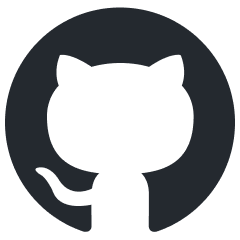
AIforEarthDataSets
Notebooks and documentation for AI-for-Earth-managed datasets on Azure
Stars: 263
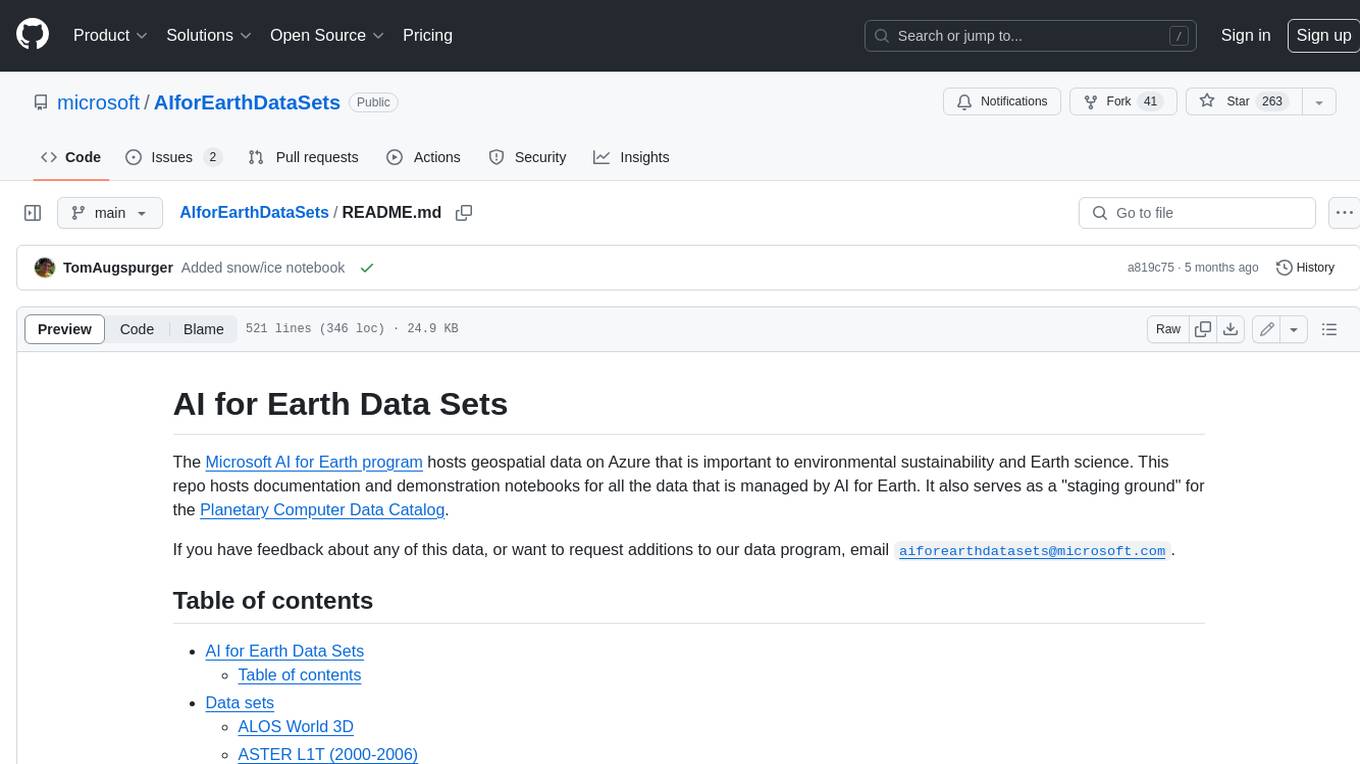
The Microsoft AI for Earth program hosts geospatial data on Azure that is important to environmental sustainability and Earth science. This repo hosts documentation and demonstration notebooks for all the data that is managed by AI for Earth. It also serves as a "staging ground" for the Planetary Computer Data Catalog.
README:
The Microsoft AI for Earth program hosts geospatial data on Azure that is important to environmental sustainability and Earth science. This repo hosts documentation and demonstration notebooks for all the data that is managed by AI for Earth. It also serves as a "staging ground" for the Planetary Computer Data Catalog.
If you have feedback about any of this data, or want to request additions to our data program, email [email protected]
.
- AI for Earth Data Sets
-
Data sets
- ALOS World 3D
- ASTER L1T (2000-2006)
- Copernicus DEM
- Daymet
- Deltares Global Flood Maps
- Deltares Global Water Availability
- Esri 10m Land Cover
- Global Biodiversity Information Facility (GBIF)
- Harmonized Global Biomass
- Harmonized Landsat Sentinel-2
- High Resolution Electricity Access (HREA)
- High Resolution Ocean Surface Wave Hindcast
- Labeled Information Library of Alexandria: Biology and Conservation (LILA BC)
- Landsat TM/MSS Collection 2
- Landsat 7 Collection 2 Level-2
- Landsat 8 Collection 2 Level-2
- MODIS (40 individual products)
- Monitoring Trends in Burn Severity Mosaics
- National Solar Radiation Database
- NASADEM
- NREL Puerto Rico 100 (PR100)
- NREL PV Rooftop Database
- NOAA Climate Data Records (CDR)
- NOAA Climate Forecast System (CFS)
- NOAA Digital Coast Imagery
- NOAA GFS Warm Start Initial Conditions
- NOAA GOES-R
- NOAA Global Ensemble Forecast System (GEFS)
- NOAA Global Forecast System (GFS)
- NOAA Global Hydro Estimator (GHE)
- NOAA High-Resolution Rapid Refresh (HRRR)
- NOAA Integrated Surface Data (ISD)
- NOAA Monthly US Climate Gridded Dataset (NClimGrid)
- NOAA National Water Model
- NOAA Rapid Refresh (RAP)
- NOAA US Climate Normals
- National Agriculture Imagery Program
- National Land Cover Database
- NatureServe Map of Biodiversity Importance (MoBI)
- Ocean Observatories Initiative CamHD
- Sentinel-1 GRD
- Sentinel-1 SLC
- Sentinel-2 L2A
- Sentinel-3 L2
- Sentinel-5P
- TerraClimate
- UK Met Office CSSP China 20CRDS
- UK Met Office Global Weather Data for COVID-19 Analysis
- University of Miami Coupled Model for Hurricanes Ike and Sandy
- USFS Forest Inventory and Analysis
- USGS 3DEP Seamless DEMs
- USGS Gap Land Cover
- Legal stuff
Global topographic information from the JAXA ALOS PRISM instrument.
The ASTER instrument, launched on-board NASA's Terra satellite in 1999, provides multispectral images of the Earth at 15m-90m resolution. This data set represents ASTER data from 2000-2006.
Global topographic information from the Copernicus program.
Estimates of daily weather parameters in North America on a one-kilometer grid, with monthly and annual summaries.
Global estimates of coastal inundation under various sea level rise conditions and return periods at 90m, 1km, and 5km resolutions. Also includes estimated coastal inundation caused by named historical storm events going back several decades.
Simulations of historical daily reservoir variations for 3,236 locations across the globe for the period 1970-2020 using the distributed wflow_sbm model. The model outputs long-term daily information on reservoir volume, inflow and outflow dynamics, as well as information on upstream hydrological forcing.
Global estimates of 10-class land use/land cover (LULC) for 2020, derived from ESA Sentinel-2 imagery at 10m resolution, produced by Impact Observatory.
Exports of global species occurrence data from the GBIF network.
Global maps of aboveground and belowground biomass carbon density for the year 2010 at 300m resolution.
Satellite imagery from the Landsat 8 and Sentinel-2 satellites, aligned to a common grid and processed to compatible color spaces.
Settlement-level measures of electricity access, reliability, and usage derived from VIIRS satellite imagery.
Long-term wave hindcast data for the U.S. Exclusive Economic Zone (EEZ), developed by the U.S. Department of Energy's Water Power Technologies Office.
AI for Earth and partners have assembled a repository of labeled information related to wildlife conservation, particularly wildlife imagery.
Global optical imagery from the Landsat MSS and TM instruments, which imaged the Earth from 1972 to 2013, aboard the Landsat 1-5 satellites.
Landsat TM/MSS data are in preview; access is granted by request.
Global optical imagery from the Landsat 7 satellite, which has imaged the Earth since 1999.
Landsat 7 data are in preview; access is granted by request.
Global optical imagery from the Landsat 8 satellite, which has imaged the Earth since 2013.
Satellite imagery from the Moderate Resolution Imaging Spectroradiometer (MODIS).
Annual burn severity mosaics for the continental United States and Alaska.
Hourly and half-hourly values of the three most common measurements of solar radiation – global horizontal, direct normal, and diffuse horizontal irradiance - along with meteorological data.
Global topographic information from the NASADEM program.
A collection of geospasial data useful for renewable energy development in Puerto Rico. The dataset is curated by the National Renewable Energy Laboratory.
A lidar-derived, geospatially-resolved dataset of suitable roof surfaces and their PV technical potential for 128 metropolitan regions in the United States.
Historical global climate information.
Model output data from the NOAA NCEP Climate Forecast System Version 2.
High resolution (1 meter or less) imagery collected by a number of sources and contributed to the NOAA Digital Coast
Warm start initial conditions for the NOAA Global Forecast System.
Weather imagery from the GOES-16, GOES-17, and GOES-18 satellites.
Model output data from the NOAA Global Ensemble Forecast System.
Model output data from the NOAA Global Forecast System.
Global rainfall estimates in 15-minute intervals.
Weather forecasts for North America at 3km spatial resolution and 15 minute temporal resolution.
Historical global weather information.
Gridded climate data for the US from 1895 to the present.
Data from the National Water Model.
Weather forecasts for North America at 13km resolution.
Typical climate conditions for the United States from 1981 to the present.
NAIP provides US-wide, high-resolution aerial imagery. This data set includes NAIP images from 2010 to the present.
US-wide data on land cover and land cover change at a 30m resolution with a 16-class legend.
Habitat information for 2,216 imperiled species occurring in the conterminous United States.
Video data from the Ocean Observatories Initiative seafloor camera deployed at Axial Volcano on the Juan de Fuca Ridge.
Global synthetic aperture radar (SAR) data from 2017-present, projected to ground range.
Sentinel-1 GRD data are in preview; access is granted by request.
Global synthetic aperture radar (SAR) data for the last 90 days.
Sentinel-1 SLC data are in preview; access is granted by request.
Global optical imagery at 10m resolution from 2016-present.
Global multispectral imagery at 300m resolution, with a revisit rate of less than two days, from 2016-present.
Sentinel-3 data are in preview; access is granted by request.
Global atmospheric data from 2018-present.
Sentinel-5P data are in preview; access is granted by request.
Monthly climate and climatic water balance for global terrestrial surfaces from 1958-2019.
Historical climate data for China, from 1851-2010.
Data for COVID-19 researchers exploring relationships between COVID-19 and environmental factors.
Modeled wind, wave, and current data for Hurricanes Ike and Sandy, produced by the National Renewable Energy Laboratory.
Status and trends on U.S. forest location, health, growth, mortality, and production, from the US Forest Service's Forest Inventory and Analysis (FIA) program.
This project welcomes contributions and suggestions. Most contributions require you to agree to a Contributor License Agreement (CLA) declaring that you have the right to, and actually do, grant us the rights to use your contribution. For details, visit https://cla.opensource.microsoft.com.
When you submit a pull request, a CLA bot will automatically determine whether you need to provide a CLA and decorate the PR appropriately (e.g., status check, comment). Simply follow the instructions provided by the bot. You will only need to do this once across all repos using our CLA.
This project has adopted the Microsoft Open Source Code of Conduct. For more information see the Code of Conduct FAQ or contact [email protected] with any additional questions or comments.
This project may contain trademarks or logos for projects, products, or services. Authorized use of Microsoft trademarks or logos is subject to and must follow Microsoft's Trademark & Brand Guidelines. Use of Microsoft trademarks or logos in modified versions of this project must not cause confusion or imply Microsoft sponsorship. Any use of third-party trademarks or logos are subject to those third-party's policies.
For Tasks:
Click tags to check more tools for each tasksFor Jobs:
Alternative AI tools for AIforEarthDataSets
Similar Open Source Tools
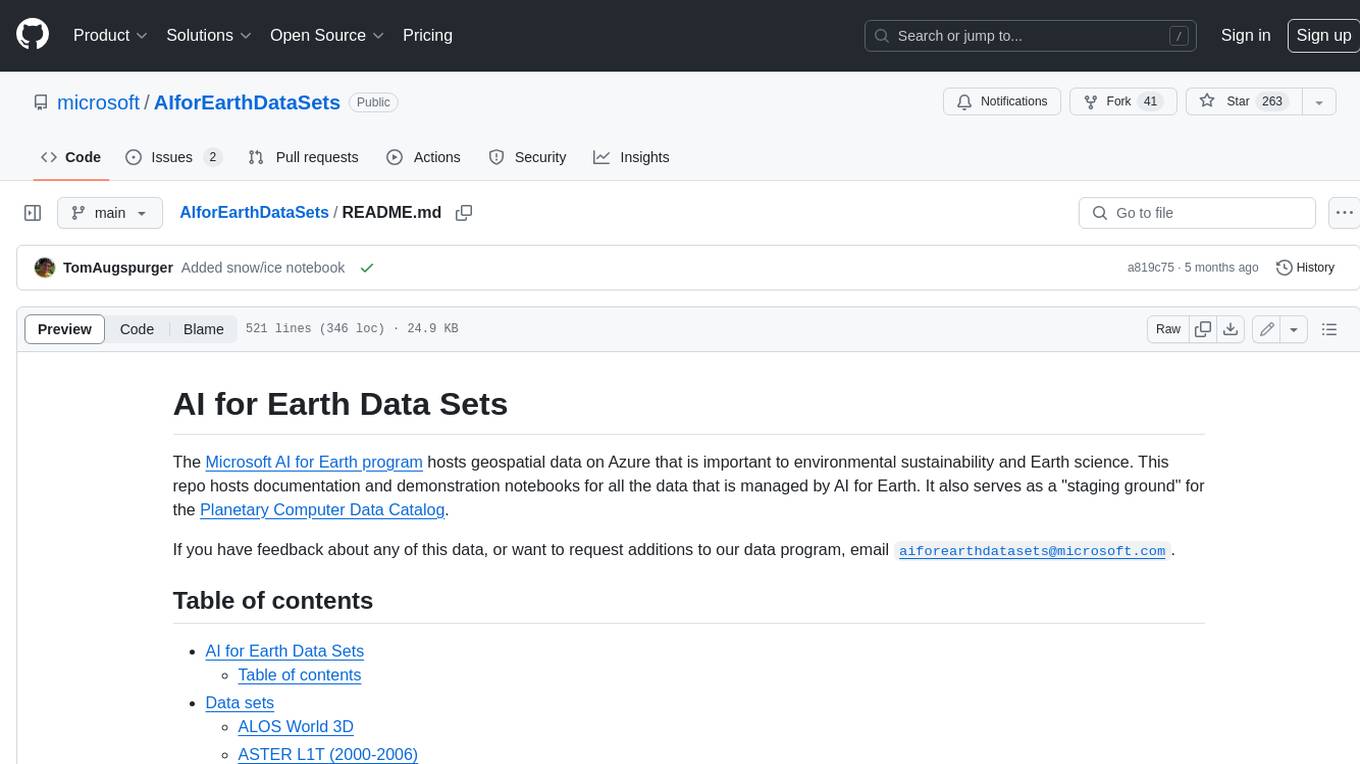
AIforEarthDataSets
The Microsoft AI for Earth program hosts geospatial data on Azure that is important to environmental sustainability and Earth science. This repo hosts documentation and demonstration notebooks for all the data that is managed by AI for Earth. It also serves as a "staging ground" for the Planetary Computer Data Catalog.
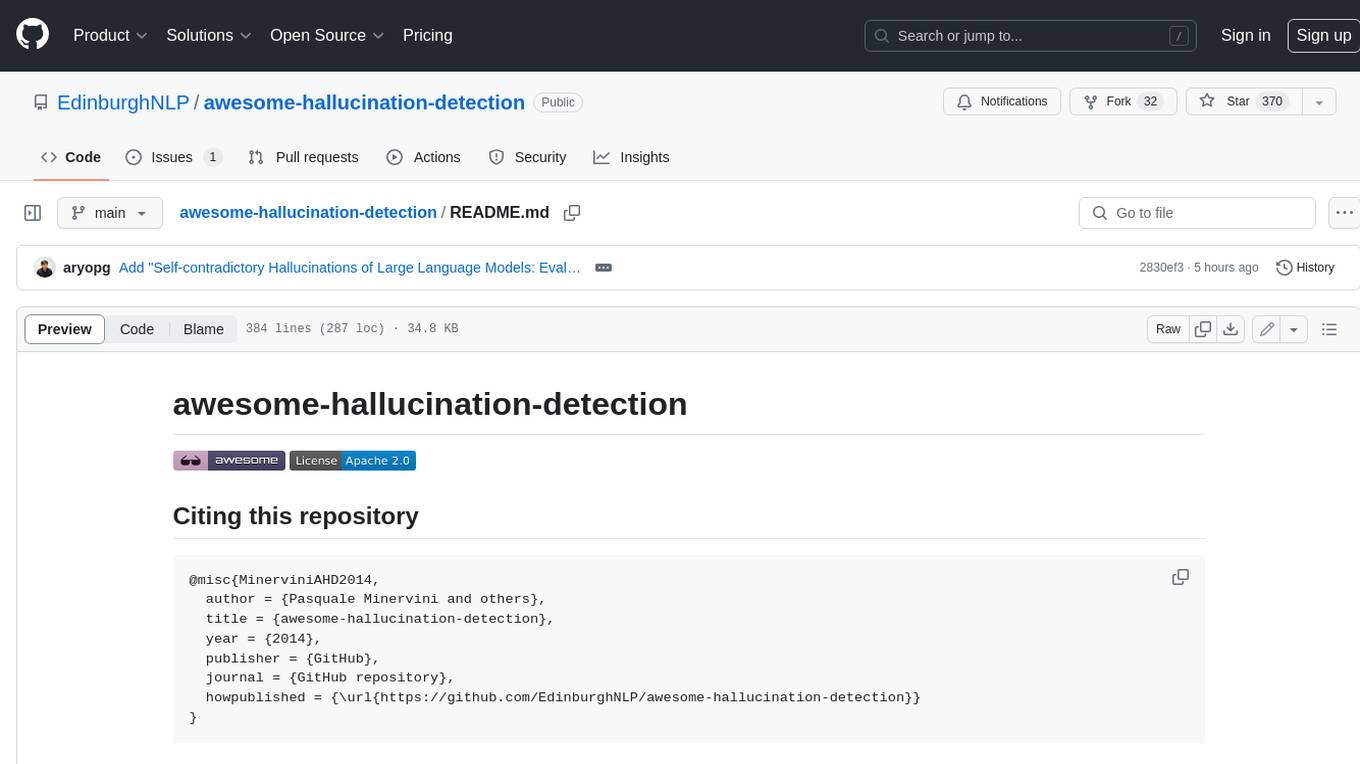
awesome-hallucination-detection
This repository provides a curated list of papers, datasets, and resources related to the detection and mitigation of hallucinations in large language models (LLMs). Hallucinations refer to the generation of factually incorrect or nonsensical text by LLMs, which can be a significant challenge for their use in real-world applications. The resources in this repository aim to help researchers and practitioners better understand and address this issue.
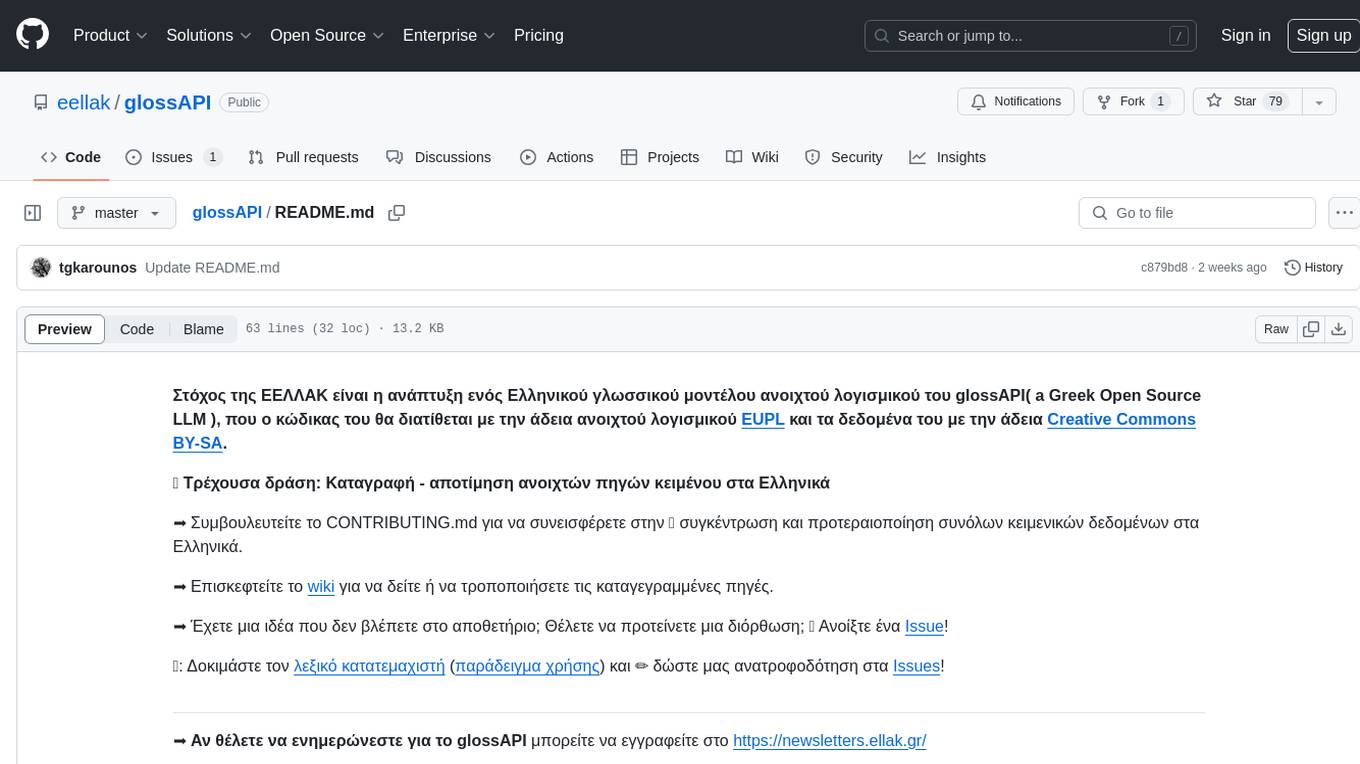
glossAPI
The glossAPI project aims to develop a Greek language model as open-source software, with code licensed under EUPL and data under Creative Commons BY-SA. The project focuses on collecting and evaluating open text sources in Greek, with efforts to prioritize and gather textual data sets. The project encourages contributions through the CONTRIBUTING.md file and provides resources in the wiki for viewing and modifying recorded sources. It also welcomes ideas and corrections through issue submissions. The project emphasizes the importance of open standards, ethically secured data, privacy protection, and addressing digital divides in the context of artificial intelligence and advanced language technologies.
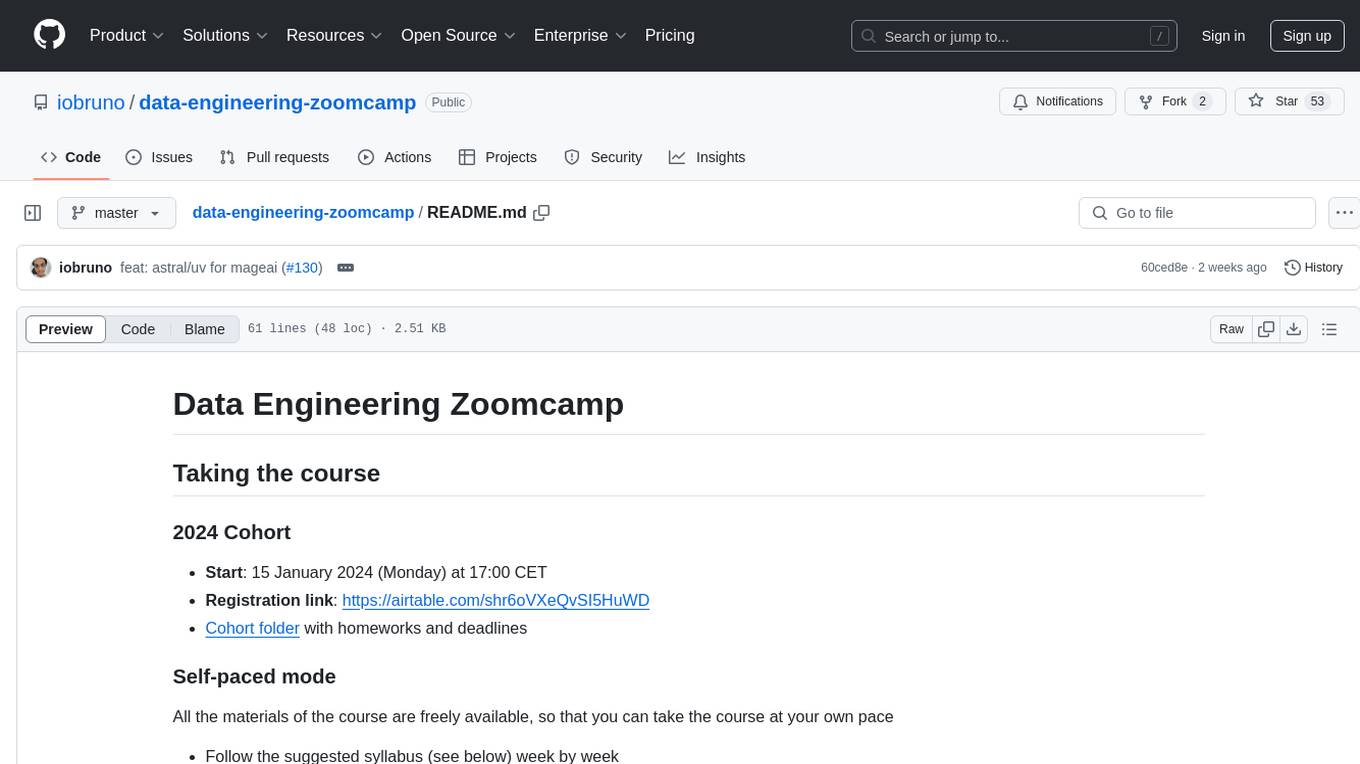
data-engineering-zoomcamp
Data Engineering Zoomcamp is a comprehensive course covering various aspects of data engineering, including data ingestion, workflow orchestration, data warehouse, analytics engineering, batch processing, and stream processing. The course provides hands-on experience with tools like Python, Rust, Terraform, Airflow, BigQuery, dbt, PySpark, Kafka, and more. Students will learn how to work with different data technologies to build scalable and efficient data pipelines for analytics and processing. The course is designed for individuals looking to enhance their data engineering skills and gain practical experience in working with big data technologies.
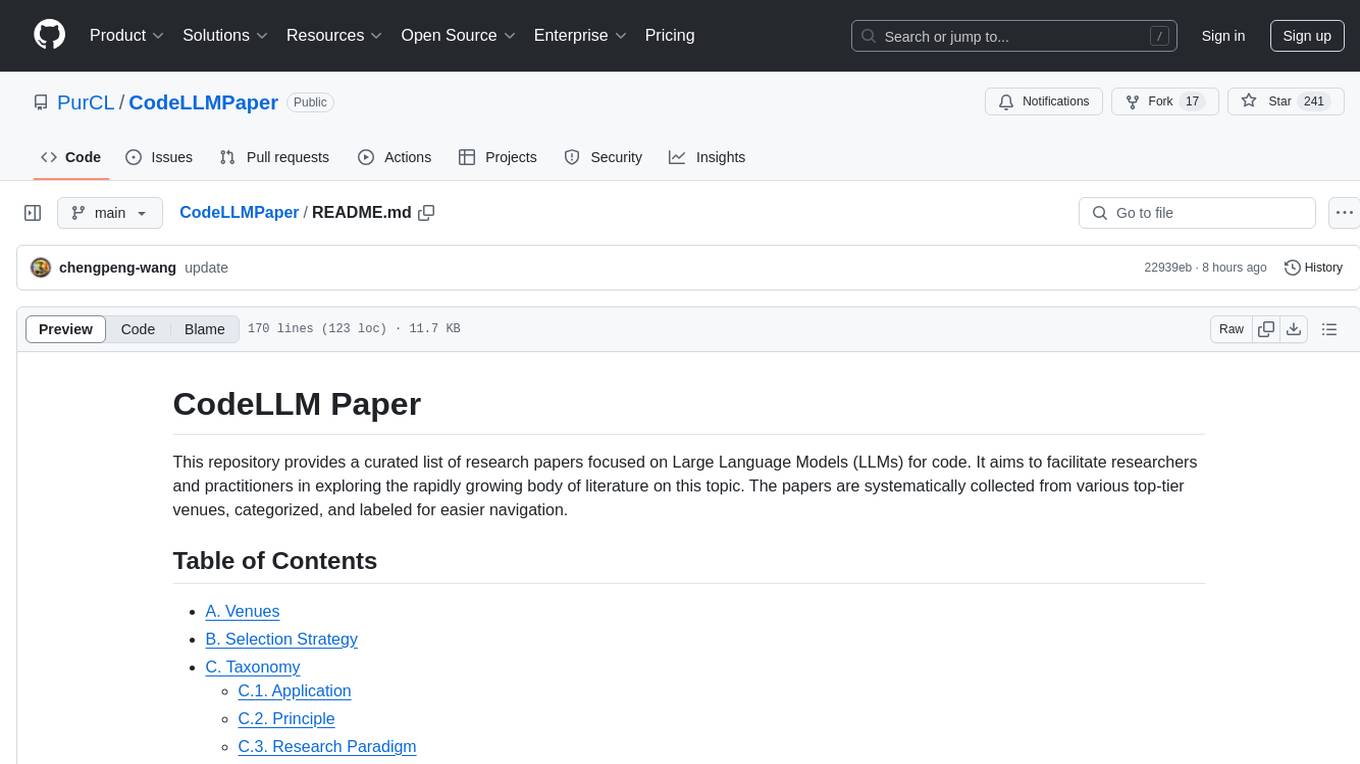
CodeLLMPaper
CodeLLM Paper repository provides a curated list of research papers focused on Large Language Models (LLMs) for code. It aims to facilitate researchers and practitioners in exploring the rapidly growing body of literature on this topic. The papers are systematically collected from various top-tier venues, categorized, and labeled for easier navigation. The selection strategy involves abstract extraction, keyword matching, relevance check using LLMs, and manual labeling. The papers are categorized based on Application, Principle, and Research Paradigm dimensions. Contributions to expand the repository are welcome through PR submission, issue submission, or request for batch updates. The repository is intended solely for research purposes, with raw data sourced from publicly available information on ACM, IEEE, and corresponding conference websites.
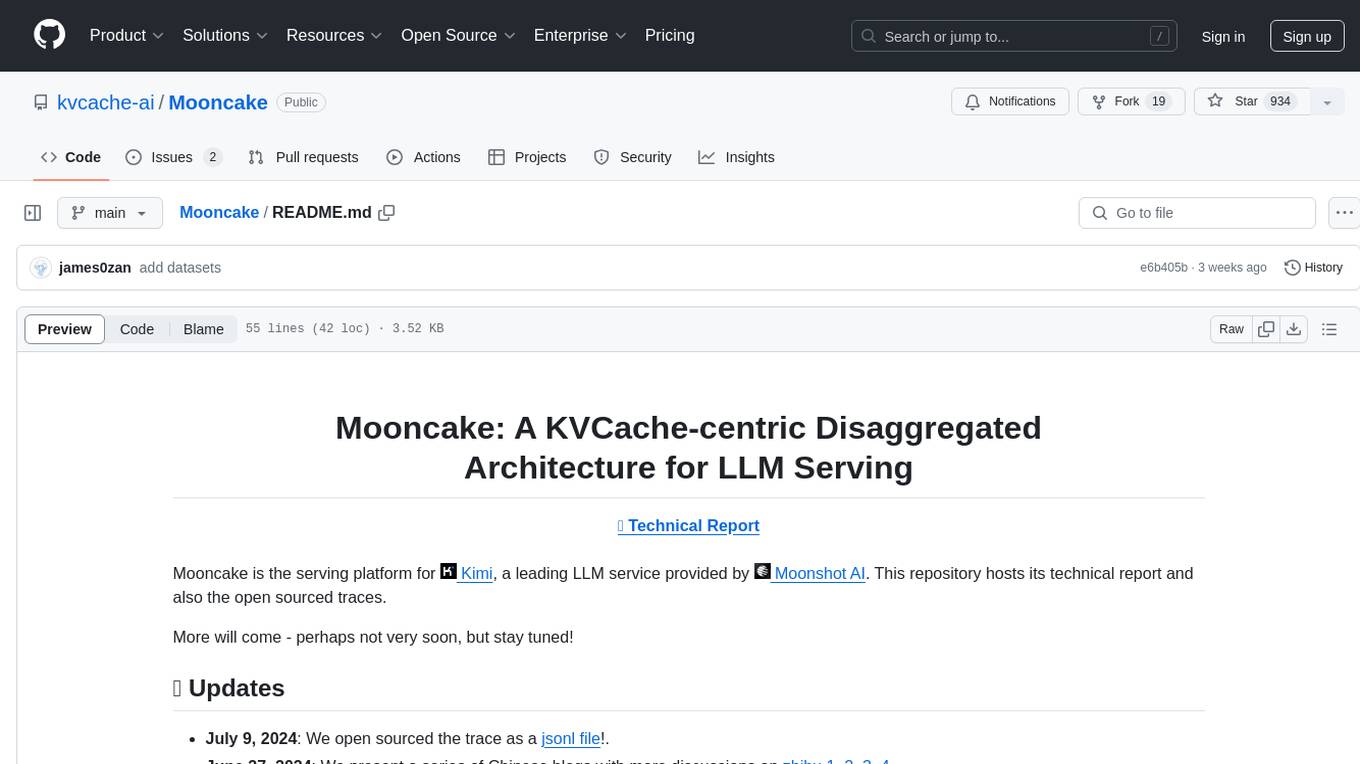
Mooncake
Mooncake is a serving platform for Kimi, a leading LLM service provided by Moonshot AI. It features a KVCache-centric disaggregated architecture that separates prefill and decoding clusters, leveraging underutilized CPU, DRAM, and SSD resources of the GPU cluster. Mooncake's scheduler balances throughput and latency-related SLOs, with a prediction-based early rejection policy for highly overloaded scenarios. It excels in long-context scenarios, achieving up to a 525% increase in throughput while handling 75% more requests under real workloads.
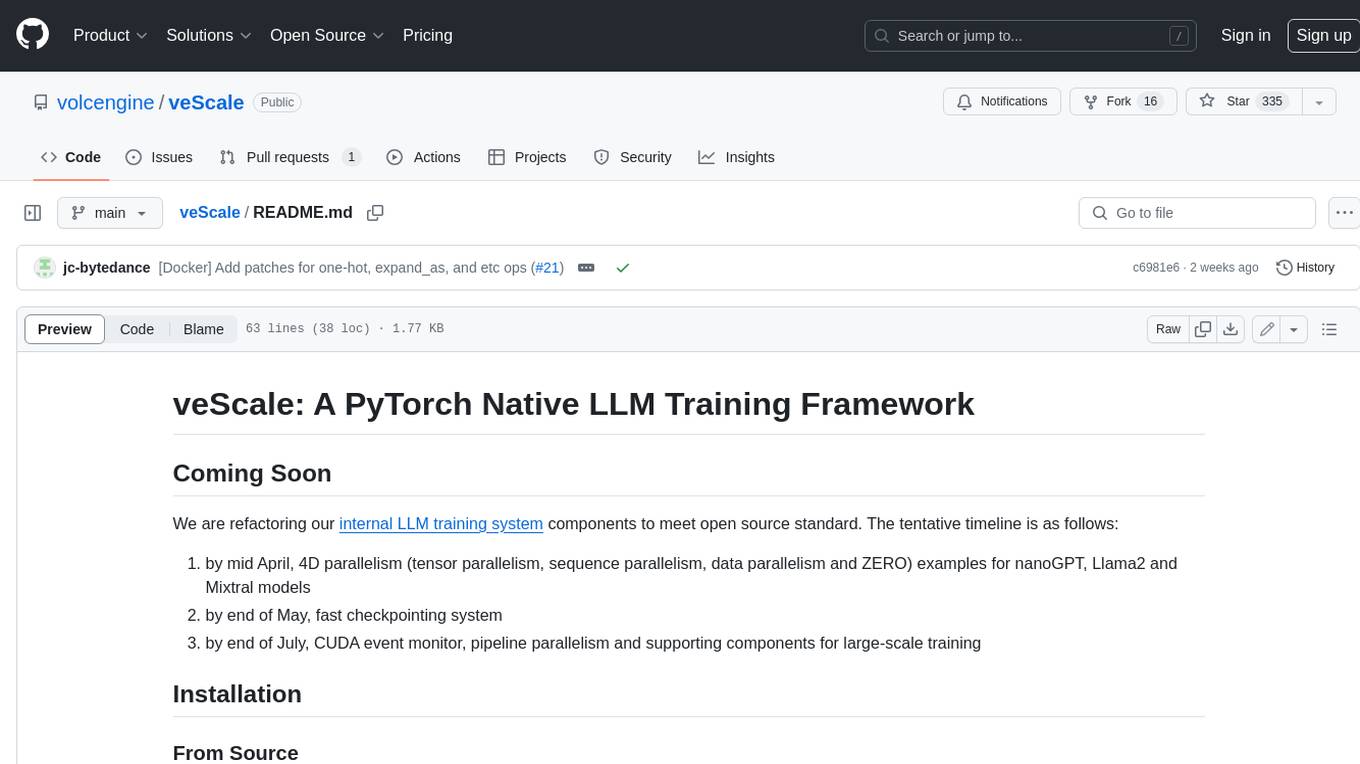
veScale
veScale is a PyTorch Native LLM Training Framework. It provides a set of tools and components to facilitate the training of large language models (LLMs) using PyTorch. veScale includes features such as 4D parallelism, fast checkpointing, and a CUDA event monitor. It is designed to be scalable and efficient, and it can be used to train LLMs on a variety of hardware platforms.
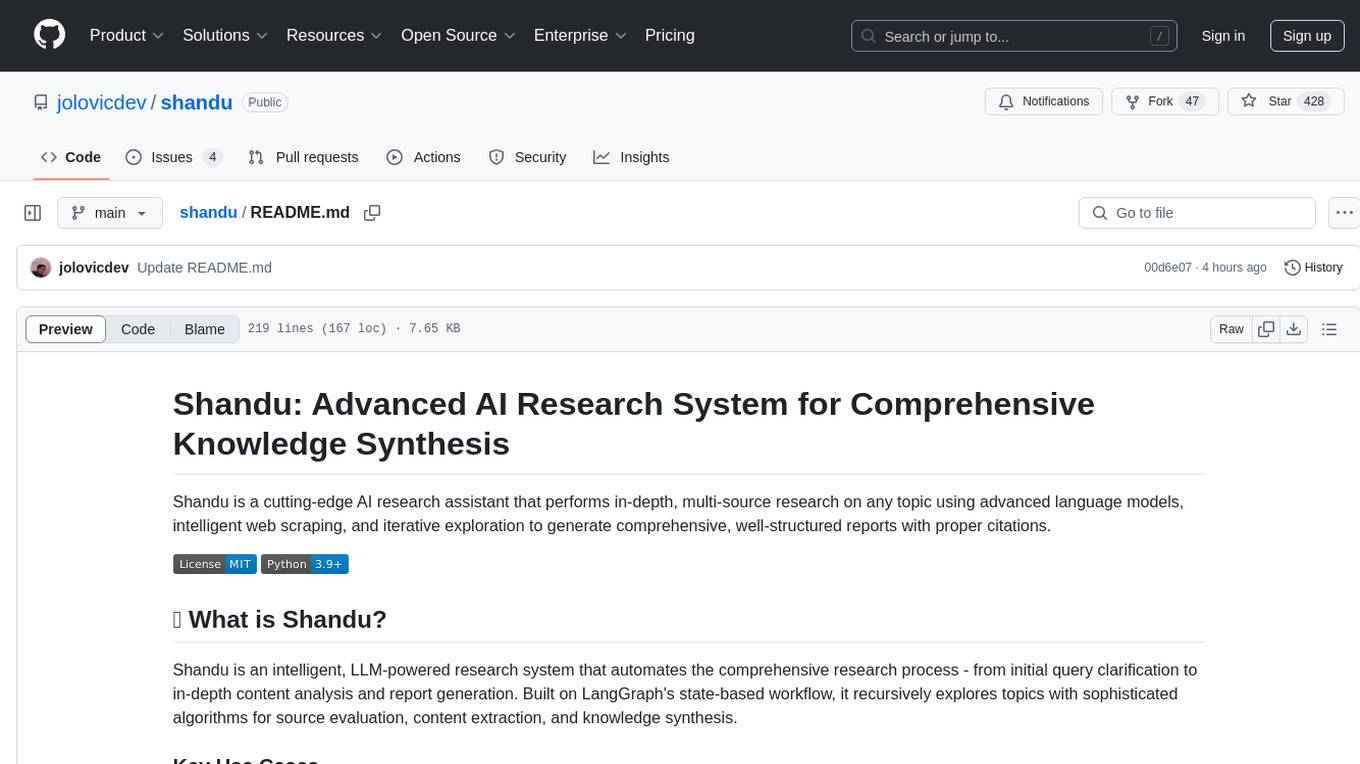
shandu
Shandu is an advanced AI research system that automates comprehensive research processes using language models, web scraping, and iterative exploration to generate well-structured reports with citations. It features intelligent state-based workflow, deep exploration, multi-source information synthesis, enhanced web scraping, smart source evaluation, content analysis pipeline, comprehensive report generation, parallel processing, adaptive search strategy, and full citation management.
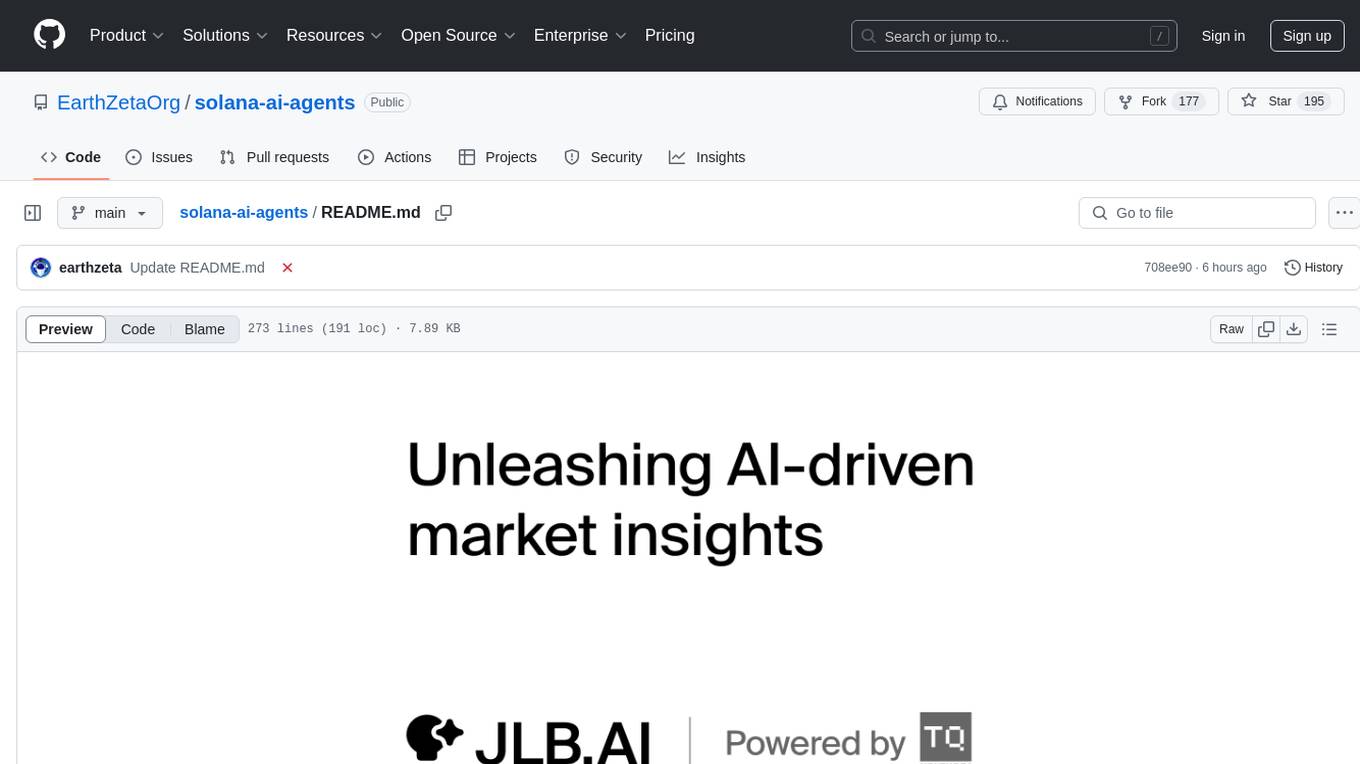
solana-ai-agents
JLB AI Agent is an innovative solution on the Solana blockchain that leverages artificial intelligence to automate complex tasks and enhance decision-making in the DeFi space. It offers real-time analytics, efficient operations, and seamless integration for both newcomers and experienced crypto enthusiasts. With features like autonomous trading, NFT management, DeFi insights, and comprehensive ecosystem integration, JLB empowers users with cutting-edge technology to navigate the dynamic landscape of blockchain.
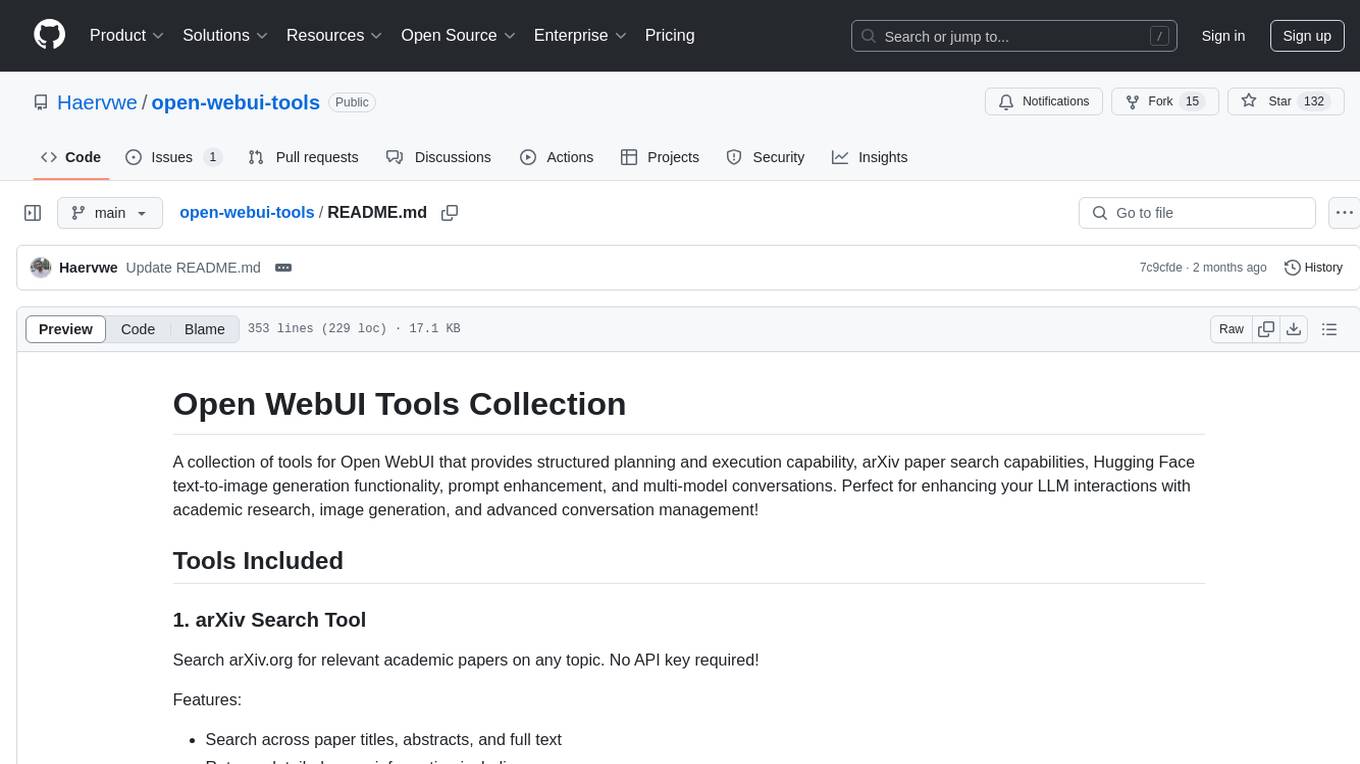
open-webui-tools
Open WebUI Tools Collection is a set of tools for structured planning, arXiv paper search, Hugging Face text-to-image generation, prompt enhancement, and multi-model conversations. It enhances LLM interactions with academic research, image generation, and conversation management. Tools include arXiv Search Tool and Hugging Face Image Generator. Function Pipes like Planner Agent offer autonomous plan generation and execution. Filters like Prompt Enhancer improve prompt quality. Installation and configuration instructions are provided for each tool and pipe.
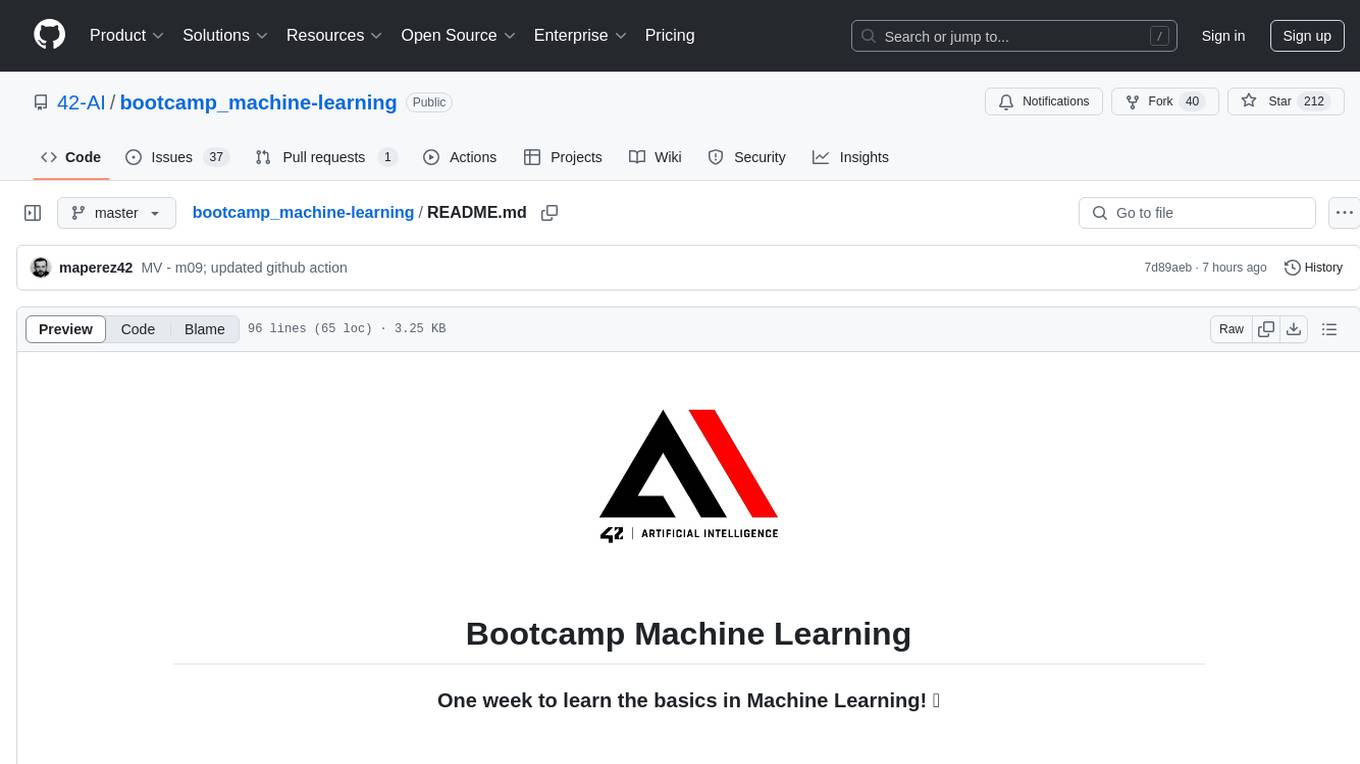
bootcamp_machine-learning
Bootcamp Machine Learning is a one-week program designed by 42 AI to teach the basics of Machine Learning. The curriculum covers topics such as linear algebra, statistics, regression, classification, and regularization. Participants will learn concepts like gradient descent, hypothesis modeling, overfitting detection, logistic regression, and more. The bootcamp is ideal for individuals with prior knowledge of Python who are interested in diving into the field of artificial intelligence.
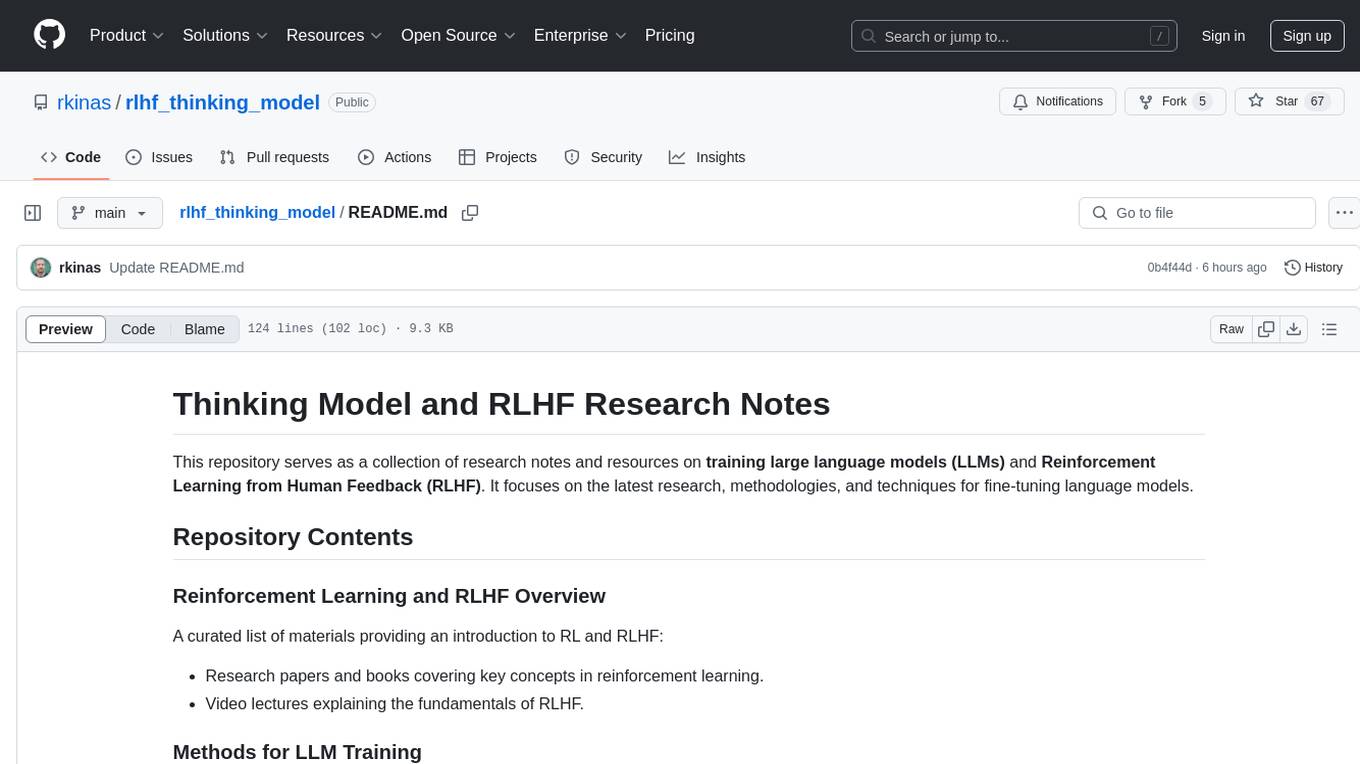
rlhf_thinking_model
This repository is a collection of research notes and resources focusing on training large language models (LLMs) and Reinforcement Learning from Human Feedback (RLHF). It includes methodologies, techniques, and state-of-the-art approaches for optimizing preferences and model alignment in LLM training. The purpose is to serve as a reference for researchers and engineers interested in reinforcement learning, large language models, model alignment, and alternative RL-based methods.
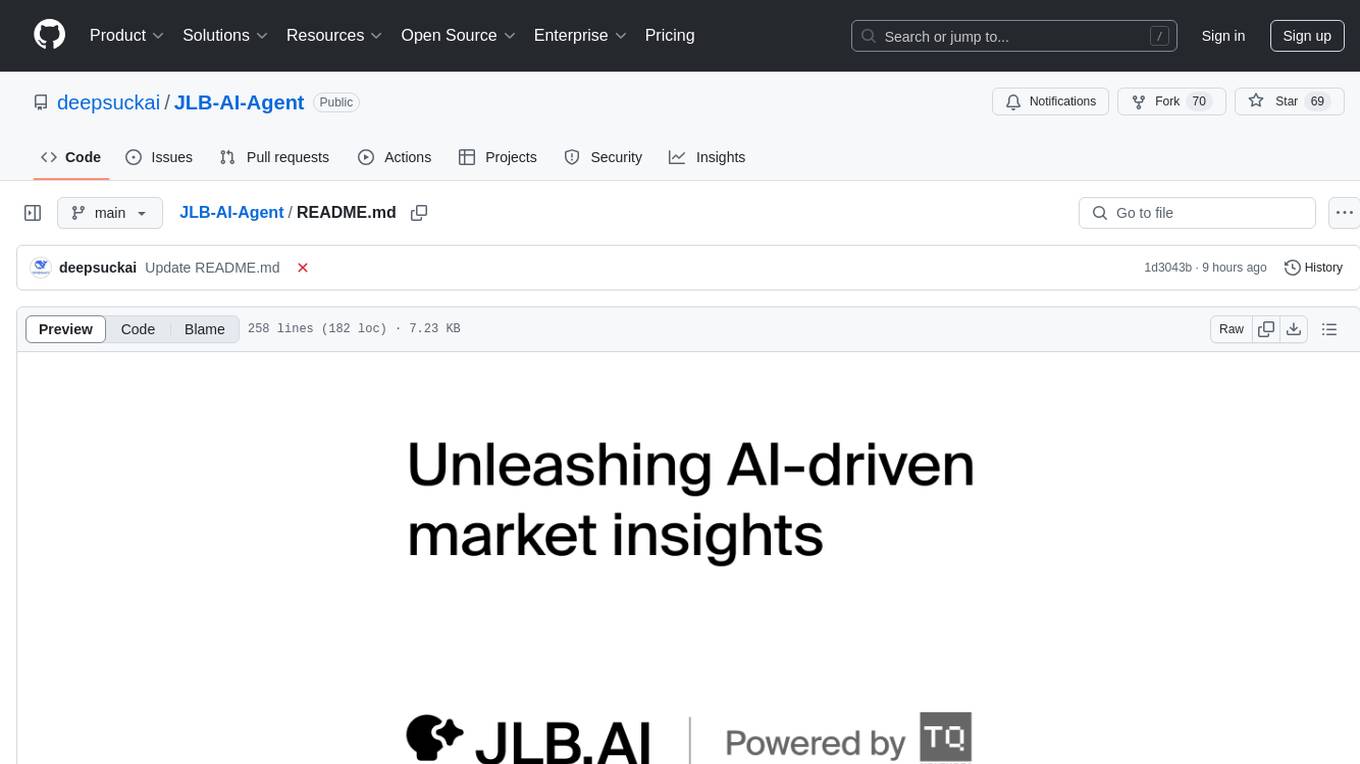
JLB-AI-Agent
JLB AI Agent is an innovative solution built on the Solana blockchain that harnesses the power of artificial intelligence to automate complex tasks and optimize decision-making in the DeFi space. It aims to provide real-time analytics, efficient operations, and seamless integration for both newcomers and experienced crypto enthusiasts. The tool offers features like blockchain agent chat terminal, real-time streaming implementation, trading infrastructure, NFT management, AI integration, and more, empowering users with autonomous technology where AI meets the dynamic landscape of blockchain.
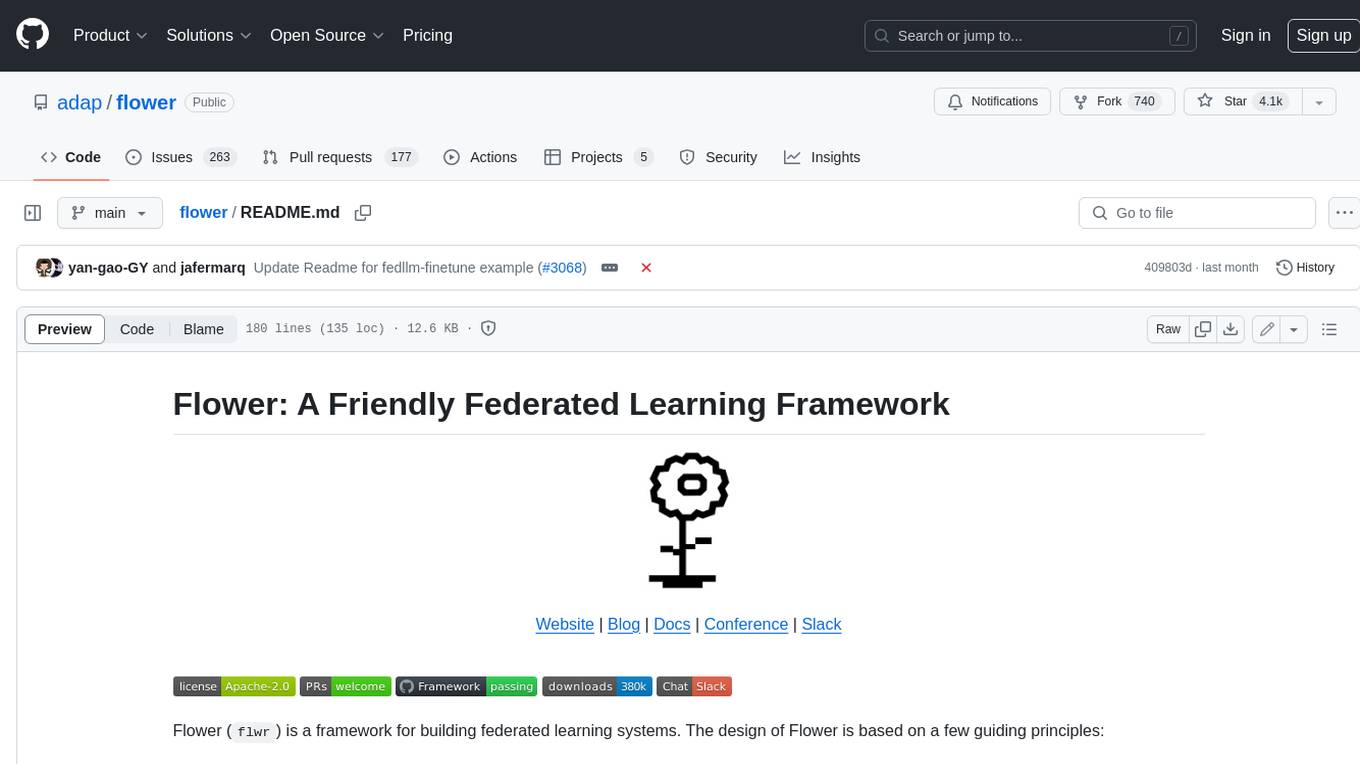
flower
Flower is a framework for building federated learning systems. It is designed to be customizable, extensible, framework-agnostic, and understandable. Flower can be used with any machine learning framework, for example, PyTorch, TensorFlow, Hugging Face Transformers, PyTorch Lightning, scikit-learn, JAX, TFLite, MONAI, fastai, MLX, XGBoost, Pandas for federated analytics, or even raw NumPy for users who enjoy computing gradients by hand.
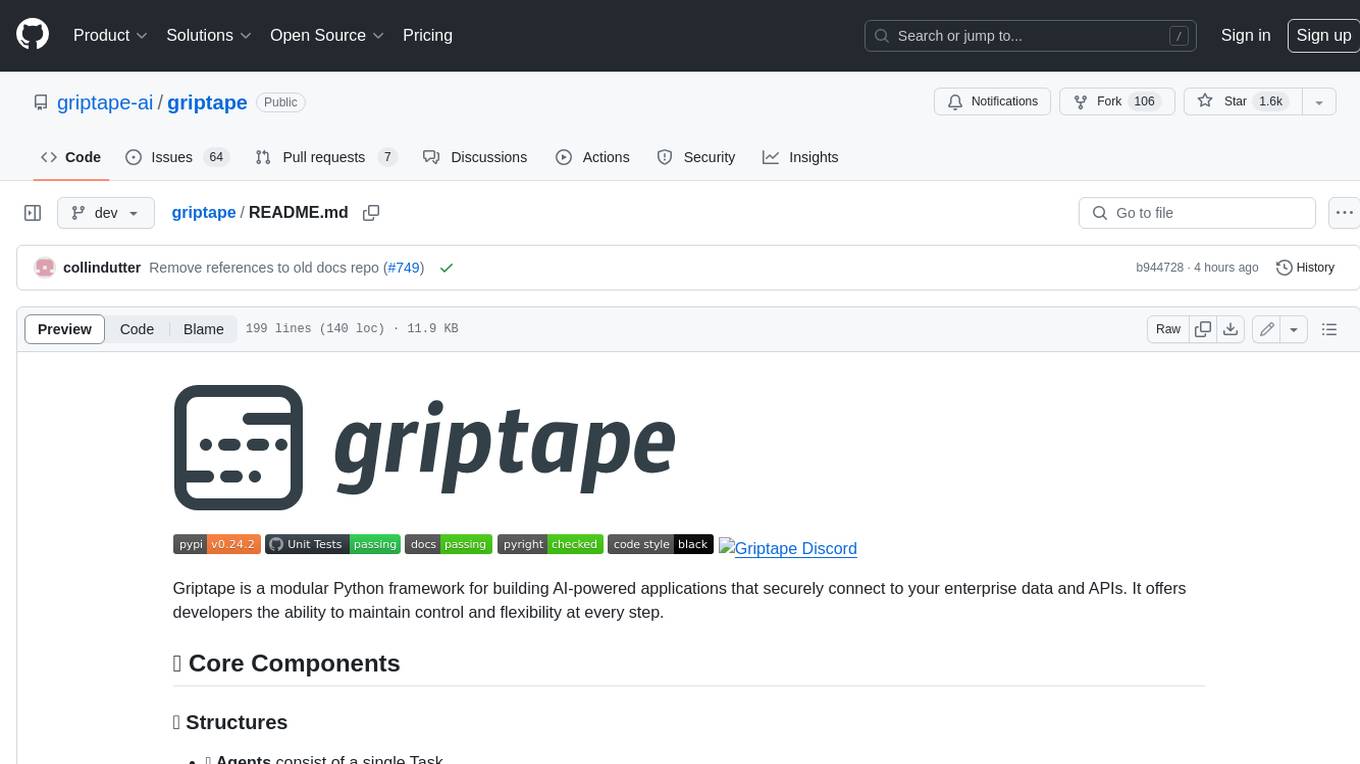
griptape
Griptape is a modular Python framework for building AI-powered applications that securely connect to your enterprise data and APIs. It offers developers the ability to maintain control and flexibility at every step. Griptape's core components include Structures (Agents, Pipelines, and Workflows), Tasks, Tools, Memory (Conversation Memory, Task Memory, and Meta Memory), Drivers (Prompt and Embedding Drivers, Vector Store Drivers, Image Generation Drivers, Image Query Drivers, SQL Drivers, Web Scraper Drivers, and Conversation Memory Drivers), Engines (Query Engines, Extraction Engines, Summary Engines, Image Generation Engines, and Image Query Engines), and additional components (Rulesets, Loaders, Artifacts, Chunkers, and Tokenizers). Griptape enables developers to create AI-powered applications with ease and efficiency.
For similar tasks
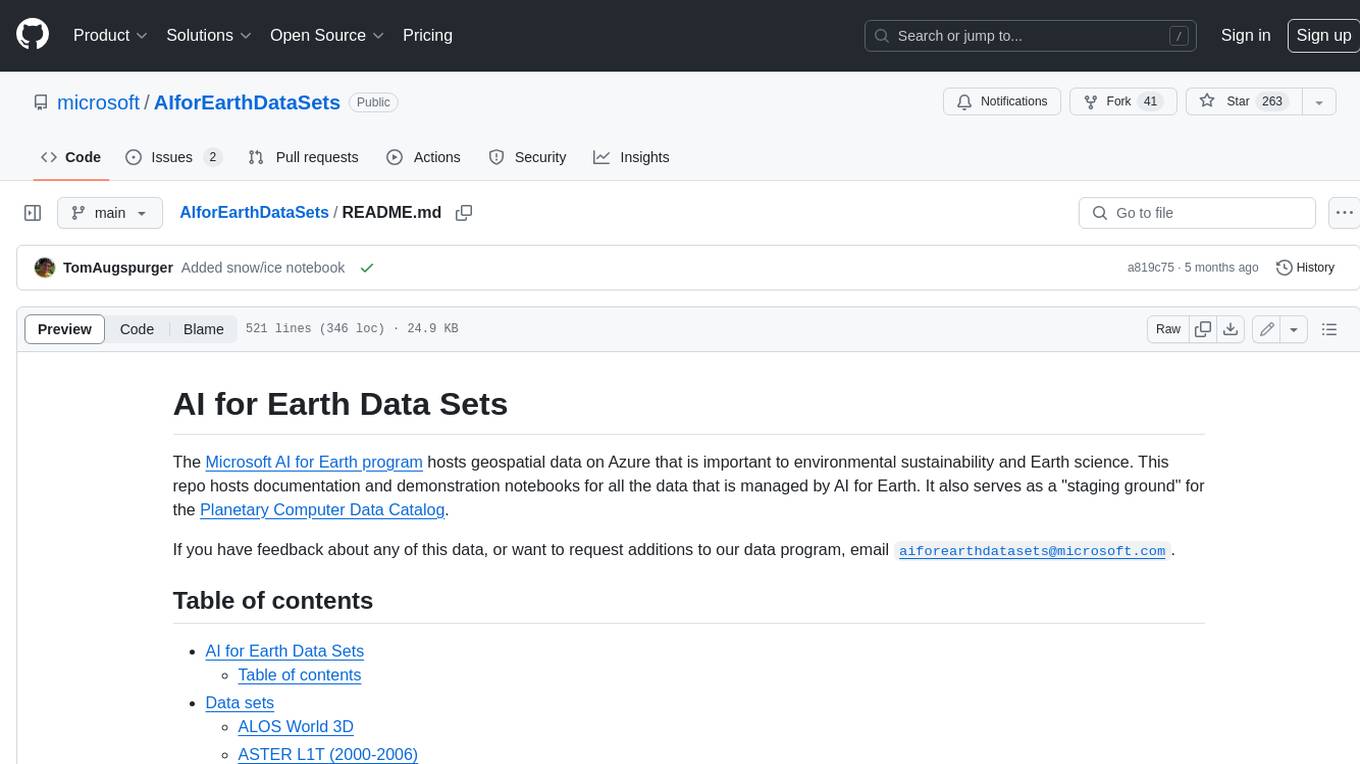
AIforEarthDataSets
The Microsoft AI for Earth program hosts geospatial data on Azure that is important to environmental sustainability and Earth science. This repo hosts documentation and demonstration notebooks for all the data that is managed by AI for Earth. It also serves as a "staging ground" for the Planetary Computer Data Catalog.
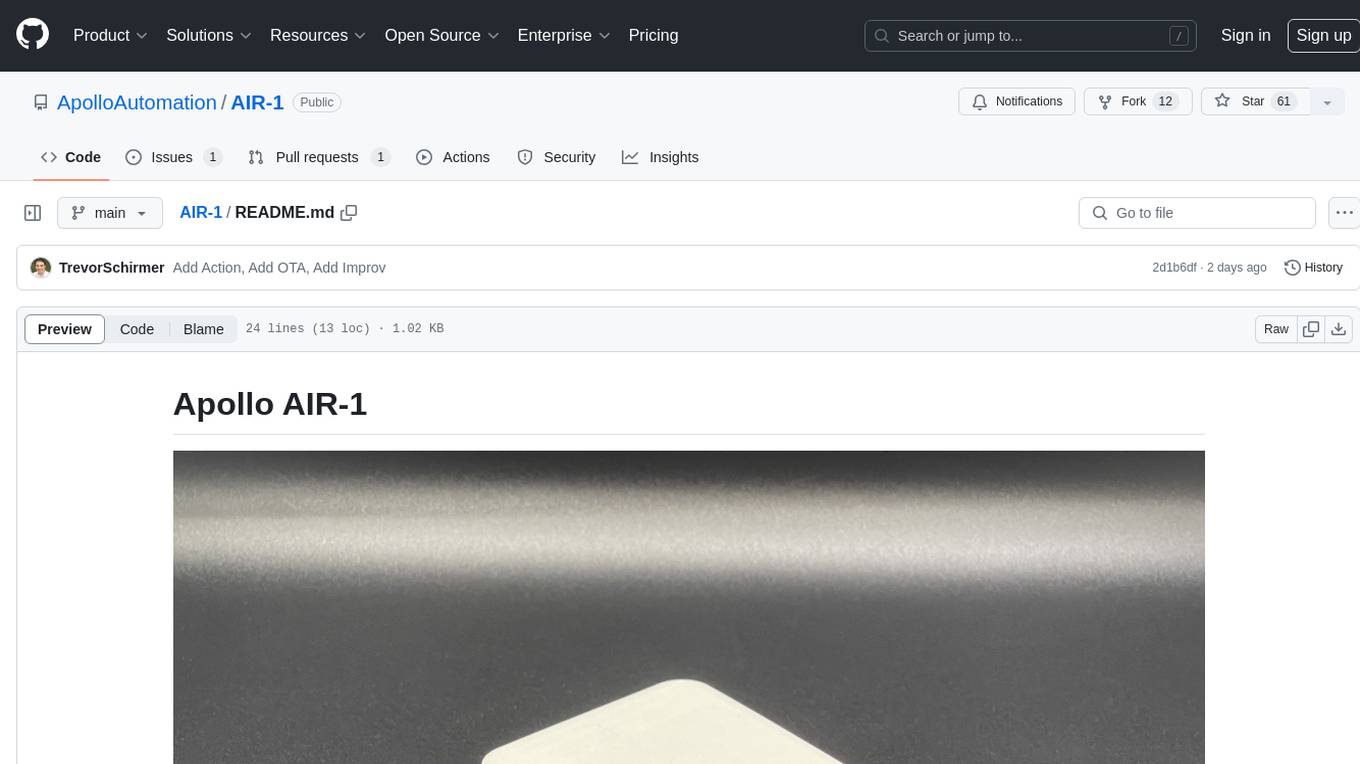
AIR-1
AIR-1 is a compact sensor device designed for monitoring various environmental parameters such as gas levels, particulate matter, temperature, and humidity. It features multiple sensors for detecting gases like CO, alcohol, H2, NO2, NH3, CO2, as well as particulate matter, VOCs, NOx, and more. The device is designed with a focus on accuracy and efficient heat management in a small form factor, making it suitable for indoor air quality monitoring and environmental sensing applications.
For similar jobs
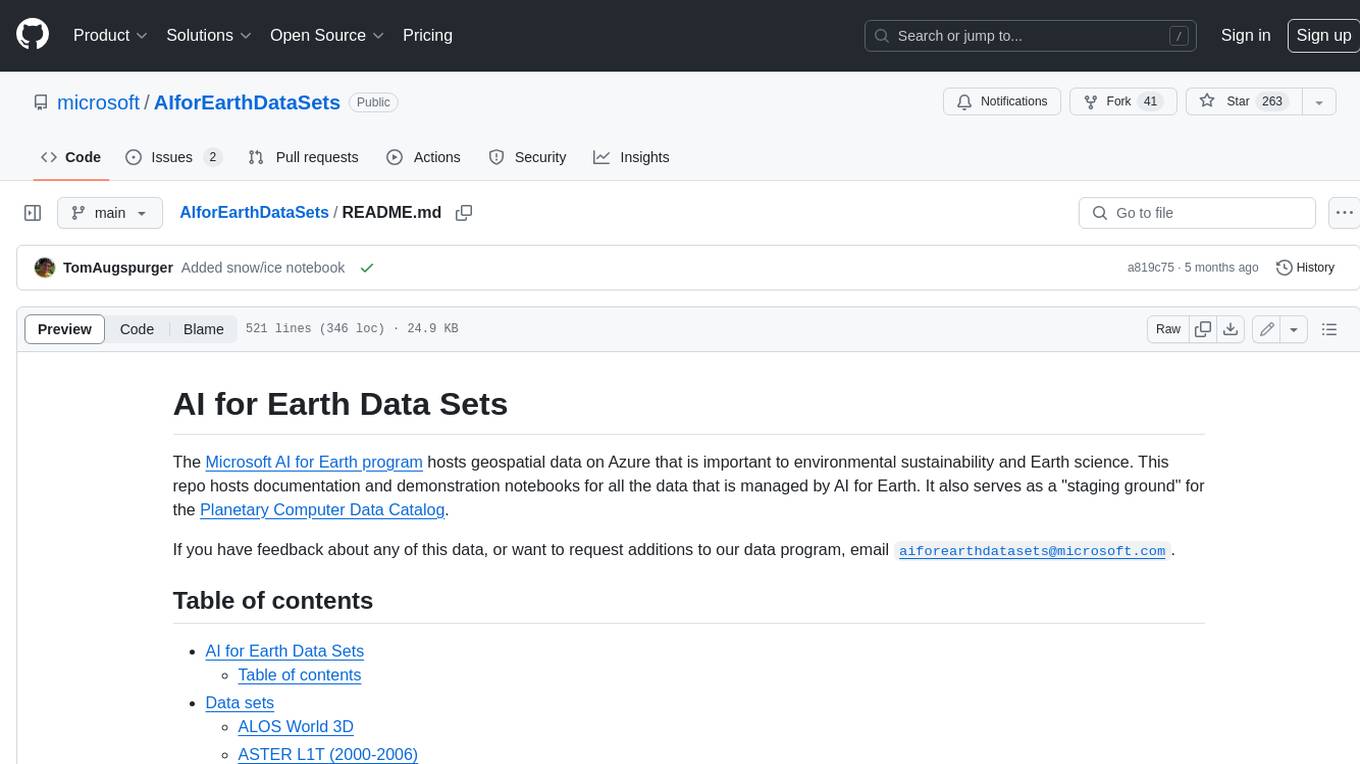
AIforEarthDataSets
The Microsoft AI for Earth program hosts geospatial data on Azure that is important to environmental sustainability and Earth science. This repo hosts documentation and demonstration notebooks for all the data that is managed by AI for Earth. It also serves as a "staging ground" for the Planetary Computer Data Catalog.
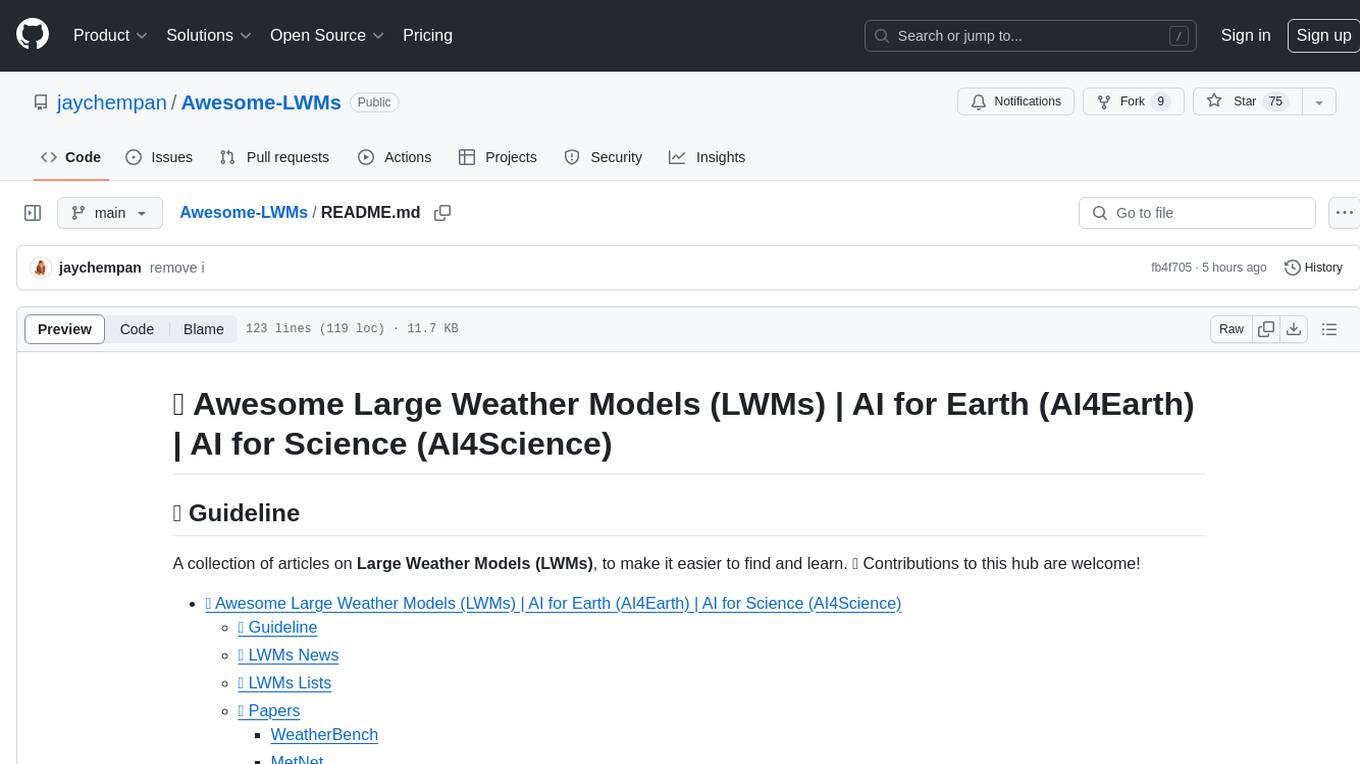
Awesome-LWMs
Awesome Large Weather Models (LWMs) is a curated collection of articles and resources related to large weather models used in AI for Earth and AI for Science. It includes information on various cutting-edge weather forecasting models, benchmark datasets, and research papers. The repository serves as a hub for researchers and enthusiasts to explore the latest advancements in weather modeling and forecasting.
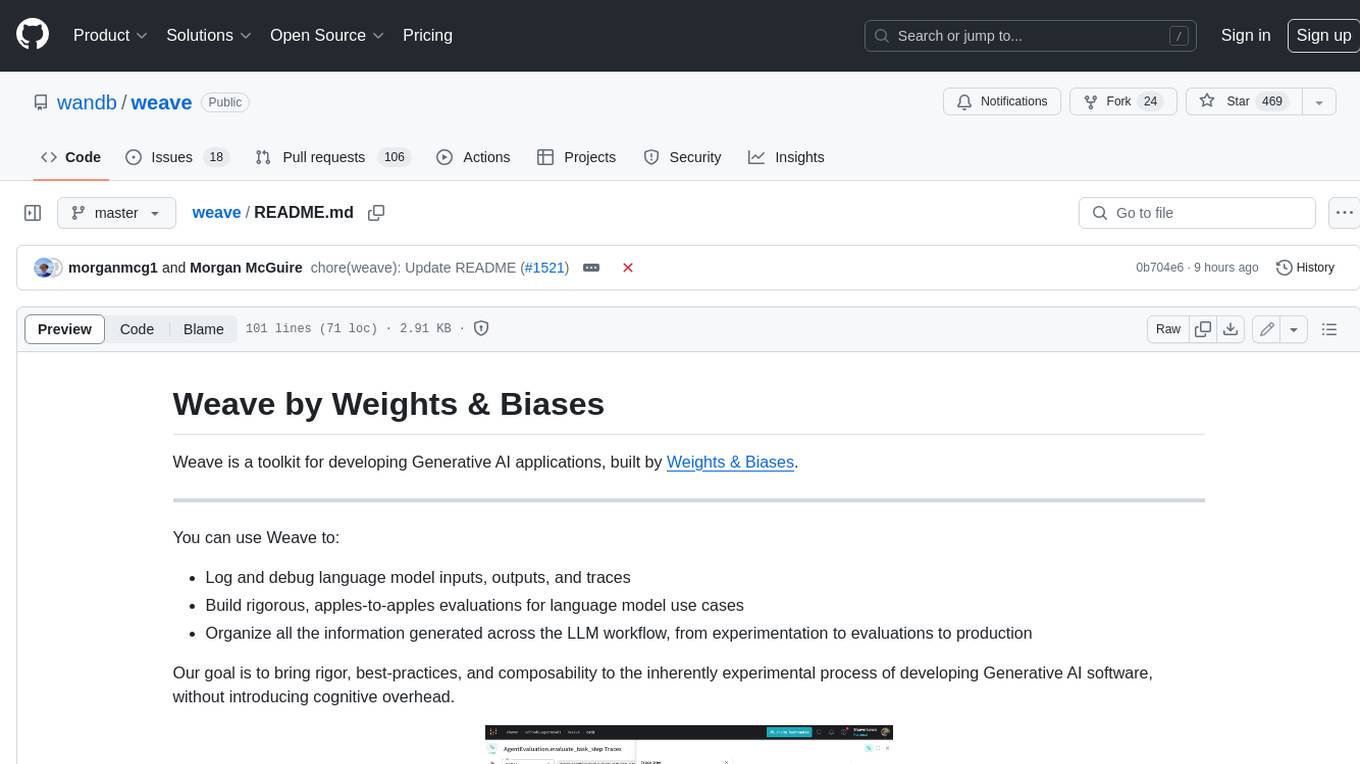
weave
Weave is a toolkit for developing Generative AI applications, built by Weights & Biases. With Weave, you can log and debug language model inputs, outputs, and traces; build rigorous, apples-to-apples evaluations for language model use cases; and organize all the information generated across the LLM workflow, from experimentation to evaluations to production. Weave aims to bring rigor, best-practices, and composability to the inherently experimental process of developing Generative AI software, without introducing cognitive overhead.
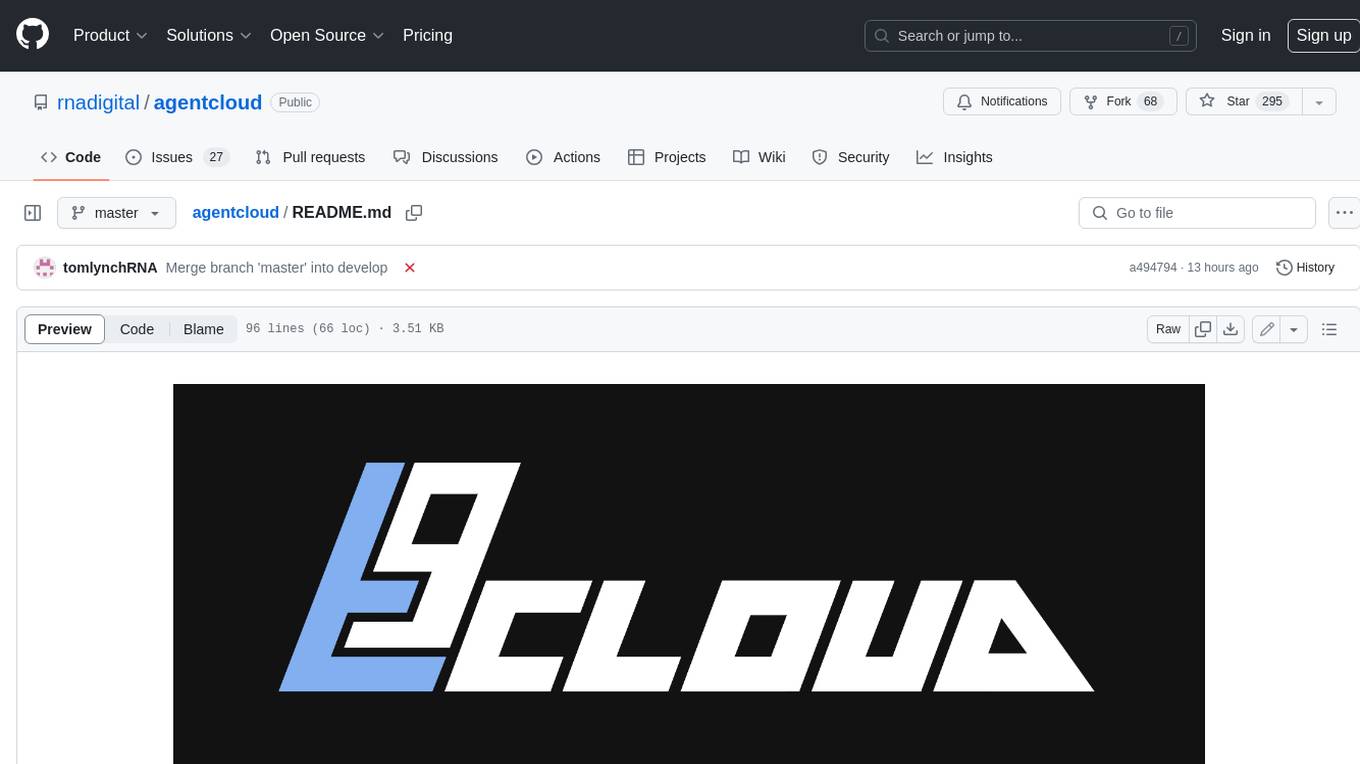
agentcloud
AgentCloud is an open-source platform that enables companies to build and deploy private LLM chat apps, empowering teams to securely interact with their data. It comprises three main components: Agent Backend, Webapp, and Vector Proxy. To run this project locally, clone the repository, install Docker, and start the services. The project is licensed under the GNU Affero General Public License, version 3 only. Contributions and feedback are welcome from the community.
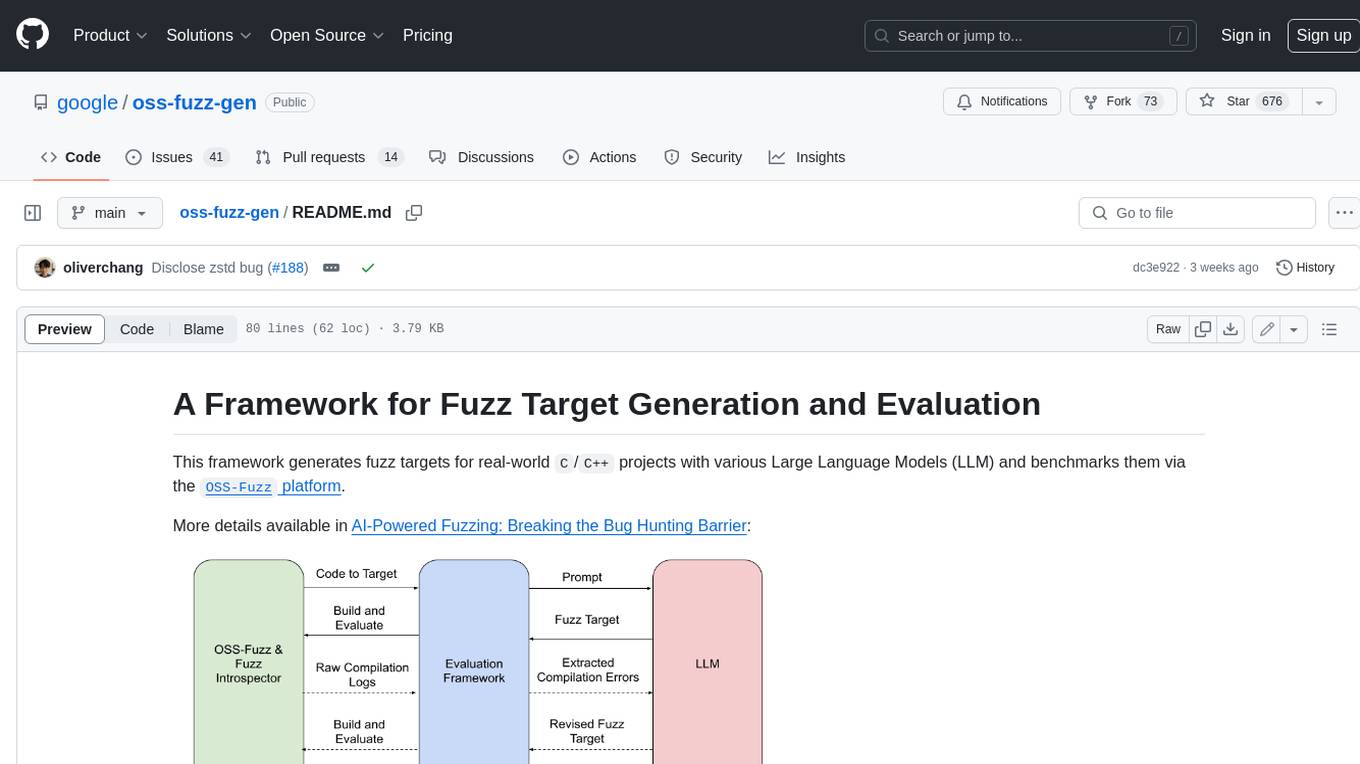
oss-fuzz-gen
This framework generates fuzz targets for real-world `C`/`C++` projects with various Large Language Models (LLM) and benchmarks them via the `OSS-Fuzz` platform. It manages to successfully leverage LLMs to generate valid fuzz targets (which generate non-zero coverage increase) for 160 C/C++ projects. The maximum line coverage increase is 29% from the existing human-written targets.
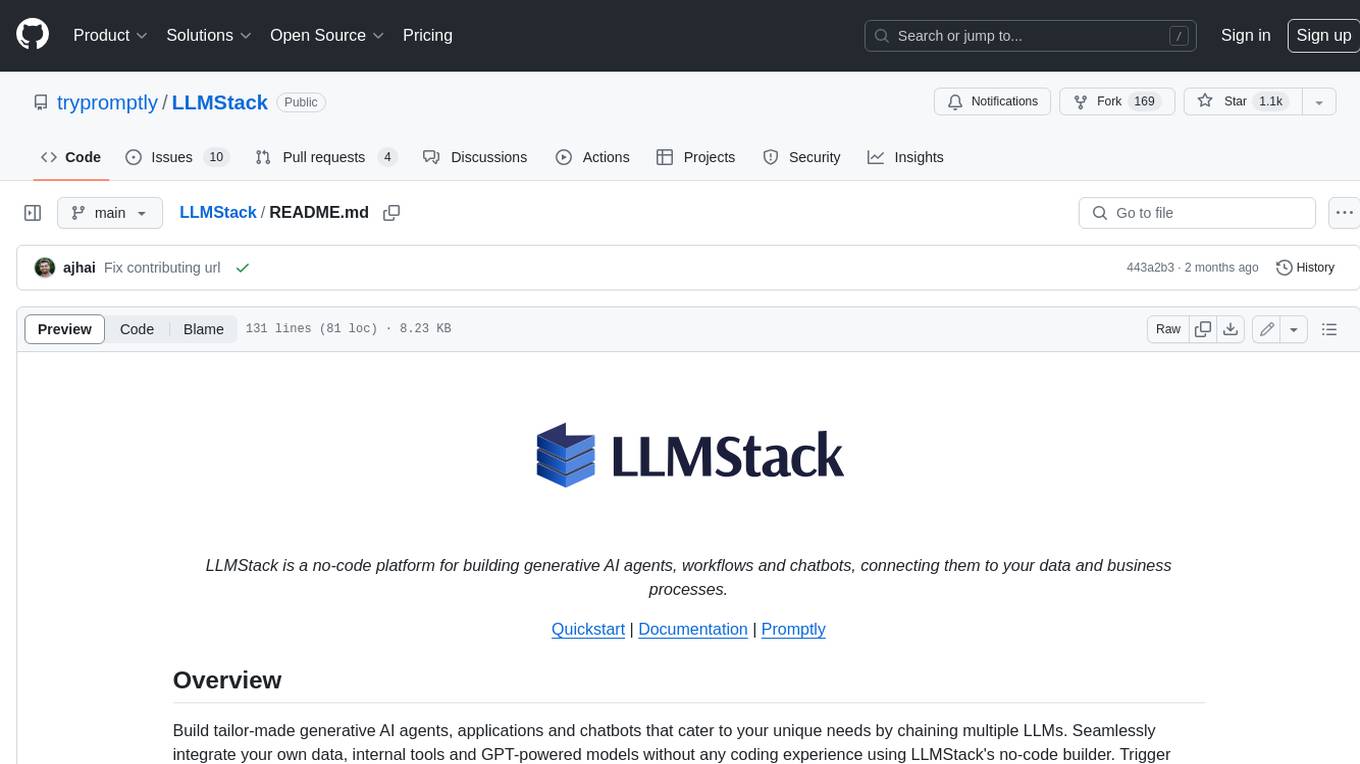
LLMStack
LLMStack is a no-code platform for building generative AI agents, workflows, and chatbots. It allows users to connect their own data, internal tools, and GPT-powered models without any coding experience. LLMStack can be deployed to the cloud or on-premise and can be accessed via HTTP API or triggered from Slack or Discord.
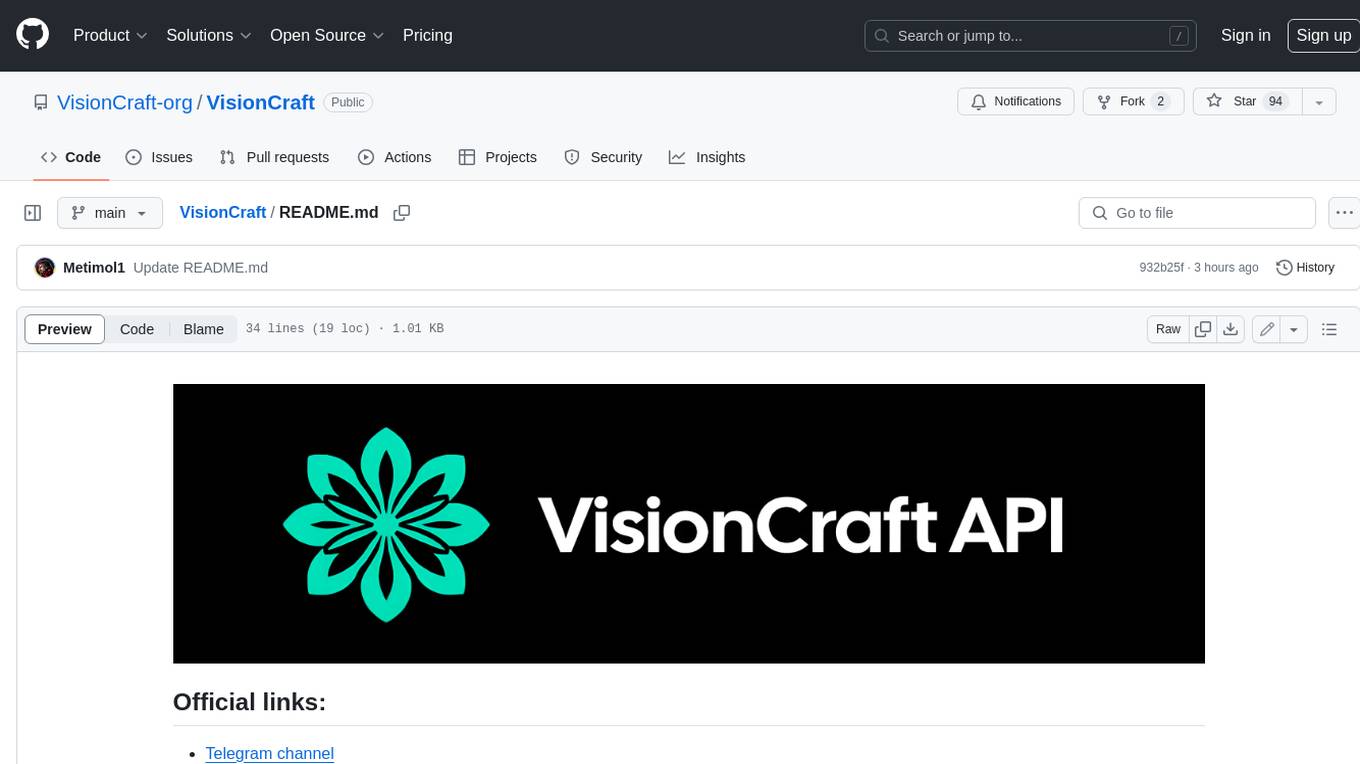
VisionCraft
The VisionCraft API is a free API for using over 100 different AI models. From images to sound.
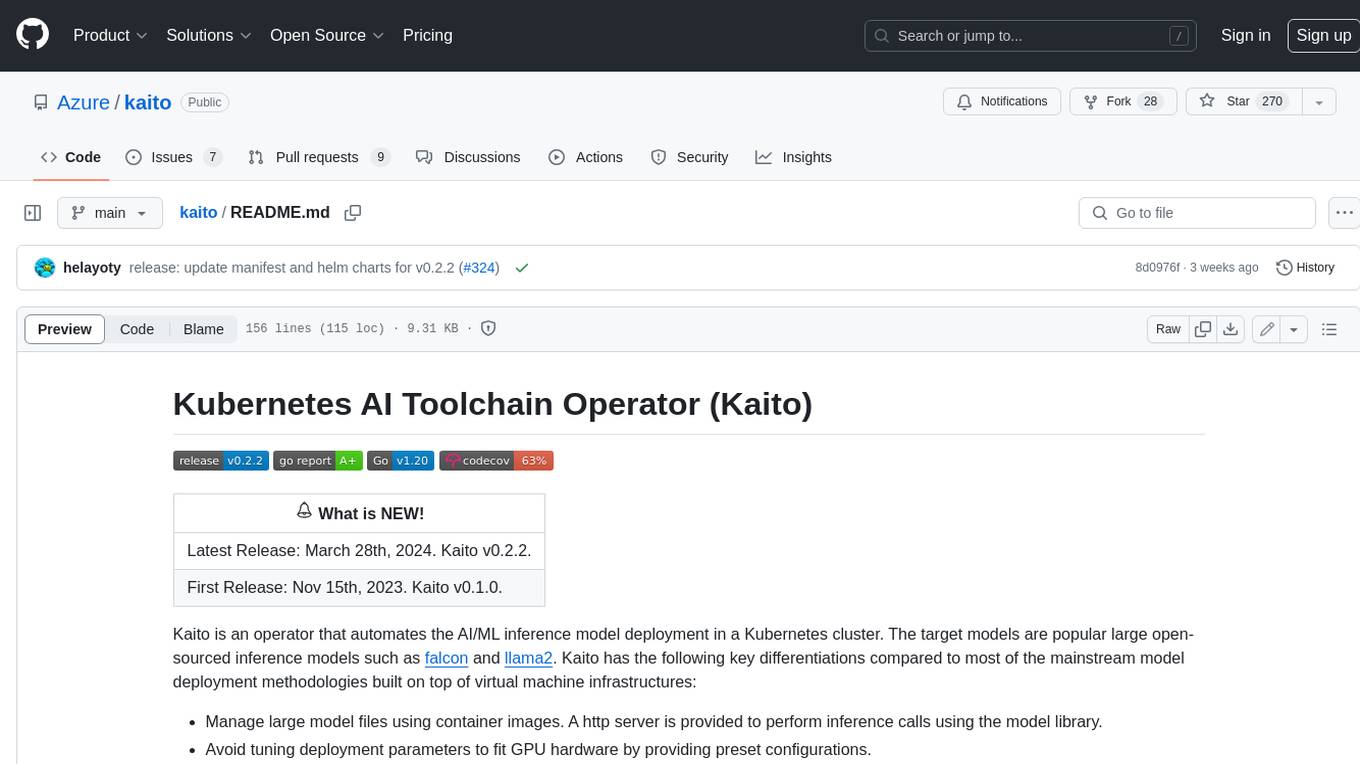
kaito
Kaito is an operator that automates the AI/ML inference model deployment in a Kubernetes cluster. It manages large model files using container images, avoids tuning deployment parameters to fit GPU hardware by providing preset configurations, auto-provisions GPU nodes based on model requirements, and hosts large model images in the public Microsoft Container Registry (MCR) if the license allows. Using Kaito, the workflow of onboarding large AI inference models in Kubernetes is largely simplified.