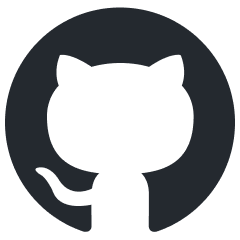
SEED-Bench
(CVPR2024)A benchmark for evaluating Multimodal LLMs using multiple-choice questions.
Stars: 240
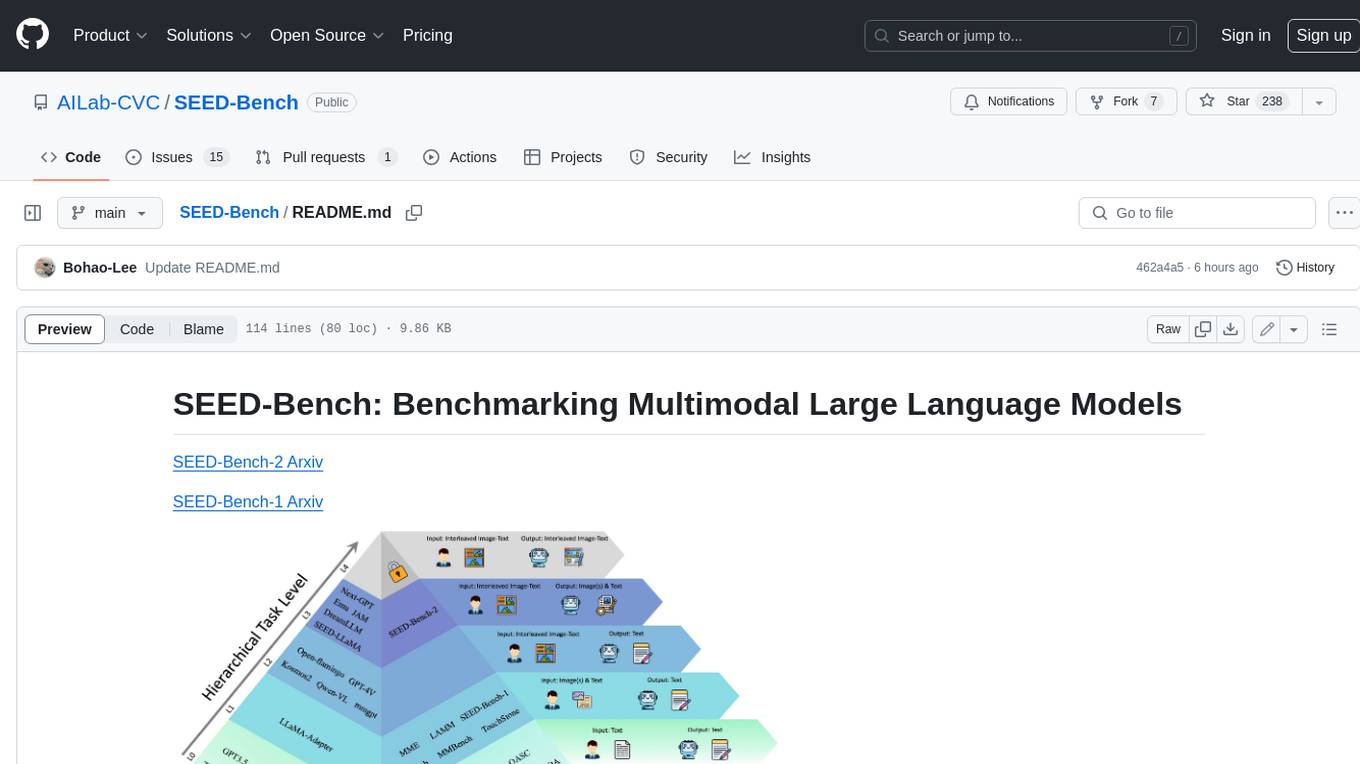
SEED-Bench is a comprehensive benchmark for evaluating the performance of multimodal large language models (LLMs) on a wide range of tasks that require both text and image understanding. It consists of two versions: SEED-Bench-1 and SEED-Bench-2. SEED-Bench-1 focuses on evaluating the spatial and temporal understanding of LLMs, while SEED-Bench-2 extends the evaluation to include text and image generation tasks. Both versions of SEED-Bench provide a diverse set of tasks that cover different aspects of multimodal understanding, making it a valuable tool for researchers and practitioners working on LLMs.
README:
SEED-Bench-2-Plus comprises 2.3K multiple-choice questions with precise human annotations, spanning three broad categories: Charts, Maps, and Webs, each of which covers a wide spectrum of textrich scenarios in the real world.
SEED-Bench-2 comprises 24K multiple-choice questions with accurate human annotations, which spans 27 dimensions, including the evaluation of both text and image generation.
SEED-Bench-1 consists of 19K multiple-choice questions with accurate human annotations, covering 12 evaluation dimensions including both the spatial and temporal understanding.
[2024.4.26] We are excited to announce the release of SEED-Bench-2-Plus, a benchmark specifically designed for text-rich visual comprehension. The accompanying dataset is released on SEED-Bench-2-Plus.
[2024.4.23] We are pleased to share the comprehensive evaluation results for Gemini-Vision-Pro and Claude-3-Opus on SEED-Bench-1 and SEED-Bench-2. You can access detailed performance on the SEED-Bench Leaderboard. Please note that for Gemini-Vision-Pro we only report task performance when the model responds with at least 50% valid data in the task.
[2024.2.27] SEED-Bench is accepted by CVPR 2024.
[2023.12.18] We have placed the comprehensive evaluation results for GPT-4v on SEED-Bench-1 and SEED-Bench-2. These can be accessed at GPT-4V for SEED-Bench-1 and GPT-4V for SEED-Bench-2. If you're interested, please feel free to take a look.
[2023.12.4] We have updated the SEED-Bench Leaderboard for SEED-Bench-2. Additionally, we have updated the evaluation results for GPT-4v on both SEED-Bench-1 and SEED-Bench-2. If you are interested, please visit the SEED-Bench Leaderboard for more details.
[2023.11.30] We have updated the SEED-Bench-v1 JSON (manually screening the multiple-choice questions for videos) and provided corresponding video frames for easier testing. Please refer to SEED-Bench for more information.
[2023.11.27] SEED-Bench-2 is released! Data and evaluation code is available now.
[2023.9.9] We are actively looking for self-motivated interns. Please feel free to reach out if you are interested.
[2023.8.16] SEED-Bench Leaderboard is released! You can upload your model's results now.
[2023.7.30] SEED-Bench is released! Data and evaluation code is available now.
Welcome to SEED-Bench Leaderboard!
You can submit your model results in SEED-Bench Leaderboard now. You can use our evaluation code to obtain 'results.json' in 'results' folder as below.
python eval.py --model instruct_blip --anno_path SEED-Bench.json --output-dir results --task all
Then you can upload 'results.json' in SEED-Bench Leaderboard.
After submitting, please press refresh button to get the latest results.
You can download the data of SEED-Bench released on HuggingFace repo SEED-Bench, SEED-Bench-2, and SEED-Bench-2-Plus. Please refer to DATASET.md for data preparation.
Please refer to INSTALL.md.
Please refer to EVALUATION.md.
SEED-Bench is released under Apache License Version 2.0.
Data Sources: Data from the internet under CC-BY licenses.
Please contact us if you believe any data infringes upon your rights, and we will remove it.
Data Sources:
- Dimensions 1-9, 23 (In-Context Captioning): Conceptual Captions Dataset (https://ai.google.com/research/ConceptualCaptions/) under its license (https://github.com/google-research-datasets/conceptual-captions/blob/master/LICENSE). Copyright belongs to the original dataset owner.
- Dimension 9 (Text Recognition): ICDAR2003 (http://www.imglab.org/db/index.html), ICDAR2013(https://rrc.cvc.uab.es/?ch=2), IIIT5k(https://cvit.iiit.ac.in/research/projects/cvit-projects/the-iiit-5k-word-dataset), and SVT(http://vision.ucsd.edu/~kai/svt/). Copyright belongs to the original dataset owner.
- Dimension 10 (Celebrity Recognition): MME (https://github.com/BradyFU/Awesome-Multimodal-Large-Language-Models/tree/Evaluation) and MMBench (https://github.com/open-compass/MMBench) under MMBench license (https://github.com/open-compass/MMBench/blob/main/LICENSE). Copyright belongs to the original dataset owners.
- Dimension 11 (Landmark Recognition): Google Landmark Dataset v2 (https://github.com/cvdfoundation/google-landmark) under CC-BY licenses without ND restrictions.
- Dimension 12 (Chart Understanding): PlotQA (https://github.com/NiteshMethani/PlotQA) under its license (https://github.com/NiteshMethani/PlotQA/blob/master/LICENSE).
- Dimension 13 (Visual Referring Expression): VCR (http://visualcommonsense.com) under its license (http://visualcommonsense.com/license/).
- Dimension 14 (Science Knowledge): ScienceQA (https://github.com/lupantech/ScienceQA) under its license (https://github.com/lupantech/ScienceQA/blob/main/LICENSE-DATA).
- Dimension 15 (Emotion Recognition): FER2013 (https://www.kaggle.com/competitions/challenges-in-representation-learning-facial-expression-recognition-challenge/data) under its license (https://www.kaggle.com/competitions/challenges-in-representation-learning-facial-expression-recognition-challenge/rules#7-competition-data).
- Dimension 16 (Visual Mathematics): MME (https://github.com/BradyFU/Awesome-Multimodal-Large-Language-Models/tree/Evaluation) and data from the internet under CC-BY licenses.
- Dimension 17 (Difference Spotting): MIMICIT (https://github.com/Luodian/Otter/blob/main/mimic-it/README.md) under its license (https://github.com/Luodian/Otter/tree/main/mimic-it#eggs).
- Dimension 18 (Meme Comprehension): Data from the internet under CC-BY licenses.
- Dimension 19 (Global Video Understanding): Charades (https://prior.allenai.org/projects/charades) under its license (https://prior.allenai.org/projects/data/charades/license.txt). SEED-Bench-2 provides 8 frames per video.
- Dimensions 20-22 (Action Recognition, Action Prediction, Procedure Understanding): Something-Something v2 (https://developer.qualcomm.com/software/ai-datasets/something-something), Epic-Kitchen 100 (https://epic-kitchens.github.io/2023), and Breakfast (https://serre-lab.clps.brown.edu/resource/breakfast-actions-dataset/). SEED-Bench-2 provides 8 frames per video.
- Dimension 24 (Interleaved Image-Text Analysis): Data from the internet under CC-BY licenses.
- Dimension 25 (Text-to-Image Generation): CC-500 (https://github.com/weixi-feng/Structured-Diffusion-Guidance) and ABC-6k (https://github.com/weixi-feng/Structured-Diffusion-Guidance) under their license (https://github.com/weixi-feng/Structured-Diffusion-Guidance/blob/master/LICENSE), with images generated by Stable-Diffusion-XL (https://huggingface.co/stabilityai/stable-diffusion-xl-base-1.0) under its license (https://huggingface.co/stabilityai/stable-diffusion-xl-base-1.0/blob/main/LICENSE.md).
- Dimension 26 (Next Image Prediction): Epic-Kitchen 100 (https://epic-kitchens.github.io/2023) under its license (https://creativecommons.org/licenses/by-nc/4.0/).
- Dimension 27 (Text-Image Creation): Data from the internet under CC-BY licenses.
Please contact us if you believe any data infringes upon your rights, and we will remove it.
For the images of SEED-Bench-1, we use the data from Conceptual Captions Dataset (https://ai.google.com/research/ConceptualCaptions/) following its license (https://github.com/google-research-datasets/conceptual-captions/blob/master/LICENSE). Tencent does not hold the copyright for these images and the copyright belongs to the original owner of Conceptual Captions Dataset.
For the videos of SEED-Bench-1, we use tha data from Something-Something v2 (https://developer.qualcomm.com/software/ai-datasets/something-something), Epic-kitchen 100 (https://epic-kitchens.github.io/2023) and Breakfast (https://serre-lab.clps.brown.edu/resource/breakfast-actions-dataset/). We only provide the video name. Please download them in their official websites.
If you find this repository helpful, please consider citing it:
@article{li2023seed2,
title={SEED-Bench-2: Benchmarking Multimodal Large Language Models},
author={Li, Bohao and Ge, Yuying and Ge, Yixiao and Wang, Guangzhi and Wang, Rui and Zhang, Ruimao and Shan, Ying},
journal={arXiv preprint arXiv:2311.17092},
year={2023}
}
@article{li2023seed,
title={Seed-bench: Benchmarking multimodal llms with generative comprehension},
author={Li, Bohao and Wang, Rui and Wang, Guangzhi and Ge, Yuying and Ge, Yixiao and Shan, Ying},
journal={arXiv preprint arXiv:2307.16125},
year={2023}
}
For Tasks:
Click tags to check more tools for each tasksFor Jobs:
Alternative AI tools for SEED-Bench
Similar Open Source Tools
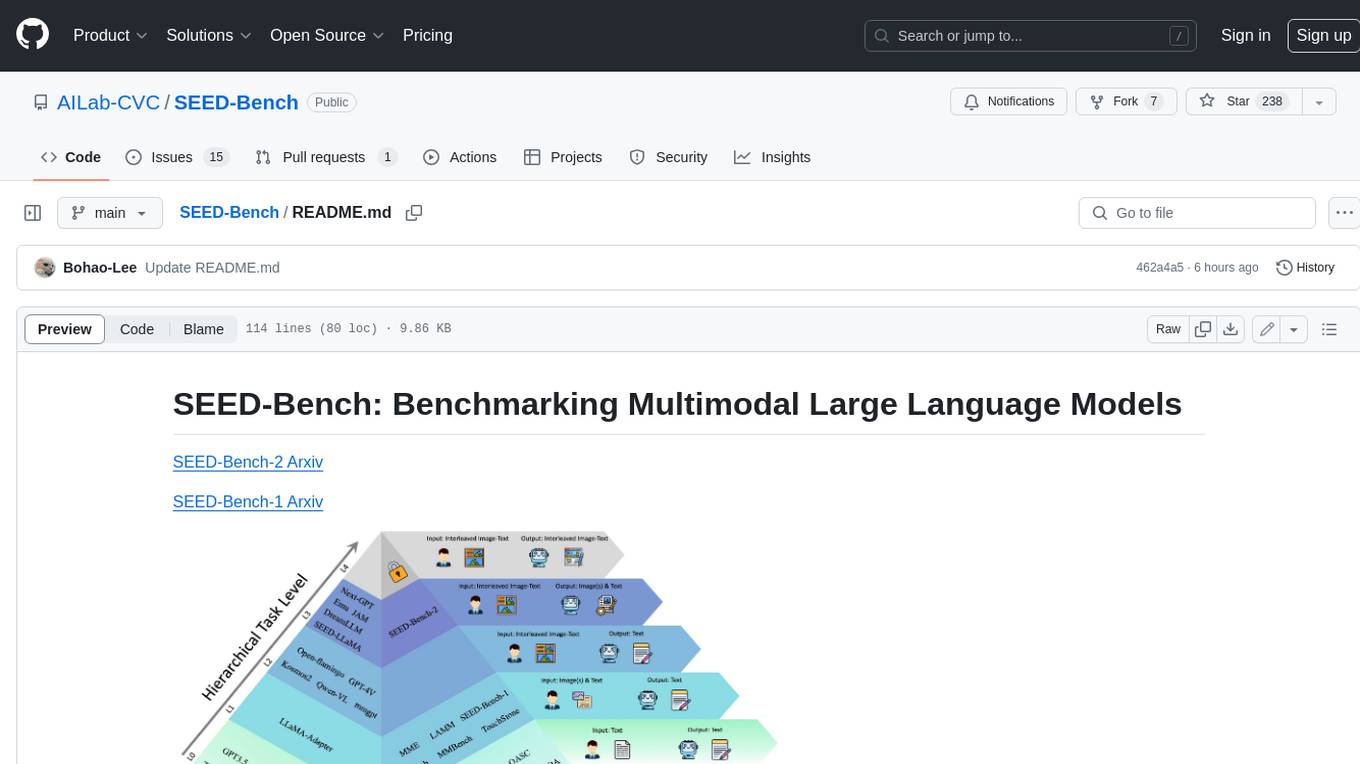
SEED-Bench
SEED-Bench is a comprehensive benchmark for evaluating the performance of multimodal large language models (LLMs) on a wide range of tasks that require both text and image understanding. It consists of two versions: SEED-Bench-1 and SEED-Bench-2. SEED-Bench-1 focuses on evaluating the spatial and temporal understanding of LLMs, while SEED-Bench-2 extends the evaluation to include text and image generation tasks. Both versions of SEED-Bench provide a diverse set of tasks that cover different aspects of multimodal understanding, making it a valuable tool for researchers and practitioners working on LLMs.
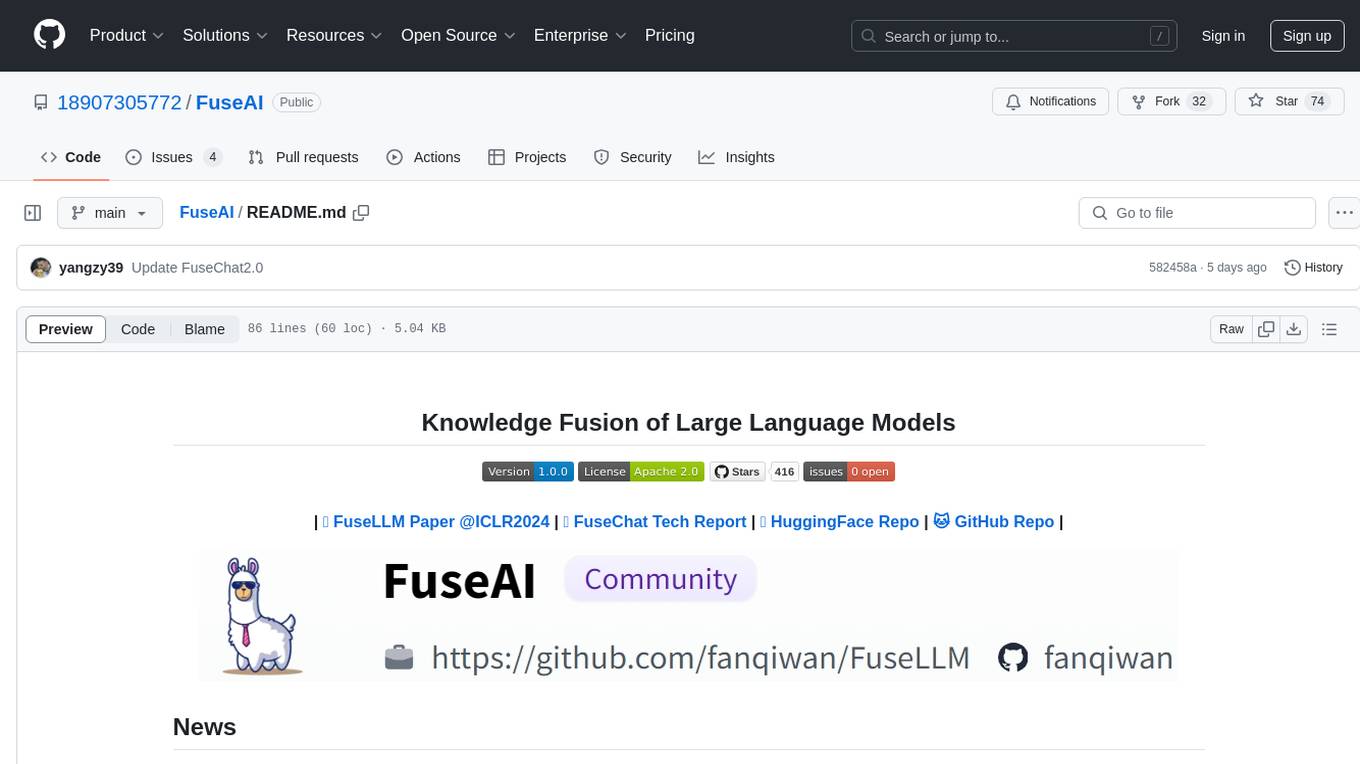
FuseAI
FuseAI is a repository that focuses on knowledge fusion of large language models. It includes FuseChat, a state-of-the-art 7B LLM on MT-Bench, and FuseLLM, which surpasses Llama-2-7B by fusing three open-source foundation LLMs. The repository provides tech reports, releases, and datasets for FuseChat and FuseLLM, showcasing their performance and advancements in the field of chat models and large language models.
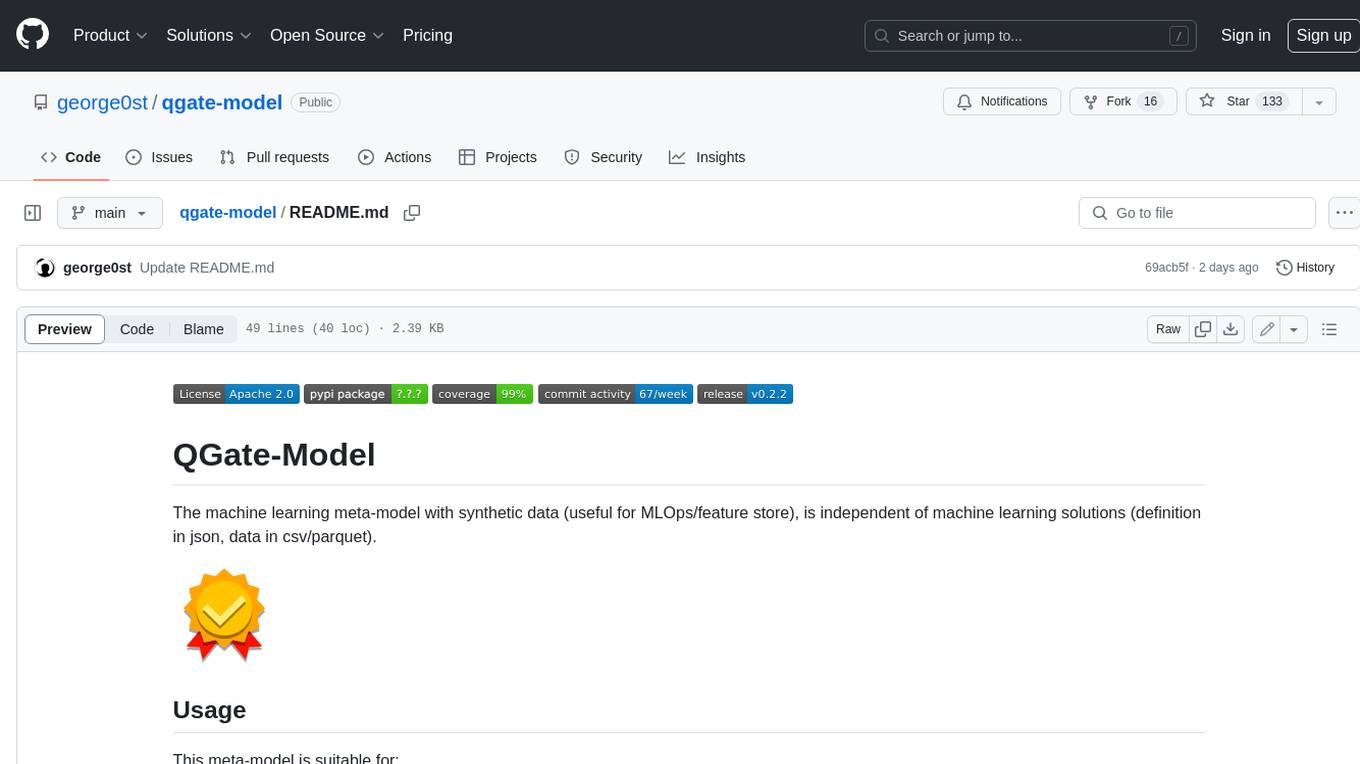
qgate-model
QGate-Model is a machine learning meta-model with synthetic data, designed for MLOps and feature store. It is independent of machine learning solutions, with definitions in JSON and data in CSV/parquet formats. This meta-model is useful for comparing capabilities and functions of machine learning solutions, independently testing new versions of machine learning solutions, and conducting various types of tests (unit, sanity, smoke, system, regression, function, acceptance, performance, shadow, etc.). It can also be used for external test coverage when internal test coverage is not available or weak.
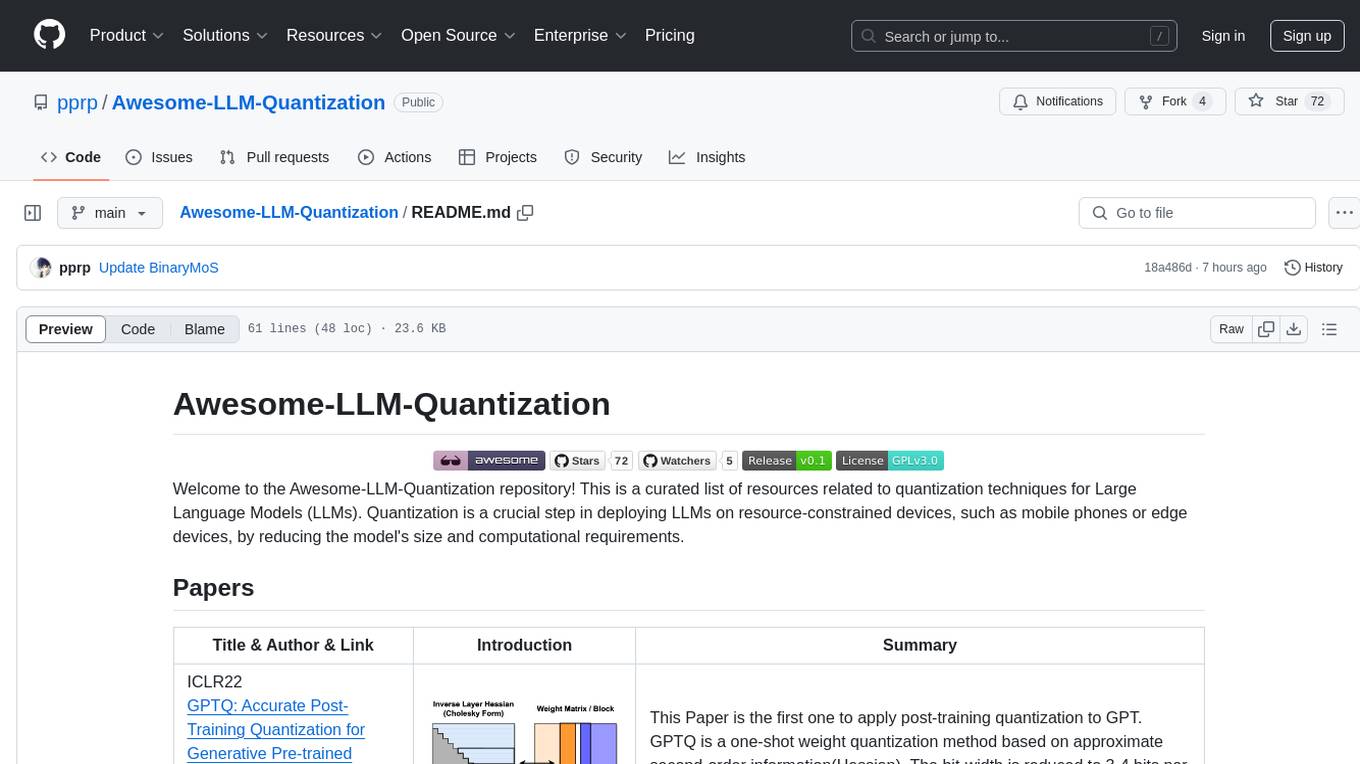
Awesome-LLM-Quantization
Awesome-LLM-Quantization is a curated list of resources related to quantization techniques for Large Language Models (LLMs). Quantization is a crucial step in deploying LLMs on resource-constrained devices, such as mobile phones or edge devices, by reducing the model's size and computational requirements.
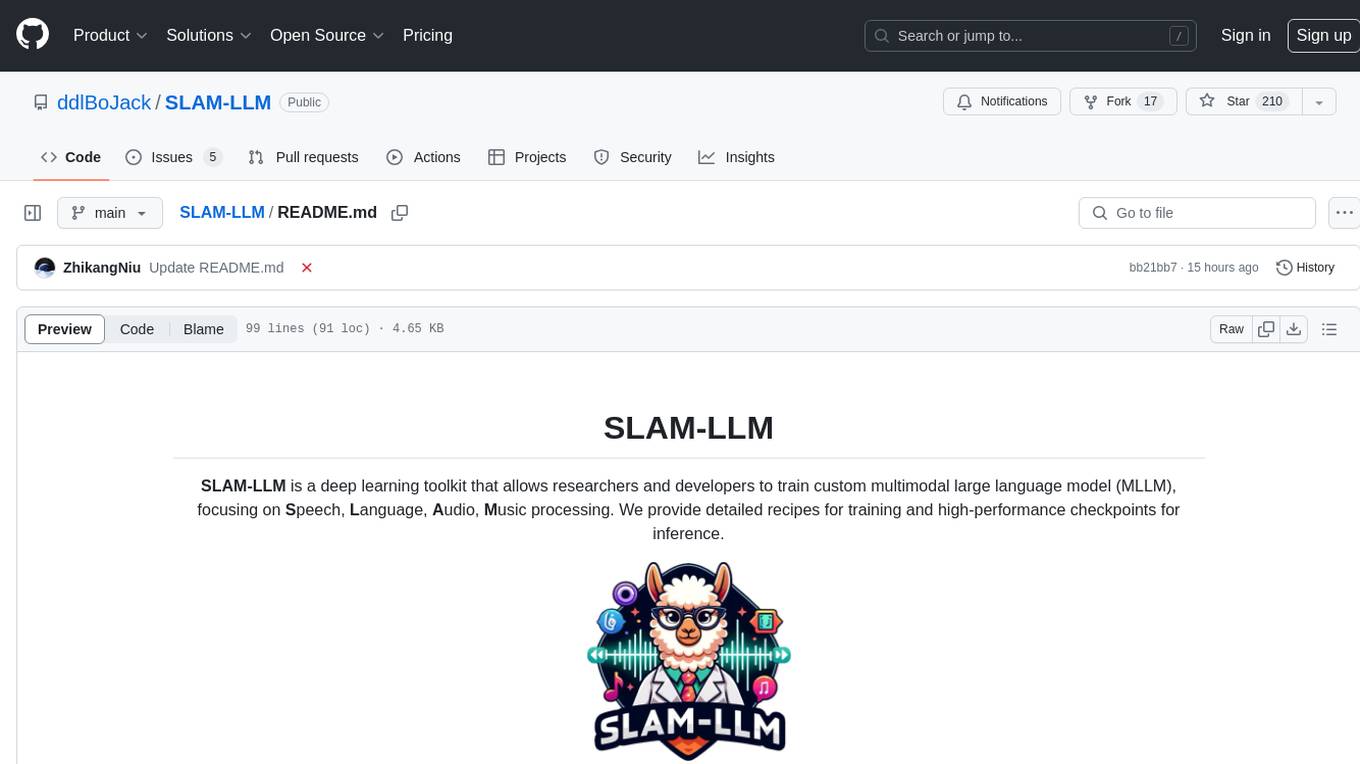
SLAM-LLM
SLAM-LLM is a deep learning toolkit designed for researchers and developers to train custom multimodal large language models (MLLM) focusing on speech, language, audio, and music processing. It provides detailed recipes for training and high-performance checkpoints for inference. The toolkit supports tasks such as automatic speech recognition (ASR), text-to-speech (TTS), visual speech recognition (VSR), automated audio captioning (AAC), spatial audio understanding, and music caption (MC). SLAM-LLM features easy extension to new models and tasks, mixed precision training for faster training with less GPU memory, multi-GPU training with data and model parallelism, and flexible configuration based on Hydra and dataclass.
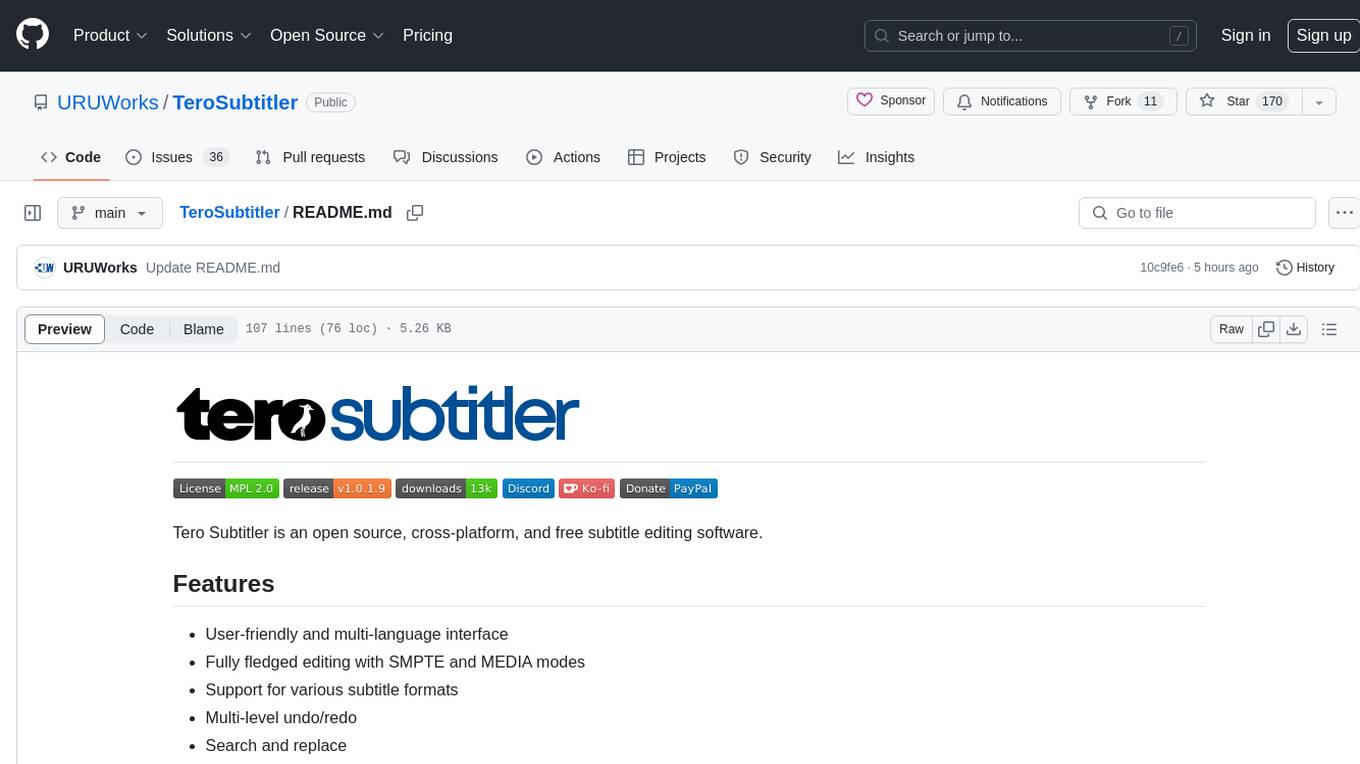
TeroSubtitler
Tero Subtitler is an open source, cross-platform, and free subtitle editing software with a user-friendly interface. It offers fully fledged editing with SMPTE and MEDIA modes, support for various subtitle formats, multi-level undo/redo, search and replace, auto-backup, source and transcription modes, translation memory, audiovisual preview, timeline with waveform visualizer, manipulation tools, formatting options, quality control features, translation and transcription capabilities, validation tools, automation for correcting errors, and more. It also includes features like exporting subtitles to MP3, importing/exporting Blu-ray SUP format, generating blank video, generating video with hardcoded subtitles, video dubbing, and more. The tool utilizes powerful multimedia playback engines like mpv, advanced audio/video manipulation tools like FFmpeg, tools for automatic transcription like whisper.cpp/Faster-Whisper, auto-translation API like Google Translate, and ElevenLabs TTS for video dubbing.
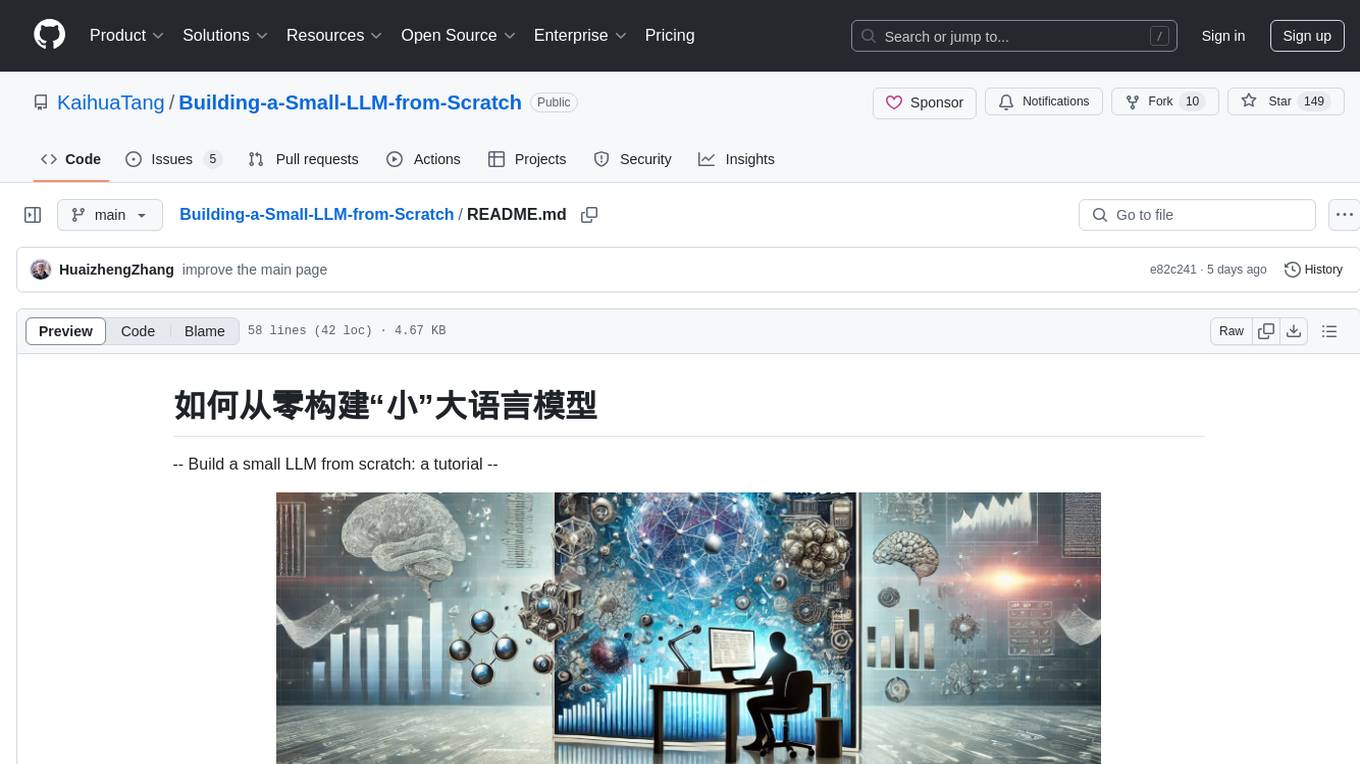
Building-a-Small-LLM-from-Scratch
This tutorial provides a comprehensive guide on building a small Large Language Model (LLM) from scratch using PyTorch. The author shares insights and experiences gained from working on LLM projects in the industry, aiming to help beginners understand the fundamental components of LLMs and training fine-tuning codes. The tutorial covers topics such as model structure overview, attention modules, optimization techniques, normalization layers, tokenizers, pretraining, and fine-tuning with dialogue data. It also addresses specific industry-related challenges and explores cutting-edge model concepts like DeepSeek network structure, causal attention, dynamic-to-static tensor conversion for ONNX inference, and performance optimizations for NPU chips. The series emphasizes hands-on practice with small models to enable local execution and plans to expand into multimodal language models and tensor parallel multi-card deployment.
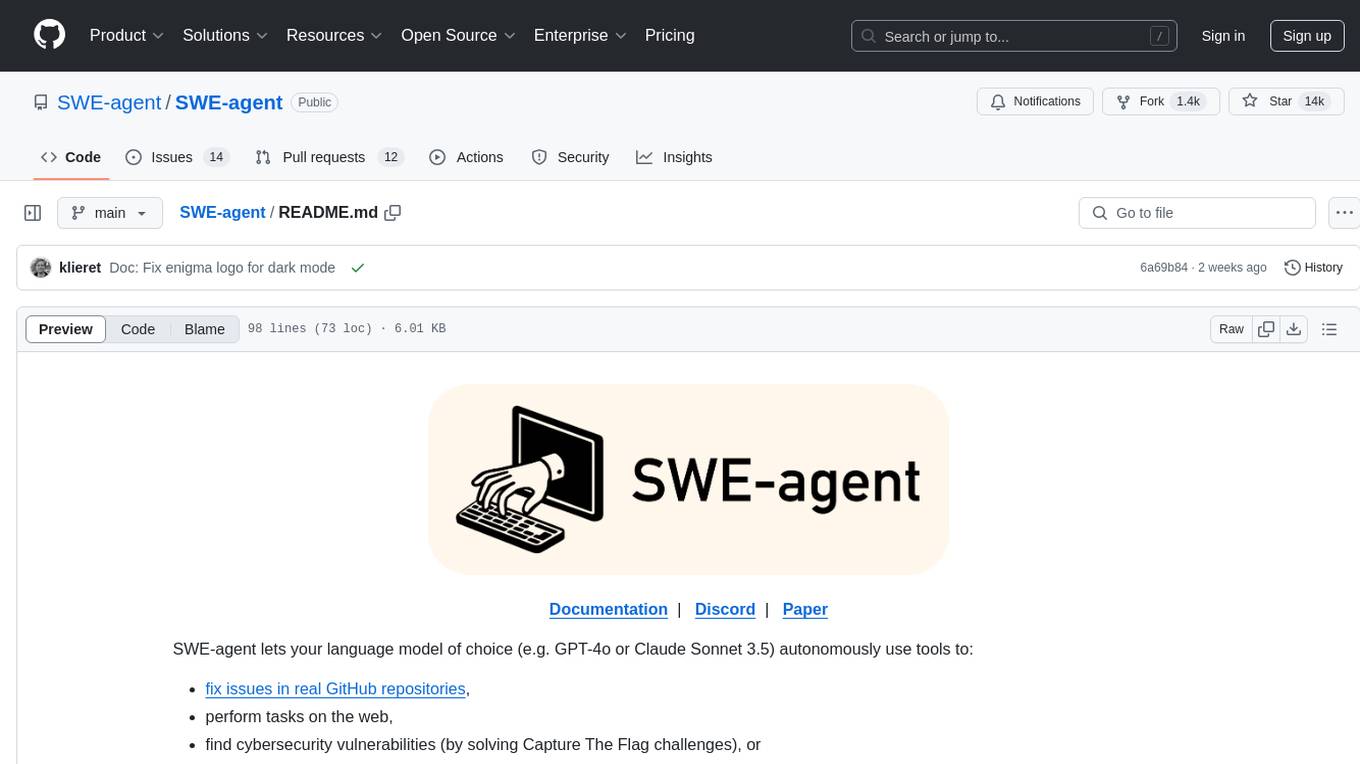
SWE-agent
SWE-agent is a tool that allows language models to autonomously fix issues in GitHub repositories, perform tasks on the web, find cybersecurity vulnerabilities, and handle custom tasks. It uses configurable agent-computer interfaces (ACIs) to interact with isolated computer environments. The tool is built and maintained by researchers from Princeton University and Stanford University.
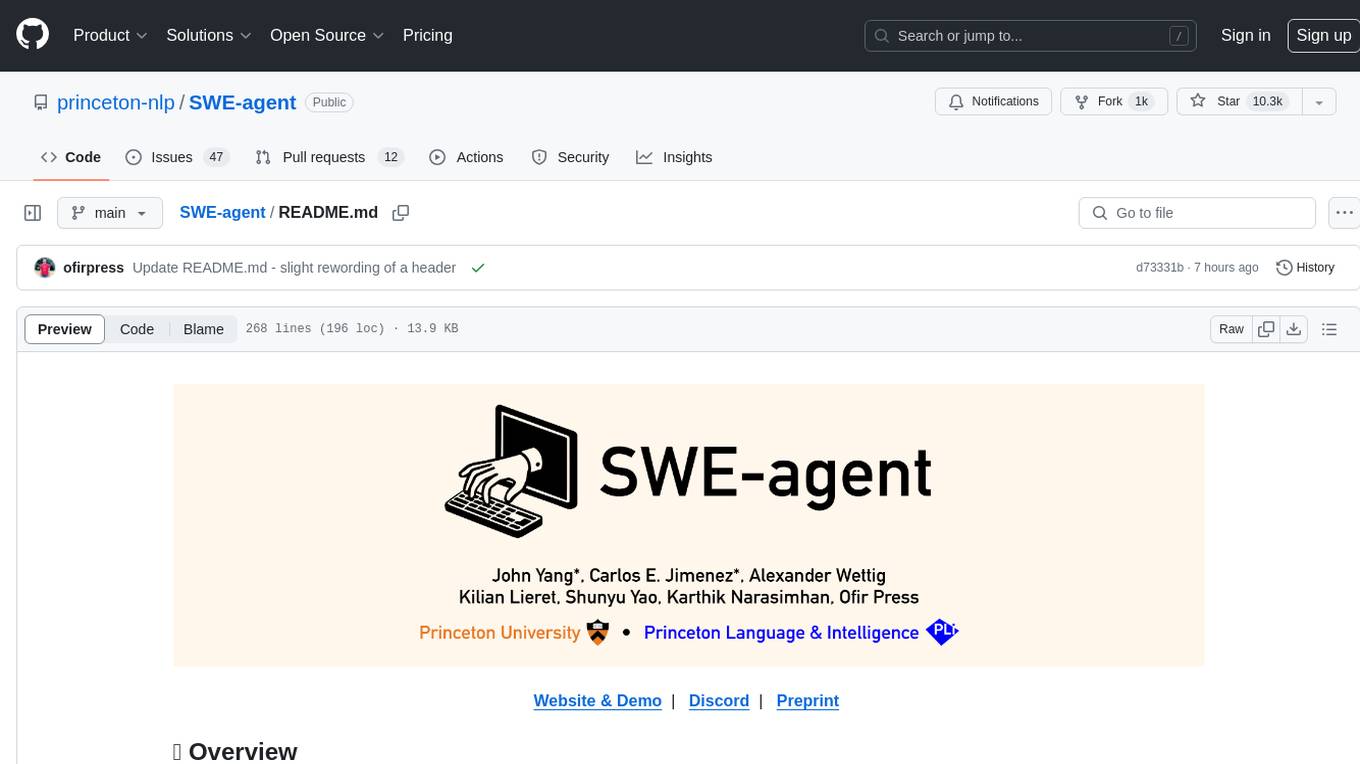
SWE-agent
SWE-agent is a tool that turns language models (e.g. GPT-4) into software engineering agents capable of fixing bugs and issues in real GitHub repositories. It achieves state-of-the-art performance on the full test set by resolving 12.29% of issues. The tool is built and maintained by researchers from Princeton University. SWE-agent provides a command line tool and a graphical web interface for developers to interact with. It introduces an Agent-Computer Interface (ACI) to facilitate browsing, viewing, editing, and executing code files within repositories. The tool includes features such as a linter for syntax checking, a specialized file viewer, and a full-directory string searching command to enhance the agent's capabilities. SWE-agent aims to improve prompt engineering and ACI design to enhance the performance of language models in software engineering tasks.
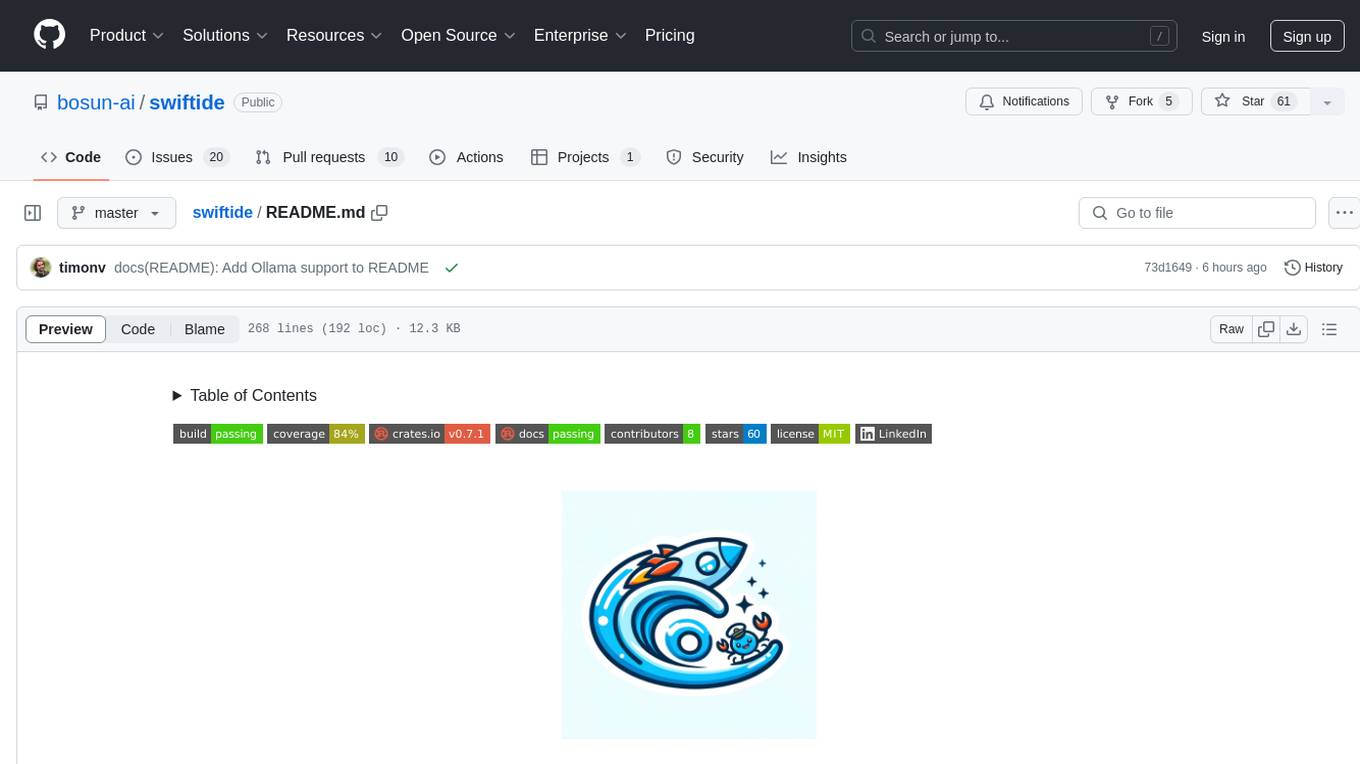
swiftide
Swiftide is a fast, streaming indexing and query library tailored for Retrieval Augmented Generation (RAG) in AI applications. It is built in Rust, utilizing parallel, asynchronous streams for blazingly fast performance. With Swiftide, users can easily build AI applications from idea to production in just a few lines of code. The tool addresses frustrations around performance, stability, and ease of use encountered while working with Python-based tooling. It offers features like fast streaming indexing pipeline, experimental query pipeline, integrations with various platforms, loaders, transformers, chunkers, embedders, and more. Swiftide aims to provide a platform for data indexing and querying to advance the development of automated Large Language Model (LLM) applications.
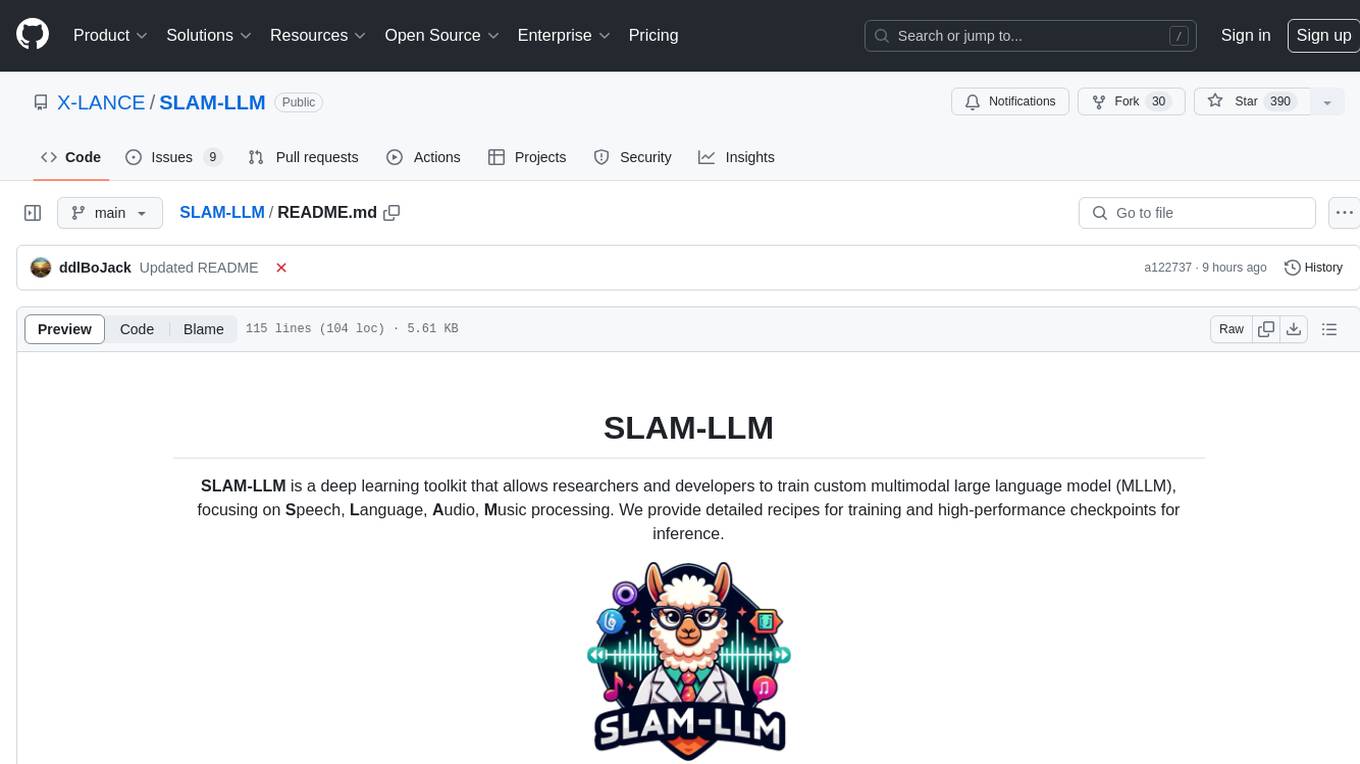
SLAM-LLM
SLAM-LLM is a deep learning toolkit for training custom multimodal large language models (MLLM) focusing on speech, language, audio, and music processing. It provides detailed recipes for training and high-performance checkpoints for inference. The toolkit supports various tasks such as automatic speech recognition (ASR), text-to-speech (TTS), visual speech recognition (VSR), automated audio captioning (AAC), spatial audio understanding, and music caption (MC). Users can easily extend to new models and tasks, utilize mixed precision training for faster training with less GPU memory, and perform multi-GPU training with data and model parallelism. Configuration is flexible based on Hydra and dataclass, allowing different configuration methods.
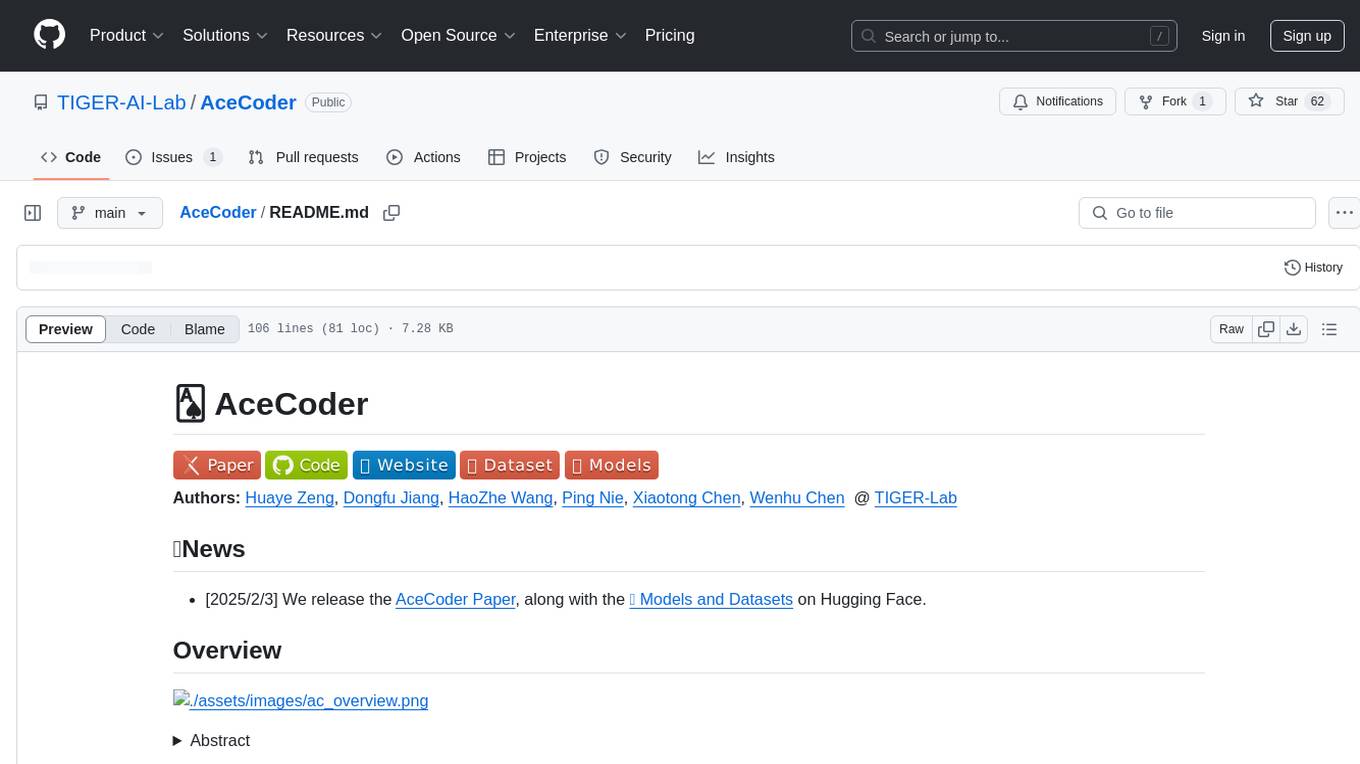
AceCoder
AceCoder is a tool that introduces a fully automated pipeline for synthesizing large-scale reliable tests used for reward model training and reinforcement learning in the coding scenario. It curates datasets, trains reward models, and performs RL training to improve coding abilities of language models. The tool aims to unlock the potential of RL training for code generation models and push the boundaries of LLM's coding abilities.
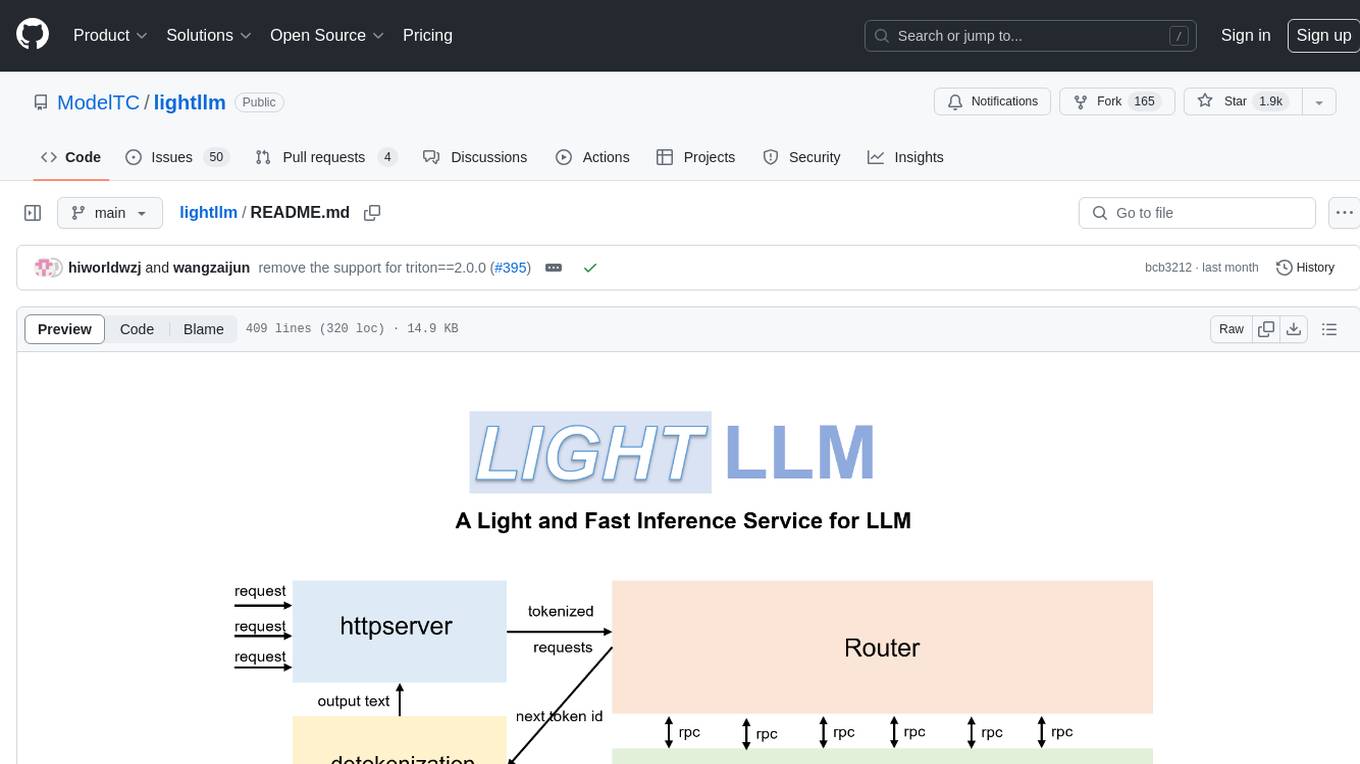
lightllm
LightLLM is a Python-based LLM (Large Language Model) inference and serving framework known for its lightweight design, scalability, and high-speed performance. It offers features like tri-process asynchronous collaboration, Nopad for efficient attention operations, dynamic batch scheduling, FlashAttention integration, tensor parallelism, Token Attention for zero memory waste, and Int8KV Cache. The tool supports various models like BLOOM, LLaMA, StarCoder, Qwen-7b, ChatGLM2-6b, Baichuan-7b, Baichuan2-7b, Baichuan2-13b, InternLM-7b, Yi-34b, Qwen-VL, Llava-7b, Mixtral, Stablelm, and MiniCPM. Users can deploy and query models using the provided server launch commands and interact with multimodal models like QWen-VL and Llava using specific queries and images.
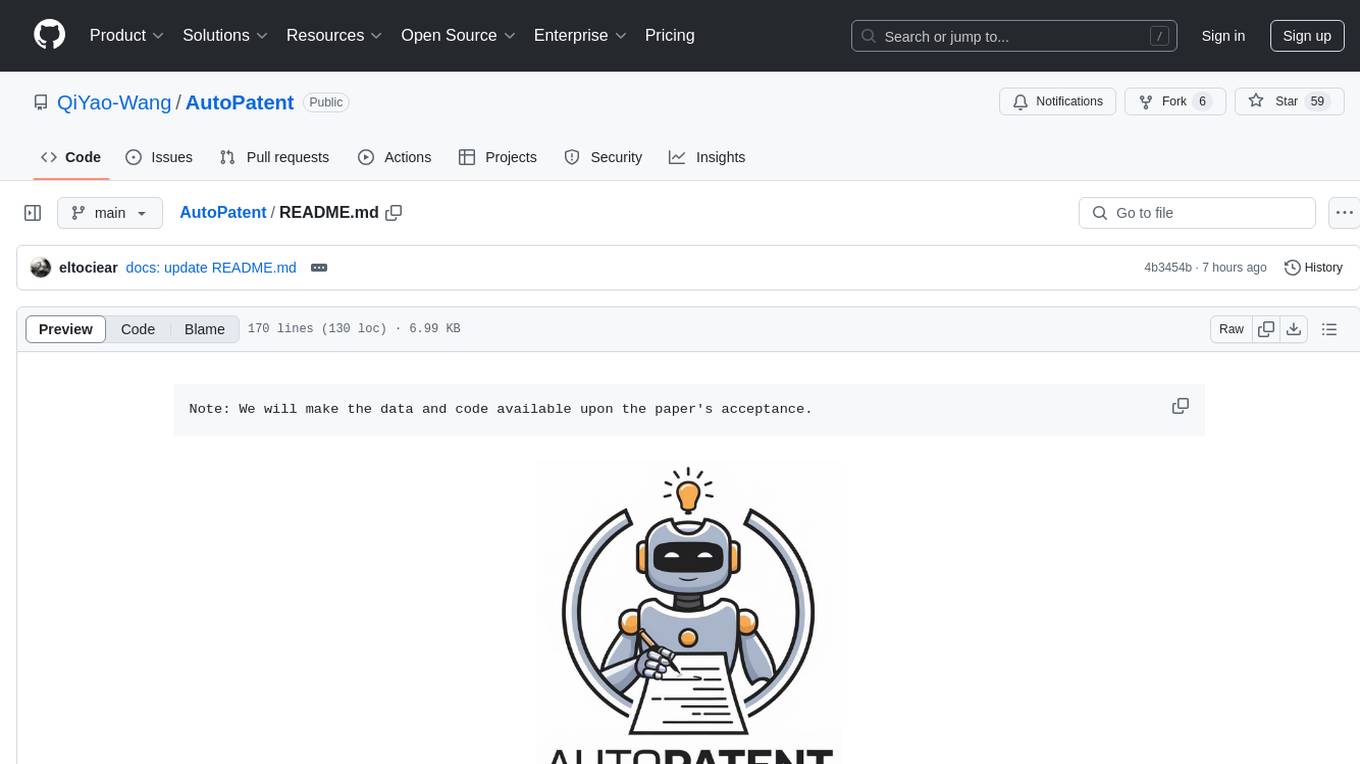
AutoPatent
AutoPatent is a multi-agent framework designed for automatic patent generation. It challenges large language models to generate full-length patents based on initial drafts. The framework leverages planner, writer, and examiner agents along with PGTree and RRAG to craft lengthy, intricate, and high-quality patent documents. It introduces a new metric, IRR (Inverse Repetition Rate), to measure sentence repetition within patents. The tool aims to streamline the patent generation process by automating the creation of detailed and specialized patent documents.
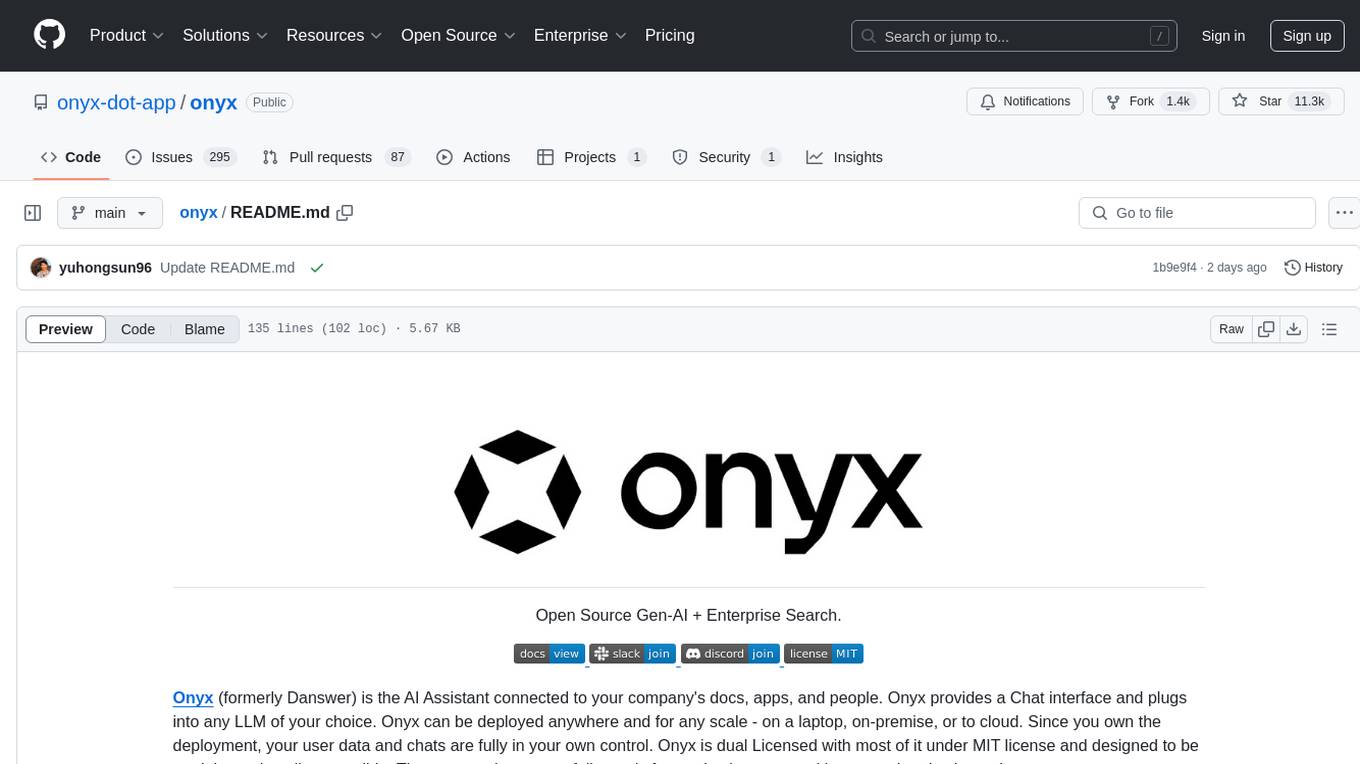
onyx
Onyx is an open-source Gen-AI and Enterprise Search tool that serves as an AI Assistant connected to company documents, apps, and people. It provides a chat interface, can be deployed anywhere, and offers features like user authentication, role management, chat persistence, and UI for configuring AI Assistants. Onyx acts as an Enterprise Search tool across various workplace platforms, enabling users to access team-specific knowledge and perform tasks like document search, AI answers for natural language queries, and integration with common workplace tools like Slack, Google Drive, Confluence, etc.
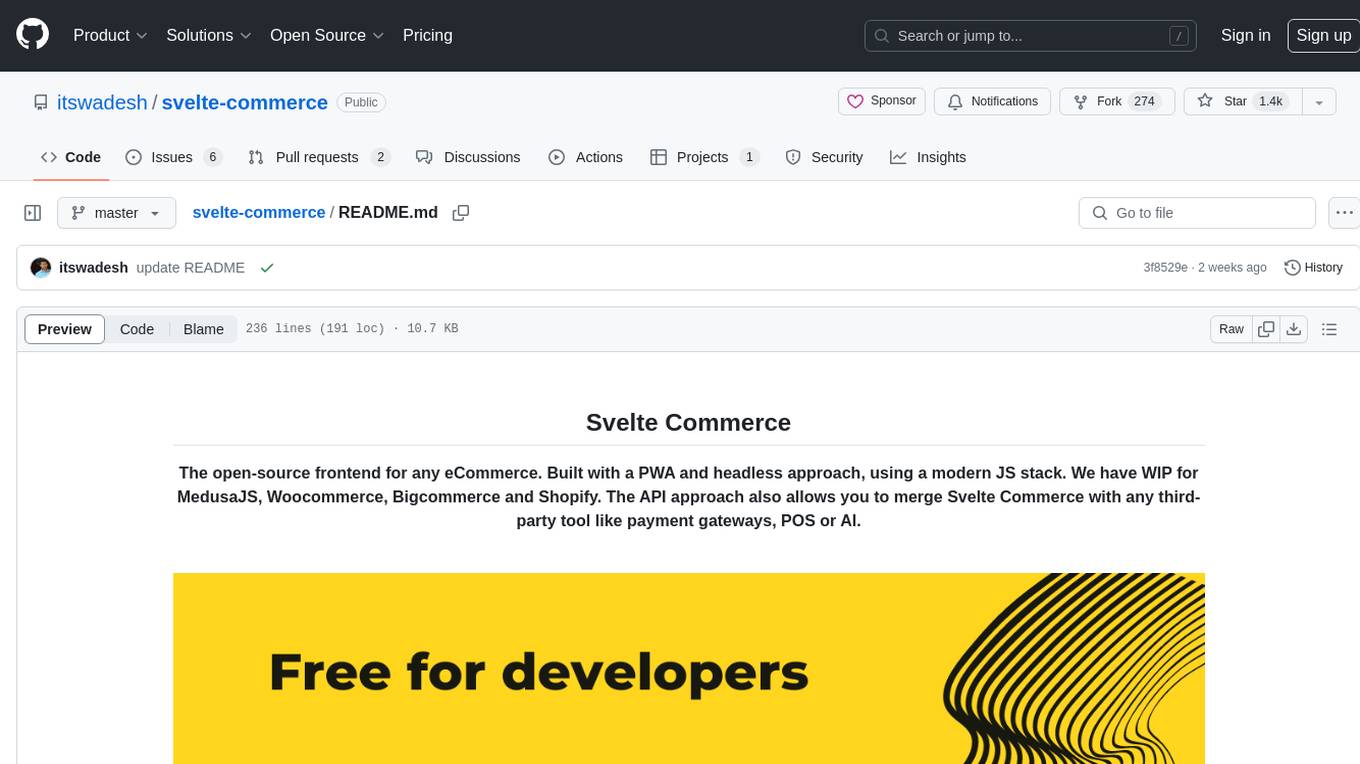
svelte-commerce
Svelte Commerce is an open-source frontend for eCommerce, utilizing a PWA and headless approach with a modern JS stack. It supports integration with various eCommerce backends like MedusaJS, Woocommerce, Bigcommerce, and Shopify. The API flexibility allows seamless connection with third-party tools such as payment gateways, POS systems, and AI services. Svelte Commerce offers essential eCommerce features, is both SSR and SPA, superfast, and free to download and modify. Users can easily deploy it on Netlify or Vercel with zero configuration. The tool provides features like headless commerce, authentication, cart & checkout, TailwindCSS styling, server-side rendering, proxy + API integration, animations, lazy loading, search functionality, faceted filters, and more.
For similar tasks
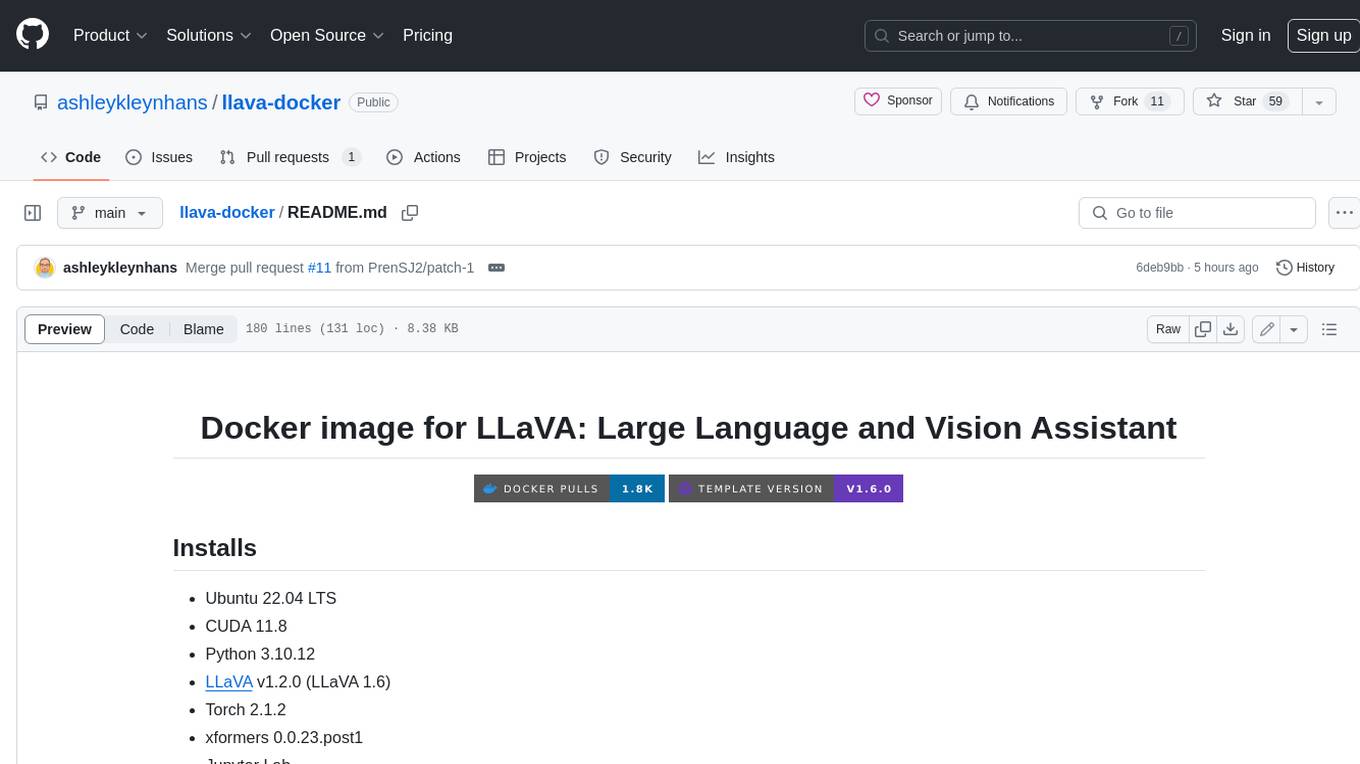
llava-docker
This Docker image for LLaVA (Large Language and Vision Assistant) provides a convenient way to run LLaVA locally or on RunPod. LLaVA is a powerful AI tool that combines natural language processing and computer vision capabilities. With this Docker image, you can easily access LLaVA's functionalities for various tasks, including image captioning, visual question answering, text summarization, and more. The image comes pre-installed with LLaVA v1.2.0, Torch 2.1.2, xformers 0.0.23.post1, and other necessary dependencies. You can customize the model used by setting the MODEL environment variable. The image also includes a Jupyter Lab environment for interactive development and exploration. Overall, this Docker image offers a comprehensive and user-friendly platform for leveraging LLaVA's capabilities.
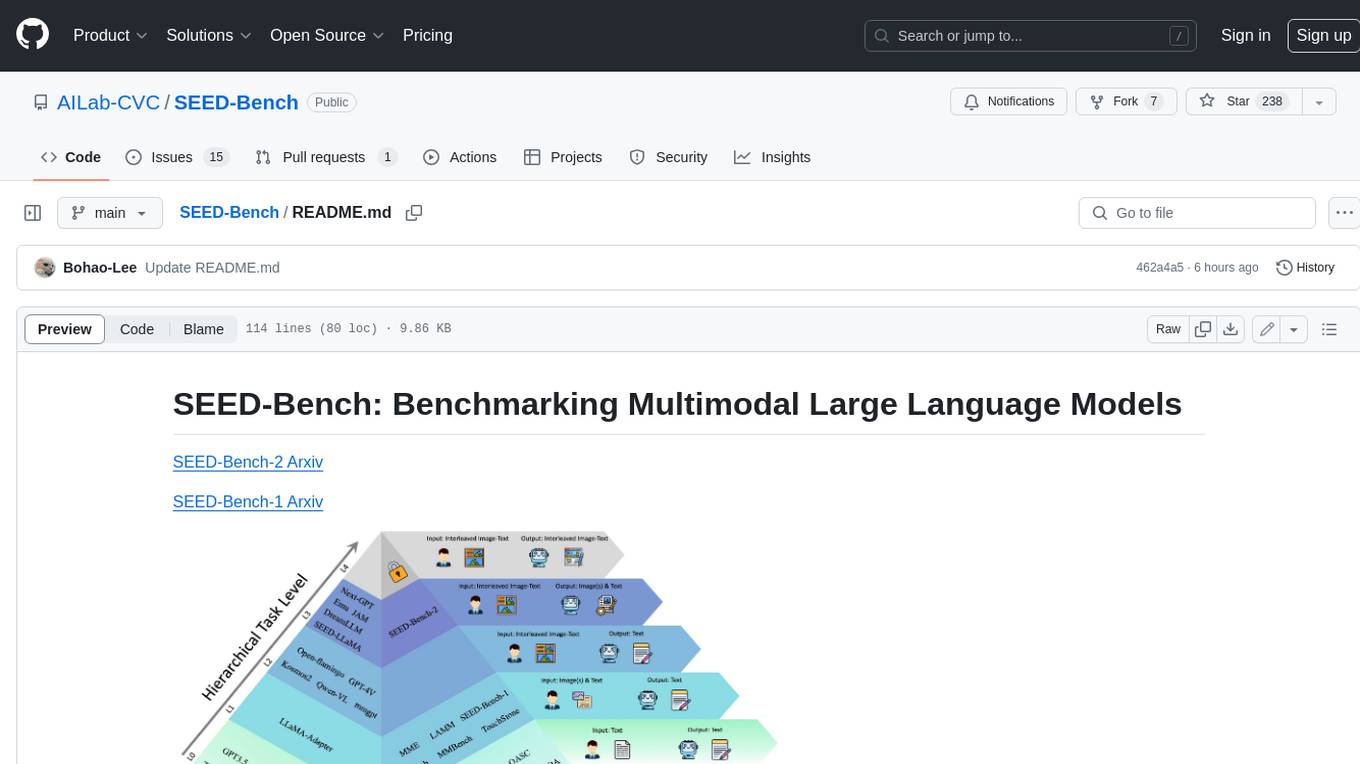
SEED-Bench
SEED-Bench is a comprehensive benchmark for evaluating the performance of multimodal large language models (LLMs) on a wide range of tasks that require both text and image understanding. It consists of two versions: SEED-Bench-1 and SEED-Bench-2. SEED-Bench-1 focuses on evaluating the spatial and temporal understanding of LLMs, while SEED-Bench-2 extends the evaluation to include text and image generation tasks. Both versions of SEED-Bench provide a diverse set of tasks that cover different aspects of multimodal understanding, making it a valuable tool for researchers and practitioners working on LLMs.
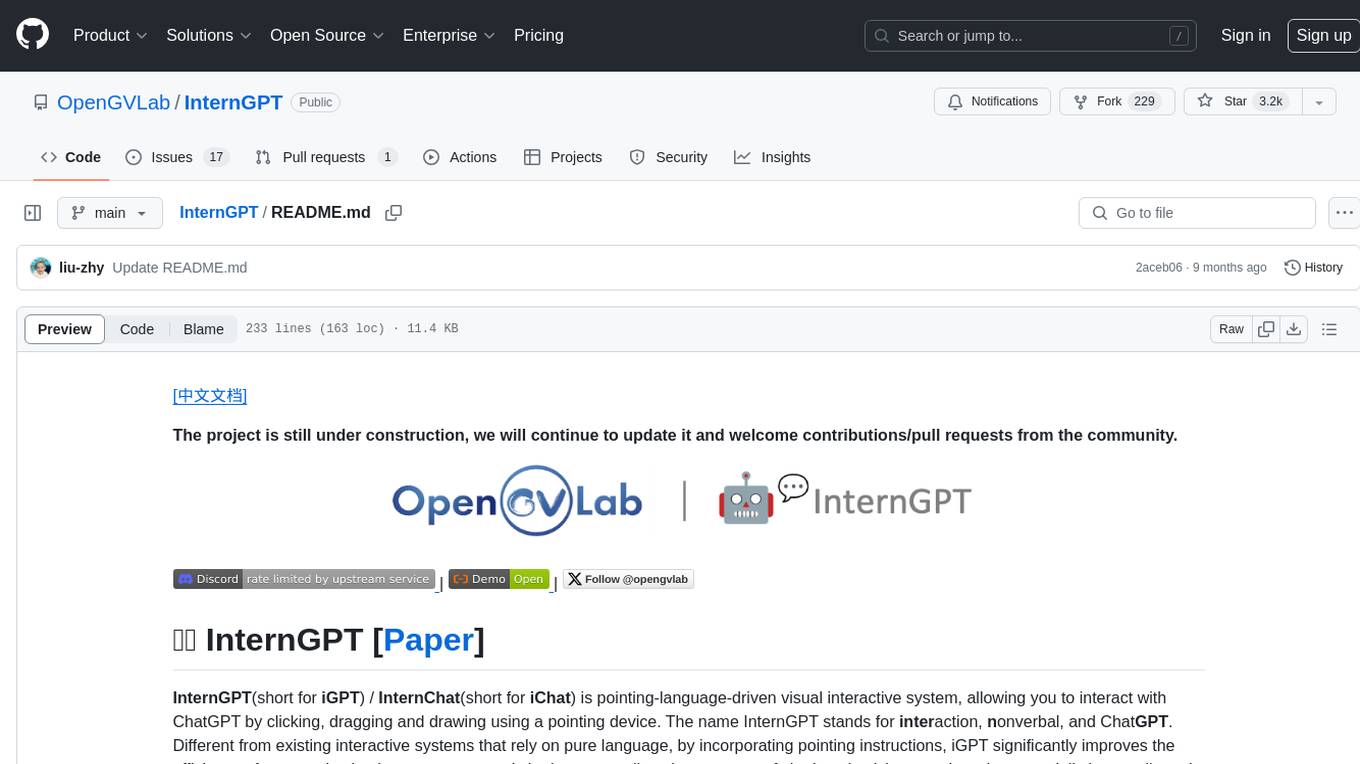
InternGPT
InternGPT (iGPT) is a pointing-language-driven visual interactive system that enhances communication between users and chatbots by incorporating pointing instructions. It improves chatbot accuracy in vision-centric tasks, especially in complex visual scenarios. The system includes an auxiliary control mechanism to enhance the control capability of the language model. InternGPT features a large vision-language model called Husky, fine-tuned for high-quality multi-modal dialogue. Users can interact with ChatGPT by clicking, dragging, and drawing using a pointing device, leading to efficient communication and improved chatbot performance in vision-related tasks.
For similar jobs
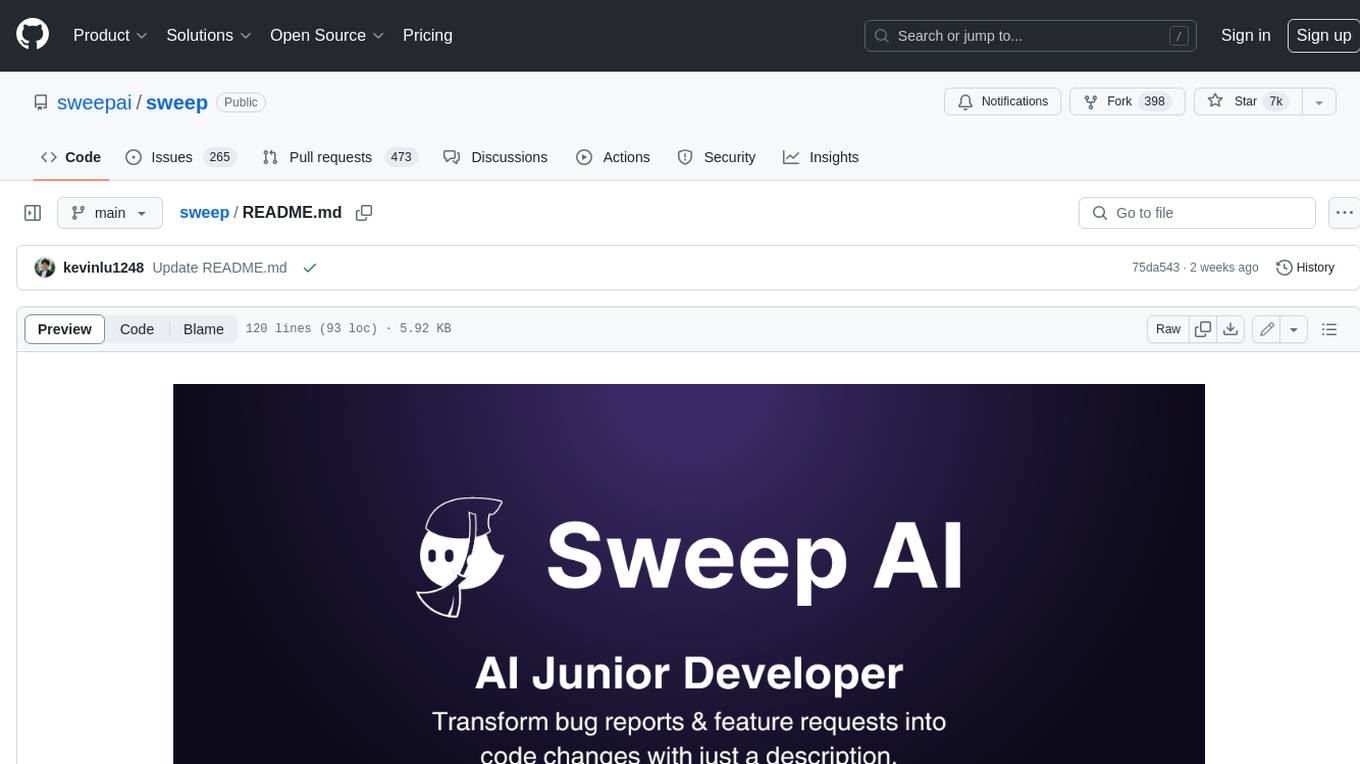
sweep
Sweep is an AI junior developer that turns bugs and feature requests into code changes. It automatically handles developer experience improvements like adding type hints and improving test coverage.
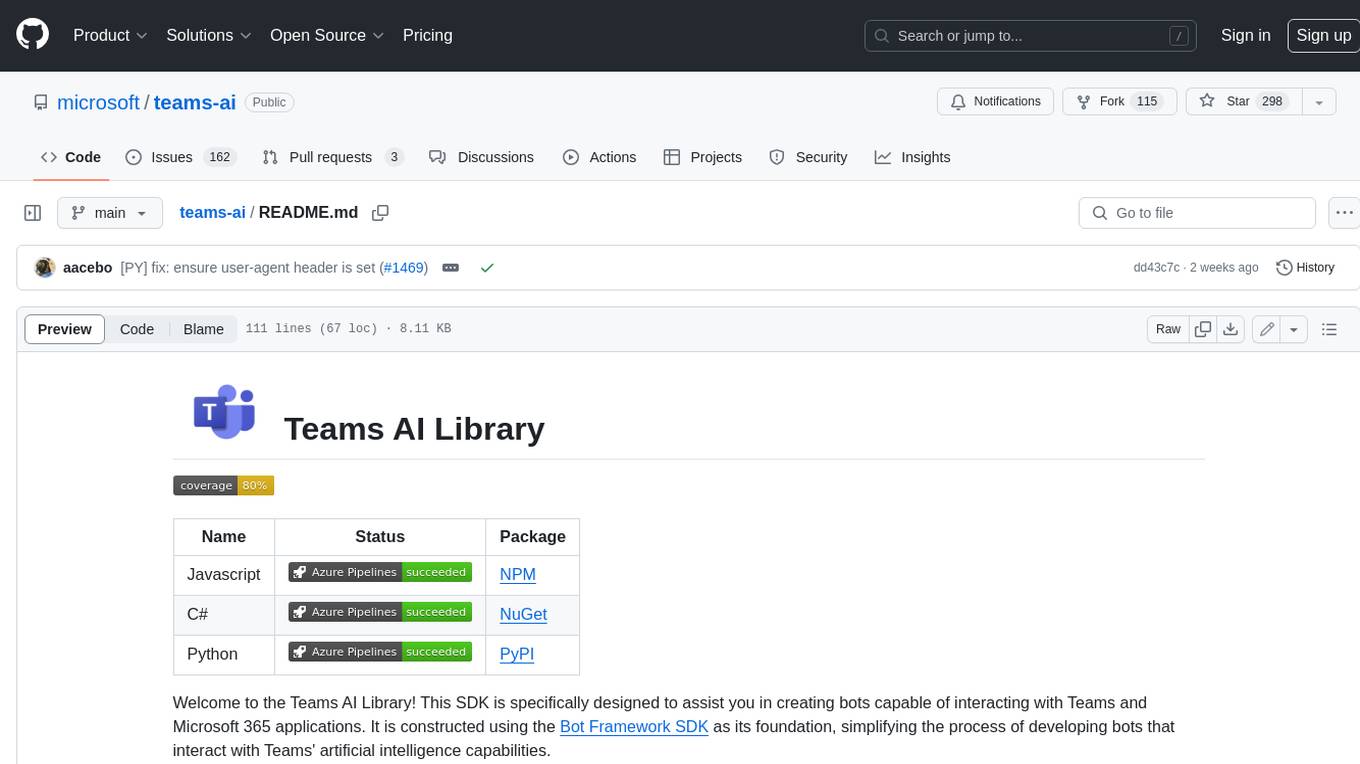
teams-ai
The Teams AI Library is a software development kit (SDK) that helps developers create bots that can interact with Teams and Microsoft 365 applications. It is built on top of the Bot Framework SDK and simplifies the process of developing bots that interact with Teams' artificial intelligence capabilities. The SDK is available for JavaScript/TypeScript, .NET, and Python.
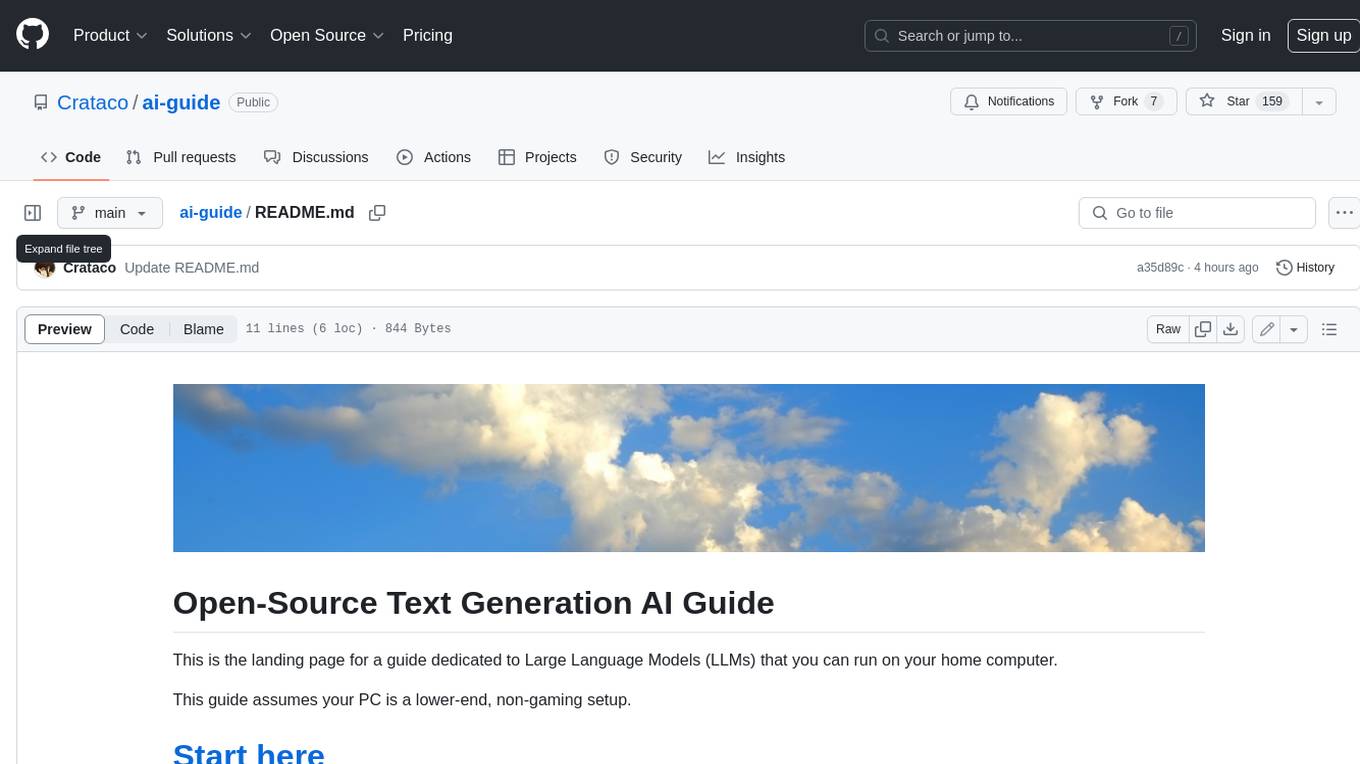
ai-guide
This guide is dedicated to Large Language Models (LLMs) that you can run on your home computer. It assumes your PC is a lower-end, non-gaming setup.
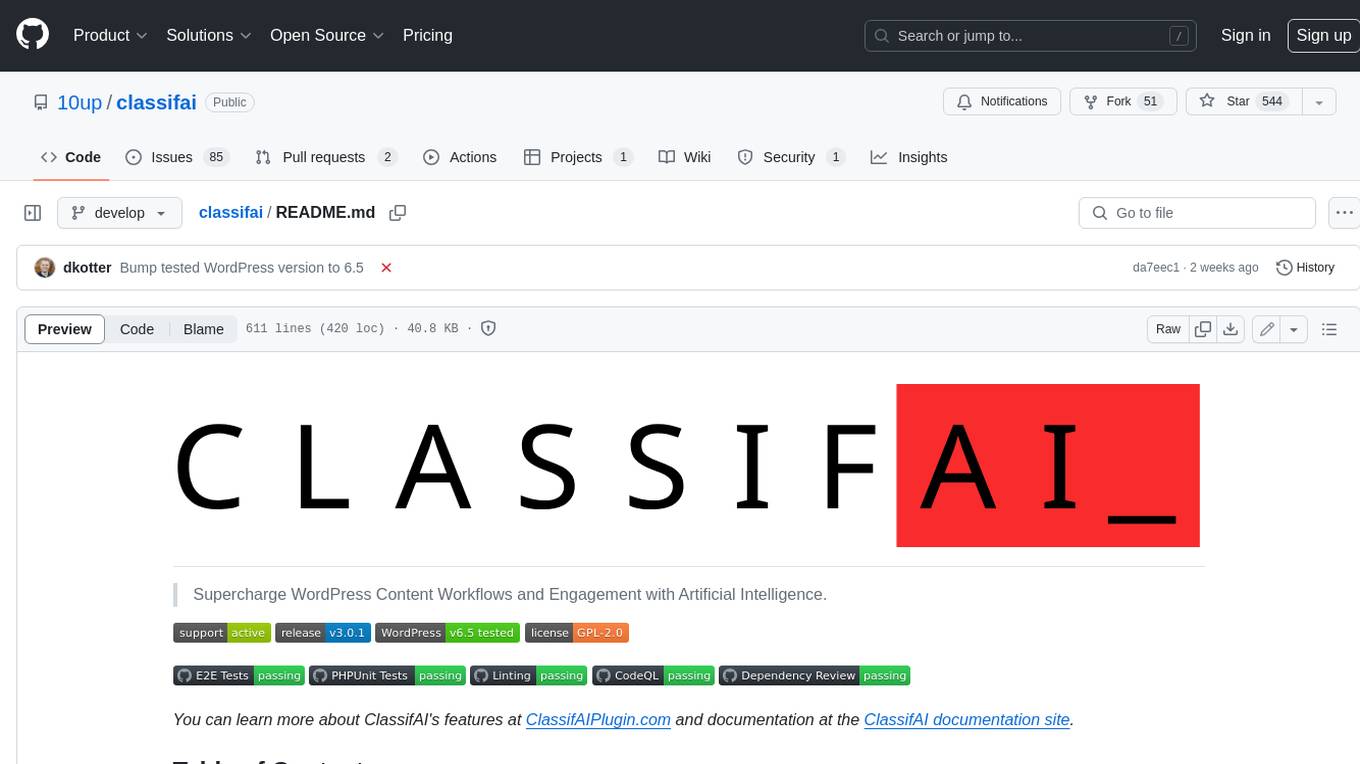
classifai
Supercharge WordPress Content Workflows and Engagement with Artificial Intelligence. Tap into leading cloud-based services like OpenAI, Microsoft Azure AI, Google Gemini and IBM Watson to augment your WordPress-powered websites. Publish content faster while improving SEO performance and increasing audience engagement. ClassifAI integrates Artificial Intelligence and Machine Learning technologies to lighten your workload and eliminate tedious tasks, giving you more time to create original content that matters.
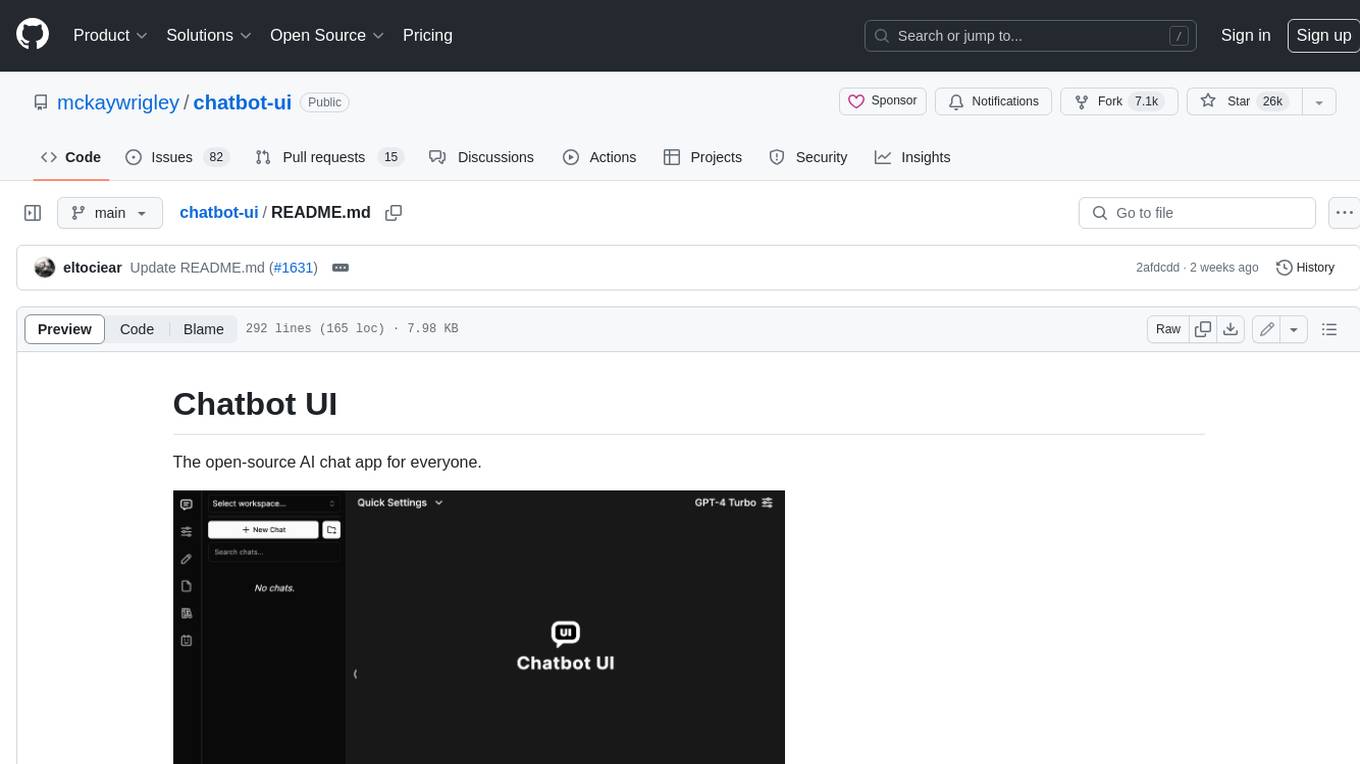
chatbot-ui
Chatbot UI is an open-source AI chat app that allows users to create and deploy their own AI chatbots. It is easy to use and can be customized to fit any need. Chatbot UI is perfect for businesses, developers, and anyone who wants to create a chatbot.
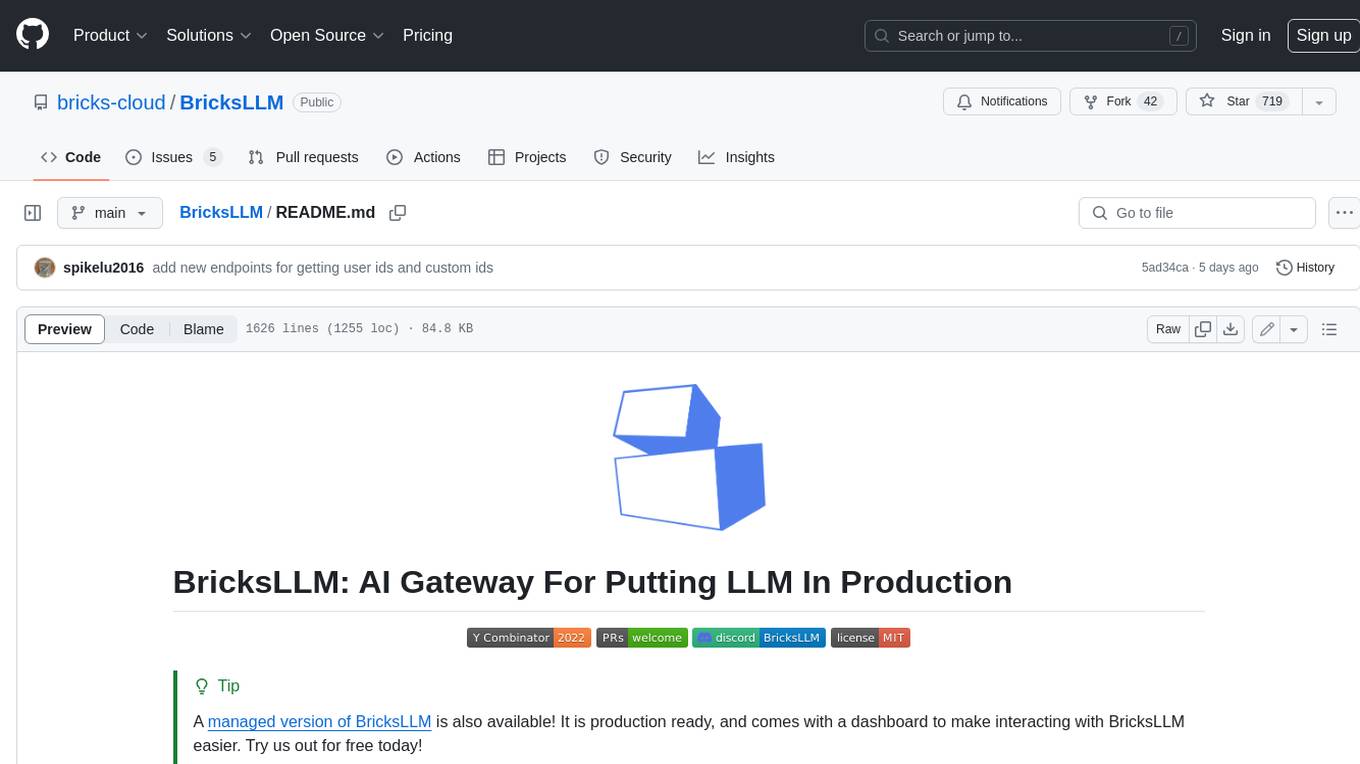
BricksLLM
BricksLLM is a cloud native AI gateway written in Go. Currently, it provides native support for OpenAI, Anthropic, Azure OpenAI and vLLM. BricksLLM aims to provide enterprise level infrastructure that can power any LLM production use cases. Here are some use cases for BricksLLM: * Set LLM usage limits for users on different pricing tiers * Track LLM usage on a per user and per organization basis * Block or redact requests containing PIIs * Improve LLM reliability with failovers, retries and caching * Distribute API keys with rate limits and cost limits for internal development/production use cases * Distribute API keys with rate limits and cost limits for students
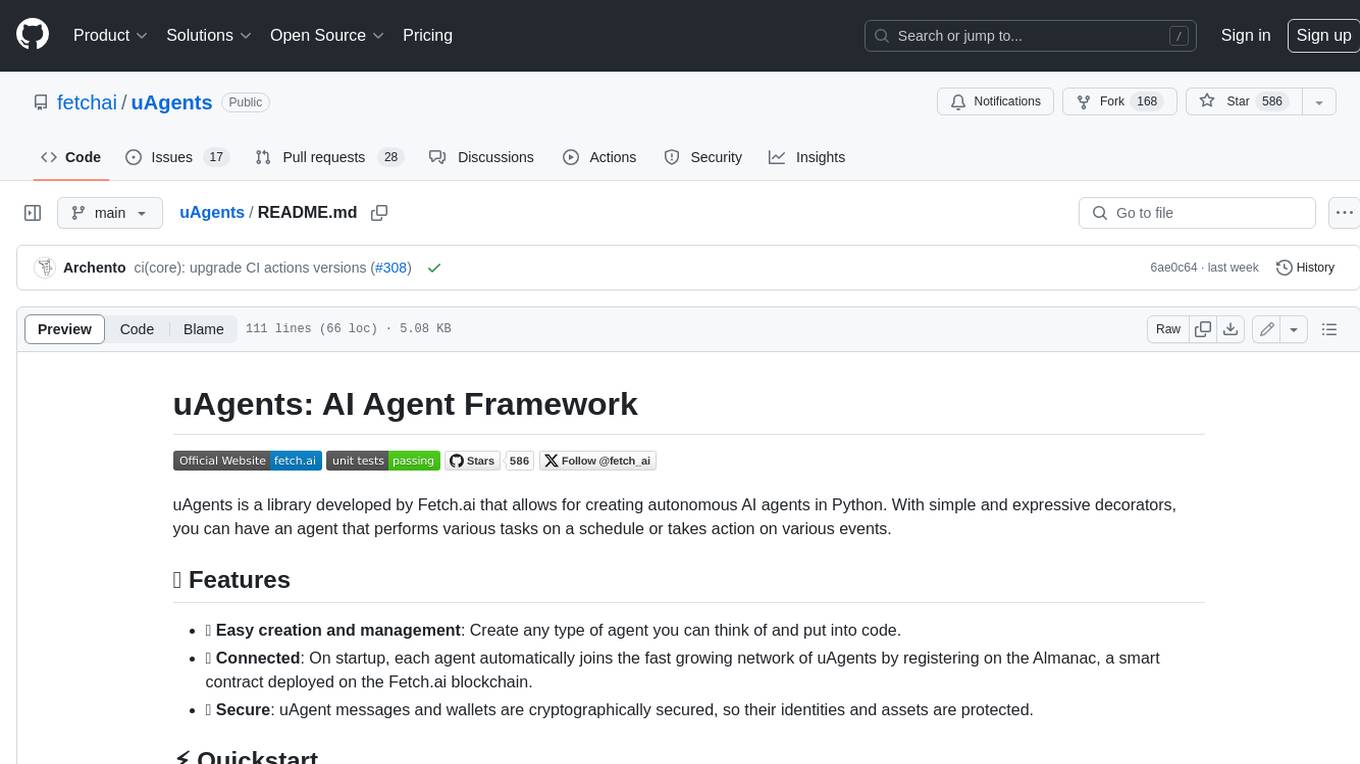
uAgents
uAgents is a Python library developed by Fetch.ai that allows for the creation of autonomous AI agents. These agents can perform various tasks on a schedule or take action on various events. uAgents are easy to create and manage, and they are connected to a fast-growing network of other uAgents. They are also secure, with cryptographically secured messages and wallets.
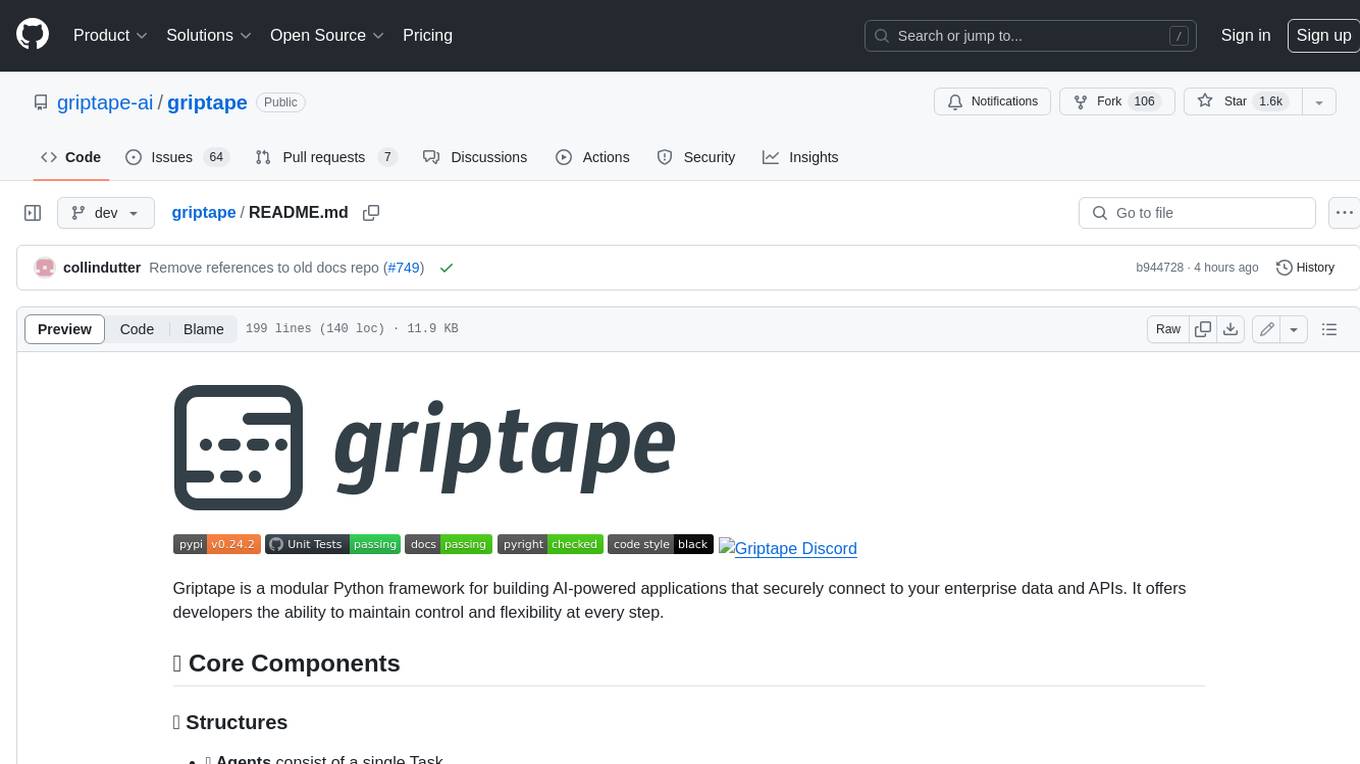
griptape
Griptape is a modular Python framework for building AI-powered applications that securely connect to your enterprise data and APIs. It offers developers the ability to maintain control and flexibility at every step. Griptape's core components include Structures (Agents, Pipelines, and Workflows), Tasks, Tools, Memory (Conversation Memory, Task Memory, and Meta Memory), Drivers (Prompt and Embedding Drivers, Vector Store Drivers, Image Generation Drivers, Image Query Drivers, SQL Drivers, Web Scraper Drivers, and Conversation Memory Drivers), Engines (Query Engines, Extraction Engines, Summary Engines, Image Generation Engines, and Image Query Engines), and additional components (Rulesets, Loaders, Artifacts, Chunkers, and Tokenizers). Griptape enables developers to create AI-powered applications with ease and efficiency.