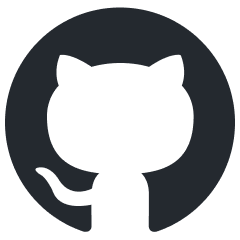
Awesome-LWMs
π A Collection of Awesome Large Weather Models (LWMs) | AI for Earth (AI4Earth) | AI for Science (AI4Science)
Stars: 188
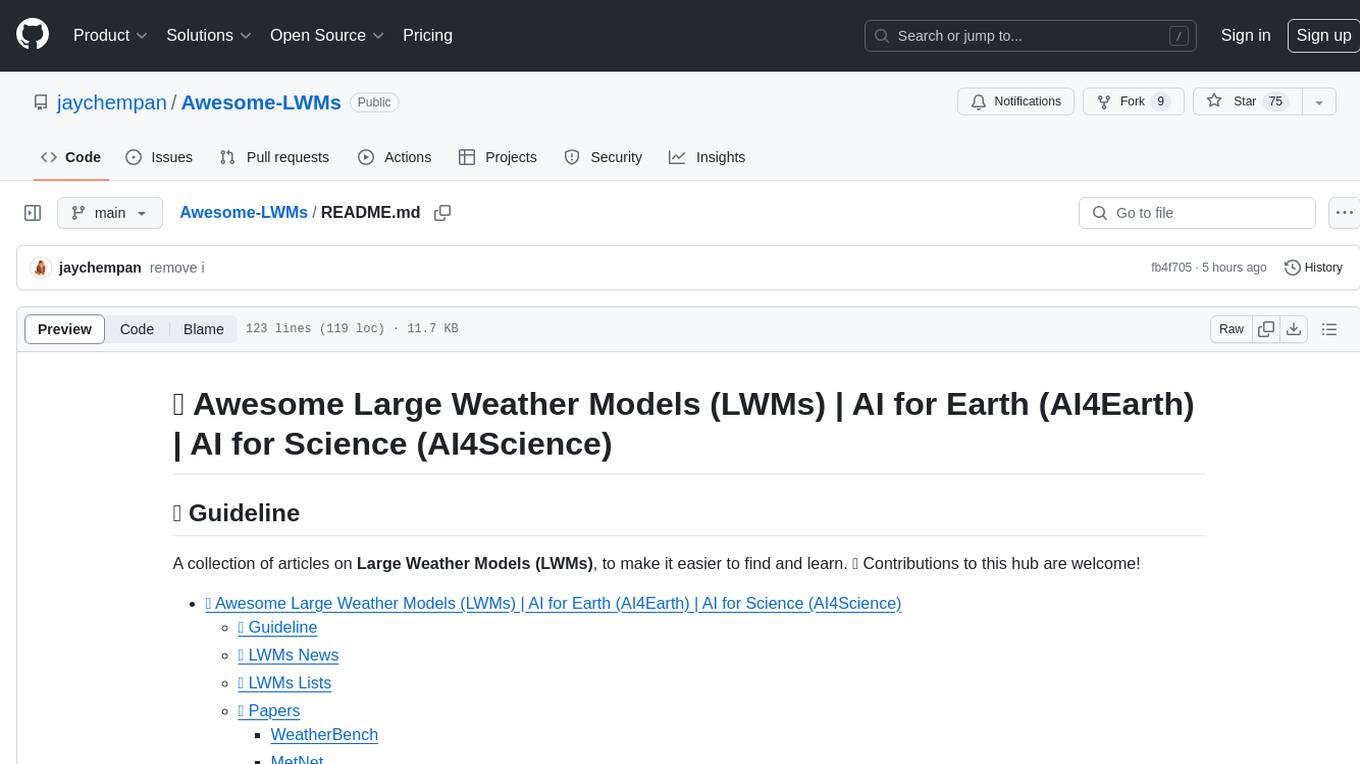
Awesome Large Weather Models (LWMs) is a curated collection of articles and resources related to large weather models used in AI for Earth and AI for Science. It includes information on various cutting-edge weather forecasting models, benchmark datasets, and research papers. The repository serves as a hub for researchers and enthusiasts to explore the latest advancements in weather modeling and forecasting.
README:
A collection of articles on Large Weather Models (LWMs), to make it easier to find and learn. π Contributions to this hub are welcome!
- 2024/12/20: GraphDOP, a new data-driven, end-to-end forecast system developed at the European Centre for Medium-Range Weather Forecasts (ECMWF) that is trained and initialised exclusively from Earth System observations, with no physics-based (re)analysis inputs or feedbacks [link].
- 2024/12/11: ECMWF releases its Artificial Intelligence Forecast System (AIFS) model and weights freely available on the web [link]
- 2024/12/04: Google DeepMind releases GenCast, an ensemble AI forecast model [link]
- 2024/09/20: IBM and Nasa Prithvi-WxC Foundation model [link]
- 2024/08/15: MetMamba, a DLWP model built on a state-of-the-art state-space model, Mamba, offers notable performance gains [link];
- 2024/07/30: FuXi-S2S published in Nature Communications [link];
- 2024/06/20: WEATHER-5K: A Large-scale Global Station Weather Dataset Towards Comprehensive Time-series Forecasting Benchmark [link];
- 2024/05/24: ORCA: A Global Ocean Emulator for Multi-year to Decadal Predictions [link];
- 2024/05/22: Generalizing Weather Forecast to Fine-grained Temporal Scales via Physics-AI Hybrid Modeling [link];
- 2024/05/20: Aurora: A Foundation Model of the Atmosphere [link];
- 2024/05/09: FuXi-ENS: A machine learning model for medium-range ensemble weather forecasting [link];
- 2024/05/06: CRA5: Extreme Compression of ERA5 for Portable Global Climate and Weather Research via an Efficient Variational Transformer [link];
- 2024/04/15: ClimODE: Climate and Weather Forecasting with Physics-informed Neural ODEs [link];
- 2024/04/12: FuXi-DA: A Generalized Deep Learning Data Assimilation Framework for Assimilating Satellite Observations [link];
- 2024/03/29: SEEDS: Generative emulation of weather forecast ensembles with diffusion models [link];
- 2024/03/13: KARINA: An Efficient Deep Learning Model for Global Weather Forecast [link];
- 2024/02/06: CasCast: Skillful High-resolution Precipitation Nowcasting via Cascaded Modelling [link];
- 2024/02/04: XiHe, the first data-driven 1/12Β° resolution global ocean eddy-resolving forecasting model [link];
- 2024/02/02: ExtremeCast: Boosting Extreme Value Prediction for Global Weather Forecast [link];
Expand to see more LWMs news
- 2024/01/28: FengWu-GHR, the first data-driven global weather forecasting model running at the 0.09β horizontal resolution [link];
- 2023/12/27: GenCast, a ML-based generative model for ensemble weather forecasting [link];
- 2023/12/16: Four-Dimensional Variational (4DVar) assimilation, and develop an AI-based cyclic weather forecasting system, FengWu-4DVar [link];
- 2023/12/15: FuXi-S2S: An accurate machine learning model for global subseasonal forecasts [link];
- 2023/12/11: A unified and flexible framework that can equip any type of spatio-temporal models is proposed based on residual diffusion DiffCast [link];
- 2023/11/13: GCMs are physics-based simulators which combine a numerical solver for large-scale dynamics with tuned representations for small-scale processes such as cloud formation. [link];
- 2023/12/13: FuXi is open source [link];
- 2023/11/14: GraphCast published in Science [link];
- 2023/10/25: IBM and Nasa Prithvi-100M Model [link];
- 2023/09/14: Pangu-Weather published in Nature [link];
- 2023/08/25: ClimaX published in ICML 2023 [link];
LWM name | From | Date(1st) | Publication | Links | Model Licence | Weights Licence |
---|---|---|---|---|---|---|
MetNet | 2020.03 | - | [arXiv paper] [github] | [MIT] | N/A | |
FourCastNet | NVIDIA | 2022.02 | PASC 23 | [arXiv paper] [github] | [BSD-3] | [BSD-3] |
MetNet-2 | 2022.09 | Nature Communications 2022 | [Nature paper] [github] | [MIT] | N/A | |
Pangu-Weather | Huaiwei | 2022.11 | Nature 2023 | [Nature paper] [github] | Not Specified | [CC-BY-NC-SA 4.0] |
GraphCast | DeepMind | 2022.12 | Science 2023 | [Science paper] [github] | [Apache 2.0] | [CC-BY-NC-SA 4.0] |
ClimaX | Microsoft | 2023.01 | ICML 2023 | [arXiv paper] [github] | [MIT] | Not specificied ([MIT]?) |
Fengwu | Shanghai AI Lab | 2023.04 | - | [arXiv paper] [github] | Not Specified | Not Specified |
MetNet-3 | 2023.06 | - | [arXiv paper] | - | - | |
FuXi | Fudan | 2023.06 | npj 2023 | [arXiv paper] [github] | [Apache 2.0] | [CC-BY-NC-SA 4.0] |
NowcastNet | Tsinghua | 2023.07 | Nature 2023 | [Nature paper] | - | - |
AI-GOMS | Tsinghua | 2023.08 | - | [arXiv paper] | - | - |
Prithvi-100M | IBM / Nasa | 2023.08 | [arXiv paper] [Hugging Face] | [Apache 2.0] | [Apache 2.0] | |
FuXi-Extreme | Fudan | 2023.10 | - | [arXiv paper] | - | - |
NeuralGCM | DeepMind | 2023.11 | - | [arXiv paper] | - | - |
FengWu-4DVar | Tsinghua | 2023.12 | ICML 2024 | [arXiv paper] | - | - |
FengWu-Adas | Shanghai AI Lab | 2023.12 | - | [arXiv paper] | - | - |
FuXi-S2S | Fudan | 2023.12 | Nature Communications 2024 | [arXiv paper] [NC paper] | - | - |
GenCast | Google DeepMind | 2023.12 | Nature | [paper] [github] | [Apache 2.0] | [CC-BY-NC-SA 4.0] |
DiffCast | HITsz | 2023.12 | CVPR 2024 | [arXiv paper] | - | - |
FengWu-GHR | Shanghai AI Lab | 2024.01 | - | [arXiv paper] | - | - |
ExtremeCast | Shanghai AI Lab | 2024.02 | - | [arXiv paper] [github] | Not Specified | Not Specified |
XiHe | NUDT | 2024.02 | - | [arXiv paper] [github] | Not Specified | Not Specified |
CasCast | Shanghai AI Lab | 2024.02 | ICML 2024 | [arXiv paper] [github] | Not Specified | Not Specified |
KARINA | KIST | 2024.03 | - | [arXiv paper] | - | - |
SEEDS | 2024.03 | Science Advances | [Science Advances paper] | - | - | |
FuXi-DA | Fudan | 2024.04 | - | [arXiv paper] | - | - |
ClimODE | Aalto University | 2024.04 | ICLR 2024 (Oral) | [arXiv paper] [github] | [MIT] | Not specified or non applicable |
FuXi-ENS | Fudan | 2024.05 | - | [arXiv paper] | - | - |
Aurora | Microsoft | 2024.05 | - | [arXiv paper] [github] | [MIT] | [CC-BY-NC-SA 4.0] |
WeatherGFT | Shanghai AI Lab | 2024.05 | NeurIPS 2024 | [arXiv paper] [github] | Not Specified | Not Specified |
ORCA | Shanghai AI Lab | 2024.05 | - | [arXiv paper] [github] | - | - |
MetMamba | Beijing PRESKY Technology | 2024.08 | - | [paper] | Not Specified | Not Specified |
Prithvi-WxC | IBM / Nasa | 2024.09 | - | [arXiv paper] [Hugging Face] | [CDLA Permissive 2.0] | [CDLA Permissive 2.0] |
AIFS | ECMWF | 2024.12 | - | [arXiv paper] [Hugging Face] | [CC BY 4.0] | [CC BY 4.0] |
GraphDOP | ECMWF | 2024.12 | - | [arXiv paper] | - | - |
Dataset name | From | Date(1st) | Publication | Links |
---|---|---|---|---|
WeatherBench | 2020.02 | JAMES 2020 | [paper] [github] | |
ERA5 | ECMWF | 2020.05 | - | [paper] [link] |
SEVIR | MIT | 2020.06 | NeurIPS 2020 | [paper] [github] [link] |
WeatherBench2 | 2023.08 | - | [paper] [github] | |
CRA5 | Shanghai AI Lab | 2024.05 | - | [paper] [github] |
WEATHER-5K | Beijing PRESKY Technology | 2024.08 | - | [paper] |
- WeatherBench: A benchmark dataset for data-driven weather forecasting [pdf]
- WeatherBench 2: A benchmark for the next generation of data-driven global weather models [pdf]
- MetNet: A Neural Weather Model for Precipitation Forecasting (MetNet) [pdf]
- Deep learning for twelve hour precipitation forecasts (MetNet-2) [pdf]
- Deep Learning for Day Forecasts from Sparse Observations (MetNet-3) [pdf]
- FourCastNet: A Global Data-driven High-resolution Weather Model using Adaptive Fourier Neural Operators (FourCastNet) [pdf]
- Accurate medium-range global weather forecasting with 3D neural networks (Pangu-Weather) [pdf]
- Learning skillful medium-range global weather forecasting (GraphCast) [pdf]
- ClimaX: A foundation model for weather and climate (ClimaX) [pdf]
- FengWu: Pushing the Skillful Global Medium-range Weather Forecast beyond 10 Days Lead (FengWu) [pdf]
- FengWu-4DVar: Coupling the Data-driven Weather Forecasting Model with 4D Variational Assimilation [pdf]
- Towards an end-to-end artificial intelligence driven global weather forecasting system [pdf]
- FengWu-GHR: Learning the Kilometer-scale Medium-range Global Weather Forecasting [pdf]
- ExtremeCast: Boosting Extreme Value Prediction for Global Weather Forecast [pdf]
- FuXi: A cascade machine learning forecasting system for 15-day global weather forecast (FuXi) [pdf]
- FuXi-Extreme: Improving extreme rainfall and wind forecasts with diffusion model (FuXi-Extreme) [pdf]
- FuXi-S2S: An accurate machine learning model for global subseasonal forecasts [pdf]
- Fuxi-DA: A Generalized Deep Learning Data Assimilation Framework for Assimilating Satellite Observations [pdf]
- FuXi-ENS: A machine learning model for medium-range ensemble weather forecasting [pdf]
- AI-GOMS: Large AI-Driven Global Ocean Modeling System (AI-GOMS) [pdf]
- XiHe: A Data-Driven Model for Global Ocean Eddy-Resolving Forecasting [pdf]
- Fourier Neural Operator with Learned Deformations for PDEs on General Geometries [pdf]
- SFNO: Spherical Fourier Neural Operators: Learning Stable Dynamics on the Sphere [pdf]
- Earthformer: Exploring Space-Time Transformers for Earth System Forecasting [pdf]
- PreDiff: Precipitation Nowcasting with Latent Diffusion Models [pdf]
- DGMR: Skilful precipitation nowcasting using deep generative models of radar [odf]
- Skilful nowcasting of extreme precipitation with NowcastNet (NowcastNet) [pdf]
- DiffCast: A Unified Framework via Residual Diffusion for Precipitation Nowcasting [pdf]
- CasCast: Skillful High-resolution Precipitation Nowcasting via Cascaded Modelling [pdf]
- Generalizing Weather Forecast to Fine-grained Temporal Scales via Physics-AI Hybrid Modeling [pdf]
- NeuralGCM: Neural General Circulation Models for Weather and Climate [pdf]
- ClimODE: Climate and Weather Forecasting with Physics-informed Neural ODEs [pdf]
- Generalizing Weather Forecast to Fine-grained Temporal Scales via Physics-AI Hybrid Modeling [pdf]
- WeatherBench: A benchmark dataset for data-driven weather forecasting [pdf]
- The ERA5 global reanalysis [pdf]
- SEVIR : A Storm Event Imagery Dataset for Deep Learning Applications in Radar and Satellite Meteorology [pdf]
- WeatherBench 2: A benchmark for the next generation of data-driven global weather models [pdf]
- CRA5: Extreme Compression of ERA5 for Portable Global Climate and Weather Research via an Efficient Variational Transformer [pdf]
- WEATHER-5K: A Large-scale Global Station Weather Dataset Towards Comprehensive Time-series Forecasting Benchmark [pdf]
- Can deep learning beat numerical weather prediction? [pdf]
- AtmoRep: A stochastic model of atmosphere dynamics using large scale representation learning [pdf]
- Anthropogenic fingerprints in daily precipitation revealed by deep learning [pdf]
- GenCast: Diffusion-based ensemble forecasting for medium-range weather [pdf]
- KARINA: An Efficient Deep Learning Model for Global Weather Forecast [pdf]
- SEEDS: Generative emulation of weather forecast ensembles with diffusion models [pdf]
- Aurora: A Foundation Model of the Atmosphere [pdf]
- ORCA: A Global Ocean Emulator for Multi-year to Decadal Predictions [pdf]
- GraphDOP: Towards skilful data-driven medium-range weather forecasts learnt and initialised directly from observations [pdf]
- ECMWF AI Models: AI-based weather forecasting models.
- Skyrim: AI weather models united.
- NVIDIA Earth2Mip: Earth-2 Model Intercomparison Project (MIP) is a python framework that enables climate researchers and scientists to inter-compare AI models for weather and climate.
- AI Models for All: Run AI NWP forecasts hassle-free, serverless in the cloud!
- OpenEarthLab: OpenEarthLab, aiming at developing cutting-edge Spatiaotemporal Generation algorithms and promoting the development of Earth Science.
For Tasks:
Click tags to check more tools for each tasksFor Jobs:
Alternative AI tools for Awesome-LWMs
Similar Open Source Tools
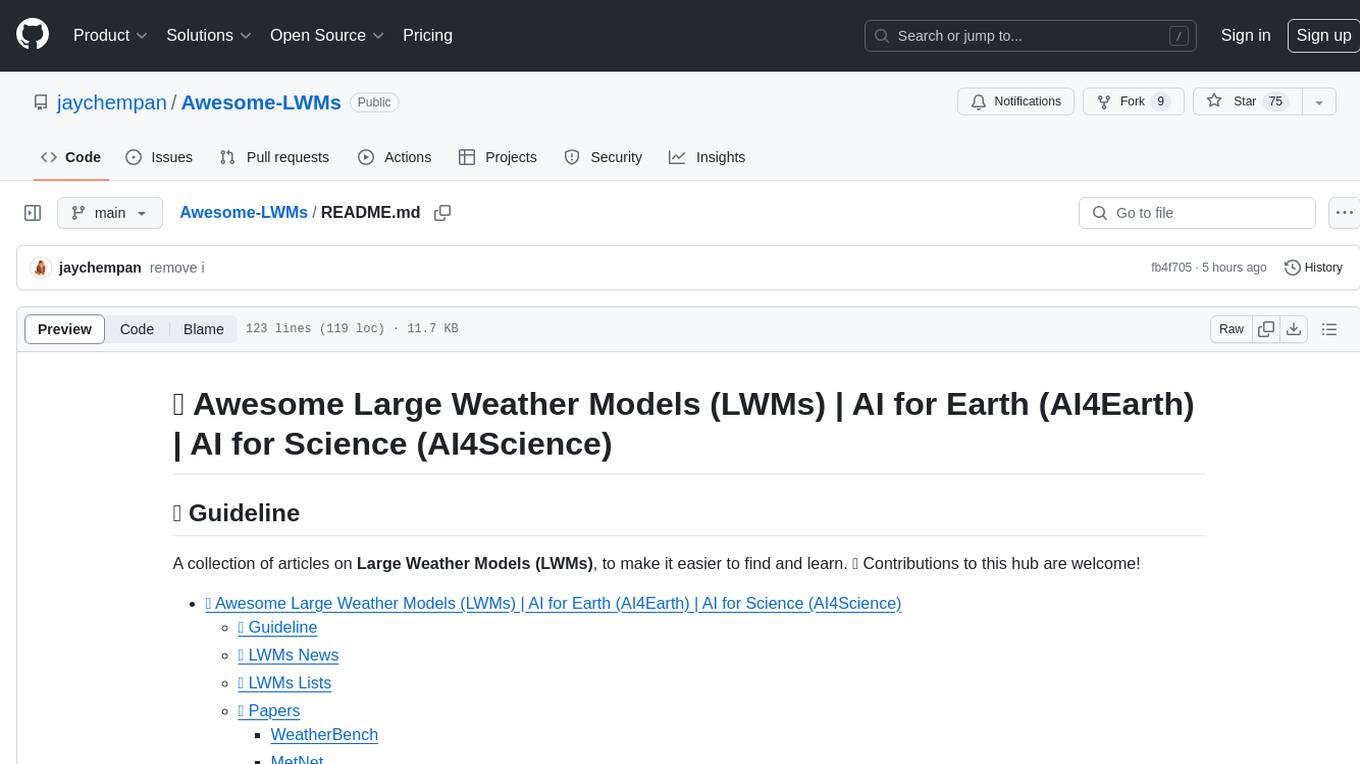
Awesome-LWMs
Awesome Large Weather Models (LWMs) is a curated collection of articles and resources related to large weather models used in AI for Earth and AI for Science. It includes information on various cutting-edge weather forecasting models, benchmark datasets, and research papers. The repository serves as a hub for researchers and enthusiasts to explore the latest advancements in weather modeling and forecasting.
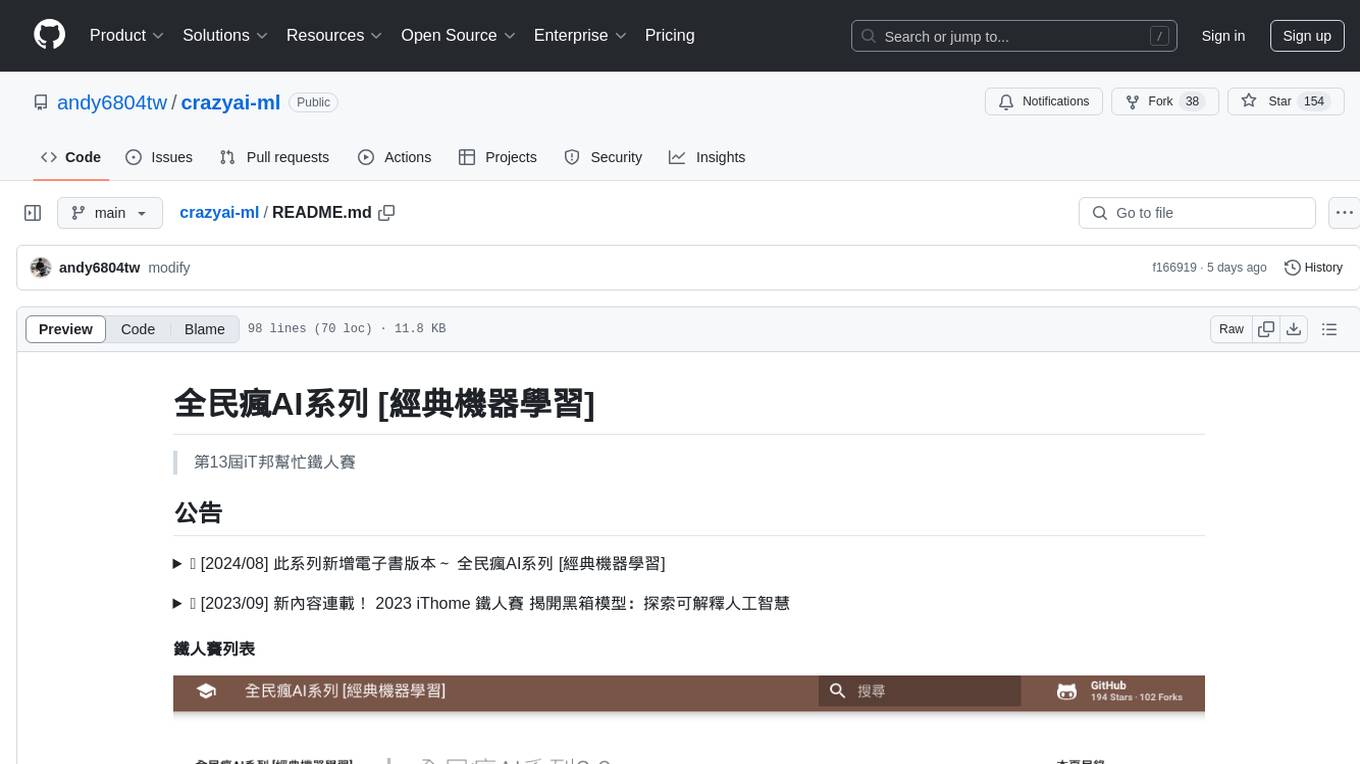
crazyai-ml
The 'crazyai-ml' repository is a collection of resources related to machine learning, specifically focusing on explaining artificial intelligence models. It includes articles, code snippets, and tutorials covering various machine learning algorithms, data analysis, model training, and deployment. The content aims to provide a comprehensive guide for beginners in the field of AI, offering practical implementations and insights into popular machine learning packages and model tuning techniques. The repository also addresses the integration of AI models and frontend-backend concepts, making it a valuable resource for individuals interested in AI applications.
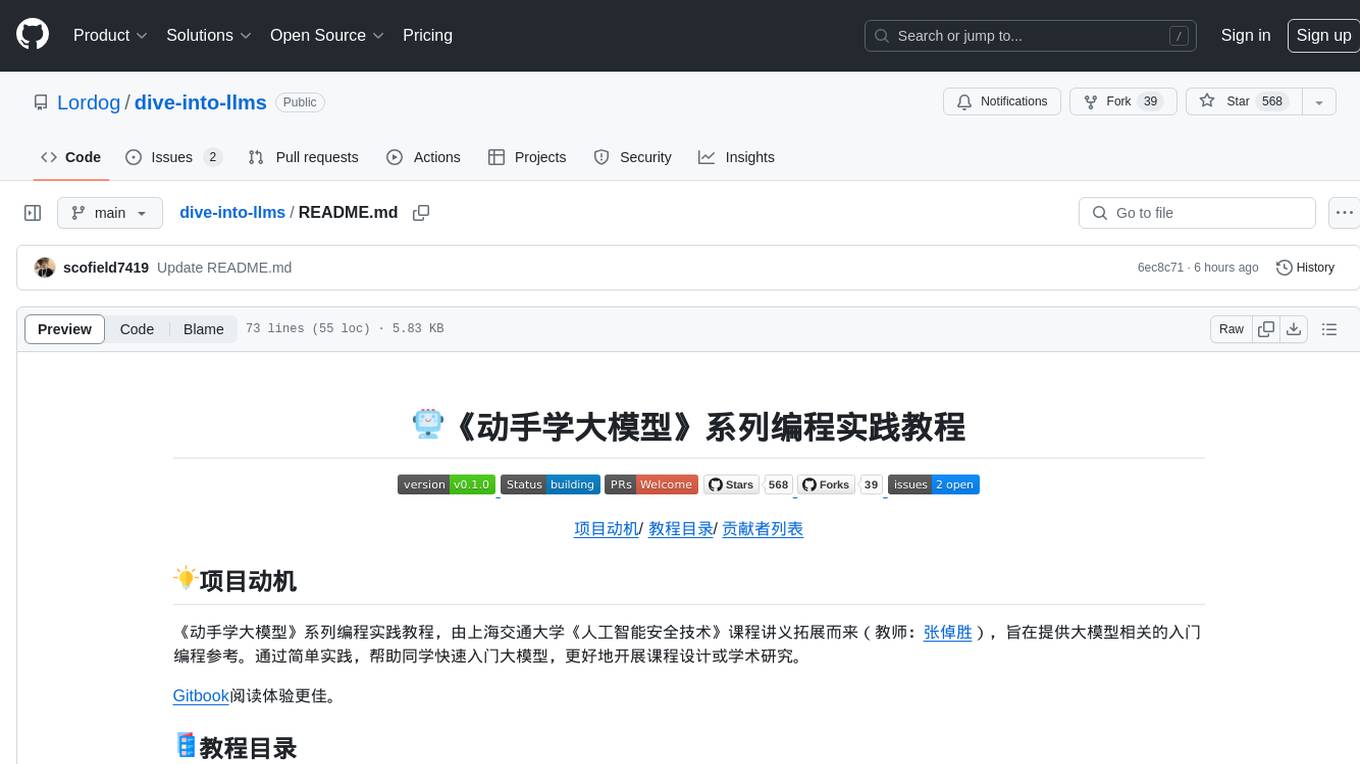
dive-into-llms
The 'Dive into Large Language Models' series programming practice tutorial is an extension of the 'Artificial Intelligence Security Technology' course lecture notes from Shanghai Jiao Tong University (Instructor: Zhang Zhuosheng). It aims to provide introductory programming references related to large models. Through simple practice, it helps students quickly grasp large models, better engage in course design, or academic research. The tutorial covers topics such as fine-tuning and deployment, prompt learning and thought chains, knowledge editing, model watermarking, jailbreak attacks, multimodal models, large model intelligent agents, and security. Disclaimer: The content is based on contributors' personal experiences, internet data, and accumulated research work, provided for reference only.
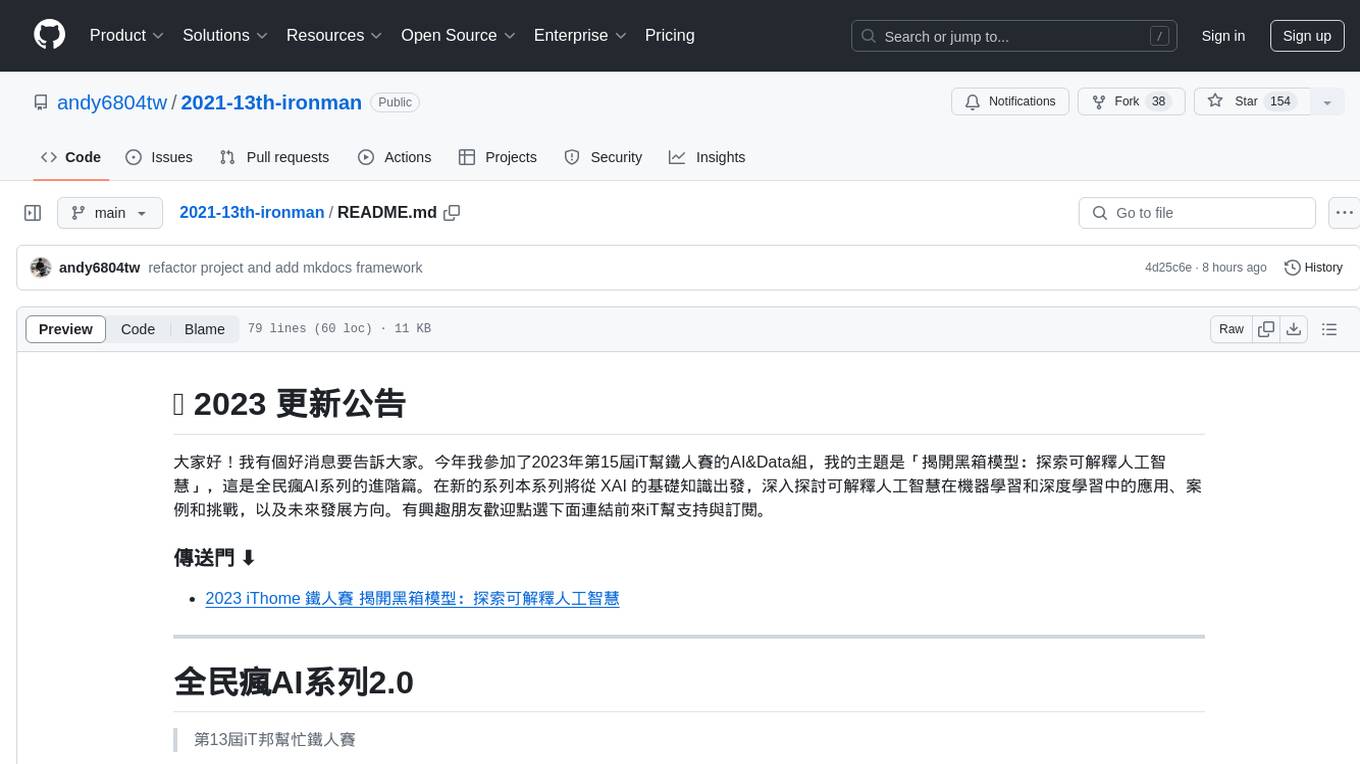
2021-13th-ironman
This repository is a part of the 13th iT Help Ironman competition, focusing on exploring explainable artificial intelligence (XAI) in machine learning and deep learning. The content covers the basics of XAI, its applications, cases, challenges, and future directions. It also includes practical machine learning algorithms, model deployment, and integration concepts. The author aims to provide detailed resources on AI and share knowledge with the audience through this competition.
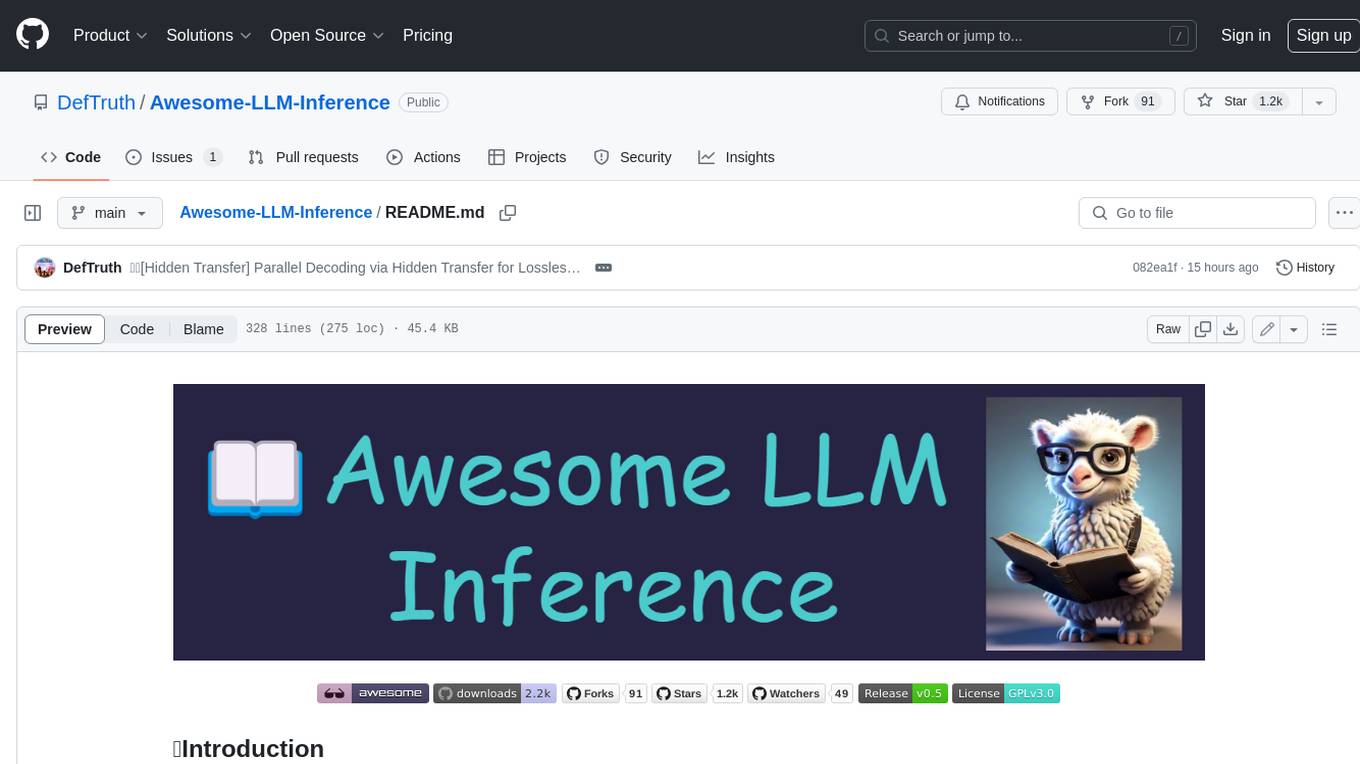
Awesome-LLM-Inference
Awesome-LLM-Inference: A curated list of πAwesome LLM Inference Papers with Codes, check πContents for more details. This repo is still updated frequently ~ π¨βπ»β Welcome to star βοΈ or submit a PR to this repo!
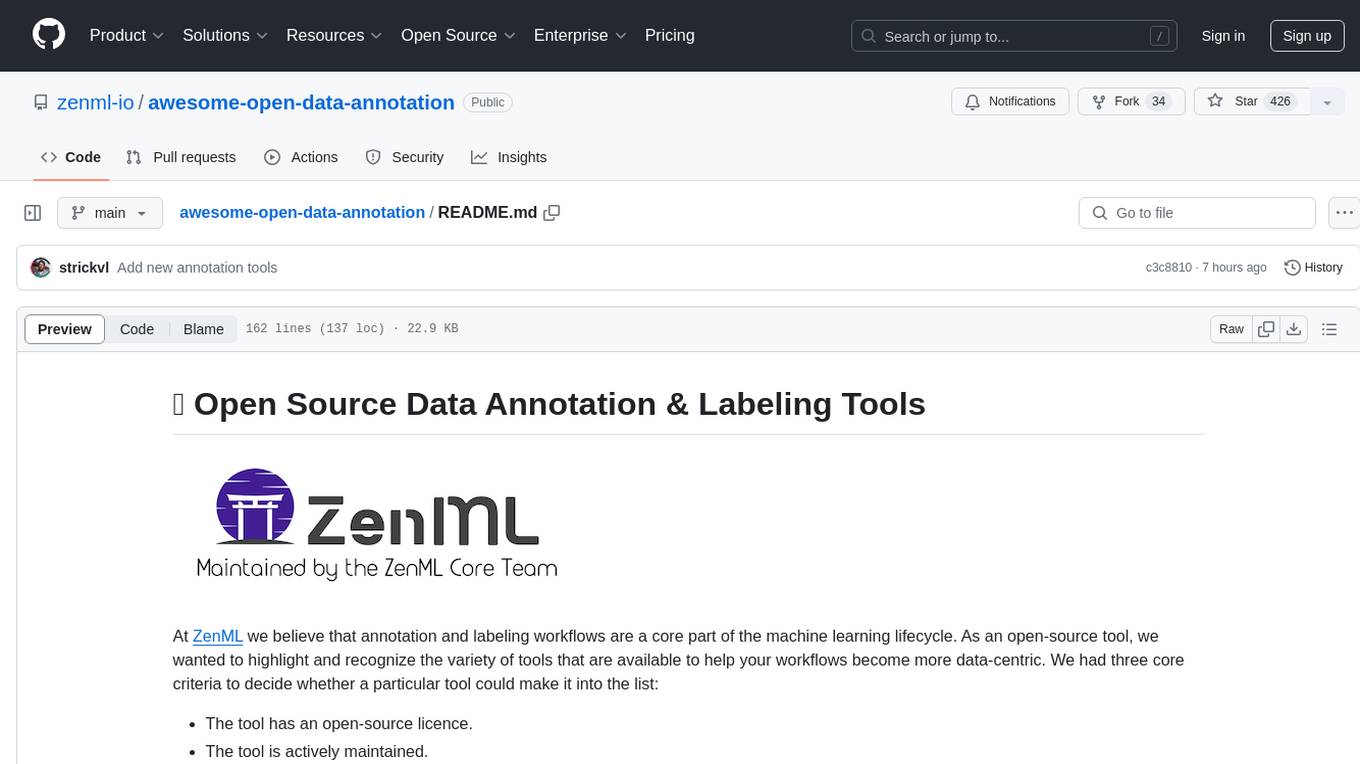
awesome-open-data-annotation
At ZenML, we believe in the importance of annotation and labeling workflows in the machine learning lifecycle. This repository showcases a curated list of open-source data annotation and labeling tools that are actively maintained and fit for purpose. The tools cover various domains such as multi-modal, text, images, audio, video, time series, and other data types. Users can contribute to the list and discover tools for tasks like named entity recognition, data annotation for machine learning, image and video annotation, text classification, sequence labeling, object detection, and more. The repository aims to help users enhance their data-centric workflows by leveraging these tools.
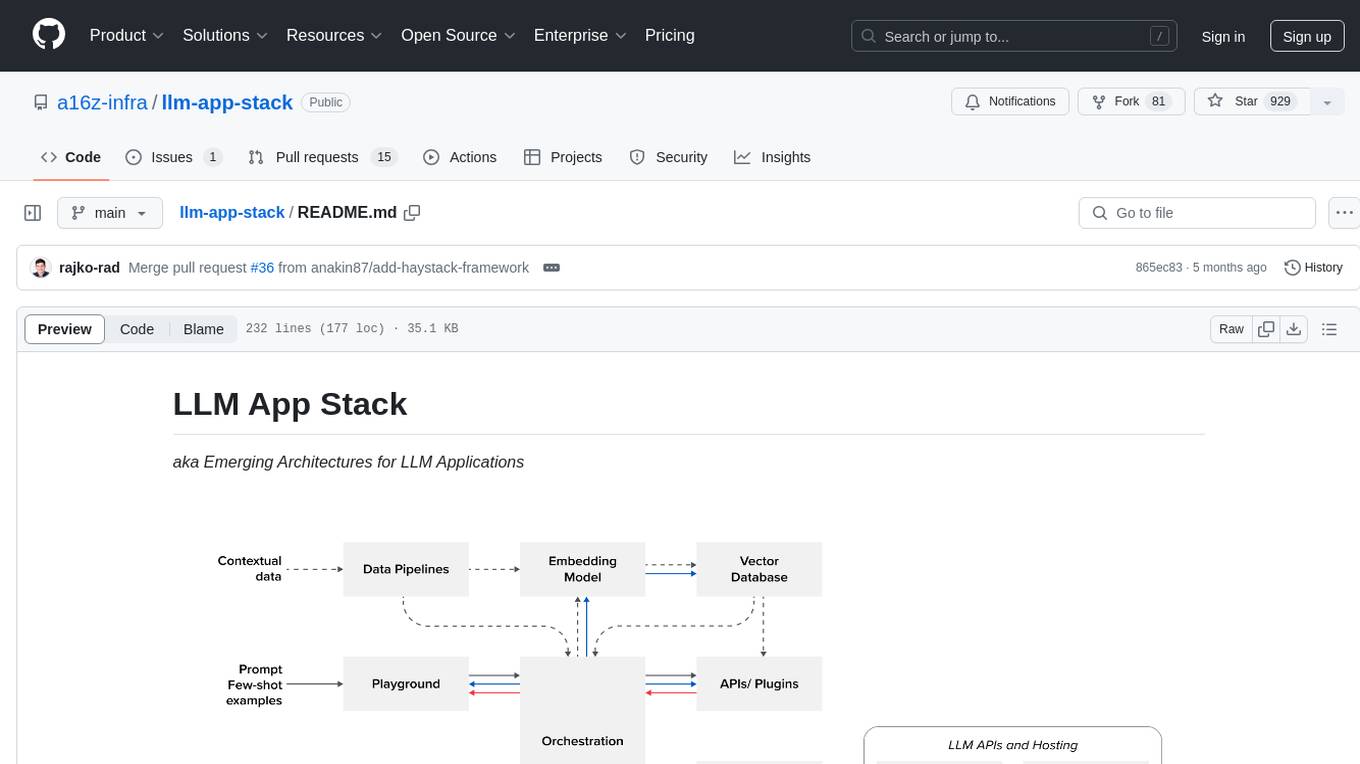
llm-app-stack
LLM App Stack, also known as Emerging Architectures for LLM Applications, is a comprehensive list of available tools, projects, and vendors at each layer of the LLM app stack. It covers various categories such as Data Pipelines, Embedding Models, Vector Databases, Playgrounds, Orchestrators, APIs/Plugins, LLM Caches, Logging/Monitoring/Eval, Validators, LLM APIs (proprietary and open source), App Hosting Platforms, Cloud Providers, and Opinionated Clouds. The repository aims to provide a detailed overview of tools and projects for building, deploying, and maintaining enterprise data solutions, AI models, and applications.
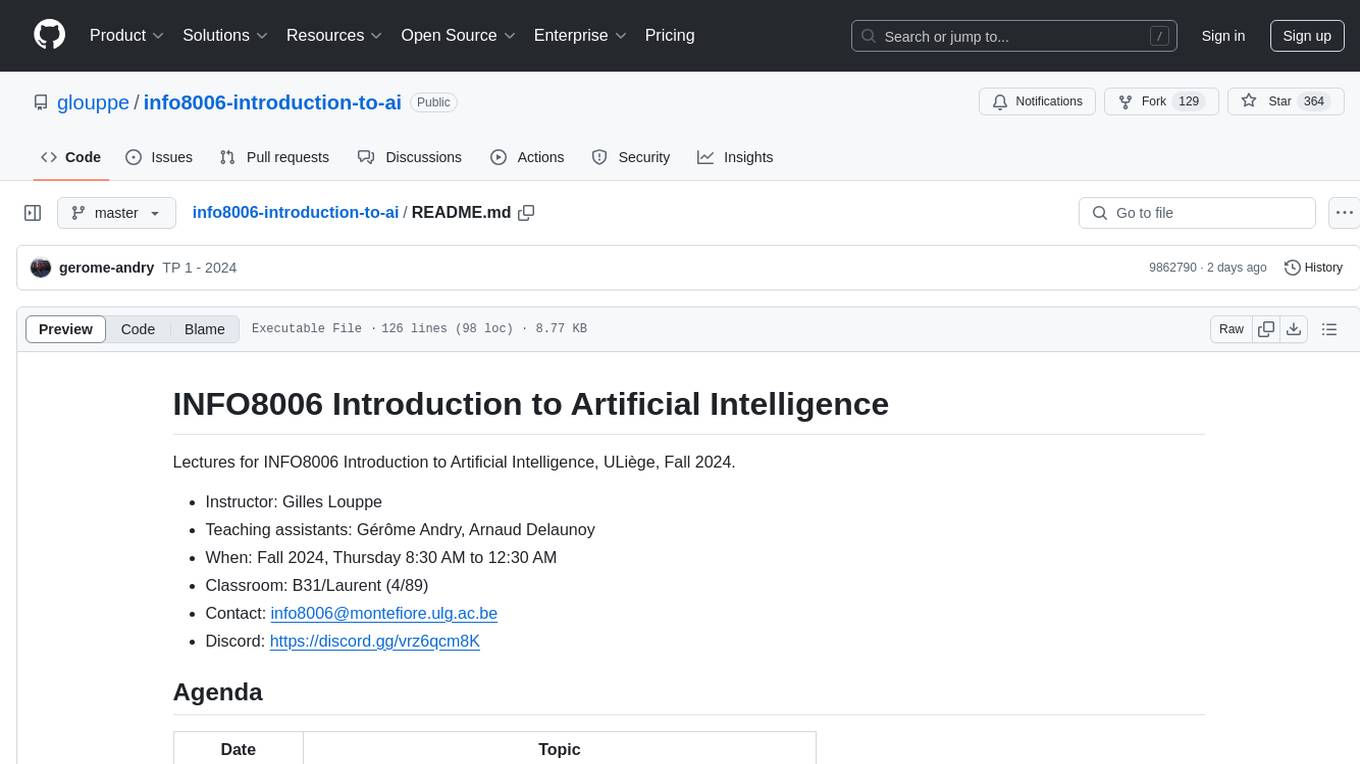
info8006-introduction-to-ai
INFO8006 Introduction to Artificial Intelligence is a course at ULiège that covers various topics in AI such as intelligent agents, problem-solving, games, probabilistic reasoning, machine learning, neural networks, reinforcement learning, and decision-making. The course includes lectures, exercises, and programming projects using Python. Students can access course materials, previous exams, and archived lectures to enhance their understanding of AI concepts.
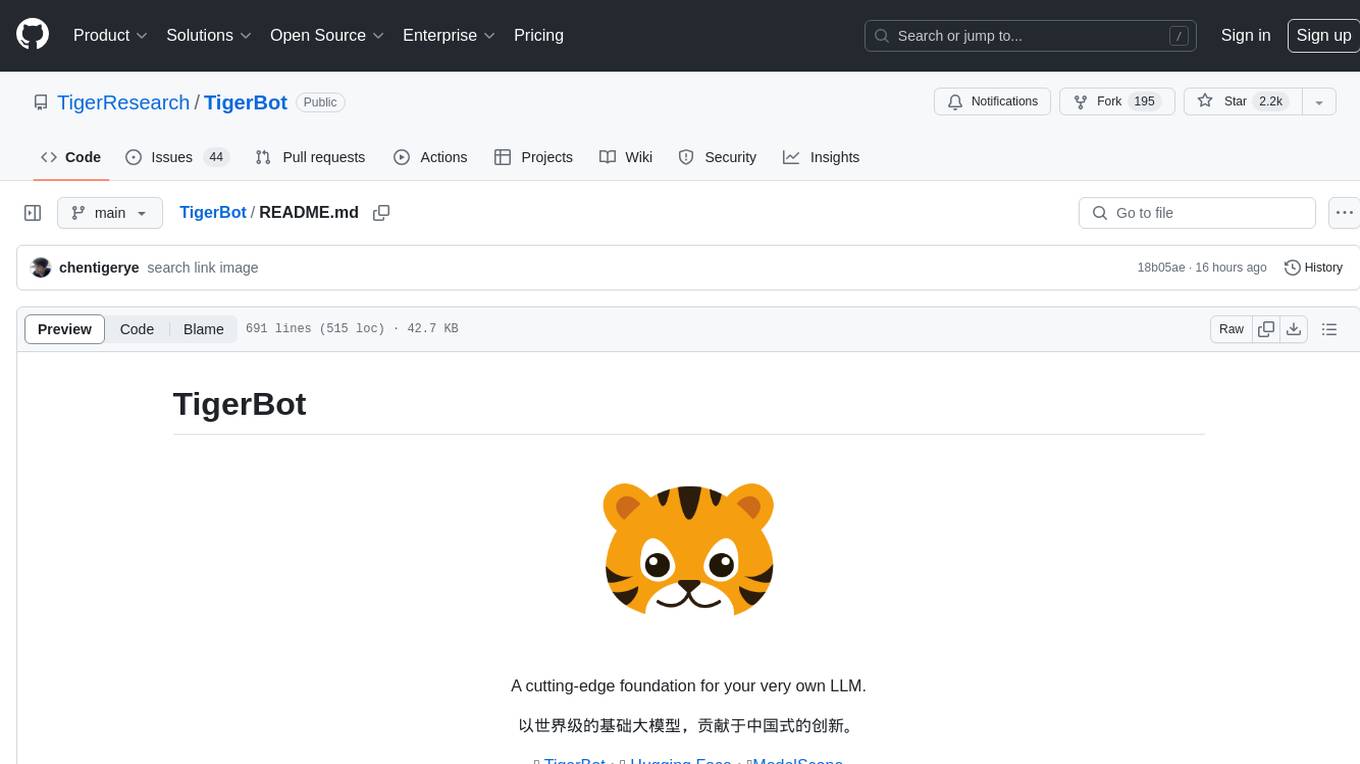
TigerBot
TigerBot is a cutting-edge foundation for your very own LLM, providing a world-class large model for innovative Chinese-style contributions. It offers various upgrades and features, such as search mode enhancements, support for large context lengths, and the ability to play text-based games. TigerBot is suitable for prompt-based game engine development, interactive game design, and real-time feedback for playable games.
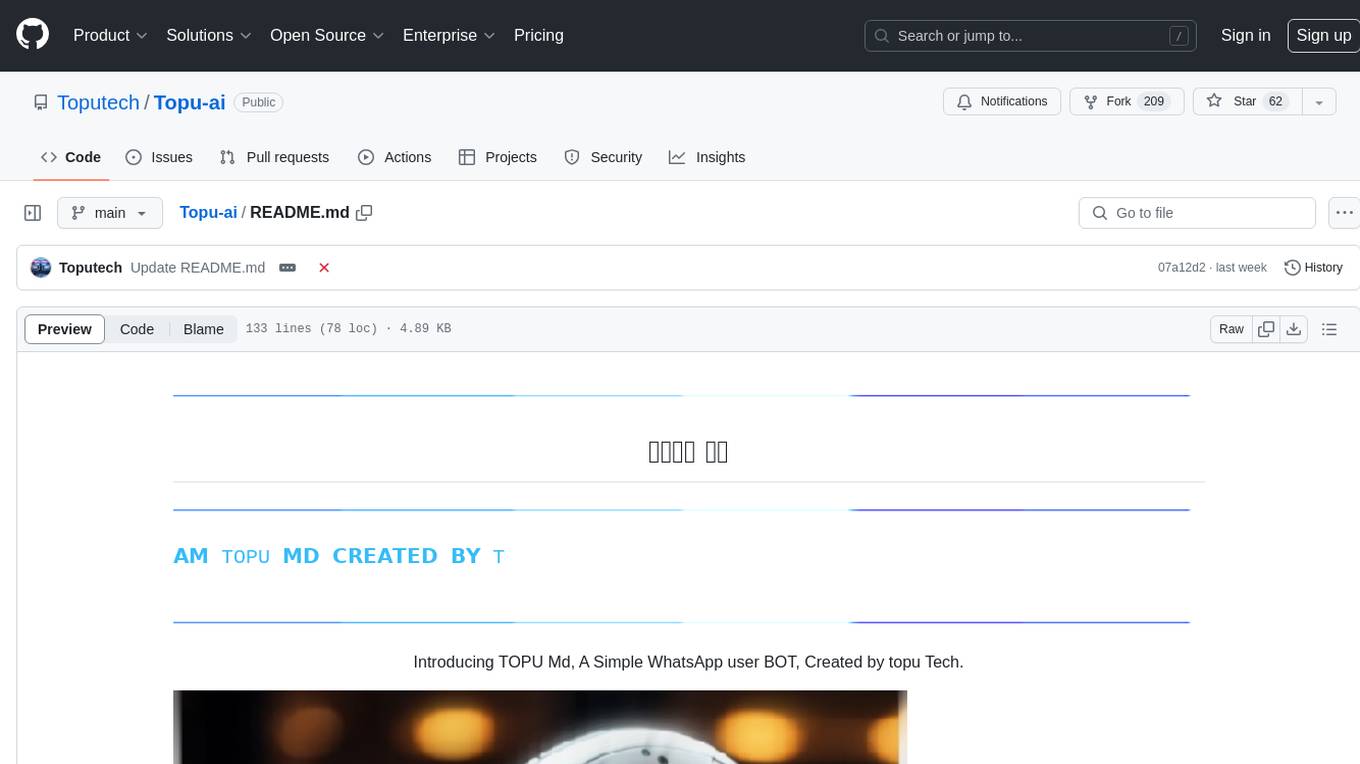
Topu-ai
TOPU Md is a simple WhatsApp user bot created by Topu Tech. It offers various features such as multi-device support, AI photo enhancement, downloader commands, hidden NSFW commands, logo commands, anime commands, economy menu, various games, and audio/video editor commands. Users can fork the repo, get a session ID by pairing code, and deploy on Heroku. The bot requires Node version 18.x or higher for optimal performance. Contributions to TOPU-MD are welcome, and the tool is safe for use on WhatsApp and Heroku. The tool is licensed under the MIT License and is designed to enhance the WhatsApp experience with diverse features.
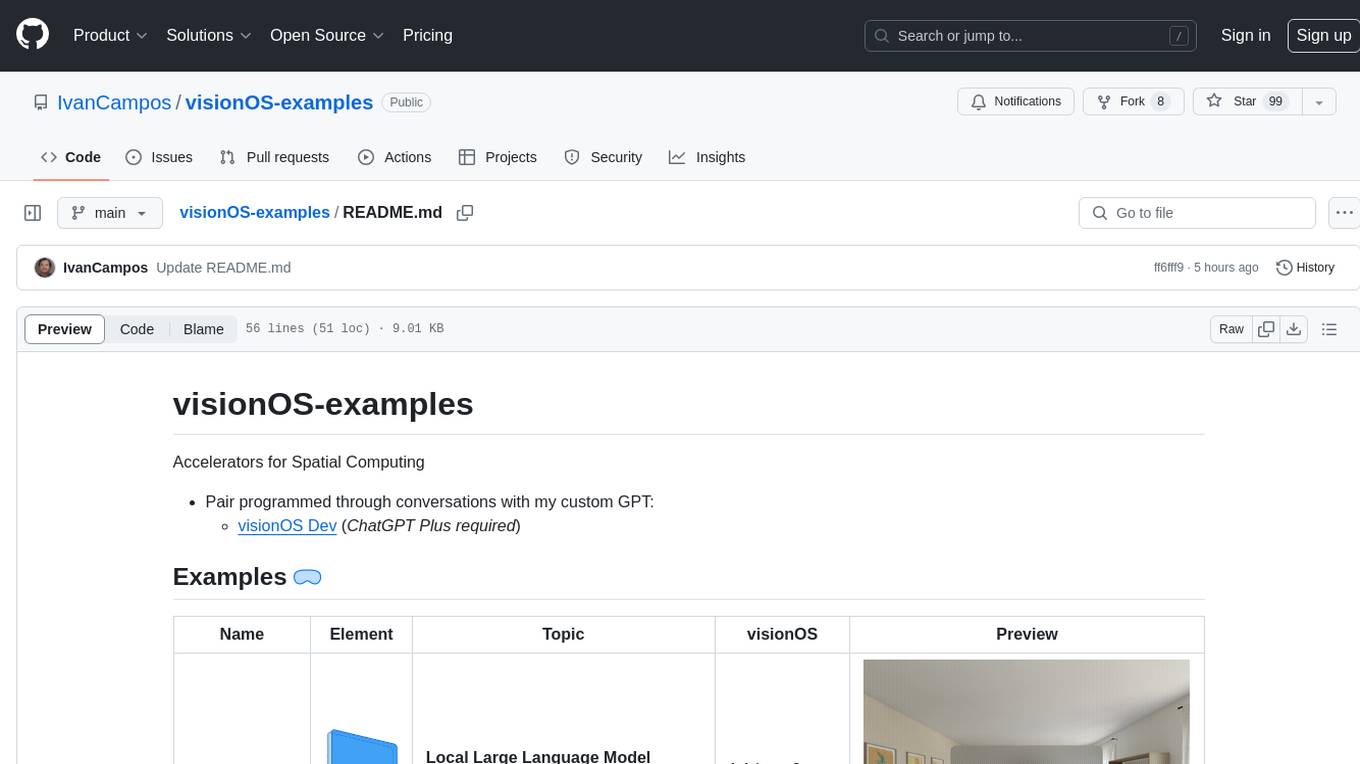
visionOS-examples
visionOS-examples is a repository containing accelerators for Spatial Computing. It includes examples such as Local Large Language Model, Chat Apple Vision Pro, WebSockets, Anchor To Head, Hand Tracking, Battery Life, Countdown, Plane Detection, Timer Vision, and PencilKit for visionOS. The repository showcases various functionalities and features for Apple Vision Pro, offering tools for developers to enhance their visionOS apps with capabilities like hand tracking, plane detection, and real-time cryptocurrency prices.
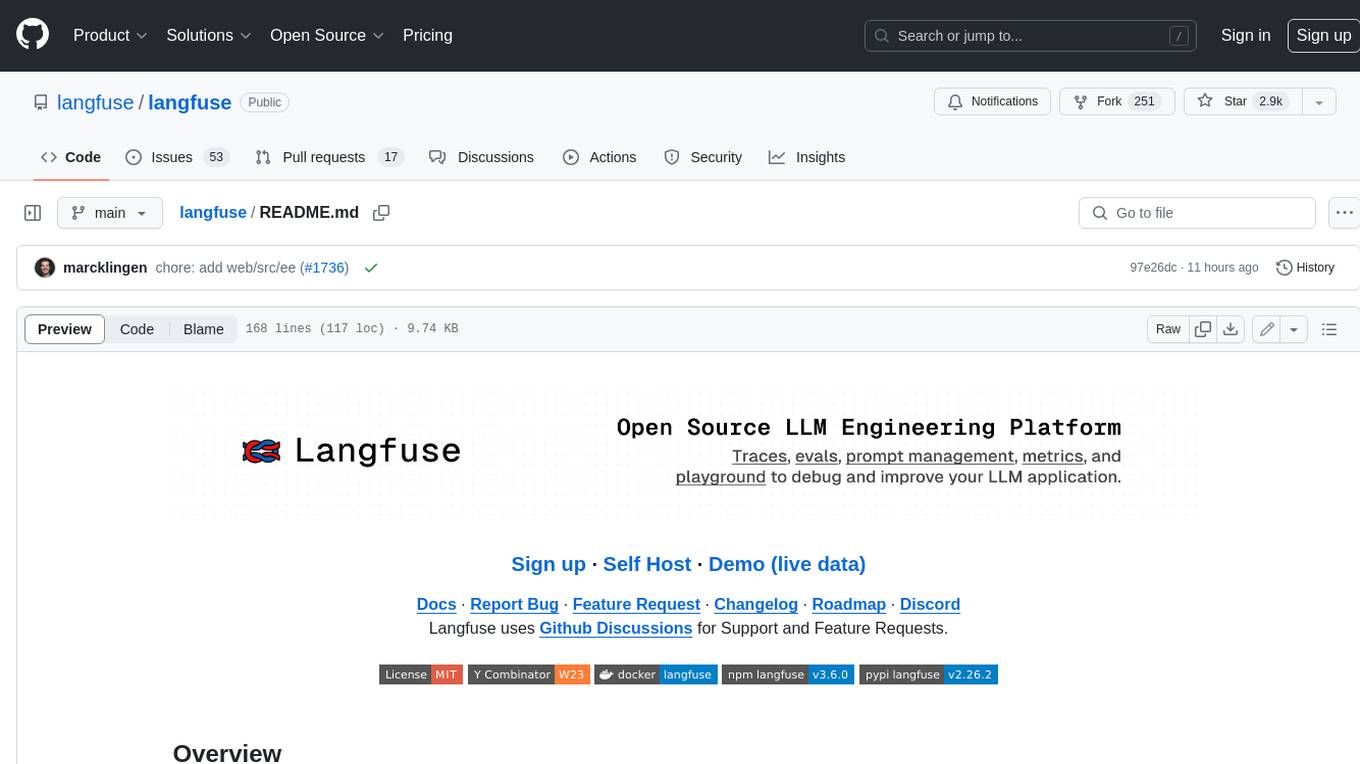
langfuse
Langfuse is a powerful tool that helps you develop, monitor, and test your LLM applications. With Langfuse, you can: * **Develop:** Instrument your app and start ingesting traces to Langfuse, inspect and debug complex logs, and manage, version, and deploy prompts from within Langfuse. * **Monitor:** Track metrics (cost, latency, quality) and gain insights from dashboards & data exports, collect and calculate scores for your LLM completions, run model-based evaluations, collect user feedback, and manually score observations in Langfuse. * **Test:** Track and test app behaviour before deploying a new version, test expected in and output pairs and benchmark performance before deploying, and track versions and releases in your application. Langfuse is easy to get started with and offers a generous free tier. You can sign up for Langfuse Cloud or deploy Langfuse locally or on your own infrastructure. Langfuse also offers a variety of integrations to make it easy to connect to your LLM applications.
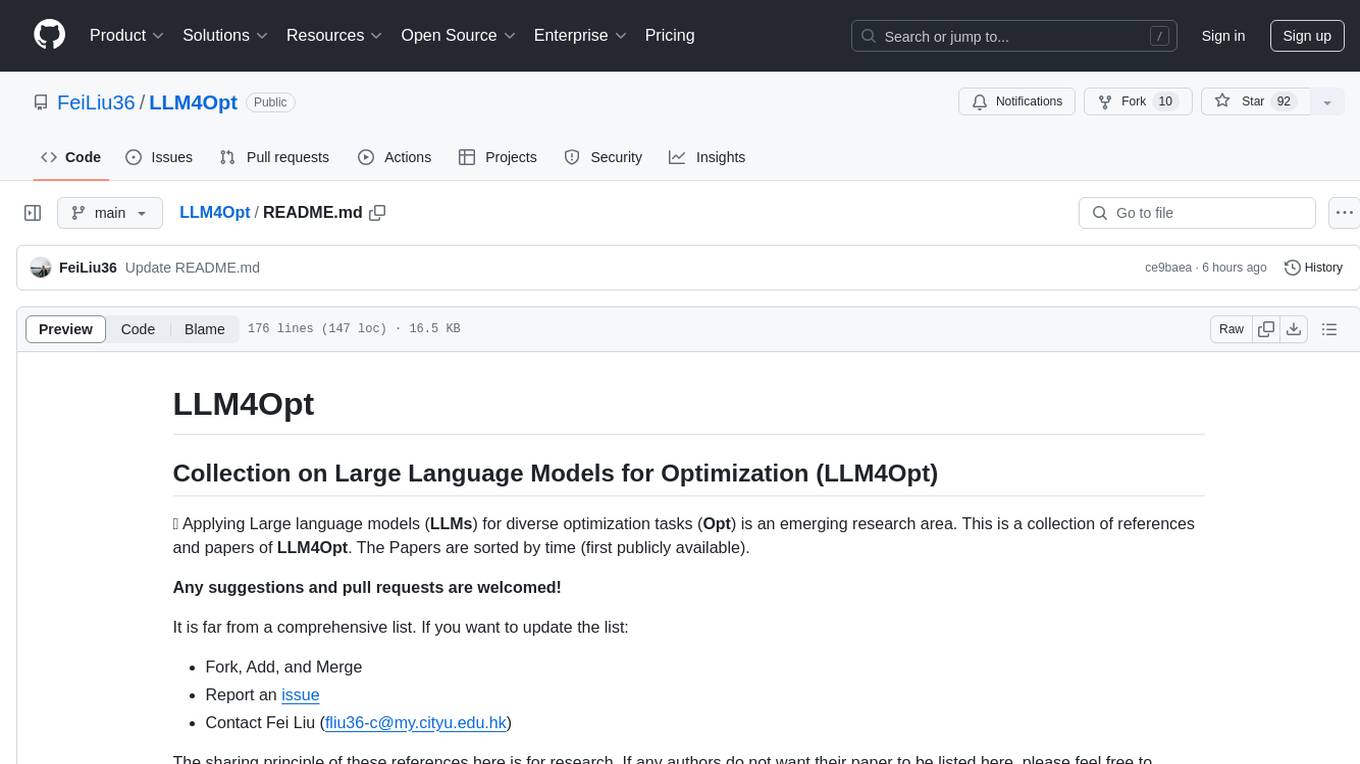
LLM4Opt
LLM4Opt is a collection of references and papers focusing on applying Large Language Models (LLMs) for diverse optimization tasks. The repository includes research papers, tutorials, workshops, competitions, and related collections related to LLMs in optimization. It covers a wide range of topics such as algorithm search, code generation, machine learning, science, industry, and more. The goal is to provide a comprehensive resource for researchers and practitioners interested in leveraging LLMs for optimization tasks.
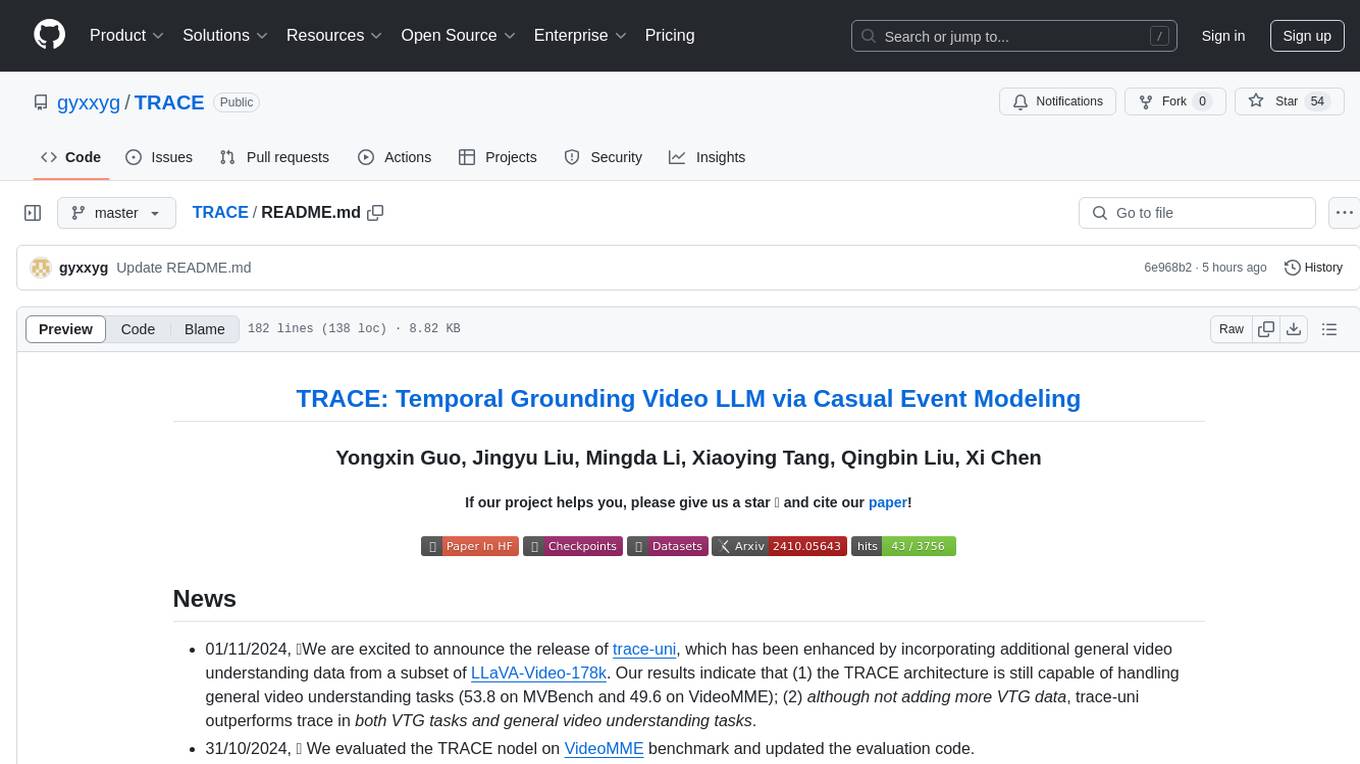
TRACE
TRACE is a temporal grounding video model that utilizes causal event modeling to capture videos' inherent structure. It presents a task-interleaved video LLM model tailored for sequential encoding/decoding of timestamps, salient scores, and textual captions. The project includes various model checkpoints for different stages and fine-tuning on specific datasets. It provides evaluation codes for different tasks like VTG, MVBench, and VideoMME. The repository also offers annotation files and links to raw videos preparation projects. Users can train the model on different tasks and evaluate the performance based on metrics like CIDER, METEOR, SODA_c, F1, mAP, Hit@1, etc. TRACE has been enhanced with trace-retrieval and trace-uni models, showing improved performance on dense video captioning and general video understanding tasks.
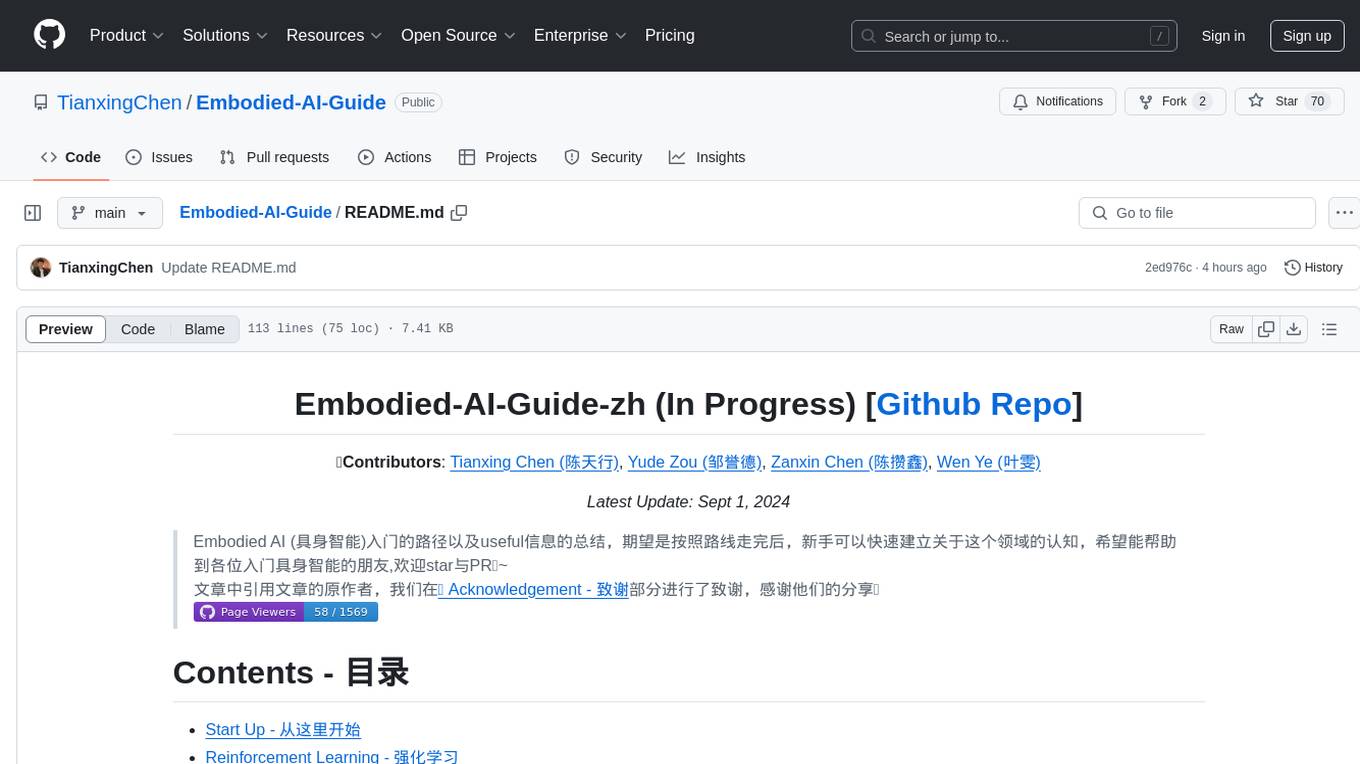
Embodied-AI-Guide
Embodied-AI-Guide is a comprehensive guide for beginners to understand Embodied AI, focusing on the path of entry and useful information in the field. It covers topics such as Reinforcement Learning, Imitation Learning, Large Language Model for Robotics, 3D Vision, Control, Benchmarks, and provides resources for building cognitive understanding. The repository aims to help newcomers quickly establish knowledge in the field of Embodied AI.
For similar tasks
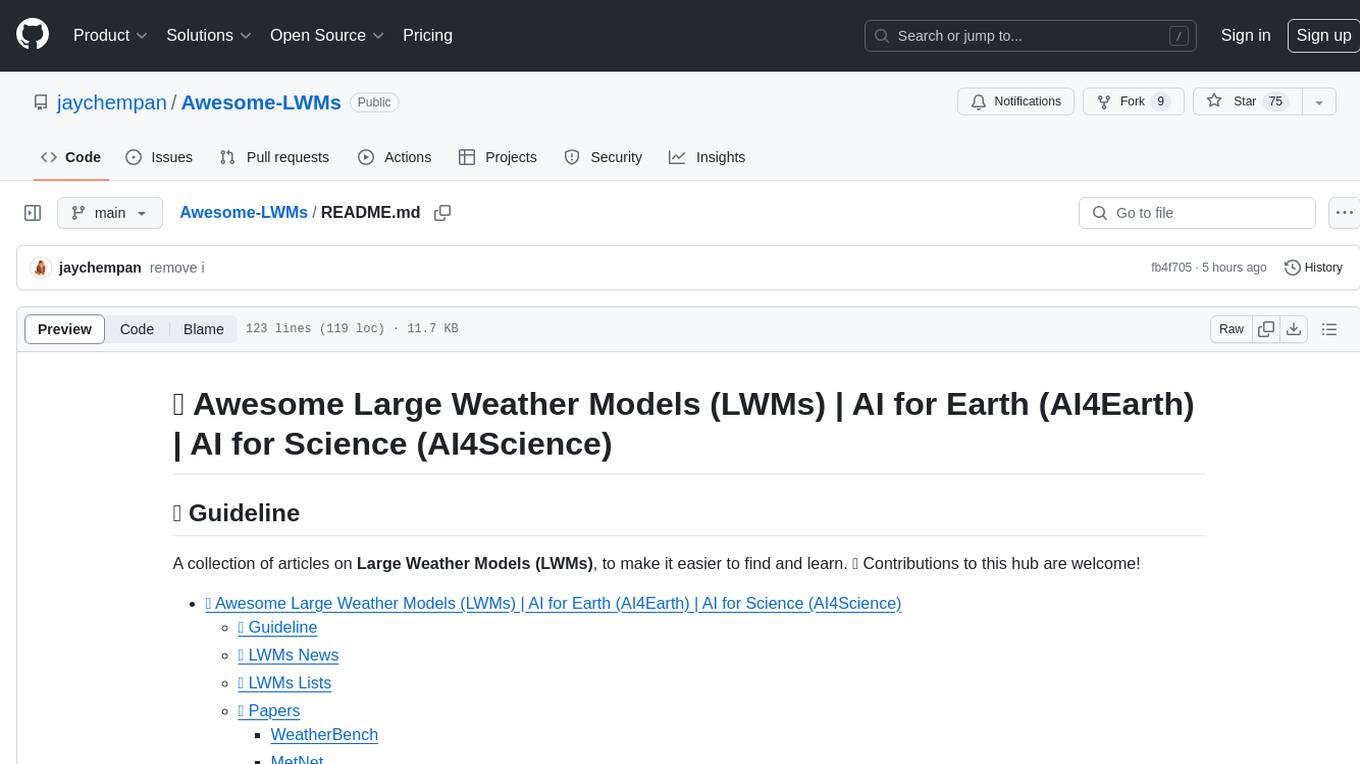
Awesome-LWMs
Awesome Large Weather Models (LWMs) is a curated collection of articles and resources related to large weather models used in AI for Earth and AI for Science. It includes information on various cutting-edge weather forecasting models, benchmark datasets, and research papers. The repository serves as a hub for researchers and enthusiasts to explore the latest advancements in weather modeling and forecasting.
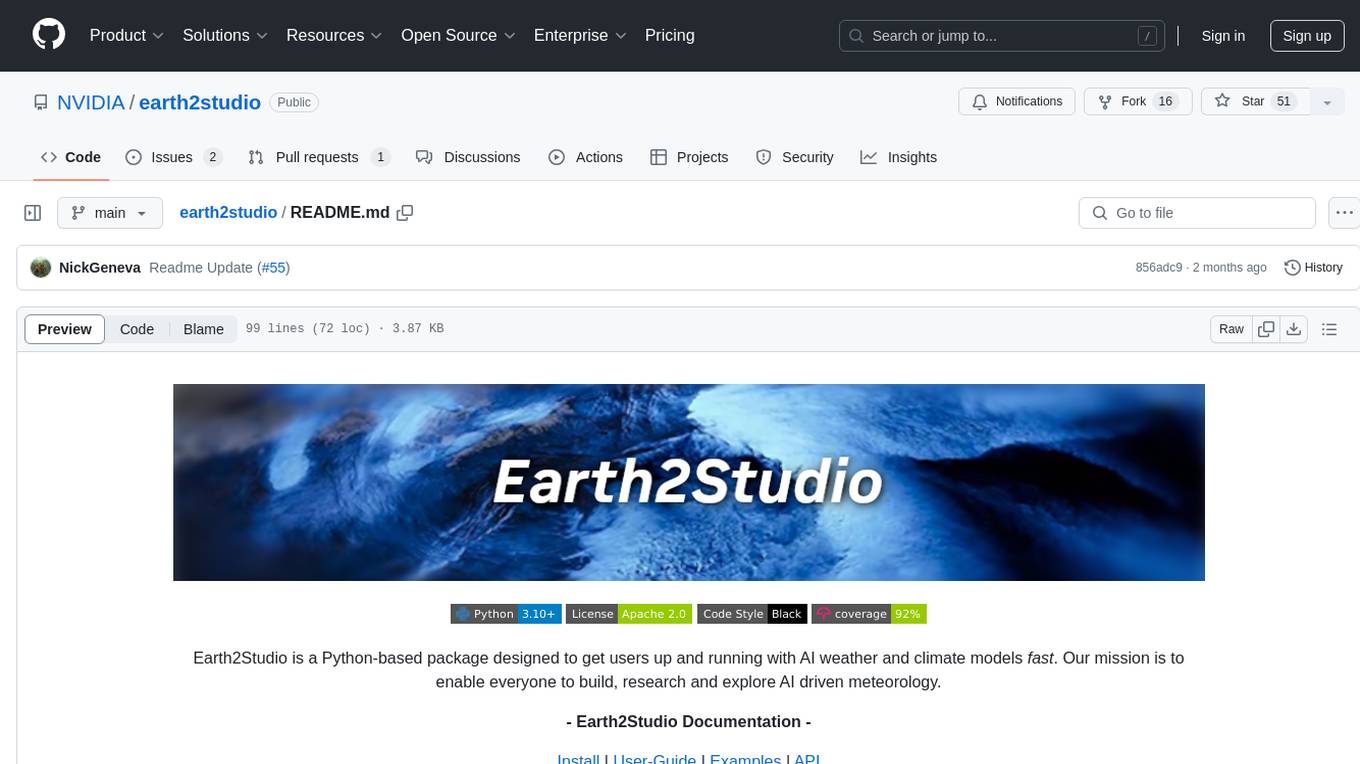
earth2studio
Earth2Studio is a Python-based package designed to enable users to quickly get started with AI weather and climate models. It provides access to pre-trained models, diagnostic tools, data sources, IO utilities, perturbation methods, and sample workflows for building custom weather prediction workflows. The package aims to empower users to explore AI-driven meteorology through modular components and seamless integration with other Nvidia packages like Modulus.
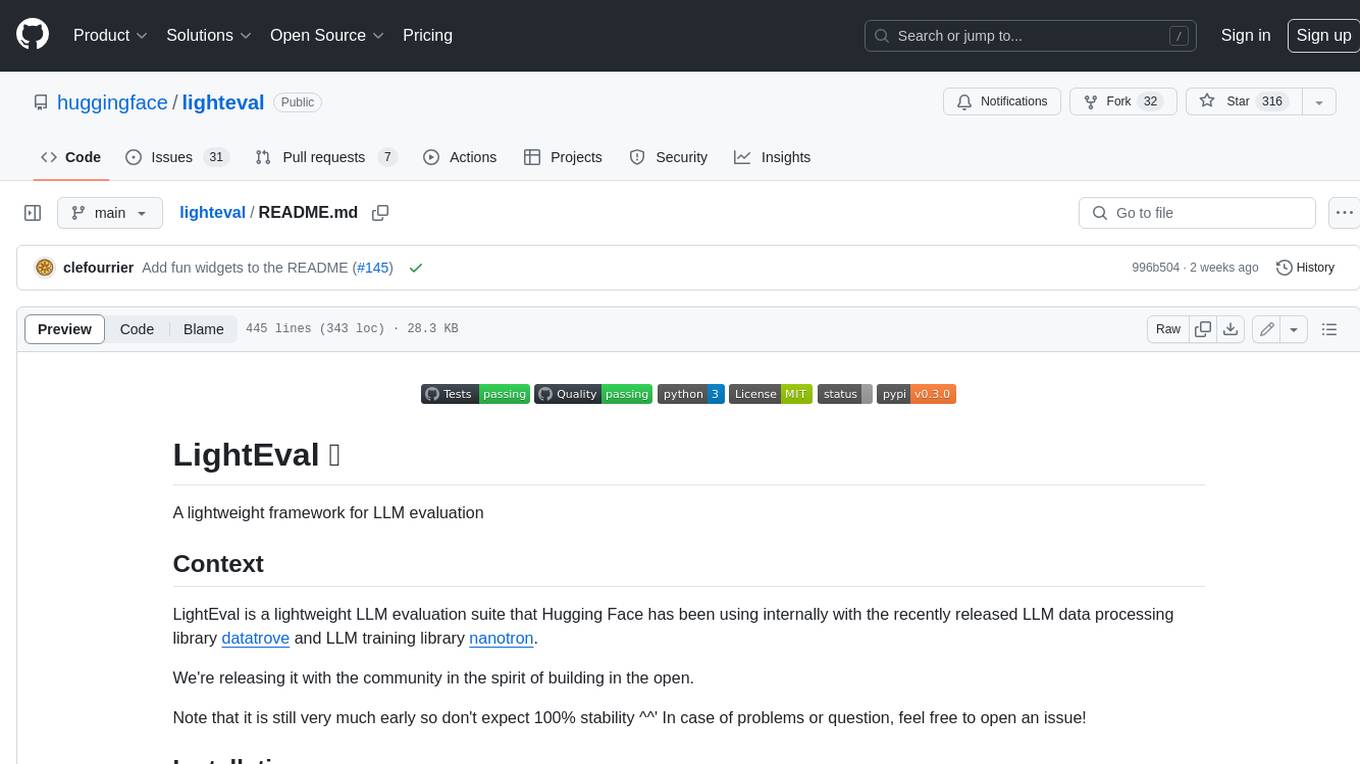
lighteval
LightEval is a lightweight LLM evaluation suite that Hugging Face has been using internally with the recently released LLM data processing library datatrove and LLM training library nanotron. We're releasing it with the community in the spirit of building in the open. Note that it is still very much early so don't expect 100% stability ^^' In case of problems or question, feel free to open an issue!
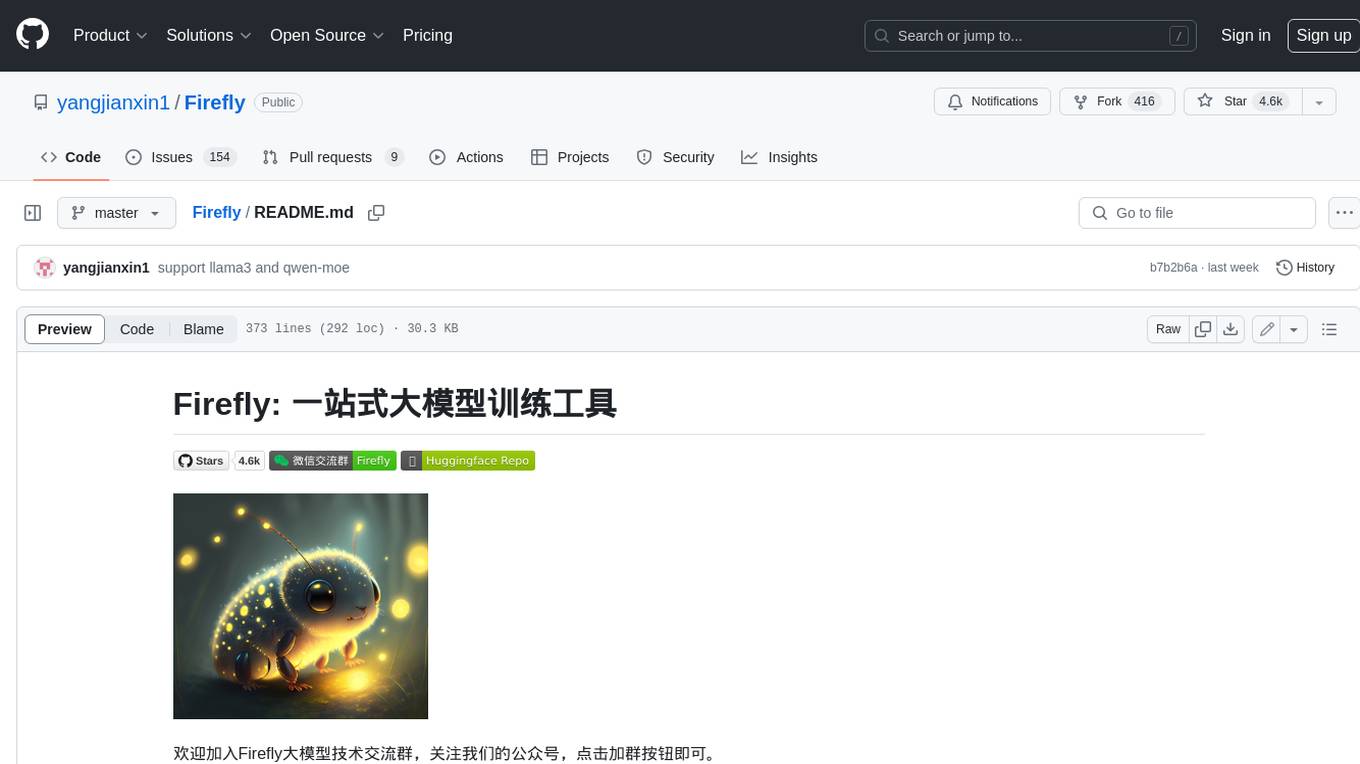
Firefly
Firefly is an open-source large model training project that supports pre-training, fine-tuning, and DPO of mainstream large models. It includes models like Llama3, Gemma, Qwen1.5, MiniCPM, Llama, InternLM, Baichuan, ChatGLM, Yi, Deepseek, Qwen, Orion, Ziya, Xverse, Mistral, Mixtral-8x7B, Zephyr, Vicuna, Bloom, etc. The project supports full-parameter training, LoRA, QLoRA efficient training, and various tasks such as pre-training, SFT, and DPO. Suitable for users with limited training resources, QLoRA is recommended for fine-tuning instructions. The project has achieved good results on the Open LLM Leaderboard with QLoRA training process validation. The latest version has significant updates and adaptations for different chat model templates.
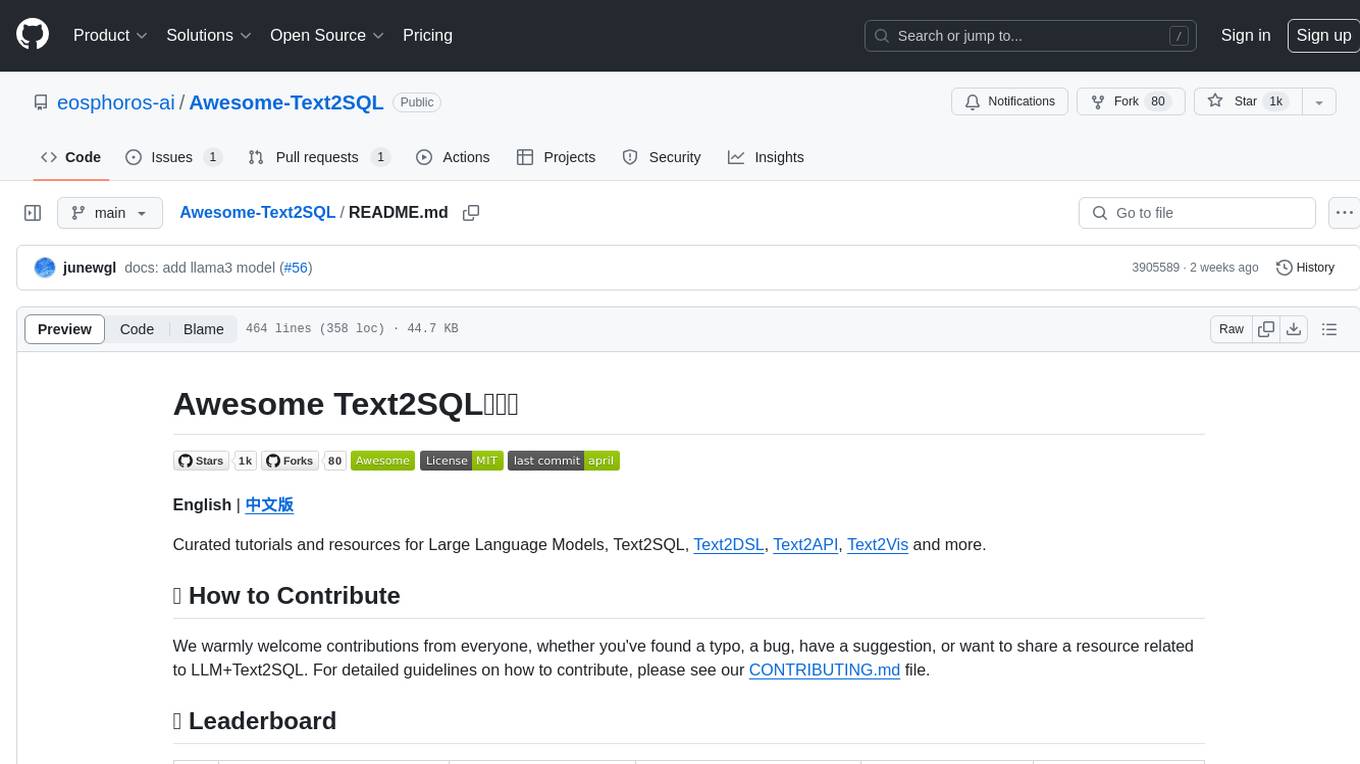
Awesome-Text2SQL
Awesome Text2SQL is a curated repository containing tutorials and resources for Large Language Models, Text2SQL, Text2DSL, Text2API, Text2Vis, and more. It provides guidelines on converting natural language questions into structured SQL queries, with a focus on NL2SQL. The repository includes information on various models, datasets, evaluation metrics, fine-tuning methods, libraries, and practice projects related to Text2SQL. It serves as a comprehensive resource for individuals interested in working with Text2SQL and related technologies.
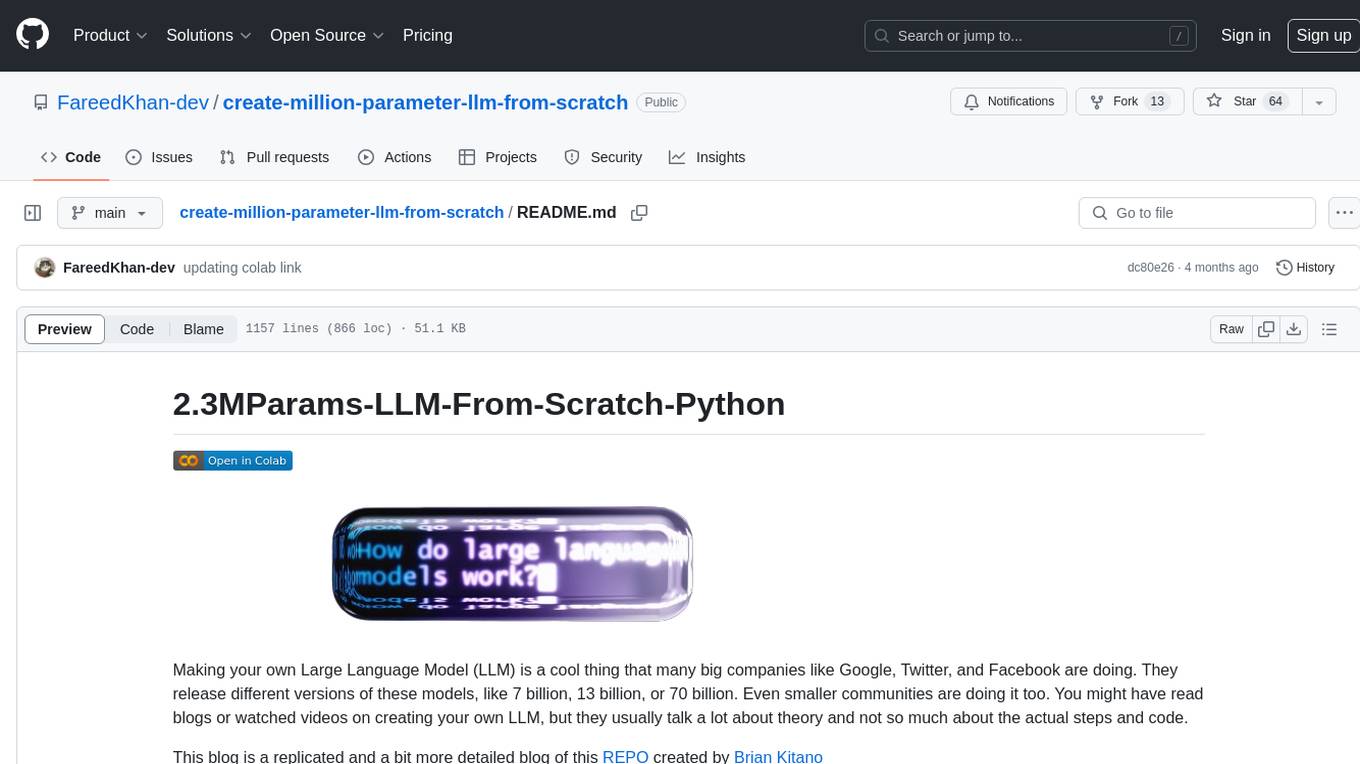
create-million-parameter-llm-from-scratch
The 'create-million-parameter-llm-from-scratch' repository provides a detailed guide on creating a Large Language Model (LLM) with 2.3 million parameters from scratch. The blog replicates the LLaMA approach, incorporating concepts like RMSNorm for pre-normalization, SwiGLU activation function, and Rotary Embeddings. The model is trained on a basic dataset to demonstrate the ease of creating a million-parameter LLM without the need for a high-end GPU.
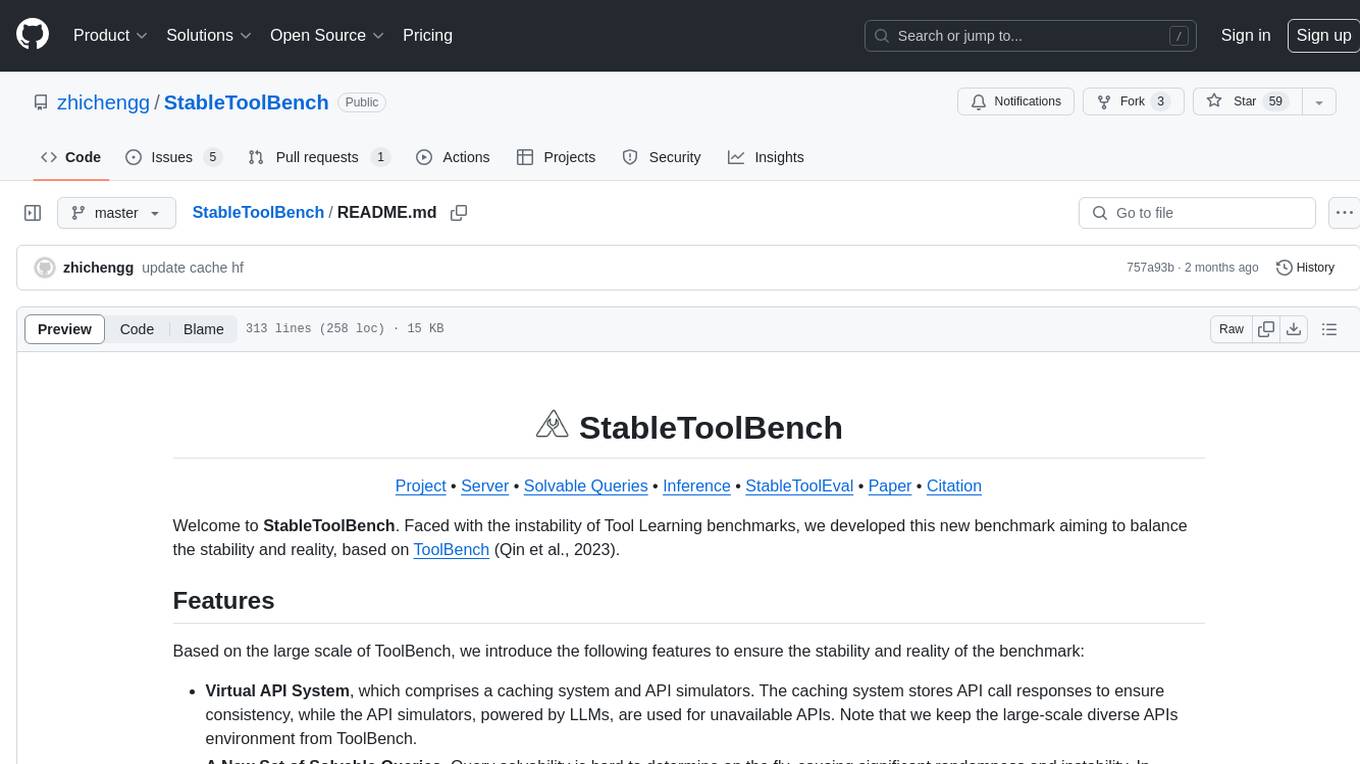
StableToolBench
StableToolBench is a new benchmark developed to address the instability of Tool Learning benchmarks. It aims to balance stability and reality by introducing features such as a Virtual API System with caching and API simulators, a new set of solvable queries determined by LLMs, and a Stable Evaluation System using GPT-4. The Virtual API Server can be set up either by building from source or using a prebuilt Docker image. Users can test the server using provided scripts and evaluate models with Solvable Pass Rate and Solvable Win Rate metrics. The tool also includes model experiments results comparing different models' performance.
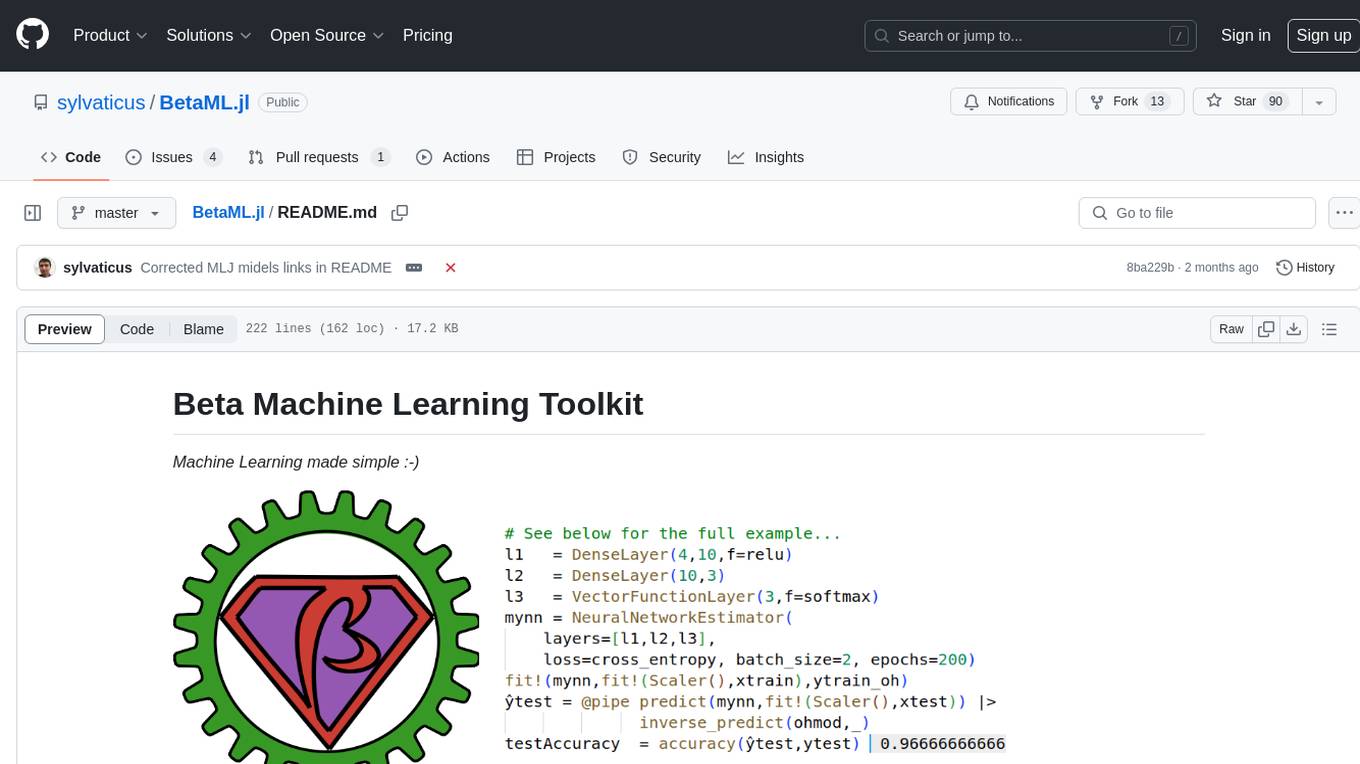
BetaML.jl
The Beta Machine Learning Toolkit is a package containing various algorithms and utilities for implementing machine learning workflows in multiple languages, including Julia, Python, and R. It offers a range of supervised and unsupervised models, data transformers, and assessment tools. The models are implemented entirely in Julia and are not wrappers for third-party models. Users can easily contribute new models or request implementations. The focus is on user-friendliness rather than computational efficiency, making it suitable for educational and research purposes.
For similar jobs
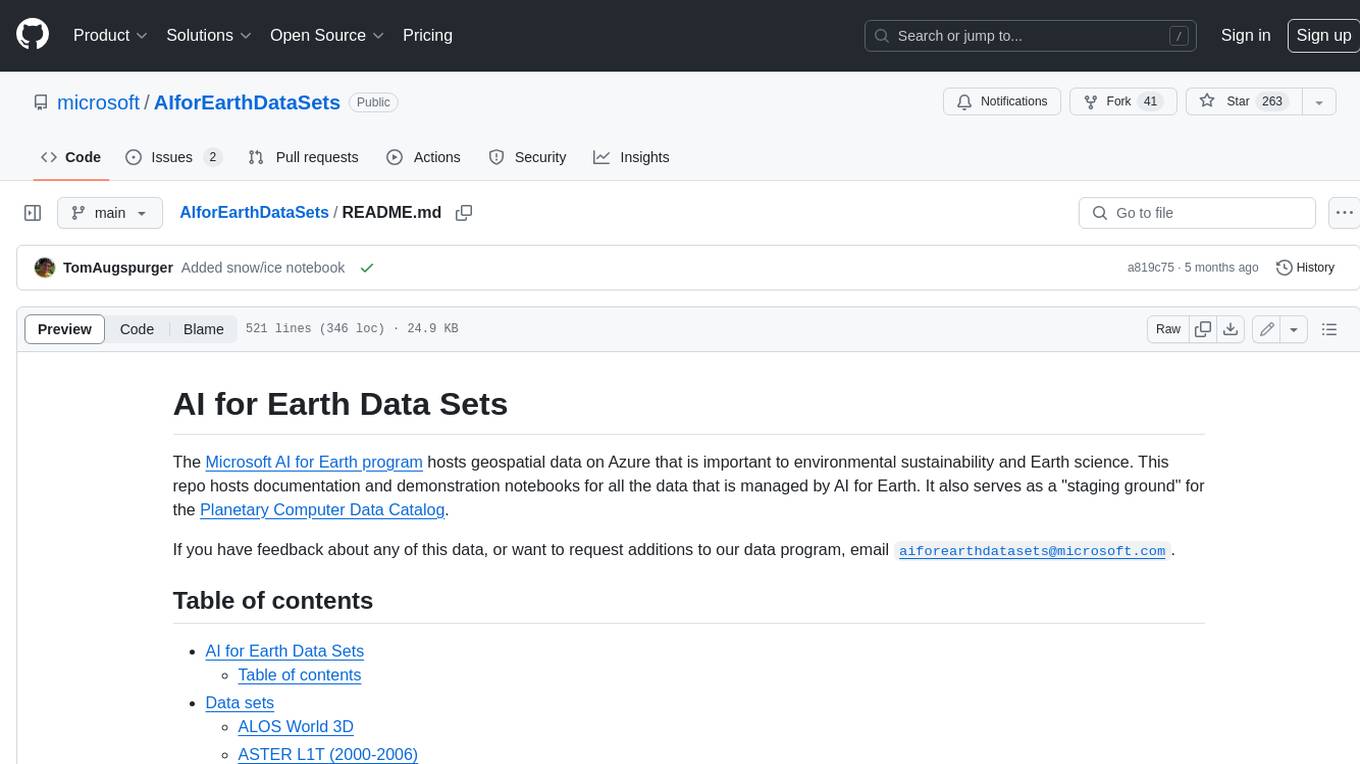
AIforEarthDataSets
The Microsoft AI for Earth program hosts geospatial data on Azure that is important to environmental sustainability and Earth science. This repo hosts documentation and demonstration notebooks for all the data that is managed by AI for Earth. It also serves as a "staging ground" for the Planetary Computer Data Catalog.
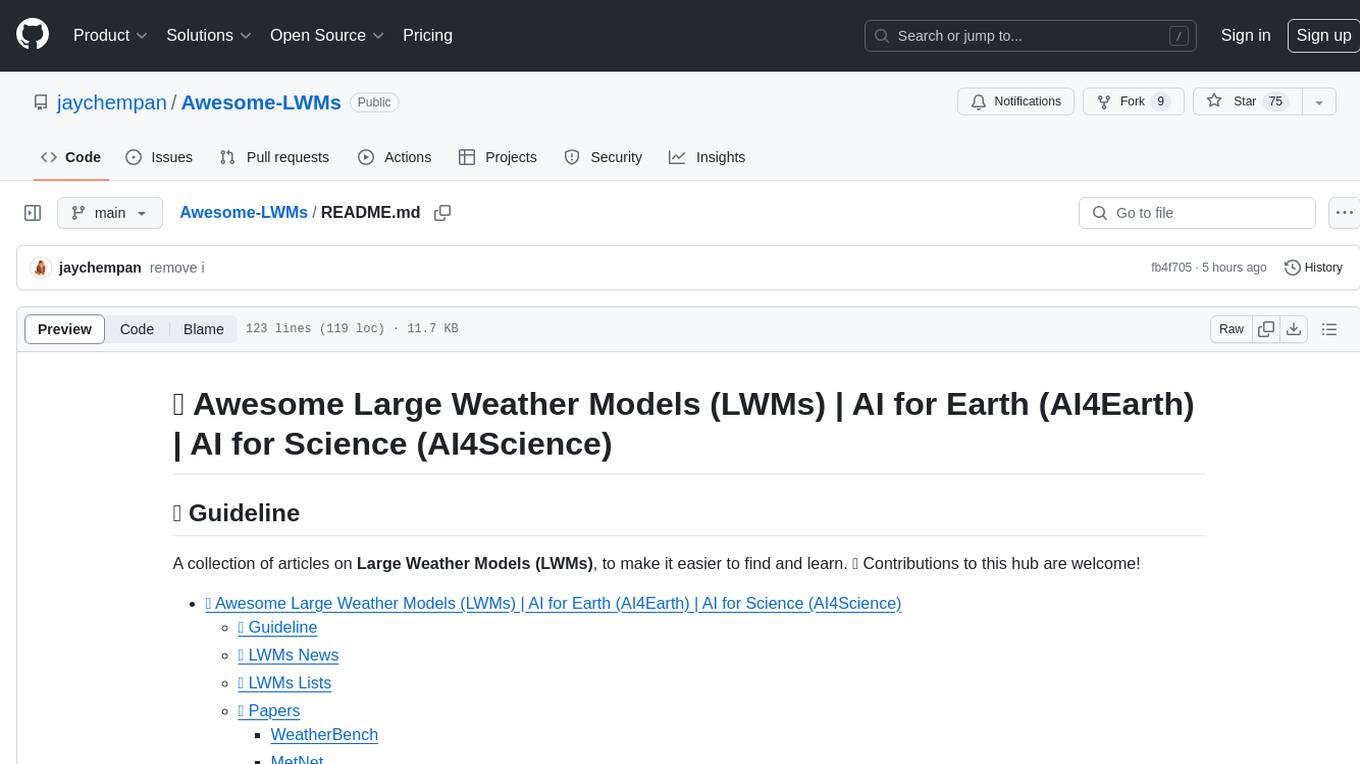
Awesome-LWMs
Awesome Large Weather Models (LWMs) is a curated collection of articles and resources related to large weather models used in AI for Earth and AI for Science. It includes information on various cutting-edge weather forecasting models, benchmark datasets, and research papers. The repository serves as a hub for researchers and enthusiasts to explore the latest advancements in weather modeling and forecasting.
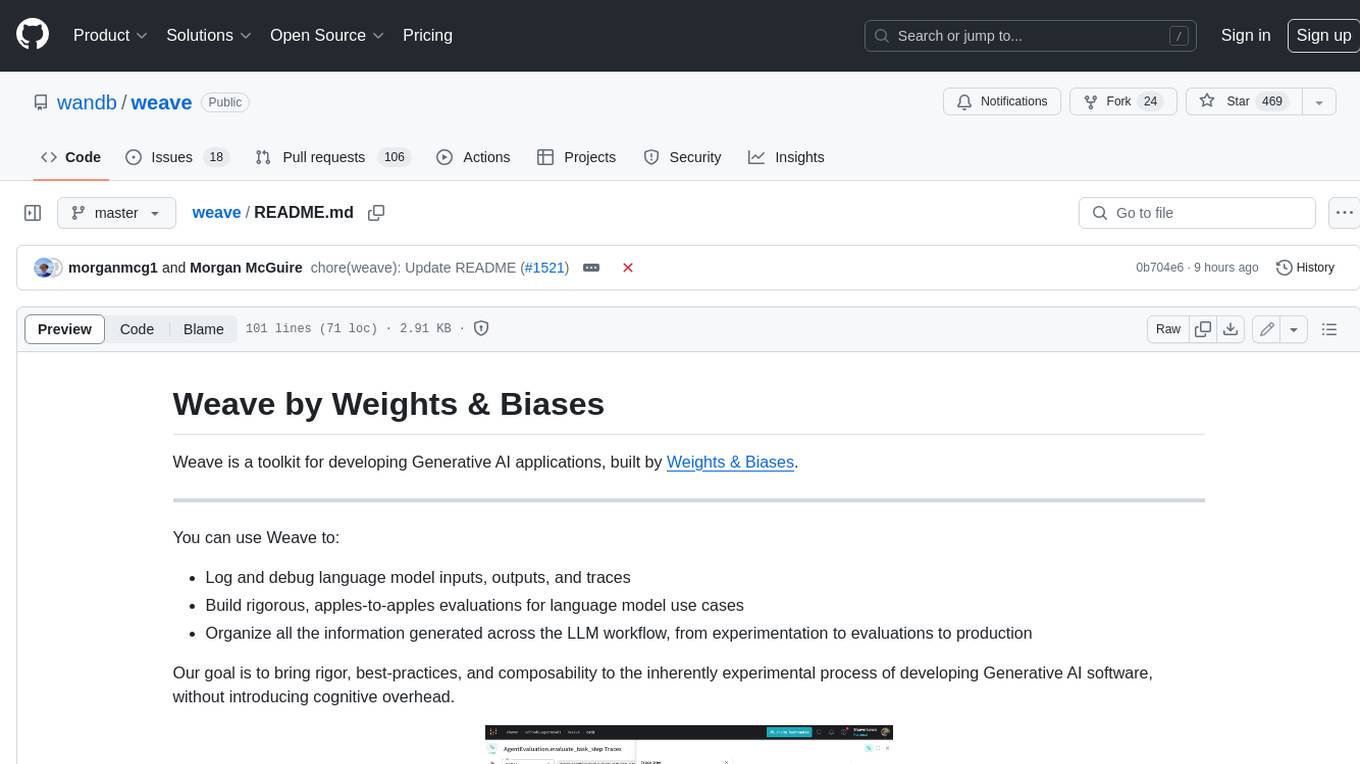
weave
Weave is a toolkit for developing Generative AI applications, built by Weights & Biases. With Weave, you can log and debug language model inputs, outputs, and traces; build rigorous, apples-to-apples evaluations for language model use cases; and organize all the information generated across the LLM workflow, from experimentation to evaluations to production. Weave aims to bring rigor, best-practices, and composability to the inherently experimental process of developing Generative AI software, without introducing cognitive overhead.
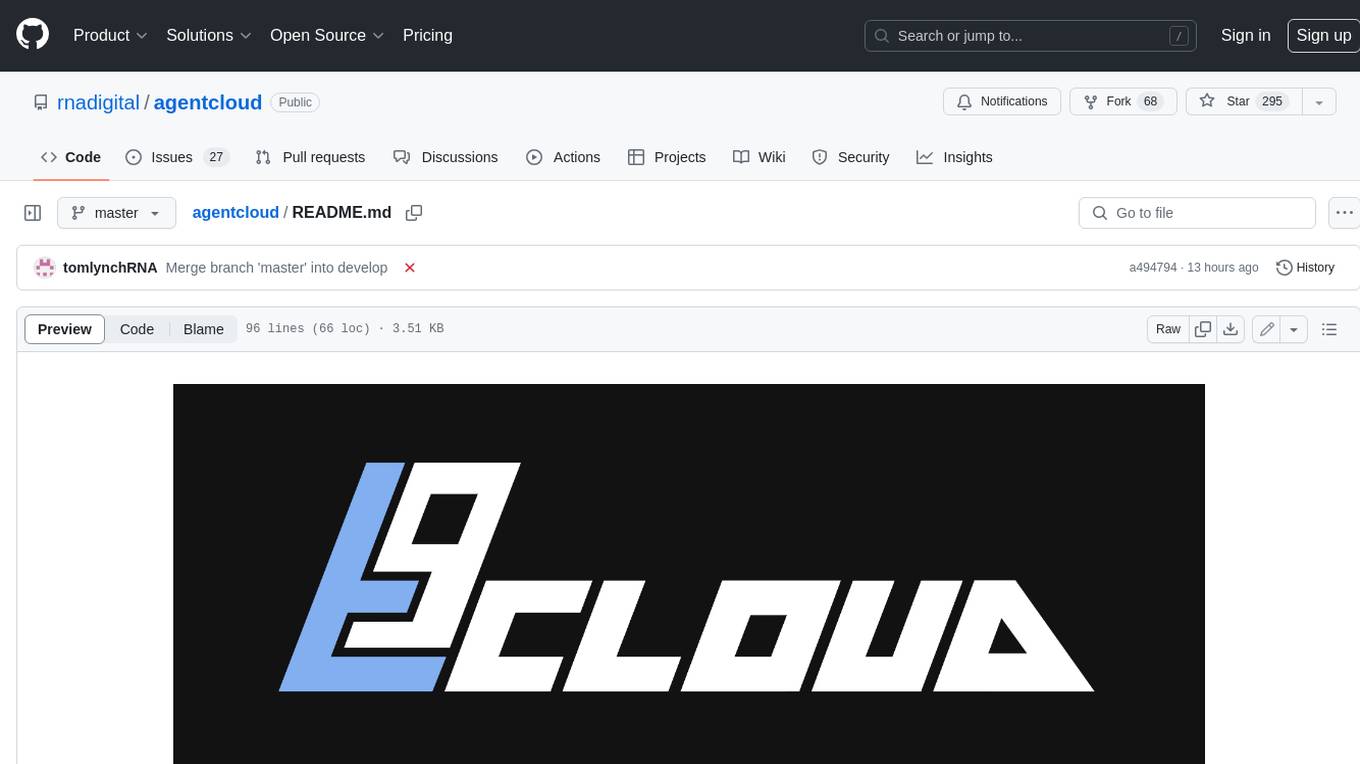
agentcloud
AgentCloud is an open-source platform that enables companies to build and deploy private LLM chat apps, empowering teams to securely interact with their data. It comprises three main components: Agent Backend, Webapp, and Vector Proxy. To run this project locally, clone the repository, install Docker, and start the services. The project is licensed under the GNU Affero General Public License, version 3 only. Contributions and feedback are welcome from the community.
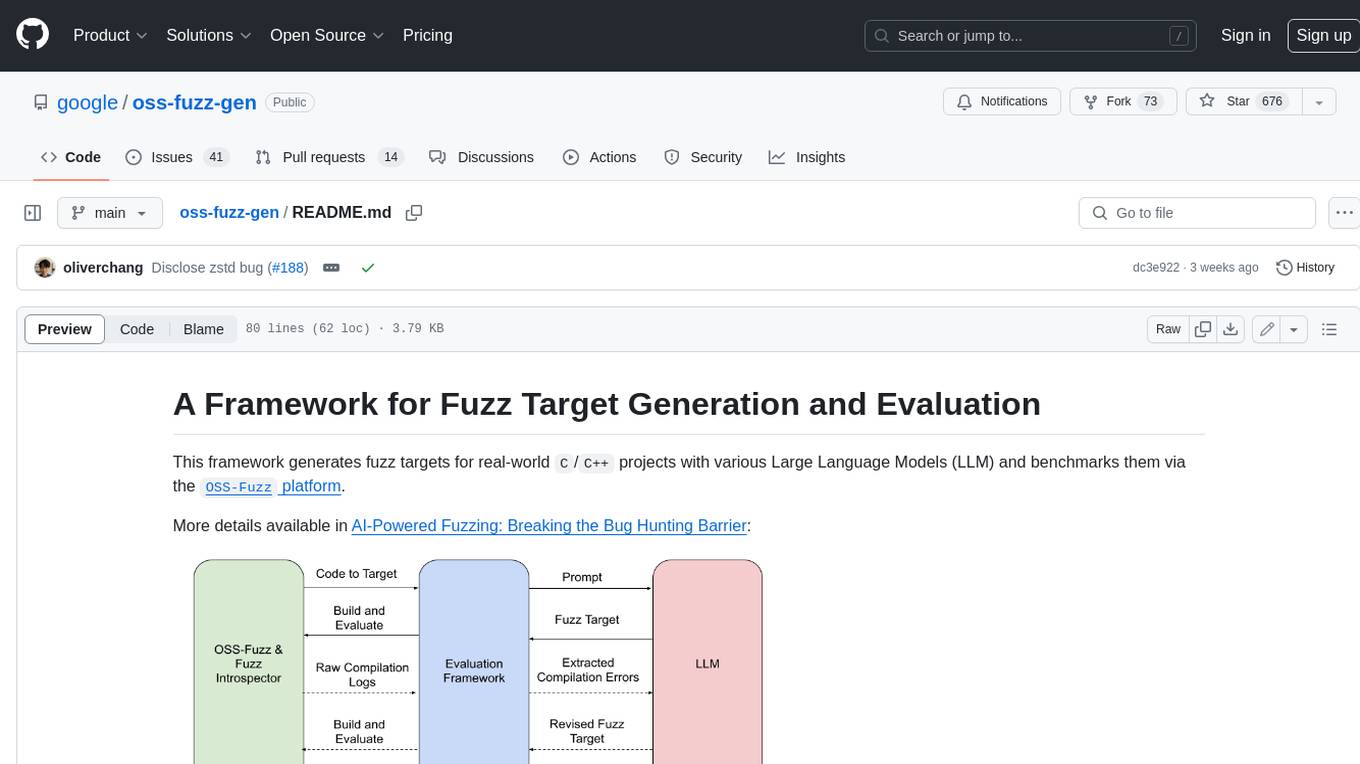
oss-fuzz-gen
This framework generates fuzz targets for real-world `C`/`C++` projects with various Large Language Models (LLM) and benchmarks them via the `OSS-Fuzz` platform. It manages to successfully leverage LLMs to generate valid fuzz targets (which generate non-zero coverage increase) for 160 C/C++ projects. The maximum line coverage increase is 29% from the existing human-written targets.
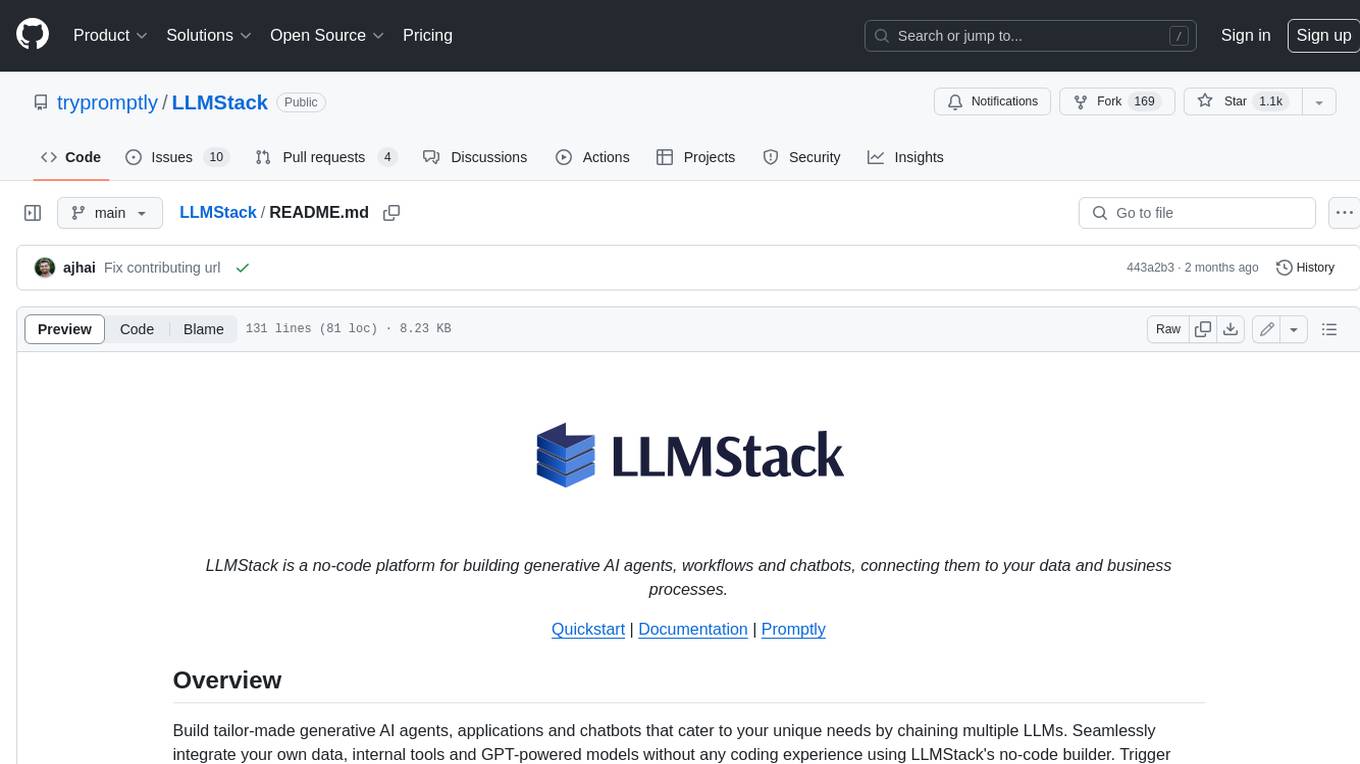
LLMStack
LLMStack is a no-code platform for building generative AI agents, workflows, and chatbots. It allows users to connect their own data, internal tools, and GPT-powered models without any coding experience. LLMStack can be deployed to the cloud or on-premise and can be accessed via HTTP API or triggered from Slack or Discord.
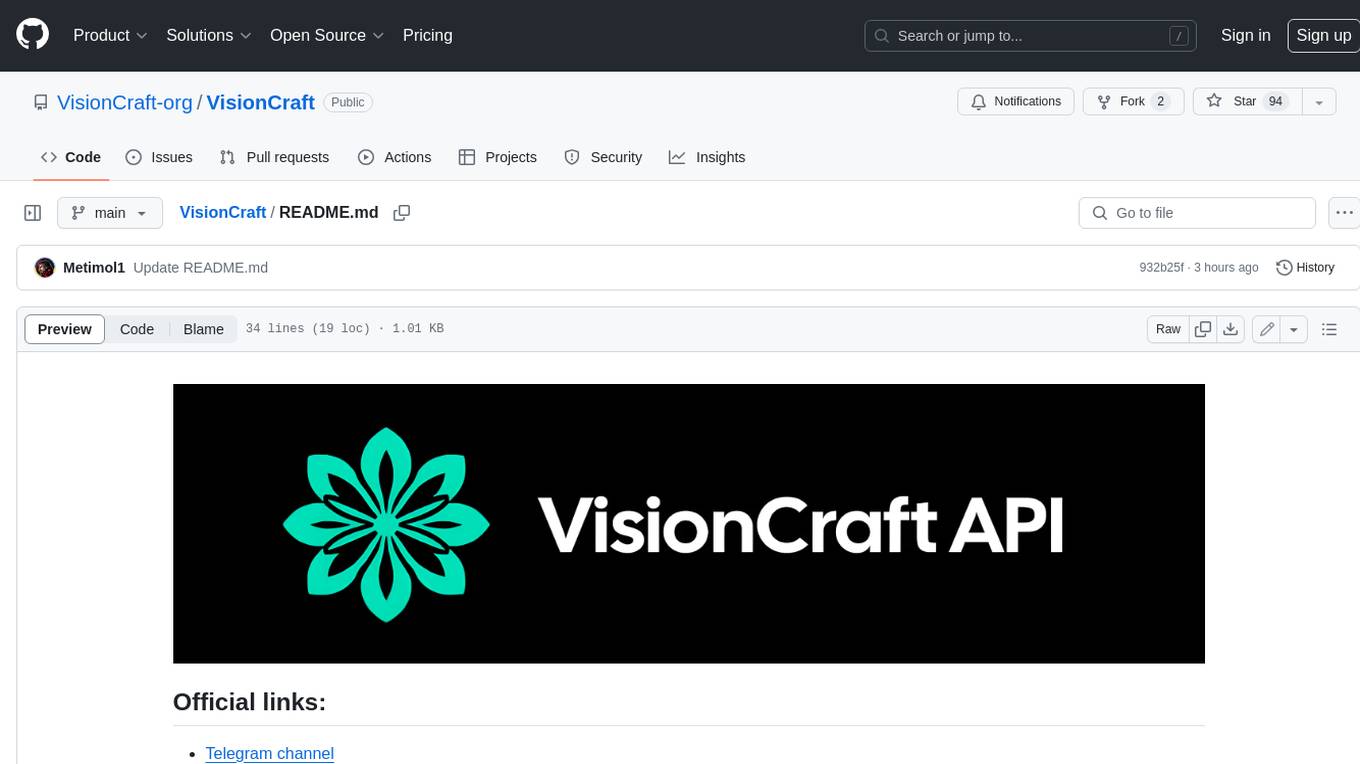
VisionCraft
The VisionCraft API is a free API for using over 100 different AI models. From images to sound.
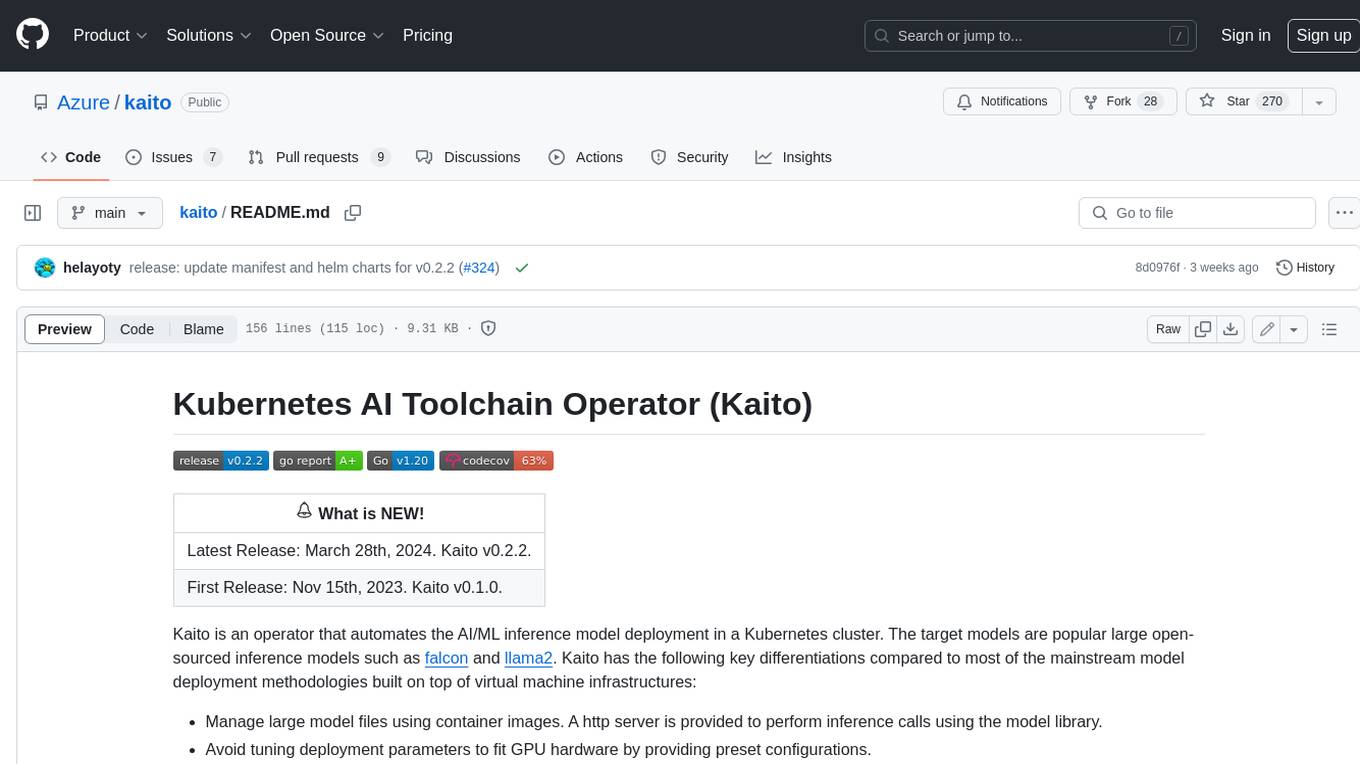
kaito
Kaito is an operator that automates the AI/ML inference model deployment in a Kubernetes cluster. It manages large model files using container images, avoids tuning deployment parameters to fit GPU hardware by providing preset configurations, auto-provisions GPU nodes based on model requirements, and hosts large model images in the public Microsoft Container Registry (MCR) if the license allows. Using Kaito, the workflow of onboarding large AI inference models in Kubernetes is largely simplified.