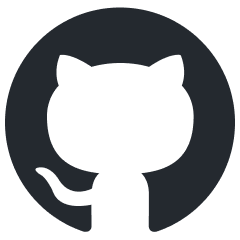
dash-infer
DashInfer is a native LLM inference engine aiming to deliver industry-leading performance atop various hardware architectures, including CUDA, x86 and ARMv9.
Stars: 237
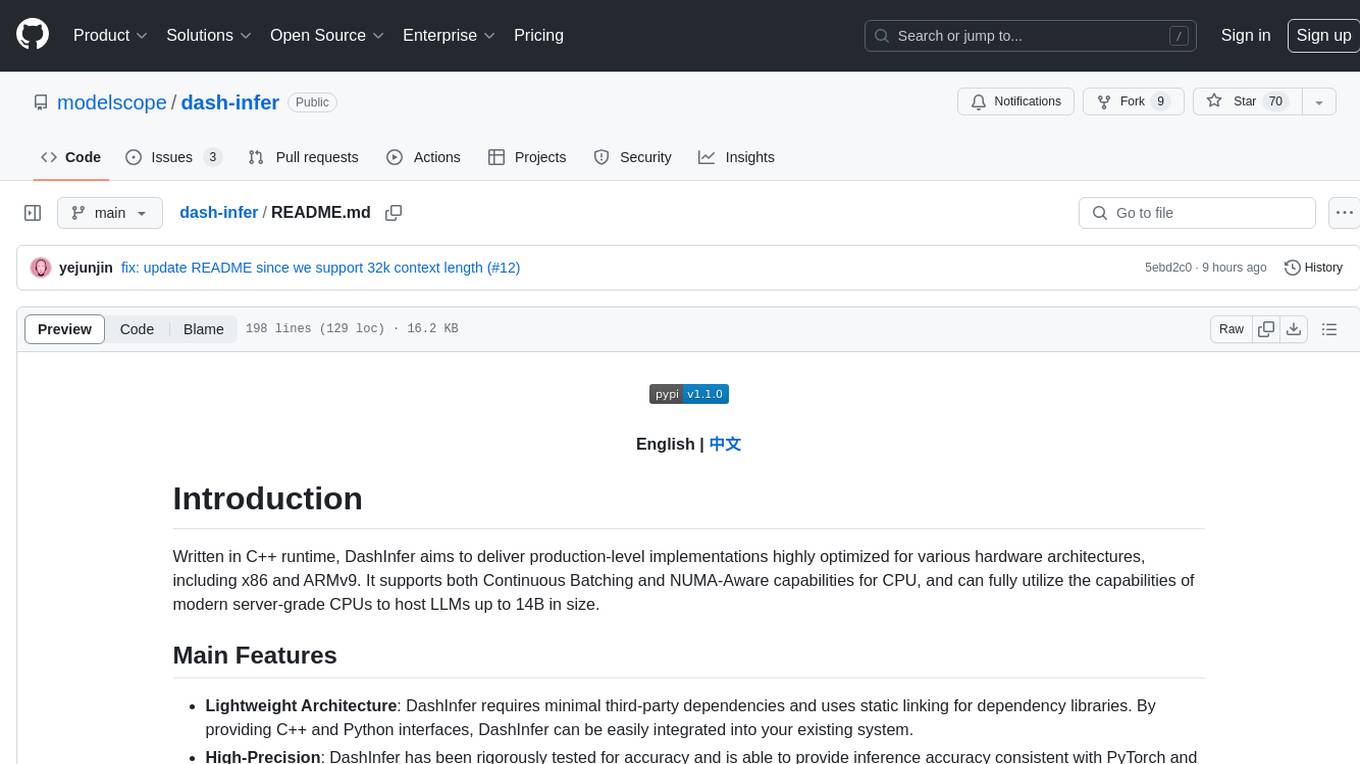
DashInfer is a C++ runtime tool designed to deliver production-level implementations highly optimized for various hardware architectures, including x86 and ARMv9. It supports Continuous Batching and NUMA-Aware capabilities for CPU, and can fully utilize modern server-grade CPUs to host large language models (LLMs) up to 14B in size. With lightweight architecture, high precision, support for mainstream open-source LLMs, post-training quantization, optimized computation kernels, NUMA-aware design, and multi-language API interfaces, DashInfer provides a versatile solution for efficient inference tasks. It supports x86 CPUs with AVX2 instruction set and ARMv9 CPUs with SVE instruction set, along with various data types like FP32, BF16, and InstantQuant. DashInfer also offers single-NUMA and multi-NUMA architectures for model inference, with detailed performance tests and inference accuracy evaluations available. The tool is supported on mainstream Linux server operating systems and provides documentation and examples for easy integration and usage.
README:
-
[2024/12] 🔥 DashInfer: Announcing the release of v2.0, now with enhanced GPU (CUDA) support! This version includes features like prefix caching (with GPU & CPU swapping), guided decoding, optimized attention for GQA, a lockless reactor engine, and newly added support for the VLM model (Qwen-VL) and MoE Models. For more details, please refer to the release notes.
-
[2024/06] DashInfer: v1.0 release with x86 & ARMv9 CPU and CPU flash attention support.
Written in C++ runtime, DashInfer aims to deliver production-level implementations highly optimized for various hardware architectures, including CUDA, x86 and ARMv9.
DashInfer is a highly optimized LLM inference engine with the following core features:
-
Lightweight Architecture: DashInfer requires minimal third-party dependencies and uses static linking for almost all dependency libraries. By providing C++ and Python interfaces, DashInfer can be easily integrated into your existing system.
-
High Precision: DashInfer has been rigorously tested to ensure accuracy, and is able to provide inference whose accuracy is consistent with PyTorch and other GPU engines (e.g., vLLM).
-
High Performance: DashInfer employs optmized kernels to provide high-performance LLM serving, as well as lots of standard LLM inference techniques, including:
-
Continuous Batching: DashInfer allows for the immediate insertion of new requests and supports streaming outputs.
-
Paged Attention: Using our self-developed paged attention technique (which we call SpanAttention), we can achieve efficient acceleration of attention operator, combined with int8 and uint4 KV cache quantization, based on highly efficient GEMM and GEMV implementations.
-
Prefix Cache: DashInfer supports highly efficient Prefix Cache for prompts, which accelerates standard LLMs and MultiModal LMs (MMLMs) like Qwen-VL, using both GPU and CPU.
-
Quantization Support: Using DashInfer's InstantQuant (IQ), weight-only quantization acceleration can be achieved without fine-tuning, improving deployment efficiency. Accuracy evaluation shows that IQ has almost no impact on model accuracy, for detail, see :doc:
quant/weight_activate_quant
. -
Asynchronous Interface: Request-based asynchronous interfaces offer individual control over generation parameters and request status of each request.
-
-
Supported Models:
-
Mainstream Open-Source LLMs: DashInfer supports mainstream open-source LLMs including Qwen, LLaMA, ChatGLM, etc., and supports loading models in the Huggingface format.
-
MultiModal LMs: DashInfer supports MultiModal Language Models (MMLMs) including Qwen-VL, Qwen-AL, and Qwen2-VL.
-
-
OpenAI API Server: DashInfer can easily serve with fastChat to achieve OpenAI-compatible API server.
-
Multi-Programming-Language API: Both C++ and Python interfaces are provided. It is possible to extend C++ interface to Java, Rust and other programming languages, via standard cross-language interfaces.
- CUDA GPUs: Support CUDA Version from 11.4 - 12.4, and supports various CUDA compute architectures like SM70 - SM90a (T4, 3090, 4090, V100, A100, A10, L20, H20, H100)
- x86 CPUs: Hardware support for AVX2 instruction set is required. For Intel's 5th generation Xeon processors (Emerald Rapids), 4th generation Xeon processors (Sapphire Rapids), corresponding to Aliyun's 8th generation ECS instances (e.g., g8i), AMX instructions are used to accelerate caculation.
- ARMv9 CPU: Hardware support for SVE instruction set is required. DashInfer supports ARMv9 architecture processors such as Yitian710, corresponding to Aliyun's 8th generation ECS instances (e.g. g8y), and adopts SVE instruction to accelerate caculation.
- CUDA GPUs: FP16, BF16, FP8, FP32, Int8(InstantQuant), Int4(InstantQuant)
- x86 CPU: FP32, BF16
- ARM Yitian710 CPU: FP32, BF16, Int8(InstantQuant)
DashInfer provides various many quantization technology for LLM weight, such as, int{8,4} weight only quantization, int8 activate quantization, and many customized fused kernel to provide best performance on specified device.
To put it simply, models fine-tuned with GPTQ will provide better accuracy, but our InstantQuant (IQ) technique, which does not require fine-tuning, can offer a faster deployment experience. Detailed explanations of IQ quantization can be found at the end of this article.
In terms of supported quantization algorithms, DashInfer supports models fine-tuned with GPTQ and dynamic quantization using the IQ quantization technique in two ways:
- IntantQuant(IQ): DashInfer provides the InstantQuant (IQ) dynamic quantization technique, which does not require fine-tuning and can offer a faster deployment experience. Detailed explanations of IQ quantization can be found at the end of this article.
- GPTQ: Models fine-tuned with GPTQ will provide better accuracy, but it requires a fine-tuning step.
The quantization strategies introduced here can be broadly divided into two categories:
- Weight Only Quantization: This quantization technique only quantizes and compresses the weights, such as storing weights in int8 format, but uses bf16/fp16 for computations. It only reduces memory access requirements, without improving computational performance compared to BF16.
- Activation Quantization: This quantization technique not only stores weights in int8 format but also performs low-precision quantized computations (such as int8) during the calculation phase. (Since Nvidia GPUs only have int8 Tensor Cores that can easily maintain precision, this quantization technique can reduce memory access requirements and improve computational performance, making it a more ideal quantization approach. In terms of accuracy, it may have a slight decrease compared to Weight Only quantization, so business data accuracy testing is required.
In terms of quantization granularity, there are two types:
- Per-Channel: DashInfer's quantization techniques at least adopt the Per-Channel (also known as Per-Token) quantization granularity, and some also provide Sub-Channel quantization granularity. Generally speaking, Per-Channel quantization can meet most accuracy requirements due to its simple implementation and optimal performance. Only when the accuracy of Per-Channel quantization is insufficient should the Sub-Channel quantization strategy be considered.
- Sub-Channel: Compared to Per-Channel quantization, Sub-Channel refers to dividing a channel into N groups, and calculating quantization parameters within each group. This quantization granularity typically provides better accuracy, but due to increased implementation complexity, it comes with many limitations. For example, performance may be slightly slower than Per-Channel quantization, and Activation quantization is difficult to implement Sub-Channel quantization due to computational formula constraints (DashInfer's Activation quantization is all Per-Channel).
For the detailed user manual, please refer to the documentation: Documentation Link.
- Using API Python Quick Start
- LLM OpenAI Server Quick Start Guide for OpenAI API Server
- VLM OpenAI Server VLM Support
In <path_to_dashinfer>/examples
there are examples for C++ and Python interfaces, and please refer to the documentation in <path_to_dashinfer>/documents/EN
to run the examples.
The VLM Support in multimodal folder, it's a toolkit to support Vision Language Models (VLMs) inference based on the DashInfer engine. It's compatible with the OpenAI Chat Completion API, supporting text and image/video inputs.
We have conducted several benchmarks to compare the performance of mainstream LLM inference engines.
We compared the performance of Qwen-VL with vllm across various model sizes:
Benchmarks were conducted using an A100-80Gx1 for 2B and 7B sizes, and an A100-80Gx4 for the 72B model. For more details, please refer to the benchmark documentation.
We evaluated the performance of the prefix cache at different cache hit rates:
The chart above shows the reduction in TTFT (Time to First Token) with varying PrefixCache hit rates in DashInfer.
Test Setup:
- Model: Qwen2-72B-Instruct
- GPU: 4x A100
- Runs: 20
- Batch Size: 1
- Input Tokens: 4000
- Output Tokens: 1
We compared the guided output (in JSON format) between different engines using the same request with a customized JSON schema (Context Length: 45, Generated Length: 63):
- HIE-DNN: an operator library for high-performance inference of deep neural network (DNN).
- SpanAttention: a high-performance decode-phase attention with paged KV cache for LLM inference on CUDA-enabled devices.
The high-performance implementation of DashInfer MoE operator is introduced in this paper, and DashInfer employs the efficient top-k operator RadiK. If you find them useful, please feel free to cite these papers:
@misc{dashinfermoe2025,
title = {Static Batching of Irregular Workloads on GPUs: Framework and Application to Efficient MoE Model Inference},
author = {Yinghan Li and Yifei Li and Jiejing Zhang and Bujiao Chen and Xiaotong Chen and Lian Duan and Yejun Jin and Zheng Li and Xuanyu Liu and Haoyu Wang and Wente Wang and Yajie Wang and Jiacheng Yang and Peiyang Zhang and Laiwen Zheng and Wenyuan Yu},
year = {2025},
eprint = {2501.16103},
archivePrefix = {arXiv},
primaryClass = {cs.DC},
url = {https://arxiv.org/abs/2501.16103}
}
@inproceedings{radik2024,
title = {RadiK: Scalable and Optimized GPU-Parallel Radix Top-K Selection},
author = {Li, Yifei and Zhou, Bole and Zhang, Jiejing and Wei, Xuechao and Li, Yinghan and Chen, Yingda},
booktitle = {Proceedings of the 38th ACM International Conference on Supercomputing},
year = {2024}
}
- [x] GPU Support
- [x] Multi Modal Model support
- [x] Accelerate attention with Flash-Attention
- [x] Expand context length to over 32k
- [x] Support 4-bit quantization
- [x] Support quantized models fine-tuned with GPTQ
- [x] Support MoE architecture
- [x] Guided output: Json Mode
- [x] Prefix Cache: Support GPU Prefix Cache and CPU Swap
- [x] Quantization: Fp8 A8W8 Activation quantization support on CUDA.
- [x] LORA: Continues Batch LORA Optimization.
- [ ] Parallel Context phase and Generation phase within engine.
- [ ] More effective MoE Operator on GPU.
- [ ] Porting to AMD(ROCm) Platform.
The DashInfer source code is licensed under the Apache 2.0 license, and you can find the full text of the license in the root of the repository.
For Tasks:
Click tags to check more tools for each tasksFor Jobs:
Alternative AI tools for dash-infer
Similar Open Source Tools
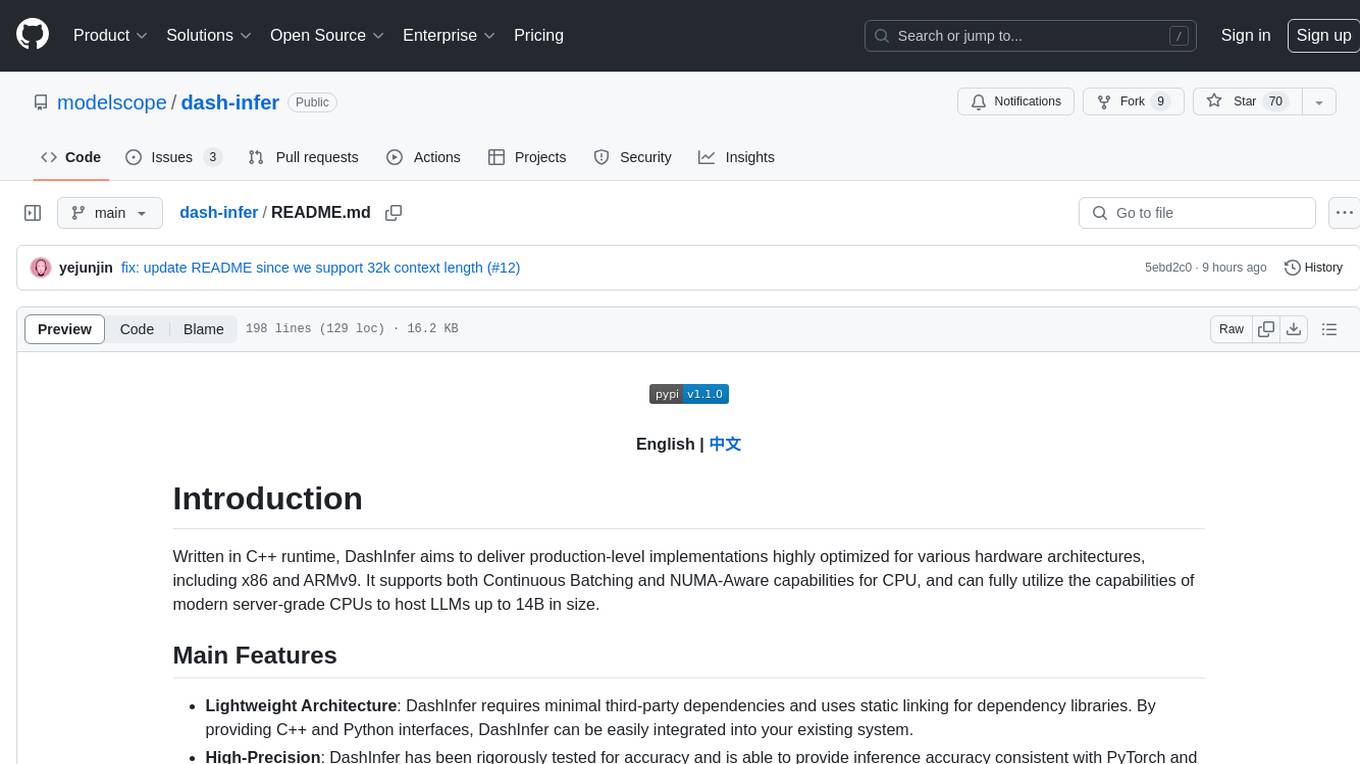
dash-infer
DashInfer is a C++ runtime tool designed to deliver production-level implementations highly optimized for various hardware architectures, including x86 and ARMv9. It supports Continuous Batching and NUMA-Aware capabilities for CPU, and can fully utilize modern server-grade CPUs to host large language models (LLMs) up to 14B in size. With lightweight architecture, high precision, support for mainstream open-source LLMs, post-training quantization, optimized computation kernels, NUMA-aware design, and multi-language API interfaces, DashInfer provides a versatile solution for efficient inference tasks. It supports x86 CPUs with AVX2 instruction set and ARMv9 CPUs with SVE instruction set, along with various data types like FP32, BF16, and InstantQuant. DashInfer also offers single-NUMA and multi-NUMA architectures for model inference, with detailed performance tests and inference accuracy evaluations available. The tool is supported on mainstream Linux server operating systems and provides documentation and examples for easy integration and usage.
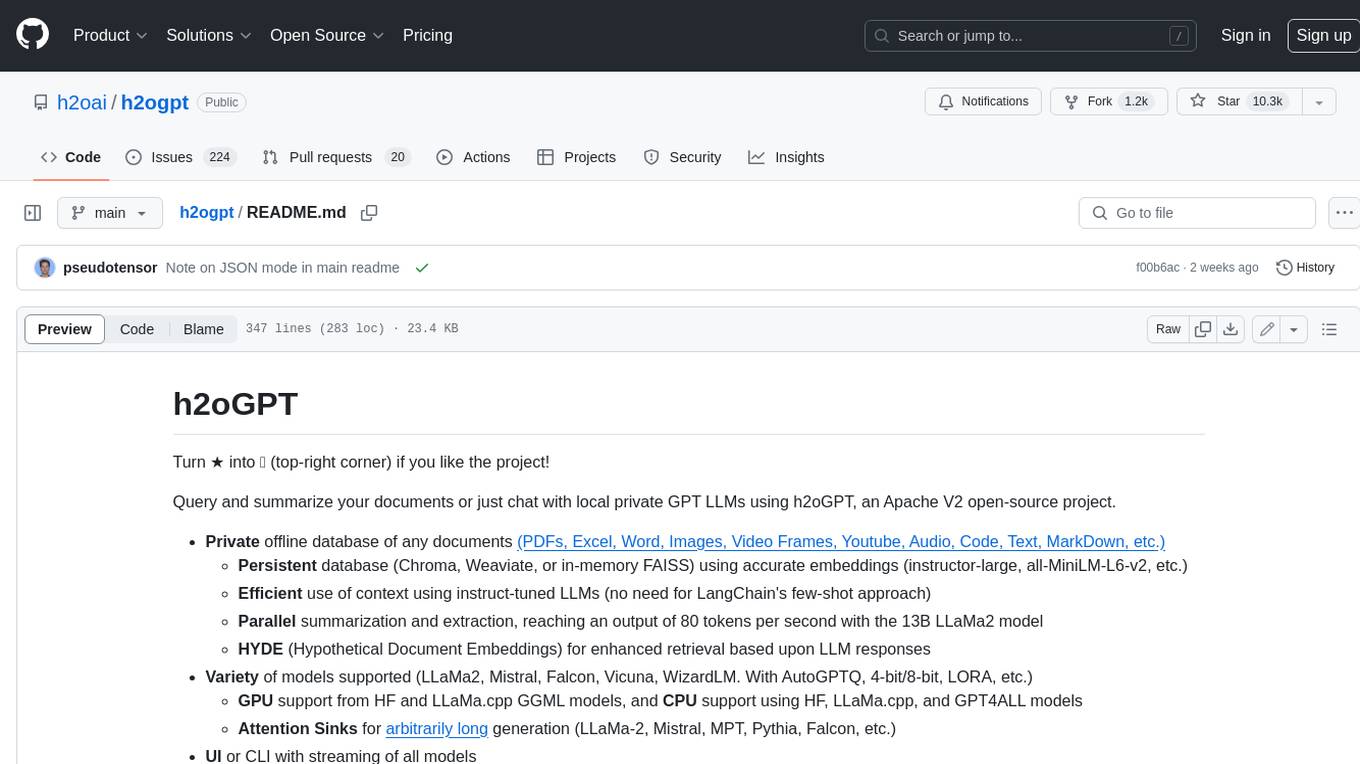
h2ogpt
h2oGPT is an Apache V2 open-source project that allows users to query and summarize documents or chat with local private GPT LLMs. It features a private offline database of any documents (PDFs, Excel, Word, Images, Video Frames, Youtube, Audio, Code, Text, MarkDown, etc.), a persistent database (Chroma, Weaviate, or in-memory FAISS) using accurate embeddings (instructor-large, all-MiniLM-L6-v2, etc.), and efficient use of context using instruct-tuned LLMs (no need for LangChain's few-shot approach). h2oGPT also offers parallel summarization and extraction, reaching an output of 80 tokens per second with the 13B LLaMa2 model, HYDE (Hypothetical Document Embeddings) for enhanced retrieval based upon LLM responses, a variety of models supported (LLaMa2, Mistral, Falcon, Vicuna, WizardLM. With AutoGPTQ, 4-bit/8-bit, LORA, etc.), GPU support from HF and LLaMa.cpp GGML models, and CPU support using HF, LLaMa.cpp, and GPT4ALL models. Additionally, h2oGPT provides Attention Sinks for arbitrarily long generation (LLaMa-2, Mistral, MPT, Pythia, Falcon, etc.), a UI or CLI with streaming of all models, the ability to upload and view documents through the UI (control multiple collaborative or personal collections), Vision Models LLaVa, Claude-3, Gemini-Pro-Vision, GPT-4-Vision, Image Generation Stable Diffusion (sdxl-turbo, sdxl) and PlaygroundAI (playv2), Voice STT using Whisper with streaming audio conversion, Voice TTS using MIT-Licensed Microsoft Speech T5 with multiple voices and Streaming audio conversion, Voice TTS using MPL2-Licensed TTS including Voice Cloning and Streaming audio conversion, AI Assistant Voice Control Mode for hands-free control of h2oGPT chat, Bake-off UI mode against many models at the same time, Easy Download of model artifacts and control over models like LLaMa.cpp through the UI, Authentication in the UI by user/password via Native or Google OAuth, State Preservation in the UI by user/password, Linux, Docker, macOS, and Windows support, Easy Windows Installer for Windows 10 64-bit (CPU/CUDA), Easy macOS Installer for macOS (CPU/M1/M2), Inference Servers support (oLLaMa, HF TGI server, vLLM, Gradio, ExLLaMa, Replicate, OpenAI, Azure OpenAI, Anthropic), OpenAI-compliant, Server Proxy API (h2oGPT acts as drop-in-replacement to OpenAI server), Python client API (to talk to Gradio server), JSON Mode with any model via code block extraction. Also supports MistralAI JSON mode, Claude-3 via function calling with strict Schema, OpenAI via JSON mode, and vLLM via guided_json with strict Schema, Web-Search integration with Chat and Document Q/A, Agents for Search, Document Q/A, Python Code, CSV frames (Experimental, best with OpenAI currently), Evaluate performance using reward models, and Quality maintained with over 1000 unit and integration tests taking over 4 GPU-hours.
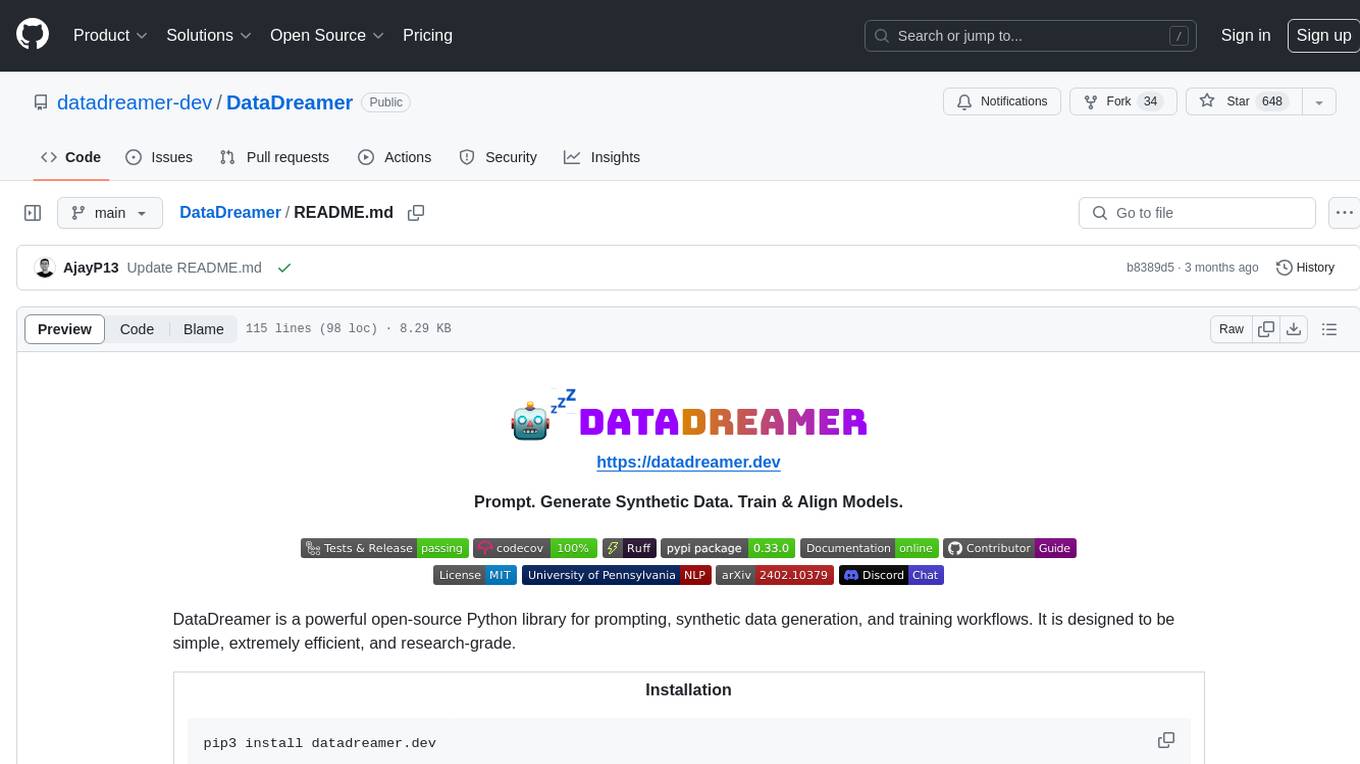
DataDreamer
DataDreamer is a powerful open-source Python library designed for prompting, synthetic data generation, and training workflows. It is simple, efficient, and research-grade, allowing users to create prompting workflows, generate synthetic datasets, and train models with ease. The library is built for researchers, by researchers, focusing on correctness, best practices, and reproducibility. It offers features like aggressive caching, resumability, support for bleeding-edge techniques, and easy sharing of datasets and models. DataDreamer enables users to run multi-step prompting workflows, generate synthetic datasets for various tasks, and train models by aligning, fine-tuning, instruction-tuning, and distilling them using existing or synthetic data.
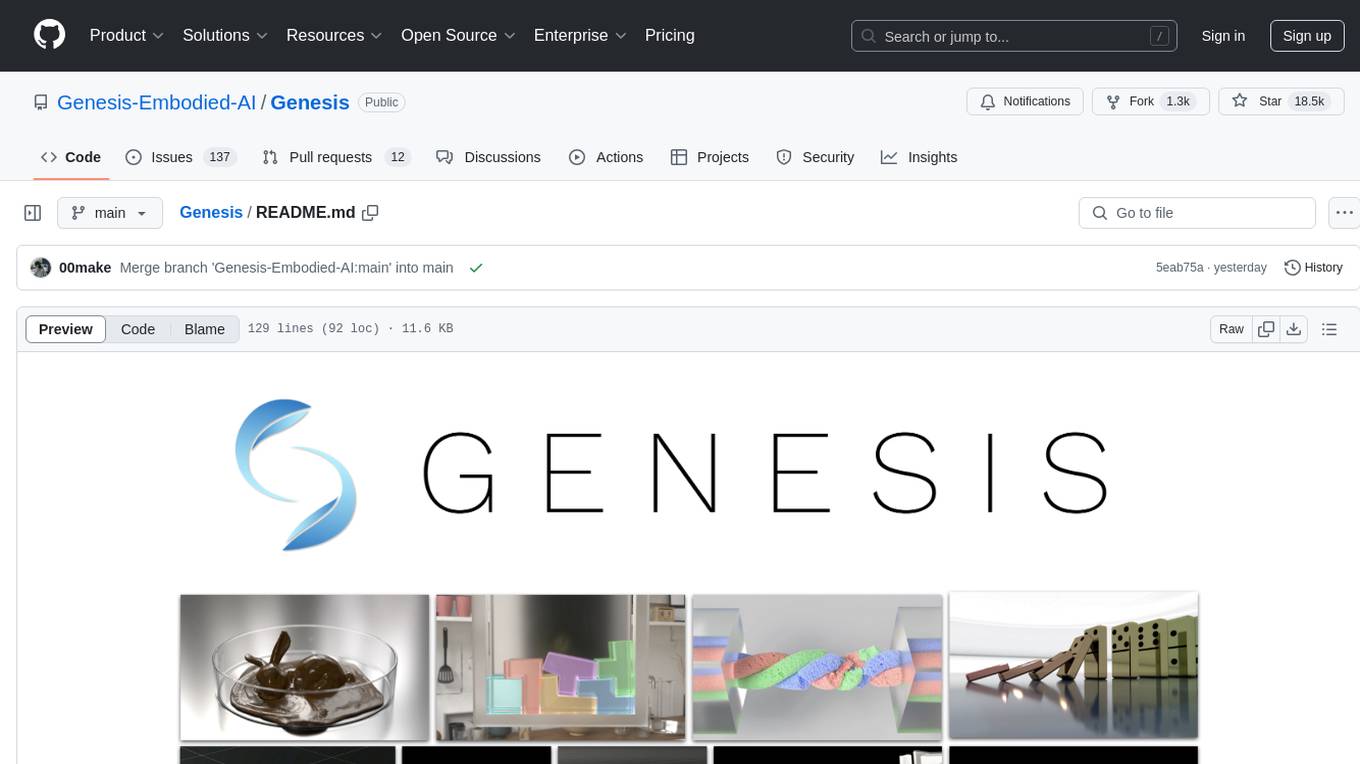
Genesis
Genesis is a physics platform designed for general purpose Robotics/Embodied AI/Physical AI applications. It includes a universal physics engine, a lightweight, ultra-fast, pythonic, and user-friendly robotics simulation platform, a powerful and fast photo-realistic rendering system, and a generative data engine that transforms user-prompted natural language description into various modalities of data. It aims to lower the barrier to using physics simulations, unify state-of-the-art physics solvers, and minimize human effort in collecting and generating data for robotics and other domains.
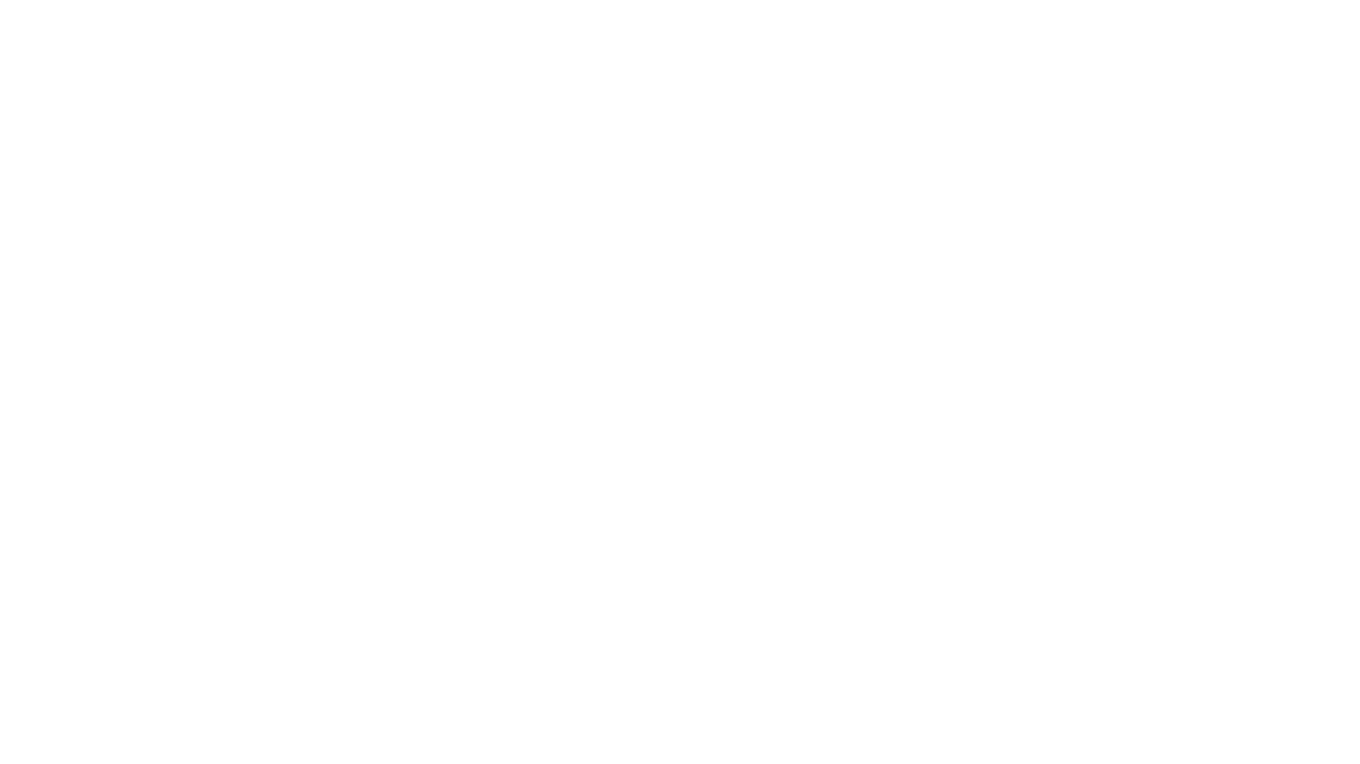
only_train_once
Only Train Once (OTO) is an automatic, architecture-agnostic DNN training and compression framework that allows users to train a general DNN from scratch or a pretrained checkpoint to achieve high performance and slimmer architecture simultaneously in a one-shot manner without fine-tuning. The framework includes features for automatic structured pruning and erasing operators, as well as hybrid structured sparse optimizers for efficient model compression. OTO provides tools for pruning zero-invariant group partitioning, constructing pruned models, and visualizing pruning and erasing dependency graphs. It supports the HESSO optimizer and offers a sanity check for compliance testing on various DNNs. The repository also includes publications, installation instructions, quick start guides, and a roadmap for future enhancements and collaborations.
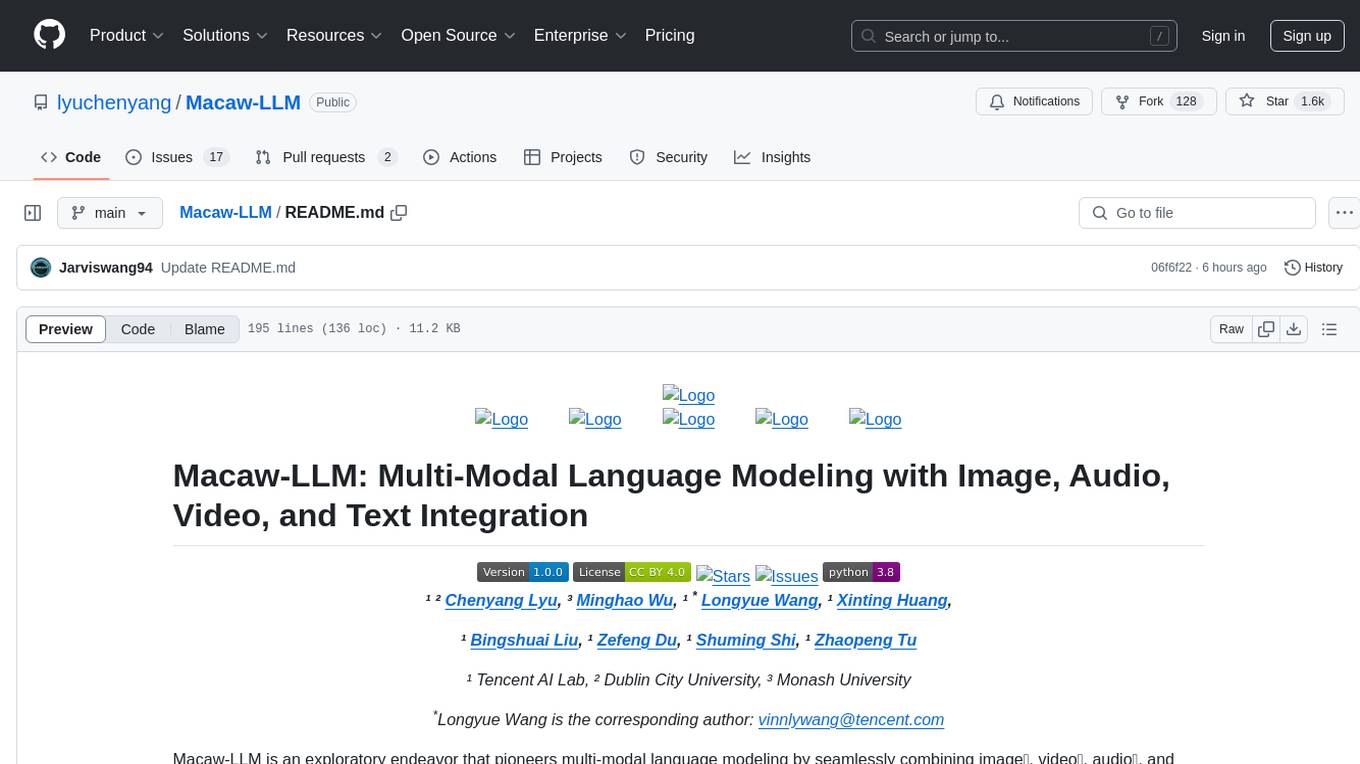
Macaw-LLM
Macaw-LLM is a pioneering multi-modal language modeling tool that seamlessly integrates image, audio, video, and text data. It builds upon CLIP, Whisper, and LLaMA models to process and analyze multi-modal information effectively. The tool boasts features like simple and fast alignment, one-stage instruction fine-tuning, and a new multi-modal instruction dataset. It enables users to align multi-modal features efficiently, encode instructions, and generate responses across different data types.
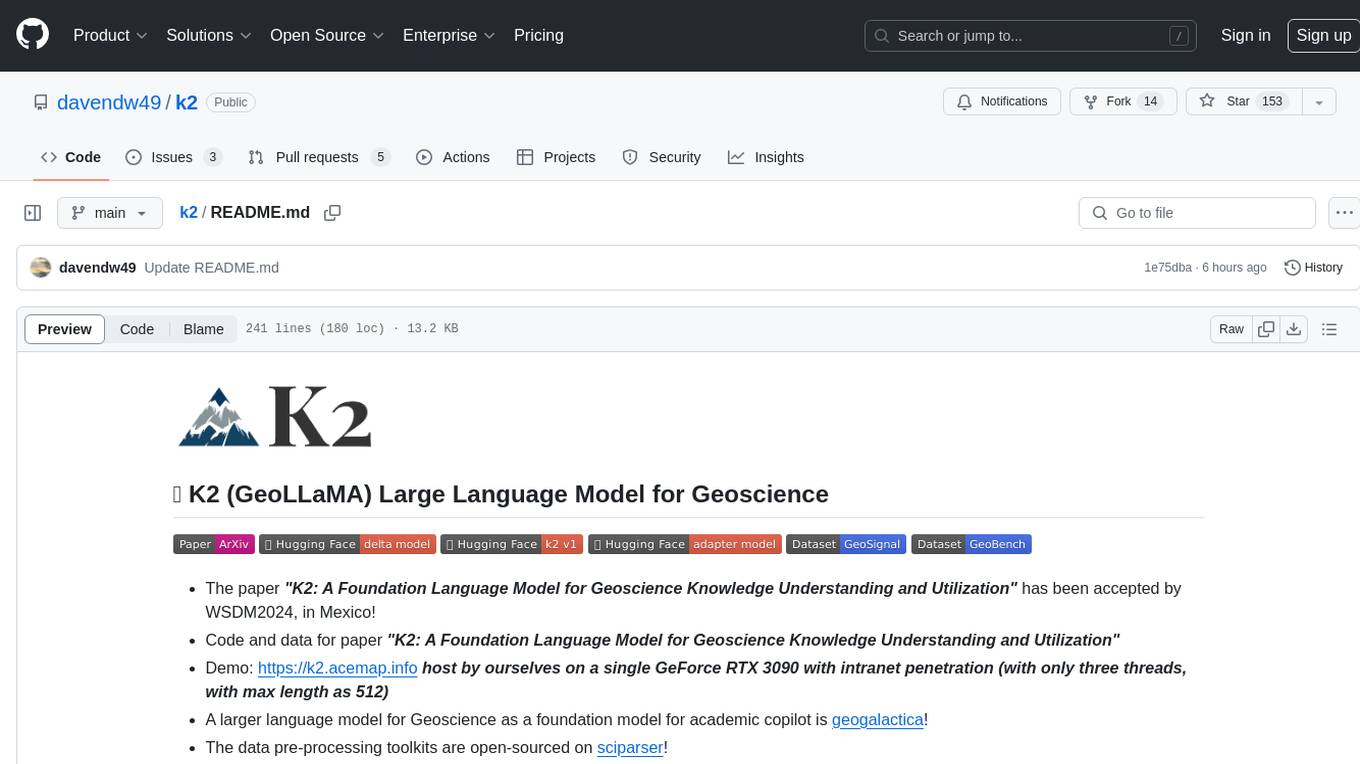
k2
K2 (GeoLLaMA) is a large language model for geoscience, trained on geoscience literature and fine-tuned with knowledge-intensive instruction data. It outperforms baseline models on objective and subjective tasks. The repository provides K2 weights, core data of GeoSignal, GeoBench benchmark, and code for further pretraining and instruction tuning. The model is available on Hugging Face for use. The project aims to create larger and more powerful geoscience language models in the future.
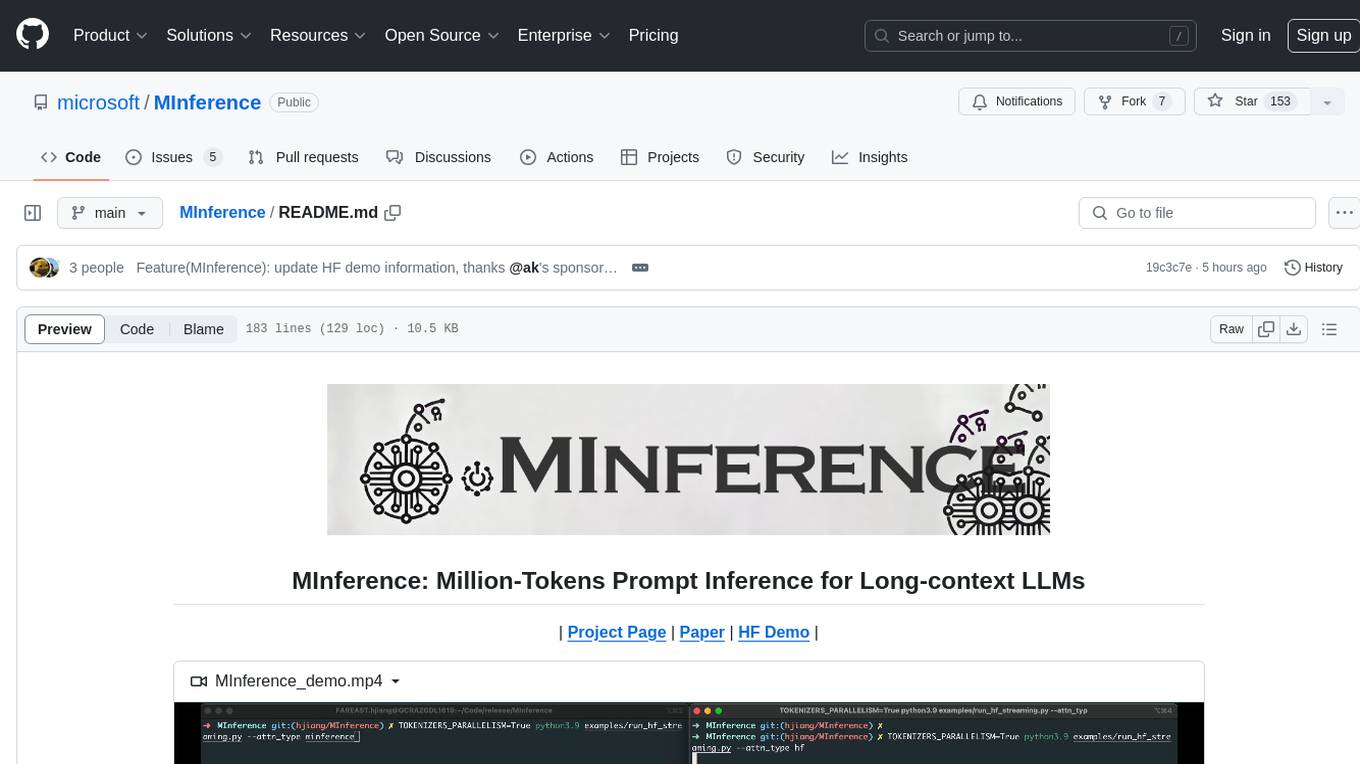
MInference
MInference is a tool designed to accelerate pre-filling for long-context Language Models (LLMs) by leveraging dynamic sparse attention. It achieves up to a 10x speedup for pre-filling on an A100 while maintaining accuracy. The tool supports various decoding LLMs, including LLaMA-style models and Phi models, and provides custom kernels for attention computation. MInference is useful for researchers and developers working with large-scale language models who aim to improve efficiency without compromising accuracy.
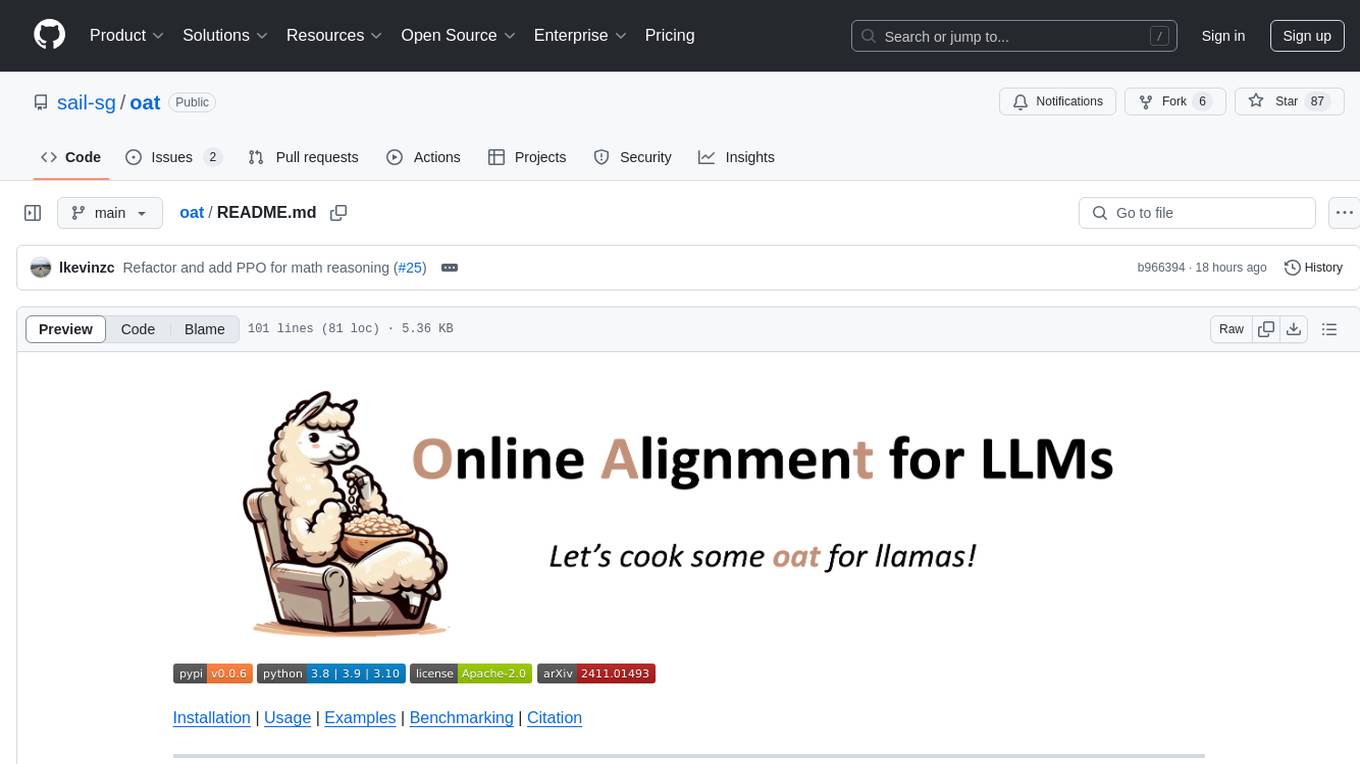
oat
Oat is a simple and efficient framework for running online LLM alignment algorithms. It implements a distributed Actor-Learner-Oracle architecture, with components optimized using state-of-the-art tools. Oat simplifies the experimental pipeline of LLM alignment by serving an Oracle online for preference data labeling and model evaluation. It provides a variety of oracles for simulating feedback and supports verifiable rewards. Oat's modular structure allows for easy inheritance and modification of classes, enabling rapid prototyping and experimentation with new algorithms. The framework implements cutting-edge online algorithms like PPO for math reasoning and various online exploration algorithms.
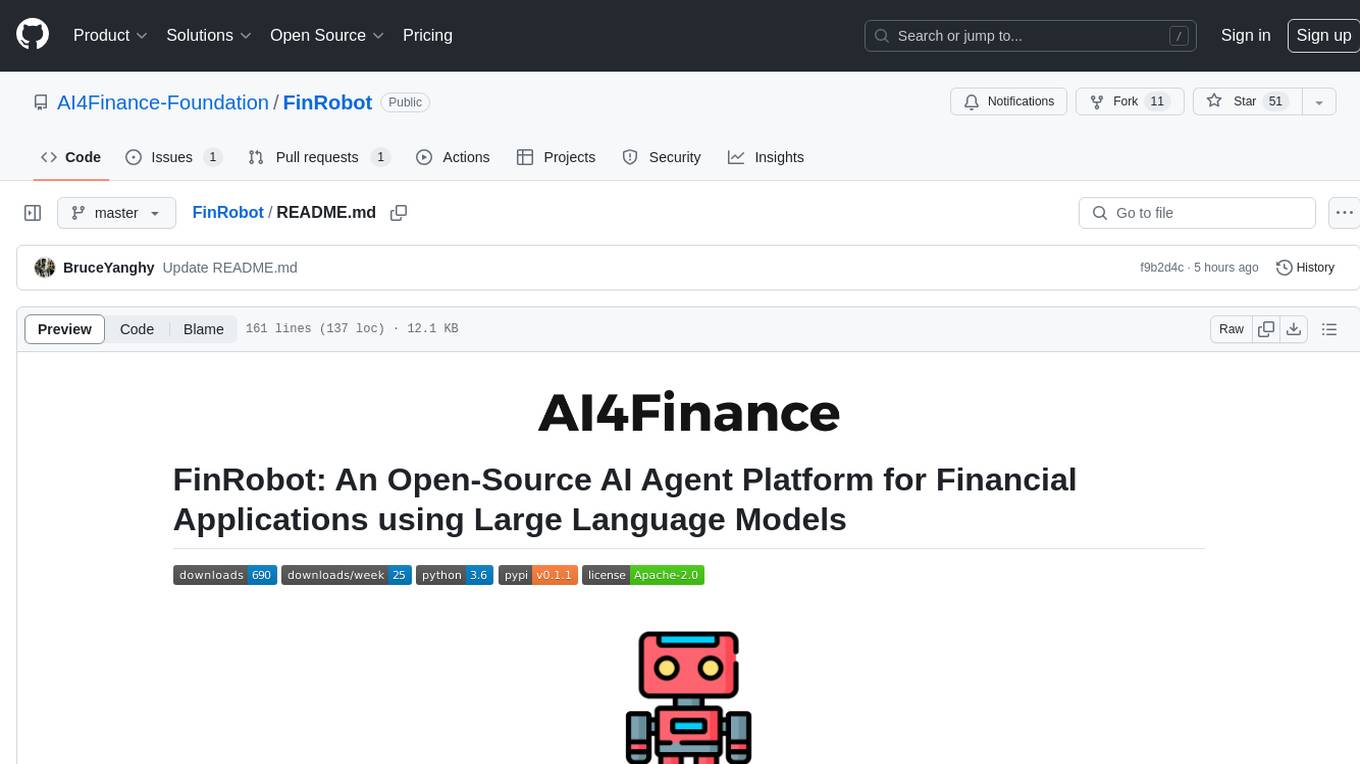
FinRobot
FinRobot is an open-source AI agent platform designed for financial applications using large language models. It transcends the scope of FinGPT, offering a comprehensive solution that integrates a diverse array of AI technologies. The platform's versatility and adaptability cater to the multifaceted needs of the financial industry. FinRobot's ecosystem is organized into four layers, including Financial AI Agents Layer, Financial LLMs Algorithms Layer, LLMOps and DataOps Layers, and Multi-source LLM Foundation Models Layer. The platform's agent workflow involves Perception, Brain, and Action modules to capture, process, and execute financial data and insights. The Smart Scheduler optimizes model diversity and selection for tasks, managed by components like Director Agent, Agent Registration, Agent Adaptor, and Task Manager. The tool provides a structured file organization with subfolders for agents, data sources, and functional modules, along with installation instructions and hands-on tutorials.
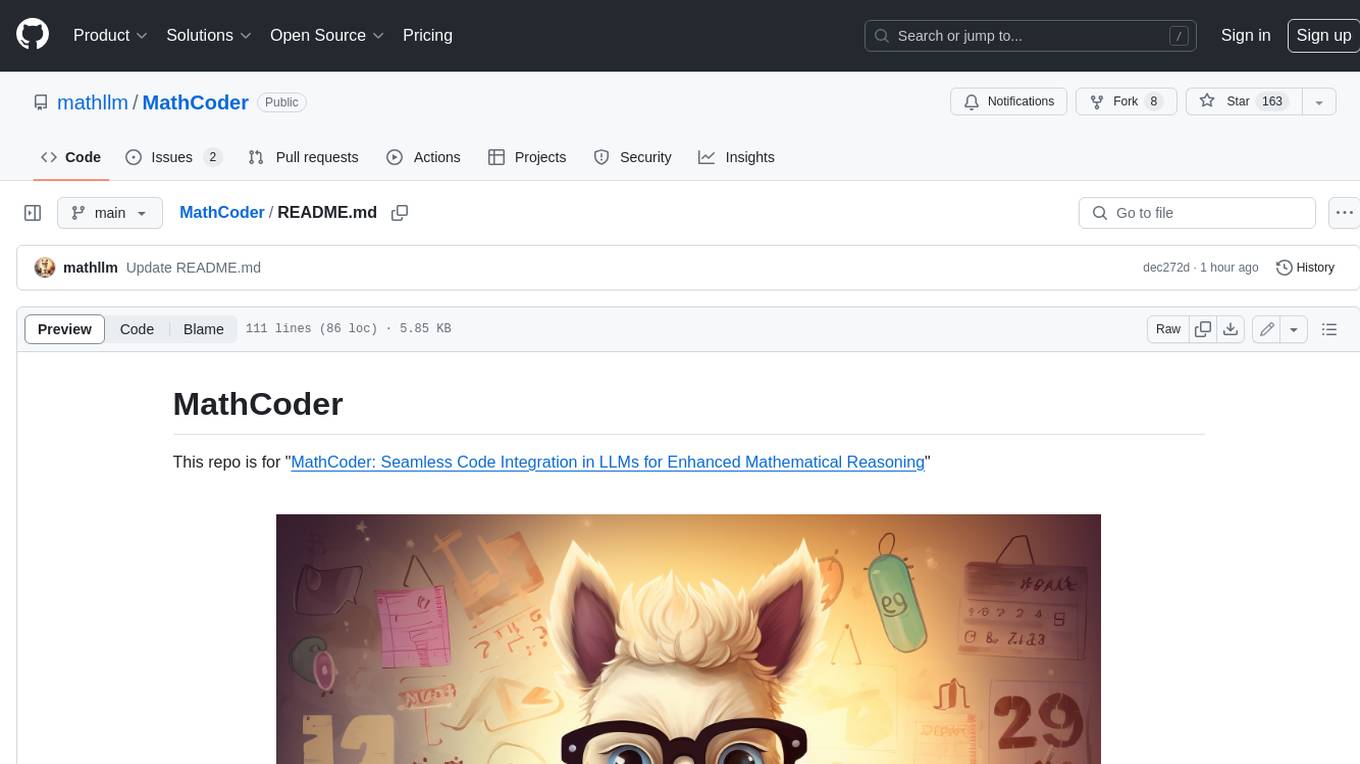
MathCoder
MathCoder is a repository focused on enhancing mathematical reasoning by fine-tuning open-source language models to use code for modeling and deriving math equations. It introduces MathCodeInstruct dataset with solutions interleaving natural language, code, and execution results. The repository provides MathCoder models capable of generating code-based solutions for challenging math problems, achieving state-of-the-art scores on MATH and GSM8K datasets. It offers tools for model deployment, inference, and evaluation, along with a citation for referencing the work.
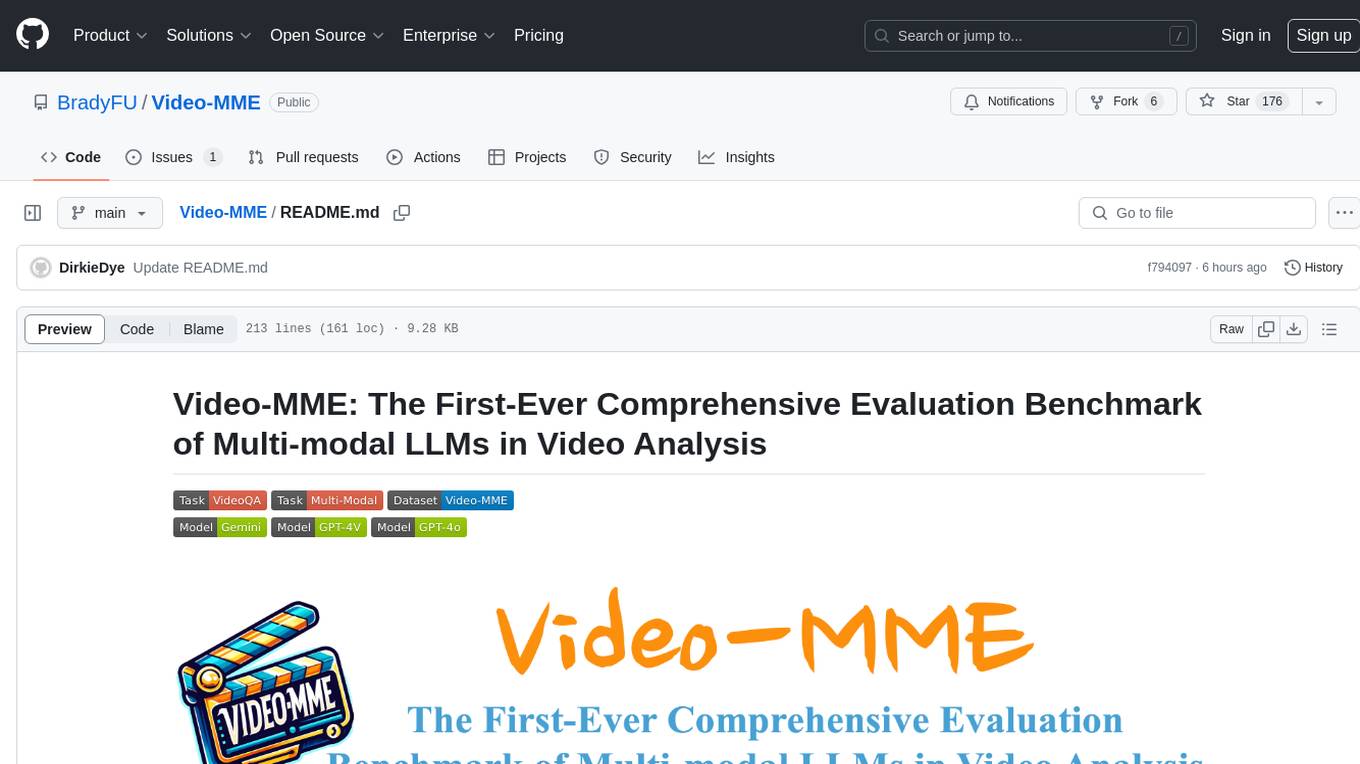
Video-MME
Video-MME is the first-ever comprehensive evaluation benchmark of Multi-modal Large Language Models (MLLMs) in Video Analysis. It assesses the capabilities of MLLMs in processing video data, covering a wide range of visual domains, temporal durations, and data modalities. The dataset comprises 900 videos with 256 hours and 2,700 human-annotated question-answer pairs. It distinguishes itself through features like duration variety, diversity in video types, breadth in data modalities, and quality in annotations.
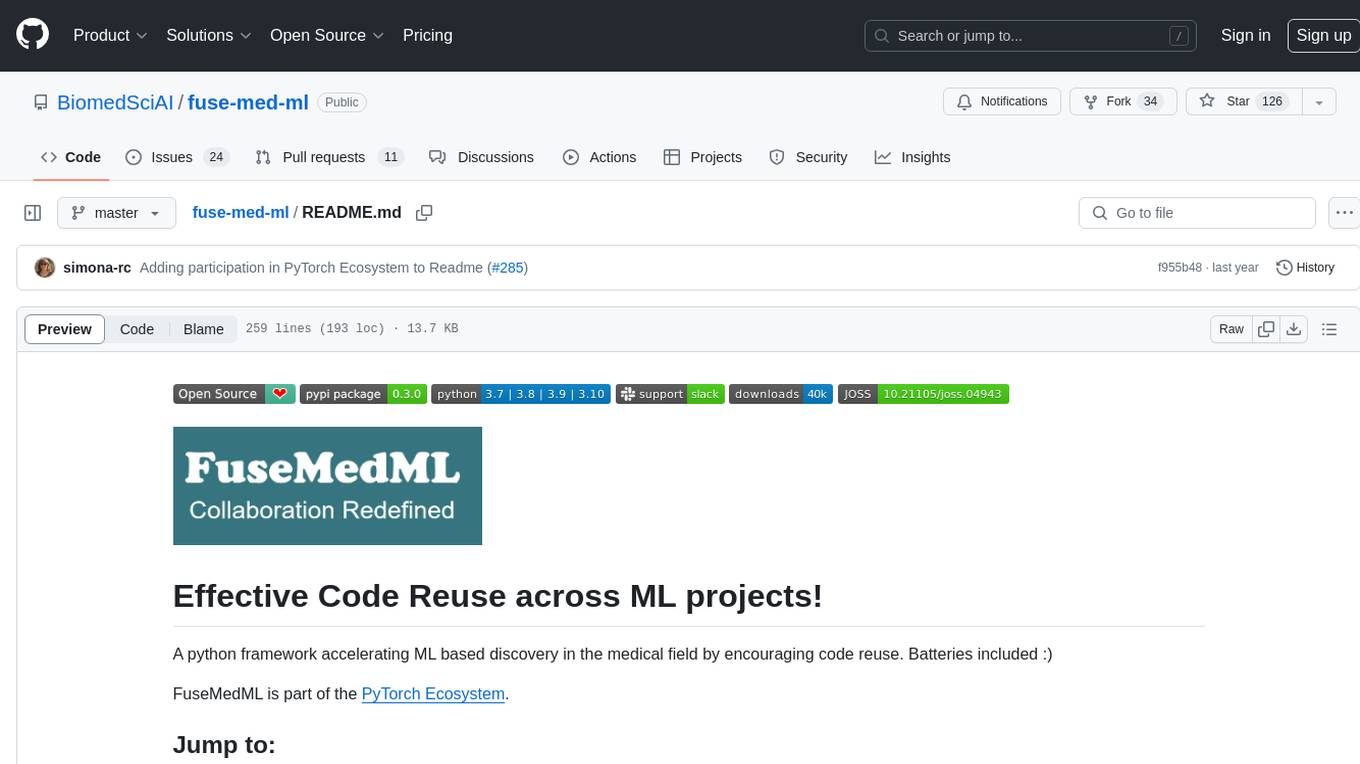
fuse-med-ml
FuseMedML is a Python framework designed to accelerate machine learning-based discovery in the medical field by promoting code reuse. It provides a flexible design concept where data is stored in a nested dictionary, allowing easy handling of multi-modality information. The framework includes components for creating custom models, loss functions, metrics, and data processing operators. Additionally, FuseMedML offers 'batteries included' key components such as fuse.data for data processing, fuse.eval for model evaluation, and fuse.dl for reusable deep learning components. It supports PyTorch and PyTorch Lightning libraries and encourages the creation of domain extensions for specific medical domains.
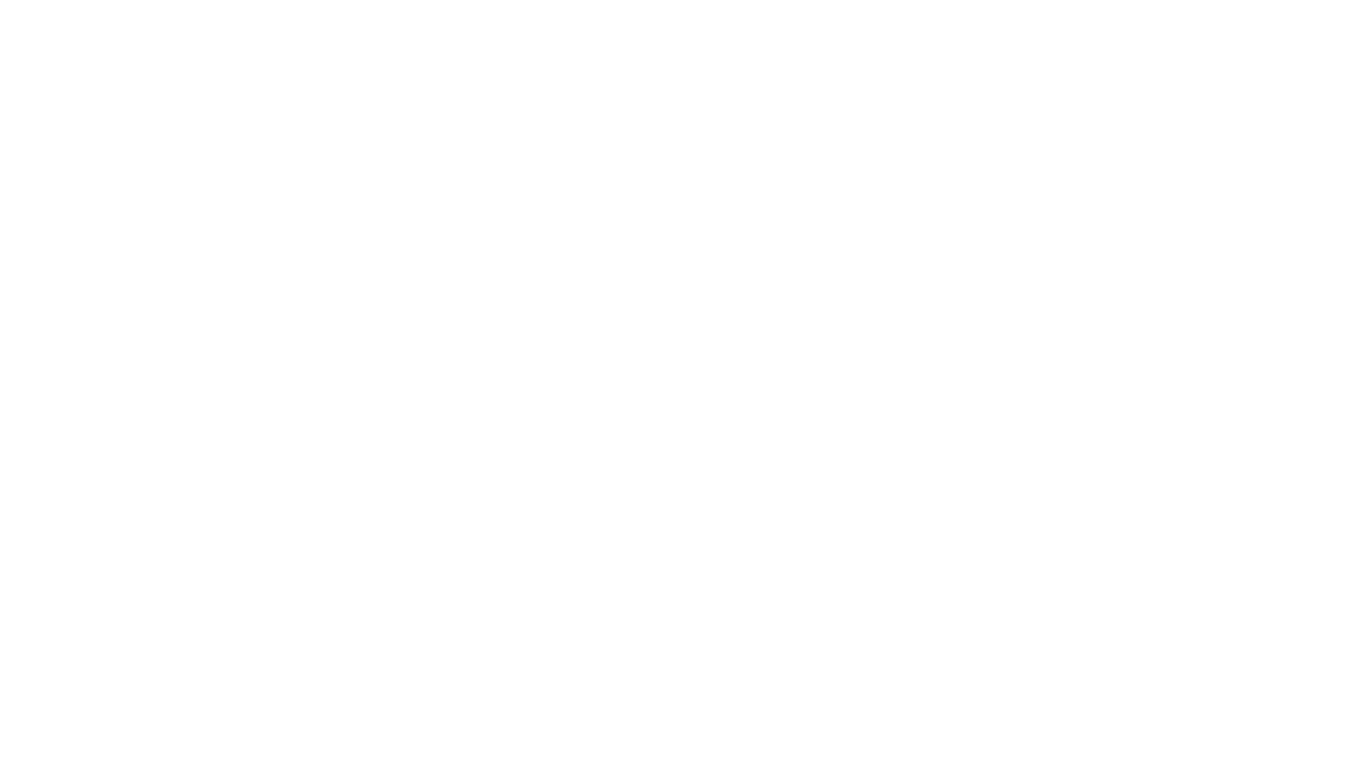
cleanlab
Cleanlab helps you **clean** data and **lab** els by automatically detecting issues in a ML dataset. To facilitate **machine learning with messy, real-world data** , this data-centric AI package uses your _existing_ models to estimate dataset problems that can be fixed to train even _better_ models.
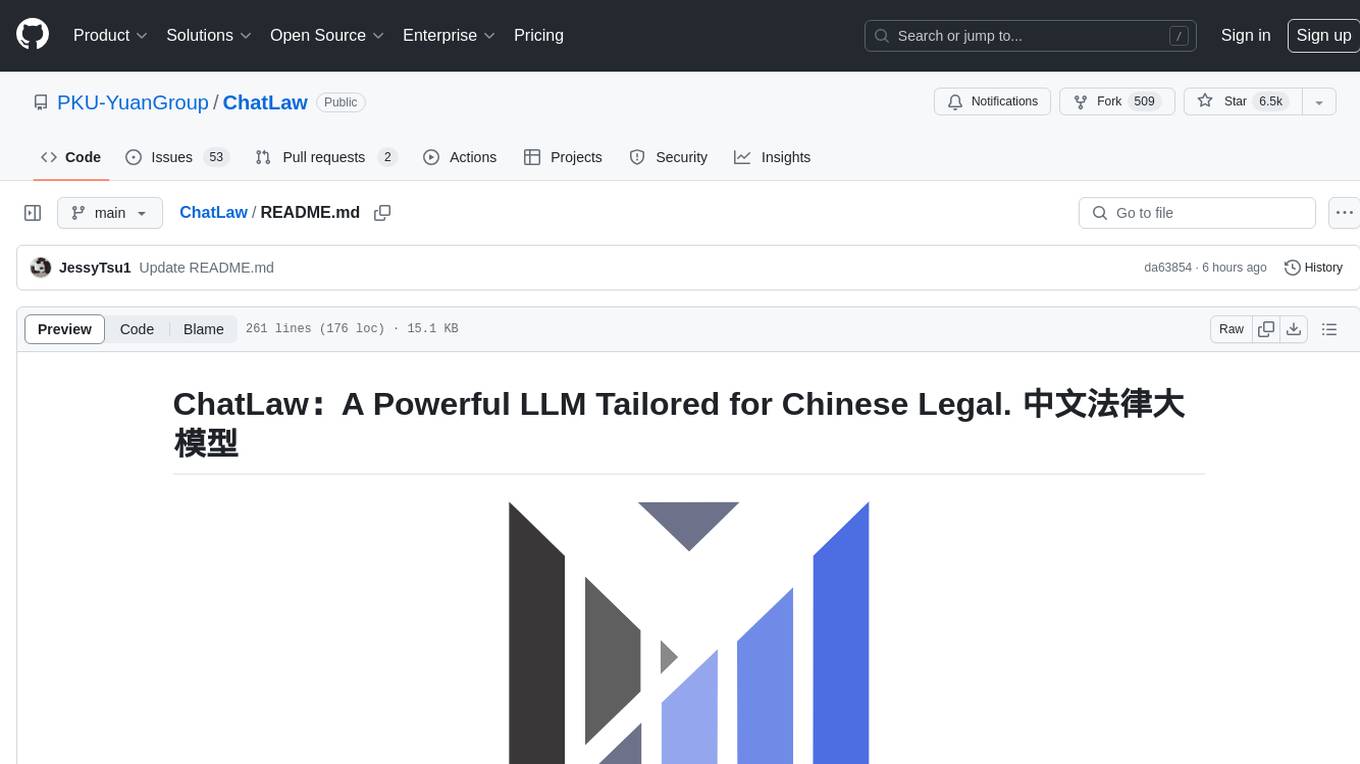
ChatLaw
ChatLaw is an open-source legal large language model tailored for Chinese legal scenarios. It aims to combine LLM and knowledge bases to provide solutions for legal scenarios. The models include ChatLaw-13B and ChatLaw-33B, trained on various legal texts to construct dialogue data. The project focuses on improving logical reasoning abilities and plans to train models with parameters exceeding 30B for better performance. The dataset consists of forum posts, news, legal texts, judicial interpretations, legal consultations, exam questions, and court judgments, cleaned and enhanced to create dialogue data. The tool is designed to assist in legal tasks requiring complex logical reasoning, with a focus on accuracy and reliability.
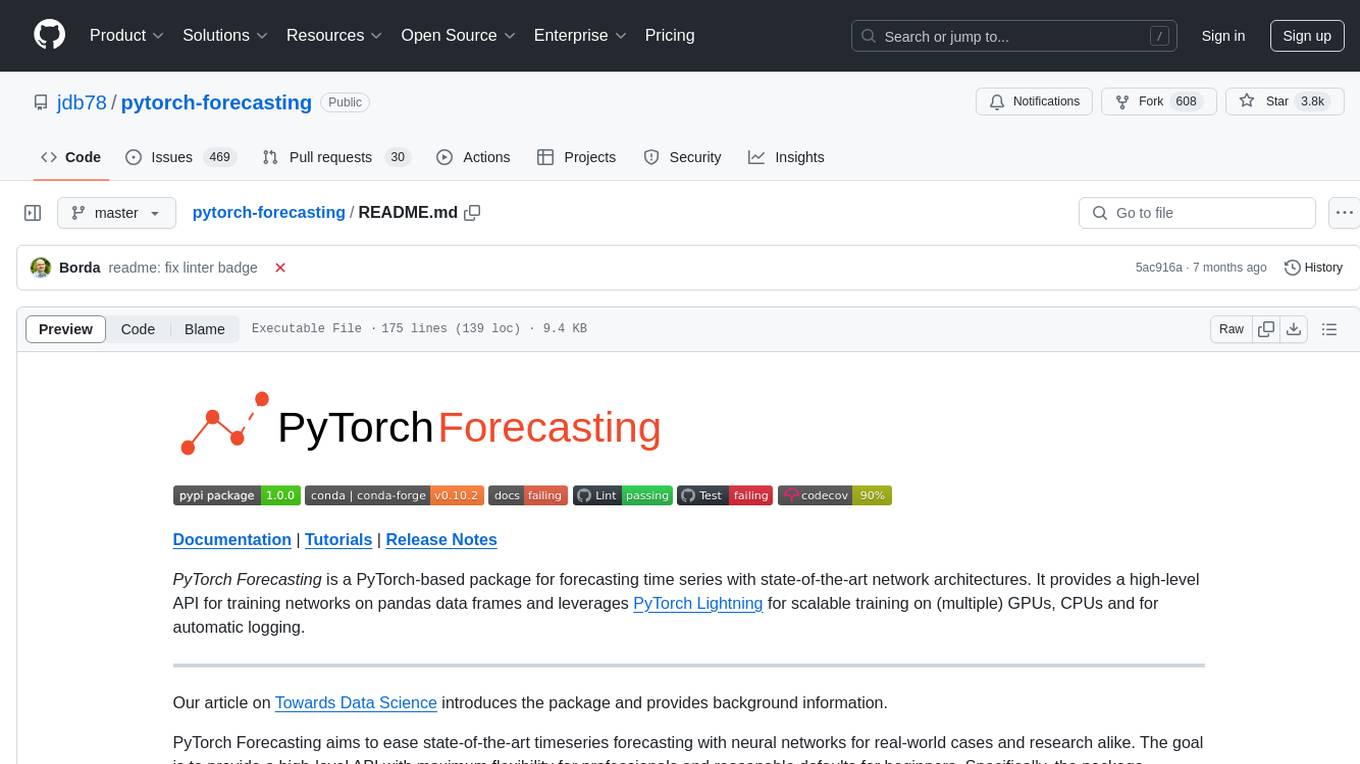
pytorch-forecasting
PyTorch Forecasting is a PyTorch-based package for time series forecasting with state-of-the-art network architectures. It offers a high-level API for training networks on pandas data frames and utilizes PyTorch Lightning for scalable training on GPUs and CPUs. The package aims to simplify time series forecasting with neural networks by providing a flexible API for professionals and default settings for beginners. It includes a timeseries dataset class, base model class, multiple neural network architectures, multi-horizon timeseries metrics, and hyperparameter tuning with optuna. PyTorch Forecasting is built on pytorch-lightning for easy training on various hardware configurations.
For similar tasks
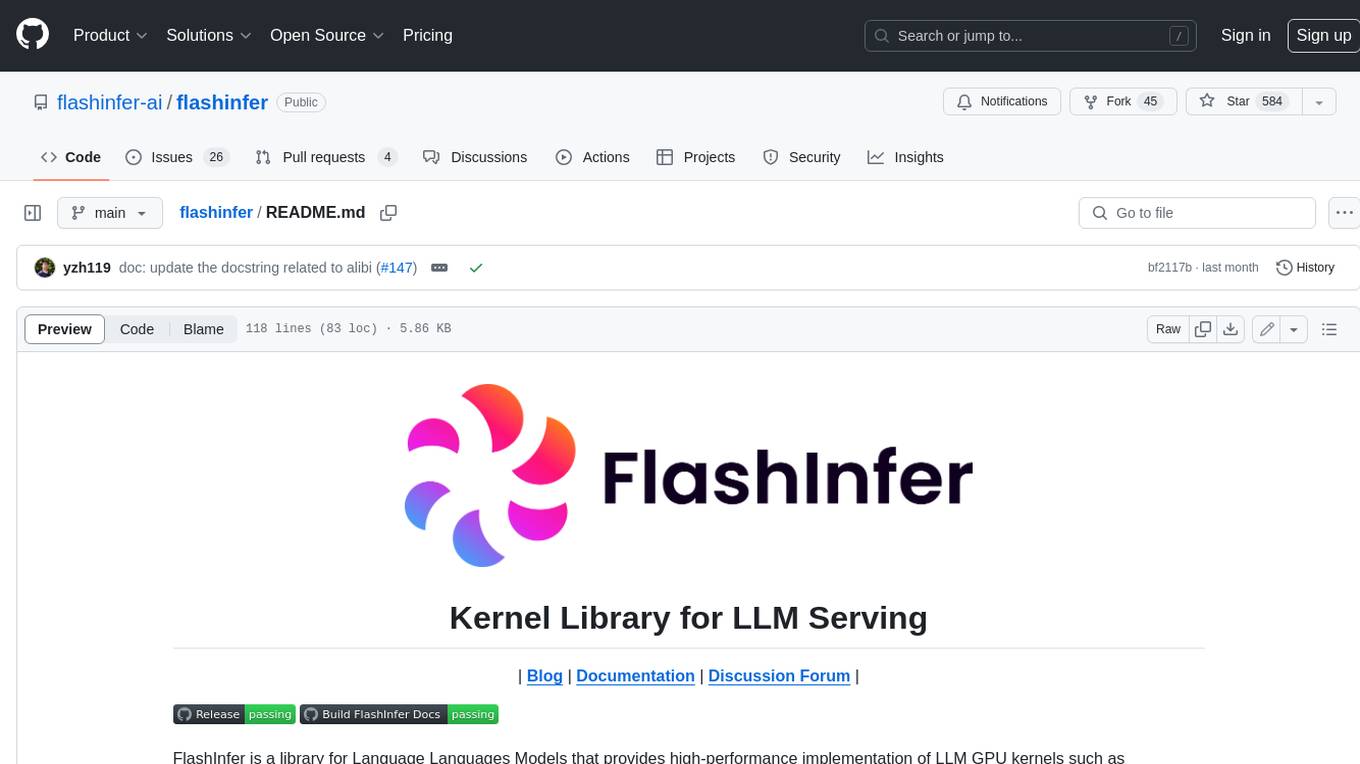
flashinfer
FlashInfer is a library for Language Languages Models that provides high-performance implementation of LLM GPU kernels such as FlashAttention, PageAttention and LoRA. FlashInfer focus on LLM serving and inference, and delivers state-the-art performance across diverse scenarios.
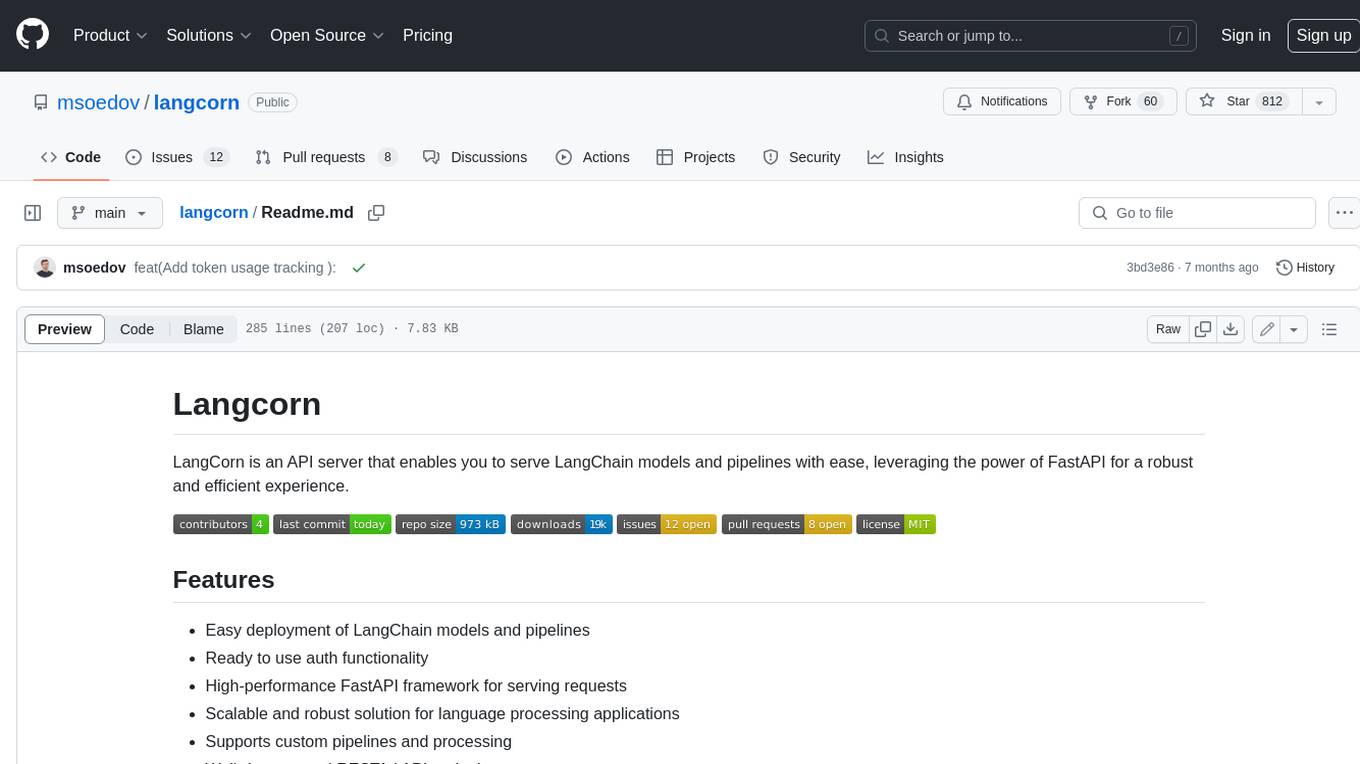
langcorn
LangCorn is an API server that enables you to serve LangChain models and pipelines with ease, leveraging the power of FastAPI for a robust and efficient experience. It offers features such as easy deployment of LangChain models and pipelines, ready-to-use authentication functionality, high-performance FastAPI framework for serving requests, scalability and robustness for language processing applications, support for custom pipelines and processing, well-documented RESTful API endpoints, and asynchronous processing for faster response times.
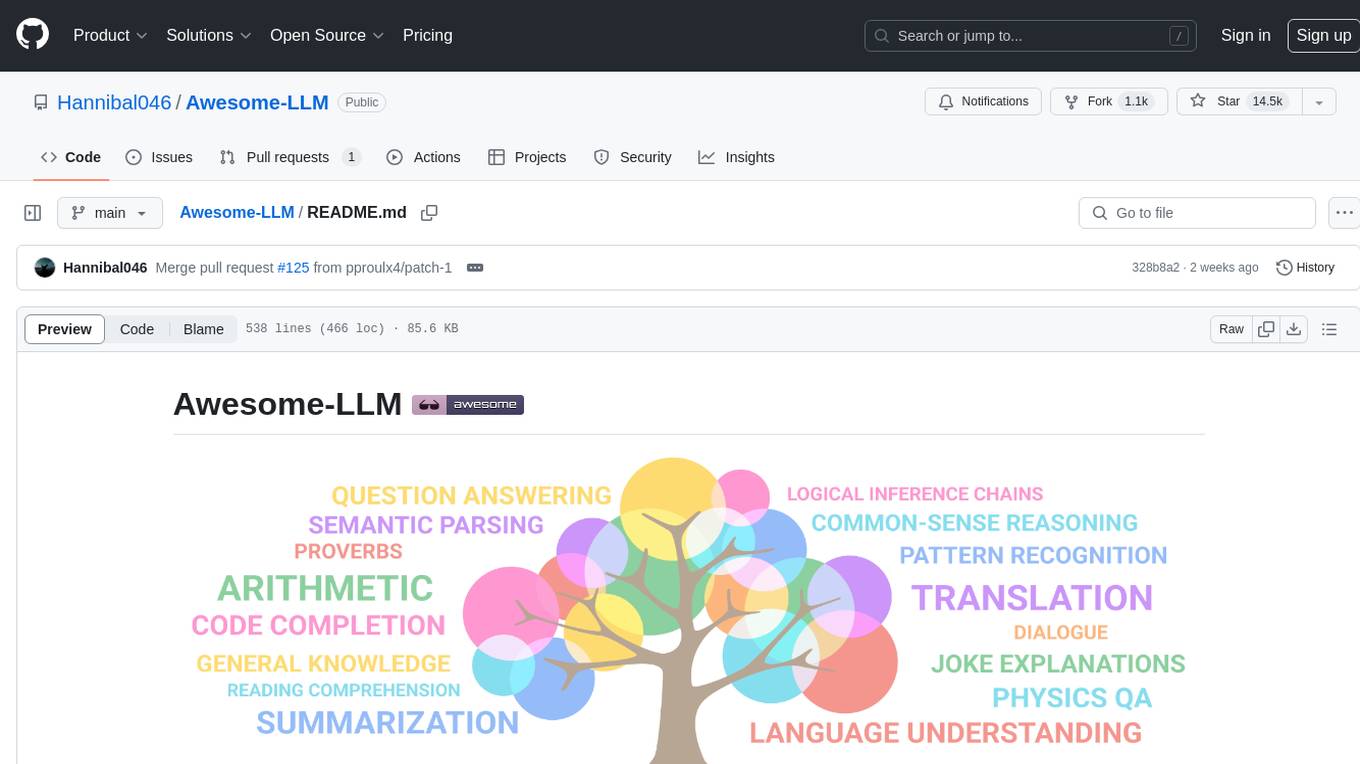
Awesome-LLM
Awesome-LLM is a curated list of resources related to large language models, focusing on papers, projects, frameworks, tools, tutorials, courses, opinions, and other useful resources in the field. It covers trending LLM projects, milestone papers, other papers, open LLM projects, LLM training frameworks, LLM evaluation frameworks, tools for deploying LLM, prompting libraries & tools, tutorials, courses, books, and opinions. The repository provides a comprehensive overview of the latest advancements and resources in the field of large language models.
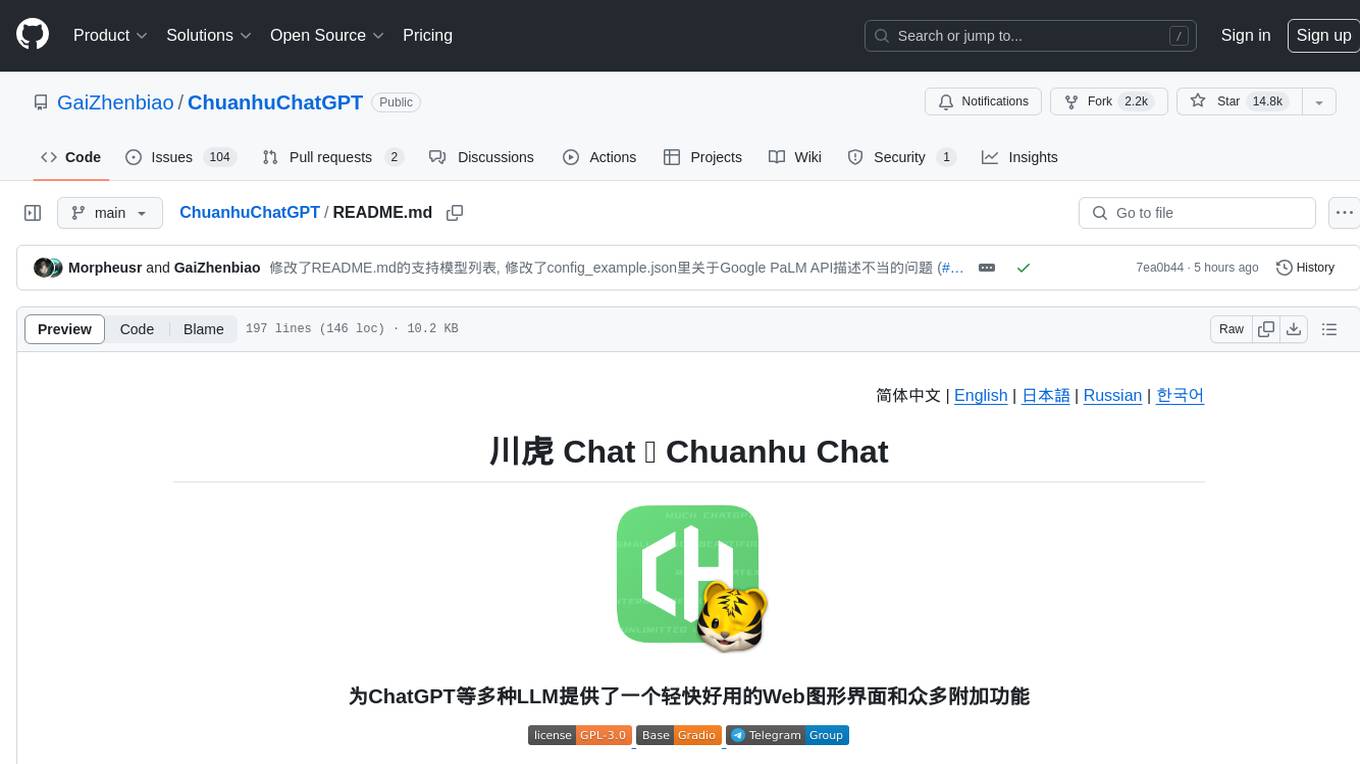
ChuanhuChatGPT
Chuanhu Chat is a user-friendly web graphical interface that provides various additional features for ChatGPT and other language models. It supports GPT-4, file-based question answering, local deployment of language models, online search, agent assistant, and fine-tuning. The tool offers a range of functionalities including auto-solving questions, online searching with network support, knowledge base for quick reading, local deployment of language models, GPT 3.5 fine-tuning, and custom model integration. It also features system prompts for effective role-playing, basic conversation capabilities with options to regenerate or delete dialogues, conversation history management with auto-saving and search functionalities, and a visually appealing user experience with themes, dark mode, LaTeX rendering, and PWA application support.
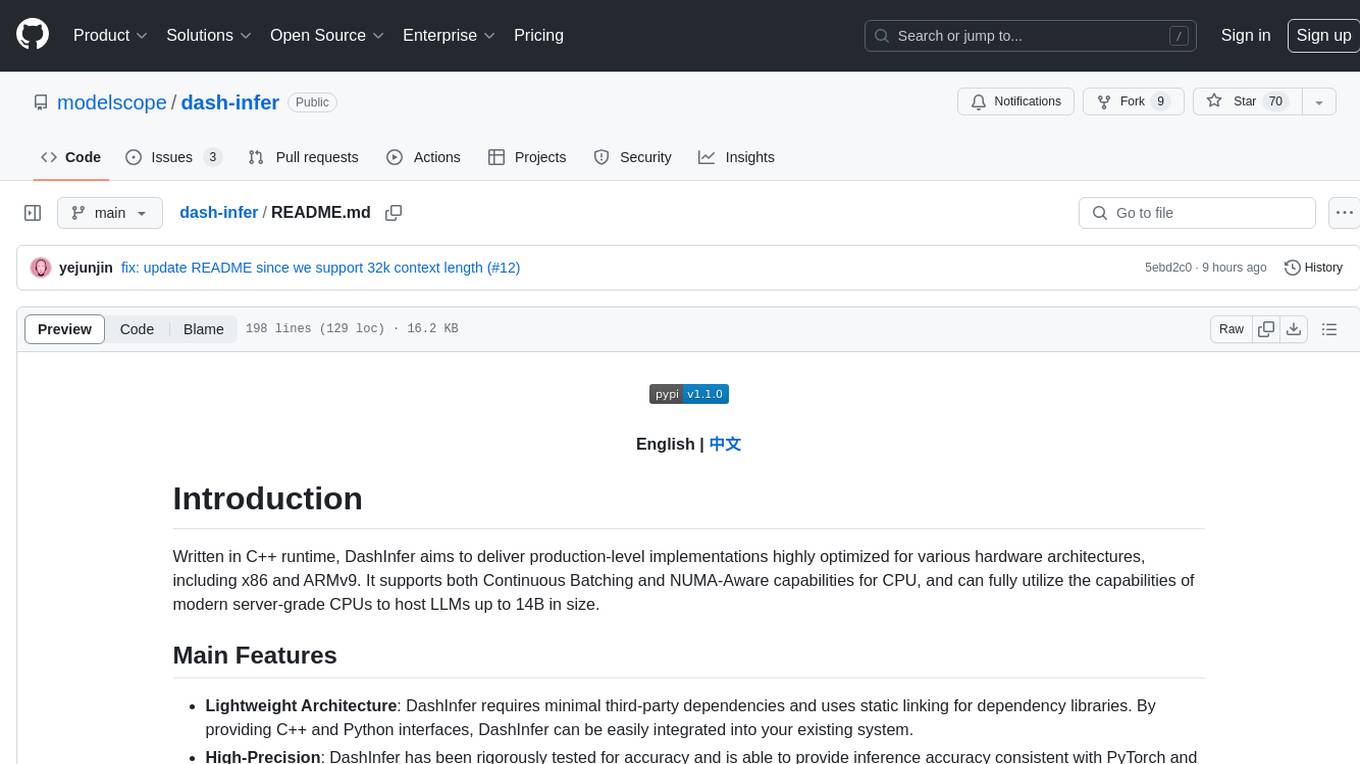
dash-infer
DashInfer is a C++ runtime tool designed to deliver production-level implementations highly optimized for various hardware architectures, including x86 and ARMv9. It supports Continuous Batching and NUMA-Aware capabilities for CPU, and can fully utilize modern server-grade CPUs to host large language models (LLMs) up to 14B in size. With lightweight architecture, high precision, support for mainstream open-source LLMs, post-training quantization, optimized computation kernels, NUMA-aware design, and multi-language API interfaces, DashInfer provides a versatile solution for efficient inference tasks. It supports x86 CPUs with AVX2 instruction set and ARMv9 CPUs with SVE instruction set, along with various data types like FP32, BF16, and InstantQuant. DashInfer also offers single-NUMA and multi-NUMA architectures for model inference, with detailed performance tests and inference accuracy evaluations available. The tool is supported on mainstream Linux server operating systems and provides documentation and examples for easy integration and usage.
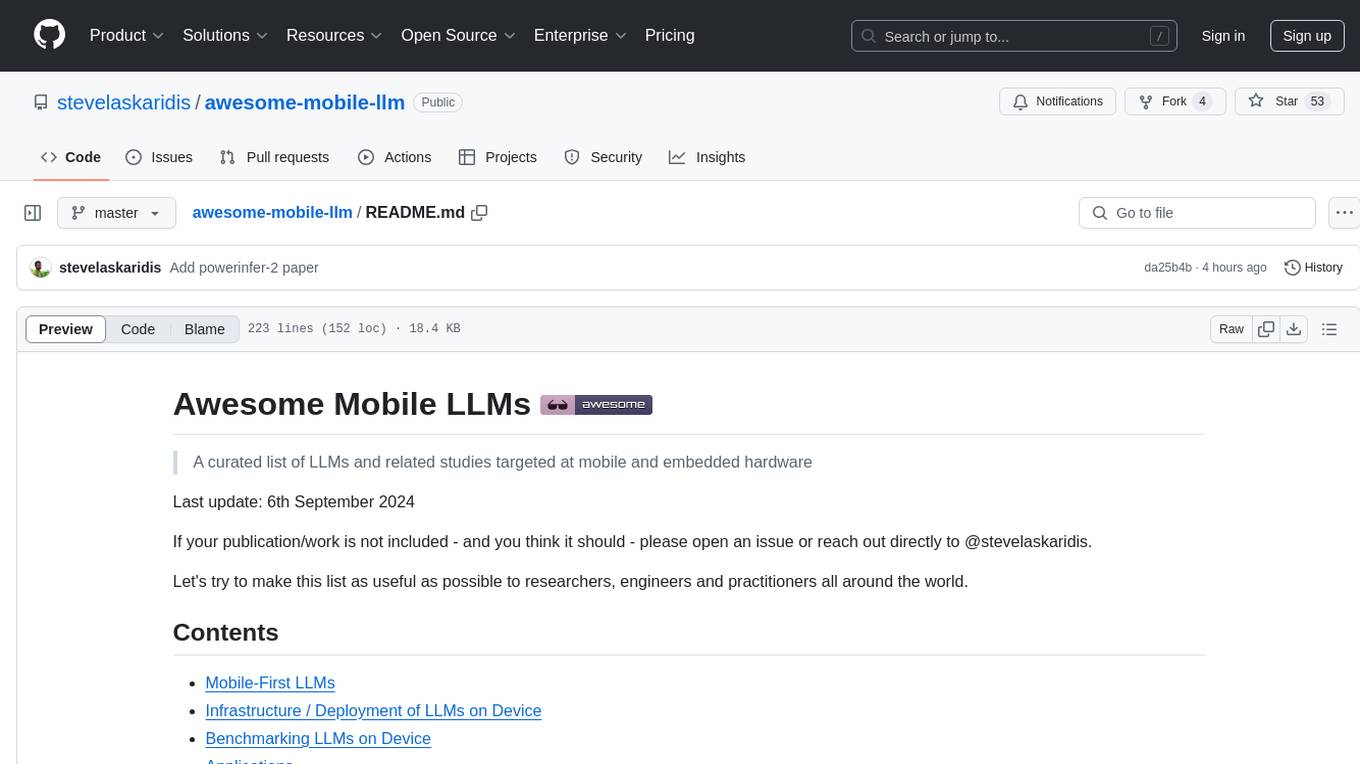
awesome-mobile-llm
Awesome Mobile LLMs is a curated list of Large Language Models (LLMs) and related studies focused on mobile and embedded hardware. The repository includes information on various LLM models, deployment frameworks, benchmarking efforts, applications, multimodal LLMs, surveys on efficient LLMs, training LLMs on device, mobile-related use-cases, industry announcements, and related repositories. It aims to be a valuable resource for researchers, engineers, and practitioners interested in mobile LLMs.
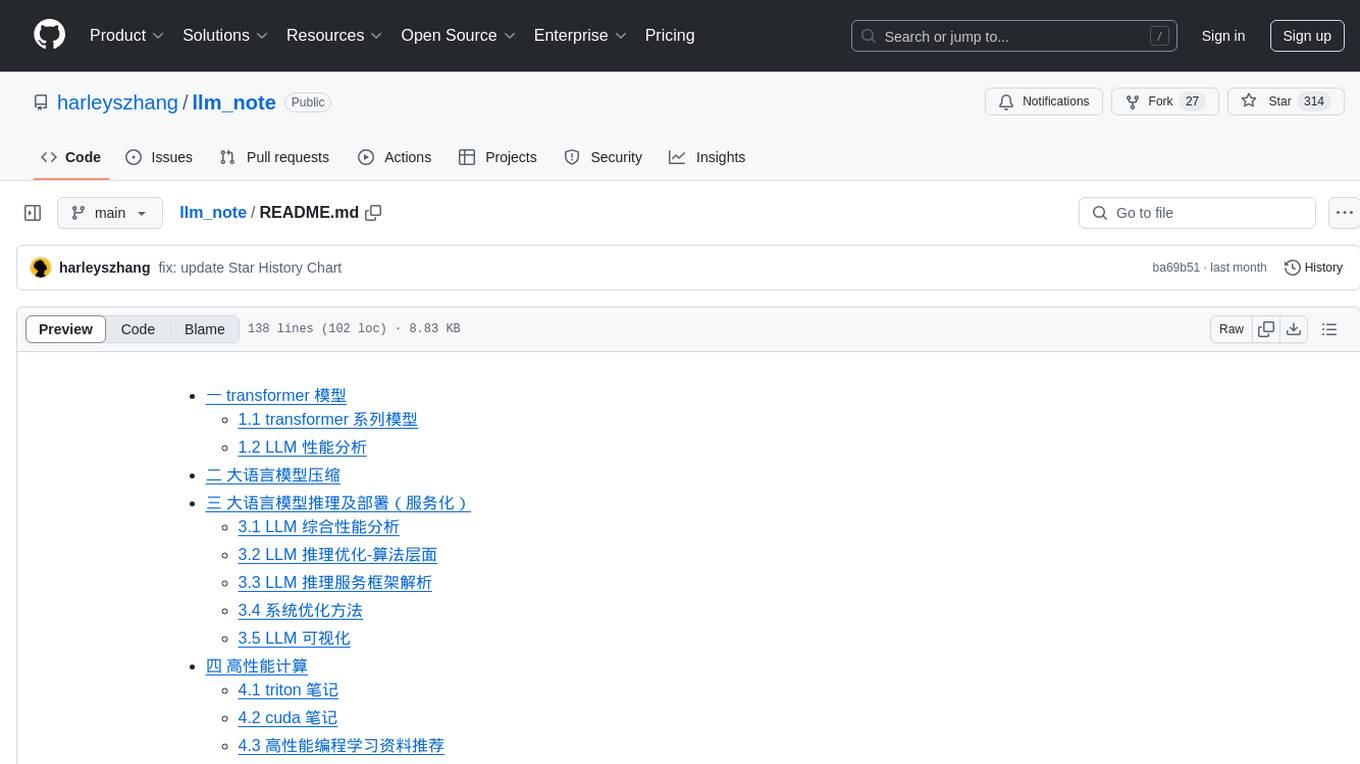
llm_note
LLM notes repository contains detailed analysis on transformer models, language model compression, inference and deployment, high-performance computing, and system optimization methods. It includes discussions on various algorithms, frameworks, and performance analysis related to large language models and high-performance computing. The repository serves as a comprehensive resource for understanding and optimizing language models and computing systems.
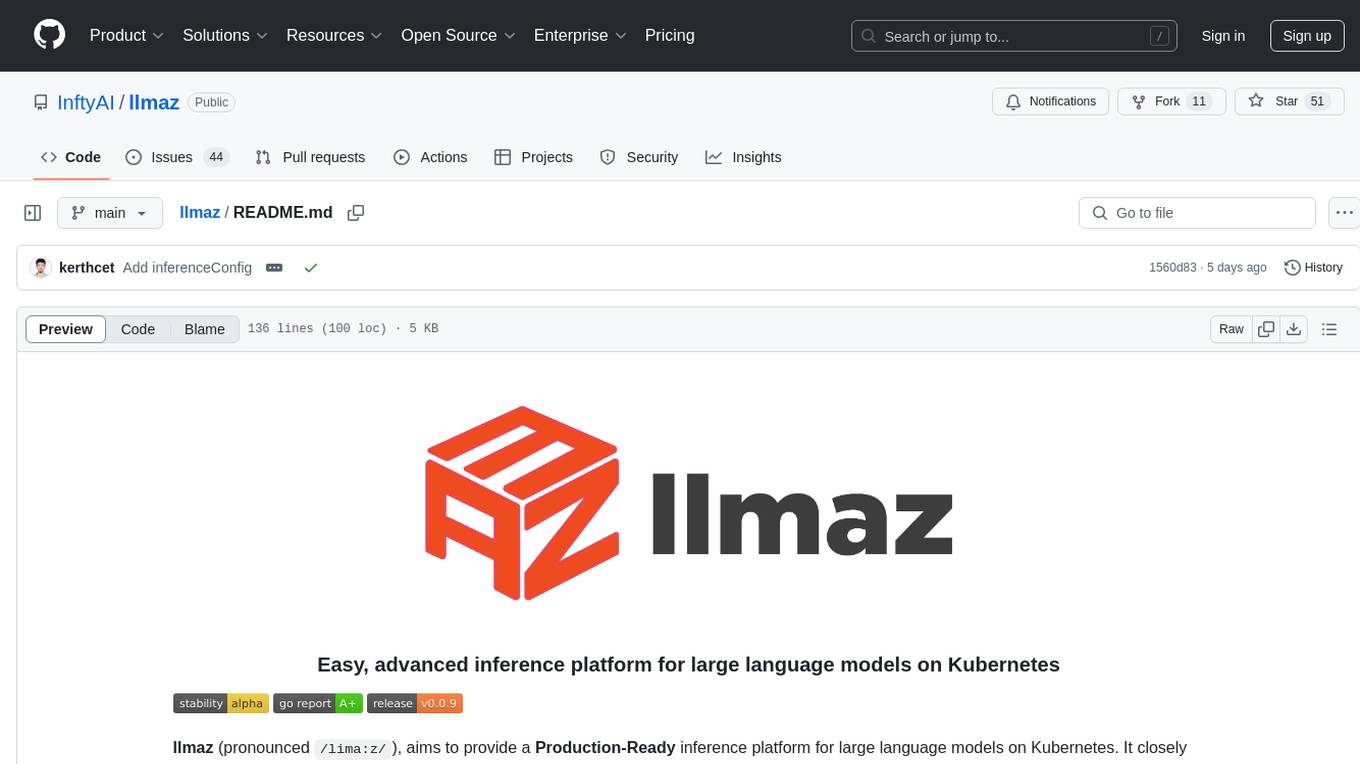
llmaz
llmaz is an easy, advanced inference platform for large language models on Kubernetes. It aims to provide a production-ready solution that integrates with state-of-the-art inference backends. The platform supports efficient model distribution, accelerator fungibility, SOTA inference, various model providers, multi-host support, and scaling efficiency. Users can quickly deploy LLM services with minimal configurations and benefit from a wide range of advanced inference backends. llmaz is designed to optimize cost and performance while supporting cutting-edge researches like Speculative Decoding or Splitwise on Kubernetes.
For similar jobs
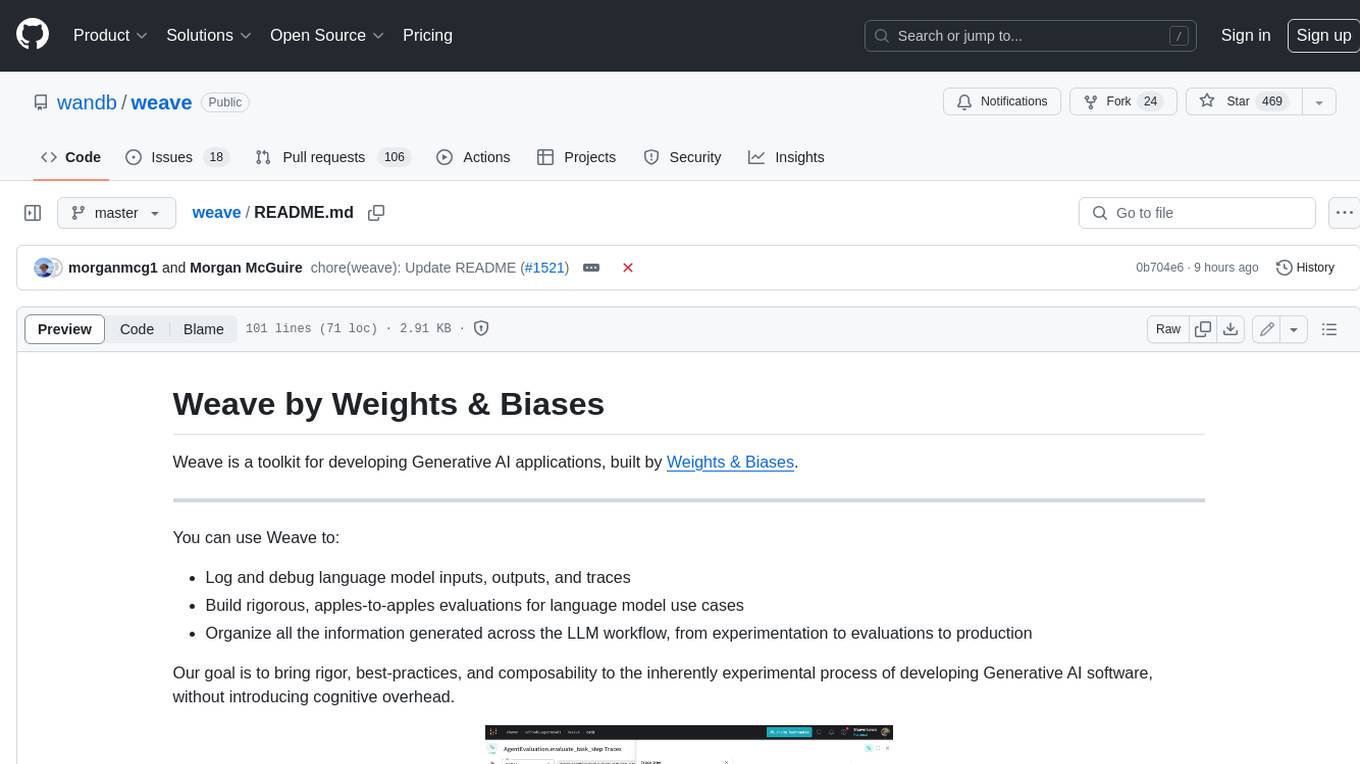
weave
Weave is a toolkit for developing Generative AI applications, built by Weights & Biases. With Weave, you can log and debug language model inputs, outputs, and traces; build rigorous, apples-to-apples evaluations for language model use cases; and organize all the information generated across the LLM workflow, from experimentation to evaluations to production. Weave aims to bring rigor, best-practices, and composability to the inherently experimental process of developing Generative AI software, without introducing cognitive overhead.
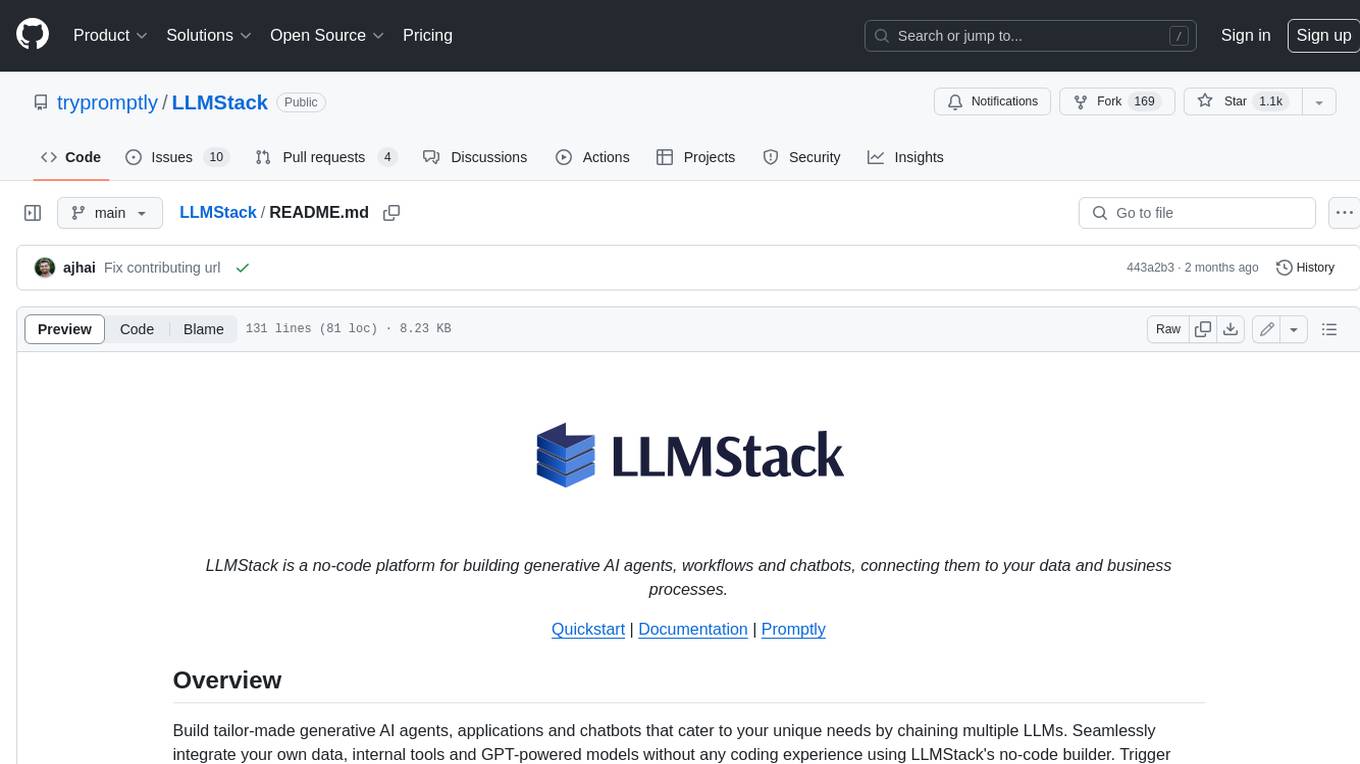
LLMStack
LLMStack is a no-code platform for building generative AI agents, workflows, and chatbots. It allows users to connect their own data, internal tools, and GPT-powered models without any coding experience. LLMStack can be deployed to the cloud or on-premise and can be accessed via HTTP API or triggered from Slack or Discord.
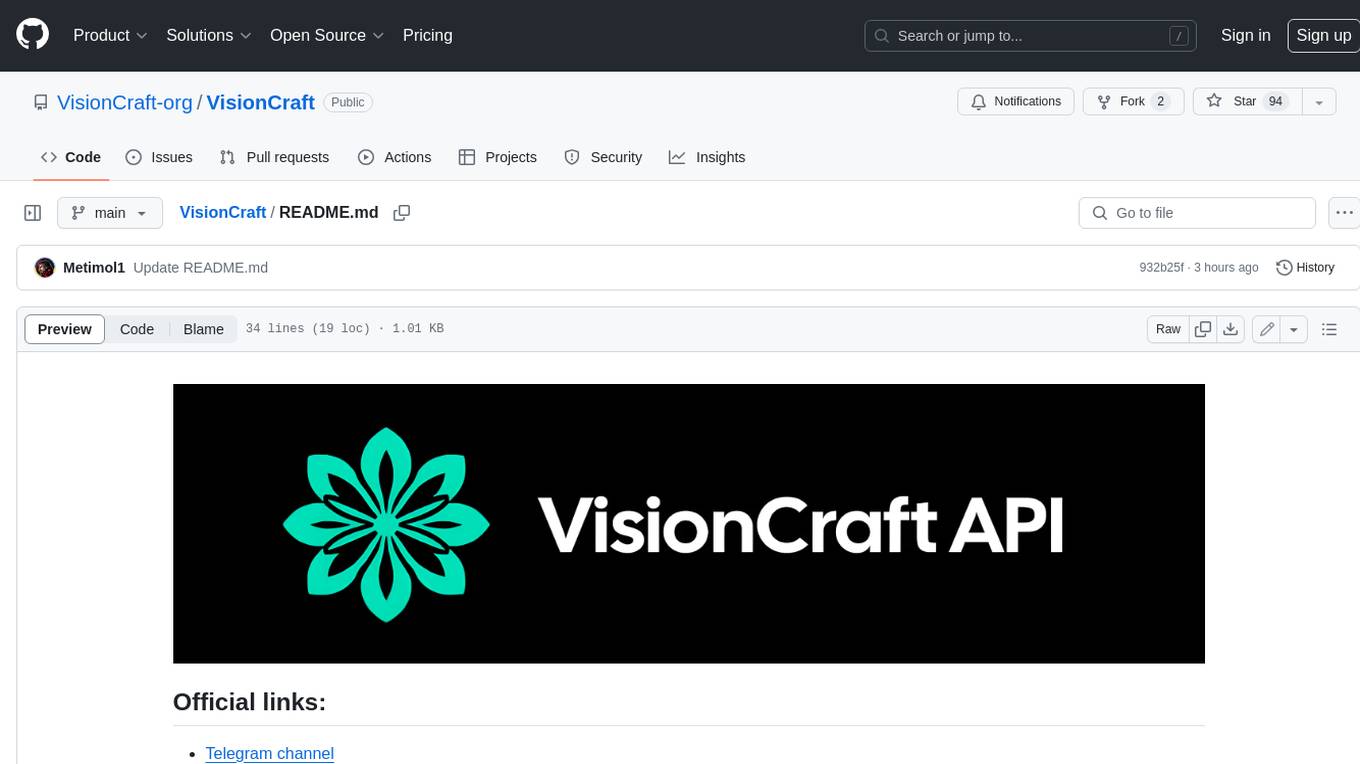
VisionCraft
The VisionCraft API is a free API for using over 100 different AI models. From images to sound.
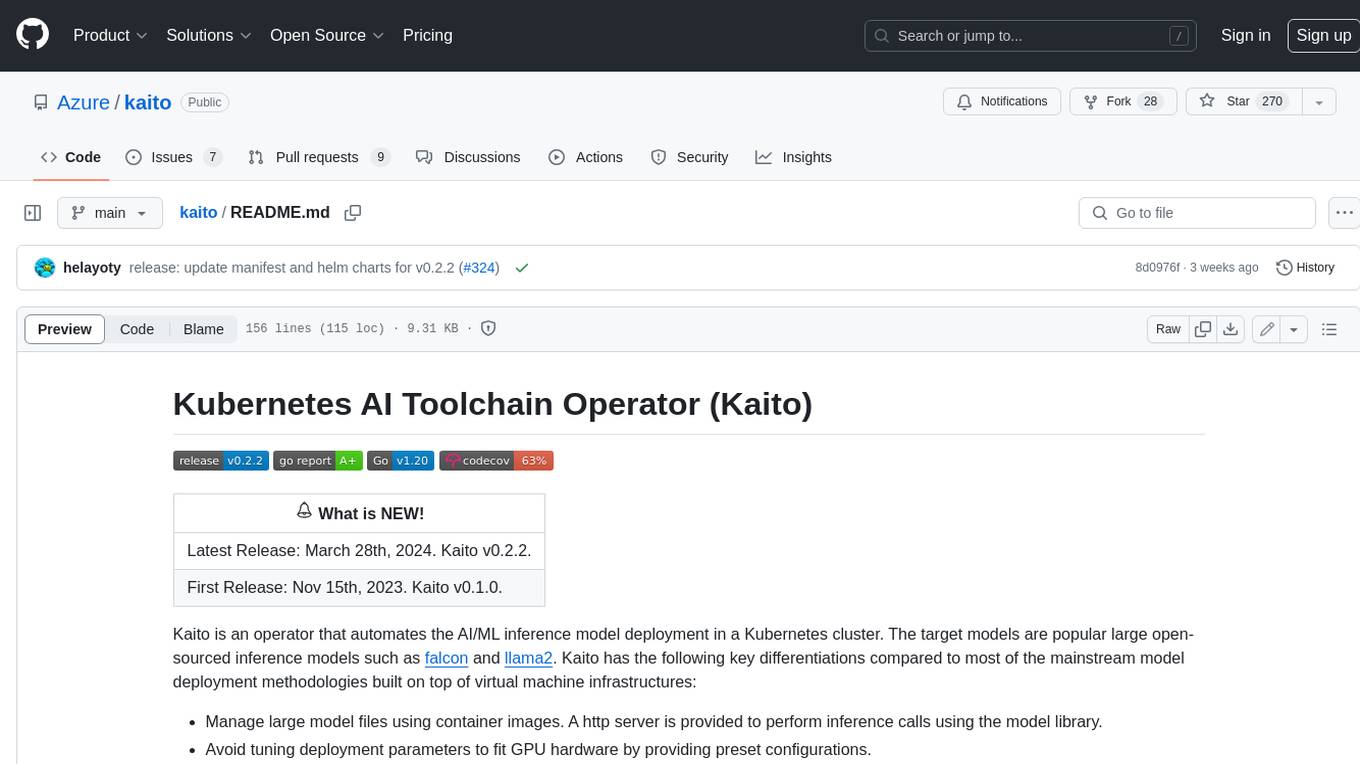
kaito
Kaito is an operator that automates the AI/ML inference model deployment in a Kubernetes cluster. It manages large model files using container images, avoids tuning deployment parameters to fit GPU hardware by providing preset configurations, auto-provisions GPU nodes based on model requirements, and hosts large model images in the public Microsoft Container Registry (MCR) if the license allows. Using Kaito, the workflow of onboarding large AI inference models in Kubernetes is largely simplified.
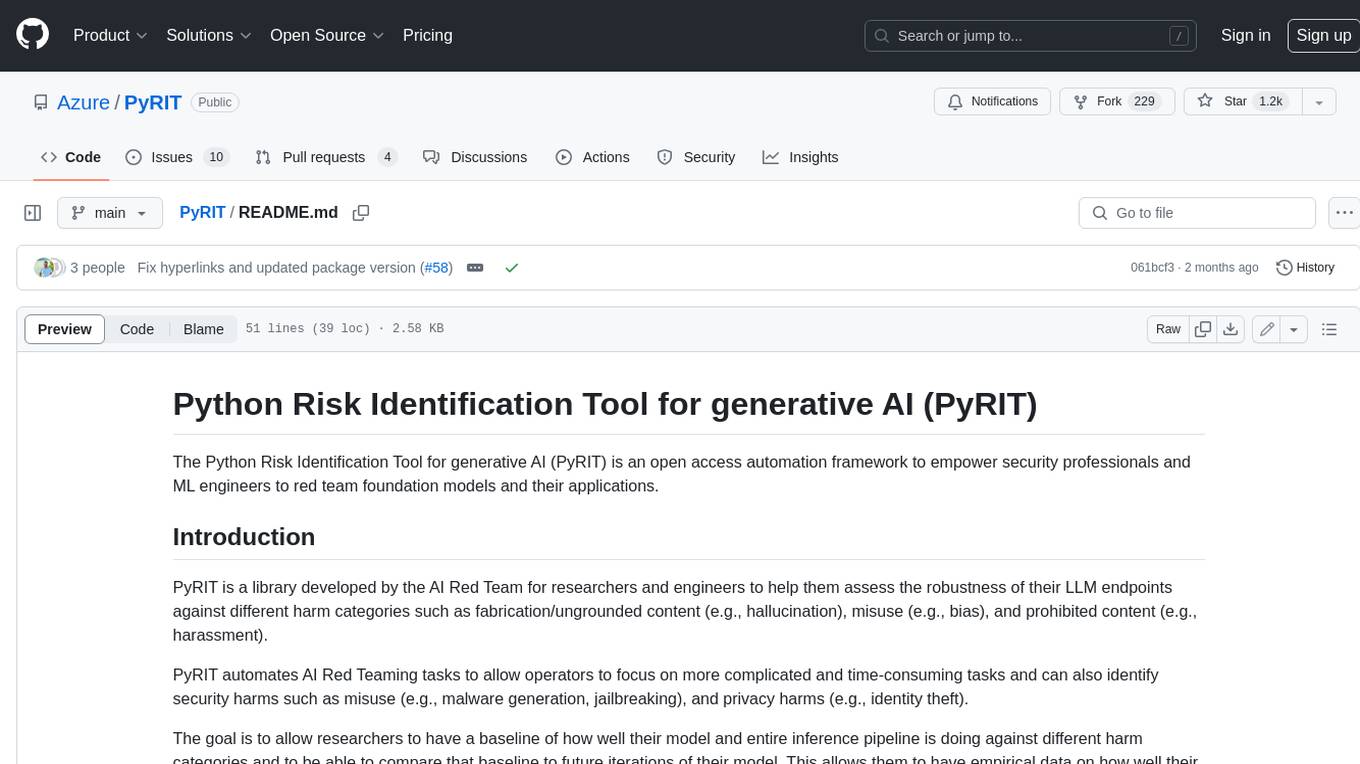
PyRIT
PyRIT is an open access automation framework designed to empower security professionals and ML engineers to red team foundation models and their applications. It automates AI Red Teaming tasks to allow operators to focus on more complicated and time-consuming tasks and can also identify security harms such as misuse (e.g., malware generation, jailbreaking), and privacy harms (e.g., identity theft). The goal is to allow researchers to have a baseline of how well their model and entire inference pipeline is doing against different harm categories and to be able to compare that baseline to future iterations of their model. This allows them to have empirical data on how well their model is doing today, and detect any degradation of performance based on future improvements.
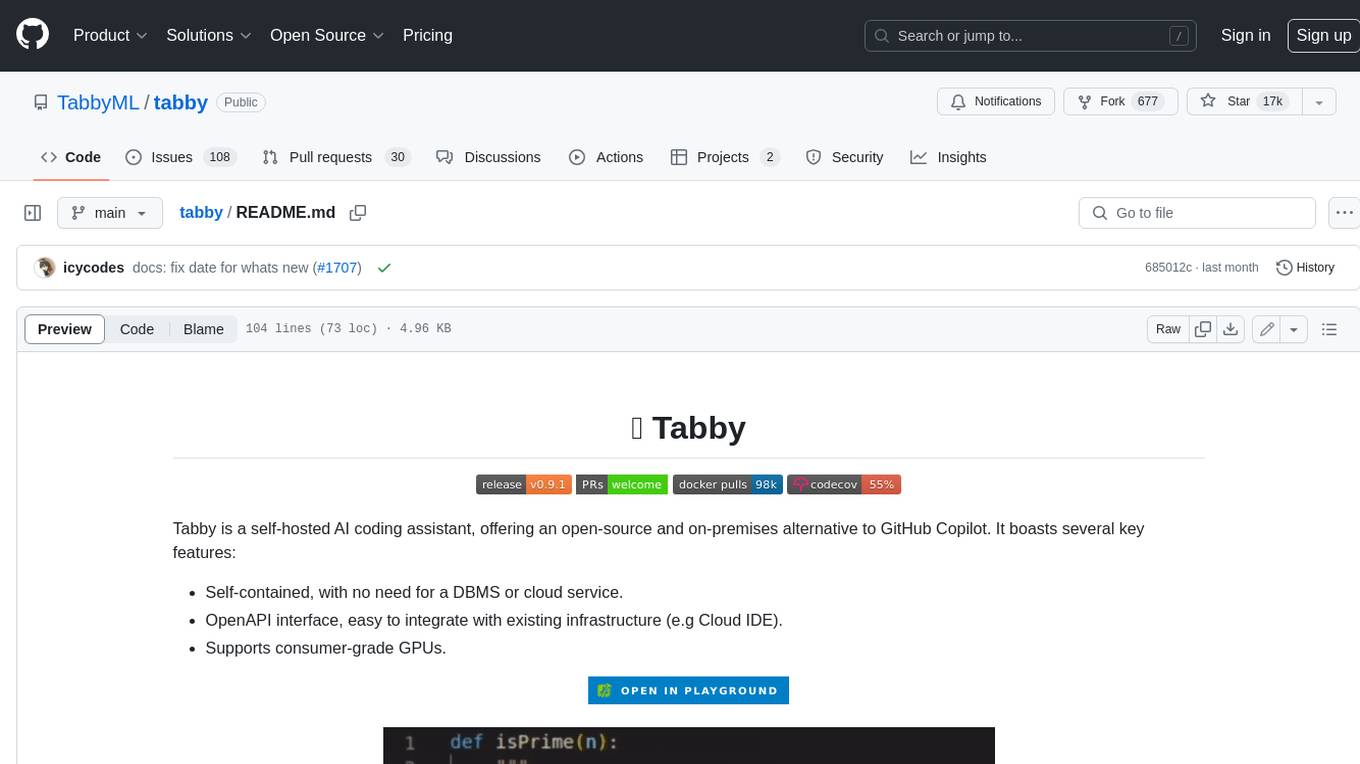
tabby
Tabby is a self-hosted AI coding assistant, offering an open-source and on-premises alternative to GitHub Copilot. It boasts several key features: * Self-contained, with no need for a DBMS or cloud service. * OpenAPI interface, easy to integrate with existing infrastructure (e.g Cloud IDE). * Supports consumer-grade GPUs.
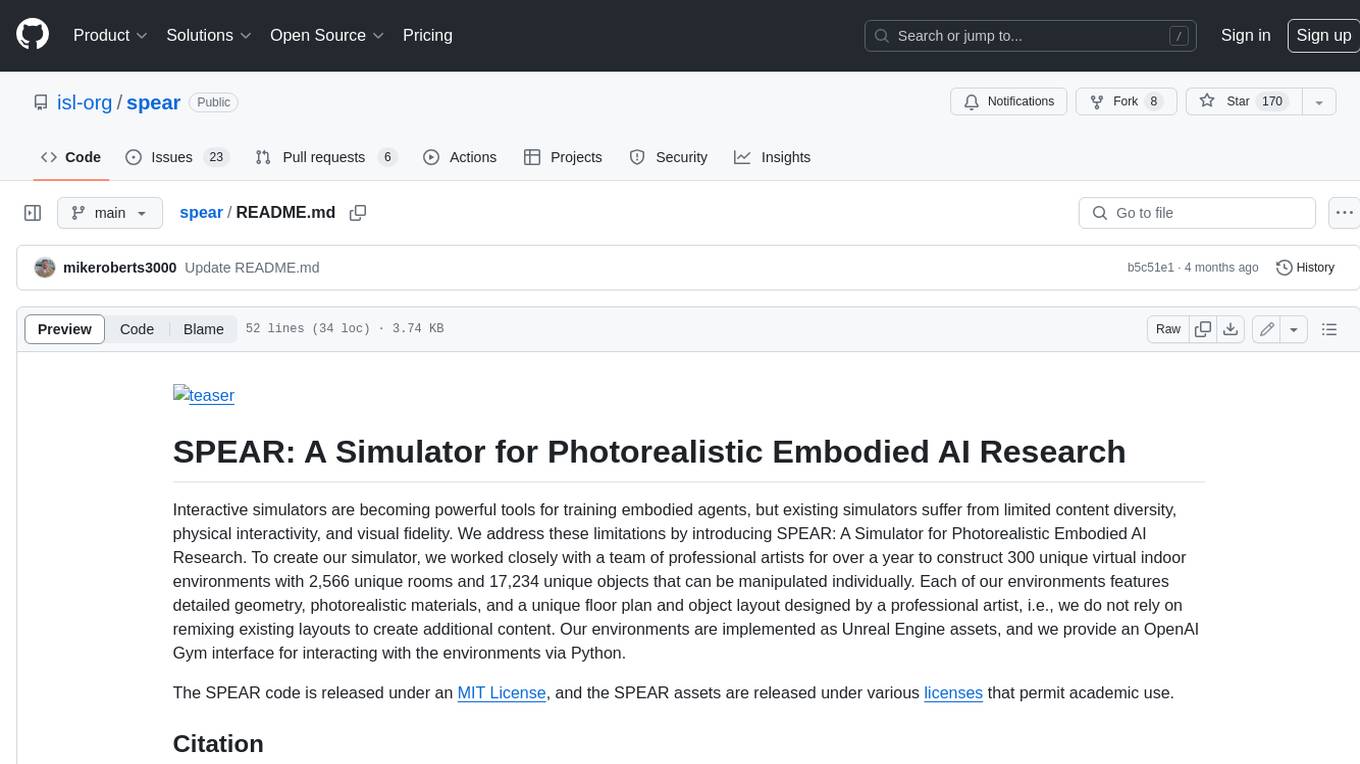
spear
SPEAR (Simulator for Photorealistic Embodied AI Research) is a powerful tool for training embodied agents. It features 300 unique virtual indoor environments with 2,566 unique rooms and 17,234 unique objects that can be manipulated individually. Each environment is designed by a professional artist and features detailed geometry, photorealistic materials, and a unique floor plan and object layout. SPEAR is implemented as Unreal Engine assets and provides an OpenAI Gym interface for interacting with the environments via Python.
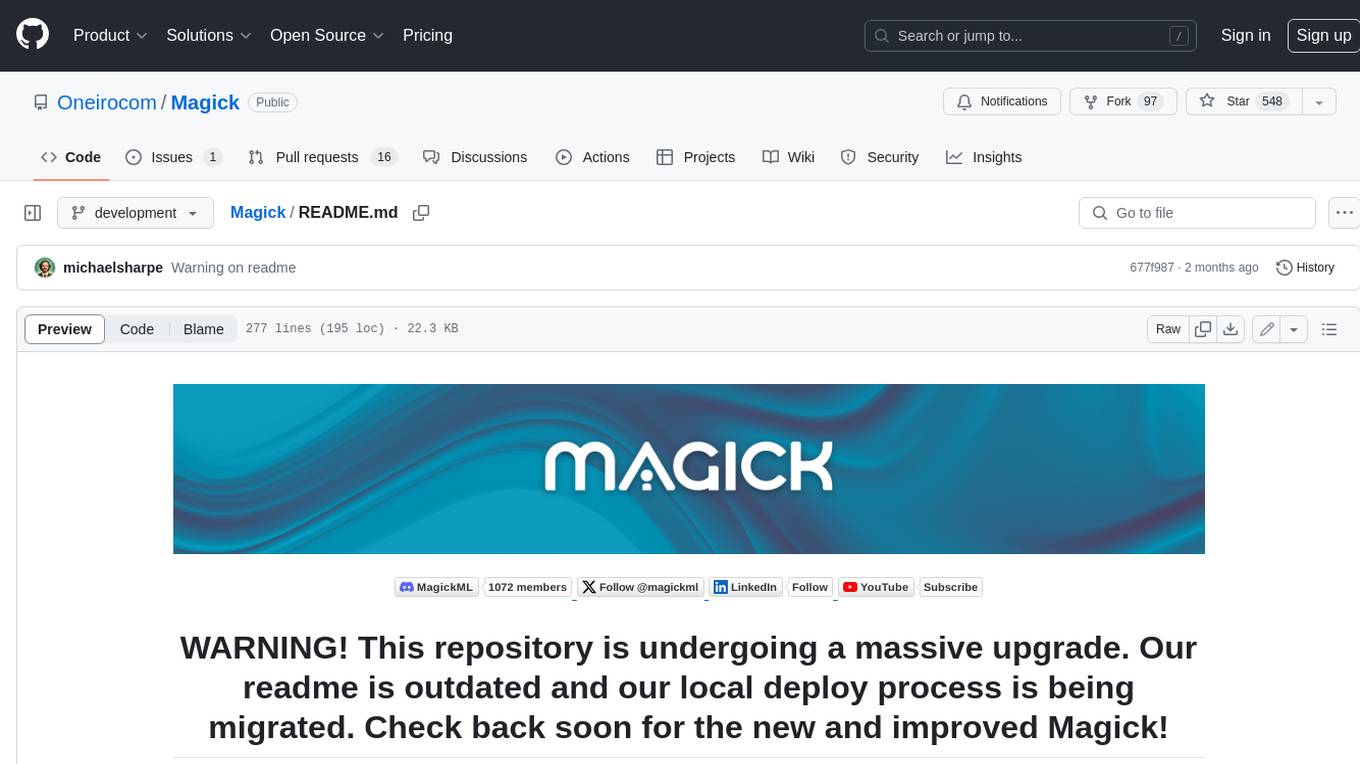
Magick
Magick is a groundbreaking visual AIDE (Artificial Intelligence Development Environment) for no-code data pipelines and multimodal agents. Magick can connect to other services and comes with nodes and templates well-suited for intelligent agents, chatbots, complex reasoning systems and realistic characters.