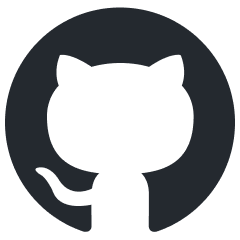
veScale
A PyTorch Native LLM Training Framework
Stars: 531
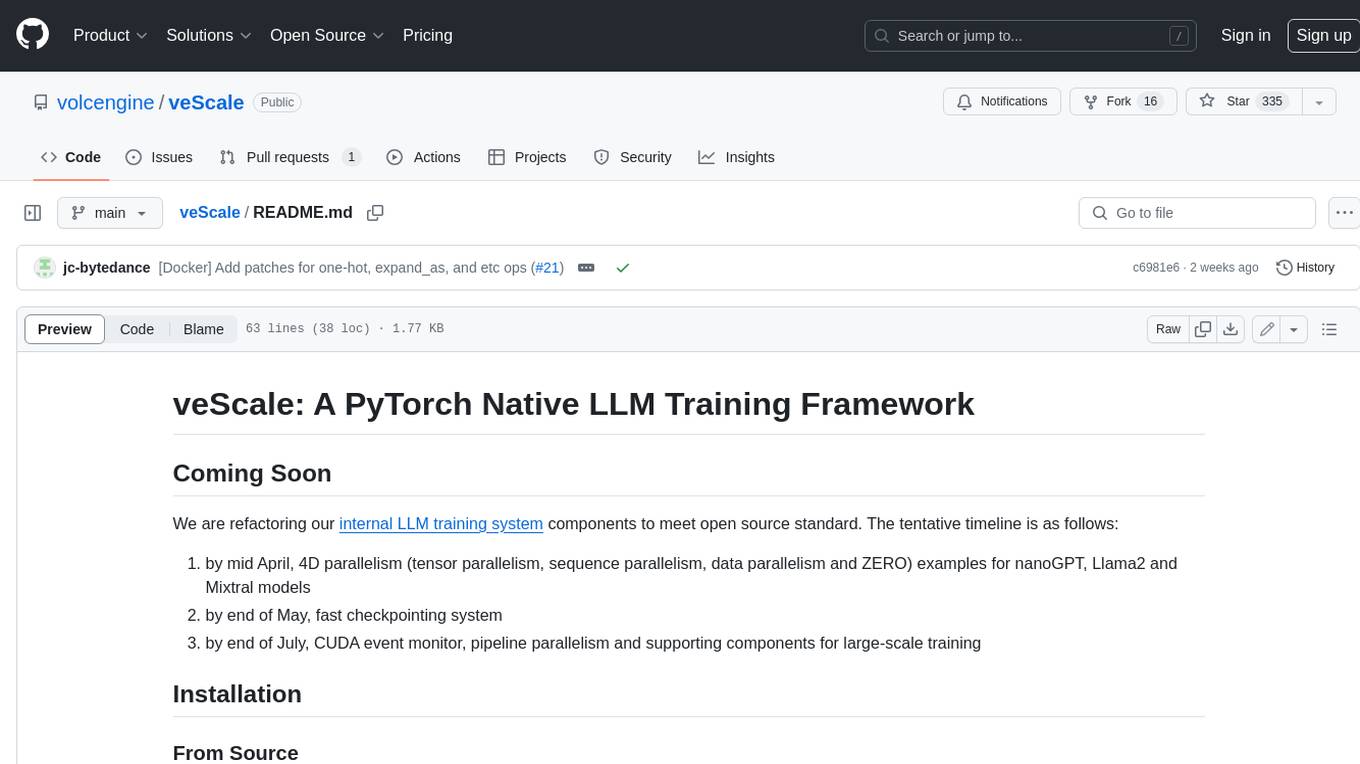
veScale is a PyTorch Native LLM Training Framework. It provides a set of tools and components to facilitate the training of large language models (LLMs) using PyTorch. veScale includes features such as 4D parallelism, fast checkpointing, and a CUDA event monitor. It is designed to be scalable and efficient, and it can be used to train LLMs on a variety of hardware platforms.
README:
An Industrial-Level Framework for Easy-of-Use
-
🔥 PyTorch Native: veScale is rooted in PyTorch-native data structures, operators, and APIs, enjoying the ecosystem of PyTorch that dominates the ML world.
-
🛡 Zero Model Code Change: veScale decouples distributed system design from model architecture, requiring near-zero or zero modification on the model code of users.
-
🚀 Single Device Abstraction: veScale provides single-device semantics to users, automatically distributing and orchestrating model execution in a cluster of devices.
-
🎯 Automatic Parallelism Planning: veScale parallelizes model execution with a synergy of strategies (tensor, sequence, data, ZeRO, pipeline parallelism) under semi- or full-automation [coming soon].
-
âš¡ Eager & Compile Mode: veScale supports not only Eager-mode automation for parallel training and inference but also Compile-mode for ultimate performance [coming soon].
-
📀 Automatic Checkpoint Resharding: veScale manages distributed checkpoints automatically with online resharding across different cluster sizes and different parallelism strategies.
-
[2024-7-25] veScale's pipeline parallelism open sourced with API, graph parser, stage abstraction, schedules and execution runtime along with nD distributed timeline.
-
[2024-5-31] veScale's fast checkpointing system open sourced with automatic checkpoint resharding, caching, load-balancing, fast copying, deduplicating, and asynchronous io.
-
[2024-5-21] veScale's examples (Mixtral, LLama2, and nanoGPT) open sourced with bit-wise correctness of training loss curves.
-
[2024-5-13] The debut of veScale in MLSys 2024 as a poster.
-
[2024-4-16] Our internal LLM training system presented in NSDI 2024.
veScale is still in its early phase. We are refactoring our internal LLM training system components to meet open source standard. The tentative timeline is as follows:
-
High-level nD parallel api for extreme ease of use
-
Power-user plan api for easy customization of nD parallel training
-
End-to-end vescale/examples with 5D parallel training (TP, SP, DP, ZeRO, PP)
Table of Content (web view)
Parallel
- Overview
- Tensor Parallel & Sequence Parallel
- Data Parallel
- Optimizer Parallel
- Pipeline Parallel
- nD Device Mesh
Plan
The veScale Project is under the Apache License v2.0.
For Tasks:
Click tags to check more tools for each tasksFor Jobs:
Alternative AI tools for veScale
Similar Open Source Tools
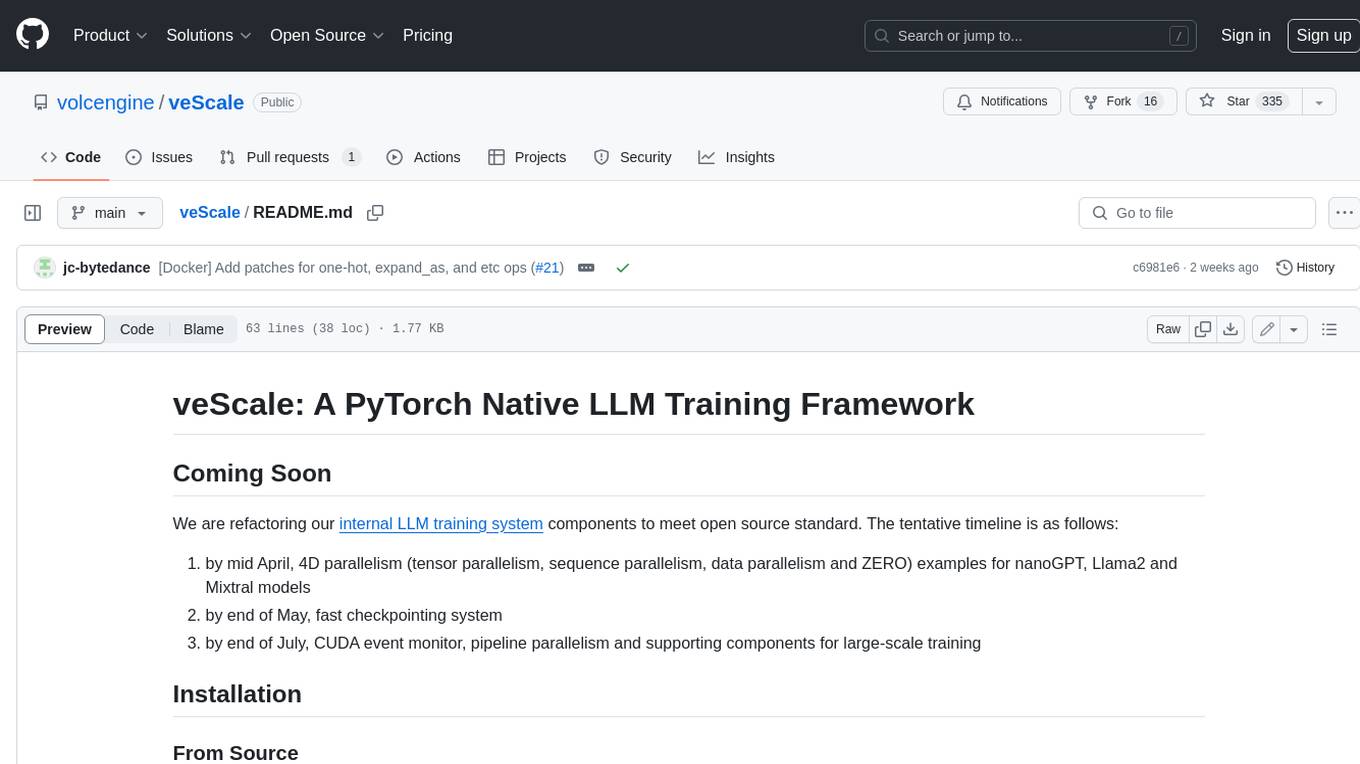
veScale
veScale is a PyTorch Native LLM Training Framework. It provides a set of tools and components to facilitate the training of large language models (LLMs) using PyTorch. veScale includes features such as 4D parallelism, fast checkpointing, and a CUDA event monitor. It is designed to be scalable and efficient, and it can be used to train LLMs on a variety of hardware platforms.
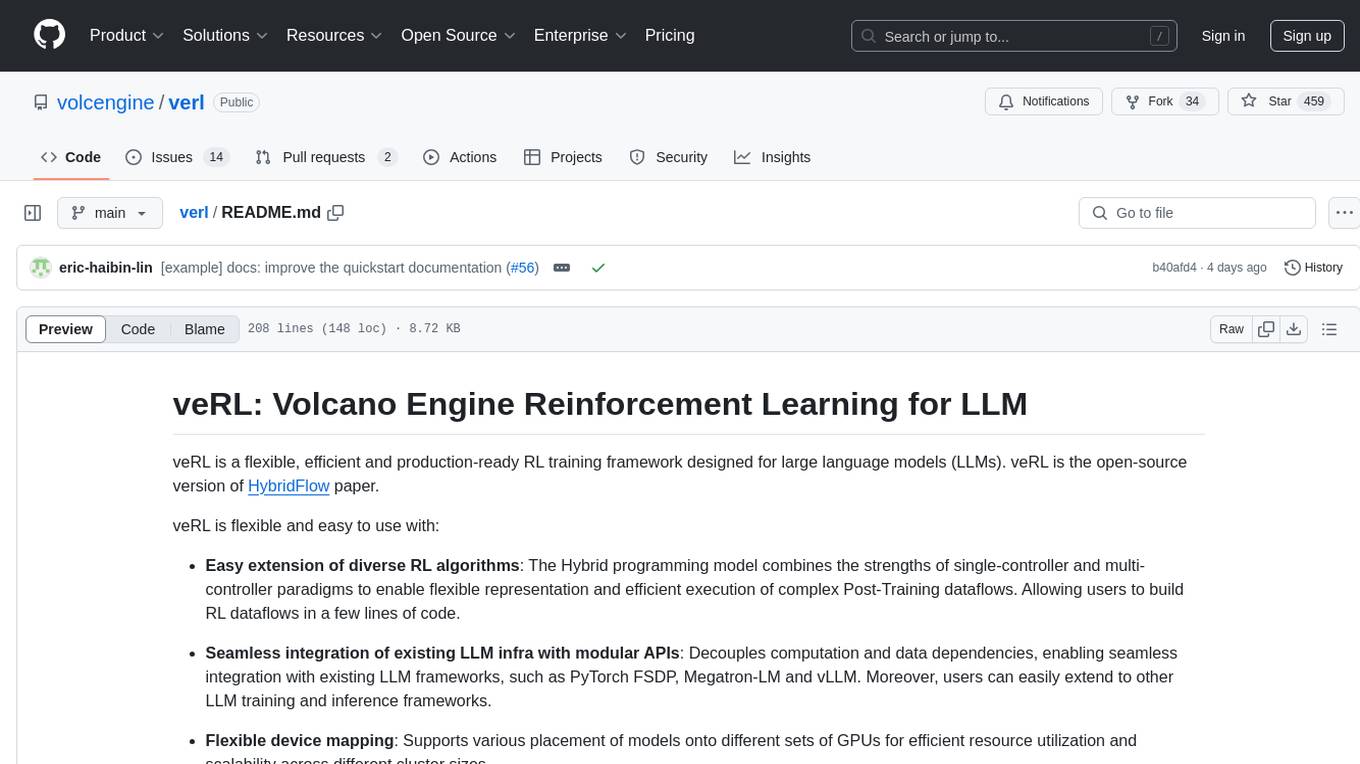
verl
veRL is a flexible and efficient reinforcement learning training framework designed for large language models (LLMs). It allows easy extension of diverse RL algorithms, seamless integration with existing LLM infrastructures, and flexible device mapping. The framework achieves state-of-the-art throughput and efficient actor model resharding with 3D-HybridEngine. It supports popular HuggingFace models and is suitable for users working with PyTorch FSDP, Megatron-LM, and vLLM backends.
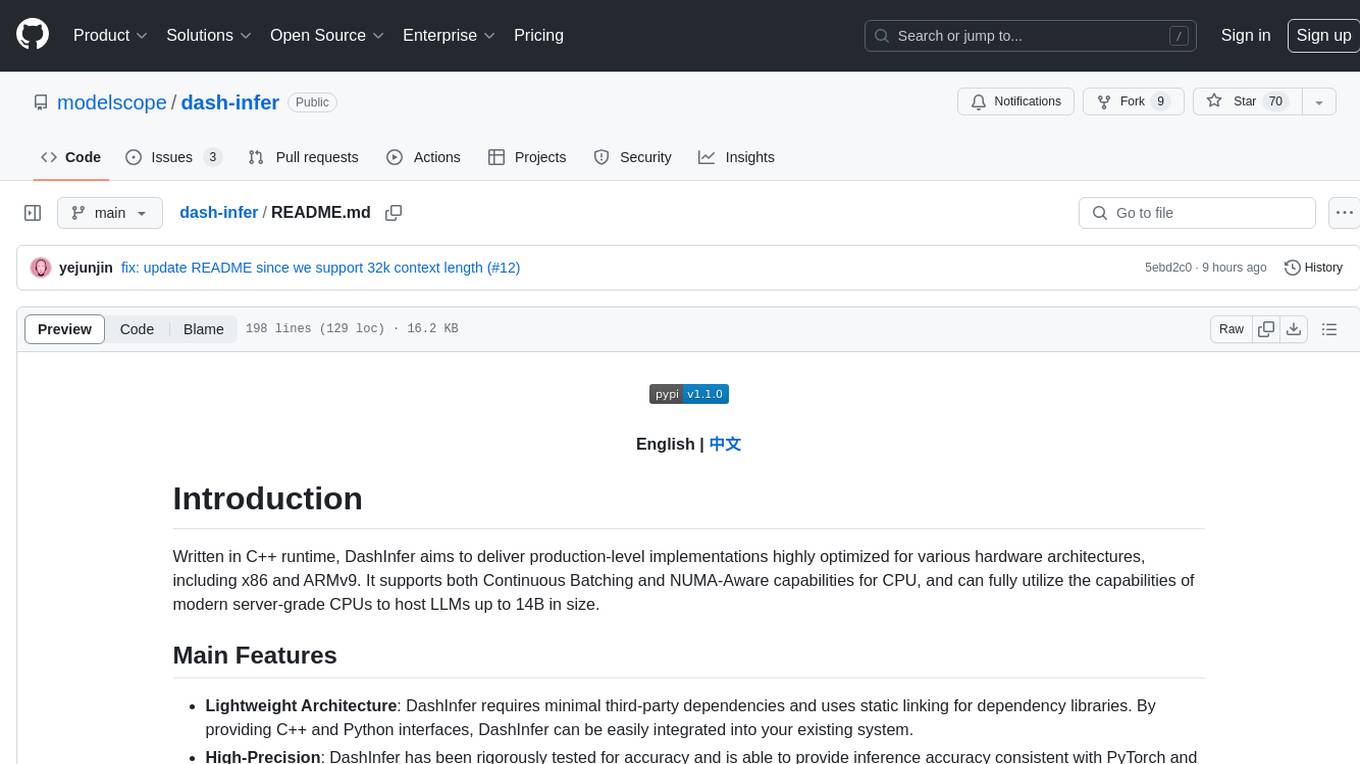
dash-infer
DashInfer is a C++ runtime tool designed to deliver production-level implementations highly optimized for various hardware architectures, including x86 and ARMv9. It supports Continuous Batching and NUMA-Aware capabilities for CPU, and can fully utilize modern server-grade CPUs to host large language models (LLMs) up to 14B in size. With lightweight architecture, high precision, support for mainstream open-source LLMs, post-training quantization, optimized computation kernels, NUMA-aware design, and multi-language API interfaces, DashInfer provides a versatile solution for efficient inference tasks. It supports x86 CPUs with AVX2 instruction set and ARMv9 CPUs with SVE instruction set, along with various data types like FP32, BF16, and InstantQuant. DashInfer also offers single-NUMA and multi-NUMA architectures for model inference, with detailed performance tests and inference accuracy evaluations available. The tool is supported on mainstream Linux server operating systems and provides documentation and examples for easy integration and usage.
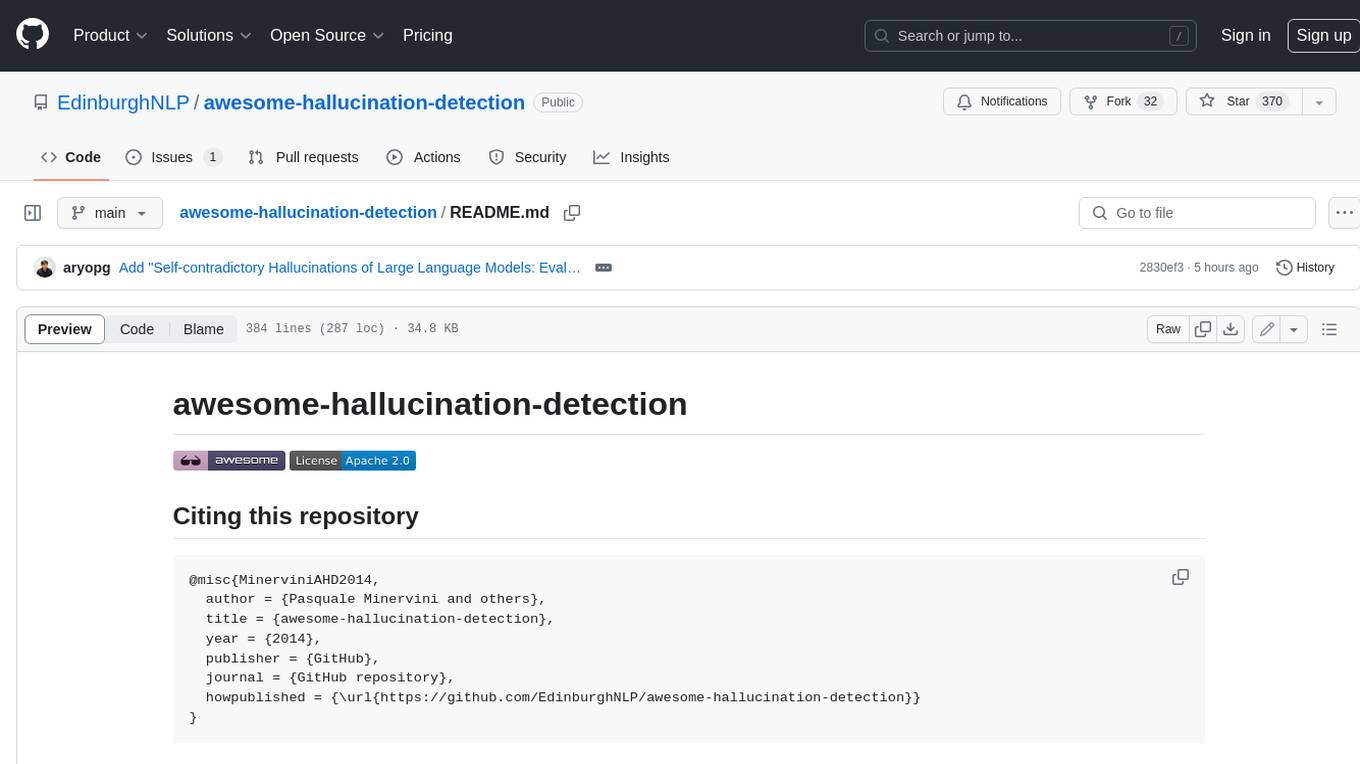
awesome-hallucination-detection
This repository provides a curated list of papers, datasets, and resources related to the detection and mitigation of hallucinations in large language models (LLMs). Hallucinations refer to the generation of factually incorrect or nonsensical text by LLMs, which can be a significant challenge for their use in real-world applications. The resources in this repository aim to help researchers and practitioners better understand and address this issue.
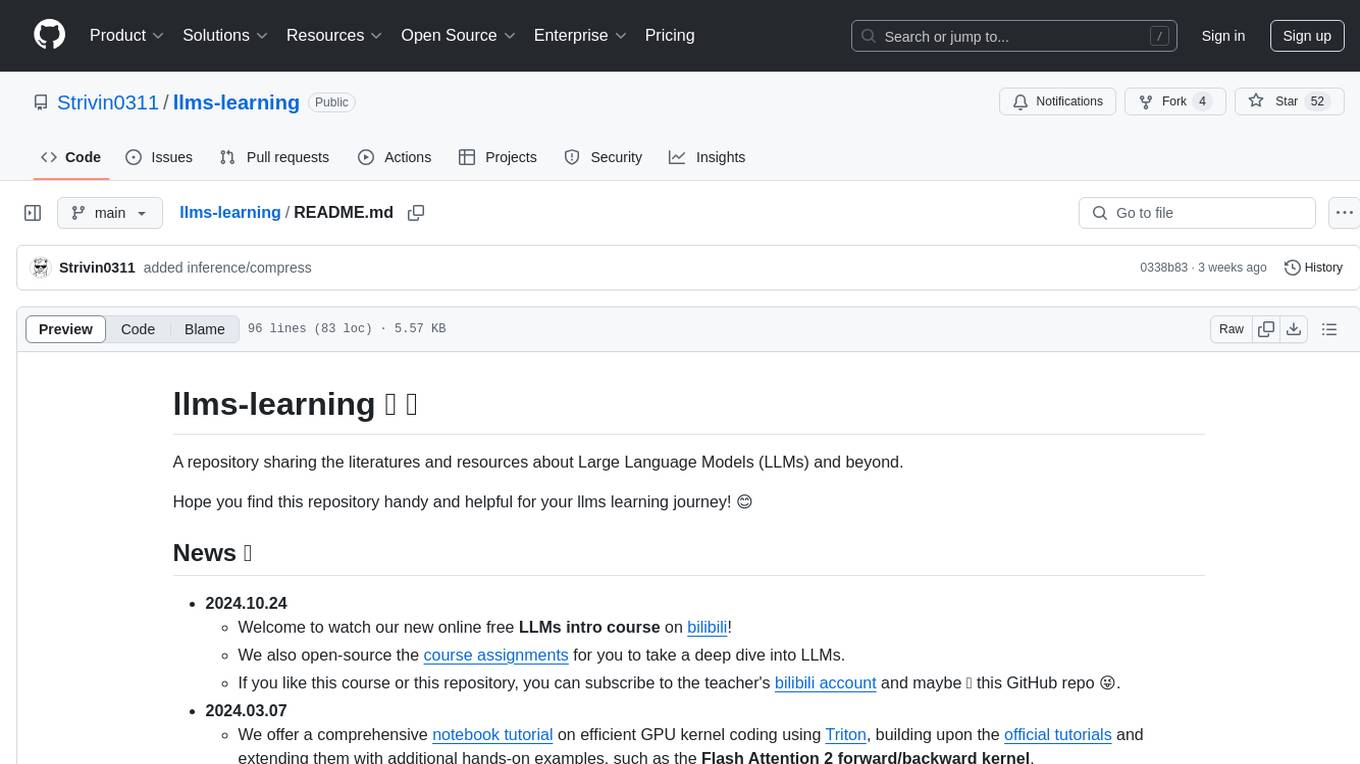
llms-learning
A repository sharing literatures and resources about Large Language Models (LLMs) and beyond. It includes tutorials, notebooks, course assignments, development stages, modeling, inference, training, applications, study, and basics related to LLMs. The repository covers various topics such as language models, transformers, state space models, multi-modal language models, training recipes, applications in autonomous driving, code, math, embodied intelligence, and more. The content is organized by different categories and provides comprehensive information on LLMs and related topics.
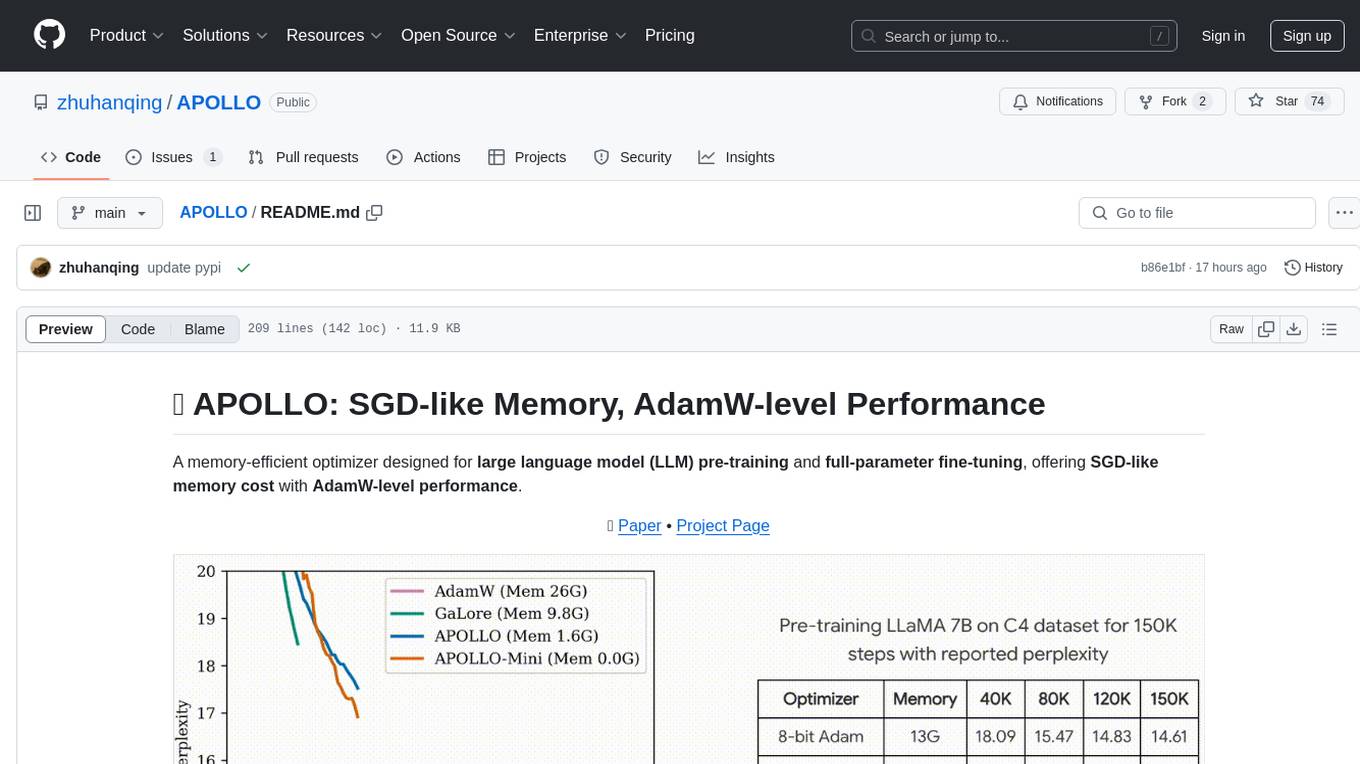
APOLLO
APOLLO is a memory-efficient optimizer designed for large language model (LLM) pre-training and full-parameter fine-tuning. It offers SGD-like memory cost with AdamW-level performance. The optimizer integrates low-rank approximation and optimizer state redundancy reduction to achieve significant memory savings while maintaining or surpassing the performance of Adam(W). Key contributions include structured learning rate updates for LLM training, approximated channel-wise gradient scaling in a low-rank auxiliary space, and minimal-rank tensor-wise gradient scaling. APOLLO aims to optimize memory efficiency during training large language models.
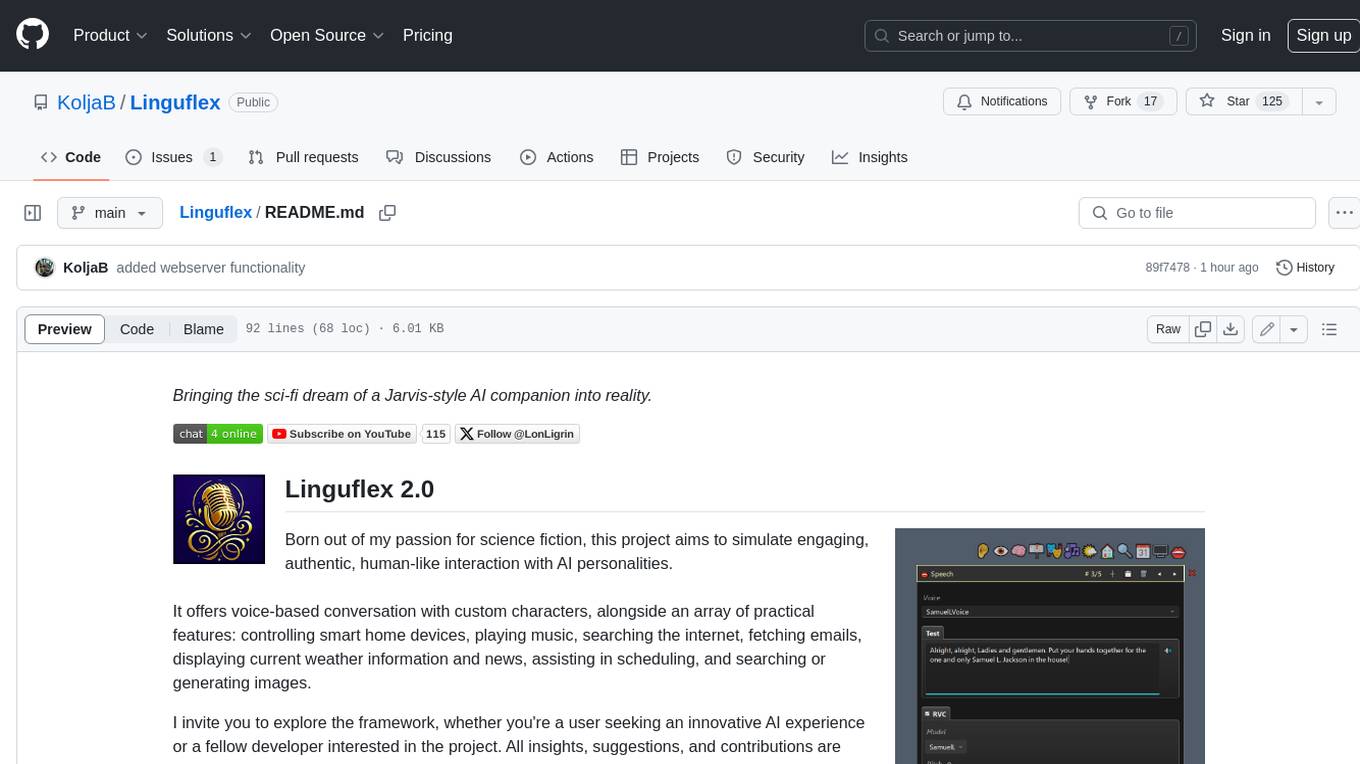
Linguflex
Linguflex is a project that aims to simulate engaging, authentic, human-like interaction with AI personalities. It offers voice-based conversation with custom characters, alongside an array of practical features such as controlling smart home devices, playing music, searching the internet, fetching emails, displaying current weather information and news, assisting in scheduling, and searching or generating images.
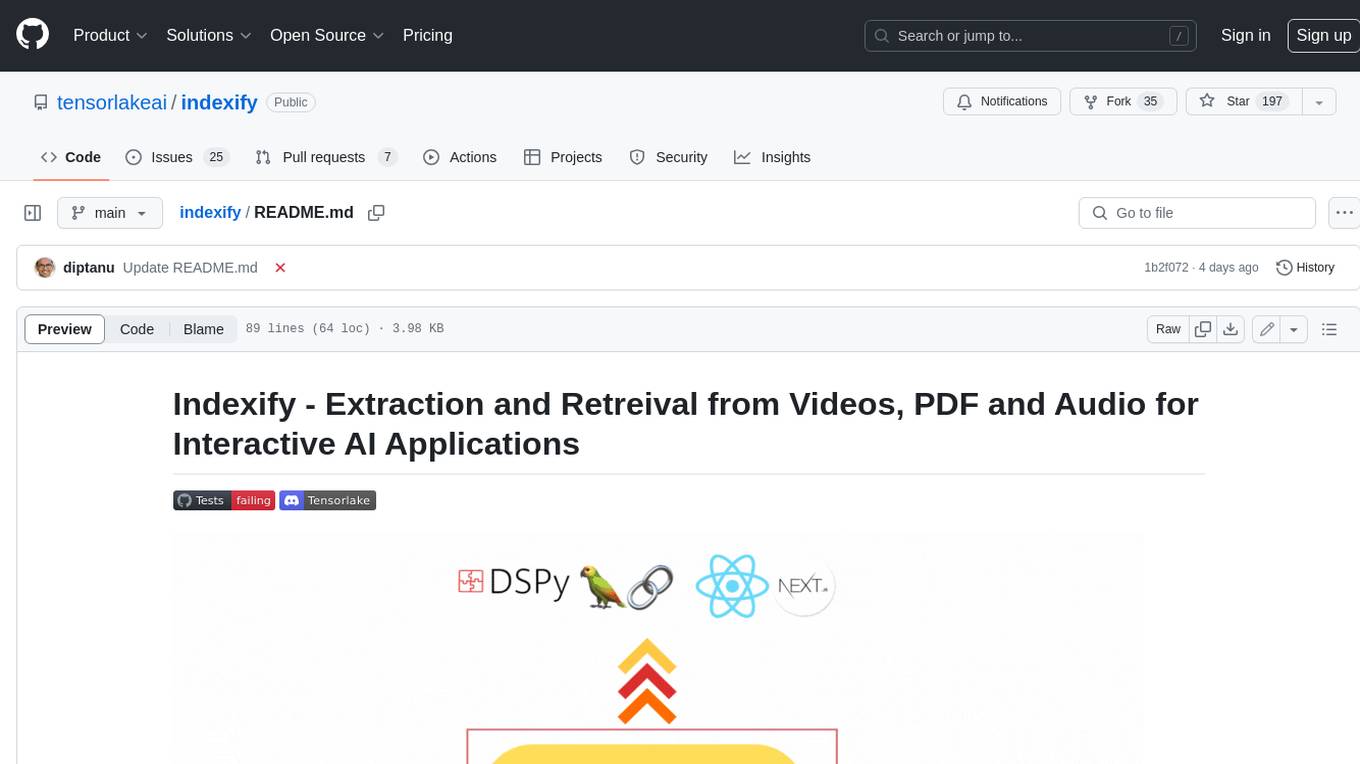
indexify
Indexify is an open-source engine for building fast data pipelines for unstructured data (video, audio, images, and documents) using reusable extractors for embedding, transformation, and feature extraction. LLM Applications can query transformed content friendly to LLMs by semantic search and SQL queries. Indexify keeps vector databases and structured databases (PostgreSQL) updated by automatically invoking the pipelines as new data is ingested into the system from external data sources. **Why use Indexify** * Makes Unstructured Data **Queryable** with **SQL** and **Semantic Search** * **Real-Time** Extraction Engine to keep indexes **automatically** updated as new data is ingested. * Create **Extraction Graph** to describe **data transformation** and extraction of **embedding** and **structured extraction**. * **Incremental Extraction** and **Selective Deletion** when content is deleted or updated. * **Extractor SDK** allows adding new extraction capabilities, and many readily available extractors for **PDF**, **Image**, and **Video** indexing and extraction. * Works with **any LLM Framework** including **Langchain**, **DSPy**, etc. * Runs on your laptop during **prototyping** and also scales to **1000s of machines** on the cloud. * Works with many **Blob Stores**, **Vector Stores**, and **Structured Databases** * We have even **Open Sourced Automation** to deploy to Kubernetes in production.
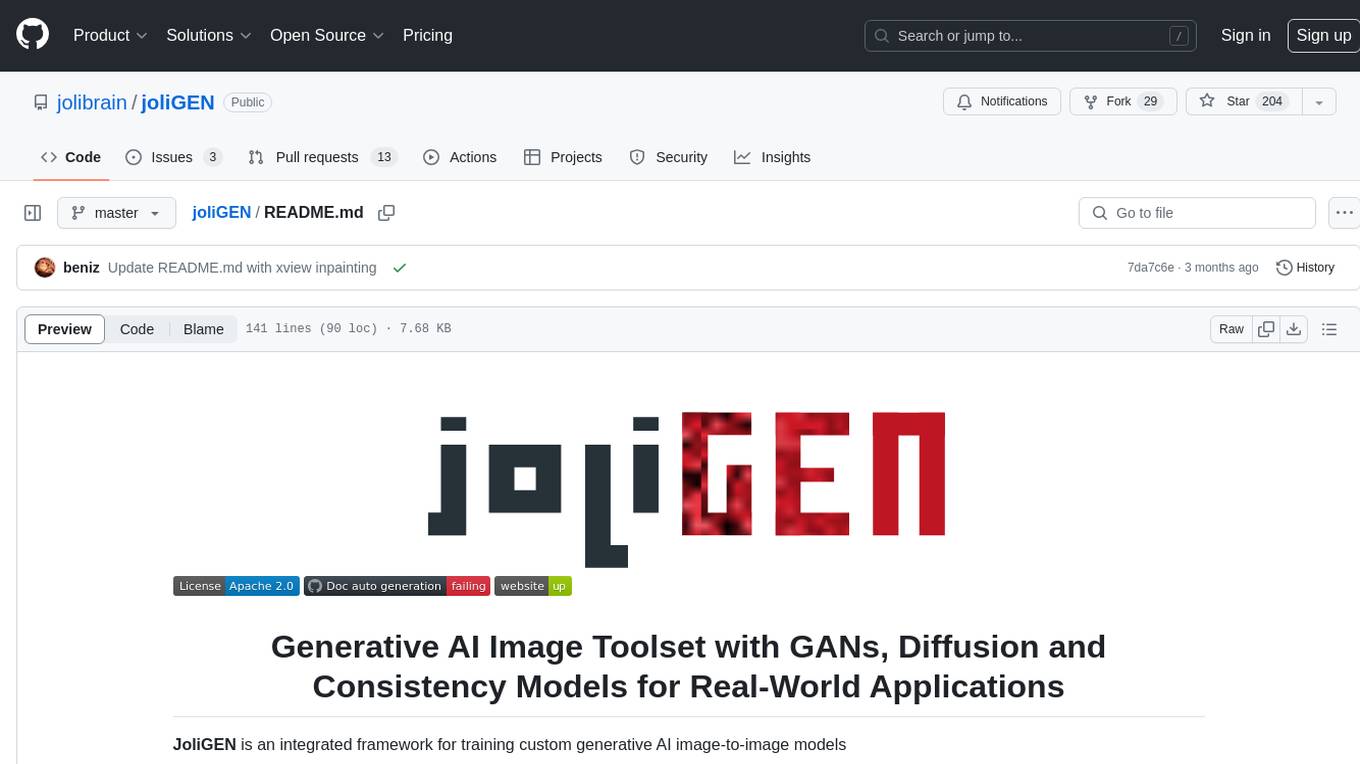
joliGEN
JoliGEN is an integrated framework for training custom generative AI image-to-image models. It implements GAN, Diffusion, and Consistency models for various image translation tasks, including domain and style adaptation with conservation of semantics. The tool is designed for real-world applications such as Controlled Image Generation, Augmented Reality, Dataset Smart Augmentation, and Synthetic to Real transforms. JoliGEN allows for fast and stable training with a REST API server for simplified deployment. It offers a wide range of options and parameters with detailed documentation available for models, dataset formats, and data augmentation.
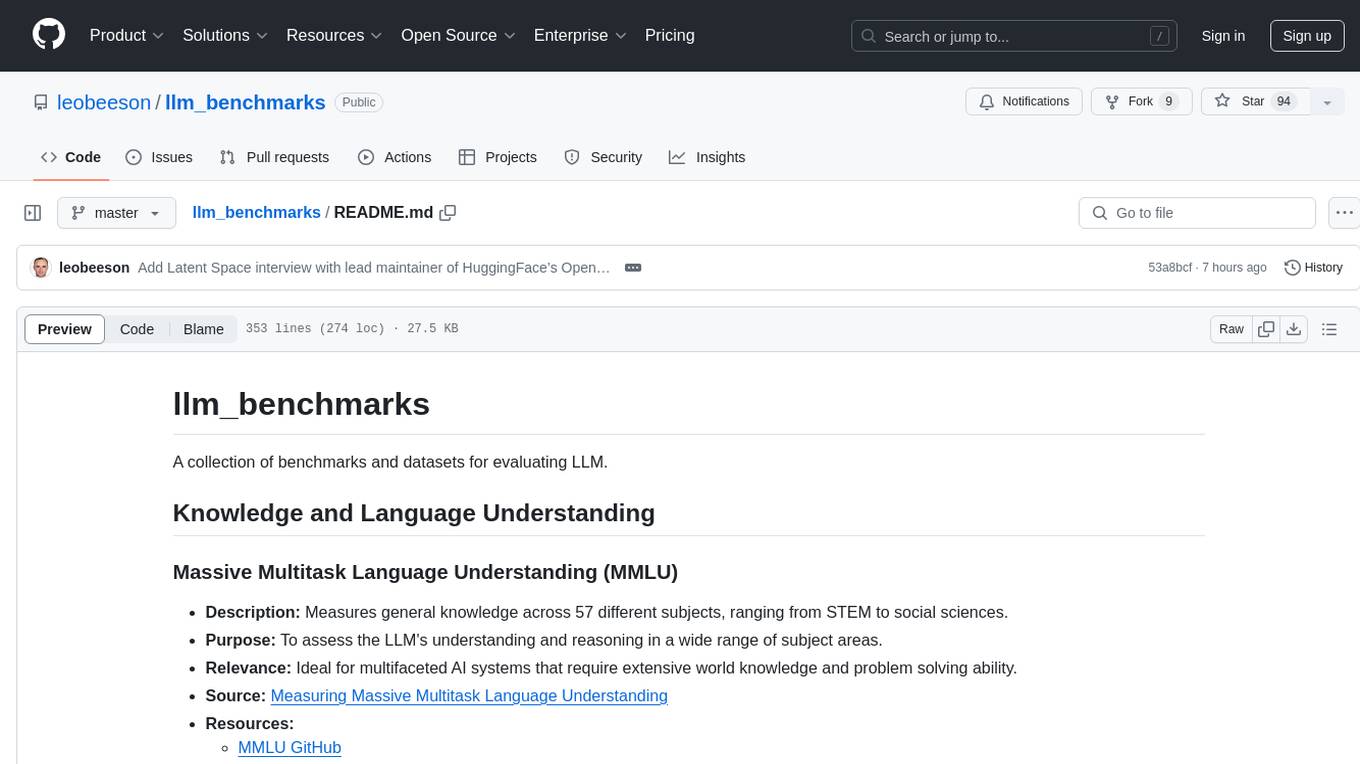
llm_benchmarks
llm_benchmarks is a collection of benchmarks and datasets for evaluating Large Language Models (LLMs). It includes various tasks and datasets to assess LLMs' knowledge, reasoning, language understanding, and conversational abilities. The repository aims to provide comprehensive evaluation resources for LLMs across different domains and applications, such as education, healthcare, content moderation, coding, and conversational AI. Researchers and developers can leverage these benchmarks to test and improve the performance of LLMs in various real-world scenarios.
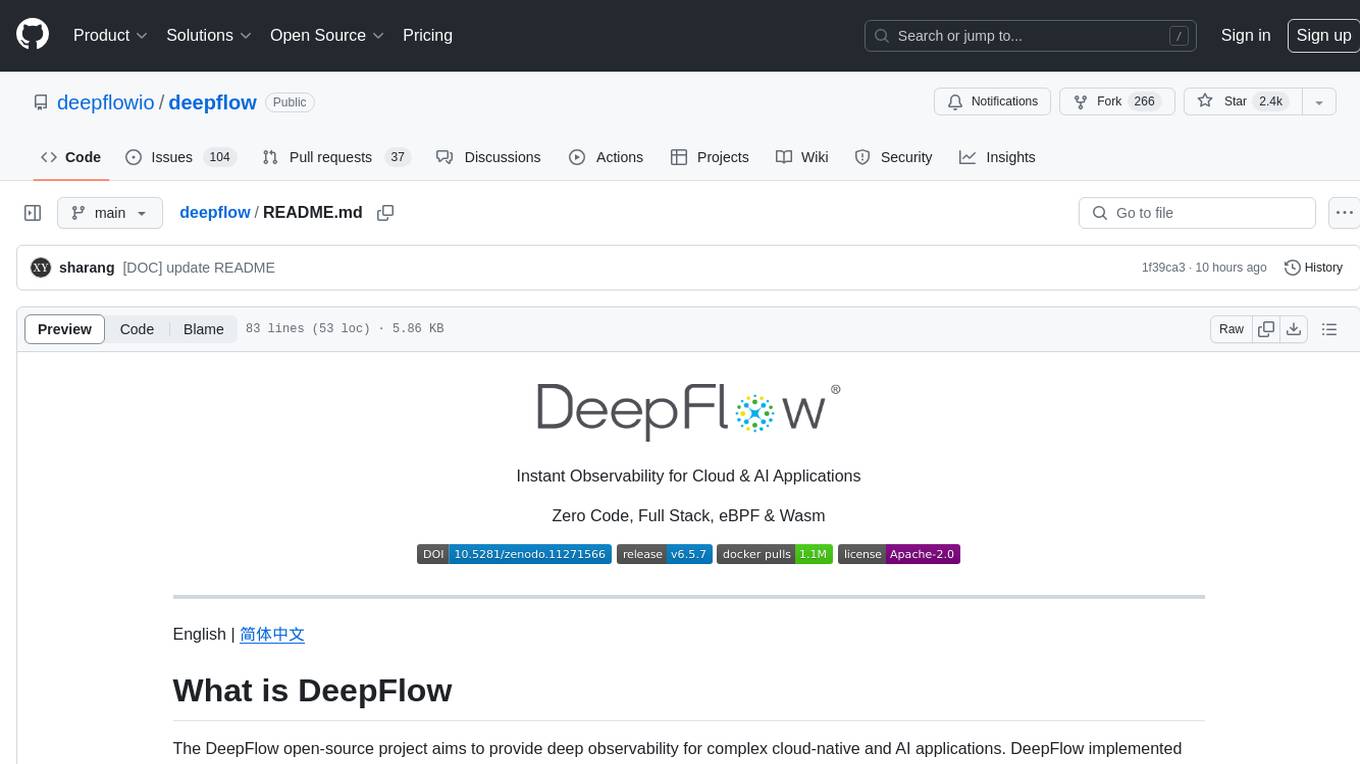
deepflow
DeepFlow is an open-source project that provides deep observability for complex cloud-native and AI applications. It offers Zero Code data collection with eBPF for metrics, distributed tracing, request logs, and function profiling. DeepFlow is integrated with SmartEncoding to achieve Full Stack correlation and efficient access to all observability data. With DeepFlow, cloud-native and AI applications automatically gain deep observability, removing the burden of developers continually instrumenting code and providing monitoring and diagnostic capabilities covering everything from code to infrastructure for DevOps/SRE teams.
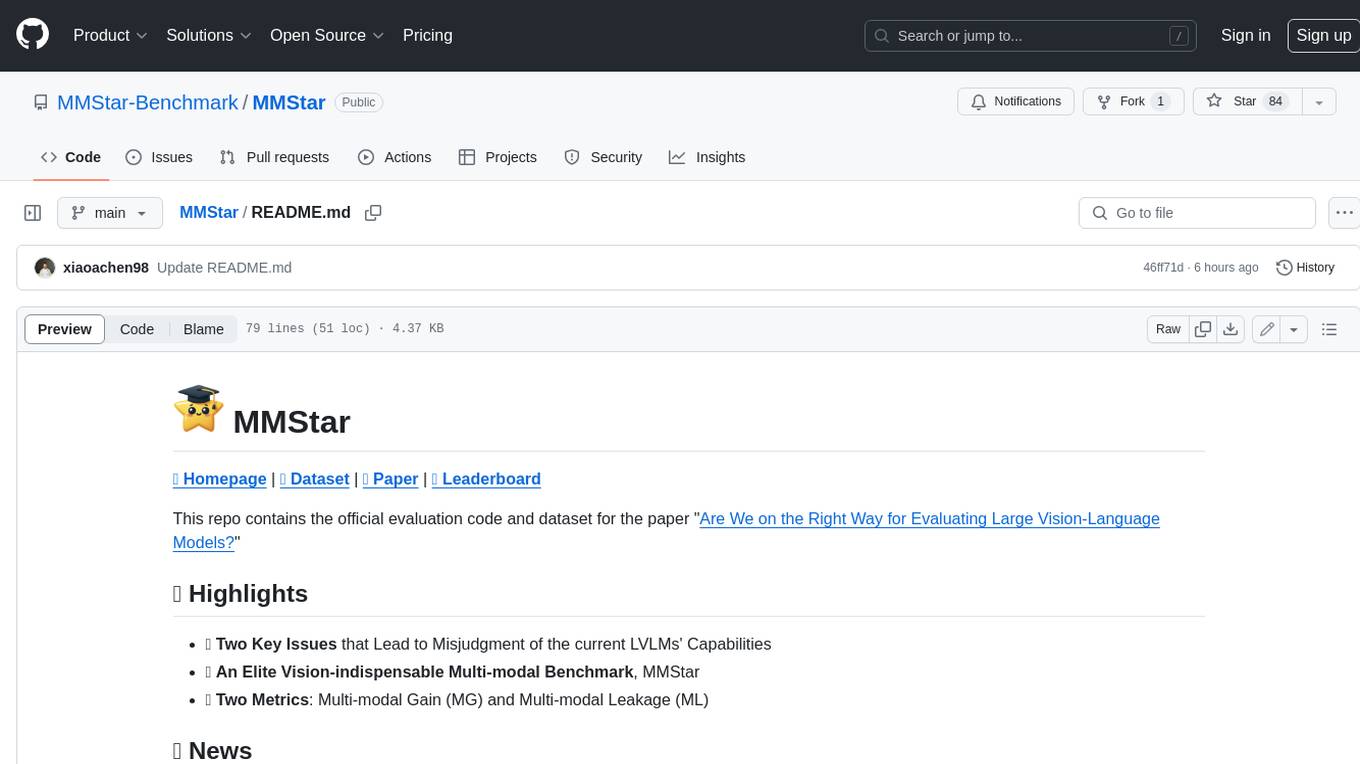
MMStar
MMStar is an elite vision-indispensable multi-modal benchmark comprising 1,500 challenge samples meticulously selected by humans. It addresses two key issues in current LLM evaluation: the unnecessary use of visual content in many samples and the existence of unintentional data leakage in LLM and LVLM training. MMStar evaluates 6 core capabilities across 18 detailed axes, ensuring a balanced distribution of samples across all dimensions.
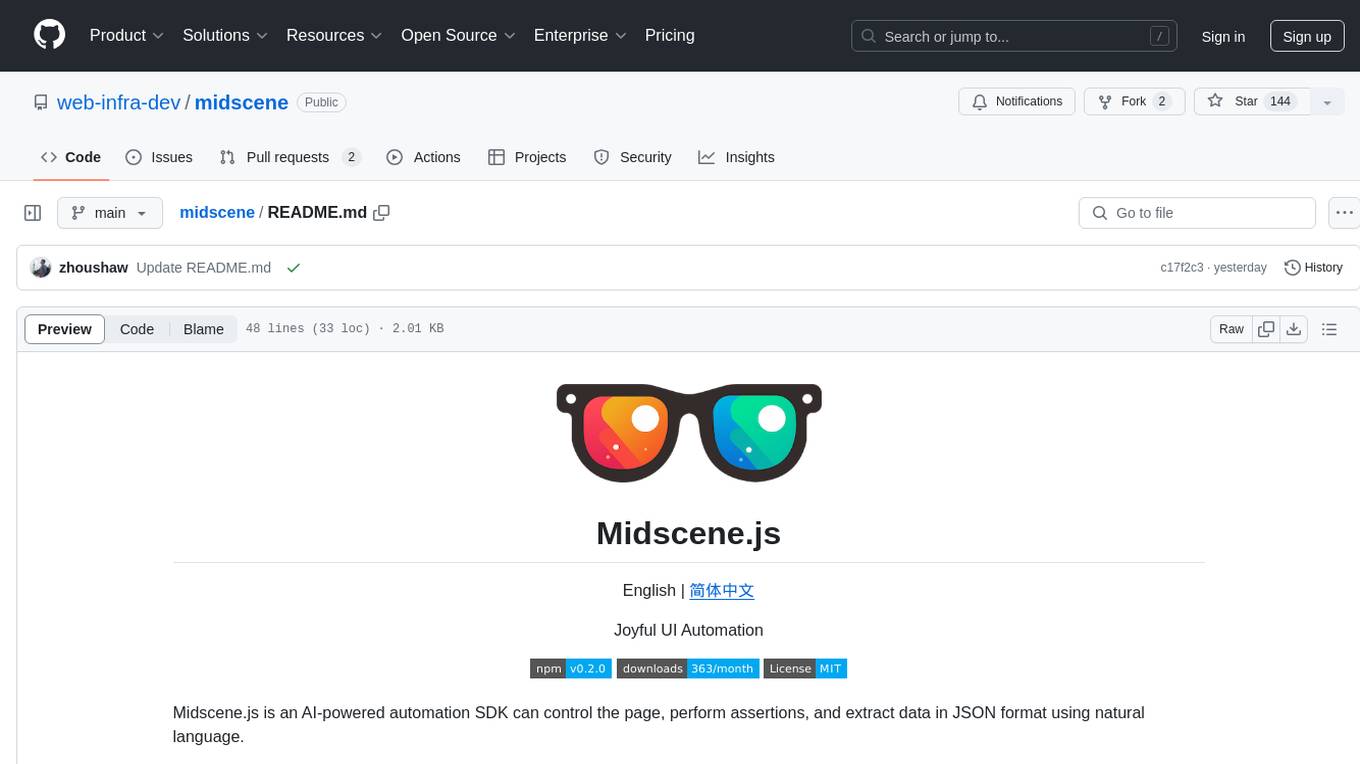
midscene
Midscene.js is an AI-powered automation SDK that allows users to control web pages, perform assertions, and extract data in JSON format using natural language. It offers features such as natural language interaction, understanding UI and providing responses in JSON, intuitive assertion based on AI understanding, compatibility with public multimodal LLMs like GPT-4o, visualization tool for easy debugging, and a brand new experience in automation development.
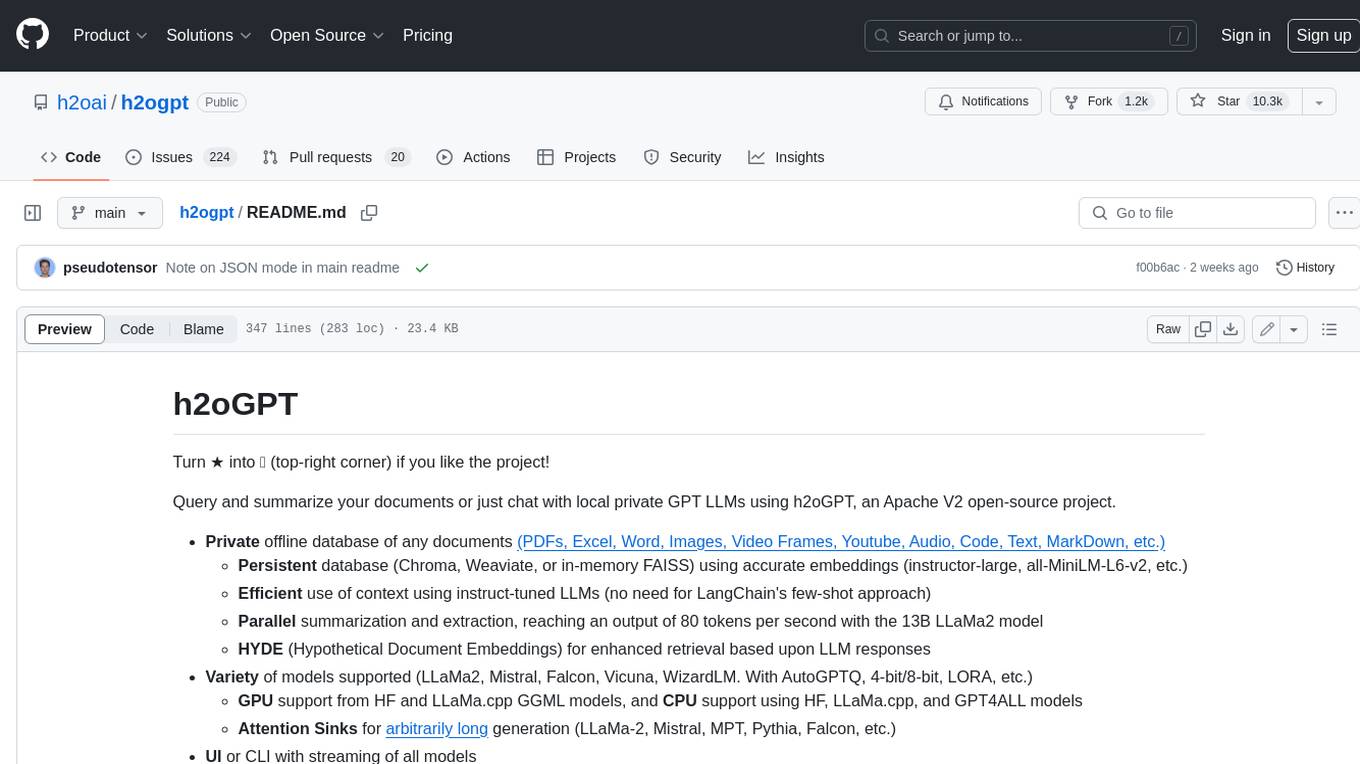
h2ogpt
h2oGPT is an Apache V2 open-source project that allows users to query and summarize documents or chat with local private GPT LLMs. It features a private offline database of any documents (PDFs, Excel, Word, Images, Video Frames, Youtube, Audio, Code, Text, MarkDown, etc.), a persistent database (Chroma, Weaviate, or in-memory FAISS) using accurate embeddings (instructor-large, all-MiniLM-L6-v2, etc.), and efficient use of context using instruct-tuned LLMs (no need for LangChain's few-shot approach). h2oGPT also offers parallel summarization and extraction, reaching an output of 80 tokens per second with the 13B LLaMa2 model, HYDE (Hypothetical Document Embeddings) for enhanced retrieval based upon LLM responses, a variety of models supported (LLaMa2, Mistral, Falcon, Vicuna, WizardLM. With AutoGPTQ, 4-bit/8-bit, LORA, etc.), GPU support from HF and LLaMa.cpp GGML models, and CPU support using HF, LLaMa.cpp, and GPT4ALL models. Additionally, h2oGPT provides Attention Sinks for arbitrarily long generation (LLaMa-2, Mistral, MPT, Pythia, Falcon, etc.), a UI or CLI with streaming of all models, the ability to upload and view documents through the UI (control multiple collaborative or personal collections), Vision Models LLaVa, Claude-3, Gemini-Pro-Vision, GPT-4-Vision, Image Generation Stable Diffusion (sdxl-turbo, sdxl) and PlaygroundAI (playv2), Voice STT using Whisper with streaming audio conversion, Voice TTS using MIT-Licensed Microsoft Speech T5 with multiple voices and Streaming audio conversion, Voice TTS using MPL2-Licensed TTS including Voice Cloning and Streaming audio conversion, AI Assistant Voice Control Mode for hands-free control of h2oGPT chat, Bake-off UI mode against many models at the same time, Easy Download of model artifacts and control over models like LLaMa.cpp through the UI, Authentication in the UI by user/password via Native or Google OAuth, State Preservation in the UI by user/password, Linux, Docker, macOS, and Windows support, Easy Windows Installer for Windows 10 64-bit (CPU/CUDA), Easy macOS Installer for macOS (CPU/M1/M2), Inference Servers support (oLLaMa, HF TGI server, vLLM, Gradio, ExLLaMa, Replicate, OpenAI, Azure OpenAI, Anthropic), OpenAI-compliant, Server Proxy API (h2oGPT acts as drop-in-replacement to OpenAI server), Python client API (to talk to Gradio server), JSON Mode with any model via code block extraction. Also supports MistralAI JSON mode, Claude-3 via function calling with strict Schema, OpenAI via JSON mode, and vLLM via guided_json with strict Schema, Web-Search integration with Chat and Document Q/A, Agents for Search, Document Q/A, Python Code, CSV frames (Experimental, best with OpenAI currently), Evaluate performance using reward models, and Quality maintained with over 1000 unit and integration tests taking over 4 GPU-hours.
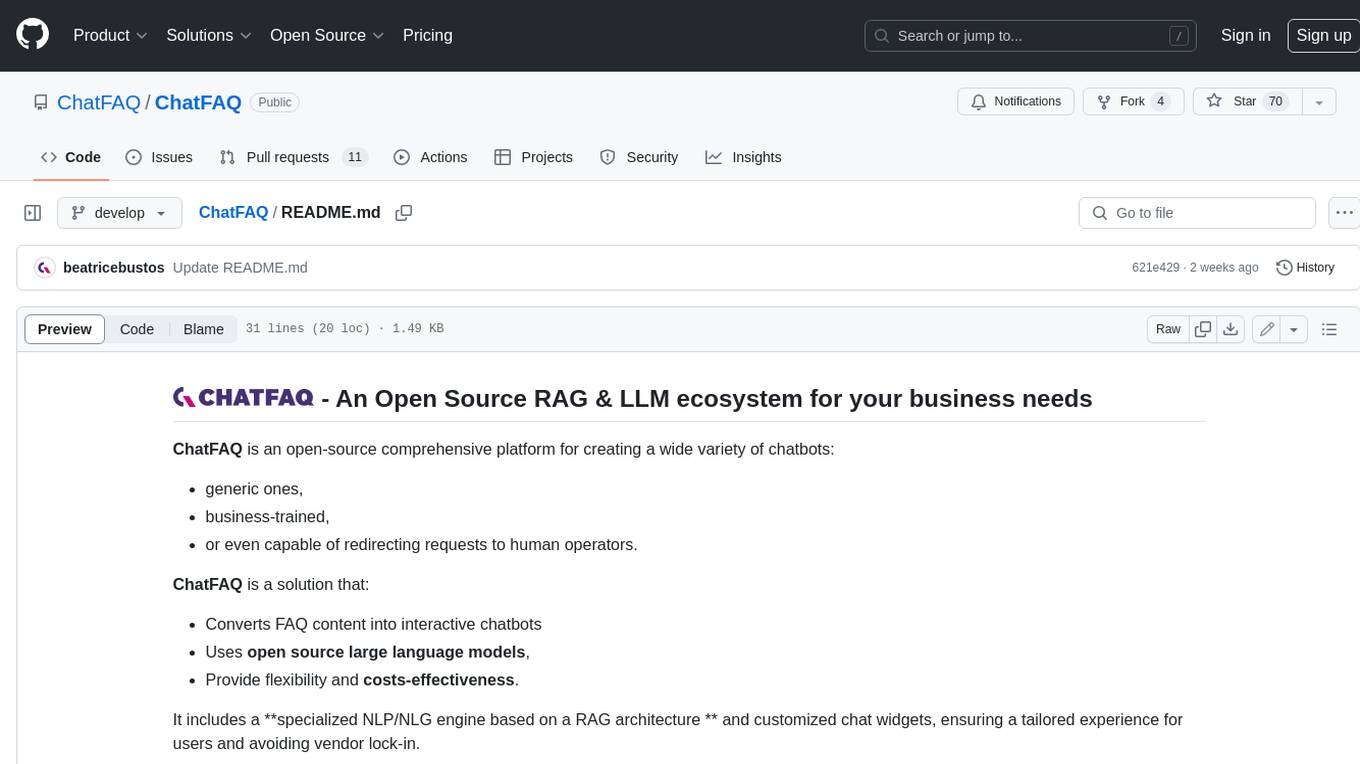
ChatFAQ
ChatFAQ is an open-source comprehensive platform for creating a wide variety of chatbots: generic ones, business-trained, or even capable of redirecting requests to human operators. It includes a specialized NLP/NLG engine based on a RAG architecture and customized chat widgets, ensuring a tailored experience for users and avoiding vendor lock-in.
For similar tasks
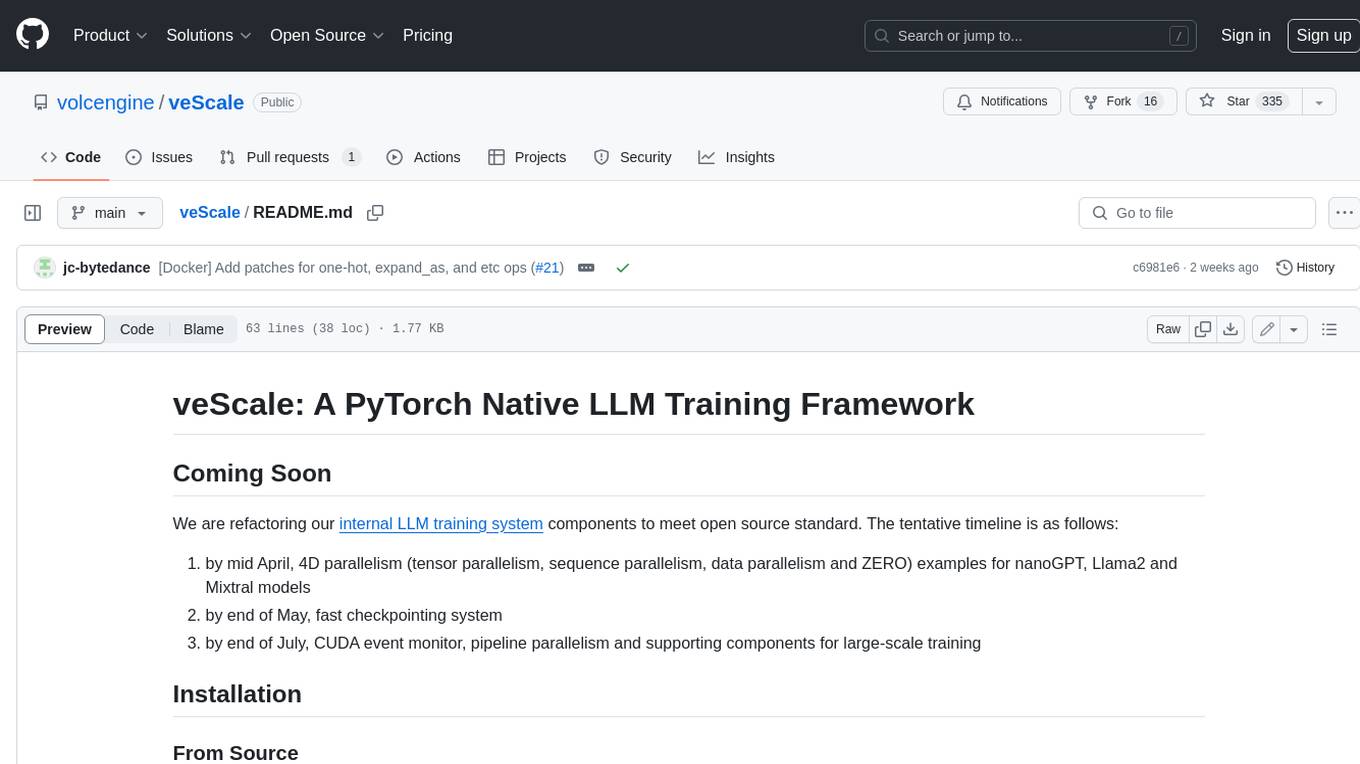
veScale
veScale is a PyTorch Native LLM Training Framework. It provides a set of tools and components to facilitate the training of large language models (LLMs) using PyTorch. veScale includes features such as 4D parallelism, fast checkpointing, and a CUDA event monitor. It is designed to be scalable and efficient, and it can be used to train LLMs on a variety of hardware platforms.
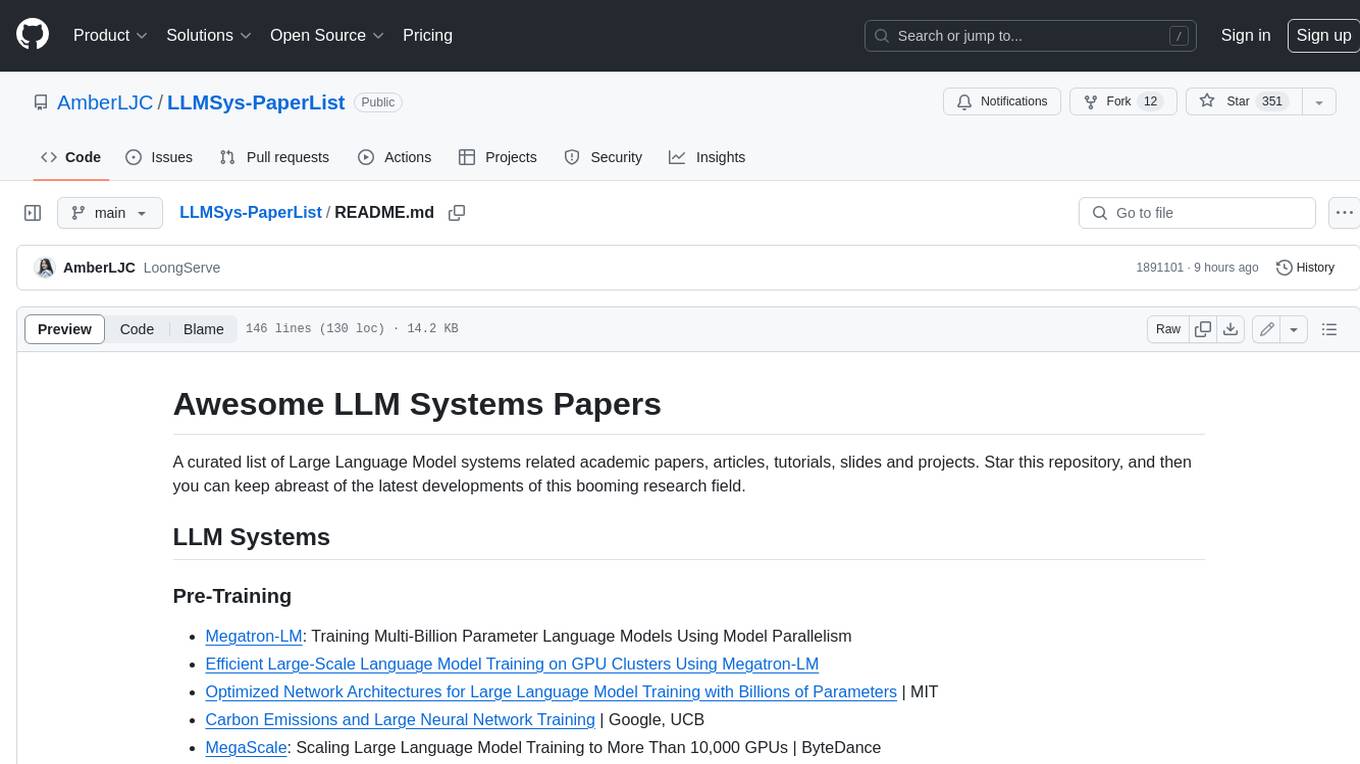
LLMSys-PaperList
This repository provides a comprehensive list of academic papers, articles, tutorials, slides, and projects related to Large Language Model (LLM) systems. It covers various aspects of LLM research, including pre-training, serving, system efficiency optimization, multi-model systems, image generation systems, LLM applications in systems, ML systems, survey papers, LLM benchmarks and leaderboards, and other relevant resources. The repository is regularly updated to include the latest developments in this rapidly evolving field, making it a valuable resource for researchers, practitioners, and anyone interested in staying abreast of the advancements in LLM technology.
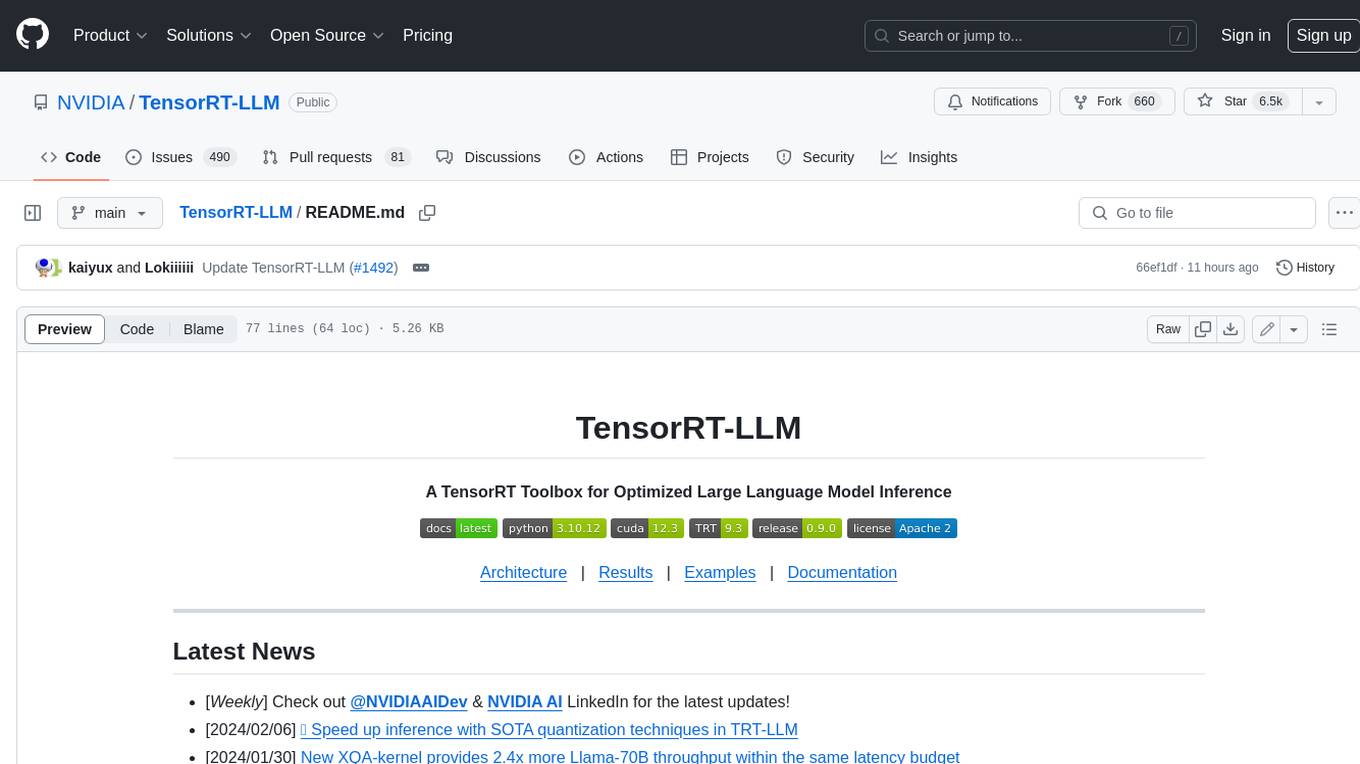
TensorRT-LLM
TensorRT-LLM is an easy-to-use Python API to define Large Language Models (LLMs) and build TensorRT engines that contain state-of-the-art optimizations to perform inference efficiently on NVIDIA GPUs. TensorRT-LLM contains components to create Python and C++ runtimes that execute those TensorRT engines. It also includes a backend for integration with the NVIDIA Triton Inference Server; a production-quality system to serve LLMs. Models built with TensorRT-LLM can be executed on a wide range of configurations going from a single GPU to multiple nodes with multiple GPUs (using Tensor Parallelism and/or Pipeline Parallelism).
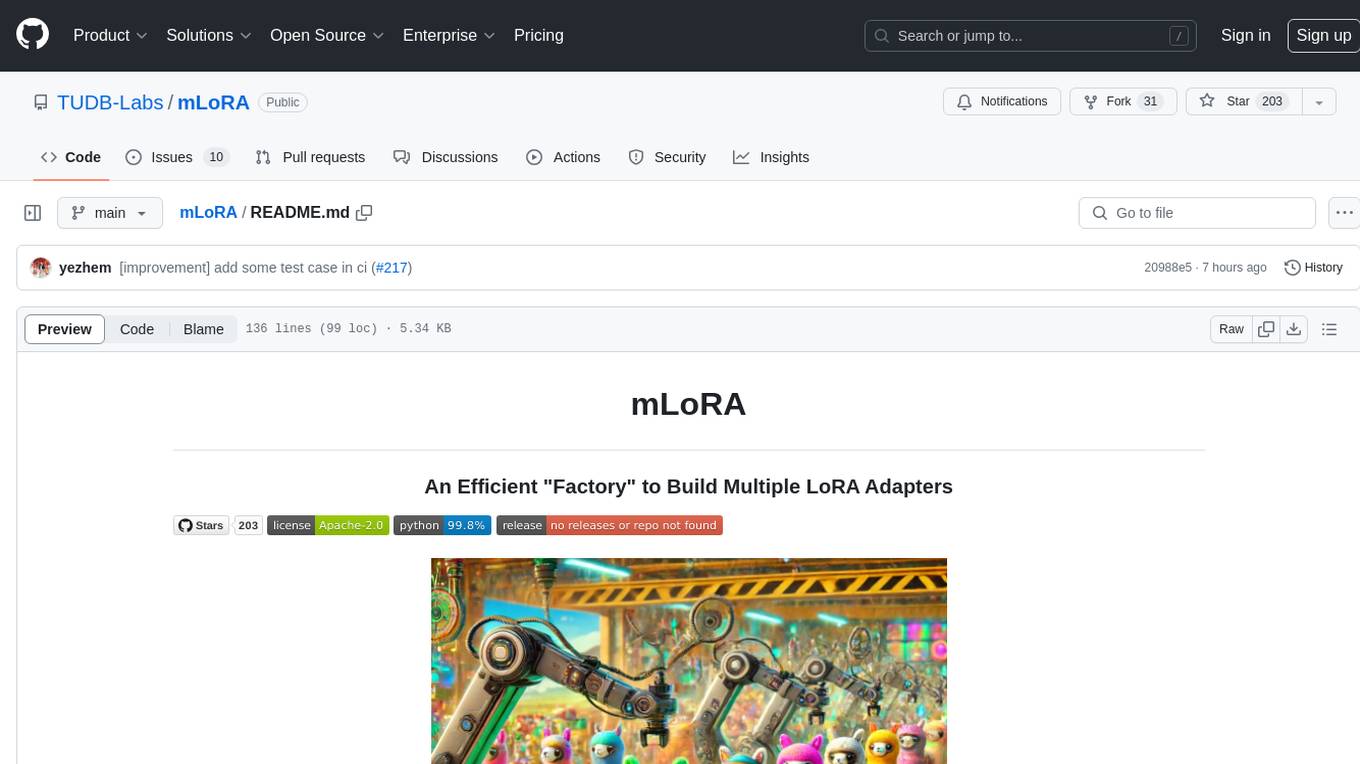
mLoRA
mLoRA (Multi-LoRA Fine-Tune) is an open-source framework for efficient fine-tuning of multiple Large Language Models (LLMs) using LoRA and its variants. It allows concurrent fine-tuning of multiple LoRA adapters with a shared base model, efficient pipeline parallelism algorithm, support for various LoRA variant algorithms, and reinforcement learning preference alignment algorithms. mLoRA helps save computational and memory resources when training multiple adapters simultaneously, achieving high performance on consumer hardware.
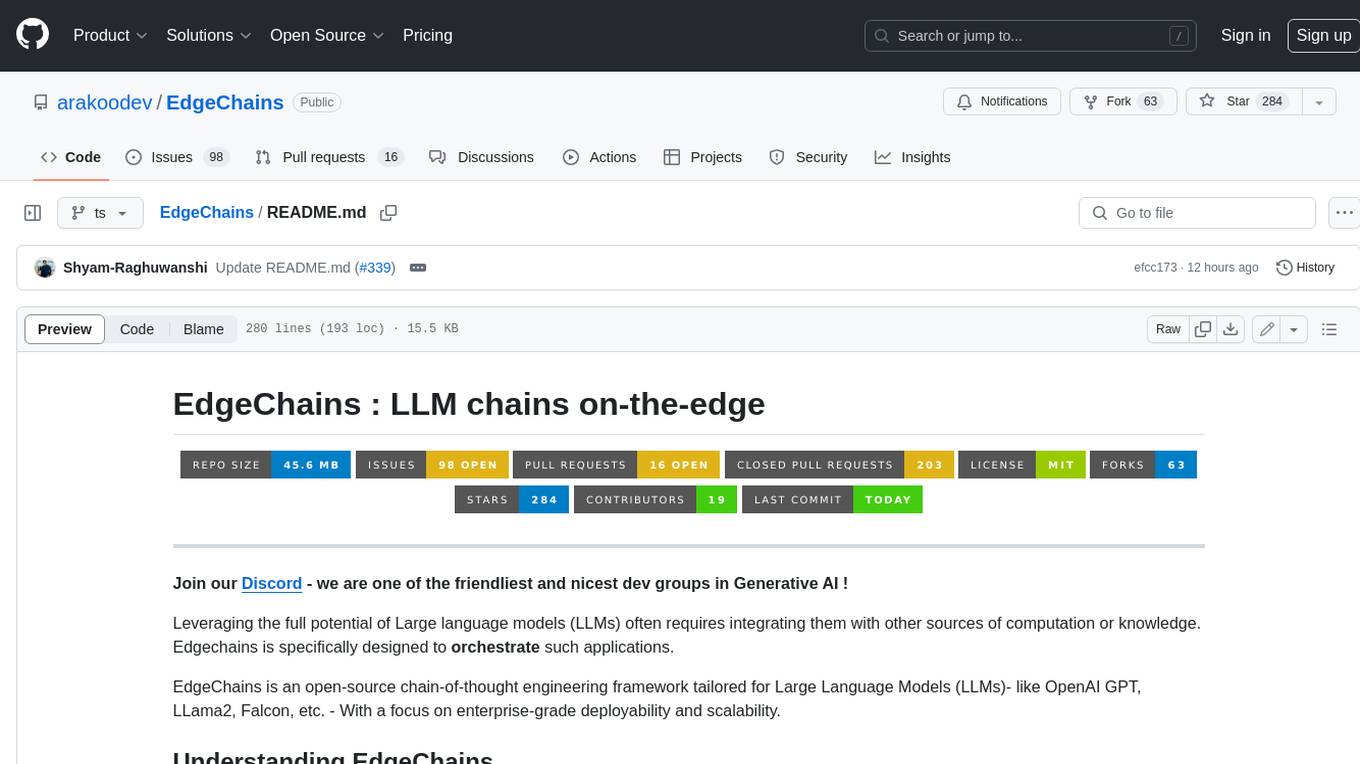
EdgeChains
EdgeChains is an open-source chain-of-thought engineering framework tailored for Large Language Models (LLMs)- like OpenAI GPT, LLama2, Falcon, etc. - With a focus on enterprise-grade deployability and scalability. EdgeChains is specifically designed to **orchestrate** such applications. At EdgeChains, we take a unique approach to Generative AI - we think Generative AI is a deployment and configuration management challenge rather than a UI and library design pattern challenge. We build on top of a tech that has solved this problem in a different domain - Kubernetes Config Management - and bring that to Generative AI. Edgechains is built on top of jsonnet, originally built by Google based on their experience managing a vast amount of configuration code in the Borg infrastructure.
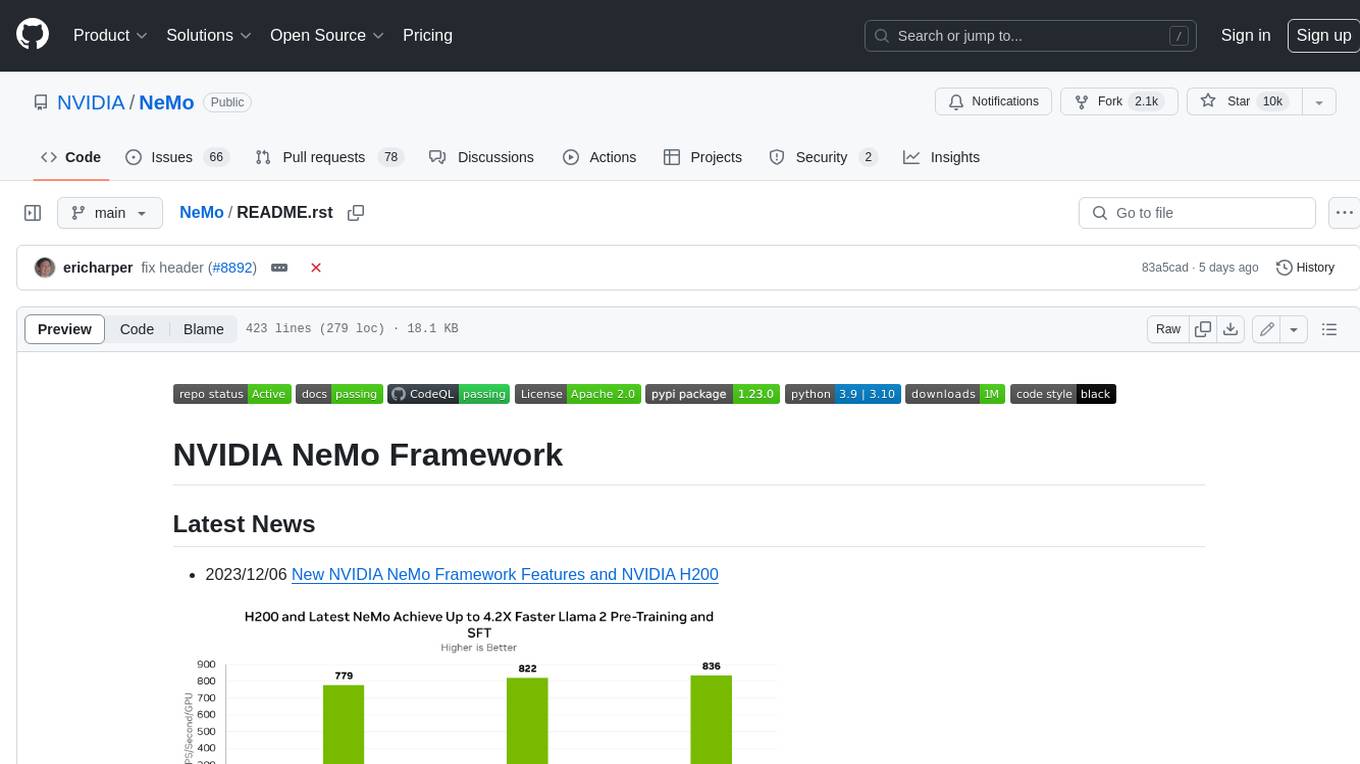
NeMo
NeMo Framework is a generative AI framework built for researchers and pytorch developers working on large language models (LLMs), multimodal models (MM), automatic speech recognition (ASR), and text-to-speech synthesis (TTS). The primary objective of NeMo is to provide a scalable framework for researchers and developers from industry and academia to more easily implement and design new generative AI models by being able to leverage existing code and pretrained models.
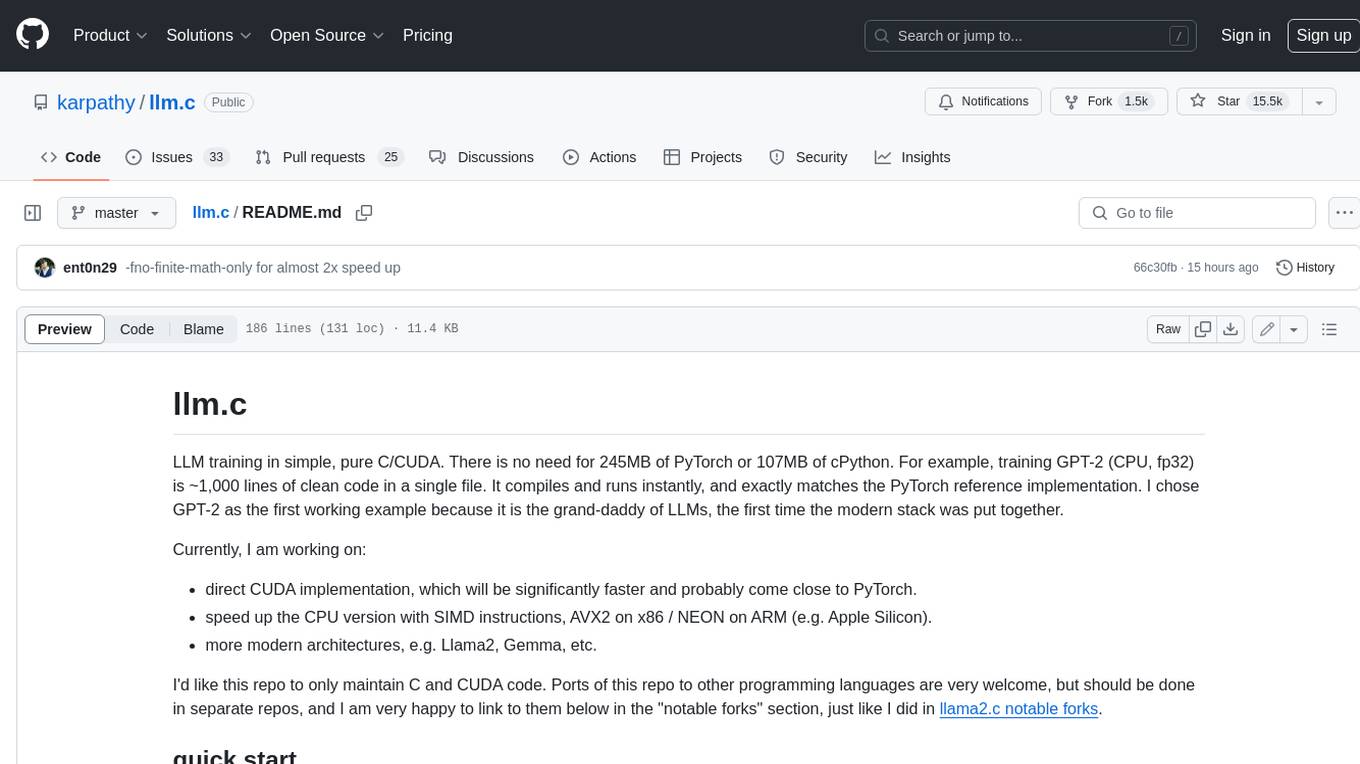
llm.c
LLM training in simple, pure C/CUDA. There is no need for 245MB of PyTorch or 107MB of cPython. For example, training GPT-2 (CPU, fp32) is ~1,000 lines of clean code in a single file. It compiles and runs instantly, and exactly matches the PyTorch reference implementation. I chose GPT-2 as the first working example because it is the grand-daddy of LLMs, the first time the modern stack was put together.
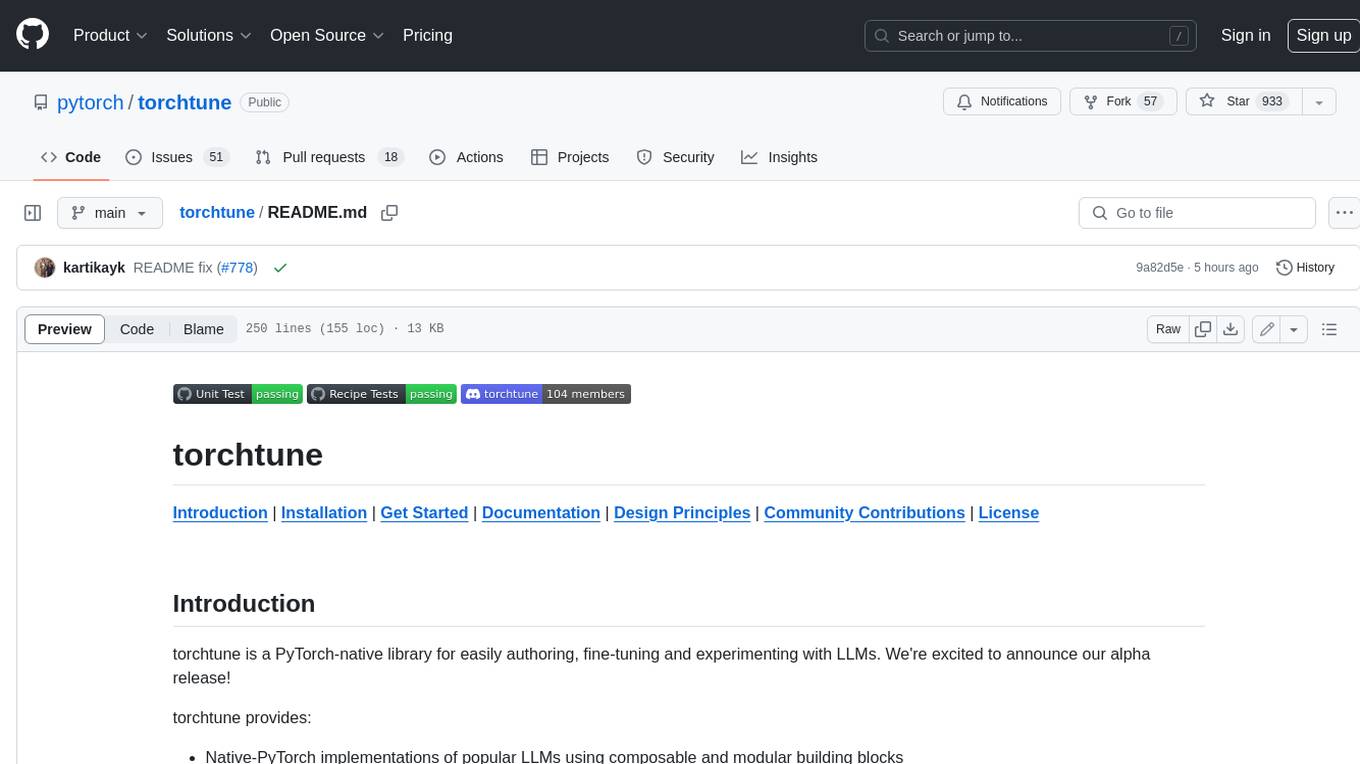
torchtune
Torchtune is a PyTorch-native library for easily authoring, fine-tuning, and experimenting with LLMs. It provides native-PyTorch implementations of popular LLMs using composable and modular building blocks, easy-to-use and hackable training recipes for popular fine-tuning techniques, YAML configs for easily configuring training, evaluation, quantization, or inference recipes, and built-in support for many popular dataset formats and prompt templates to help you quickly get started with training.
For similar jobs
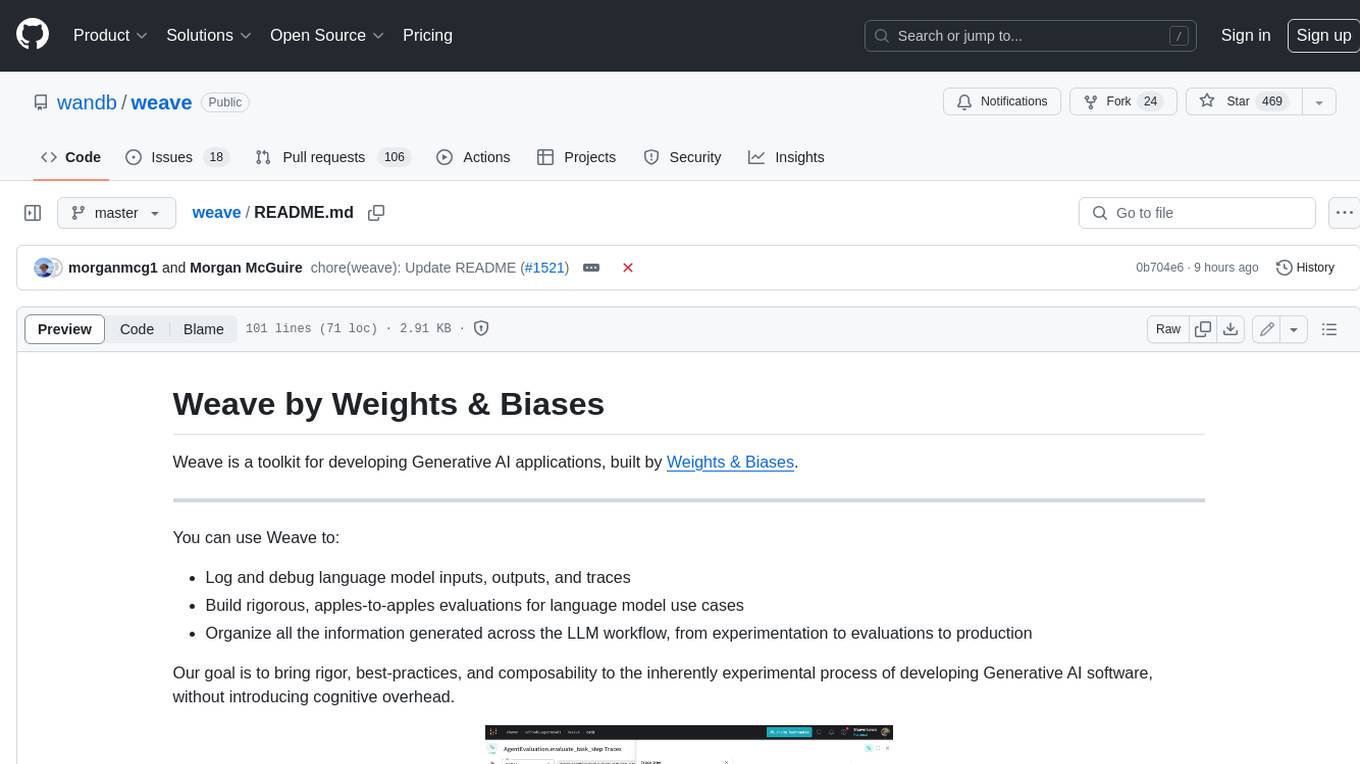
weave
Weave is a toolkit for developing Generative AI applications, built by Weights & Biases. With Weave, you can log and debug language model inputs, outputs, and traces; build rigorous, apples-to-apples evaluations for language model use cases; and organize all the information generated across the LLM workflow, from experimentation to evaluations to production. Weave aims to bring rigor, best-practices, and composability to the inherently experimental process of developing Generative AI software, without introducing cognitive overhead.
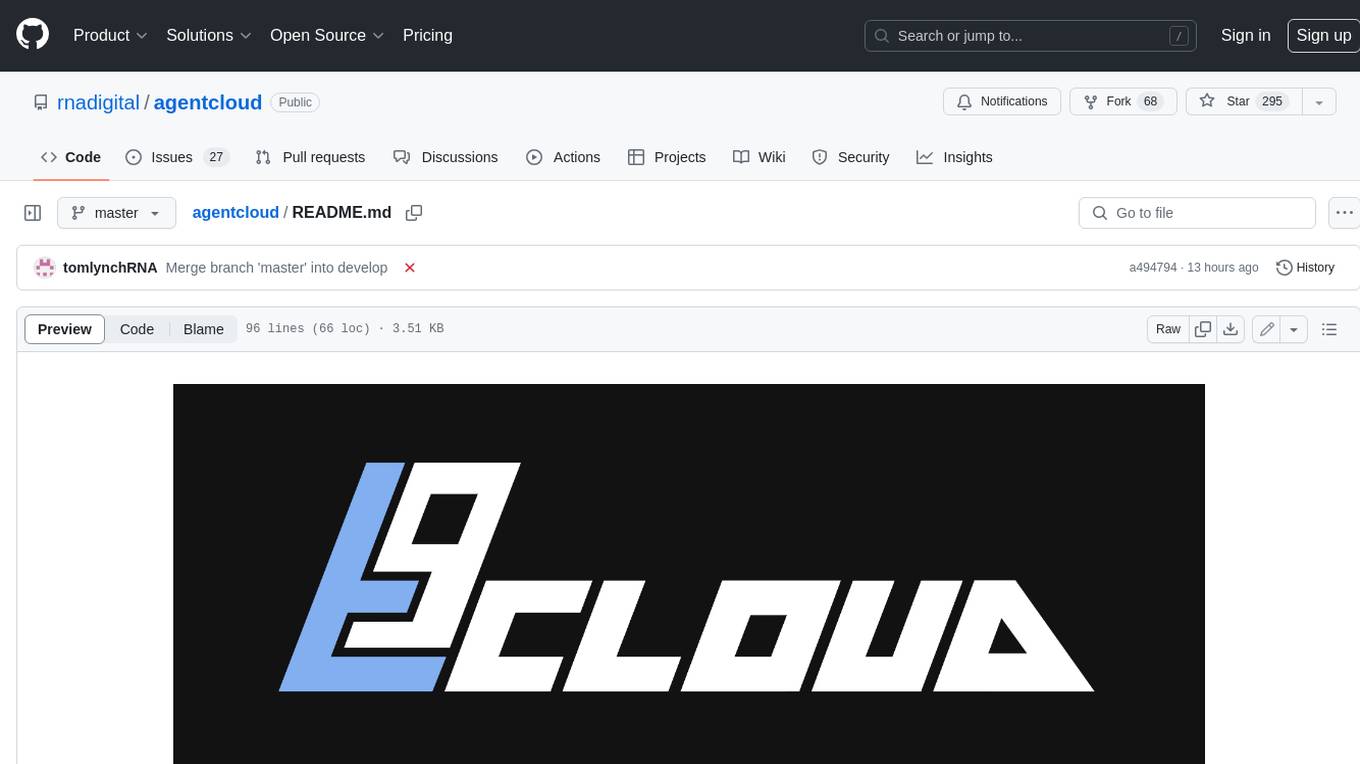
agentcloud
AgentCloud is an open-source platform that enables companies to build and deploy private LLM chat apps, empowering teams to securely interact with their data. It comprises three main components: Agent Backend, Webapp, and Vector Proxy. To run this project locally, clone the repository, install Docker, and start the services. The project is licensed under the GNU Affero General Public License, version 3 only. Contributions and feedback are welcome from the community.
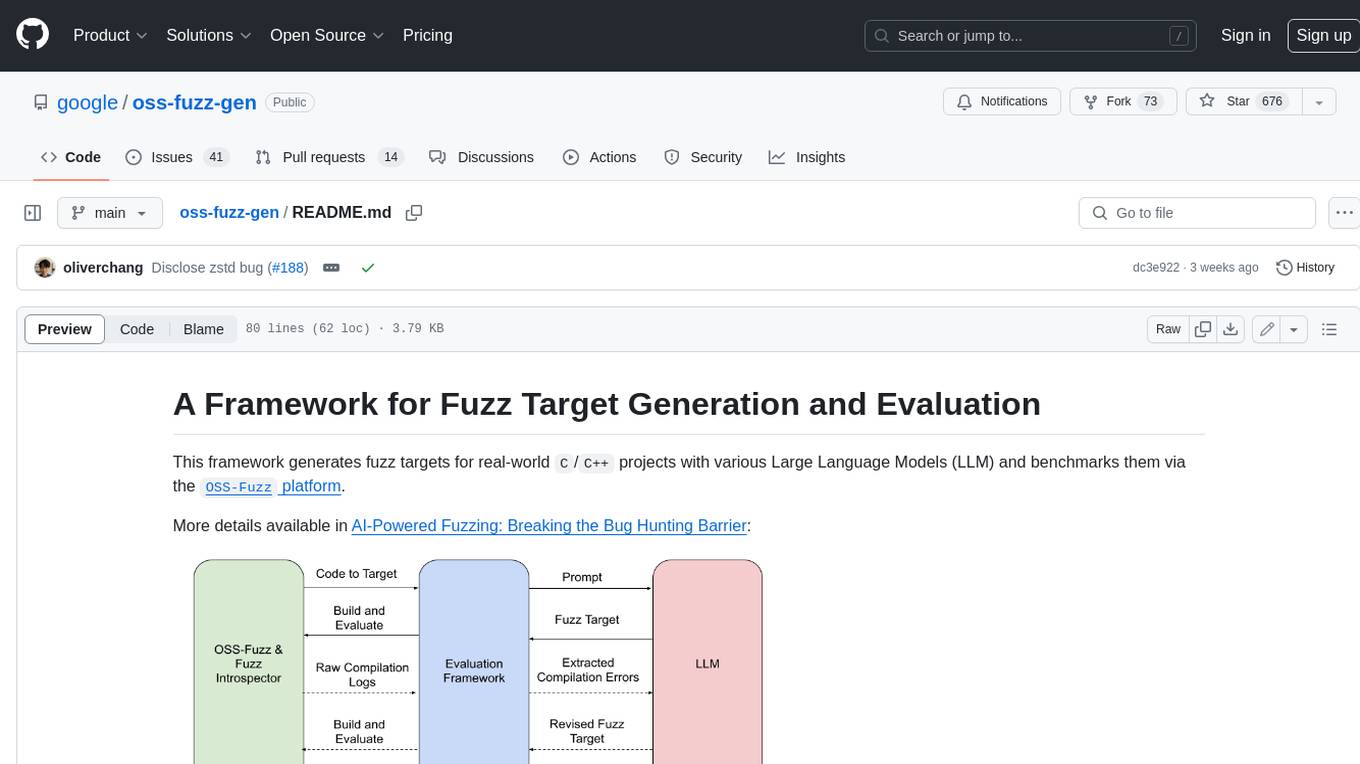
oss-fuzz-gen
This framework generates fuzz targets for real-world `C`/`C++` projects with various Large Language Models (LLM) and benchmarks them via the `OSS-Fuzz` platform. It manages to successfully leverage LLMs to generate valid fuzz targets (which generate non-zero coverage increase) for 160 C/C++ projects. The maximum line coverage increase is 29% from the existing human-written targets.
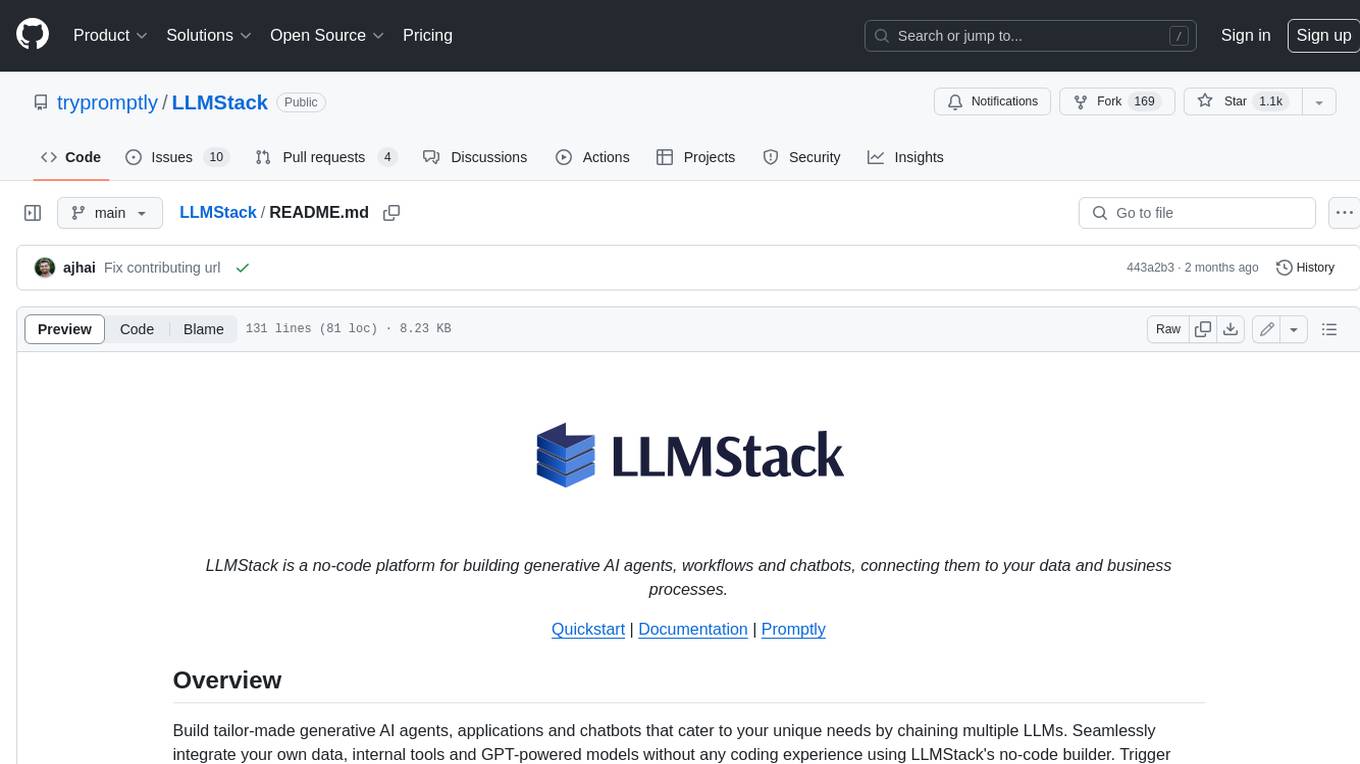
LLMStack
LLMStack is a no-code platform for building generative AI agents, workflows, and chatbots. It allows users to connect their own data, internal tools, and GPT-powered models without any coding experience. LLMStack can be deployed to the cloud or on-premise and can be accessed via HTTP API or triggered from Slack or Discord.
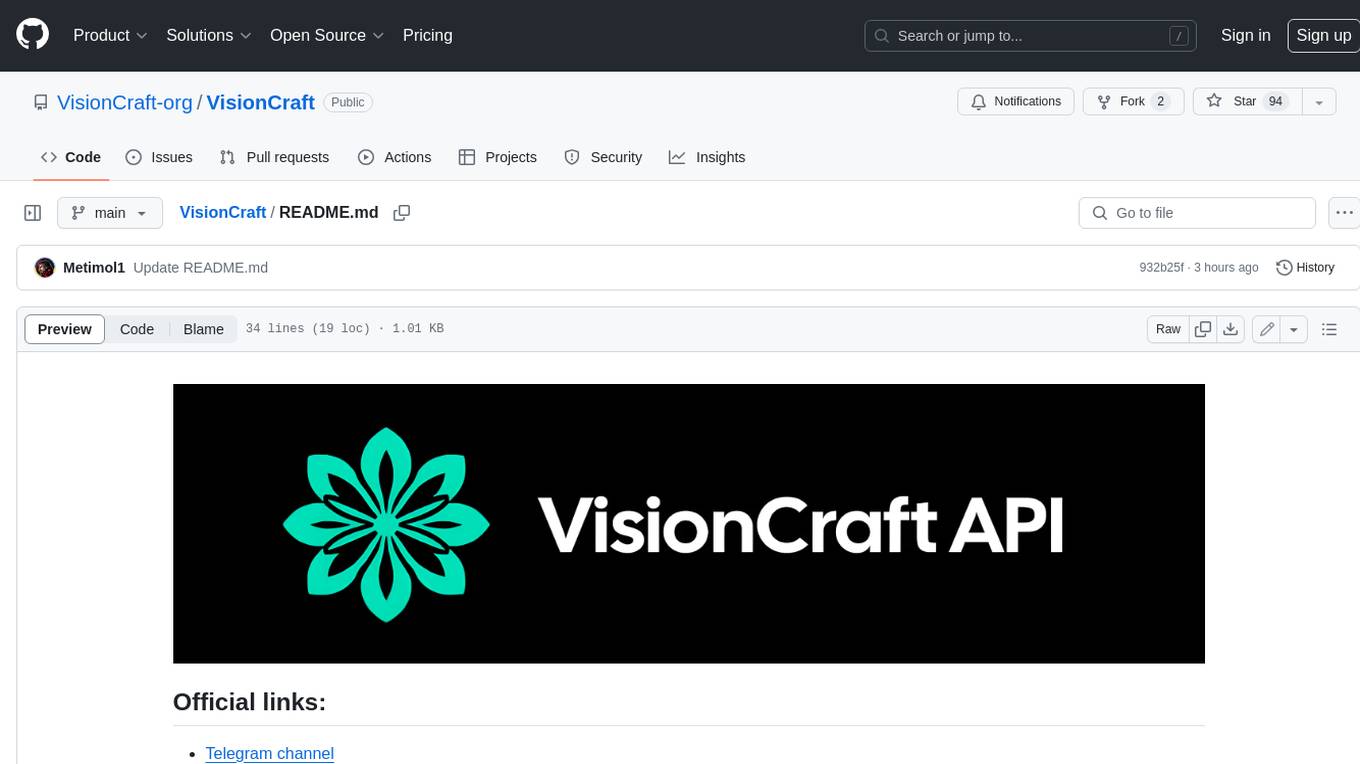
VisionCraft
The VisionCraft API is a free API for using over 100 different AI models. From images to sound.
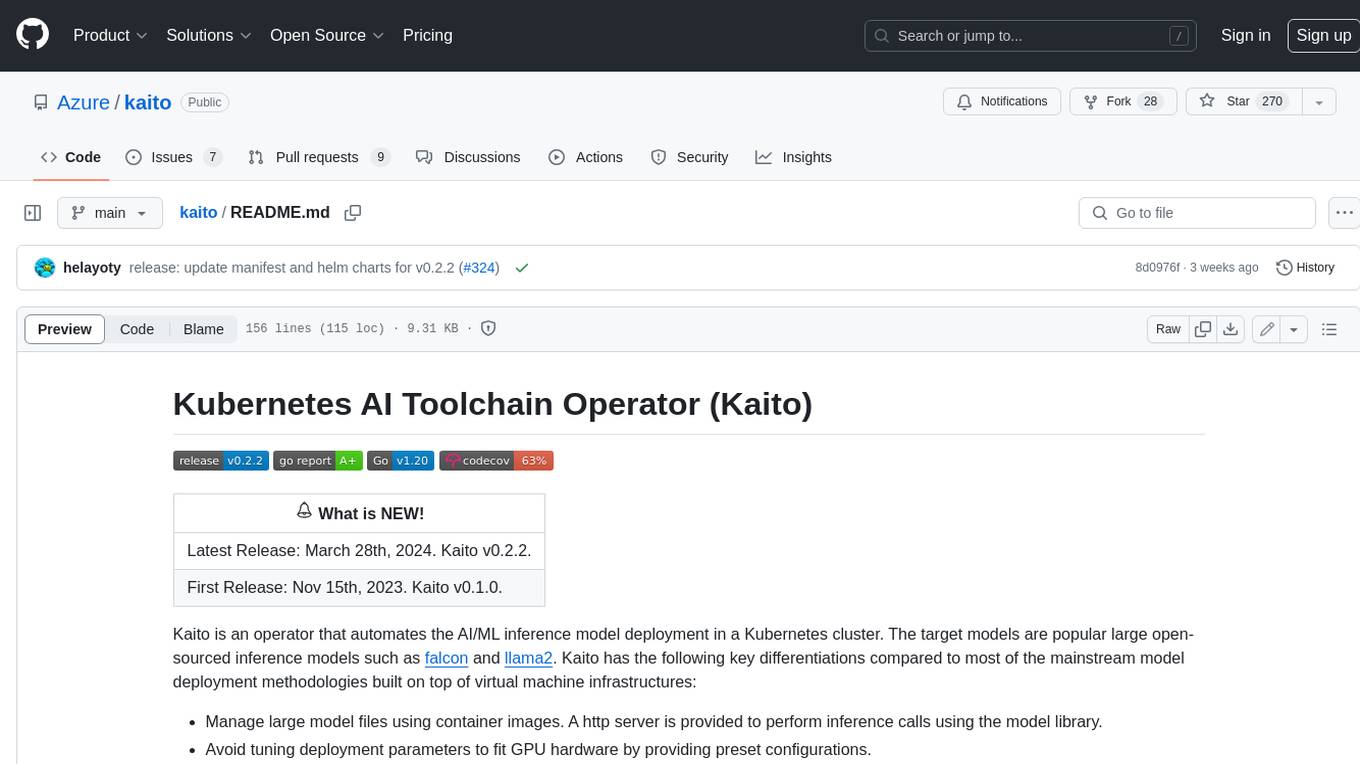
kaito
Kaito is an operator that automates the AI/ML inference model deployment in a Kubernetes cluster. It manages large model files using container images, avoids tuning deployment parameters to fit GPU hardware by providing preset configurations, auto-provisions GPU nodes based on model requirements, and hosts large model images in the public Microsoft Container Registry (MCR) if the license allows. Using Kaito, the workflow of onboarding large AI inference models in Kubernetes is largely simplified.
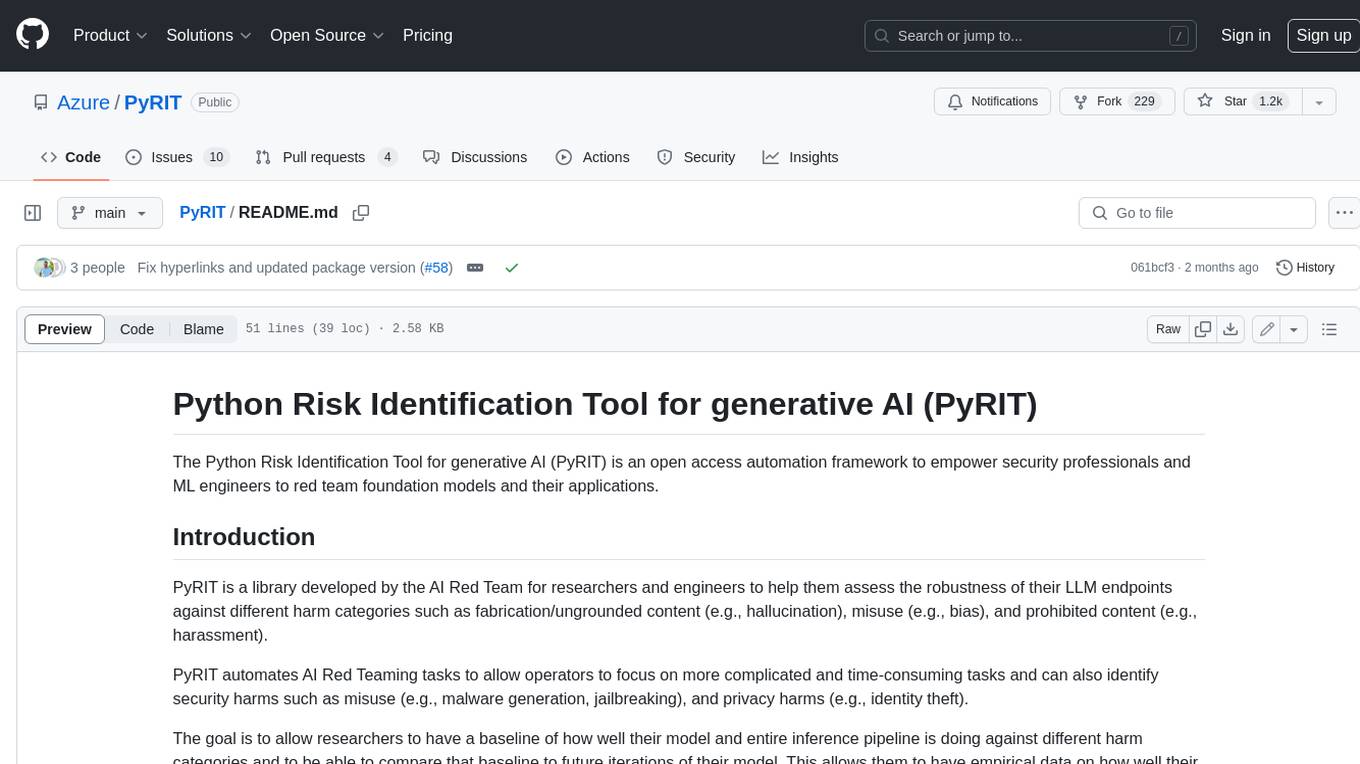
PyRIT
PyRIT is an open access automation framework designed to empower security professionals and ML engineers to red team foundation models and their applications. It automates AI Red Teaming tasks to allow operators to focus on more complicated and time-consuming tasks and can also identify security harms such as misuse (e.g., malware generation, jailbreaking), and privacy harms (e.g., identity theft). The goal is to allow researchers to have a baseline of how well their model and entire inference pipeline is doing against different harm categories and to be able to compare that baseline to future iterations of their model. This allows them to have empirical data on how well their model is doing today, and detect any degradation of performance based on future improvements.
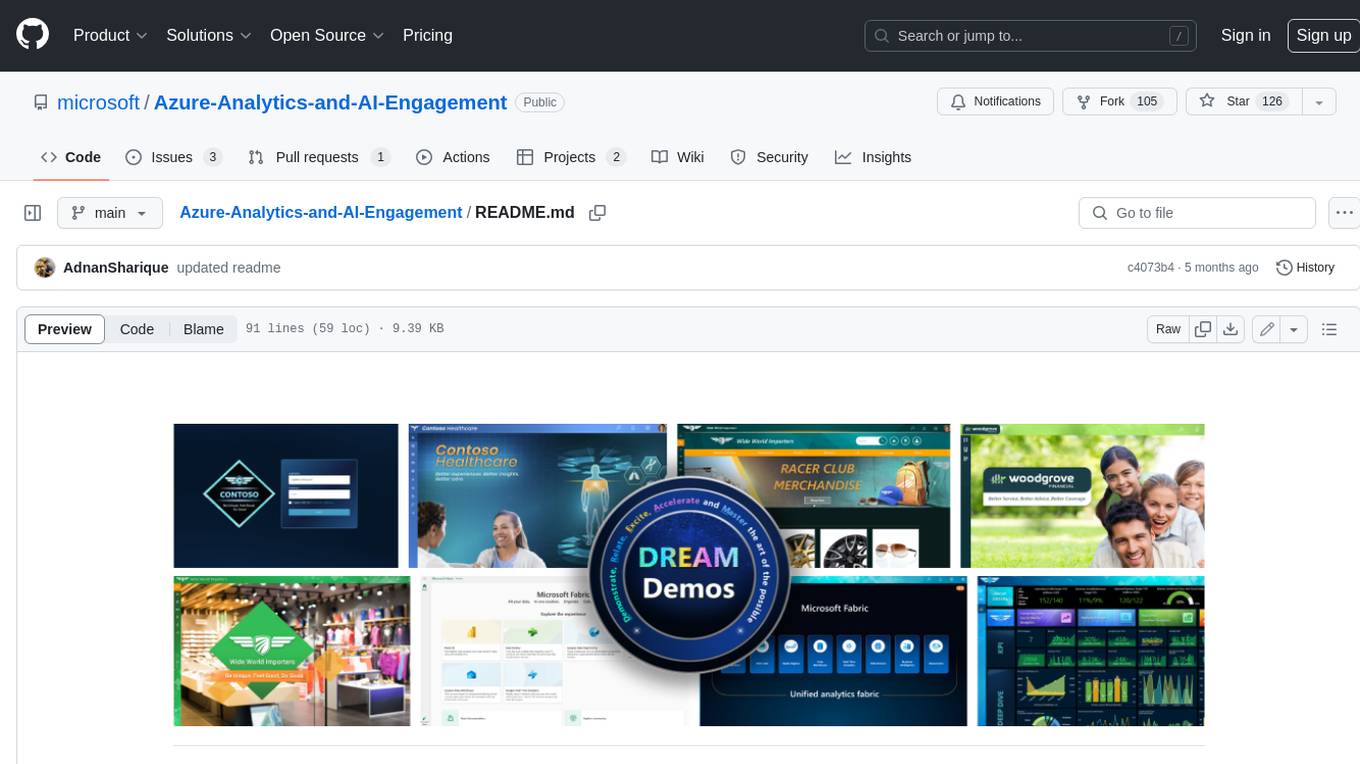
Azure-Analytics-and-AI-Engagement
The Azure-Analytics-and-AI-Engagement repository provides packaged Industry Scenario DREAM Demos with ARM templates (Containing a demo web application, Power BI reports, Synapse resources, AML Notebooks etc.) that can be deployed in a customer’s subscription using the CAPE tool within a matter of few hours. Partners can also deploy DREAM Demos in their own subscriptions using DPoC.