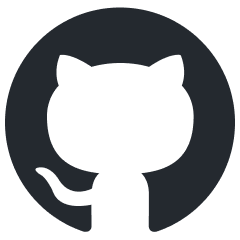
Awesome-LLM
Awesome-LLM: a curated list of Large Language Model
Stars: 22089
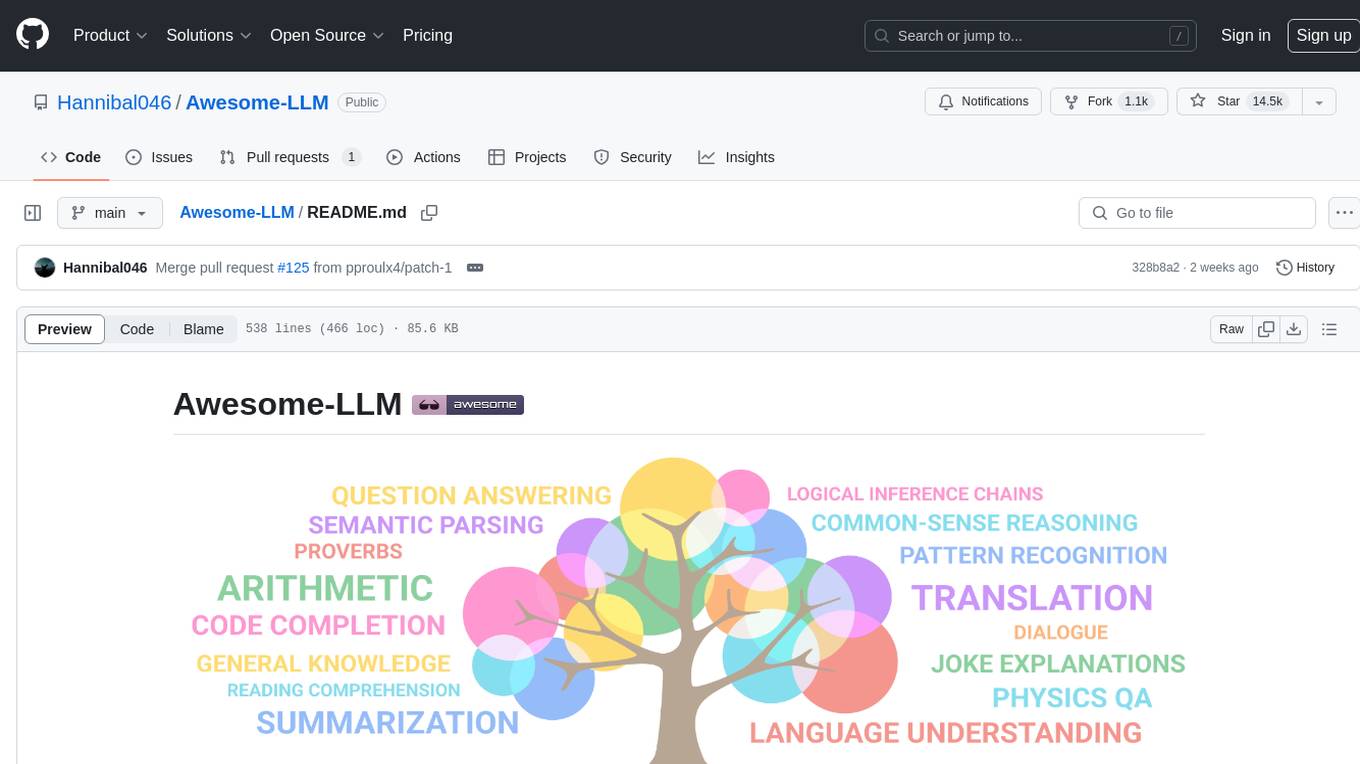
Awesome-LLM is a curated list of resources related to large language models, focusing on papers, projects, frameworks, tools, tutorials, courses, opinions, and other useful resources in the field. It covers trending LLM projects, milestone papers, other papers, open LLM projects, LLM training frameworks, LLM evaluation frameworks, tools for deploying LLM, prompting libraries & tools, tutorials, courses, books, and opinions. The repository provides a comprehensive overview of the latest advancements and resources in the field of large language models.
README:
🔥 Large Language Models(LLM) have taken the NLP community AI community the Whole World by storm. Here is a curated list of papers about large language models, especially relating to ChatGPT. It also contains frameworks for LLM training, tools to deploy LLM, courses and tutorials about LLM and all publicly available LLM checkpoints and APIs.
- TinyZero - Clean, minimal, accessible reproduction of DeepSeek R1-Zero
- open-r1 - Fully open reproduction of DeepSeek-R1
- DeepSeek-R1 - First-generation reasoning models from DeepSeek.
- Qwen2.5-Max - Exploring the Intelligence of Large-scale MoE Model.
- OpenAI o3-mini - Pushing the frontier of cost-effective reasoning.
- DeepSeek-V3 - First open-sourced GPT-4o level model.
milestone papers
[!NOTE] If you're interested in the field of LLM, you may find the above list of milestone papers helpful to explore its history and state-of-the-art. However, each direction of LLM offers a unique set of insights and contributions, which are essential to understanding the field as a whole. For a detailed list of papers in various subfields, please refer to the following link:
other papers
-
Awesome-LLM-hallucination - LLM hallucination paper list.
-
awesome-hallucination-detection - List of papers on hallucination detection in LLMs.
-
LLMsPracticalGuide - A curated list of practical guide resources of LLMs
-
Awesome ChatGPT Prompts - A collection of prompt examples to be used with the ChatGPT model.
-
awesome-chatgpt-prompts-zh - A Chinese collection of prompt examples to be used with the ChatGPT model.
-
Awesome ChatGPT - Curated list of resources for ChatGPT and GPT-3 from OpenAI.
-
Chain-of-Thoughts Papers - A trend starts from "Chain of Thought Prompting Elicits Reasoning in Large Language Models.
-
Awesome Deliberative Prompting - How to ask LLMs to produce reliable reasoning and make reason-responsive decisions.
-
Instruction-Tuning-Papers - A trend starts from
Natrural-Instruction
(ACL 2022),FLAN
(ICLR 2022) andT0
(ICLR 2022). -
LLM Reading List - A paper & resource list of large language models.
-
Reasoning using Language Models - Collection of papers and resources on Reasoning using Language Models.
-
Chain-of-Thought Hub - Measuring LLMs' Reasoning Performance
-
Awesome GPT - A curated list of awesome projects and resources related to GPT, ChatGPT, OpenAI, LLM, and more.
-
Awesome GPT-3 - a collection of demos and articles about the OpenAI GPT-3 API.
-
Awesome LLM Human Preference Datasets - a collection of human preference datasets for LLM instruction tuning, RLHF and evaluation.
-
RWKV-howto - possibly useful materials and tutorial for learning RWKV.
-
ModelEditingPapers - A paper & resource list on model editing for large language models.
-
Awesome LLM Security - A curation of awesome tools, documents and projects about LLM Security.
-
Awesome-Align-LLM-Human - A collection of papers and resources about aligning large language models (LLMs) with human.
-
Awesome-Code-LLM - An awesome and curated list of best code-LLM for research.
-
Awesome-LLM-Compression - Awesome LLM compression research papers and tools.
-
Awesome-LLM-Systems - Awesome LLM systems research papers.
-
awesome-llm-webapps - A collection of open source, actively maintained web apps for LLM applications.
-
awesome-japanese-llm - 日本語LLMまとめ - Overview of Japanese LLMs.
-
Awesome-LLM-Healthcare - The paper list of the review on LLMs in medicine.
-
Awesome-LLM-Inference - A curated list of Awesome LLM Inference Paper with codes.
-
Awesome-LLM-3D - A curated list of Multi-modal Large Language Model in 3D world, including 3D understanding, reasoning, generation, and embodied agents.
-
LLMDatahub - a curated collection of datasets specifically designed for chatbot training, including links, size, language, usage, and a brief description of each dataset
-
Awesome-Chinese-LLM - 整理开源的中文大语言模型,以规模较小、可私有化部署、训练成本较低的模型为主,包括底座模型,垂直领域微调及应用,数据集与教程等。
-
LLM4Opt - Applying Large language models (LLMs) for diverse optimization tasks (Opt) is an emerging research area. This is a collection of references and papers of LLM4Opt.
-
awesome-language-model-analysis - This paper list focuses on the theoretical or empirical analysis of language models, e.g., the learning dynamics, expressive capacity, interpretability, generalization, and other interesting topics.
- Chatbot Arena Leaderboard - a benchmark platform for large language models (LLMs) that features anonymous, randomized battles in a crowdsourced manner.
- LiveBench - A Challenging, Contamination-Free LLM Benchmark.
- Open LLM Leaderboard - aims to track, rank, and evaluate LLMs and chatbots as they are released.
- AlpacaEval - An Automatic Evaluator for Instruction-following Language Models using Nous benchmark suite.
other leaderboards
- ACLUE - an evaluation benchmark focused on ancient Chinese language comprehension.
- BeHonest - A pioneering benchmark specifically designed to assess honesty in LLMs comprehensively.
- Berkeley Function-Calling Leaderboard - evaluates LLM's ability to call external functions/tools.
- Chinese Large Model Leaderboard - an expert-driven benchmark for Chineses LLMs.
- CompassRank - CompassRank is dedicated to exploring the most advanced language and visual models, offering a comprehensive, objective, and neutral evaluation reference for the industry and research.
- CompMix - a benchmark evaluating QA methods that operate over a mixture of heterogeneous input sources (KB, text, tables, infoboxes).
- DreamBench++ - a benchmark for evaluating the performance of large language models (LLMs) in various tasks related to both textual and visual imagination.
- FELM - a meta-benchmark that evaluates how well factuality evaluators assess the outputs of large language models (LLMs).
- InfiBench - a benchmark designed to evaluate large language models (LLMs) specifically in their ability to answer real-world coding-related questions.
- LawBench - a benchmark designed to evaluate large language models in the legal domain.
- LLMEval - focuses on understanding how these models perform in various scenarios and analyzing results from an interpretability perspective.
- M3CoT - a benchmark that evaluates large language models on a variety of multimodal reasoning tasks, including language, natural and social sciences, physical and social commonsense, temporal reasoning, algebra, and geometry.
- MathEval - a comprehensive benchmarking platform designed to evaluate large models' mathematical abilities across 20 fields and nearly 30,000 math problems.
- MixEval - a ground-truth-based dynamic benchmark derived from off-the-shelf benchmark mixtures, which evaluates LLMs with a highly capable model ranking (i.e., 0.96 correlation with Chatbot Arena) while running locally and quickly (6% the time and cost of running MMLU).
- MMedBench - a benchmark that evaluates large language models' ability to answer medical questions across multiple languages.
- MMToM-QA - a multimodal question-answering benchmark designed to evaluate AI models' cognitive ability to understand human beliefs and goals.
- OlympicArena - a benchmark for evaluating AI models across multiple academic disciplines like math, physics, chemistry, biology, and more.
- PubMedQA - a biomedical question-answering benchmark designed for answering research-related questions using PubMed abstracts.
- SciBench - benchmark designed to evaluate large language models (LLMs) on solving complex, college-level scientific problems from domains like chemistry, physics, and mathematics.
- SuperBench - a benchmark platform designed for evaluating large language models (LLMs) on a range of tasks, particularly focusing on their performance in different aspects such as natural language understanding, reasoning, and generalization.
- SuperLim - a Swedish language understanding benchmark that evaluates natural language processing (NLP) models on various tasks such as argumentation analysis, semantic similarity, and textual entailment.
- TAT-DQA - a large-scale Document Visual Question Answering (VQA) dataset designed for complex document understanding, particularly in financial reports.
- TAT-QA - a large-scale question-answering benchmark focused on real-world financial data, integrating both tabular and textual information.
- VisualWebArena - a benchmark designed to assess the performance of multimodal web agents on realistic visually grounded tasks.
- We-Math - a benchmark that evaluates large multimodal models (LMMs) on their ability to perform human-like mathematical reasoning.
- WHOOPS! - a benchmark dataset testing AI's ability to reason about visual commonsense through images that defy normal expectations.
DeepSeek
Alibaba
- Qwen-1.8B|7B|14B|72B
- Qwen1.5-0.5B|1.8B|4B|7B|14B|32B|72B|110B|MoE-A2.7B
- Qwen2-0.5B|1.5B|7B|57B-A14B-MoE|72B
- Qwen2.5-0.5B|1.5B|3B|7B|14B|32B|72B
- CodeQwen1.5-7B
- Qwen2.5-Coder-1.5B|7B|32B
- Qwen2-Math-1.5B|7B|72B
- Qwen2.5-Math-1.5B|7B|72B
- Qwen-VL-7B
- Qwen2-VL-2B|7B|72B
- Qwen2-Audio-7B
- Qwen2.5-VL-3|7|72B
- Qwen2.5-1M-7|14B
Meta
Mistral AI
Apple
Microsoft
AllenAI
xAI
Cohere
01-ai
Baichuan
Nvidia
BLOOM
Zhipu AI
RWKV Foundation
- RWKV-v4|5|6minicpm-2b-65d48bf958302b9fd25b698f)
ElutherAI
Stability AI
BigCode
DataBricks
Shanghai AI Laboratory
Reference: LLMDataHub
- IBM data-prep-kit - Open-Source Toolkit for Efficient Unstructured Data Processing with Pre-built Modules and Local to Cluster Scalability.
- Datatrove - Freeing data processing from scripting madness by providing a set of platform-agnostic customizable pipeline processing blocks.
- Dingo - Dingo: A Comprehensive Data Quality Evaluation Tool
- lm-evaluation-harness - A framework for few-shot evaluation of language models.
- lighteval - a lightweight LLM evaluation suite that Hugging Face has been using internally.
- simple-evals - Eval tools by OpenAI.
other evaluation frameworks
- OLMO-eval - a repository for evaluating open language models.
- MixEval - A reliable click-and-go evaluation suite compatible with both open-source and proprietary models, supporting MixEval and other benchmarks.
- HELM - Holistic Evaluation of Language Models (HELM), a framework to increase the transparency of language models.
- instruct-eval - This repository contains code to quantitatively evaluate instruction-tuned models such as Alpaca and Flan-T5 on held-out tasks.
- Giskard - Testing & evaluation library for LLM applications, in particular RAGs
- LangSmith - a unified platform from LangChain framework for: evaluation, collaboration HITL (Human In The Loop), logging and monitoring LLM applications.
- Ragas - a framework that helps you evaluate your Retrieval Augmented Generation (RAG) pipelines.
- Meta Lingua - a lean, efficient, and easy-to-hack codebase to research LLMs.
- Litgpt - 20+ high-performance LLMs with recipes to pretrain, finetune and deploy at scale.
- nanotron - Minimalistic large language model 3D-parallelism training.
- DeepSpeed - DeepSpeed is a deep learning optimization library that makes distributed training and inference easy, efficient, and effective.
- Megatron-LM - Ongoing research training transformer models at scale.
- torchtitan - A native PyTorch Library for large model training.
other frameworks
- Megatron-DeepSpeed - DeepSpeed version of NVIDIA's Megatron-LM that adds additional support for several features such as MoE model training, Curriculum Learning, 3D Parallelism, and others.
- torchtune - A Native-PyTorch Library for LLM Fine-tuning.
- veRL - veRL is a flexible and efficient RL framework for LLMs.
- NeMo Framework - Generative AI framework built for researchers and PyTorch developers working on Large Language Models (LLMs), Multimodal Models (MMs), Automatic Speech Recognition (ASR), Text to Speech (TTS), and Computer Vision (CV) domains.
- Colossal-AI - Making large AI models cheaper, faster, and more accessible.
- BMTrain - Efficient Training for Big Models.
- Mesh Tensorflow - Mesh TensorFlow: Model Parallelism Made Easier.
- maxtext - A simple, performant and scalable Jax LLM!
- GPT-NeoX - An implementation of model parallel autoregressive transformers on GPUs, based on the DeepSpeed library.
- Transformer Engine - A library for accelerating Transformer model training on NVIDIA GPUs.
- OpenRLHF - An Easy-to-use, Scalable and High-performance RLHF Framework (70B+ PPO Full Tuning & Iterative DPO & LoRA & RingAttention & RFT).
- TRL - TRL is a full stack library where we provide a set of tools to train transformer language models with Reinforcement Learning, from the Supervised Fine-tuning step (SFT), Reward Modeling step (RM) to the Proximal Policy Optimization (PPO) step.
- unslothai - A framework that specializes in efficient fine-tuning. On its GitHub page, you can find ready-to-use fine-tuning templates for various LLMs, allowing you to easily train your own data for free on the Google Colab cloud.
Reference: llm-inference-solutions
- SGLang - SGLang is a fast serving framework for large language models and vision language models.
- vLLM - A high-throughput and memory-efficient inference and serving engine for LLMs.
- llama.cpp - LLM inference in C/C++.
- ollama - Get up and running with Llama 3, Mistral, Gemma, and other large language models.
- TGI - a toolkit for deploying and serving Large Language Models (LLMs).
- TensorRT-LLM - Nvidia Framework for LLM Inference
other deployment tools
- FasterTransformer - NVIDIA Framework for LLM Inference(Transitioned to TensorRT-LLM)
- MInference - To speed up Long-context LLMs' inference, approximate and dynamic sparse calculate the attention, which reduces inference latency by up to 10x for pre-filling on an A100 while maintaining accuracy.
- exllama - A more memory-efficient rewrite of the HF transformers implementation of Llama for use with quantized weights.
- FastChat - A distributed multi-model LLM serving system with web UI and OpenAI-compatible RESTful APIs.
- mistral.rs - Blazingly fast LLM inference.
- SkyPilot - Run LLMs and batch jobs on any cloud. Get maximum cost savings, highest GPU availability, and managed execution -- all with a simple interface.
- Haystack - an open-source NLP framework that allows you to use LLMs and transformer-based models from Hugging Face, OpenAI and Cohere to interact with your own data.
- OpenLLM - Fine-tune, serve, deploy, and monitor any open-source LLMs in production. Used in production at BentoML for LLMs-based applications.
- DeepSpeed-Mii - MII makes low-latency and high-throughput inference, similar to vLLM powered by DeepSpeed.
- Text-Embeddings-Inference - Inference for text-embeddings in Rust, HFOIL Licence.
- Infinity - Inference for text-embeddings in Python
- LMDeploy - A high-throughput and low-latency inference and serving framework for LLMs and VLs
- Liger-Kernel - Efficient Triton Kernels for LLM Training.
Reference: awesome-llm-apps
- dspy - DSPy: The framework for programming—not prompting—foundation models.
- LangChain — A popular Python/JavaScript library for chaining sequences of language model prompts.
- LlamaIndex — A Python library for augmenting LLM apps with data.
more applications
-
MLflow - MLflow: An open-source framework for the end-to-end machine learning lifecycle, helping developers track experiments, evaluate models/prompts, deploy models, and add observability with tracing.
-
Swiss Army Llama - Comprehensive set of tools for working with local LLMs for various tasks.
-
LiteChain - Lightweight alternative to LangChain for composing LLMs
-
magentic - Seamlessly integrate LLMs as Python functions
-
wechat-chatgpt - Use ChatGPT On Wechat via wechaty
-
promptfoo - Test your prompts. Evaluate and compare LLM outputs, catch regressions, and improve prompt quality.
-
Agenta - Easily build, version, evaluate and deploy your LLM-powered apps.
-
Serge - a chat interface crafted with llama.cpp for running Alpaca models. No API keys, entirely self-hosted!
-
Langroid - Harness LLMs with Multi-Agent Programming
-
Embedchain - Framework to create ChatGPT like bots over your dataset.
-
Opik - Confidently evaluate, test, and ship LLM applications with a suite of observability tools to calibrate language model outputs across your dev and production lifecycle.
-
IntelliServer - simplifies the evaluation of LLMs by providing a unified microservice to access and test multiple AI models.
-
Langchain-Chatchat - Formerly langchain-ChatGLM, local knowledge based LLM (like ChatGLM) QA app with langchain.
-
Search with Lepton - Build your own conversational search engine using less than 500 lines of code by LeptonAI.
-
Robocorp - Create, deploy and operate Actions using Python anywhere to enhance your AI agents and assistants. Batteries included with an extensive set of libraries, helpers and logging.
-
Tune Studio - Playground for devs to finetune & deploy LLMs
-
LLocalSearch - Locally running websearch using LLM chains
-
AI Gateway — Gateway streamlines requests to 100+ open & closed source models with a unified API. It is also production-ready with support for caching, fallbacks, retries, timeouts, loadbalancing, and can be edge-deployed for minimum latency.
-
talkd.ai dialog - Simple API for deploying any RAG or LLM that you want adding plugins.
-
Wllama - WebAssembly binding for llama.cpp - Enabling in-browser LLM inference
-
GPUStack - An open-source GPU cluster manager for running LLMs
-
MNN-LLM -- A Device-Inference framework, including LLM Inference on device(Mobile Phone/PC/IOT)
-
CAMEL - First LLM Multi-agent framework.
-
QA-Pilot - An interactive chat project that leverages Ollama/OpenAI/MistralAI LLMs for rapid understanding and navigation of GitHub code repository or compressed file resources.
-
Shell-Pilot - Interact with LLM using Ollama models(or openAI, mistralAI)via pure shell scripts on your Linux(or MacOS) system, enhancing intelligent system management without any dependencies.
-
MindSQL - A python package for Txt-to-SQL with self hosting functionalities and RESTful APIs compatible with proprietary as well as open source LLM.
-
Langfuse - Open Source LLM Engineering Platform 🪢 Tracing, Evaluations, Prompt Management, Evaluations and Playground.
-
AdalFlow - AdalFlow: The library to build&auto-optimize LLM applications.
-
Guidance — A handy looking Python library from Microsoft that uses Handlebars templating to interleave generation, prompting, and logical control.
-
Evidently — An open-source framework to evaluate, test and monitor ML and LLM-powered systems.
-
Chainlit — A Python library for making chatbot interfaces.
-
Guardrails.ai — A Python library for validating outputs and retrying failures. Still in alpha, so expect sharp edges and bugs.
-
Semantic Kernel — A Python/C#/Java library from Microsoft that supports prompt templating, function chaining, vectorized memory, and intelligent planning.
-
Prompttools — Open-source Python tools for testing and evaluating models, vector DBs, and prompts.
-
Outlines — A Python library that provides a domain-specific language to simplify prompting and constrain generation.
-
Promptify — A small Python library for using language models to perform NLP tasks.
-
Scale Spellbook — A paid product for building, comparing, and shipping language model apps.
-
PromptPerfect — A paid product for testing and improving prompts.
-
Weights & Biases — A paid product for tracking model training and prompt engineering experiments.
-
OpenAI Evals — An open-source library for evaluating task performance of language models and prompts.
-
Arthur Shield — A paid product for detecting toxicity, hallucination, prompt injection, etc.
-
LMQL — A programming language for LLM interaction with support for typed prompting, control flow, constraints, and tools.
-
ModelFusion - A TypeScript library for building apps with LLMs and other ML models (speech-to-text, text-to-speech, image generation).
-
OneKE — A bilingual Chinese-English knowledge extraction model with knowledge graphs and natural language processing technologies.
-
llm-ui - A React library for building LLM UIs.
-
Wordware - A web-hosted IDE where non-technical domain experts work with AI Engineers to build task-specific AI agents. We approach prompting as a new programming language rather than low/no-code blocks.
-
Wallaroo.AI - Deploy, manage, optimize any model at scale across any environment from cloud to edge. Let's you go from python notebook to inferencing in minutes.
-
Dify - An open-source LLM app development platform with an intuitive interface that streamlines AI workflows, model management, and production deployment.
-
LazyLLM - An open-source LLM app for building multi-agent LLMs applications in an easy and lazy way, supports model deployment and fine-tuning.
-
MemFree - Open Source Hybrid AI Search Engine, Instantly Get Accurate Answers from the Internet, Bookmarks, Notes, and Docs. Support One-Click Deployment
-
AutoRAG - Open source AutoML tool for RAG. Optimize the RAG answer quality automatically. From generation evaluation datset to deploying optimized RAG pipeline.
-
Epsilla - An all-in-one LLM Agent platform with your private data and knowledge, delivers your production-ready AI Agents on Day 1.
-
Arize-Phoenix - Open-source tool for ML observability that runs in your notebook environment. Monitor and fine tune LLM, CV and Tabular Models.
-
LLM - A CLI utility and Python library for interacting with Large Language Models, both via remote APIs and models that can be installed and run on your own machine.
- Andrej Karpathy Series - My favorite!
- Umar Jamil Series - high quality and educational videos you don't want to miss.
- Alexander Rush Series - high quality and educational materials you don't want to miss.
- llm-course - Course to get into Large Language Models (LLMs) with roadmaps and Colab notebooks.
- UWaterloo CS 886 - Recent Advances on Foundation Models.
- CS25-Transformers United
- ChatGPT Prompt Engineering
- Princeton: Understanding Large Language Models
- CS324 - Large Language Models
- State of GPT
- A Visual Guide to Mamba and State Space Models
- Let's build GPT: from scratch, in code, spelled out.
- minbpe - Minimal, clean code for the Byte Pair Encoding (BPE) algorithm commonly used in LLM tokenization.
- femtoGPT - Pure Rust implementation of a minimal Generative Pretrained Transformer.
- Neurips2022-Foundational Robustness of Foundation Models
- ICML2022-Welcome to the "Big Model" Era: Techniques and Systems to Train and Serve Bigger Models
- GPT in 60 Lines of NumPy
- Generative AI with LangChain: Build large language model (LLM) apps with Python, ChatGPT, and other LLMs - it comes with a GitHub repository that showcases a lot of the functionality
- Build a Large Language Model (From Scratch) - A guide to building your own working LLM.
- BUILD GPT: HOW AI WORKS - explains how to code a Generative Pre-trained Transformer, or GPT, from scratch.
- Hands-On Large Language Models: Language Understanding and Generation - Explore the world of Large Language Models with over 275 custom made figures in this illustrated guide!
- The Chinese Book for Large Language Models - An Introductory LLM Textbook Based on A Survey of Large Language Models.
- Why did all of the public reproduction of GPT-3 fail?
- A Stage Review of Instruction Tuning
- LLM Powered Autonomous Agents
- Why you should work on AI AGENTS!
- Google "We Have No Moat, And Neither Does OpenAI"
- AI competition statement
- Prompt Engineering
- Noam Chomsky: The False Promise of ChatGPT
- Is ChatGPT 175 Billion Parameters? Technical Analysis
- The Next Generation Of Large Language Models
- Large Language Model Training in 2023
- How does GPT Obtain its Ability? Tracing Emergent Abilities of Language Models to their Sources
- Open Pretrained Transformers
- Scaling, emergence, and reasoning in large language models
- Emergent Mind - The latest AI news, curated & explained by GPT-4.
- ShareGPT - Share your wildest ChatGPT conversations with one click.
- Major LLMs + Data Availability
- 500+ Best AI Tools
- Cohere Summarize Beta - Introducing Cohere Summarize Beta: A New Endpoint for Text Summarization
- chatgpt-wrapper - ChatGPT Wrapper is an open-source unofficial Python API and CLI that lets you interact with ChatGPT.
- Cursor - Write, edit, and chat about your code with a powerful AI.
- AutoGPT - an experimental open-source application showcasing the capabilities of the GPT-4 language model.
- OpenAGI - When LLM Meets Domain Experts.
- EasyEdit - An easy-to-use framework to edit large language models.
- chatgpt-shroud - A Chrome extension for OpenAI's ChatGPT, enhancing user privacy by enabling easy hiding and unhiding of chat history. Ideal for privacy during screen shares.
This is an active repository and your contributions are always welcome!
I will keep some pull requests open if I'm not sure if they are awesome for LLM, you could vote for them by adding 👍 to them.
If you have any question about this opinionated list, do not hesitate to contact me [email protected].
For Tasks:
Click tags to check more tools for each tasksFor Jobs:
Alternative AI tools for Awesome-LLM
Similar Open Source Tools
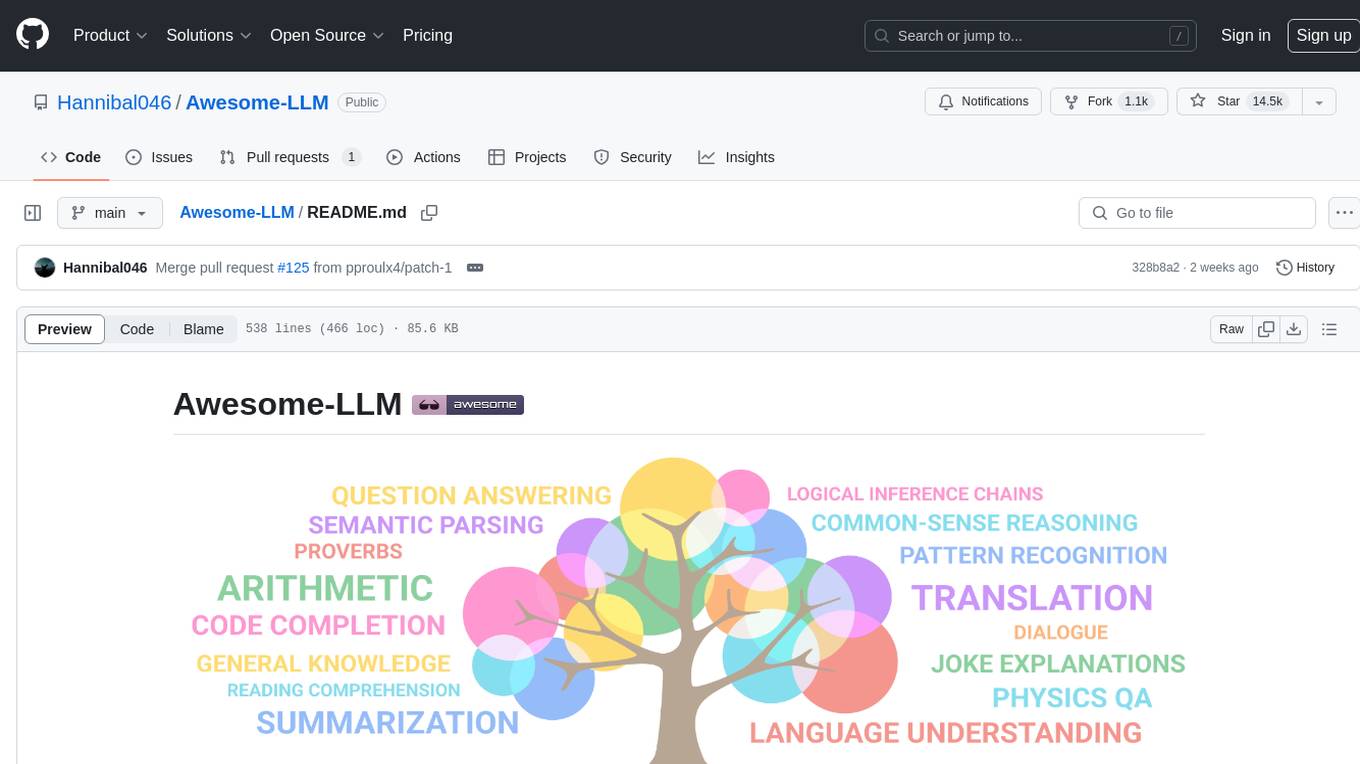
Awesome-LLM
Awesome-LLM is a curated list of resources related to large language models, focusing on papers, projects, frameworks, tools, tutorials, courses, opinions, and other useful resources in the field. It covers trending LLM projects, milestone papers, other papers, open LLM projects, LLM training frameworks, LLM evaluation frameworks, tools for deploying LLM, prompting libraries & tools, tutorials, courses, books, and opinions. The repository provides a comprehensive overview of the latest advancements and resources in the field of large language models.
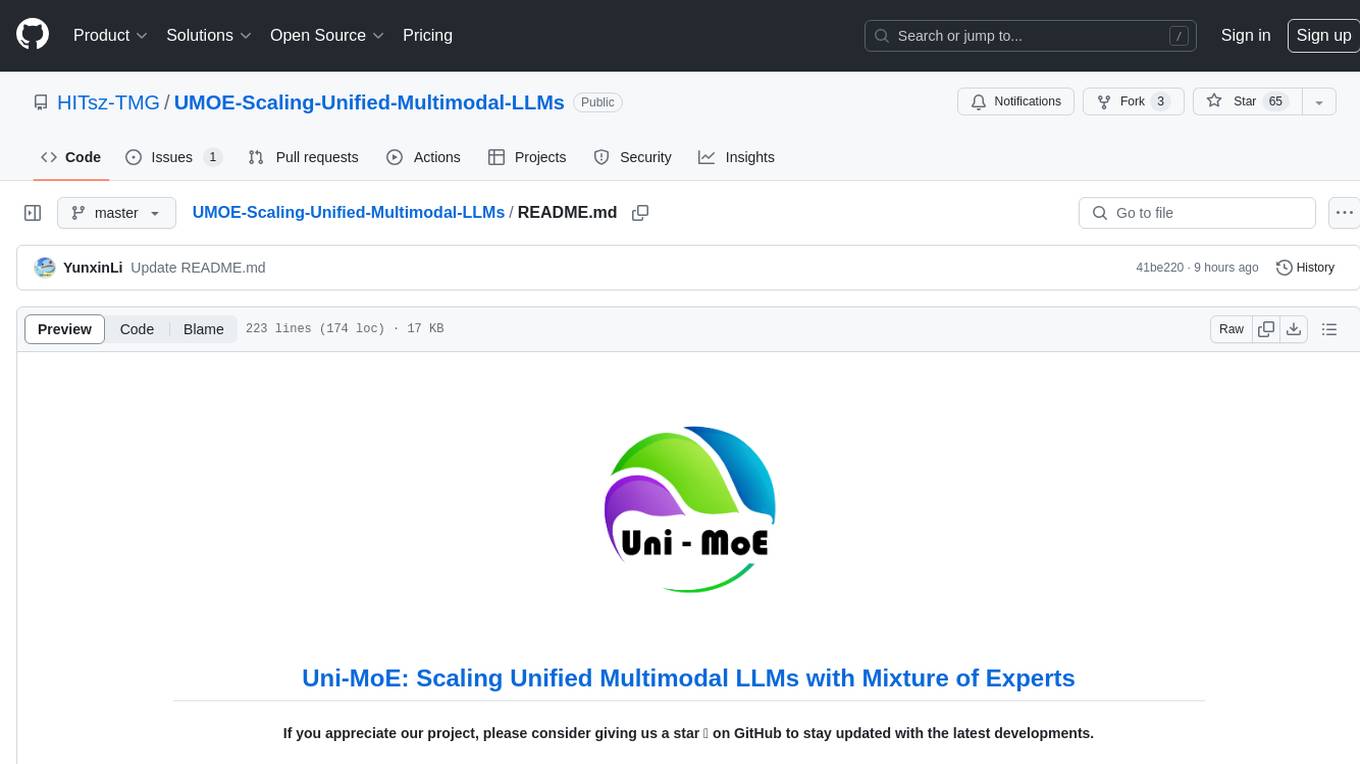
UMOE-Scaling-Unified-Multimodal-LLMs
Uni-MoE is a MoE-based unified multimodal model that can handle diverse modalities including audio, speech, image, text, and video. The project focuses on scaling Unified Multimodal LLMs with a Mixture of Experts framework. It offers enhanced functionality for training across multiple nodes and GPUs, as well as parallel processing at both the expert and modality levels. The model architecture involves three training stages: building connectors for multimodal understanding, developing modality-specific experts, and incorporating multiple trained experts into LLMs using the LoRA technique on mixed multimodal data. The tool provides instructions for installation, weights organization, inference, training, and evaluation on various datasets.
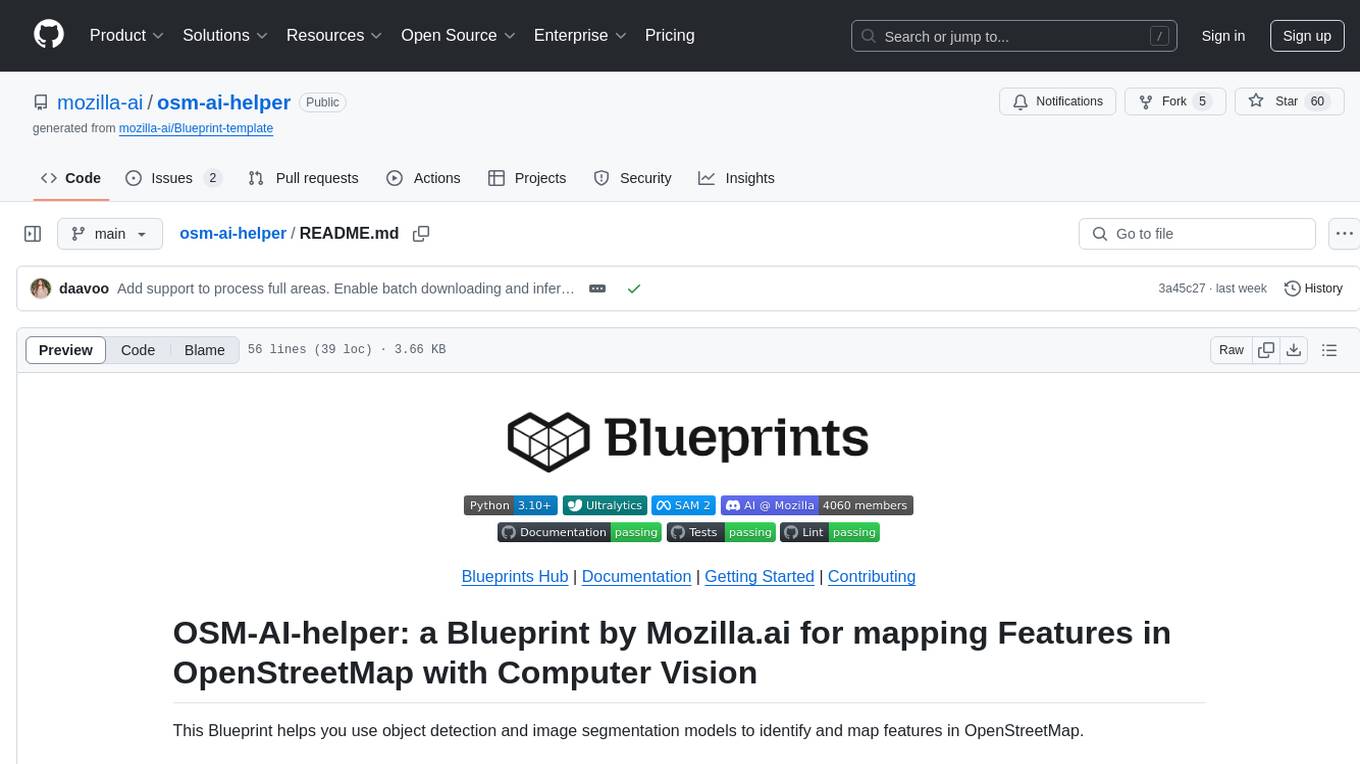
osm-ai-helper
OSM-AI-helper is a Blueprint by Mozilla.ai designed to assist users in mapping features in OpenStreetMap using object detection and image segmentation models. It provides tools for identifying and mapping various features, such as swimming pools, in OpenStreetMap. Users can also create custom datasets and fine-tune models for different use cases. The project is licensed under the AGPL-3.0 License and welcomes contributions from the community.
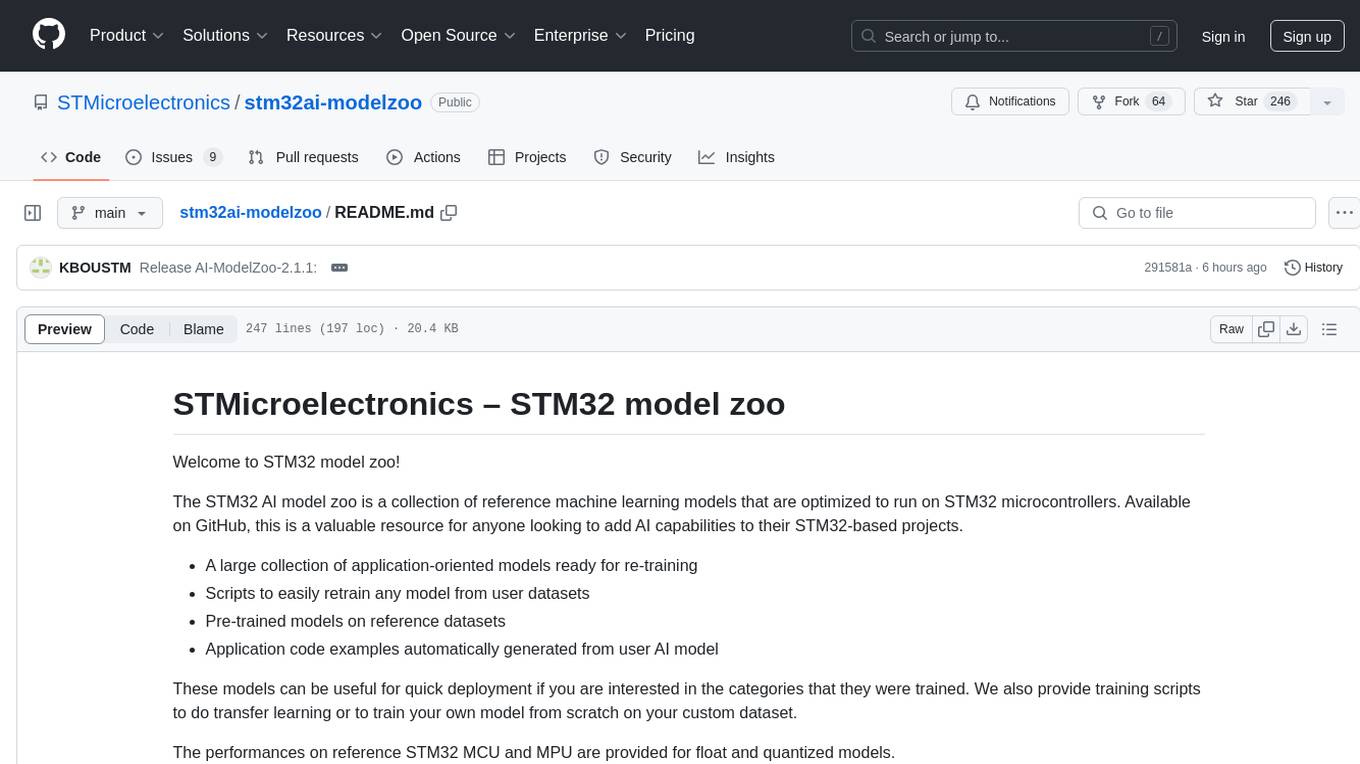
stm32ai-modelzoo
The STM32 AI model zoo is a collection of reference machine learning models optimized to run on STM32 microcontrollers. It provides a large collection of application-oriented models ready for re-training, scripts for easy retraining from user datasets, pre-trained models on reference datasets, and application code examples generated from user AI models. The project offers training scripts for transfer learning or training custom models from scratch. It includes performances on reference STM32 MCU and MPU for float and quantized models. The project is organized by application, providing step-by-step guides for training and deploying models.
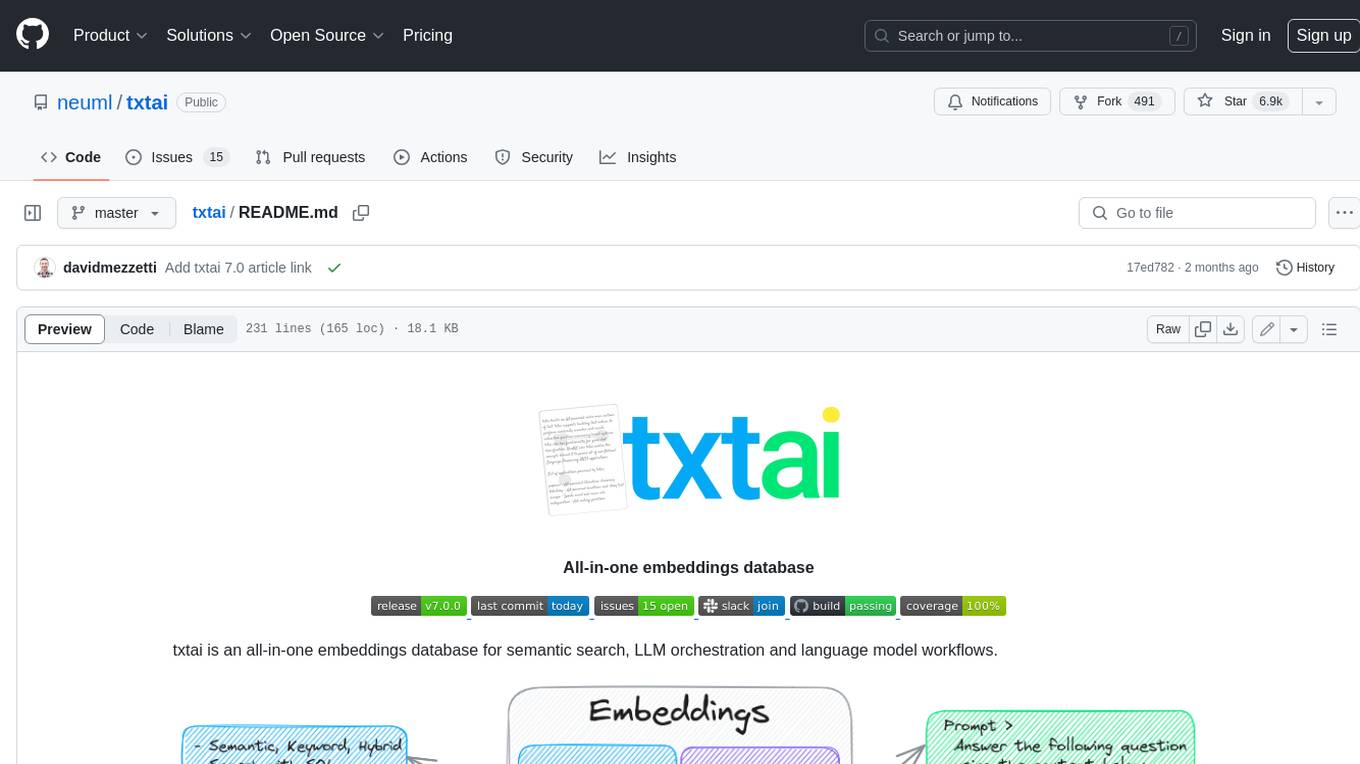
txtai
Txtai is an all-in-one embeddings database for semantic search, LLM orchestration, and language model workflows. It combines vector indexes, graph networks, and relational databases to enable vector search with SQL, topic modeling, retrieval augmented generation, and more. Txtai can stand alone or serve as a knowledge source for large language models (LLMs). Key features include vector search with SQL, object storage, topic modeling, graph analysis, multimodal indexing, embedding creation for various data types, pipelines powered by language models, workflows to connect pipelines, and support for Python, JavaScript, Java, Rust, and Go. Txtai is open-source under the Apache 2.0 license.
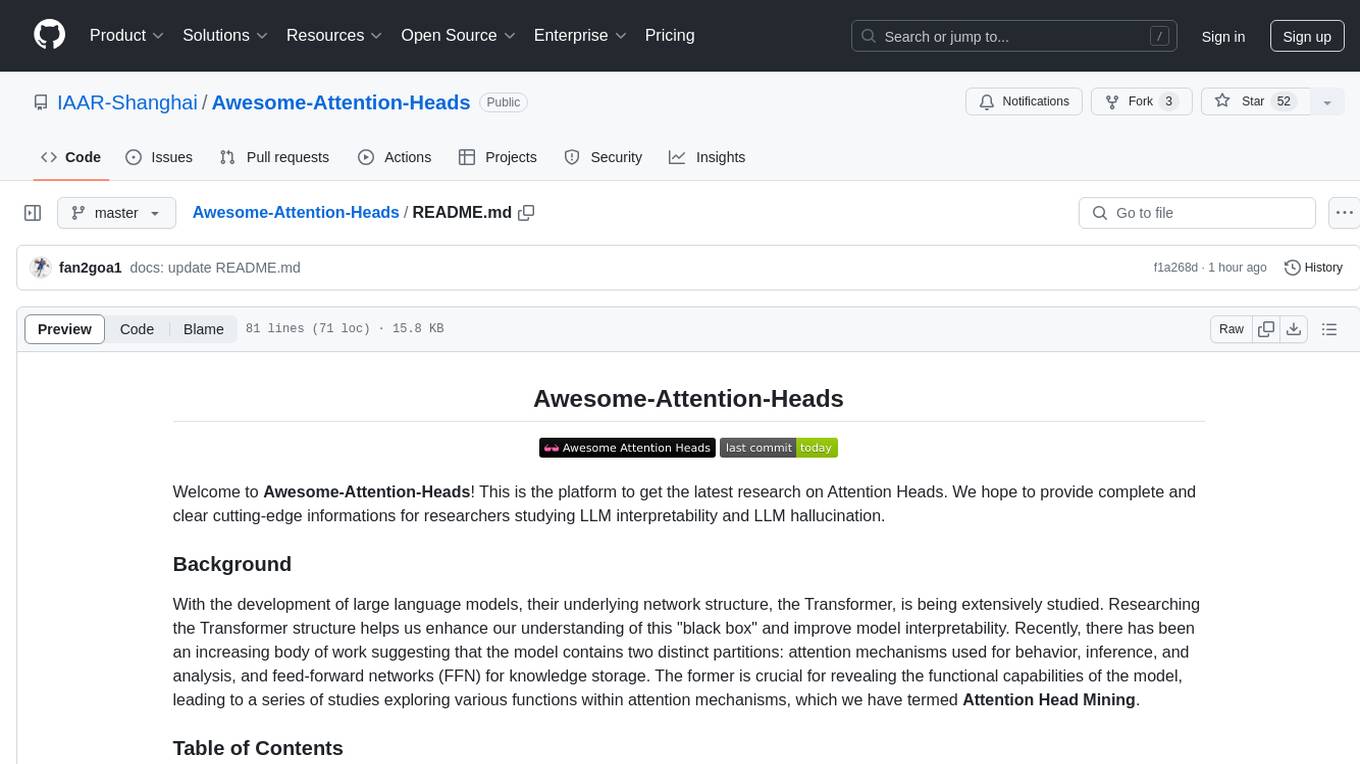
Awesome-Attention-Heads
Awesome-Attention-Heads is a platform providing the latest research on Attention Heads, focusing on enhancing understanding of Transformer structure for model interpretability. It explores attention mechanisms for behavior, inference, and analysis, alongside feed-forward networks for knowledge storage. The repository aims to support researchers studying LLM interpretability and hallucination by offering cutting-edge information on Attention Head Mining.
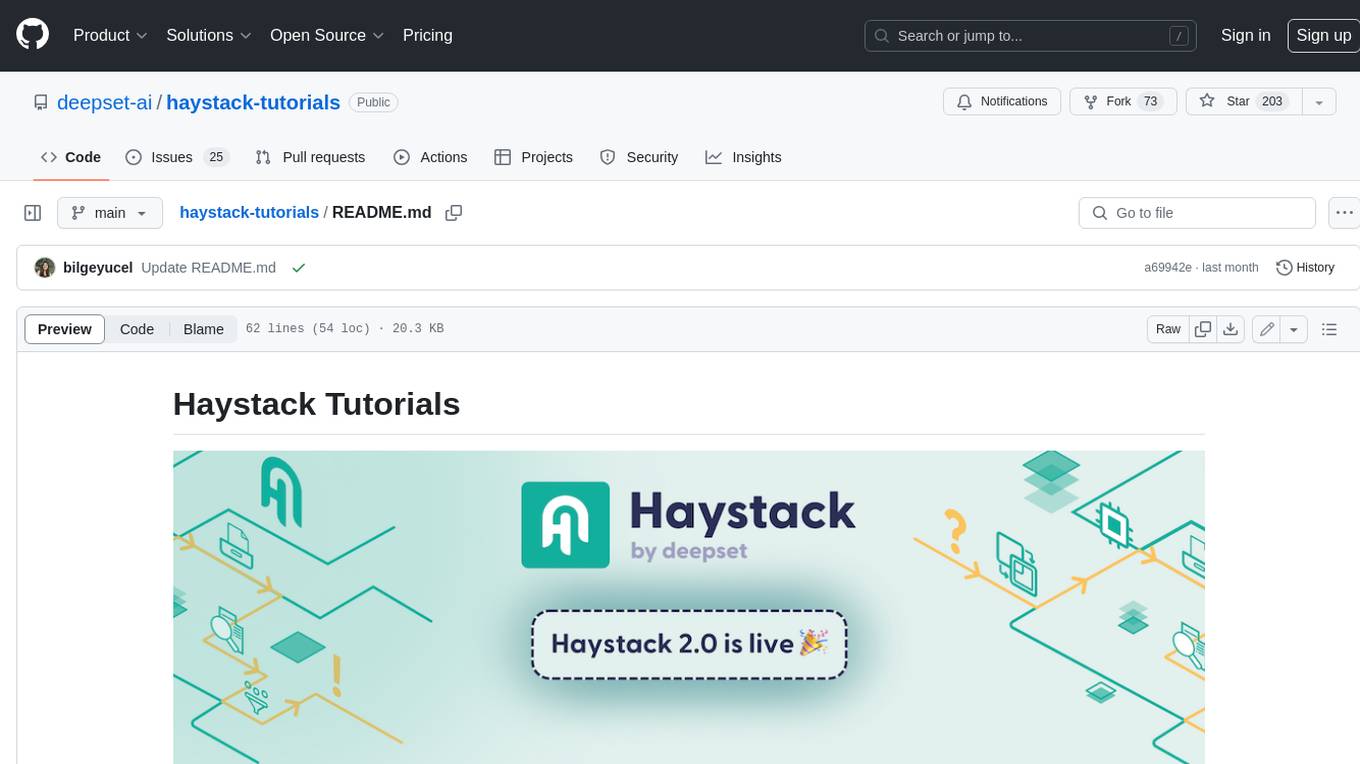
haystack-tutorials
Haystack is an open-source framework for building production-ready LLM applications, retrieval-augmented generative pipelines, and state-of-the-art search systems that work intelligently over large document collections. It lets you quickly try out the latest models in natural language processing (NLP) while being flexible and easy to use.
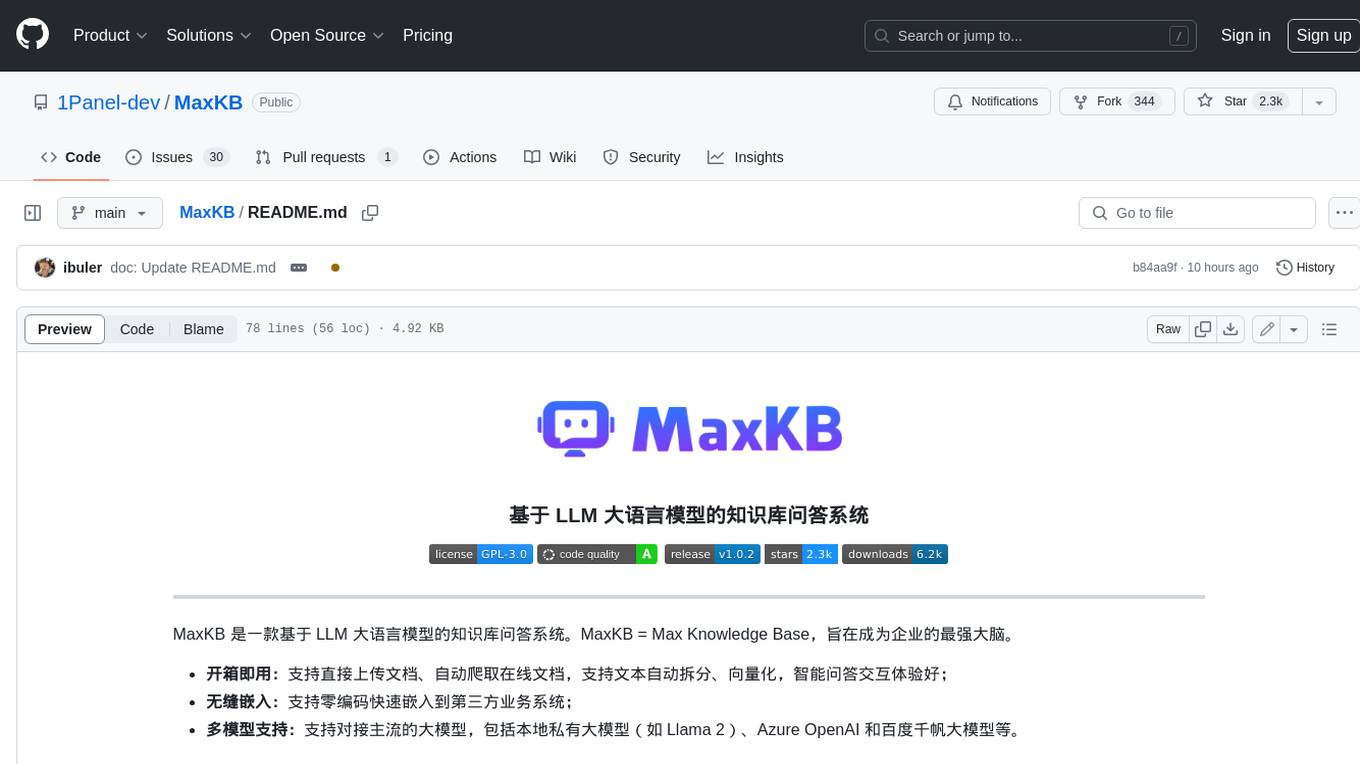
MaxKB
MaxKB is a knowledge base Q&A system based on the LLM large language model. MaxKB = Max Knowledge Base, which aims to become the most powerful brain of the enterprise.
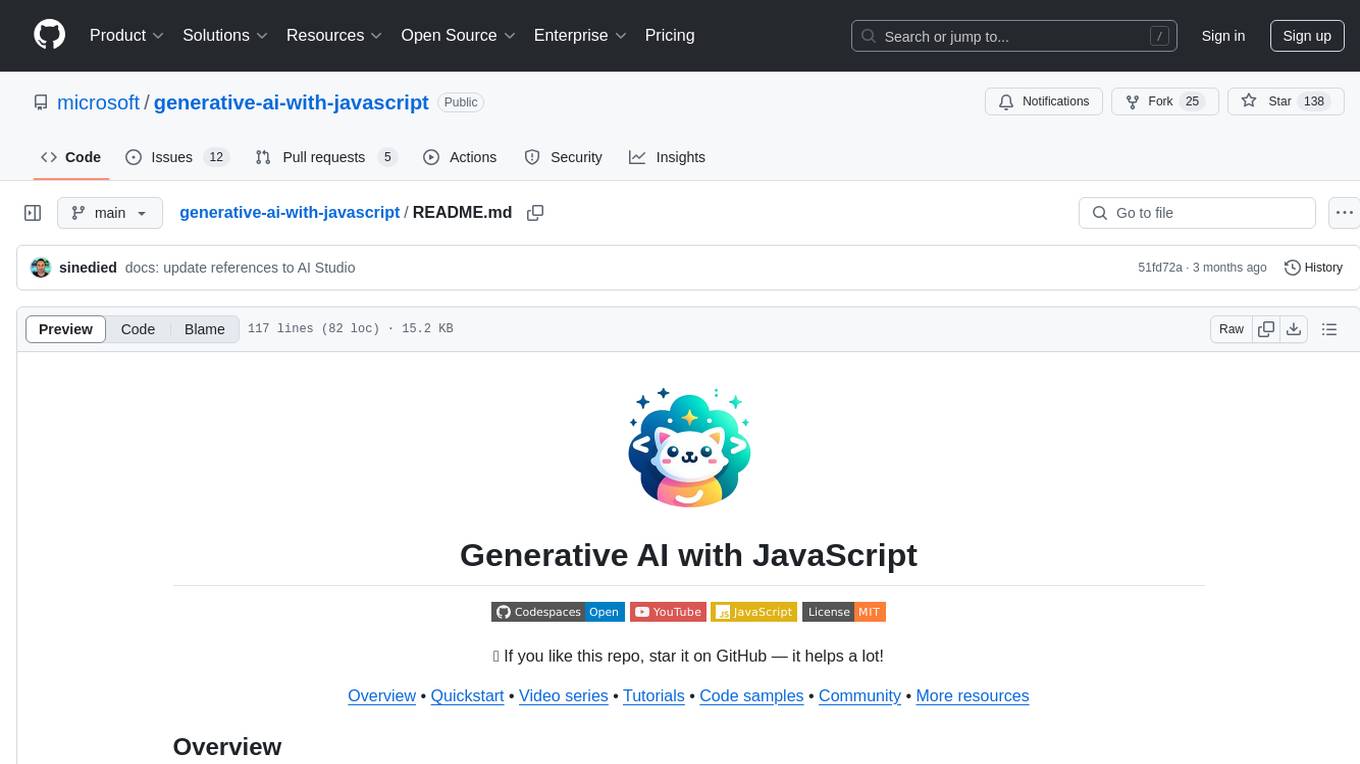
generative-ai-with-javascript
The 'Generative AI with JavaScript' repository is a comprehensive resource hub for JavaScript developers interested in delving into the world of Generative AI. It provides code samples, tutorials, and resources from a video series, offering best practices and tips to enhance AI skills. The repository covers the basics of generative AI, guides on building AI applications using JavaScript, from local development to deployment on Azure, and scaling AI models. It is a living repository with continuous updates, making it a valuable resource for both beginners and experienced developers looking to explore AI with JavaScript.
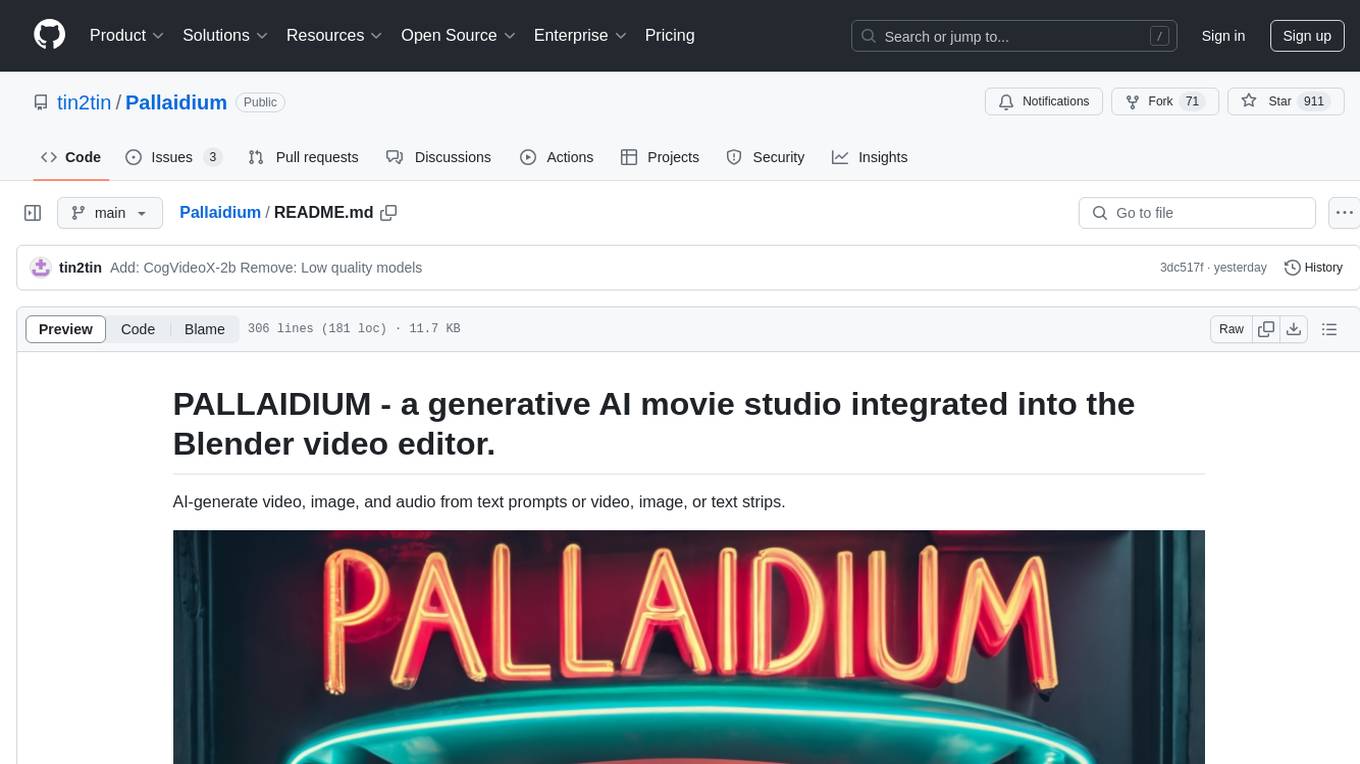
Pallaidium
Pallaidium is a generative AI movie studio integrated into the Blender video editor. It allows users to AI-generate video, image, and audio from text prompts or existing media files. The tool provides various features such as text to video, text to audio, text to speech, text to image, image to image, image to video, video to video, image to text, and more. It requires a Windows system with a CUDA-supported Nvidia card and at least 6 GB VRAM. Pallaidium offers batch processing capabilities, text to audio conversion using Bark, and various performance optimization tips. Users can install the tool by downloading the add-on and following the installation instructions provided. The tool comes with a set of restrictions on usage, prohibiting the generation of harmful, pornographic, violent, or false content.
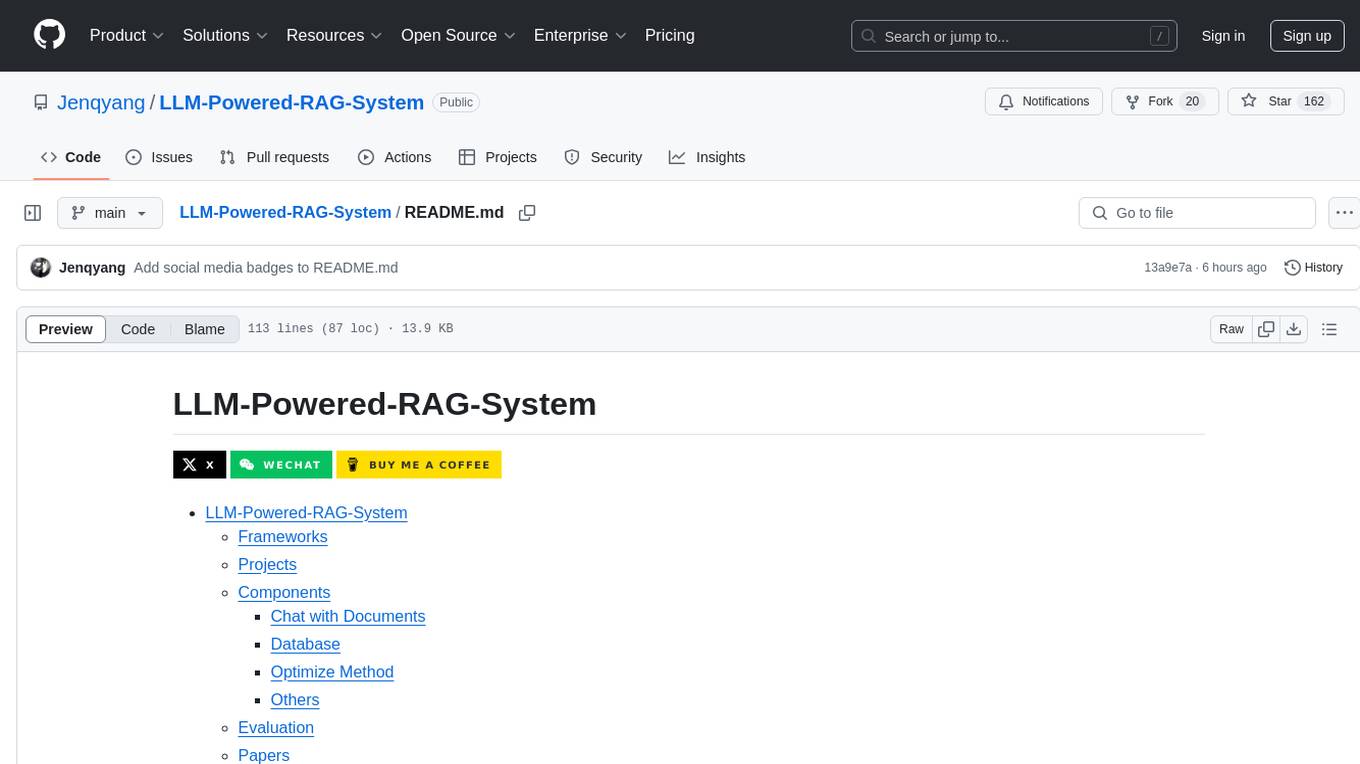
LLM-Powered-RAG-System
LLM-Powered-RAG-System is a comprehensive repository containing frameworks, projects, components, evaluation tools, papers, blogs, and other resources related to Retrieval-Augmented Generation (RAG) systems powered by Large Language Models (LLMs). The repository includes various frameworks for building applications with LLMs, data frameworks, modular graph-based RAG systems, dense retrieval models, and efficient retrieval augmentation and generation frameworks. It also features projects such as personal productivity assistants, knowledge-based platforms, chatbots, question and answer systems, and code assistants. Additionally, the repository provides components for interacting with documents, databases, and optimization methods using ML and LLM technologies. Evaluation frameworks, papers, blogs, and other resources related to RAG systems are also included.
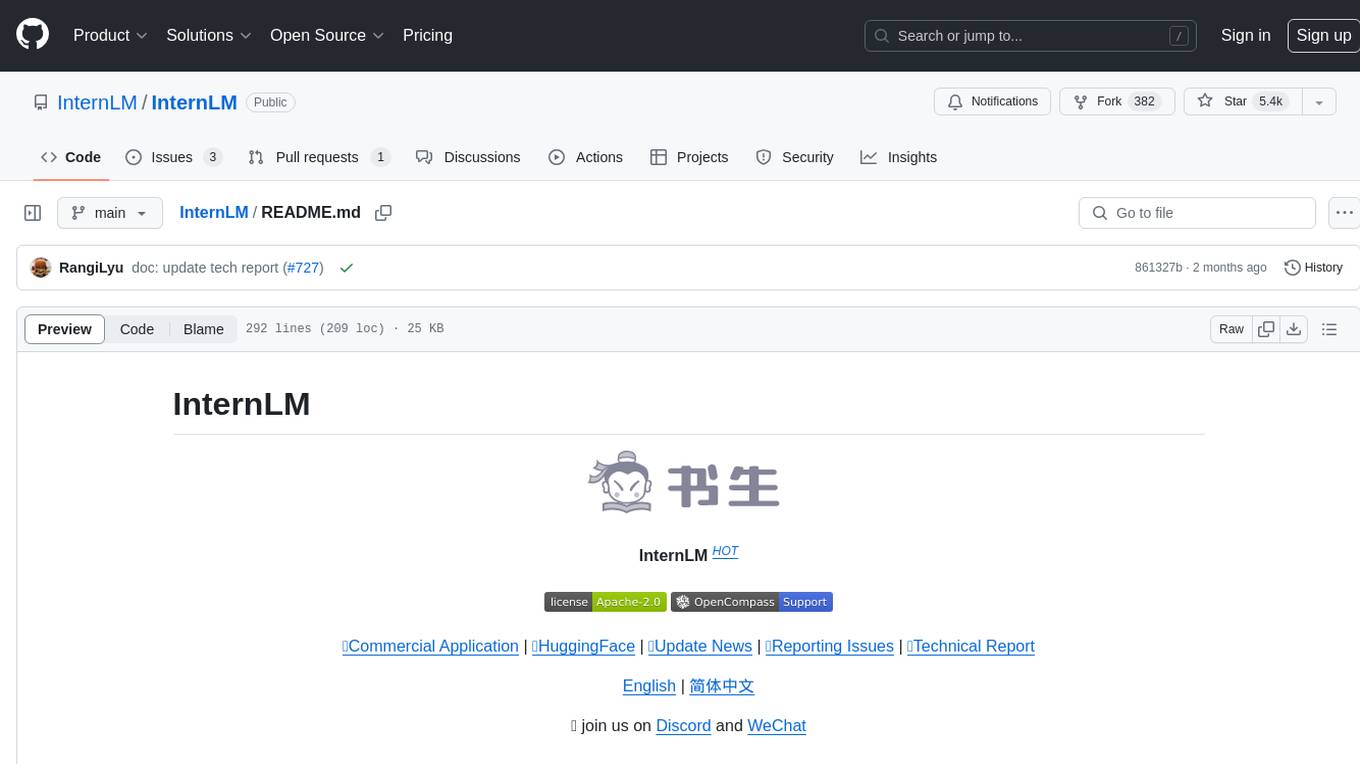
InternLM
InternLM is a powerful language model series with features such as 200K context window for long-context tasks, outstanding comprehensive performance in reasoning, math, code, chat experience, instruction following, and creative writing, code interpreter & data analysis capabilities, and stronger tool utilization capabilities. It offers models in sizes of 7B and 20B, suitable for research and complex scenarios. The models are recommended for various applications and exhibit better performance than previous generations. InternLM models may match or surpass other open-source models like ChatGPT. The tool has been evaluated on various datasets and has shown superior performance in multiple tasks. It requires Python >= 3.8, PyTorch >= 1.12.0, and Transformers >= 4.34 for usage. InternLM can be used for tasks like chat, agent applications, fine-tuning, deployment, and long-context inference.
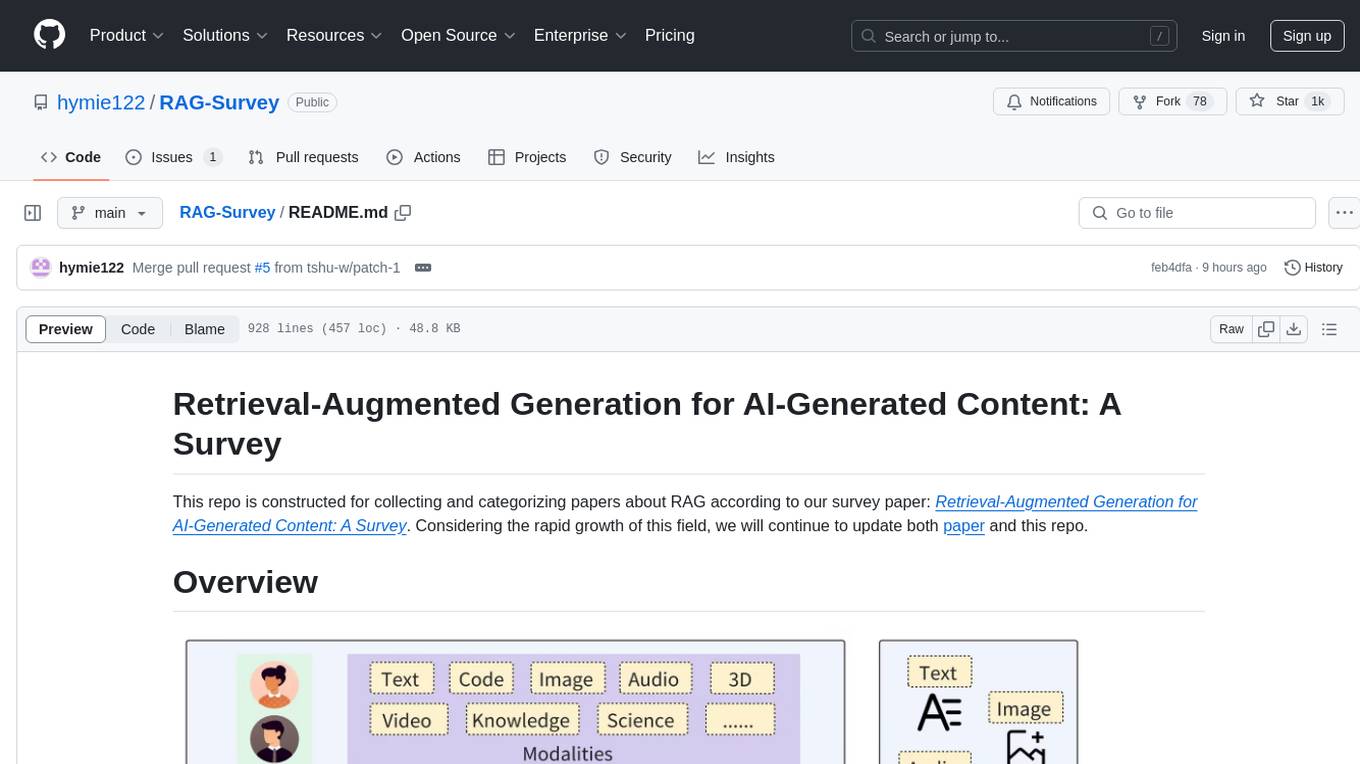
RAG-Survey
This repository is dedicated to collecting and categorizing papers related to Retrieval-Augmented Generation (RAG) for AI-generated content. It serves as a survey repository based on the paper 'Retrieval-Augmented Generation for AI-Generated Content: A Survey'. The repository is continuously updated to keep up with the rapid growth in the field of RAG.
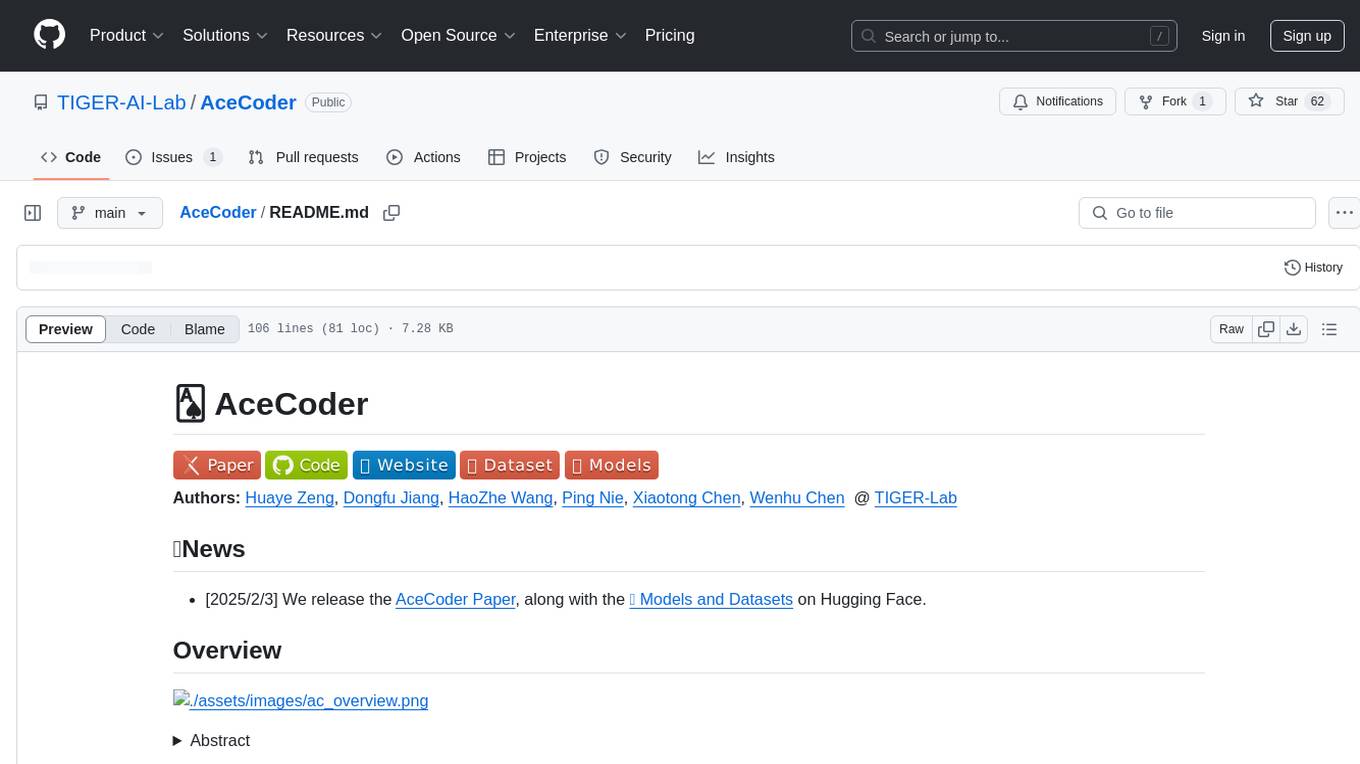
AceCoder
AceCoder is a tool that introduces a fully automated pipeline for synthesizing large-scale reliable tests used for reward model training and reinforcement learning in the coding scenario. It curates datasets, trains reward models, and performs RL training to improve coding abilities of language models. The tool aims to unlock the potential of RL training for code generation models and push the boundaries of LLM's coding abilities.
For similar tasks
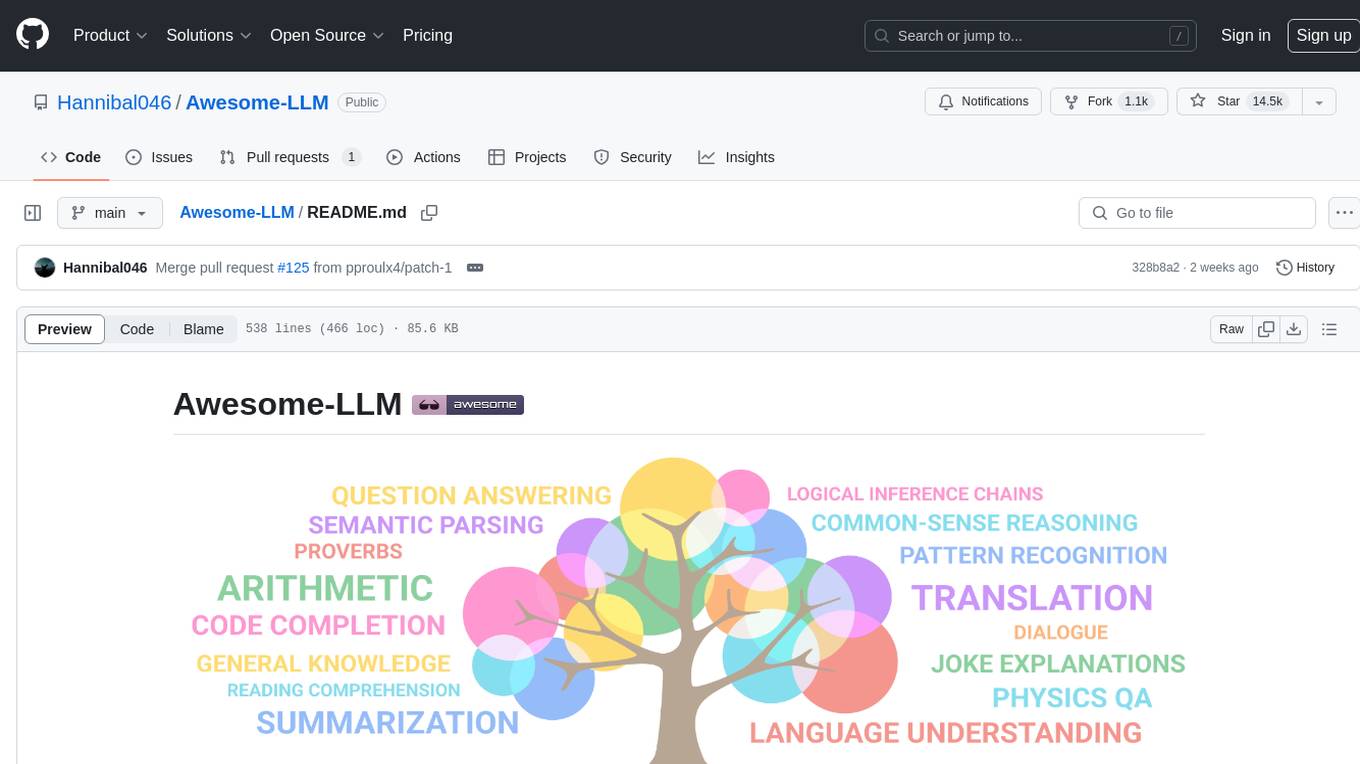
Awesome-LLM
Awesome-LLM is a curated list of resources related to large language models, focusing on papers, projects, frameworks, tools, tutorials, courses, opinions, and other useful resources in the field. It covers trending LLM projects, milestone papers, other papers, open LLM projects, LLM training frameworks, LLM evaluation frameworks, tools for deploying LLM, prompting libraries & tools, tutorials, courses, books, and opinions. The repository provides a comprehensive overview of the latest advancements and resources in the field of large language models.
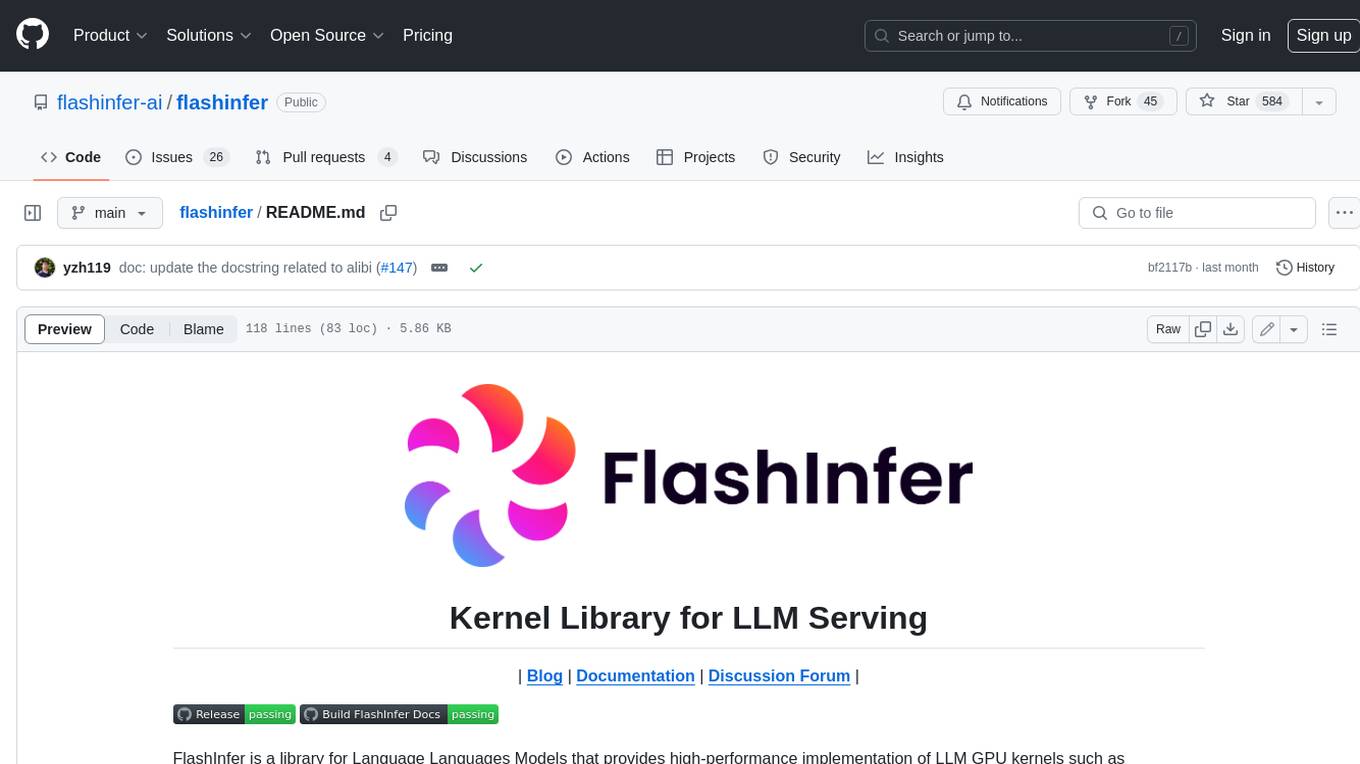
flashinfer
FlashInfer is a library for Language Languages Models that provides high-performance implementation of LLM GPU kernels such as FlashAttention, PageAttention and LoRA. FlashInfer focus on LLM serving and inference, and delivers state-the-art performance across diverse scenarios.
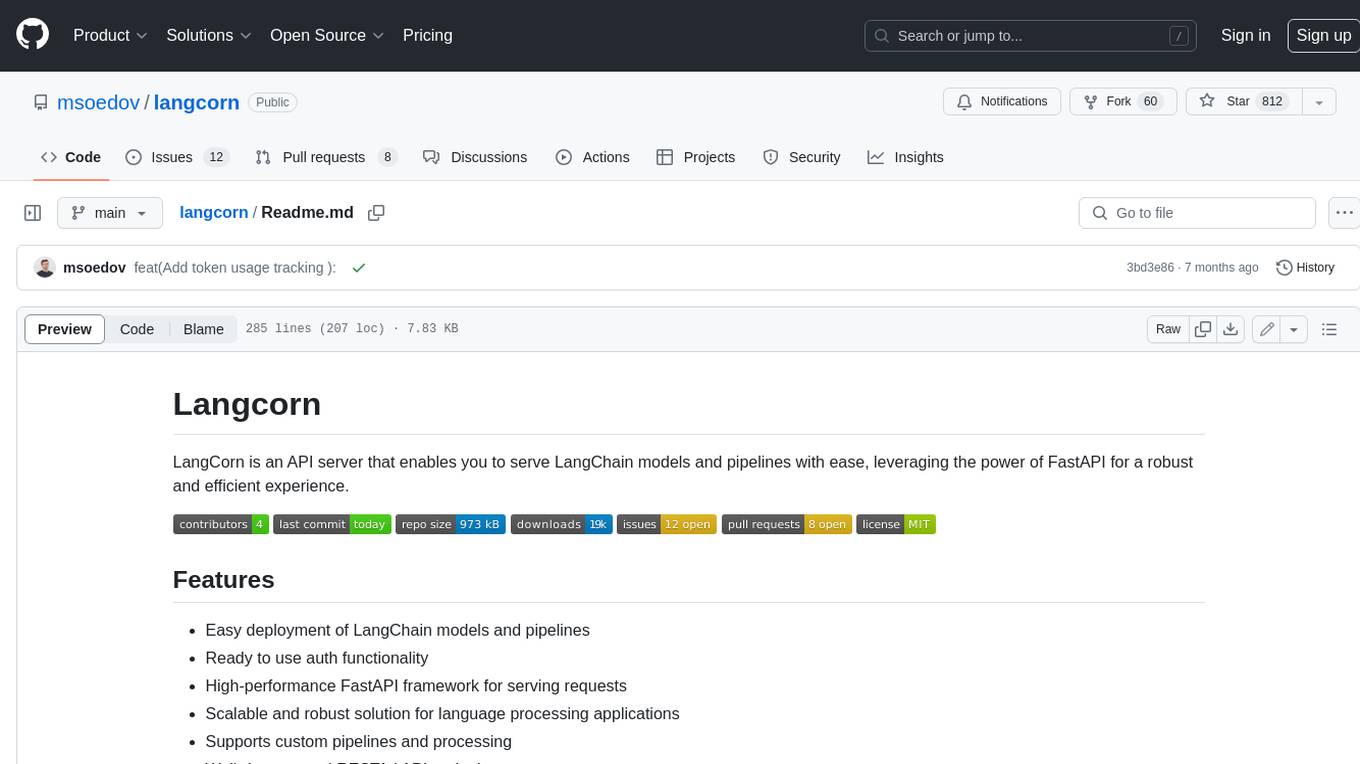
langcorn
LangCorn is an API server that enables you to serve LangChain models and pipelines with ease, leveraging the power of FastAPI for a robust and efficient experience. It offers features such as easy deployment of LangChain models and pipelines, ready-to-use authentication functionality, high-performance FastAPI framework for serving requests, scalability and robustness for language processing applications, support for custom pipelines and processing, well-documented RESTful API endpoints, and asynchronous processing for faster response times.
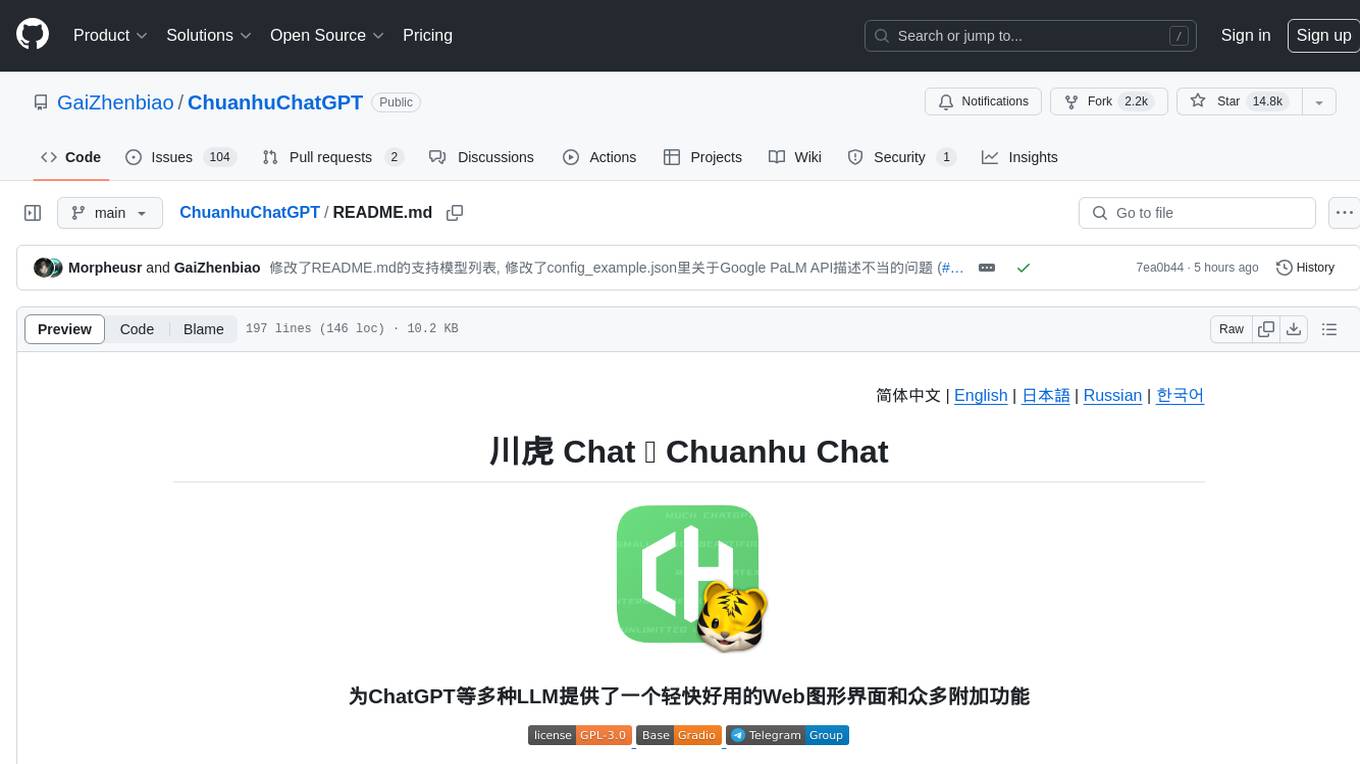
ChuanhuChatGPT
Chuanhu Chat is a user-friendly web graphical interface that provides various additional features for ChatGPT and other language models. It supports GPT-4, file-based question answering, local deployment of language models, online search, agent assistant, and fine-tuning. The tool offers a range of functionalities including auto-solving questions, online searching with network support, knowledge base for quick reading, local deployment of language models, GPT 3.5 fine-tuning, and custom model integration. It also features system prompts for effective role-playing, basic conversation capabilities with options to regenerate or delete dialogues, conversation history management with auto-saving and search functionalities, and a visually appealing user experience with themes, dark mode, LaTeX rendering, and PWA application support.
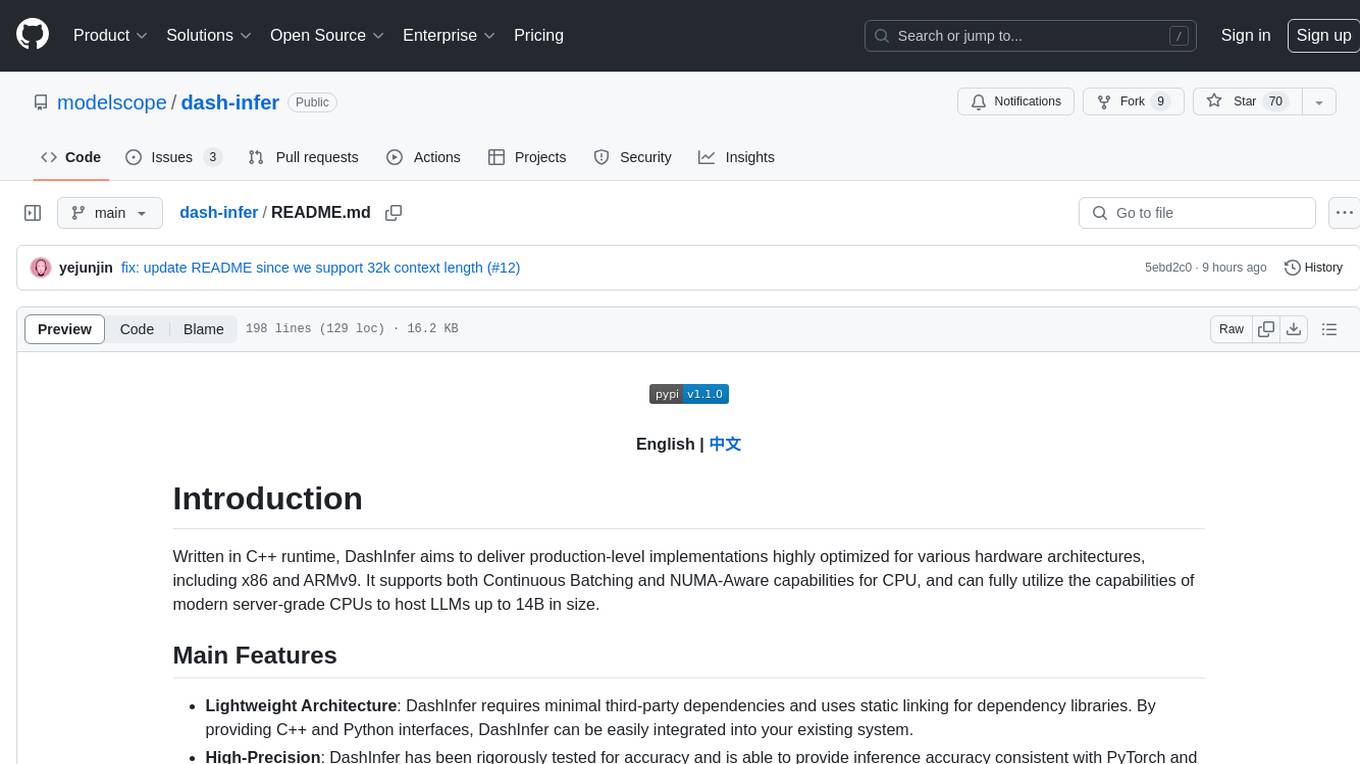
dash-infer
DashInfer is a C++ runtime tool designed to deliver production-level implementations highly optimized for various hardware architectures, including x86 and ARMv9. It supports Continuous Batching and NUMA-Aware capabilities for CPU, and can fully utilize modern server-grade CPUs to host large language models (LLMs) up to 14B in size. With lightweight architecture, high precision, support for mainstream open-source LLMs, post-training quantization, optimized computation kernels, NUMA-aware design, and multi-language API interfaces, DashInfer provides a versatile solution for efficient inference tasks. It supports x86 CPUs with AVX2 instruction set and ARMv9 CPUs with SVE instruction set, along with various data types like FP32, BF16, and InstantQuant. DashInfer also offers single-NUMA and multi-NUMA architectures for model inference, with detailed performance tests and inference accuracy evaluations available. The tool is supported on mainstream Linux server operating systems and provides documentation and examples for easy integration and usage.
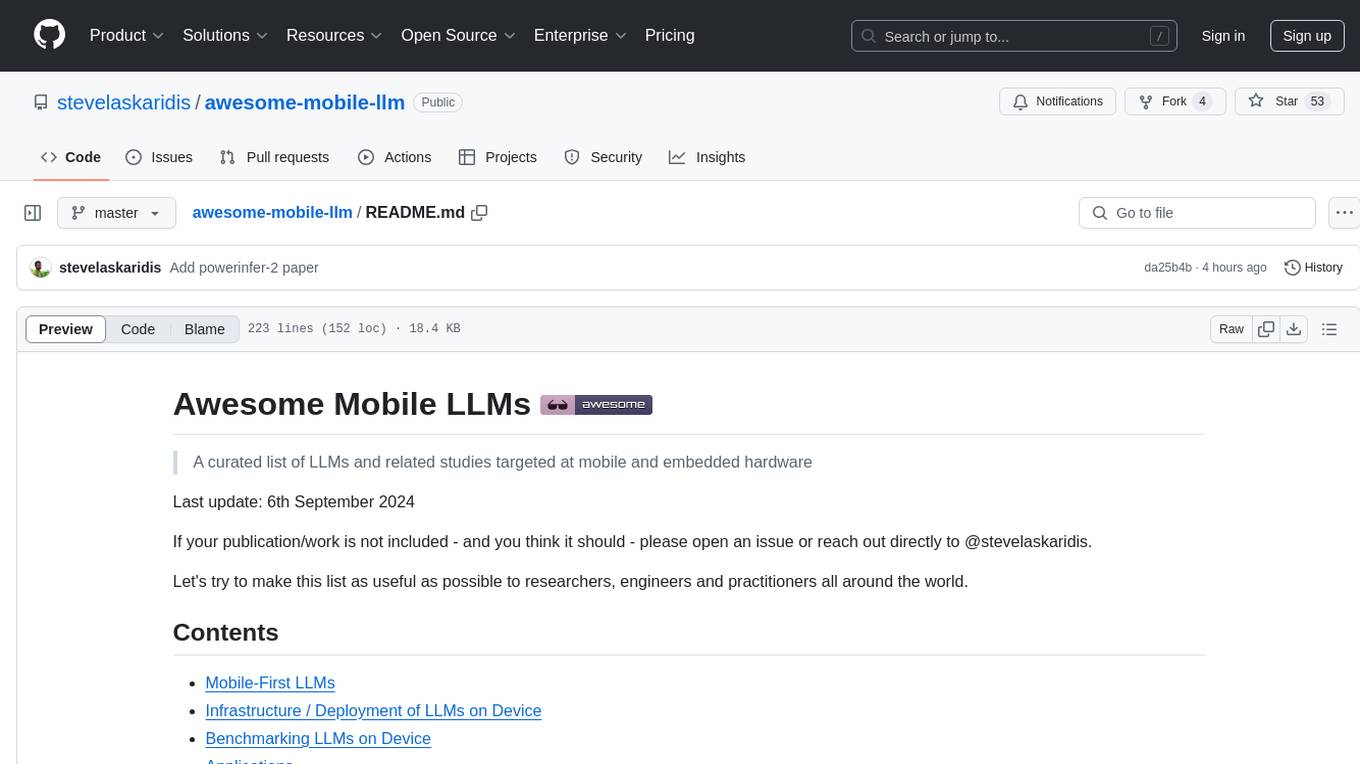
awesome-mobile-llm
Awesome Mobile LLMs is a curated list of Large Language Models (LLMs) and related studies focused on mobile and embedded hardware. The repository includes information on various LLM models, deployment frameworks, benchmarking efforts, applications, multimodal LLMs, surveys on efficient LLMs, training LLMs on device, mobile-related use-cases, industry announcements, and related repositories. It aims to be a valuable resource for researchers, engineers, and practitioners interested in mobile LLMs.
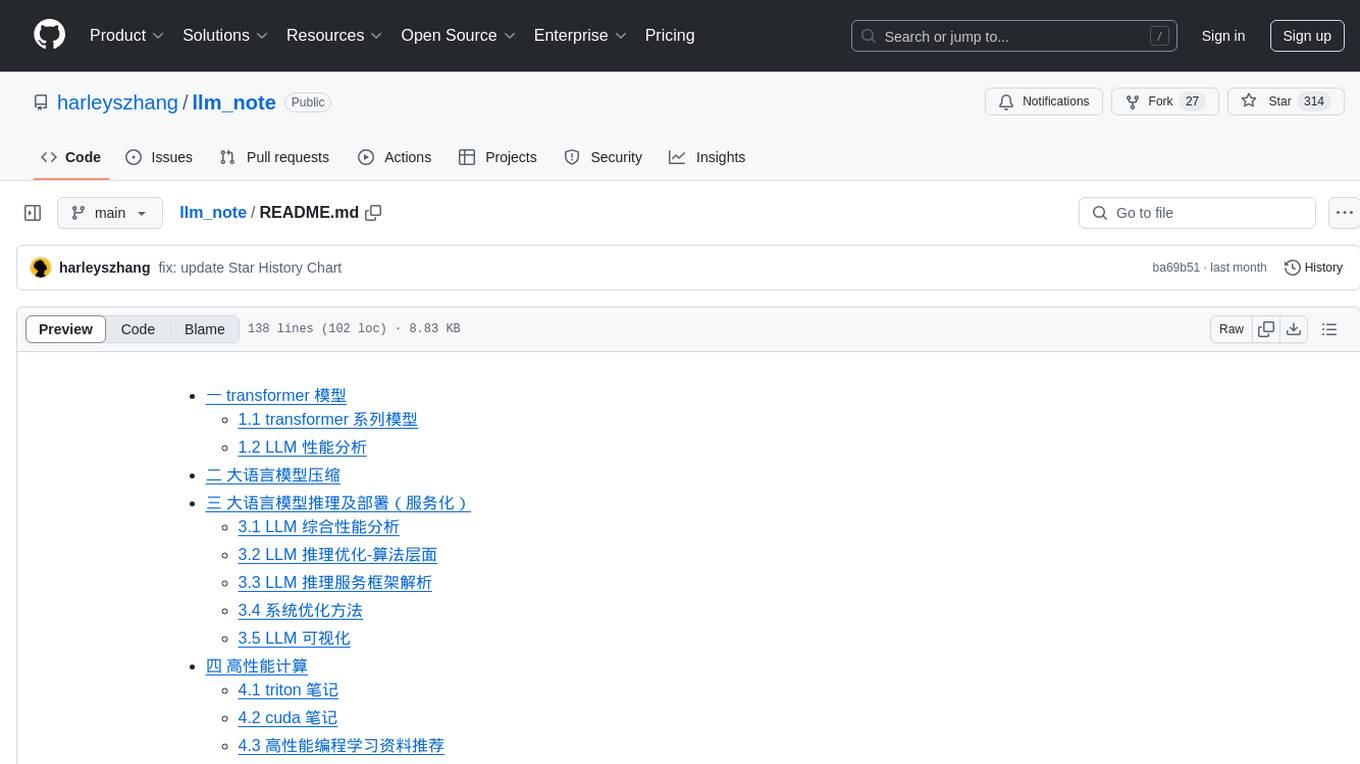
llm_note
LLM notes repository contains detailed analysis on transformer models, language model compression, inference and deployment, high-performance computing, and system optimization methods. It includes discussions on various algorithms, frameworks, and performance analysis related to large language models and high-performance computing. The repository serves as a comprehensive resource for understanding and optimizing language models and computing systems.
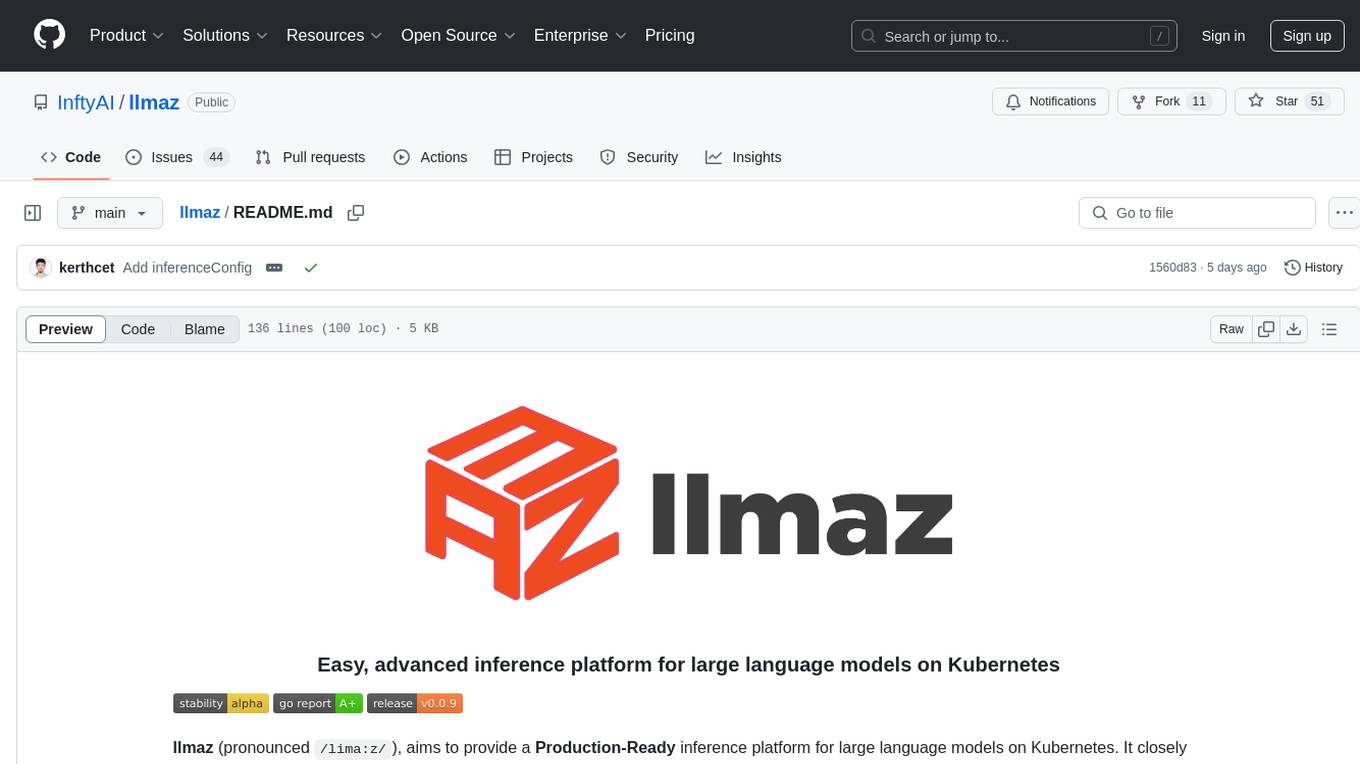
llmaz
llmaz is an easy, advanced inference platform for large language models on Kubernetes. It aims to provide a production-ready solution that integrates with state-of-the-art inference backends. The platform supports efficient model distribution, accelerator fungibility, SOTA inference, various model providers, multi-host support, and scaling efficiency. Users can quickly deploy LLM services with minimal configurations and benefit from a wide range of advanced inference backends. llmaz is designed to optimize cost and performance while supporting cutting-edge researches like Speculative Decoding or Splitwise on Kubernetes.
For similar jobs
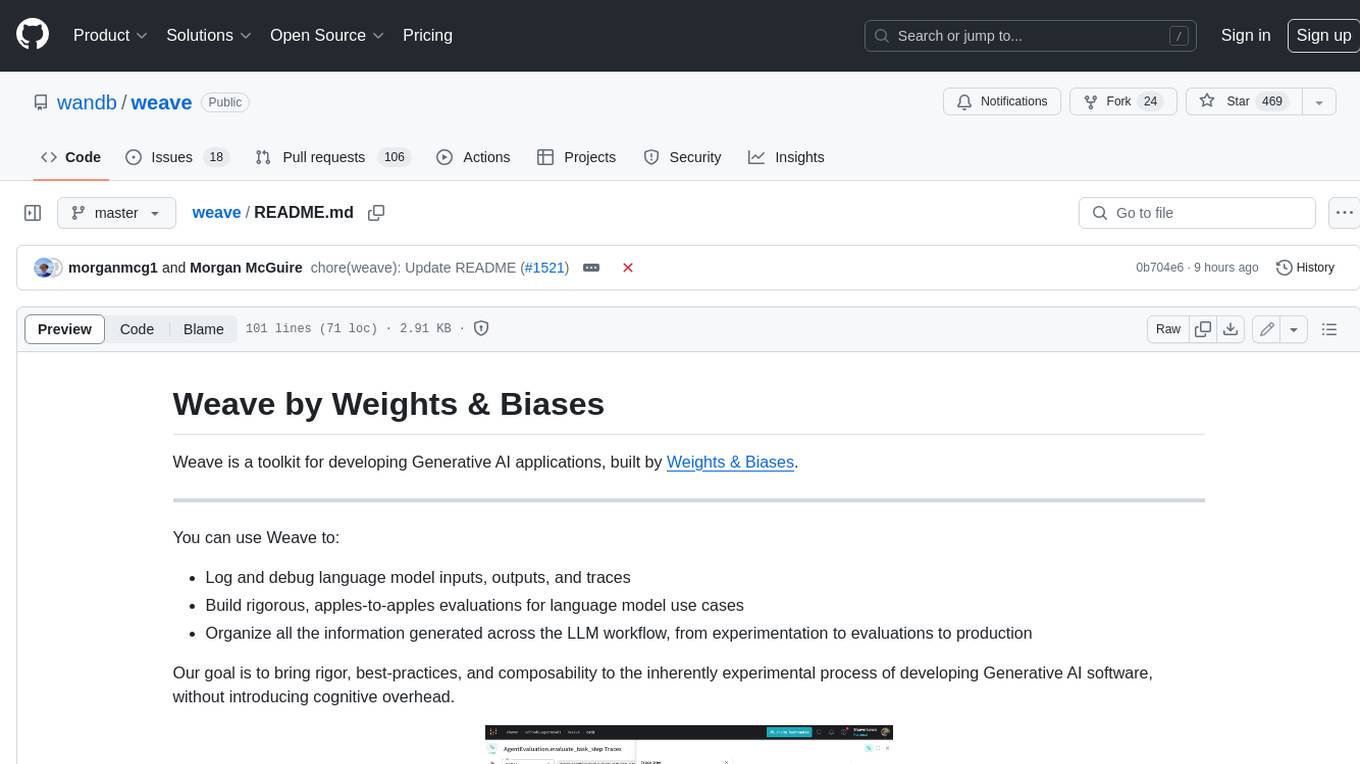
weave
Weave is a toolkit for developing Generative AI applications, built by Weights & Biases. With Weave, you can log and debug language model inputs, outputs, and traces; build rigorous, apples-to-apples evaluations for language model use cases; and organize all the information generated across the LLM workflow, from experimentation to evaluations to production. Weave aims to bring rigor, best-practices, and composability to the inherently experimental process of developing Generative AI software, without introducing cognitive overhead.
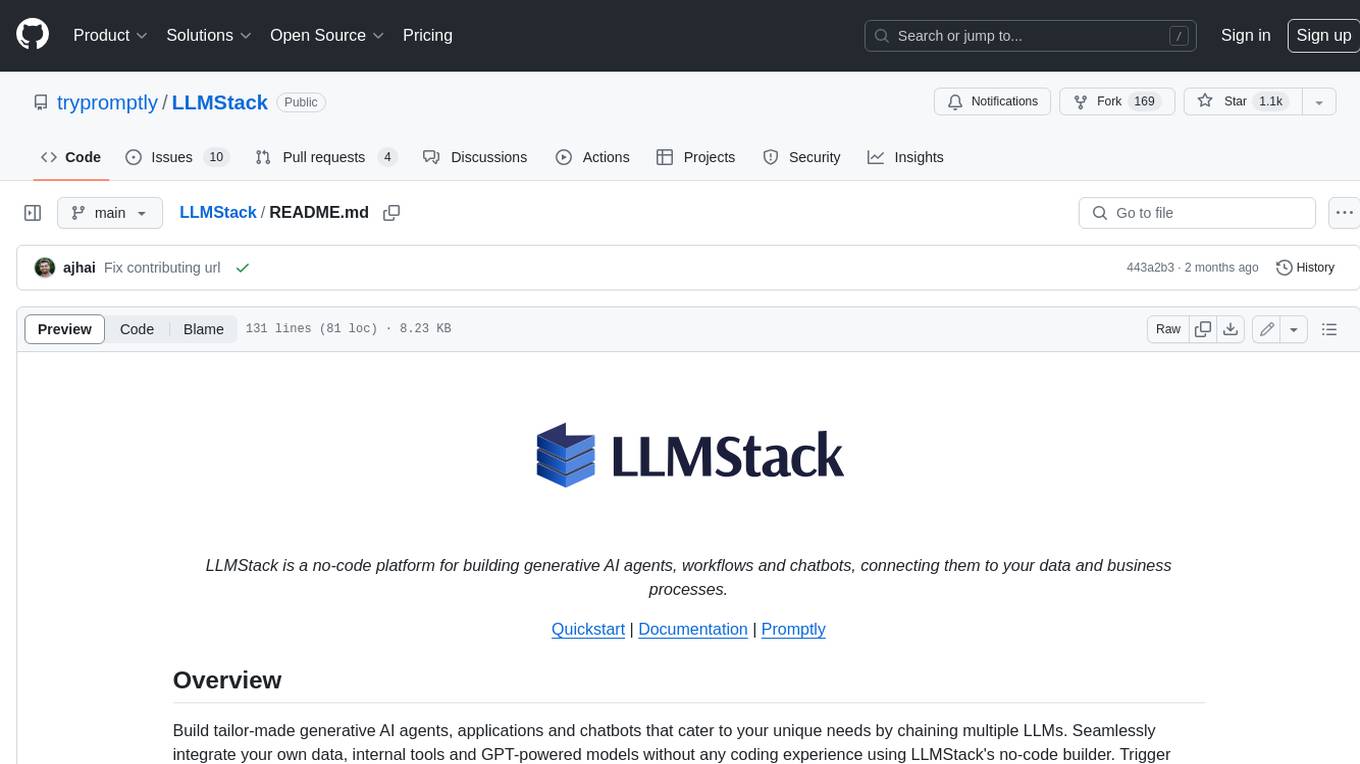
LLMStack
LLMStack is a no-code platform for building generative AI agents, workflows, and chatbots. It allows users to connect their own data, internal tools, and GPT-powered models without any coding experience. LLMStack can be deployed to the cloud or on-premise and can be accessed via HTTP API or triggered from Slack or Discord.
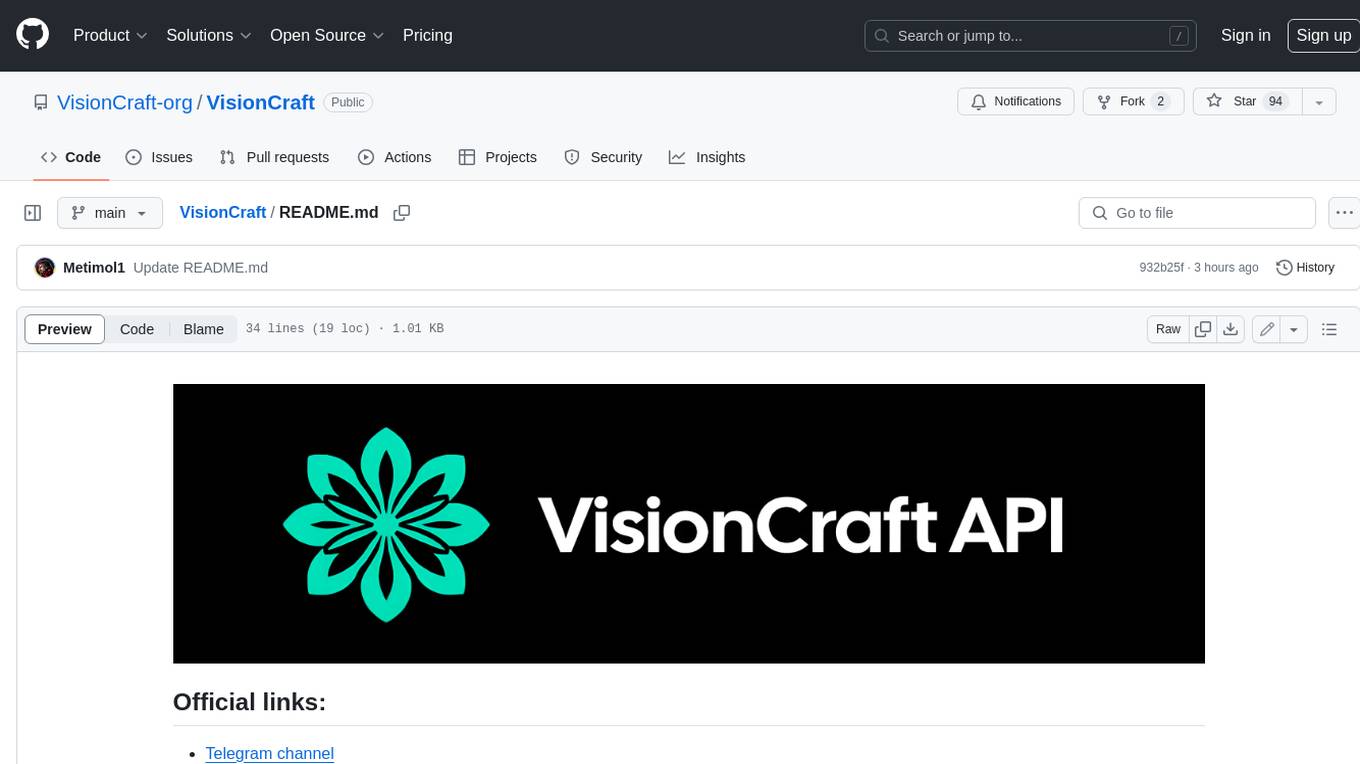
VisionCraft
The VisionCraft API is a free API for using over 100 different AI models. From images to sound.
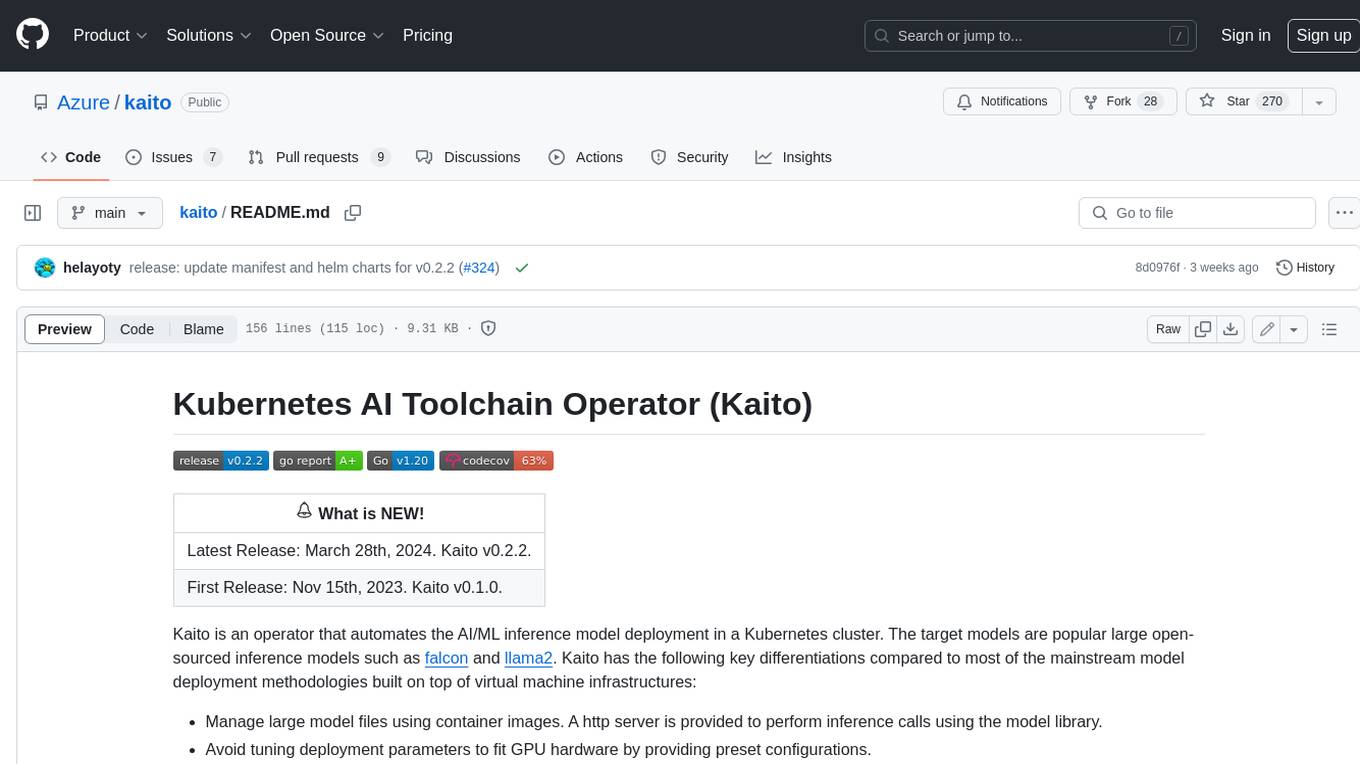
kaito
Kaito is an operator that automates the AI/ML inference model deployment in a Kubernetes cluster. It manages large model files using container images, avoids tuning deployment parameters to fit GPU hardware by providing preset configurations, auto-provisions GPU nodes based on model requirements, and hosts large model images in the public Microsoft Container Registry (MCR) if the license allows. Using Kaito, the workflow of onboarding large AI inference models in Kubernetes is largely simplified.
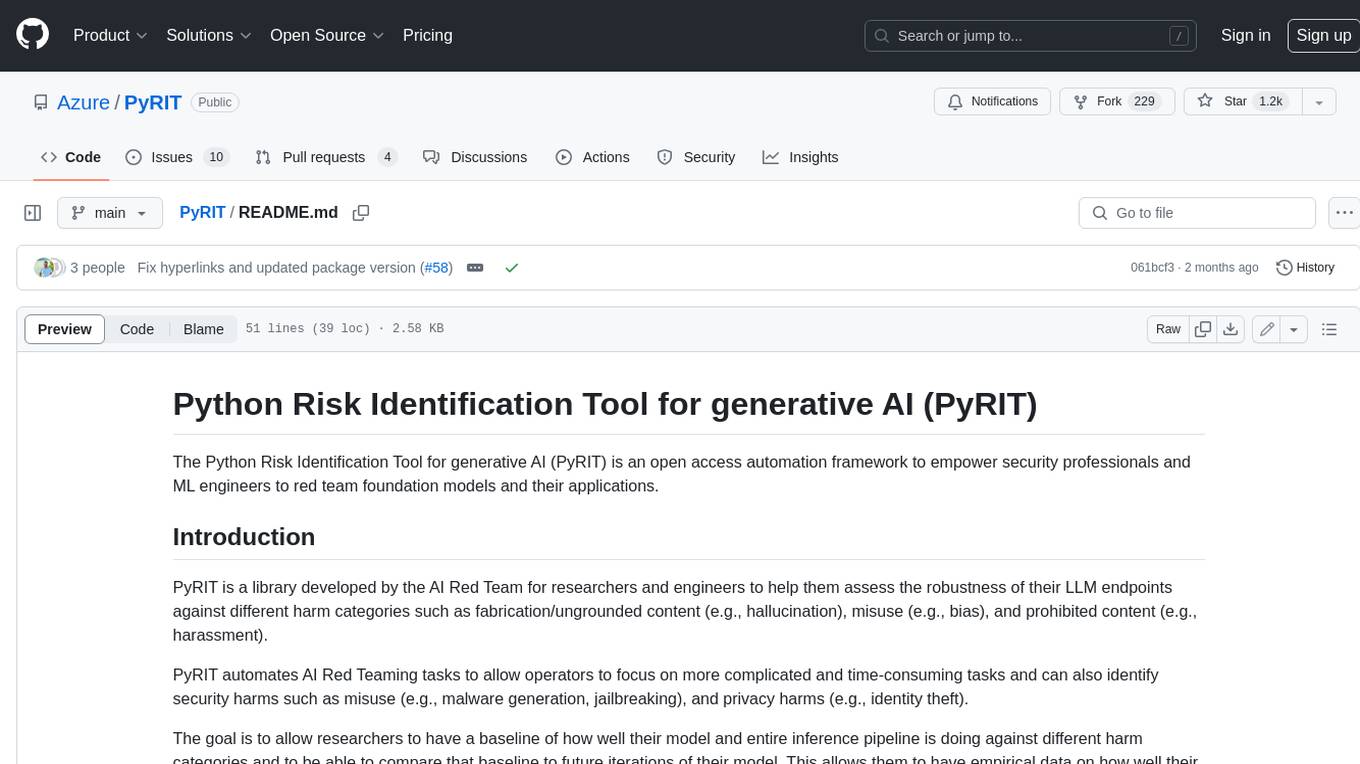
PyRIT
PyRIT is an open access automation framework designed to empower security professionals and ML engineers to red team foundation models and their applications. It automates AI Red Teaming tasks to allow operators to focus on more complicated and time-consuming tasks and can also identify security harms such as misuse (e.g., malware generation, jailbreaking), and privacy harms (e.g., identity theft). The goal is to allow researchers to have a baseline of how well their model and entire inference pipeline is doing against different harm categories and to be able to compare that baseline to future iterations of their model. This allows them to have empirical data on how well their model is doing today, and detect any degradation of performance based on future improvements.
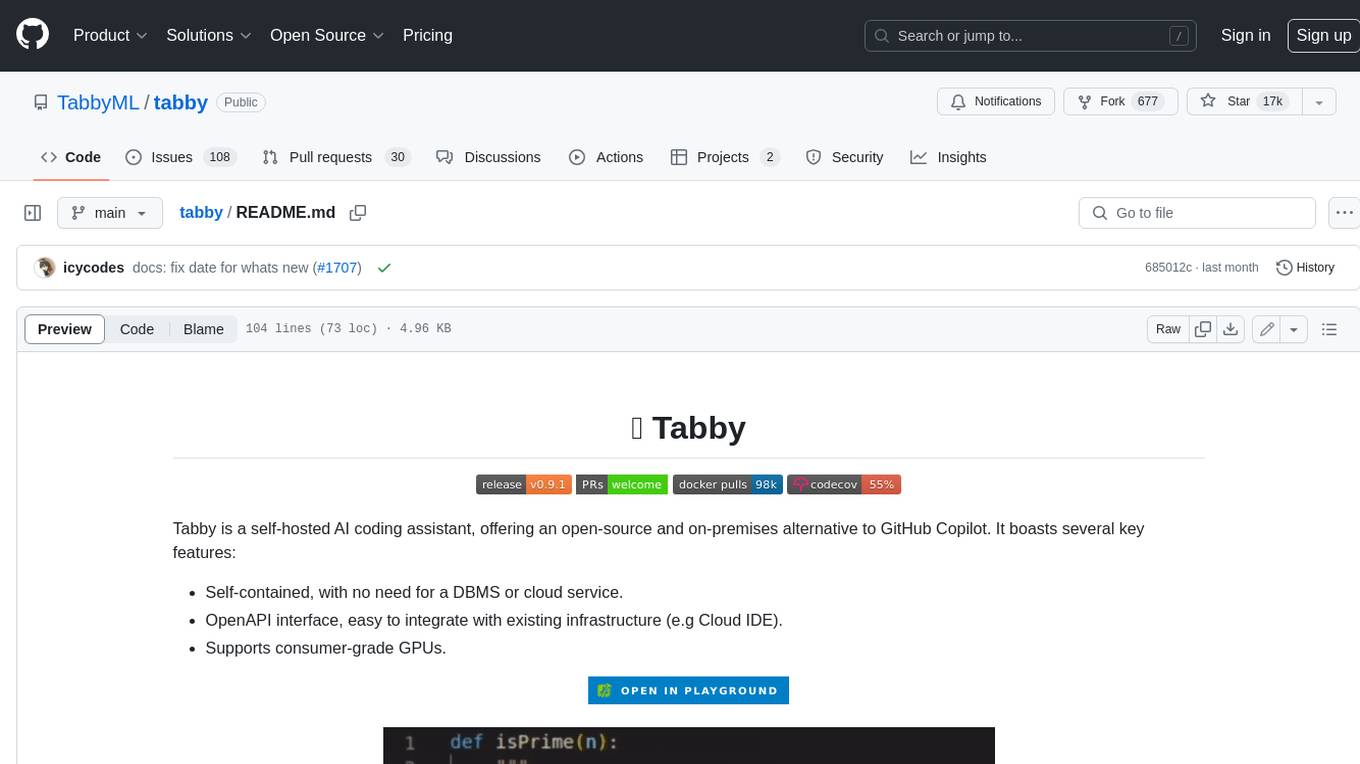
tabby
Tabby is a self-hosted AI coding assistant, offering an open-source and on-premises alternative to GitHub Copilot. It boasts several key features: * Self-contained, with no need for a DBMS or cloud service. * OpenAPI interface, easy to integrate with existing infrastructure (e.g Cloud IDE). * Supports consumer-grade GPUs.
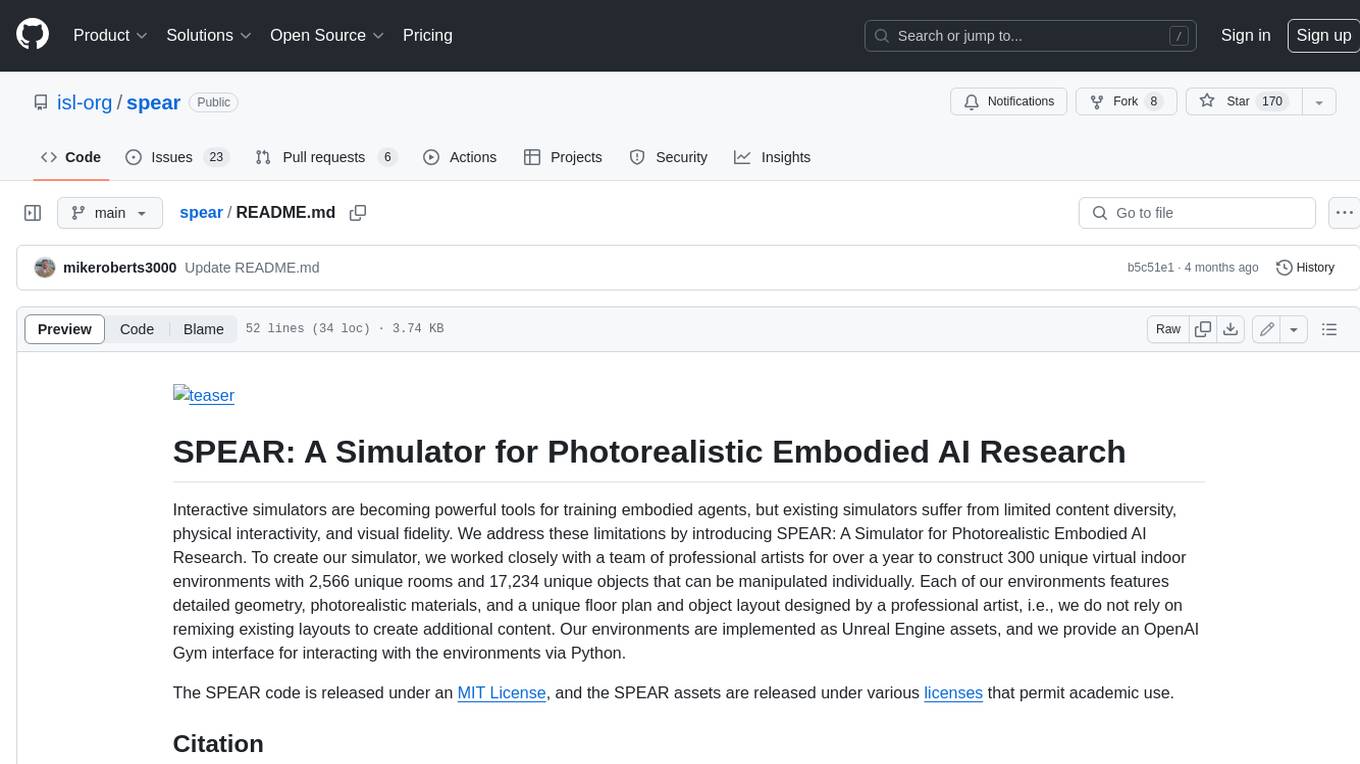
spear
SPEAR (Simulator for Photorealistic Embodied AI Research) is a powerful tool for training embodied agents. It features 300 unique virtual indoor environments with 2,566 unique rooms and 17,234 unique objects that can be manipulated individually. Each environment is designed by a professional artist and features detailed geometry, photorealistic materials, and a unique floor plan and object layout. SPEAR is implemented as Unreal Engine assets and provides an OpenAI Gym interface for interacting with the environments via Python.
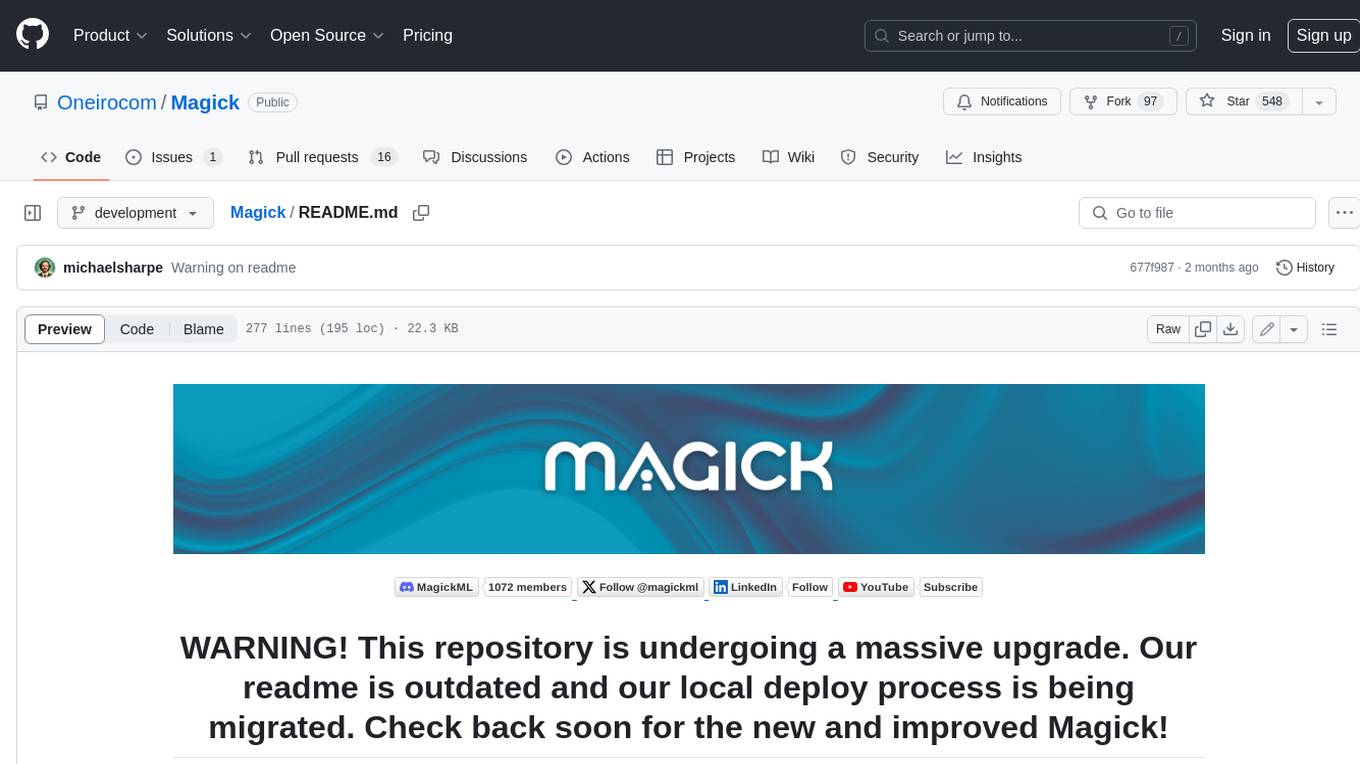
Magick
Magick is a groundbreaking visual AIDE (Artificial Intelligence Development Environment) for no-code data pipelines and multimodal agents. Magick can connect to other services and comes with nodes and templates well-suited for intelligent agents, chatbots, complex reasoning systems and realistic characters.