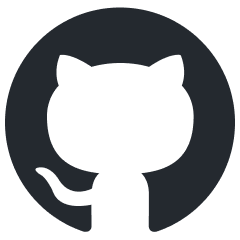
HuatuoGPT-II
HuatuoGPT2, One-stage Training for Medical Adaption of LLMs. (An Open Medical GPT)
Stars: 308
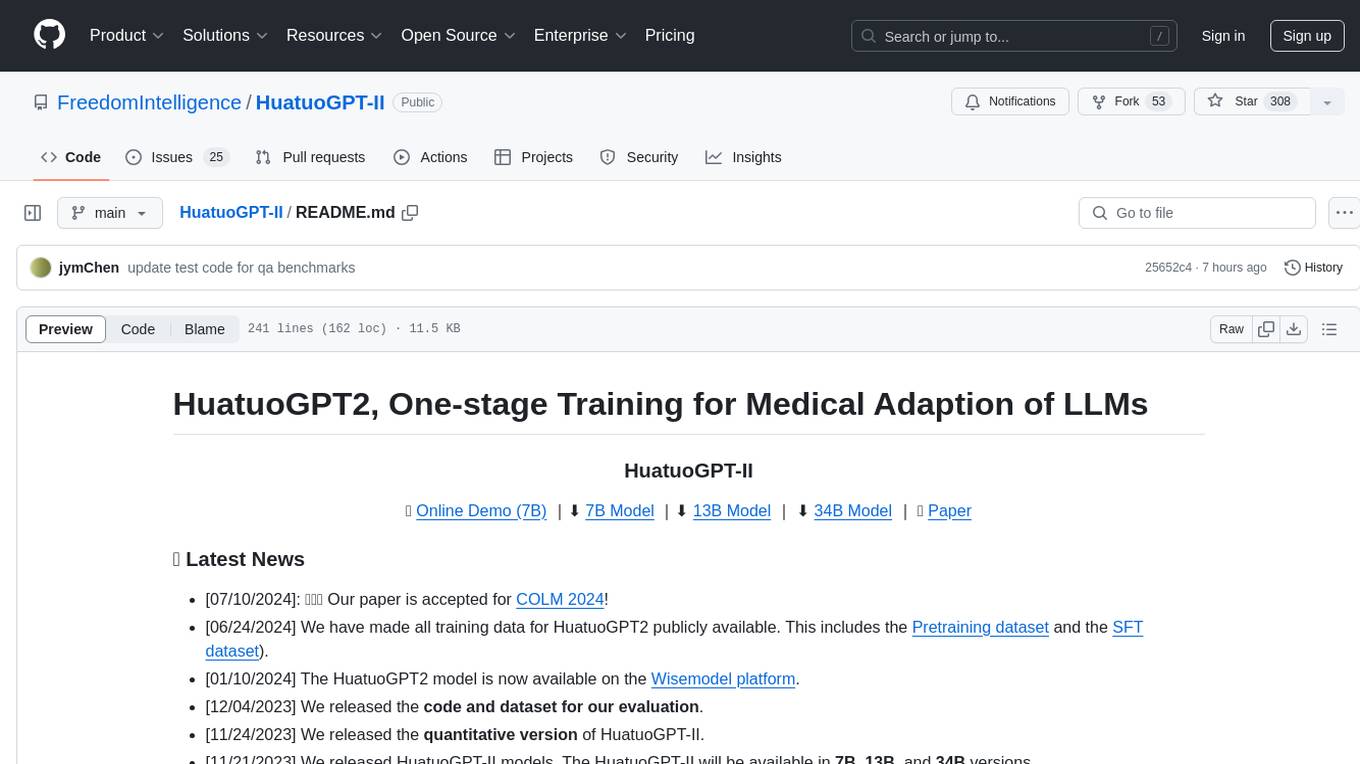
HuatuoGPT2 is an innovative domain-adapted medical large language model that excels in medical knowledge and dialogue proficiency. It showcases state-of-the-art performance in various medical benchmarks, surpassing GPT-4 in expert evaluations and fresh medical licensing exams. The open-source release includes HuatuoGPT2 models in 7B, 13B, and 34B versions, training code for one-stage adaptation, partial pre-training and fine-tuning instructions, and evaluation methods for medical response capabilities and professional pharmacist exams. The tool aims to enhance LLM capabilities in the Chinese medical field through open-source principles.
README:
🖥️ Online Demo (7B) |⬇️ 7B Model |⬇️ 13B Model | ⬇️ 34B Model | 📃 Paper
- [07/10/2024]: 🎉🎉🎉 Our paper is accepted for COLM 2024!
- [06/24/2024] We have made all training data for HuatuoGPT2 publicly available. This includes the Pretraining dataset and the SFT dataset).
- [01/10/2024] The HuatuoGPT2 model is now available on the Wisemodel platform.
- [12/04/2023] We released the code and dataset for our evaluation.
- [11/24/2023] We released the quantitative version of HuatuoGPT-II.
- [11/21/2023] We released HuatuoGPT-II models. The HuatuoGPT-II will be available in 7B, 13B, and 34B versions.
- [11/17/2023] We released the HuatuoGPT-II paper, achieving a new state-of-the-art in Chinese medical applications! Try our demo!
Hello! Welcome to the repository for HuatuoGPT2.
HuatuoGPT2 employs an innovative domain adaptation method to significantly boost its medical knowledge and dialogue proficiency. It showcases state-of-the-art performance in several medical benchmarks, especially surpassing GPT-4 in expert evaluations and the fresh medical licensing exams.
The open-source release of HuatuoGPT-2 includes:
- HuatuoGPT2 Model: Open-sourcing of 7B, 13B, and 34B versions.
- Training Code: Training code for one-stage adaptation will be provided, enabling better model adaptation across various languages and domains.
- HuatuoGPT2 Data: Release of partial pre-training and fine-tuning instructions.
- Evaluation for Chinese Medical LLM: Comprehensive automatic evaluation methods for medical response capabilities of LLM and the fresh professional pharmacist exam assessment.
Note that we're still actively organizing our code and data. Please stay tuned for updates coming soon!
Compared with representative open-source models and closed-source models (including GPT-4), HuatuoGPT2 showed impressive performance on medical benchmarks. Here, we present two of the results.
- Expert Evaluation: In assessments by medical professionals, HuatuoGPT-II's responses in Chinese medical contexts were favored over counterparts like GPT-4:
HuatuoGPT-II Win Rate | Win | Tie | Fail |
---|---|---|---|
Single-round Medical Response | |||
HuatuoGPT-II(7B) vs GPT-4 | 38 | 38 | 24 |
HuatuoGPT-II(7B) vs ChatGPT | 52 | 33 | 15 |
HuatuoGPT-II(7B) vs Baichuan2-13B-Chat | 63 | 19 | 18 |
HuatuoGPT-II(7B) vs HuatuoGPT | 81 | 11 | 8 |
Multi-round Medical Dialogue | |||
HuatuoGPT-II(7B) vs GPT-4 | 53 | 17 | 30 |
HuatuoGPT-II(7B) vs ChatGPT | 56 | 11 | 33 |
HuatuoGPT-II(7B) vs Baichuan2-13B-Chat | 63 | 19 | 18 |
HuatuoGPT-II(7B) vs HuatuoGPT | 68 | 6 | 26 |
- The Fresh Medical Exams: We collected the fresh 2023 Chinese National Pharmacist Licensure Examination, which started on October 21, 2023. This date is later than our data finalization. HuatuoGPT2 achieved the best results in this exam, as shown below.
Our model is now available on Huggingface. You can Try our model in https://www.huatuogpt.cn/.
Model | Backbone | Checkpoint |
---|---|---|
HuatuoGPT2-7B | Baichuan2-7B-Base | HF Lnik |
HuatuoGPT2-13B | Baichuan2-13B-Base | HF Lnik |
HuatuoGPT2-34B | Yi-34B | HF Lnik |
A quantized version of HuatuoGPT2 is also provided, allowing users with constrained memory or computing resources to access our HuatuoGPT2.
Quantization | Backbone | Checkpoint |
---|---|---|
HuatuoGPT2-7B-4bits | Baichuan2-7B-Base | HF Lnik |
HuatuoGPT2-7B-8bits | Baichuan2-7B-Base | HF Lnik |
HuatuoGPT2-34B-4bits | Yi-34B | HF Lnik |
HuatuoGPT2-34B-8bits | Yi-34B | HF Lnik |
import torch
from transformers import AutoModelForCausalLM, AutoTokenizer
tokenizer = AutoTokenizer.from_pretrained("FreedomIntelligence/HuatuoGPT2-7B", use_fast=True, trust_remote_code=True)
model = AutoModelForCausalLM.from_pretrained("FreedomIntelligence/HuatuoGPT2-7B", device_map="auto", torch_dtype=torch.bfloat16, trust_remote_code=True)
messages = []
messages.append({"role": "user", "content": "肚子疼怎么办?"})
response = model.HuatuoChat(tokenizer, messages)
print(response)
python cli_demo.py --model_name FreedomIntelligence/HuatuoGPT2-7B
We open source part of the training data.
Data Type | # Training data | Link |
---|---|---|
Medical Fine-tuning Instruction (GPT-4) | 142,248 | HF Link |
Medical Pre-training Instruction | 5,286,308 | HF Link |
- HuatuoGPT2 transforms the pre-training corpus into (instruction, output) pairs using LLM. Utilize the script for Data Unification.
python adaption/data_unification/rewrite.py
- We introduce a priority sampling approach, pre-processing data with this algorithm:
python adaption/one_stage_training/data_process.py
- Then, training is conducted using one-stage training:
bash adaption/one_stage_training/train.sh
By adopting the One-stage Adaptation method, you will observe the following loss curve:
-- Evaluation code for the QA benchmarks.
accelerate launch evaluation/eval_qa.py --model_path=FreedomIntelligence/HuatuoGPT2-7B --data_path=./evaluation/data/eval_qa.json
- Single-turn response evaluation using GPT-4:
python evaluation/eval_huatuo_inst.py
- Multi-turn dialogue evaluation using GPT-4:
python evaluation/eval_huatuo_conv.py
Access our newest medical exam dataset via the link provided. The dataset includes complete exam questions, with exam dates noted to alert for potential leaks. We plan to release more updated exams in the future.
Examination | #Question | Exam Time | Links |
---|---|---|---|
2023 Chinese National Pharmacist Licensure Examination (Pharmacy) | 480 | 2023.10.22 | huggingface |
2023 Chinese National Pharmacist Licensure Examination (TCM) | 480 | 2023.10.22 | huggingface |
Other Fresh Medical Examinations is in coming |
The HuatuoGPT series has so far launched two generations:
- HuatuoGPT: A Doctor-like Medical Large Language Model
- HuatuoGPT-II: An Domain-enhanced Medical Large Language Model
In the future, we will continue to release new versions of HuatuoGPT. Our goal is to enhance the capabilities of LLM in the Chinese medical field and to adhere to open-source principles (aligned with the ethos of FreedomIntelligence). We hope to work together with everyone to promote the development of medical LLM!
We are from the School of Data Science, the Chinese University of Hong Kong, Shenzhen (CUHKSZ) and the Shenzhen Research Institute of Big Data (SRIBD).
@misc{chen2023huatuogptii,
title={HuatuoGPT-II, One-stage Training for Medical Adaption of LLMs},
author={Junying Chen and Xidong Wang and Anningzhe Gao and Feng Jiang and Shunian Chen and Hongbo Zhang and Dingjie Song and Wenya Xie and Chuyi Kong and Jianquan Li and Xiang Wan and Haizhou Li and Benyou Wang},
year={2023},
eprint={2311.09774},
archivePrefix={arXiv},
primaryClass={cs.CL}
}
@article{huatuogpt-2023,
title={HuatuoGPT, Towards Taming Language Models To Be a Doctor},
author={Hongbo Zhang and Junying Chen and Feng Jiang and Fei Yu and Zhihong Chen and Jianquan Li and Guiming Chen and Xiangbo Wu and Zhiyi Zhang and Qingying Xiao and Xiang Wan and Benyou Wang and Haizhou Li},
journal={arXiv preprint arXiv:2305.15075},
year={2023}
}
For Tasks:
Click tags to check more tools for each tasksFor Jobs:
Alternative AI tools for HuatuoGPT-II
Similar Open Source Tools
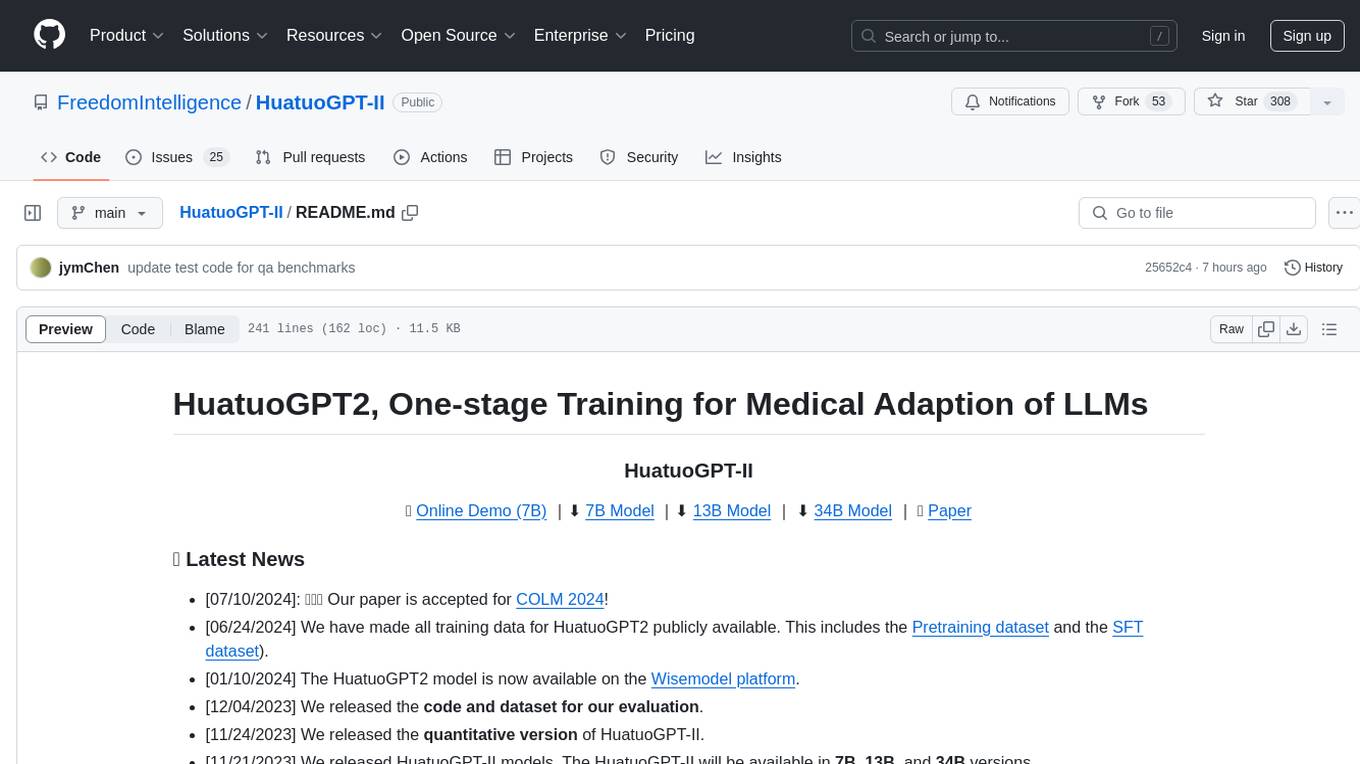
HuatuoGPT-II
HuatuoGPT2 is an innovative domain-adapted medical large language model that excels in medical knowledge and dialogue proficiency. It showcases state-of-the-art performance in various medical benchmarks, surpassing GPT-4 in expert evaluations and fresh medical licensing exams. The open-source release includes HuatuoGPT2 models in 7B, 13B, and 34B versions, training code for one-stage adaptation, partial pre-training and fine-tuning instructions, and evaluation methods for medical response capabilities and professional pharmacist exams. The tool aims to enhance LLM capabilities in the Chinese medical field through open-source principles.
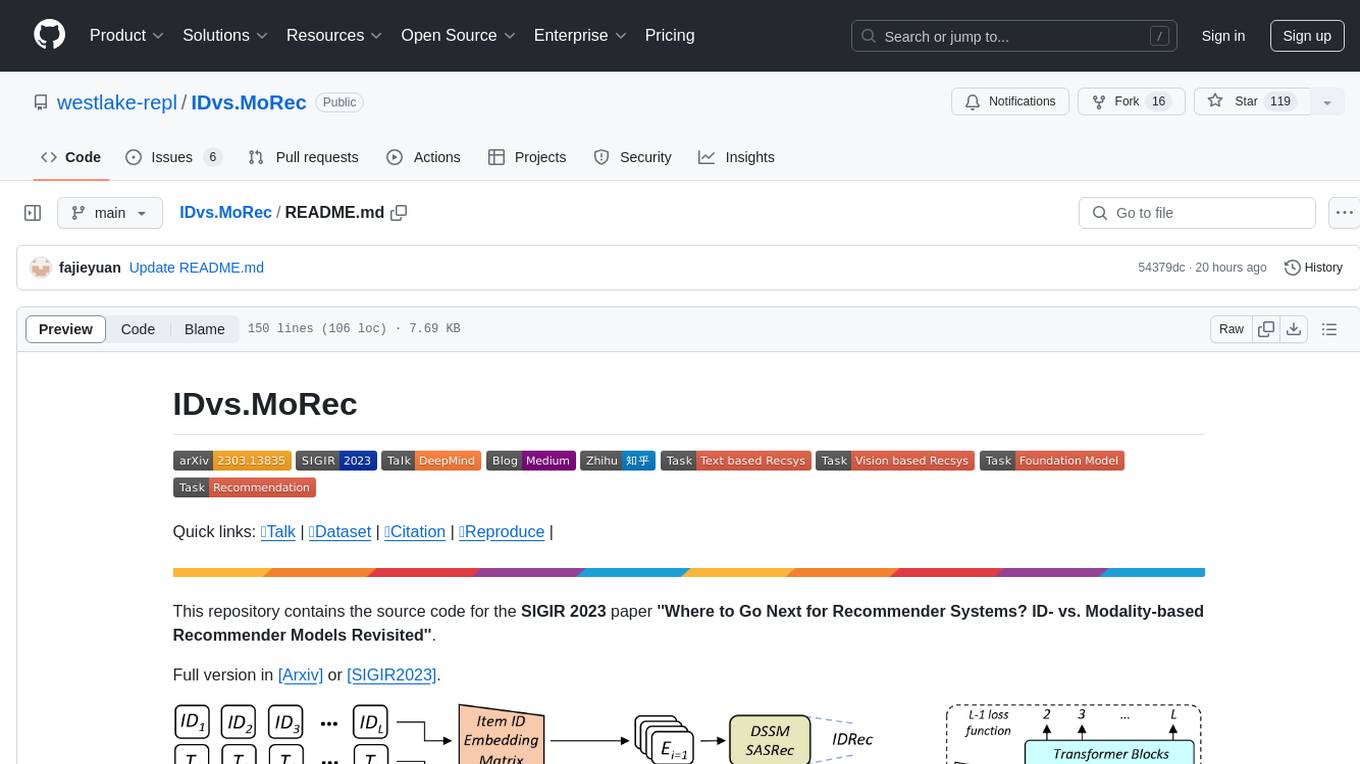
IDvs.MoRec
This repository contains the source code for the SIGIR 2023 paper 'Where to Go Next for Recommender Systems? ID- vs. Modality-based Recommender Models Revisited'. It provides resources for evaluating foundation, transferable, multi-modal, and LLM recommendation models, along with datasets, pre-trained models, and training strategies for IDRec and MoRec using in-batch debiased cross-entropy loss. The repository also offers large-scale datasets, code for SASRec with in-batch debias cross-entropy loss, and information on joining the lab for research opportunities.
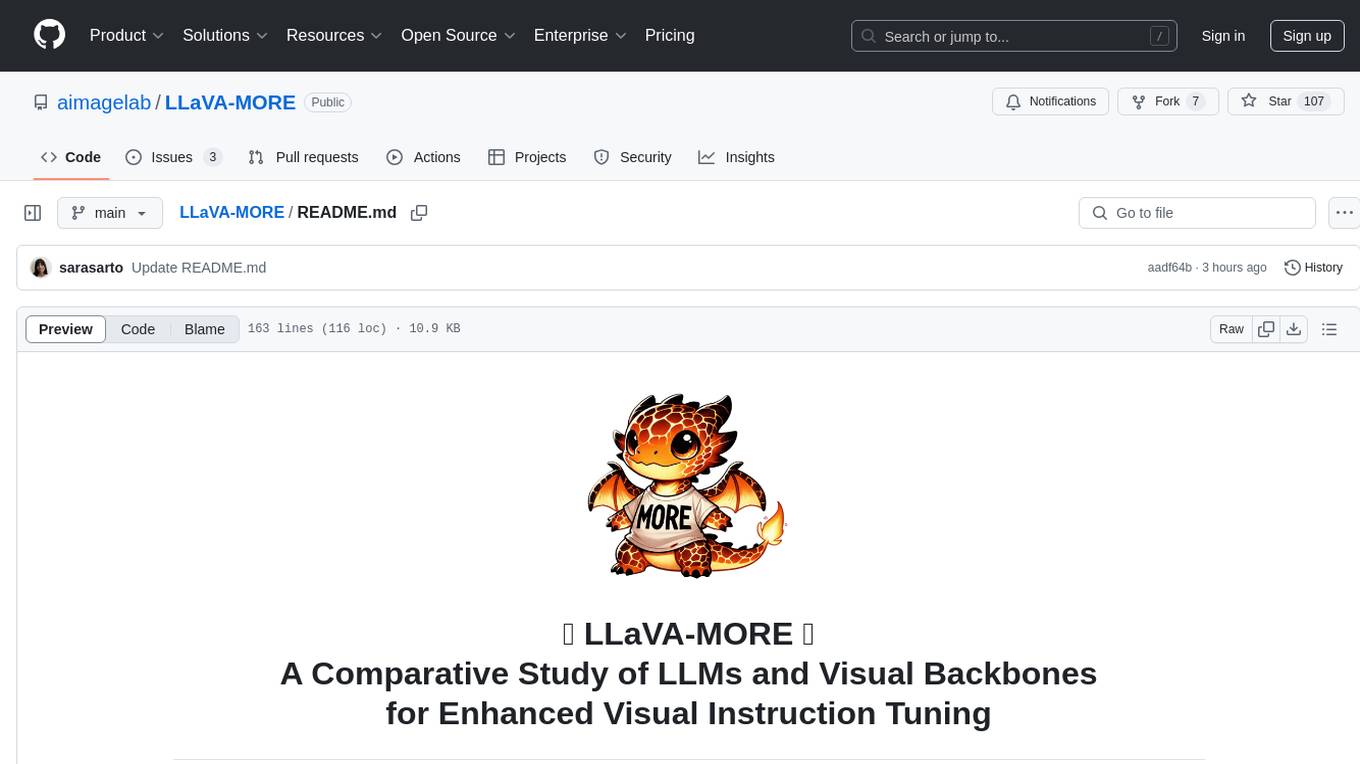
LLaVA-MORE
LLaVA-MORE is a new family of Multimodal Language Models (MLLMs) that integrates recent language models with diverse visual backbones. The repository provides a unified training protocol for fair comparisons across all architectures and releases training code and scripts for distributed training. It aims to enhance Multimodal LLM performance and offers various models for different tasks. Users can explore different visual backbones like SigLIP and methods for managing image resolutions (S2) to improve the connection between images and language. The repository is a starting point for expanding the study of Multimodal LLMs and enhancing new features in the field.
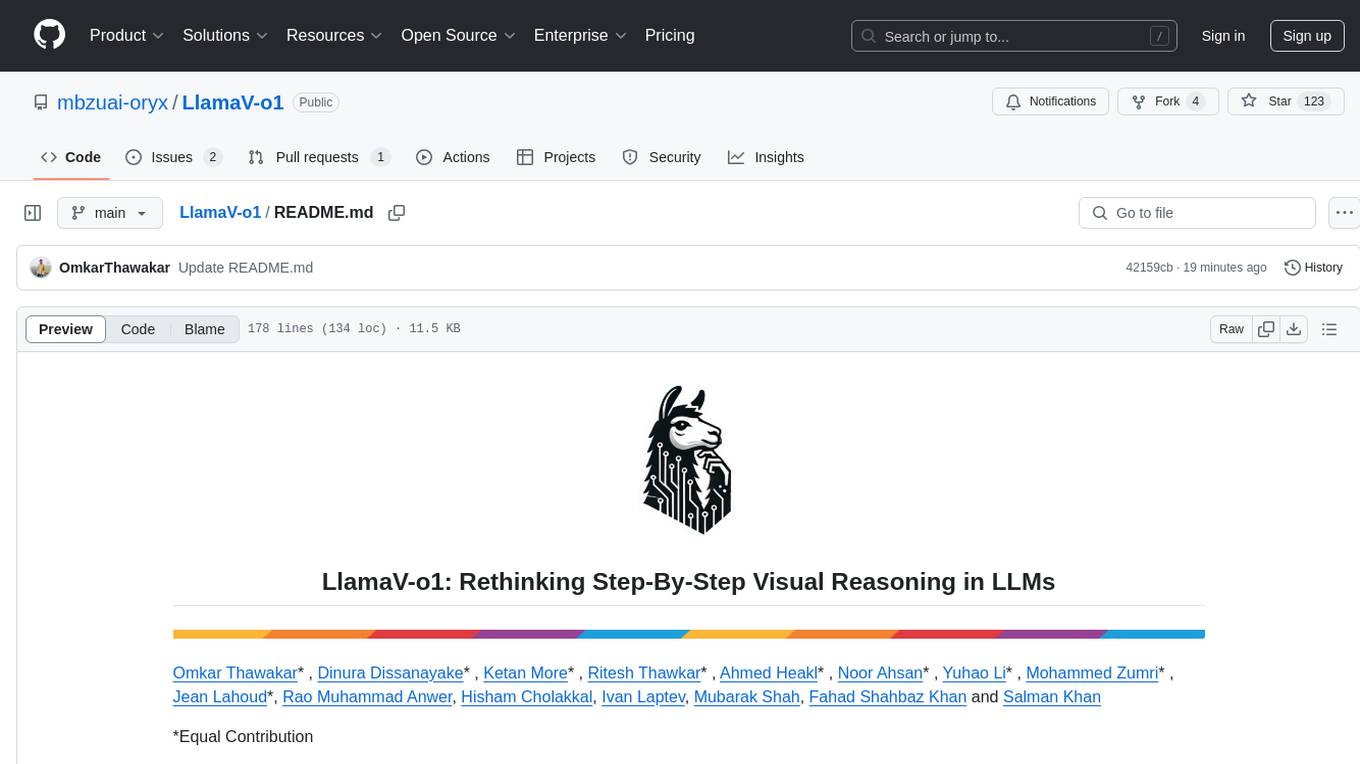
LlamaV-o1
LlamaV-o1 is a Large Multimodal Model designed for spontaneous reasoning tasks. It outperforms various existing models on multimodal reasoning benchmarks. The project includes a Step-by-Step Visual Reasoning Benchmark, a novel evaluation metric, and a combined Multi-Step Curriculum Learning and Beam Search Approach. The model achieves superior performance in complex multi-step visual reasoning tasks in terms of accuracy and efficiency.
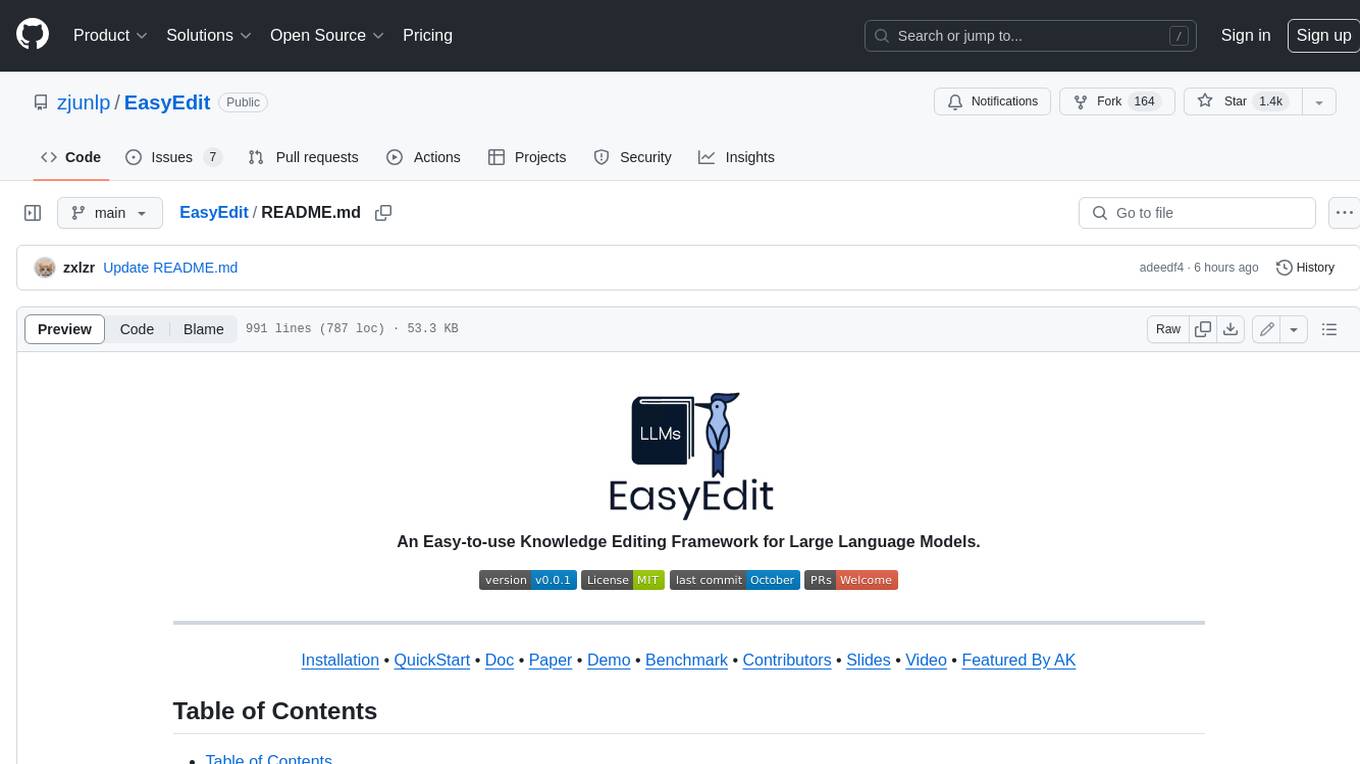
EasyEdit
EasyEdit is a Python package for edit Large Language Models (LLM) like `GPT-J`, `Llama`, `GPT-NEO`, `GPT2`, `T5`(support models from **1B** to **65B**), the objective of which is to alter the behavior of LLMs efficiently within a specific domain without negatively impacting performance across other inputs. It is designed to be easy to use and easy to extend.
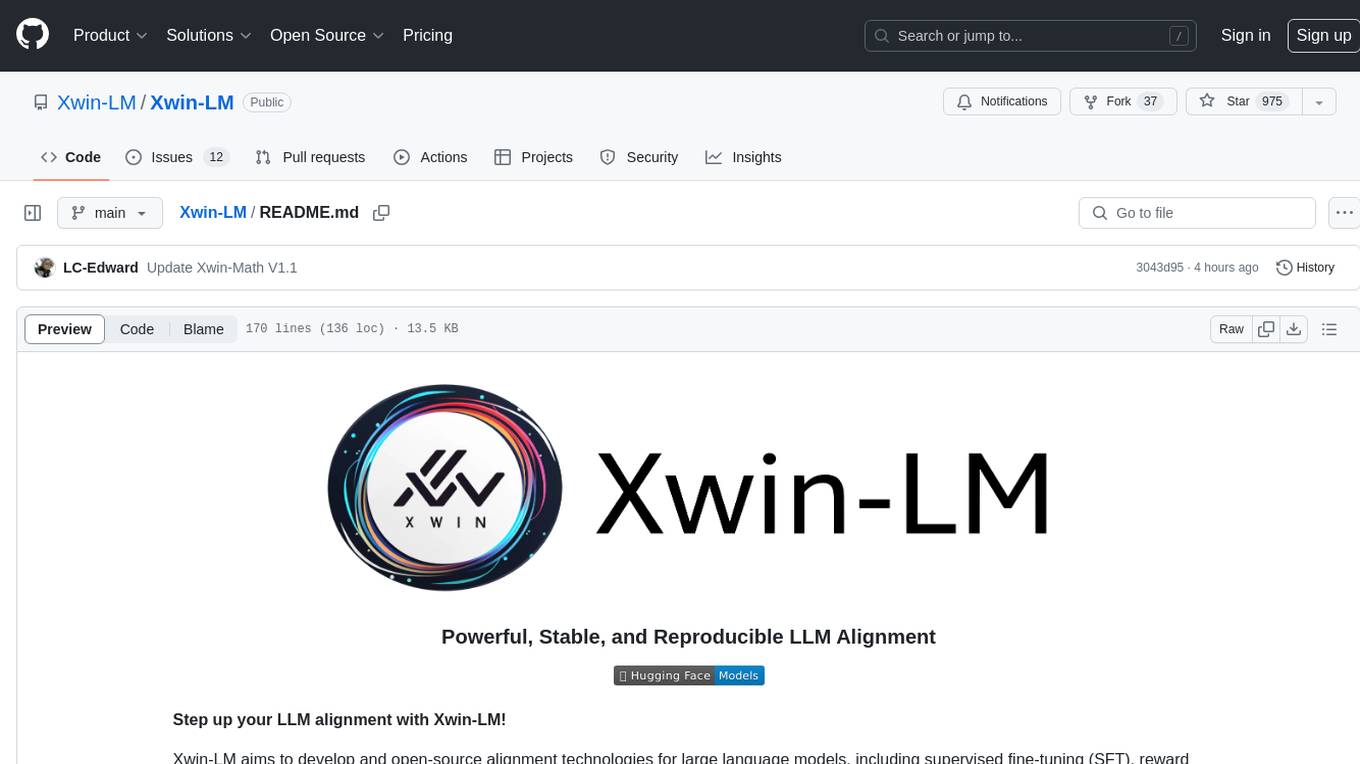
Xwin-LM
Xwin-LM is a powerful and stable open-source tool for aligning large language models, offering various alignment technologies like supervised fine-tuning, reward models, reject sampling, and reinforcement learning from human feedback. It has achieved top rankings in benchmarks like AlpacaEval and surpassed GPT-4. The tool is continuously updated with new models and features.
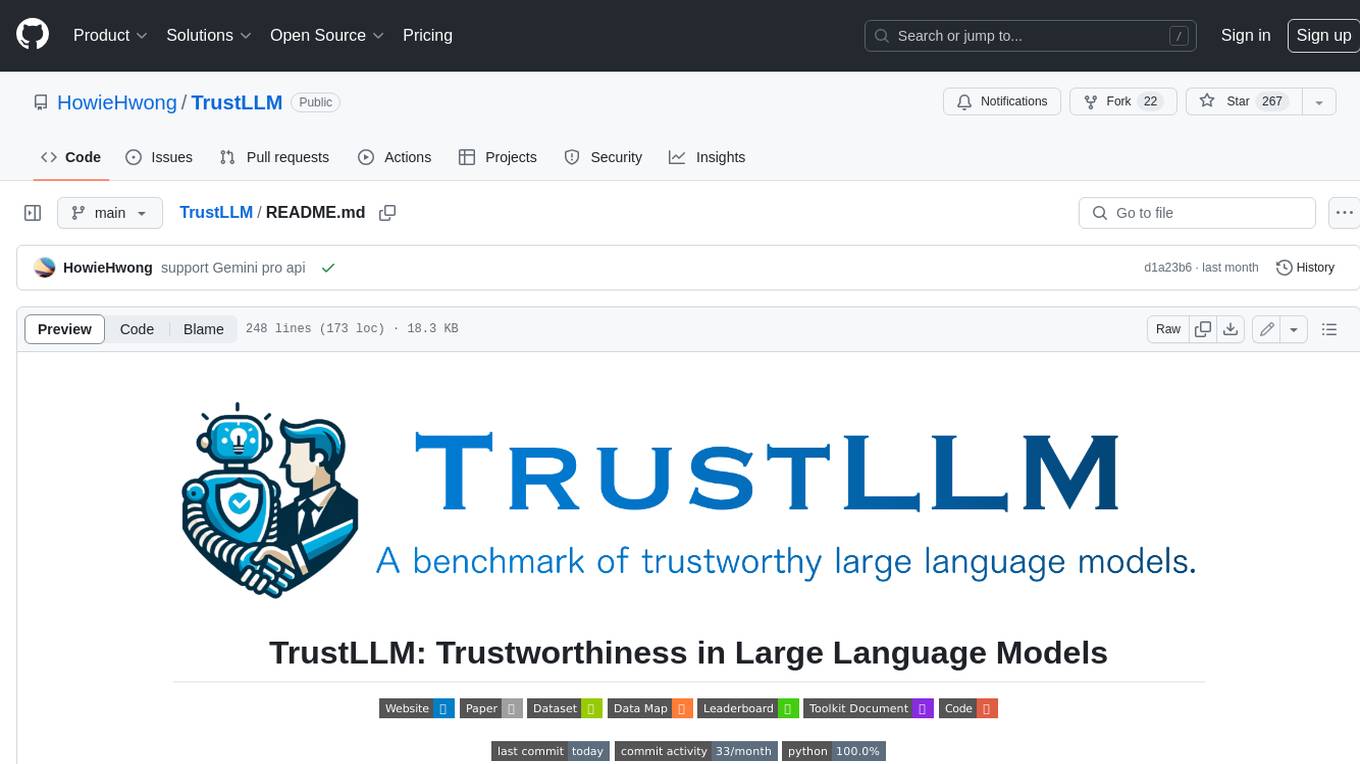
TrustLLM
TrustLLM is a comprehensive study of trustworthiness in LLMs, including principles for different dimensions of trustworthiness, established benchmark, evaluation, and analysis of trustworthiness for mainstream LLMs, and discussion of open challenges and future directions. Specifically, we first propose a set of principles for trustworthy LLMs that span eight different dimensions. Based on these principles, we further establish a benchmark across six dimensions including truthfulness, safety, fairness, robustness, privacy, and machine ethics. We then present a study evaluating 16 mainstream LLMs in TrustLLM, consisting of over 30 datasets. The document explains how to use the trustllm python package to help you assess the performance of your LLM in trustworthiness more quickly. For more details about TrustLLM, please refer to project website.
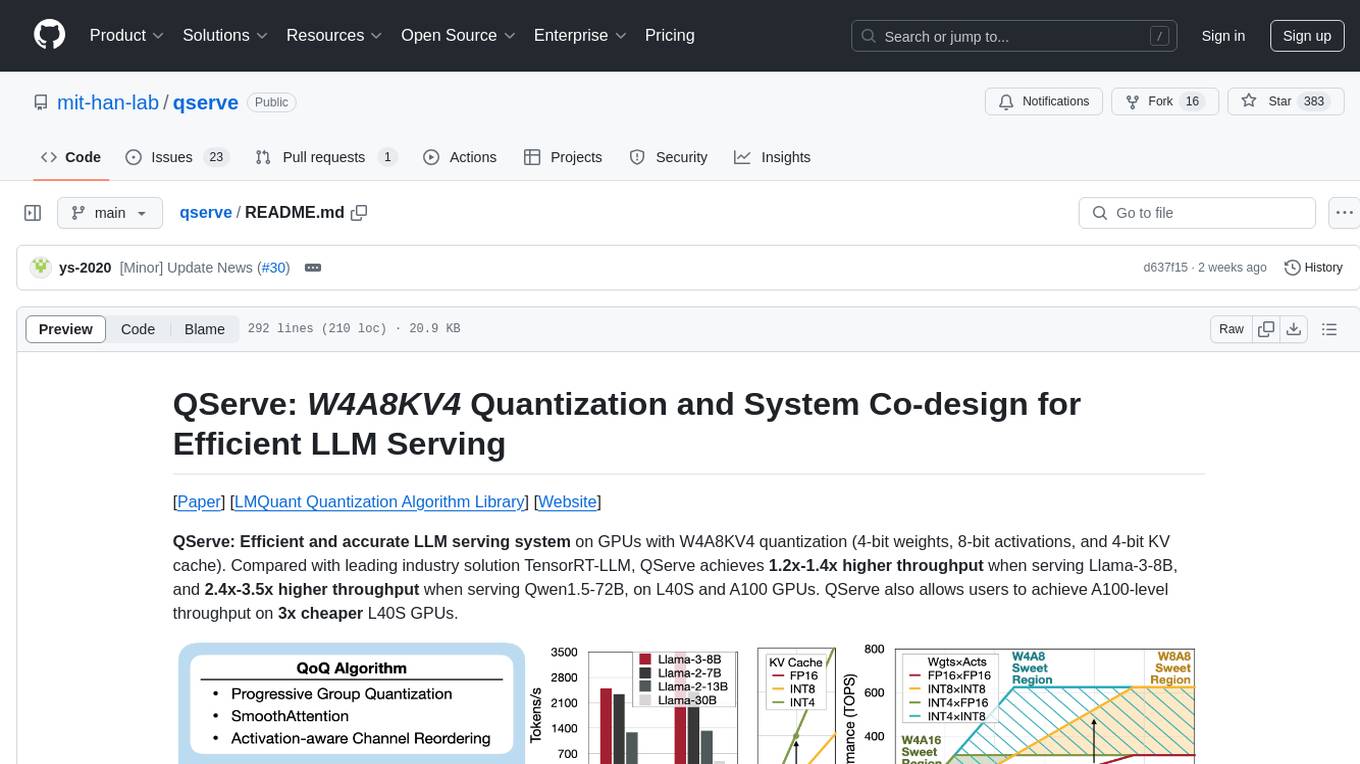
qserve
QServe is a serving system designed for efficient and accurate Large Language Models (LLM) on GPUs with W4A8KV4 quantization. It achieves higher throughput compared to leading industry solutions, allowing users to achieve A100-level throughput on cheaper L40S GPUs. The system introduces the QoQ quantization algorithm with 4-bit weight, 8-bit activation, and 4-bit KV cache, addressing runtime overhead challenges. QServe improves serving throughput for various LLM models by implementing compute-aware weight reordering, register-level parallelism, and fused attention memory-bound techniques.
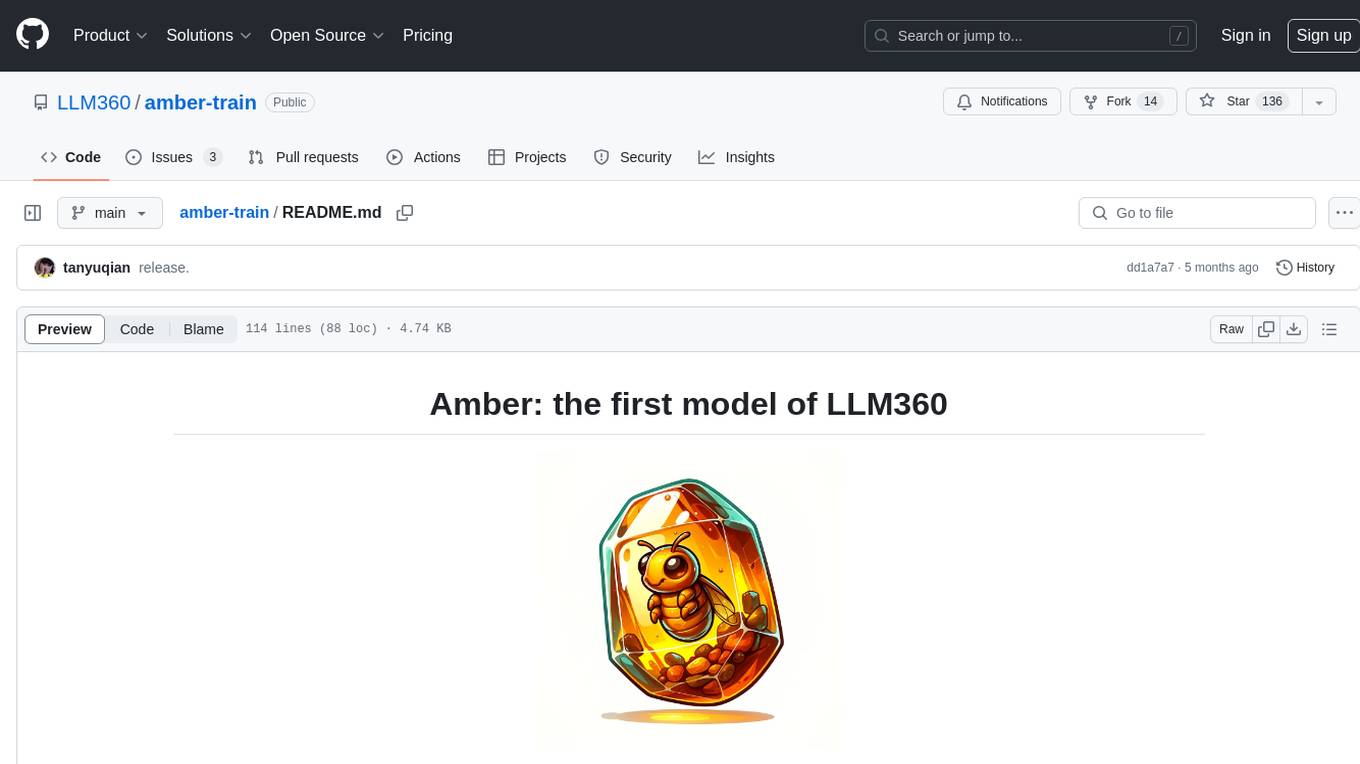
amber-train
Amber is the first model in the LLM360 family, an initiative for comprehensive and fully open-sourced LLMs. It is a 7B English language model with the LLaMA architecture. The model type is a language model with the same architecture as LLaMA-7B. It is licensed under Apache 2.0. The resources available include training code, data preparation, metrics, and fully processed Amber pretraining data. The model has been trained on various datasets like Arxiv, Book, C4, Refined-Web, StarCoder, StackExchange, and Wikipedia. The hyperparameters include a total of 6.7B parameters, hidden size of 4096, intermediate size of 11008, 32 attention heads, 32 hidden layers, RMSNorm ε of 1e^-6, max sequence length of 2048, and a vocabulary size of 32000.
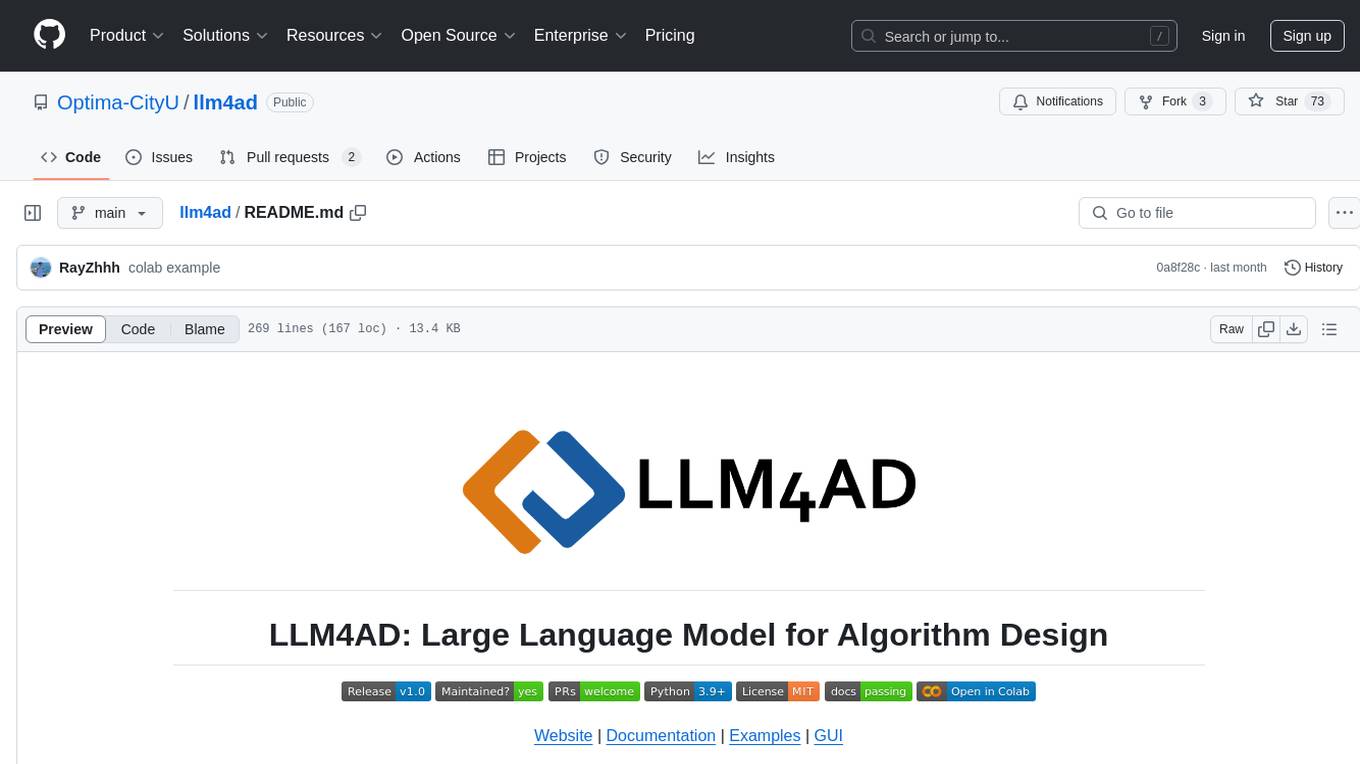
llm4ad
LLM4AD is an open-source Python-based platform leveraging Large Language Models (LLMs) for Automatic Algorithm Design (AD). It provides unified interfaces for methods, tasks, and LLMs, along with features like evaluation acceleration, secure evaluation, logs, GUI support, and more. The platform was originally developed for optimization tasks but is versatile enough to be used in other areas such as machine learning, science discovery, game theory, and engineering design. It offers various search methods and algorithm design tasks across different domains. LLM4AD supports remote LLM API, local HuggingFace LLM deployment, and custom LLM interfaces. The project is licensed under the MIT License and welcomes contributions, collaborations, and issue reports.
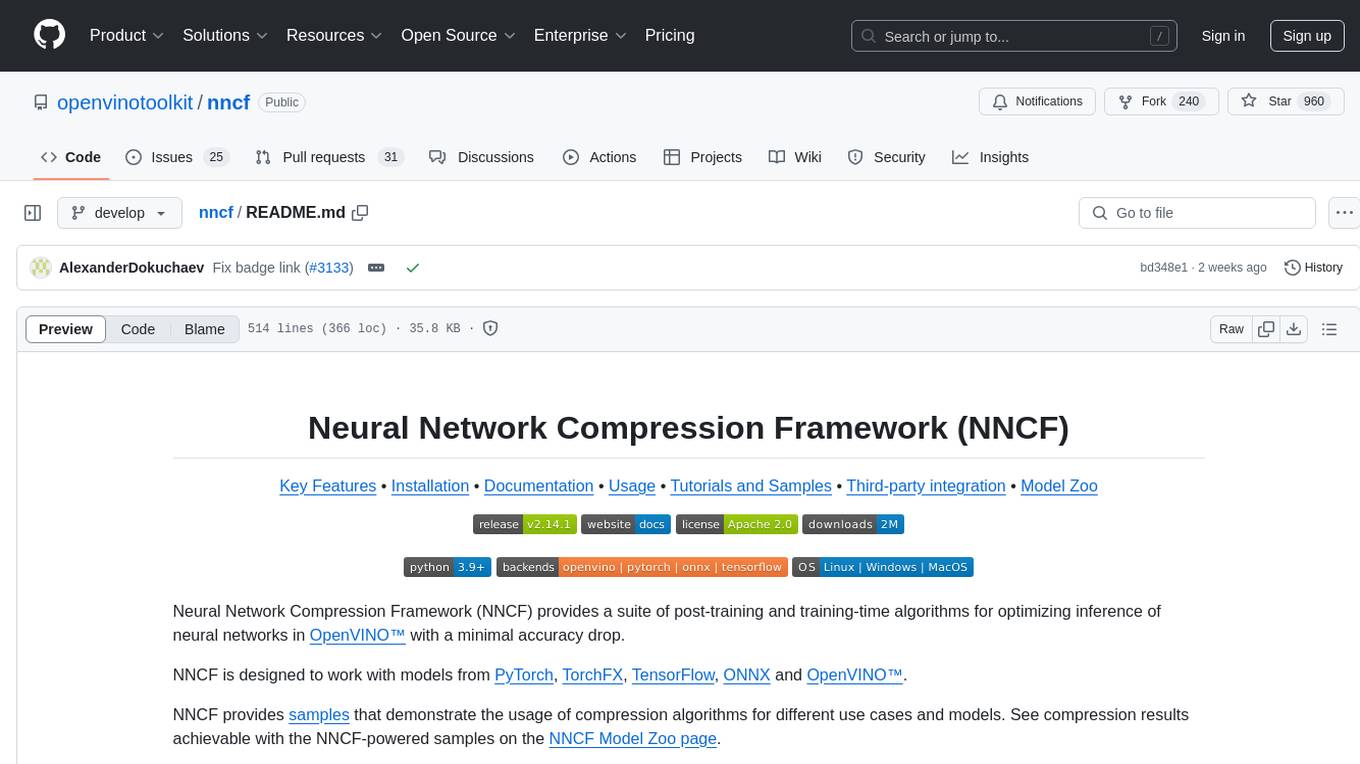
nncf
Neural Network Compression Framework (NNCF) provides a suite of post-training and training-time algorithms for optimizing inference of neural networks in OpenVINO™ with a minimal accuracy drop. It is designed to work with models from PyTorch, TorchFX, TensorFlow, ONNX, and OpenVINO™. NNCF offers samples demonstrating compression algorithms for various use cases and models, with the ability to add different compression algorithms easily. It supports GPU-accelerated layers, distributed training, and seamless combination of pruning, sparsity, and quantization algorithms. NNCF allows exporting compressed models to ONNX or TensorFlow formats for use with OpenVINO™ toolkit, and supports Accuracy-Aware model training pipelines via Adaptive Compression Level Training and Early Exit Training.
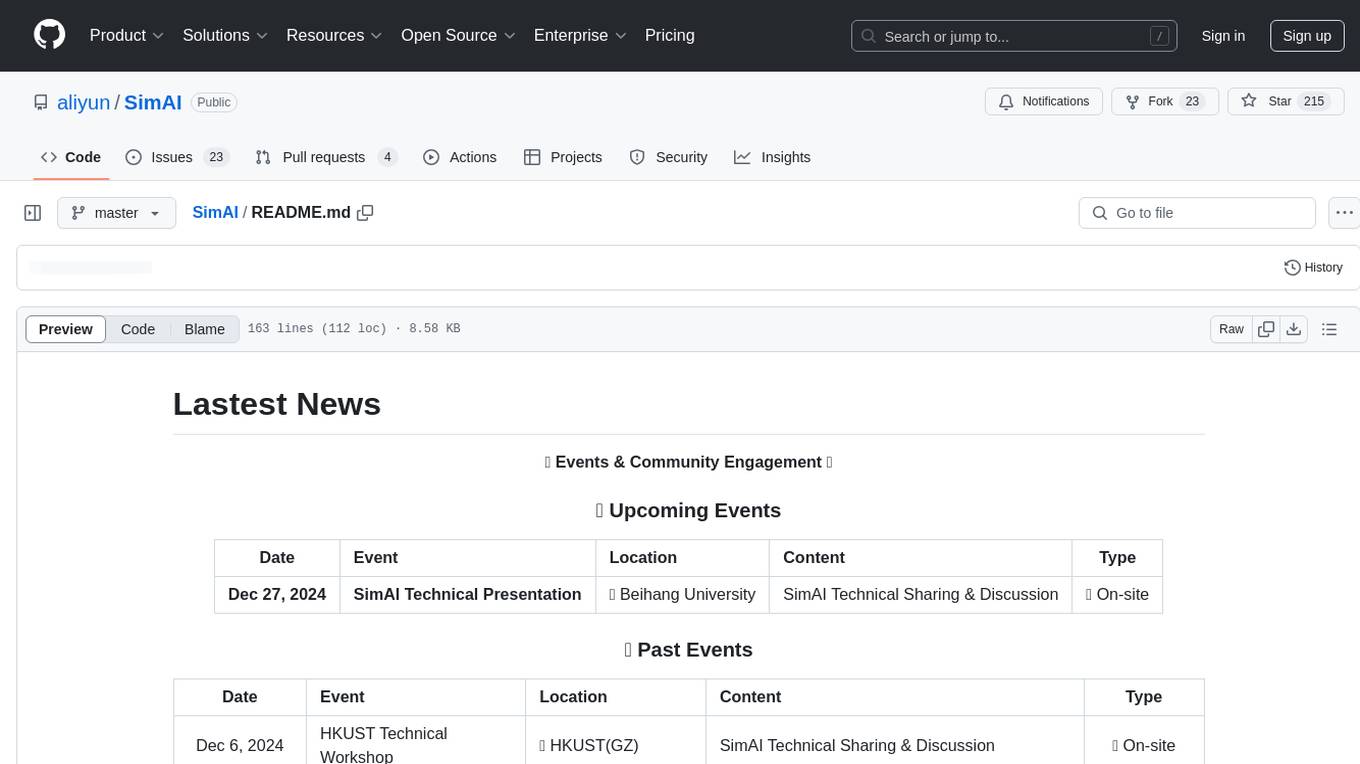
SimAI
SimAI is the industry's first full-stack, high-precision simulator for AI large-scale training. It provides detailed modeling and simulation of the entire LLM training process, encompassing framework, collective communication, network layers, and more. This comprehensive approach offers end-to-end performance data, enabling researchers to analyze training process details, evaluate time consumption of AI tasks under specific conditions, and assess performance gains from various algorithmic optimizations.
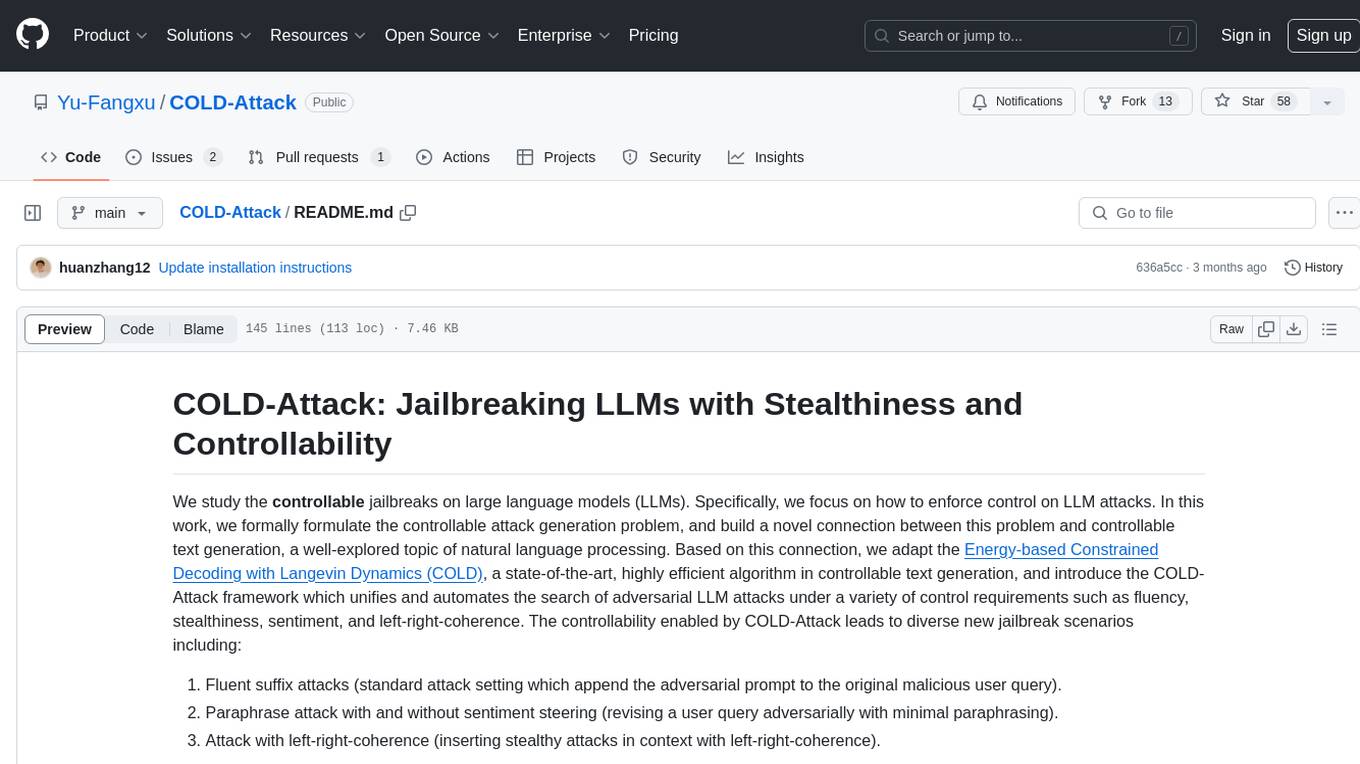
COLD-Attack
COLD-Attack is a framework designed for controllable jailbreaks on large language models (LLMs). It formulates the controllable attack generation problem and utilizes the Energy-based Constrained Decoding with Langevin Dynamics (COLD) algorithm to automate the search of adversarial LLM attacks with control over fluency, stealthiness, sentiment, and left-right-coherence. The framework includes steps for energy function formulation, Langevin dynamics sampling, and decoding process to generate discrete text attacks. It offers diverse jailbreak scenarios such as fluent suffix attacks, paraphrase attacks, and attacks with left-right-coherence.
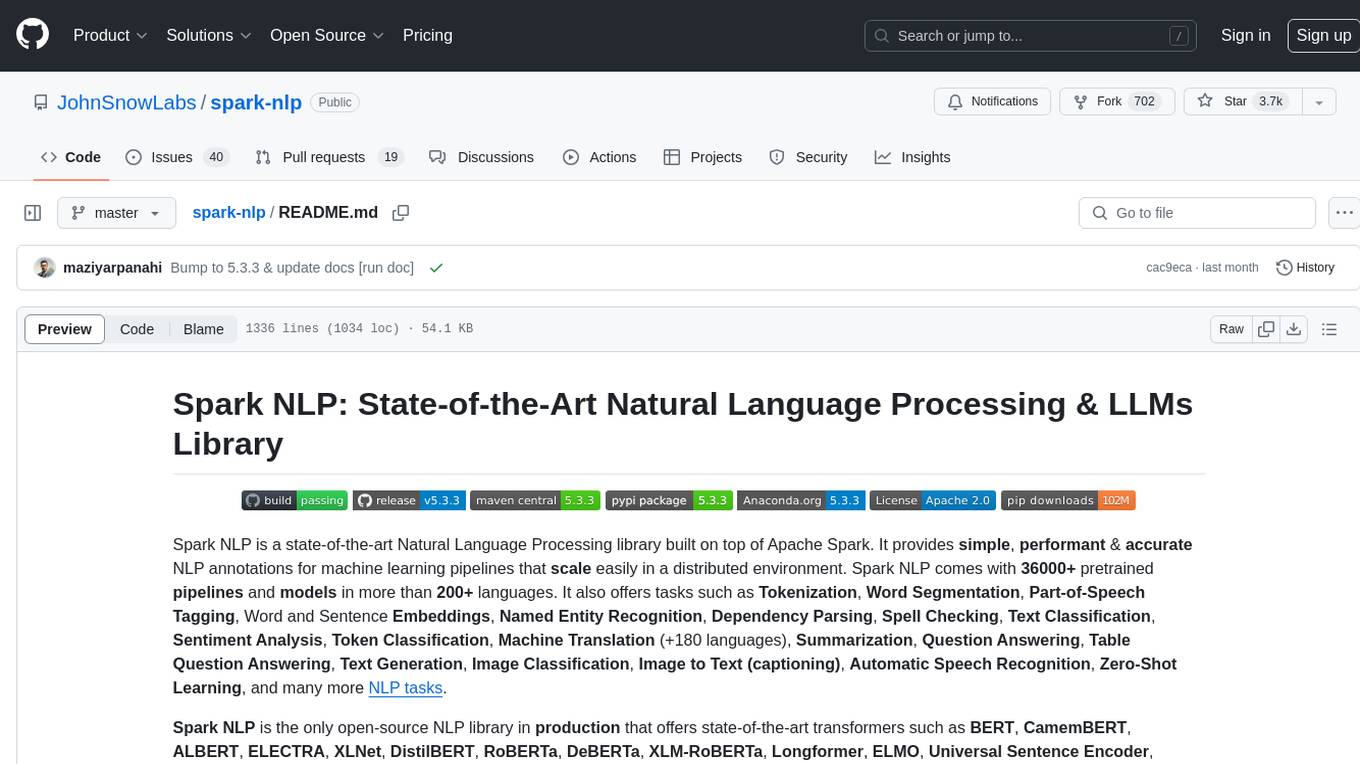
spark-nlp
Spark NLP is a state-of-the-art Natural Language Processing library built on top of Apache Spark. It provides simple, performant, and accurate NLP annotations for machine learning pipelines that scale easily in a distributed environment. Spark NLP comes with 36000+ pretrained pipelines and models in more than 200+ languages. It offers tasks such as Tokenization, Word Segmentation, Part-of-Speech Tagging, Named Entity Recognition, Dependency Parsing, Spell Checking, Text Classification, Sentiment Analysis, Token Classification, Machine Translation, Summarization, Question Answering, Table Question Answering, Text Generation, Image Classification, Image to Text (captioning), Automatic Speech Recognition, Zero-Shot Learning, and many more NLP tasks. Spark NLP is the only open-source NLP library in production that offers state-of-the-art transformers such as BERT, CamemBERT, ALBERT, ELECTRA, XLNet, DistilBERT, RoBERTa, DeBERTa, XLM-RoBERTa, Longformer, ELMO, Universal Sentence Encoder, Llama-2, M2M100, BART, Instructor, E5, Google T5, MarianMT, OpenAI GPT2, Vision Transformers (ViT), OpenAI Whisper, and many more not only to Python and R, but also to JVM ecosystem (Java, Scala, and Kotlin) at scale by extending Apache Spark natively.
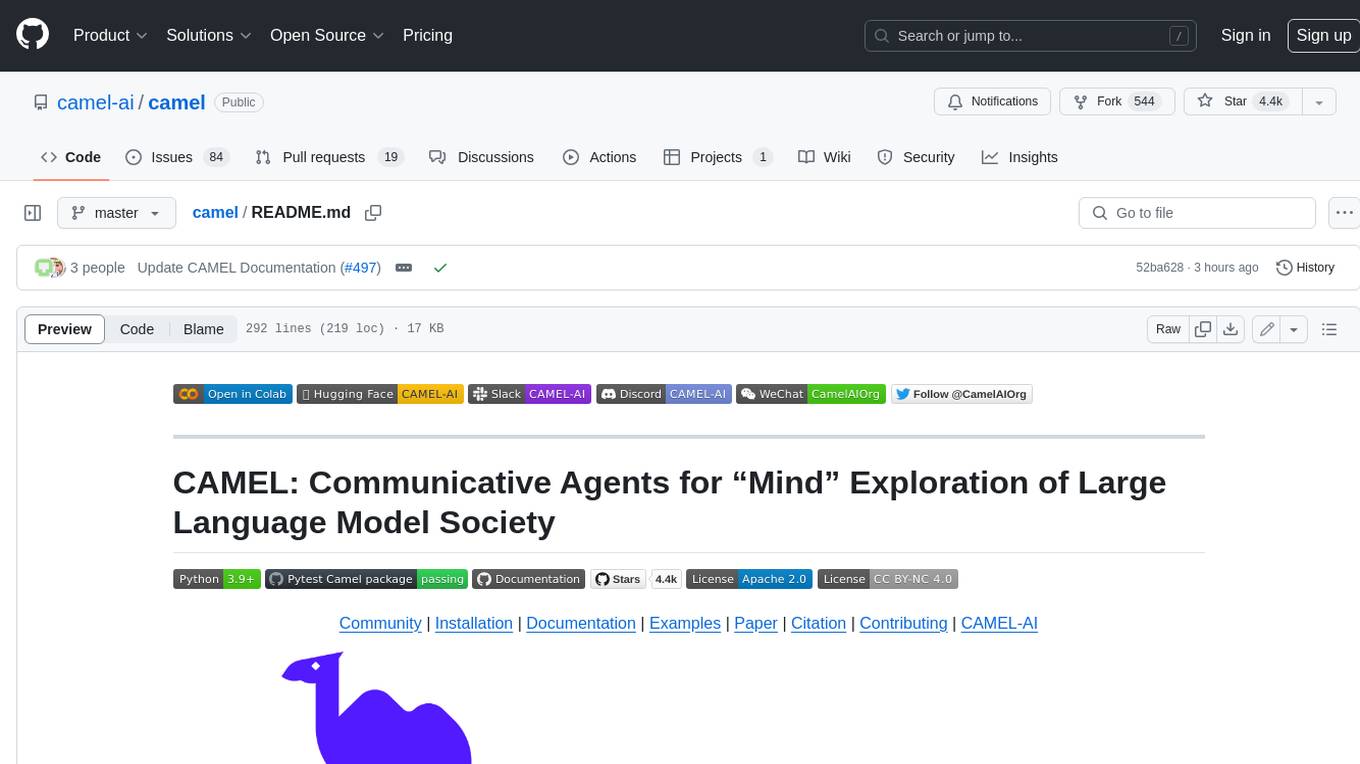
camel
CAMEL is an open-source library designed for the study of autonomous and communicative agents. We believe that studying these agents on a large scale offers valuable insights into their behaviors, capabilities, and potential risks. To facilitate research in this field, we implement and support various types of agents, tasks, prompts, models, and simulated environments.
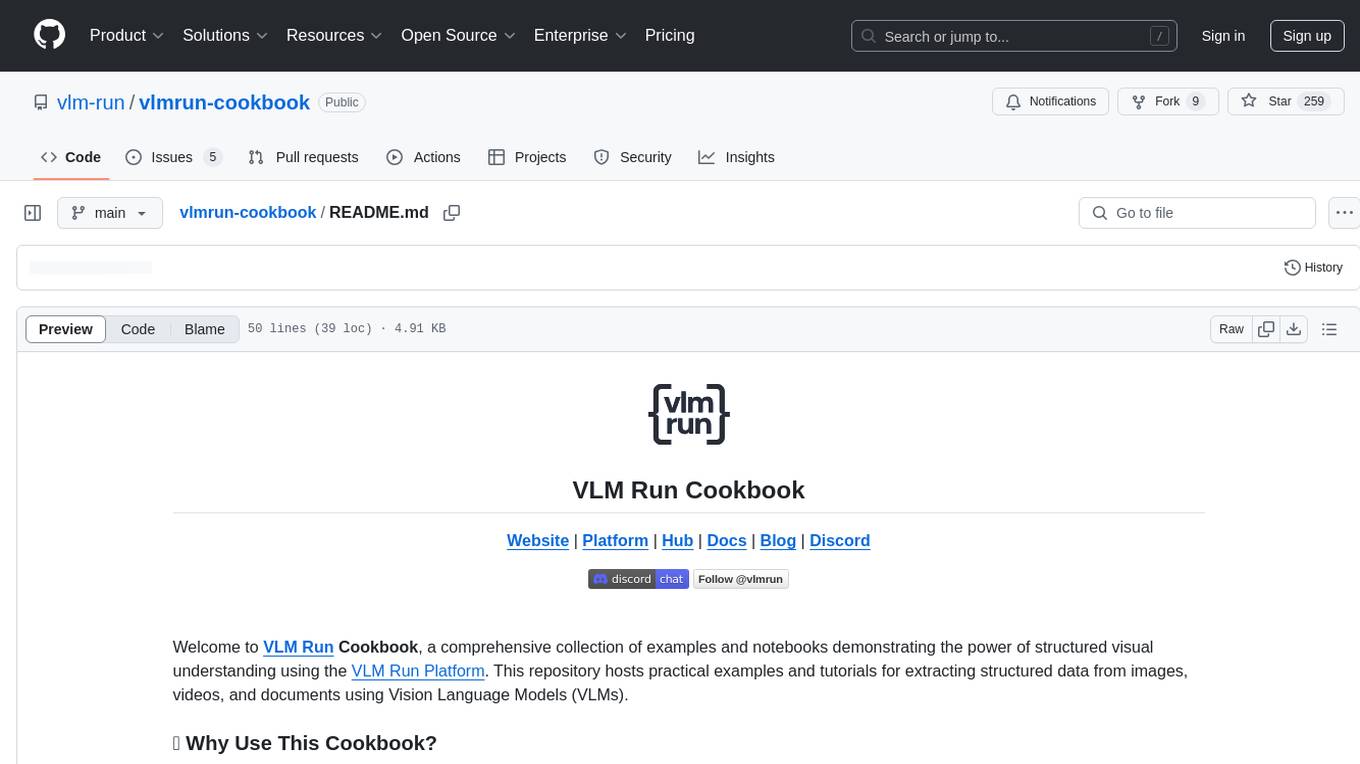
vlmrun-cookbook
VLM Run Cookbook is a repository containing practical examples and tutorials for extracting structured data from images, videos, and documents using Vision Language Models (VLMs). It offers comprehensive Colab notebooks demonstrating real-world applications of VLM Run, with complete code and documentation for easy adaptation. The examples cover various domains such as financial documents and TV news analysis.
For similar tasks
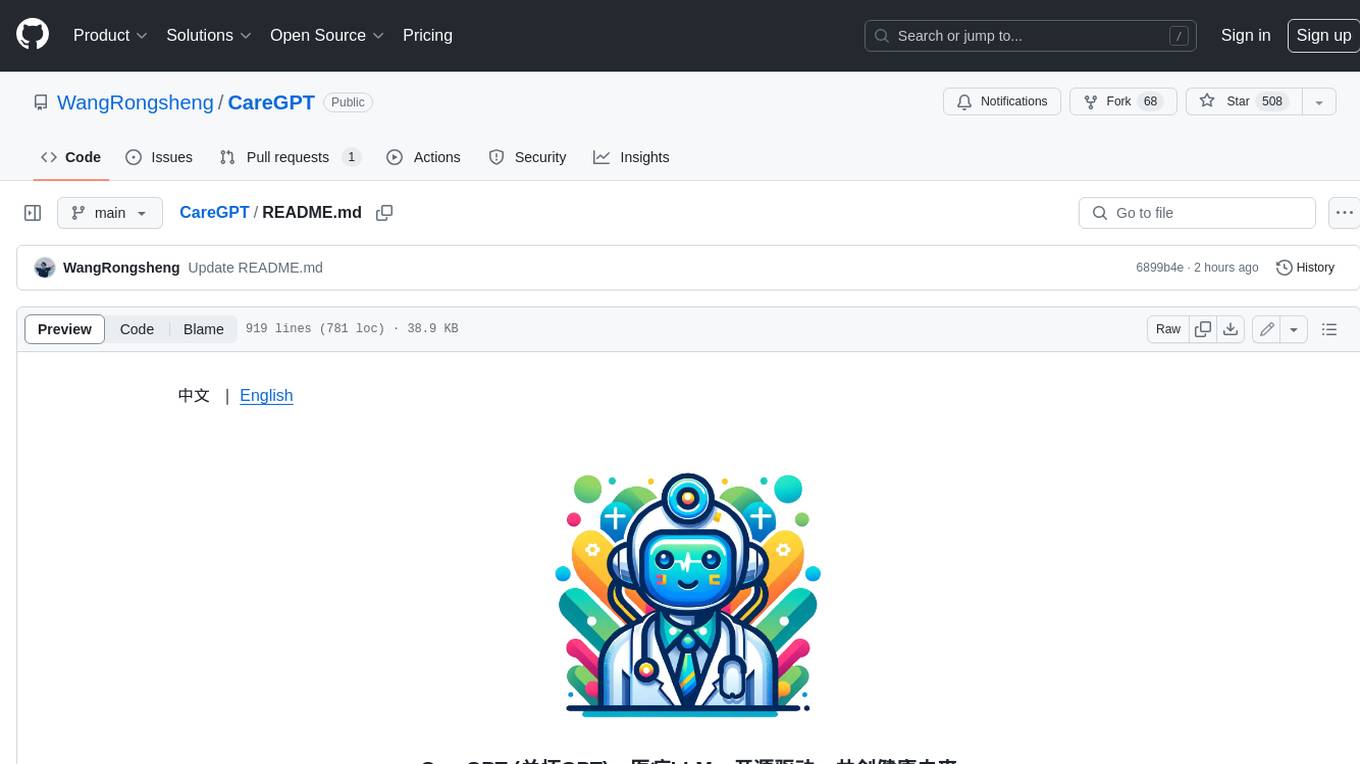
CareGPT
CareGPT is a medical large language model (LLM) that explores medical data, training, and deployment related research work. It integrates resources, open-source models, rich data, and efficient deployment methods. It supports various medical tasks, including patient diagnosis, medical dialogue, and medical knowledge integration. The model has been fine-tuned on diverse medical datasets to enhance its performance in the healthcare domain.
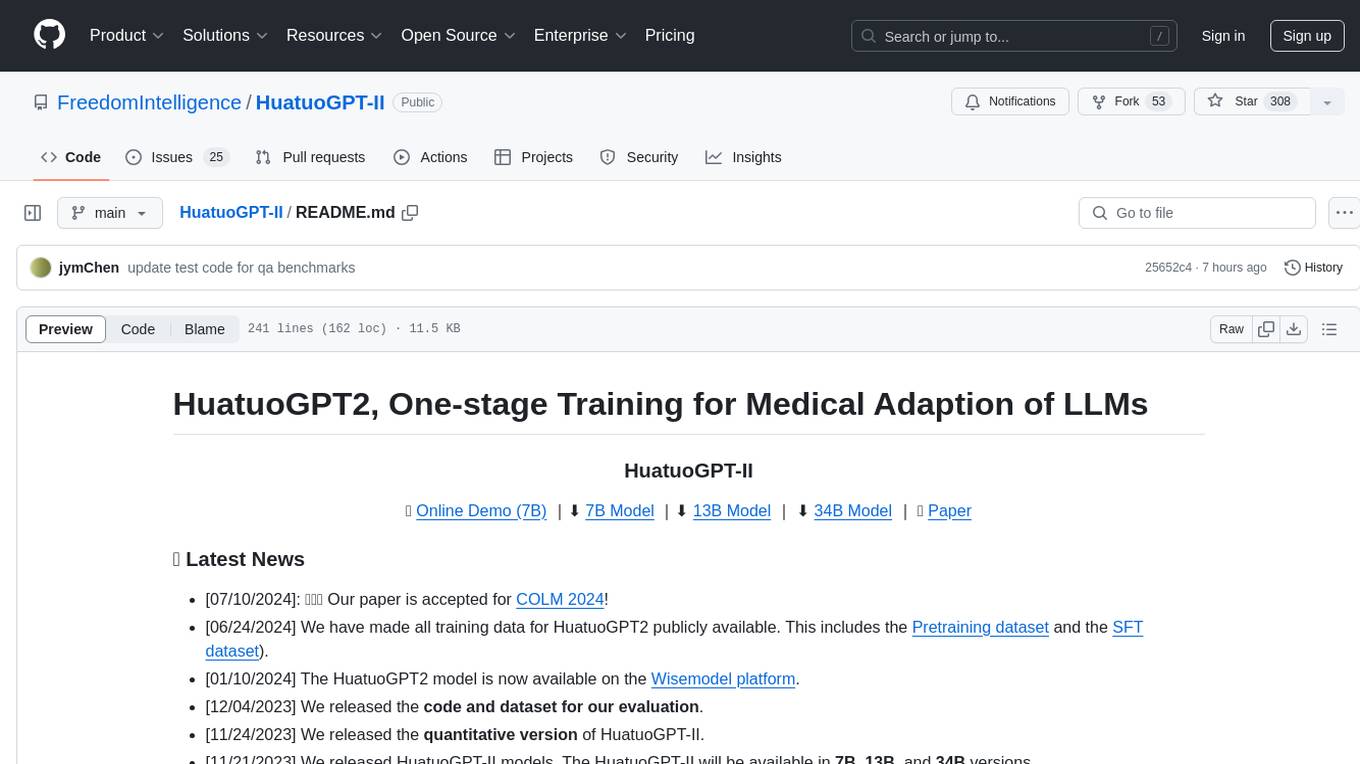
HuatuoGPT-II
HuatuoGPT2 is an innovative domain-adapted medical large language model that excels in medical knowledge and dialogue proficiency. It showcases state-of-the-art performance in various medical benchmarks, surpassing GPT-4 in expert evaluations and fresh medical licensing exams. The open-source release includes HuatuoGPT2 models in 7B, 13B, and 34B versions, training code for one-stage adaptation, partial pre-training and fine-tuning instructions, and evaluation methods for medical response capabilities and professional pharmacist exams. The tool aims to enhance LLM capabilities in the Chinese medical field through open-source principles.
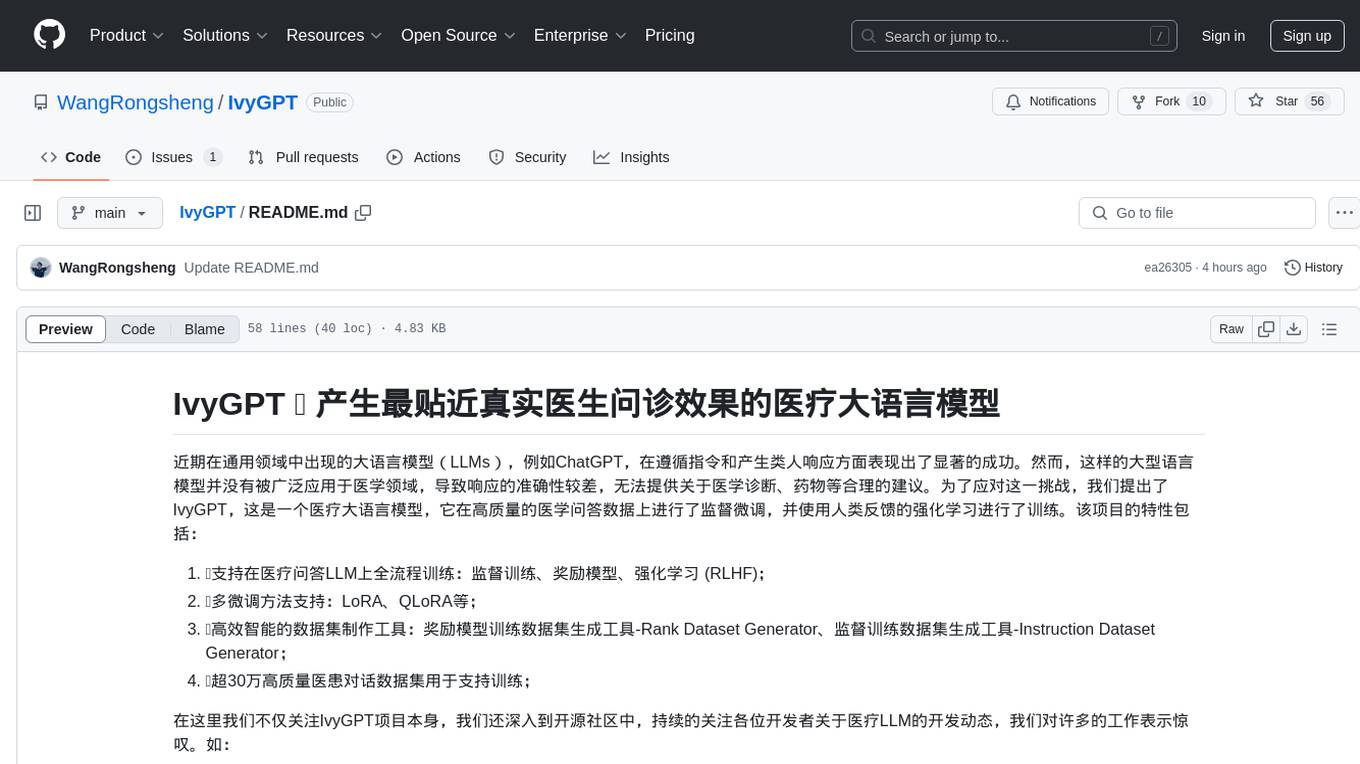
IvyGPT
IvyGPT is a medical large language model that aims to generate the most realistic doctor consultation effects. It has been fine-tuned on high-quality medical Q&A data and trained using human feedback reinforcement learning. The project features full-process training on medical Q&A LLM, multiple fine-tuning methods support, efficient dataset creation tools, and a dataset of over 300,000 high-quality doctor-patient dialogues for training.
For similar jobs
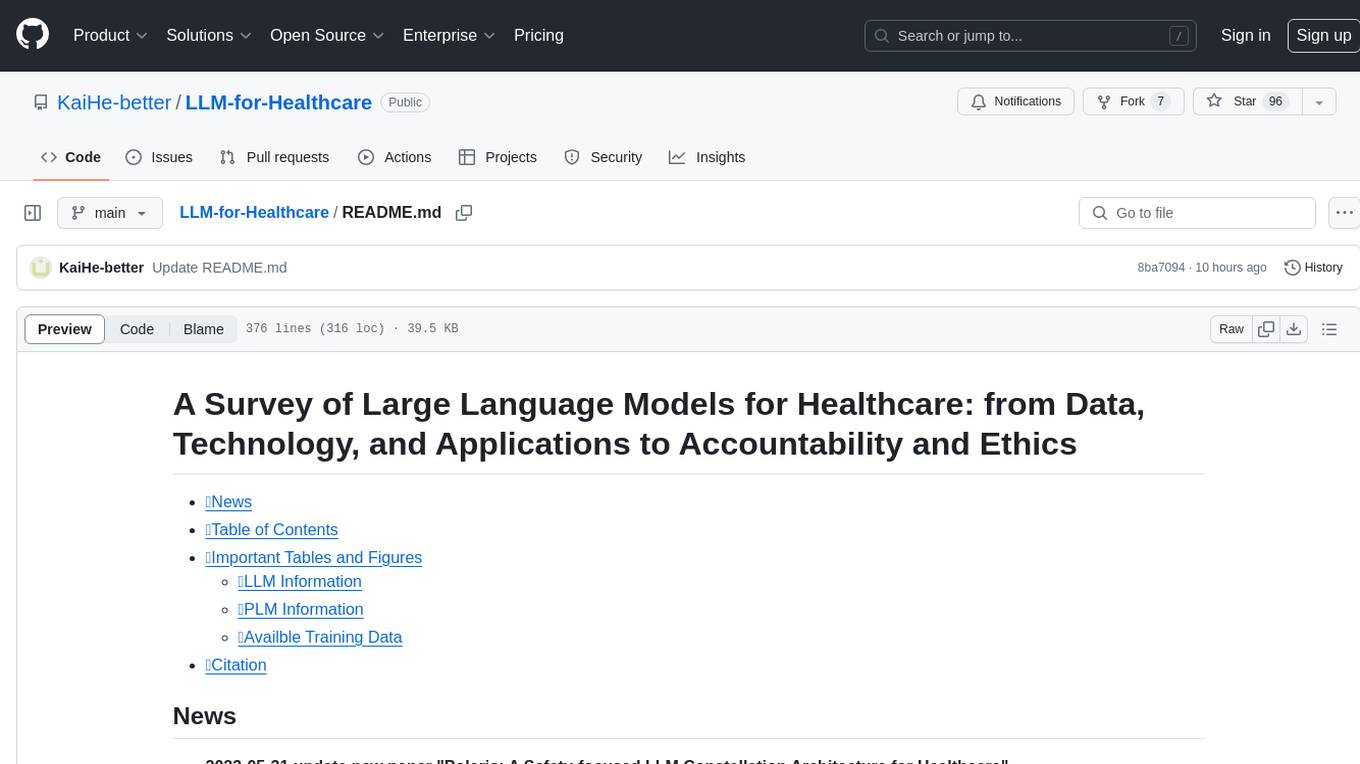
LLM-for-Healthcare
The repository 'LLM-for-Healthcare' provides a comprehensive survey of large language models (LLMs) for healthcare, covering data, technology, applications, and accountability and ethics. It includes information on various LLM models, training data, evaluation methods, and computation costs. The repository also discusses tasks such as NER, text classification, question answering, dialogue systems, and generation of medical reports from images in the healthcare domain.
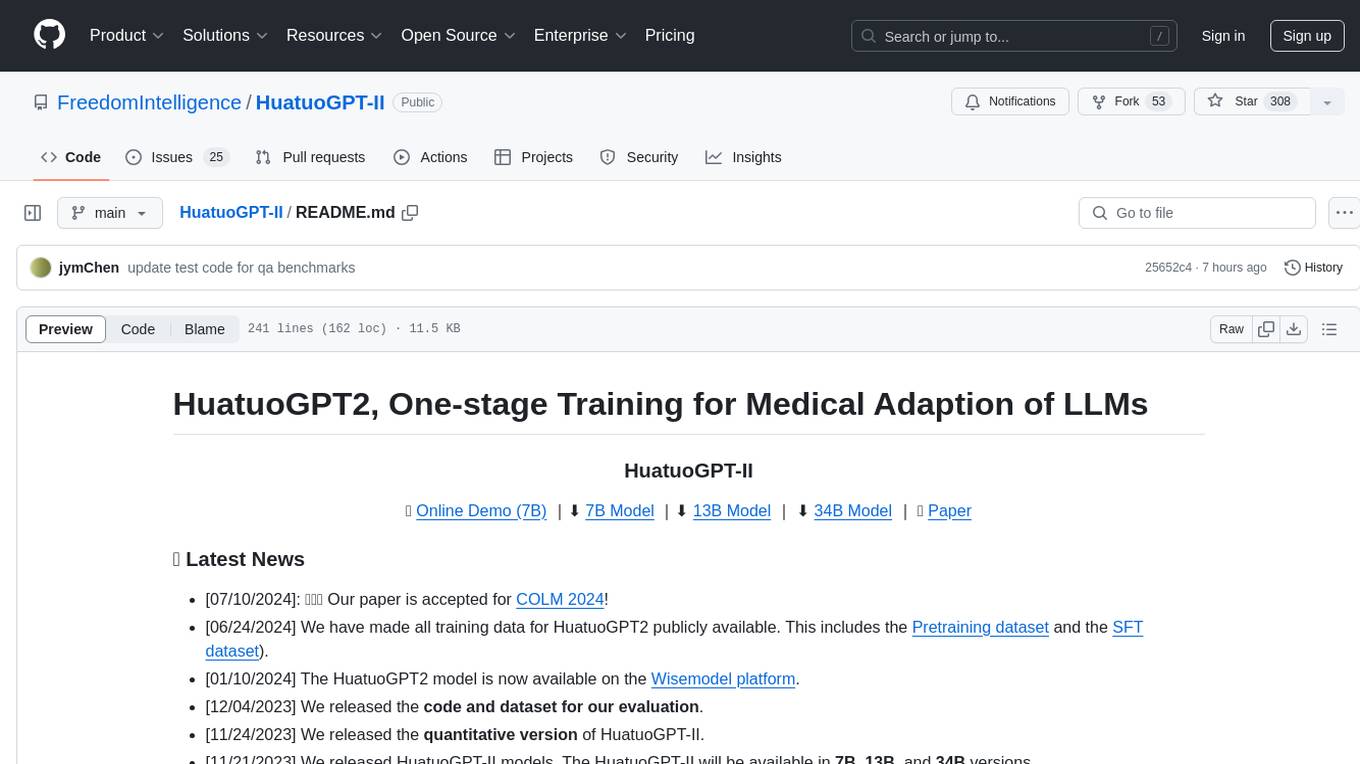
HuatuoGPT-II
HuatuoGPT2 is an innovative domain-adapted medical large language model that excels in medical knowledge and dialogue proficiency. It showcases state-of-the-art performance in various medical benchmarks, surpassing GPT-4 in expert evaluations and fresh medical licensing exams. The open-source release includes HuatuoGPT2 models in 7B, 13B, and 34B versions, training code for one-stage adaptation, partial pre-training and fine-tuning instructions, and evaluation methods for medical response capabilities and professional pharmacist exams. The tool aims to enhance LLM capabilities in the Chinese medical field through open-source principles.
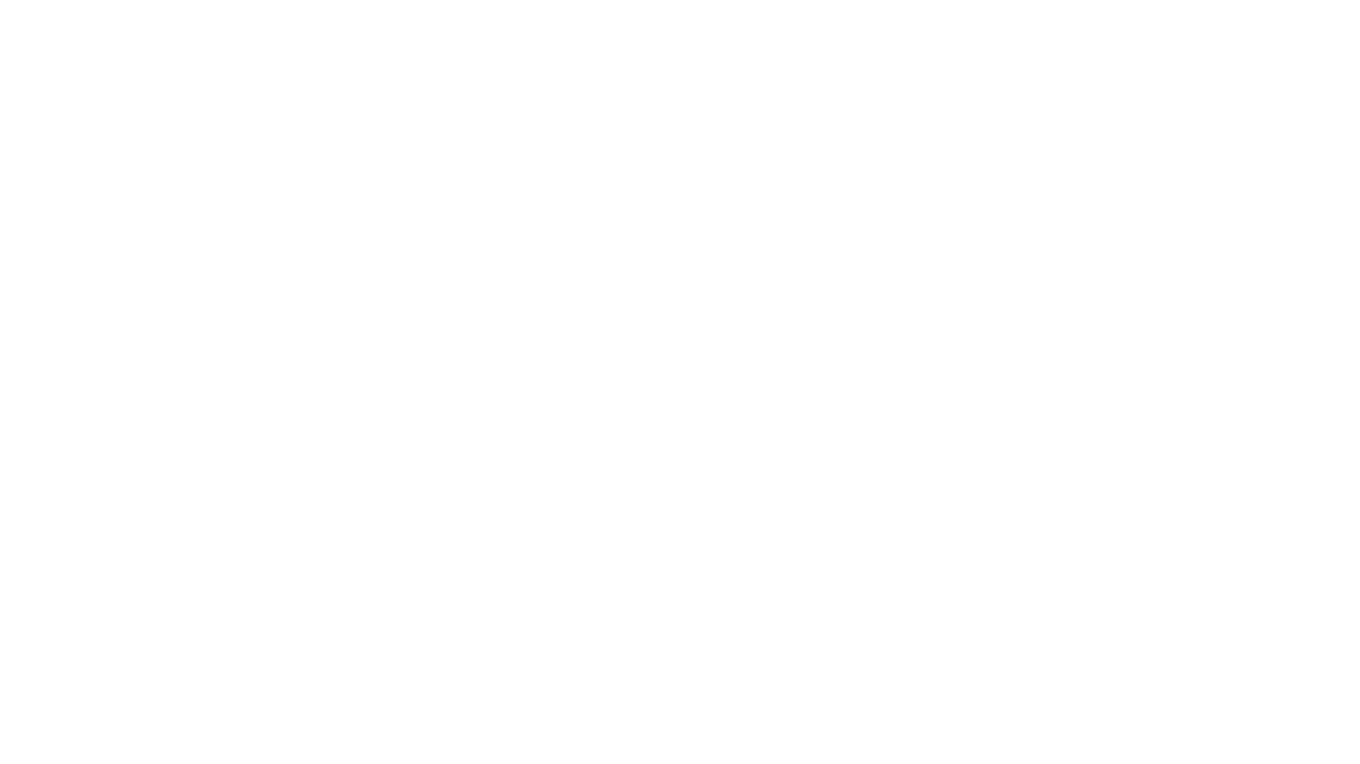
Me-LLaMA
Me LLaMA introduces a suite of open-source medical Large Language Models (LLMs), including Me LLaMA 13B/70B and their chat-enhanced versions. Developed through innovative continual pre-training and instruction tuning, these models leverage a vast medical corpus comprising PubMed papers, medical guidelines, and general domain data. Me LLaMA sets new benchmarks on medical reasoning tasks, making it a significant asset for medical NLP applications and research. The models are intended for computational linguistics and medical research, not for clinical decision-making without validation and regulatory approval.
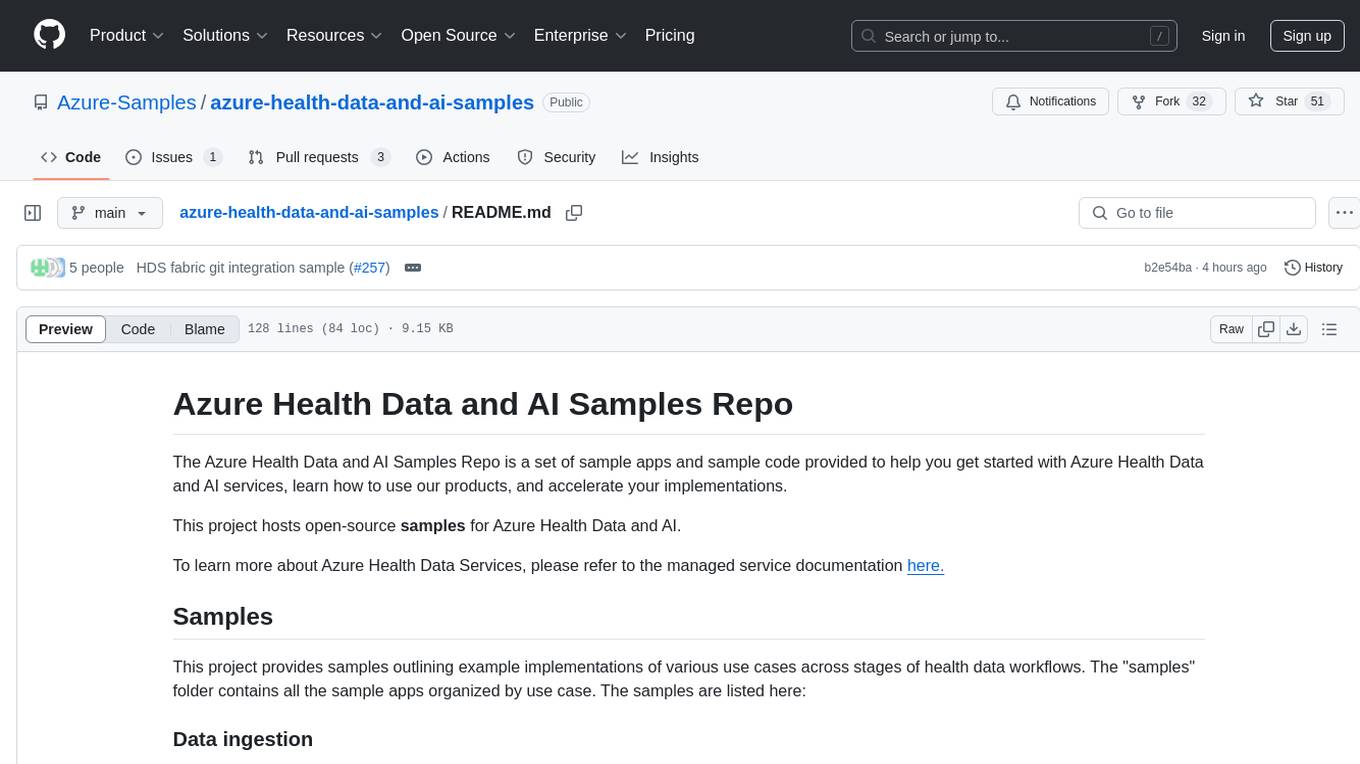
azure-health-data-and-ai-samples
The Azure Health Data and AI Samples Repo is a collection of sample apps and code to help users start with Azure Health Data and AI services, learn product usage, and speed up implementations. It includes samples for various health data workflows, such as data ingestion, analytics, machine learning, SMART on FHIR, patient services, FHIR service integration, Azure AD B2C access, DICOM service, MedTech service, and healthcare data solutions in Microsoft Fabric. These samples are simplified scenarios for testing purposes only.
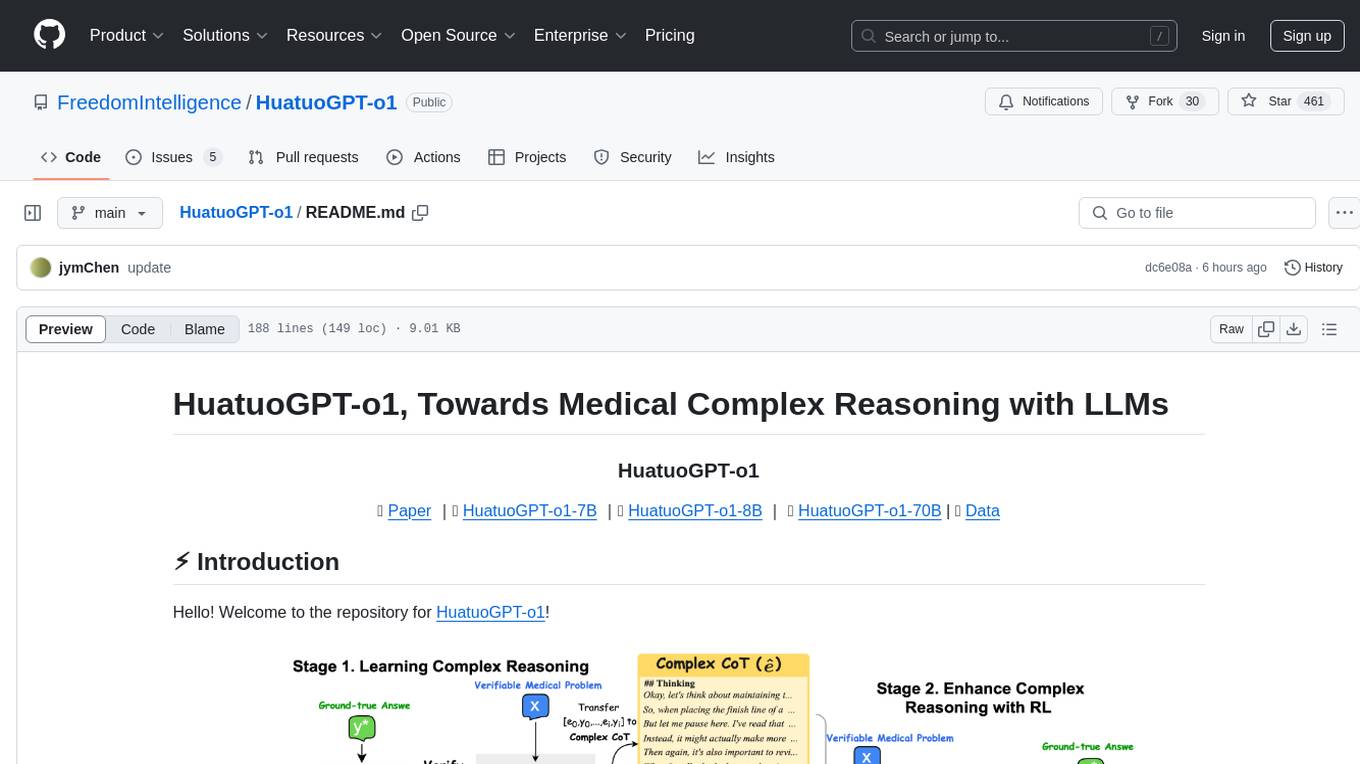
HuatuoGPT-o1
HuatuoGPT-o1 is a medical language model designed for advanced medical reasoning. It can identify mistakes, explore alternative strategies, and refine answers. The model leverages verifiable medical problems and a specialized medical verifier to guide complex reasoning trajectories and enhance reasoning through reinforcement learning. The repository provides access to models, data, and code for HuatuoGPT-o1, allowing users to deploy the model for medical reasoning tasks.
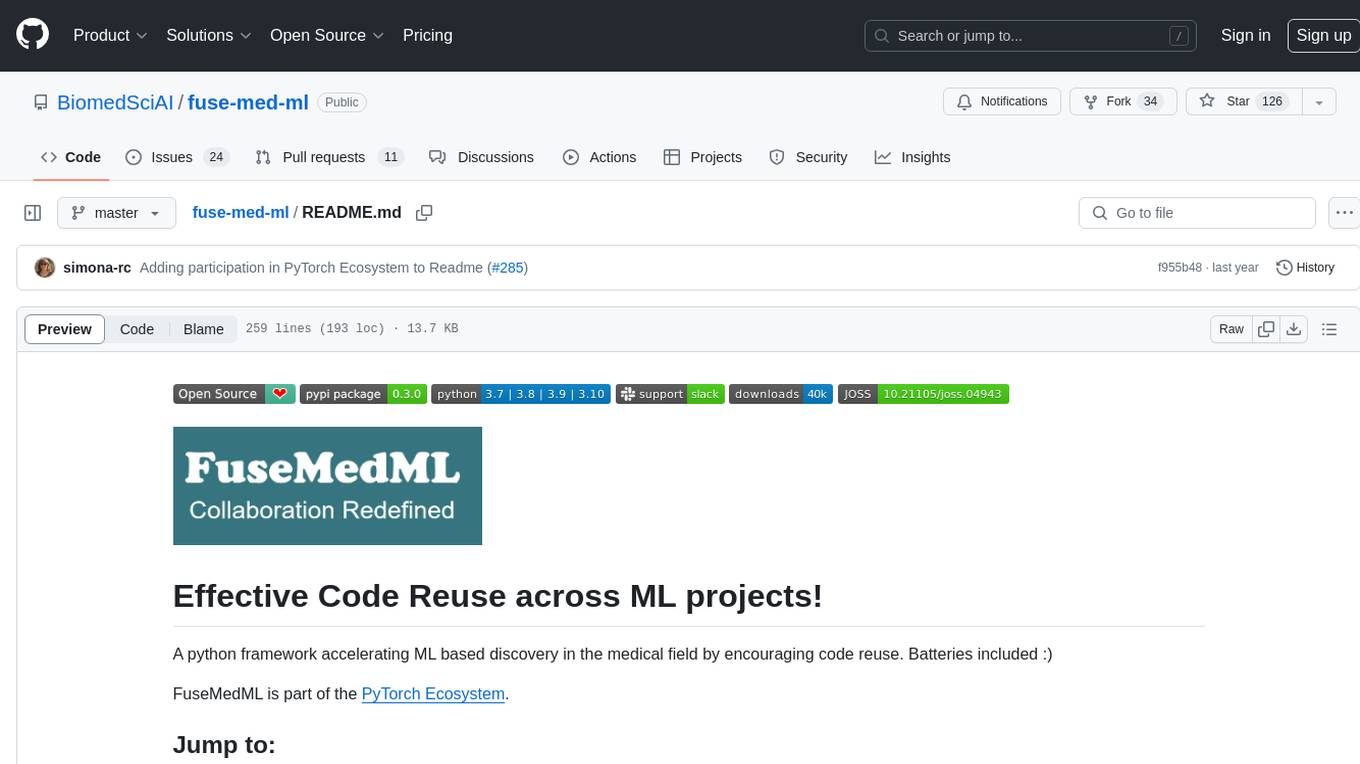
fuse-med-ml
FuseMedML is a Python framework designed to accelerate machine learning-based discovery in the medical field by promoting code reuse. It provides a flexible design concept where data is stored in a nested dictionary, allowing easy handling of multi-modality information. The framework includes components for creating custom models, loss functions, metrics, and data processing operators. Additionally, FuseMedML offers 'batteries included' key components such as fuse.data for data processing, fuse.eval for model evaluation, and fuse.dl for reusable deep learning components. It supports PyTorch and PyTorch Lightning libraries and encourages the creation of domain extensions for specific medical domains.
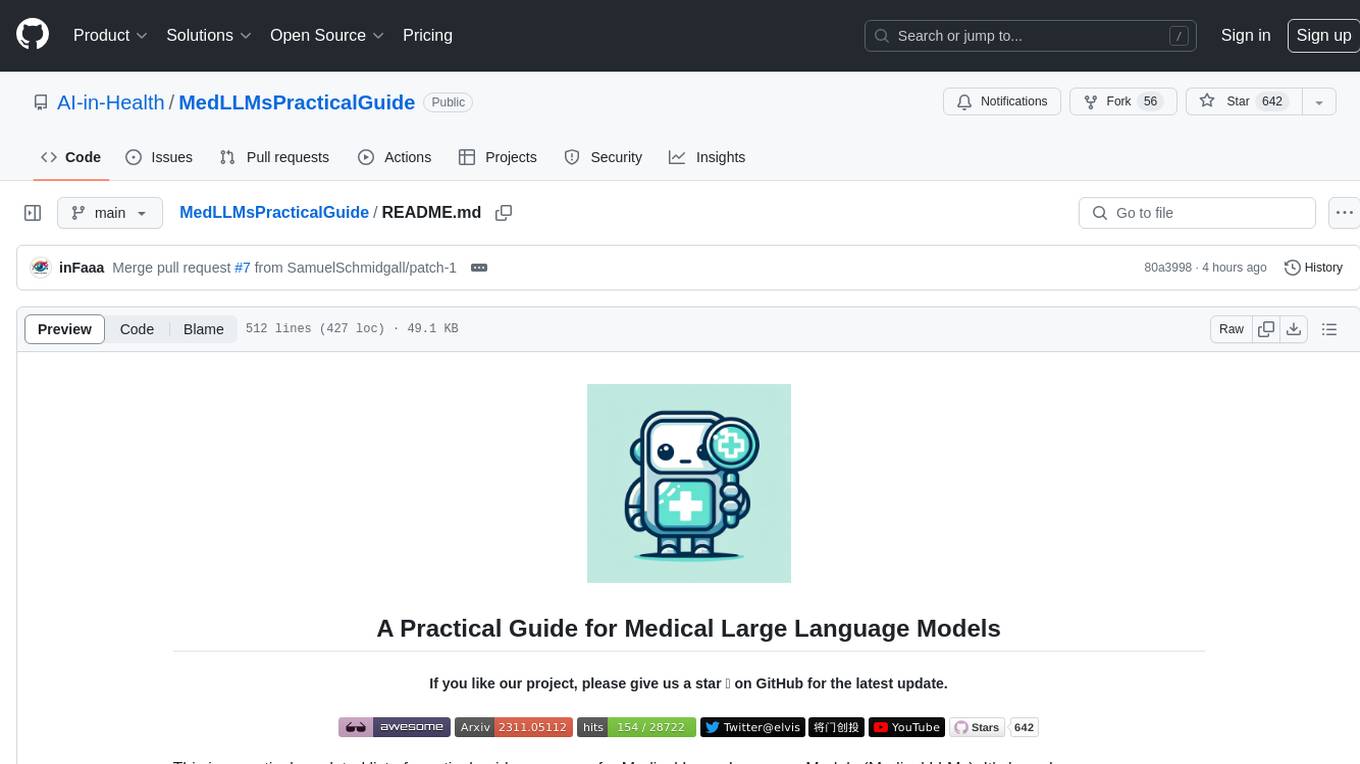
MedLLMsPracticalGuide
This repository serves as a practical guide for Medical Large Language Models (Medical LLMs) and provides resources, surveys, and tools for building, fine-tuning, and utilizing LLMs in the medical domain. It covers a wide range of topics including pre-training, fine-tuning, downstream biomedical tasks, clinical applications, challenges, future directions, and more. The repository aims to provide insights into the opportunities and challenges of LLMs in medicine and serve as a practical resource for constructing effective medical LLMs.
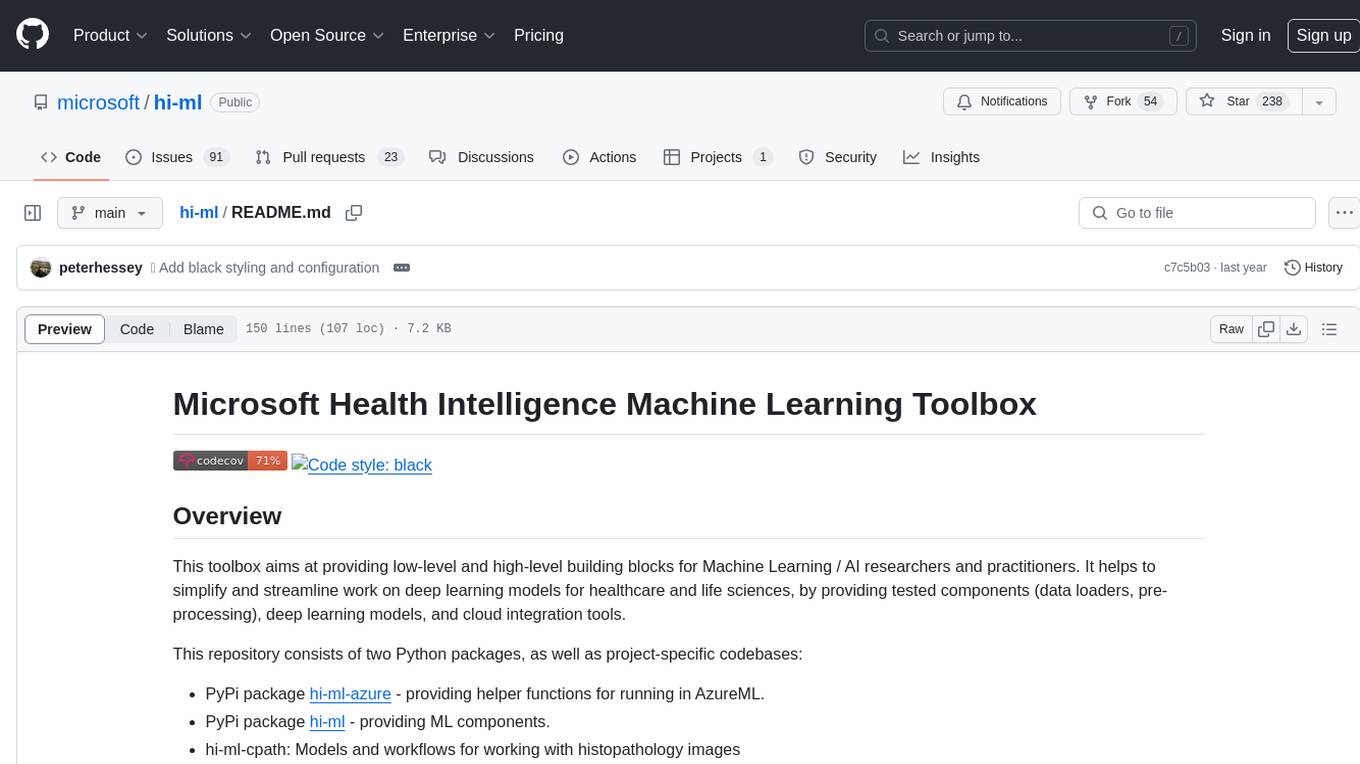
hi-ml
The Microsoft Health Intelligence Machine Learning Toolbox is a repository that provides low-level and high-level building blocks for Machine Learning / AI researchers and practitioners. It simplifies and streamlines work on deep learning models for healthcare and life sciences by offering tested components such as data loaders, pre-processing tools, deep learning models, and cloud integration utilities. The repository includes two Python packages, 'hi-ml-azure' for helper functions in AzureML, 'hi-ml' for ML components, and 'hi-ml-cpath' for models and workflows related to histopathology images.