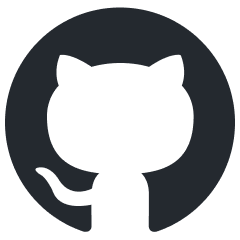
IvyGPT
[CICAI 2023] The official codes for "Ivygpt: Interactive chinese pathway language model in medical domain"
Stars: 56
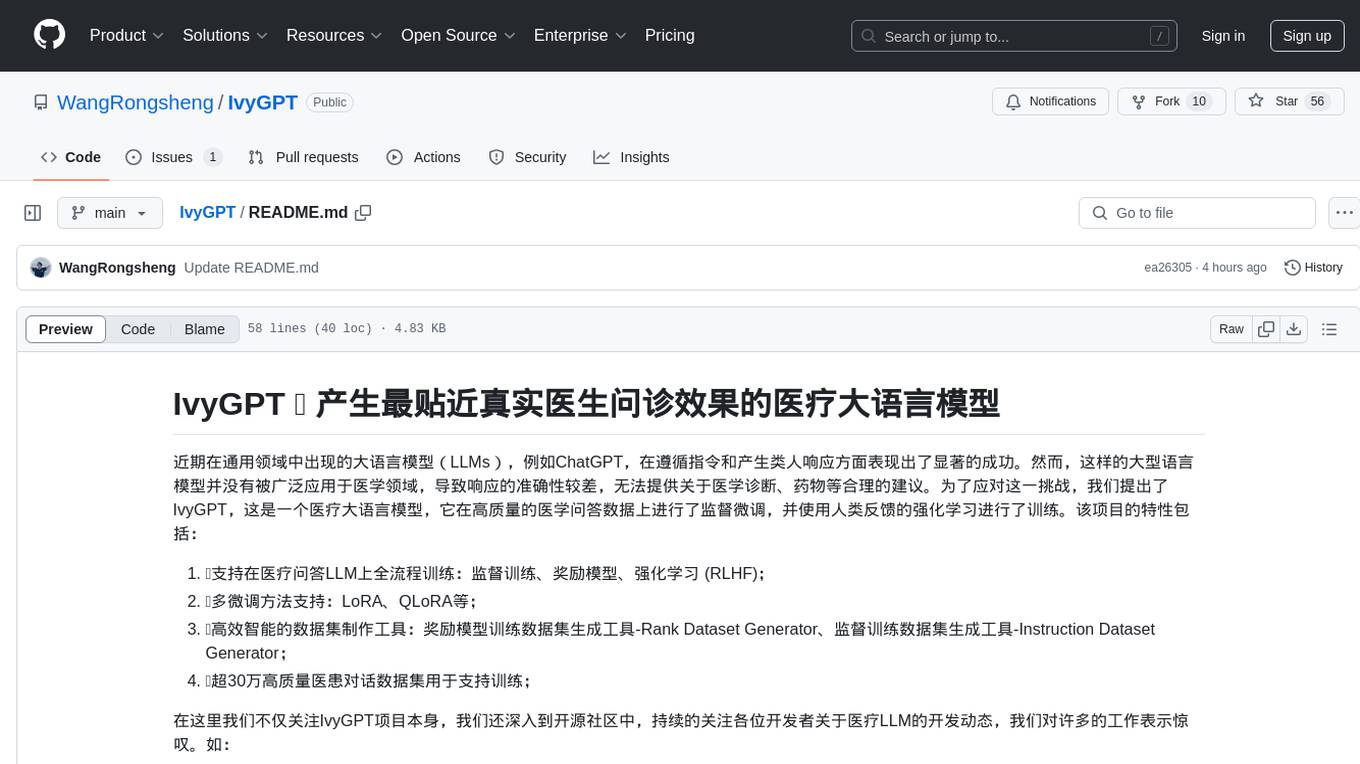
IvyGPT is a medical large language model that aims to generate the most realistic doctor consultation effects. It has been fine-tuned on high-quality medical Q&A data and trained using human feedback reinforcement learning. The project features full-process training on medical Q&A LLM, multiple fine-tuning methods support, efficient dataset creation tools, and a dataset of over 300,000 high-quality doctor-patient dialogues for training.
README:
近期在通用领域中出现的大语言模型(LLMs),例如ChatGPT,在遵循指令和产生类人响应方面表现出了显著的成功。然而,这样的大型语言模型并没有被广泛应用于医学领域,导致响应的准确性较差,无法提供关于医学诊断、药物等合理的建议。为了应对这一挑战,我们提出了IvyGPT,这是一个医疗大语言模型,它在高质量的医学问答数据上进行了监督微调,并使用人类反馈的强化学习进行了训练。该项目的特性包括:
- 🍦支持在医疗问答LLM上全流程训练:监督训练、奖励模型、强化学习 (RLHF);
- 🏵️多微调方法支持:LoRA、QLoRA等;
- 🥄高效智能的数据集制作工具:奖励模型训练数据集生成工具-Rank Dataset Generator、监督训练数据集生成工具-Instruction Dataset Generator;
- 🧽超30万高质量医患对话数据集用于支持训练;
在这里我们不仅关注IvyGPT项目本身,我们还深入到开源社区中,持续的关注各位开发者关于医疗LLM的开发动态,我们对许多的工作表示惊叹。如:
- 英文医疗LLM领域:ChatDoctor、PMC-LLaMA、medAlpaca;
- 中文医疗LLM领域:ChatMed、Med-ChatGLM、Huatuo-Llama-Med-Chinese、DoctorGLM、MedicalGPT-zh、QiZhenGPT、BianQue、MedicalGPT、LLM-Pretrain-FineTune;
关于常春藤:
- 常春藤是一种常见的攀援植物,其拉丁学名为Hedera helix。常春藤的叶子呈现出深绿色,具有闪亮的光泽,常被用作装饰植物。此外,常春藤在医学领域也有其应用,其叶子中含有一些活性成分,可以用于治疗一些疾病。例如,常春藤可以用于治疗呼吸道疾病、消化系统疾病、皮肤病等。此外,常春藤还具有镇静、镇痛、抗炎等作用,可以用于缓解焦虑、失眠、疼痛等症状。
- 常春藤是一种常绿的攀援植物,它的寓意在医学上也很美好。常春藤的攀爬和延伸象征着医学的不断发展和进步,它的常绿象征着医学的持久不变和永恒的价值。此外,常春藤还有着坚韧、适应力强等特点,这也是医学工作者所需要具备的品质。因此,常春藤在医学上被赋予了积极向上的寓意,它象征着医学工作者不断追求进步和创新的精神。
- 常春藤在医院患者身上也有着美好的寓意。常春藤的攀爬和延伸象征着患者的希望和努力,他们在疾病的折磨下仍然坚强地向前迈进,不断寻求治疗和康复的方法。常春藤的常绿象征着患者们的生命力和坚韧不拔,他们在面对疾病时不会轻易放弃,而是坚持不懈地与疾病作斗争。因此,常春藤在医院患者身上也被赋予了积极向上的寓意,它象征着患者们对生命的热爱和追求,以及对未来的信心和希望。
这项工作由澳门理工大学应用科学学院硕士生王荣胜完成,指导老师为檀韬副教授。
本项目相关资源仅供学术研究之用,严禁用于商业用途。使用涉及第三方代码的部分时,请严格遵循相应的开源协议。模型生成的内容受模型计算、随机性和量化精度损失等因素影响,本项目无法对其准确性作出保证。即使本项目模型输出符合医学事实,也不能被用作实际医学诊断的依据。对于模型输出的任何内容,本项目不承担任何法律责任,亦不对因使用相关资源和输出结果而可能产生的任何损失承担责任。
如果您觉得此项目有帮助,请引用:
@inproceedings{wang2023ivygpt,
title={Ivygpt: Interactive chinese pathway language model in medical domain},
author={Wang, Rongsheng and Duan, Yaofei and Lam, ChanTong and Chen, Jiexin and Xu, Jiangsheng and Chen, Haoming and Liu, Xiaohong and Pang, Patrick Cheong-Iao and Tan, Tao},
booktitle={CAAI International Conference on Artificial Intelligence},
pages={378--382},
year={2023},
organization={Springer}
}
For Tasks:
Click tags to check more tools for each tasksFor Jobs:
Alternative AI tools for IvyGPT
Similar Open Source Tools
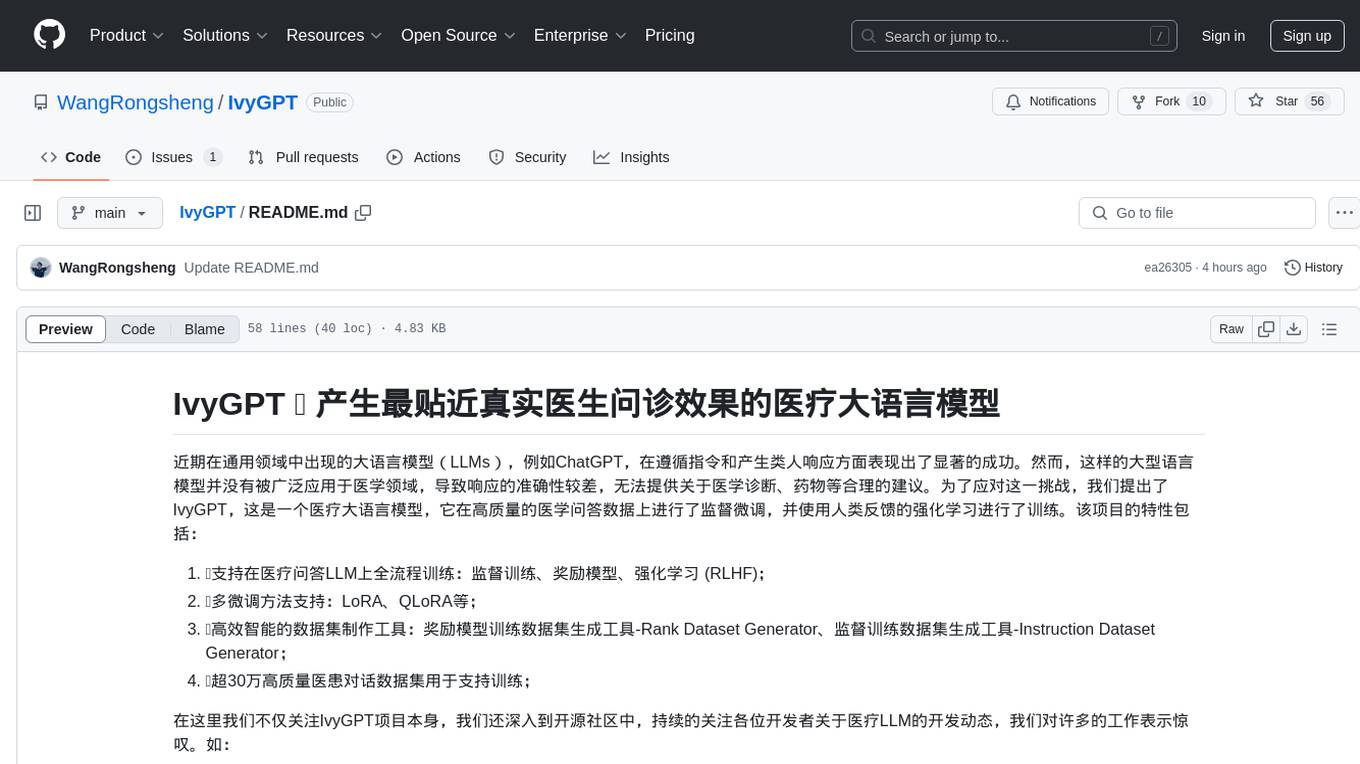
IvyGPT
IvyGPT is a medical large language model that aims to generate the most realistic doctor consultation effects. It has been fine-tuned on high-quality medical Q&A data and trained using human feedback reinforcement learning. The project features full-process training on medical Q&A LLM, multiple fine-tuning methods support, efficient dataset creation tools, and a dataset of over 300,000 high-quality doctor-patient dialogues for training.
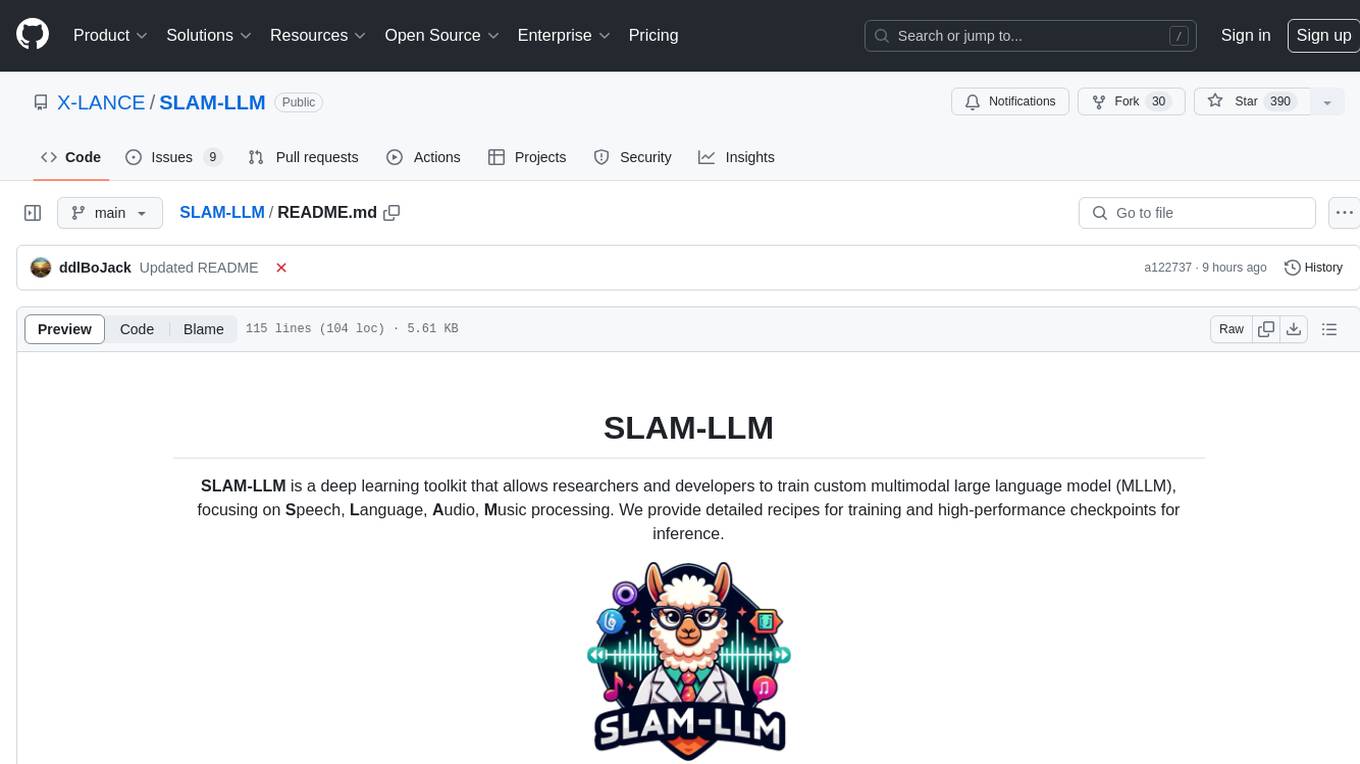
SLAM-LLM
SLAM-LLM is a deep learning toolkit for training custom multimodal large language models (MLLM) focusing on speech, language, audio, and music processing. It provides detailed recipes for training and high-performance checkpoints for inference. The toolkit supports various tasks such as automatic speech recognition (ASR), text-to-speech (TTS), visual speech recognition (VSR), automated audio captioning (AAC), spatial audio understanding, and music caption (MC). Users can easily extend to new models and tasks, utilize mixed precision training for faster training with less GPU memory, and perform multi-GPU training with data and model parallelism. Configuration is flexible based on Hydra and dataclass, allowing different configuration methods.
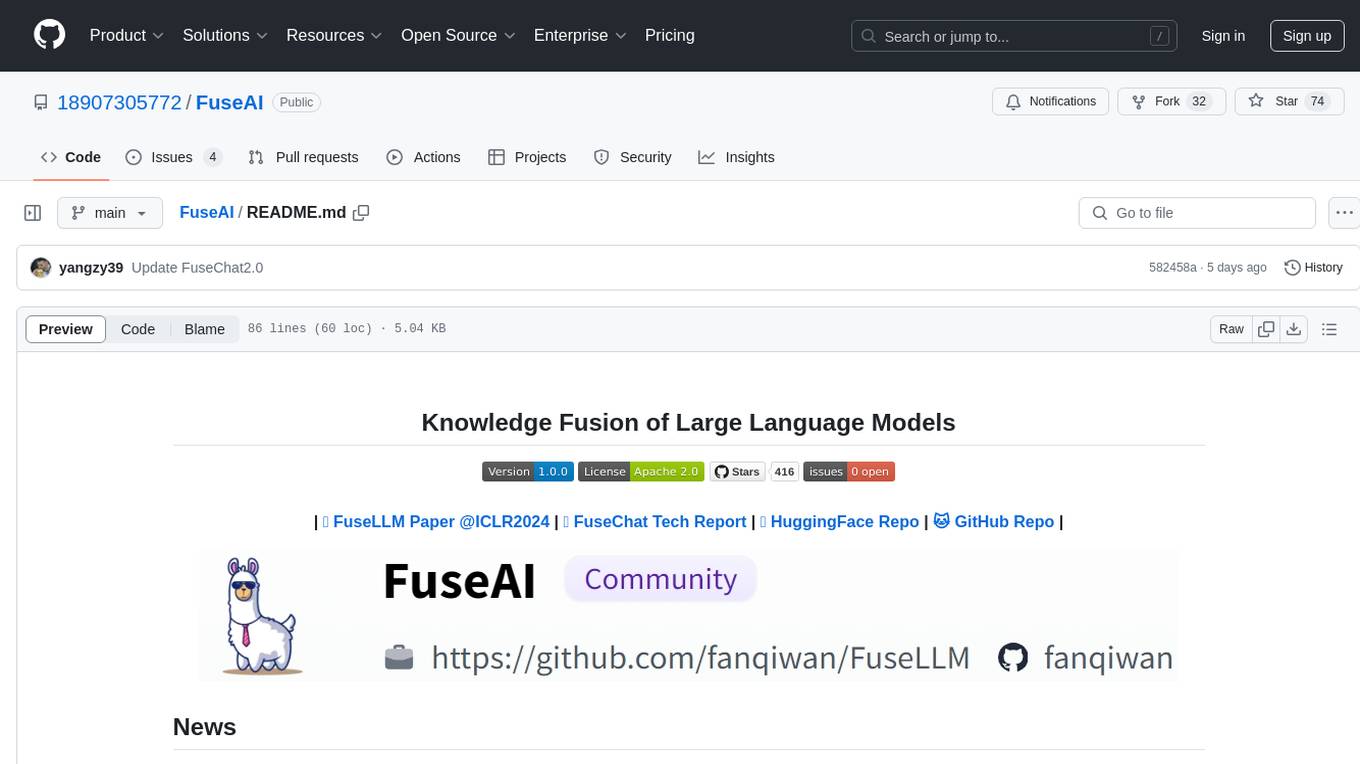
FuseAI
FuseAI is a repository that focuses on knowledge fusion of large language models. It includes FuseChat, a state-of-the-art 7B LLM on MT-Bench, and FuseLLM, which surpasses Llama-2-7B by fusing three open-source foundation LLMs. The repository provides tech reports, releases, and datasets for FuseChat and FuseLLM, showcasing their performance and advancements in the field of chat models and large language models.
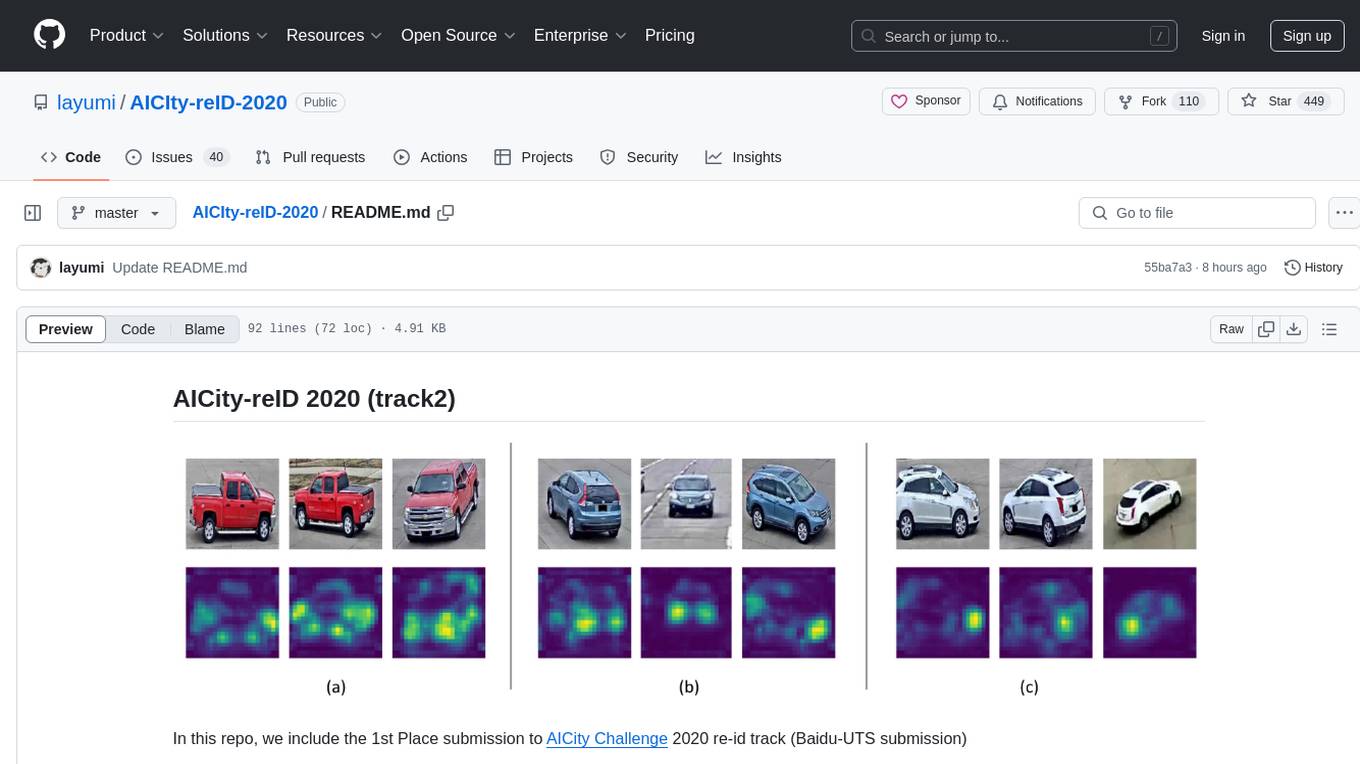
AICIty-reID-2020
AICIty-reID 2020 is a repository containing the 1st Place submission to AICity Challenge 2020 re-id track by Baidu-UTS. It includes models trained on Paddlepaddle and Pytorch, with performance metrics and trained models provided. Users can extract features, perform camera and direction prediction, and access related repositories for drone-based building re-id, vehicle re-ID, person re-ID baseline, and person/vehicle generation. Citations are also provided for research purposes.
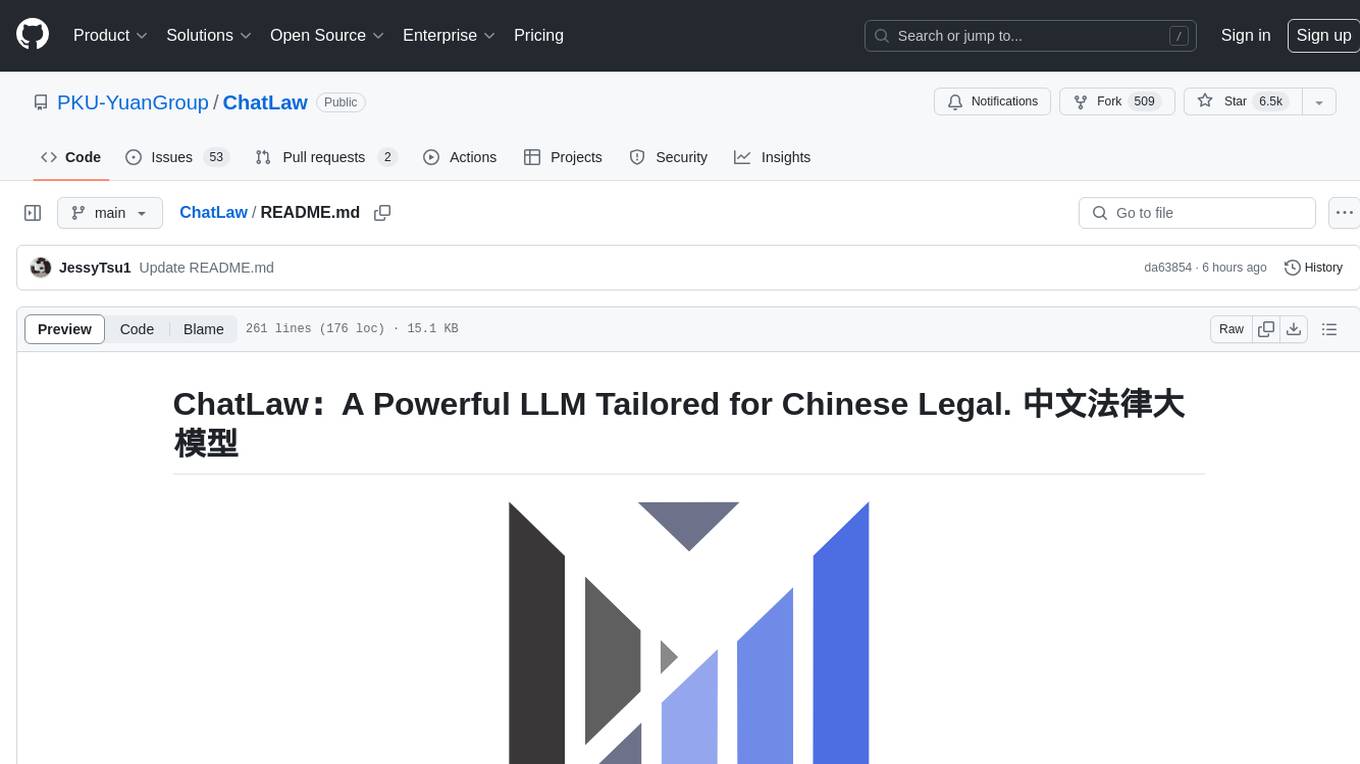
ChatLaw
ChatLaw is an open-source legal large language model tailored for Chinese legal scenarios. It aims to combine LLM and knowledge bases to provide solutions for legal scenarios. The models include ChatLaw-13B and ChatLaw-33B, trained on various legal texts to construct dialogue data. The project focuses on improving logical reasoning abilities and plans to train models with parameters exceeding 30B for better performance. The dataset consists of forum posts, news, legal texts, judicial interpretations, legal consultations, exam questions, and court judgments, cleaned and enhanced to create dialogue data. The tool is designed to assist in legal tasks requiring complex logical reasoning, with a focus on accuracy and reliability.
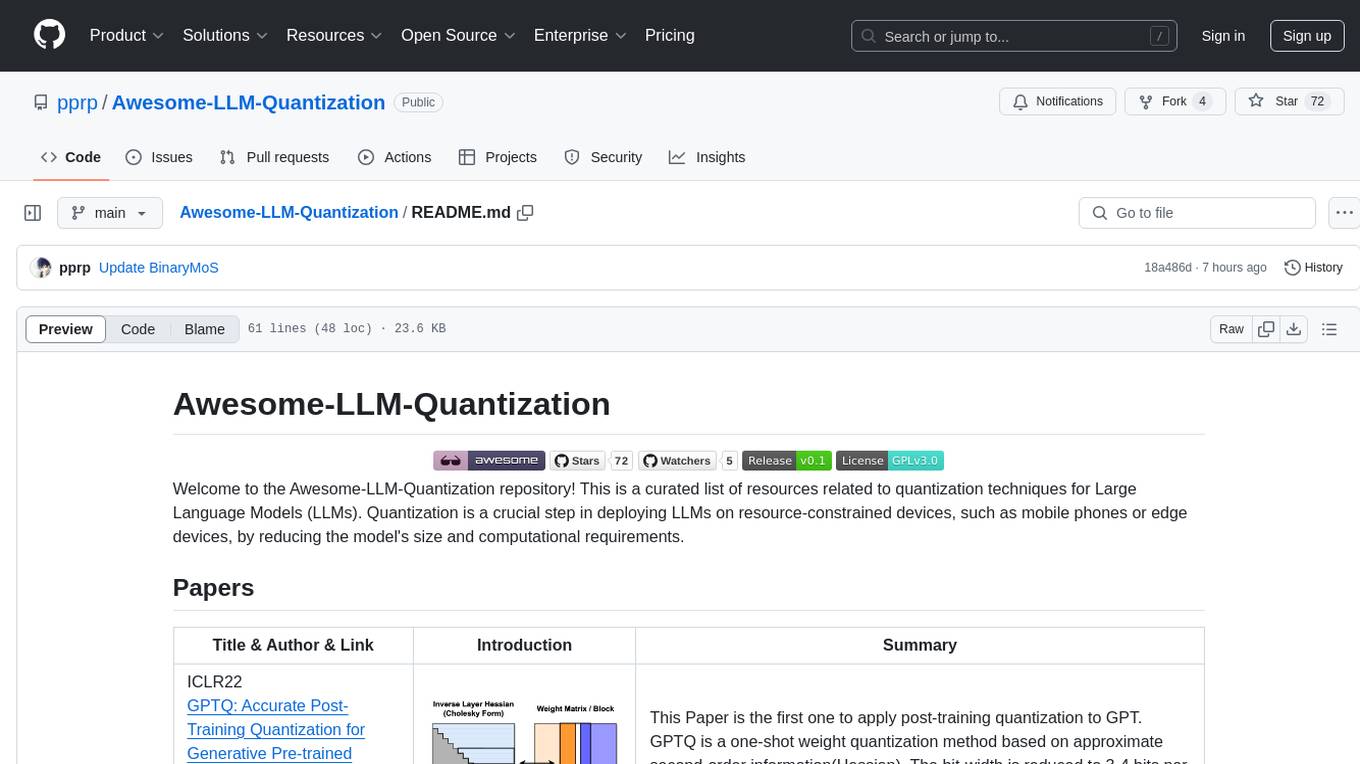
Awesome-LLM-Quantization
Awesome-LLM-Quantization is a curated list of resources related to quantization techniques for Large Language Models (LLMs). Quantization is a crucial step in deploying LLMs on resource-constrained devices, such as mobile phones or edge devices, by reducing the model's size and computational requirements.
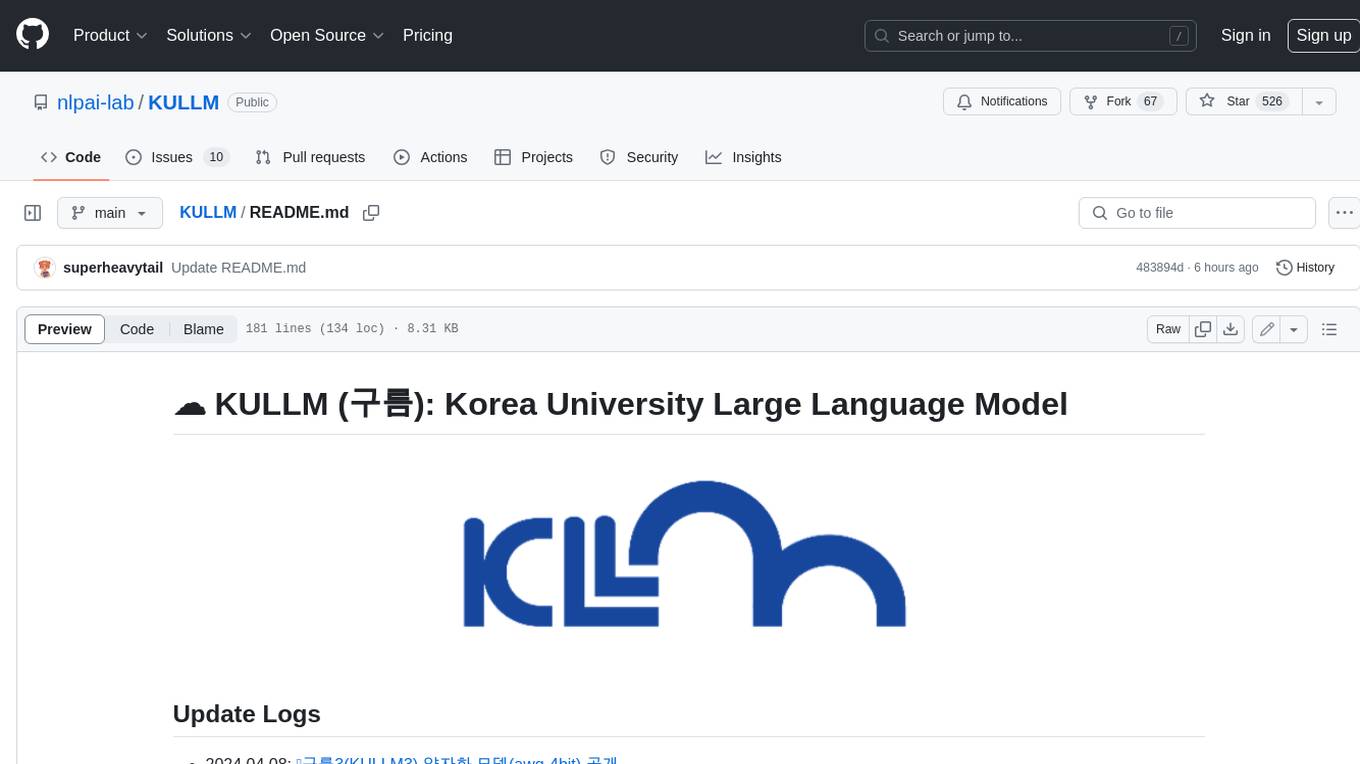
KULLM
KULLM (구름) is a Korean Large Language Model developed by Korea University NLP & AI Lab and HIAI Research Institute. It is based on the upstage/SOLAR-10.7B-v1.0 model and has been fine-tuned for instruction. The model has been trained on 8×A100 GPUs and is capable of generating responses in Korean language. KULLM exhibits hallucination and repetition phenomena due to its decoding strategy. Users should be cautious as the model may produce inaccurate or harmful results. Performance may vary in benchmarks without a fixed system prompt.
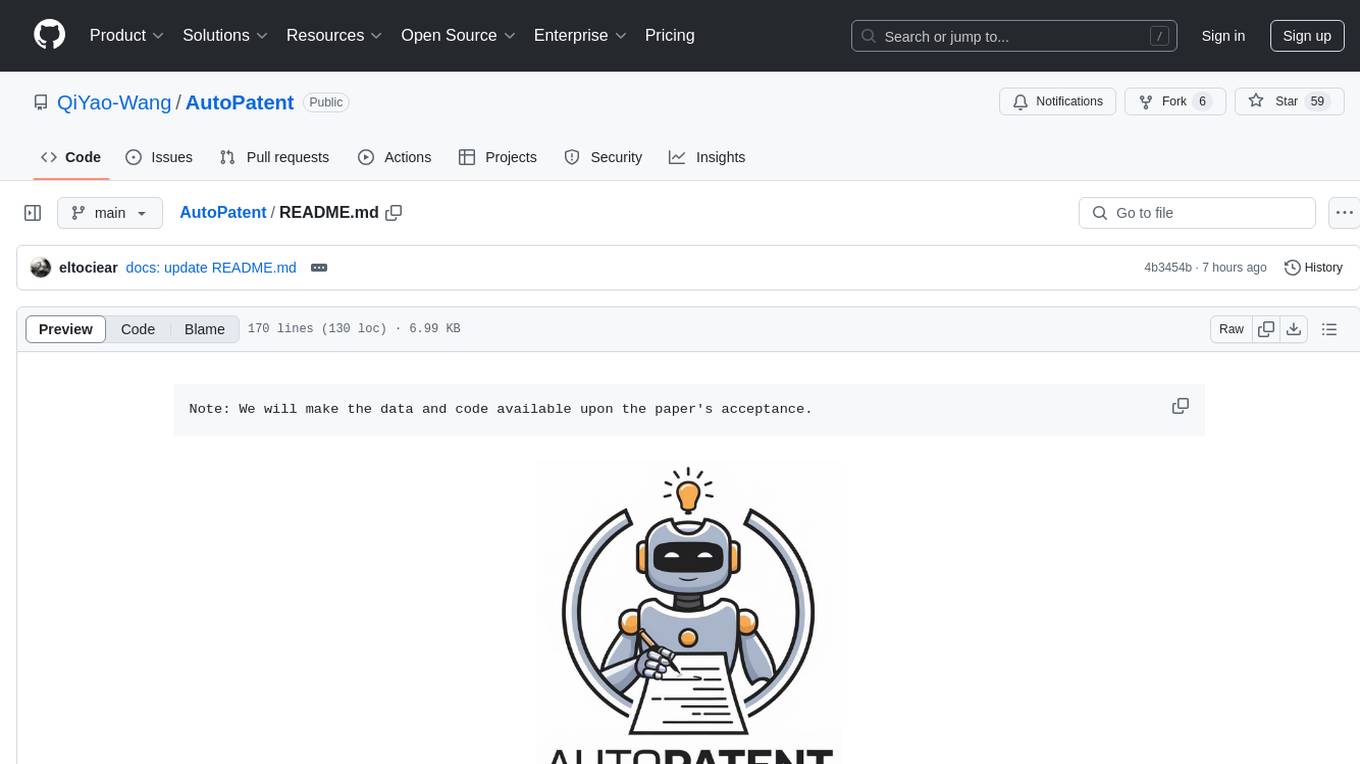
AutoPatent
AutoPatent is a multi-agent framework designed for automatic patent generation. It challenges large language models to generate full-length patents based on initial drafts. The framework leverages planner, writer, and examiner agents along with PGTree and RRAG to craft lengthy, intricate, and high-quality patent documents. It introduces a new metric, IRR (Inverse Repetition Rate), to measure sentence repetition within patents. The tool aims to streamline the patent generation process by automating the creation of detailed and specialized patent documents.
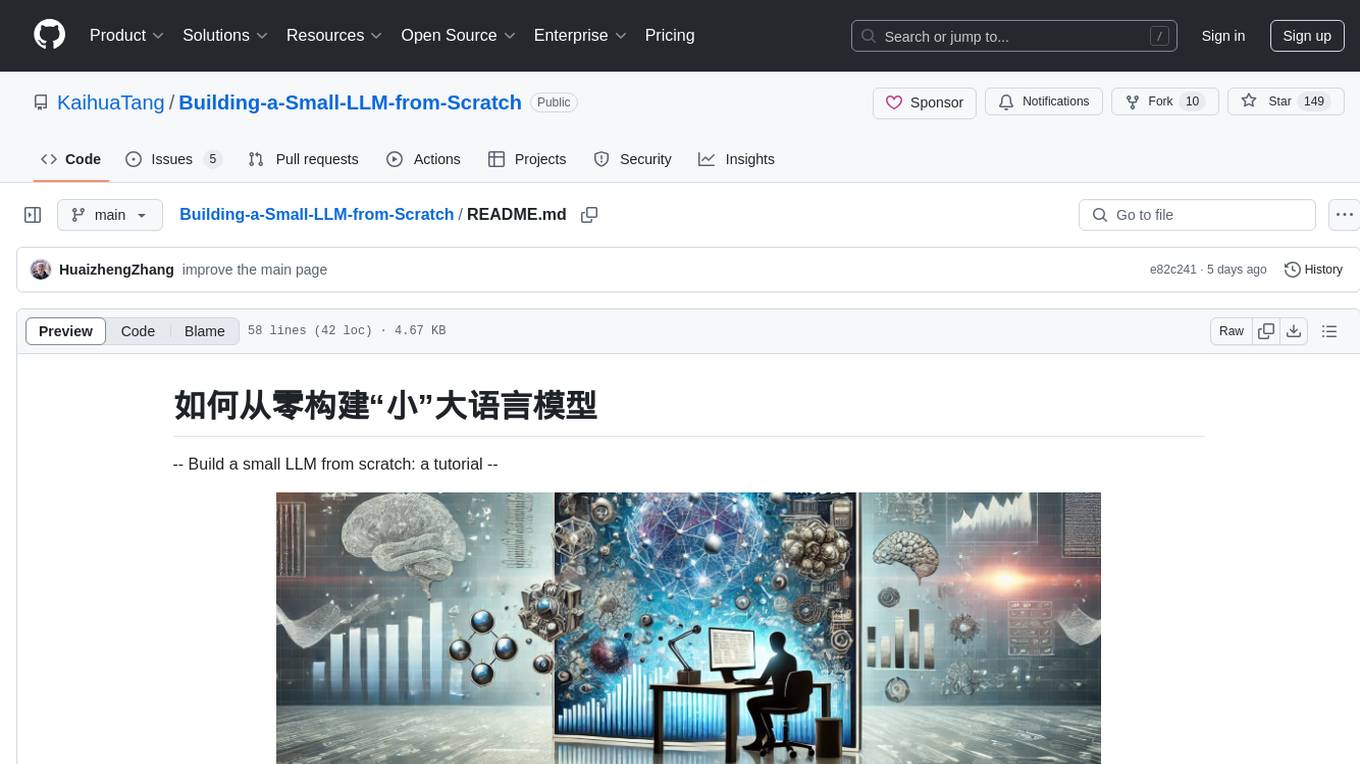
Building-a-Small-LLM-from-Scratch
This tutorial provides a comprehensive guide on building a small Large Language Model (LLM) from scratch using PyTorch. The author shares insights and experiences gained from working on LLM projects in the industry, aiming to help beginners understand the fundamental components of LLMs and training fine-tuning codes. The tutorial covers topics such as model structure overview, attention modules, optimization techniques, normalization layers, tokenizers, pretraining, and fine-tuning with dialogue data. It also addresses specific industry-related challenges and explores cutting-edge model concepts like DeepSeek network structure, causal attention, dynamic-to-static tensor conversion for ONNX inference, and performance optimizations for NPU chips. The series emphasizes hands-on practice with small models to enable local execution and plans to expand into multimodal language models and tensor parallel multi-card deployment.
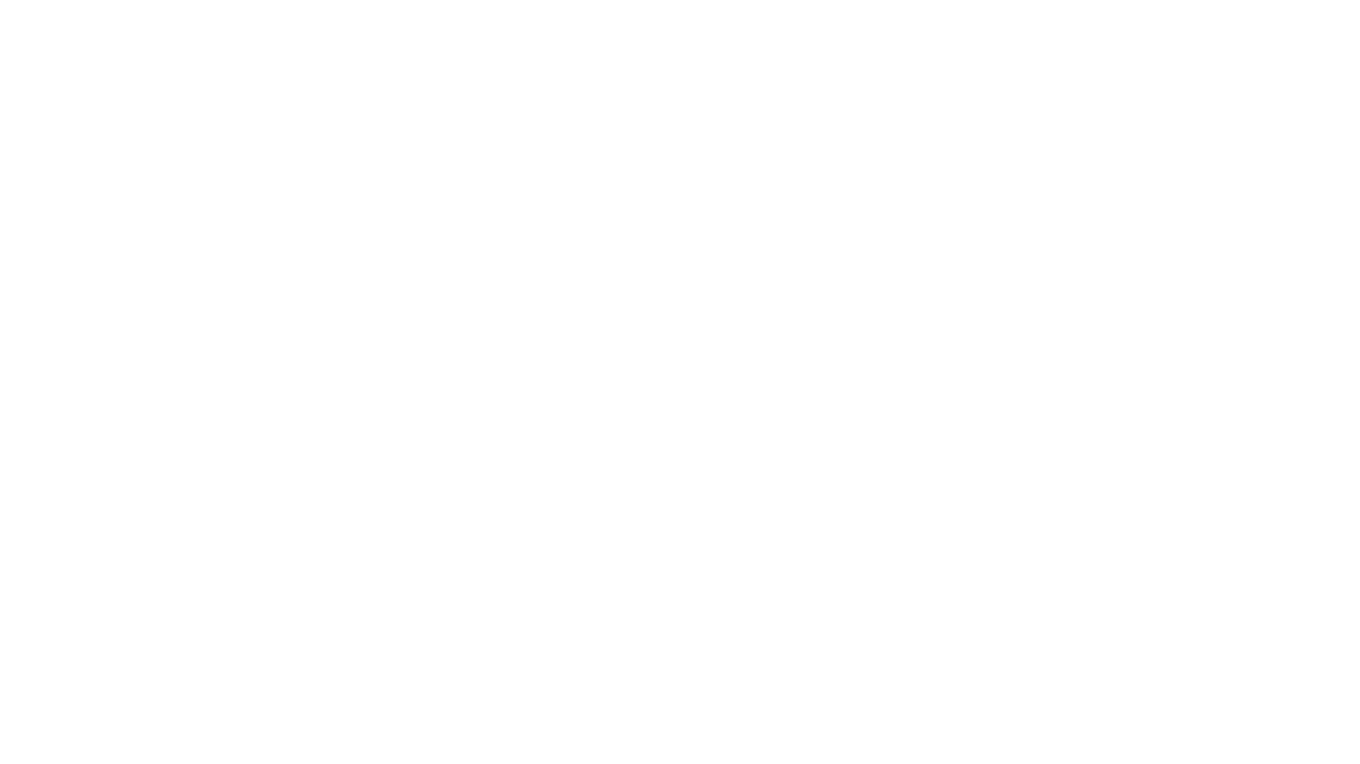
only_train_once
Only Train Once (OTO) is an automatic, architecture-agnostic DNN training and compression framework that allows users to train a general DNN from scratch or a pretrained checkpoint to achieve high performance and slimmer architecture simultaneously in a one-shot manner without fine-tuning. The framework includes features for automatic structured pruning and erasing operators, as well as hybrid structured sparse optimizers for efficient model compression. OTO provides tools for pruning zero-invariant group partitioning, constructing pruned models, and visualizing pruning and erasing dependency graphs. It supports the HESSO optimizer and offers a sanity check for compliance testing on various DNNs. The repository also includes publications, installation instructions, quick start guides, and a roadmap for future enhancements and collaborations.
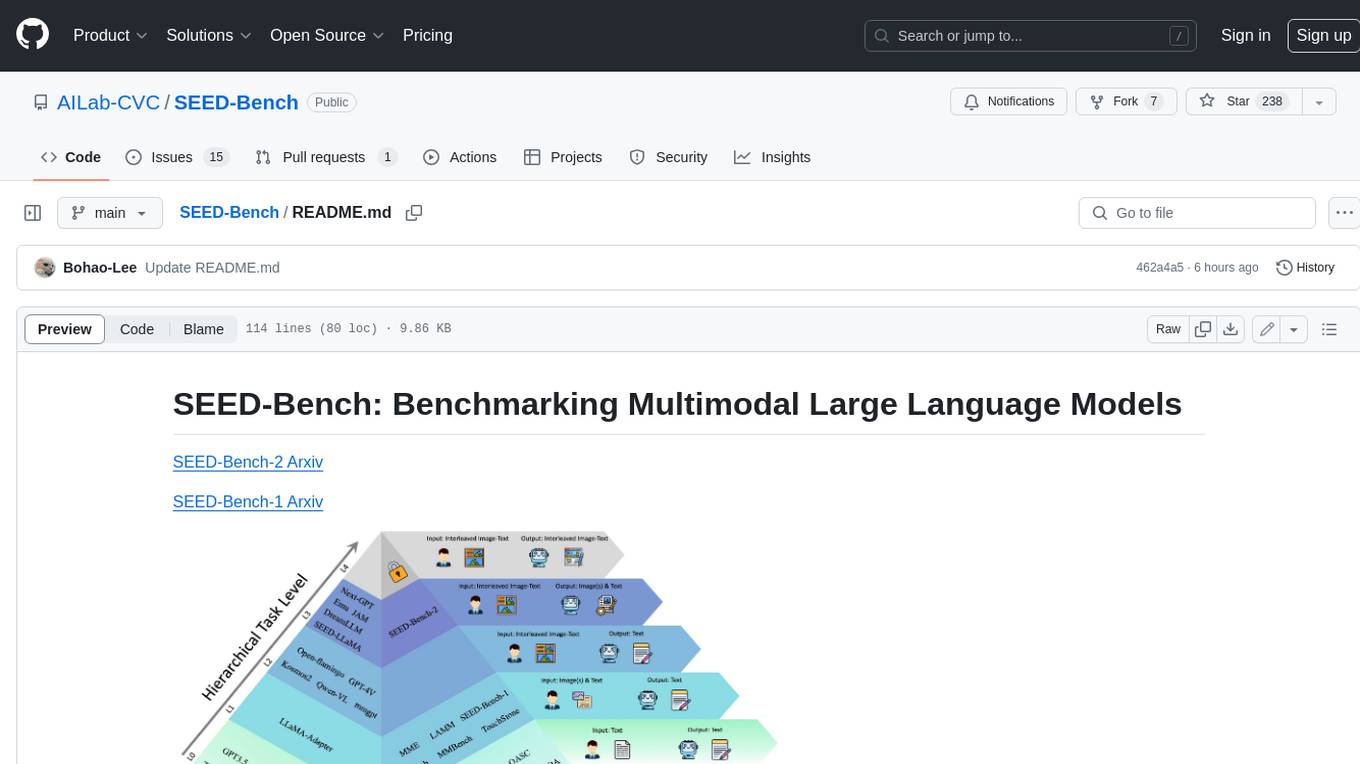
SEED-Bench
SEED-Bench is a comprehensive benchmark for evaluating the performance of multimodal large language models (LLMs) on a wide range of tasks that require both text and image understanding. It consists of two versions: SEED-Bench-1 and SEED-Bench-2. SEED-Bench-1 focuses on evaluating the spatial and temporal understanding of LLMs, while SEED-Bench-2 extends the evaluation to include text and image generation tasks. Both versions of SEED-Bench provide a diverse set of tasks that cover different aspects of multimodal understanding, making it a valuable tool for researchers and practitioners working on LLMs.
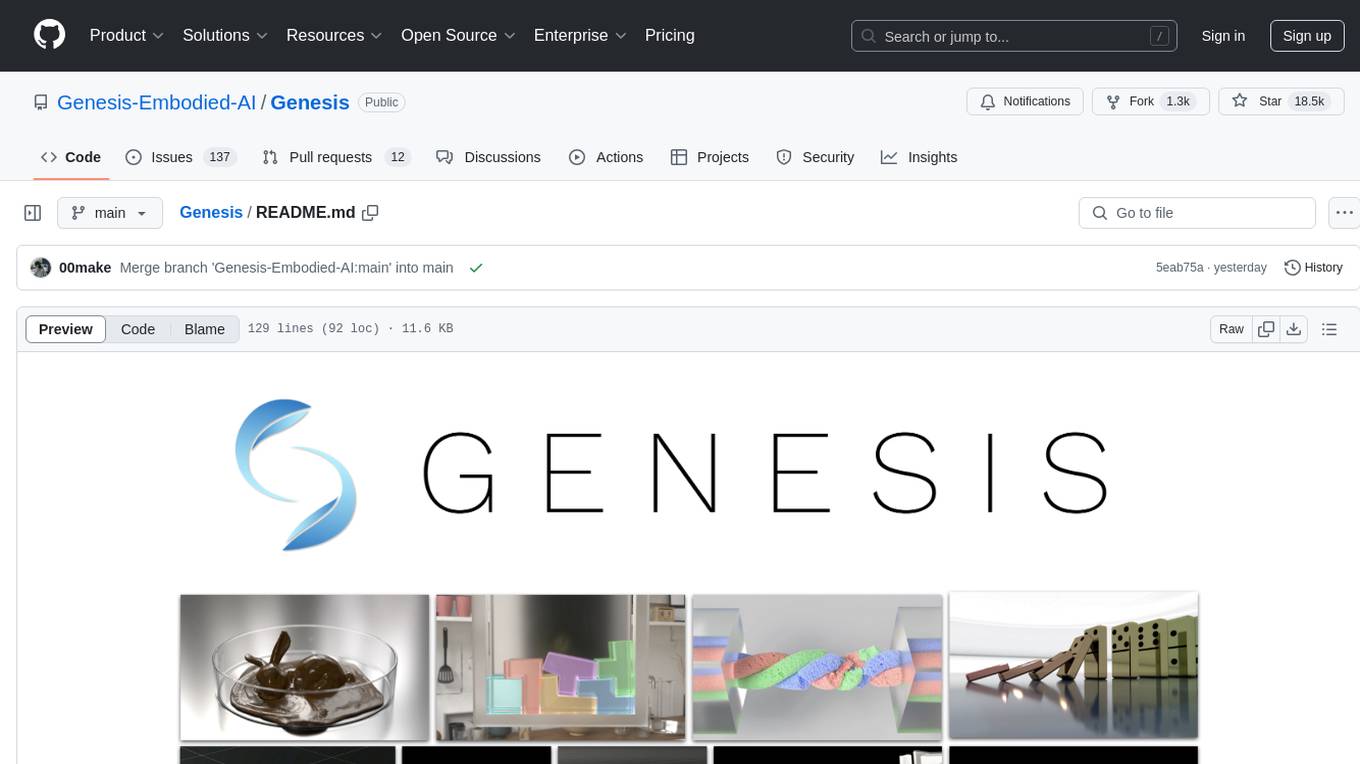
Genesis
Genesis is a physics platform designed for general purpose Robotics/Embodied AI/Physical AI applications. It includes a universal physics engine, a lightweight, ultra-fast, pythonic, and user-friendly robotics simulation platform, a powerful and fast photo-realistic rendering system, and a generative data engine that transforms user-prompted natural language description into various modalities of data. It aims to lower the barrier to using physics simulations, unify state-of-the-art physics solvers, and minimize human effort in collecting and generating data for robotics and other domains.
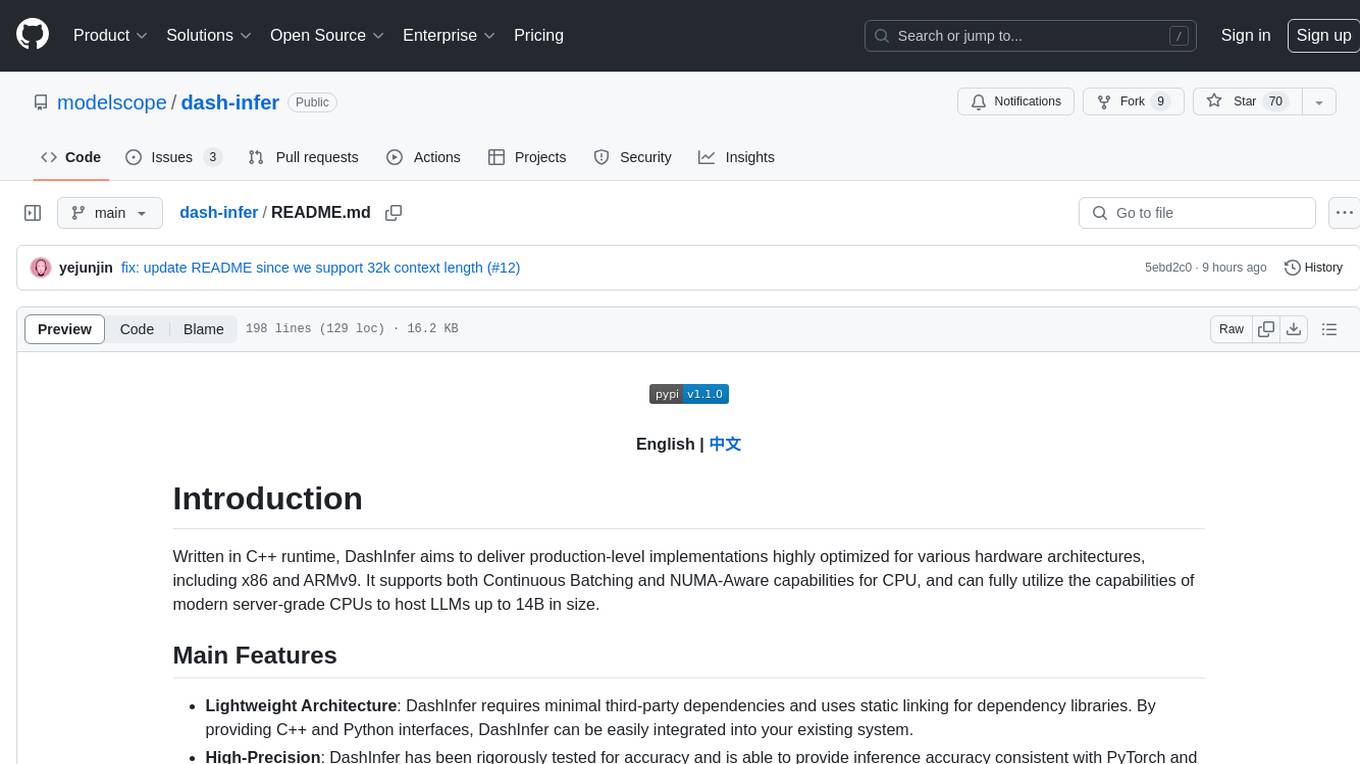
dash-infer
DashInfer is a C++ runtime tool designed to deliver production-level implementations highly optimized for various hardware architectures, including x86 and ARMv9. It supports Continuous Batching and NUMA-Aware capabilities for CPU, and can fully utilize modern server-grade CPUs to host large language models (LLMs) up to 14B in size. With lightweight architecture, high precision, support for mainstream open-source LLMs, post-training quantization, optimized computation kernels, NUMA-aware design, and multi-language API interfaces, DashInfer provides a versatile solution for efficient inference tasks. It supports x86 CPUs with AVX2 instruction set and ARMv9 CPUs with SVE instruction set, along with various data types like FP32, BF16, and InstantQuant. DashInfer also offers single-NUMA and multi-NUMA architectures for model inference, with detailed performance tests and inference accuracy evaluations available. The tool is supported on mainstream Linux server operating systems and provides documentation and examples for easy integration and usage.
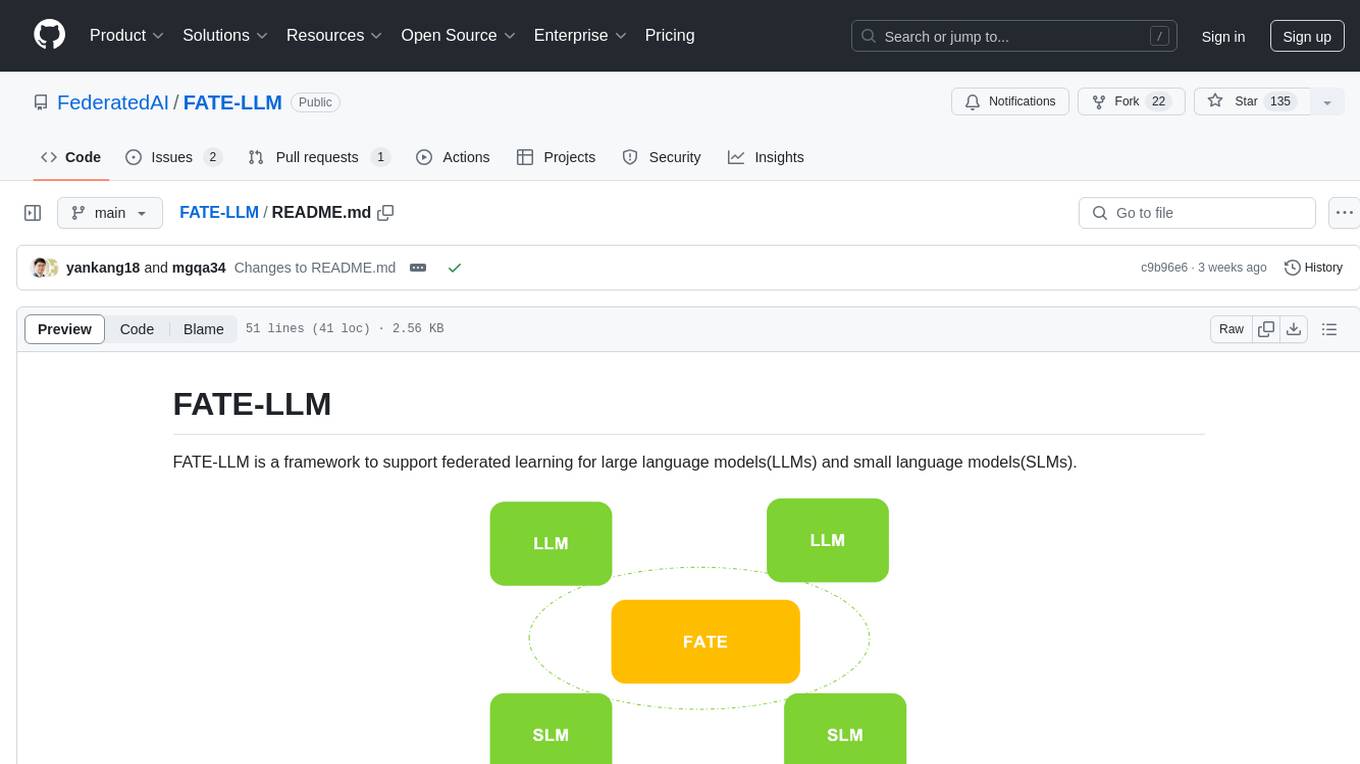
FATE-LLM
FATE-LLM is a framework supporting federated learning for large and small language models. It promotes training efficiency of federated LLMs using Parameter-Efficient methods, protects the IP of LLMs using FedIPR, and ensures data privacy during training and inference through privacy-preserving mechanisms.
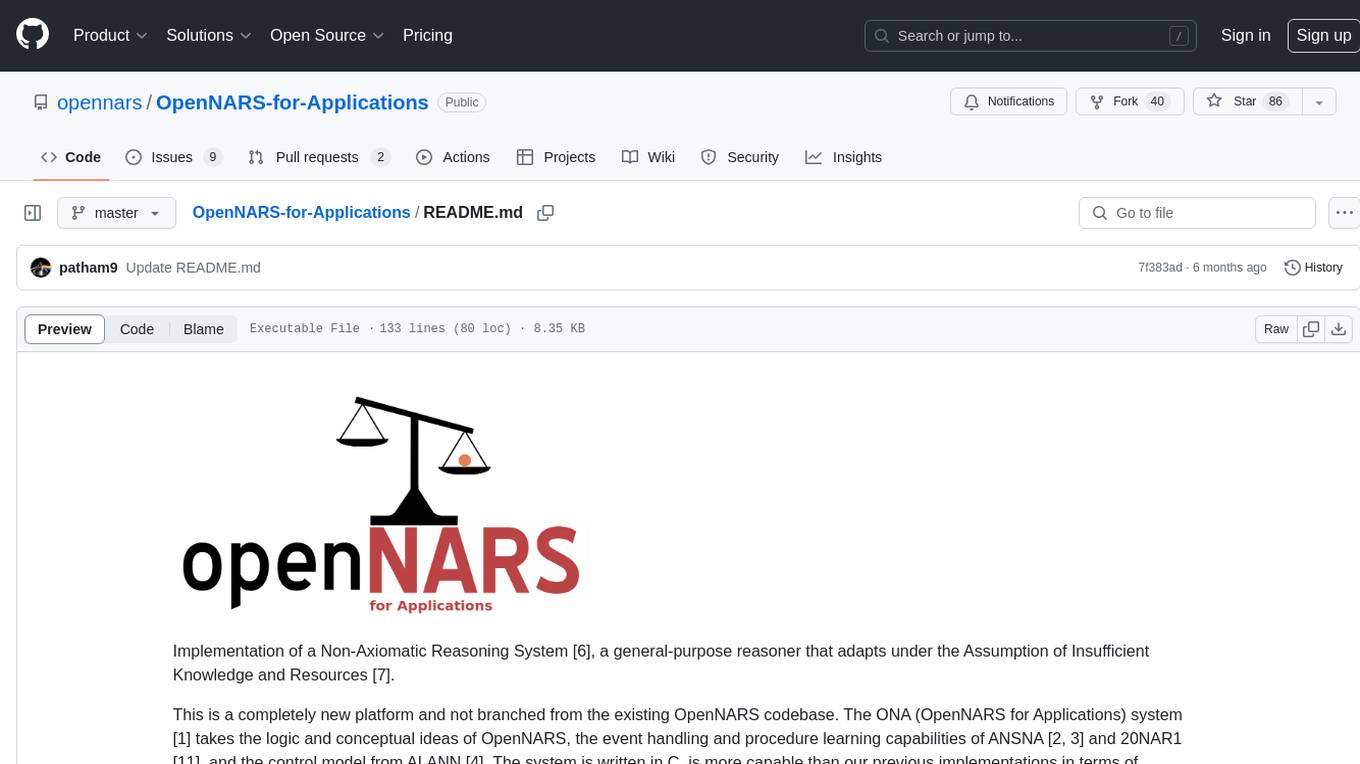
OpenNARS-for-Applications
OpenNARS-for-Applications is an implementation of a Non-Axiomatic Reasoning System, a general-purpose reasoner that adapts under the Assumption of Insufficient Knowledge and Resources. The system combines the logic and conceptual ideas of OpenNARS, event handling and procedure learning capabilities of ANSNA and 20NAR1, and the control model from ALANN. It is written in C, offers improved reasoning performance, and has been compared with Reinforcement Learning and means-end reasoning approaches. The system has been used in real-world applications such as assisting first responders, real-time traffic surveillance, and experiments with autonomous robots. It has been developed with a pragmatic mindset focusing on effective implementation of existing theory.
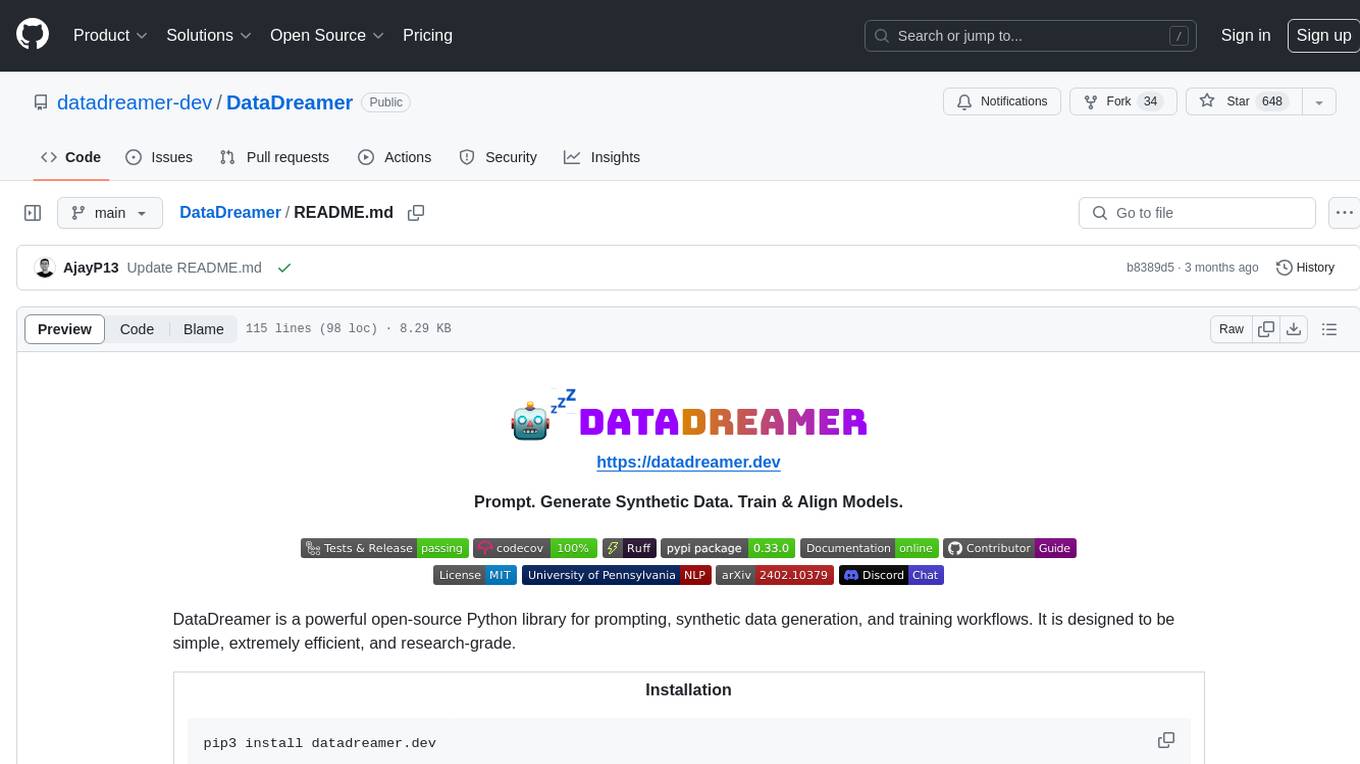
DataDreamer
DataDreamer is a powerful open-source Python library designed for prompting, synthetic data generation, and training workflows. It is simple, efficient, and research-grade, allowing users to create prompting workflows, generate synthetic datasets, and train models with ease. The library is built for researchers, by researchers, focusing on correctness, best practices, and reproducibility. It offers features like aggressive caching, resumability, support for bleeding-edge techniques, and easy sharing of datasets and models. DataDreamer enables users to run multi-step prompting workflows, generate synthetic datasets for various tasks, and train models by aligning, fine-tuning, instruction-tuning, and distilling them using existing or synthetic data.
For similar tasks
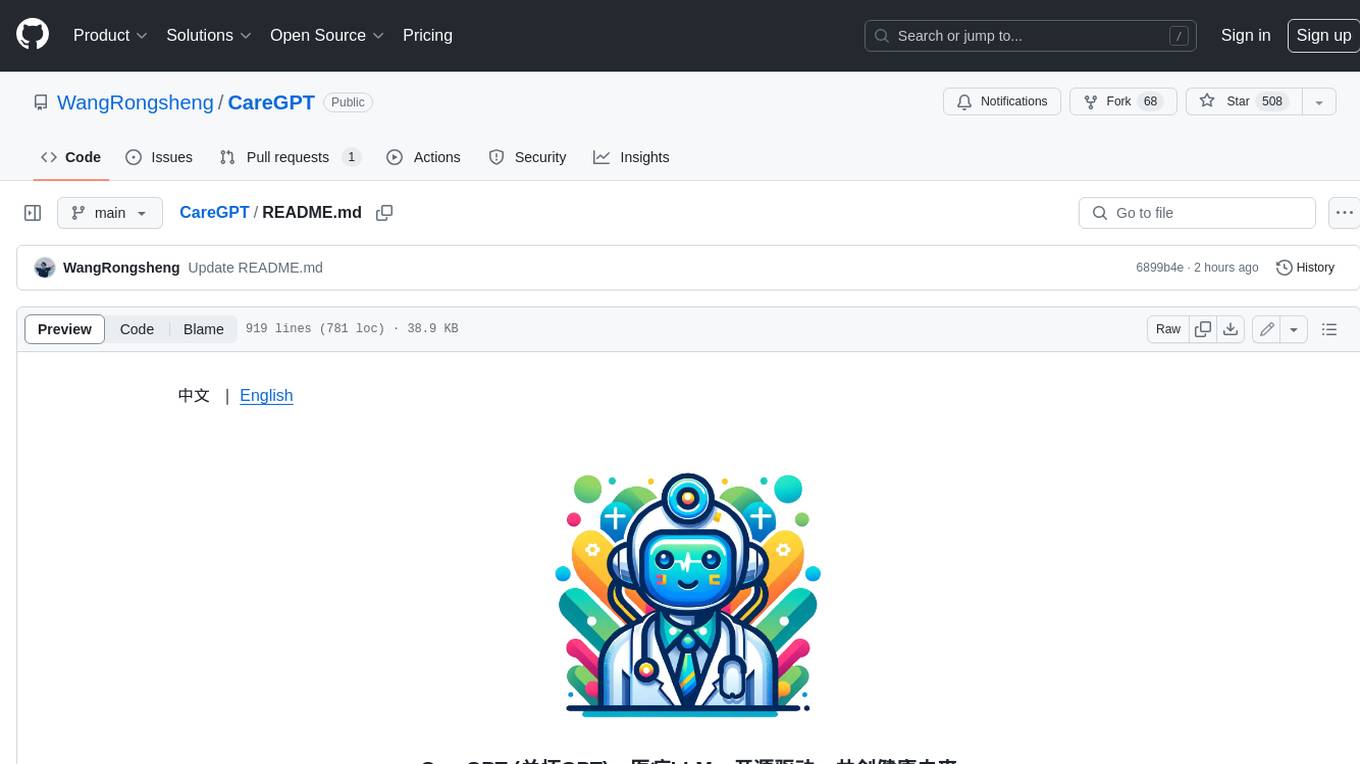
CareGPT
CareGPT is a medical large language model (LLM) that explores medical data, training, and deployment related research work. It integrates resources, open-source models, rich data, and efficient deployment methods. It supports various medical tasks, including patient diagnosis, medical dialogue, and medical knowledge integration. The model has been fine-tuned on diverse medical datasets to enhance its performance in the healthcare domain.
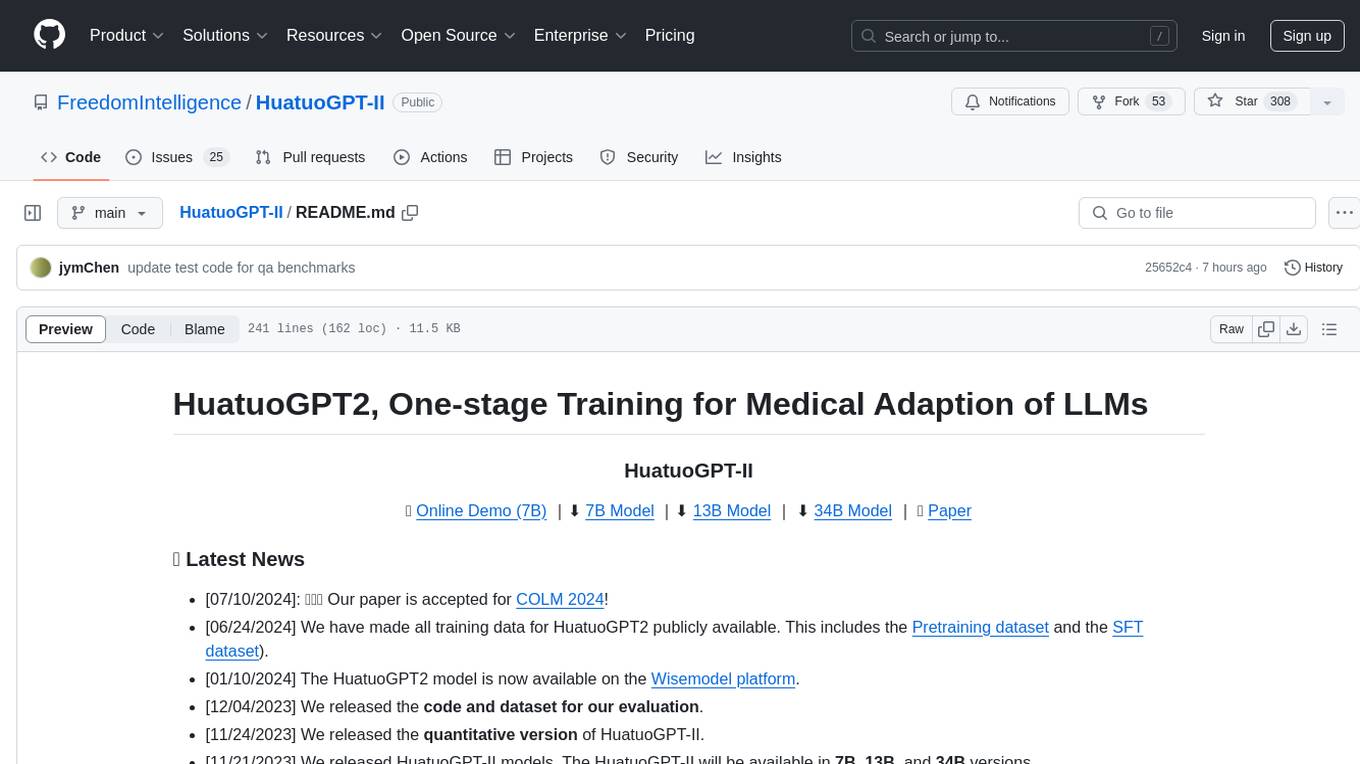
HuatuoGPT-II
HuatuoGPT2 is an innovative domain-adapted medical large language model that excels in medical knowledge and dialogue proficiency. It showcases state-of-the-art performance in various medical benchmarks, surpassing GPT-4 in expert evaluations and fresh medical licensing exams. The open-source release includes HuatuoGPT2 models in 7B, 13B, and 34B versions, training code for one-stage adaptation, partial pre-training and fine-tuning instructions, and evaluation methods for medical response capabilities and professional pharmacist exams. The tool aims to enhance LLM capabilities in the Chinese medical field through open-source principles.
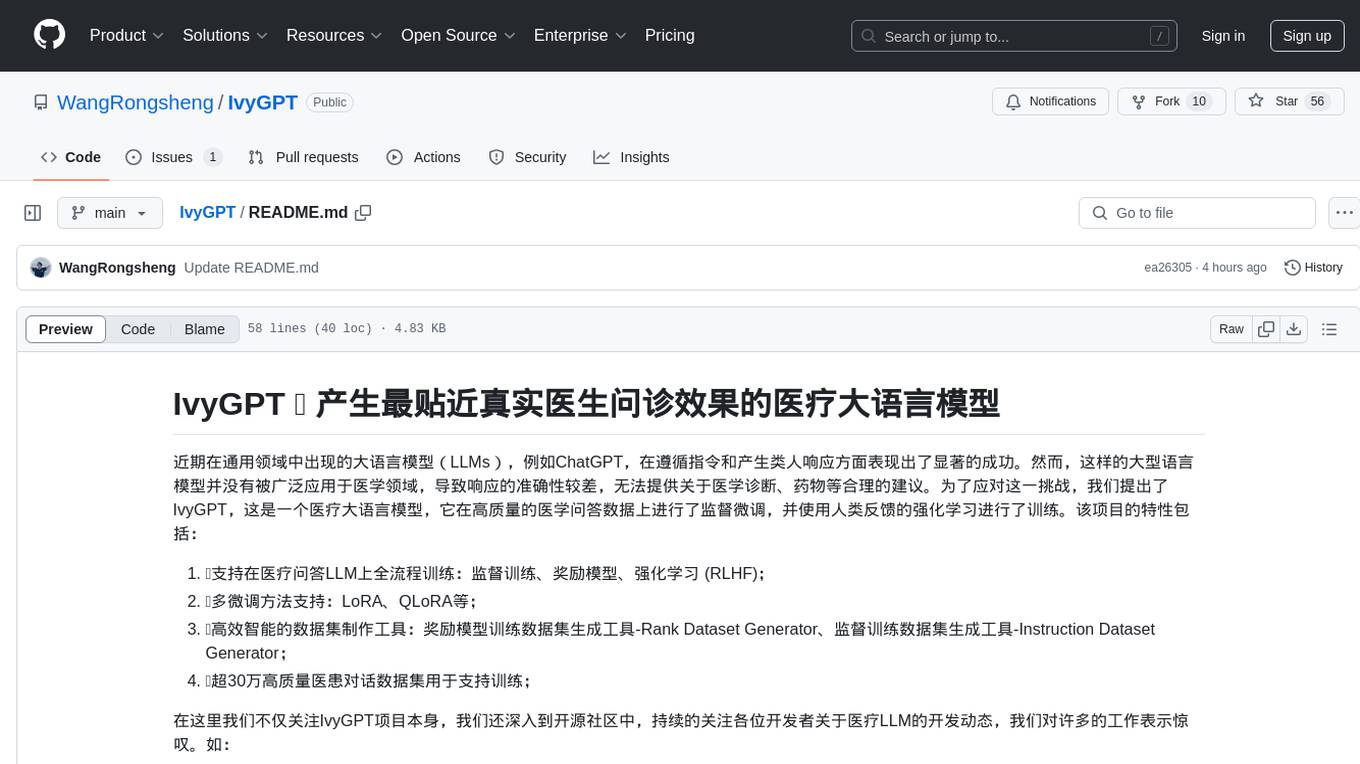
IvyGPT
IvyGPT is a medical large language model that aims to generate the most realistic doctor consultation effects. It has been fine-tuned on high-quality medical Q&A data and trained using human feedback reinforcement learning. The project features full-process training on medical Q&A LLM, multiple fine-tuning methods support, efficient dataset creation tools, and a dataset of over 300,000 high-quality doctor-patient dialogues for training.
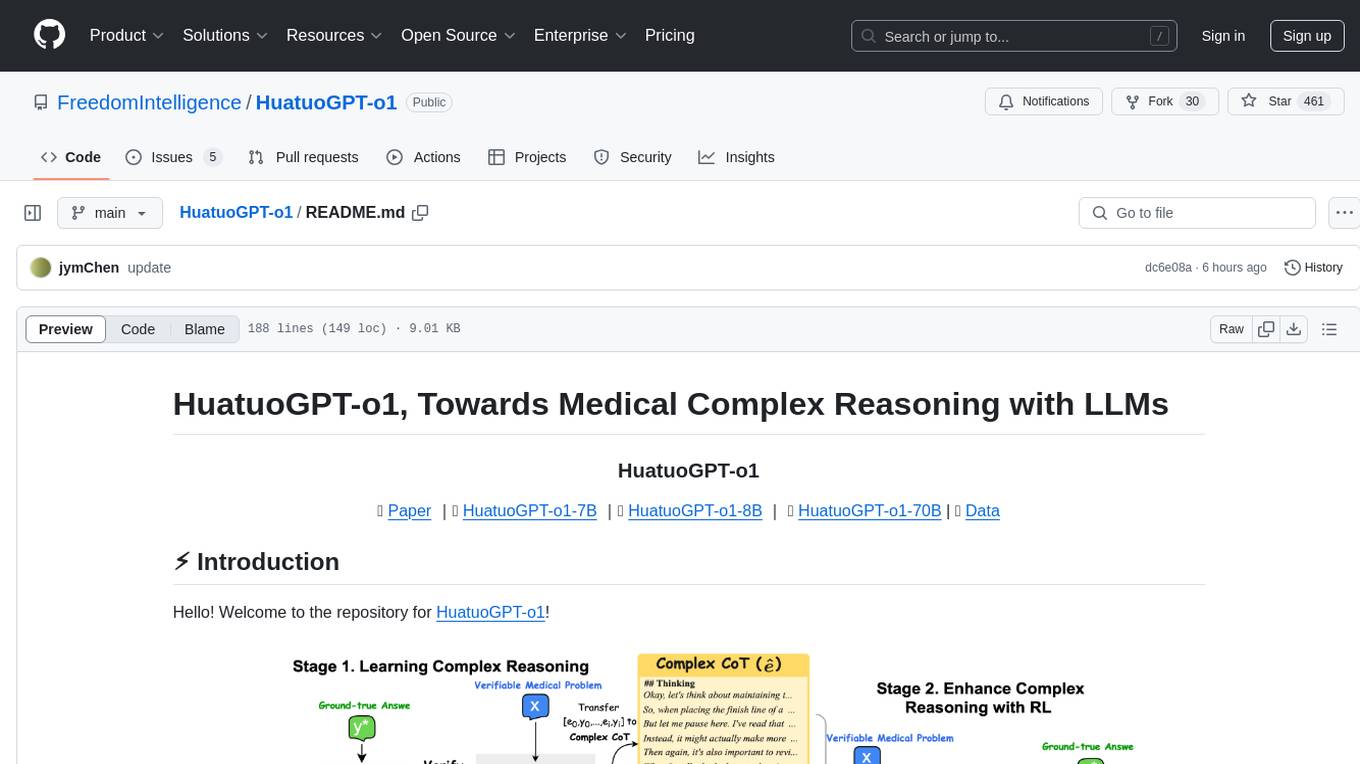
HuatuoGPT-o1
HuatuoGPT-o1 is a medical language model designed for advanced medical reasoning. It can identify mistakes, explore alternative strategies, and refine answers. The model leverages verifiable medical problems and a specialized medical verifier to guide complex reasoning trajectories and enhance reasoning through reinforcement learning. The repository provides access to models, data, and code for HuatuoGPT-o1, allowing users to deploy the model for medical reasoning tasks.
For similar jobs
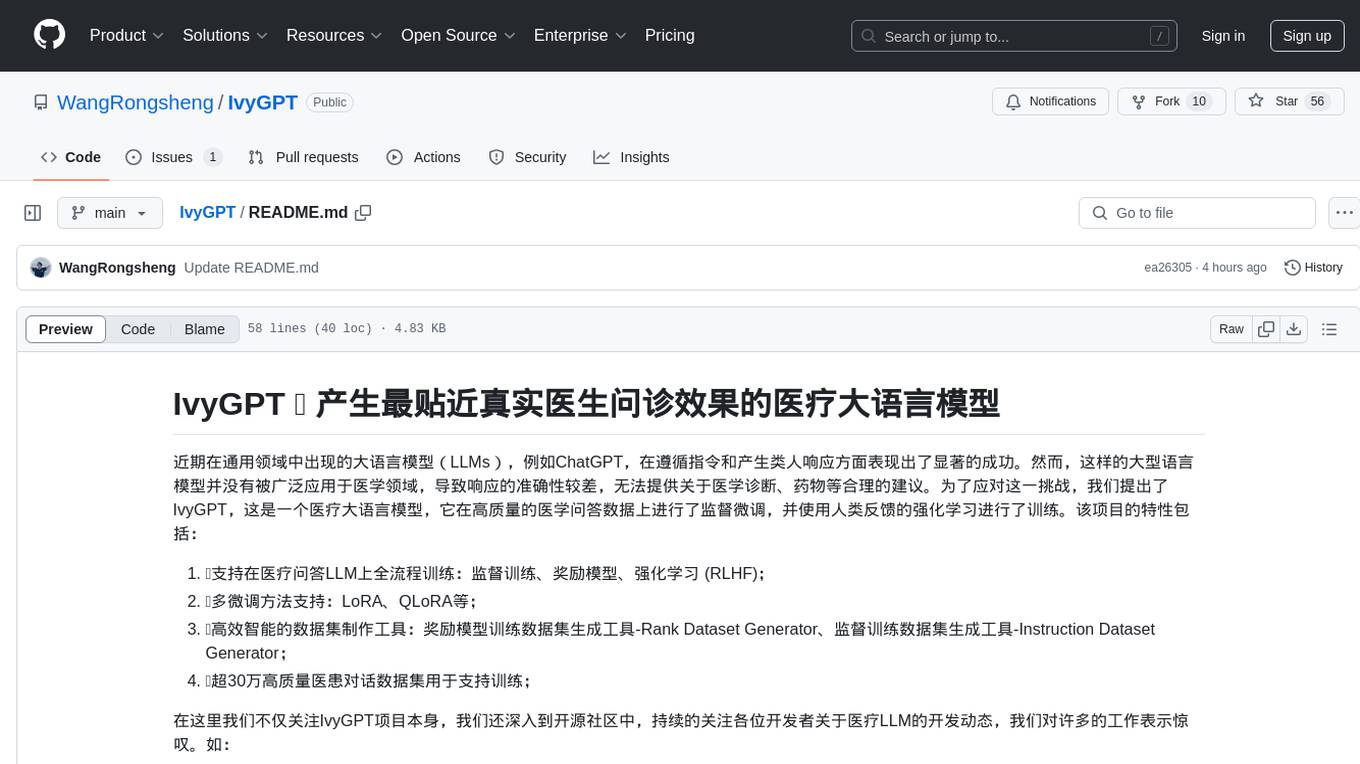
IvyGPT
IvyGPT is a medical large language model that aims to generate the most realistic doctor consultation effects. It has been fine-tuned on high-quality medical Q&A data and trained using human feedback reinforcement learning. The project features full-process training on medical Q&A LLM, multiple fine-tuning methods support, efficient dataset creation tools, and a dataset of over 300,000 high-quality doctor-patient dialogues for training.
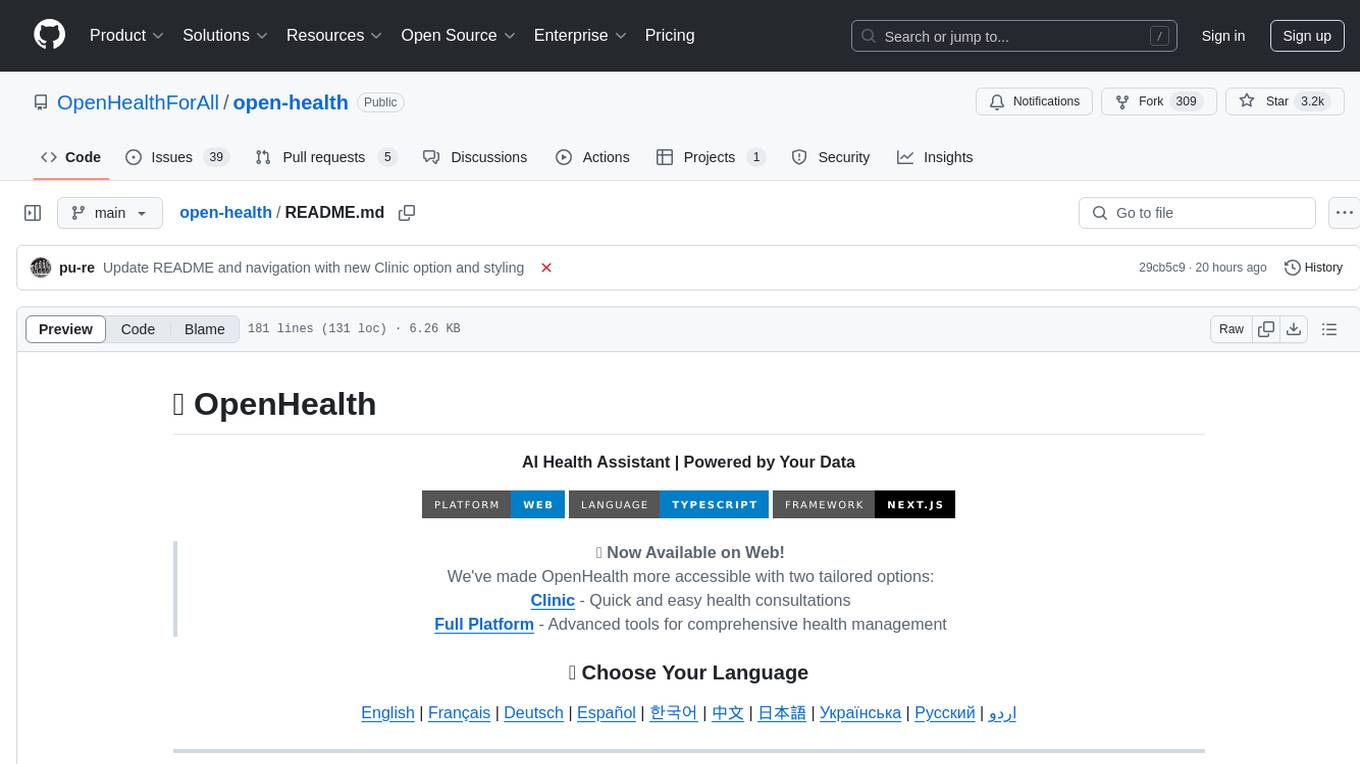
open-health
OpenHealth is an AI health assistant that helps users manage their health data by leveraging AI and personal health information. It allows users to consolidate health data, parse it smartly, and engage in contextual conversations with GPT-powered AI. The tool supports various data sources like blood test results, health checkup data, personal physical information, family history, and symptoms. OpenHealth aims to empower users to take control of their health by combining data and intelligence for actionable health management.
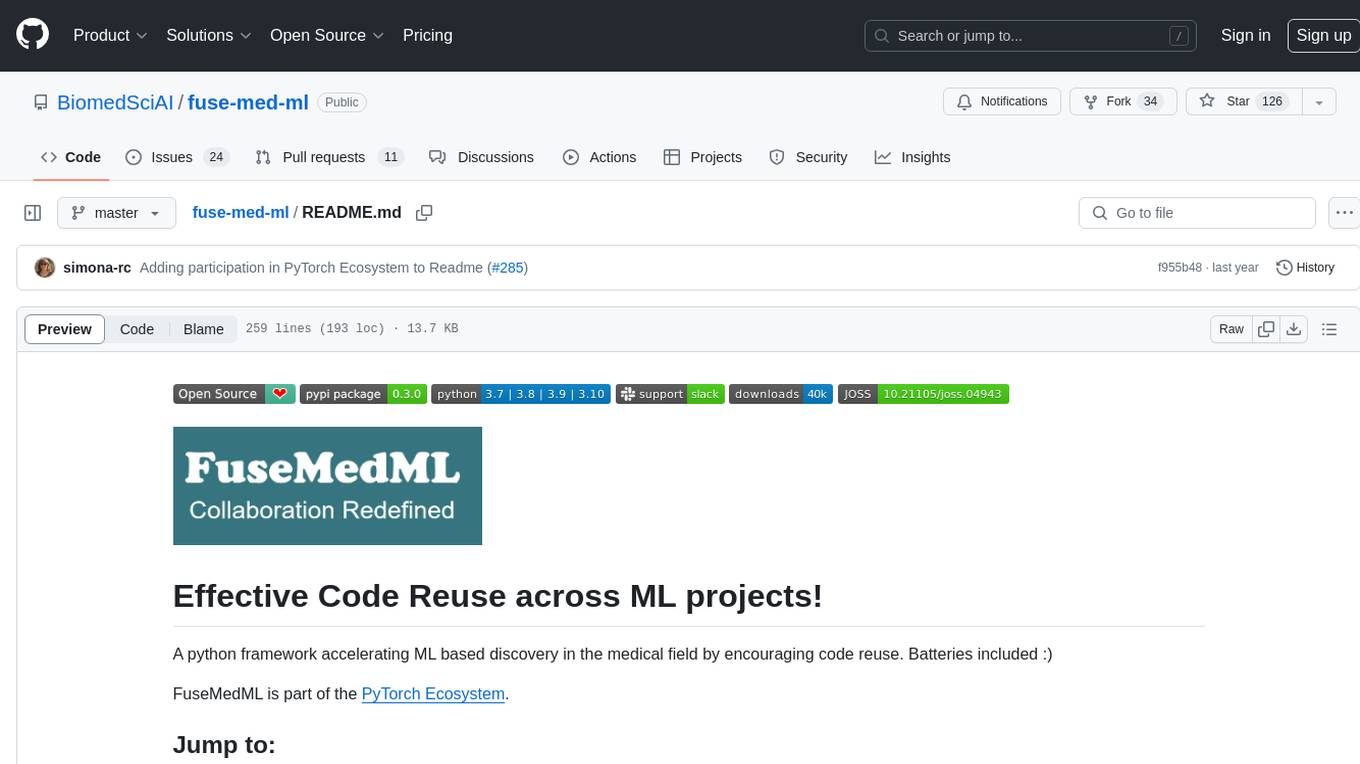
fuse-med-ml
FuseMedML is a Python framework designed to accelerate machine learning-based discovery in the medical field by promoting code reuse. It provides a flexible design concept where data is stored in a nested dictionary, allowing easy handling of multi-modality information. The framework includes components for creating custom models, loss functions, metrics, and data processing operators. Additionally, FuseMedML offers 'batteries included' key components such as fuse.data for data processing, fuse.eval for model evaluation, and fuse.dl for reusable deep learning components. It supports PyTorch and PyTorch Lightning libraries and encourages the creation of domain extensions for specific medical domains.
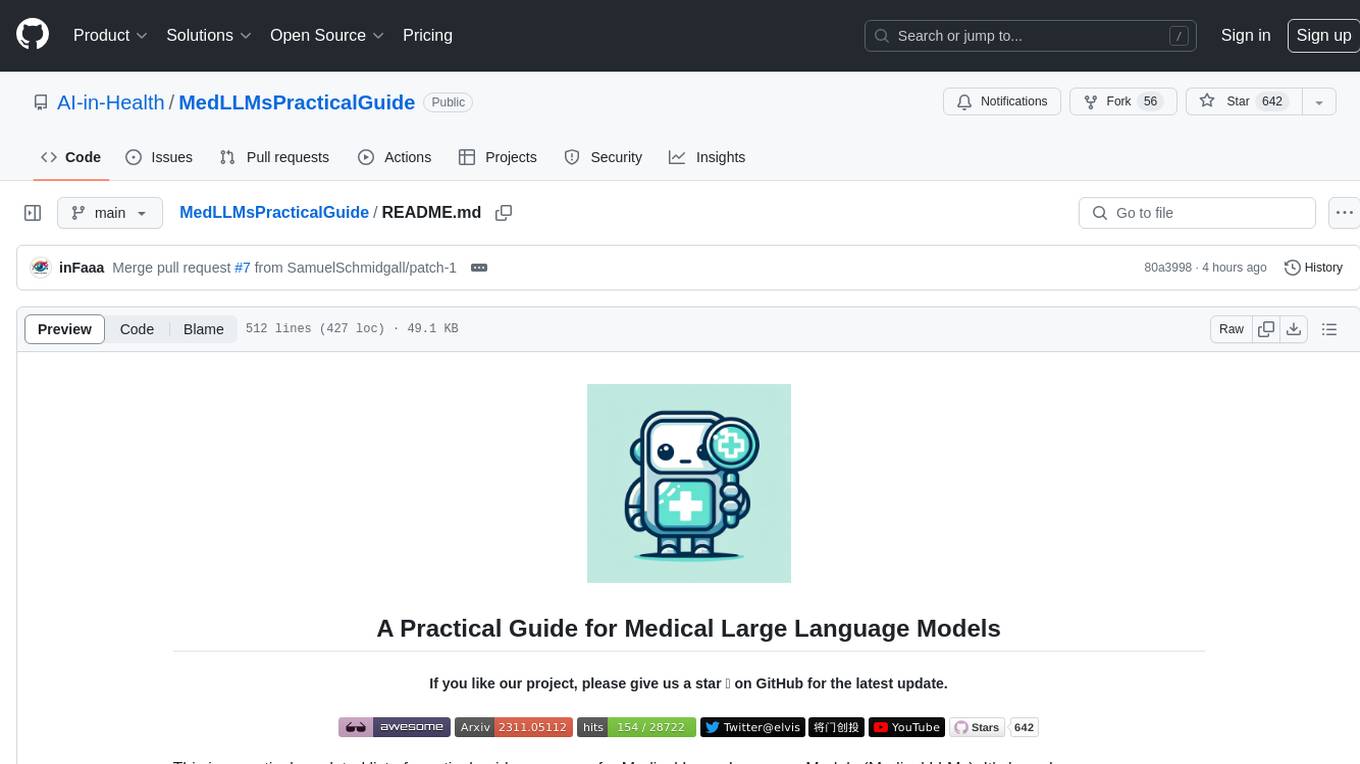
MedLLMsPracticalGuide
This repository serves as a practical guide for Medical Large Language Models (Medical LLMs) and provides resources, surveys, and tools for building, fine-tuning, and utilizing LLMs in the medical domain. It covers a wide range of topics including pre-training, fine-tuning, downstream biomedical tasks, clinical applications, challenges, future directions, and more. The repository aims to provide insights into the opportunities and challenges of LLMs in medicine and serve as a practical resource for constructing effective medical LLMs.
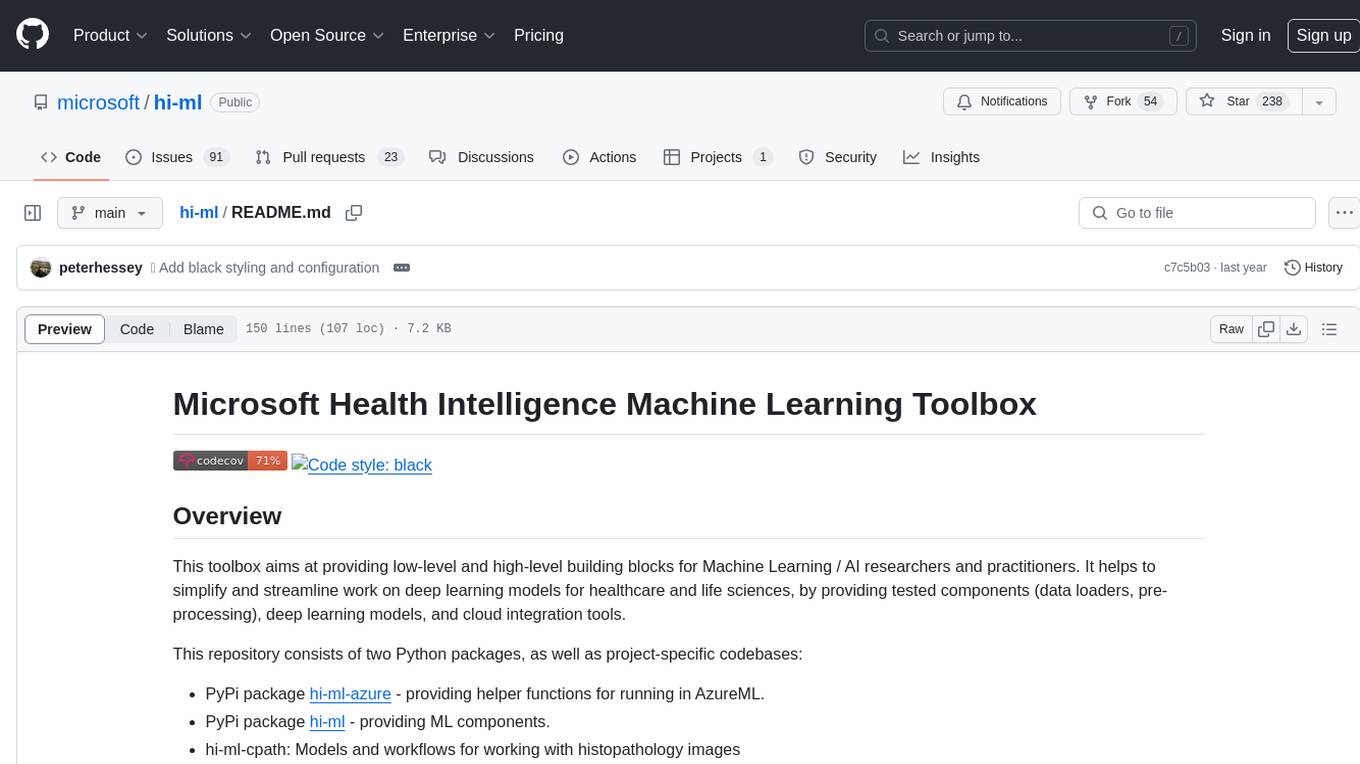
hi-ml
The Microsoft Health Intelligence Machine Learning Toolbox is a repository that provides low-level and high-level building blocks for Machine Learning / AI researchers and practitioners. It simplifies and streamlines work on deep learning models for healthcare and life sciences by offering tested components such as data loaders, pre-processing tools, deep learning models, and cloud integration utilities. The repository includes two Python packages, 'hi-ml-azure' for helper functions in AzureML, 'hi-ml' for ML components, and 'hi-ml-cpath' for models and workflows related to histopathology images.
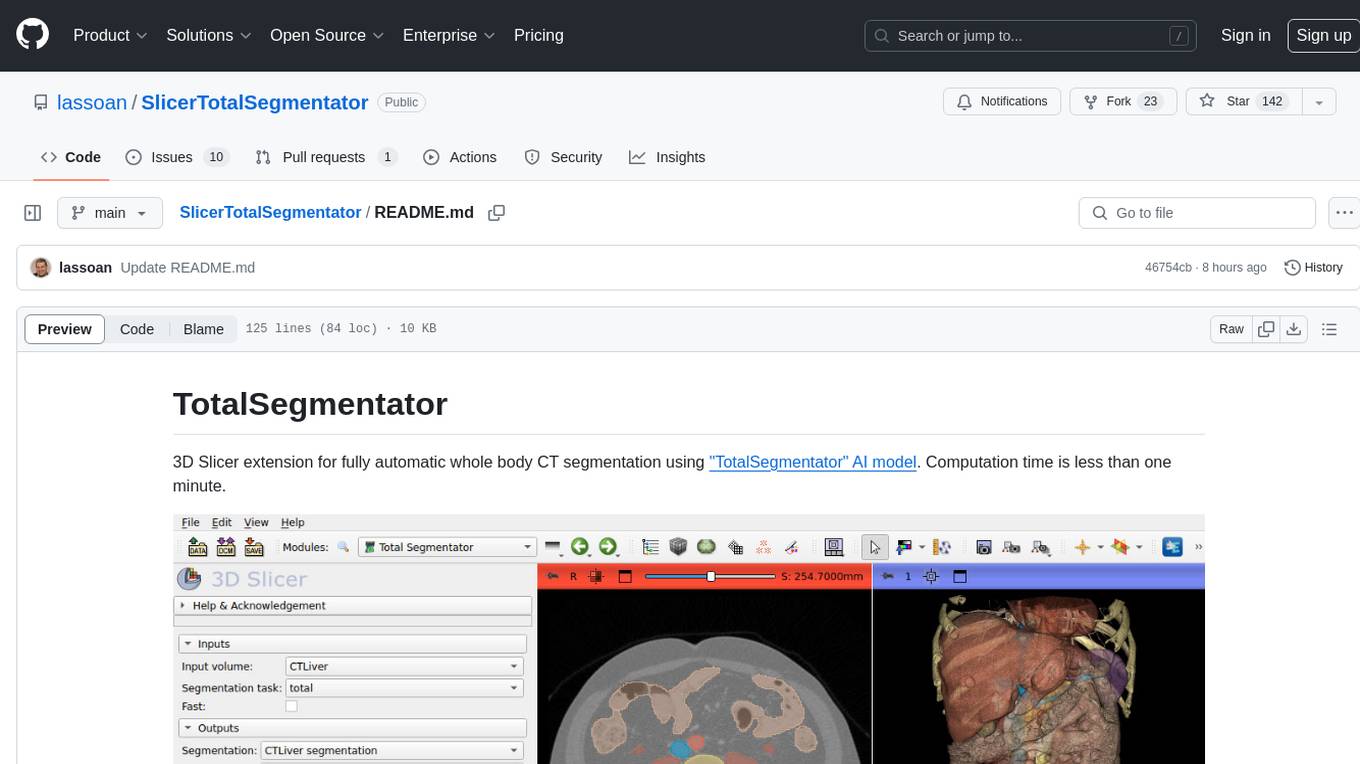
SlicerTotalSegmentator
TotalSegmentator is a 3D Slicer extension designed for fully automatic whole body CT segmentation using the 'TotalSegmentator' AI model. The computation time is less than one minute, making it efficient for research purposes. Users can set up GPU acceleration for faster segmentation. The tool provides a user-friendly interface for loading CT images, creating segmentations, and displaying results in 3D. Troubleshooting steps are available for common issues such as failed computation, GPU errors, and inaccurate segmentations. Contributions to the extension are welcome, following 3D Slicer contribution guidelines.
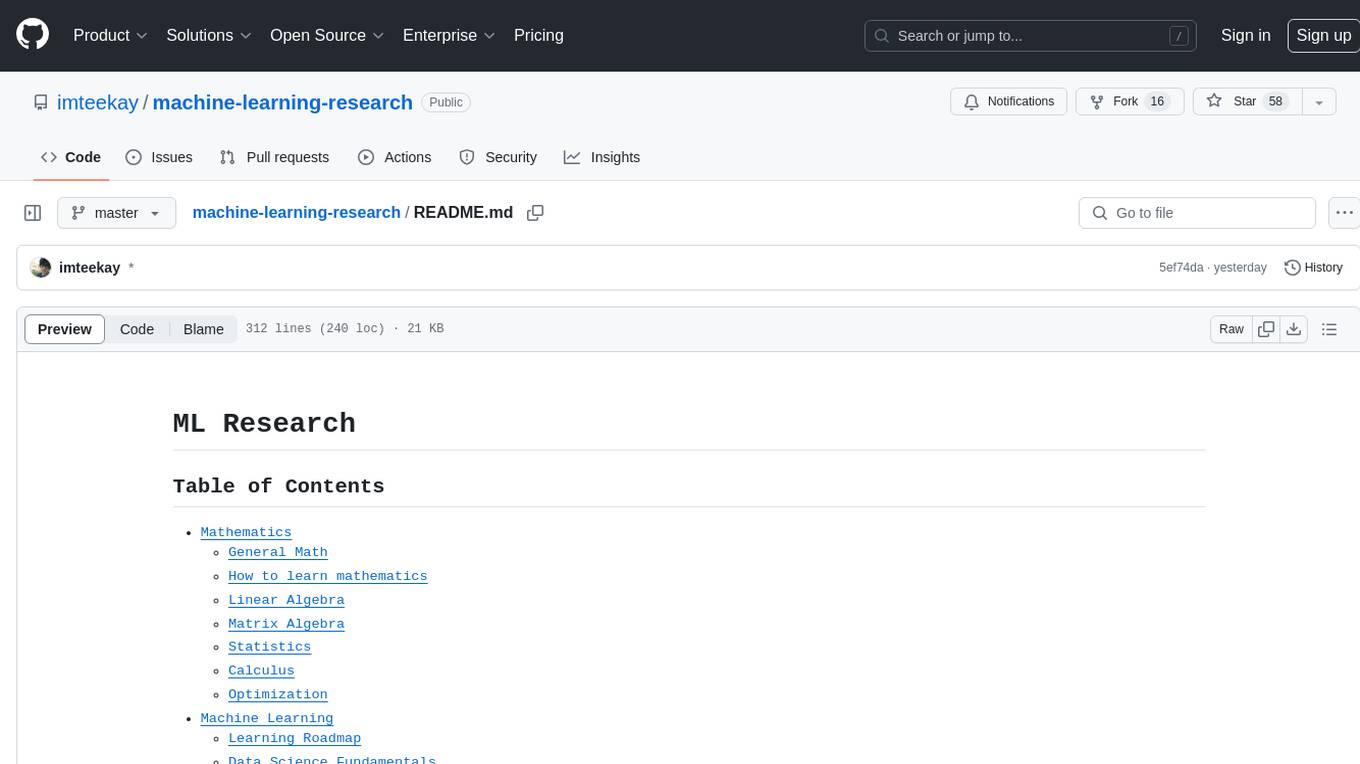
machine-learning-research
The 'machine-learning-research' repository is a comprehensive collection of resources related to mathematics, machine learning, deep learning, artificial intelligence, data science, and various scientific fields. It includes materials such as courses, tutorials, books, podcasts, communities, online courses, papers, and dissertations. The repository covers topics ranging from fundamental math skills to advanced machine learning concepts, with a focus on applications in healthcare, genetics, computational biology, precision health, and AI in science. It serves as a valuable resource for individuals interested in learning and researching in the fields of machine learning and related disciplines.
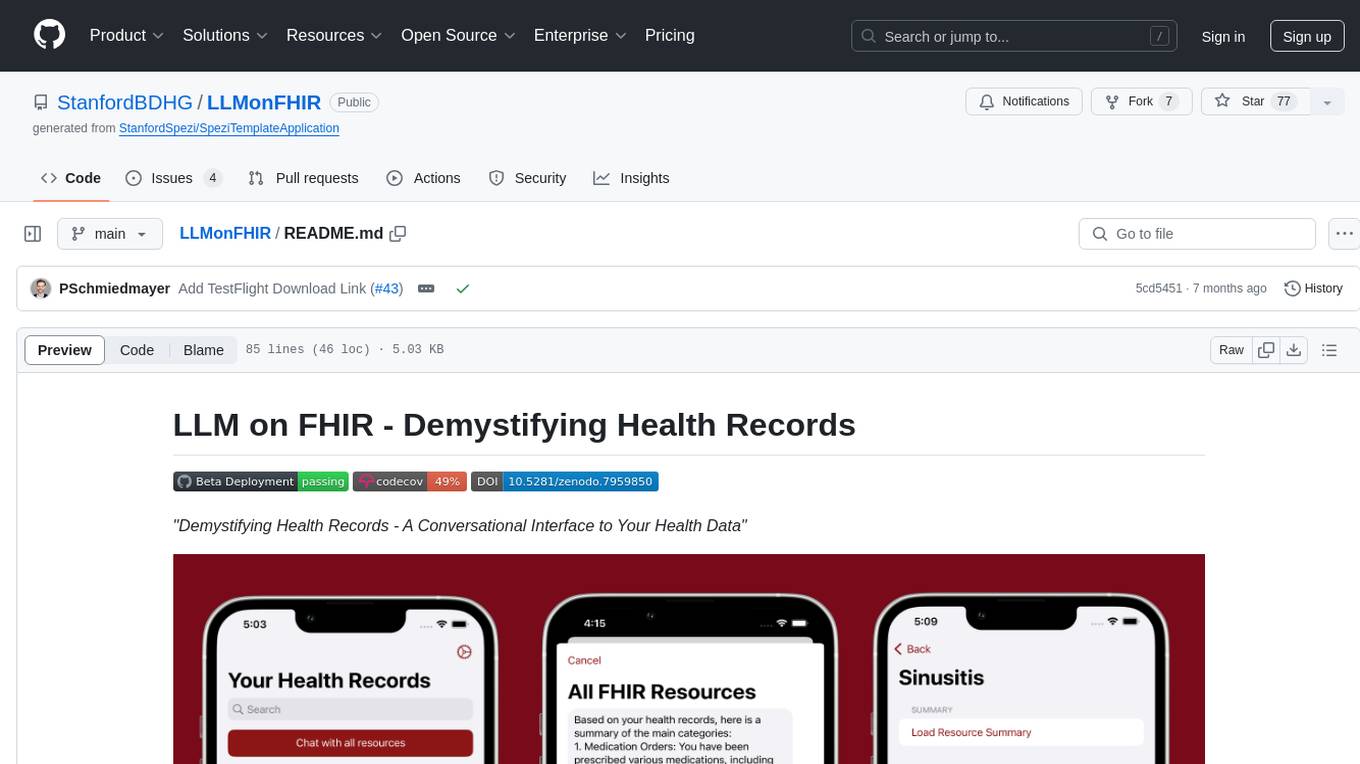
LLMonFHIR
LLMonFHIR is an iOS application that utilizes large language models (LLMs) to interpret and provide context around patient data in the Fast Healthcare Interoperability Resources (FHIR) format. It connects to the OpenAI GPT API to analyze FHIR resources, supports multiple languages, and allows users to interact with their health data stored in the Apple Health app. The app aims to simplify complex health records, provide insights, and facilitate deeper understanding through a conversational interface. However, it is an experimental app for informational purposes only and should not be used as a substitute for professional medical advice. Users are advised to verify information provided by AI models and consult healthcare professionals for personalized advice.