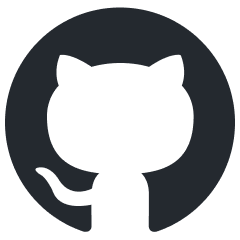
Gemini
The open source implementation of Gemini, the model that will "eclipse ChatGPT" by Google
Stars: 361
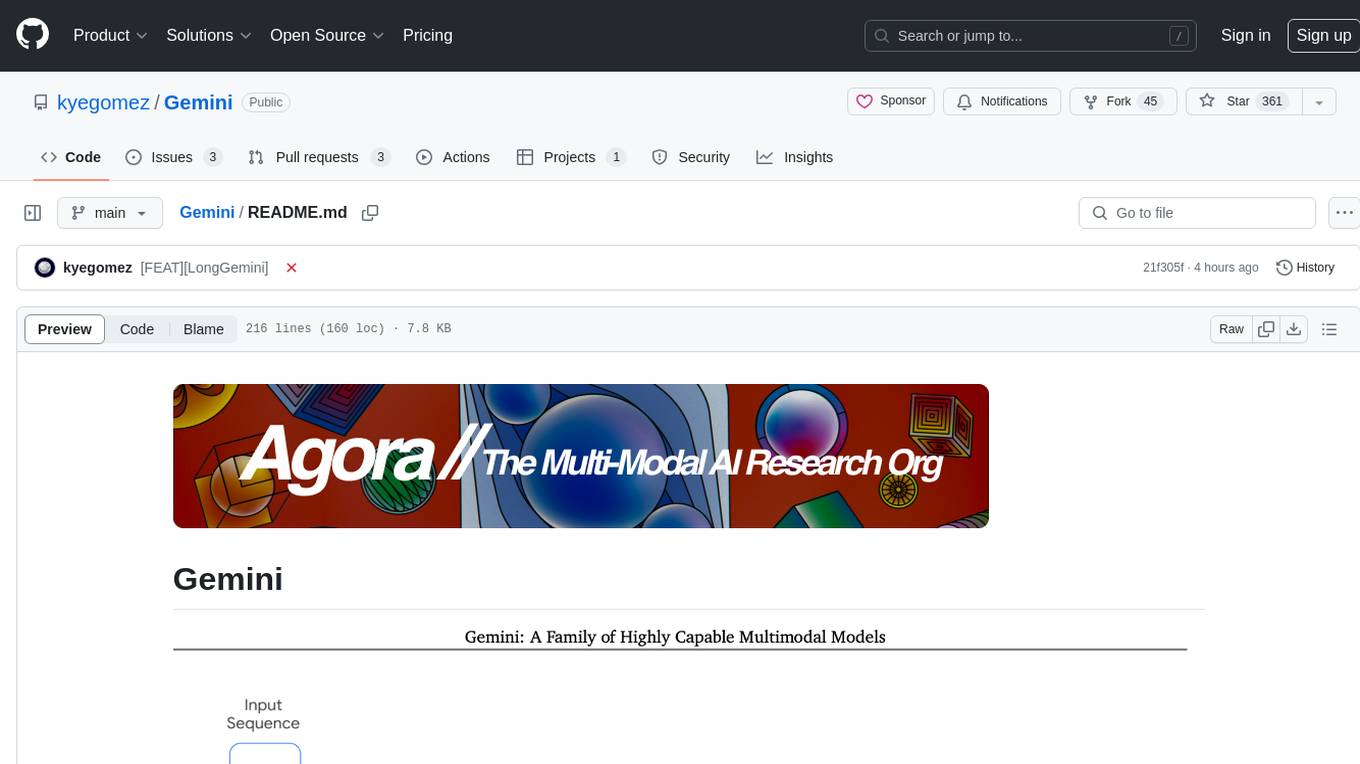
Gemini is an open-source model designed to handle multiple modalities such as text, audio, images, and videos. It utilizes a transformer architecture with special decoders for text and image generation. The model processes input sequences by transforming them into tokens and then decoding them to generate image outputs. Gemini differs from other models by directly feeding image embeddings into the transformer instead of using a visual transformer encoder. The model also includes a component called Codi for conditional generation. Gemini aims to effectively integrate image, audio, and video embeddings to enhance its performance.
README:
The open source implementation of Gemini, the model that will "eclipse ChatGPT", it seems to work by directly taking in all modalities all at once into a transformer with special decoders for text or img generation!
Join the Agora discord channel to help with the implementation! and Here is the project board:
The input sequences for Gemini consist of texts, audio, images, and videos. These inputs are transformed into tokens, which are then processed by a transformer. Subsequently, conditional decoding takes place to generate image outputs. Interestingly, the architecture of Gemini bears resemblance to Fuyu's architecture but is expanded to encompass multiple modalities. Instead of utilizing a visual transformer (vit) encoder, Gemini simply feeds image embeddings directly into the transformer. For Gemini, the token inputs will likely be indicated by special modality tokens such as [IMG], , [AUDIO], or . Codi, a component of Gemini, also employs conditional generation and makes use of the tokenized outputs. To implement this model effectively, I intend to initially focus on the image embeddings to ensure their smooth integration. Subsequently, I will proceed with incorporating audio embeddings and then video embeddings.
pip3 install gemini-torch
- Base transformer
- Multi Grouped Query Attn / flash attn
- rope
- alibi
- xpos
- qk norm
- no pos embeds
- kv cache
import torch
from gemini_torch.model import Gemini
# Initialize model with smaller dimensions
model = Gemini(
num_tokens=50432,
max_seq_len=4096, # Reduced from 8192
dim=1280, # Reduced from 2560
depth=16, # Reduced from 32
dim_head=64, # Reduced from 128
heads=12, # Reduced from 24
use_abs_pos_emb=False,
attn_flash=True,
attn_kv_heads=2,
qk_norm=True,
attn_qk_norm=True,
attn_qk_norm_dim_scale=True,
)
# Text shape: [batch, seq_len, dim]
text = torch.randint(0, 50432, (1, 4096)) # Reduced seq_len from 8192
# Apply model to text
y = model(
text,
)
# Output shape: [batch, seq_len, dim]
print(y)
- Processes images and audio through a series of reshapes
- Ready to train for production grade usage
- Hyper optimized with flash attention, qk norm, and other methods
import torch
from gemini_torch.model import Gemini
# Initialize model with smaller dimensions
model = Gemini(
num_tokens=10000, # Reduced from 50432
max_seq_len=1024, # Reduced from 4096
dim=320, # Reduced from 1280
depth=8, # Reduced from 16
dim_head=32, # Reduced from 64
heads=6, # Reduced from 12
use_abs_pos_emb=False,
attn_flash=True,
attn_kv_heads=2,
qk_norm=True,
attn_qk_norm=True,
attn_qk_norm_dim_scale=True,
post_fusion_norm=True,
post_modal_transform_norm=True,
)
# Text shape: [batch, seq_len, dim]
text = torch.randint(0, 10000, (1, 1024)) # Reduced seq_len from 4096
# Img shape: [batch, channels, height, width]
img = torch.randn(1, 3, 64, 64) # Reduced height and width from 128
# Audio shape: [batch, audio_seq_len, dim]
audio = torch.randn(1, 32) # Reduced audio_seq_len from 64
# Apply model to text and img
y, _ = model(text=text, img=img, audio=audio)
# Output shape: [batch, seq_len, dim]
print(y)
print(y.shape)
# After much training
model.eval()
text = tokenize(texts)
logits = model(text)
text = detokenize(logits)
An implementation of Gemini with Ring Attention, no multi-modality processing yet.
import torch
from gemini_torch import LongGemini
# Text tokens
x = torch.randint(0, 10000, (1, 1024))
# Create an instance of the LongGemini model
model = LongGemini(
dim=512, # Dimension of the input tensor
depth=32, # Number of transformer blocks
dim_head=128, # Dimension of the query, key, and value vectors
long_gemini_depth=9, # Number of long gemini transformer blocks
heads=24, # Number of attention heads
qk_norm=True, # Whether to apply layer normalization to query and key vectors
ring_seq_size=512, # The size of the ring sequence
)
# Apply the model to the input tensor
out = model(x)
# Print the output tensor
print(out)
- Sentencepiece, tokenizer
- We're using the same tokenizer as LLAMA with special tokens denoting the beginning and end of the multi modality tokens.
- Does not fully process img, audio, or videos now we need help on that
from gemini_torch.tokenizer import MultimodalSentencePieceTokenizer
# Example usage
tokenizer_name = "hf-internal-testing/llama-tokenizer"
tokenizer = MultimodalSentencePieceTokenizer(tokenizer_name=tokenizer_name)
# Encoding and decoding examples
encoded_audio = tokenizer.encode("Audio description", modality="audio")
decoded_audio = tokenizer.decode(encoded_audio)
print("Encoded audio:", encoded_audio)
print("Decoded audio:", decoded_audio)
- Combine Reinforcment learning with modular pretrained transformer, multi-modal capabilities, image, audio,
- self improving mechanisms like robocat
- PPO? or MPO
- get good at backtracking and exploring alternative paths
- speculative decoding
- Algorithm of Thoughts
- RLHF
- Gemini Report
- Gemini Landing Page
-
[x] Implement the img feature embedder and align imgs with text and pass into transformer:
Gemini models are trained to accommodate textual input interleaved with a wide variety of audio and visual inputs, such as natural images, charts, screenshots, PDFs, and videos, and they can produce text and image outputs (see Figure 2). The visual encoding of Gemini models is inspired by our own foundational work on Flamingo (Alayrac et al., 2022), CoCa (Yu et al., 2022a), and PaLI (Chen et al., 2022), with the important distinction that the models are multimodal from the beginning and can natively output images using discrete image tokens (Ramesh et al., 2021; Yu et al., 2022b).
-
[x] Implement the audio processing using USM by Google:
In addition, Gemini can directly ingest audio signals at 16kHz from Universal Speech Model (USM) (Zhang et al., 2023) features. This enables the model to capture nuances that are typically lost when the audio is naively mapped to a text input (for example, see audio understanding demo on the website).
-
[ ] Video Processing Technique: " Video understanding is accomplished by encoding the video as a sequence of frames in the large context window. Video frames or images can be interleaved naturally with text or audio as part of the model input"
-
[ ] Prompting Technique:
We find Gemini Ultra achieves highest accuracy when used in combination with a chain-of-thought prompting approach (Wei et al., 2022) that accounts for model uncertainty. The model produces a chain of thought with k samples, for example 8 or 32. If there is a consensus above a preset threshold (selected based on the validation split), it selects this answer, otherwise it reverts to a greedy sample based on maximum likelihood choice without chain of thought. We refer the reader to appendix for a detailed breakdown of how this approach compares with only chain-of-thought prompting or only greedy sampling.
-
[ ] Train a 1.8B + 3.25 Model:
Nano-1 and Nano-2 model sizes are only 1.8B and 3.25B parameters respectively. Despite their size, they show exceptionally strong performance on factuality, i.e. retrieval-related tasks, and significant performance on reasoning, STEM, coding, multimodal and
For Tasks:
Click tags to check more tools for each tasksFor Jobs:
Alternative AI tools for Gemini
Similar Open Source Tools
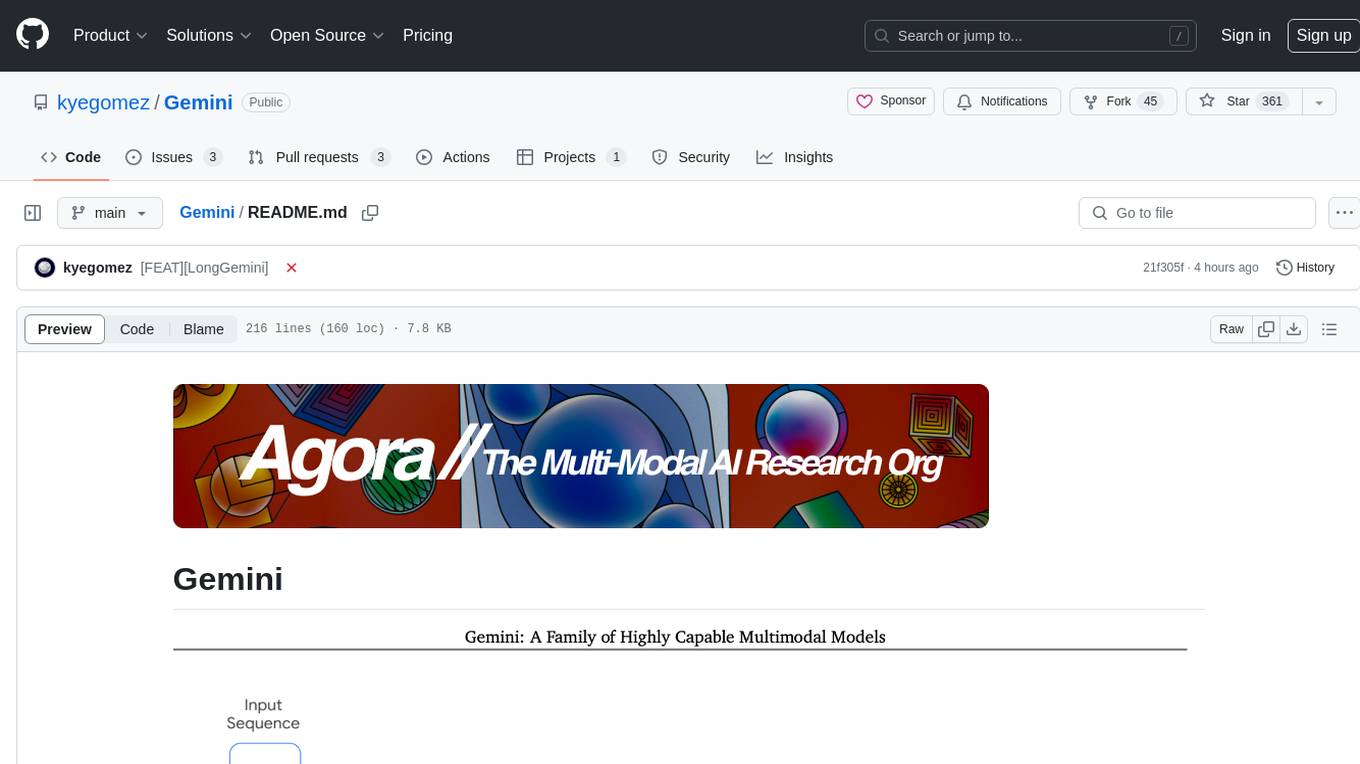
Gemini
Gemini is an open-source model designed to handle multiple modalities such as text, audio, images, and videos. It utilizes a transformer architecture with special decoders for text and image generation. The model processes input sequences by transforming them into tokens and then decoding them to generate image outputs. Gemini differs from other models by directly feeding image embeddings into the transformer instead of using a visual transformer encoder. The model also includes a component called Codi for conditional generation. Gemini aims to effectively integrate image, audio, and video embeddings to enhance its performance.
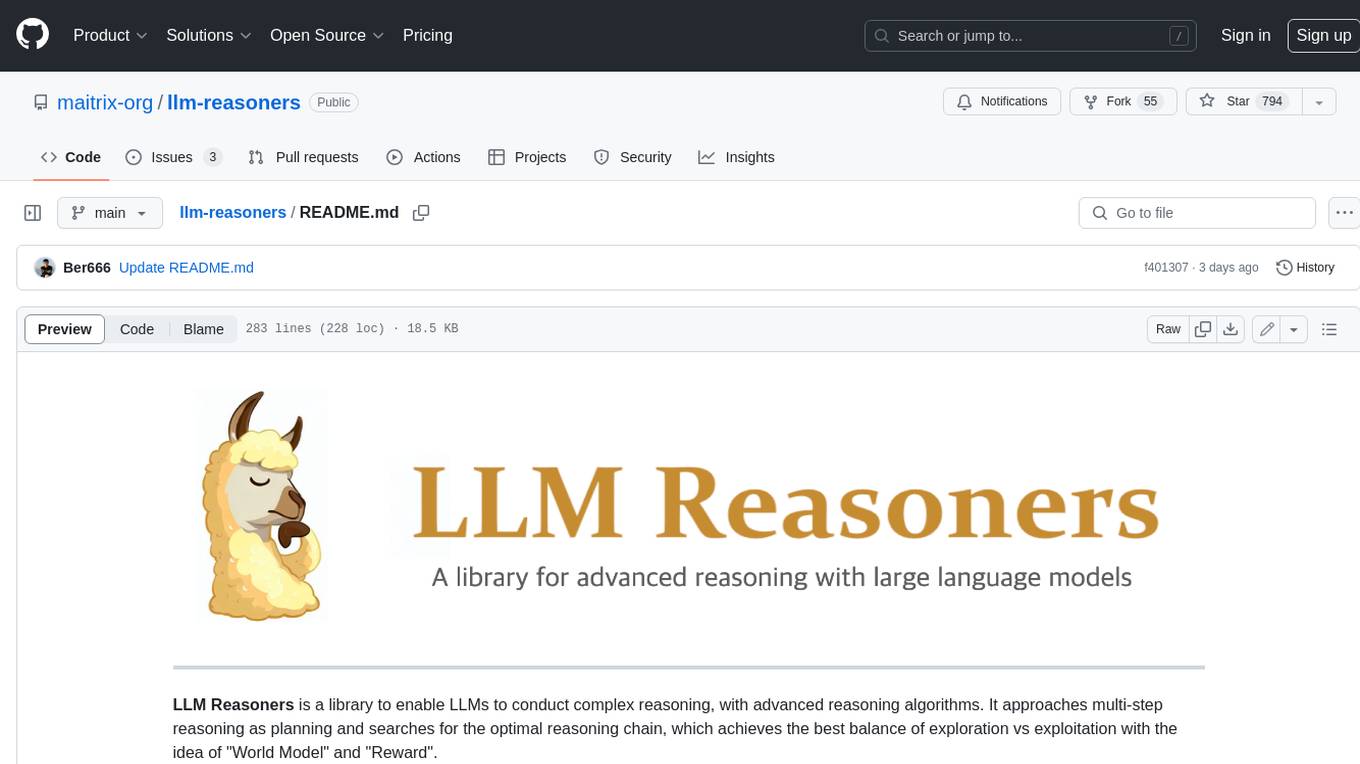
llm-reasoners
LLM Reasoners is a library that enables LLMs to conduct complex reasoning, with advanced reasoning algorithms. It approaches multi-step reasoning as planning and searches for the optimal reasoning chain, which achieves the best balance of exploration vs exploitation with the idea of "World Model" and "Reward". Given any reasoning problem, simply define the reward function and an optional world model (explained below), and let LLM reasoners take care of the rest, including Reasoning Algorithms, Visualization, LLM calling, and more!
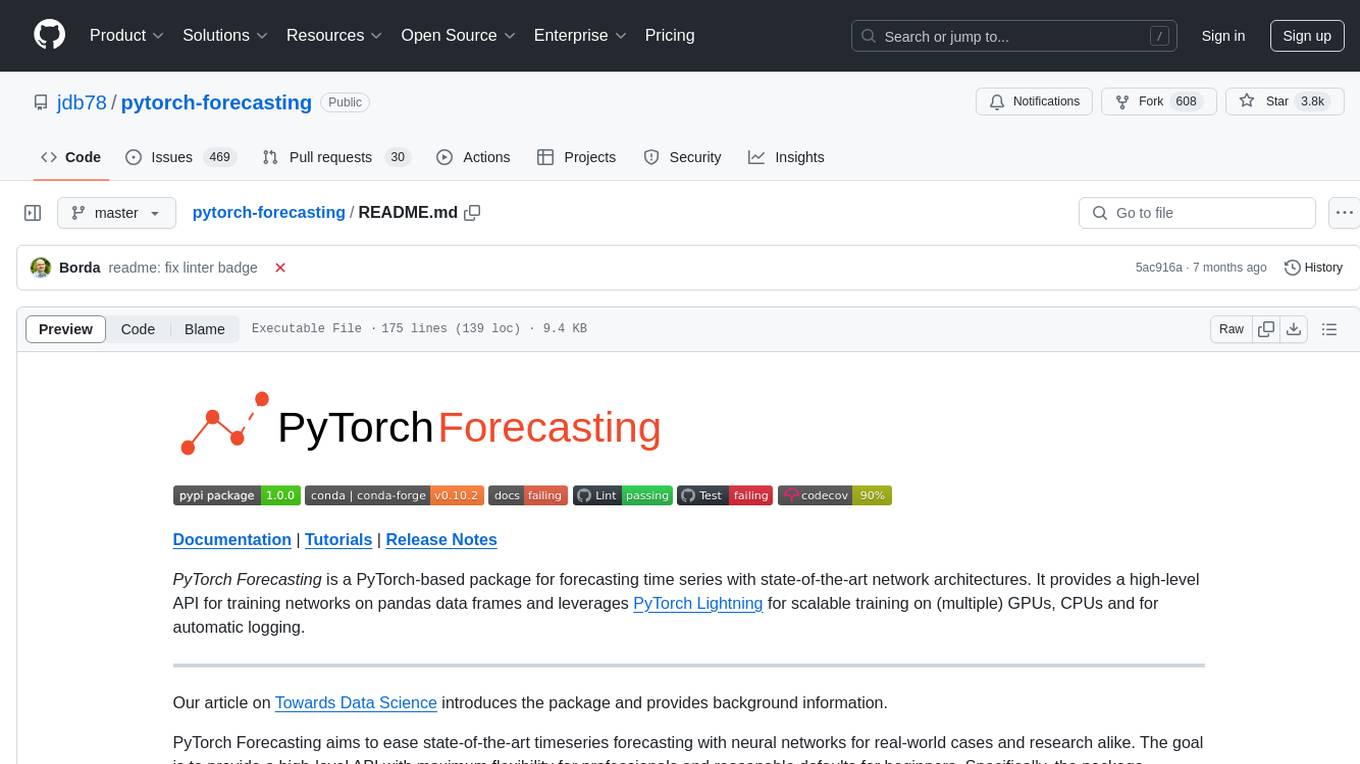
pytorch-forecasting
PyTorch Forecasting is a PyTorch-based package for time series forecasting with state-of-the-art network architectures. It offers a high-level API for training networks on pandas data frames and utilizes PyTorch Lightning for scalable training on GPUs and CPUs. The package aims to simplify time series forecasting with neural networks by providing a flexible API for professionals and default settings for beginners. It includes a timeseries dataset class, base model class, multiple neural network architectures, multi-horizon timeseries metrics, and hyperparameter tuning with optuna. PyTorch Forecasting is built on pytorch-lightning for easy training on various hardware configurations.
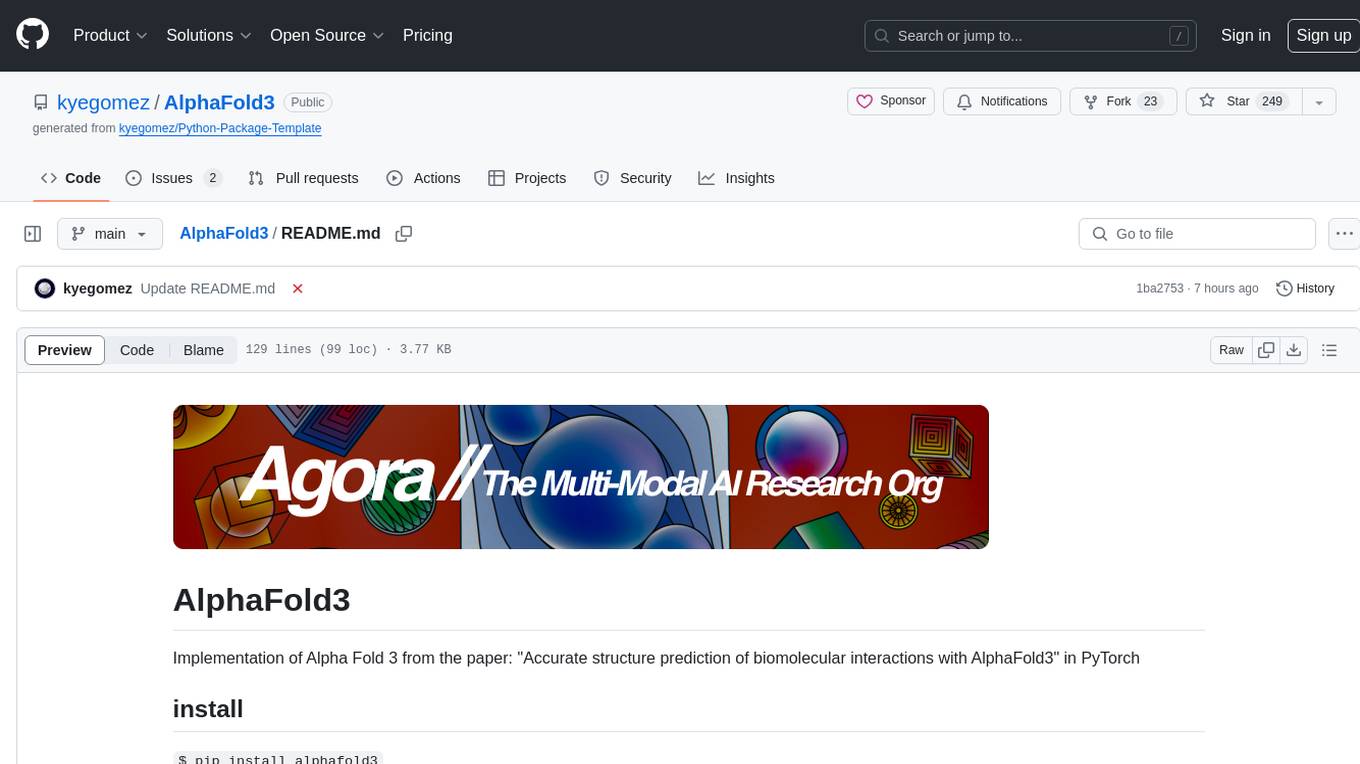
AlphaFold3
AlphaFold3 is an implementation of the Alpha Fold 3 model in PyTorch for accurate structure prediction of biomolecular interactions. It includes modules for genetic diffusion and full model examples for forward pass computations. The tool allows users to generate random pair and single representations, operate on atomic coordinates, and perform structure predictions based on input tensors. The implementation also provides functionalities for training and evaluating the model.
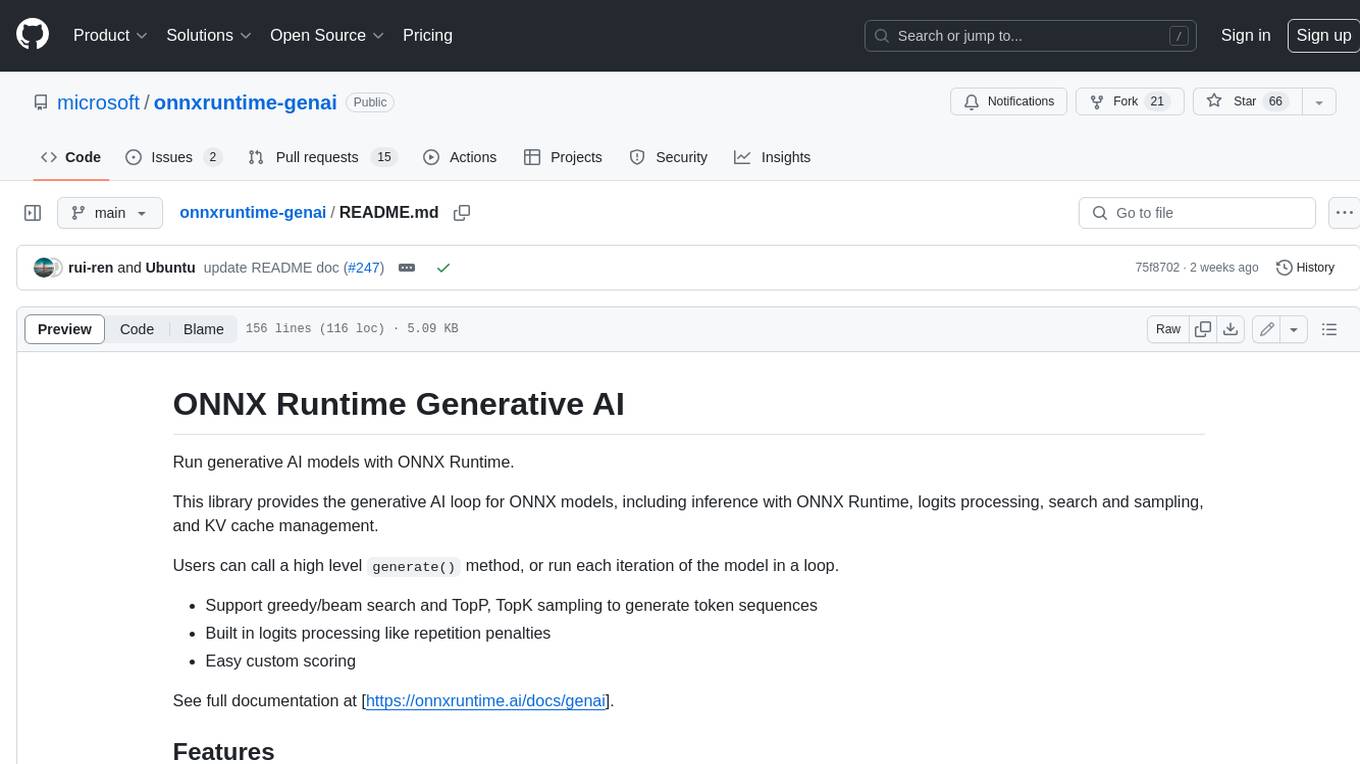
onnxruntime-genai
ONNX Runtime Generative AI is a library that provides the generative AI loop for ONNX models, including inference with ONNX Runtime, logits processing, search and sampling, and KV cache management. Users can call a high level `generate()` method, or run each iteration of the model in a loop. It supports greedy/beam search and TopP, TopK sampling to generate token sequences, has built in logits processing like repetition penalties, and allows for easy custom scoring.
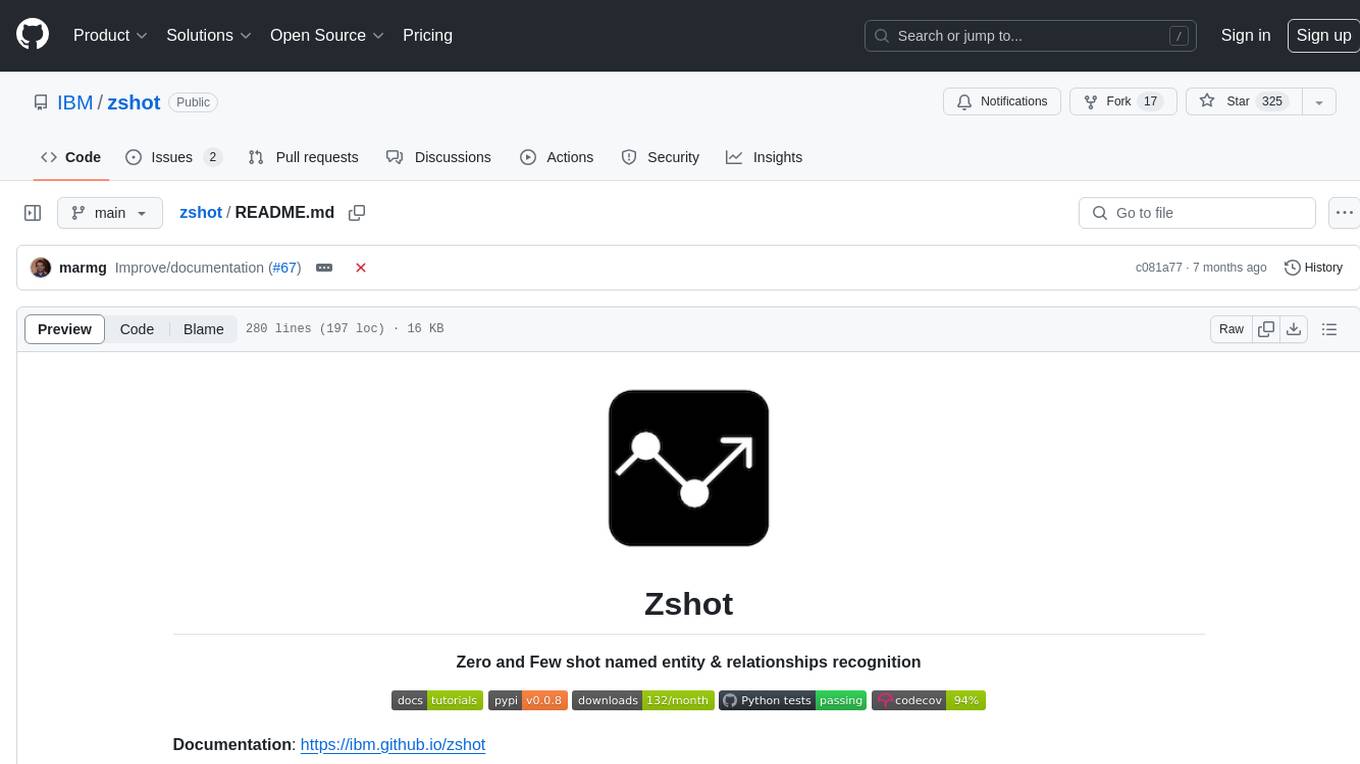
zshot
Zshot is a highly customizable framework for performing Zero and Few shot named entity and relationships recognition. It can be used for mentions extraction, wikification, zero and few shot named entity recognition, zero and few shot named relationship recognition, and visualization of zero-shot NER and RE extraction. The framework consists of two main components: the mentions extractor and the linker. There are multiple mentions extractors and linkers available, each serving a specific purpose. Zshot also includes a relations extractor and a knowledge extractor for extracting relations among entities and performing entity classification. The tool requires Python 3.6+ and dependencies like spacy, torch, transformers, evaluate, and datasets for evaluation over datasets like OntoNotes. Optional dependencies include flair and blink for additional functionalities. Zshot provides examples, tutorials, and evaluation methods to assess the performance of the components.
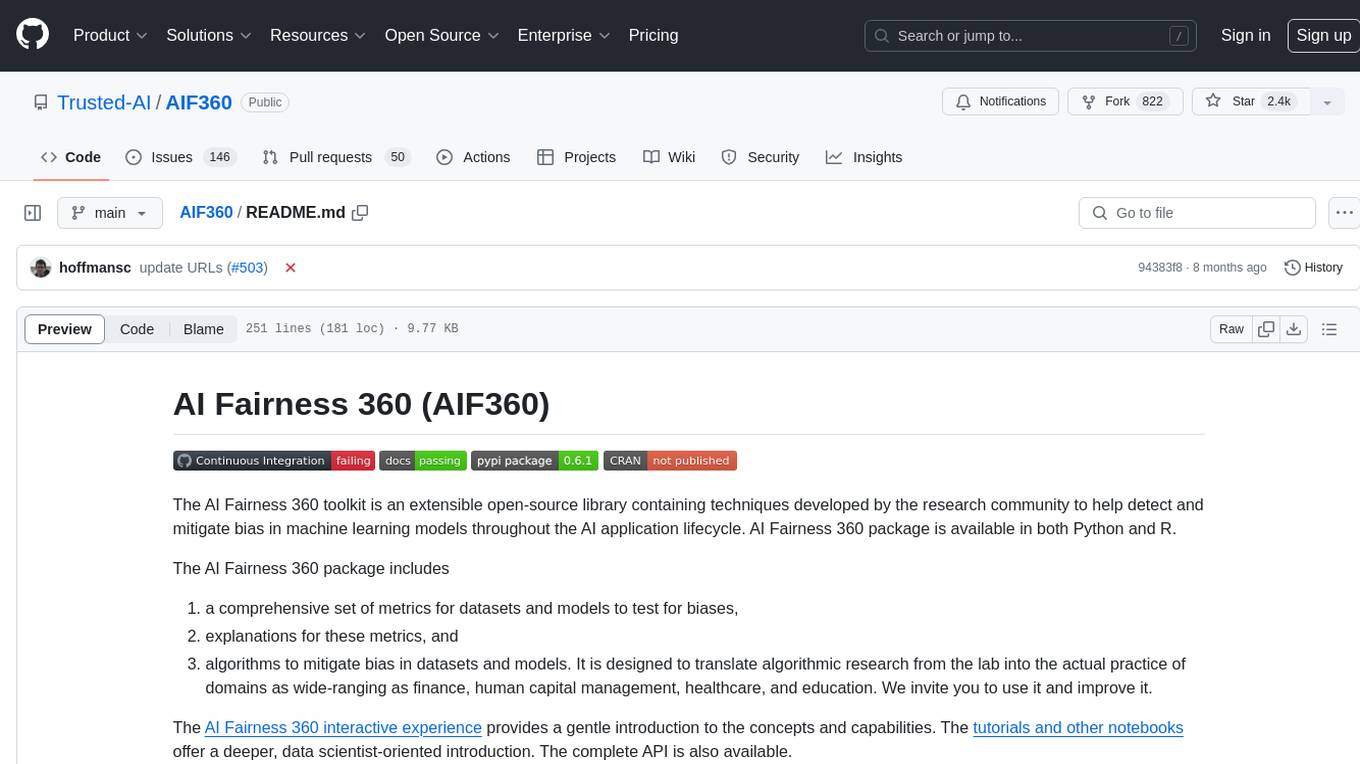
AIF360
The AI Fairness 360 toolkit is an open-source library designed to detect and mitigate bias in machine learning models. It provides a comprehensive set of metrics, explanations, and algorithms for bias mitigation in various domains such as finance, healthcare, and education. The toolkit supports multiple bias mitigation algorithms and fairness metrics, and is available in both Python and R. Users can leverage the toolkit to ensure fairness in AI applications and contribute to its development for extensibility.
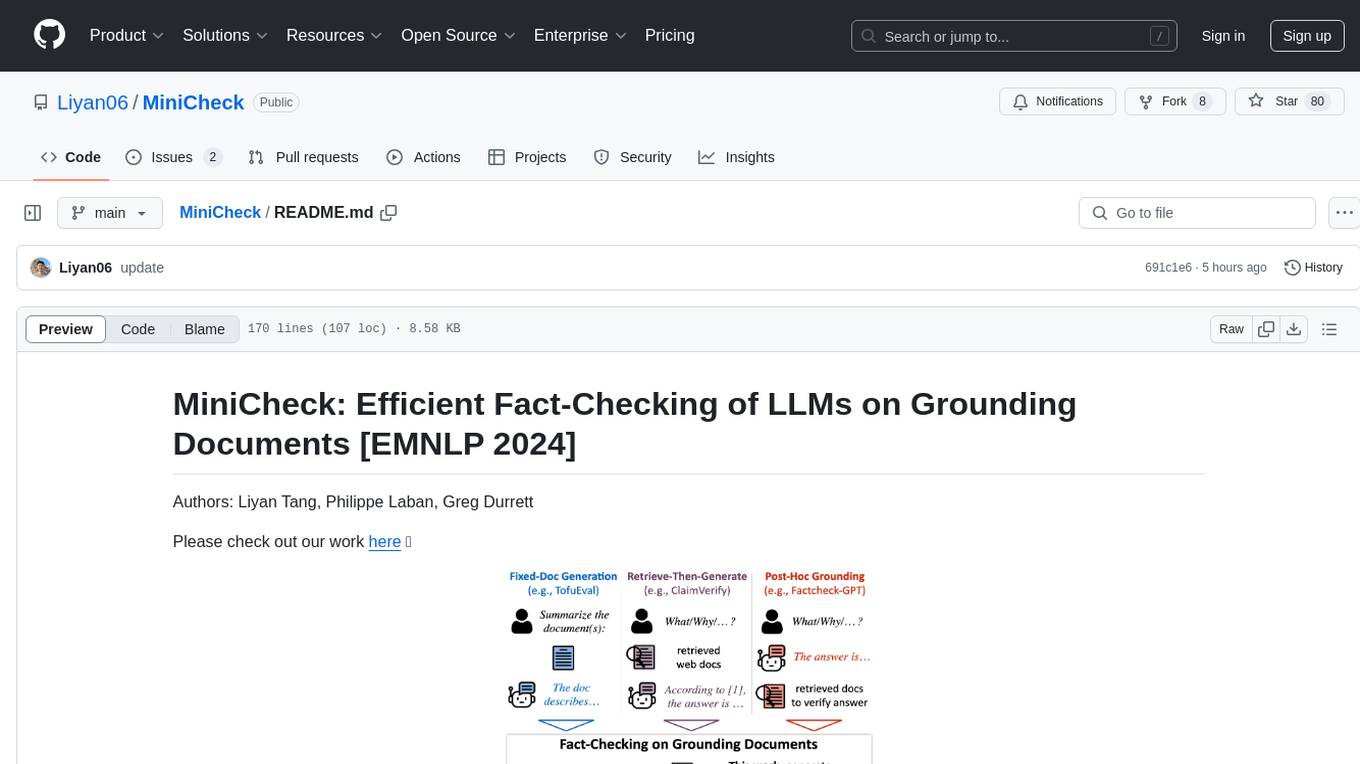
MiniCheck
MiniCheck is an efficient fact-checking tool designed to verify claims against grounding documents using large language models. It provides a sentence-level fact-checking model that can be used to evaluate the consistency of claims with the provided documents. MiniCheck offers different models, including Bespoke-MiniCheck-7B, which is the state-of-the-art and commercially usable. The tool enables users to fact-check multi-sentence claims by breaking them down into individual sentences for optimal performance. It also supports automatic prefix caching for faster inference when repeatedly fact-checking the same document with different claims.
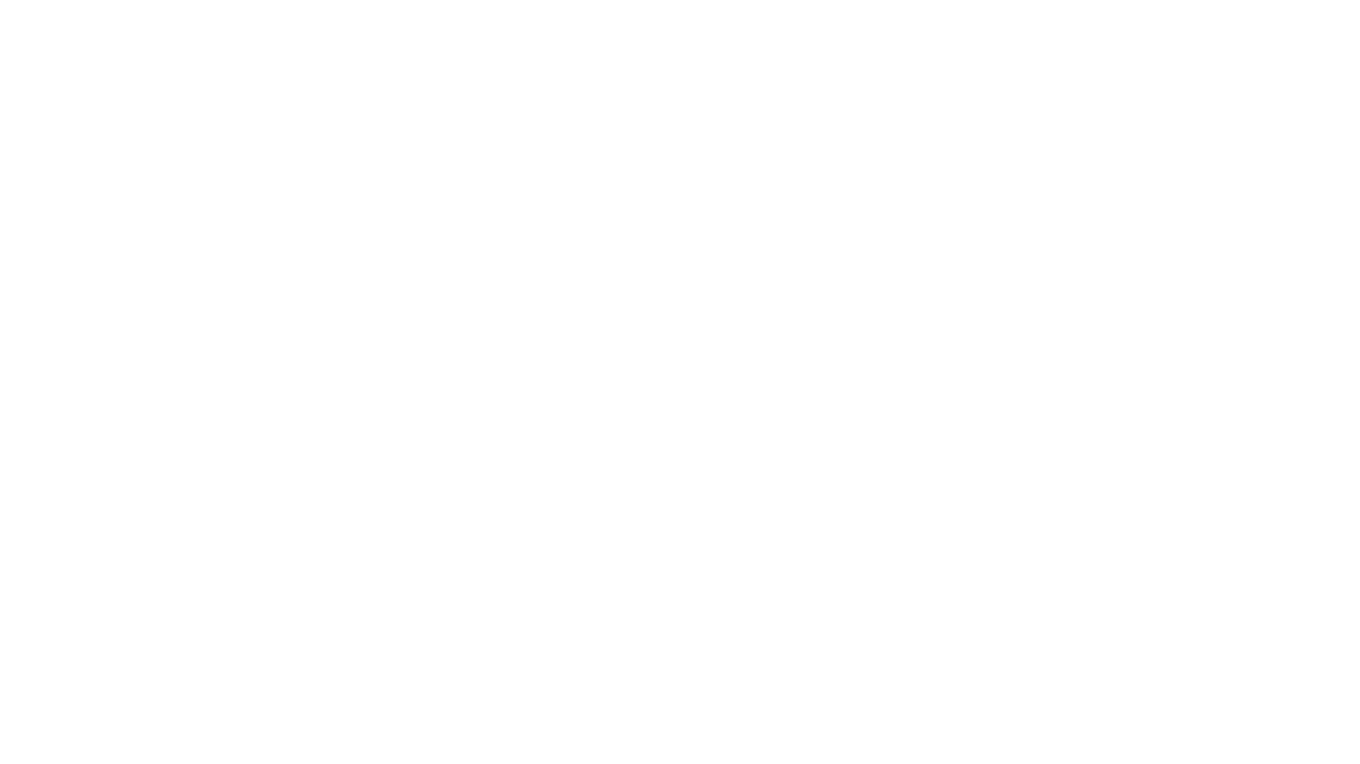
only_train_once
Only Train Once (OTO) is an automatic, architecture-agnostic DNN training and compression framework that allows users to train a general DNN from scratch or a pretrained checkpoint to achieve high performance and slimmer architecture simultaneously in a one-shot manner without fine-tuning. The framework includes features for automatic structured pruning and erasing operators, as well as hybrid structured sparse optimizers for efficient model compression. OTO provides tools for pruning zero-invariant group partitioning, constructing pruned models, and visualizing pruning and erasing dependency graphs. It supports the HESSO optimizer and offers a sanity check for compliance testing on various DNNs. The repository also includes publications, installation instructions, quick start guides, and a roadmap for future enhancements and collaborations.
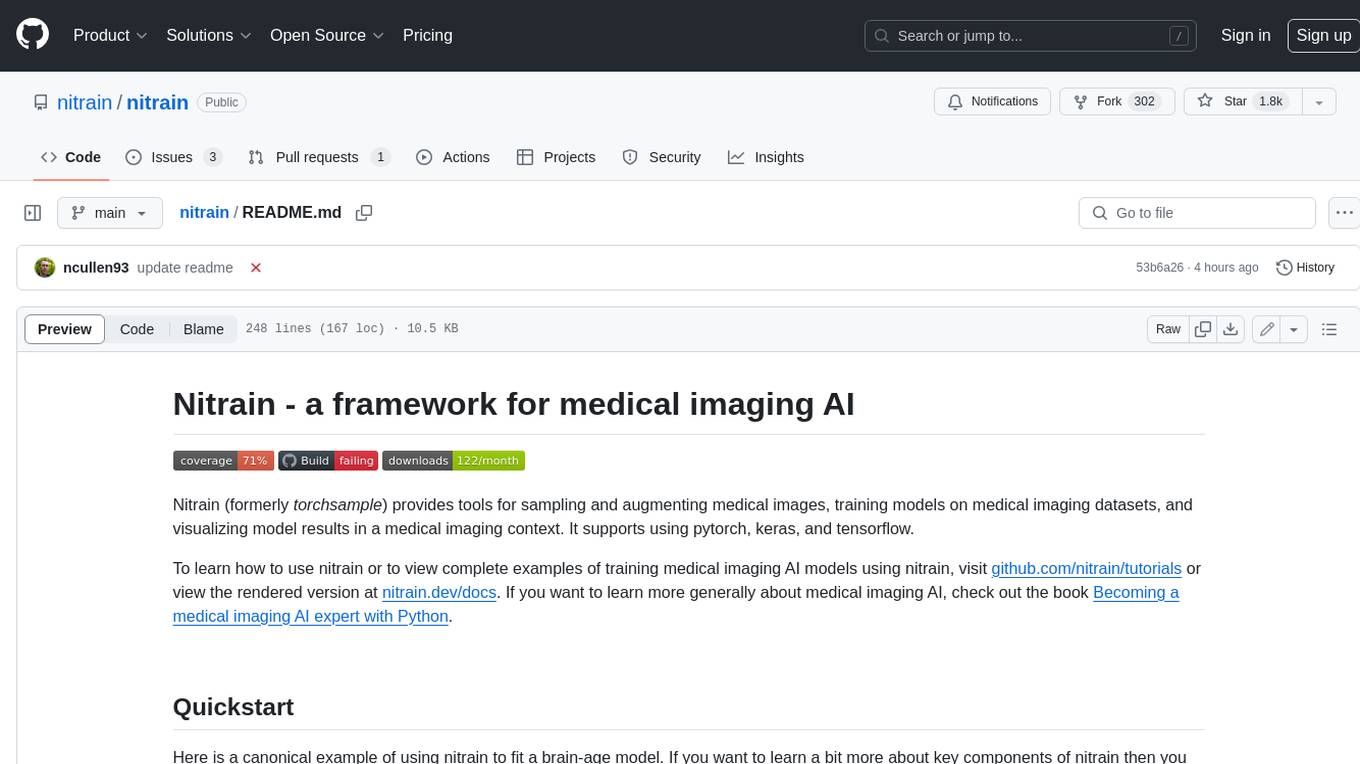
nitrain
Nitrain is a framework for medical imaging AI that provides tools for sampling and augmenting medical images, training models on medical imaging datasets, and visualizing model results in a medical imaging context. It supports using pytorch, keras, and tensorflow.
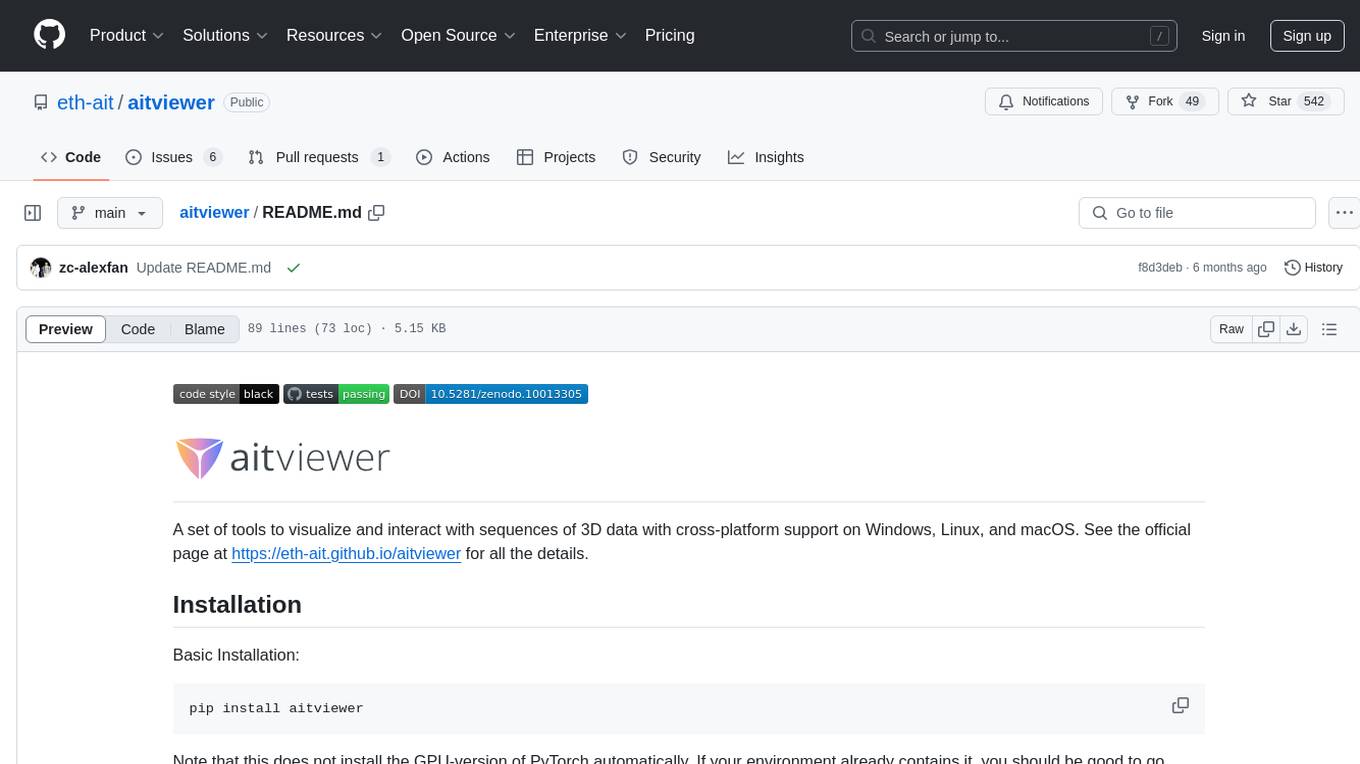
aitviewer
A set of tools to visualize and interact with sequences of 3D data with cross-platform support on Windows, Linux, and macOS. It provides a native Python interface for loading and displaying SMPL[-H/-X], MANO, FLAME, STAR, and SUPR sequences in an interactive viewer. Users can render 3D data on top of images, edit SMPL sequences and poses, export screenshots and videos, and utilize a high-performance ModernGL-based rendering pipeline. The tool is designed for easy use and hacking, with features like headless mode, remote mode, animatable camera paths, and a built-in extensible GUI.
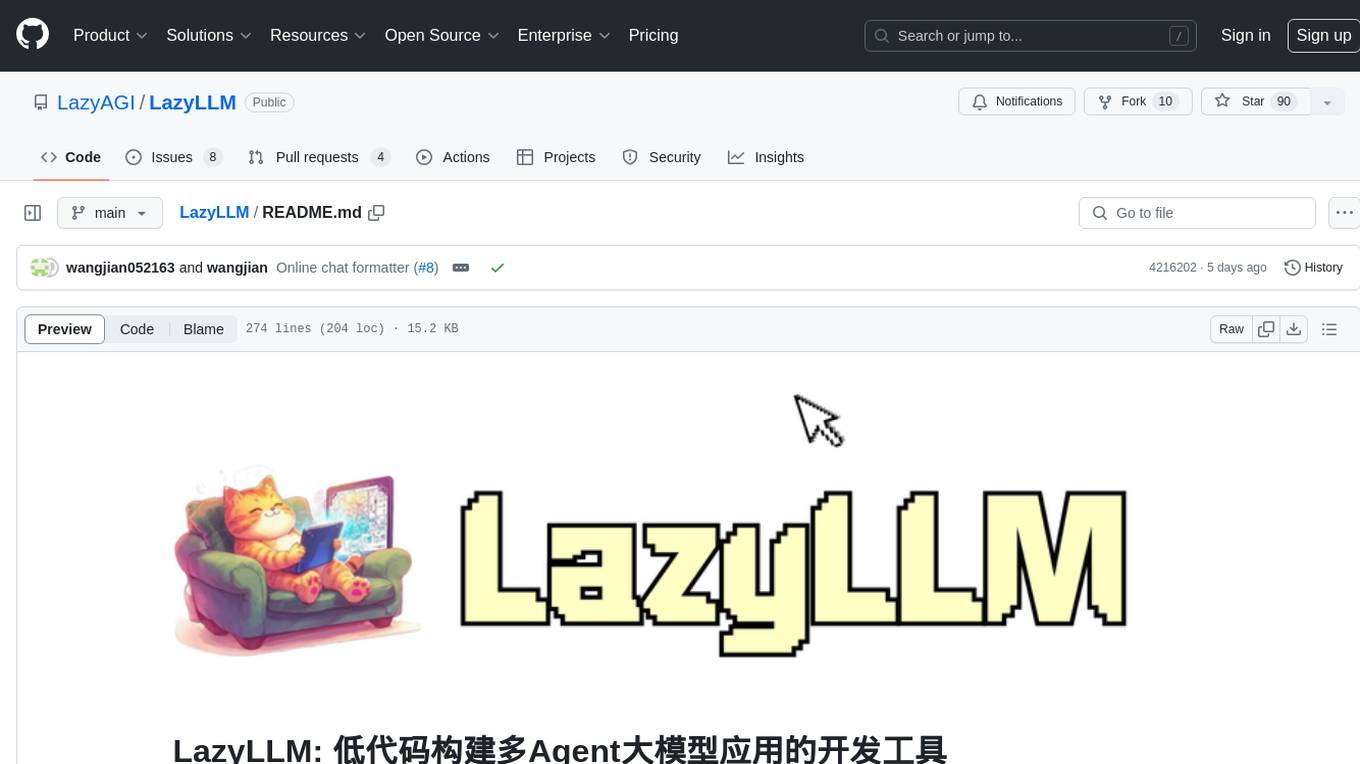
LazyLLM
LazyLLM is a low-code development tool for building complex AI applications with multiple agents. It assists developers in building AI applications at a low cost and continuously optimizing their performance. The tool provides a convenient workflow for application development and offers standard processes and tools for various stages of application development. Users can quickly prototype applications with LazyLLM, analyze bad cases with scenario task data, and iteratively optimize key components to enhance the overall application performance. LazyLLM aims to simplify the AI application development process and provide flexibility for both beginners and experts to create high-quality applications.
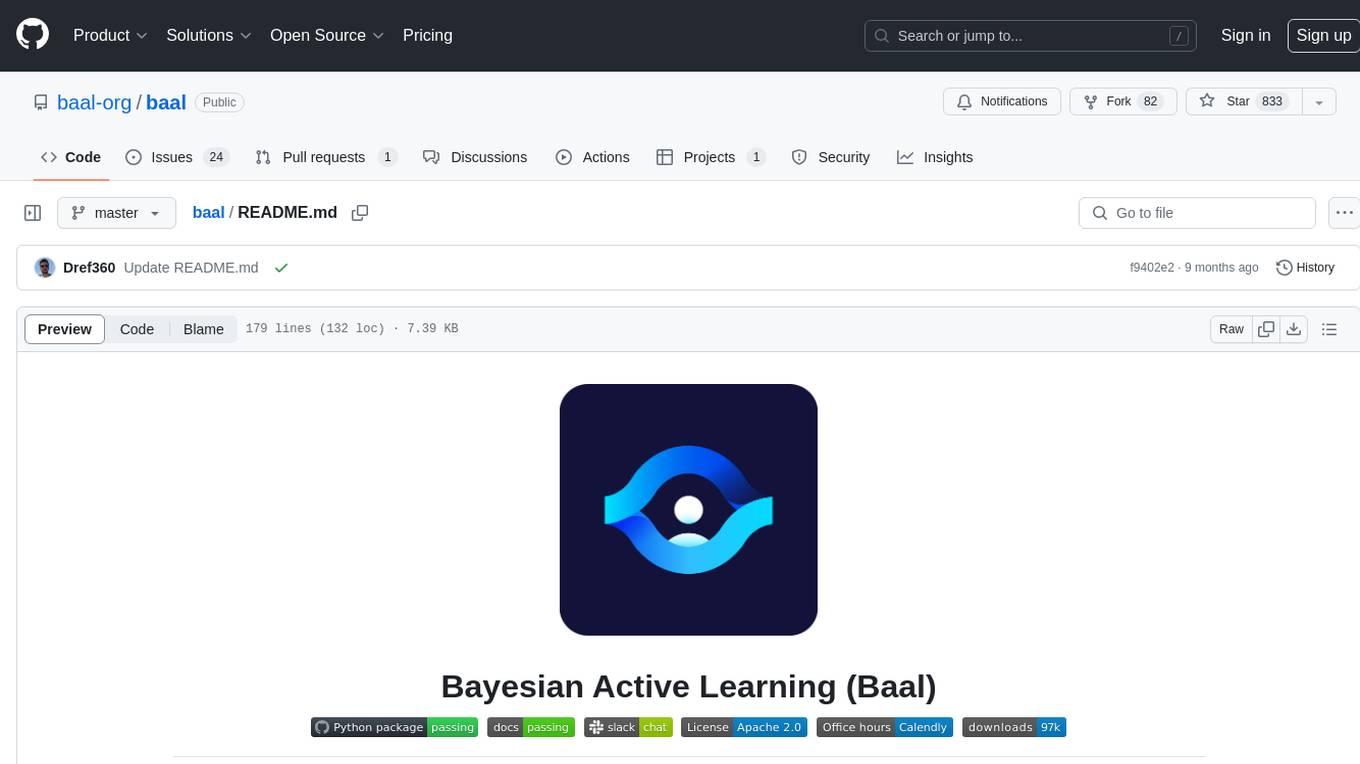
baal
Baal is an active learning library that supports both industrial applications and research use cases. It provides a framework for Bayesian active learning methods such as Monte-Carlo Dropout, MCDropConnect, Deep ensembles, and Semi-supervised learning. Baal helps in labeling the most uncertain items in the dataset pool to improve model performance and reduce annotation effort. The library is actively maintained by a dedicated team and has been used in various research papers for production and experimentation.
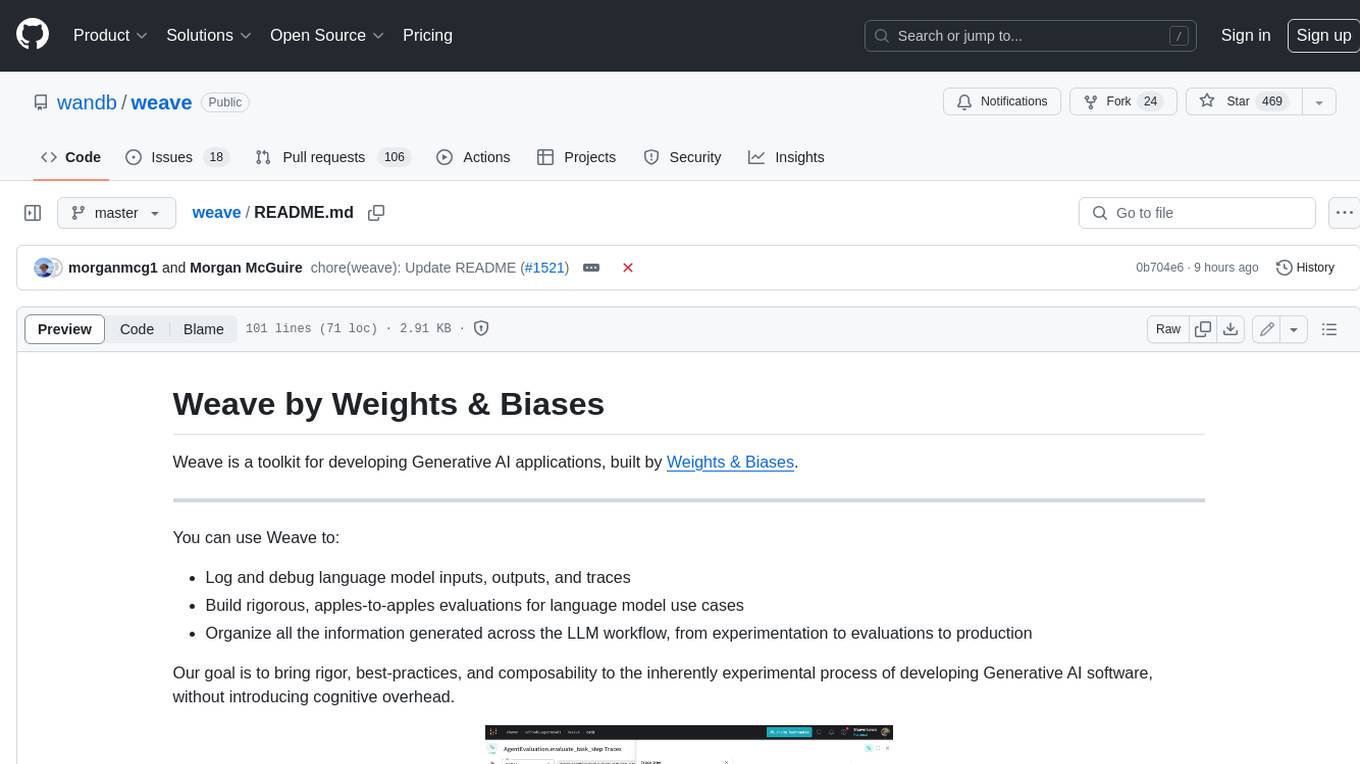
weave
Weave is a toolkit for developing Generative AI applications, built by Weights & Biases. With Weave, you can log and debug language model inputs, outputs, and traces; build rigorous, apples-to-apples evaluations for language model use cases; and organize all the information generated across the LLM workflow, from experimentation to evaluations to production. Weave aims to bring rigor, best-practices, and composability to the inherently experimental process of developing Generative AI software, without introducing cognitive overhead.
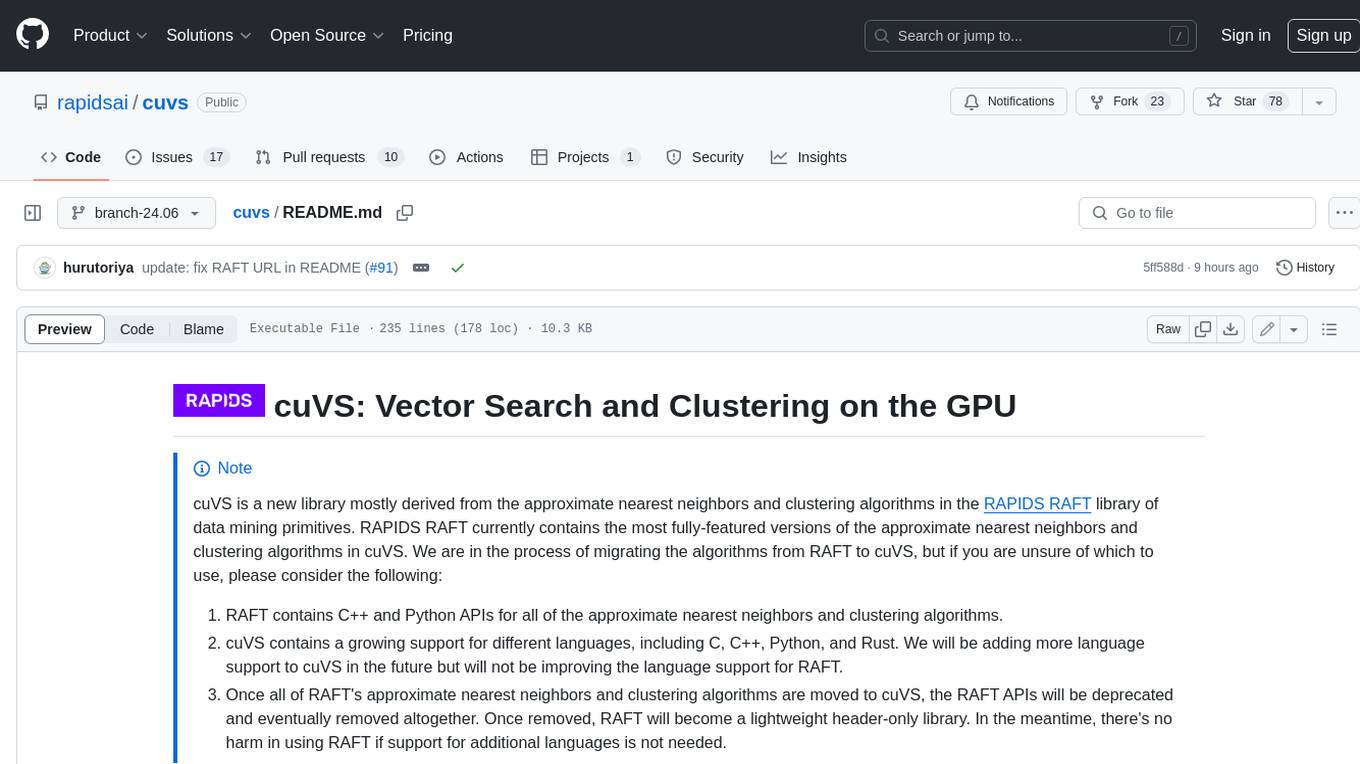
cuvs
cuVS is a library that contains state-of-the-art implementations of several algorithms for running approximate nearest neighbors and clustering on the GPU. It can be used directly or through the various databases and other libraries that have integrated it. The primary goal of cuVS is to simplify the use of GPUs for vector similarity search and clustering.
For similar tasks
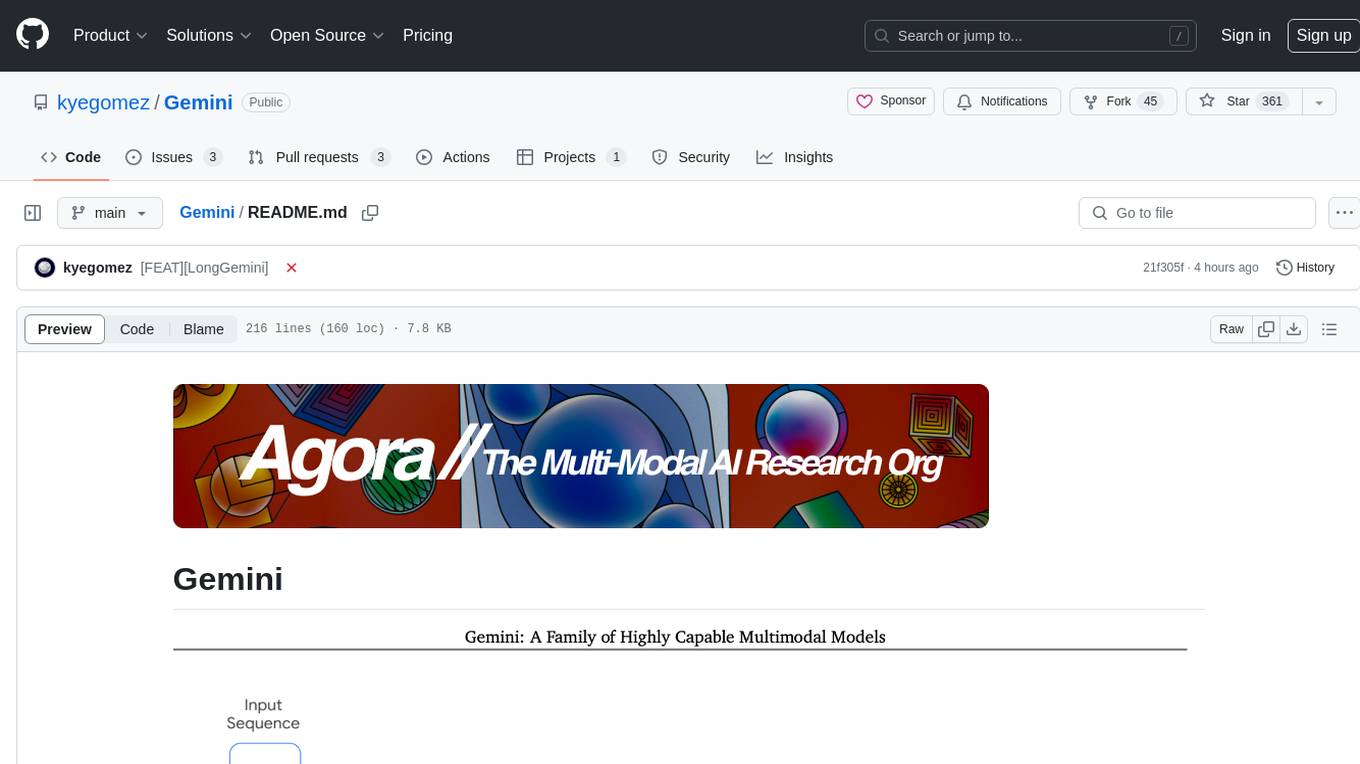
Gemini
Gemini is an open-source model designed to handle multiple modalities such as text, audio, images, and videos. It utilizes a transformer architecture with special decoders for text and image generation. The model processes input sequences by transforming them into tokens and then decoding them to generate image outputs. Gemini differs from other models by directly feeding image embeddings into the transformer instead of using a visual transformer encoder. The model also includes a component called Codi for conditional generation. Gemini aims to effectively integrate image, audio, and video embeddings to enhance its performance.
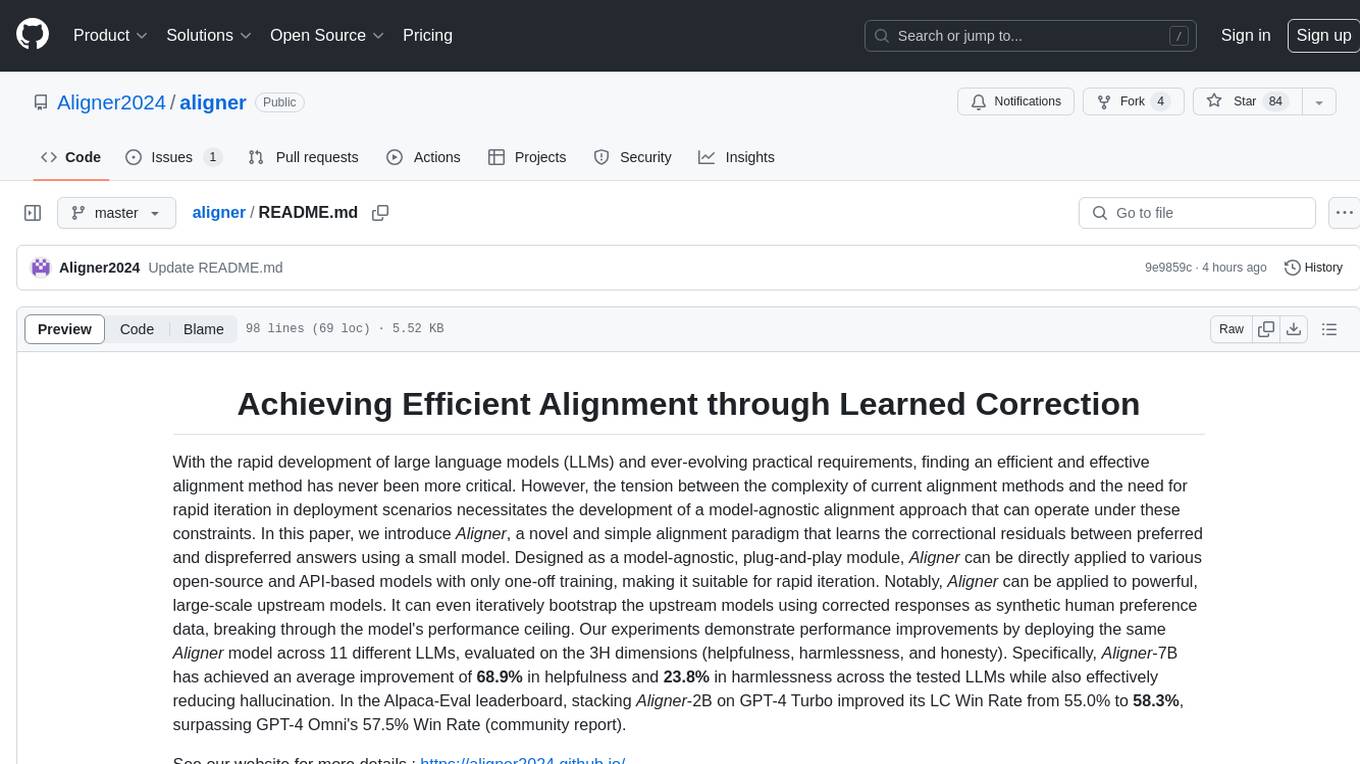
aligner
Aligner is a model-agnostic alignment tool that learns correctional residuals between preferred and dispreferred answers using a small model. It can be directly applied to various open-source and API-based models with only one-off training, suitable for rapid iteration and improving model performance. Aligner has shown significant improvements in helpfulness, harmlessness, and honesty dimensions across different large language models.
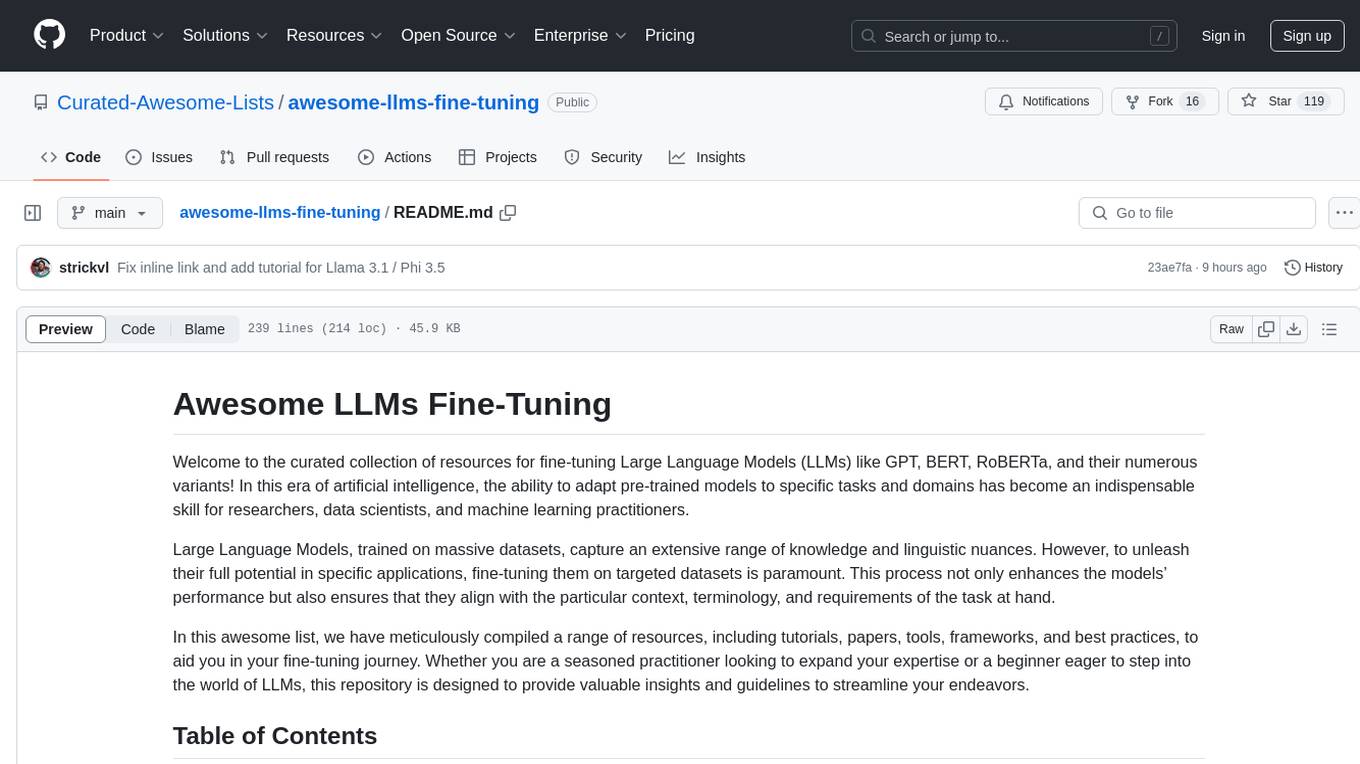
awesome-llms-fine-tuning
This repository is a curated collection of resources for fine-tuning Large Language Models (LLMs) like GPT, BERT, RoBERTa, and their variants. It includes tutorials, papers, tools, frameworks, and best practices to aid researchers, data scientists, and machine learning practitioners in adapting pre-trained models to specific tasks and domains. The resources cover a wide range of topics related to fine-tuning LLMs, providing valuable insights and guidelines to streamline the process and enhance model performance.
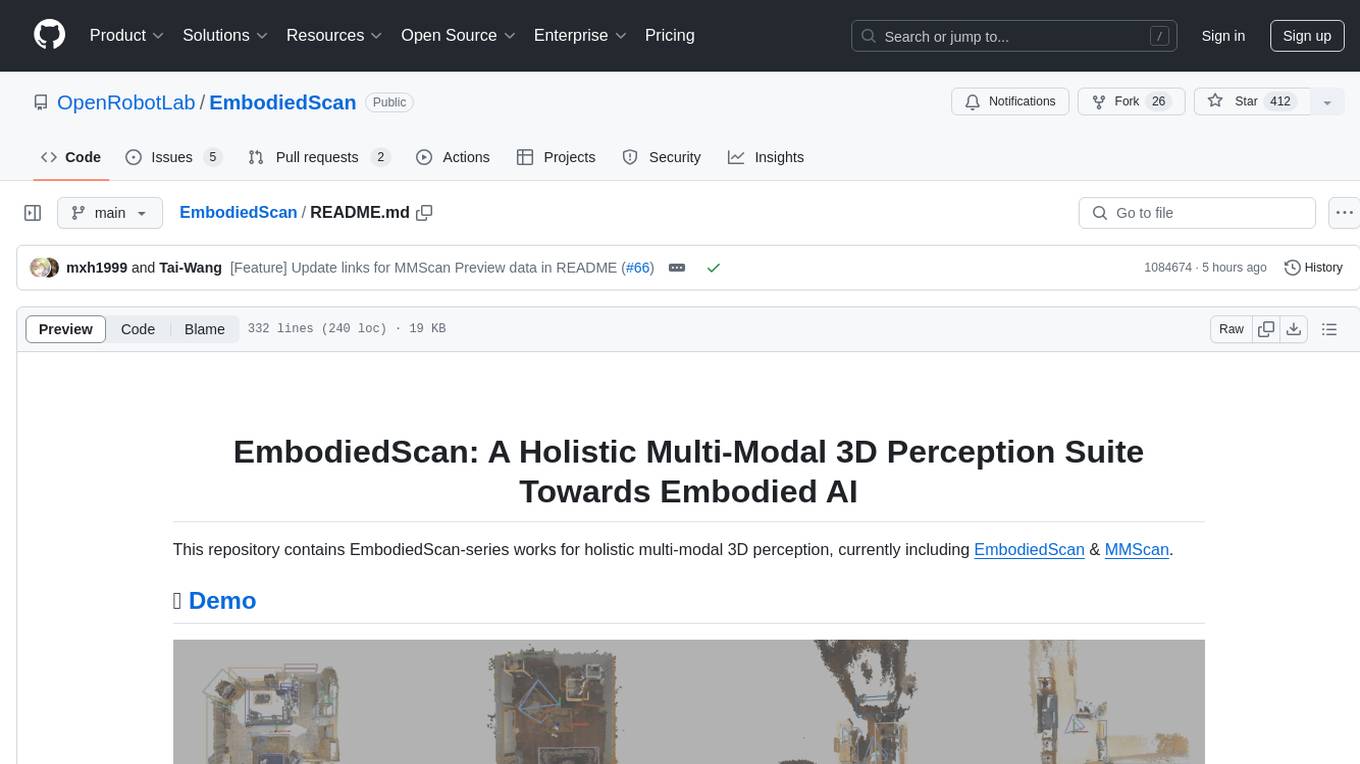
EmbodiedScan
EmbodiedScan is a holistic multi-modal 3D perception suite designed for embodied AI. It introduces a multi-modal, ego-centric 3D perception dataset and benchmark for holistic 3D scene understanding. The dataset includes over 5k scans with 1M ego-centric RGB-D views, 1M language prompts, 160k 3D-oriented boxes spanning 760 categories, and dense semantic occupancy with 80 common categories. The suite includes a baseline framework named Embodied Perceptron, capable of processing multi-modal inputs for 3D perception tasks and language-grounded tasks.
For similar jobs
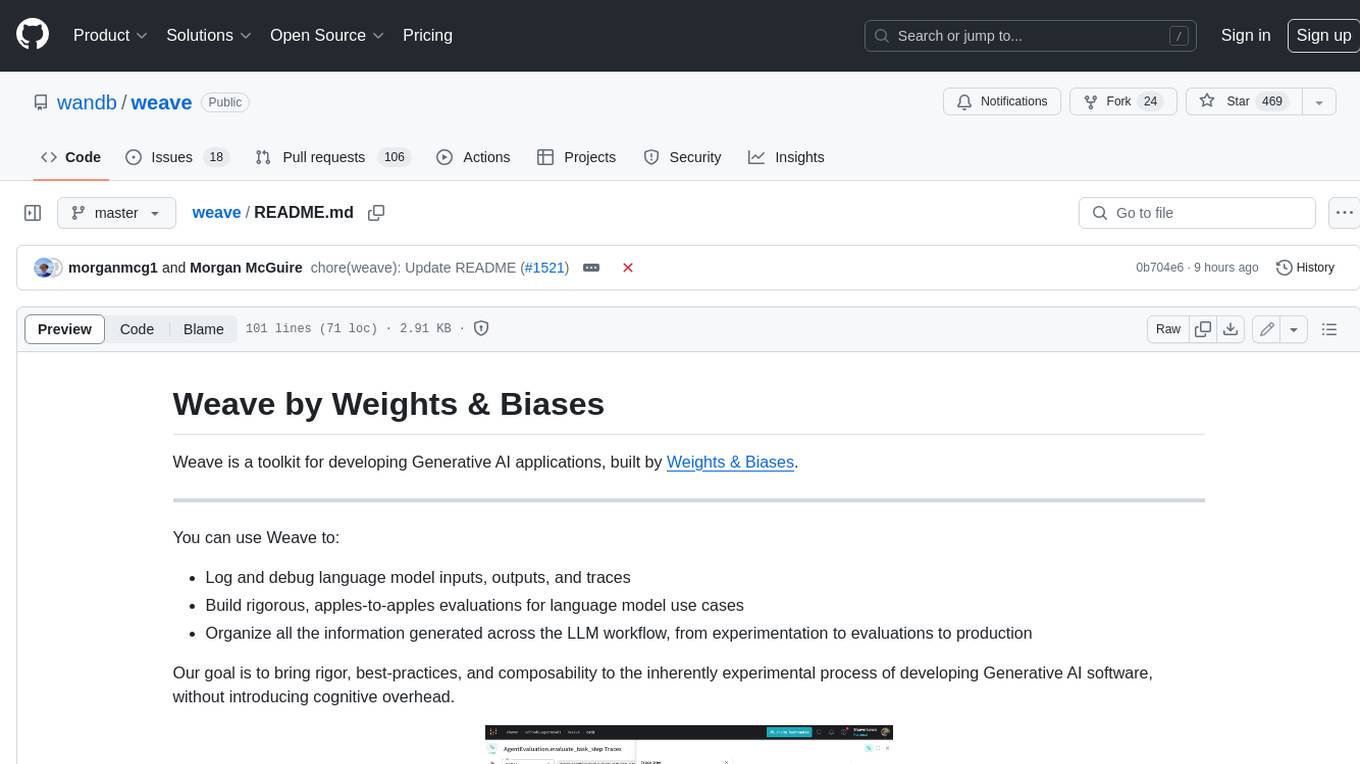
weave
Weave is a toolkit for developing Generative AI applications, built by Weights & Biases. With Weave, you can log and debug language model inputs, outputs, and traces; build rigorous, apples-to-apples evaluations for language model use cases; and organize all the information generated across the LLM workflow, from experimentation to evaluations to production. Weave aims to bring rigor, best-practices, and composability to the inherently experimental process of developing Generative AI software, without introducing cognitive overhead.
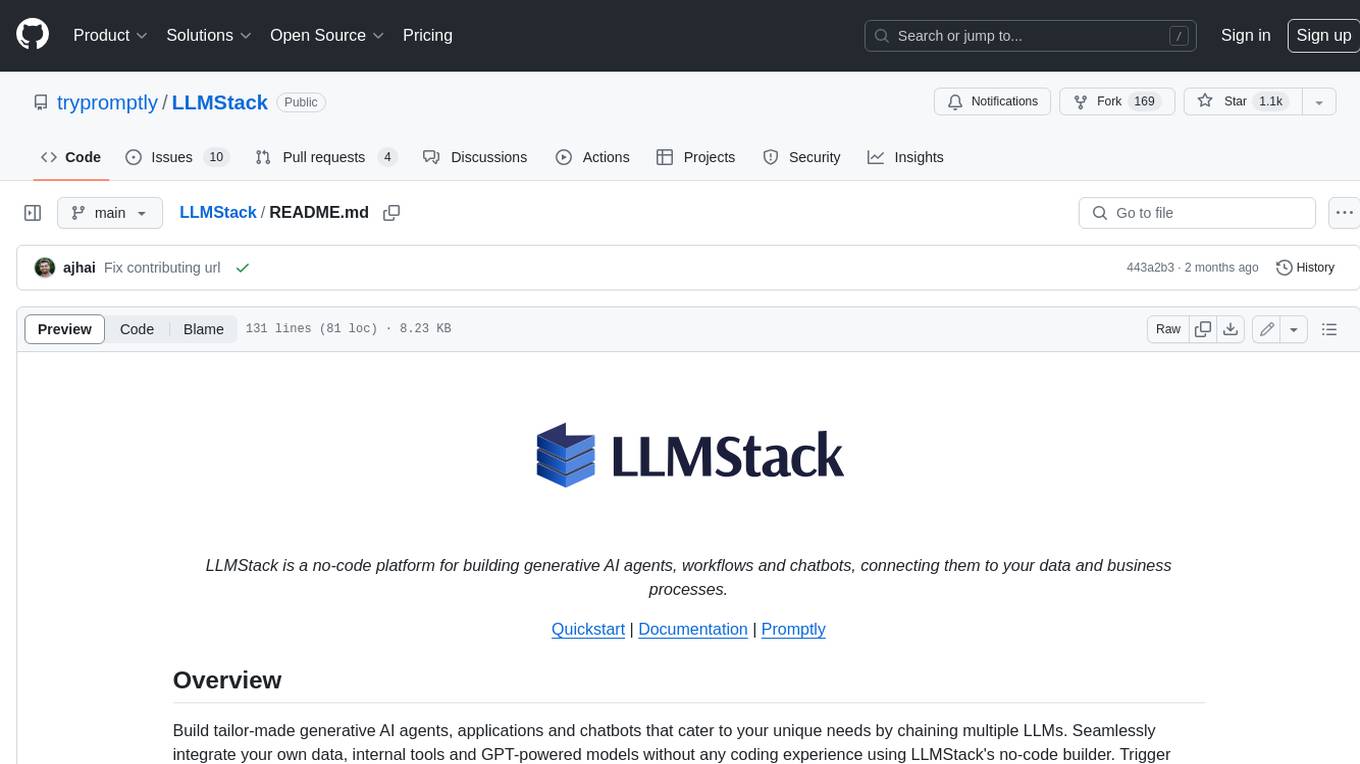
LLMStack
LLMStack is a no-code platform for building generative AI agents, workflows, and chatbots. It allows users to connect their own data, internal tools, and GPT-powered models without any coding experience. LLMStack can be deployed to the cloud or on-premise and can be accessed via HTTP API or triggered from Slack or Discord.
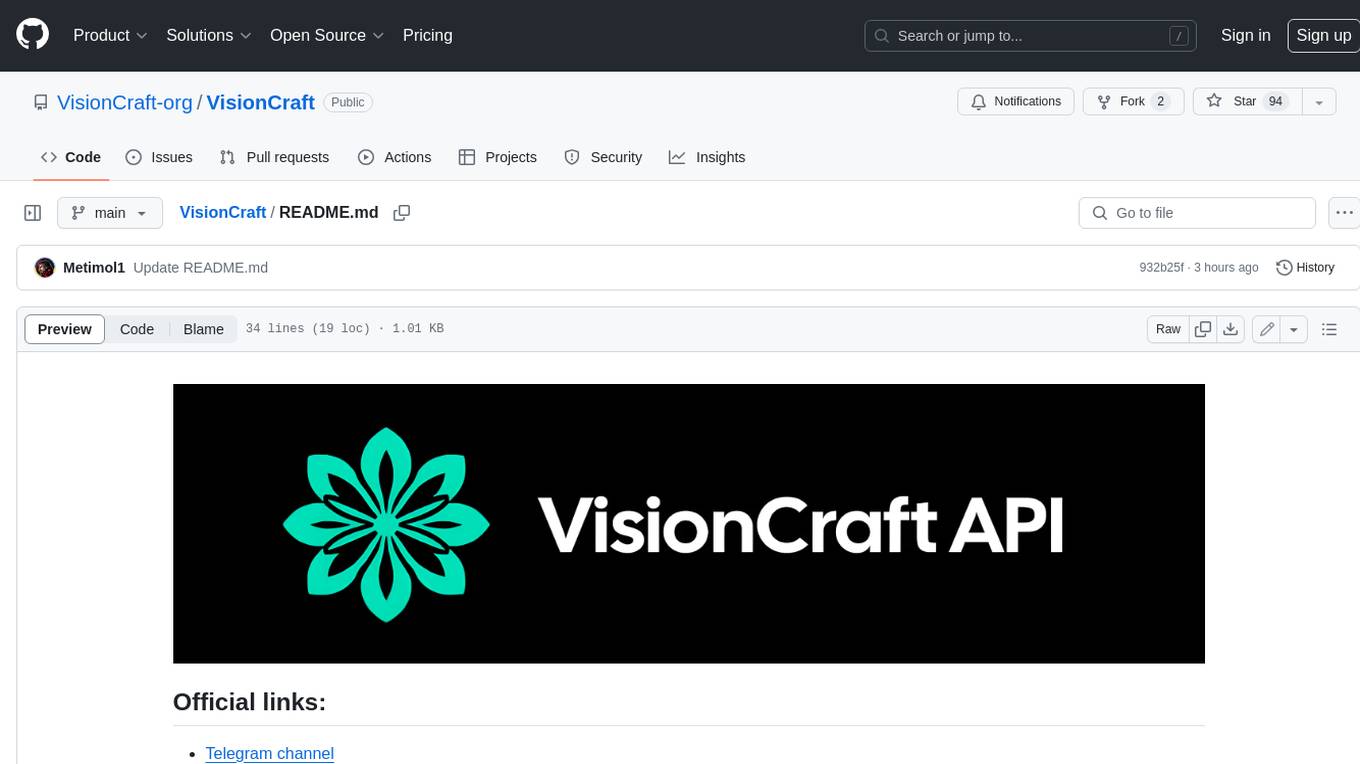
VisionCraft
The VisionCraft API is a free API for using over 100 different AI models. From images to sound.
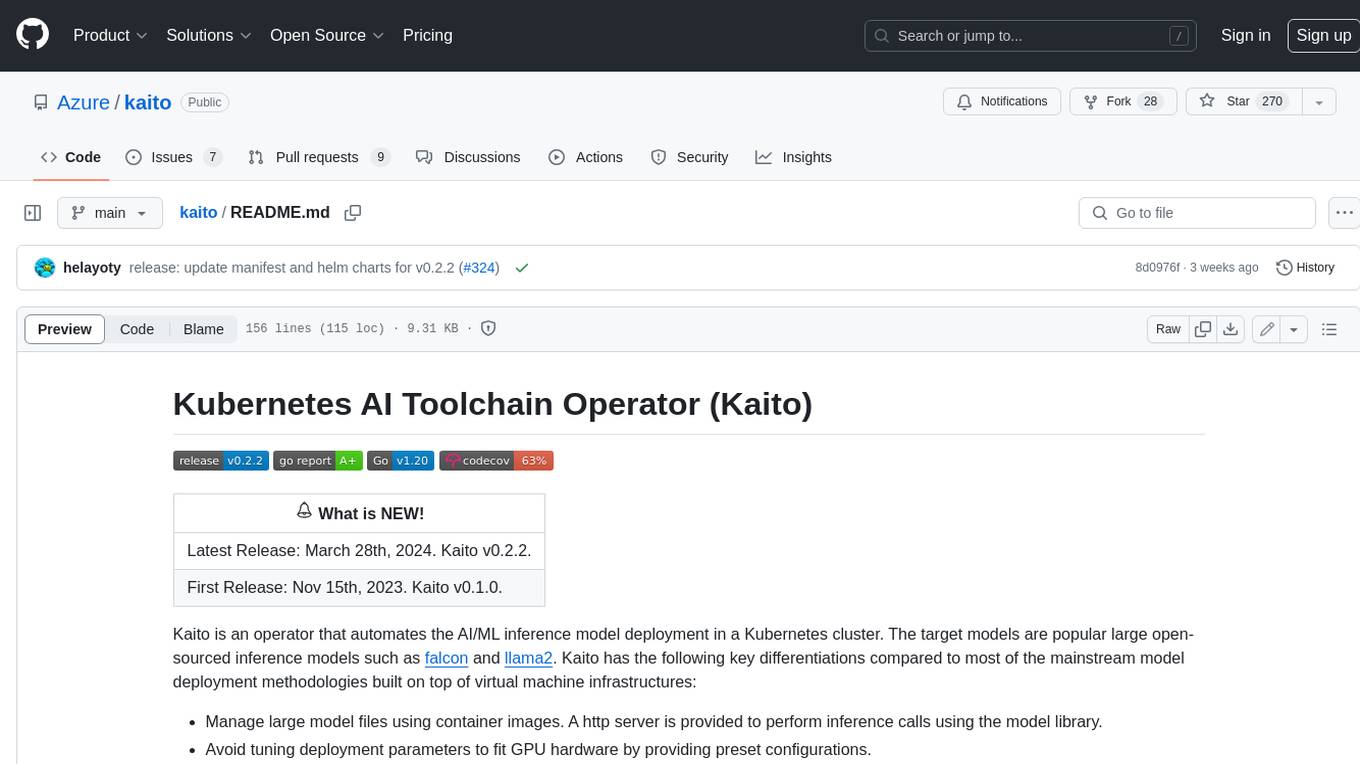
kaito
Kaito is an operator that automates the AI/ML inference model deployment in a Kubernetes cluster. It manages large model files using container images, avoids tuning deployment parameters to fit GPU hardware by providing preset configurations, auto-provisions GPU nodes based on model requirements, and hosts large model images in the public Microsoft Container Registry (MCR) if the license allows. Using Kaito, the workflow of onboarding large AI inference models in Kubernetes is largely simplified.
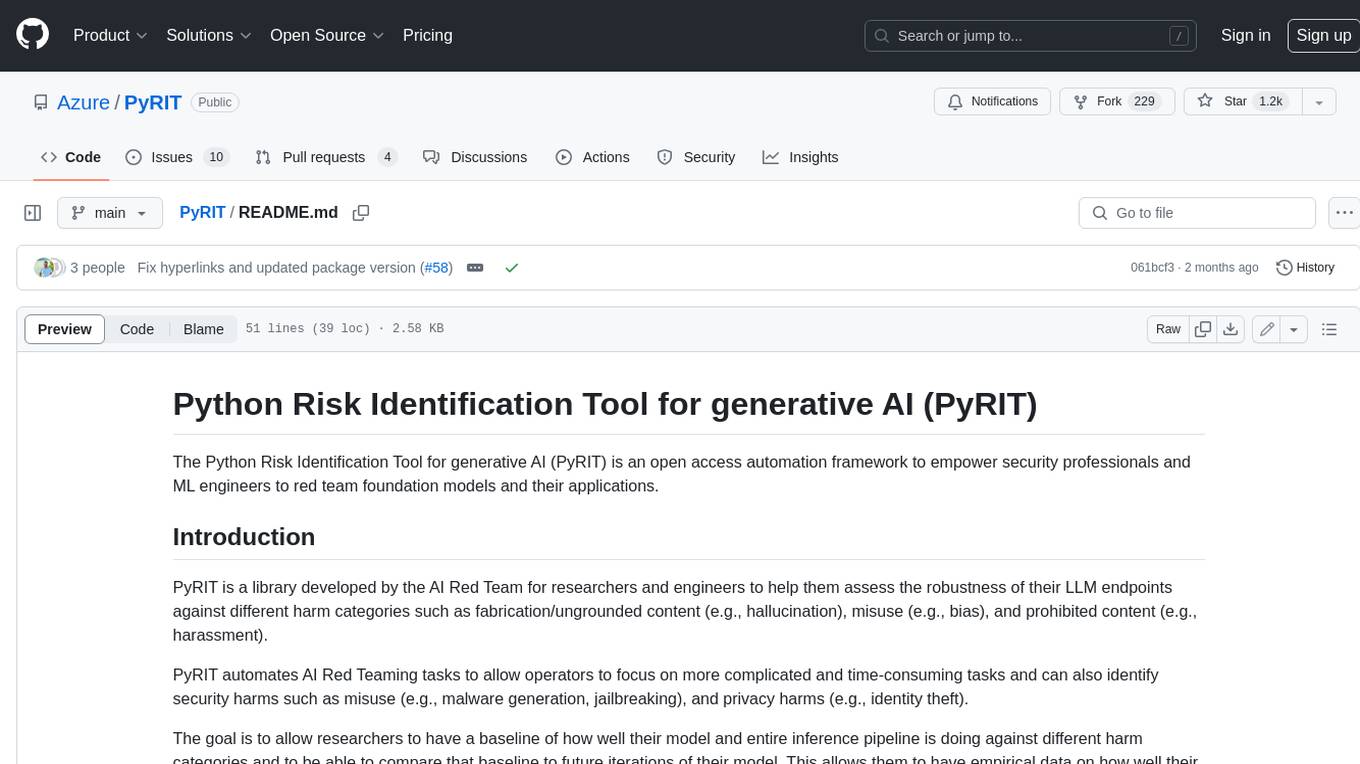
PyRIT
PyRIT is an open access automation framework designed to empower security professionals and ML engineers to red team foundation models and their applications. It automates AI Red Teaming tasks to allow operators to focus on more complicated and time-consuming tasks and can also identify security harms such as misuse (e.g., malware generation, jailbreaking), and privacy harms (e.g., identity theft). The goal is to allow researchers to have a baseline of how well their model and entire inference pipeline is doing against different harm categories and to be able to compare that baseline to future iterations of their model. This allows them to have empirical data on how well their model is doing today, and detect any degradation of performance based on future improvements.
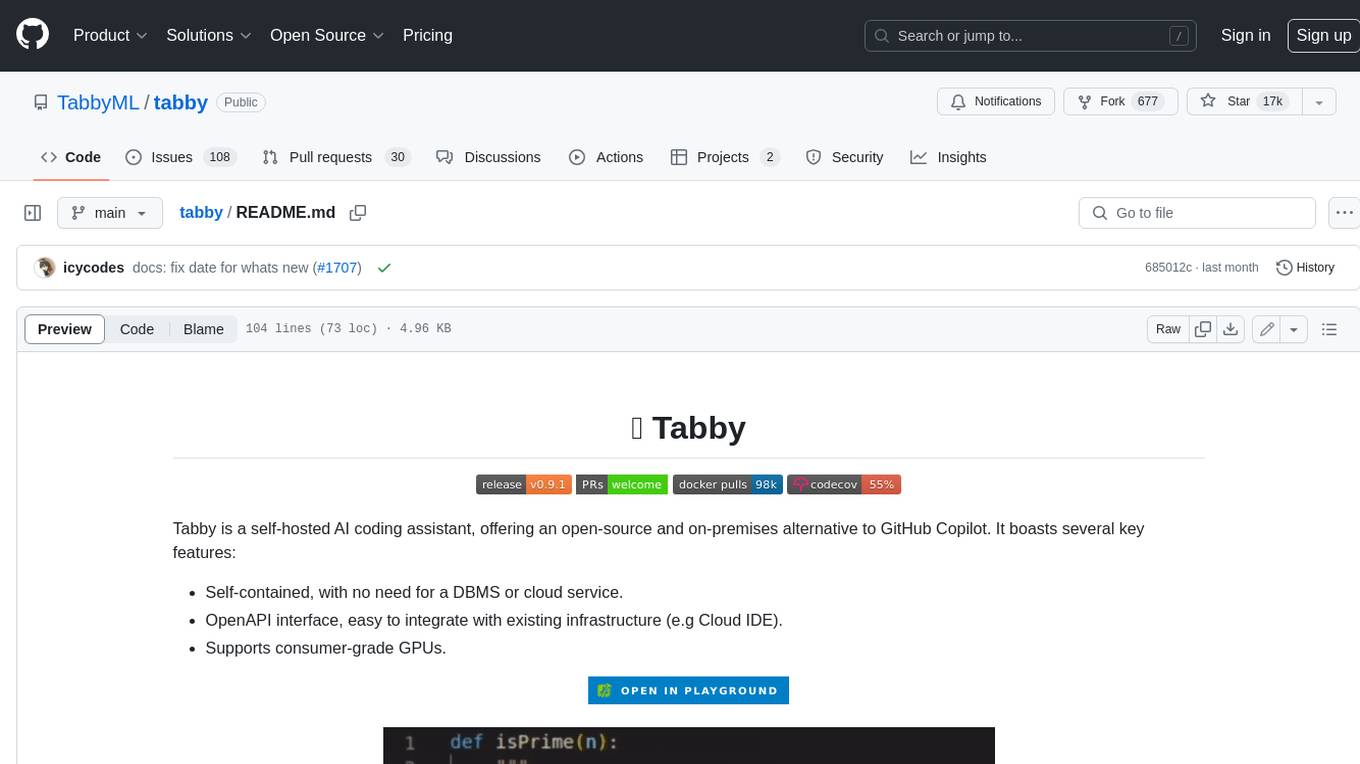
tabby
Tabby is a self-hosted AI coding assistant, offering an open-source and on-premises alternative to GitHub Copilot. It boasts several key features: * Self-contained, with no need for a DBMS or cloud service. * OpenAPI interface, easy to integrate with existing infrastructure (e.g Cloud IDE). * Supports consumer-grade GPUs.
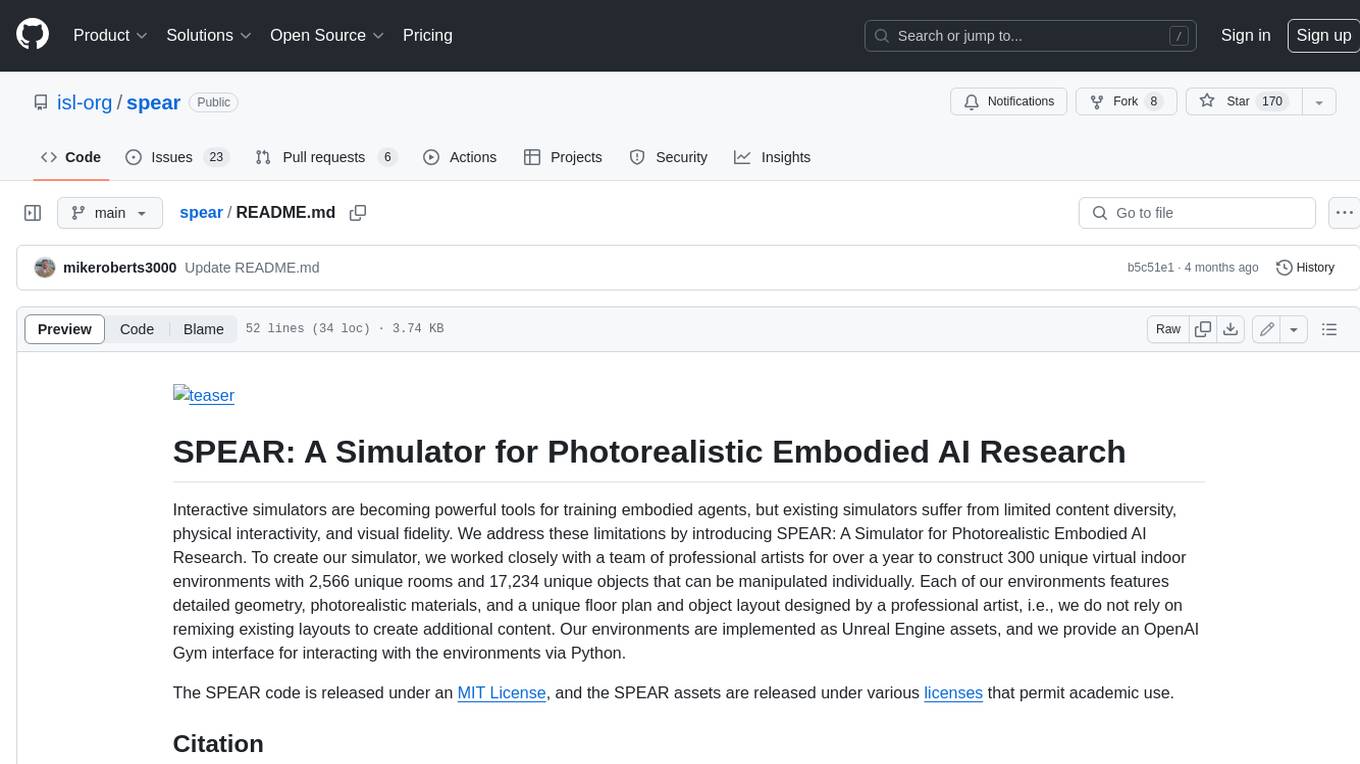
spear
SPEAR (Simulator for Photorealistic Embodied AI Research) is a powerful tool for training embodied agents. It features 300 unique virtual indoor environments with 2,566 unique rooms and 17,234 unique objects that can be manipulated individually. Each environment is designed by a professional artist and features detailed geometry, photorealistic materials, and a unique floor plan and object layout. SPEAR is implemented as Unreal Engine assets and provides an OpenAI Gym interface for interacting with the environments via Python.
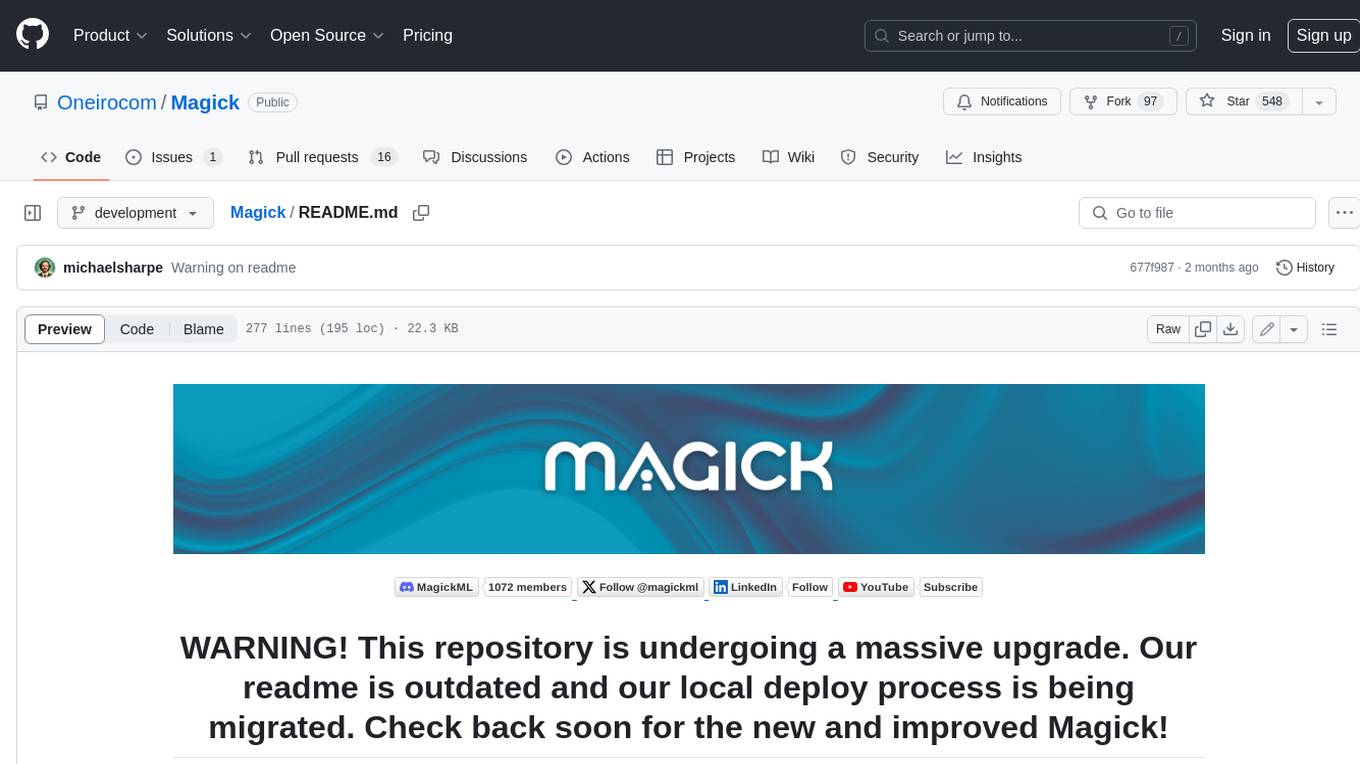
Magick
Magick is a groundbreaking visual AIDE (Artificial Intelligence Development Environment) for no-code data pipelines and multimodal agents. Magick can connect to other services and comes with nodes and templates well-suited for intelligent agents, chatbots, complex reasoning systems and realistic characters.