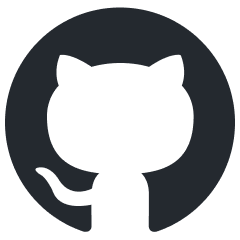
llm_note
LLM notes, including model inference, transformer model structure, and llm framework code analysis notes.
Stars: 686
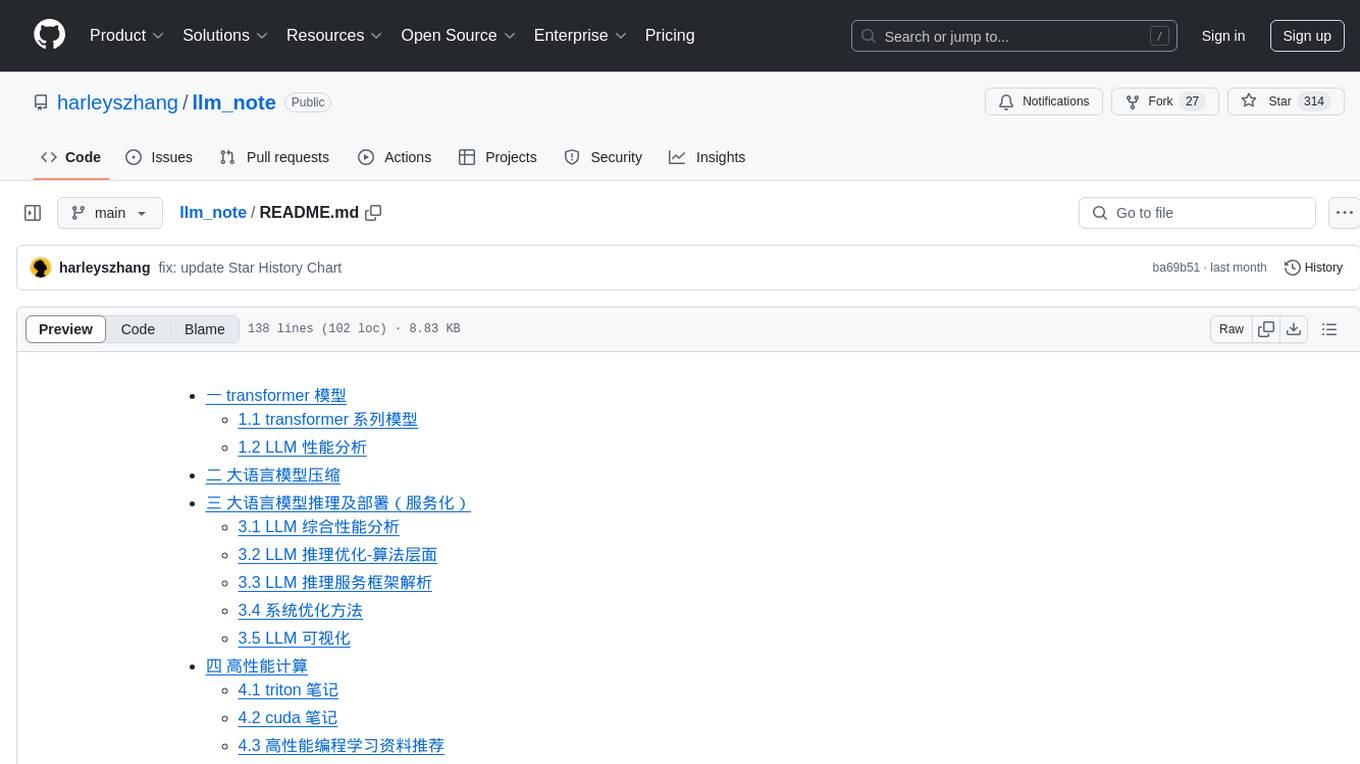
LLM notes repository contains detailed analysis on transformer models, language model compression, inference and deployment, high-performance computing, and system optimization methods. It includes discussions on various algorithms, frameworks, and performance analysis related to large language models and high-performance computing. The repository serves as a comprehensive resource for understanding and optimizing language models and computing systems.
README:
LLM notes, including model inference, transformer model structure, and lightllm framework code analysis notes.
-
框架亮点:基于
Triton + PyTorch
开发的轻量级、且简单易用的大模型推理框架,采用类Pytorch
语法的Triton
编写算子,绕开 Cuda 复杂语法实现 GPU 内核开发。 - 价格:499。非常实惠和便宜,课程、项目、面经、答疑质量绝对对得起这个价格。
-
课程优势:
- 手把手教你从 0 到 1 实现大模型推理框架。
- 项目导向 + 面试导向 + 分类总结的面试题。
- 2025 最新的高性能计算/推理框架岗位的大厂面试题汇总
-
项目优势:
- 架构清晰,代码简洁且注释详尽,覆盖大模型离线推理全流程。
- 运用 OpenAI
Triton
编写高性能计算Kernel
,其中矩阵乘法内核效率堪比cuBLAS
。 - 基于
PyTorch
实现高效显存管理。 - 课程项目完美支持
FlashAttentionV1、V2、V3
与GQA
,以及PageAttention
的具体实现。 - 使用
Triton
编写融合算子,如 KV 线性层融合等。 - 适配最新的
llama/qwen2.5/llava1.5
模型,相较 transformers 库,在 llama3 1B 和 3B 模型上,加速比最高可达4
倍。
- 分类总结部分面试题:
![]() |
![]() |
- 项目运行效果:
llama3.2-1.5B-Instruct
模型流式输出结果测试:
Qwen2.5-3B
模型(社区版本)流式输出结果测试:
Llava1.5-7b-hf
模型流式输出结果测试:
![]() |
![]() |
感兴趣的同学可以扫码联系课程购买,这个课程是我和《自制深度学习推理框架》作者一起合力打造的,内容也会持续更新优化。
- transformer 论文解读
- transformer 模型代码实现
- llama1-3 模型结构详解
- vit 论文速读
- gpt1-3 论文解读
- RoPE 位置编码算法详解
- Sinusoida 位置编码算法详解
- online-softmax 论文解读
- flashattention-1 论文解读
- flashattention-2 论文解读
- flashattention-3 论文解读
- flashattention1-2-3 系列总结
LLM
推理服务框架技术总结和源码解析:
DeepSpeed 框架学习笔记:
图优化、算子融合、深度学习推理框架系统层面的优化。
- 英伟达 GPU 架构总结
- 英伟达 GPU 通信理解
- 英伟达 GPU 性能分析指导
- 理解 Roofline 性能分析模型
- CUDA 背景知识
- CUDA 编程模型概述
- CUDA 编程模型进阶
- CUDA 内存组织
- CUDA 执行模型
- CUDA 内核执行配置及线程索引计算
- CUDA 内核优化策略
- CUDA 流介绍
1, 英伟达 gpu cuda 编程语法和特性学习资料推荐:
- GPU Architecture and Programming: 了解 GPU 架构和 cuda 编程的入门文档资料,学完可以理解 gpu 架构的基本原理和理解 cuda 编程模型(cuda 并行计算的基本流程)。建议当作学习 cuda 高性能计算编程的第一篇文档(文章)。
- CUDA Tutorial: CUDA 教程,分成四部分:CUDA 基础、GPU 硬件细节、最近的特性和趋势和基于任务的编程实例,提供了完整清晰的 PDF 文档和 cuda 代码实例。建议当作系统性学习 cuda 编程的教程。
- learn-cuda: 完整的 cuda 学习教程,包含高级异步方法内容,特点是有性能实验的代码实例。建议当作学习 cuda 高级特性的教程。
- CUDA C++ Programming Guide:内容很全,直接上手学习比较难,建议当作查缺补漏和验证细节的 cuda 百科全书,目前版本是 12.6。
- 《CUDA C 编程权威指南》:翻译的国外资料,说实话很多内容翻译的非常不行,我最开始跟着这个学习的,学了一周,只是了解了线程、内存概念和编程模型的概述,但是细节和系统性思维没学到,而且翻译的不行,内容也比较过时,完全不推荐,我已经替大家踩过坑了。
- 《CUDA 编程:基础与实践_樊哲勇》:国内自己写的教材,我查资料时候挑着看了一点,基本逻辑是通的,虽然很多原理、概念都讲的特别啰嗦,但实践需要的关键知识点都有讲到,想学中文教程的,可以当作当作了解一个方向的快速阅读资料。
- CUDA-Kernels-Learn-Notes: CUDA 内核编程笔记及实战代码,有很强的实践性,后期可以重点学习,我也准备认真看下代码和文档。
2, cuda/triton
编写 kernel
笔记资料:
- 最基本的通用矩阵乘法(gemm):https://zhuanlan.zhihu.com/p/657632577
- kernl: 提供了一些 llm 的 triton 版 kernels
-
unsloth:专注于大型语言模型推理加速的微调训练方向。提供了开源版本,可以显著提高训练效率,减少内存使用,并且支持 NVIDIA、Intel 和 AMD 的 GPU。Unsloth 的主要特点包括使用 OpenAI 的 Triton 语言重写所有内核。Gemma LLMs 速度提高 2-5 倍,内存减少
80%
内核基于 triton 实现。 - Liger-Kernel: 用于训练的高效 triton 内核实现。
- Efficient-LLM-Inferencing-on-GPUs: README 图片不错,改天看看。
3, Star History Chart:
For Tasks:
Click tags to check more tools for each tasksFor Jobs:
Alternative AI tools for llm_note
Similar Open Source Tools
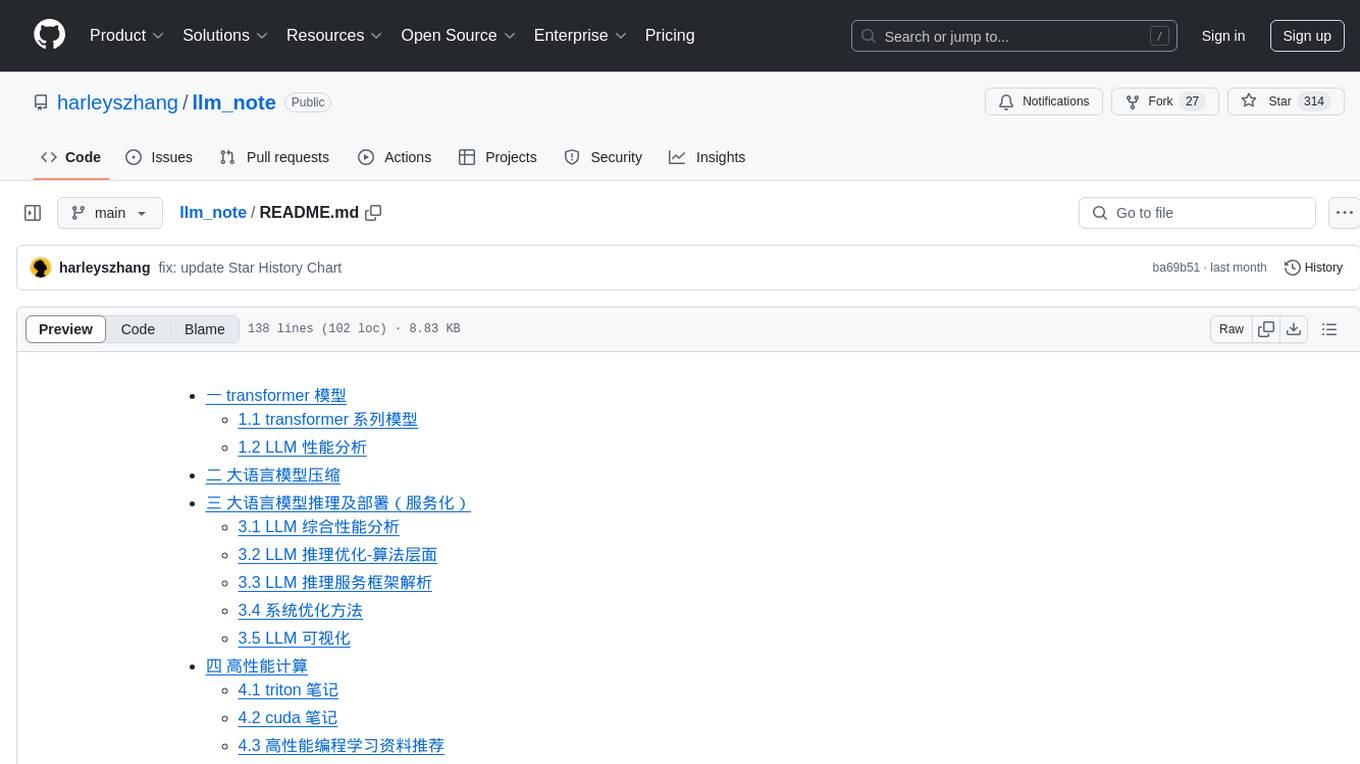
llm_note
LLM notes repository contains detailed analysis on transformer models, language model compression, inference and deployment, high-performance computing, and system optimization methods. It includes discussions on various algorithms, frameworks, and performance analysis related to large language models and high-performance computing. The repository serves as a comprehensive resource for understanding and optimizing language models and computing systems.
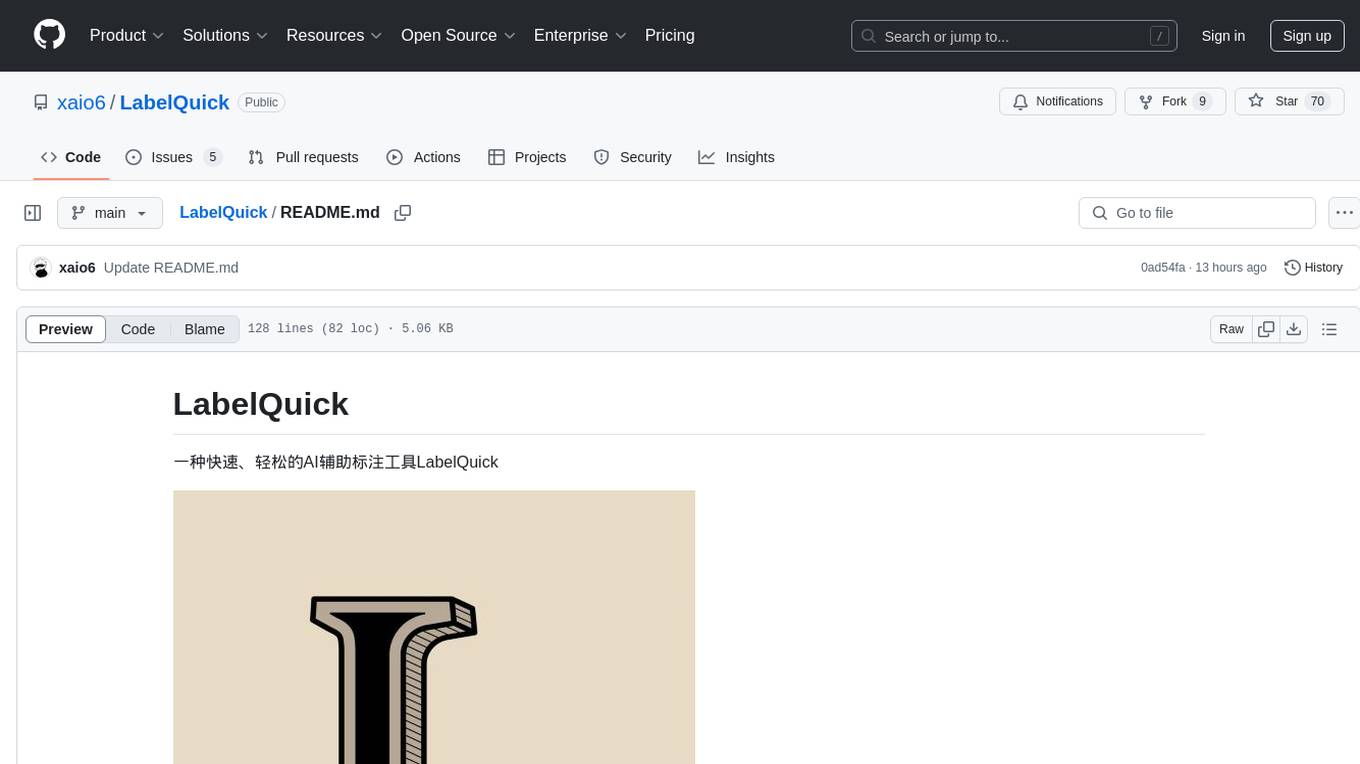
LabelQuick
LabelQuick_V2.0 is a fast image annotation tool designed and developed by the AI Horizon team. This version has been optimized and improved based on the previous version. It provides an intuitive interface and powerful annotation and segmentation functions to efficiently complete dataset annotation work. The tool supports video object tracking annotation, quick annotation by clicking, and various video operations. It introduces the SAM2 model for accurate and efficient object detection in video frames, reducing manual intervention and improving annotation quality. The tool is designed for Windows systems and requires a minimum of 6GB of memory.
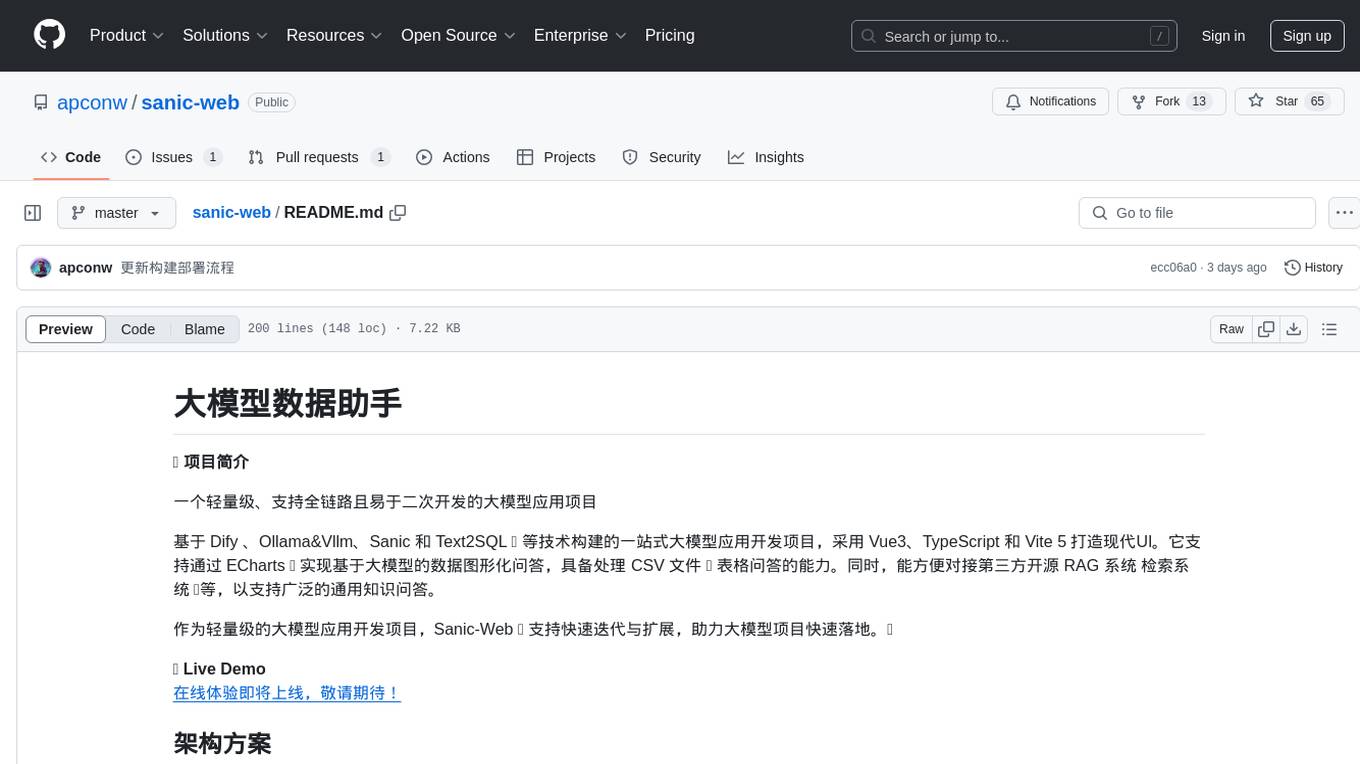
sanic-web
Sanic-Web is a lightweight, end-to-end, and easily customizable large model application project built on technologies such as Dify, Ollama & Vllm, Sanic, and Text2SQL. It provides a one-stop solution for developing large model applications, supporting graphical data-driven Q&A using ECharts, handling table-based Q&A with CSV files, and integrating with third-party RAG systems for general knowledge Q&A. As a lightweight framework, Sanic-Web enables rapid iteration and extension to facilitate the quick implementation of large model projects.
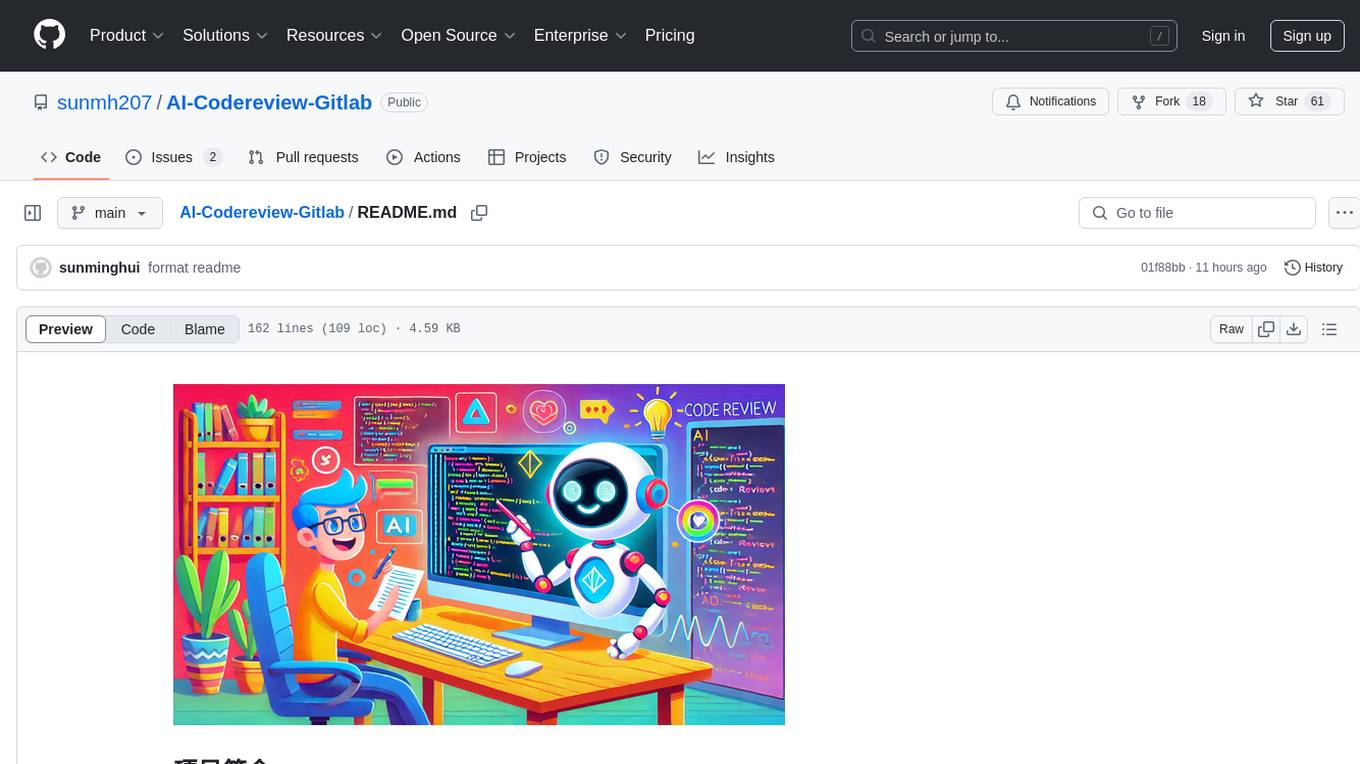
AI-Codereview-Gitlab
AI-Codereview-Gitlab is an automated code review tool based on large models, designed to help development teams conduct intelligent code reviews quickly during code merging or submission. It supports multiple large models including DeepSeek, ZhipuAI, OpenAI, and Ollama. The tool can automatically push review results to DingTalk, WeChat Work, and Feishu, generate daily reports based on GitLab commit records, and provide a visual dashboard to display code review records. The tool works by triggering webhook events on GitLab when users submit code, calling third-party large models to review the code, and recording the review results in corresponding Merge Requests or Commit Notes.
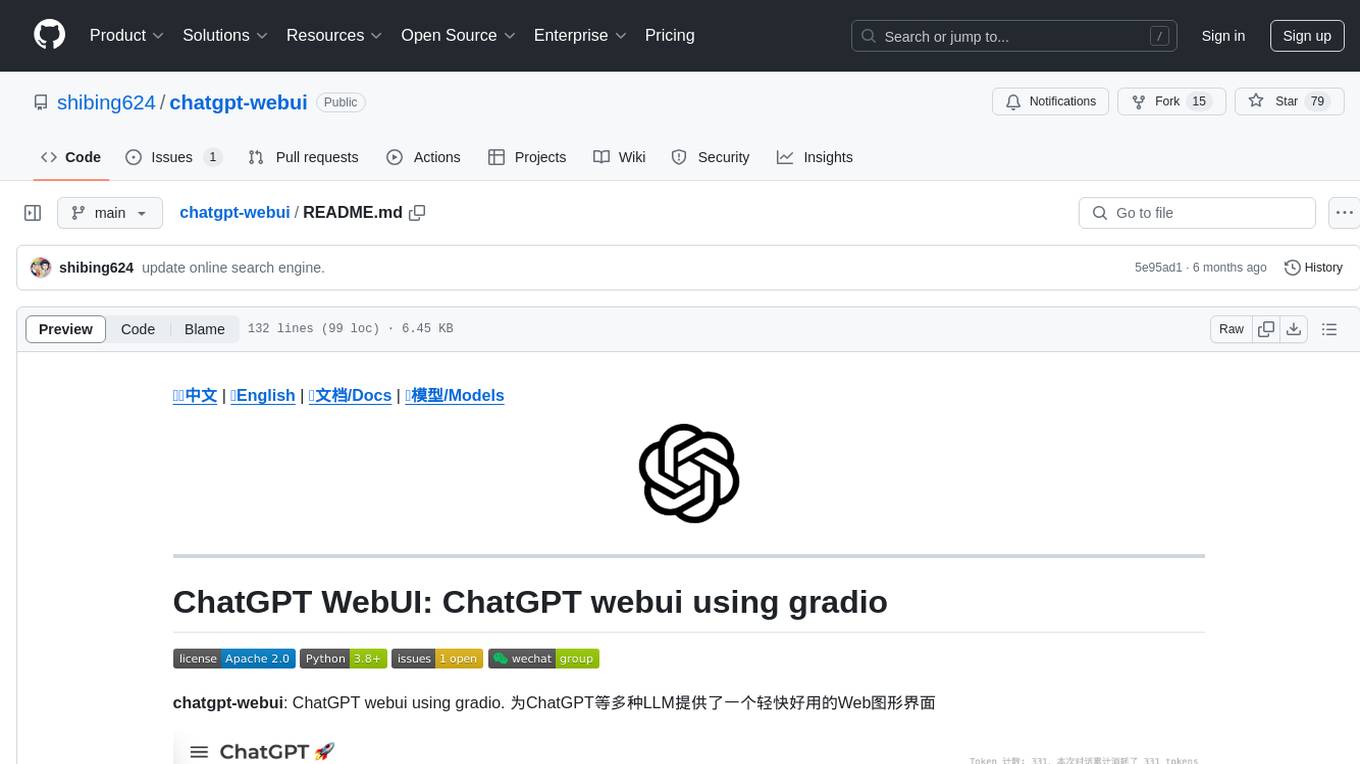
chatgpt-webui
ChatGPT WebUI is a user-friendly web graphical interface for various LLMs like ChatGPT, providing simplified features such as core ChatGPT conversation and document retrieval dialogues. It has been optimized for better RAG retrieval accuracy and supports various search engines. Users can deploy local language models easily and interact with different LLMs like GPT-4, Azure OpenAI, and more. The tool offers powerful functionalities like GPT4 API configuration, system prompt setup for role-playing, and basic conversation features. It also provides a history of conversations, customization options, and a seamless user experience with themes, dark mode, and PWA installation support.
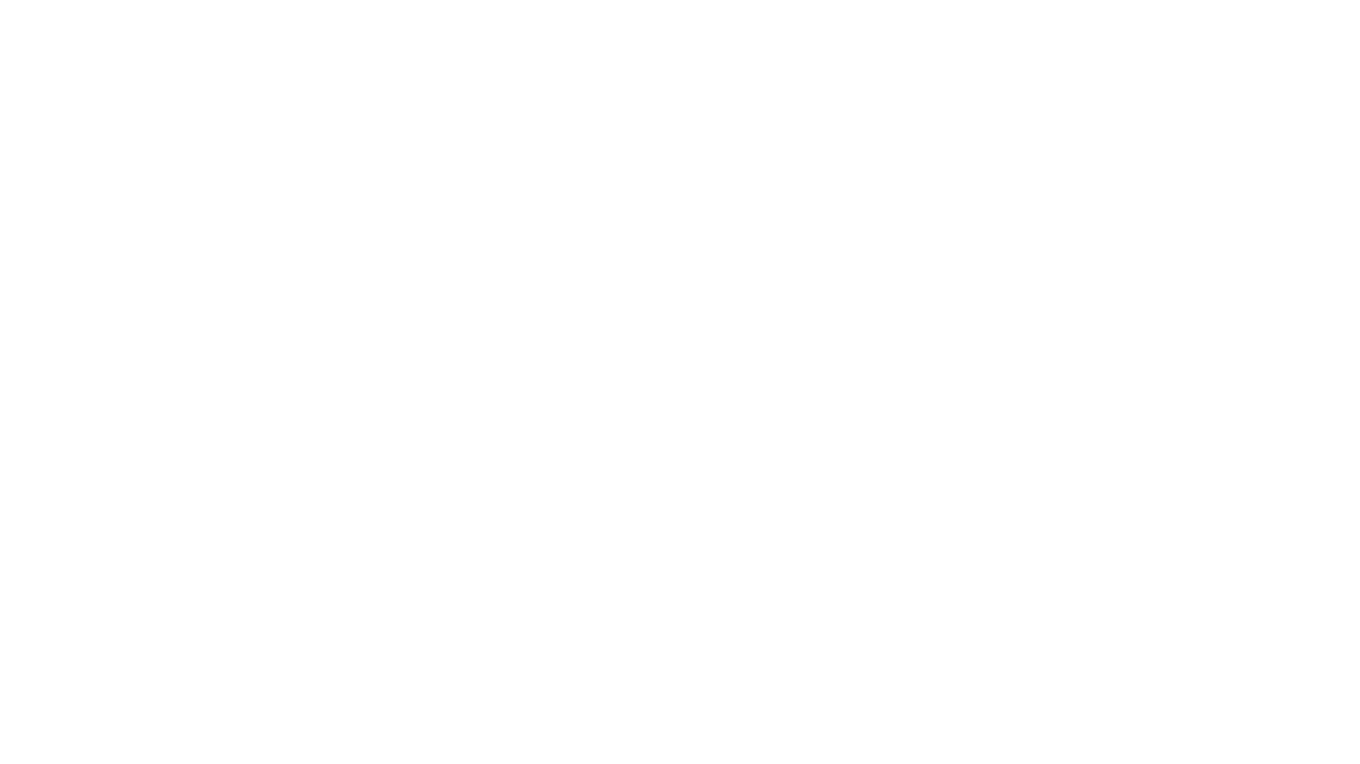
AI-automatically-generates-novels
AI Novel Writing Assistant is an intelligent productivity tool for novel creation based on AI + prompt words. It has been used by hundreds of studios and individual authors to quickly and batch generate novels. With AI technology to enhance writing efficiency and a comprehensive prompt word management feature, it achieves 20 times efficiency improvement in intelligent book disassembly, intelligent book title and synopsis generation, text polishing, and shift+L quick term insertion, making writing easier and more professional. It has been upgraded to v5.2. The tool supports mind map construction of outlines and chapters, AI self-optimization of novels, writing knowledge base management, shift+L quick term insertion in the text input field, support for any mainstream large models integration, custom skin color, prompt word import and export, support for large text memory, right-click polishing, expansion, and de-AI flavoring of outlines, chapters, and text, multiple sets of novel prompt word library management, and book disassembly function.
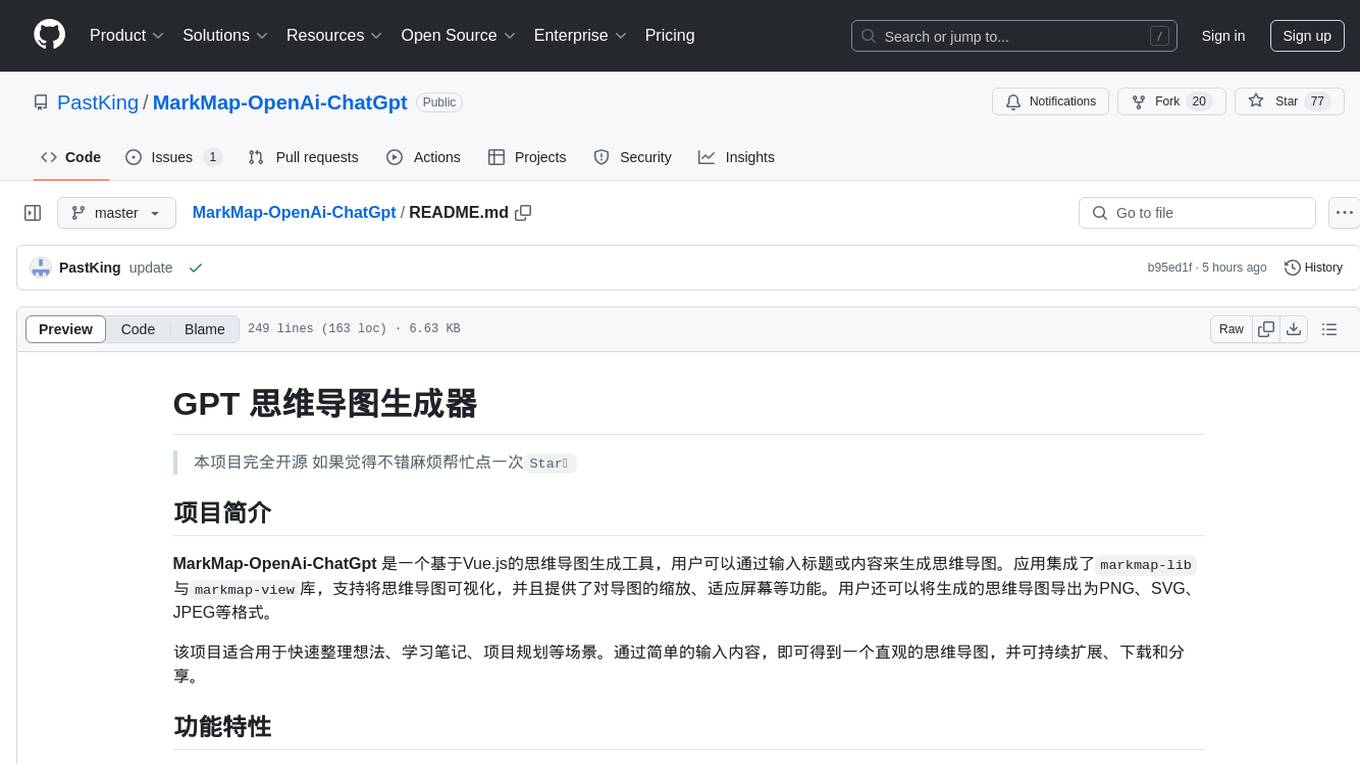
MarkMap-OpenAi-ChatGpt
MarkMap-OpenAi-ChatGpt is a Vue.js-based mind map generation tool that allows users to generate mind maps by entering titles or content. The application integrates the markmap-lib and markmap-view libraries, supports visualizing mind maps, and provides functions for zooming and adapting the map to the screen. Users can also export the generated mind map in PNG, SVG, JPEG, and other formats. This project is suitable for quickly organizing ideas, study notes, project planning, etc. By simply entering content, users can get an intuitive mind map that can be continuously expanded, downloaded, and shared.
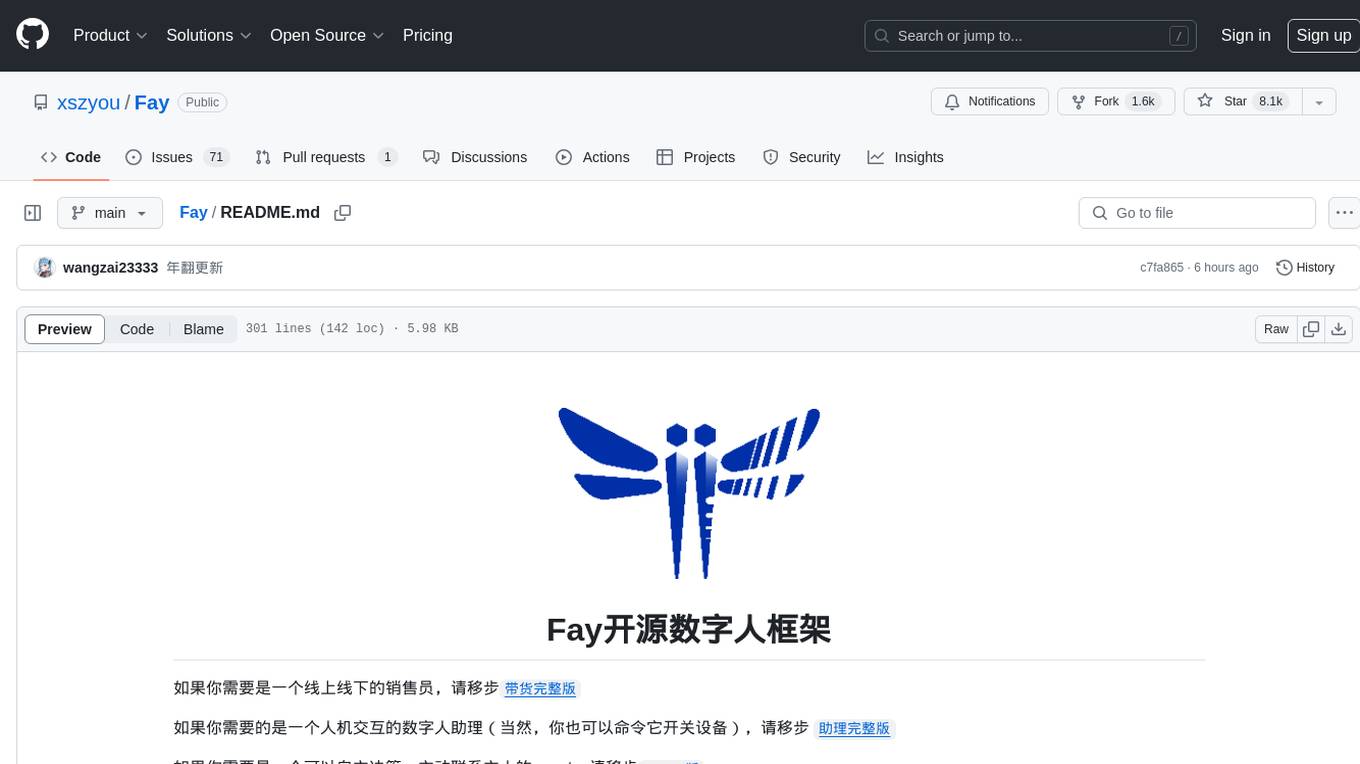
Fay
Fay is an open-source digital human framework that offers different versions for various purposes. The '带货完整版' is suitable for online and offline salespersons. The '助理完整版' serves as a human-machine interactive digital assistant that can also control devices upon command. The 'agent版' is designed to be an autonomous agent capable of making decisions and contacting its owner. The framework provides updates and improvements across its different versions, including features like emotion analysis integration, model optimizations, and compatibility enhancements. Users can access detailed documentation for each version through the provided links.
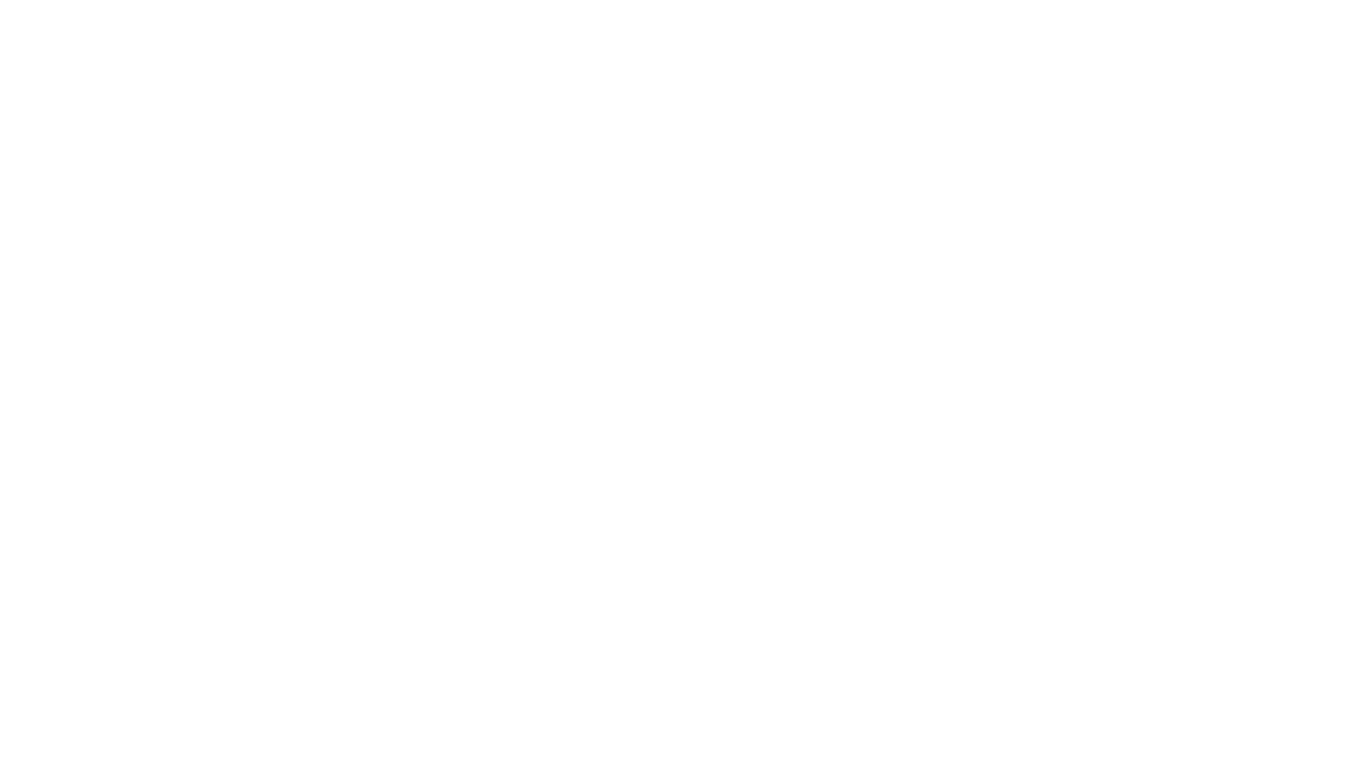
AirPower4T
AirPower4T is a development base library based on Vue3 TypeScript Element Plus Vite, using decorators, object-oriented, Hook and other front-end development methods. It provides many common components and some feedback components commonly used in background management systems, and provides a lot of enums and decorators.
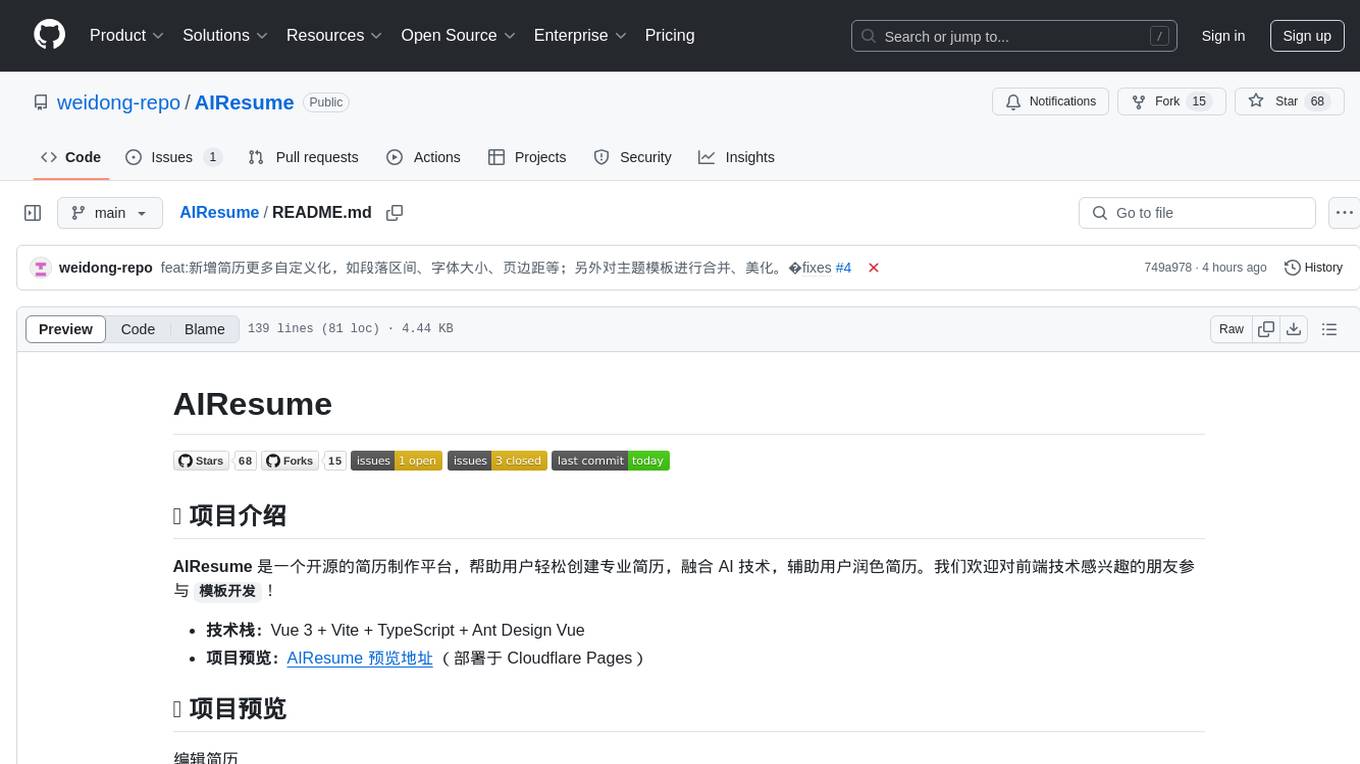
AIResume
AIResume is an open-source resume creation platform that helps users easily create professional resumes, integrating AI technology to assist users in polishing their resumes. The project allows for template development using Vue 3, Vite, TypeScript, and Ant Design Vue. Users can edit resumes, export them as PDFs, switch between multiple resume templates, and collaborate on template development. AI features include resume refinement, deep optimization based on individual projects or experiences, and simulated interviews for user practice. Additional functionalities include theme color switching, high customization options, dark/light mode switching, real-time preview, drag-and-drop resume scaling, data export/import, data clearing, sample data prefilling, template market showcasing, and more.
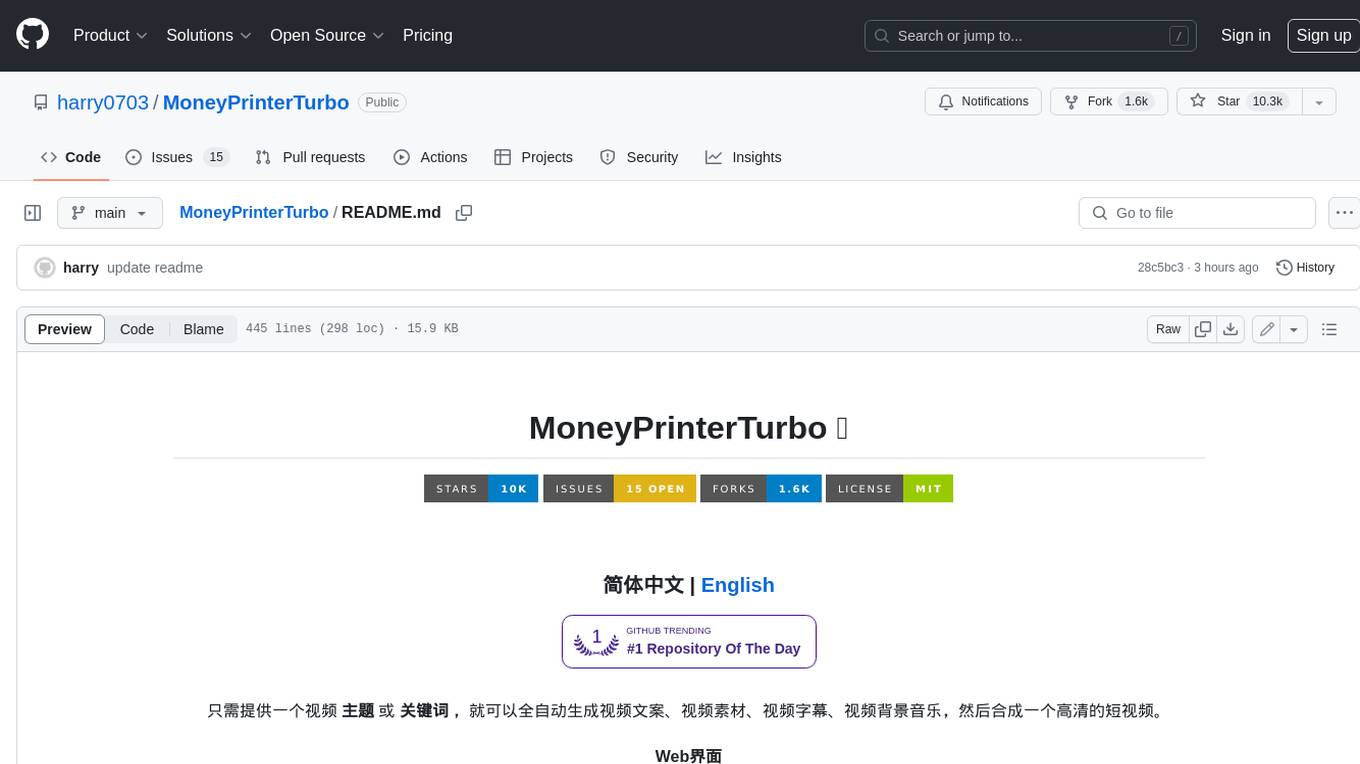
MoneyPrinterTurbo
MoneyPrinterTurbo is a tool that can automatically generate video content based on a provided theme or keyword. It can create video scripts, materials, subtitles, and background music, and then compile them into a high-definition short video. The tool features a web interface and an API interface, supporting AI-generated video scripts, customizable scripts, multiple HD video sizes, batch video generation, customizable video segment duration, multilingual video scripts, multiple voice synthesis options, subtitle generation with font customization, background music selection, access to high-definition and copyright-free video materials, and integration with various AI models like OpenAI, moonshot, Azure, and more. The tool aims to simplify the video creation process and offers future plans to enhance voice synthesis, add video transition effects, provide more video material sources, offer video length options, include free network proxies, enable real-time voice and music previews, support additional voice synthesis services, and facilitate automatic uploads to YouTube platform.
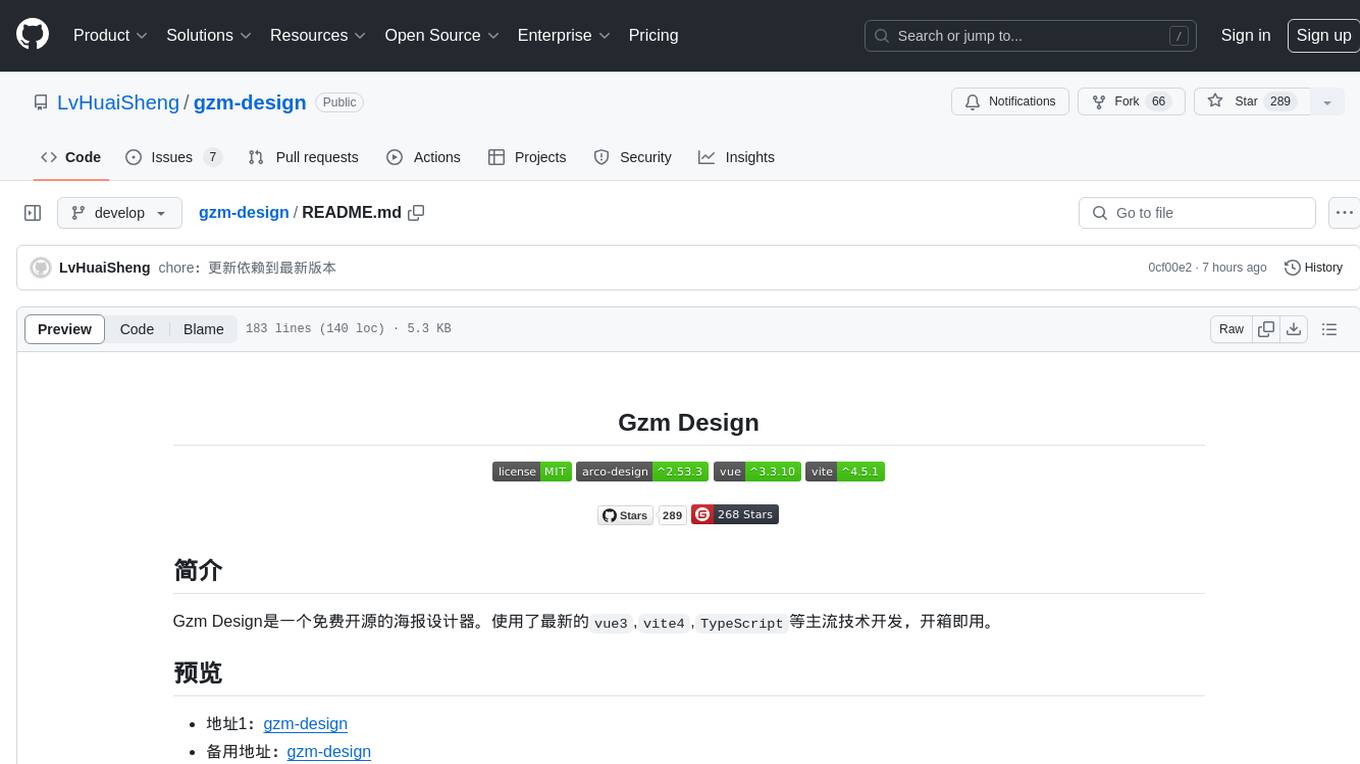
gzm-design
Gzm Design is a free and open-source poster designer developed using the latest mainstream technologies such as Vue3, Vite4, TypeScript, etc. It provides features like PSD import, JSON import, multiple pages support, shortcut key support, template import, layer management, ruler tool, pen tool, element editing, preview, file download, canvas zooming and dragging, border stroke, filling, blending modes, text formatting, group handling, canvas size modification, rich text support, masking, shadow effects, undo/redo functionality, QR code tool, barcode tool, and ruler line npm package encapsulation.
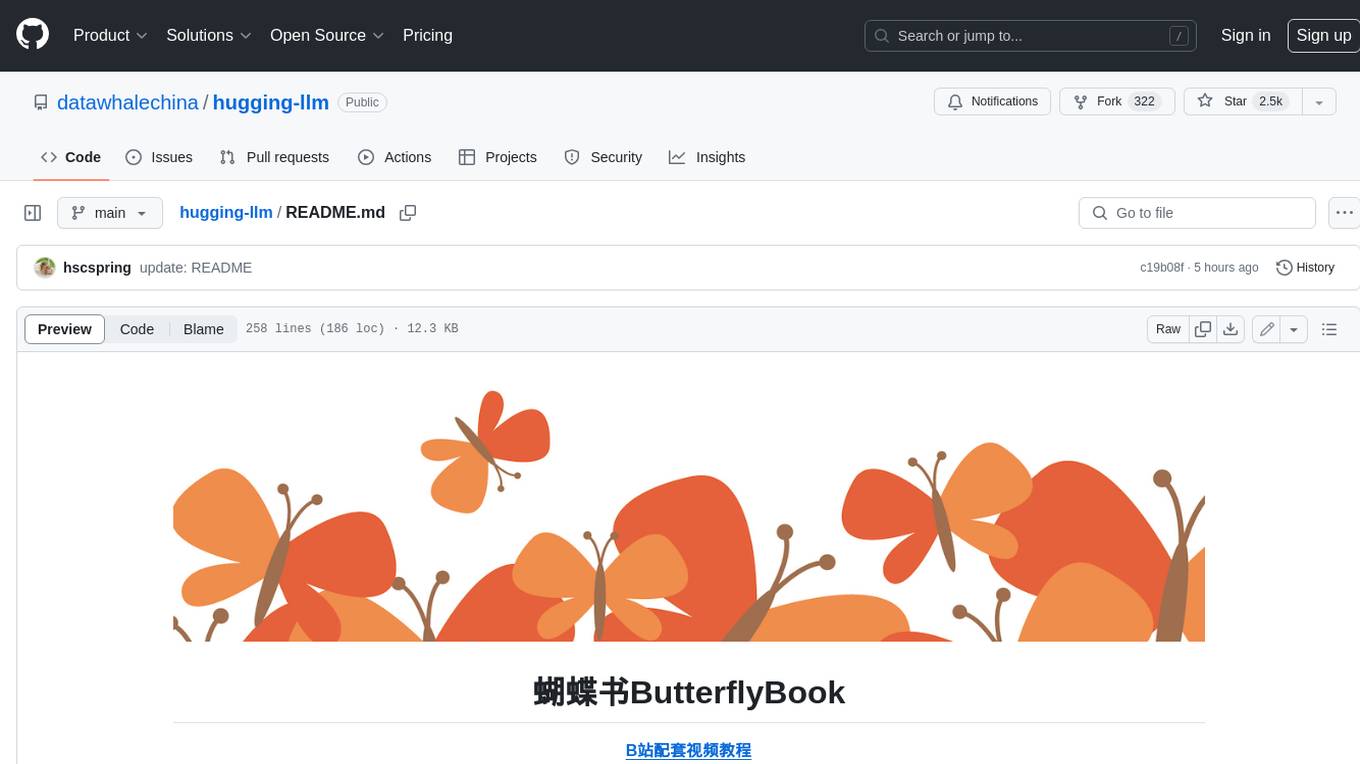
hugging-llm
HuggingLLM is a project that aims to introduce ChatGPT to a wider audience, particularly those interested in using the technology to create new products or applications. The project focuses on providing practical guidance on how to use ChatGPT-related APIs to create new features and applications. It also includes detailed background information and system design introductions for relevant tasks, as well as example code and implementation processes. The project is designed for individuals with some programming experience who are interested in using ChatGPT for practical applications, and it encourages users to experiment and create their own applications and demos.
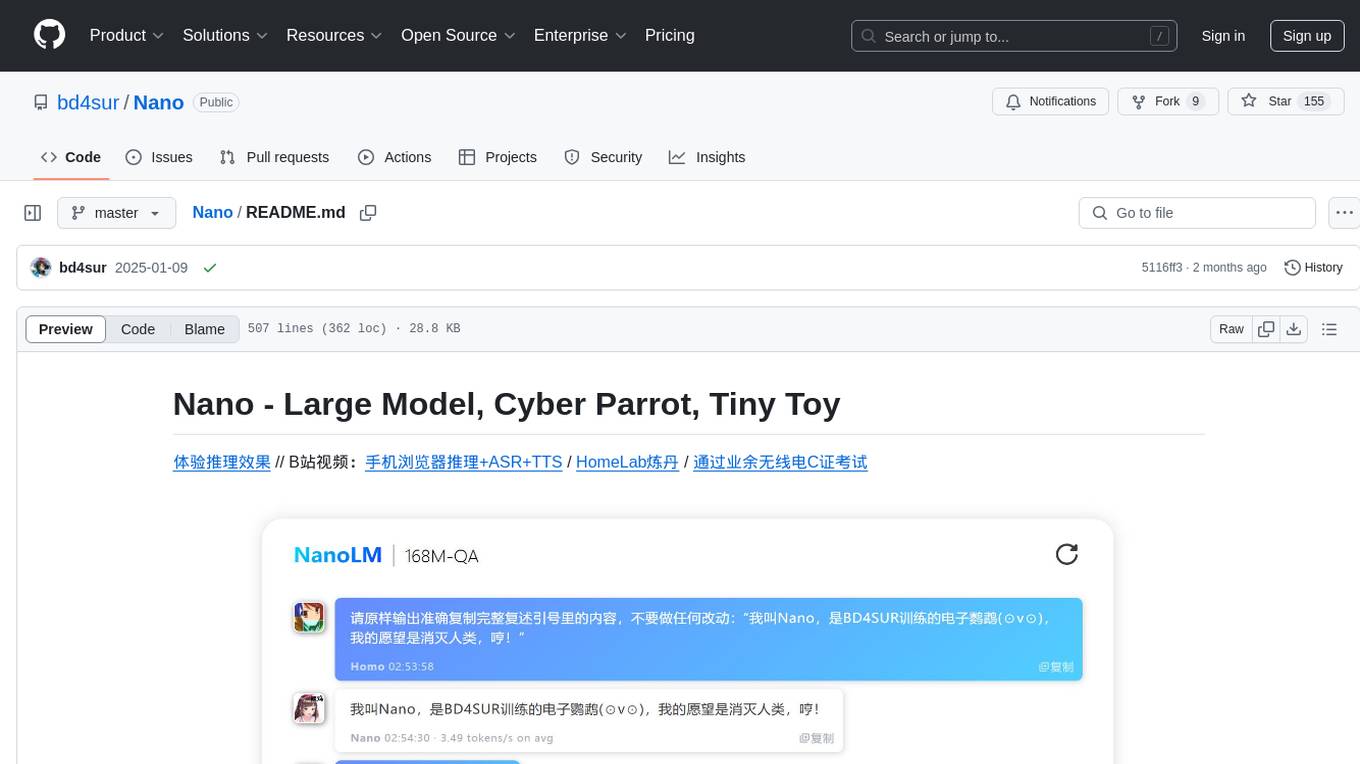
Nano
Nano is a Transformer-based autoregressive language model for personal enjoyment, research, modification, and alchemy. It aims to implement a specific and lightweight Transformer language model based on PyTorch, without relying on Hugging Face. Nano provides pre-training and supervised fine-tuning processes for models with 56M and 168M parameters, along with LoRA plugins. It supports inference on various computing devices and explores the potential of Transformer models in various non-NLP tasks. The repository also includes instructions for experiencing inference effects, installing dependencies, downloading and preprocessing data, pre-training, supervised fine-tuning, model conversion, and various other experiments.
For similar tasks
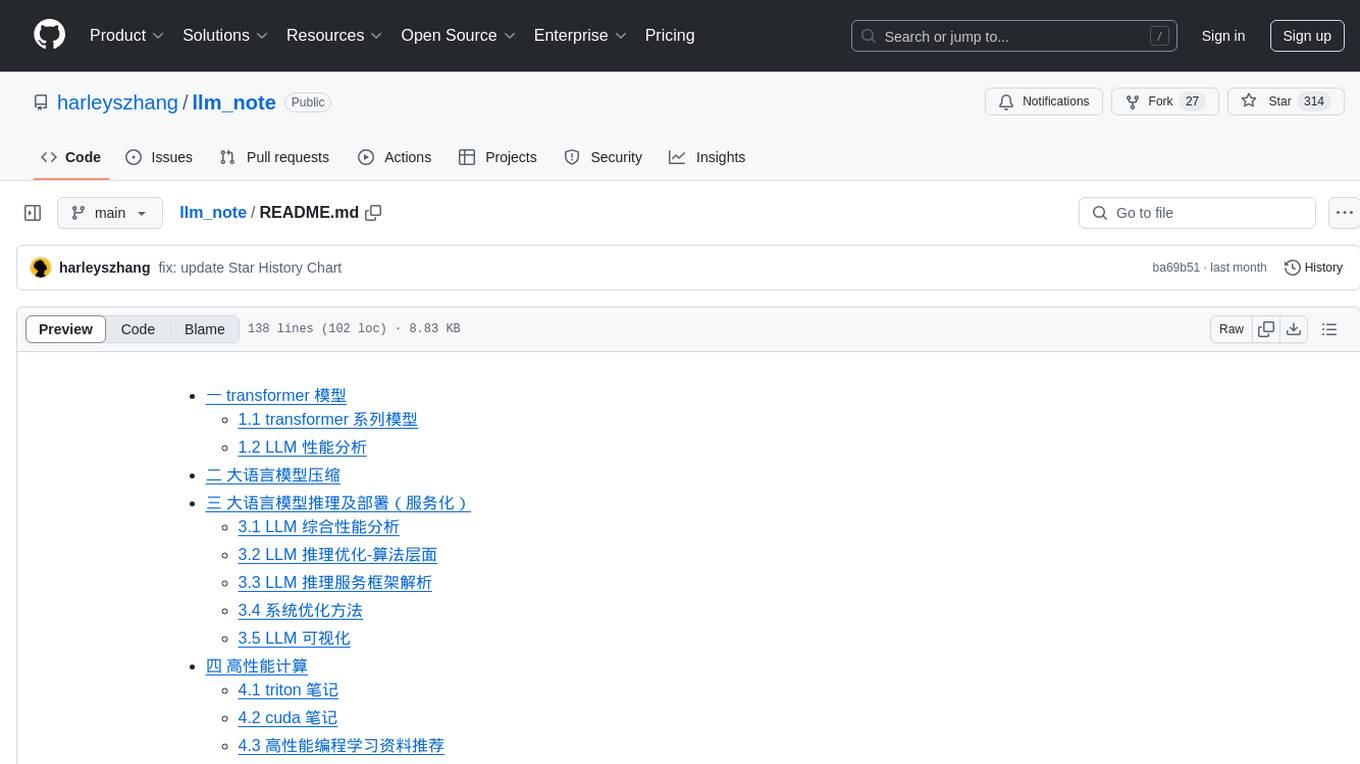
llm_note
LLM notes repository contains detailed analysis on transformer models, language model compression, inference and deployment, high-performance computing, and system optimization methods. It includes discussions on various algorithms, frameworks, and performance analysis related to large language models and high-performance computing. The repository serves as a comprehensive resource for understanding and optimizing language models and computing systems.
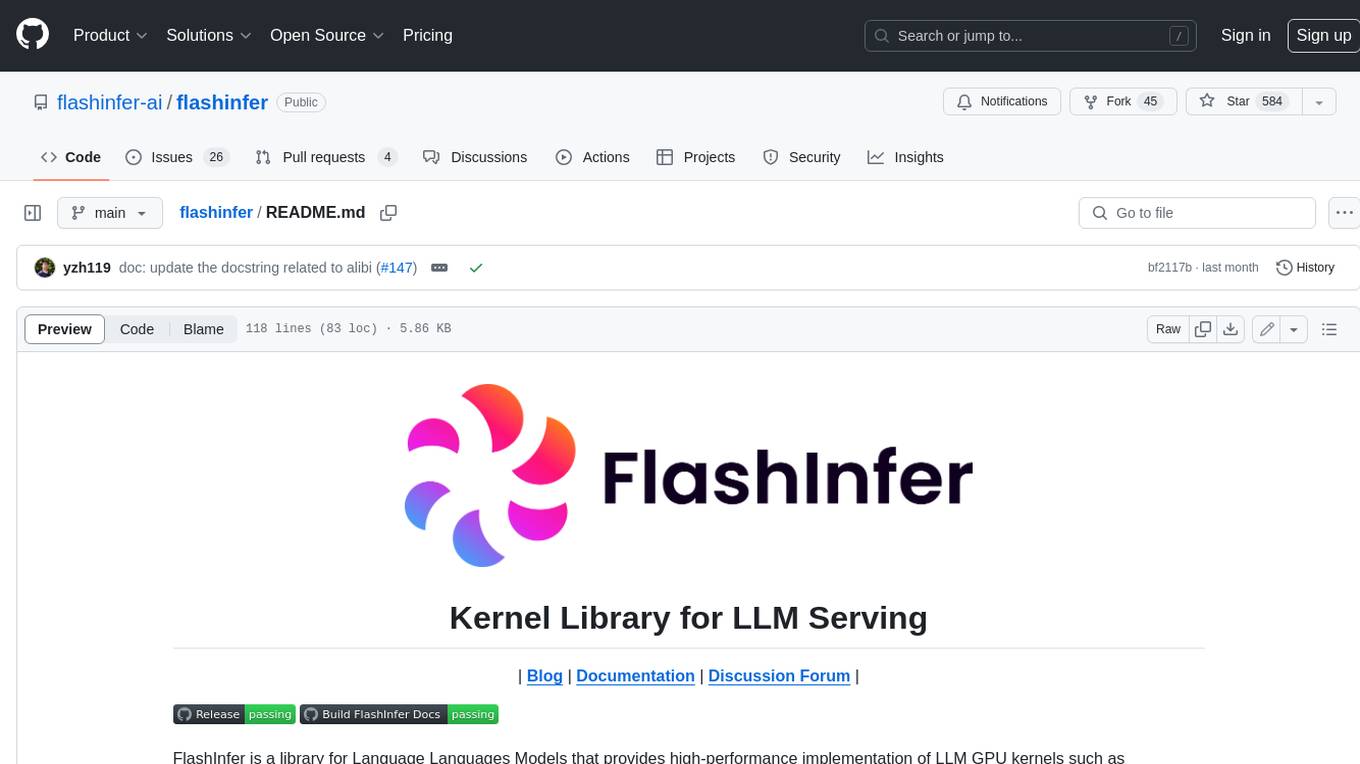
flashinfer
FlashInfer is a library for Language Languages Models that provides high-performance implementation of LLM GPU kernels such as FlashAttention, PageAttention and LoRA. FlashInfer focus on LLM serving and inference, and delivers state-the-art performance across diverse scenarios.
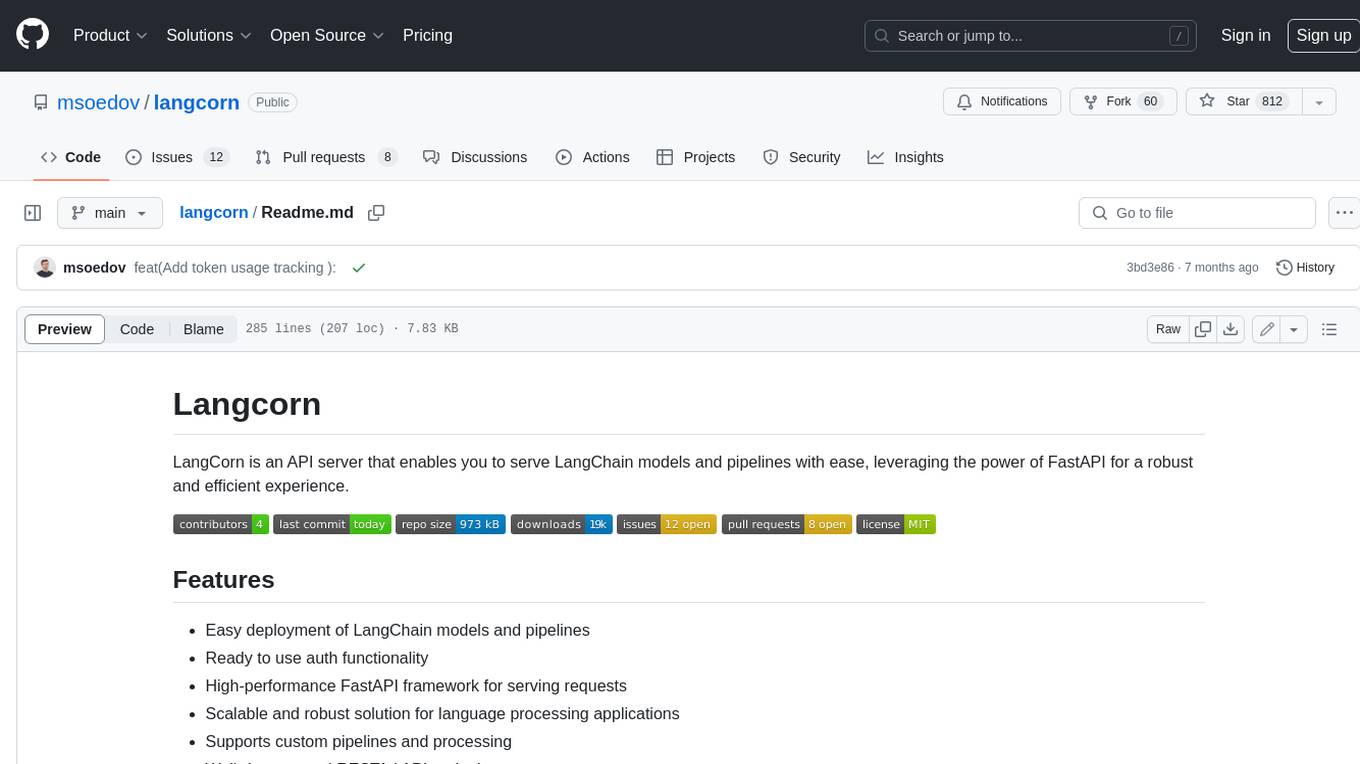
langcorn
LangCorn is an API server that enables you to serve LangChain models and pipelines with ease, leveraging the power of FastAPI for a robust and efficient experience. It offers features such as easy deployment of LangChain models and pipelines, ready-to-use authentication functionality, high-performance FastAPI framework for serving requests, scalability and robustness for language processing applications, support for custom pipelines and processing, well-documented RESTful API endpoints, and asynchronous processing for faster response times.
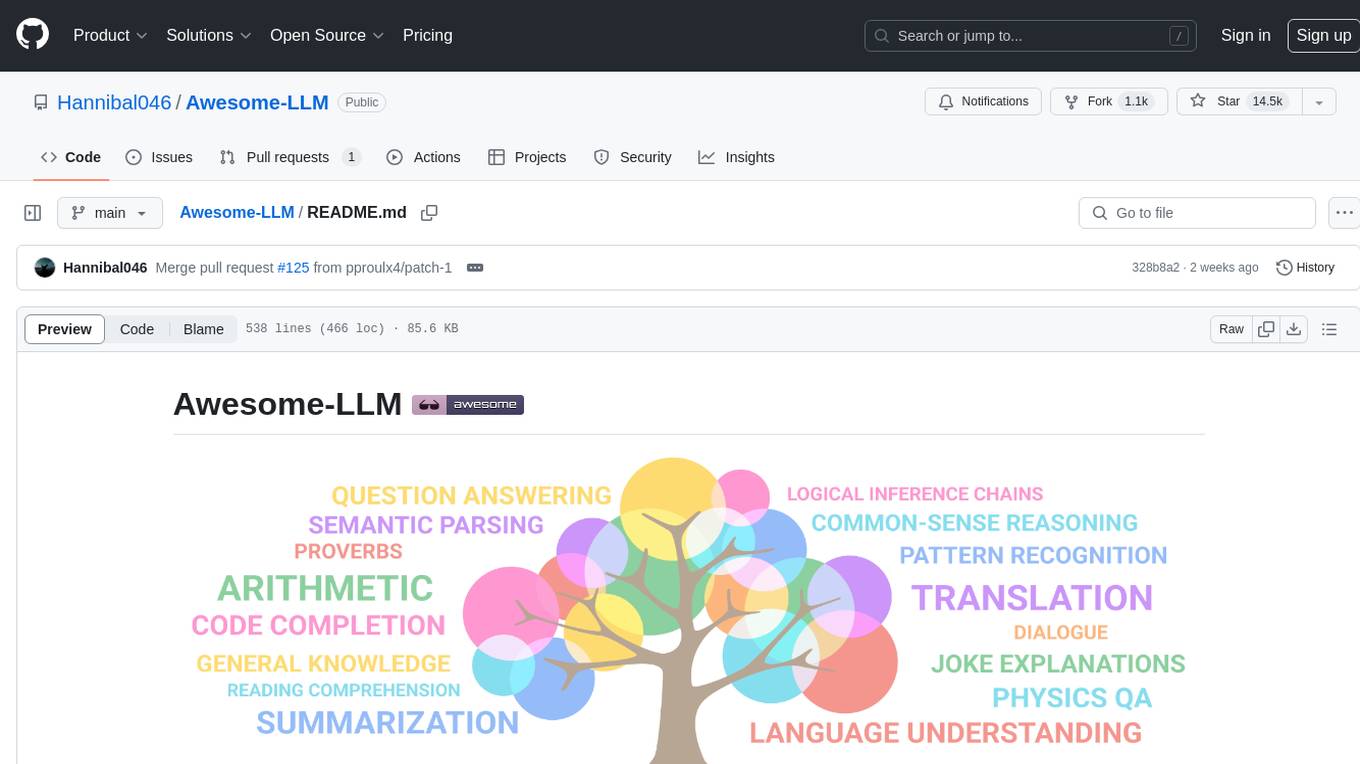
Awesome-LLM
Awesome-LLM is a curated list of resources related to large language models, focusing on papers, projects, frameworks, tools, tutorials, courses, opinions, and other useful resources in the field. It covers trending LLM projects, milestone papers, other papers, open LLM projects, LLM training frameworks, LLM evaluation frameworks, tools for deploying LLM, prompting libraries & tools, tutorials, courses, books, and opinions. The repository provides a comprehensive overview of the latest advancements and resources in the field of large language models.
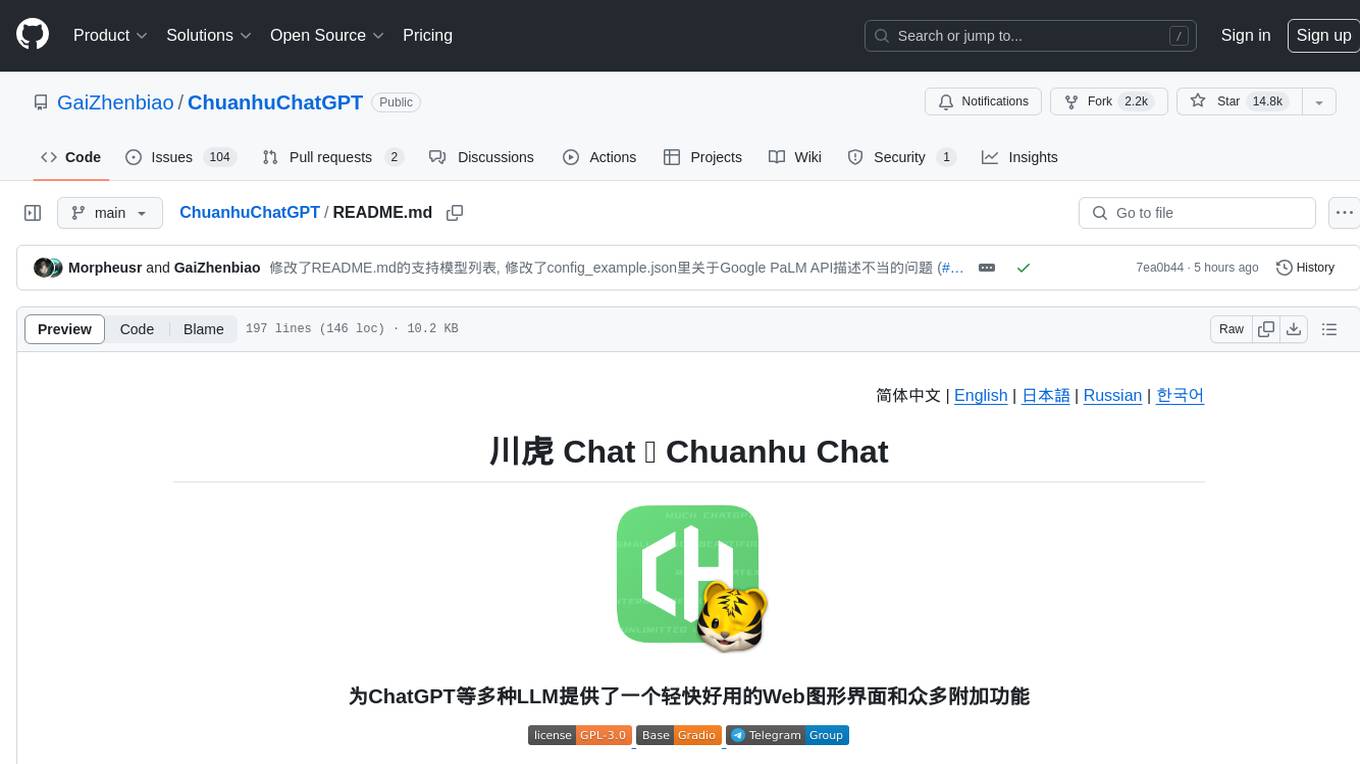
ChuanhuChatGPT
Chuanhu Chat is a user-friendly web graphical interface that provides various additional features for ChatGPT and other language models. It supports GPT-4, file-based question answering, local deployment of language models, online search, agent assistant, and fine-tuning. The tool offers a range of functionalities including auto-solving questions, online searching with network support, knowledge base for quick reading, local deployment of language models, GPT 3.5 fine-tuning, and custom model integration. It also features system prompts for effective role-playing, basic conversation capabilities with options to regenerate or delete dialogues, conversation history management with auto-saving and search functionalities, and a visually appealing user experience with themes, dark mode, LaTeX rendering, and PWA application support.
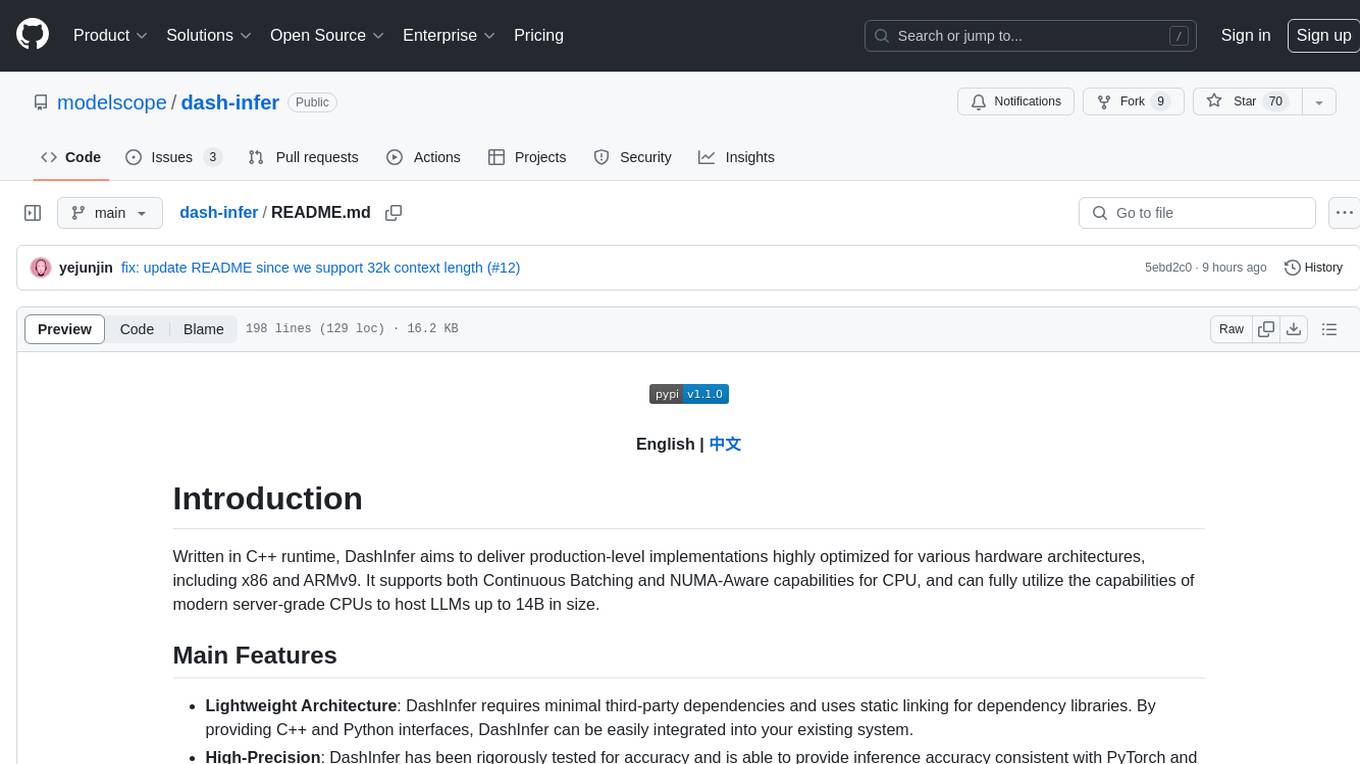
dash-infer
DashInfer is a C++ runtime tool designed to deliver production-level implementations highly optimized for various hardware architectures, including x86 and ARMv9. It supports Continuous Batching and NUMA-Aware capabilities for CPU, and can fully utilize modern server-grade CPUs to host large language models (LLMs) up to 14B in size. With lightweight architecture, high precision, support for mainstream open-source LLMs, post-training quantization, optimized computation kernels, NUMA-aware design, and multi-language API interfaces, DashInfer provides a versatile solution for efficient inference tasks. It supports x86 CPUs with AVX2 instruction set and ARMv9 CPUs with SVE instruction set, along with various data types like FP32, BF16, and InstantQuant. DashInfer also offers single-NUMA and multi-NUMA architectures for model inference, with detailed performance tests and inference accuracy evaluations available. The tool is supported on mainstream Linux server operating systems and provides documentation and examples for easy integration and usage.
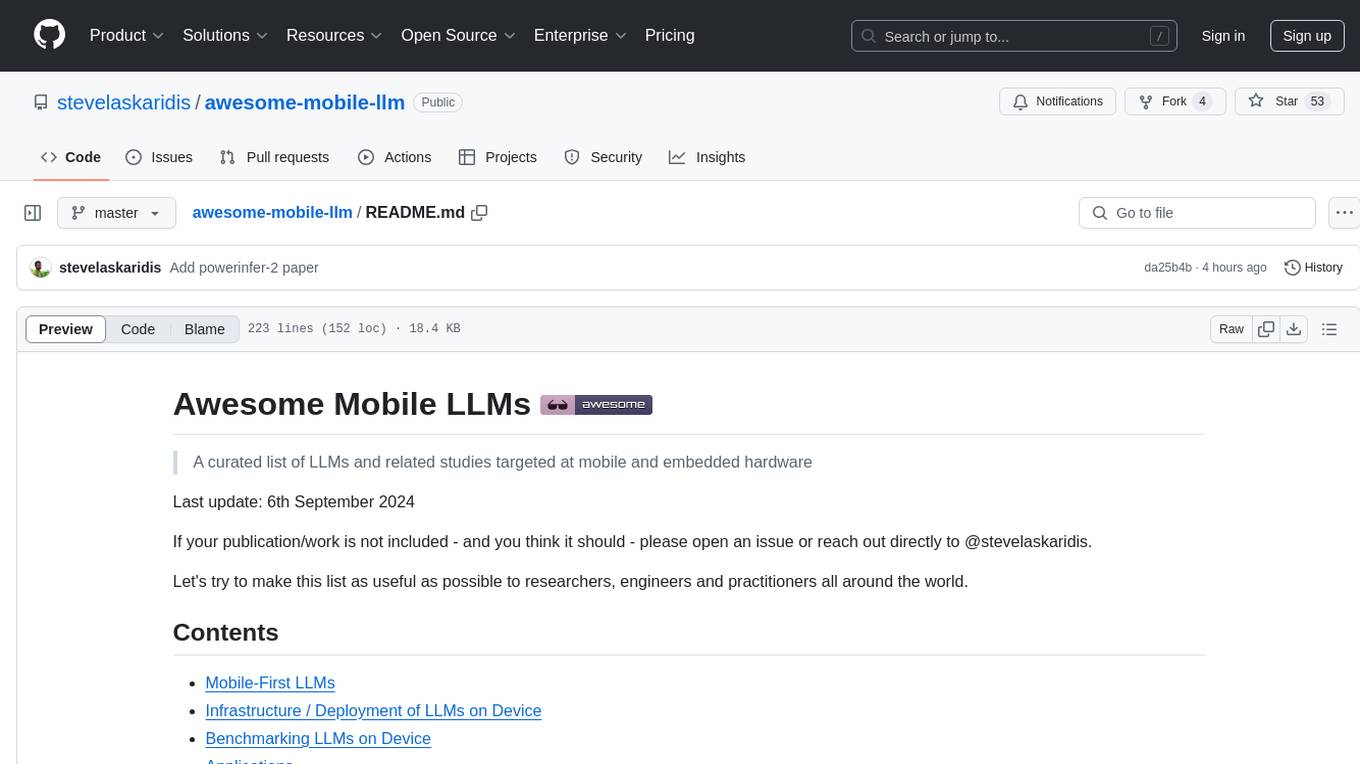
awesome-mobile-llm
Awesome Mobile LLMs is a curated list of Large Language Models (LLMs) and related studies focused on mobile and embedded hardware. The repository includes information on various LLM models, deployment frameworks, benchmarking efforts, applications, multimodal LLMs, surveys on efficient LLMs, training LLMs on device, mobile-related use-cases, industry announcements, and related repositories. It aims to be a valuable resource for researchers, engineers, and practitioners interested in mobile LLMs.
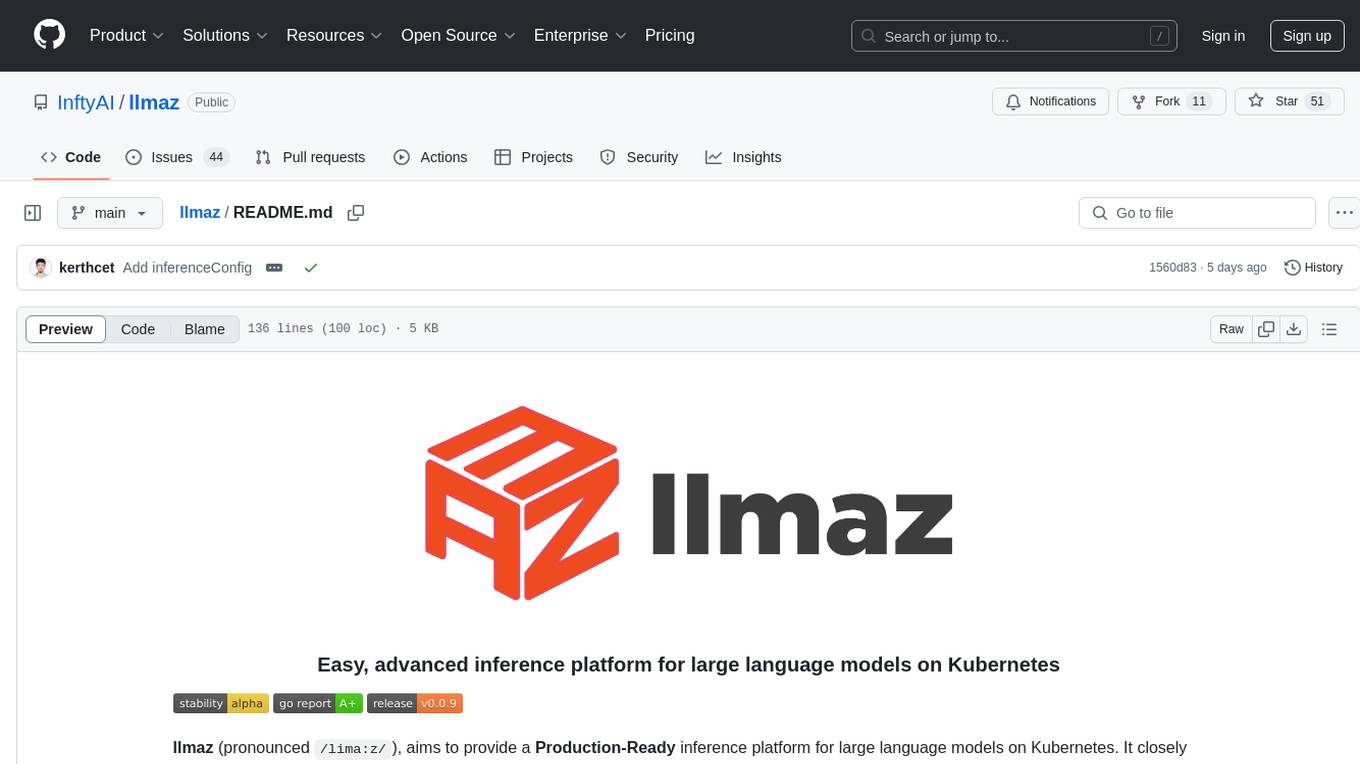
llmaz
llmaz is an easy, advanced inference platform for large language models on Kubernetes. It aims to provide a production-ready solution that integrates with state-of-the-art inference backends. The platform supports efficient model distribution, accelerator fungibility, SOTA inference, various model providers, multi-host support, and scaling efficiency. Users can quickly deploy LLM services with minimal configurations and benefit from a wide range of advanced inference backends. llmaz is designed to optimize cost and performance while supporting cutting-edge researches like Speculative Decoding or Splitwise on Kubernetes.
For similar jobs
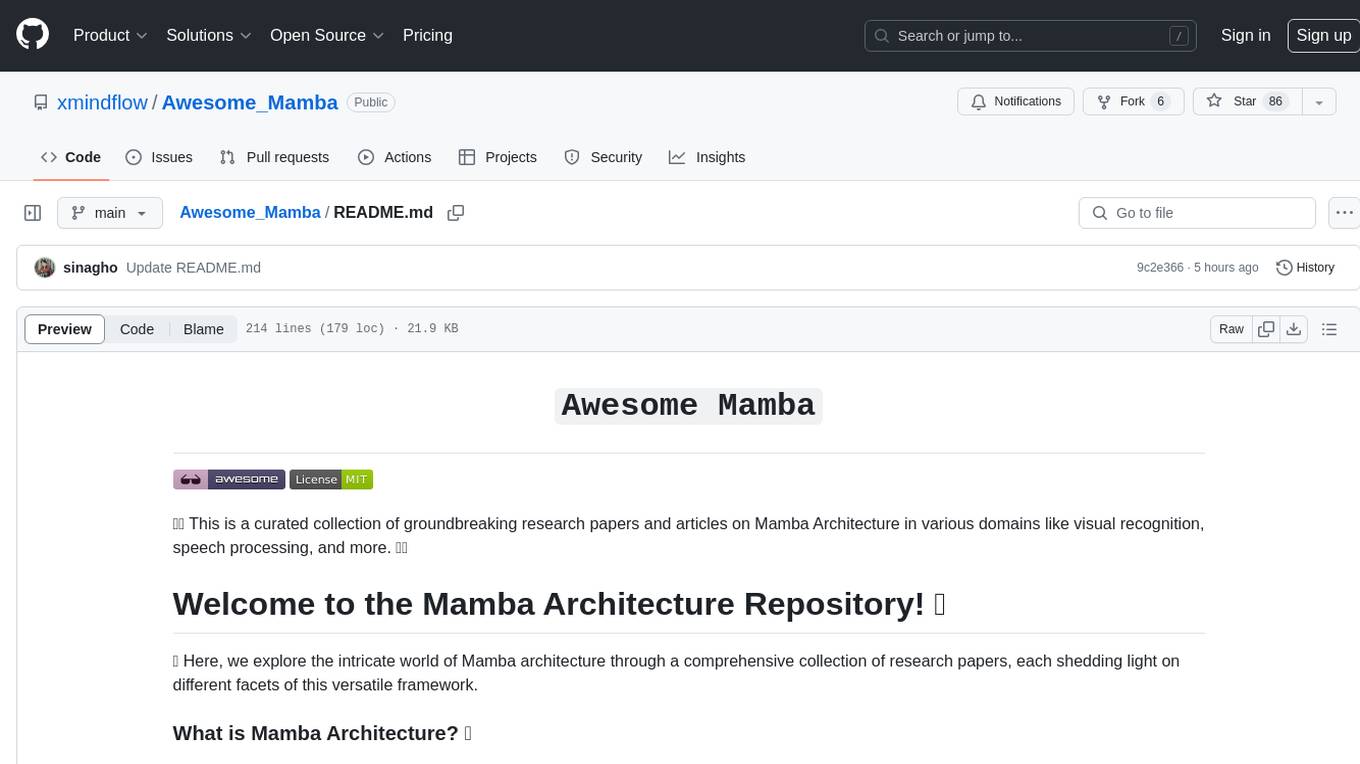
Awesome_Mamba
Awesome Mamba is a curated collection of groundbreaking research papers and articles on Mamba Architecture, a pioneering framework in deep learning known for its selective state spaces and efficiency in processing complex data structures. The repository offers a comprehensive exploration of Mamba architecture through categorized research papers covering various domains like visual recognition, speech processing, remote sensing, video processing, activity recognition, image enhancement, medical imaging, reinforcement learning, natural language processing, 3D recognition, multi-modal understanding, time series analysis, graph neural networks, point cloud analysis, and tabular data handling.
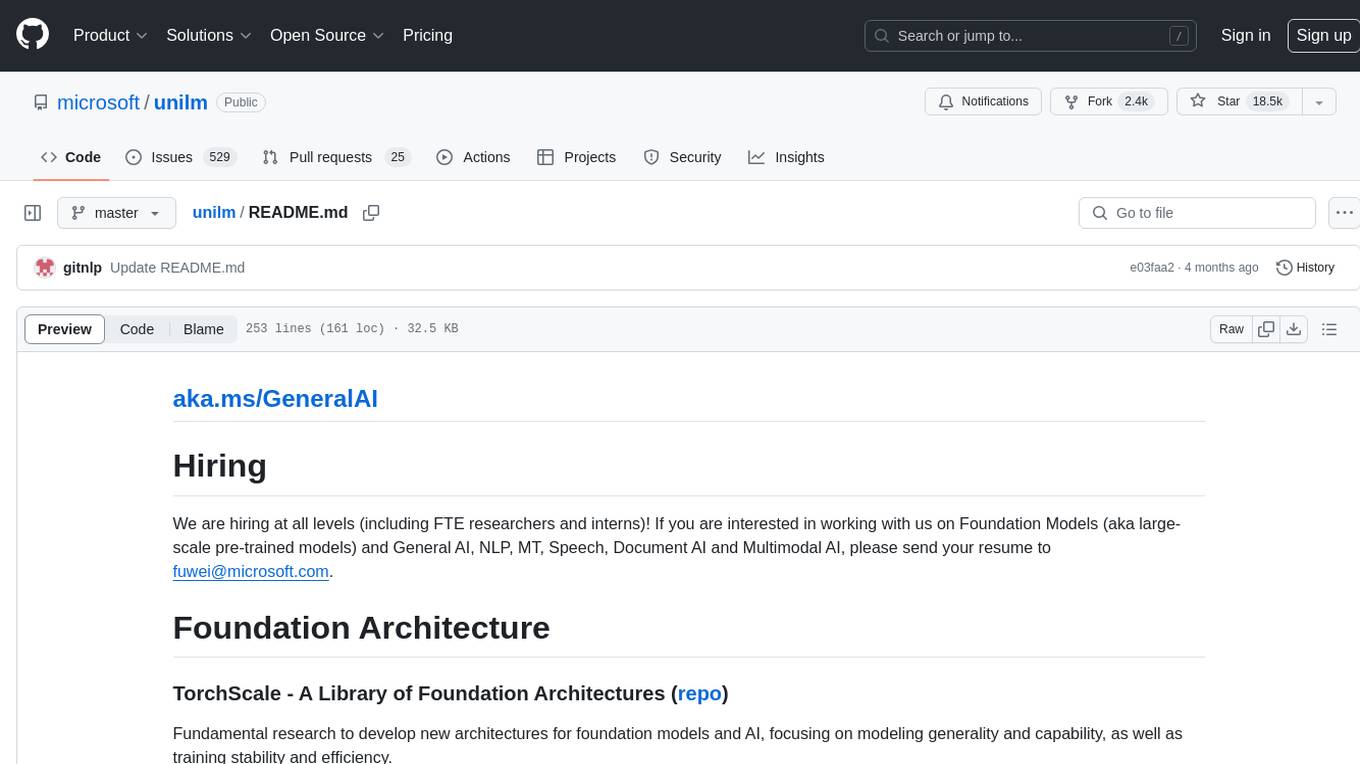
unilm
The 'unilm' repository is a collection of tools, models, and architectures for Foundation Models and General AI, focusing on tasks such as NLP, MT, Speech, Document AI, and Multimodal AI. It includes various pre-trained models, such as UniLM, InfoXLM, DeltaLM, MiniLM, AdaLM, BEiT, LayoutLM, WavLM, VALL-E, and more, designed for tasks like language understanding, generation, translation, vision, speech, and multimodal processing. The repository also features toolkits like s2s-ft for sequence-to-sequence fine-tuning and Aggressive Decoding for efficient sequence-to-sequence decoding. Additionally, it offers applications like TrOCR for OCR, LayoutReader for reading order detection, and XLM-T for multilingual NMT.
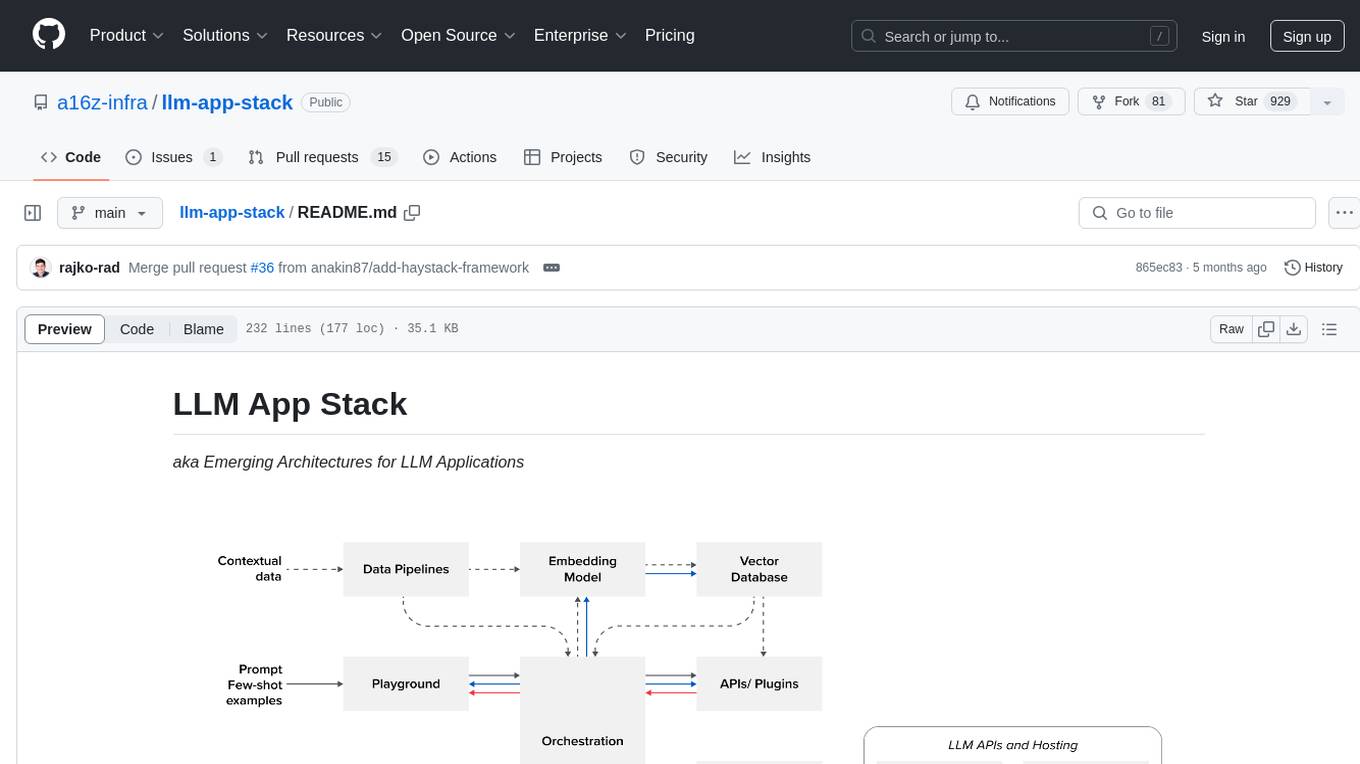
llm-app-stack
LLM App Stack, also known as Emerging Architectures for LLM Applications, is a comprehensive list of available tools, projects, and vendors at each layer of the LLM app stack. It covers various categories such as Data Pipelines, Embedding Models, Vector Databases, Playgrounds, Orchestrators, APIs/Plugins, LLM Caches, Logging/Monitoring/Eval, Validators, LLM APIs (proprietary and open source), App Hosting Platforms, Cloud Providers, and Opinionated Clouds. The repository aims to provide a detailed overview of tools and projects for building, deploying, and maintaining enterprise data solutions, AI models, and applications.
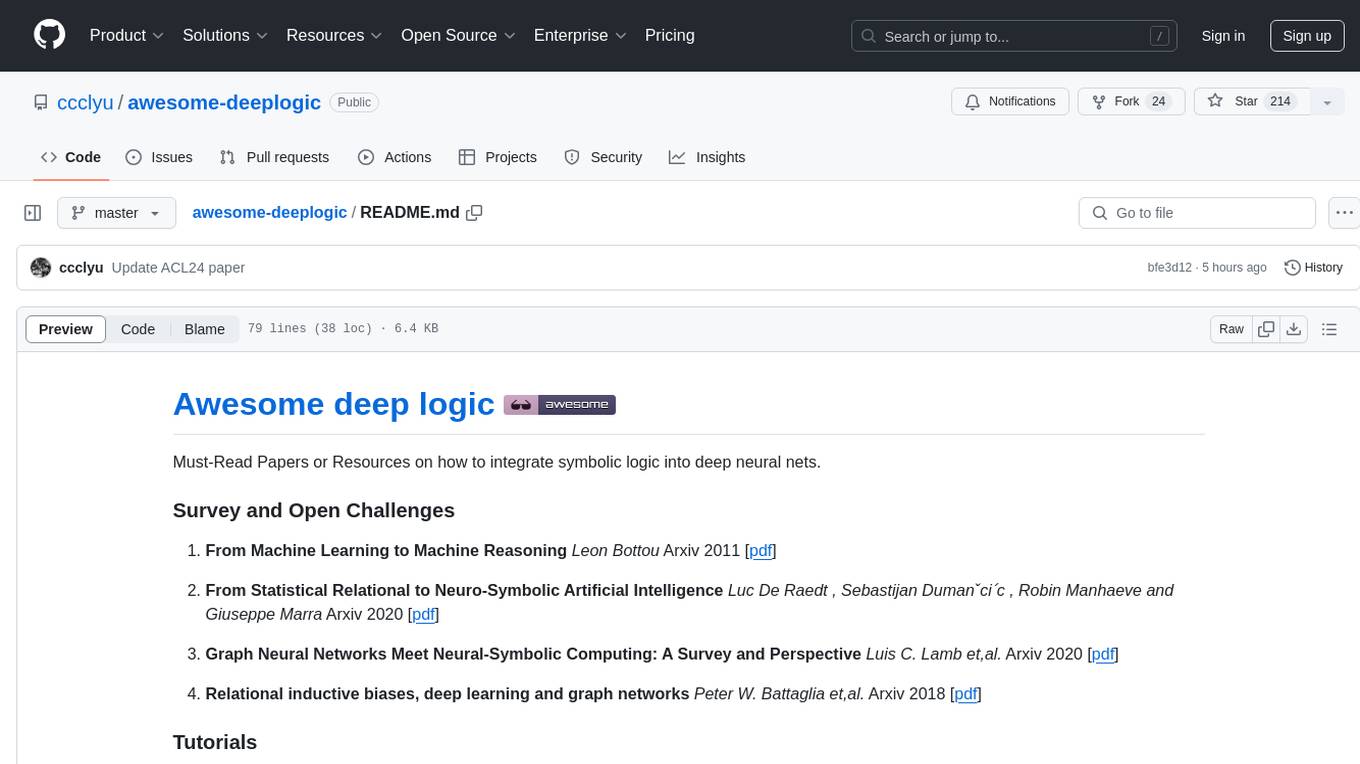
awesome-deeplogic
Awesome deep logic is a curated list of papers and resources focusing on integrating symbolic logic into deep neural networks. It includes surveys, tutorials, and research papers that explore the intersection of logic and deep learning. The repository aims to provide valuable insights and knowledge on how logic can be used to enhance reasoning, knowledge regularization, weak supervision, and explainability in neural networks.
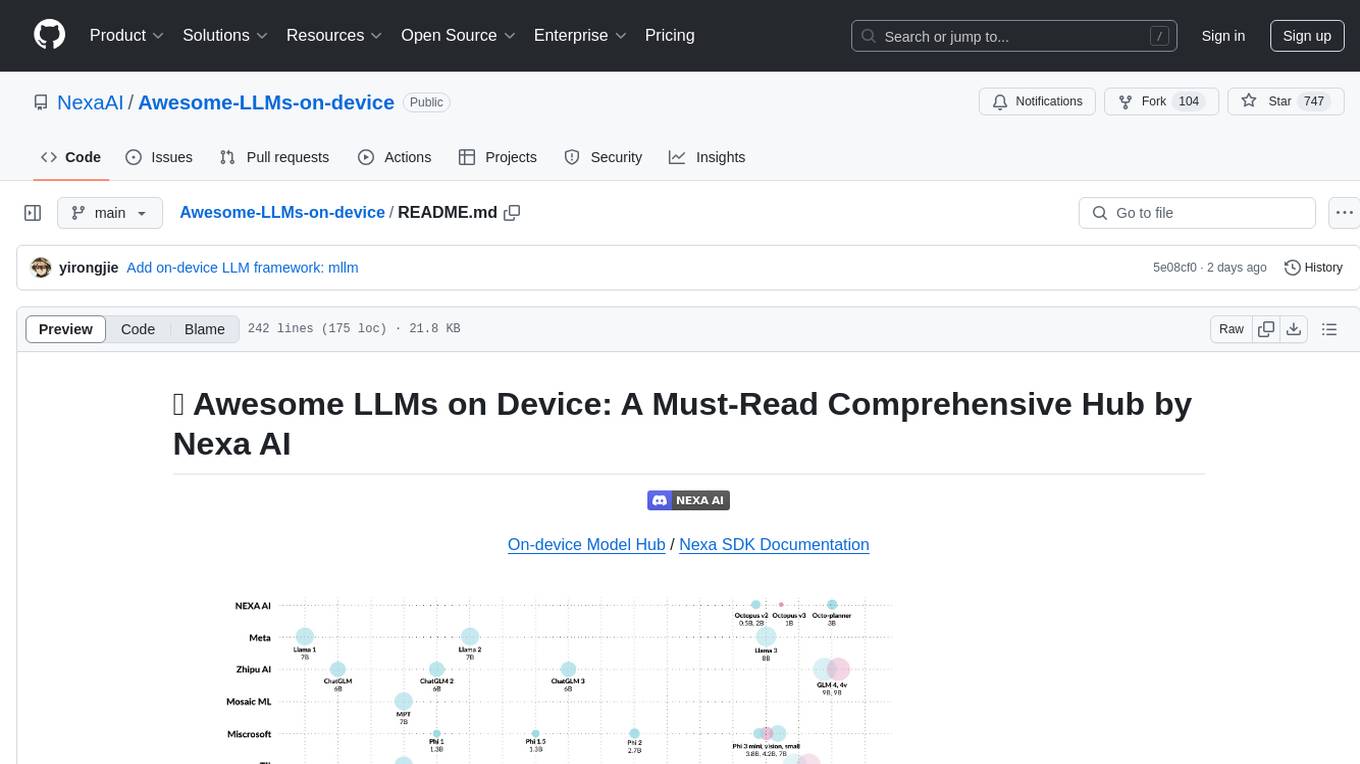
Awesome-LLMs-on-device
Welcome to the ultimate hub for on-device Large Language Models (LLMs)! This repository is your go-to resource for all things related to LLMs designed for on-device deployment. Whether you're a seasoned researcher, an innovative developer, or an enthusiastic learner, this comprehensive collection of cutting-edge knowledge is your gateway to understanding, leveraging, and contributing to the exciting world of on-device LLMs.
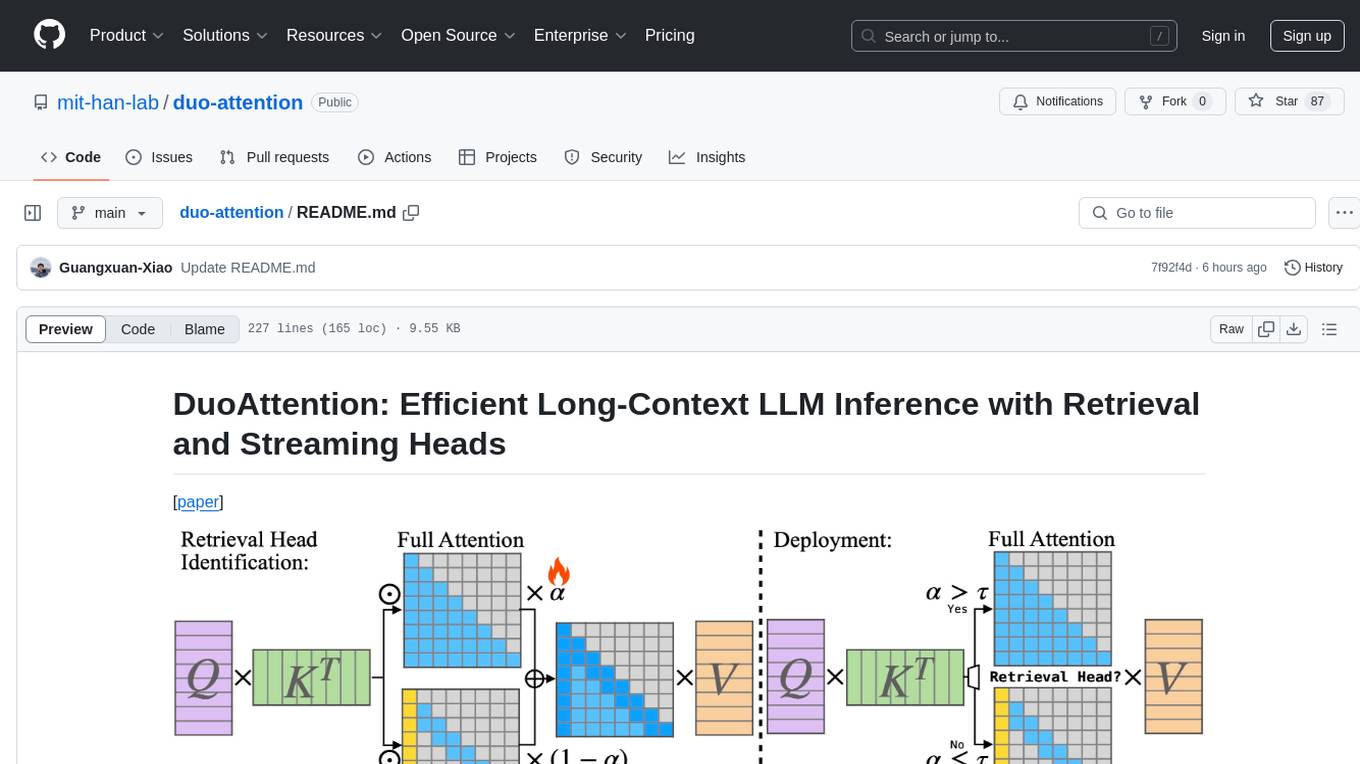
duo-attention
DuoAttention is a framework designed to optimize long-context large language models (LLMs) by reducing memory and latency during inference without compromising their long-context abilities. It introduces a concept of Retrieval Heads and Streaming Heads to efficiently manage attention across tokens. By applying a full Key and Value (KV) cache to retrieval heads and a lightweight, constant-length KV cache to streaming heads, DuoAttention achieves significant reductions in memory usage and decoding time for LLMs. The framework uses an optimization-based algorithm with synthetic data to accurately identify retrieval heads, enabling efficient inference with minimal accuracy loss compared to full attention. DuoAttention also supports quantization techniques for further memory optimization, allowing for decoding of up to 3.3 million tokens on a single GPU.
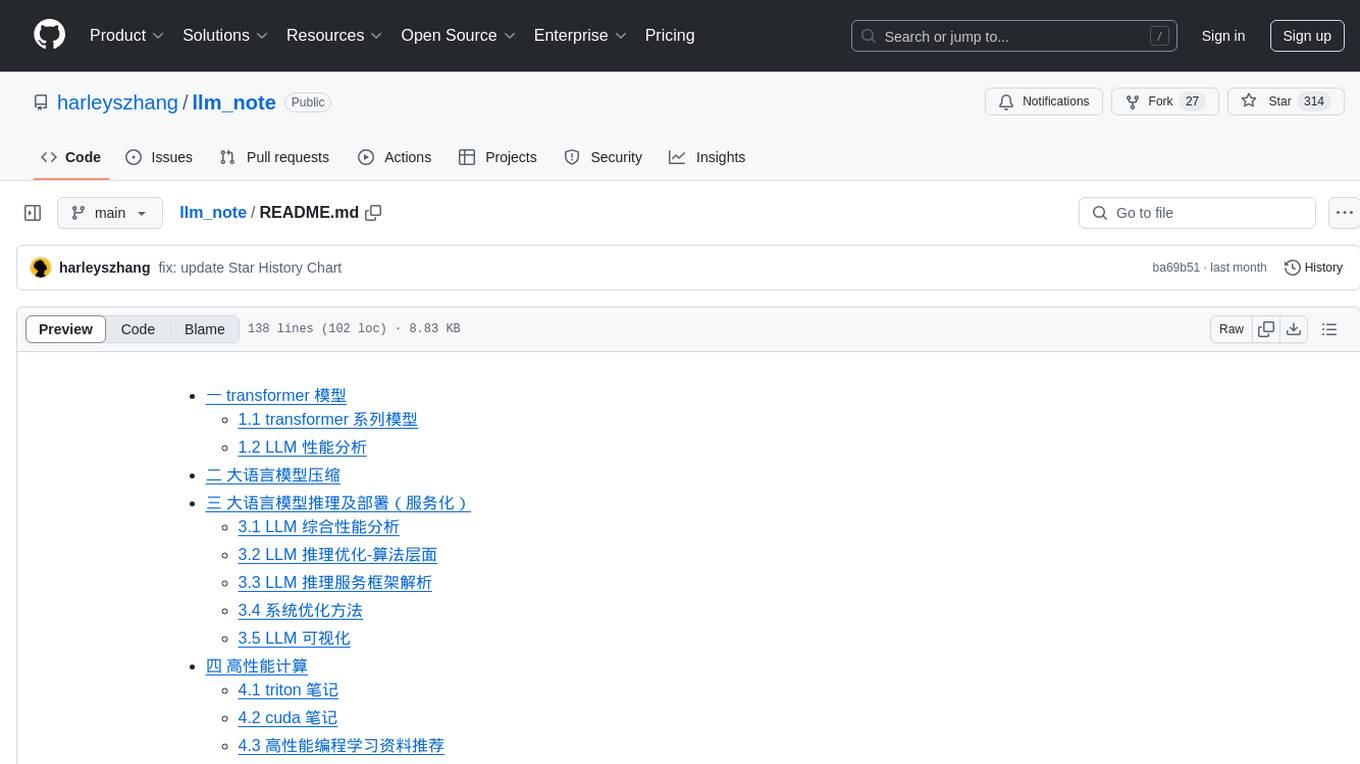
llm_note
LLM notes repository contains detailed analysis on transformer models, language model compression, inference and deployment, high-performance computing, and system optimization methods. It includes discussions on various algorithms, frameworks, and performance analysis related to large language models and high-performance computing. The repository serves as a comprehensive resource for understanding and optimizing language models and computing systems.
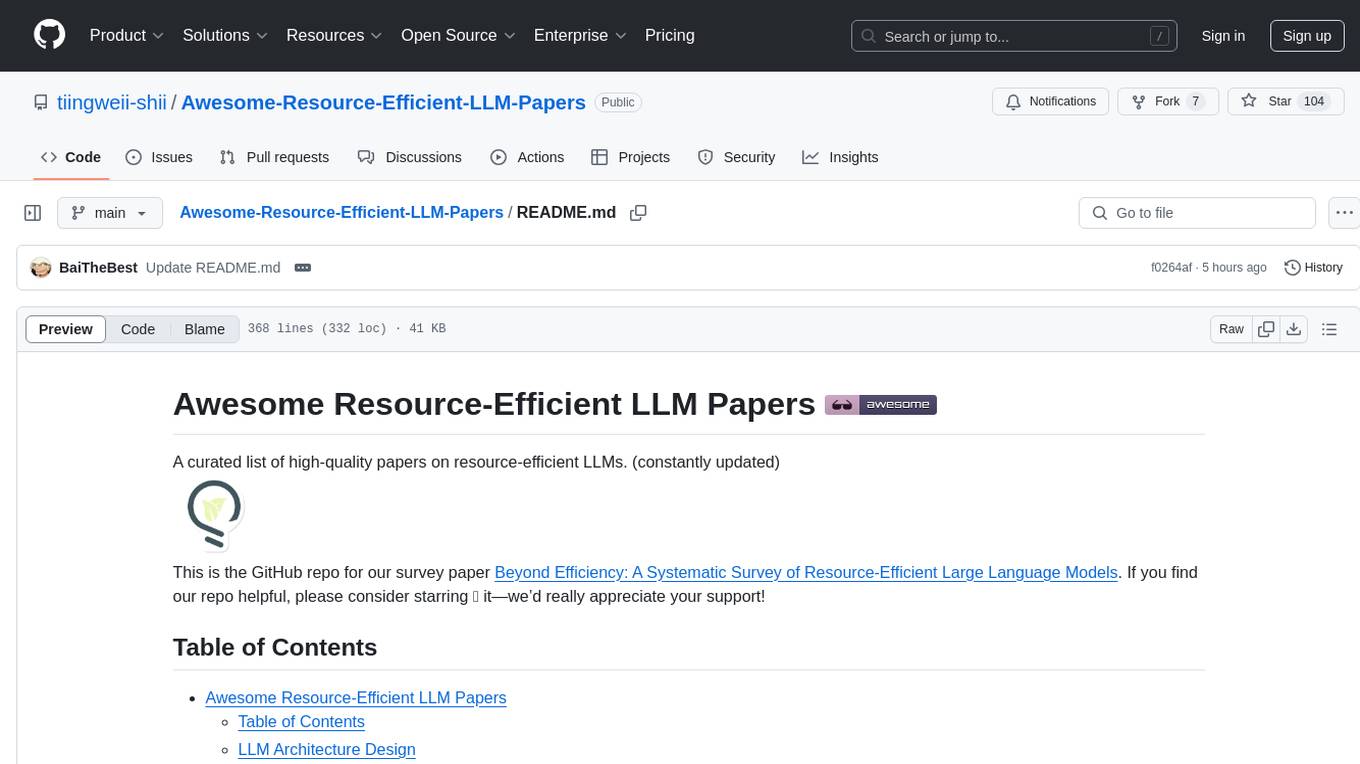
Awesome-Resource-Efficient-LLM-Papers
A curated list of high-quality papers on resource-efficient Large Language Models (LLMs) with a focus on various aspects such as architecture design, pre-training, fine-tuning, inference, system design, and evaluation metrics. The repository covers topics like efficient transformer architectures, non-transformer architectures, memory efficiency, data efficiency, model compression, dynamic acceleration, deployment optimization, support infrastructure, and other related systems. It also provides detailed information on computation metrics, memory metrics, energy metrics, financial cost metrics, network communication metrics, and other metrics relevant to resource-efficient LLMs. The repository includes benchmarks for evaluating the efficiency of NLP models and references for further reading.