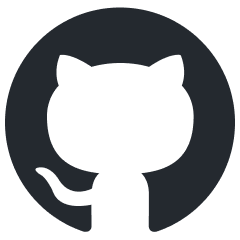
LLMSys-PaperList
Large Language Model (LLM) Systems Paper List
Stars: 869
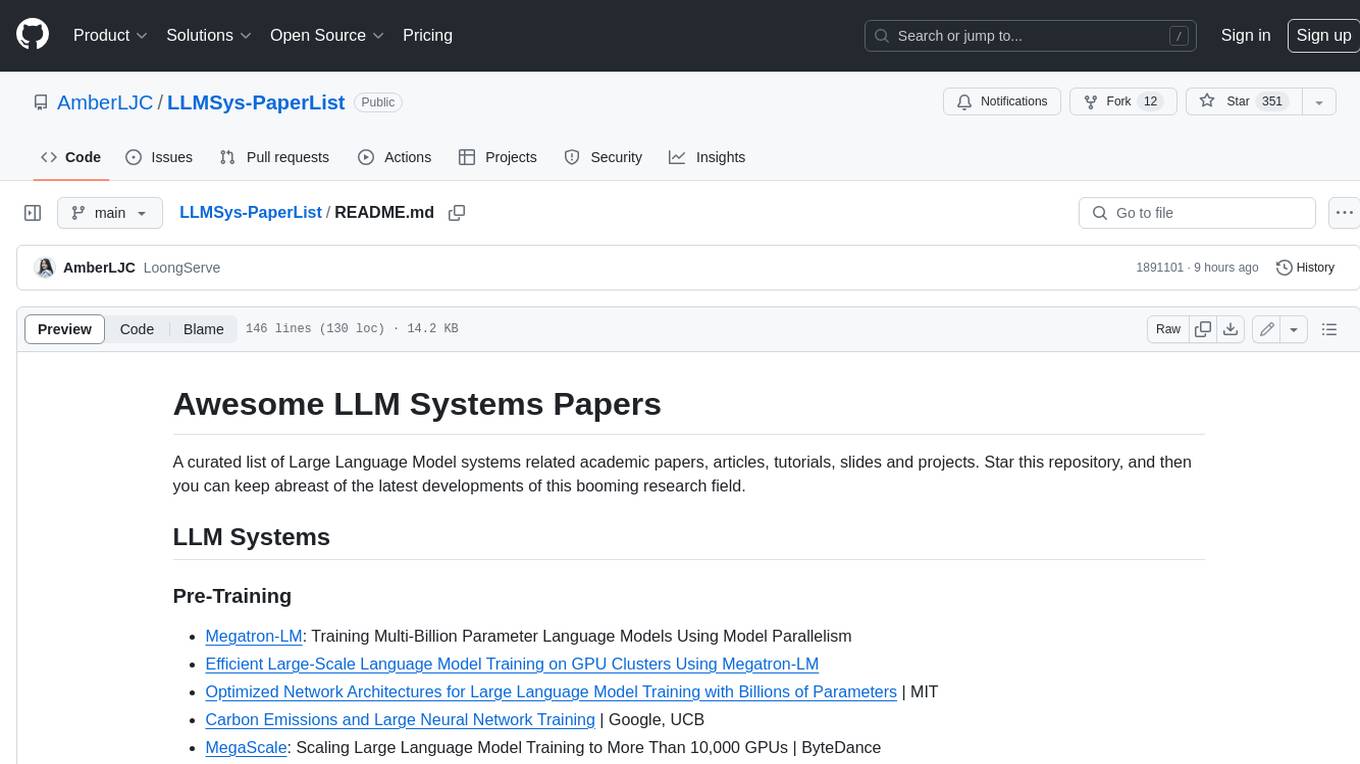
This repository provides a comprehensive list of academic papers, articles, tutorials, slides, and projects related to Large Language Model (LLM) systems. It covers various aspects of LLM research, including pre-training, serving, system efficiency optimization, multi-model systems, image generation systems, LLM applications in systems, ML systems, survey papers, LLM benchmarks and leaderboards, and other relevant resources. The repository is regularly updated to include the latest developments in this rapidly evolving field, making it a valuable resource for researchers, practitioners, and anyone interested in staying abreast of the advancements in LLM technology.
README:
A curated list of Large Language Model systems related academic papers, articles, tutorials, slides and projects. Star this repository, and then you can keep abreast of the latest developments of this booming research field.
- LLM Systems
- LLM for Systems
- ML Systems
- Survey Paper
- LLM Benchmark / Leaderboard / Traces
- LLM Frameworks
- Related ML Readings
- Megatron-LM: Training Multi-Billion Parameter Language Models Using Model Parallelism
- Efficient Large-Scale Language Model Training on GPU Clusters Using Megatron-LM
- Reducing Activation Recomputation in Large Transformer Models
- Optimized Network Architectures for Large Language Model Training with Billions of Parameters | MIT
- Carbon Emissions and Large Neural Network Training | Google, UCB
- Perseus: Removing Energy Bloat from Large Model Training | SOSP' 24
- MegaScale: Scaling Large Language Model Training to More Than 10,000 GPUs | ByteDance
- DISTMM: Accelerating distributed multimodal model training | NSDI' 24
- A Codesign of Scheduling and Parallelization for Large Model Training in Heterogeneous Clusters
- Pipeline Parallelism with Controllable Memory | Sea AI Lab
- Boosting Large-scale Parallel Training Efficiency with C4: A Communication-Driven Approach
- Scaling Beyond the GPU Memory Limit for Large Mixture-of-Experts Model Training | ICML' 24
- Alibaba HPN: A Data Center Network for Large Language ModelTraining
- FlashAttention-3: Fast and Accurate Attention with Asynchrony and Low-precision
- The Llama 3 Herd of Models (Section 3)
- FALCON: Pinpointing and Mitigating Stragglers for Large-Scale Hybrid-Parallel Training
- Enabling Parallelism Hot Switching for Efficient Training of Large Language Models | SOSP' 24
- Revisiting Reliability in Large-Scale Machine Learning Research Clusters
- ScheMoE: An Extensible Mixture-of-Experts Distributed Training System with Tasks Scheduling | EuroSys '24
- DynaPipe : Optimizing Multi-task Training through Dynamic Pipelines | EuroSys '24
- HAP: SPMD DNN Training on Heterogeneous GPU Clusters with Automated Program Synthesis | EuroSys'24
- Demystifying Workload Imbalances in Large Transformer Model Training over Variable-length Sequences | PKU
- Improving training time and GPU utilization in geo-distributed language model training
- DeepSeek-V3 Technical Report
- Comet: Fine-grained Computation-communication Overlapping for Mixture-of-Experts | ByteDance
- ByteScale : Efficient Scaling of LLM Training with a 2048K Context Length on More Than 12,000 GPUs | ByteDance
- Megalodon: Efficient LLM Pretraining and Inference with Unlimited Context Length
- SPPO:Efficient Long-sequence LLM Training via Adaptive Sequence Pipeline Parallel Offloading
- ByteScale: Efficient Scaling of LLM Training with a 2048K Context Length on More Than 12,000 GPUs | Bytedance
- TileLink: Generating Efficient Compute-Communication Overlapping Kernels using Tile-Centric Primitives | MLSys' 25
- Every FLOP Counts: Scaling a 300B Mixture-of-Experts LING LLM without Premium GPUs| Ant Group
- RLHFuse: Efficient RLHF Training for Large Language Models with Inter- and Intra-Stage Fusion | NSDI'25
- HybridFlow: A Flexible and Efficient RLHF Framework
- Oobleck: Resilient Distributed Training of Large Models Using Pipeline Templates | SOSP' 23
- Malleus: Straggler-Resilient Hybrid Parallel Training of Large-scale Models via Malleable Data and Model Parallelization
- Fire-Flyer AI-HPC: A Cost-Effective Software-Hardware Co-Design for Deep Learning | DeepSeek SC' 24
- Lazarus: Resilient and Elastic Training of Mixture-of-Experts Models with Adaptive Expert Placement
- GEMINI: Fast Failure Recovery in Distributed Training with In-Memory Checkpoints
- ByteCheckpoint: A Unified Checkpointing System for LLM Development
- ReCycle: Resilient Training of Large DNNs using Pipeline Adaptation | SOSP' 24
- Minder: Faulty Machine Detection for Large-scale Distributed Model Training | THU
- The Streaming Batch Model for Efficient and Fault-Tolerant Heterogeneous Execution
- TrainMover: Efficient ML Training Live Migration with No Memory Overhead | Alibaba
- Characterizing GPU Resilience and Impact on AI/HPC Systems | UIUC
- Orca: A Distributed Serving System for Transformer-Based Generative Models | OSDI'22
- Response Length Perception and Sequence Scheduling: An LLM-Empowered LLM Inference Pipeline | NUS
- Efficiently Scaling Transformer Inference | MLSys' 23
- Flover: A Temporal Fusion Framework for Efficient Autoregressive Model Parallel Inference
- FlashAttention: Fast and Memory-Efficient Exact Attention with IO-Awareness
- DeepSpeed Inference : Enabling Efficient Inference of Transformer Models at Unprecedented Scale.
- TurboTransformers: An Efficient GPU Serving System For Transformer Models
- FlexGen: High-throughput Generative Inference of Large Language Models with a Single GPU | ICML' 23
- MPCFormer : fast, performant, and private transformer inference with MPC | ICLR'23
- POLCA: Power Oversubscription in LLM Cloud Providers | Microsoft
- SARATHI: Efficient LLM Inference by Piggybacking Decodes with Chunked Prefills | Microsoft
- AttMemo: Accelerating Self-Attention with Memoization on Big Memory Systems
- vLLM: Easy, Fast, and Cheap LLM Serving with PagedAttention | SOSP' 23
- Tabi: An Efficient Multi-Level Inference System for Large Language Models | EuroSys' 23
- Flash-LLM: Enabling Cost-Effective and Highly-Efficient Large Generative Model Inference with Unstructured Sparsity | VLDB' 24
- AutoGen: Enabling Next-Gen LLM Applications via Multi-Agent Conversation | Microsoft
- FlashDecoding++: Faster Large Language Model Inference on GPUs | Tsinghua
- DeepSpeed-MII: Model Implementations for Inference (MII) | Microsoft
- Punica: Multi-Tenant LoRA Serving | MLSys' 24
- S-LoRA: Serving Thousands of Concurrent LoRA Adapters | MLSys' 24
- SpotServe: Serving Generative Large Language Models on Preemptible Instances | CMU
- SuperServe: Fine-Grained Inference Serving for Unpredictable Workloads
- Fairness in Serving Large Language Models | OSDI' 24
- Infinite-LLM: Efficient LLM Service for Long Context with DistAttention and Distributed KVCache
- CaraServe: CPU-Assisted and Rank-Aware LoRA Serving for Generative LLM Inference
- DistServe: Disaggregating Prefill and Decoding for Goodput-optimized Large Language Model Serving| OSDI' 24
- Inference without Interference: Disaggregate LLM Inference for Mixed Downstream Workloads
- APIServe: Efficient API Support for Large-Language Model Inferencing
- FlexLLM: A System for Co-Serving Large Language Model Inference and Parameter-Efficient Finetuning
- DéjàVu: KV-cache Streaming for Fast, Fault-tolerant Generative LLM Serving
- Optimizing LLM Queries in Relational Workloads | UCB
- AttentionStore: Cost-effective Attention Reuse across Multi-turn Conversations in Large Language Model Serving | NUS
- MuxServe: Flexible Multiplexing for Efficient Multiple LLM Serving
- LoongServe: Efficiently Serving Long-context Large Language Models with Elastic Sequence Parallelism | SOSP' 24
- RAGCache: Efficient Knowledge Caching for Retrieval-Augmented Generation | PKU
- Andes: Defining and Enhancing Quality-of-Experience in LLM-Based Text Streaming Services | Umich
- BlockLLM: Multi-tenant Finer-grained Serving for Large Language Models
- vAttention: Dynamic Memory Management for Serving LLMs without PagedAttention
- Helix: Distributed Serving of Large Language Models via Max-Flow on Heterogeneous GPUs | CMU
- Eloquent: A More Robust Transmission Scheme for LLM Token Streaming | NAIC' 24
- Optimizing Speculative Decoding for Serving Large Language Models Using Goodput | UCB
- Enabling Elastic Model Serving with MultiWorld | Cisco Research
- Prepacking: A Simple Method for Fast Prefilling and Increased Throughput in Large Language Models
- NanoFlow: Towards Optimal Large Language Model Serving Throughput
- Responsive ML inference in multi-tenanted environments using AQUA
- One Queue Is All You Need: Resolving Head-of-Line Blocking in Large Language Model Serving
- MemServe: Context Caching for Disaggregated LLM Serving with Elastic Memory Pool
- dLoRA: Dynamically Orchestrating Requests and Adapters for LoRA LLM Serving | OSDI' 24
- Llumnix: Dynamic Scheduling for Large Language Model Serving | OSDI' 24
- Taming Throughput-Latency Tradeoff in LLM Inference with Sarathi-Serve | OSDI' 24
- InfiniGen: Efficient Generative Inference of Large Language Models with Dynamic KV Cache Management
- ServerlessLLM: Low-Latency Serverless Inference for Large Language Models | OSDI' 24
- Preble: Efficient Distributed Prompt Scheduling for LLM Serving
- Mnemosyne: Parallelization Strategies for Efficiently Serving Multi-Million Context Length LLM Inference Requests Without Approximations
- ConServe: Harvesting GPUs for Low-Latency and High-Throughput Large Language Model Serving
- BlockLLM: Multi-tenant Finer-grained Serving for Large Language Models
- Context Parallelism for Scalable Million-Token Inference
- xDiT: an Inference Engine for Diffusion Transformers (DiTs) with Massive Parallelism
- Pie: Pooling CPU Memory for LLM Inference
- NEO: Saving GPU Memory Crisis with CPU Offloading for Online LLM Inference
- FastSwitch: Optimizing Context Switching Efficiency in Fairness-aware Large Language Model Serving
- Flash Communication: Reducing Tensor Parallelization Bottleneck for Fast Large Language Model Inference
- FlashInfer: Efficient and Customizable Attention Engine for LLM Inference Serving
- Fast Inference for Augmented Large Language Models
- A System for Microserving of LLMs | CMU
- iServe : An Intent-based Serving System for LLMs| UT Austin
- Locality-aware Fair Scheduling in LLM Serving | UCB
- Towards Efficient Large Multimodal Model Serving | MSFT
- DeltaZip: Efficient Serving of Multiple Full-Model-Tuned LLMs
- PIM Is All You Need: A CXL-Enabled GPU-Free System for Large Language Model Inference | ASPLOS' 25
- λScale: Enabling Fast Scaling for Serverless Large Language Model Inference
- AIBrix: Towards Scalable and Cost-Effective LLM Inference Infrastructure | vLLM
- Serving Models, Fast and Slow:Optimizing Heterogeneous LLM Inferencing Workloads at Scale
- Make LLM Inference Affordable to Everyone: Augmenting GPU Memory with NDP-DIMM
- Jenga: Effective Memory Management for Serving LLM with Heterogeneity
- ALTO: An Efficient Network Orchestrator for Compound AI Systems | Stanford & UCB
- Parrot: Efficient Serving of LLM-based Applications with Semantic Variable | OSDI' 24
- Efficiently Serving LLM Reasoning Programs with Certaindex | UCSD
- Autellix: An Efficient Serving Engine for LLM Agents as General Programs | UCB
- LLM in a flash: Efficient Large Language Model Inference with Limited Memory | Apple
- STI: Turbocharge NLP Inference at the Edge via Elastic Pipelining | ASPLOS 23
- PowerInfer: Fast Large Language Model Serving with a Consumer-grade GPU | SOSP' 24
- MoE-Lightning: High-Throughput MoE Inference on Memory-constrained GPUs
- InfiniteHiP: Extending Language Model Context Up to 3 Million Tokens on a Single GPU
- Fast Distributed Inference Serving for Large Language Models | PKU
- FrugalGPT: How to Use Large Language Models While Reducing Cost and Improving Performance | Stanford
- H2O: Heavy-Hitter Oracle for Efficient Generative Inference of Large Language Models | ICML ES-FoMo Workshop 2023
- Inference with Reference: Lossless Acceleration of Large Language Models
- SkipDecode: Autoregressive Skip Decoding with Batching and Caching for Efficient LLM Inferencex
- Scissorhands: Exploiting the Persistence of Importance Hypothesis for LLM KV Cache Compression at Test Time
- Knowledge-preserving Pruning for Pre-trained Language Models without Retraining | SNU
- Accelerating LLM Inference with Staged Speculative Decoding | ICML' 23
- SpecInfer: Accelerating Generative LLM Serving with Speculative Inference and Token Tree Verification | CMU
- Deja Vu: Contextual Sparsity for Efficient LLMs at Inference Time | ICML' 23
- S3: Increasing GPU Utilization during Generative Inference for Higher Throughput | Havard
- LLMCad: Fast and Scalable On-device Large Language Model Inference
- Skeleton-of-Thought: Large Language Models Can Do Parallel Decoding | THU
- LoRAShear: Efficient Large Language Model Structured Pruning and Knowledge Recovery | Microsoft
- Ring Attention with Blockwise Transformers for Near-Infinite Context | UCB
- Learned Best-Effort LLM Serving | UCB
- Star Attention : Efficient LLM Inference over Long Sequences| NVIDIA
- Ymir: A Scheduler for Foundation Model Fine-tuning Workloads in Datacenters | ICS' 24
- MOSEL: Inference Serving Using Dynamic Modality Selection
- DISTMM: Accelerating distributed multimodal model training | NSDI' 24
- Approximate Caching for Efficiently Serving Diffusion Models | Adobe Research
- Generative AI Beyond LLMs: System Implications of Multi-Modal Generation | Meta
- Characterizing and Efficiently Accelerating Multimodal Generation Model Inference | Meta
- DistriFusion: Distributed Parallel Inference for High-Resolution Diffusion Models | MIT
- Optimus: Accelerating Large-Scale Multi-Modal LLM Training by Bubble Exploitation
- Addressing Model and Data Heterogeneity in Multimodal Large Language Model Training | PKU
- LongVILA: Scaling Long-Context Visual Language Models for Long Videos | NVIDIA
- FlexCache: Flexible Approximate Cache System for Video Diffusion | University of Waterloo
- Large Language Models for Compiler Optimization
- The Hitchhiker's Guide to Program Analysis: A Journey with Large Language Models
- LLM-Assisted Code Cleaning For Training Accurate Code Generators | UCB
- Efficient Multi-Task Large Model Training via Data Heterogeneity-aware Model Management
- If At First You Don’t Succeed, Try, Try, Again...? | SOSP' 24
- Aceso: Efficient Parallel DNN Training through Iterative Bottleneck Alleviation | EuroSys '24
- GMorph: Accelerating Multi-DNN Inference via Model Fusion | EuroSys '24
- Automatic Root Cause Analysis via Large Language Models for Cloud Incidents | EuroSys '24
- Efficient Large Language Models: A Survey
- Challenges and Applications of Large Language Models
- Beyond Efficiency: A Systematic Survey of Resource-Efficient Large Language Models
- Towards Efficient Generative Large Language Model Serving: A Survey from Algorithms to Systems
- LLM Energy Leaderboard | Umich
- LLM-Perf Leaderboard | HuggingFace
- Aviary Explorer | Anyscale
- Open LLM Leaderboard | HuggingFace
- HELM | Stanford
- LMSYS | UCB
- Towards Efficient and Reliable LLM Serving: A Real-World Workload Study
- DeepSpeed: a deep learning optimization library that makes distributed training and inference easy, efficient, and effective | Microsoft
- TensorRT-LLM | Nvidia
- Accelerate | Hugging Face
- Ray-LLM | Ray
- LLaVA
- Megatron | Nvidia
- NeMo | Nvidia
- torchtitan | PyTorch
- vLLM | UCB
- SGLang | UCB
- TGI | Hugging Face
- OpenRLHF
- veRL | ByteDance
- veScale | ByteDance
- KV Transformers
- DeepSeek Open Infra
- Dynamo | NVIDA
- Cornstarch: Build, Train, Run Your Own Multimodal Model | UMich
- Large Transformer Model Inference Optimization
- Transformer Inference Arithmetic
- The Transformer Family Version 2.0
- Full Stack Optimization of Transformer Inference: a Survey | UCB
- Systems for Machine Learning | (Stanford)[https://cs229s.stanford.edu/fall2023/]
- Systems for Generative AI | (Umich)[https://github.com/mosharaf/eecs598/tree/w24-genai]
- Systems for AI - LLMs | (GT)[https://cs8803-sp24.anand-iyer.com/]
- A curated list of Large Language Model
- AI systems paper list
- A baseline repository of Auto-Parallelism in Training Neural Networks
- Numbers every LLM Developer should know
- 100,000 H100 Clusters: Power, Network Topology, Ethernet vs InfiniBand, Reliability, Failures, Checkpointing
- OpenAI Keynote on Building Scalable AI Infrastructure
For Tasks:
Click tags to check more tools for each tasksFor Jobs:
Alternative AI tools for LLMSys-PaperList
Similar Open Source Tools
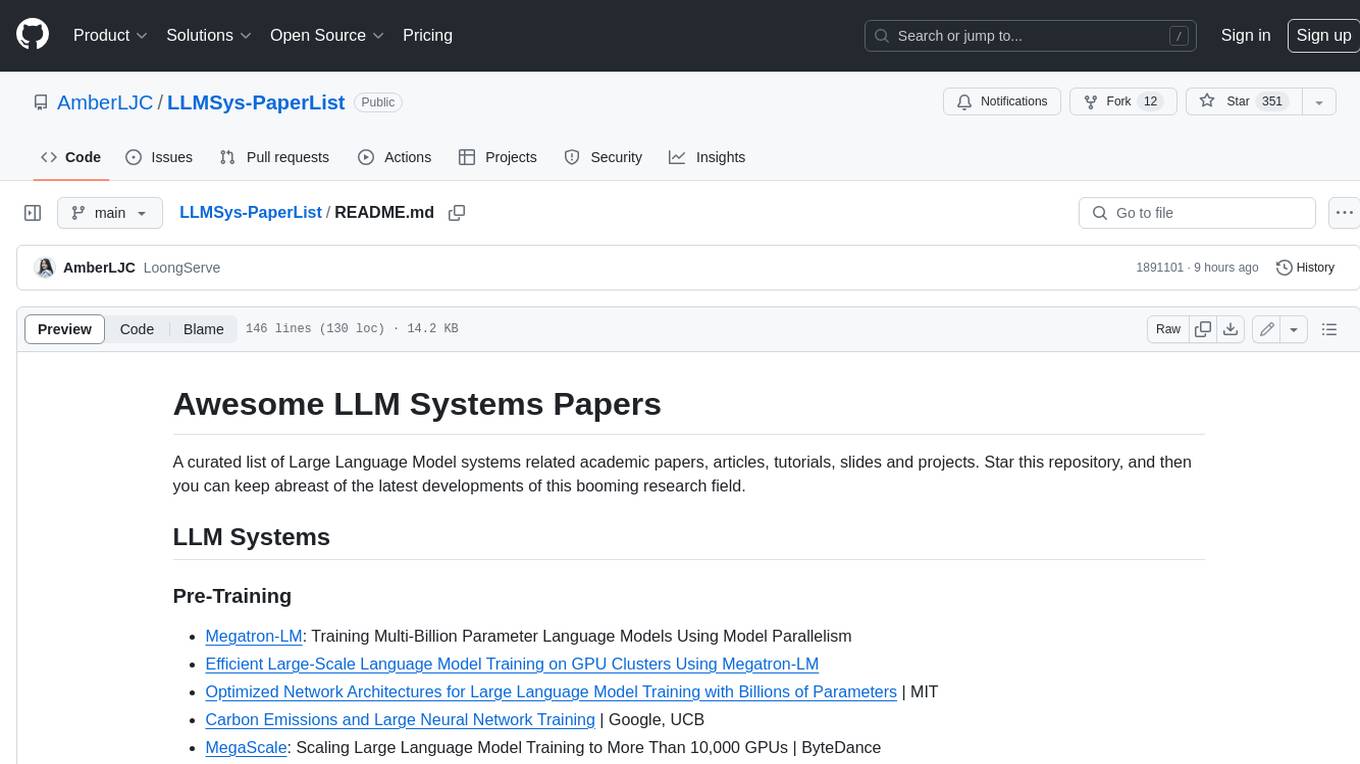
LLMSys-PaperList
This repository provides a comprehensive list of academic papers, articles, tutorials, slides, and projects related to Large Language Model (LLM) systems. It covers various aspects of LLM research, including pre-training, serving, system efficiency optimization, multi-model systems, image generation systems, LLM applications in systems, ML systems, survey papers, LLM benchmarks and leaderboards, and other relevant resources. The repository is regularly updated to include the latest developments in this rapidly evolving field, making it a valuable resource for researchers, practitioners, and anyone interested in staying abreast of the advancements in LLM technology.
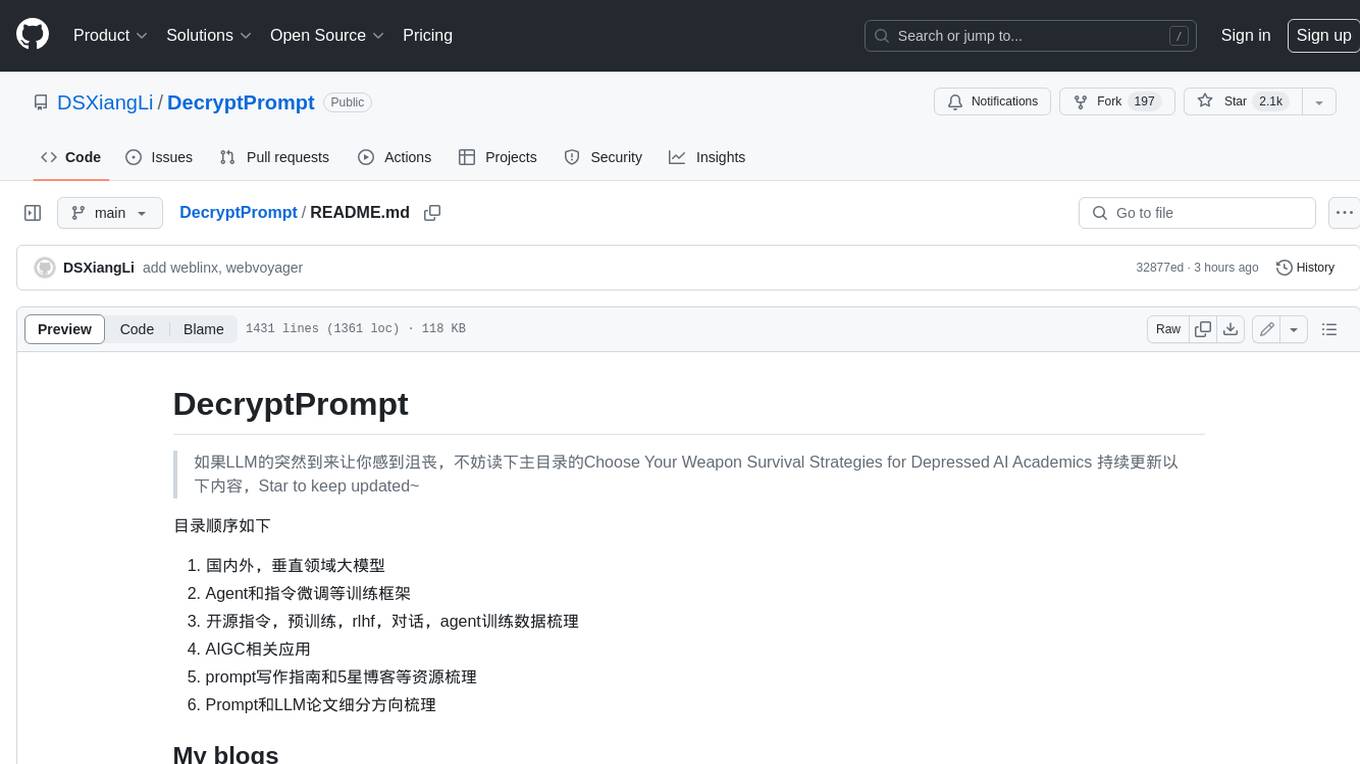
DecryptPrompt
This repository does not provide a tool, but rather a collection of resources and strategies for academics in the field of artificial intelligence who are feeling depressed or overwhelmed by the rapid advancements in the field. The resources include articles, blog posts, and other materials that offer advice on how to cope with the challenges of working in a fast-paced and competitive environment.
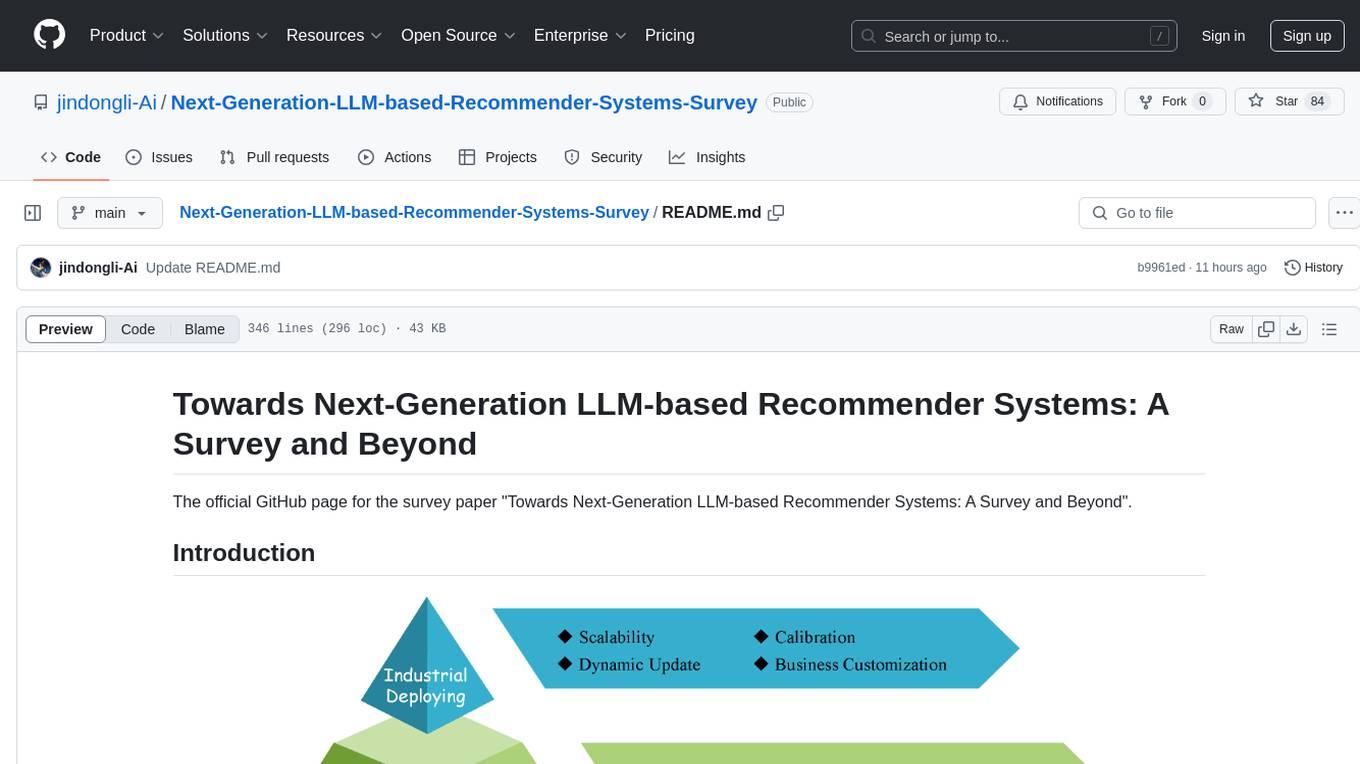
Next-Generation-LLM-based-Recommender-Systems-Survey
The Next-Generation LLM-based Recommender Systems Survey is a comprehensive overview of the latest advancements in recommender systems leveraging Large Language Models (LLMs). The survey covers various paradigms, approaches, and applications of LLMs in recommendation tasks, including generative and non-generative models, multimodal recommendations, personalized explanations, and industrial deployment. It discusses the comparison with existing surveys, different paradigms, and specific works in the field. The survey also addresses challenges and future directions in the domain of LLM-based recommender systems.
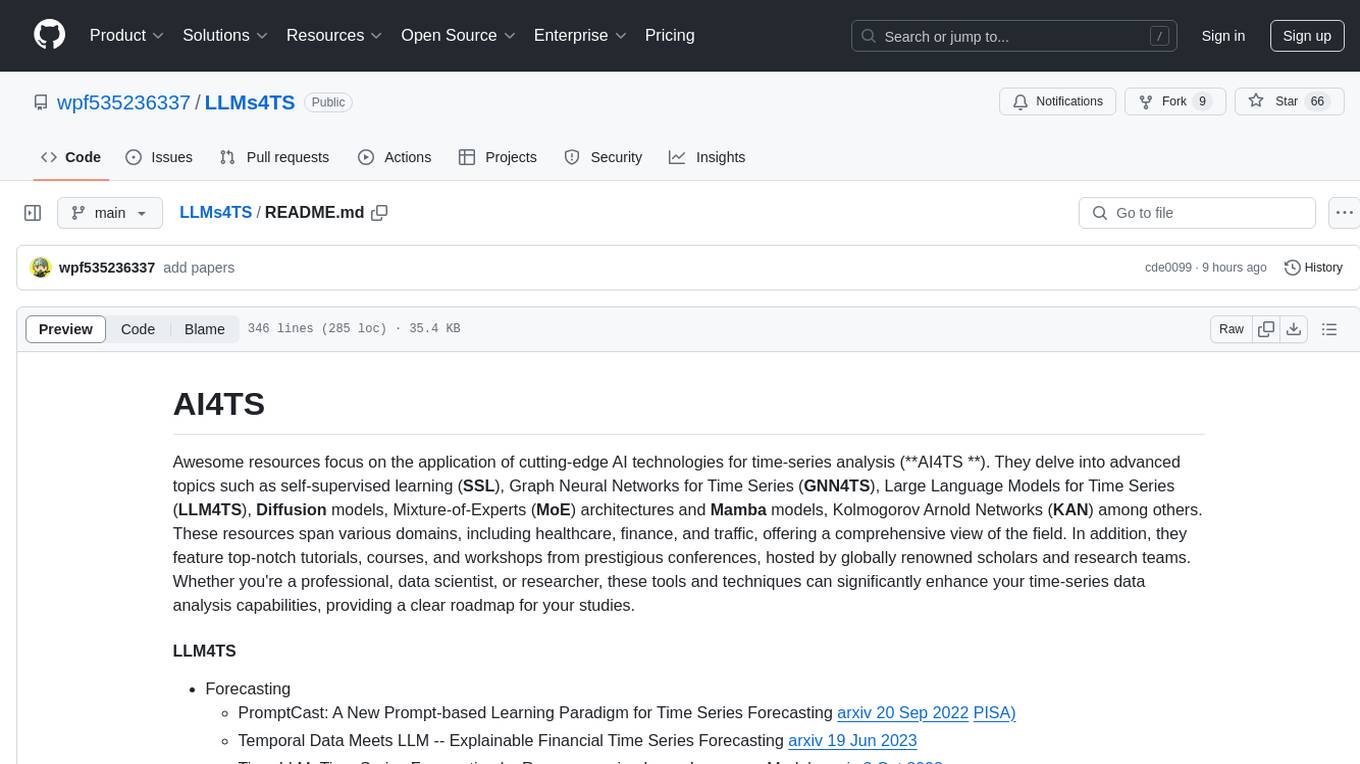
LLMs4TS
LLMs4TS is a repository focused on the application of cutting-edge AI technologies for time-series analysis. It covers advanced topics such as self-supervised learning, Graph Neural Networks for Time Series, Large Language Models for Time Series, Diffusion models, Mixture-of-Experts architectures, and Mamba models. The resources in this repository span various domains like healthcare, finance, and traffic, offering tutorials, courses, and workshops from prestigious conferences. Whether you're a professional, data scientist, or researcher, the tools and techniques in this repository can enhance your time-series data analysis capabilities.
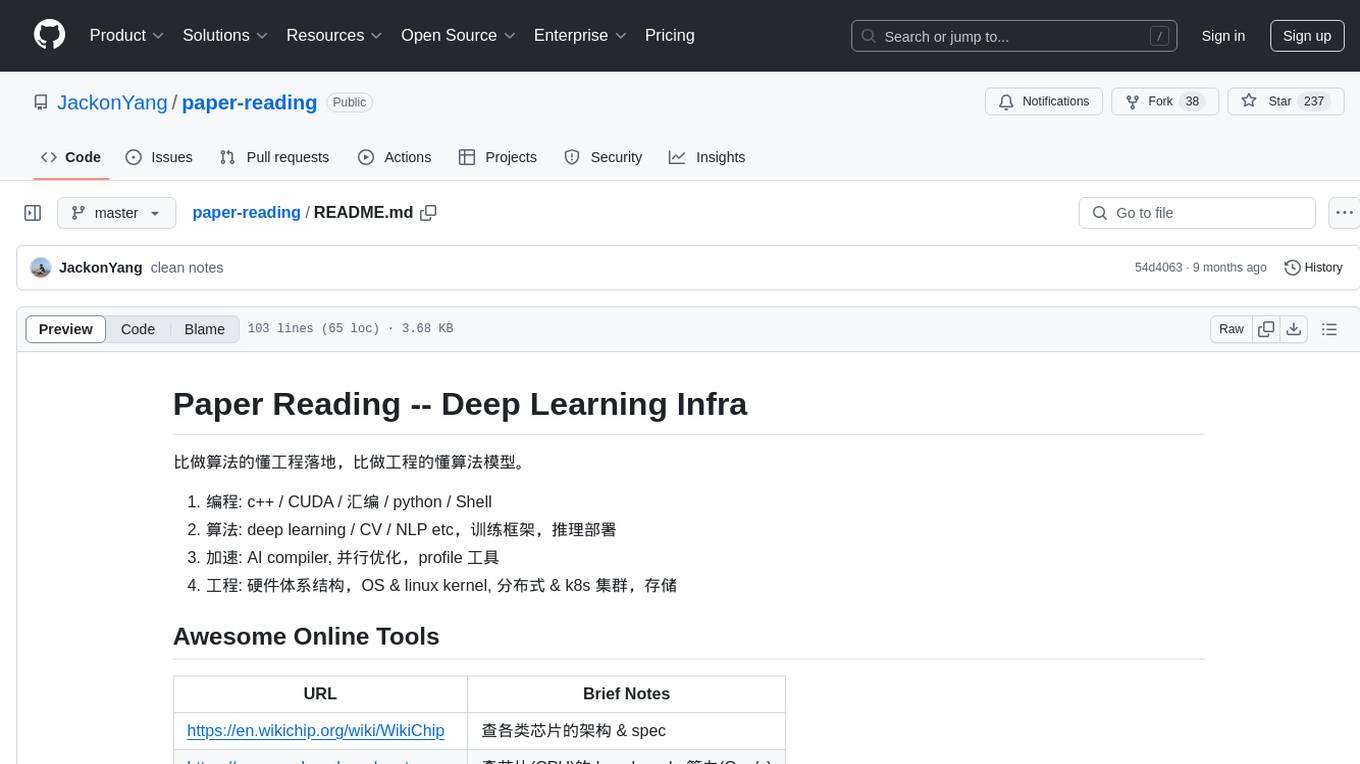
paper-reading
This repository is a collection of tools and resources for deep learning infrastructure, covering programming languages, algorithms, acceleration techniques, and engineering aspects. It provides information on various online tools for chip architecture, CPU and GPU benchmarks, and code analysis. Additionally, it includes content on AI compilers, deep learning models, high-performance computing, Docker and Kubernetes tutorials, Protobuf and gRPC guides, and programming languages such as C++, Python, and Shell. The repository aims to bridge the gap between algorithm understanding and engineering implementation in the fields of AI and deep learning.
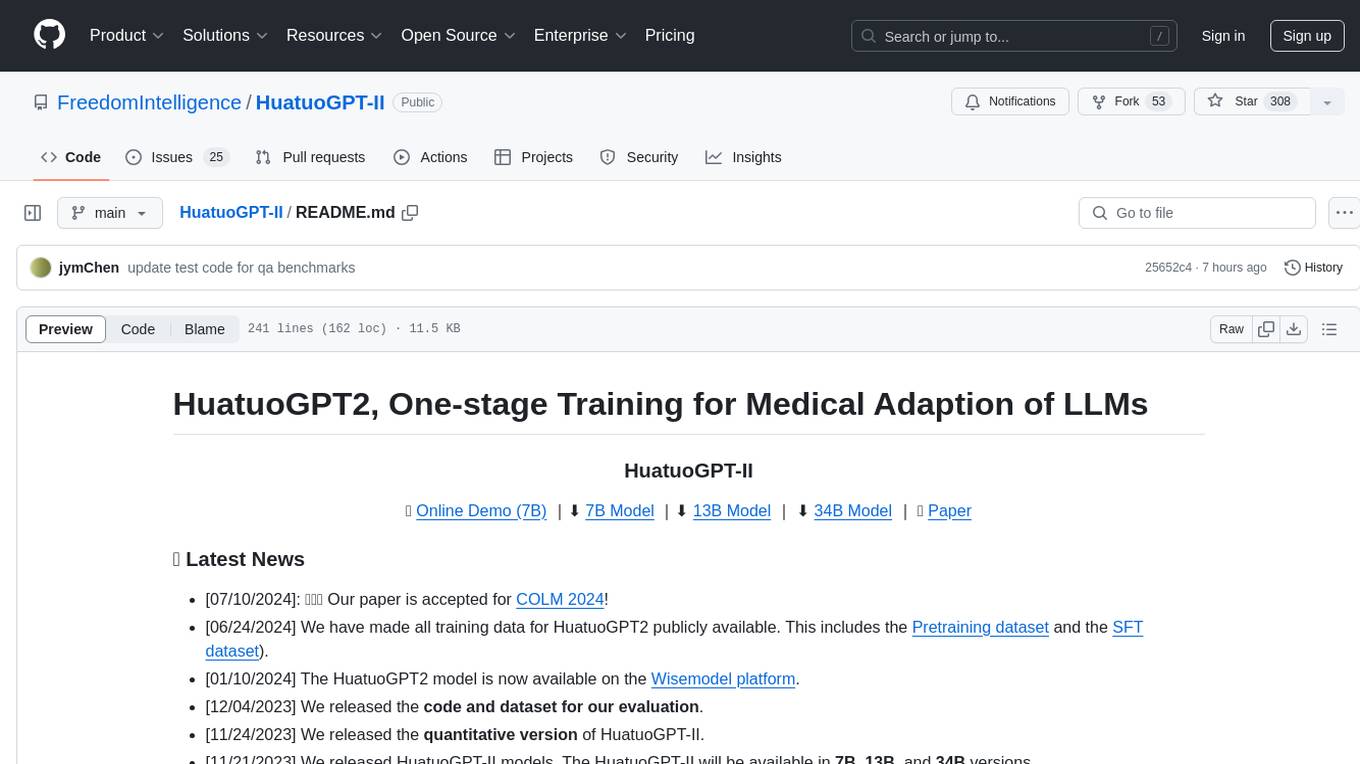
HuatuoGPT-II
HuatuoGPT2 is an innovative domain-adapted medical large language model that excels in medical knowledge and dialogue proficiency. It showcases state-of-the-art performance in various medical benchmarks, surpassing GPT-4 in expert evaluations and fresh medical licensing exams. The open-source release includes HuatuoGPT2 models in 7B, 13B, and 34B versions, training code for one-stage adaptation, partial pre-training and fine-tuning instructions, and evaluation methods for medical response capabilities and professional pharmacist exams. The tool aims to enhance LLM capabilities in the Chinese medical field through open-source principles.
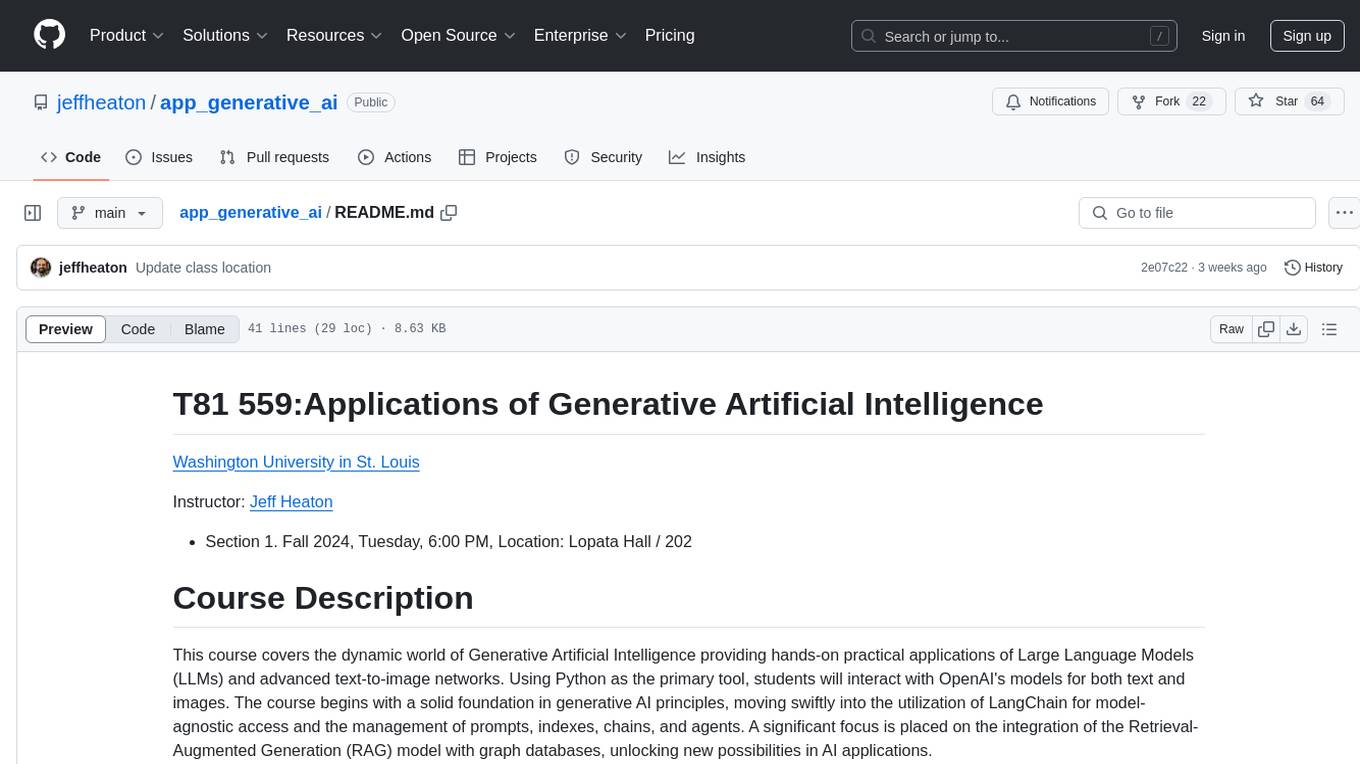
app_generative_ai
This repository contains course materials for T81 559: Applications of Generative Artificial Intelligence at Washington University in St. Louis. The course covers practical applications of Large Language Models (LLMs) and text-to-image networks using Python. Students learn about generative AI principles, LangChain, Retrieval-Augmented Generation (RAG) model, image generation techniques, fine-tuning neural networks, and prompt engineering. Ideal for students, researchers, and professionals in computer science, the course offers a transformative learning experience in the realm of Generative AI.
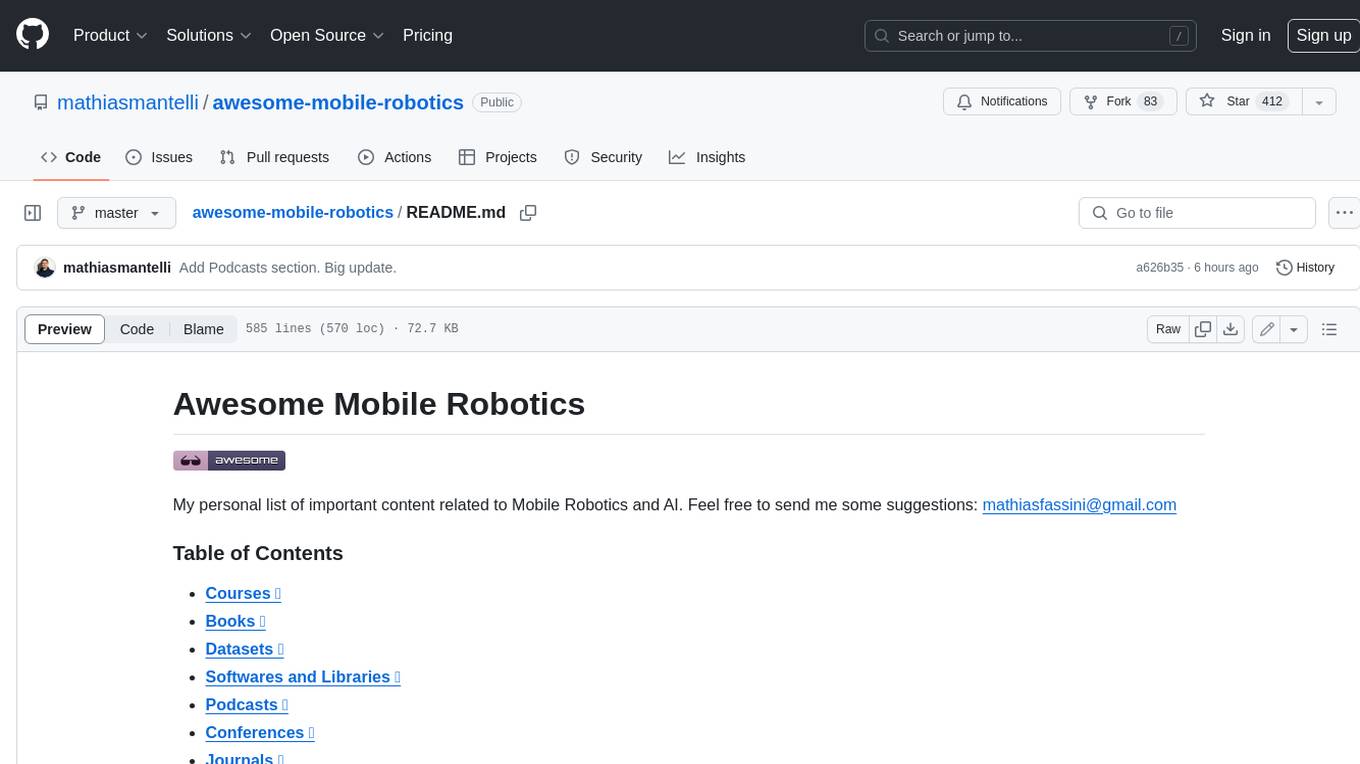
awesome-mobile-robotics
The 'awesome-mobile-robotics' repository is a curated list of important content related to Mobile Robotics and AI. It includes resources such as courses, books, datasets, software and libraries, podcasts, conferences, journals, companies and jobs, laboratories and research groups, and miscellaneous resources. The repository covers a wide range of topics in the field of Mobile Robotics and AI, providing valuable information for enthusiasts, researchers, and professionals in the domain.
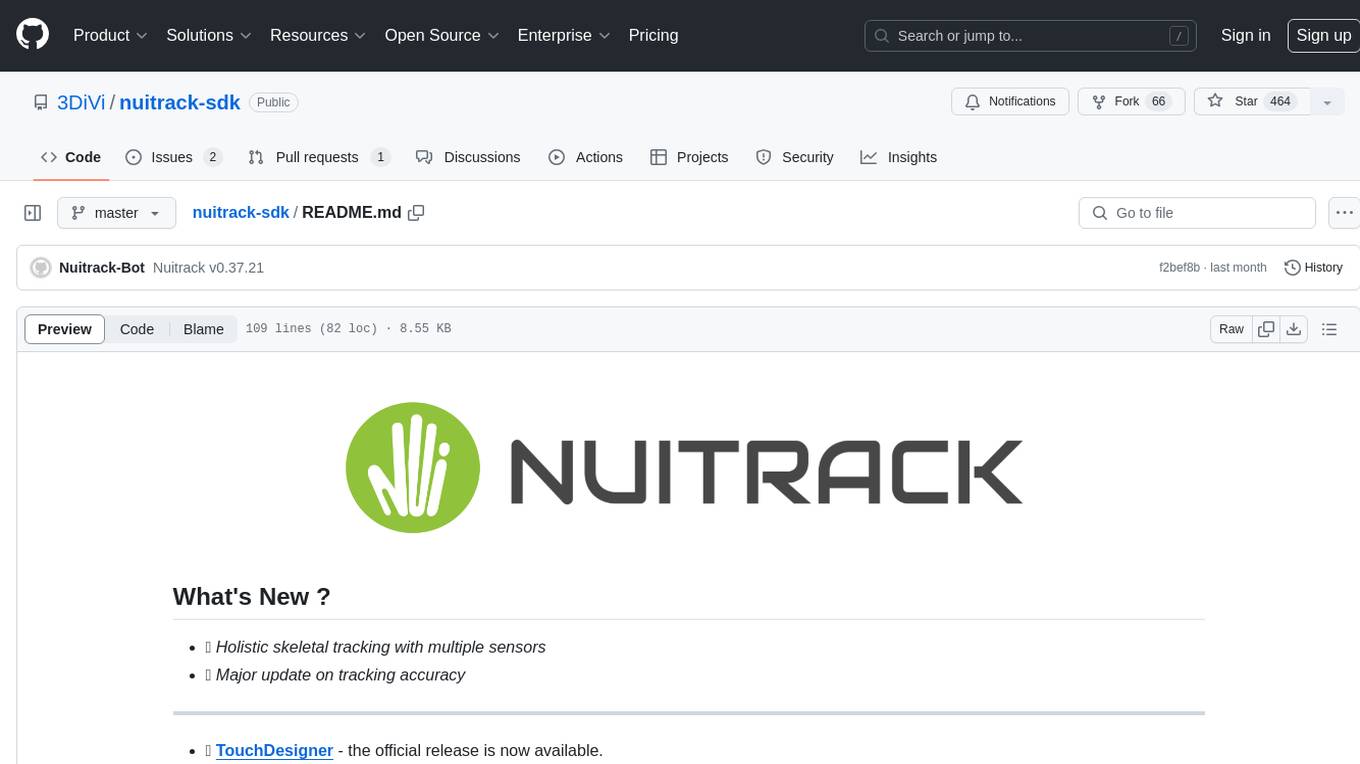
nuitrack-sdk
Nuitrack™ is an ultimate 3D body tracking solution developed by 3DiVi Inc. It enables body motion analytics applications for virtually any widespread depth sensors and hardware platforms, supporting a wide range of applications from real-time gesture recognition on embedded platforms to large-scale multisensor analytical systems. Nuitrack provides highly-sophisticated 3D skeletal tracking, basic facial analysis, hand tracking, and gesture recognition APIs for UI control. It offers two skeletal tracking engines: classical for embedded hardware and AI for complex poses, providing a human-centric spatial understanding tool for natural and intelligent user engagement.
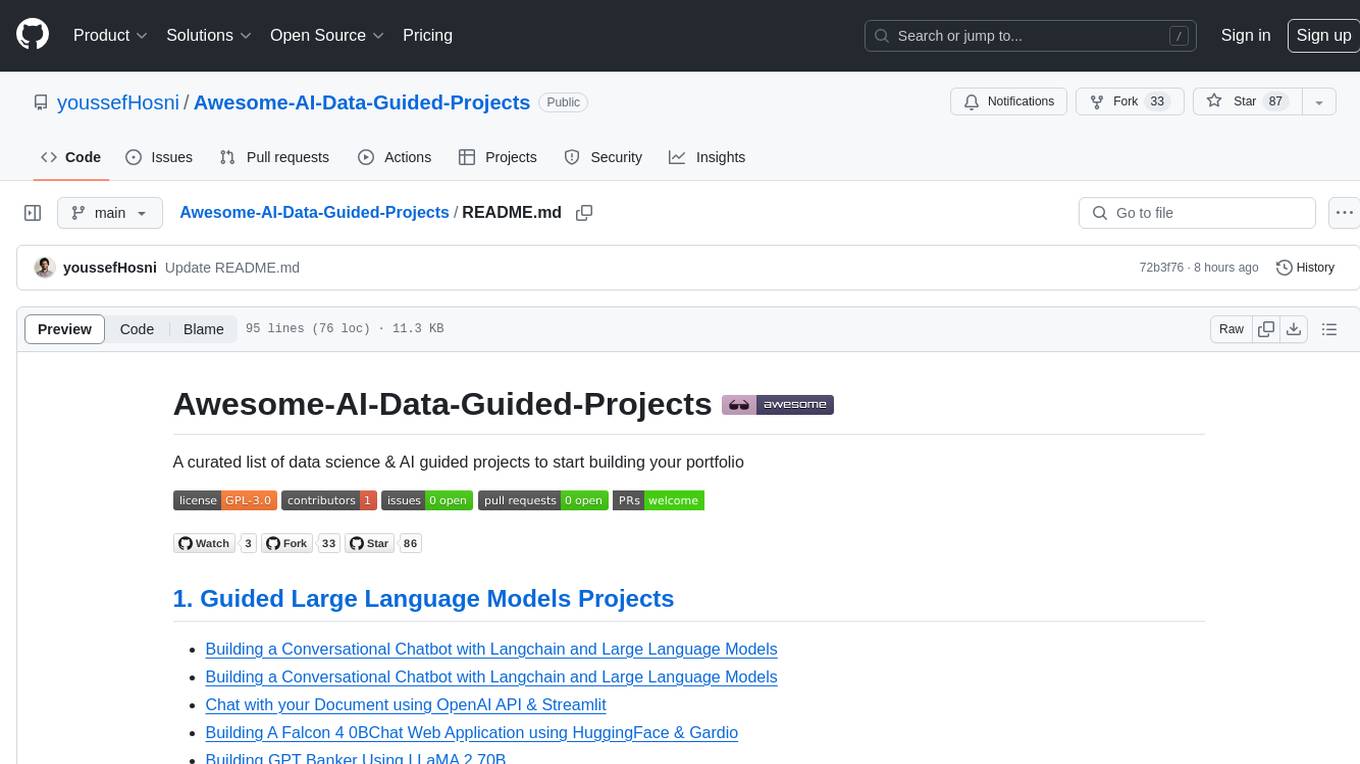
Awesome-AI-Data-Guided-Projects
A curated list of data science & AI guided projects to start building your portfolio. The repository contains guided projects covering various topics such as large language models, time series analysis, computer vision, natural language processing (NLP), and data science. Each project provides detailed instructions on how to implement specific tasks using different tools and technologies.
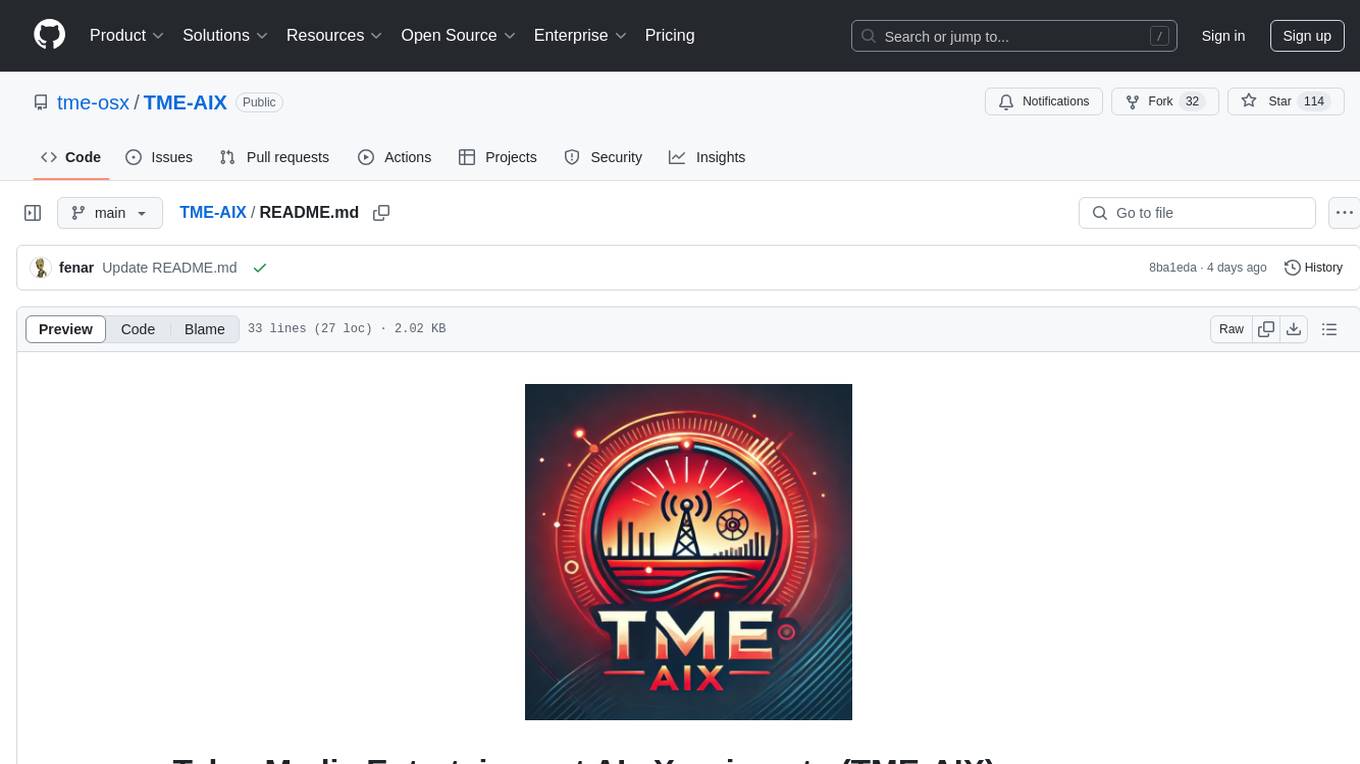
TME-AIX
The TME-AIX repository is a collaborative workspace dedicated to exploring Telco Media Entertainment use-cases using open source AI capabilities and datasets. It focuses on projects like Revenue Assurance, Service Assurance Predictions, 5G Network Fault Predictions, Sustainability, SecOps-AI, SmartGrid, IoT Security, Customer Relation Management, Anomaly Detection, Starlink Quality Predictions, and NoC AI Augmentation for OSS.
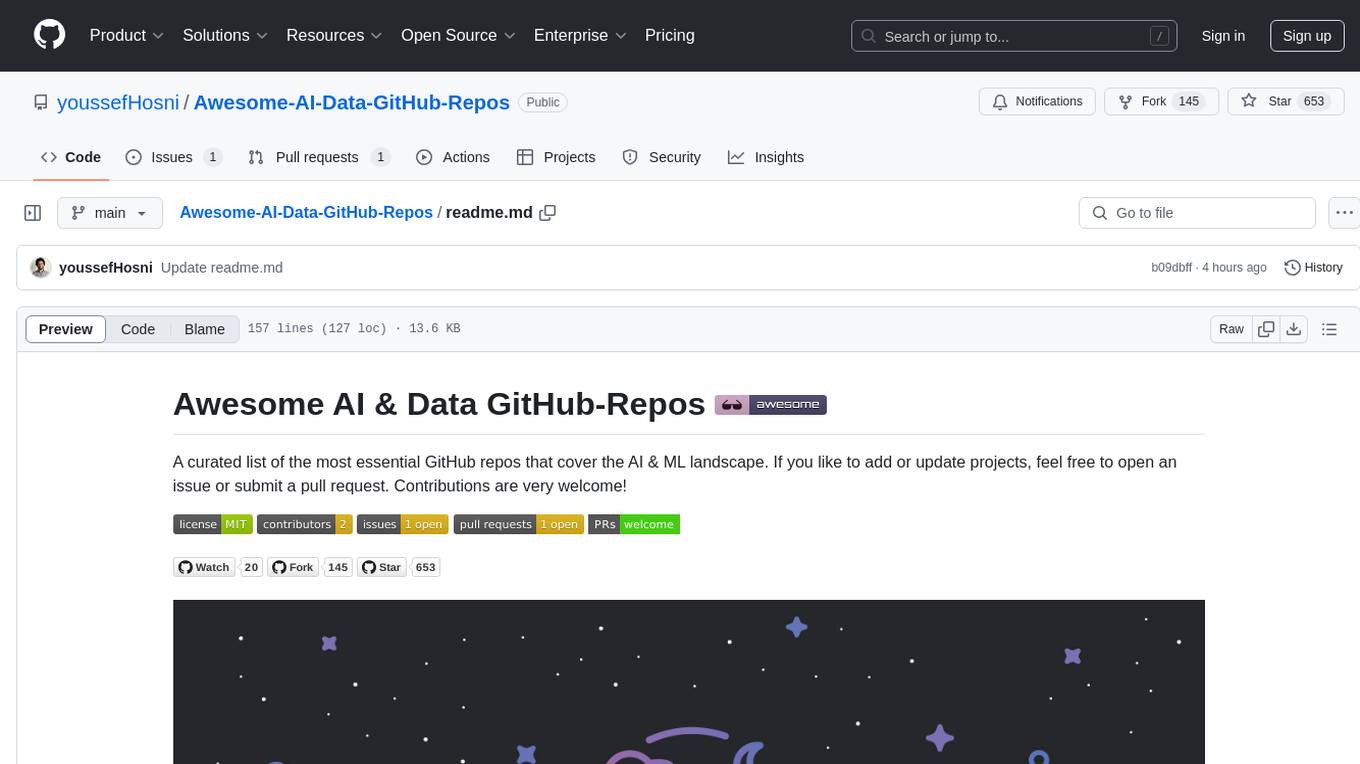
Awesome-AI-Data-GitHub-Repos
Awesome AI & Data GitHub-Repos is a curated list of essential GitHub repositories covering the AI & ML landscape. It includes resources for Natural Language Processing, Large Language Models, Computer Vision, Data Science, Machine Learning, MLOps, Data Engineering, SQL & Database, and Statistics. The repository aims to provide a comprehensive collection of projects and resources for individuals studying or working in the field of AI and data science.
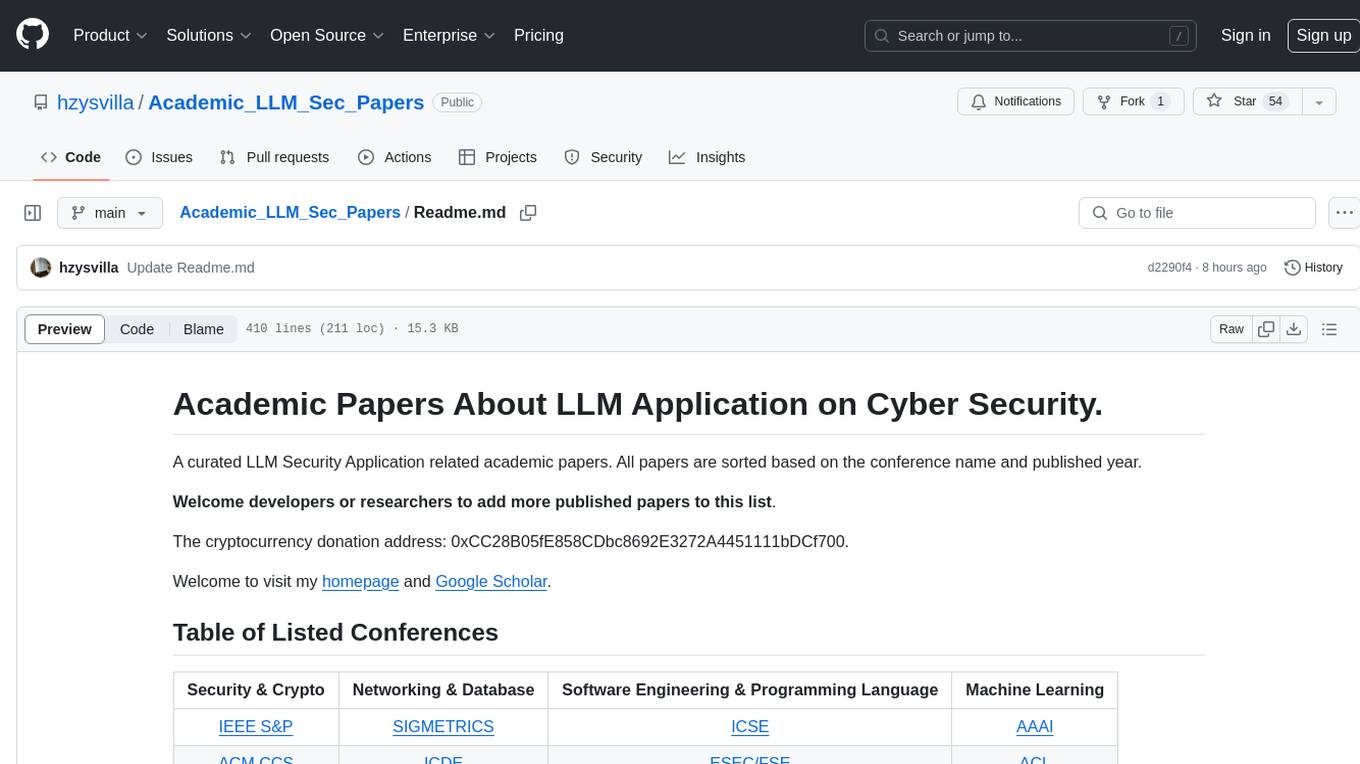
Academic_LLM_Sec_Papers
Academic_LLM_Sec_Papers is a curated collection of academic papers related to LLM Security Application. The repository includes papers sorted by conference name and published year, covering topics such as large language models for blockchain security, software engineering, machine learning, and more. Developers and researchers are welcome to contribute additional published papers to the list. The repository also provides information on listed conferences and journals related to security, networking, software engineering, and cryptography. The papers cover a wide range of topics including privacy risks, ethical concerns, vulnerabilities, threat modeling, code analysis, fuzzing, and more.
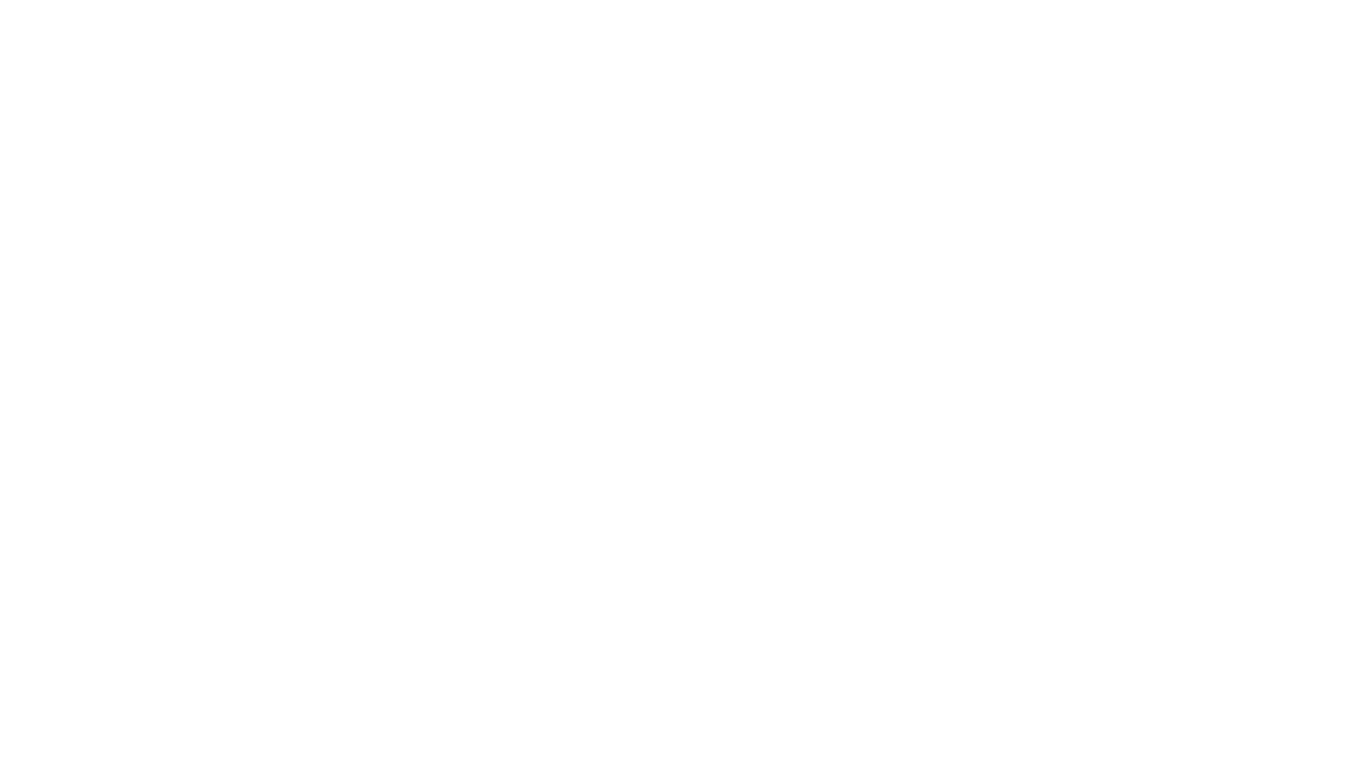
Telco-AIX
Telco-AIX is a collaborative experimental workspace dedicated to exploring data-driven decision-making use-cases using open source AI capabilities and open datasets. The repository focuses on projects related to revenue assurance, fraud management, service assurance, latency predictions, 5G network operations, sustainability, energy efficiency, SecOps-AI for networking, AI-powered SmartGrid, IoT perimeter security, anomaly detection, root cause analysis, customer relationship management voice app, Starlink quality of experience predictions, and NoC AI augmentation for OSS.
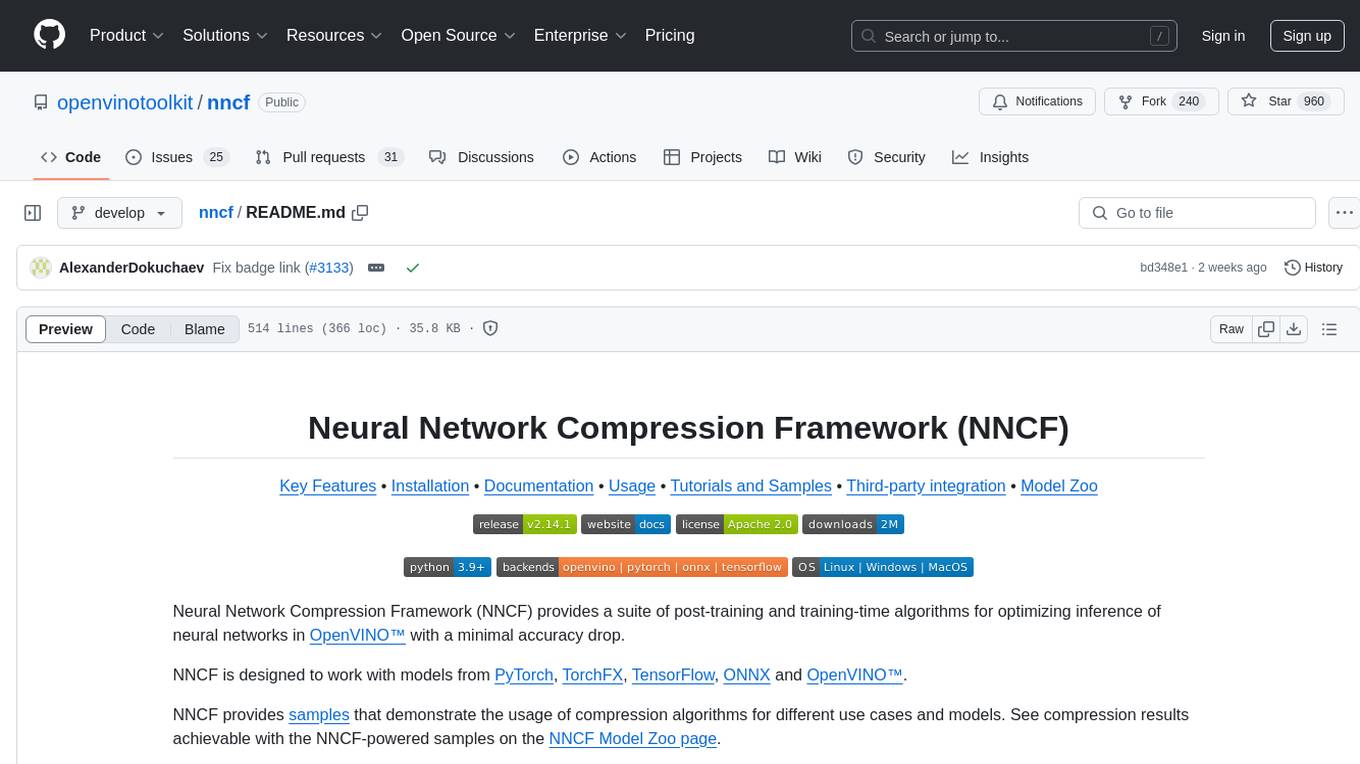
nncf
Neural Network Compression Framework (NNCF) provides a suite of post-training and training-time algorithms for optimizing inference of neural networks in OpenVINO™ with a minimal accuracy drop. It is designed to work with models from PyTorch, TorchFX, TensorFlow, ONNX, and OpenVINO™. NNCF offers samples demonstrating compression algorithms for various use cases and models, with the ability to add different compression algorithms easily. It supports GPU-accelerated layers, distributed training, and seamless combination of pruning, sparsity, and quantization algorithms. NNCF allows exporting compressed models to ONNX or TensorFlow formats for use with OpenVINO™ toolkit, and supports Accuracy-Aware model training pipelines via Adaptive Compression Level Training and Early Exit Training.
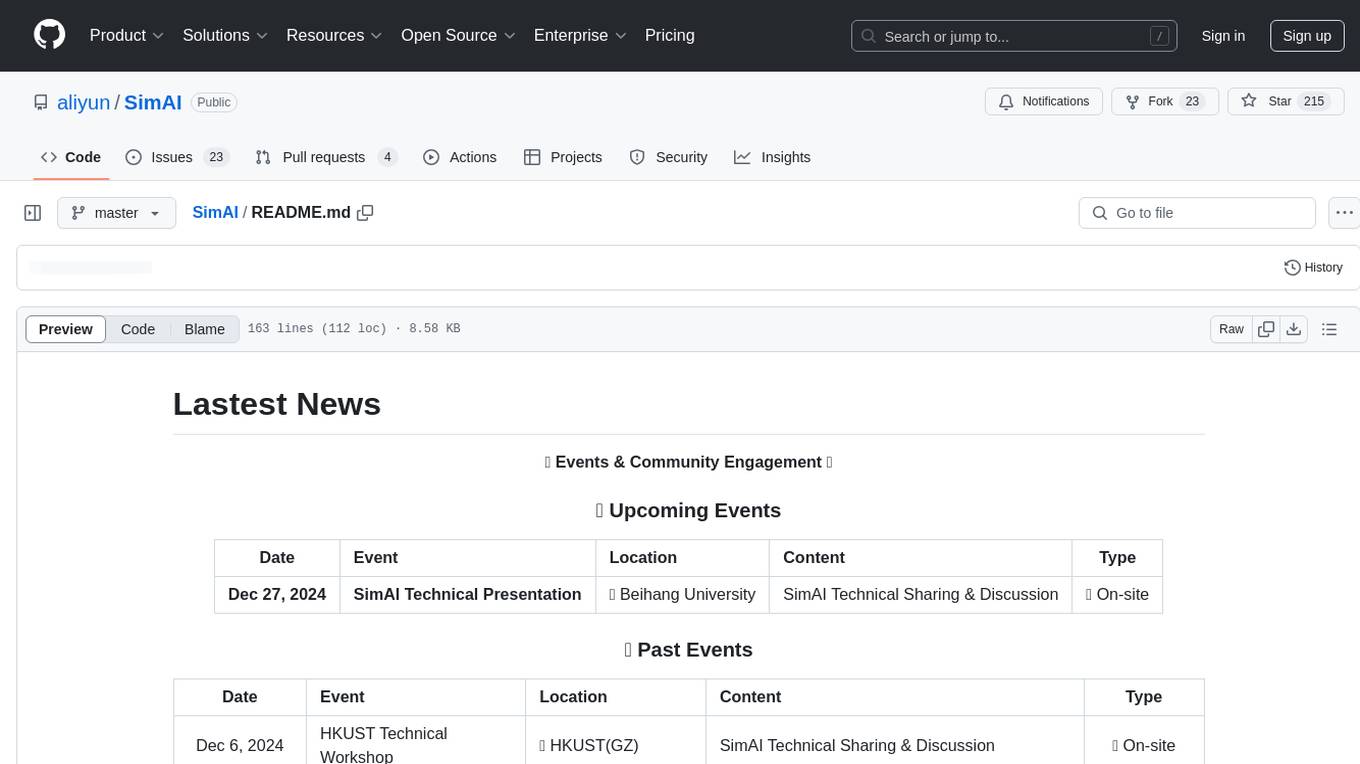
SimAI
SimAI is the industry's first full-stack, high-precision simulator for AI large-scale training. It provides detailed modeling and simulation of the entire LLM training process, encompassing framework, collective communication, network layers, and more. This comprehensive approach offers end-to-end performance data, enabling researchers to analyze training process details, evaluate time consumption of AI tasks under specific conditions, and assess performance gains from various algorithmic optimizations.
For similar tasks
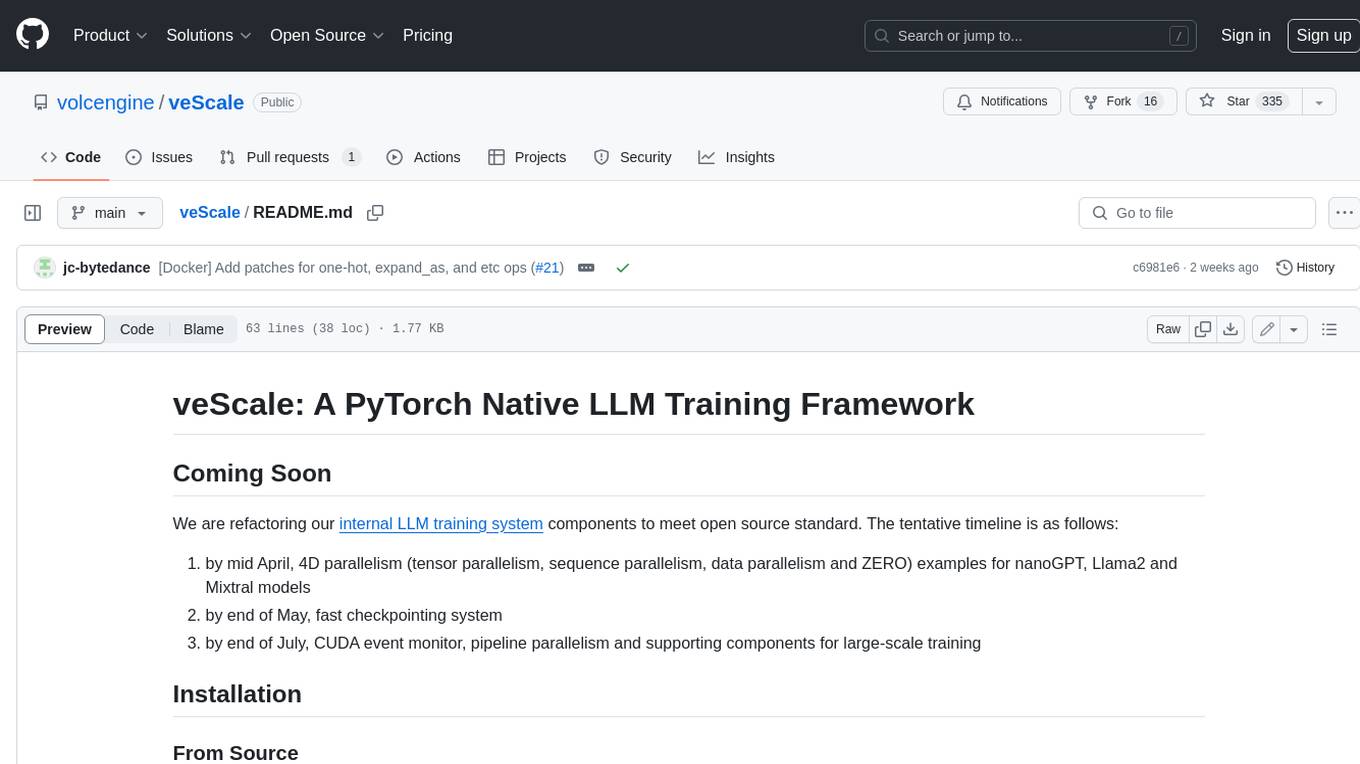
veScale
veScale is a PyTorch Native LLM Training Framework. It provides a set of tools and components to facilitate the training of large language models (LLMs) using PyTorch. veScale includes features such as 4D parallelism, fast checkpointing, and a CUDA event monitor. It is designed to be scalable and efficient, and it can be used to train LLMs on a variety of hardware platforms.
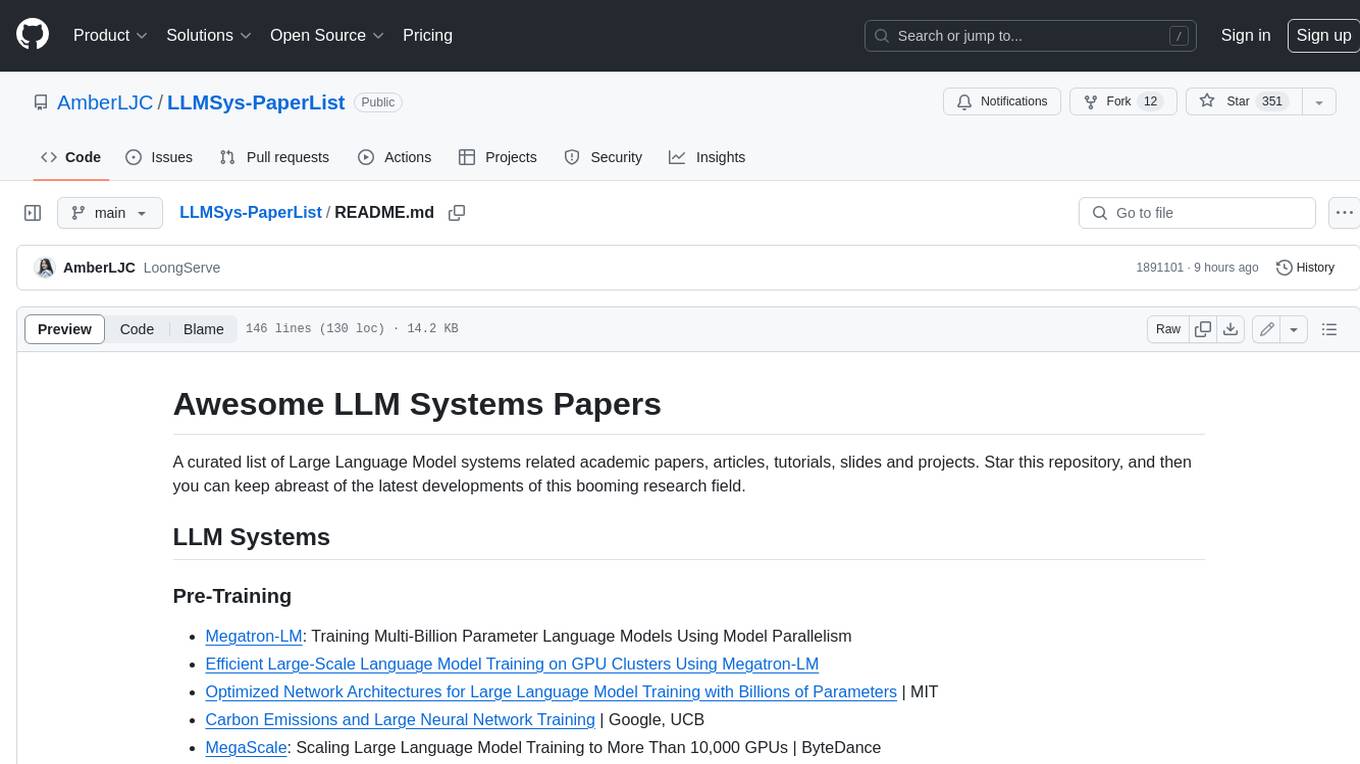
LLMSys-PaperList
This repository provides a comprehensive list of academic papers, articles, tutorials, slides, and projects related to Large Language Model (LLM) systems. It covers various aspects of LLM research, including pre-training, serving, system efficiency optimization, multi-model systems, image generation systems, LLM applications in systems, ML systems, survey papers, LLM benchmarks and leaderboards, and other relevant resources. The repository is regularly updated to include the latest developments in this rapidly evolving field, making it a valuable resource for researchers, practitioners, and anyone interested in staying abreast of the advancements in LLM technology.
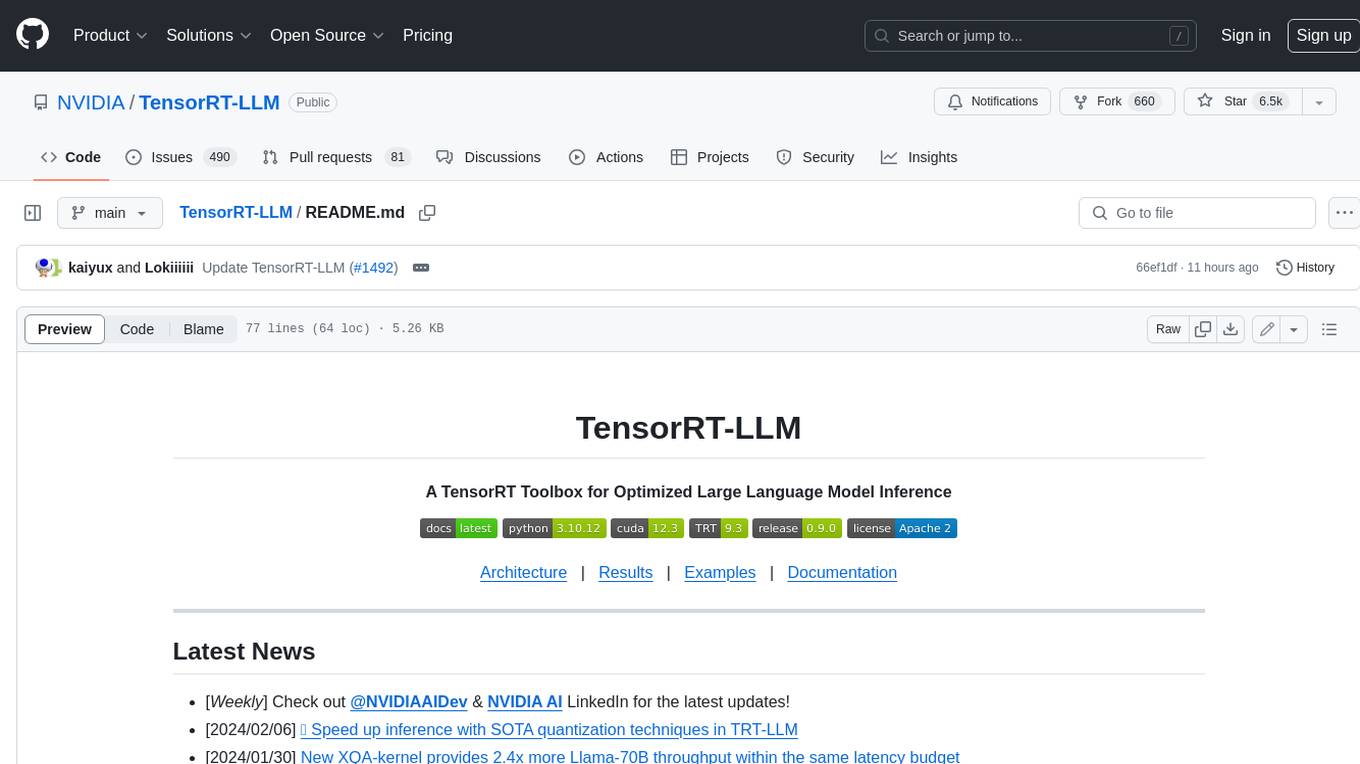
TensorRT-LLM
TensorRT-LLM is an easy-to-use Python API to define Large Language Models (LLMs) and build TensorRT engines that contain state-of-the-art optimizations to perform inference efficiently on NVIDIA GPUs. TensorRT-LLM contains components to create Python and C++ runtimes that execute those TensorRT engines. It also includes a backend for integration with the NVIDIA Triton Inference Server; a production-quality system to serve LLMs. Models built with TensorRT-LLM can be executed on a wide range of configurations going from a single GPU to multiple nodes with multiple GPUs (using Tensor Parallelism and/or Pipeline Parallelism).
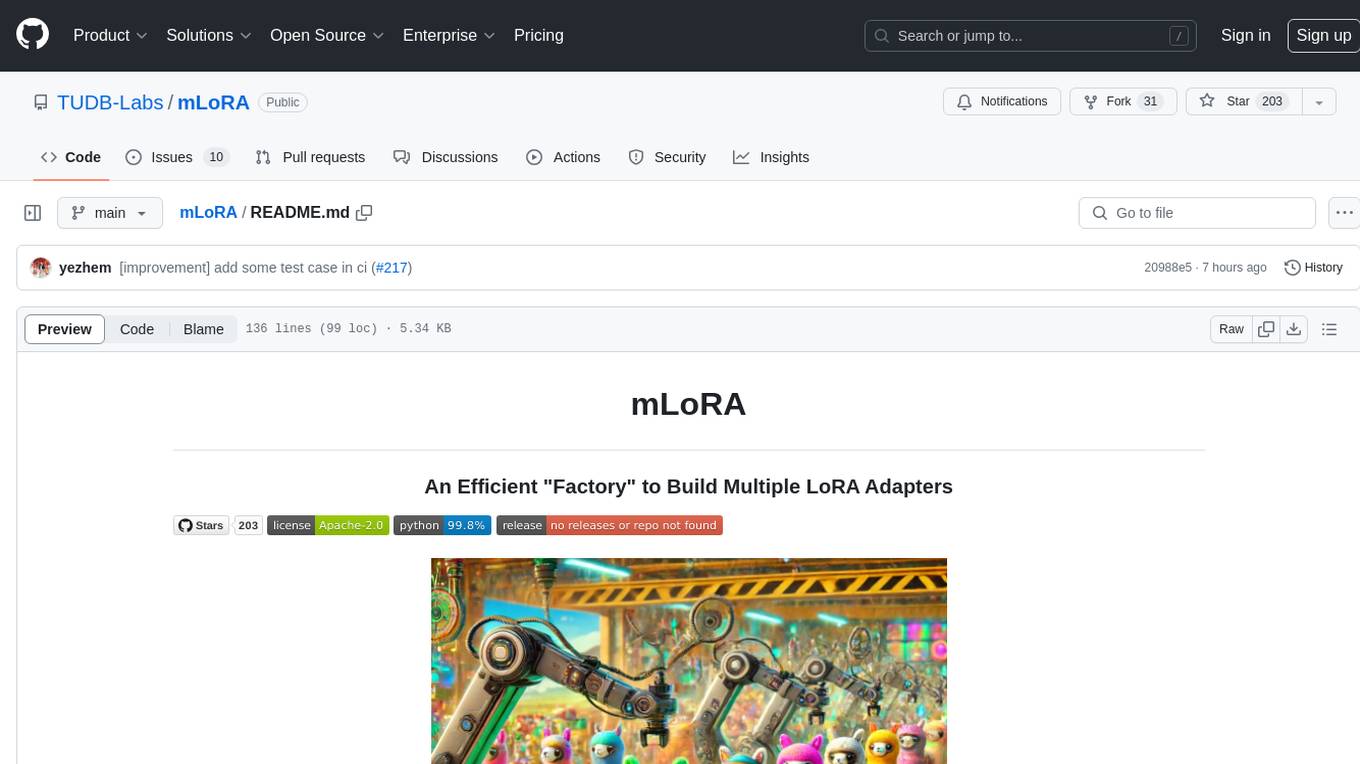
mLoRA
mLoRA (Multi-LoRA Fine-Tune) is an open-source framework for efficient fine-tuning of multiple Large Language Models (LLMs) using LoRA and its variants. It allows concurrent fine-tuning of multiple LoRA adapters with a shared base model, efficient pipeline parallelism algorithm, support for various LoRA variant algorithms, and reinforcement learning preference alignment algorithms. mLoRA helps save computational and memory resources when training multiple adapters simultaneously, achieving high performance on consumer hardware.
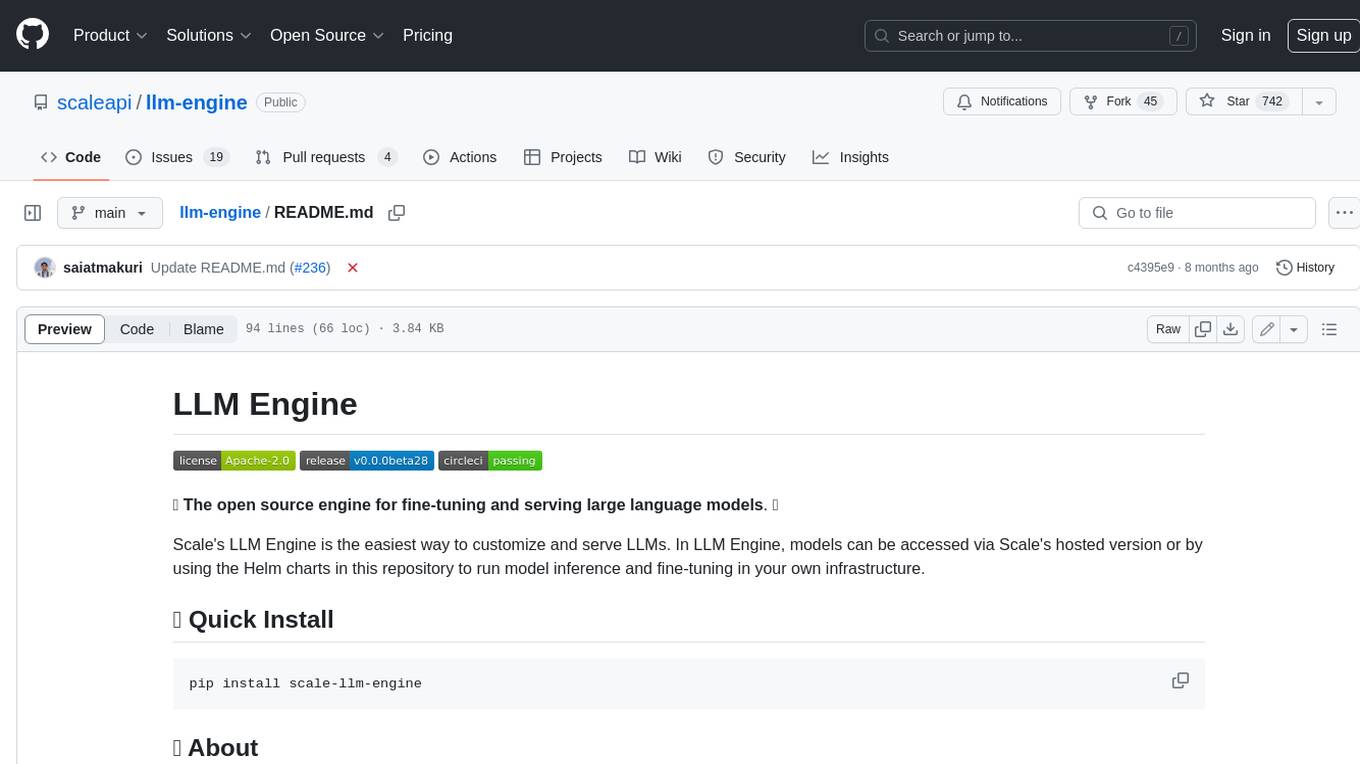
llm-engine
Scale's LLM Engine is an open-source Python library, CLI, and Helm chart that provides everything you need to serve and fine-tune foundation models, whether you use Scale's hosted infrastructure or do it in your own cloud infrastructure using Kubernetes.
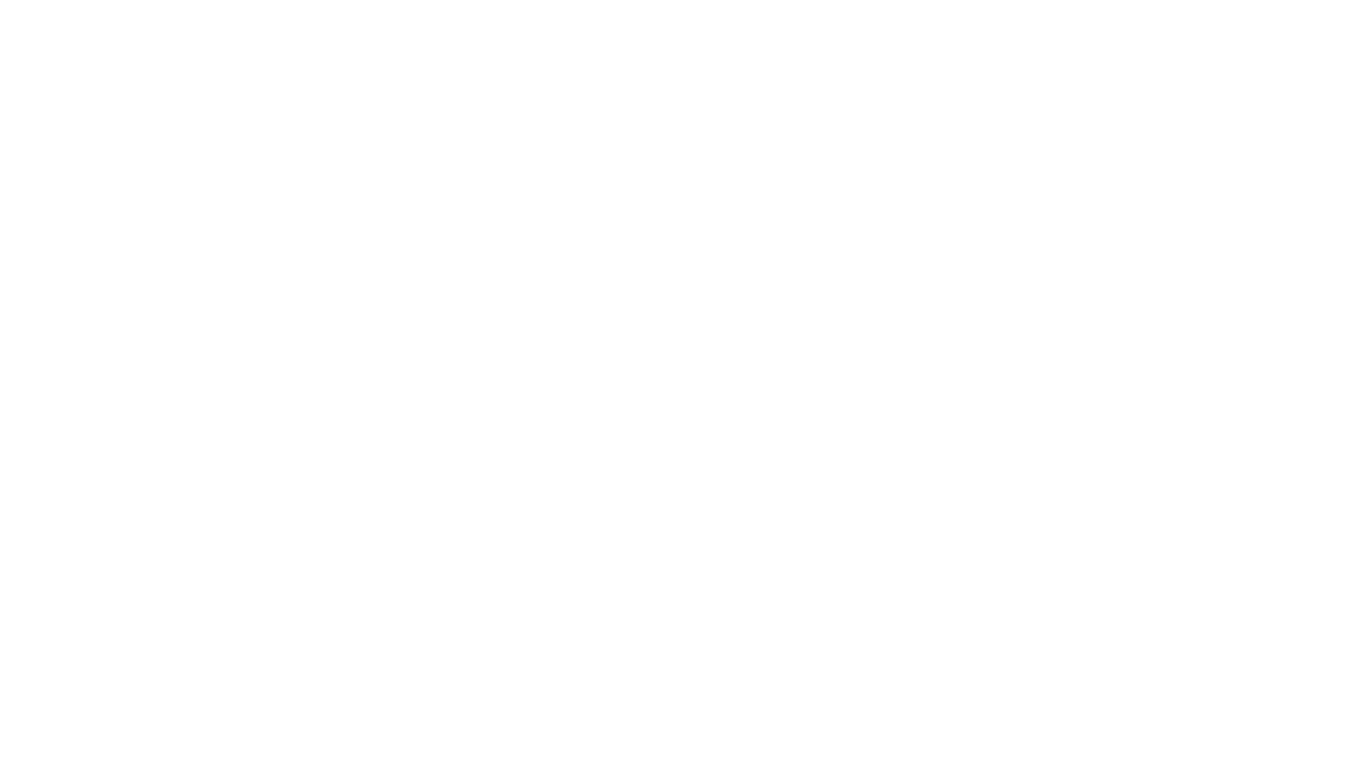
llm-on-openshift
This repository provides resources, demos, and recipes for working with Large Language Models (LLMs) on OpenShift using OpenShift AI or Open Data Hub. It includes instructions for deploying inference servers for LLMs, such as vLLM, Hugging Face TGI, Caikit-TGIS-Serving, and Ollama. Additionally, it offers guidance on deploying serving runtimes, such as vLLM Serving Runtime and Hugging Face Text Generation Inference, in the Single-Model Serving stack of Open Data Hub or OpenShift AI. The repository also covers vector databases that can be used as a Vector Store for Retrieval Augmented Generation (RAG) applications, including Milvus, PostgreSQL+pgvector, and Redis. Furthermore, it provides examples of inference and application usage, such as Caikit, Langchain, Langflow, and UI examples.
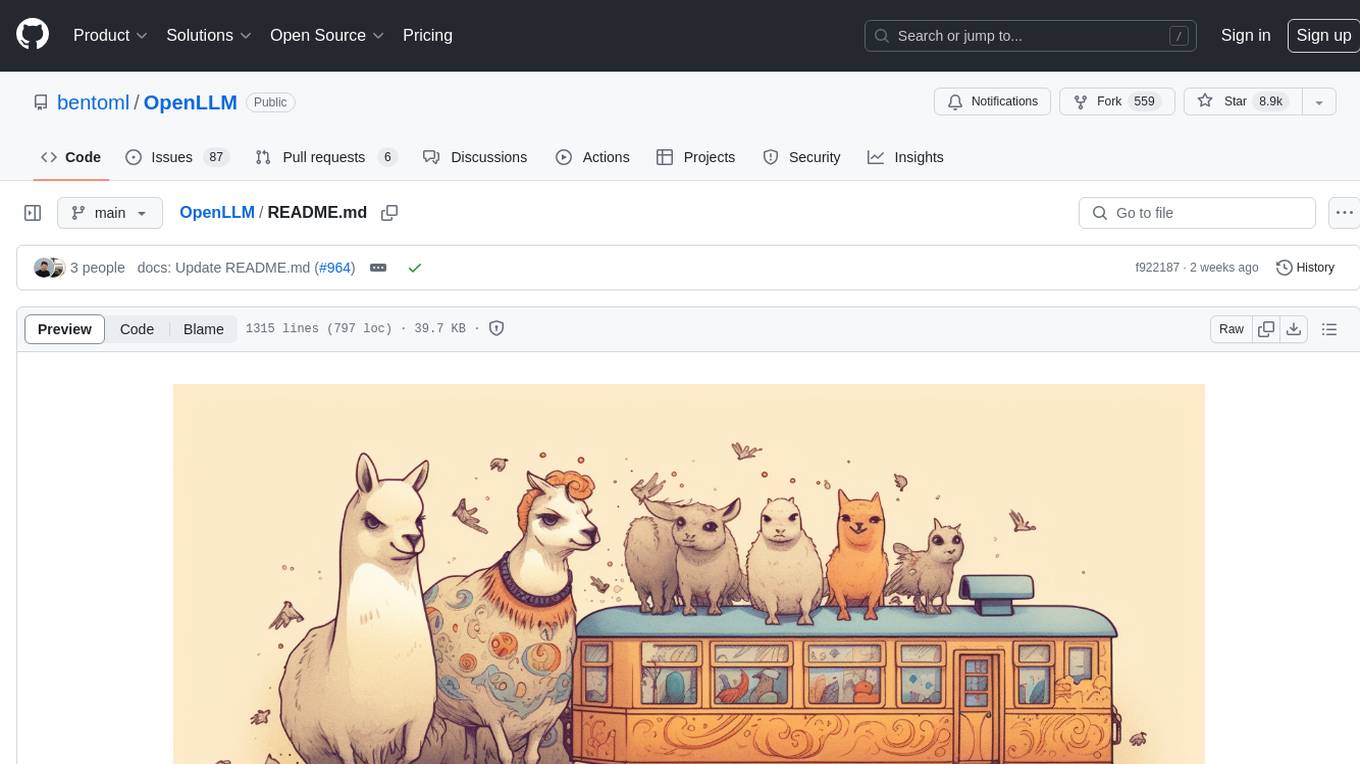
OpenLLM
OpenLLM is a platform that helps developers run any open-source Large Language Models (LLMs) as OpenAI-compatible API endpoints, locally and in the cloud. It supports a wide range of LLMs, provides state-of-the-art serving and inference performance, and simplifies cloud deployment via BentoML. Users can fine-tune, serve, deploy, and monitor any LLMs with ease using OpenLLM. The platform also supports various quantization techniques, serving fine-tuning layers, and multiple runtime implementations. OpenLLM seamlessly integrates with other tools like OpenAI Compatible Endpoints, LlamaIndex, LangChain, and Transformers Agents. It offers deployment options through Docker containers, BentoCloud, and provides a community for collaboration and contributions.
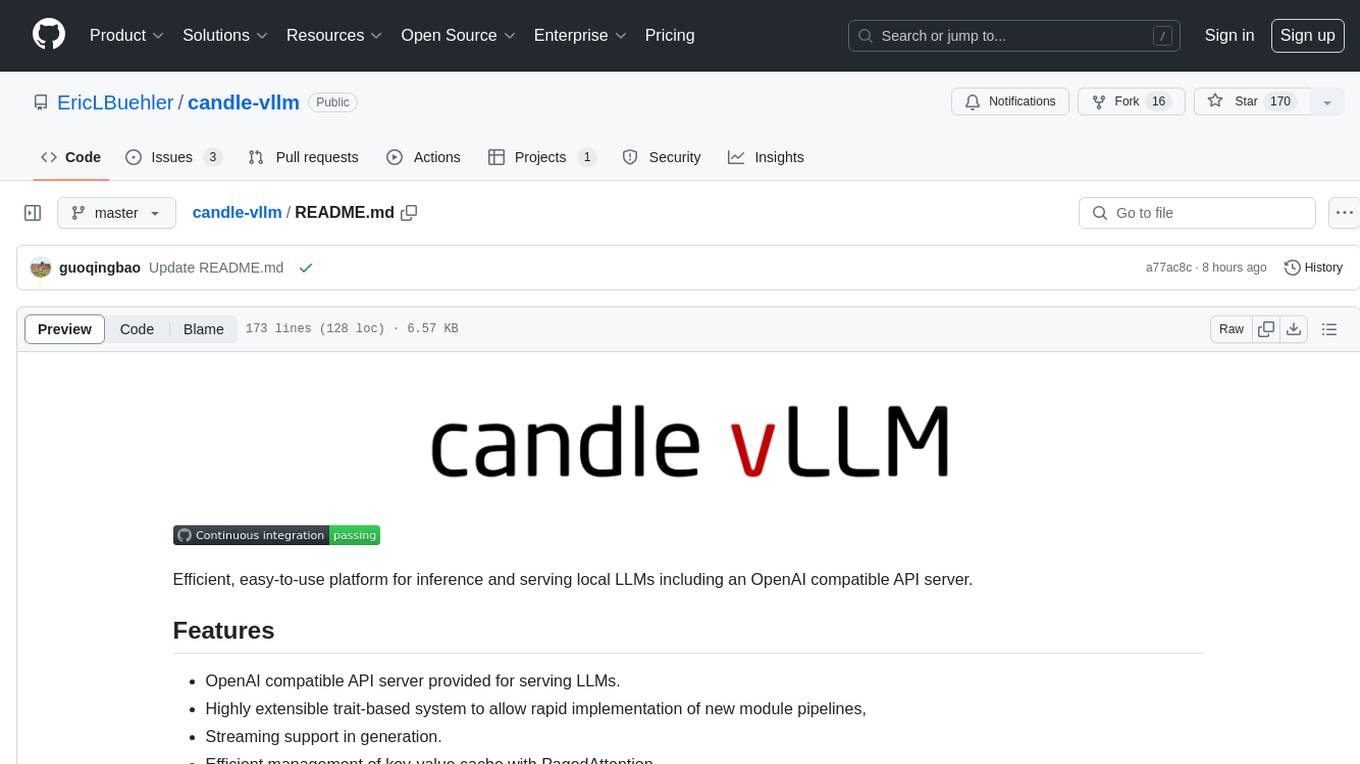
candle-vllm
Candle-vllm is an efficient and easy-to-use platform designed for inference and serving local LLMs, featuring an OpenAI compatible API server. It offers a highly extensible trait-based system for rapid implementation of new module pipelines, streaming support in generation, efficient management of key-value cache with PagedAttention, and continuous batching. The tool supports chat serving for various models and provides a seamless experience for users to interact with LLMs through different interfaces.
For similar jobs
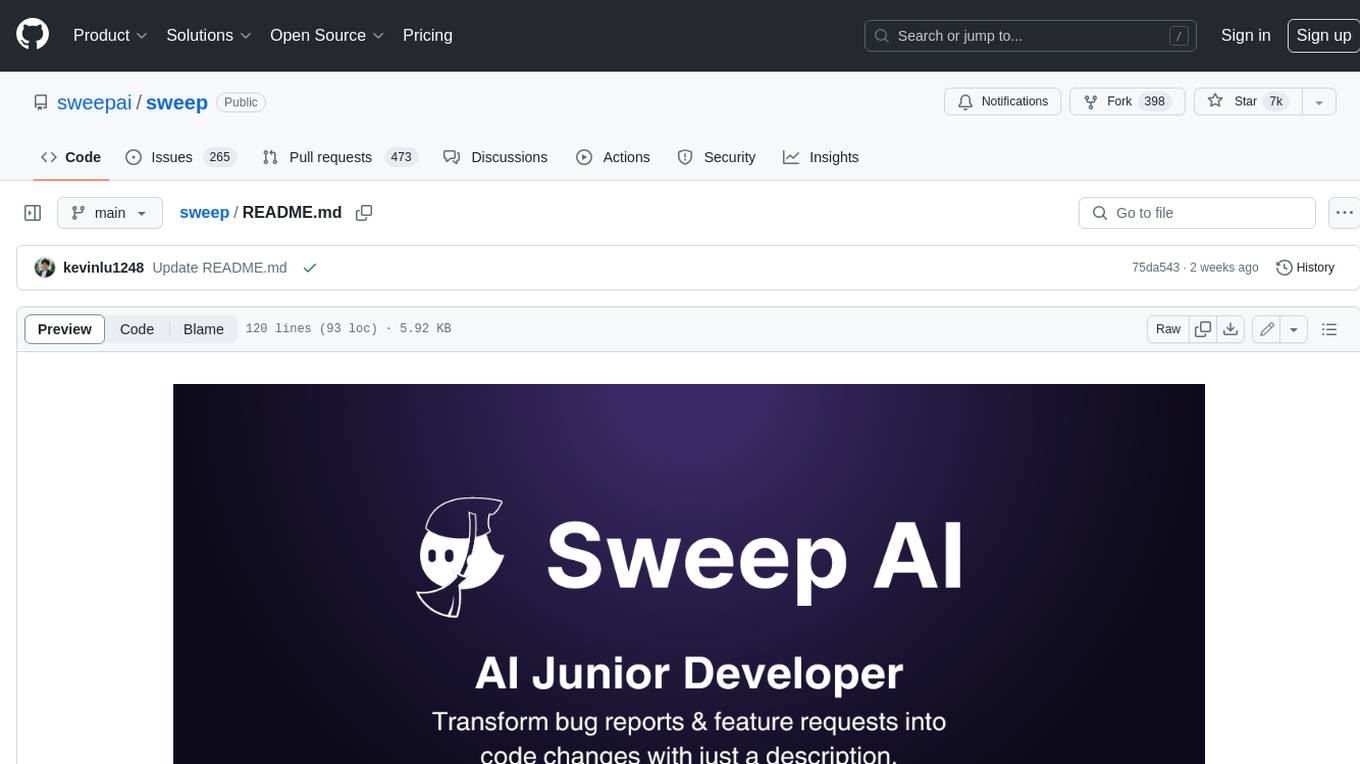
sweep
Sweep is an AI junior developer that turns bugs and feature requests into code changes. It automatically handles developer experience improvements like adding type hints and improving test coverage.
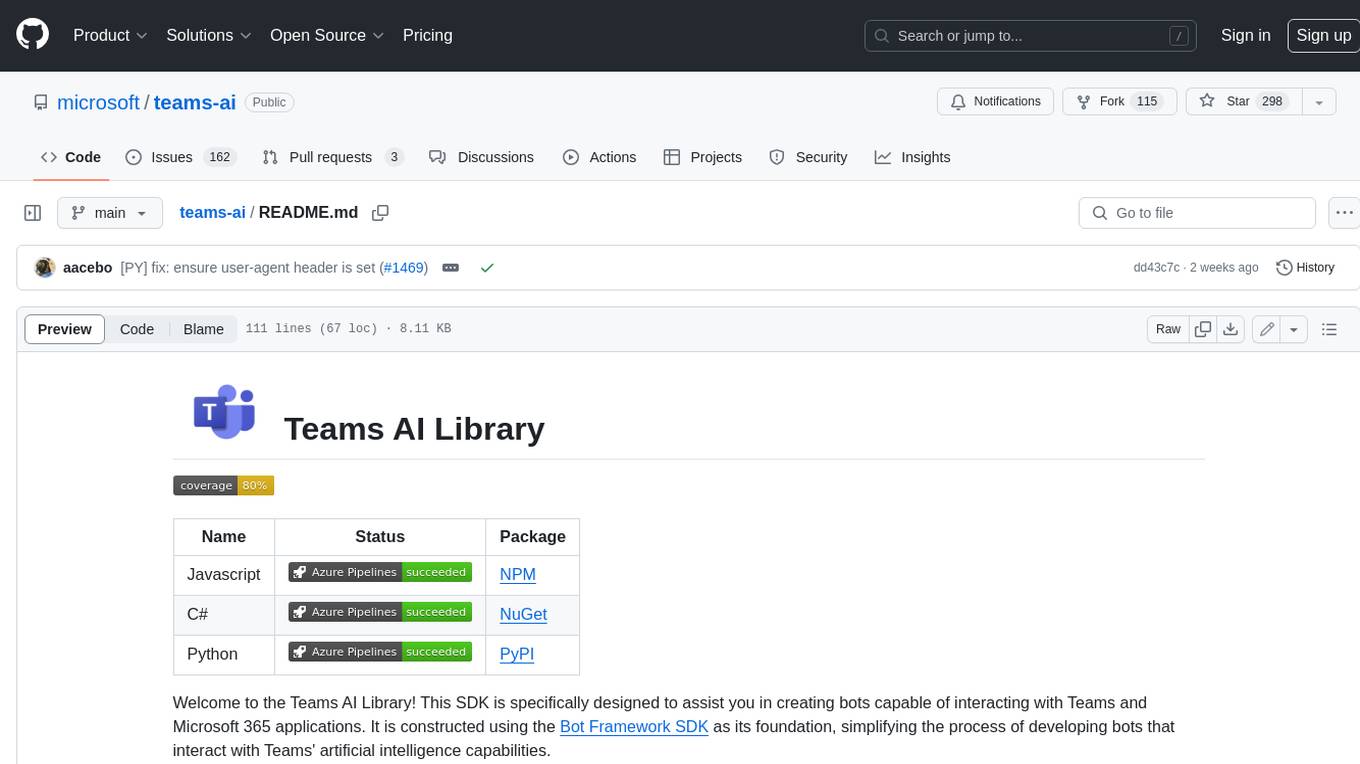
teams-ai
The Teams AI Library is a software development kit (SDK) that helps developers create bots that can interact with Teams and Microsoft 365 applications. It is built on top of the Bot Framework SDK and simplifies the process of developing bots that interact with Teams' artificial intelligence capabilities. The SDK is available for JavaScript/TypeScript, .NET, and Python.
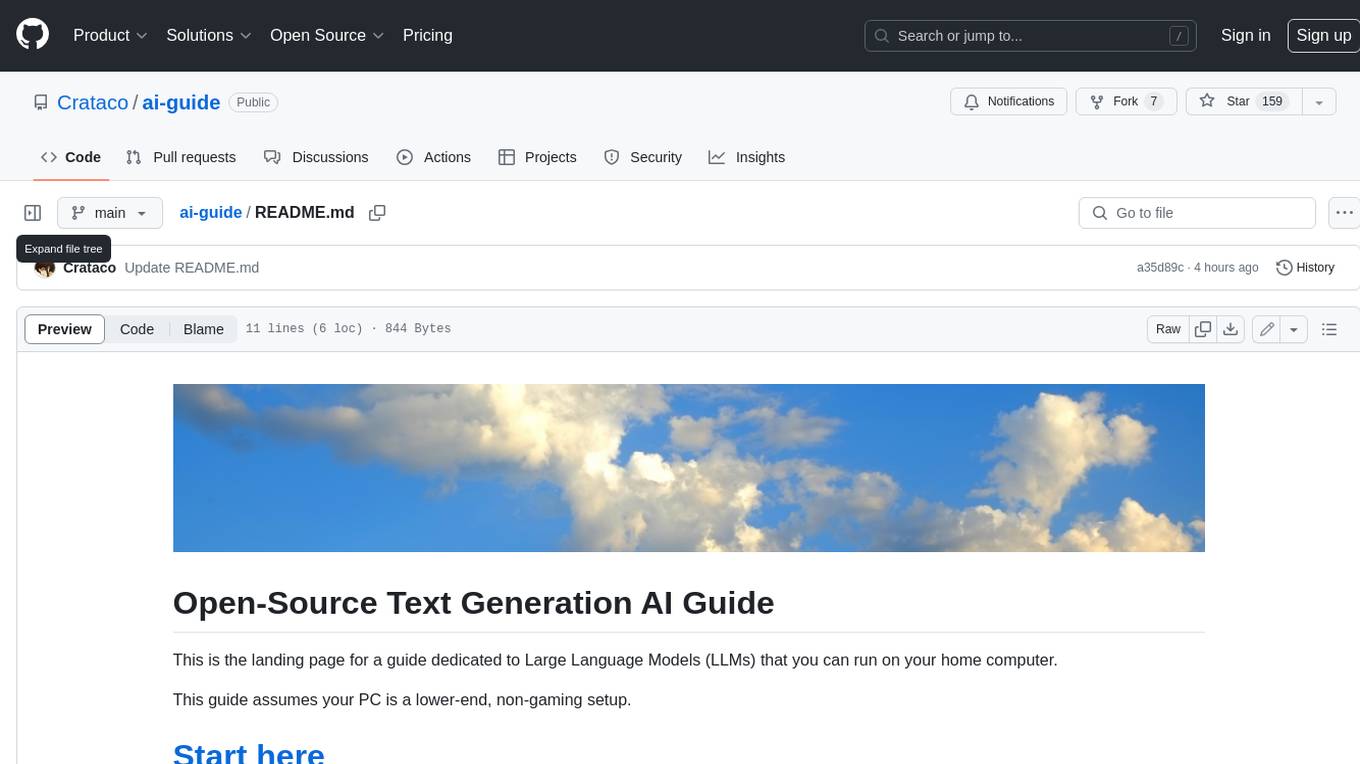
ai-guide
This guide is dedicated to Large Language Models (LLMs) that you can run on your home computer. It assumes your PC is a lower-end, non-gaming setup.
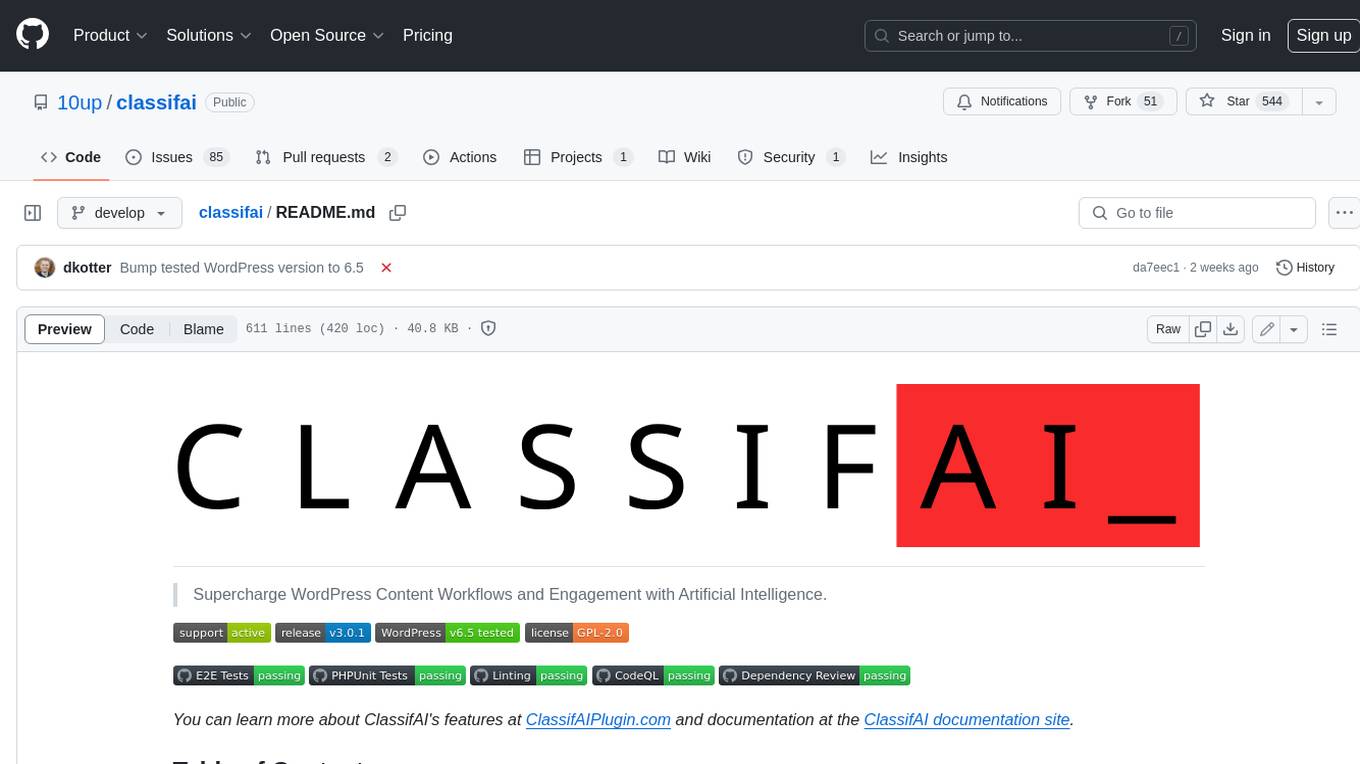
classifai
Supercharge WordPress Content Workflows and Engagement with Artificial Intelligence. Tap into leading cloud-based services like OpenAI, Microsoft Azure AI, Google Gemini and IBM Watson to augment your WordPress-powered websites. Publish content faster while improving SEO performance and increasing audience engagement. ClassifAI integrates Artificial Intelligence and Machine Learning technologies to lighten your workload and eliminate tedious tasks, giving you more time to create original content that matters.
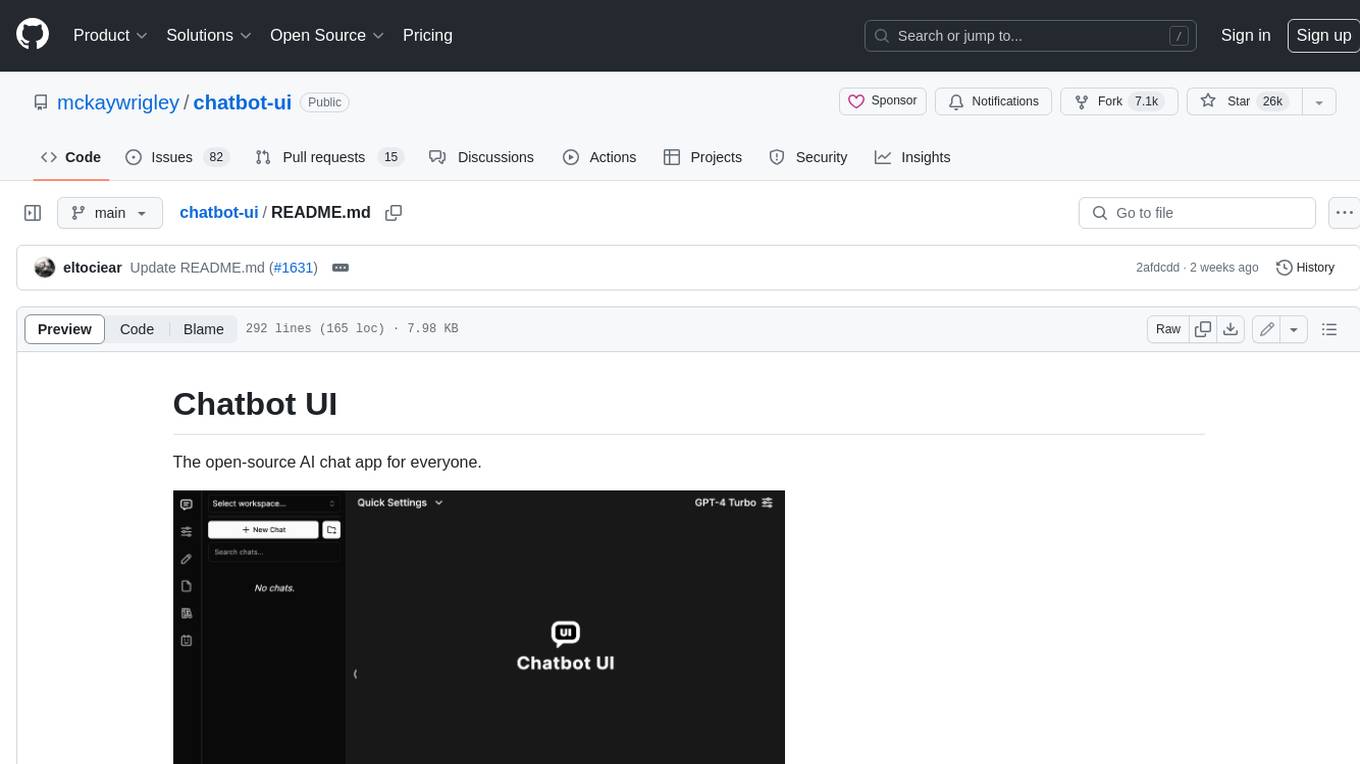
chatbot-ui
Chatbot UI is an open-source AI chat app that allows users to create and deploy their own AI chatbots. It is easy to use and can be customized to fit any need. Chatbot UI is perfect for businesses, developers, and anyone who wants to create a chatbot.
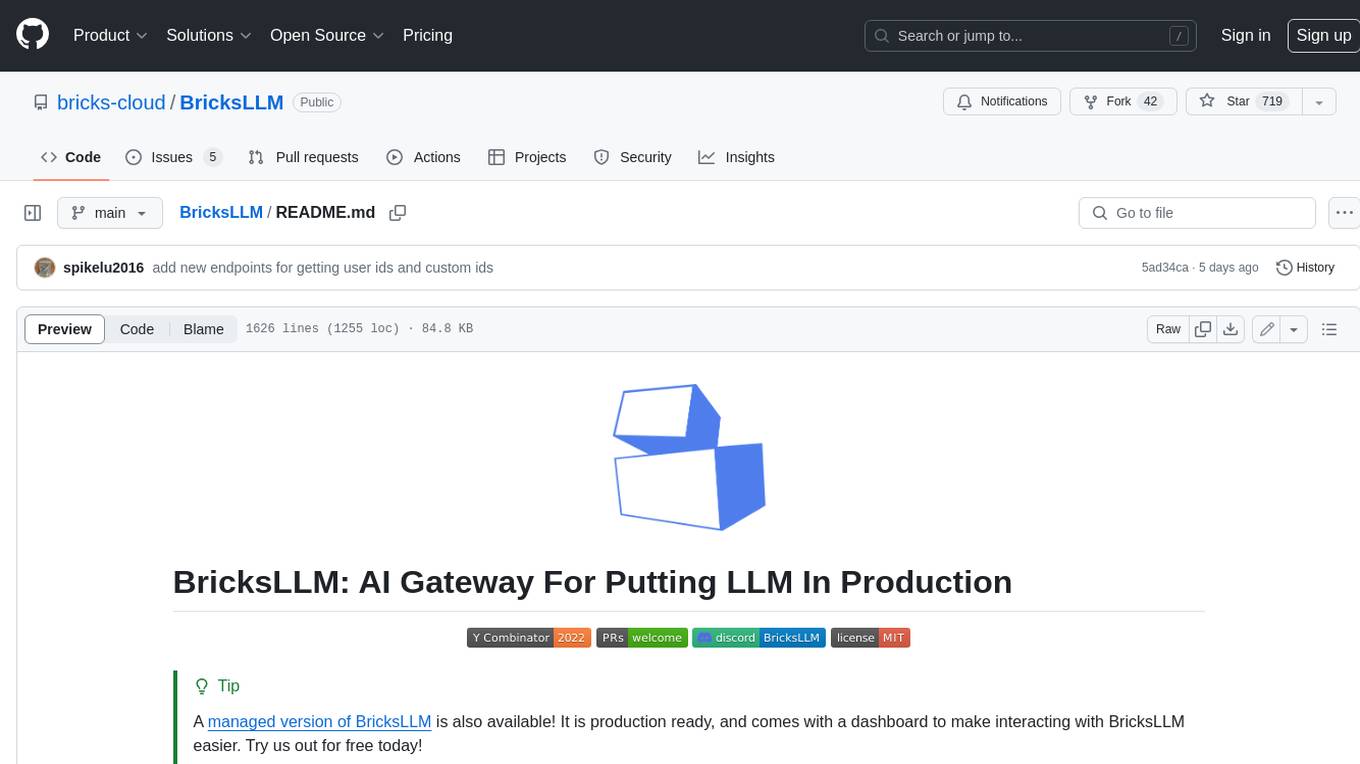
BricksLLM
BricksLLM is a cloud native AI gateway written in Go. Currently, it provides native support for OpenAI, Anthropic, Azure OpenAI and vLLM. BricksLLM aims to provide enterprise level infrastructure that can power any LLM production use cases. Here are some use cases for BricksLLM: * Set LLM usage limits for users on different pricing tiers * Track LLM usage on a per user and per organization basis * Block or redact requests containing PIIs * Improve LLM reliability with failovers, retries and caching * Distribute API keys with rate limits and cost limits for internal development/production use cases * Distribute API keys with rate limits and cost limits for students
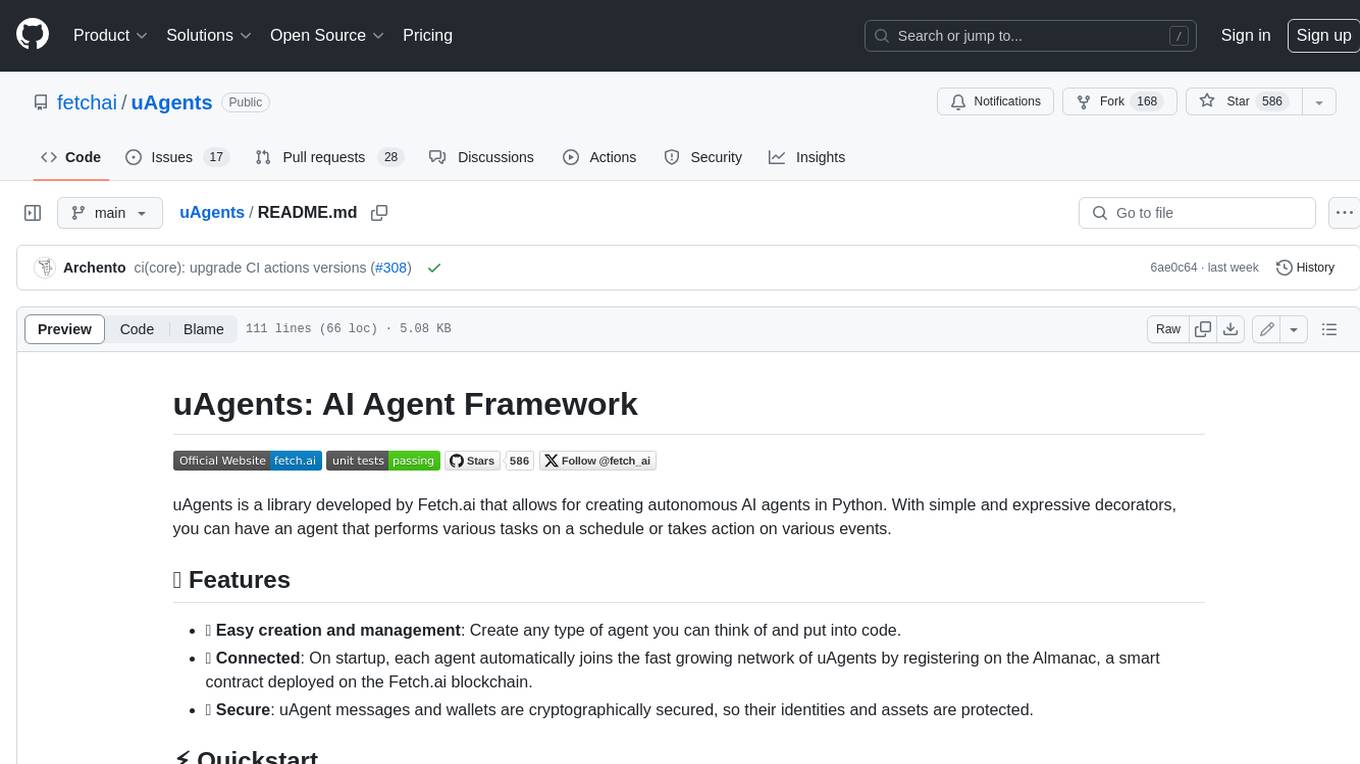
uAgents
uAgents is a Python library developed by Fetch.ai that allows for the creation of autonomous AI agents. These agents can perform various tasks on a schedule or take action on various events. uAgents are easy to create and manage, and they are connected to a fast-growing network of other uAgents. They are also secure, with cryptographically secured messages and wallets.
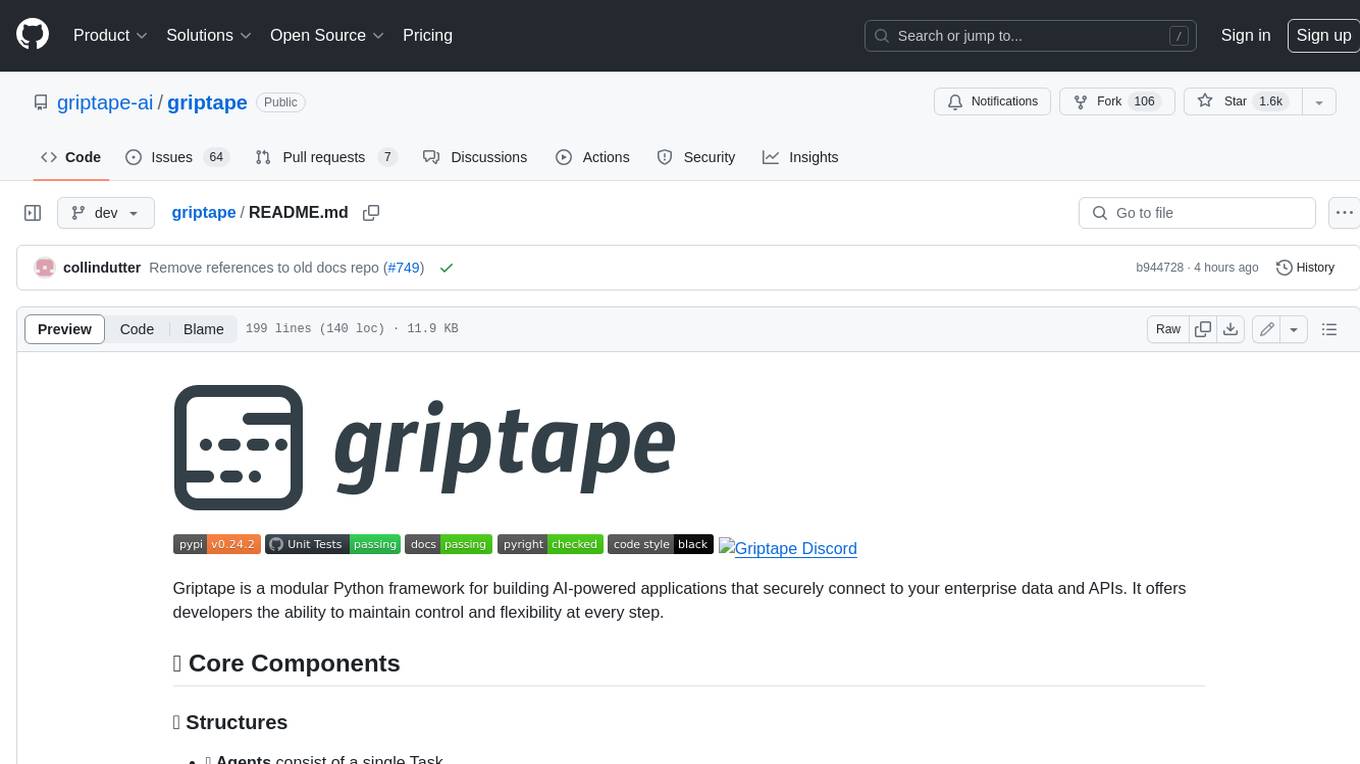
griptape
Griptape is a modular Python framework for building AI-powered applications that securely connect to your enterprise data and APIs. It offers developers the ability to maintain control and flexibility at every step. Griptape's core components include Structures (Agents, Pipelines, and Workflows), Tasks, Tools, Memory (Conversation Memory, Task Memory, and Meta Memory), Drivers (Prompt and Embedding Drivers, Vector Store Drivers, Image Generation Drivers, Image Query Drivers, SQL Drivers, Web Scraper Drivers, and Conversation Memory Drivers), Engines (Query Engines, Extraction Engines, Summary Engines, Image Generation Engines, and Image Query Engines), and additional components (Rulesets, Loaders, Artifacts, Chunkers, and Tokenizers). Griptape enables developers to create AI-powered applications with ease and efficiency.