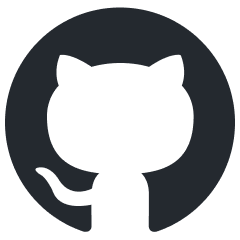
OpenNARS-for-Applications
General reasoning component for applications based on NARS theory.
Stars: 93
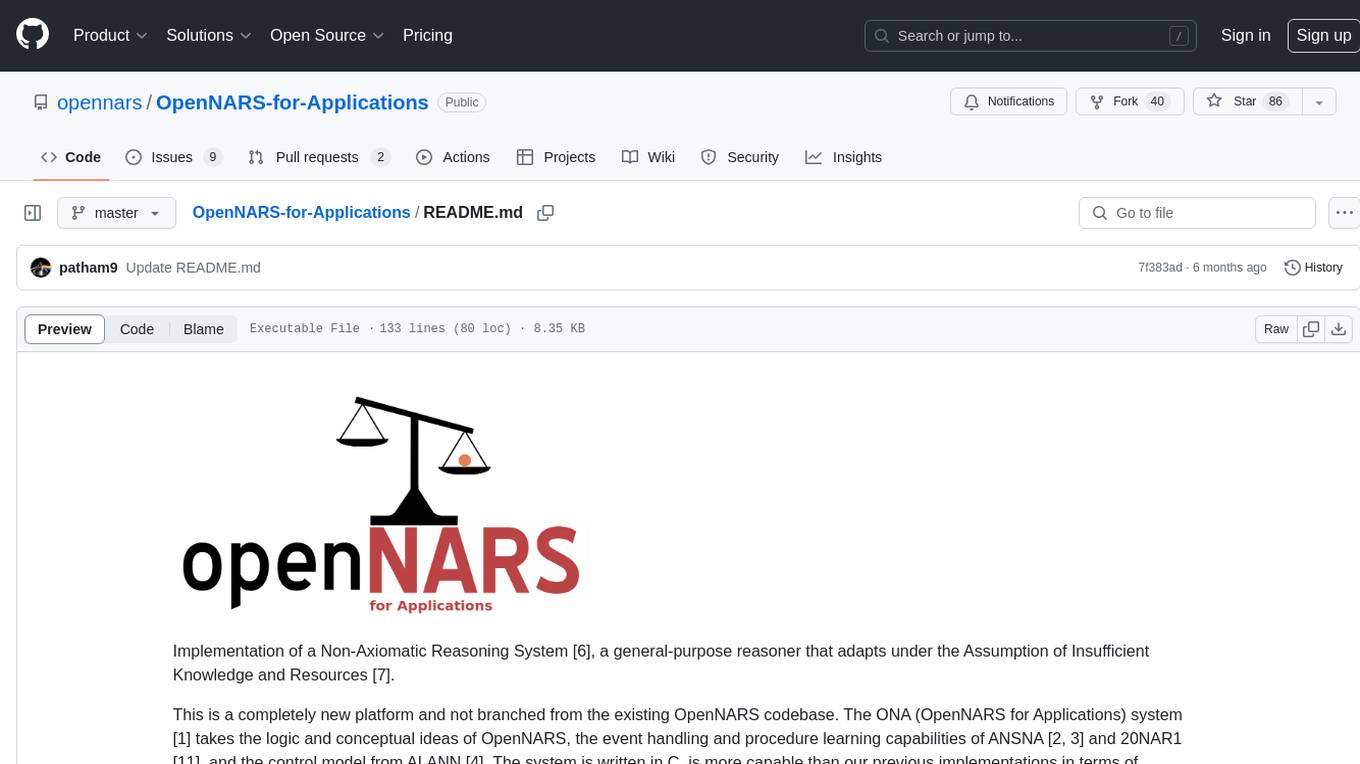
OpenNARS-for-Applications is an implementation of a Non-Axiomatic Reasoning System, a general-purpose reasoner that adapts under the Assumption of Insufficient Knowledge and Resources. The system combines the logic and conceptual ideas of OpenNARS, event handling and procedure learning capabilities of ANSNA and 20NAR1, and the control model from ALANN. It is written in C, offers improved reasoning performance, and has been compared with Reinforcement Learning and means-end reasoning approaches. The system has been used in real-world applications such as assisting first responders, real-time traffic surveillance, and experiments with autonomous robots. It has been developed with a pragmatic mindset focusing on effective implementation of existing theory.
README:
Implementation of a Non-Axiomatic Reasoning System [6], a general-purpose reasoner that adapts under the Assumption of Insufficient Knowledge and Resources [7].
This is a completely new platform and not branched from the existing OpenNARS codebase. The ONA (OpenNARS for Applications) system [1] takes the logic and conceptual ideas of OpenNARS, the event handling and procedure learning capabilities of ANSNA [2, 3] and 20NAR1 [11], and the control model from ALANN [4]. The system is written in C, is more capable than our previous implementations in terms of reasoning performance, and has also been experimentally compared with Reinforcement Learning [5, 6] and means-end reasoning approaches such as BDI models [6]. Additionally, it has become the core reasoning component of a system assisting first responders (Trusted and explainable Artificial Intelligence for Saving Lives, [6]) while driving and completing their mission. This was done in cooperation with NASA Jet Propulsion Laboratory. Also it has been tried for real-time traffic surveillance in cooperation with Cisco Systems [7]. Last, initial experiments for using the system for autonomous robots have been carried out [6], and more is yet to come.
The ONA implementation has been developed with a pragmatic mindset. The focus on the design has been to implement the 'existing' theory [8, 9] as effectively as possible and make firm decisions rather than keep as many options open as possible. This has led to some small conceptual differences to OpenNARS [10] which was developed for research purposes.
Video tutorials and demo videos can be found here: Video tutorials Or click on the picture to watch the newest summary videos (summary and demo):
Procedure learning demos (variants of Pong and Space Invaders, Test Chamber, Cartpole, food collecting agent, ...): https://www.youtube.com/watch?v=oyQ250H5owE
How to clone and compile (tested with GCC and Clang for x64, x86 and ARM):
git clone https://github.com/opennars/OpenNARS-for-Applications
cd OpenNARS-for-Applications
./build.sh
Additionally the parameter -DHARDENED can be passed to build.sh to end up with a slimmer system without language learning abilities.
How to set the amount of threads the system should run with: (to be tested more, compile with ./build.sh -fopenmp)
export OMP_NUM_THREADS=4 // 4 threads seems to be the sweet spot. More threads leads to more contention and less speed currently
If you have trouble building with OpenMP, then you probably need to specify library (and / or sources) directory alongside the -fopenmp
option, like -L<path to your openmp>
or -I<path to your openmp>
.
How to run the interactive Narsese shell:
./NAR shell
with syntax highlighting:
./NAR shell | python3 colorize.py
For a proper reliable GPT-based English language channel
Check out NARS-GPT !
with legacy English NLP shell and syntax highlighting:
python3 english_to_narsese.py | ./NAR shell | python3 colorize.py
How to run the C tests and then receive instructions how to run the current example programs:
./NAR
How to run all C tests, and all Narsese and English examples as integration tests, and collect metrics across all examples:
python3 evaluation.py
For the current output, see Evaluation results
How to run an example file:
Narsese:
./NAR shell < ./examples/nal/example1.nal
English: (tested with NLTK v3.4.5, v3.5)
python3 english_to_narsese.py < ./examples/english/story1.english | ./NAR shell
How to run an UDPNAR:
./NAR UDPNAR IP PORT timestep(ns per cycle) printDerivations
./NAR UDPNAR 127.0.0.1 50000 10000000 true
where the output can be logged simply by appending
> output.log
How to reach us:
Real-time team chat: #nars IRC channel @ libera.chat, #nars:matrix.org (accessible via Riot.im)
Google discussion group: https://groups.google.com/forum/#!forum/open-nars
Acknowledgement
Over the years, research and development on this reasoning system has been funded by Digital Futures, Cisco and NASA Jet Propulsion Laboratory.
References
[1] Hammer, P., & Lofthouse, T. (2020, September). ‘OpenNARS for Applications’: Architecture and Control. In International Conference on Artificial General Intelligence (pp. 193-204). Springer, Cham.
[2] Hammer, P. (2019, August). Adaptive Neuro-Symbolic Network Agent. In International Conference on Artificial General Intelligence (pp. 80-90). Springer, Cham.
[3] Hammer, P., & Lofthouse, T. (2018, August). Goal-directed procedure learning. In International Conference on Artificial General Intelligence (pp. 77-86). Springer, Cham.
[4] Lofthouse, T. (2019). ALANN: An event driven control mechanism for a non-axiomatic reasoning system (NARS). NARS2019 workshop at AGI 2019.
[5] Eberding, L. M., Thórisson, K. R., Sheikhlar, A., & Andrason, S. P. (2020). SAGE: Task-Environment Platform for Evaluating a Broad Range of AI Learners. In Artificial General Intelligence: 13th International Conference, AGI 2020, St. Petersburg, Russia, September 16–19, 2020, Proceedings (Vol. 12177, p. 72). Springer Nature.
[6] Hammer, P. (2021, July). Autonomy through real-time learning and OpenNARS for Applications. PhD thesis at Department of Computer and Information Sciences, Temple Universitiy
[7] Hammer, P., Lofthouse, T., Fenoglio, E., Latapie, H., & Wang, P. (2020, September). A reasoning based model for anomaly detection in the Smart City domain. In Proceedings of SAI Intelligent Systems Conference (pp. 144-159). Springer, Cham.
[8] Wang, P. (2013). Non-axiomatic logic: A model of intelligent reasoning. World Scientific.
[9] Wang, P. (2009, October). Insufficient Knowledge and Resources-A Biological Constraint and Its Functional Implications. In AAAI Fall Symposium: Biologically Inspired Cognitive Architectures.
[10] Hammer, P., Lofthouse, T., & Wang, P. (2016, July). The OpenNARS implementation of the non-axiomatic reasoning system. In International conference on artificial general intelligence (pp. 160-170). Springer, Cham.
[11] Wünsche, R. (2021, October). 20NAR1-An Alternative NARS Implementation Design. In International Conference on Artificial General Intelligence (pp. 283-291). Springer, Cham.
For Tasks:
Click tags to check more tools for each tasksFor Jobs:
Alternative AI tools for OpenNARS-for-Applications
Similar Open Source Tools
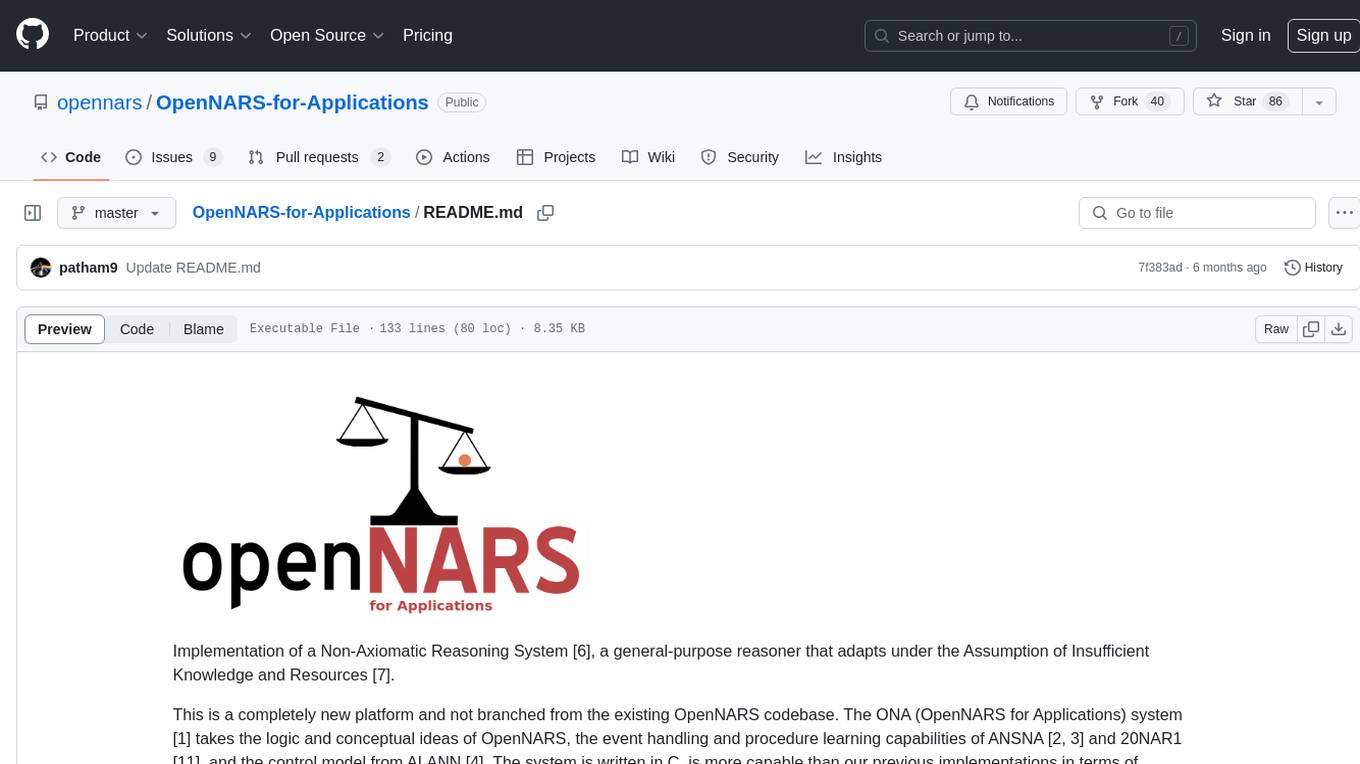
OpenNARS-for-Applications
OpenNARS-for-Applications is an implementation of a Non-Axiomatic Reasoning System, a general-purpose reasoner that adapts under the Assumption of Insufficient Knowledge and Resources. The system combines the logic and conceptual ideas of OpenNARS, event handling and procedure learning capabilities of ANSNA and 20NAR1, and the control model from ALANN. It is written in C, offers improved reasoning performance, and has been compared with Reinforcement Learning and means-end reasoning approaches. The system has been used in real-world applications such as assisting first responders, real-time traffic surveillance, and experiments with autonomous robots. It has been developed with a pragmatic mindset focusing on effective implementation of existing theory.
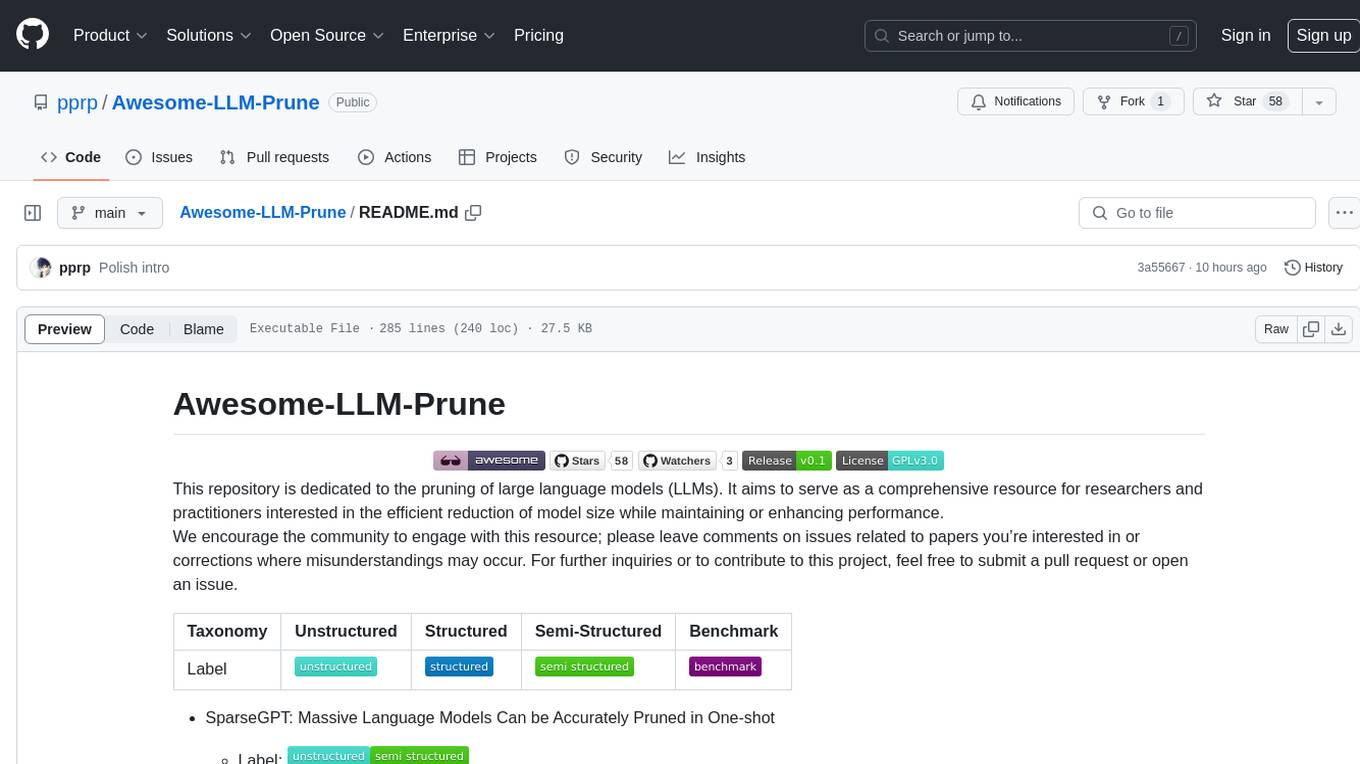
Awesome-LLM-Prune
This repository is dedicated to the pruning of large language models (LLMs). It aims to serve as a comprehensive resource for researchers and practitioners interested in the efficient reduction of model size while maintaining or enhancing performance. The repository contains various papers, summaries, and links related to different pruning approaches for LLMs, along with author information and publication details. It covers a wide range of topics such as structured pruning, unstructured pruning, semi-structured pruning, and benchmarking methods. Researchers and practitioners can explore different pruning techniques, understand their implications, and access relevant resources for further study and implementation.
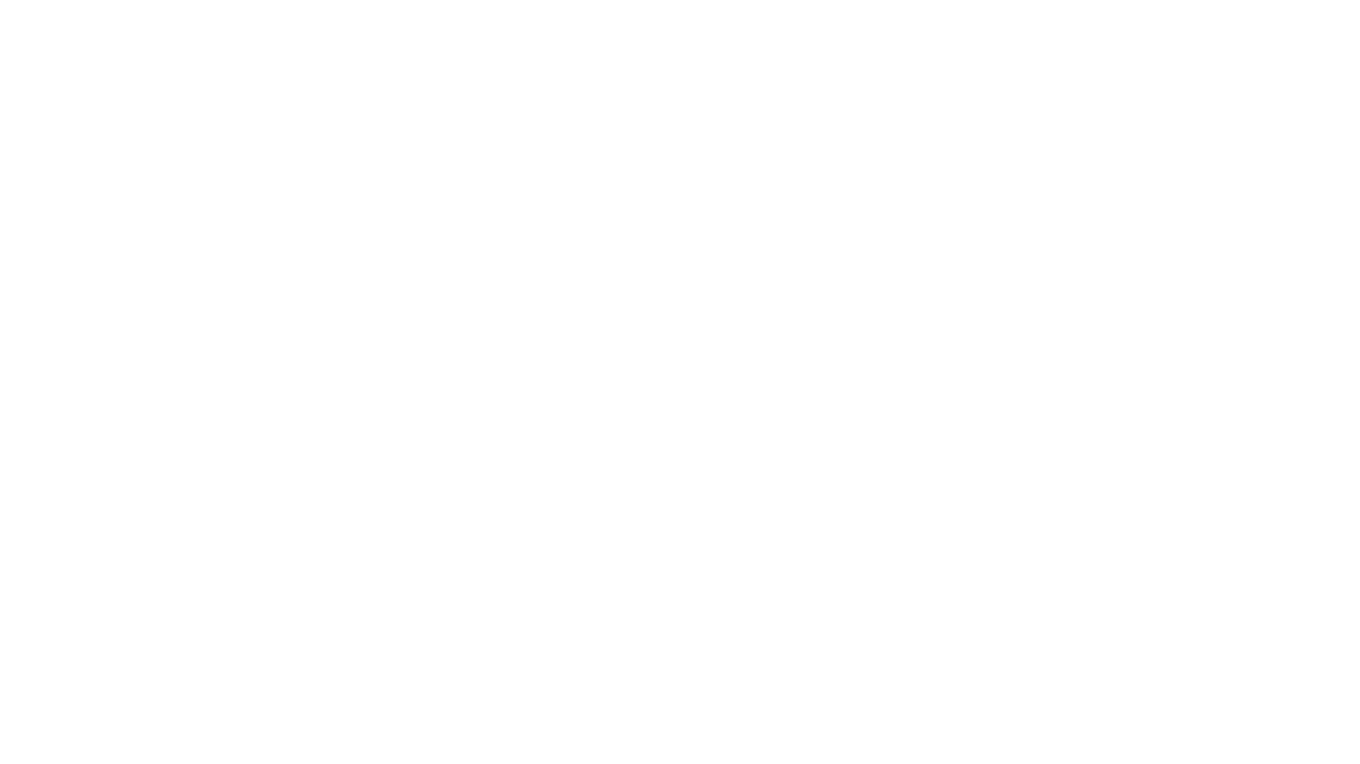
only_train_once
Only Train Once (OTO) is an automatic, architecture-agnostic DNN training and compression framework that allows users to train a general DNN from scratch or a pretrained checkpoint to achieve high performance and slimmer architecture simultaneously in a one-shot manner without fine-tuning. The framework includes features for automatic structured pruning and erasing operators, as well as hybrid structured sparse optimizers for efficient model compression. OTO provides tools for pruning zero-invariant group partitioning, constructing pruned models, and visualizing pruning and erasing dependency graphs. It supports the HESSO optimizer and offers a sanity check for compliance testing on various DNNs. The repository also includes publications, installation instructions, quick start guides, and a roadmap for future enhancements and collaborations.
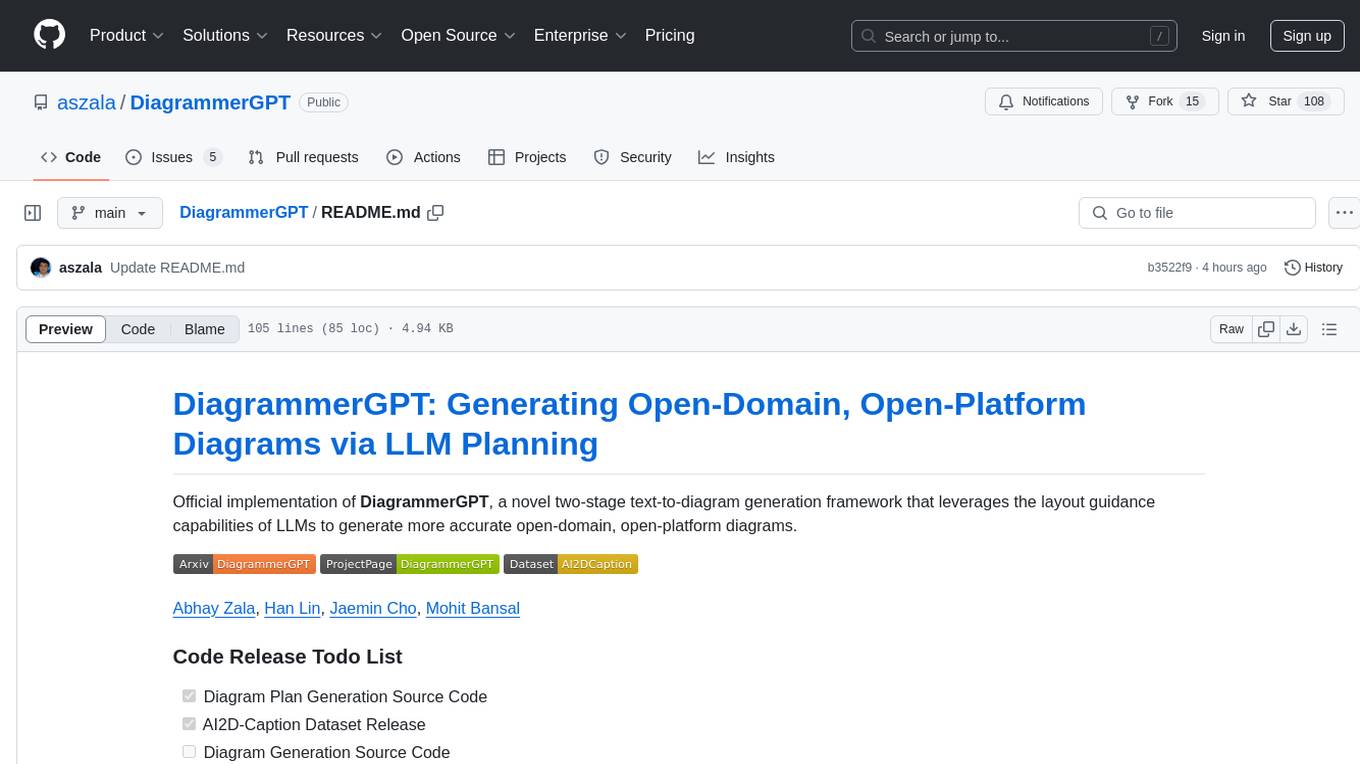
DiagrammerGPT
DiagrammerGPT is an official implementation of a two-stage text-to-diagram generation framework that utilizes the layout guidance capabilities of LLMs to create accurate open-domain, open-platform diagrams. The tool first generates a diagram plan based on a prompt, which includes dense entities, fine-grained relationships, and precise layouts. Then, it refines the plan iteratively before generating the final diagram. DiagrammerGPT has been used to create various diagrams such as layers of the earth, Earth's position around the sun, and different types of rocks with labels.
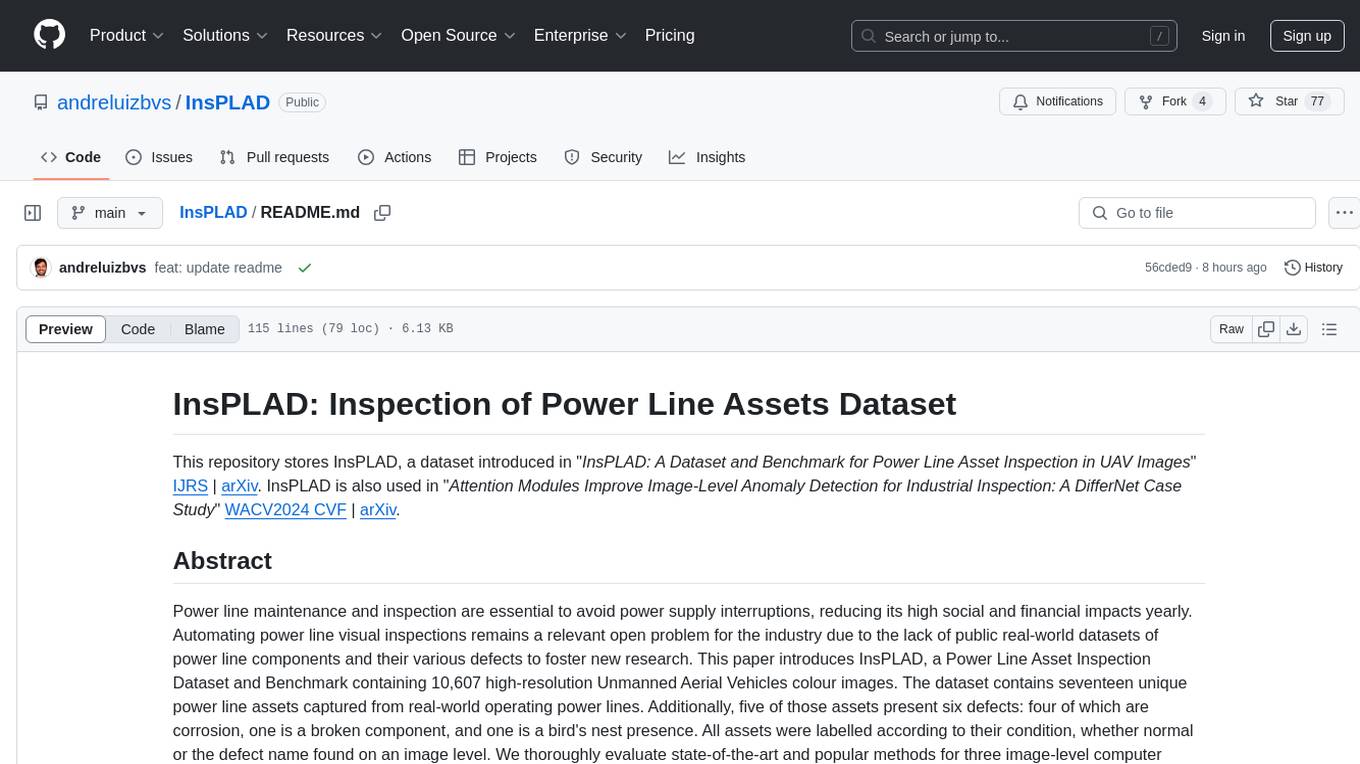
InsPLAD
InsPLAD is a dataset and benchmark for power line asset inspection in UAV images. It contains 10,607 high-resolution UAV color images of seventeen unique power line assets with six defects. The dataset is used for object detection, defect classification, and anomaly detection tasks in computer vision. InsPLAD offers challenges like multi-scale objects, intra-class variation, cluttered background, and varied lighting conditions, aiming to improve state-of-the-art methods in the field.
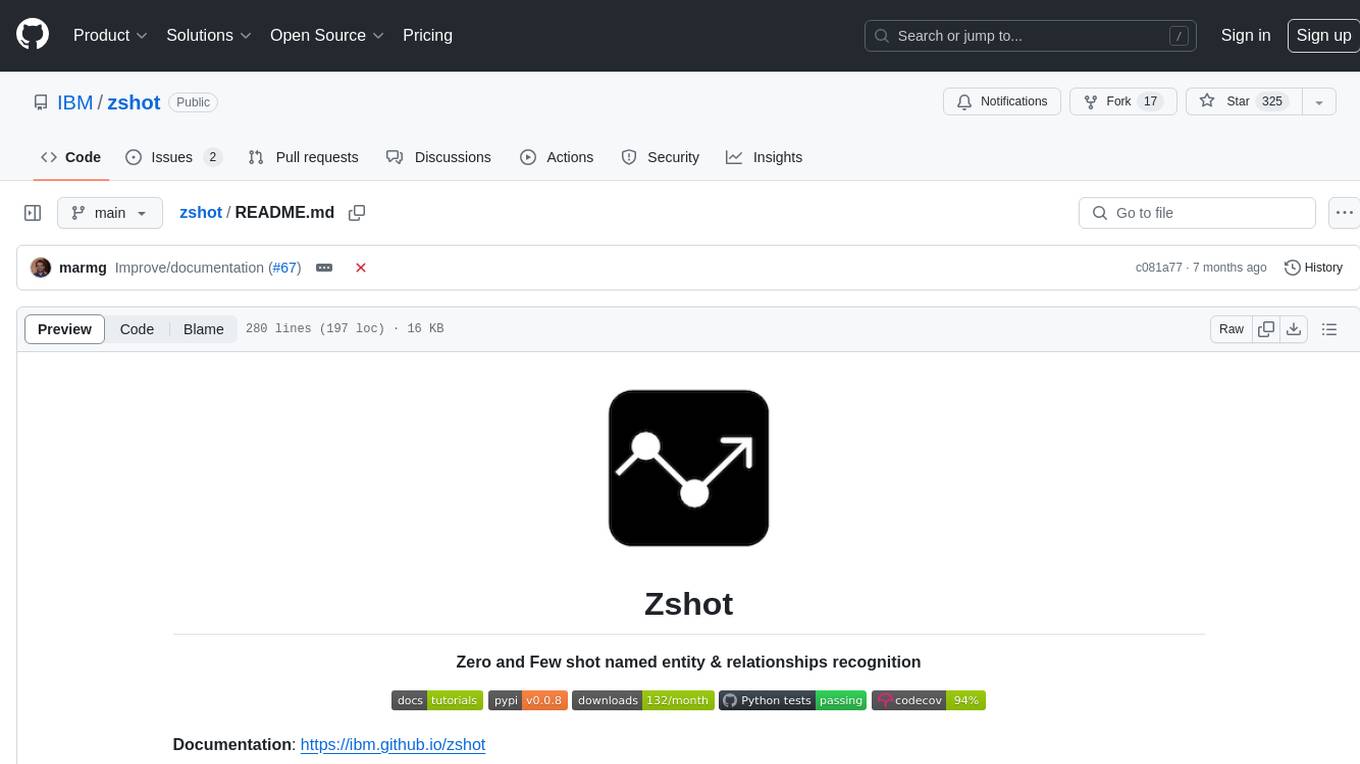
zshot
Zshot is a highly customizable framework for performing Zero and Few shot named entity and relationships recognition. It can be used for mentions extraction, wikification, zero and few shot named entity recognition, zero and few shot named relationship recognition, and visualization of zero-shot NER and RE extraction. The framework consists of two main components: the mentions extractor and the linker. There are multiple mentions extractors and linkers available, each serving a specific purpose. Zshot also includes a relations extractor and a knowledge extractor for extracting relations among entities and performing entity classification. The tool requires Python 3.6+ and dependencies like spacy, torch, transformers, evaluate, and datasets for evaluation over datasets like OntoNotes. Optional dependencies include flair and blink for additional functionalities. Zshot provides examples, tutorials, and evaluation methods to assess the performance of the components.
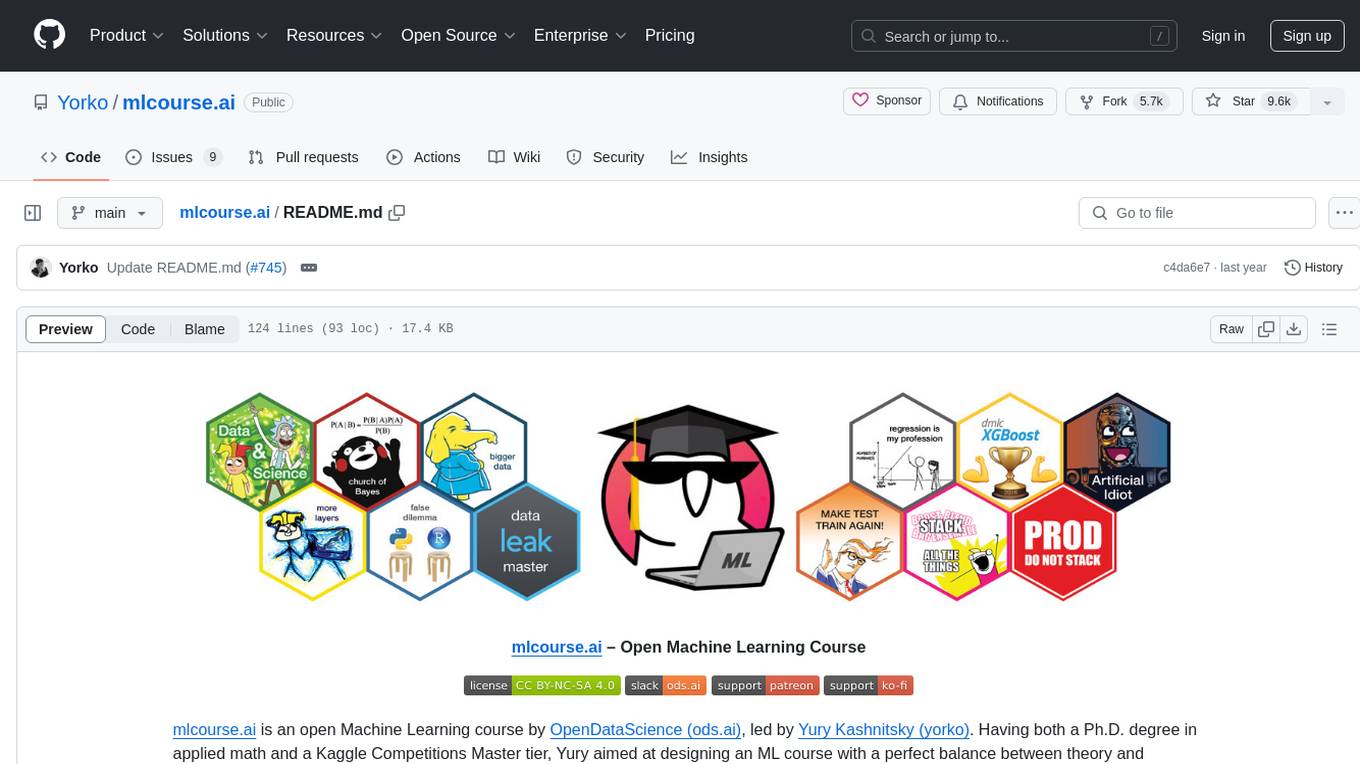
mlcourse.ai
mlcourse.ai is an open Machine Learning course by OpenDataScience (ods.ai), led by Yury Kashnitsky (yorko). The course offers a perfect balance between theory and practice, with math formulae in lectures and practical assignments including Kaggle Inclass competitions. It is currently in a self-paced mode, guiding users through 10 weeks of content covering topics from Pandas to Gradient Boosting. The course provides articles, lectures, and assignments to enhance understanding and application of machine learning concepts.
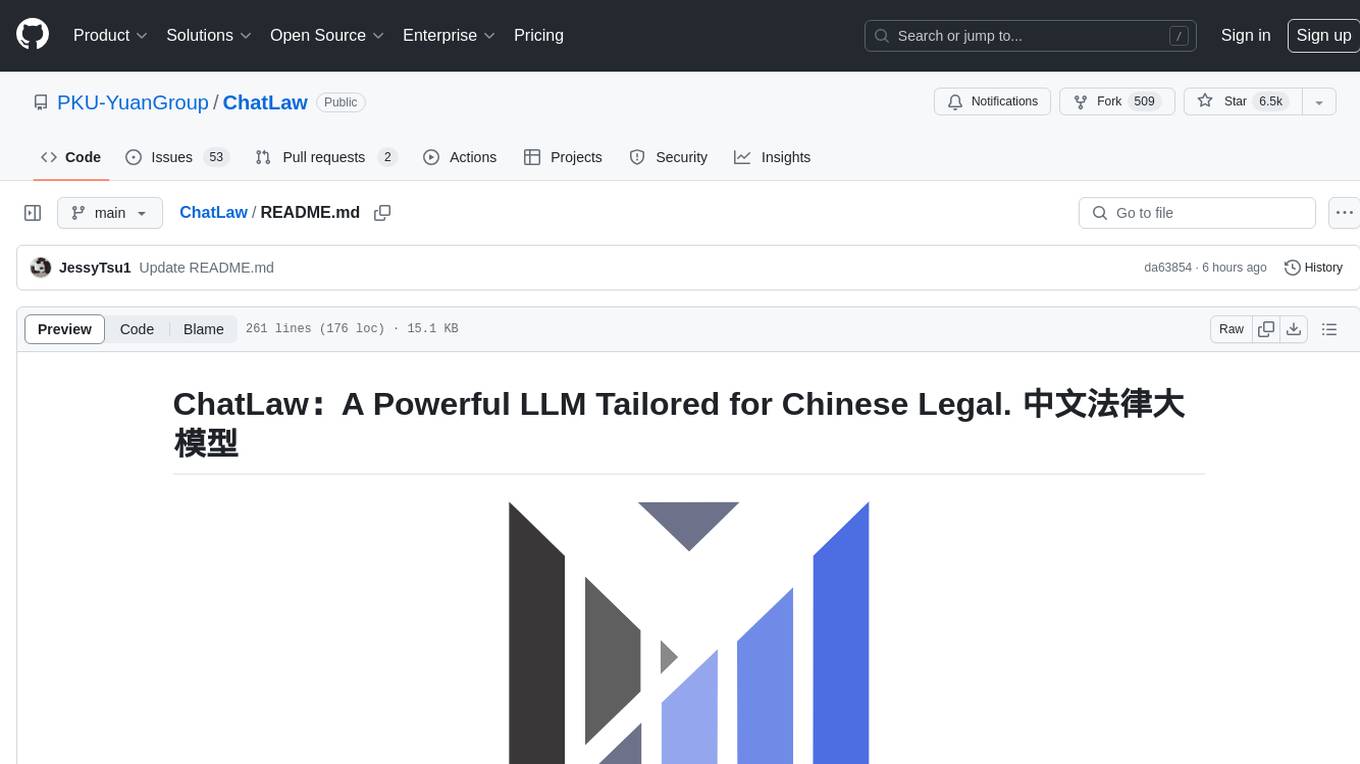
ChatLaw
ChatLaw is an open-source legal large language model tailored for Chinese legal scenarios. It aims to combine LLM and knowledge bases to provide solutions for legal scenarios. The models include ChatLaw-13B and ChatLaw-33B, trained on various legal texts to construct dialogue data. The project focuses on improving logical reasoning abilities and plans to train models with parameters exceeding 30B for better performance. The dataset consists of forum posts, news, legal texts, judicial interpretations, legal consultations, exam questions, and court judgments, cleaned and enhanced to create dialogue data. The tool is designed to assist in legal tasks requiring complex logical reasoning, with a focus on accuracy and reliability.
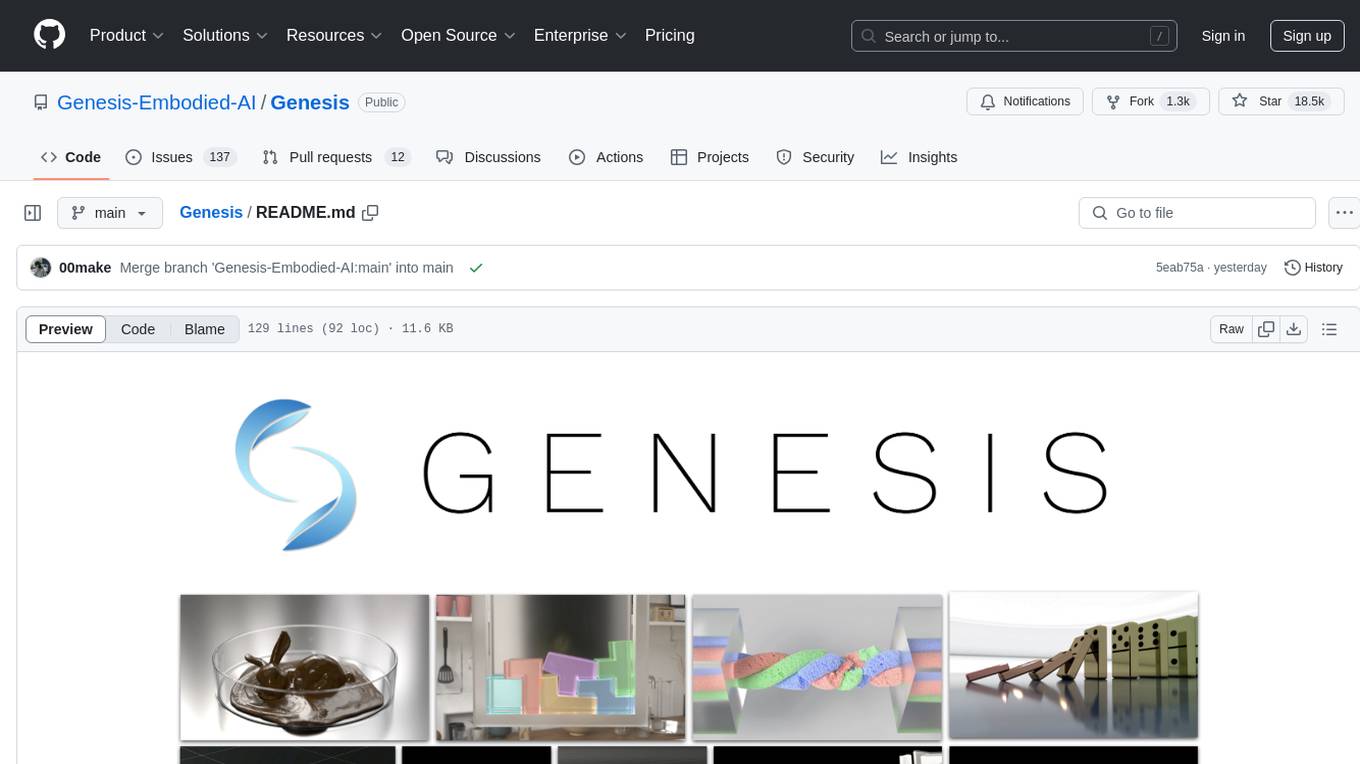
Genesis
Genesis is a physics platform designed for general purpose Robotics/Embodied AI/Physical AI applications. It includes a universal physics engine, a lightweight, ultra-fast, pythonic, and user-friendly robotics simulation platform, a powerful and fast photo-realistic rendering system, and a generative data engine that transforms user-prompted natural language description into various modalities of data. It aims to lower the barrier to using physics simulations, unify state-of-the-art physics solvers, and minimize human effort in collecting and generating data for robotics and other domains.
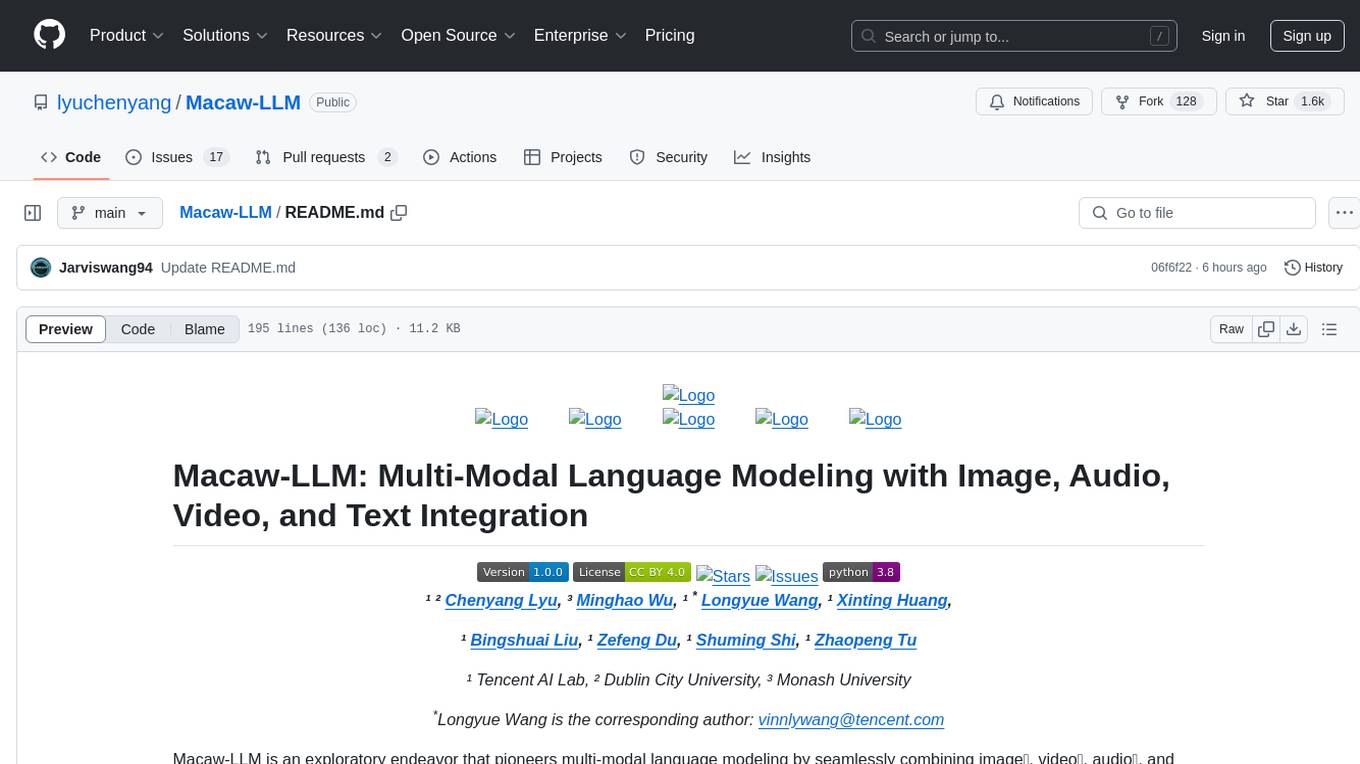
Macaw-LLM
Macaw-LLM is a pioneering multi-modal language modeling tool that seamlessly integrates image, audio, video, and text data. It builds upon CLIP, Whisper, and LLaMA models to process and analyze multi-modal information effectively. The tool boasts features like simple and fast alignment, one-stage instruction fine-tuning, and a new multi-modal instruction dataset. It enables users to align multi-modal features efficiently, encode instructions, and generate responses across different data types.
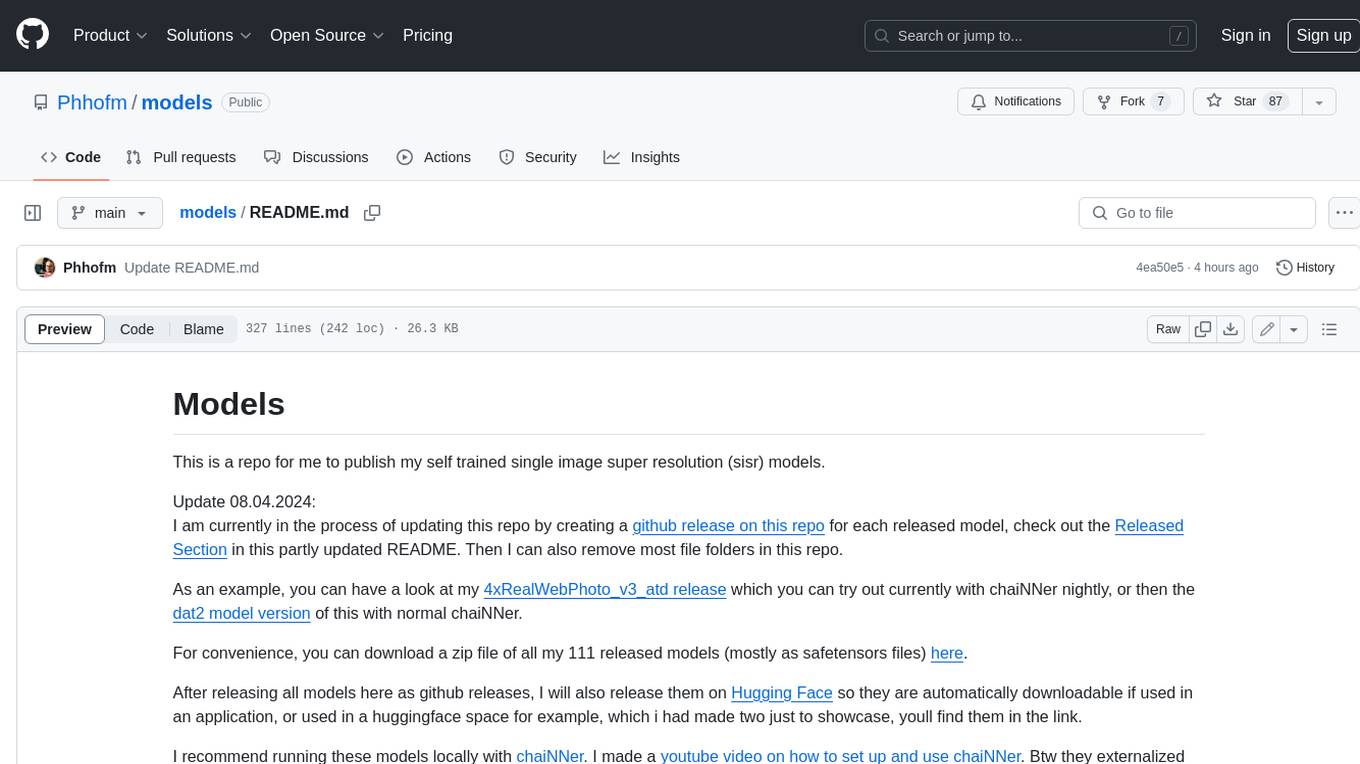
models
This repository contains self-trained single image super resolution (SISR) models. The models are trained on various datasets and use different network architectures. They can be used to upscale images by 2x, 4x, or 8x, and can handle various types of degradation, such as JPEG compression, noise, and blur. The models are provided as safetensors files, which can be loaded into a variety of deep learning frameworks, such as PyTorch and TensorFlow. The repository also includes a number of resources, such as examples, results, and a website where you can compare the outputs of different models.
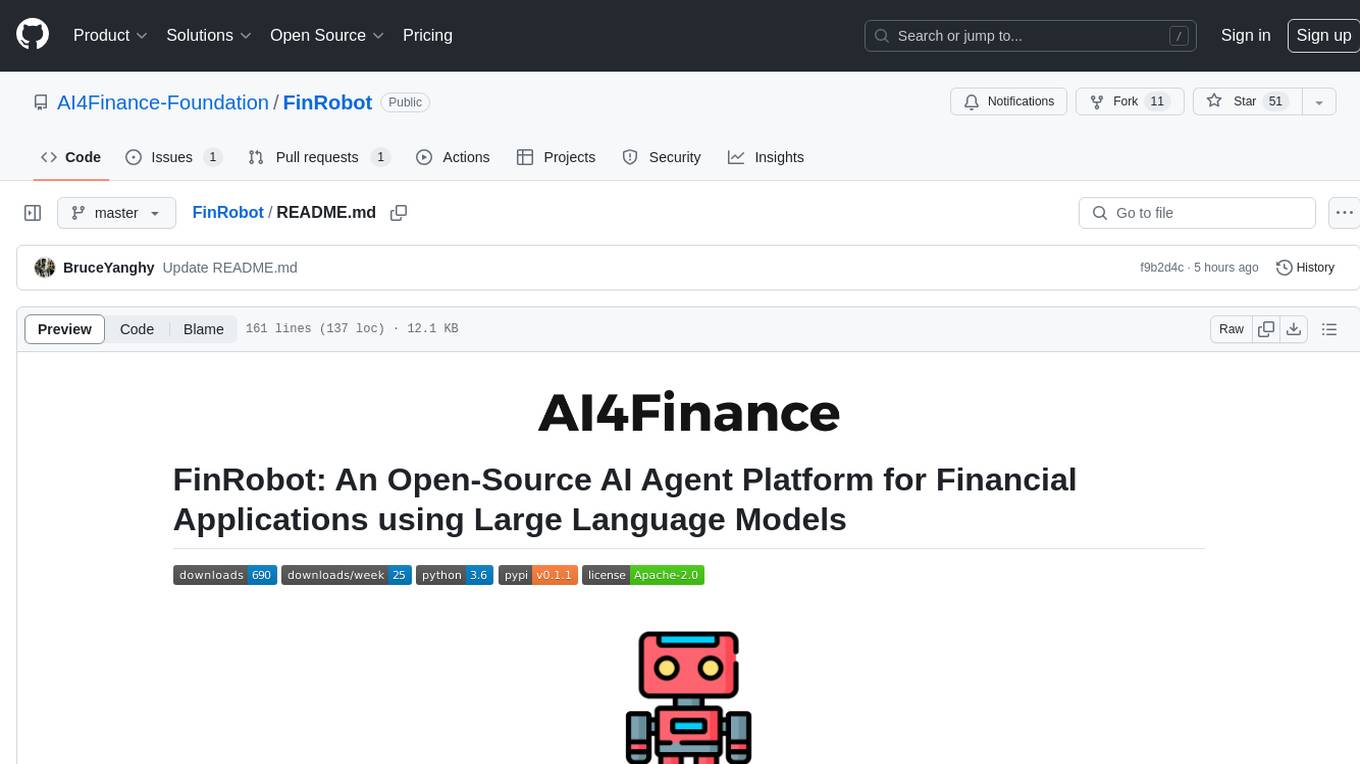
FinRobot
FinRobot is an open-source AI agent platform designed for financial applications using large language models. It transcends the scope of FinGPT, offering a comprehensive solution that integrates a diverse array of AI technologies. The platform's versatility and adaptability cater to the multifaceted needs of the financial industry. FinRobot's ecosystem is organized into four layers, including Financial AI Agents Layer, Financial LLMs Algorithms Layer, LLMOps and DataOps Layers, and Multi-source LLM Foundation Models Layer. The platform's agent workflow involves Perception, Brain, and Action modules to capture, process, and execute financial data and insights. The Smart Scheduler optimizes model diversity and selection for tasks, managed by components like Director Agent, Agent Registration, Agent Adaptor, and Task Manager. The tool provides a structured file organization with subfolders for agents, data sources, and functional modules, along with installation instructions and hands-on tutorials.
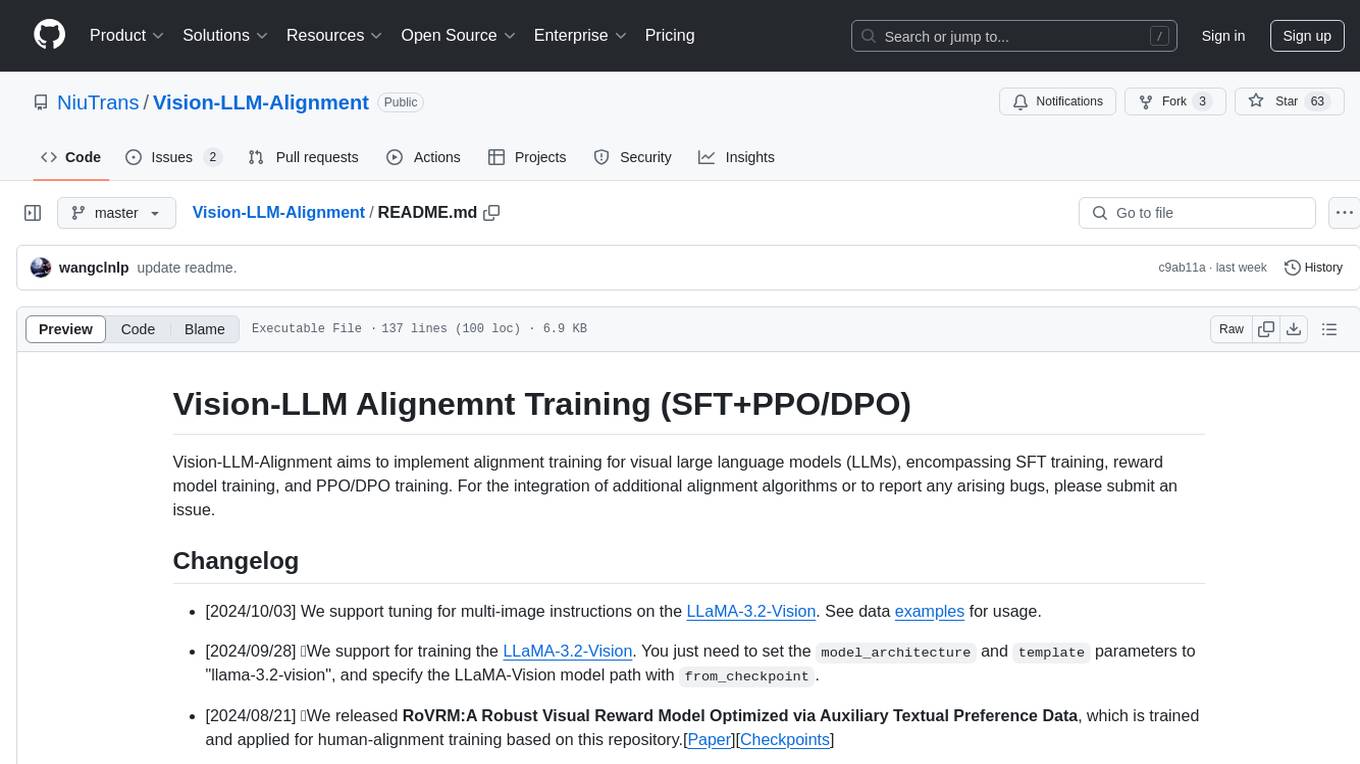
Vision-LLM-Alignment
Vision-LLM-Alignment is a repository focused on implementing alignment training for visual large language models (LLMs), including SFT training, reward model training, and PPO/DPO training. It supports various model architectures and provides datasets for training. The repository also offers benchmark results and installation instructions for users.
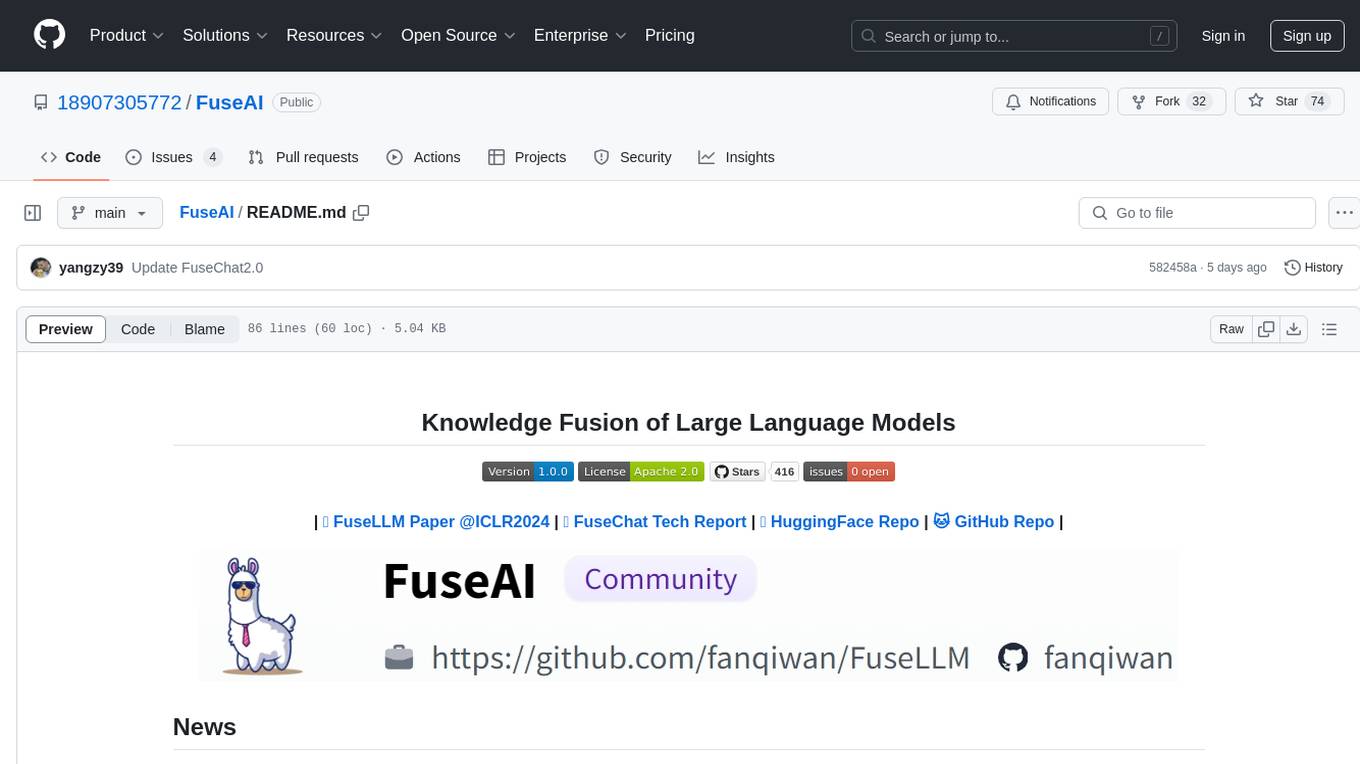
FuseAI
FuseAI is a repository that focuses on knowledge fusion of large language models. It includes FuseChat, a state-of-the-art 7B LLM on MT-Bench, and FuseLLM, which surpasses Llama-2-7B by fusing three open-source foundation LLMs. The repository provides tech reports, releases, and datasets for FuseChat and FuseLLM, showcasing their performance and advancements in the field of chat models and large language models.
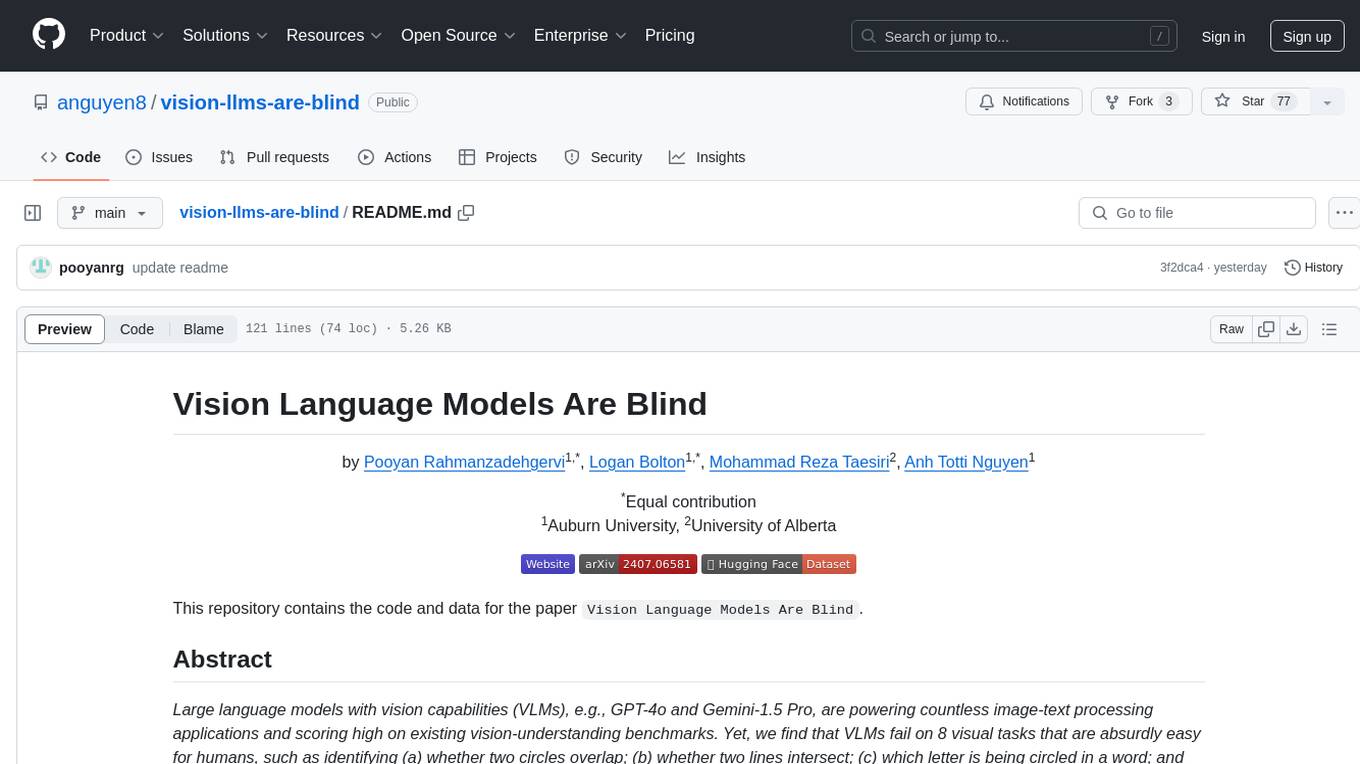
vision-llms-are-blind
This repository contains the code and data for the paper 'Vision Language Models Are Blind'. It explores the limitations of large language models with vision capabilities (VLMs) in performing basic visual tasks that are easy for humans. The repository presents benchmark results showcasing the poor performance of state-of-the-art VLMs on tasks like counting line intersections, identifying circles, letters, and shapes, and following color-coded paths. The research highlights the challenges faced by VLMs in understanding visual information accurately, drawing parallels to myopia and blindness in human vision.
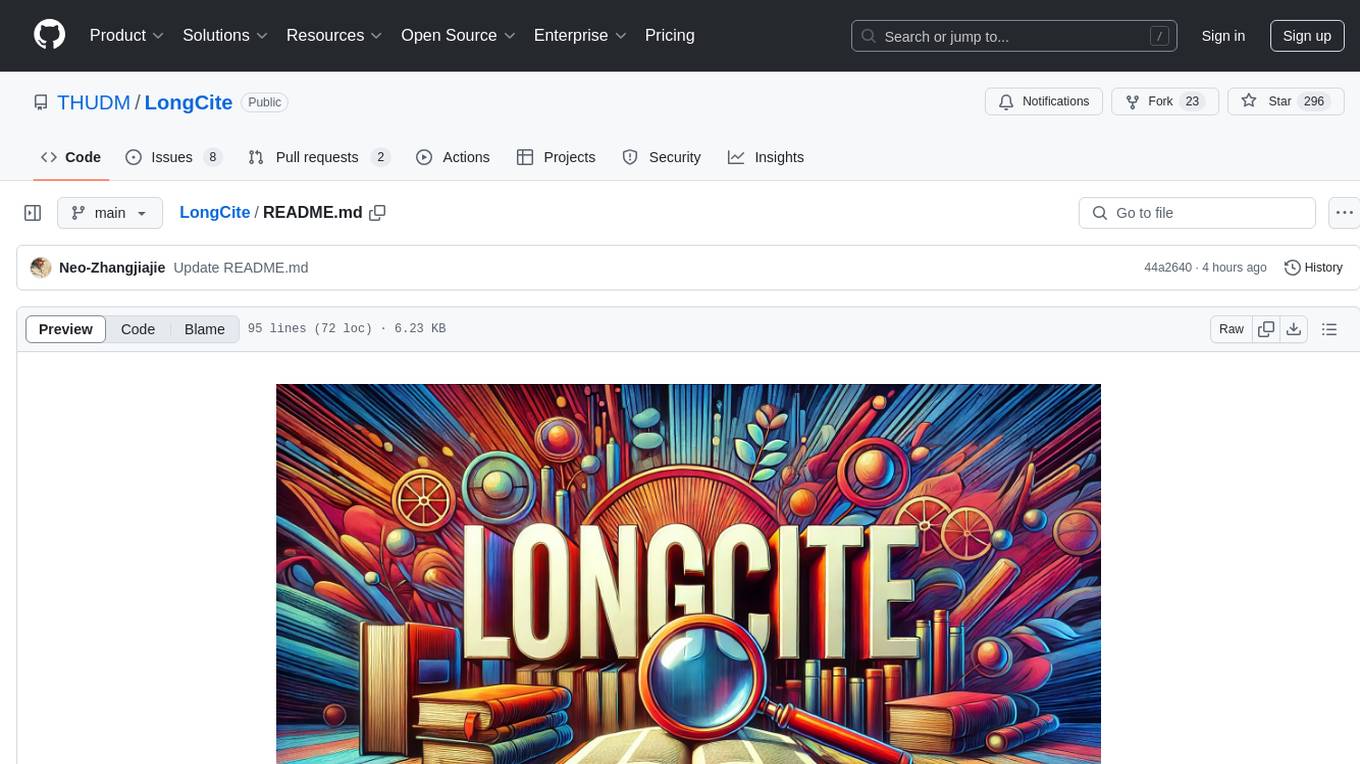
LongCite
LongCite is a tool that enables Large Language Models (LLMs) to generate fine-grained citations in long-context Question Answering (QA) scenarios. It provides models trained on GLM-4-9B and Meta-Llama-3.1-8B, supporting up to 128K context. Users can deploy LongCite chatbots, generate accurate responses, and obtain precise sentence-level citations. The tool includes components for model deployment, Coarse to Fine (CoF) pipeline for data construction, model training using LongCite-45k dataset, evaluation with LongBench-Cite benchmark, and citation generation.
For similar tasks
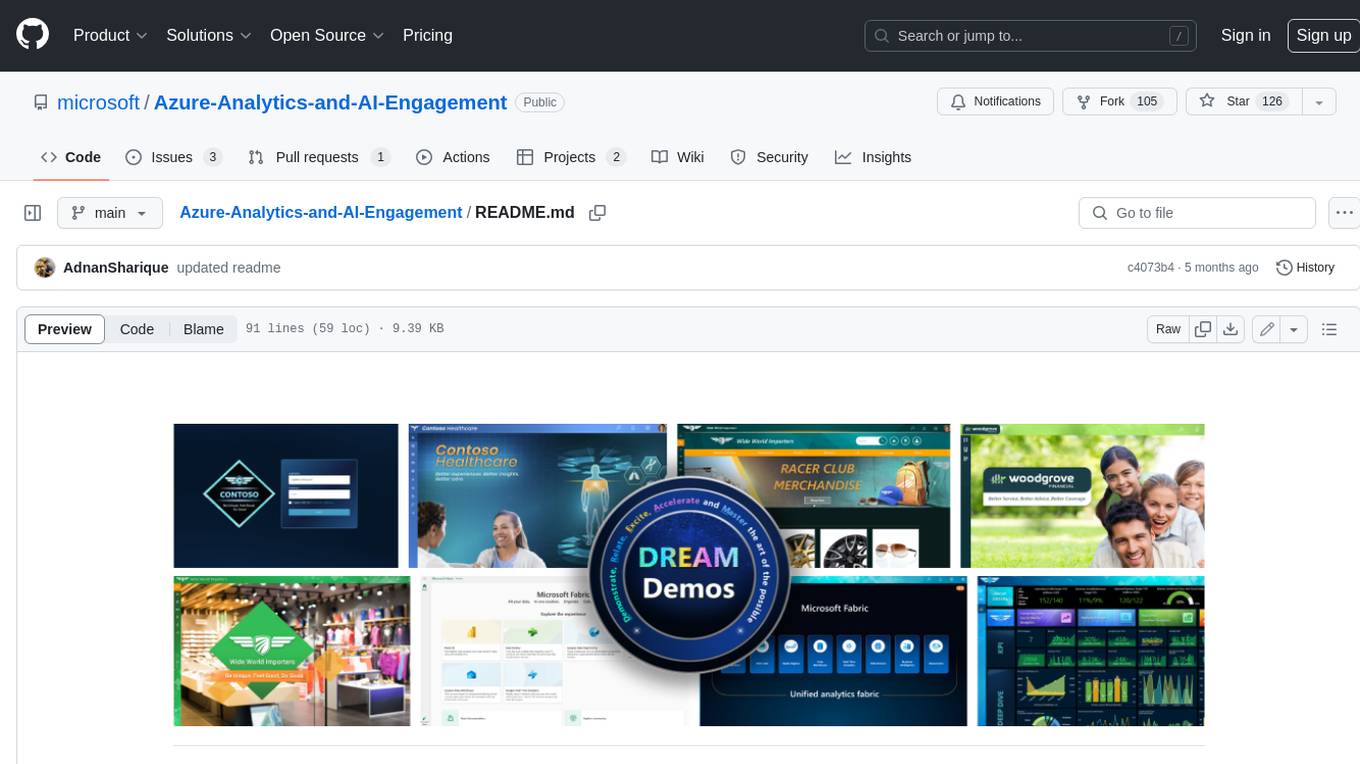
Azure-Analytics-and-AI-Engagement
The Azure-Analytics-and-AI-Engagement repository provides packaged Industry Scenario DREAM Demos with ARM templates (Containing a demo web application, Power BI reports, Synapse resources, AML Notebooks etc.) that can be deployed in a customer’s subscription using the CAPE tool within a matter of few hours. Partners can also deploy DREAM Demos in their own subscriptions using DPoC.
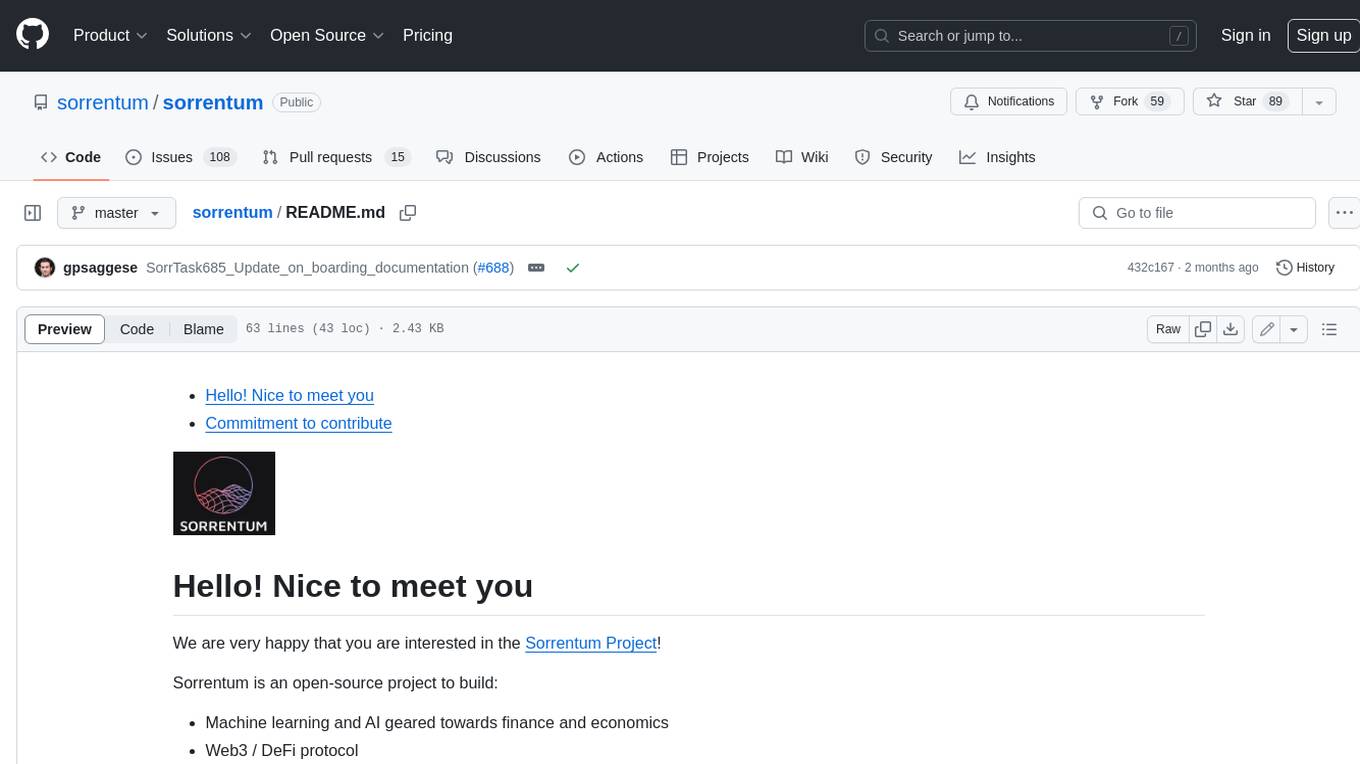
sorrentum
Sorrentum is an open-source project that aims to combine open-source development, startups, and brilliant students to build machine learning, AI, and Web3 / DeFi protocols geared towards finance and economics. The project provides opportunities for internships, research assistantships, and development grants, as well as the chance to work on cutting-edge problems, learn about startups, write academic papers, and get internships and full-time positions at companies working on Sorrentum applications.
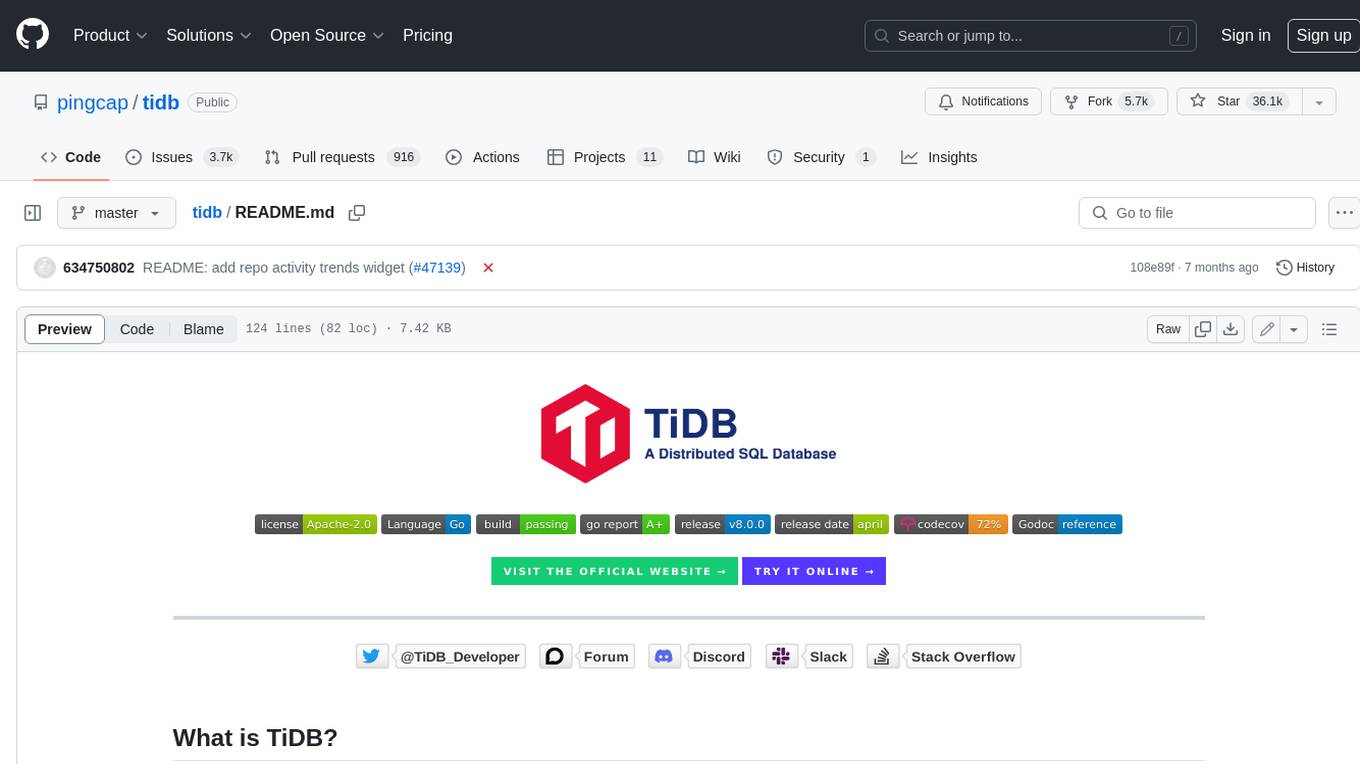
tidb
TiDB is an open-source distributed SQL database that supports Hybrid Transactional and Analytical Processing (HTAP) workloads. It is MySQL compatible and features horizontal scalability, strong consistency, and high availability.
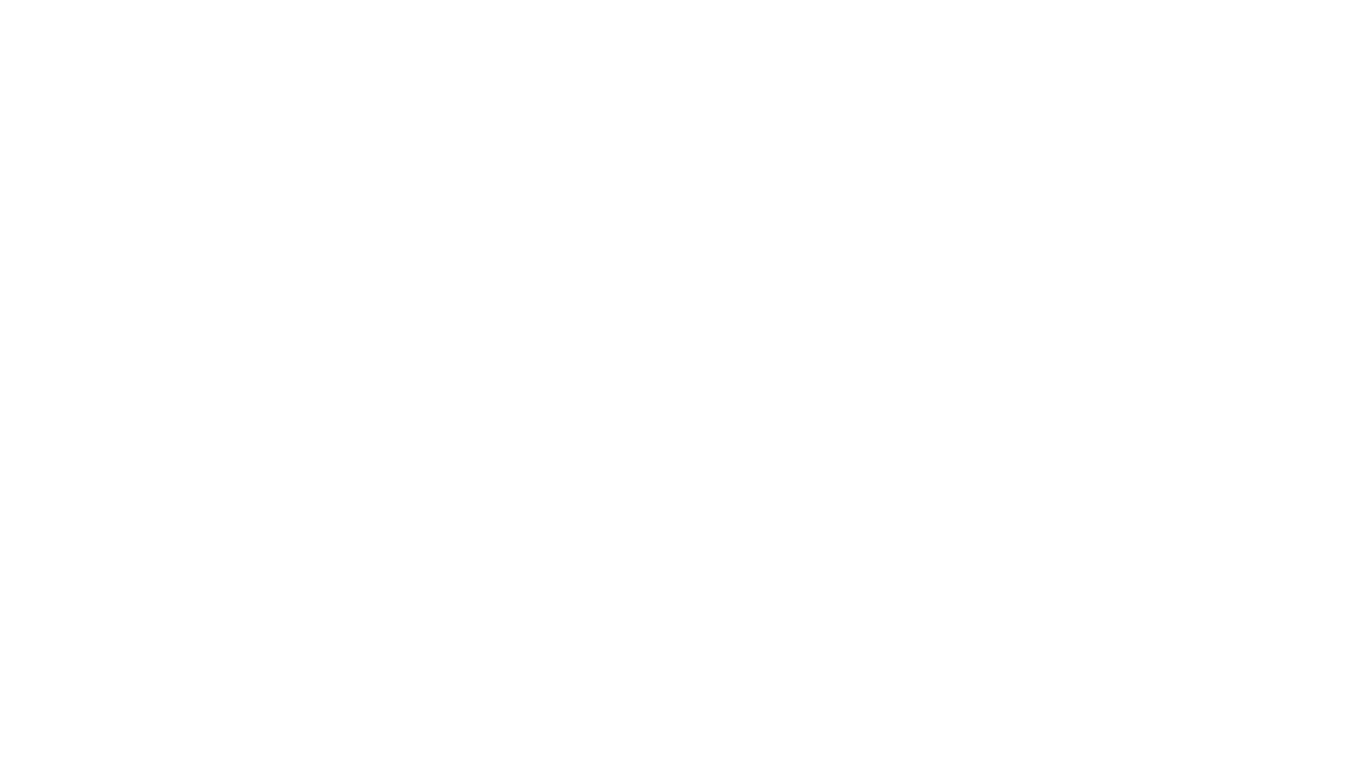
zep-python
Zep is an open-source platform for building and deploying large language model (LLM) applications. It provides a suite of tools and services that make it easy to integrate LLMs into your applications, including chat history memory, embedding, vector search, and data enrichment. Zep is designed to be scalable, reliable, and easy to use, making it a great choice for developers who want to build LLM-powered applications quickly and easily.
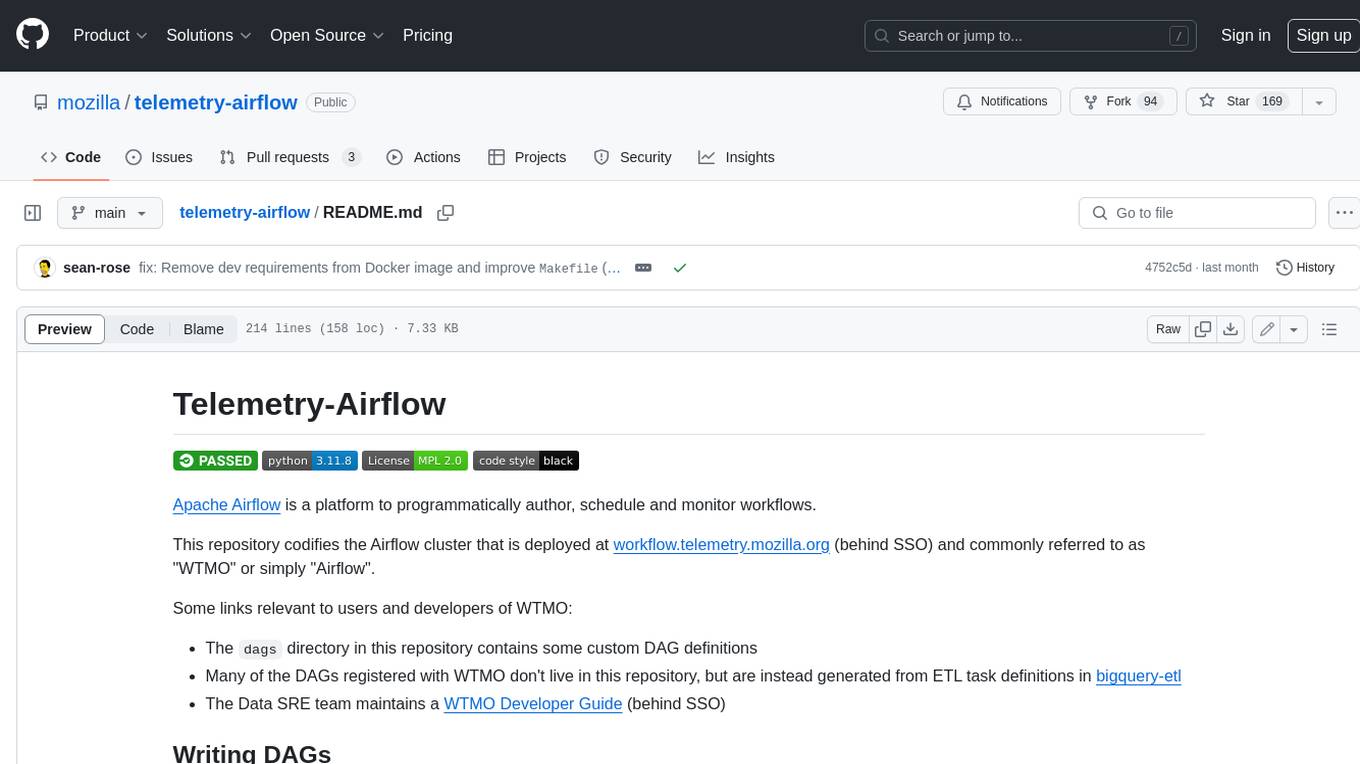
telemetry-airflow
This repository codifies the Airflow cluster that is deployed at workflow.telemetry.mozilla.org (behind SSO) and commonly referred to as "WTMO" or simply "Airflow". Some links relevant to users and developers of WTMO: * The `dags` directory in this repository contains some custom DAG definitions * Many of the DAGs registered with WTMO don't live in this repository, but are instead generated from ETL task definitions in bigquery-etl * The Data SRE team maintains a WTMO Developer Guide (behind SSO)
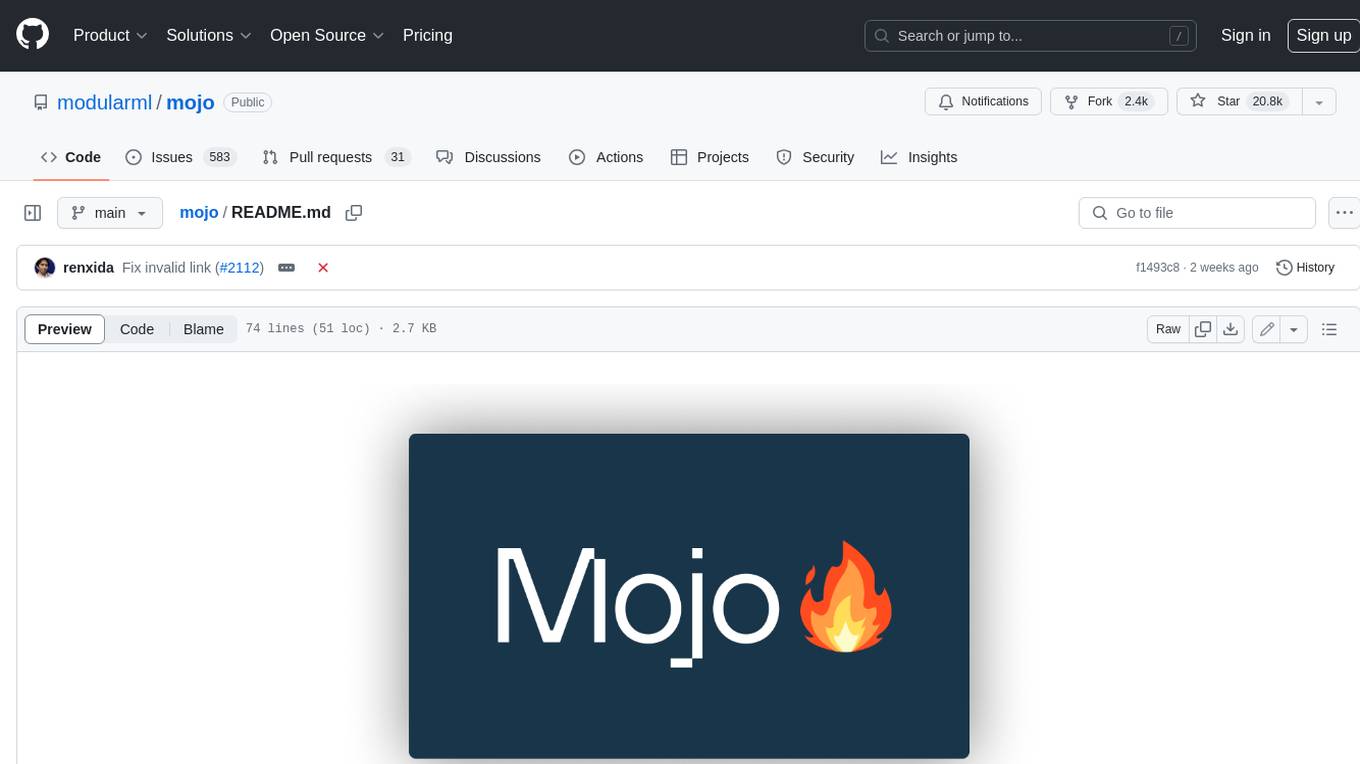
mojo
Mojo is a new programming language that bridges the gap between research and production by combining Python syntax and ecosystem with systems programming and metaprogramming features. Mojo is still young, but it is designed to become a superset of Python over time.
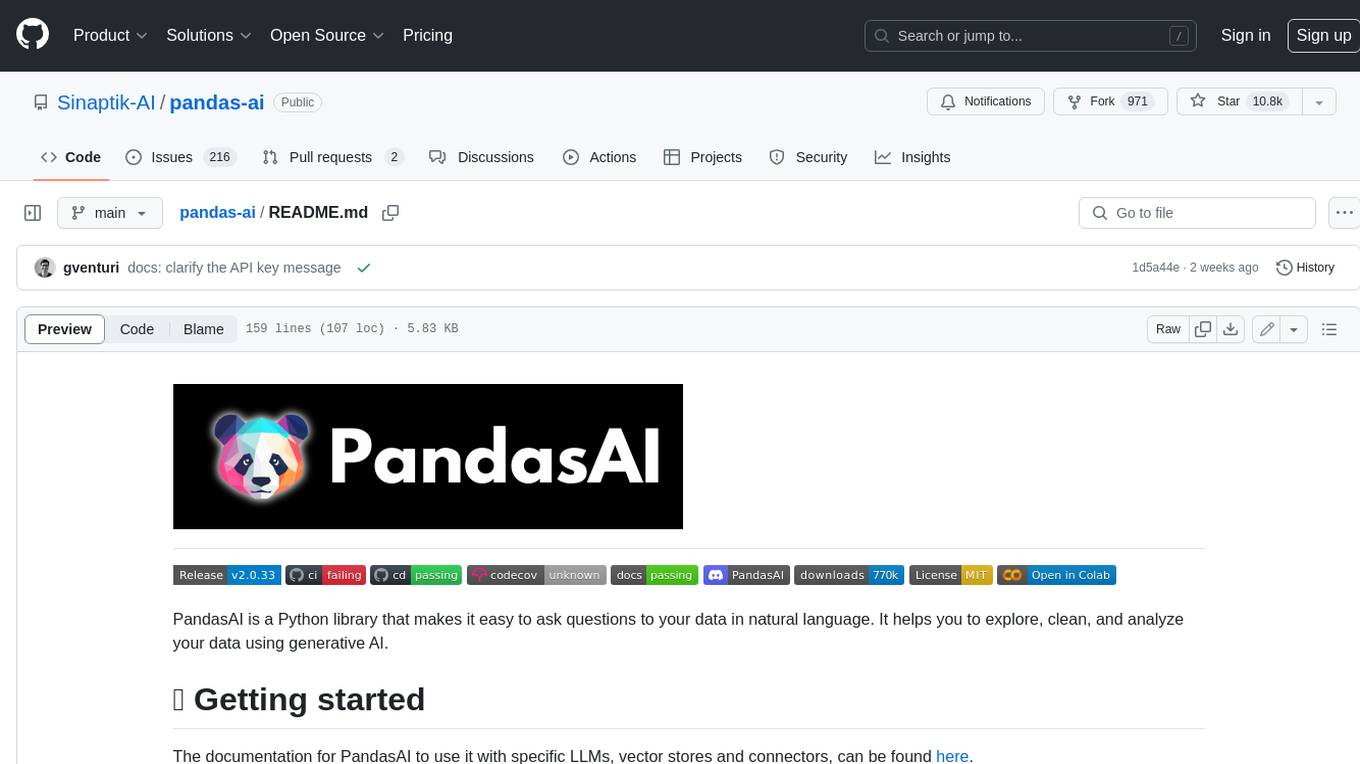
pandas-ai
PandasAI is a Python library that makes it easy to ask questions to your data in natural language. It helps you to explore, clean, and analyze your data using generative AI.
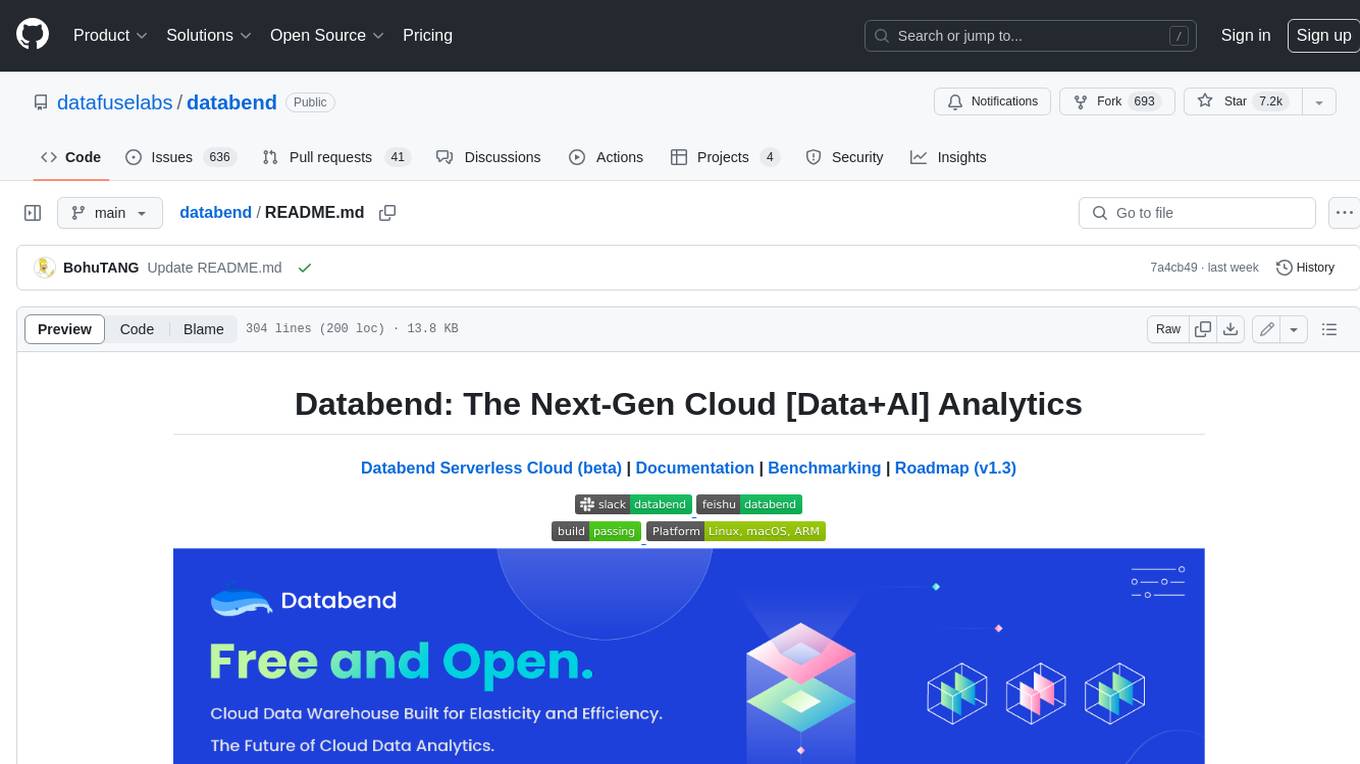
databend
Databend is an open-source cloud data warehouse that serves as a cost-effective alternative to Snowflake. With its focus on fast query execution and data ingestion, it's designed for complex analysis of the world's largest datasets.
For similar jobs
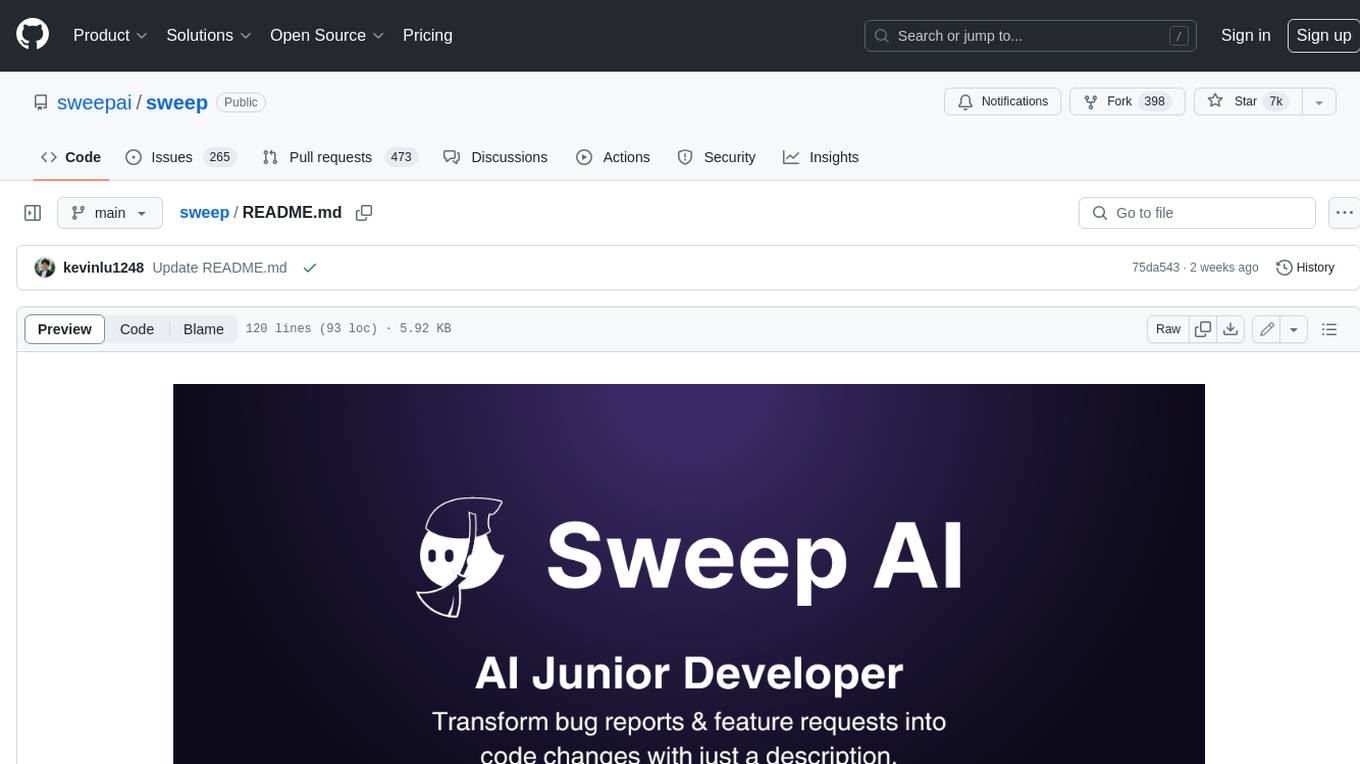
sweep
Sweep is an AI junior developer that turns bugs and feature requests into code changes. It automatically handles developer experience improvements like adding type hints and improving test coverage.
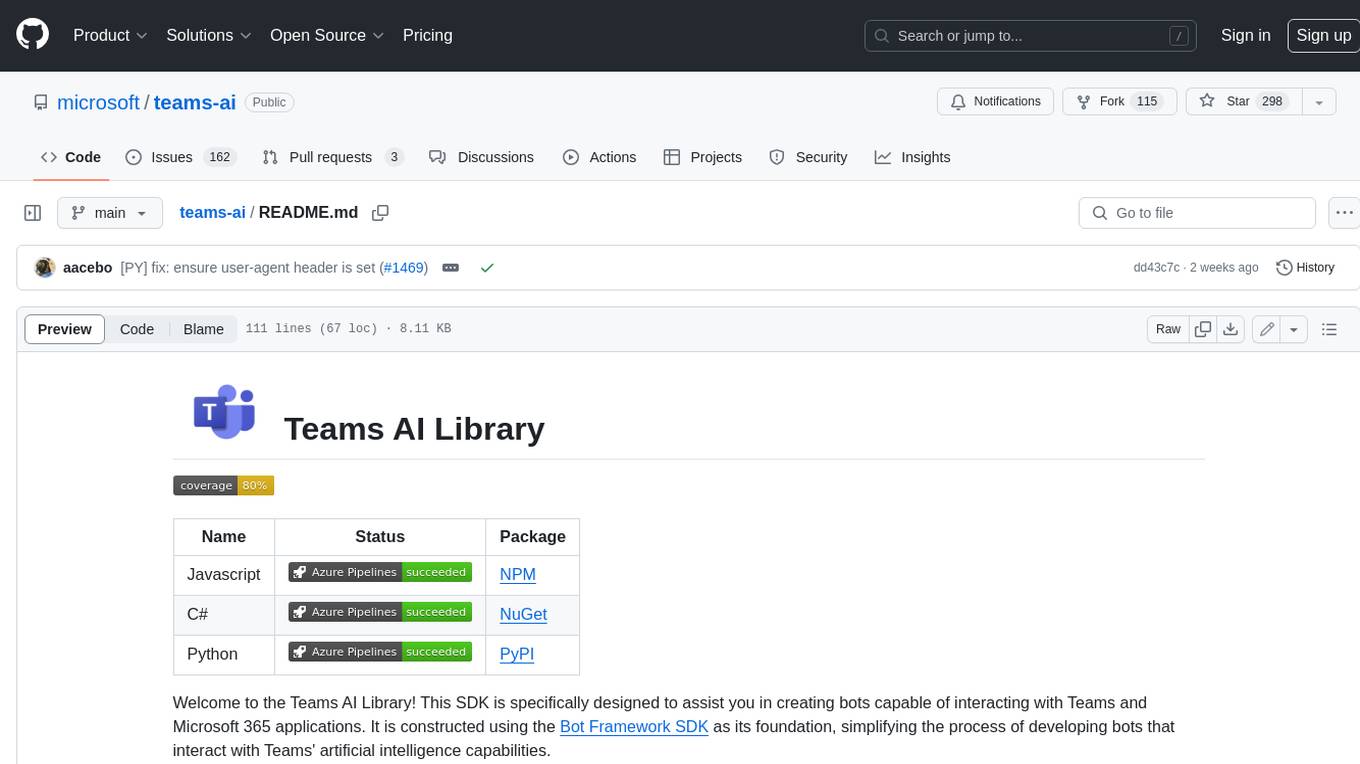
teams-ai
The Teams AI Library is a software development kit (SDK) that helps developers create bots that can interact with Teams and Microsoft 365 applications. It is built on top of the Bot Framework SDK and simplifies the process of developing bots that interact with Teams' artificial intelligence capabilities. The SDK is available for JavaScript/TypeScript, .NET, and Python.
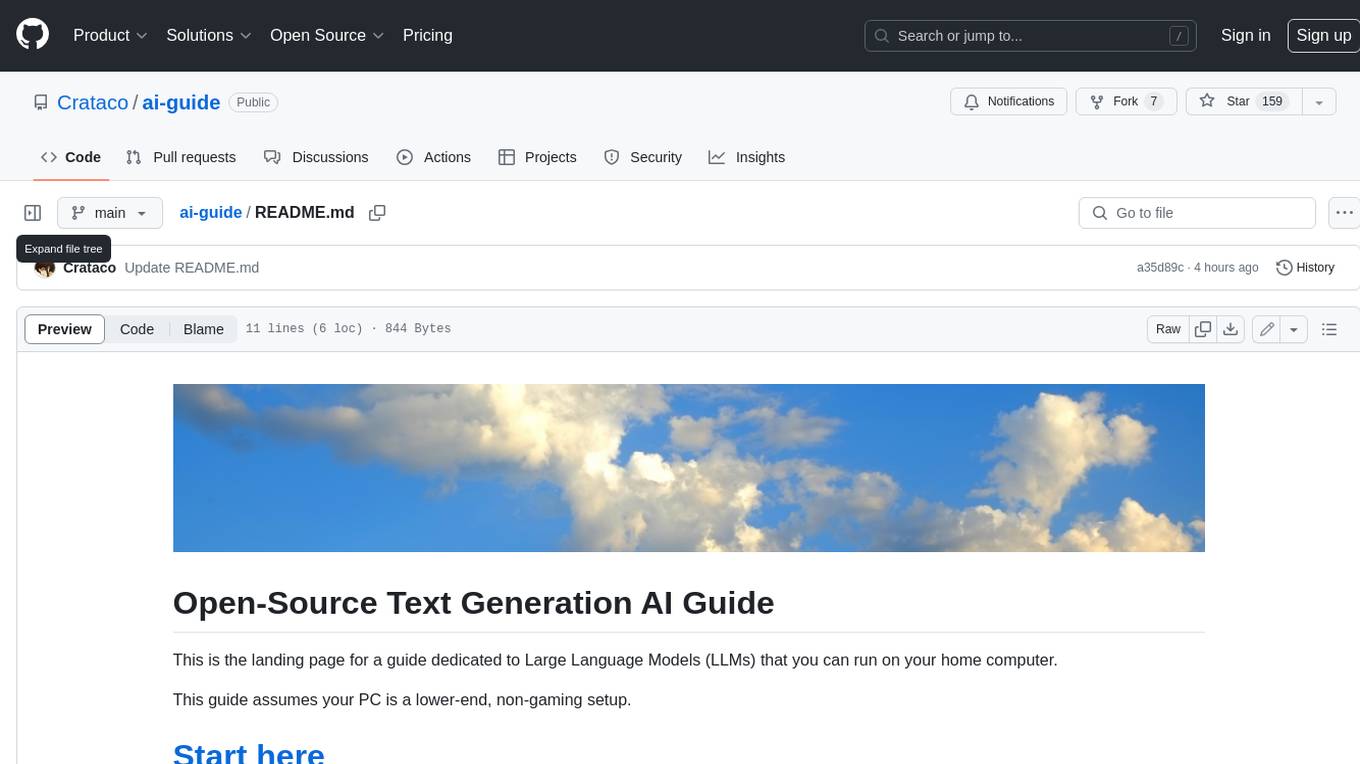
ai-guide
This guide is dedicated to Large Language Models (LLMs) that you can run on your home computer. It assumes your PC is a lower-end, non-gaming setup.
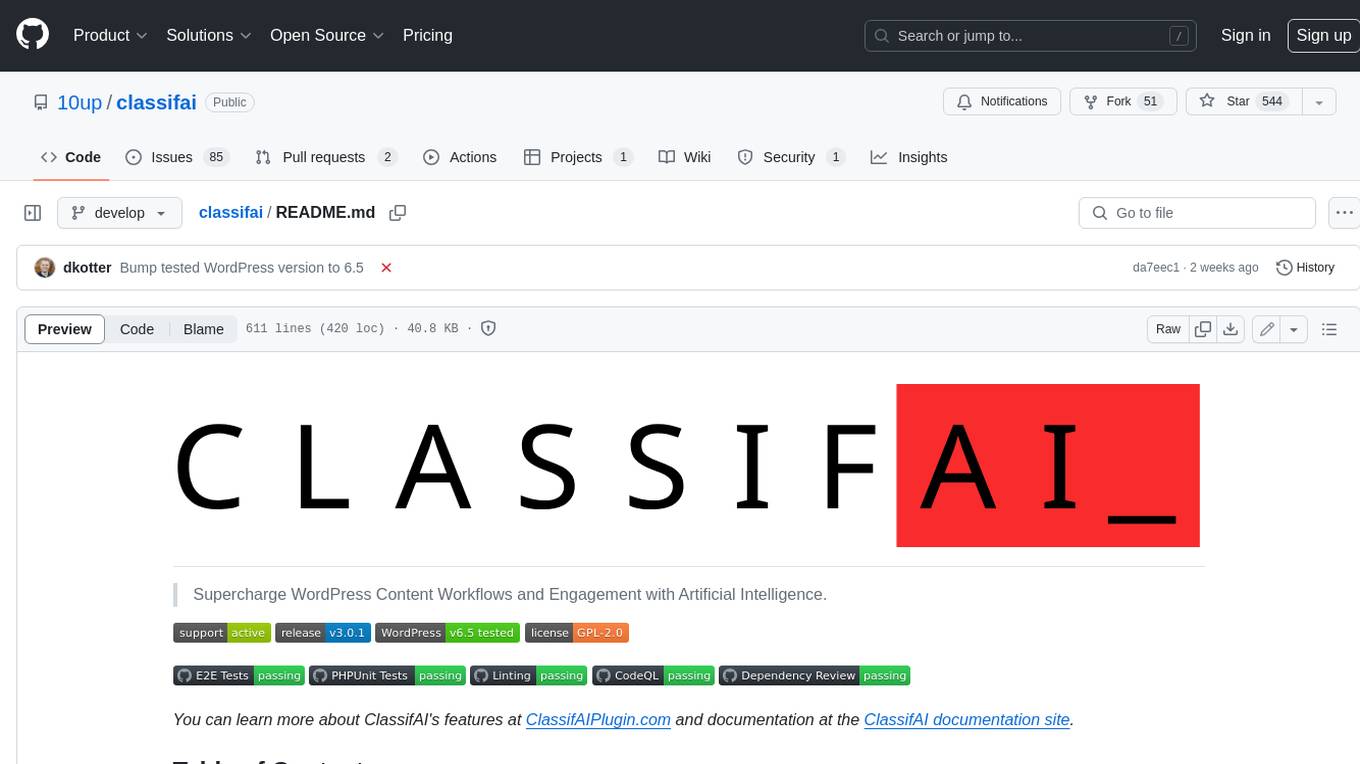
classifai
Supercharge WordPress Content Workflows and Engagement with Artificial Intelligence. Tap into leading cloud-based services like OpenAI, Microsoft Azure AI, Google Gemini and IBM Watson to augment your WordPress-powered websites. Publish content faster while improving SEO performance and increasing audience engagement. ClassifAI integrates Artificial Intelligence and Machine Learning technologies to lighten your workload and eliminate tedious tasks, giving you more time to create original content that matters.
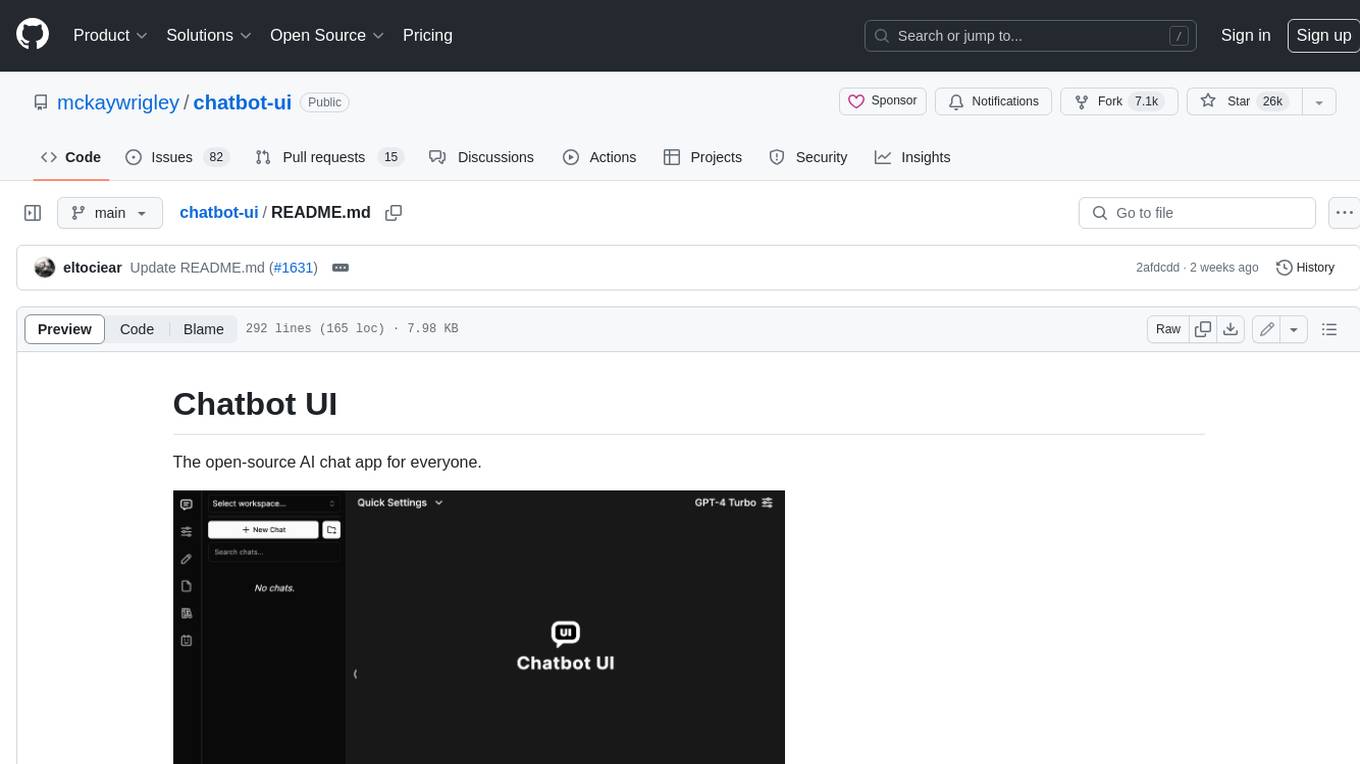
chatbot-ui
Chatbot UI is an open-source AI chat app that allows users to create and deploy their own AI chatbots. It is easy to use and can be customized to fit any need. Chatbot UI is perfect for businesses, developers, and anyone who wants to create a chatbot.
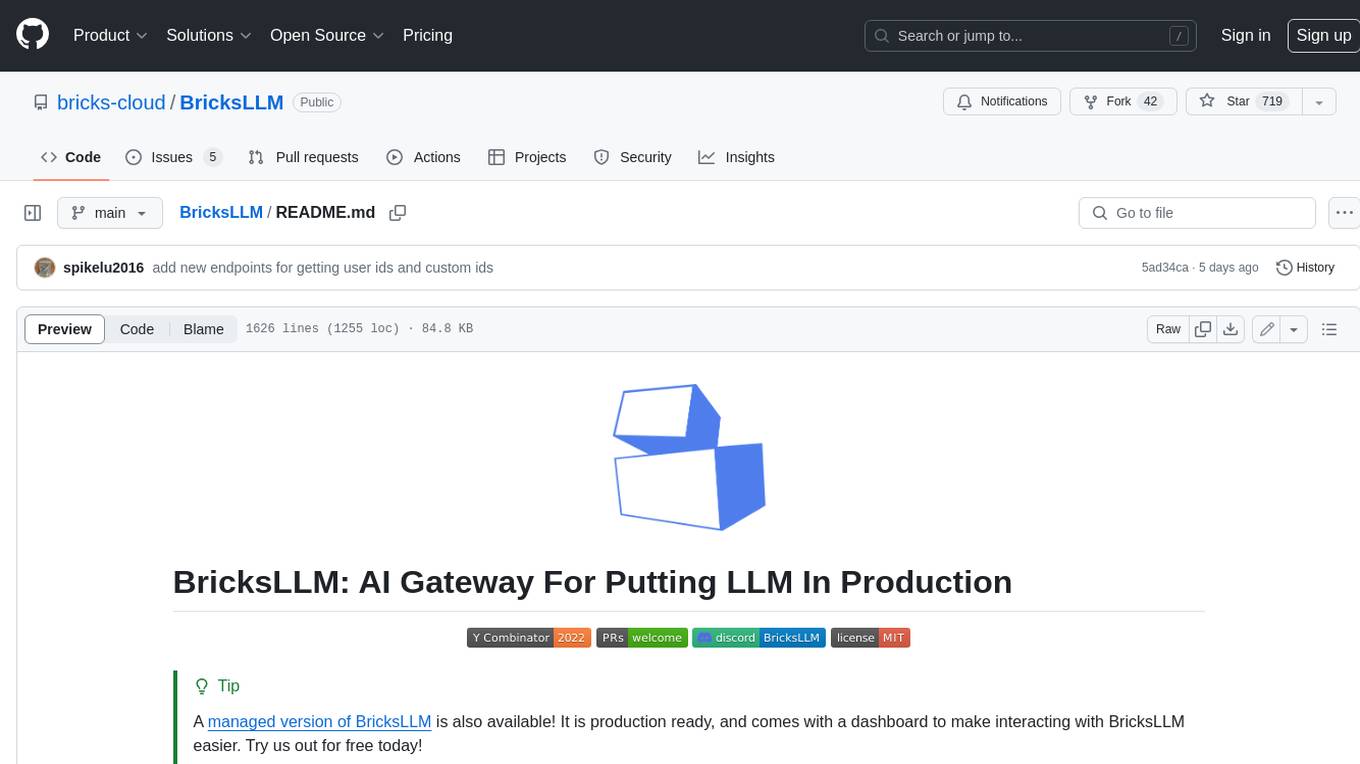
BricksLLM
BricksLLM is a cloud native AI gateway written in Go. Currently, it provides native support for OpenAI, Anthropic, Azure OpenAI and vLLM. BricksLLM aims to provide enterprise level infrastructure that can power any LLM production use cases. Here are some use cases for BricksLLM: * Set LLM usage limits for users on different pricing tiers * Track LLM usage on a per user and per organization basis * Block or redact requests containing PIIs * Improve LLM reliability with failovers, retries and caching * Distribute API keys with rate limits and cost limits for internal development/production use cases * Distribute API keys with rate limits and cost limits for students
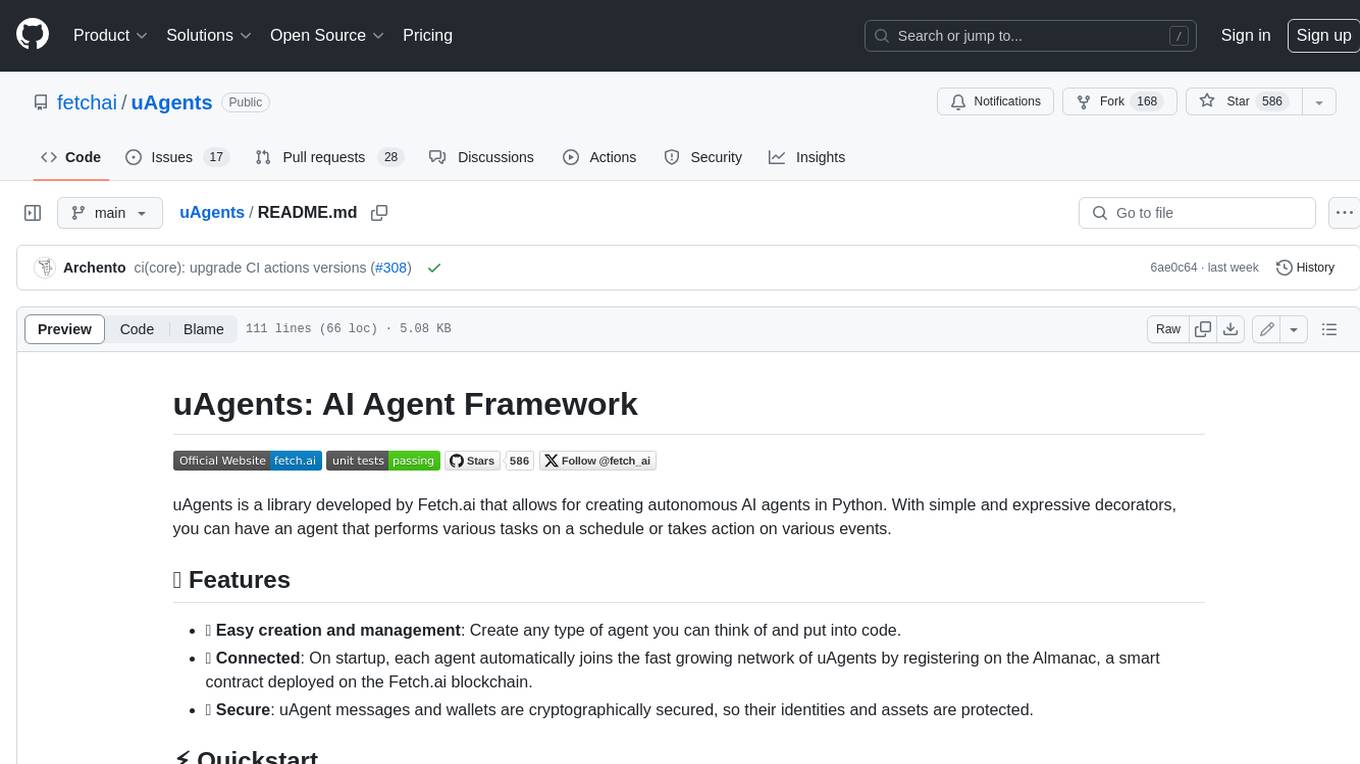
uAgents
uAgents is a Python library developed by Fetch.ai that allows for the creation of autonomous AI agents. These agents can perform various tasks on a schedule or take action on various events. uAgents are easy to create and manage, and they are connected to a fast-growing network of other uAgents. They are also secure, with cryptographically secured messages and wallets.
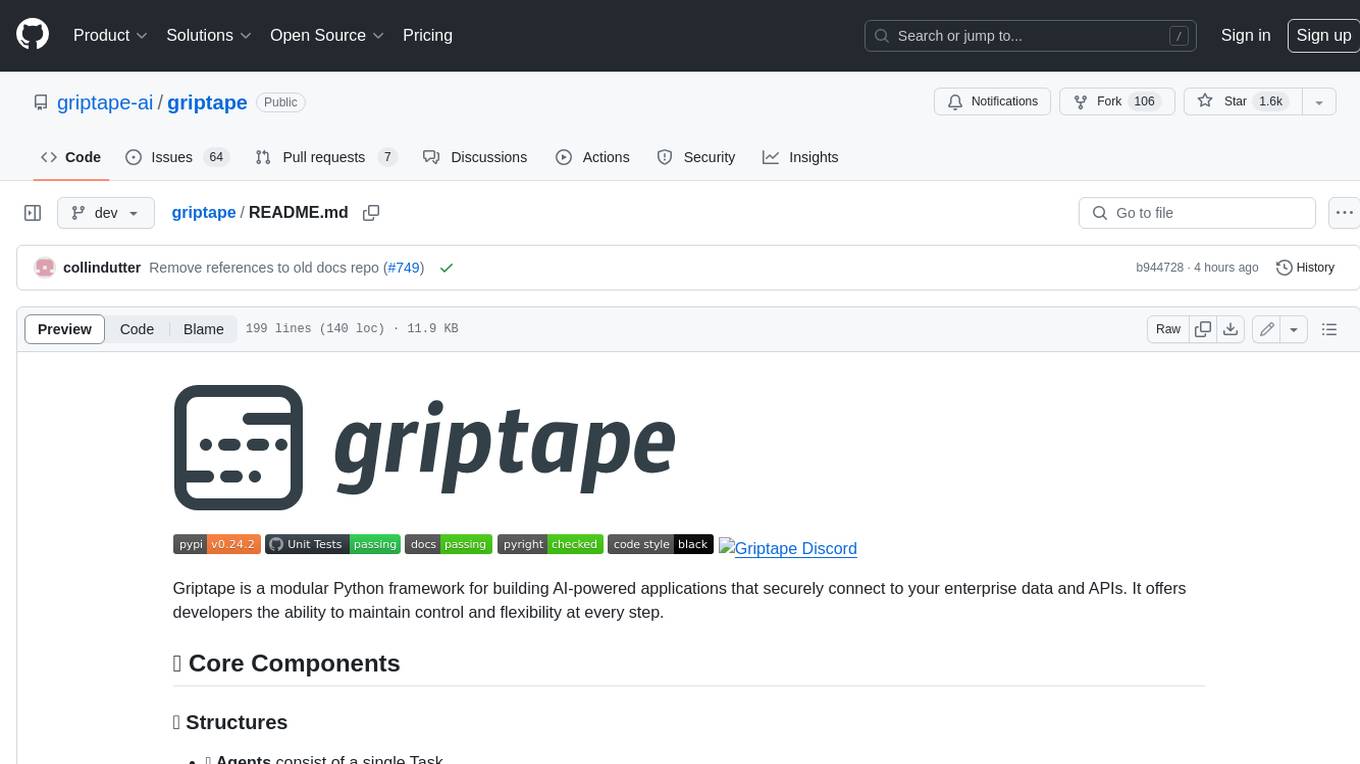
griptape
Griptape is a modular Python framework for building AI-powered applications that securely connect to your enterprise data and APIs. It offers developers the ability to maintain control and flexibility at every step. Griptape's core components include Structures (Agents, Pipelines, and Workflows), Tasks, Tools, Memory (Conversation Memory, Task Memory, and Meta Memory), Drivers (Prompt and Embedding Drivers, Vector Store Drivers, Image Generation Drivers, Image Query Drivers, SQL Drivers, Web Scraper Drivers, and Conversation Memory Drivers), Engines (Query Engines, Extraction Engines, Summary Engines, Image Generation Engines, and Image Query Engines), and additional components (Rulesets, Loaders, Artifacts, Chunkers, and Tokenizers). Griptape enables developers to create AI-powered applications with ease and efficiency.