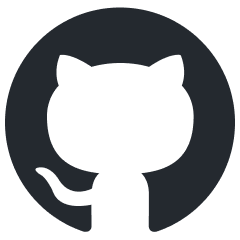
FinRobot
FinRobot: An Open-Source AI Agent Platform for Financial Applications using LLMs π π π
Stars: 1008
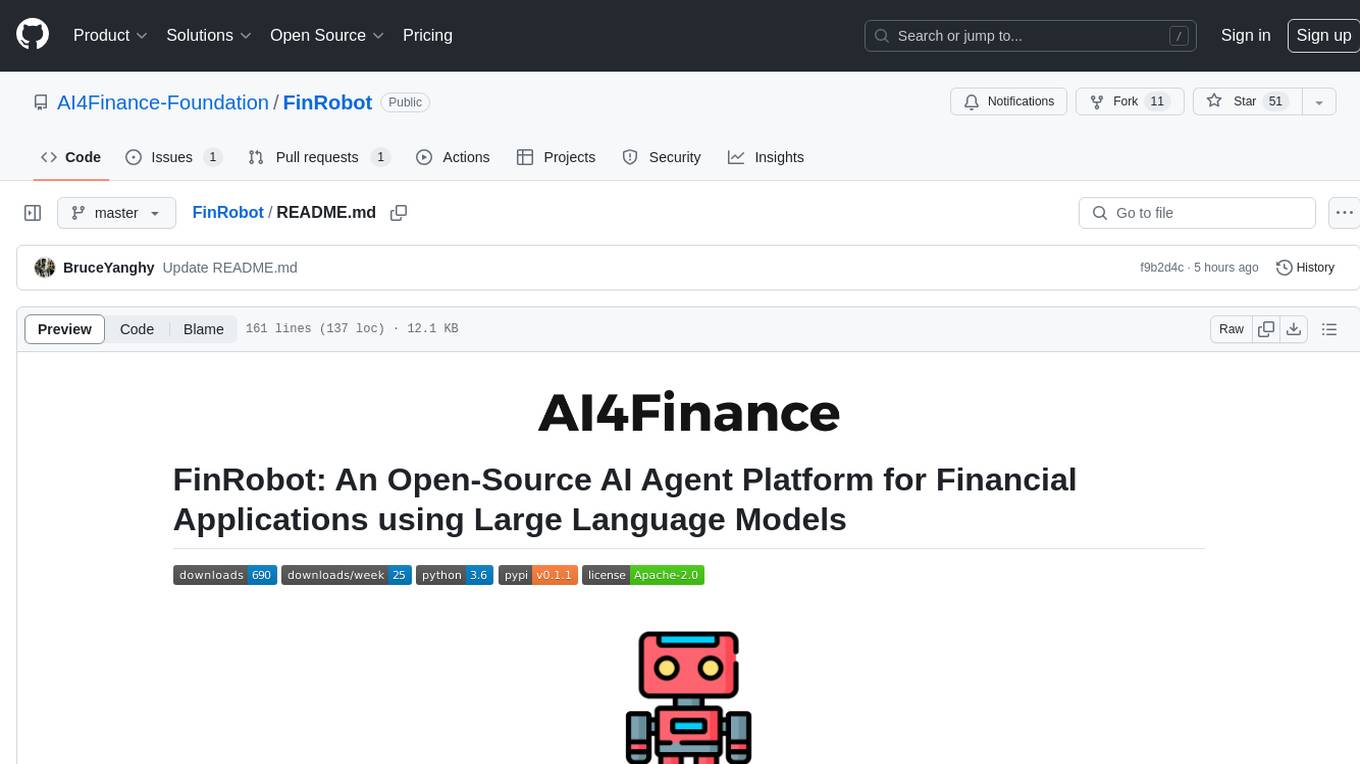
FinRobot is an open-source AI agent platform designed for financial applications using large language models. It transcends the scope of FinGPT, offering a comprehensive solution that integrates a diverse array of AI technologies. The platform's versatility and adaptability cater to the multifaceted needs of the financial industry. FinRobot's ecosystem is organized into four layers, including Financial AI Agents Layer, Financial LLMs Algorithms Layer, LLMOps and DataOps Layers, and Multi-source LLM Foundation Models Layer. The platform's agent workflow involves Perception, Brain, and Action modules to capture, process, and execute financial data and insights. The Smart Scheduler optimizes model diversity and selection for tasks, managed by components like Director Agent, Agent Registration, Agent Adaptor, and Task Manager. The tool provides a structured file organization with subfolders for agents, data sources, and functional modules, along with installation instructions and hands-on tutorials.
README:
FinRobot is an AI Agent Platform that transcends the scope of FinGPT, representing a comprehensive solution meticulously designed for financial applications. It integrates a diverse array of AI technologies, extending beyond mere language models. This expansive vision highlights the platform's versatility and adaptability, addressing the multifaceted needs of the financial industry.
Concept of AI Agent: an AI Agent is an intelligent entity that uses large language models as its brain to perceive its environment, make decisions, and execute actions. Unlike traditional artificial intelligence, AI Agents possess the ability to independently think and utilize tools to progressively achieve given objectives.
The overall framework of FinRobot is organized into four distinct layers, each designed to address specific aspects of financial AI processing and application:
- Financial AI Agents Layer: The Financial AI Agents Layer now includes Financial Chain-of-Thought (CoT) prompting, enhancing complex analysis and decision-making capacity. Market Forecasting Agents, Document Analysis Agents, and Trading Strategies Agents utilize CoT to dissect financial challenges into logical steps, aligning their advanced algorithms and domain expertise with the evolving dynamics of financial markets for precise, actionable insights.
- Financial LLMs Algorithms Layer: The Financial LLMs Algorithms Layer configures and utilizes specially tuned models tailored to specific domains and global market analysis.
- LLMOps and DataOps Layers: The LLMOps layer implements a multi-source integration strategy that selects the most suitable LLMs for specific financial tasks, utilizing a range of state-of-the-art models.
- Multi-source LLM Foundation Models Layer: This foundational layer supports the plug-and-play functionality of various general and specialized LLMs.
-
Perception: This module captures and interprets multimodal financial data from market feeds, news, and economic indicators, using sophisticated techniques to structure the data for thorough analysis.
-
Brain: Acting as the core processing unit, this module perceives data from the Perception module with LLMs and utilizes Financial Chain-of-Thought (CoT) processes to generate structured instructions.
-
Action: This module executes instructions from the Brain module, applying tools to translate analytical insights into actionable outcomes. Actions include trading, portfolio adjustments, generating reports, or sending alerts, thereby actively influencing the financial environment.
The Smart Scheduler is central to ensuring model diversity and optimizing the integration and selection of the most appropriate LLM for each task.
- Director Agent: This component orchestrates the task assignment process, ensuring that tasks are allocated to agents based on their performance metrics and suitability for specific tasks.
- Agent Registration: Manages the registration and tracks the availability of agents within the system, facilitating an efficient task allocation process.
- Agent Adaptor: Tailor agent functionalities to specific tasks, enhancing their performance and integration within the overall system.
- Task Manager: Manages and stores different general and fine-tuned LLMs-based agents tailored for various financial tasks, updated periodically to ensure relevance and efficacy.
The main folder finrobot has three subfolders agents, data_source, functional.
FinRobot
βββ finrobot (main folder)
β βββ agents
β βββ agent_library.py
β βββ workflow.py
β βββ data_source
β βββ finnhub_utils.py
β βββ finnlp_utils.py
β βββ fmp_utils.py
β βββ sec_utils.py
β βββ yfinance_utils.py
β βββ functional
β βββ analyzer.py
β βββ charting.py
β βββ coding.py
β βββ quantitative.py
β βββ reportlab.py
β βββ text.py
β βββ toolkits.py
β βββ utils.py
β
βββ configs
βββ experiments
βββ tutorials_beginner (hands-on tutorial)
β βββ agent_fingpt_forecaster.ipynb
β βββ agent_annual_report.ipynb
βββ tutorials_advanced (advanced tutorials for potential finrobot developers)
β βββ agent_trade_strategist.ipynb
β βββ agent_fingpt_forecaster.ipynb
β βββ agent_annual_report.ipynb
β βββ lmm_agent_mplfinance.ipynb
β βββ lmm_agent_opt_smacross.ipynb
βββ setup.py
βββ OAI_CONFIG_LIST_sample
βββ config_api_keys_sample
βββ requirements.txt
βββ README.md
1. (Recommended) Create a new virtual environment
conda create --name finrobot python=3.10
conda activate finrobot
2. download the FinRobot repo use terminal or download it manually
git clone https://github.com/AI4Finance-Foundation/FinRobot.git
cd FinRobot
3. install finrobot & dependencies from source or pypi
get our latest release from pypi
pip install -U finrobot
or install from this repo directly
pip install -e .
4. modify OAI_CONFIG_LIST_sample file
1) rename OAI_CONFIG_LIST_sample to OAI_CONFIG_LIST
2) remove the four lines of comment within the OAI_CONFIG_LIST file
3) add your own openai api-key <your OpenAI API key here>
5. modify config_api_keys_sample file
1) rename config_api_keys_sample to config_api_keys
2) remove the comment within the config_api_keys file
3) add your own finnhub-api "YOUR_FINNHUB_API_KEY"
4) add your own financialmodelingprep and sec-api keys "YOUR_FMP_API_KEY" and "YOUR_SEC_API_KEY" (for financial report generation)
6. start navigating the tutorials or the demos below:
# find these notebooks in tutorials
1) agent_annual_report.ipynb
2) agent_fingpt_forecaster.ipynb
3) agent_trade_strategist.ipynb
4) lmm_agent_mplfinance.ipynb
5) lmm_agent_opt_smacross.ipynb
Takes a company's ticker symbol, recent basic financials, and market news as input and predicts its stock movements.
- Import
import autogen
from finrobot.utils import get_current_date, register_keys_from_json
from finrobot.agents.workflow import SingleAssistant
- Config
# Read OpenAI API keys from a JSON file
llm_config = {
"config_list": autogen.config_list_from_json(
"../OAI_CONFIG_LIST",
filter_dict={"model": ["gpt-4-0125-preview"]},
),
"timeout": 120,
"temperature": 0,
}
# Register FINNHUB API keys
register_keys_from_json("../config_api_keys")
- Run
company = "NVDA"
assitant = SingleAssistant(
"Market_Analyst",
llm_config,
# set to "ALWAYS" if you want to chat instead of simply receiving the prediciton
human_input_mode="NEVER",
)
assitant.chat(
f"Use all the tools provided to retrieve information available for {company} upon {get_current_date()}. Analyze the positive developments and potential concerns of {company} "
"with 2-4 most important factors respectively and keep them concise. Most factors should be inferred from company related news. "
f"Then make a rough prediction (e.g. up/down by 2-3%) of the {company} stock price movement for next week. Provide a summary analysis to support your prediction."
)
- Result
Take a company's 10-k form, financial data, and market data as input and output an equity research report
- Import
import os
import autogen
from textwrap import dedent
from finrobot.utils import register_keys_from_json
from finrobot.agents.workflow import SingleAssistantShadow
- Config
llm_config = {
"config_list": autogen.config_list_from_json(
"../OAI_CONFIG_LIST",
filter_dict={
"model": ["gpt-4-0125-preview"],
},
),
"timeout": 120,
"temperature": 0.5,
}
register_keys_from_json("../config_api_keys")
# Intermediate strategy modules will be saved in this directory
work_dir = "../report"
os.makedirs(work_dir, exist_ok=True)
assistant = SingleAssistantShadow(
"Expert_Investor",
llm_config,
max_consecutive_auto_reply=None,
human_input_mode="TERMINATE",
)
- Run
company = "Microsoft"
fyear = "2023"
message = dedent(
f"""
With the tools you've been provided, write an annual report based on {company}'s {fyear} 10-k report, format it into a pdf.
Pay attention to the followings:
- Explicitly explain your working plan before you kick off.
- Use tools one by one for clarity, especially when asking for instructions.
- All your file operations should be done in "{work_dir}".
- Display any image in the chat once generated.
- All the paragraphs should combine between 400 and 450 words, don't generate the pdf until this is explicitly fulfilled.
"""
)
assistant.chat(message, use_cache=True, max_turns=50,
summary_method="last_msg")
- Result
Financial CoT:
- Gather Preliminary Data: 10-K report, market data, financial ratios
- Analyze Financial Statements: balance sheet, income statement, cash flow
- Company Overview and Performance: company description, business highlights, segment analysis
- Risk Assessment: assess risks
- Financial Performance Visualization: plot PE ratio and EPS
- Synthesize Findings into Paragraphs: combine all parts into a coherent summary
- Generate PDF Report: use tools to generate PDF automatically
- Quality Assurance: check word counts
- [Stanford University + Microsoft Research] Agent AI: Surveying the Horizons of Multimodal Interaction
- [Stanford University] Generative Agents: Interactive Simulacra of Human Behavior
- [Fudan NLP Group] The Rise and Potential of Large Language Model Based Agents: A Survey
- [Fudan NLP Group] LLM-Agent-Paper-List
- [Tsinghua University] Large Language Models Empowered Agent-based Modeling and Simulation: A Survey and Perspectives
- [Renmin University] A Survey on Large Language Model-based Autonomous Agents
- [Nanyang Technological University] FinAgent: A Multimodal Foundation Agent for Financial Trading: Tool-Augmented, Diversified, and Generalist
- [Medium] An Introduction to AI Agents
- [Medium] Unmasking the Best Character AI Chatbots | 2024
- [big-picture] ChatGPT, Next Level: Meet 10 Autonomous AI Agents
- [TowardsDataScience] Navigating the World of LLM Agents: A Beginnerβs Guide
- [YouTube] Introducing Devin - The "First" AI Agent Software Engineer
- AutoGPT (163k stars) is a tool for everyone to use, aiming to democratize AI, making it accessible for everyone to use and build upon.
- LangChain (87.4k stars) is a framework for developing context-aware applications powered by language models, enabling them to connect to sources of context and rely on the model's reasoning capabilities for responses and actions.
- MetaGPT (41k stars) is a multi-agent open-source framework that assigns different roles to GPTs, forming a collaborative software entity to execute complex tasks.
- dify (34.1.7k stars) is an LLM application development platform. It integrates the concepts of Backend as a Service and LLMOps, covering the core tech stack required for building generative AI-native applications, including a built-in RAG engine
- AutoGen (27.4k stars) is a framework for developing LLM applications with conversational agents that collaborate to solve tasks. These agents are customizable, support human interaction, and operate in modes combining LLMs, human inputs, and tools.
- ChatDev (24.1k stars) is a framework that focuses on developing conversational AI Agents capable of dialogue and question-answering. It provides a range of pre-trained models and interactive interfaces, facilitating the development of customized chat Agents for users.
- BabyAGI (19.5k stars) is an AI-powered task management system, dedicated to building AI Agents with preliminary general intelligence.
- CrewAI (16k stars) is a framework for orchestrating role-playing, autonomous AI agents. By fostering collaborative intelligence, CrewAI empowers agents to work together seamlessly, tackling complex tasks.
- SuperAGI (14.8k stars) is a dev-first open-source autonomous AI agent framework enabling developers to build, manage & run useful autonomous agents.
- FastGPT (14.6k stars) is a knowledge-based platform built on the LLM, offers out-of-the-box data processing and model invocation capabilities, allows for workflow orchestration through Flow visualization.
- XAgent (7.8k stars) is an open-source experimental Large Language Model (LLM) driven autonomous agent that can automatically solve various tasks.
- Bisheng (7.8k stars) is a leading open-source platform for developing LLM applications.
- Voyager (5.3k stars) An Open-Ended Embodied Agent with Large Language Models.
- CAMEL (4.7k stars) is a framework that offers a comprehensive set of tools and algorithms for building multimodal AI Agents, enabling them to handle various data forms such as text, images, and speech.
- Langfuse (4.3k stars) is a language fusion framework that can integrate the language abilities of multiple AI Agents, enabling them to simultaneously possess multilingual understanding and generation capabilities.
Disclaimer: The codes and documents provided herein are released under the Apache-2.0 license. They should not be construed as financial counsel or recommendations for live trading. It is imperative to exercise caution and consult with qualified financial professionals prior to any trading or investment actions.
For Tasks:
Click tags to check more tools for each tasksFor Jobs:
Alternative AI tools for FinRobot
Similar Open Source Tools
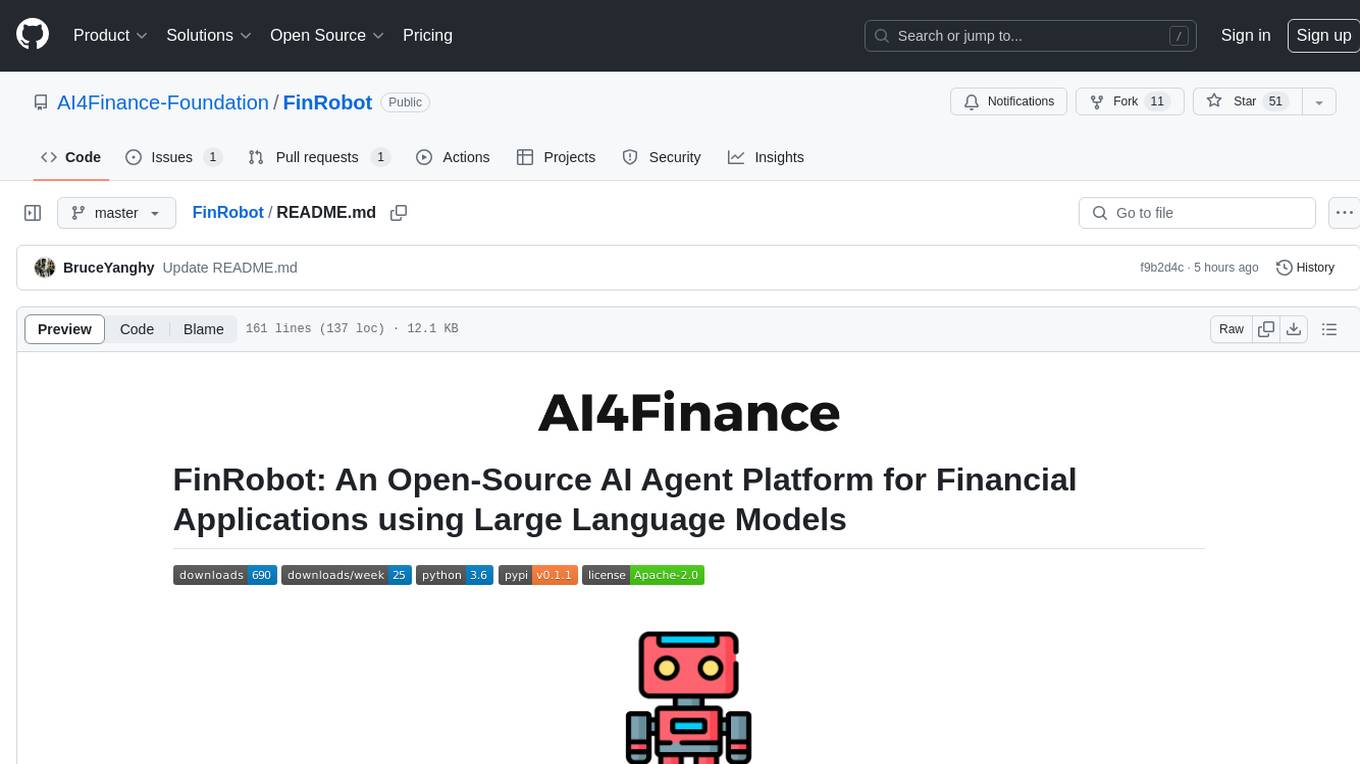
FinRobot
FinRobot is an open-source AI agent platform designed for financial applications using large language models. It transcends the scope of FinGPT, offering a comprehensive solution that integrates a diverse array of AI technologies. The platform's versatility and adaptability cater to the multifaceted needs of the financial industry. FinRobot's ecosystem is organized into four layers, including Financial AI Agents Layer, Financial LLMs Algorithms Layer, LLMOps and DataOps Layers, and Multi-source LLM Foundation Models Layer. The platform's agent workflow involves Perception, Brain, and Action modules to capture, process, and execute financial data and insights. The Smart Scheduler optimizes model diversity and selection for tasks, managed by components like Director Agent, Agent Registration, Agent Adaptor, and Task Manager. The tool provides a structured file organization with subfolders for agents, data sources, and functional modules, along with installation instructions and hands-on tutorials.
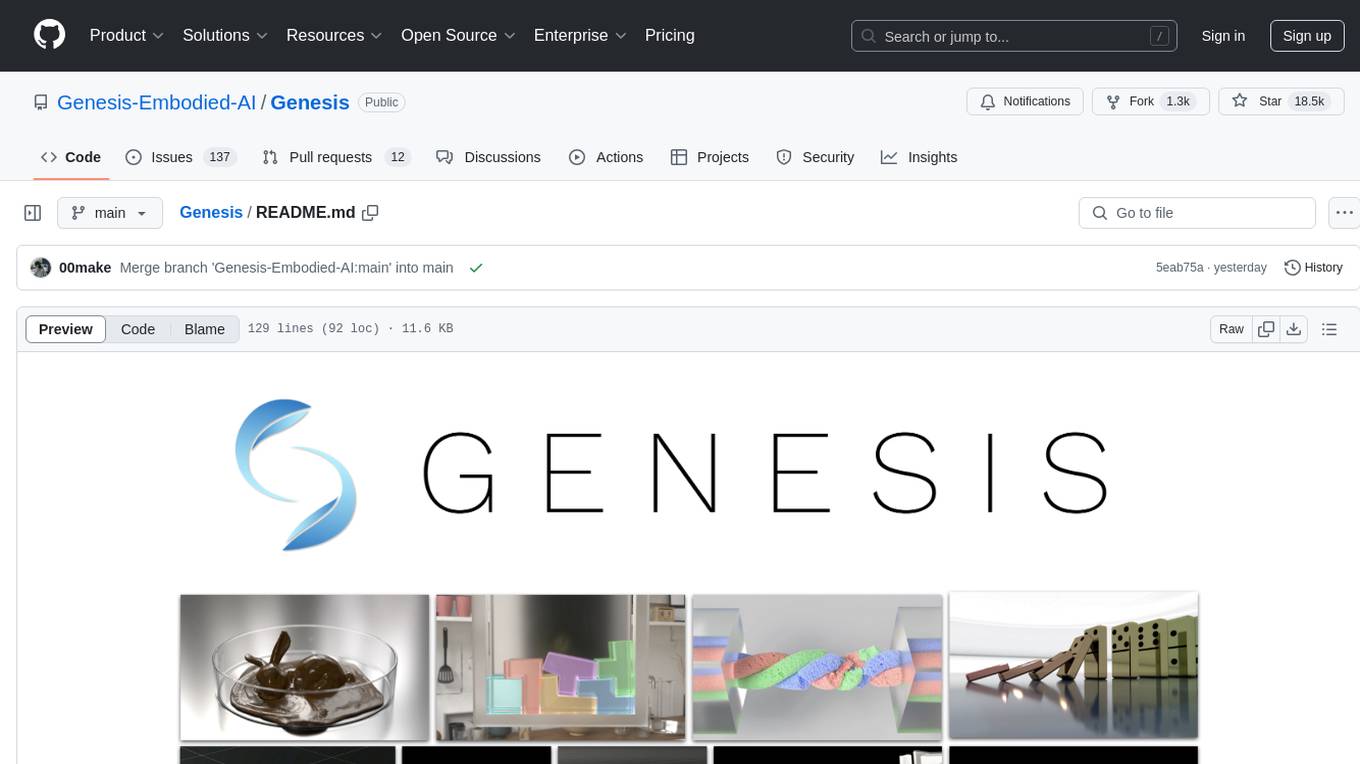
Genesis
Genesis is a physics platform designed for general purpose Robotics/Embodied AI/Physical AI applications. It includes a universal physics engine, a lightweight, ultra-fast, pythonic, and user-friendly robotics simulation platform, a powerful and fast photo-realistic rendering system, and a generative data engine that transforms user-prompted natural language description into various modalities of data. It aims to lower the barrier to using physics simulations, unify state-of-the-art physics solvers, and minimize human effort in collecting and generating data for robotics and other domains.
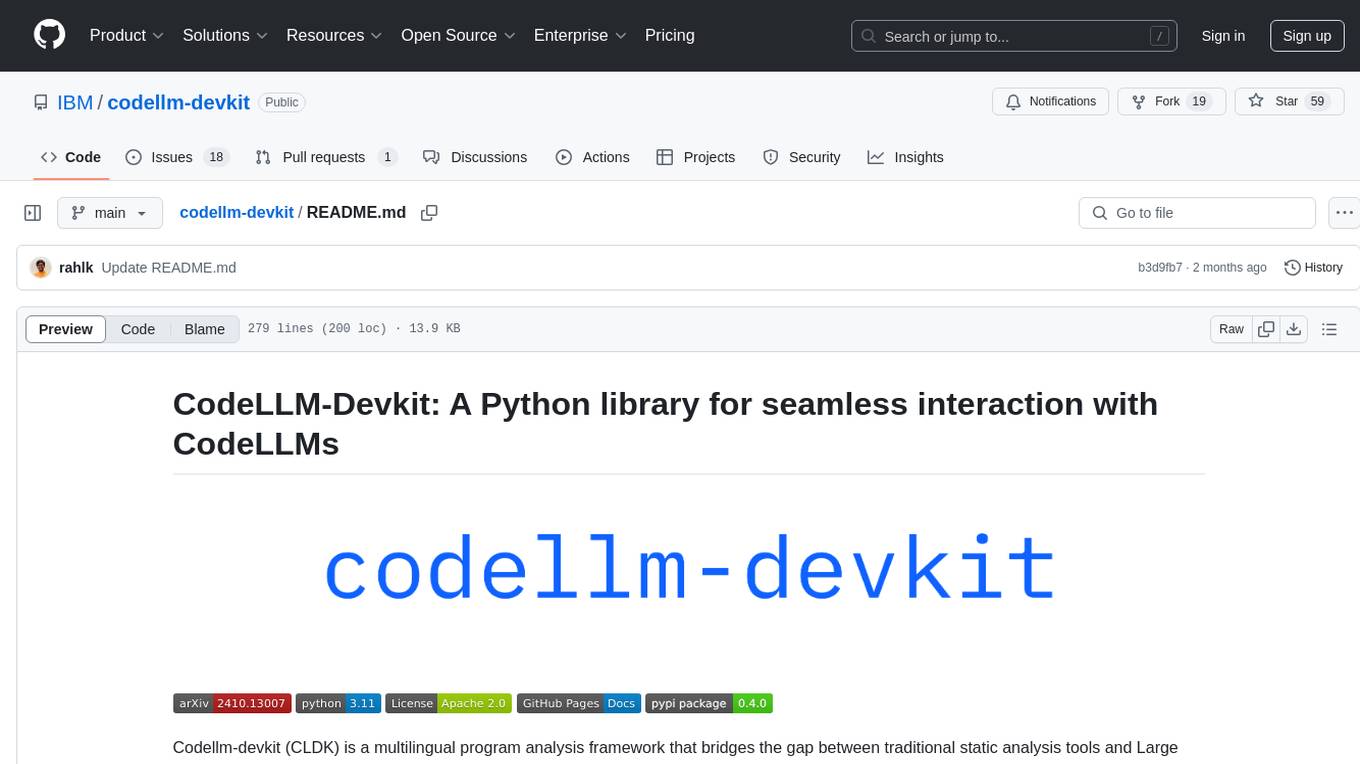
codellm-devkit
Codellm-devkit (CLDK) is a Python library that serves as a multilingual program analysis framework bridging traditional static analysis tools and Large Language Models (LLMs) specialized for code (CodeLLMs). It simplifies the process of analyzing codebases across multiple programming languages, enabling the extraction of meaningful insights and facilitating LLM-based code analysis. The library provides a unified interface for integrating outputs from various analysis tools and preparing them for effective use by CodeLLMs. Codellm-devkit aims to enable the development and experimentation of robust analysis pipelines that combine traditional program analysis tools and CodeLLMs, reducing friction in multi-language code analysis and ensuring compatibility across different tools and LLM platforms. It is designed to seamlessly integrate with popular analysis tools like WALA, Tree-sitter, LLVM, and CodeQL, acting as a crucial intermediary layer for efficient communication between these tools and CodeLLMs. The project is continuously evolving to include new tools and frameworks, maintaining its versatility for code analysis and LLM integration.
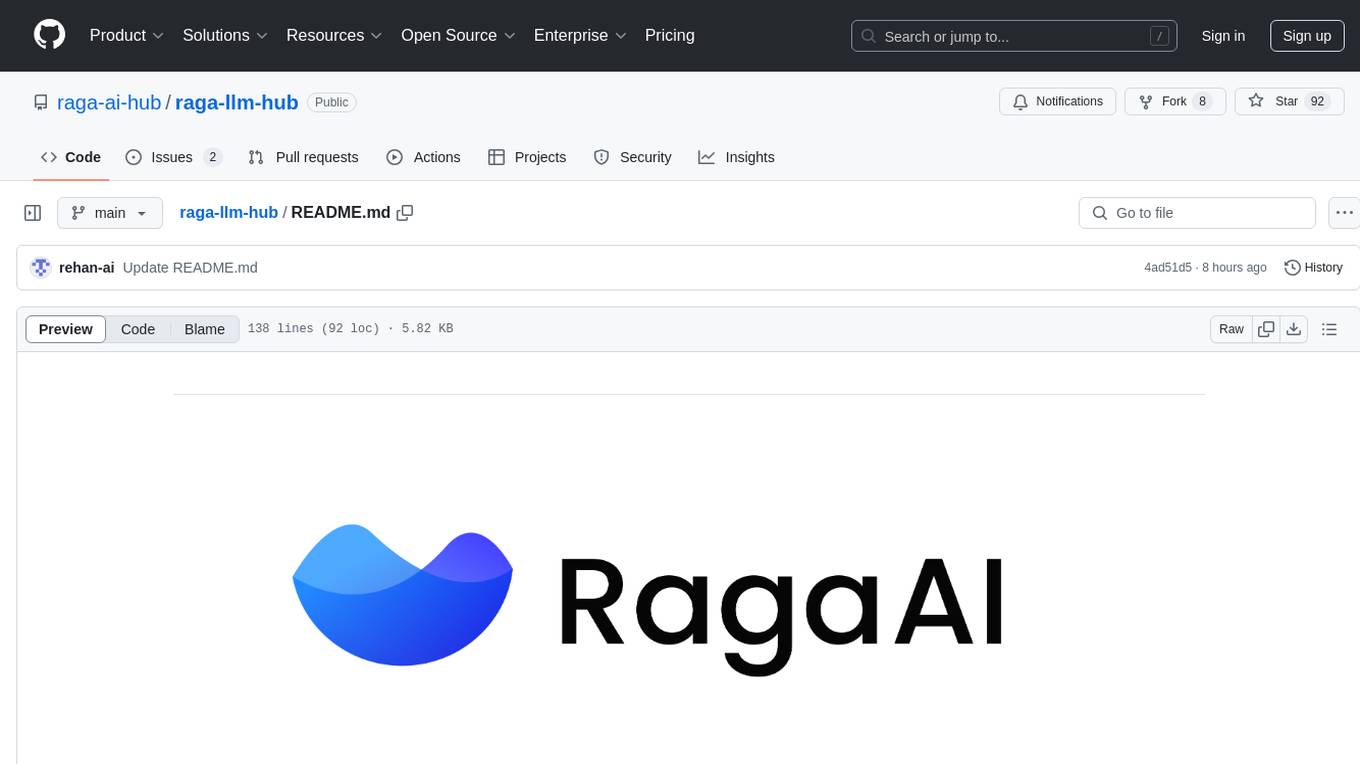
raga-llm-hub
Raga LLM Hub is a comprehensive evaluation toolkit for Language and Learning Models (LLMs) with over 100 meticulously designed metrics. It allows developers and organizations to evaluate and compare LLMs effectively, establishing guardrails for LLMs and Retrieval Augmented Generation (RAG) applications. The platform assesses aspects like Relevance & Understanding, Content Quality, Hallucination, Safety & Bias, Context Relevance, Guardrails, and Vulnerability scanning, along with Metric-Based Tests for quantitative analysis. It helps teams identify and fix issues throughout the LLM lifecycle, revolutionizing reliability and trustworthiness.
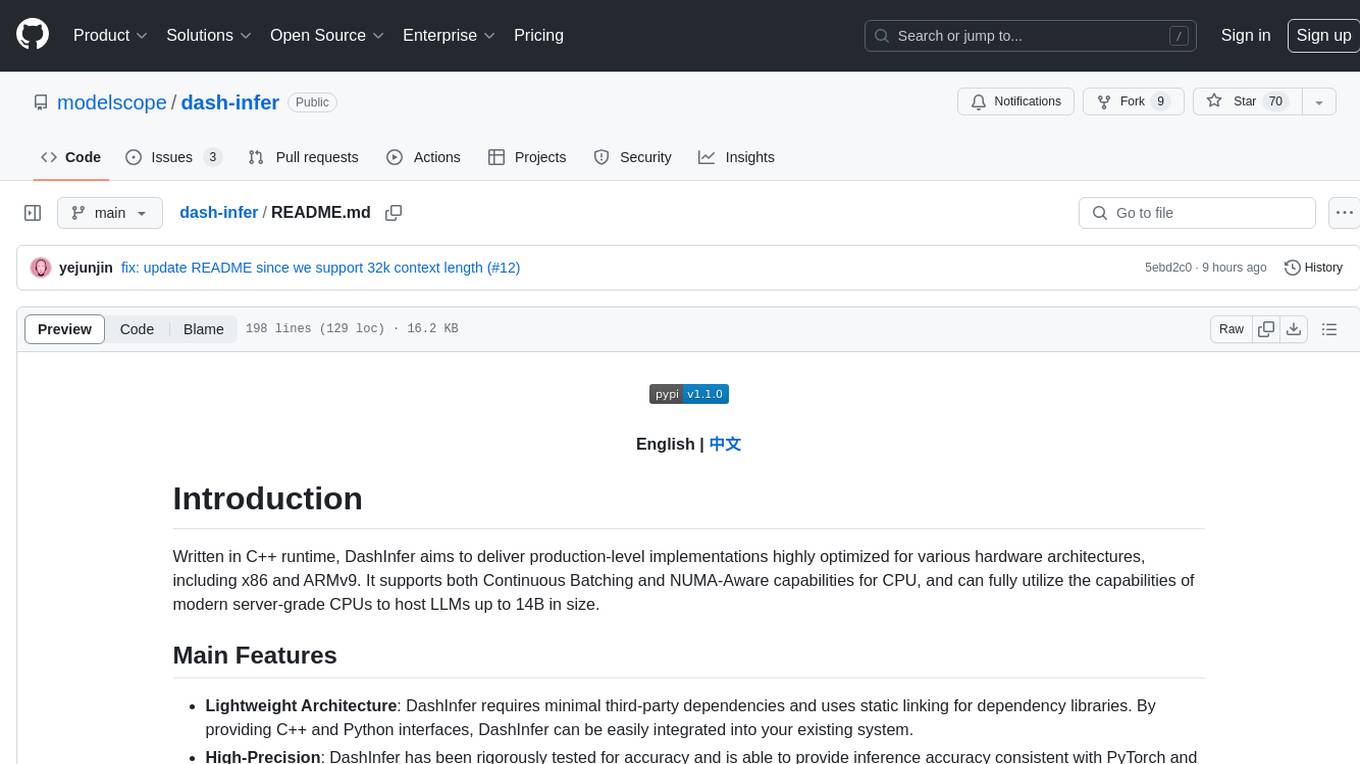
dash-infer
DashInfer is a C++ runtime tool designed to deliver production-level implementations highly optimized for various hardware architectures, including x86 and ARMv9. It supports Continuous Batching and NUMA-Aware capabilities for CPU, and can fully utilize modern server-grade CPUs to host large language models (LLMs) up to 14B in size. With lightweight architecture, high precision, support for mainstream open-source LLMs, post-training quantization, optimized computation kernels, NUMA-aware design, and multi-language API interfaces, DashInfer provides a versatile solution for efficient inference tasks. It supports x86 CPUs with AVX2 instruction set and ARMv9 CPUs with SVE instruction set, along with various data types like FP32, BF16, and InstantQuant. DashInfer also offers single-NUMA and multi-NUMA architectures for model inference, with detailed performance tests and inference accuracy evaluations available. The tool is supported on mainstream Linux server operating systems and provides documentation and examples for easy integration and usage.
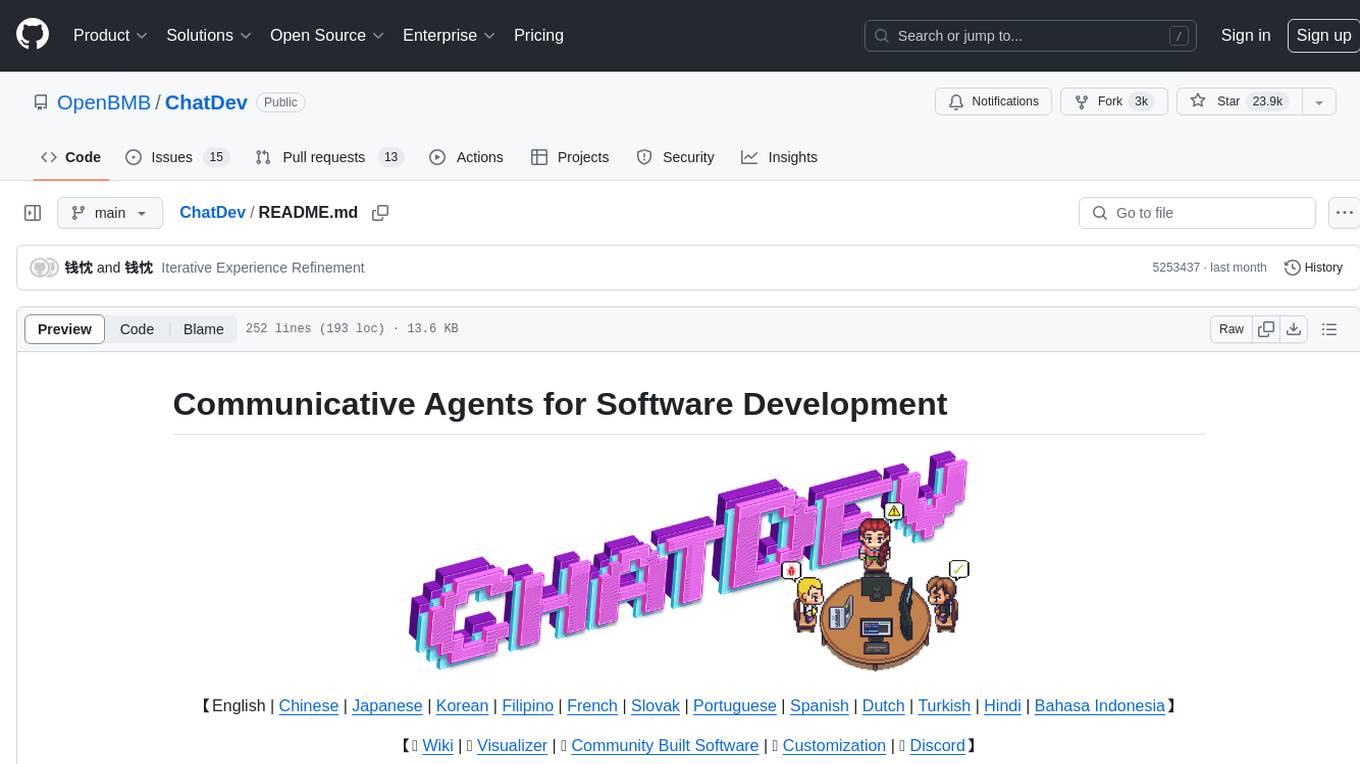
ChatDev
ChatDev is a virtual software company powered by intelligent agents like CEO, CPO, CTO, programmer, reviewer, tester, and art designer. These agents collaborate to revolutionize the digital world through programming. The platform offers an easy-to-use, highly customizable, and extendable framework based on large language models, ideal for studying collective intelligence. ChatDev introduces innovative methods like Iterative Experience Refinement and Experiential Co-Learning to enhance software development efficiency. It supports features like incremental development, Docker integration, Git mode, and Human-Agent-Interaction mode. Users can customize ChatChain, Phase, and Role settings, and share their software creations easily. The project is open-source under the Apache 2.0 License and utilizes data licensed under CC BY-NC 4.0.
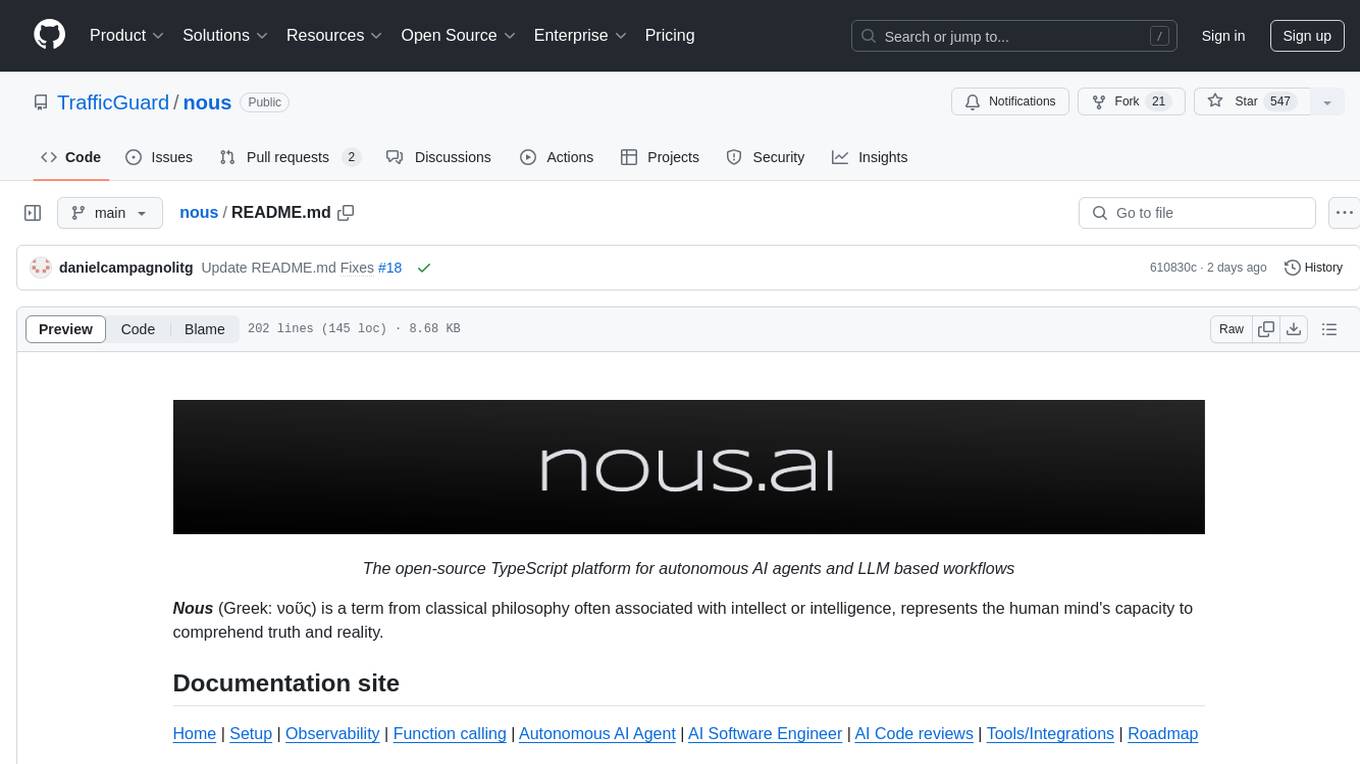
nous
Nous is an open-source TypeScript platform for autonomous AI agents and LLM based workflows. It aims to automate processes, support requests, review code, assist with refactorings, and more. The platform supports various integrations, multiple LLMs/services, CLI and web interface, human-in-the-loop interactions, flexible deployment options, observability with OpenTelemetry tracing, and specific agents for code editing, software engineering, and code review. It offers advanced features like reasoning/planning, memory and function call history, hierarchical task decomposition, and control-loop function calling options. Nous is designed to be a flexible platform for the TypeScript community to expand and support different use cases and integrations.
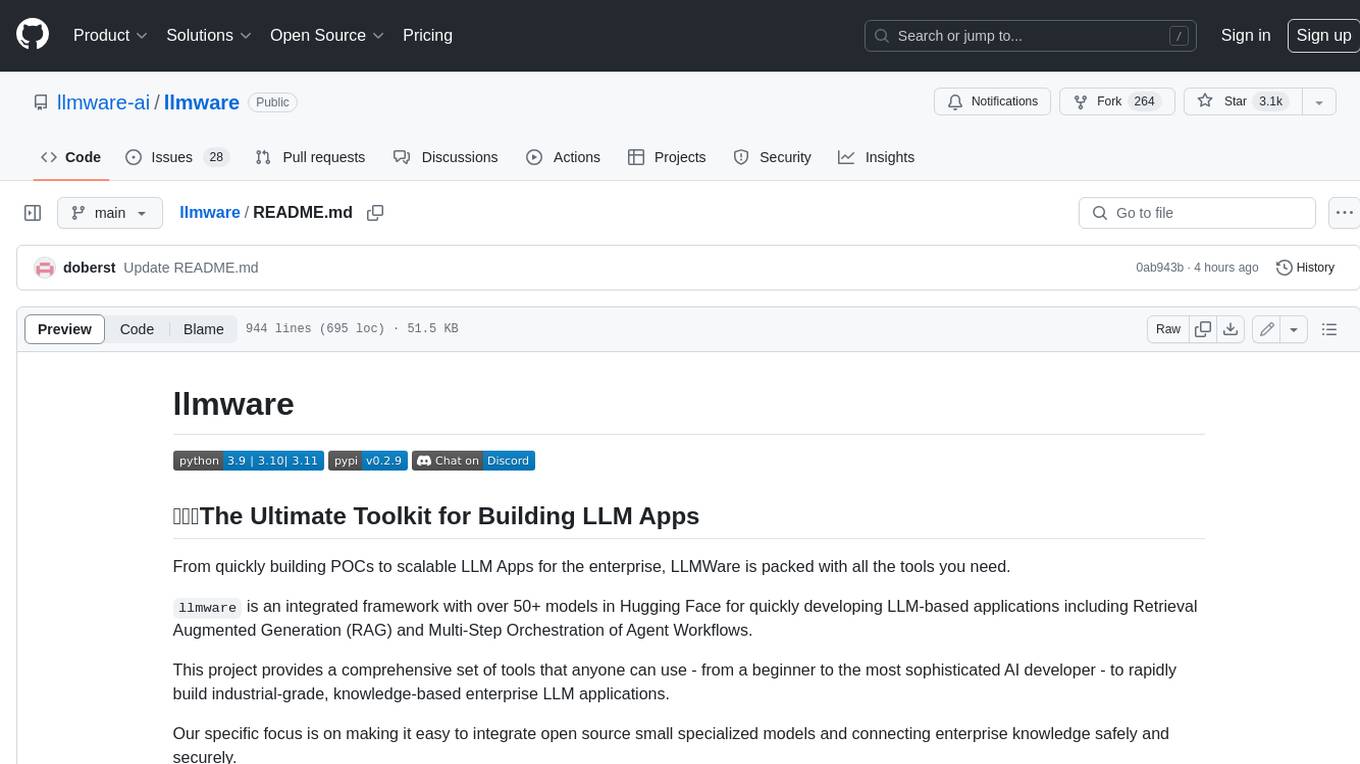
llmware
LLMWare is a framework for quickly developing LLM-based applications including Retrieval Augmented Generation (RAG) and Multi-Step Orchestration of Agent Workflows. This project provides a comprehensive set of tools that anyone can use - from a beginner to the most sophisticated AI developer - to rapidly build industrial-grade, knowledge-based enterprise LLM applications. Our specific focus is on making it easy to integrate open source small specialized models and connecting enterprise knowledge safely and securely.
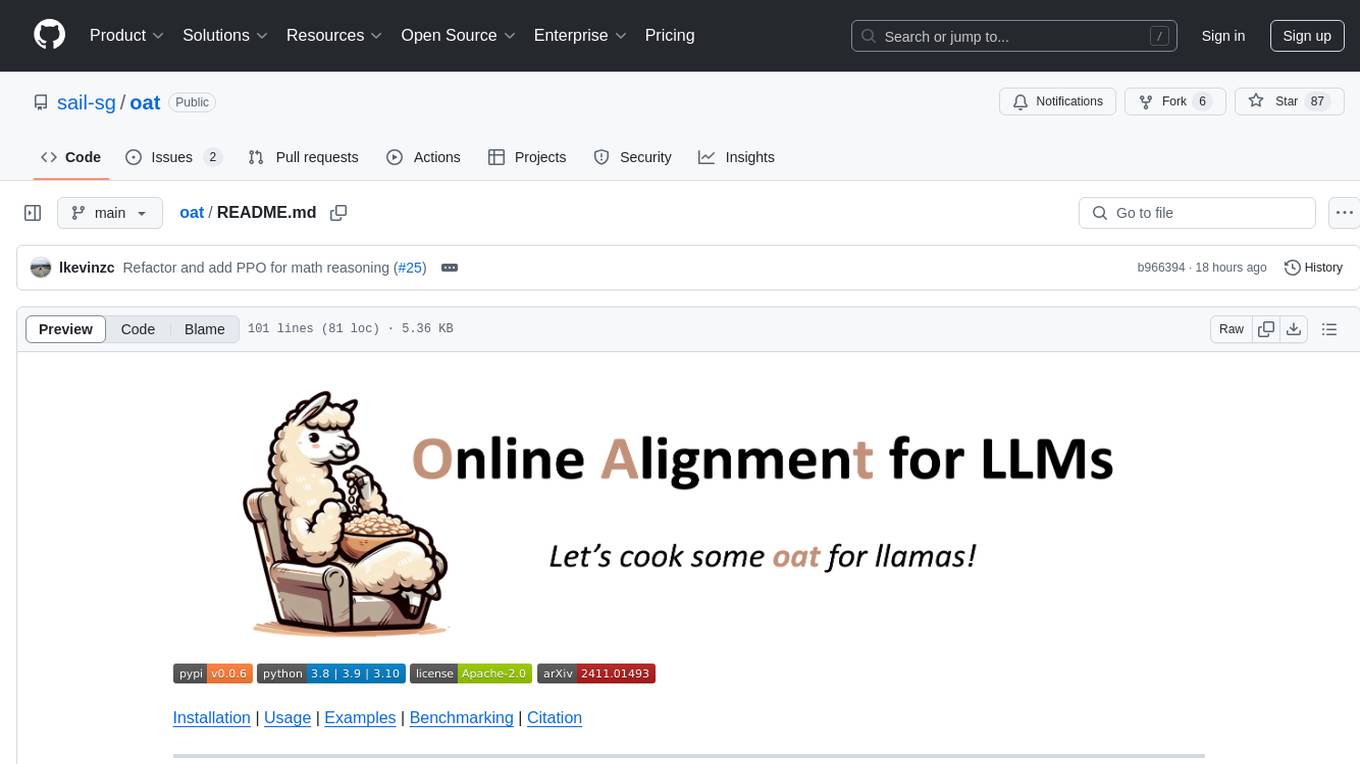
oat
Oat is a simple and efficient framework for running online LLM alignment algorithms. It implements a distributed Actor-Learner-Oracle architecture, with components optimized using state-of-the-art tools. Oat simplifies the experimental pipeline of LLM alignment by serving an Oracle online for preference data labeling and model evaluation. It provides a variety of oracles for simulating feedback and supports verifiable rewards. Oat's modular structure allows for easy inheritance and modification of classes, enabling rapid prototyping and experimentation with new algorithms. The framework implements cutting-edge online algorithms like PPO for math reasoning and various online exploration algorithms.
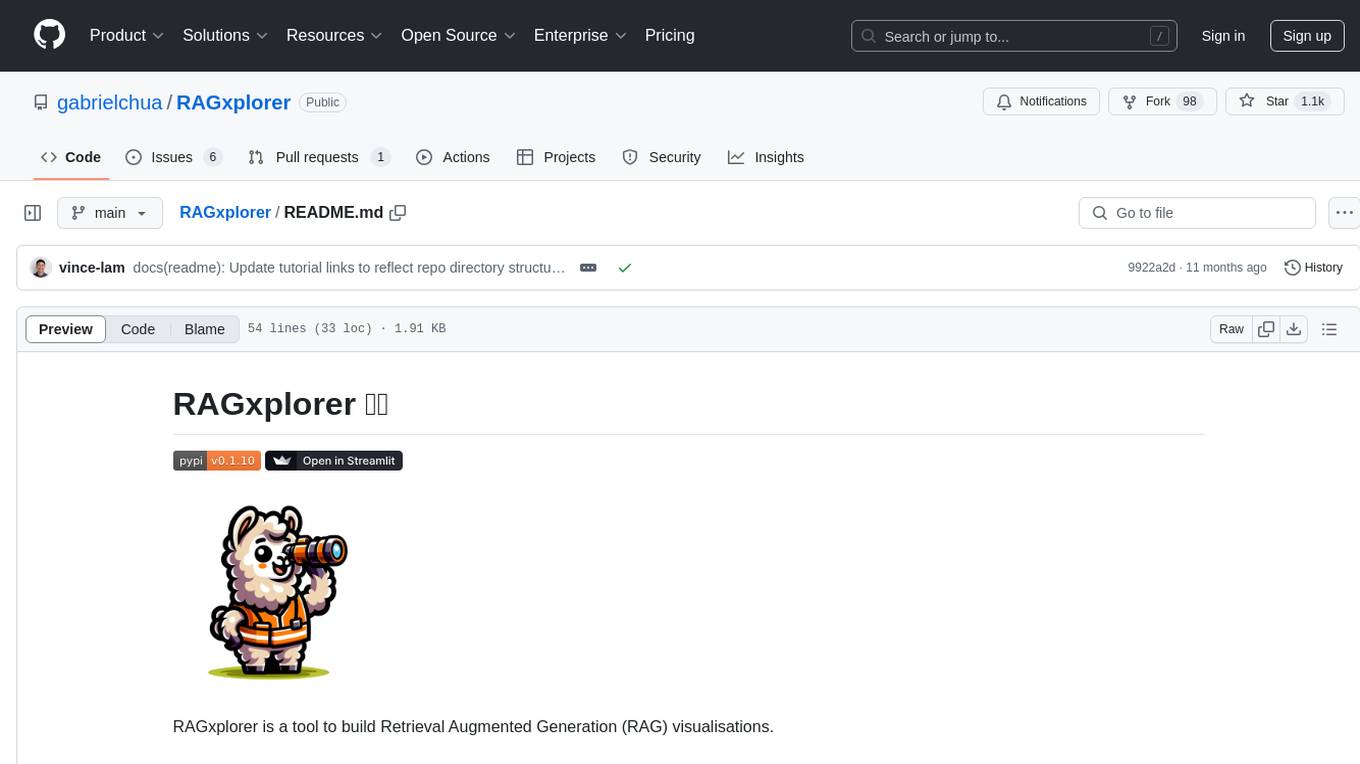
RAGxplorer
RAGxplorer is a tool designed to build visualisations for Retrieval Augmented Generation (RAG). It provides functionalities to interact with RAG models, visualize queries, and explore information retrieval tasks. The tool aims to simplify the process of working with RAG models and enhance the understanding of retrieval and generation processes.
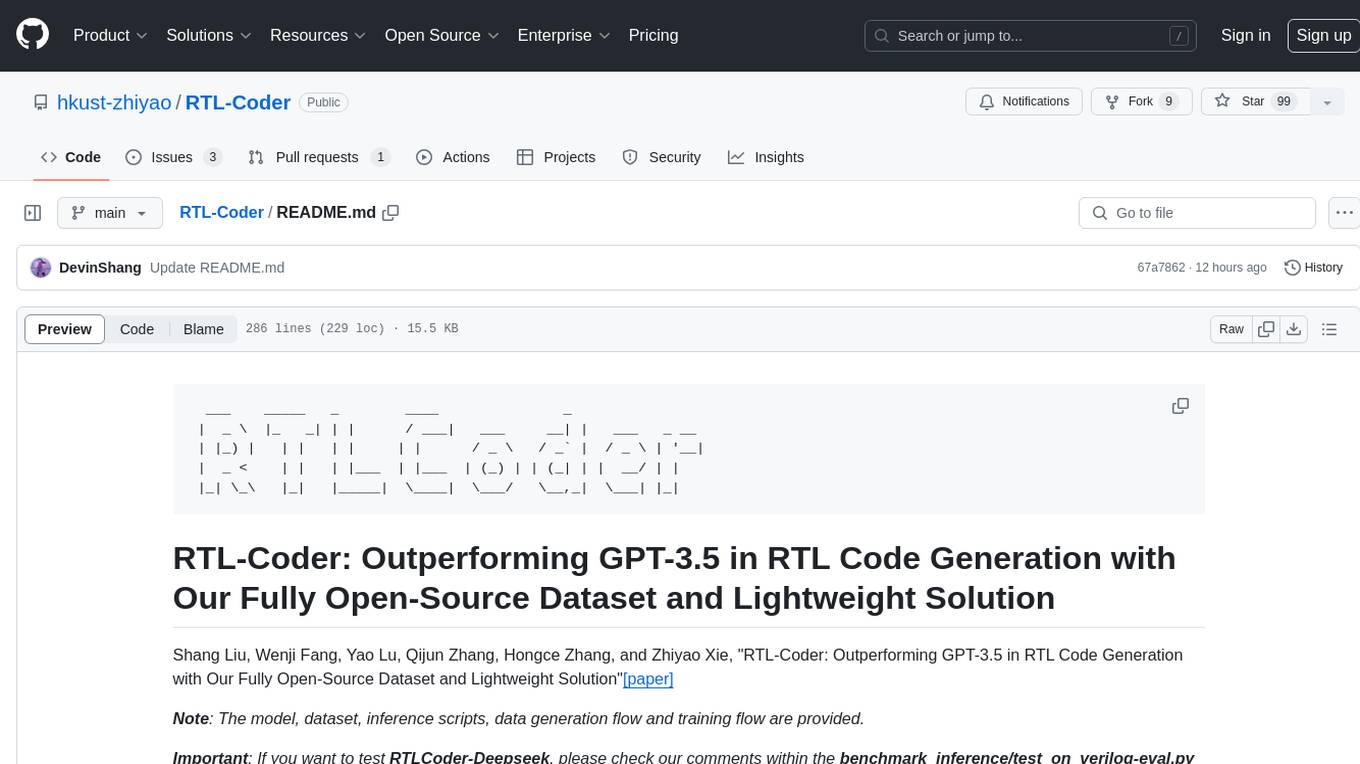
RTL-Coder
RTL-Coder is a tool designed to outperform GPT-3.5 in RTL code generation by providing a fully open-source dataset and a lightweight solution. It targets Verilog code generation and offers an automated flow to generate a large labeled dataset with over 27,000 diverse Verilog design problems and answers. The tool addresses the data availability challenge in IC design-related tasks and can be used for various applications beyond LLMs. The tool includes four RTL code generation models available on the HuggingFace platform, each with specific features and performance characteristics. Additionally, RTL-Coder introduces a new LLM training scheme based on code quality feedback to further enhance model performance and reduce GPU memory consumption.
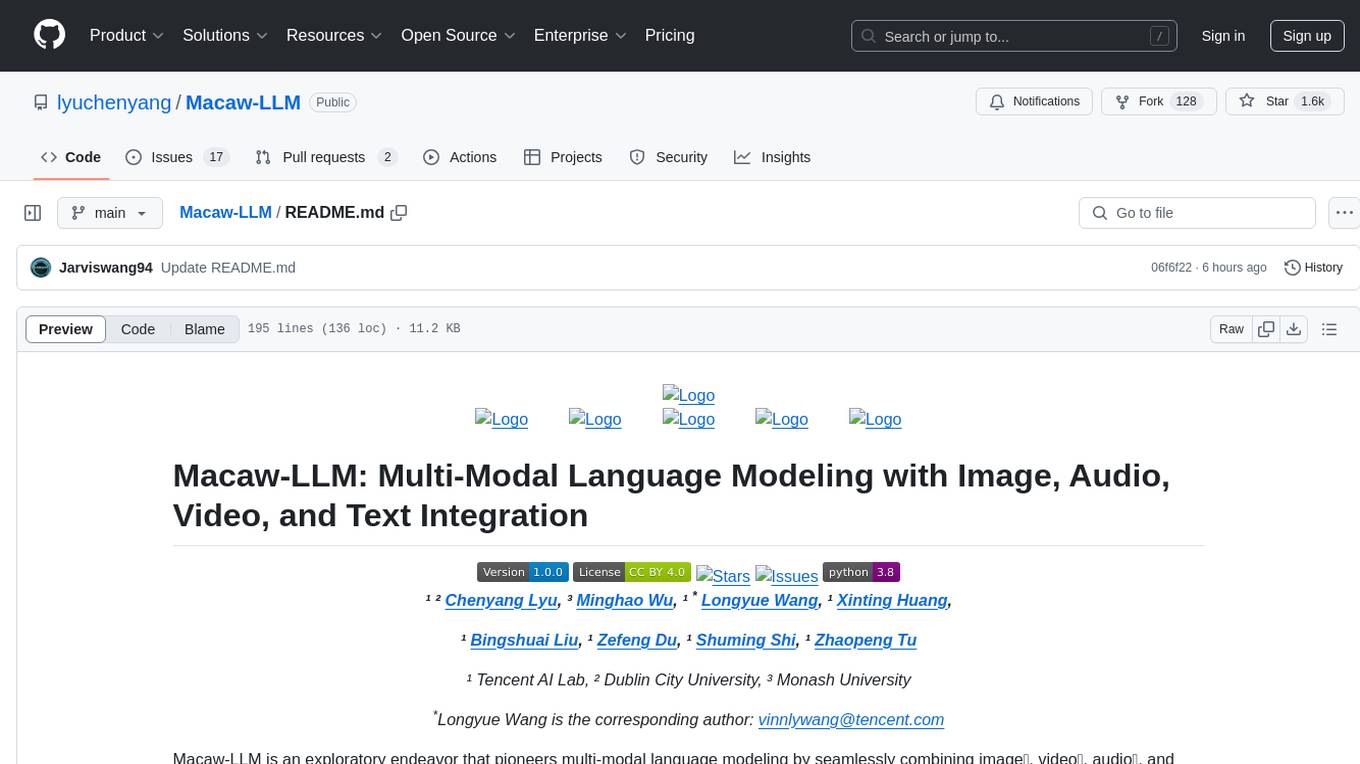
Macaw-LLM
Macaw-LLM is a pioneering multi-modal language modeling tool that seamlessly integrates image, audio, video, and text data. It builds upon CLIP, Whisper, and LLaMA models to process and analyze multi-modal information effectively. The tool boasts features like simple and fast alignment, one-stage instruction fine-tuning, and a new multi-modal instruction dataset. It enables users to align multi-modal features efficiently, encode instructions, and generate responses across different data types.
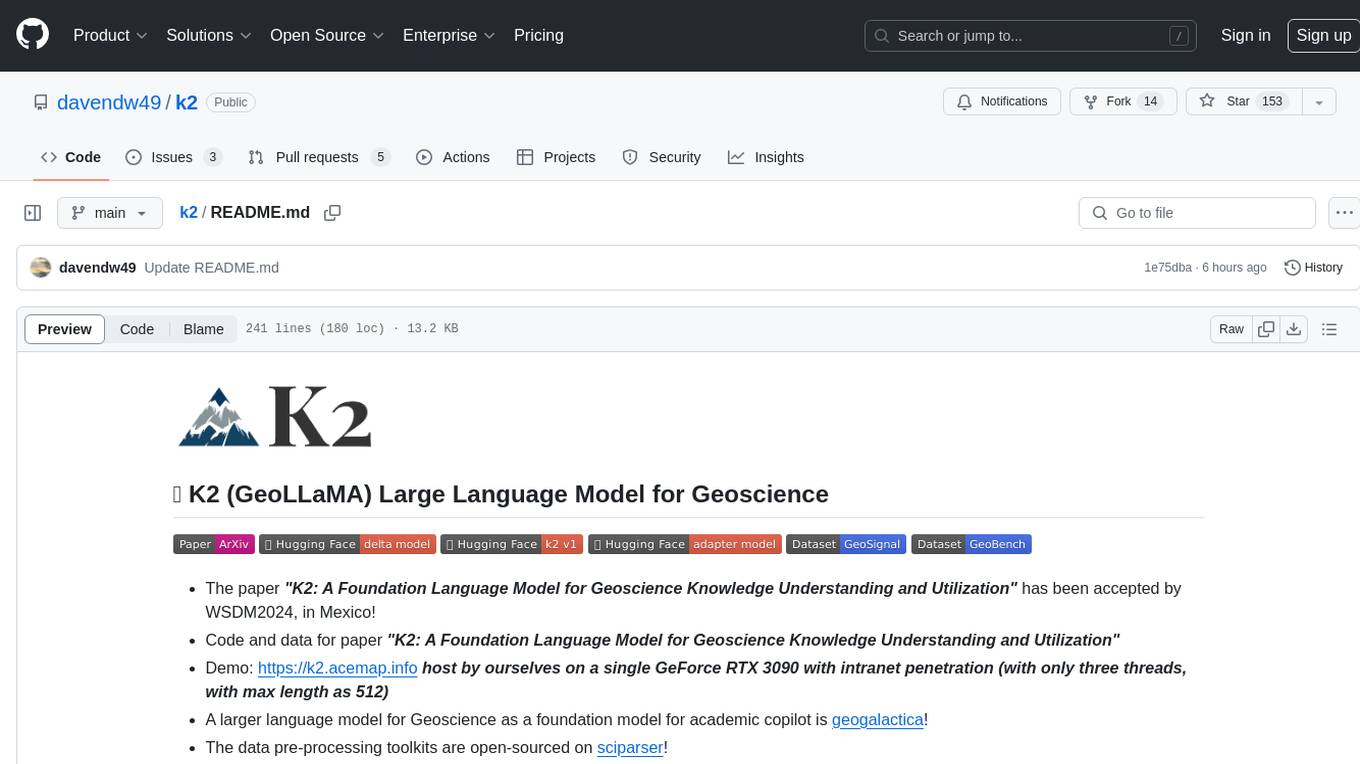
k2
K2 (GeoLLaMA) is a large language model for geoscience, trained on geoscience literature and fine-tuned with knowledge-intensive instruction data. It outperforms baseline models on objective and subjective tasks. The repository provides K2 weights, core data of GeoSignal, GeoBench benchmark, and code for further pretraining and instruction tuning. The model is available on Hugging Face for use. The project aims to create larger and more powerful geoscience language models in the future.
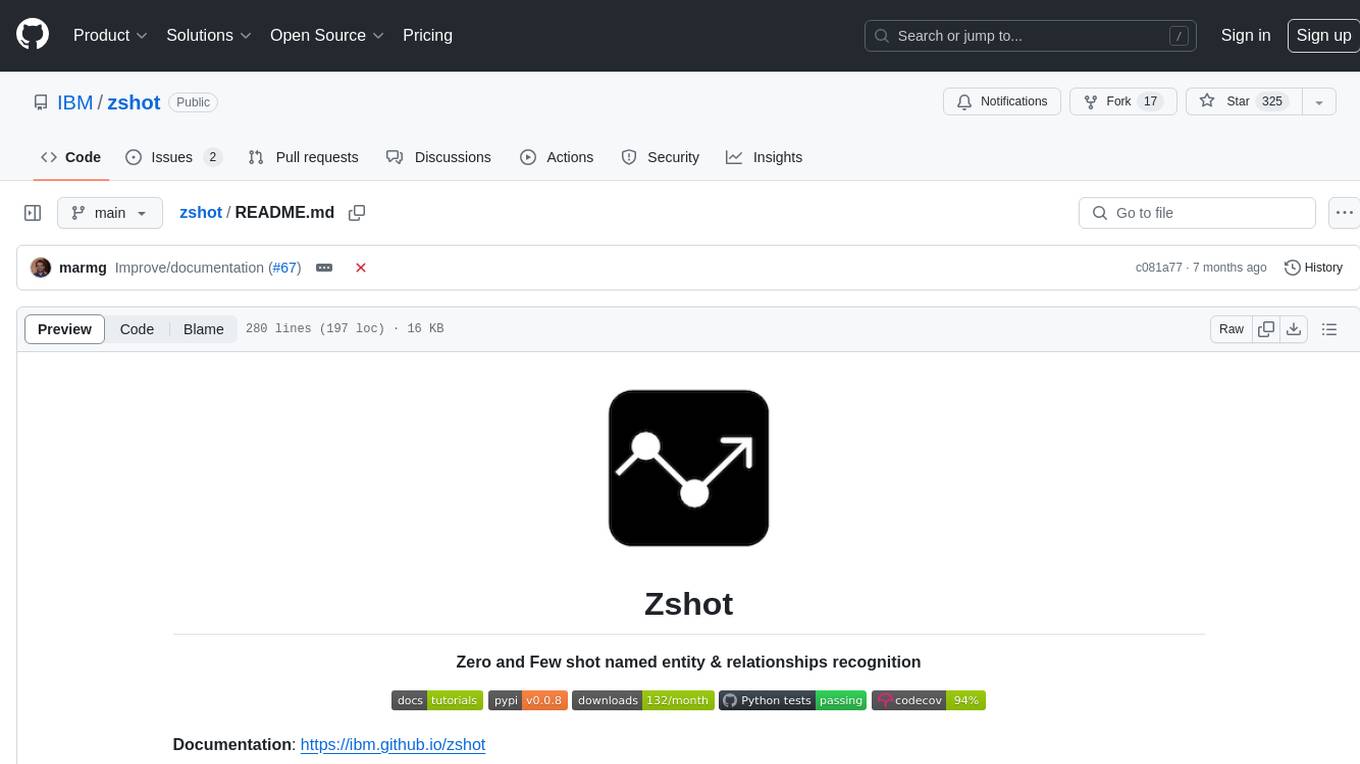
zshot
Zshot is a highly customizable framework for performing Zero and Few shot named entity and relationships recognition. It can be used for mentions extraction, wikification, zero and few shot named entity recognition, zero and few shot named relationship recognition, and visualization of zero-shot NER and RE extraction. The framework consists of two main components: the mentions extractor and the linker. There are multiple mentions extractors and linkers available, each serving a specific purpose. Zshot also includes a relations extractor and a knowledge extractor for extracting relations among entities and performing entity classification. The tool requires Python 3.6+ and dependencies like spacy, torch, transformers, evaluate, and datasets for evaluation over datasets like OntoNotes. Optional dependencies include flair and blink for additional functionalities. Zshot provides examples, tutorials, and evaluation methods to assess the performance of the components.
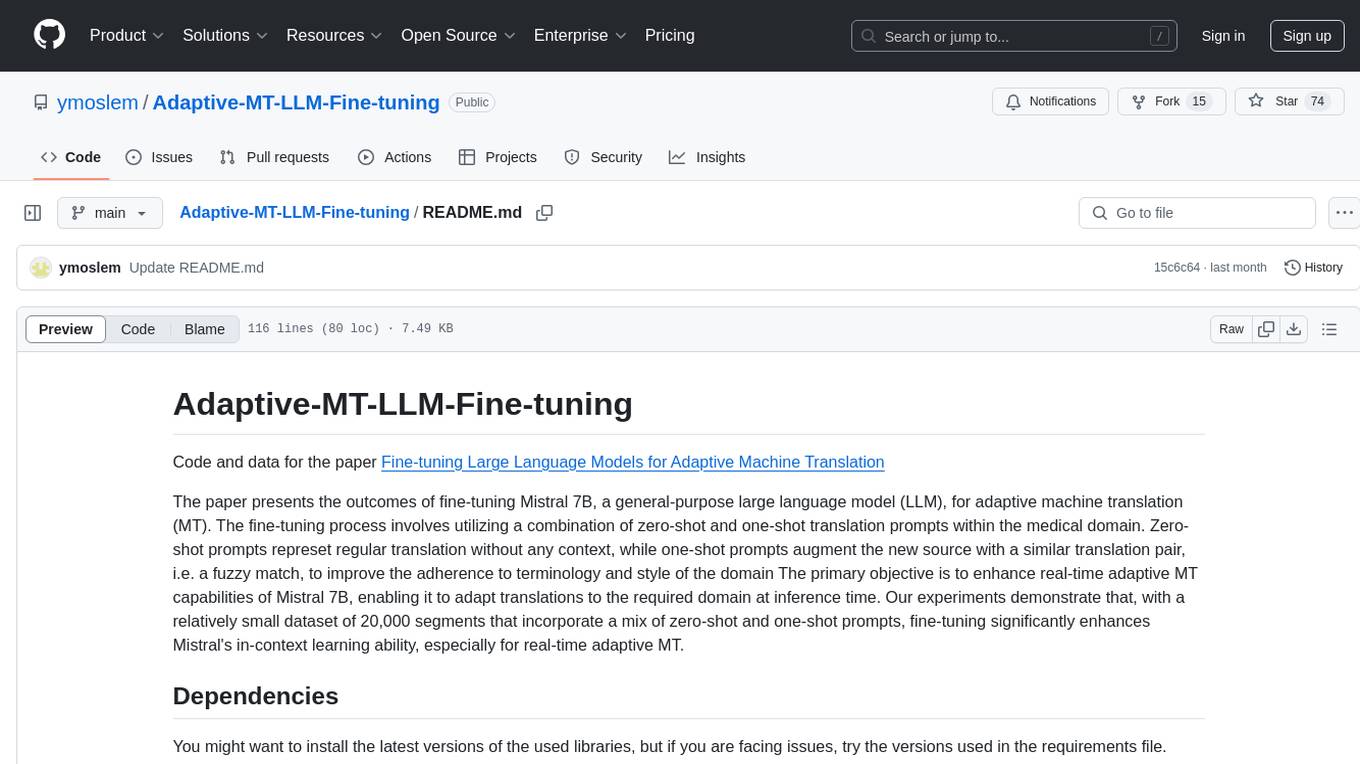
Adaptive-MT-LLM-Fine-tuning
The repository Adaptive-MT-LLM-Fine-tuning contains code and data for the paper 'Fine-tuning Large Language Models for Adaptive Machine Translation'. It focuses on enhancing Mistral 7B, a large language model, for real-time adaptive machine translation in the medical domain. The fine-tuning process involves using zero-shot and one-shot translation prompts to improve terminology and style adherence. The repository includes training and test data, data processing code, fuzzy match retrieval techniques, fine-tuning methods, conversion to CTranslate2 format, tokenizers, translation codes, and evaluation metrics.
For similar tasks
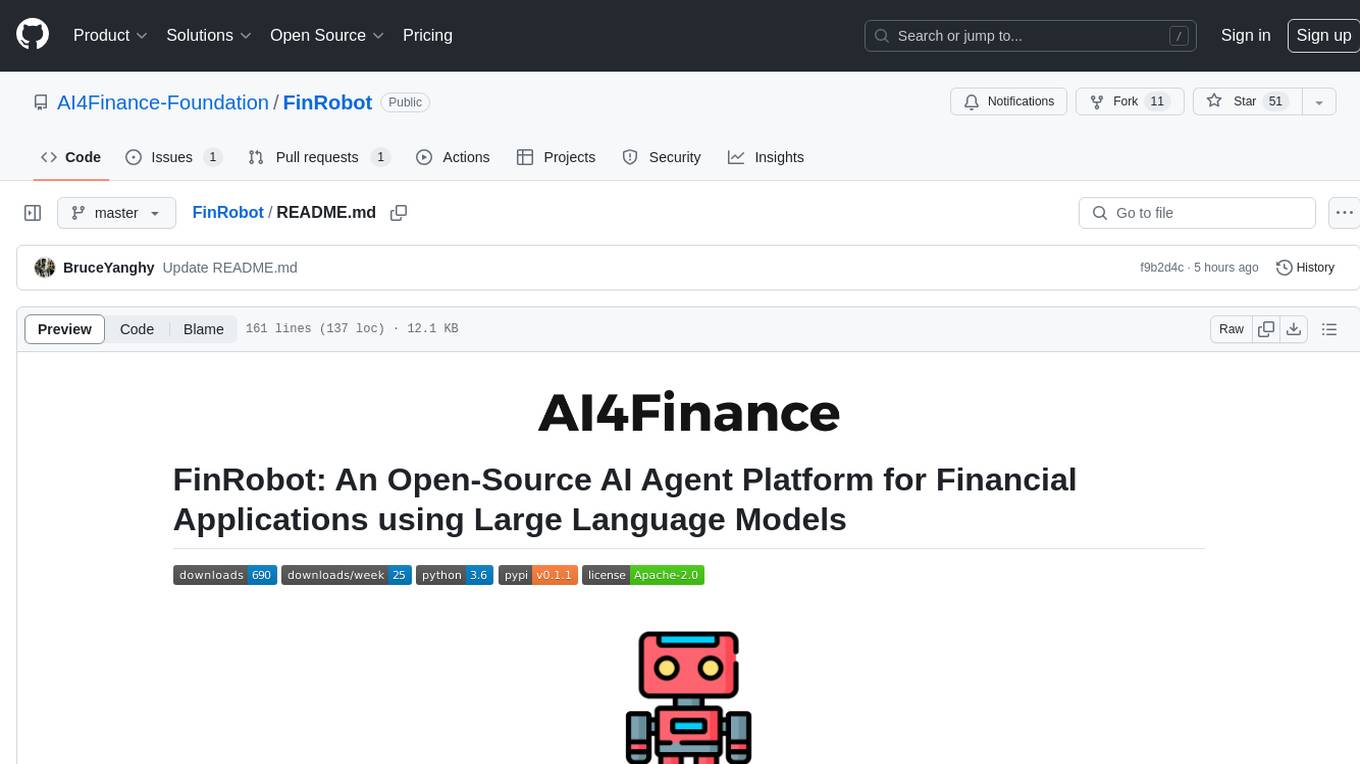
FinRobot
FinRobot is an open-source AI agent platform designed for financial applications using large language models. It transcends the scope of FinGPT, offering a comprehensive solution that integrates a diverse array of AI technologies. The platform's versatility and adaptability cater to the multifaceted needs of the financial industry. FinRobot's ecosystem is organized into four layers, including Financial AI Agents Layer, Financial LLMs Algorithms Layer, LLMOps and DataOps Layers, and Multi-source LLM Foundation Models Layer. The platform's agent workflow involves Perception, Brain, and Action modules to capture, process, and execute financial data and insights. The Smart Scheduler optimizes model diversity and selection for tasks, managed by components like Director Agent, Agent Registration, Agent Adaptor, and Task Manager. The tool provides a structured file organization with subfolders for agents, data sources, and functional modules, along with installation instructions and hands-on tutorials.
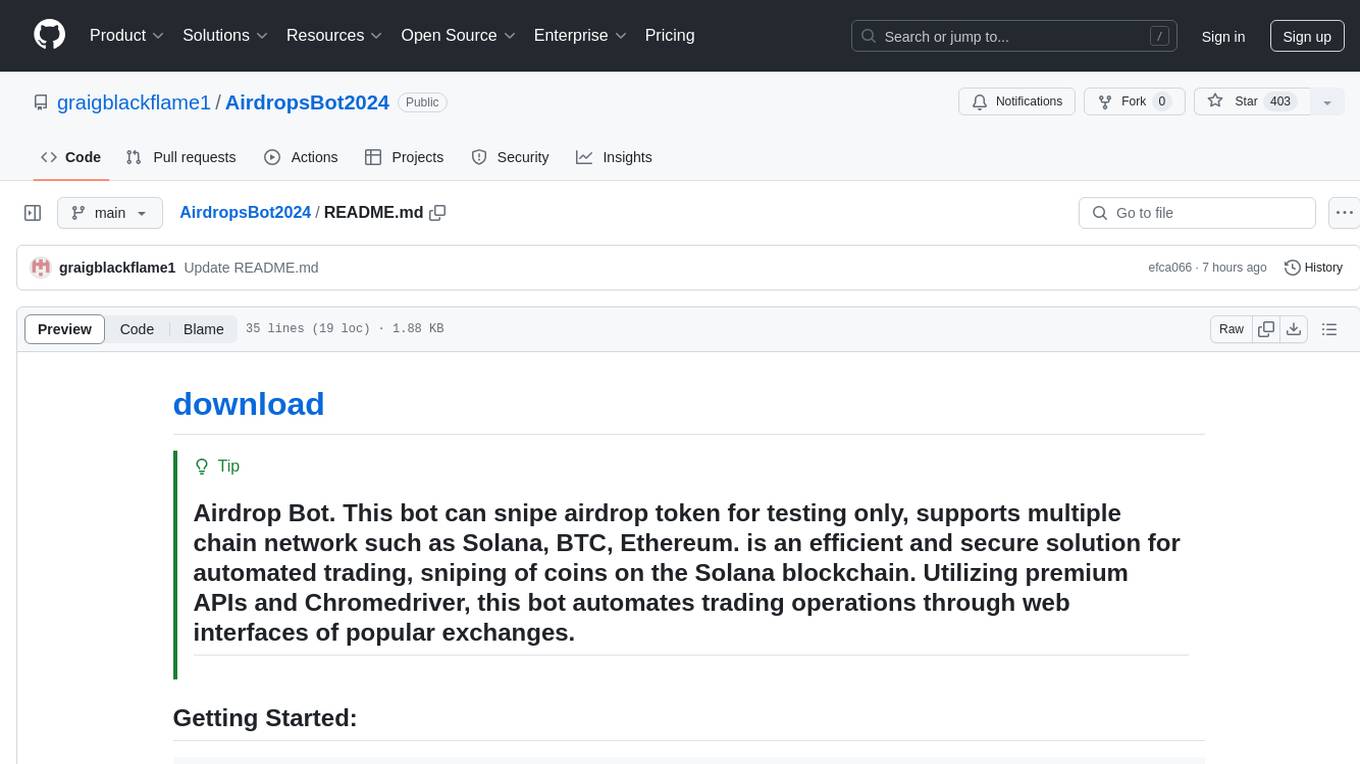
AirdropsBot2024
AirdropsBot2024 is an efficient and secure solution for automated trading and sniping of coins on the Solana blockchain. It supports multiple chain networks such as Solana, BTC, and Ethereum. The bot utilizes premium APIs and Chromedriver to automate trading operations through web interfaces of popular exchanges. It offers high-speed data analysis, in-depth market analysis, support for major exchanges, complete security and control, data visualization, advanced notification options, flexibility and adaptability in trading strategies, and profile management.
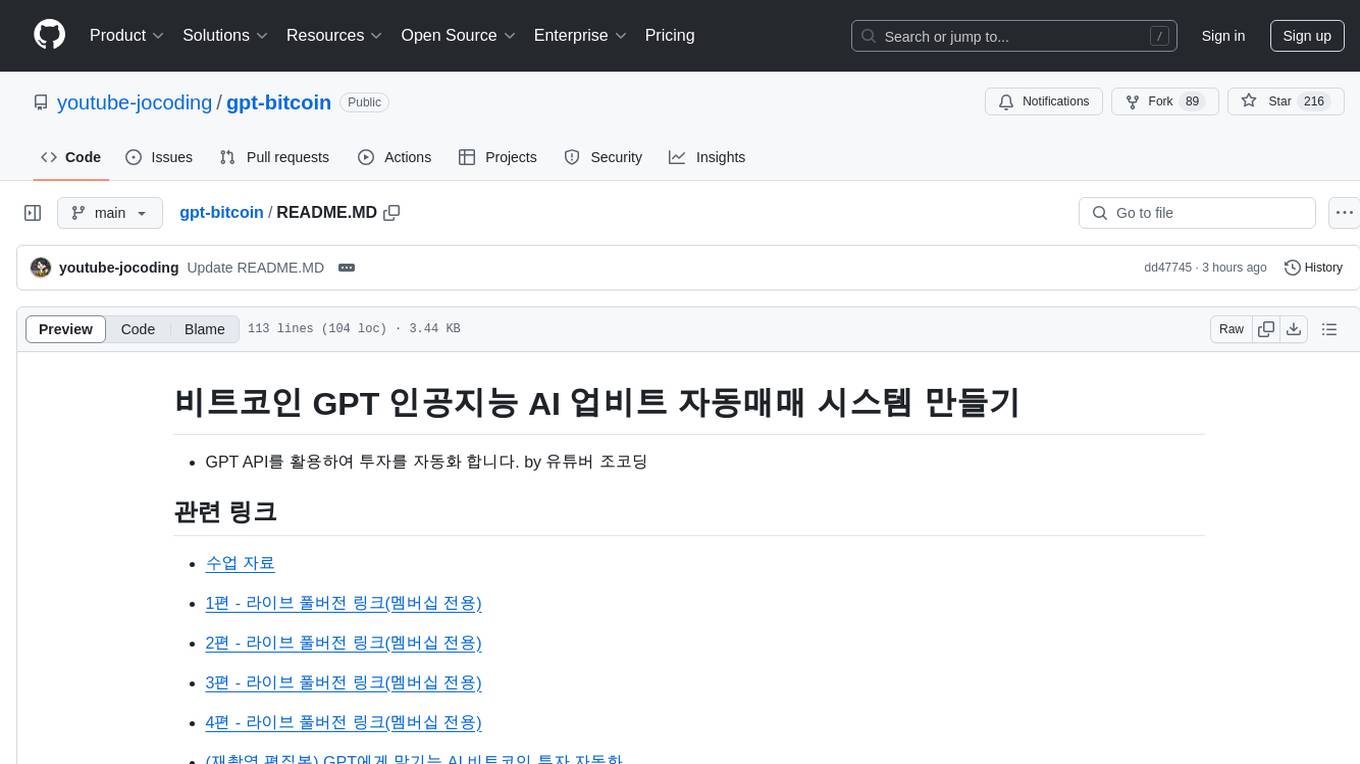
gpt-bitcoin
The gpt-bitcoin repository is focused on creating an automated trading system for Bitcoin using GPT AI technology. It provides different versions of trading strategies utilizing various data sources such as OHLCV, Moving Averages, RSI, Stochastic Oscillator, MACD, Bollinger Bands, Orderbook Data, news data, fear/greed index, and chart images. Users can set up the system by creating a .env file with necessary API keys and installing required dependencies. The repository also includes instructions for setting up the environment on local machines and AWS EC2 Ubuntu servers. The future plan includes expanding the system to support other cryptocurrency exchanges like Bithumb, Binance, Coinbase, OKX, and Bybit.
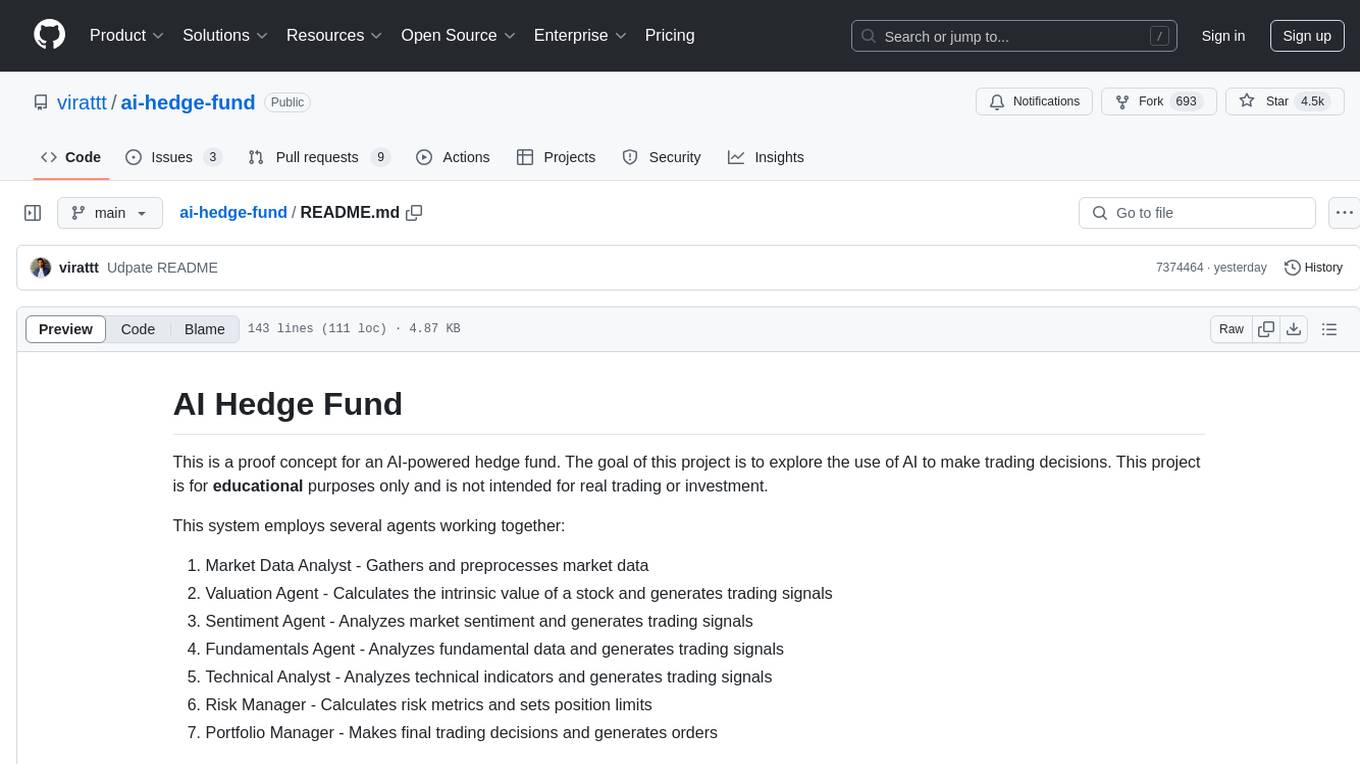
ai-hedge-fund
AI Hedge Fund is a proof of concept for an AI-powered hedge fund that explores the use of AI to make trading decisions. The project is for educational purposes only and simulates trading decisions without actual trading. It employs agents like Market Data Analyst, Valuation Agent, Sentiment Agent, Fundamentals Agent, Technical Analyst, Risk Manager, and Portfolio Manager to gather and analyze data, calculate risk metrics, and make trading decisions.
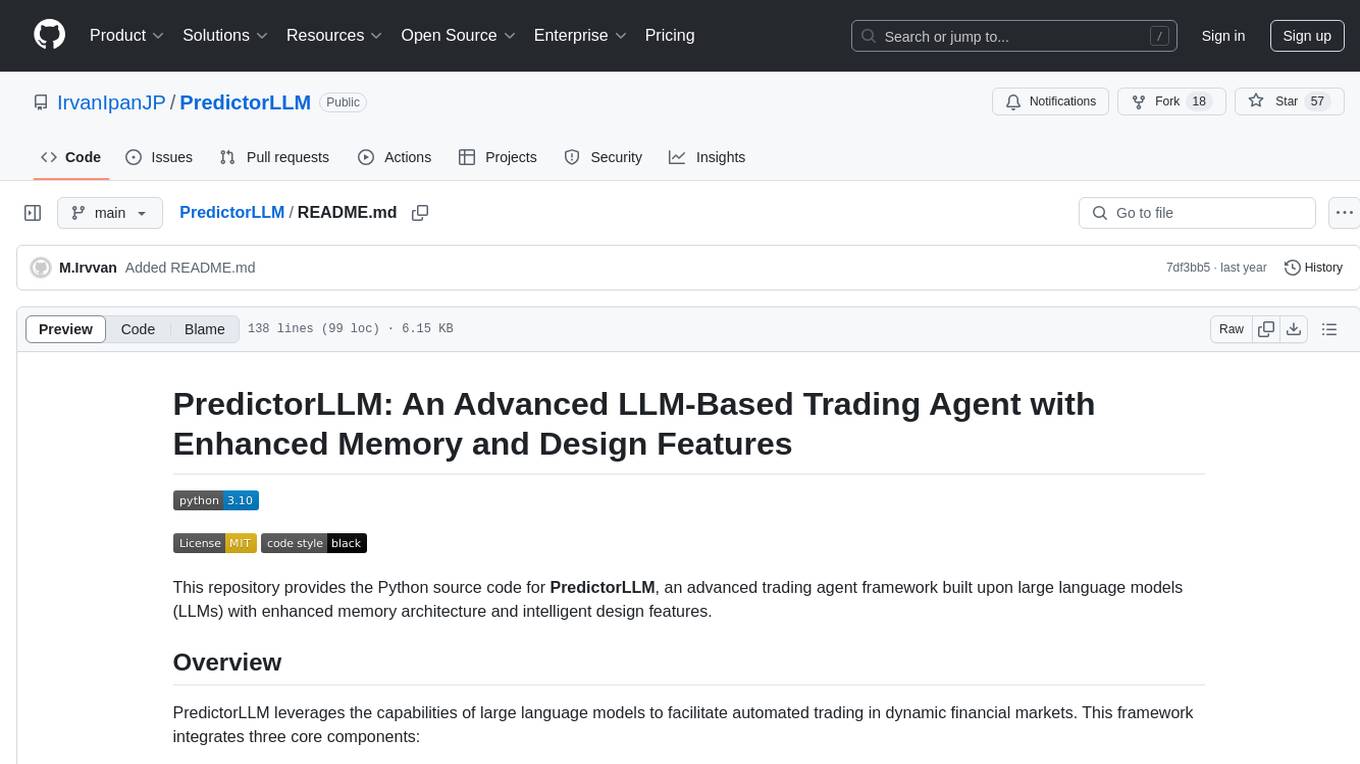
PredictorLLM
PredictorLLM is an advanced trading agent framework that utilizes large language models to automate trading in financial markets. It includes a profiling module to establish agent characteristics, a layered memory module for retaining and prioritizing financial data, and a decision-making module to convert insights into trading strategies. The framework mimics professional traders' behavior, surpassing human limitations in data processing and continuously evolving to adapt to market conditions for superior investment outcomes.
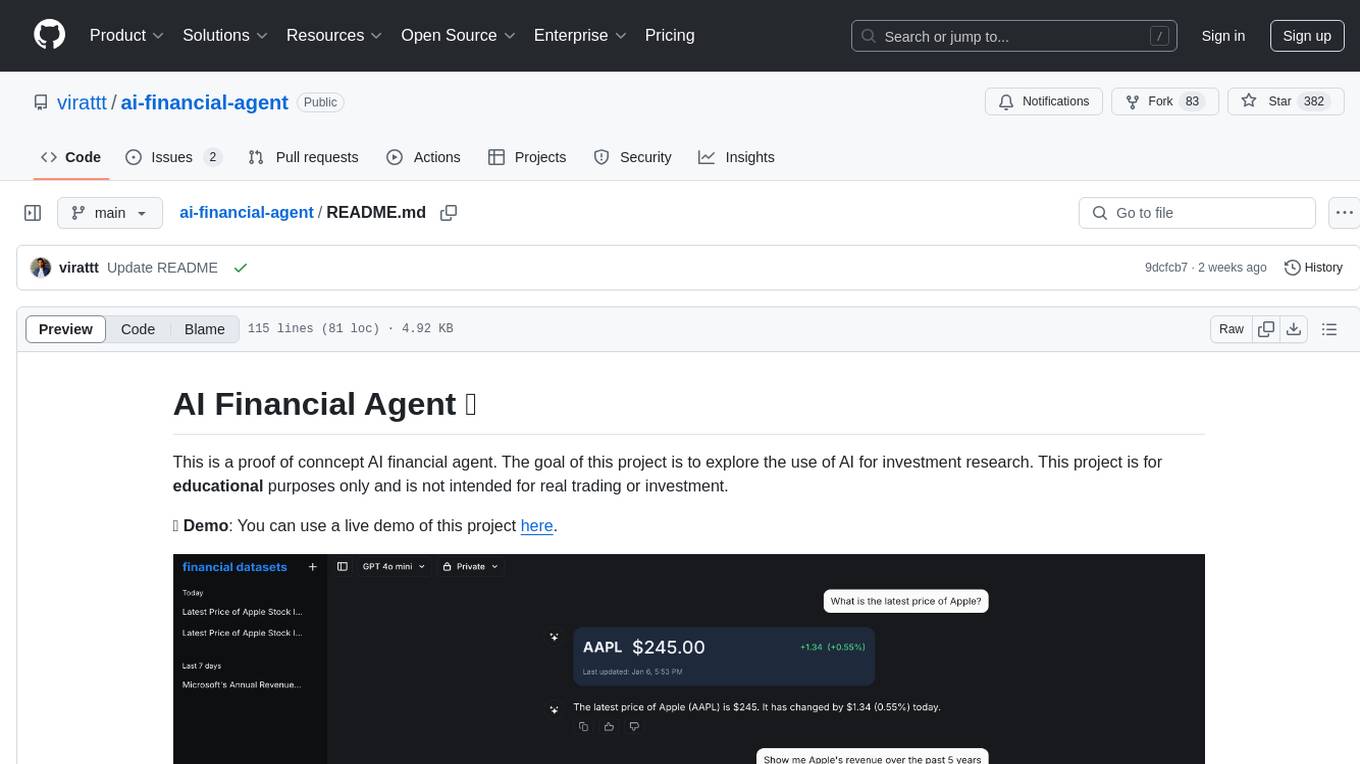
ai-financial-agent
AI Financial Agent is a proof of concept project exploring the use of AI for investment research. It provides an AI SDK with a unified API for generating text and structured objects, along with access to real-time and historical stock market data optimized for AI financial agents. The project includes features like dynamic chat interfaces, support for multiple model providers, and styling with Tailwind CSS. Users can deploy their own version of the AI Financial Agent using Vercel and GitHub integration.
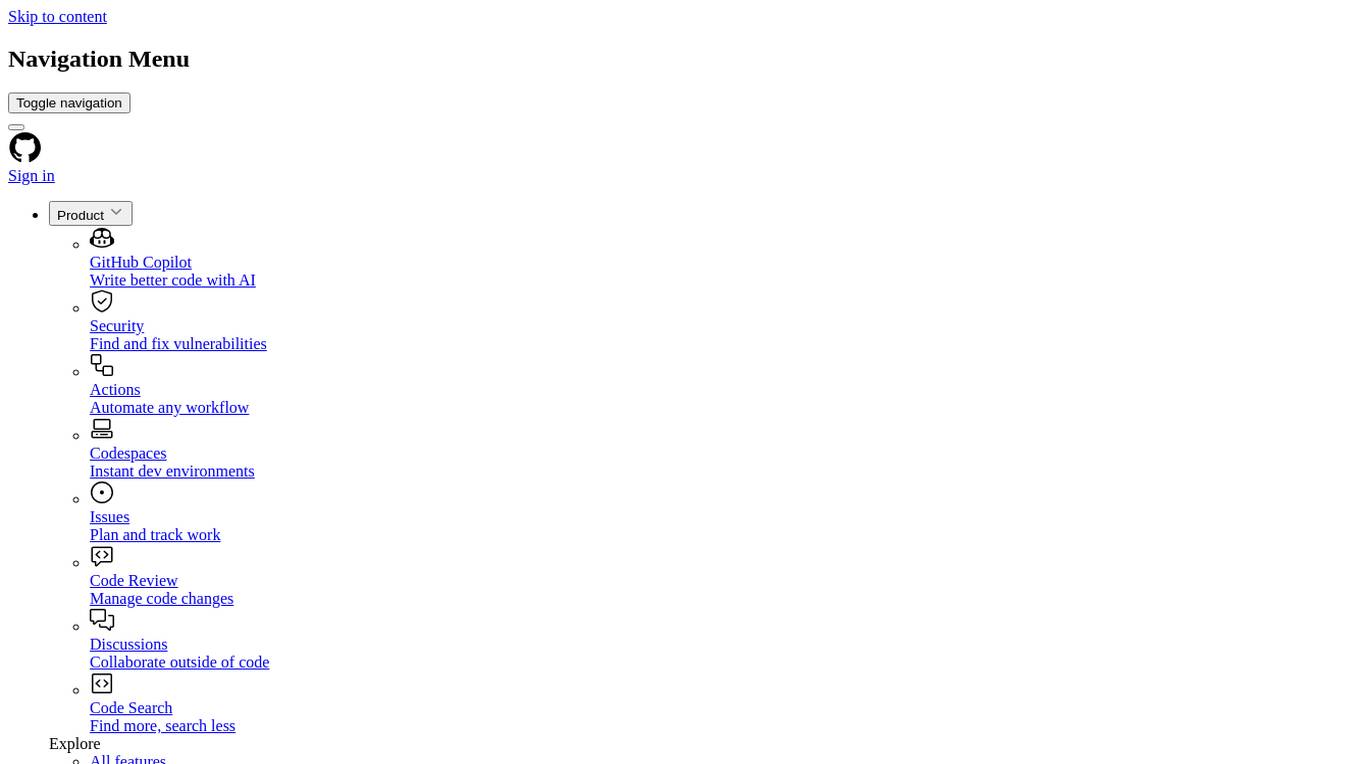
agentUniverse
agentUniverse is a multi-agent framework based on large language models, providing flexible capabilities for building individual agents. It focuses on collaborative pattern components to solve problems in various fields and integrates domain experience. The framework supports LLM model integration and offers various pattern components like PEER and DOE. Users can easily configure models and set up agents for tasks. agentUniverse aims to assist developers and enterprises in constructing domain-expert-level intelligent agents for seamless collaboration.
For similar jobs
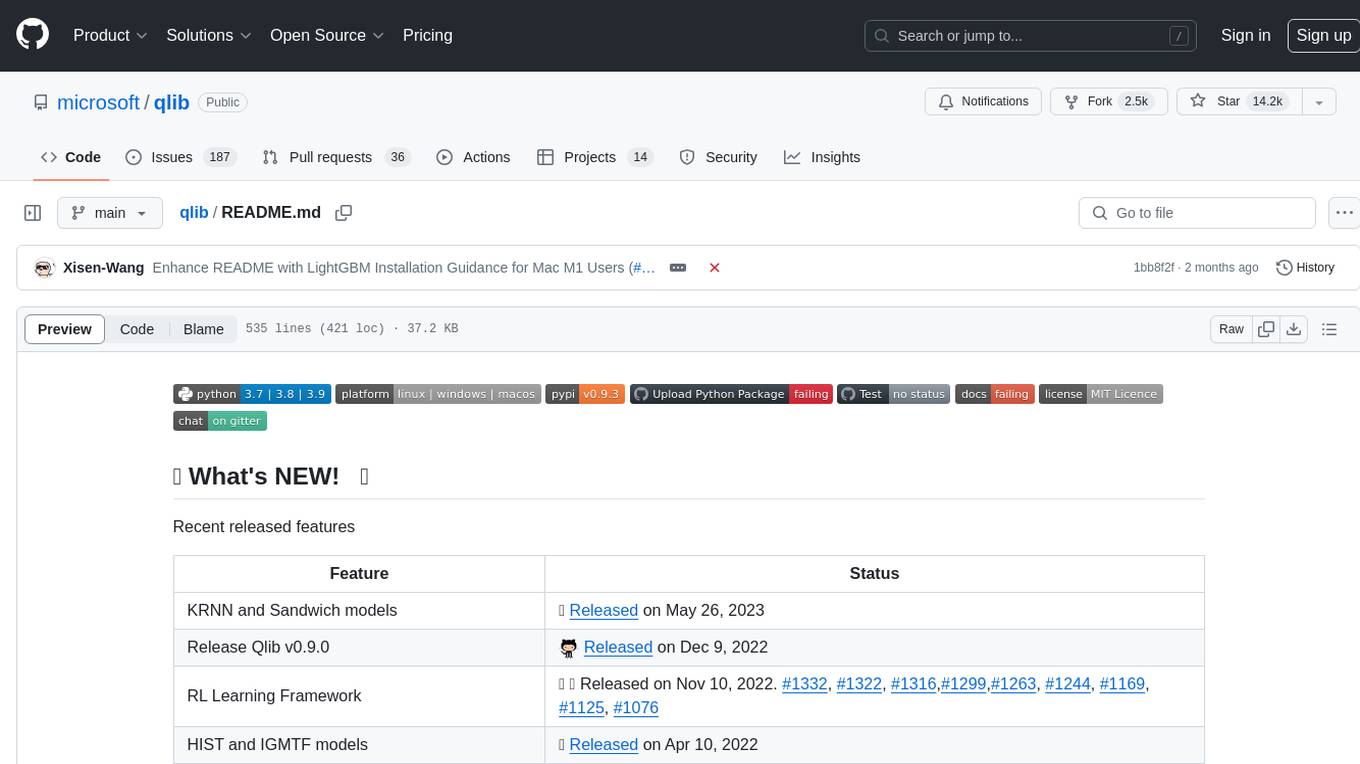
qlib
Qlib is an open-source, AI-oriented quantitative investment platform that supports diverse machine learning modeling paradigms, including supervised learning, market dynamics modeling, and reinforcement learning. It covers the entire chain of quantitative investment, from alpha seeking to order execution. The platform empowers researchers to explore ideas and implement productions using AI technologies in quantitative investment. Qlib collaboratively solves key challenges in quantitative investment by releasing state-of-the-art research works in various paradigms. It provides a full ML pipeline for data processing, model training, and back-testing, enabling users to perform tasks such as forecasting market patterns, adapting to market dynamics, and modeling continuous investment decisions.
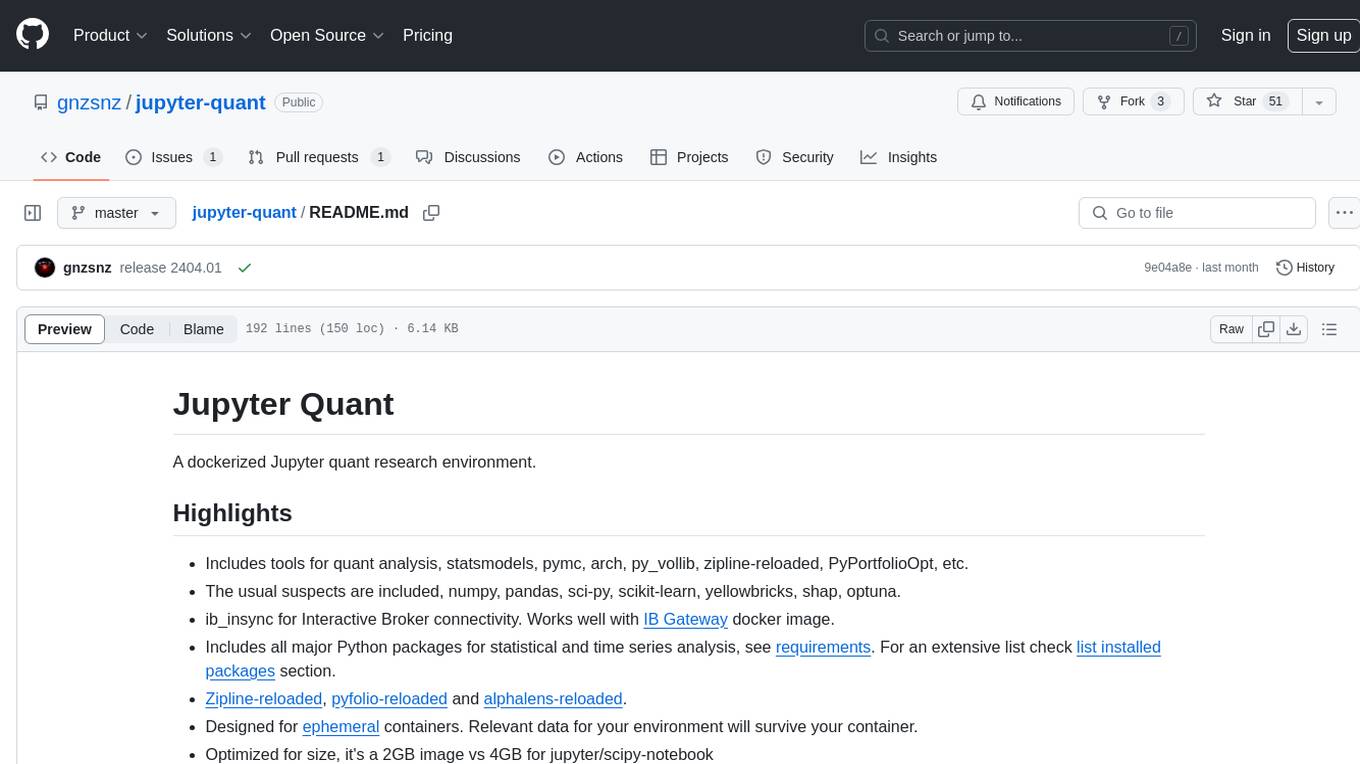
jupyter-quant
Jupyter Quant is a dockerized environment tailored for quantitative research, equipped with essential tools like statsmodels, pymc, arch, py_vollib, zipline-reloaded, PyPortfolioOpt, numpy, pandas, sci-py, scikit-learn, yellowbricks, shap, optuna, ib_insync, Cython, Numba, bottleneck, numexpr, jedi language server, jupyterlab-lsp, black, isort, and more. It does not include conda/mamba and relies on pip for package installation. The image is optimized for size, includes common command line utilities, supports apt cache, and allows for the installation of additional packages. It is designed for ephemeral containers, ensuring data persistence, and offers volumes for data, configuration, and notebooks. Common tasks include setting up the server, managing configurations, setting passwords, listing installed packages, passing parameters to jupyter-lab, running commands in the container, building wheels outside the container, installing dotfiles and SSH keys, and creating SSH tunnels.
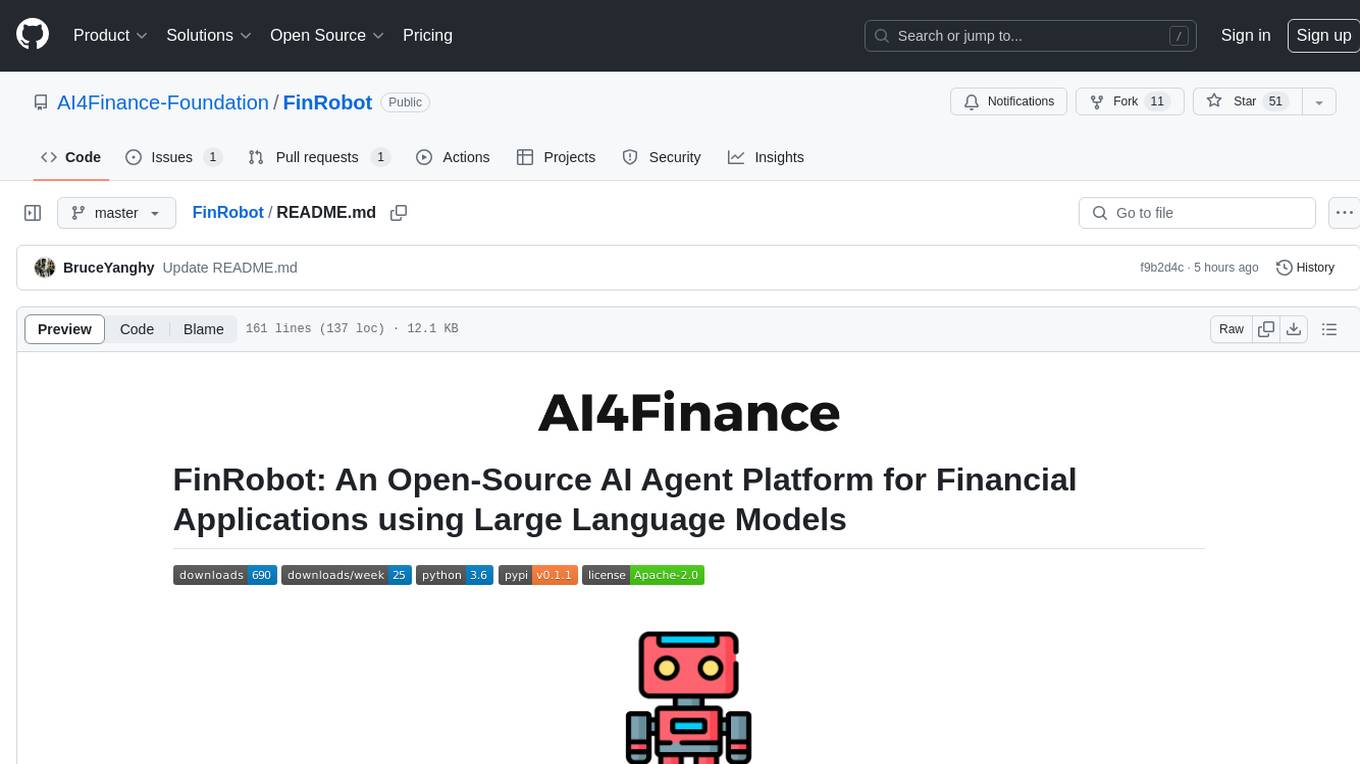
FinRobot
FinRobot is an open-source AI agent platform designed for financial applications using large language models. It transcends the scope of FinGPT, offering a comprehensive solution that integrates a diverse array of AI technologies. The platform's versatility and adaptability cater to the multifaceted needs of the financial industry. FinRobot's ecosystem is organized into four layers, including Financial AI Agents Layer, Financial LLMs Algorithms Layer, LLMOps and DataOps Layers, and Multi-source LLM Foundation Models Layer. The platform's agent workflow involves Perception, Brain, and Action modules to capture, process, and execute financial data and insights. The Smart Scheduler optimizes model diversity and selection for tasks, managed by components like Director Agent, Agent Registration, Agent Adaptor, and Task Manager. The tool provides a structured file organization with subfolders for agents, data sources, and functional modules, along with installation instructions and hands-on tutorials.
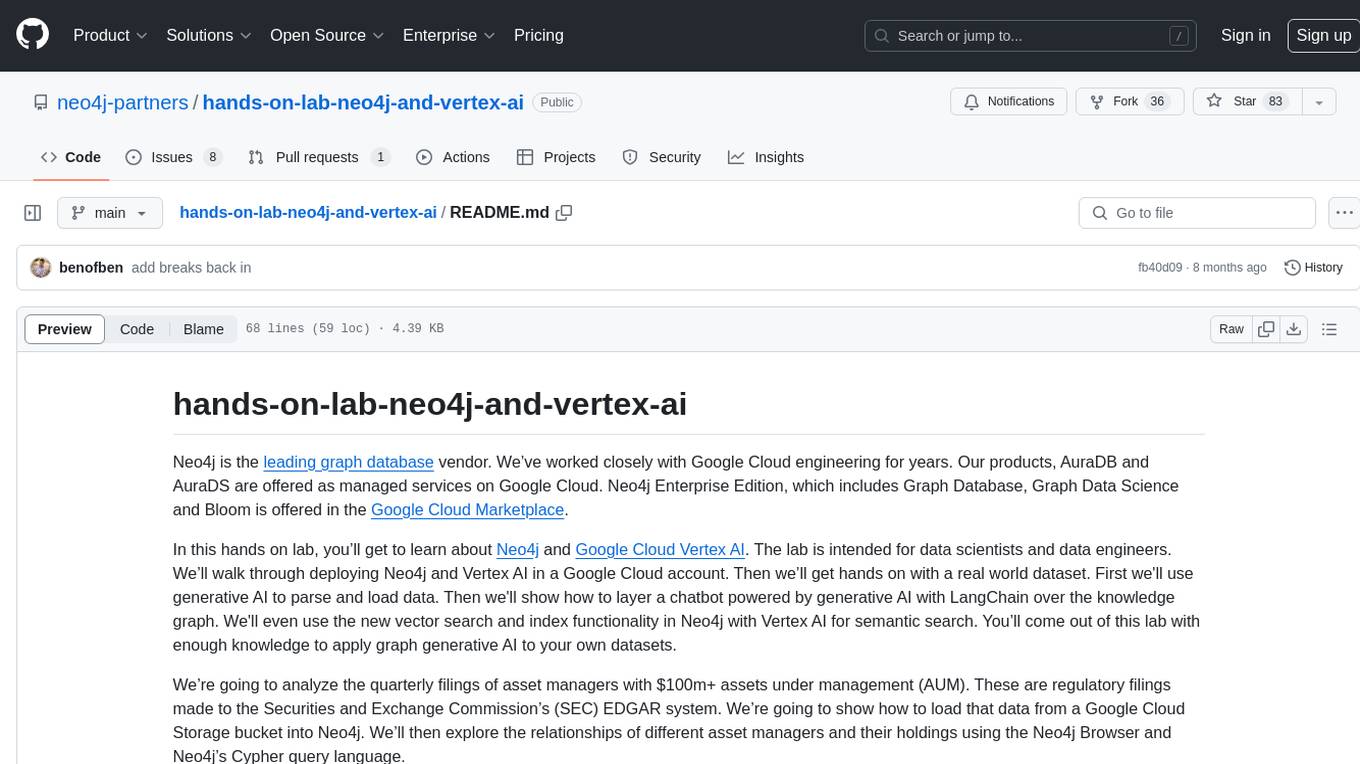
hands-on-lab-neo4j-and-vertex-ai
This repository provides a hands-on lab for learning about Neo4j and Google Cloud Vertex AI. It is intended for data scientists and data engineers to deploy Neo4j and Vertex AI in a Google Cloud account, work with real-world datasets, apply generative AI, build a chatbot over a knowledge graph, and use vector search and index functionality for semantic search. The lab focuses on analyzing quarterly filings of asset managers with $100m+ assets under management, exploring relationships using Neo4j Browser and Cypher query language, and discussing potential applications in capital markets such as algorithmic trading and securities master data management.
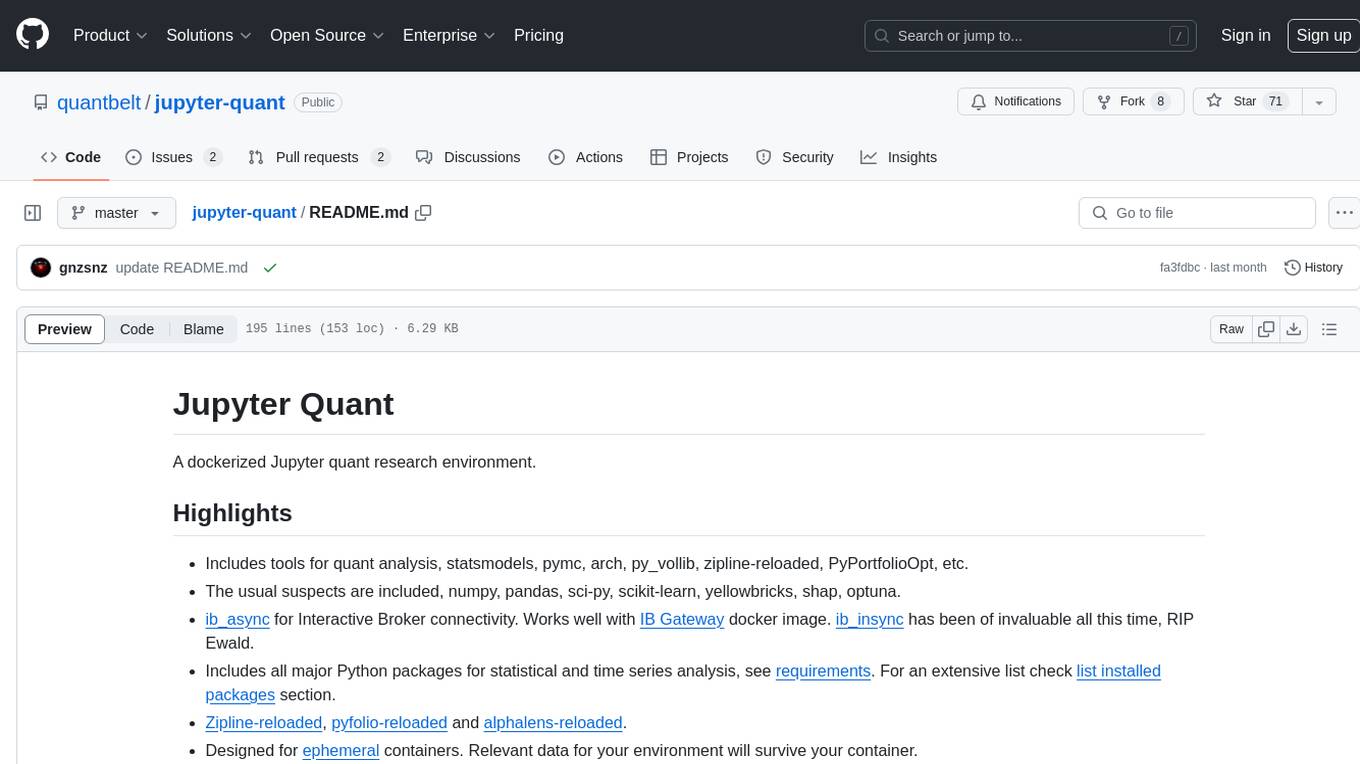
jupyter-quant
Jupyter Quant is a dockerized environment tailored for quantitative research, equipped with essential tools like statsmodels, pymc, arch, py_vollib, zipline-reloaded, PyPortfolioOpt, numpy, pandas, sci-py, scikit-learn, yellowbricks, shap, optuna, and more. It provides Interactive Broker connectivity via ib_async and includes major Python packages for statistical and time series analysis. The image is optimized for size, includes jedi language server, jupyterlab-lsp, and common command line utilities. Users can install new packages with sudo, leverage apt cache, and bring their own dot files and SSH keys. The tool is designed for ephemeral containers, ensuring data persistence and flexibility for quantitative analysis tasks.
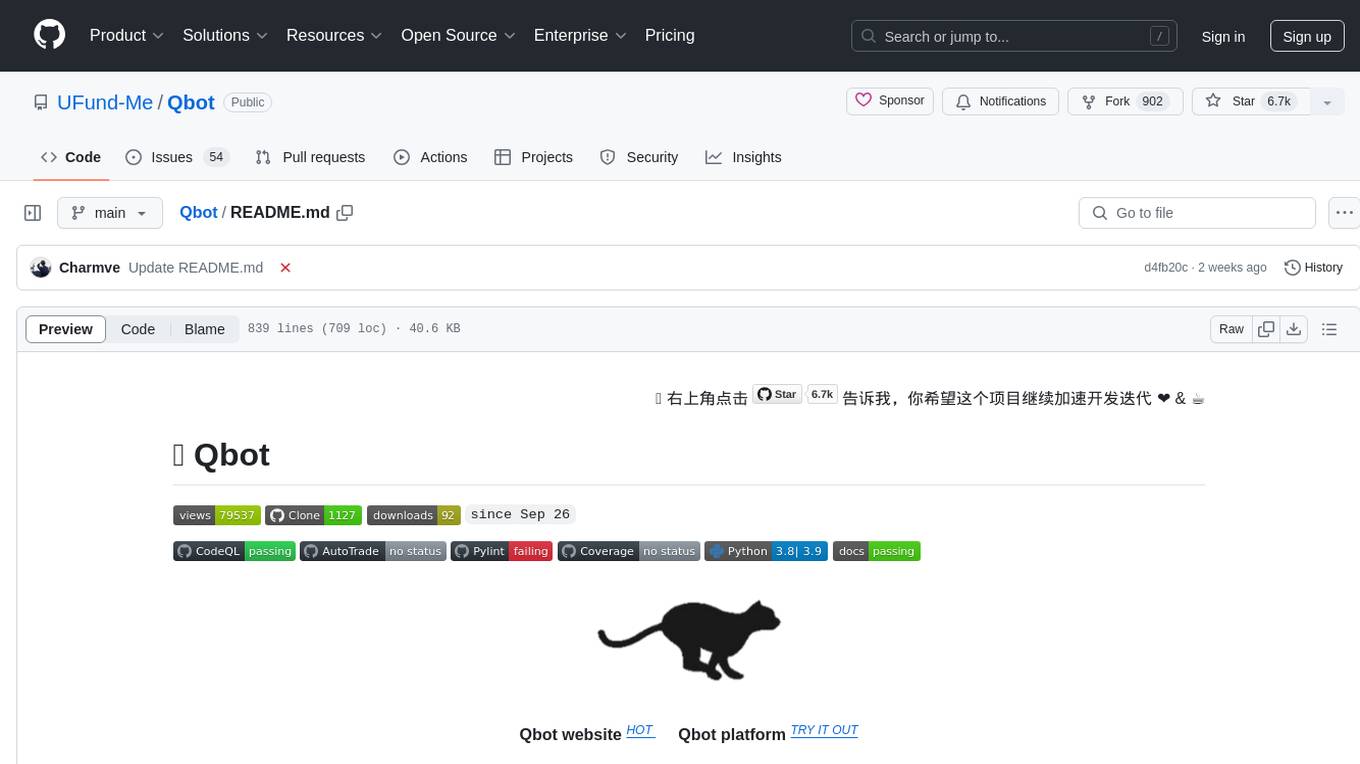
Qbot
Qbot is an AI-oriented automated quantitative investment platform that supports diverse machine learning modeling paradigms, including supervised learning, market dynamics modeling, and reinforcement learning. It provides a full closed-loop process from data acquisition, strategy development, backtesting, simulation trading to live trading. The platform emphasizes AI strategies such as machine learning, reinforcement learning, and deep learning, combined with multi-factor models to enhance returns. Users with some Python knowledge and trading experience can easily utilize the platform to address trading pain points and gaps in the market.
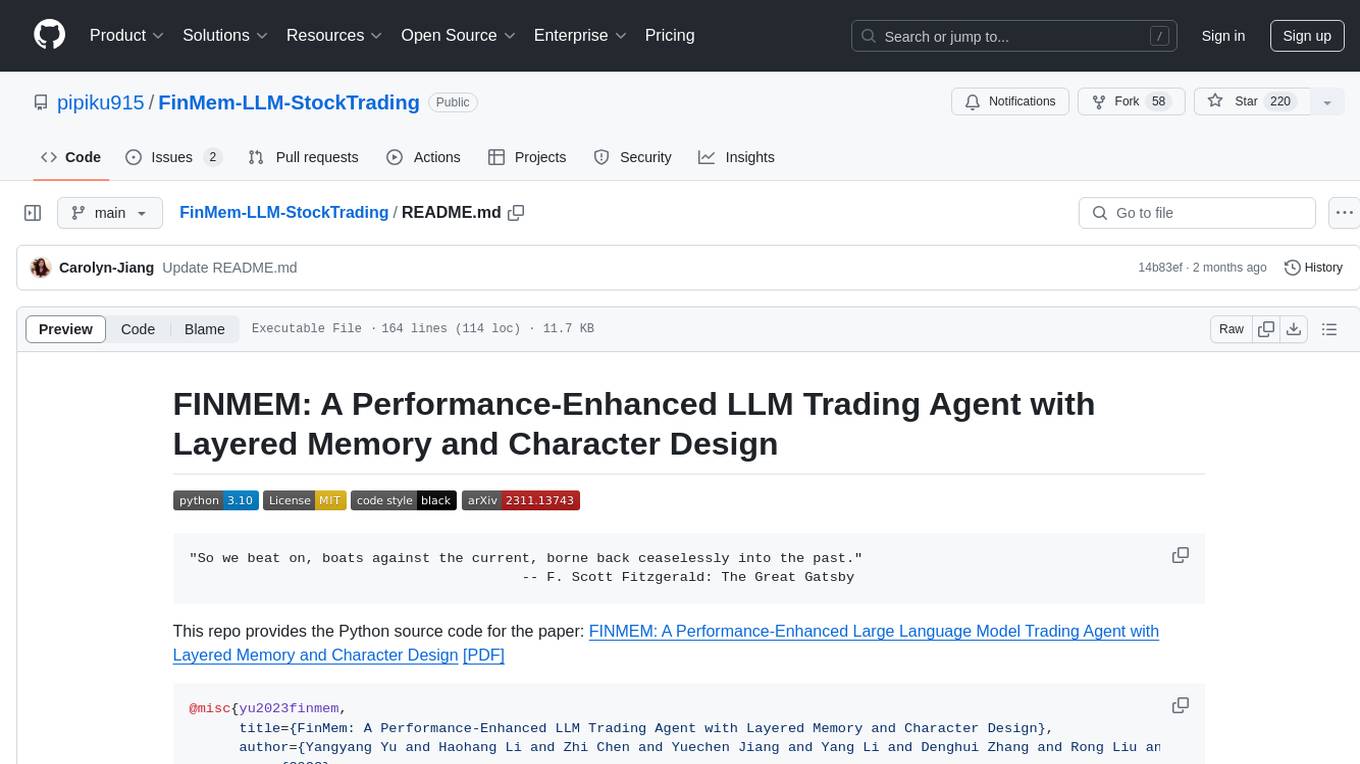
FinMem-LLM-StockTrading
This repository contains the Python source code for FINMEM, a Performance-Enhanced Large Language Model Trading Agent with Layered Memory and Character Design. It introduces FinMem, a novel LLM-based agent framework devised for financial decision-making, encompassing three core modules: Profiling, Memory with layered processing, and Decision-making. FinMem's memory module aligns closely with the cognitive structure of human traders, offering robust interpretability and real-time tuning. The framework enables the agent to self-evolve its professional knowledge, react agilely to new investment cues, and continuously refine trading decisions in the volatile financial environment. It presents a cutting-edge LLM agent framework for automated trading, boosting cumulative investment returns.
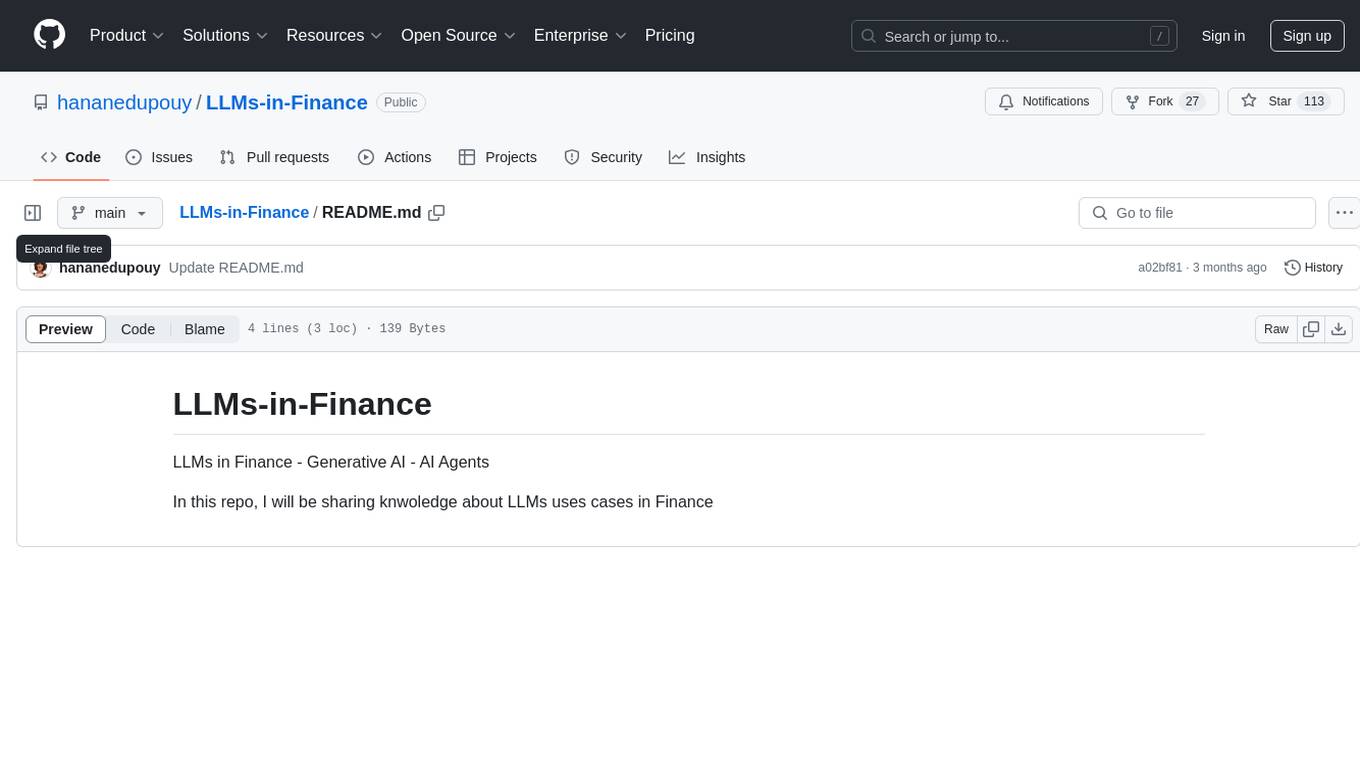
LLMs-in-Finance
This repository focuses on the application of Large Language Models (LLMs) in the field of finance. It provides insights and knowledge about how LLMs can be utilized in various scenarios within the finance industry, particularly in generating AI agents. The repository aims to explore the potential of LLMs to enhance financial processes and decision-making through the use of advanced natural language processing techniques.