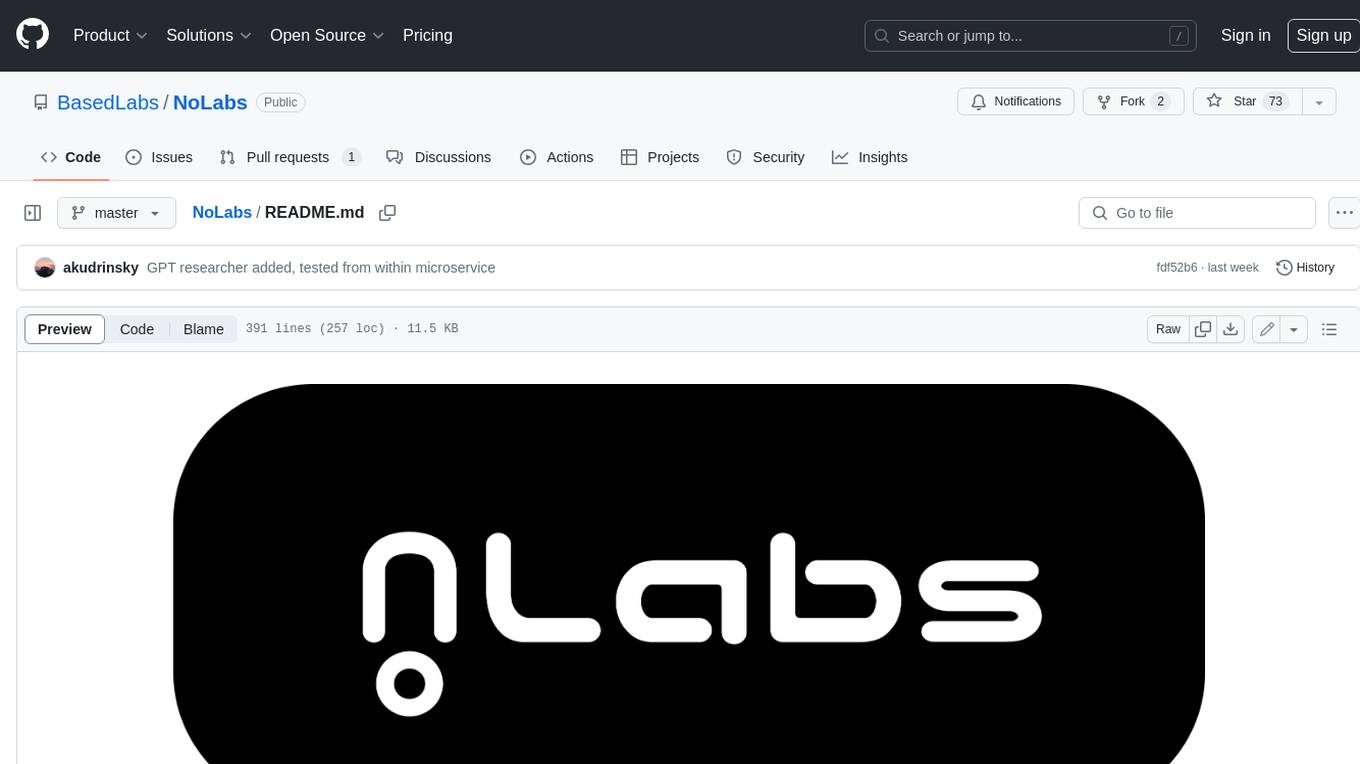
NoLabs
NoLabs is an open-source biolab that provides easy access to state-of-the-art models for bio research. It supports various tasks, including drug discovery, protein analysis, and small molecule design. NoLabs aims to accelerate bio research by making inference models accessible to everyone.
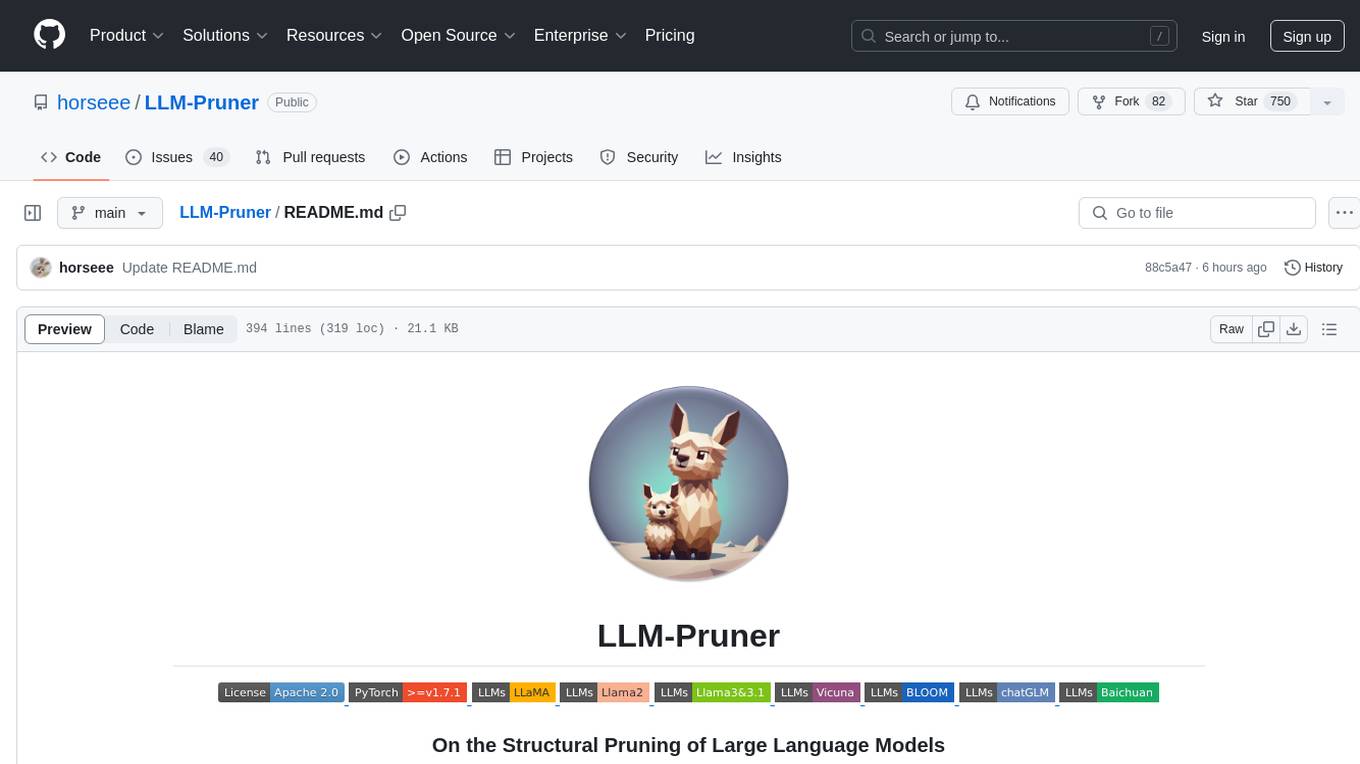
LLM-Pruner
LLM-Pruner is a tool for structural pruning of large language models, allowing task-agnostic compression while retaining multi-task solving ability. It supports automatic structural pruning of various LLMs with minimal human effort. The tool is efficient, requiring only 3 minutes for pruning and 3 hours for post-training. Supported LLMs include Llama-3.1, Llama-3, Llama-2, LLaMA, BLOOM, Vicuna, and Baichuan. Updates include support for new LLMs like GQA and BLOOM, as well as fine-tuning results achieving high accuracy. The tool provides step-by-step instructions for pruning, post-training, and evaluation, along with a Gradio interface for text generation. Limitations include issues with generating repetitive or nonsensical tokens in compressed models and manual operations for certain models.
For similar tasks
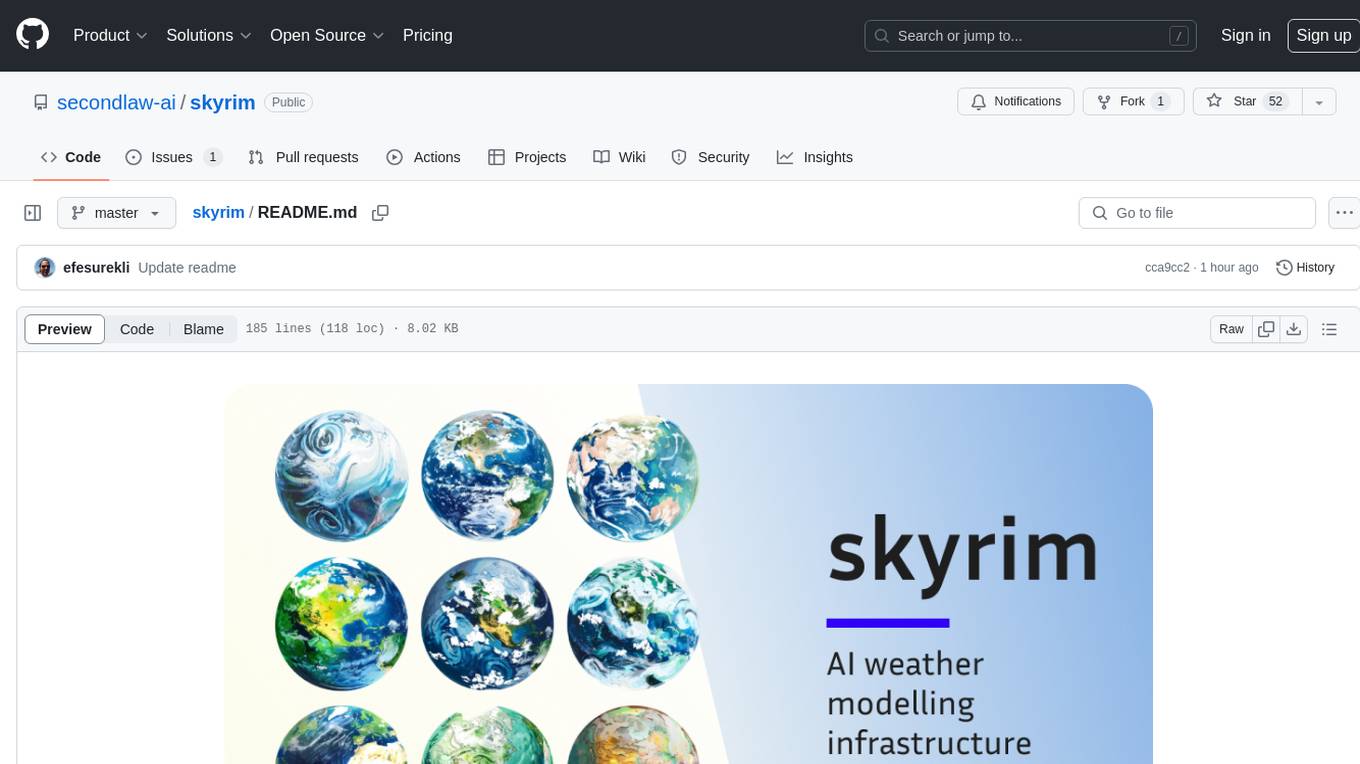
skyrim
Skyrim is a weather forecasting tool that enables users to run large weather models using consumer-grade GPUs. It provides access to state-of-the-art foundational weather models through a well-maintained infrastructure. Users can forecast weather conditions, such as wind speed and direction, by running simulations on their own GPUs or using modal volume or cloud services like s3 buckets. Skyrim supports various large weather models like Graphcast, Pangu, Fourcastnet, and DLWP, with plans for future enhancements like ensemble prediction and model quantization.
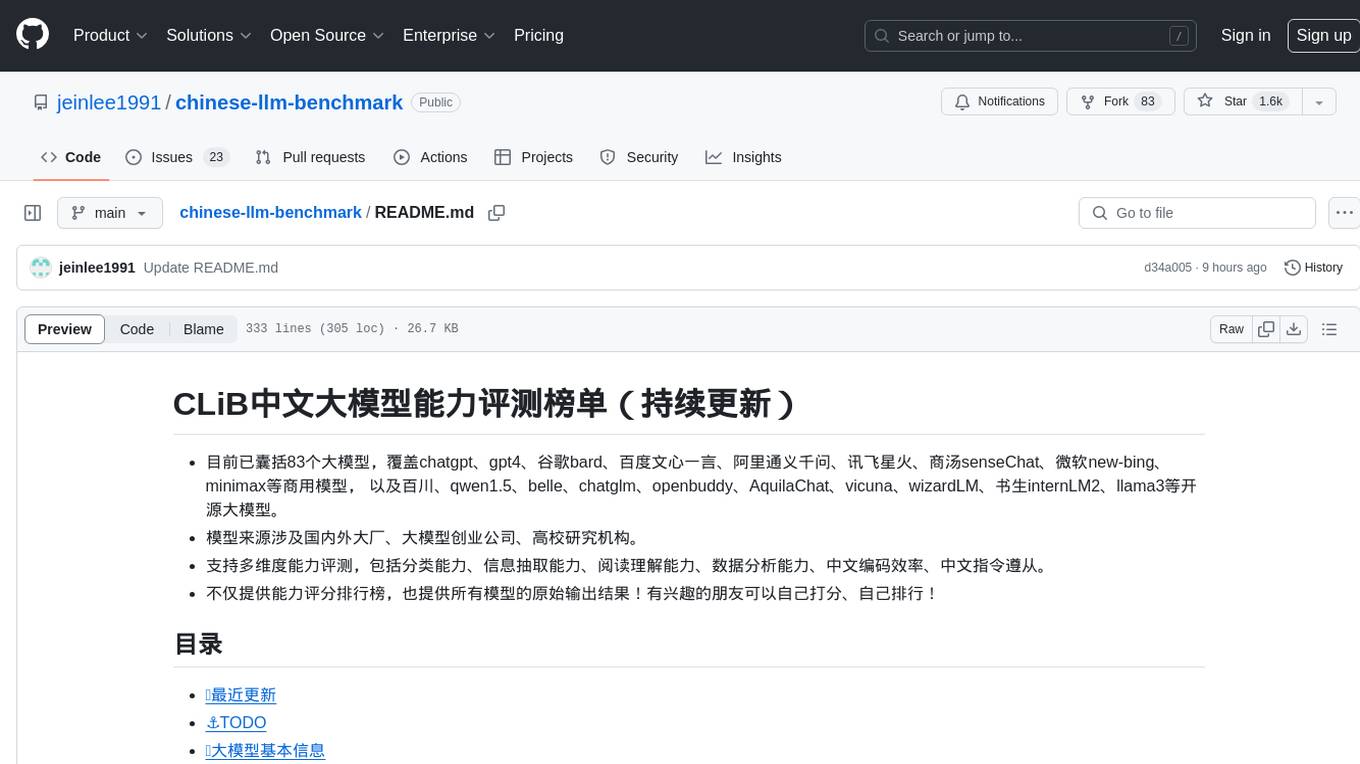
chinese-llm-benchmark
The Chinese LLM Benchmark is a continuous evaluation list of large models in CLiB, covering a wide range of commercial and open-source models from various companies and research institutions. It supports multidimensional evaluation of capabilities including classification, information extraction, reading comprehension, data analysis, Chinese encoding efficiency, and Chinese instruction compliance. The benchmark not only provides capability score rankings but also offers the original output results of all models for interested individuals to score and rank themselves.
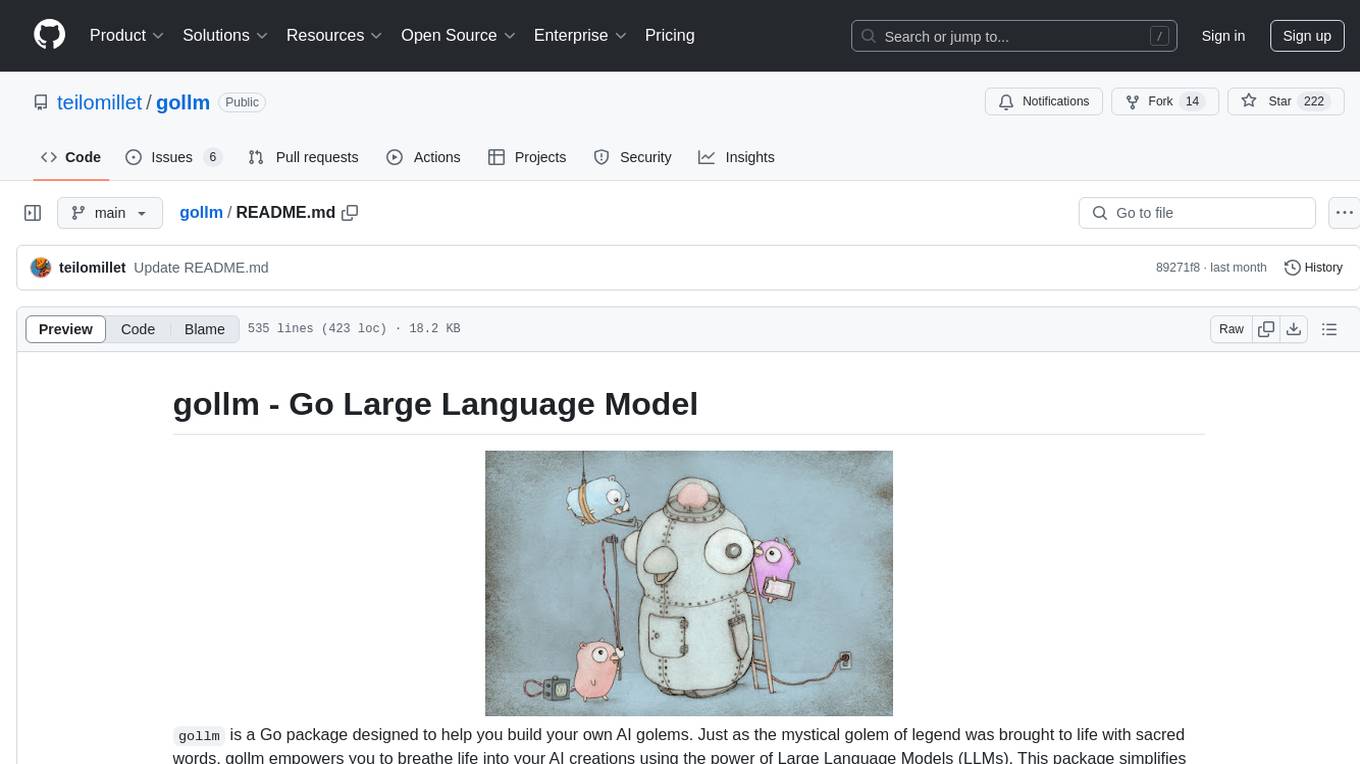
gollm
gollm is a Go package designed to simplify interactions with Large Language Models (LLMs) for AI engineers and developers. It offers a unified API for multiple LLM providers, easy provider and model switching, flexible configuration options, advanced prompt engineering, prompt optimization, memory retention, structured output and validation, provider comparison tools, high-level AI functions, robust error handling and retries, and extensible architecture. The package enables users to create AI-powered golems for tasks like content creation workflows, complex reasoning tasks, structured data generation, model performance analysis, prompt optimization, and creating a mixture of agents.
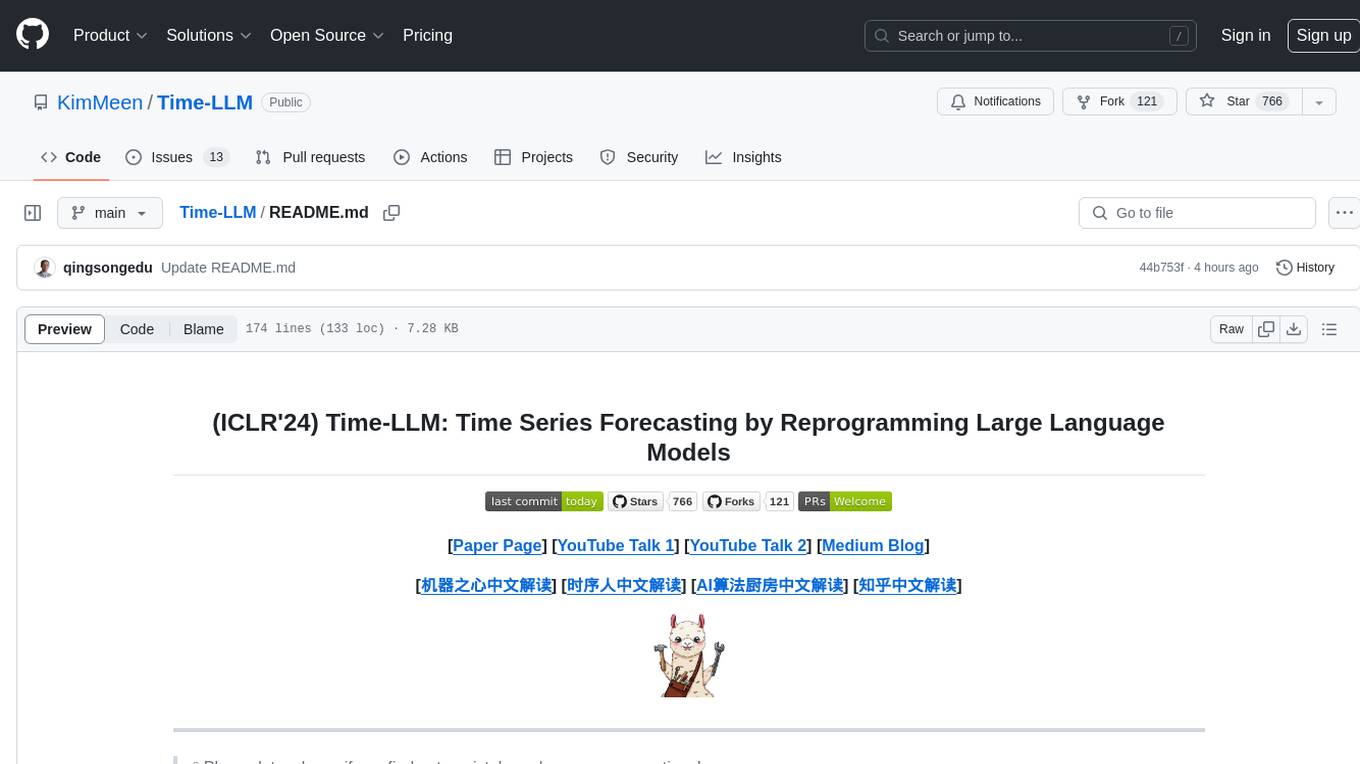
Time-LLM
Time-LLM is a reprogramming framework that repurposes large language models (LLMs) for time series forecasting. It allows users to treat time series analysis as a 'language task' and effectively leverage pre-trained LLMs for forecasting. The framework involves reprogramming time series data into text representations and providing declarative prompts to guide the LLM reasoning process. Time-LLM supports various backbone models such as Llama-7B, GPT-2, and BERT, offering flexibility in model selection. The tool provides a general framework for repurposing language models for time series forecasting tasks.
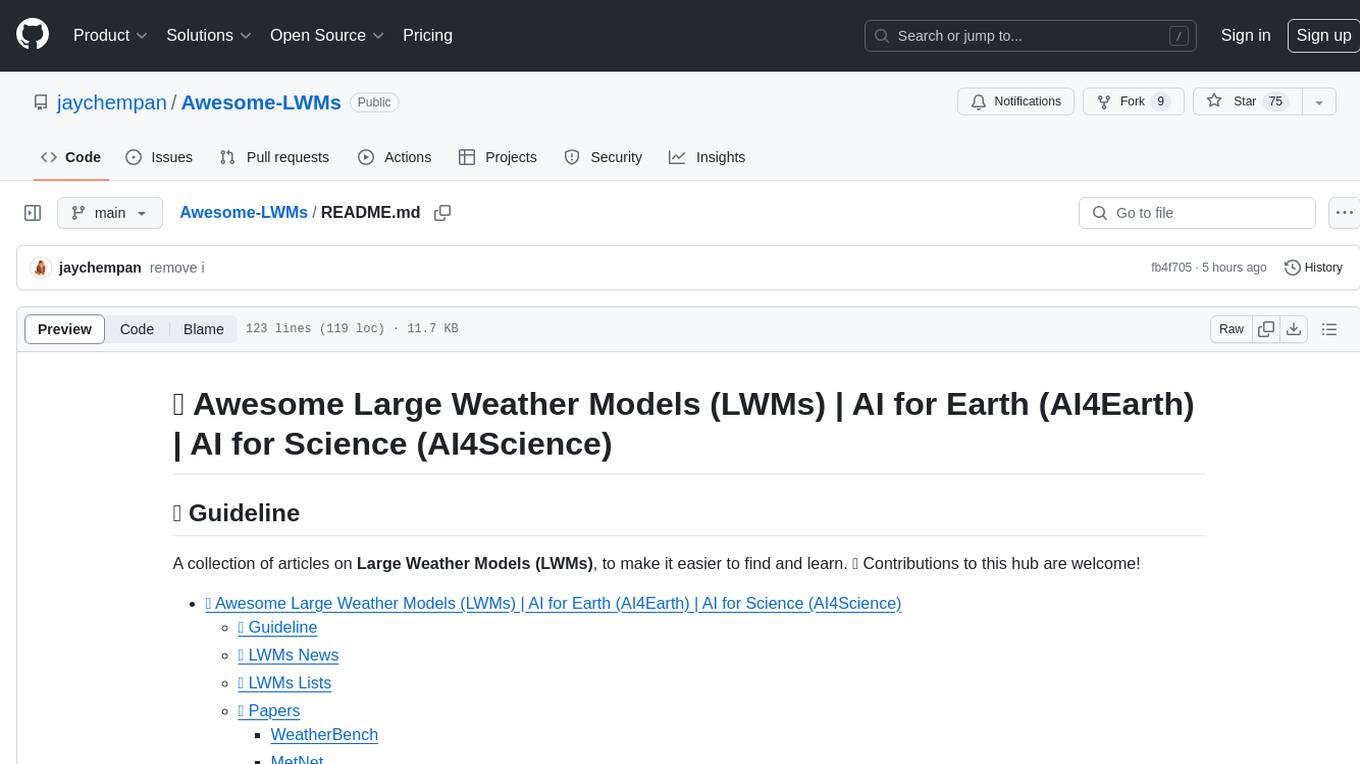
Awesome-LWMs
Awesome Large Weather Models (LWMs) is a curated collection of articles and resources related to large weather models used in AI for Earth and AI for Science. It includes information on various cutting-edge weather forecasting models, benchmark datasets, and research papers. The repository serves as a hub for researchers and enthusiasts to explore the latest advancements in weather modeling and forecasting.
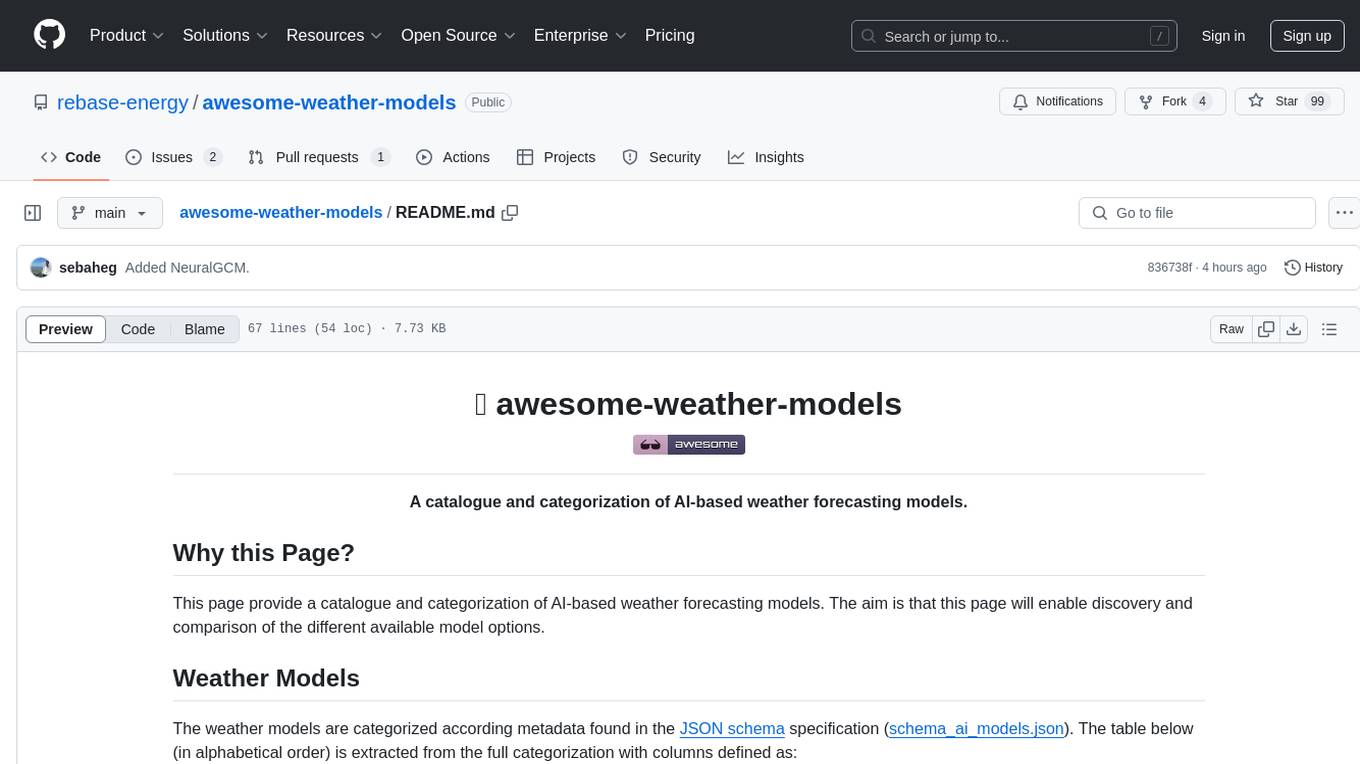
awesome-weather-models
A catalogue and categorization of AI-based weather forecasting models. This page provides a catalogue and categorization of AI-based weather forecasting models to enable discovery and comparison of different available model options. The weather models are categorized based on metadata found in the JSON schema specification. The table includes information such as the name of the weather model, the organization that developed it, operational data availability, open-source status, and links for further details.
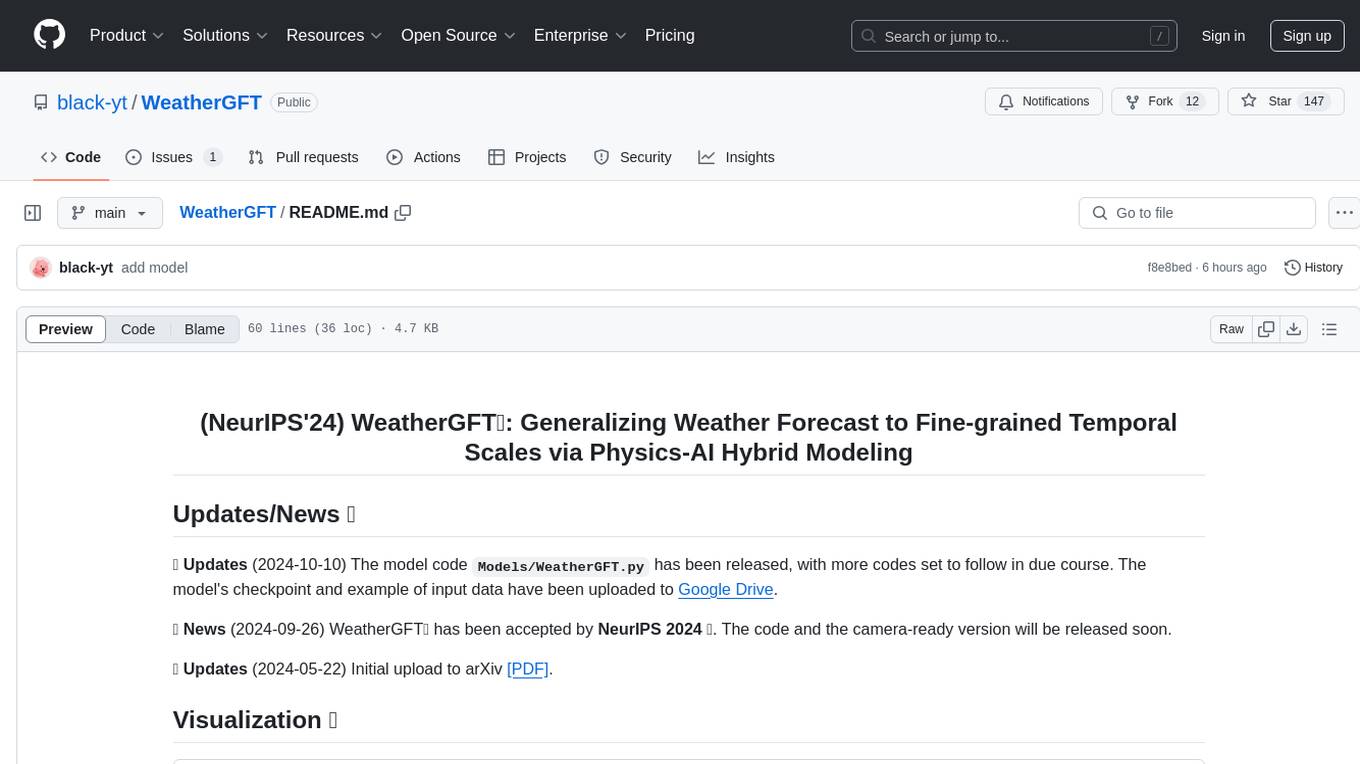
WeatherGFT
WeatherGFT is a physics-AI hybrid model designed to generalize weather forecasts to finer-grained temporal scales beyond the training dataset. It incorporates physical partial differential equations (PDEs) into neural networks to simulate fine-grained physical evolution and correct biases. The model achieves state-of-the-art performance in forecasting tasks at different time scales, from nowcasting to medium-range forecasts, by utilizing a lead time-aware training framework and a carefully designed PDE kernel. WeatherGFT bridges the gap between nowcast and medium-range forecast by extending forecasting abilities to predict accurately at a 30-minute time scale.
For similar jobs
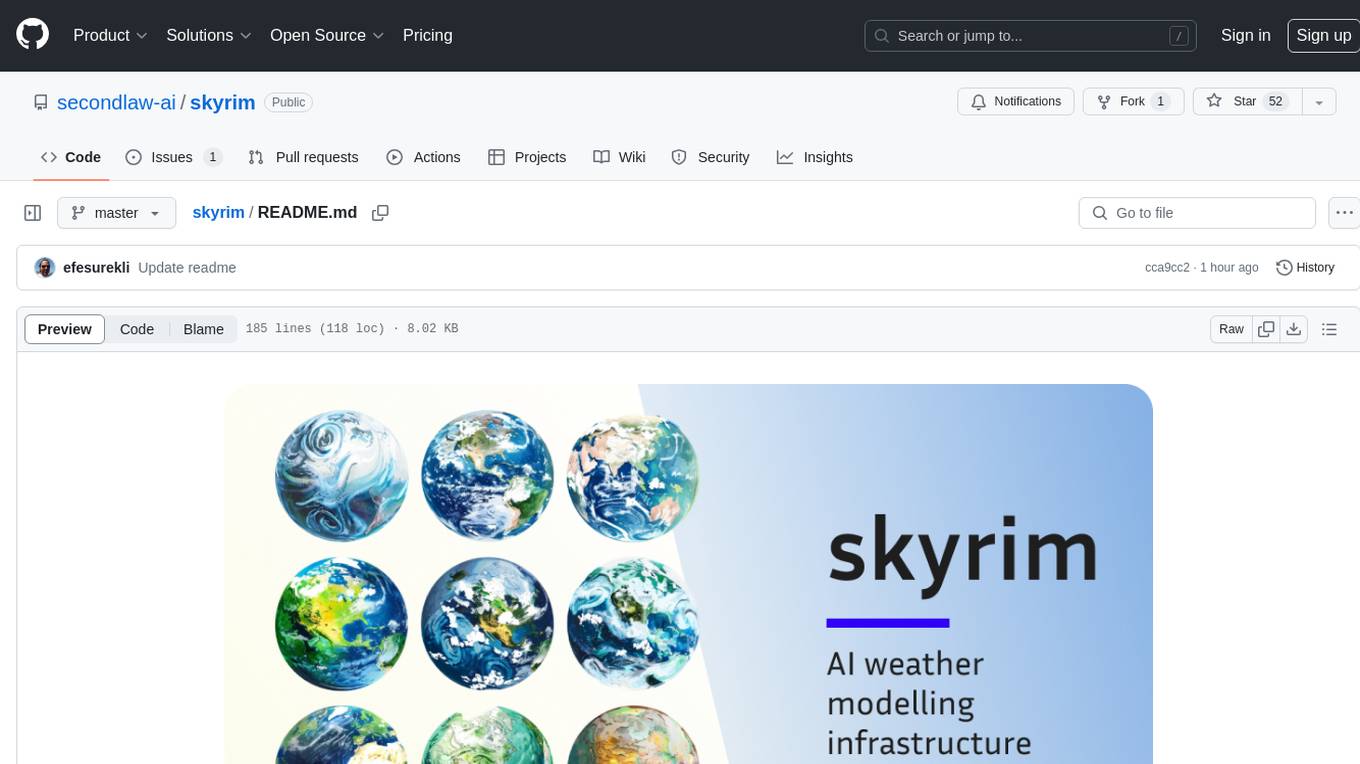
skyrim
Skyrim is a weather forecasting tool that enables users to run large weather models using consumer-grade GPUs. It provides access to state-of-the-art foundational weather models through a well-maintained infrastructure. Users can forecast weather conditions, such as wind speed and direction, by running simulations on their own GPUs or using modal volume or cloud services like s3 buckets. Skyrim supports various large weather models like Graphcast, Pangu, Fourcastnet, and DLWP, with plans for future enhancements like ensemble prediction and model quantization.
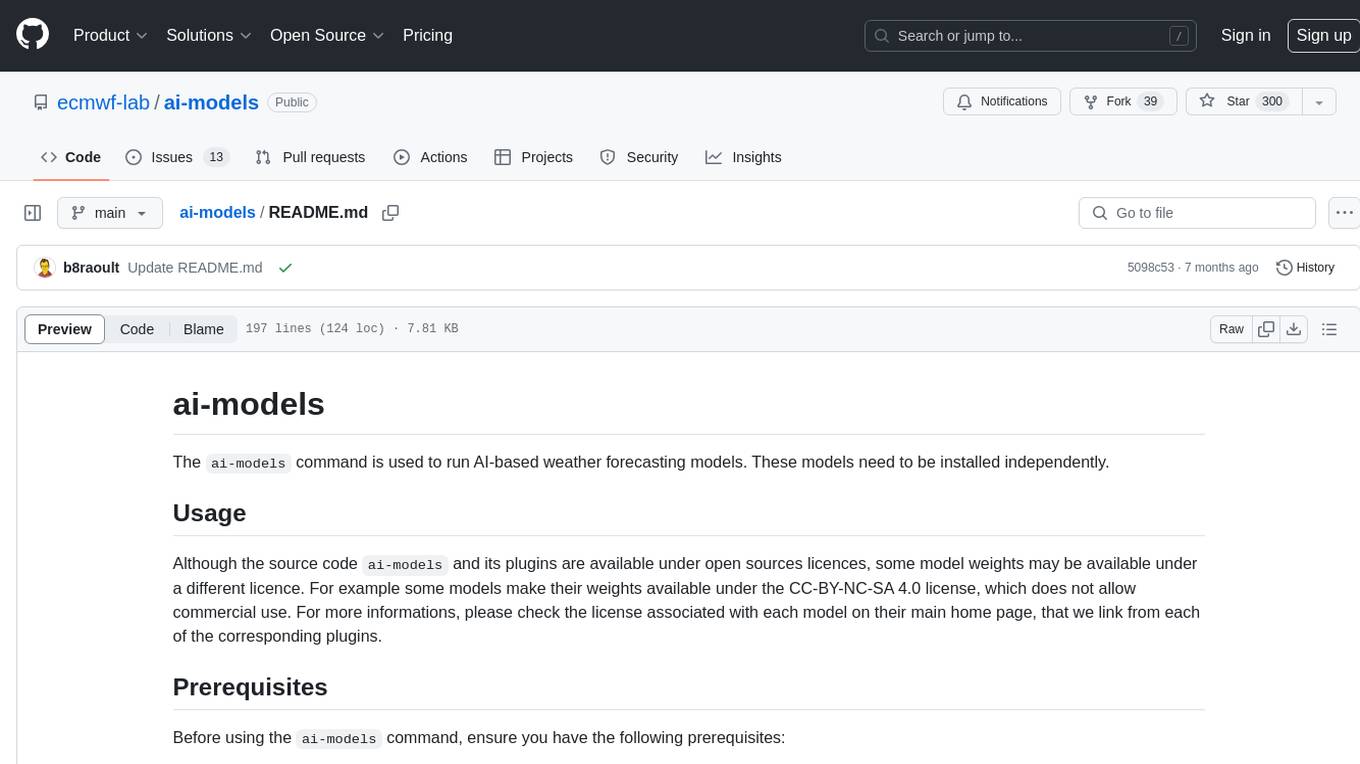
ai-models
The `ai-models` command is a tool used to run AI-based weather forecasting models. It provides functionalities to install, run, and manage different AI models for weather forecasting. Users can easily install and run various models, customize model settings, download assets, and manage input data from different sources such as ECMWF, CDS, and GRIB files. The tool is designed to optimize performance by running on GPUs and provides options for better organization of assets and output files. It offers a range of command line options for users to interact with the models and customize their forecasting tasks.
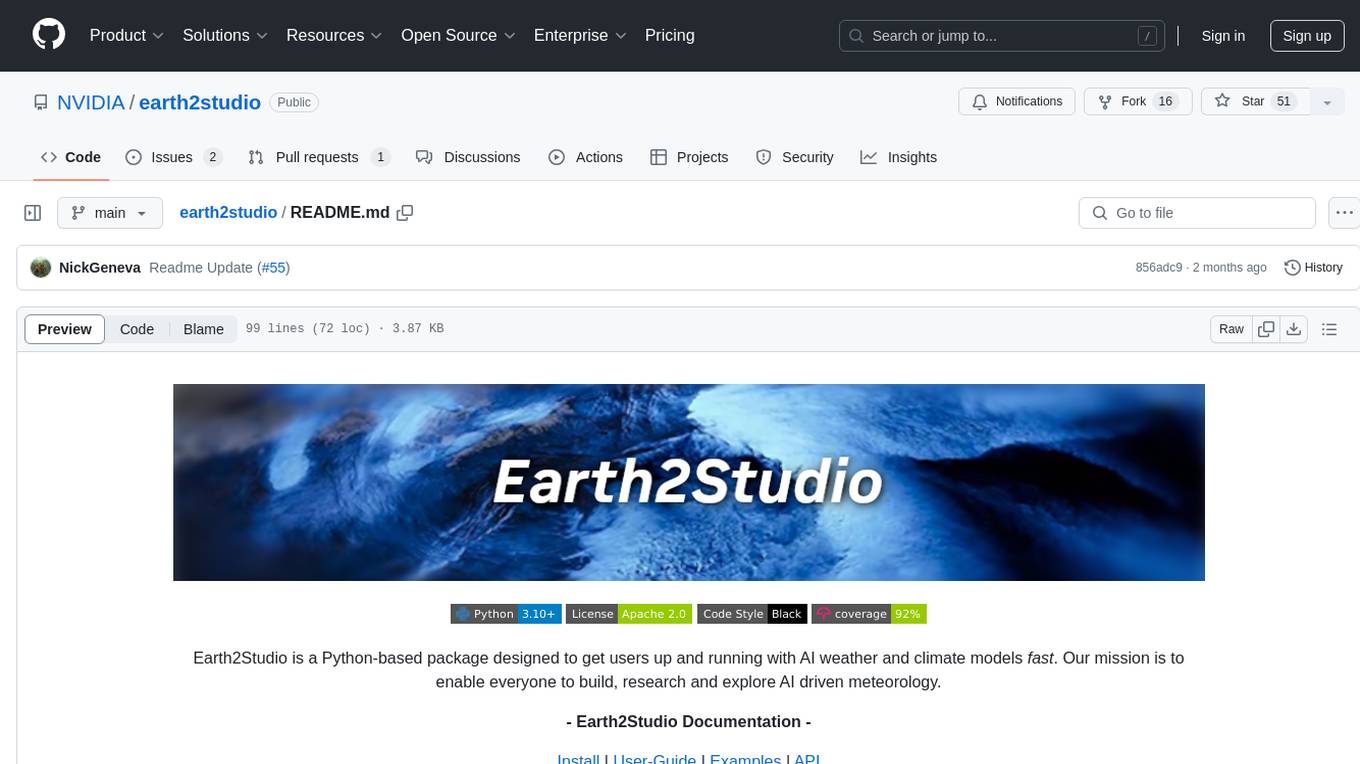
earth2studio
Earth2Studio is a Python-based package designed to enable users to quickly get started with AI weather and climate models. It provides access to pre-trained models, diagnostic tools, data sources, IO utilities, perturbation methods, and sample workflows for building custom weather prediction workflows. The package aims to empower users to explore AI-driven meteorology through modular components and seamless integration with other Nvidia packages like Modulus.
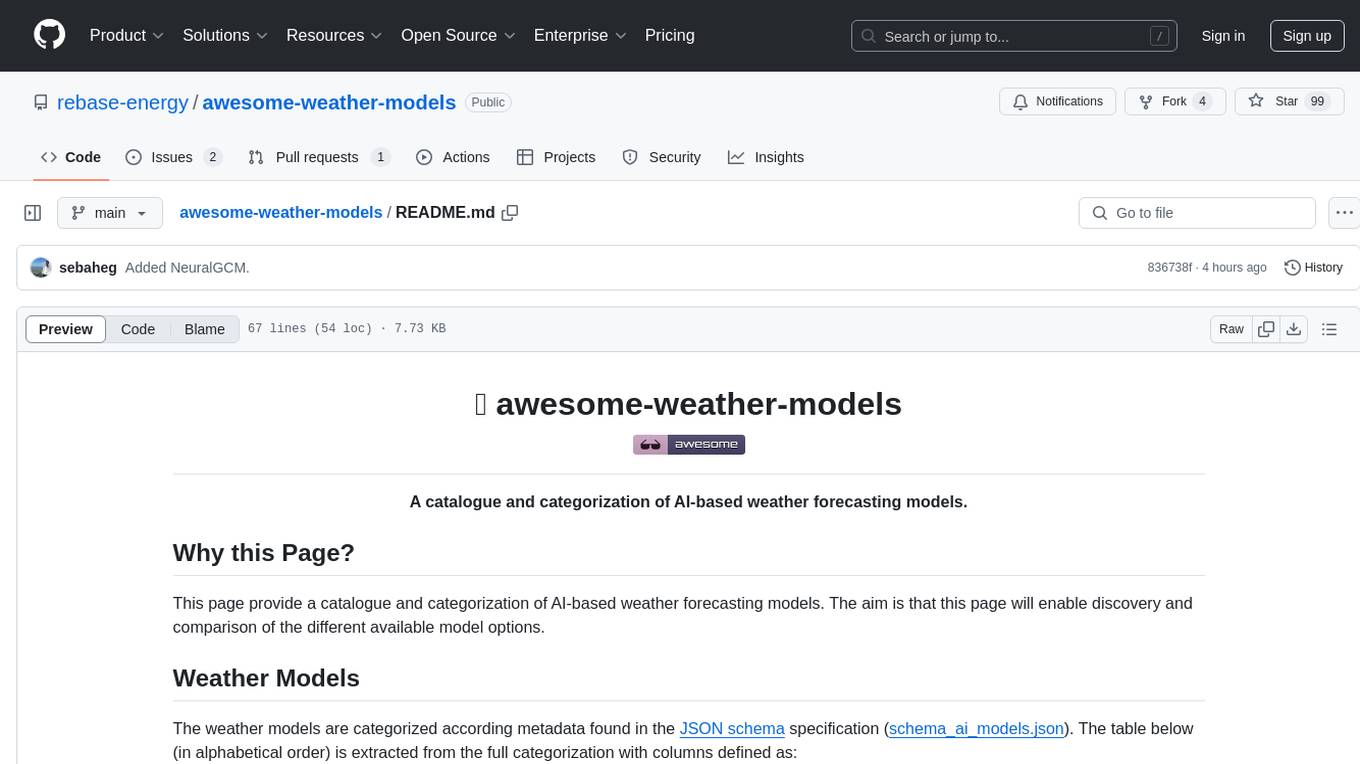
awesome-weather-models
A catalogue and categorization of AI-based weather forecasting models. This page provides a catalogue and categorization of AI-based weather forecasting models to enable discovery and comparison of different available model options. The weather models are categorized based on metadata found in the JSON schema specification. The table includes information such as the name of the weather model, the organization that developed it, operational data availability, open-source status, and links for further details.
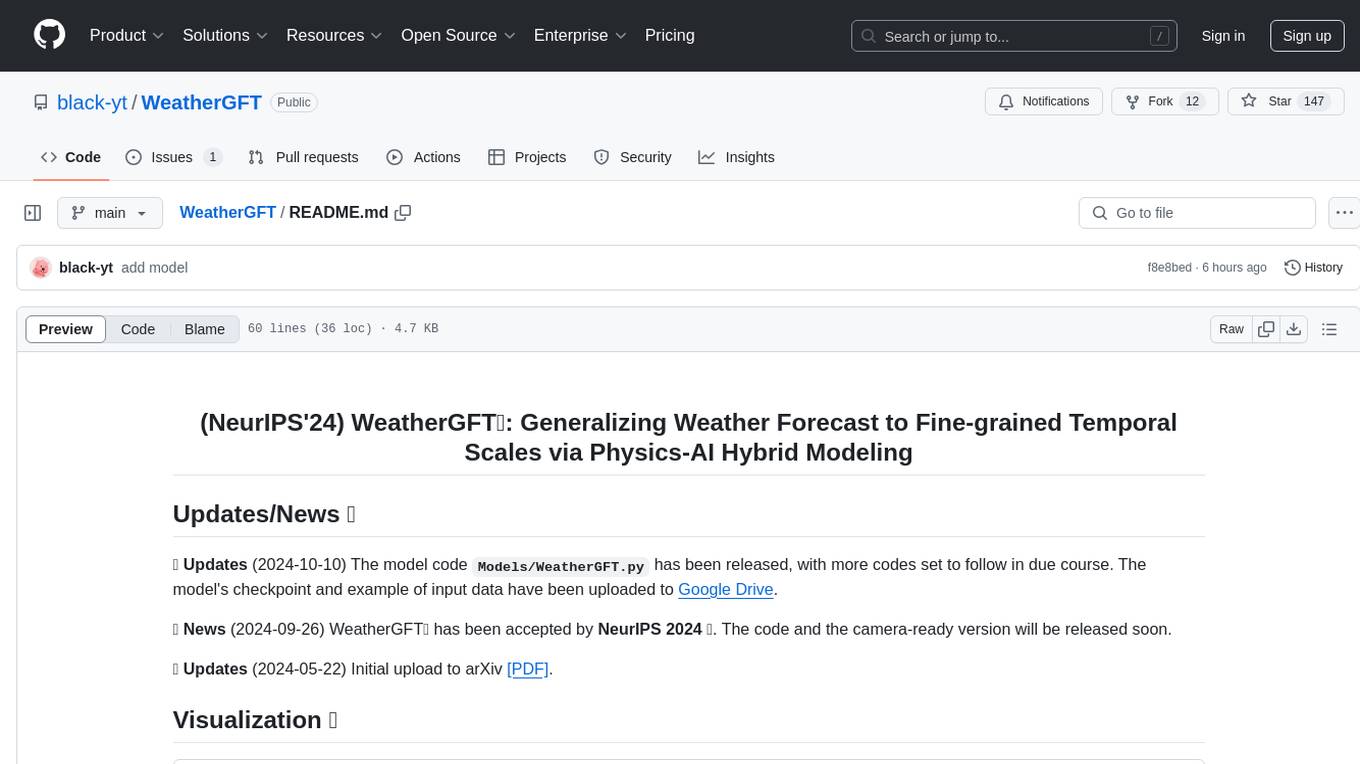
WeatherGFT
WeatherGFT is a physics-AI hybrid model designed to generalize weather forecasts to finer-grained temporal scales beyond the training dataset. It incorporates physical partial differential equations (PDEs) into neural networks to simulate fine-grained physical evolution and correct biases. The model achieves state-of-the-art performance in forecasting tasks at different time scales, from nowcasting to medium-range forecasts, by utilizing a lead time-aware training framework and a carefully designed PDE kernel. WeatherGFT bridges the gap between nowcast and medium-range forecast by extending forecasting abilities to predict accurately at a 30-minute time scale.
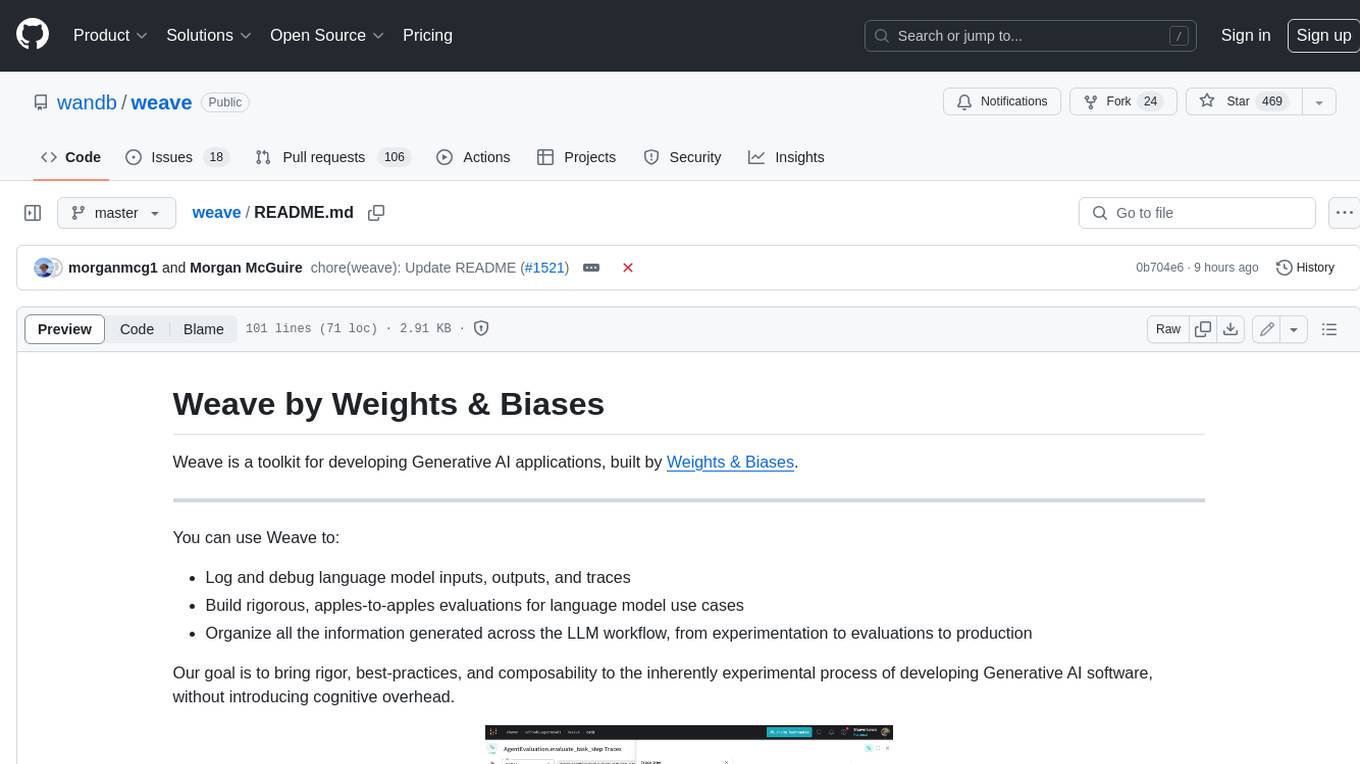
weave
Weave is a toolkit for developing Generative AI applications, built by Weights & Biases. With Weave, you can log and debug language model inputs, outputs, and traces; build rigorous, apples-to-apples evaluations for language model use cases; and organize all the information generated across the LLM workflow, from experimentation to evaluations to production. Weave aims to bring rigor, best-practices, and composability to the inherently experimental process of developing Generative AI software, without introducing cognitive overhead.
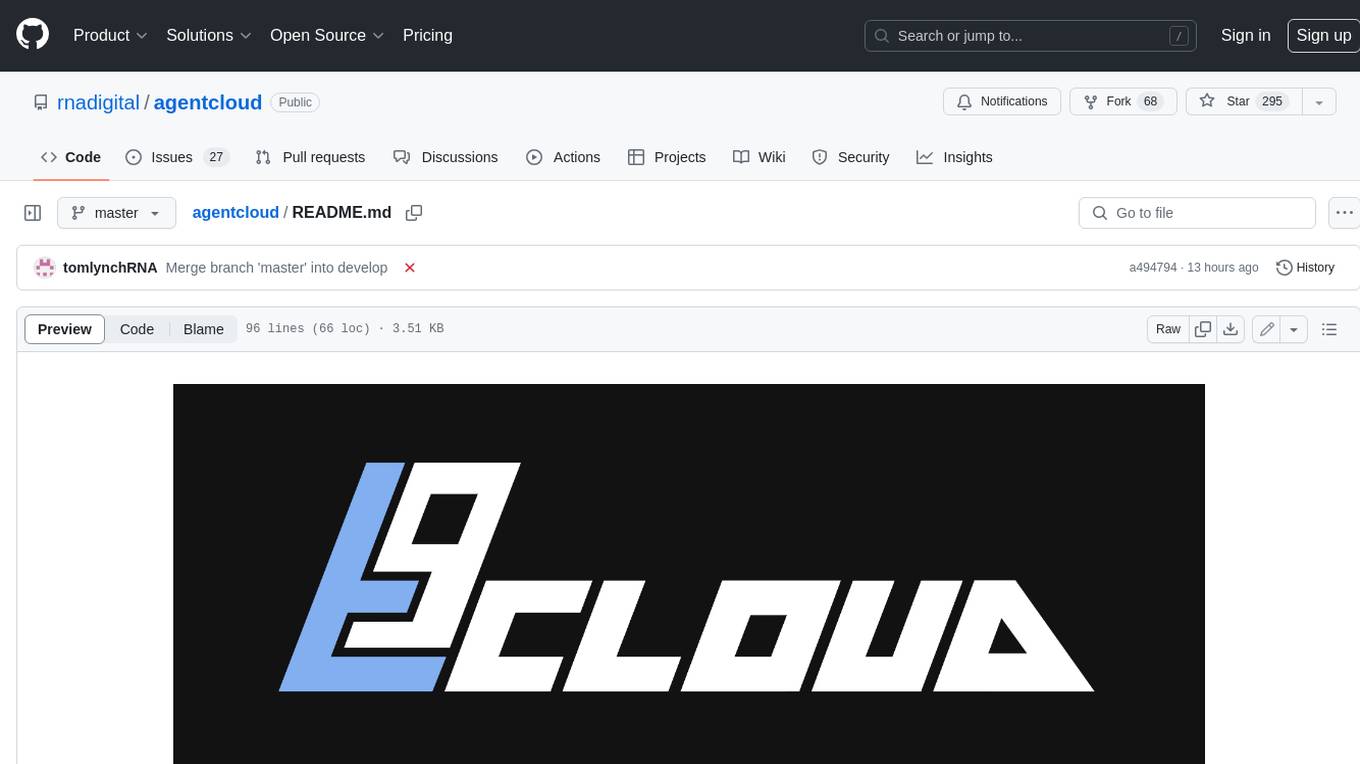
agentcloud
AgentCloud is an open-source platform that enables companies to build and deploy private LLM chat apps, empowering teams to securely interact with their data. It comprises three main components: Agent Backend, Webapp, and Vector Proxy. To run this project locally, clone the repository, install Docker, and start the services. The project is licensed under the GNU Affero General Public License, version 3 only. Contributions and feedback are welcome from the community.
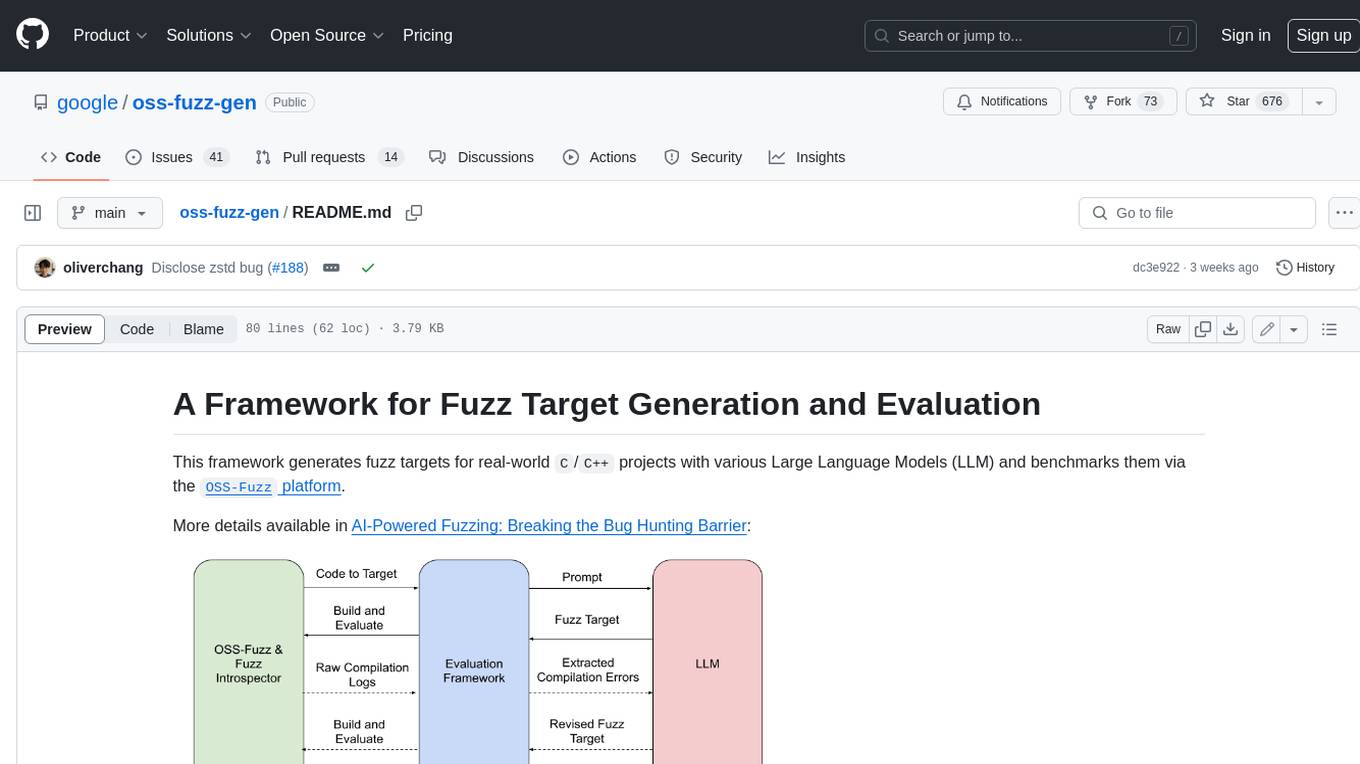
oss-fuzz-gen
This framework generates fuzz targets for real-world `C`/`C++` projects with various Large Language Models (LLM) and benchmarks them via the `OSS-Fuzz` platform. It manages to successfully leverage LLMs to generate valid fuzz targets (which generate non-zero coverage increase) for 160 C/C++ projects. The maximum line coverage increase is 29% from the existing human-written targets.