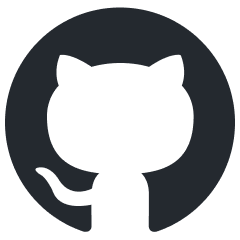
baal
Bayesian active learning library for research and industrial usecases.
Stars: 833
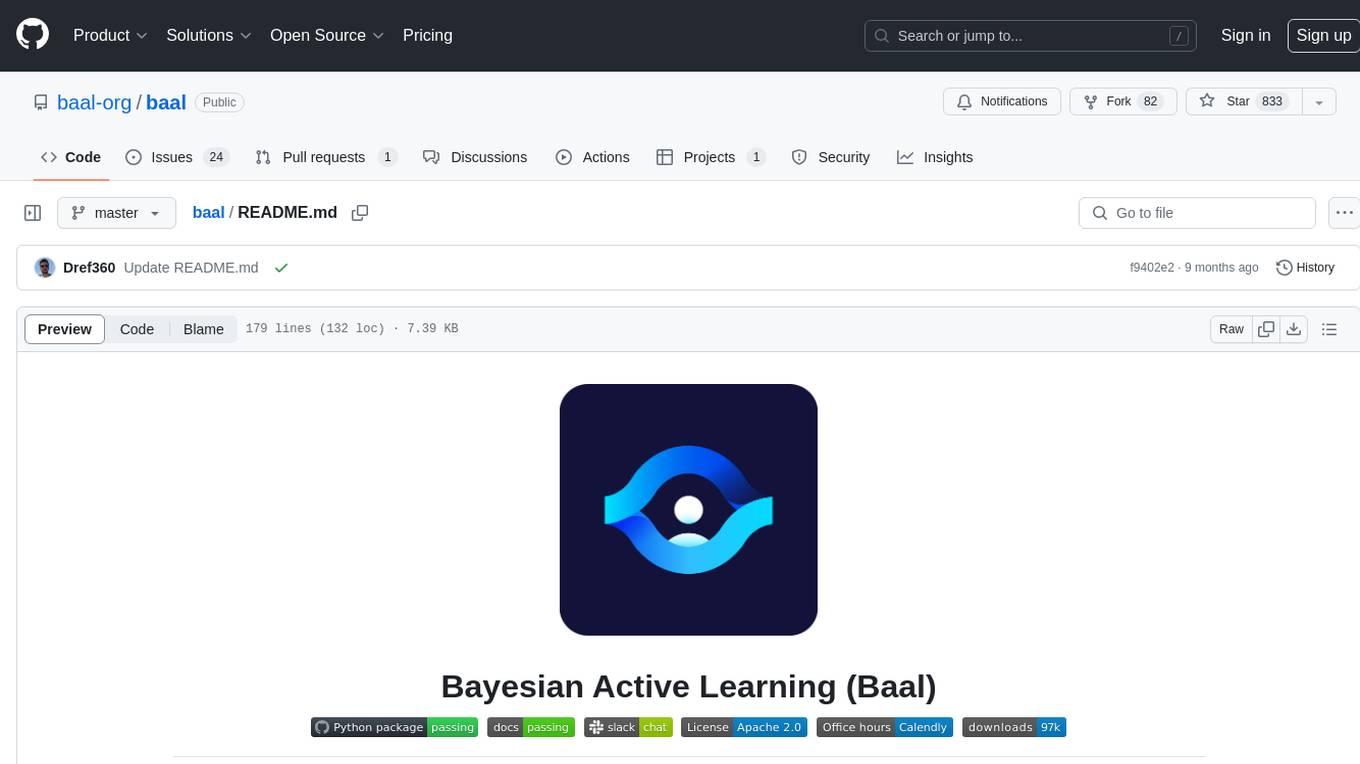
Baal is an active learning library that supports both industrial applications and research use cases. It provides a framework for Bayesian active learning methods such as Monte-Carlo Dropout, MCDropConnect, Deep ensembles, and Semi-supervised learning. Baal helps in labeling the most uncertain items in the dataset pool to improve model performance and reduce annotation effort. The library is actively maintained by a dedicated team and has been used in various research papers for production and experimentation.
README:
Baal is an active learning library that supports both industrial applications and research usecases.
Read the documentation at https://baal.readthedocs.io.
Our paper can be read on arXiv. It includes tips and tricks to make active learning usable in production.
For a quick introduction to Baal and Bayesian active learning, please see these links:
Baal was initially developed at ElementAI (acquired by ServiceNow in 2021), but is now independant.
Baal requires Python>=3.8
.
To install Baal using pip: pip install baal
We use Poetry as our package manager.
To install Baal from source: poetry install
- Bayesian active learning for production, a systematic study and a reusable library (Atighehchian et al. 2020)
- Synbols: Probing Learning Algorithms with Synthetic Datasets (Lacoste et al. 2020)
- Can Active Learning Preemptively Mitigate Fairness Issues? (Branchaud-Charron et al. 2021)
- Active learning with MaskAL reduces annotation effort for training Mask R-CNN ( Blok et al. 2021)
- Stochastic Batch Acquisition for Deep Active Learning (Kirsch et al. 2022)
Active learning is a special case of machine learning in which a learning algorithm is able to interactively query the user (or some other information source) to obtain the desired outputs at new data points (to understand the concept in more depth, refer to our tutorial).
At the moment Baal supports the following methods to perform active learning.
- Monte-Carlo Dropout (Gal et al. 2015)
- MCDropConnect (Mobiny et al. 2019)
- Deep ensembles
- Semi-supervised learning
If you want to propose new methods, please submit an issue.
The Monte-Carlo Dropout method is a known approximation for Bayesian neural networks. In this method, the Dropout layer is used both in training and test time. By running the model multiple times whilst randomly dropping weights, we calculate the uncertainty of the prediction using one of the uncertainty measurements in heuristics.py.
The framework consists of four main parts, as demonstrated in the flowchart below:
- ActiveLearningDataset
- Heuristics
- ModelWrapper
- ActiveLearningLoop
To get started, wrap your dataset in our ActiveLearningDataset class. This will ensure
that the dataset is split into
training
and pool
sets. The pool
set represents the portion of the training set which is yet to be labelled.
We provide a lightweight object ModelWrapper similar to keras.Model
to make it easier to
train and test the model. If your model is not ready for active learning, we provide Modules to prepare them.
For example, the MCDropoutModule wrapper changes the existing dropout layer to be used
in both training and inference time and the ModelWrapper
makes the specifies the number of iterations to run at
training and inference.
Finally, ActiveLearningLoop automatically computes the uncertainty and label the most uncertain items in the pool.
In conclusion, your script should be similar to this:
dataset = ActiveLearningDataset(your_dataset)
dataset.label_randomly(INITIAL_POOL) # label some data
model = MCDropoutModule(your_model)
model = ModelWrapper(model, your_criterion)
active_loop = ActiveLearningLoop(dataset,
get_probabilities=model.predict_on_dataset,
heuristic=heuristics.BALD(),
iterations=20, # Number of MC sampling.
query_size=QUERY_SIZE) # Number of item to label.
for al_step in range(N_ALSTEP):
model.train_on_dataset(dataset, optimizer, BATCH_SIZE, use_cuda=use_cuda)
metrics = model.test_on_dataset(test_dataset, BATCH_SIZE)
# Label the next most uncertain items.
if not active_loop.step():
# We're done!
break
For a complete experiment, see experiments/vgg_mcdropout_cifar10.py .
docker build [--target base_baal] -t baal .
docker run --rm baal --gpus all python3 experiments/vgg_mcdropout_cifar10.py
Simply clone the repo, and create your own experiment script similar to the example at experiments/vgg_mcdropout_cifar10.py. Make sure to use the four main parts of Baal framework. Happy running experiments
To contribute, see CONTRIBUTING.md.
"There is passion, yet peace; serenity, yet emotion; chaos, yet order."
The Baal team tests and implements the most recent papers on uncertainty estimation and active learning.
Current maintainers:
If you used Baal in one of your project, we would greatly appreciate if you cite this library using this Bibtex:
@misc{atighehchian2019baal,
title={Baal, a bayesian active learning library},
author={Atighehchian, Parmida and Branchaud-Charron, Frederic and Freyberg, Jan and Pardinas, Rafael and Schell, Lorne
and Pearse, George},
year={2022},
howpublished={\url{https://github.com/baal-org/baal/}},
}
To get information on licence of this API please read LICENCE
For Tasks:
Click tags to check more tools for each tasksFor Jobs:
Alternative AI tools for baal
Similar Open Source Tools
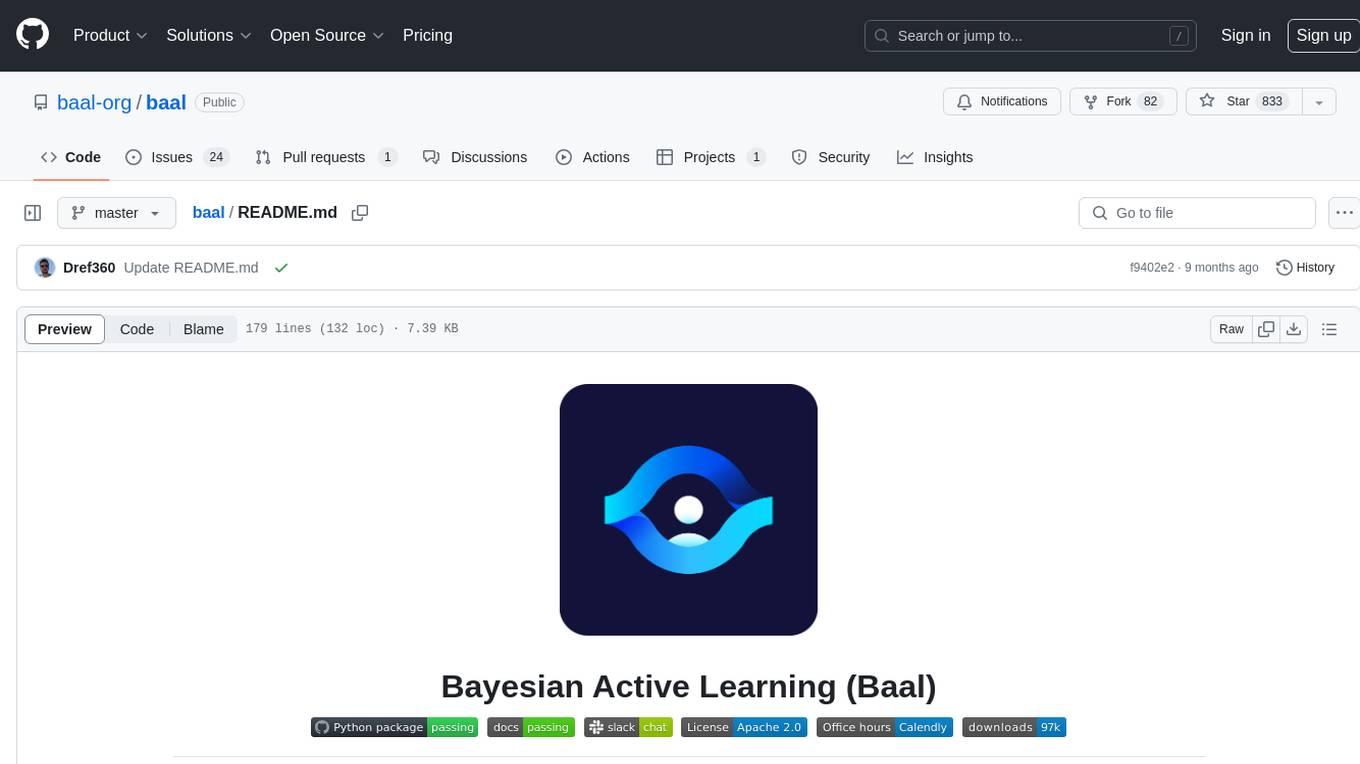
baal
Baal is an active learning library that supports both industrial applications and research use cases. It provides a framework for Bayesian active learning methods such as Monte-Carlo Dropout, MCDropConnect, Deep ensembles, and Semi-supervised learning. Baal helps in labeling the most uncertain items in the dataset pool to improve model performance and reduce annotation effort. The library is actively maintained by a dedicated team and has been used in various research papers for production and experimentation.
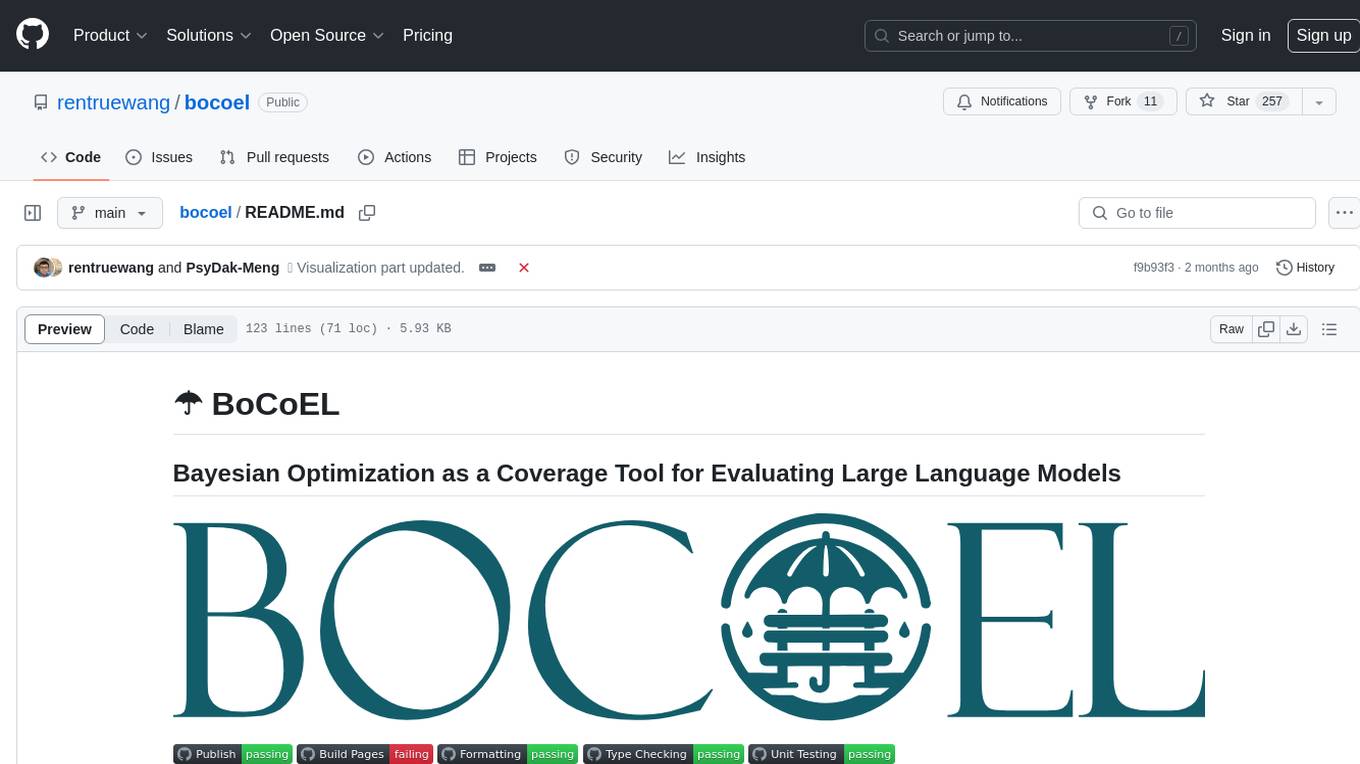
bocoel
BoCoEL is a tool that leverages Bayesian Optimization to efficiently evaluate large language models by selecting a subset of the corpus for evaluation. It encodes individual entries into embeddings, uses Bayesian optimization to select queries, retrieves from the corpus, and provides easily managed evaluations. The tool aims to reduce computation costs during evaluation with a dynamic budget, supporting models like GPT2, Pythia, and LLAMA through integration with Hugging Face transformers and datasets. BoCoEL offers a modular design and efficient representation of the corpus to enhance evaluation quality.
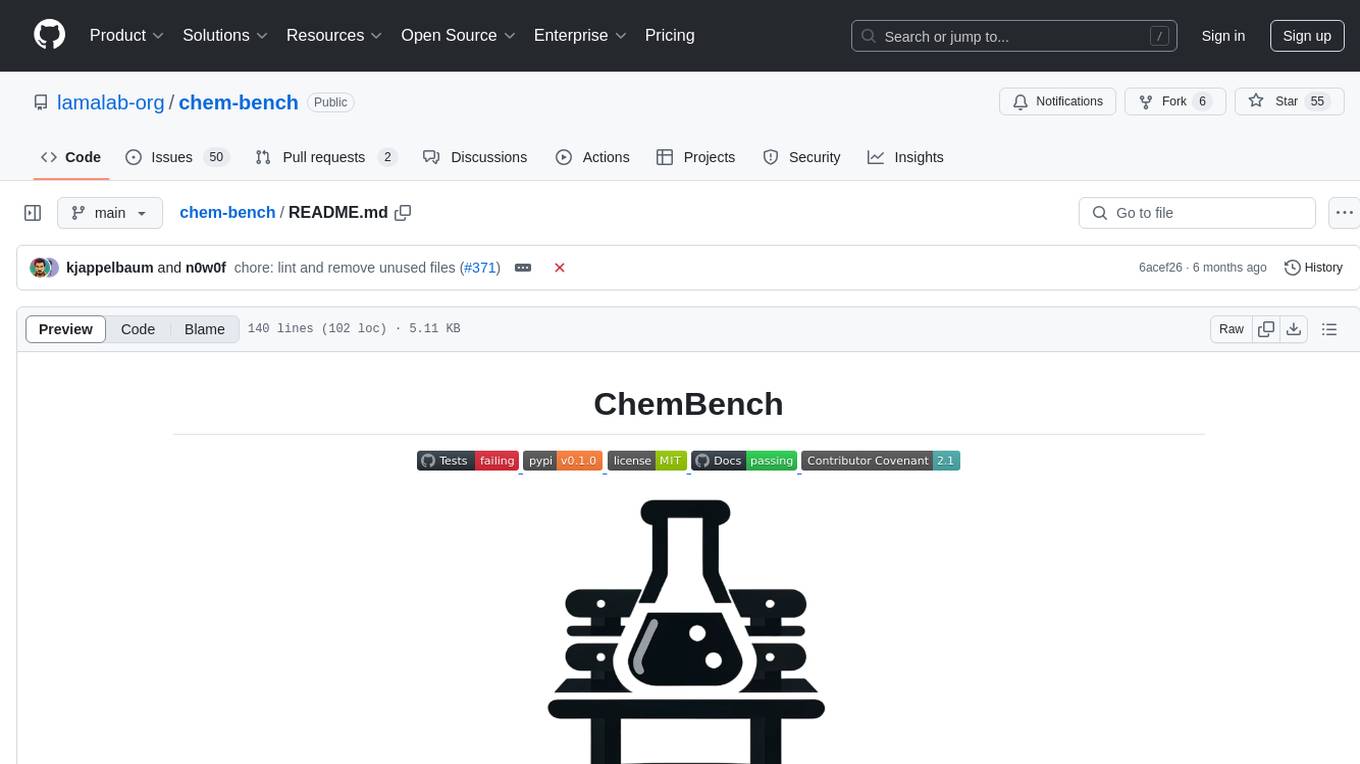
chem-bench
ChemBench is a project aimed at expanding chemistry benchmark tasks in a BIG-bench compatible way, providing a pipeline to benchmark frontier and open models. It allows users to run benchmarking tasks on models with existing presets, offering predefined parameters and processing steps. The library facilitates benchmarking models on the entire suite, addressing challenges such as prompt structure, parsing, and scoring methods. Users can contribute to the project by following the developer notes.
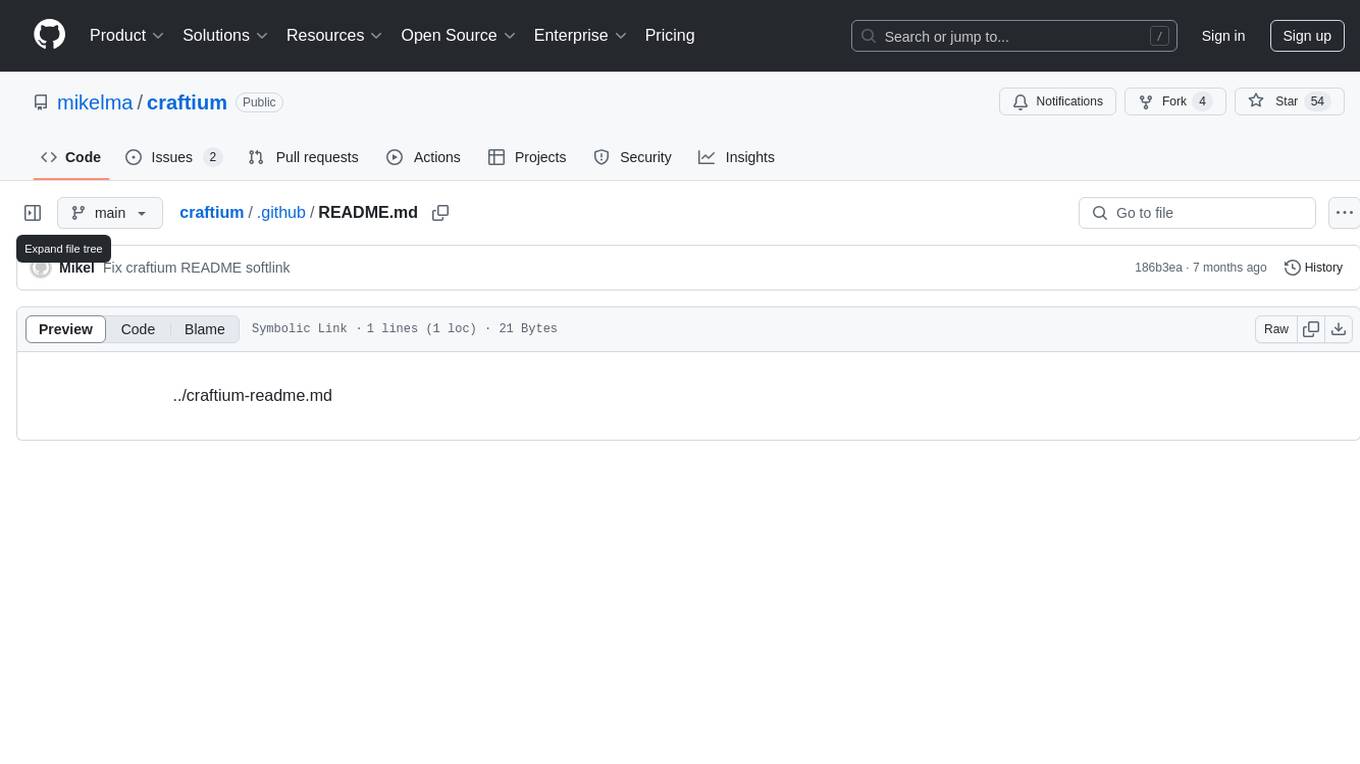
craftium
Craftium is an open-source platform based on the Minetest voxel game engine and the Gymnasium and PettingZoo APIs, designed for creating fast, rich, and diverse single and multi-agent environments. It allows for connecting to Craftium's Python process, executing actions as keyboard and mouse controls, extending the Lua API for creating RL environments and tasks, and supporting client/server synchronization for slow agents. Craftium is fully extensible, extensively documented, modern RL API compatible, fully open source, and eliminates the need for Java. It offers a variety of environments for research and development in reinforcement learning.
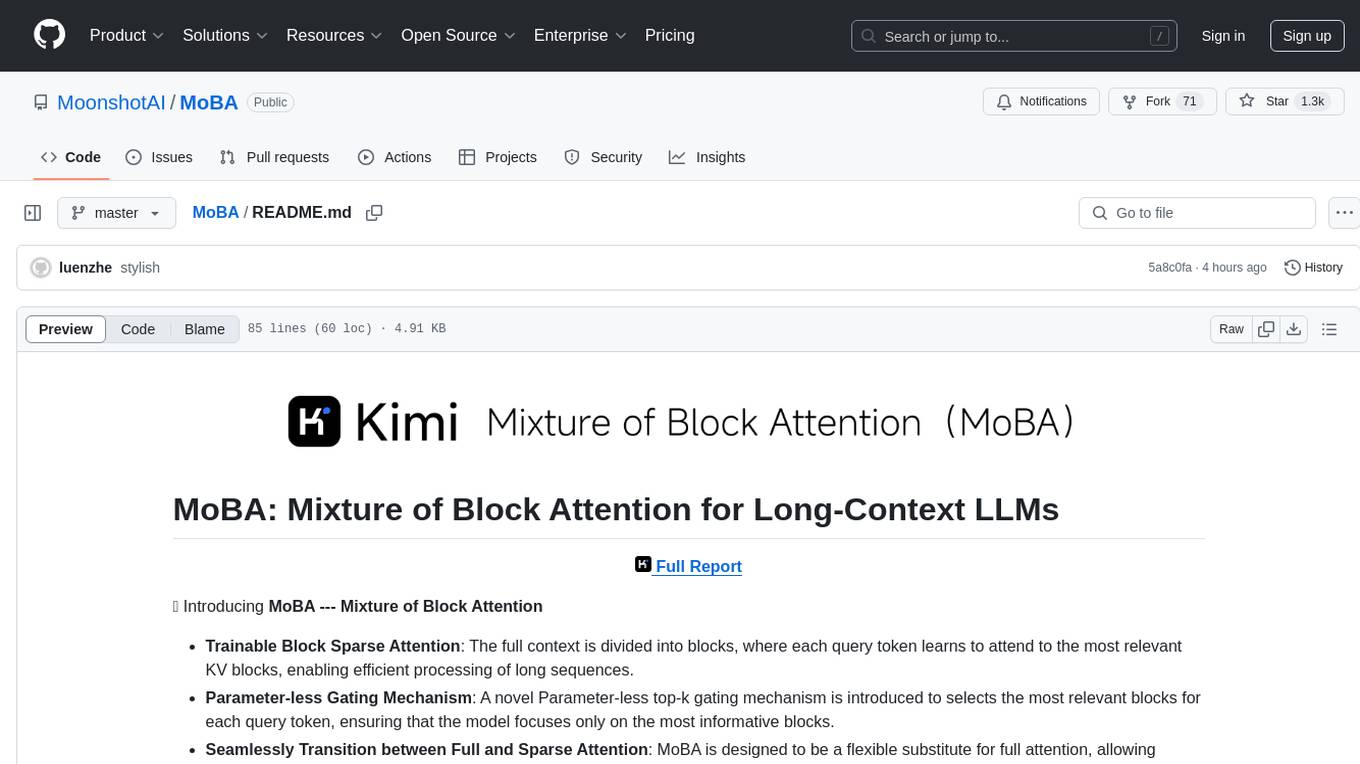
MoBA
MoBA (Mixture of Block Attention) is an innovative approach for long-context language models, enabling efficient processing of long sequences by dividing the full context into blocks and introducing a parameter-less gating mechanism. It allows seamless transitions between full and sparse attention modes, enhancing efficiency without compromising performance. MoBA has been deployed to support long-context requests and demonstrates significant advancements in efficient attention computation for large language models.
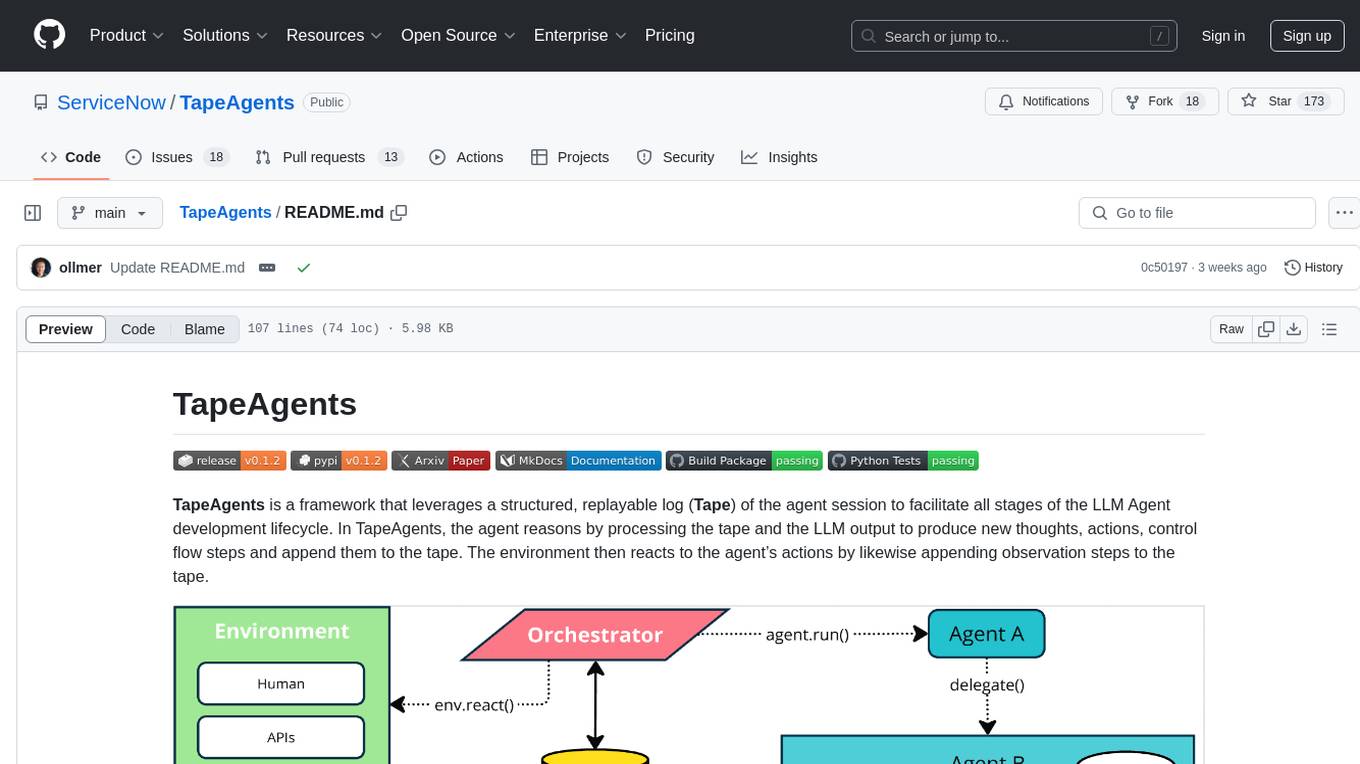
TapeAgents
TapeAgents is a framework that leverages a structured, replayable log of the agent session to facilitate all stages of the LLM Agent development lifecycle. The agent reasons by processing the tape and the LLM output to produce new thoughts, actions, control flow steps, and append them to the tape. Key features include building agents as low-level state machines or high-level multi-agent team configurations, debugging agents with TapeAgent studio or TapeBrowser apps, serving agents with response streaming, and optimizing agent configurations using successful tapes. The Tape-centric design of TapeAgents provides ultimate flexibility in project development, allowing access to tapes for making prompts, generating next steps, and controlling agent behavior.
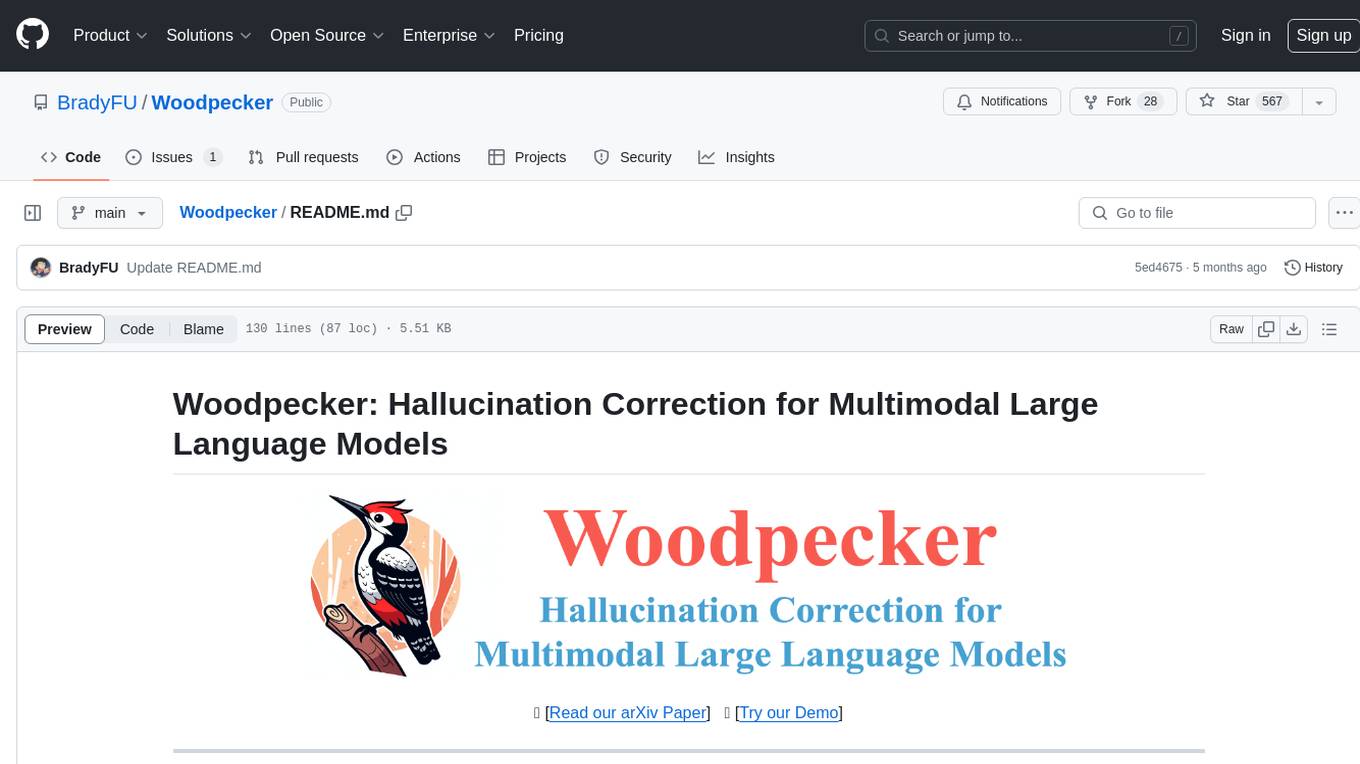
Woodpecker
Woodpecker is a tool designed to correct hallucinations in Multimodal Large Language Models (MLLMs) by introducing a training-free method that picks out and corrects inconsistencies between generated text and image content. It consists of five stages: key concept extraction, question formulation, visual knowledge validation, visual claim generation, and hallucination correction. Woodpecker can be easily integrated with different MLLMs and provides interpretable results by accessing intermediate outputs of the stages. The tool has shown significant improvements in accuracy over baseline models like MiniGPT-4 and mPLUG-Owl.
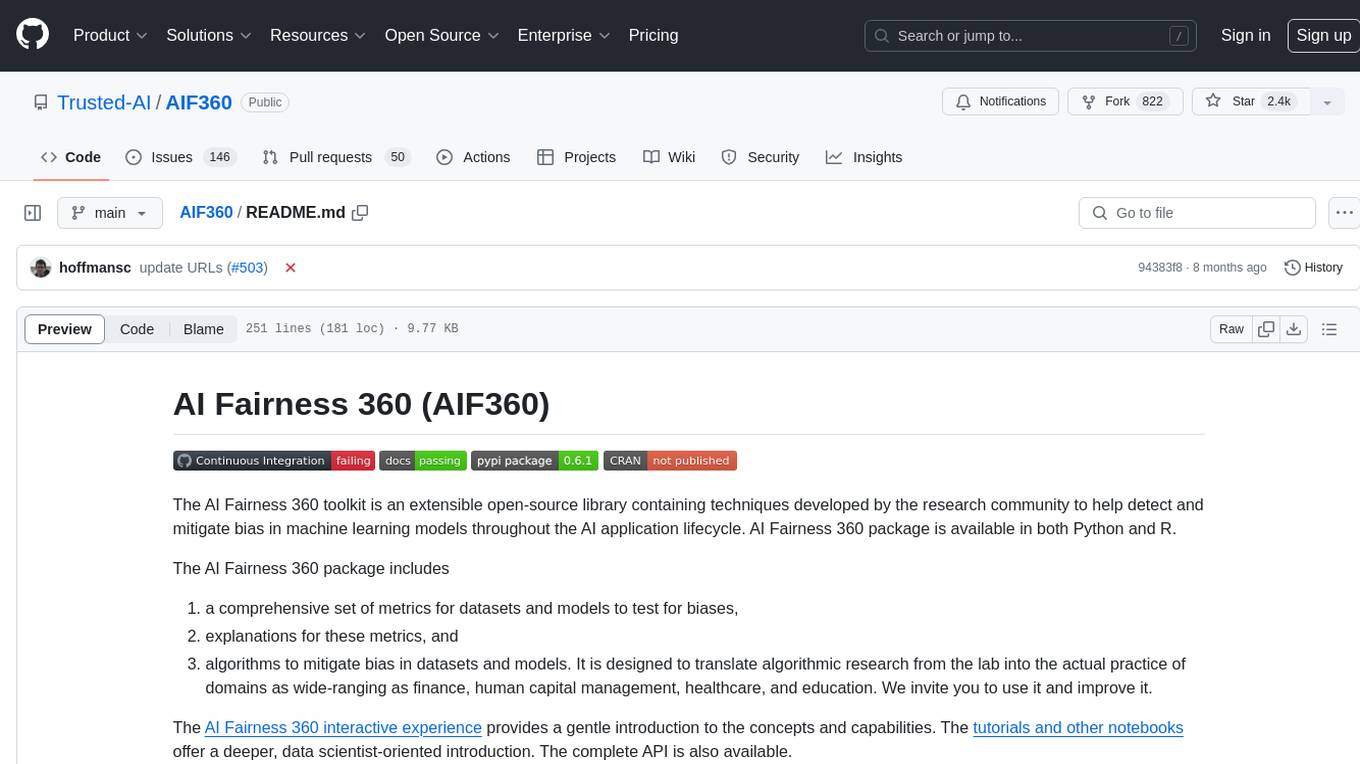
AIF360
The AI Fairness 360 toolkit is an open-source library designed to detect and mitigate bias in machine learning models. It provides a comprehensive set of metrics, explanations, and algorithms for bias mitigation in various domains such as finance, healthcare, and education. The toolkit supports multiple bias mitigation algorithms and fairness metrics, and is available in both Python and R. Users can leverage the toolkit to ensure fairness in AI applications and contribute to its development for extensibility.
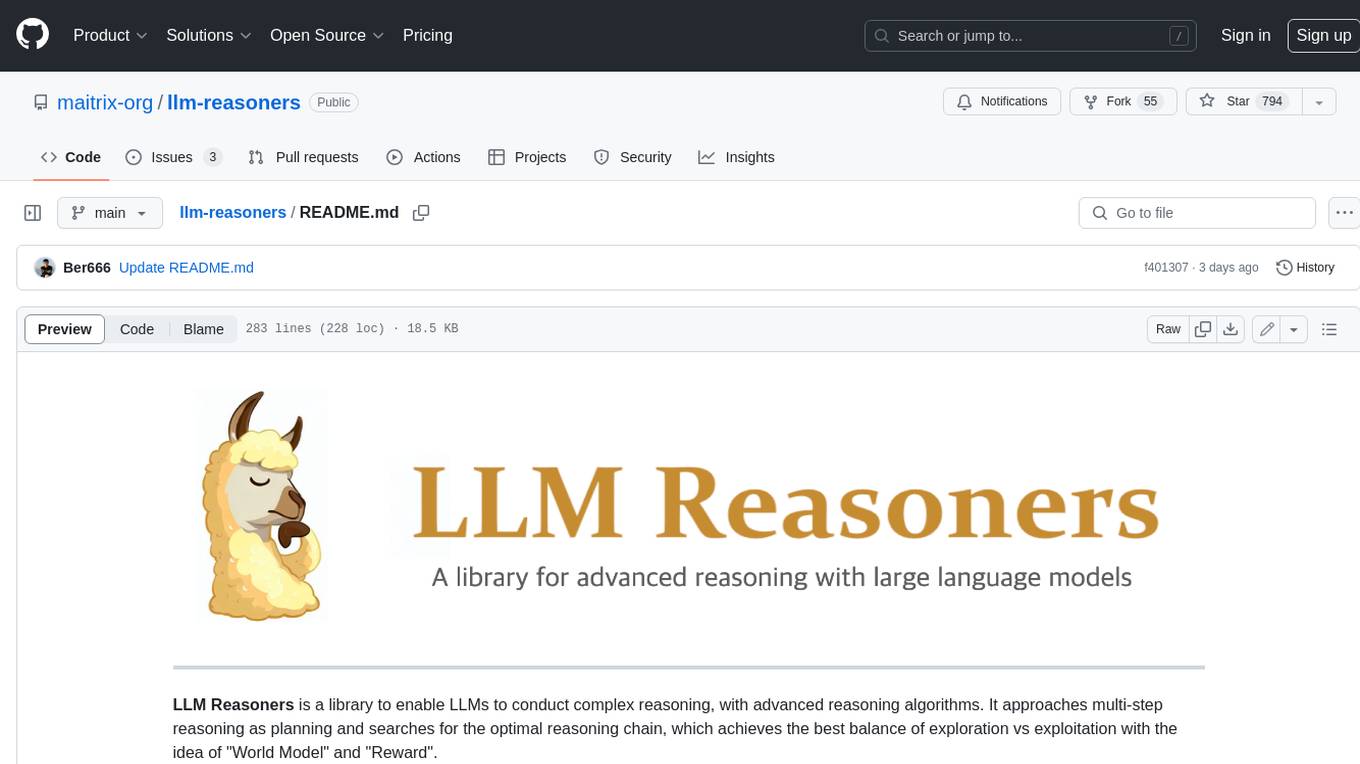
llm-reasoners
LLM Reasoners is a library that enables LLMs to conduct complex reasoning, with advanced reasoning algorithms. It approaches multi-step reasoning as planning and searches for the optimal reasoning chain, which achieves the best balance of exploration vs exploitation with the idea of "World Model" and "Reward". Given any reasoning problem, simply define the reward function and an optional world model (explained below), and let LLM reasoners take care of the rest, including Reasoning Algorithms, Visualization, LLM calling, and more!
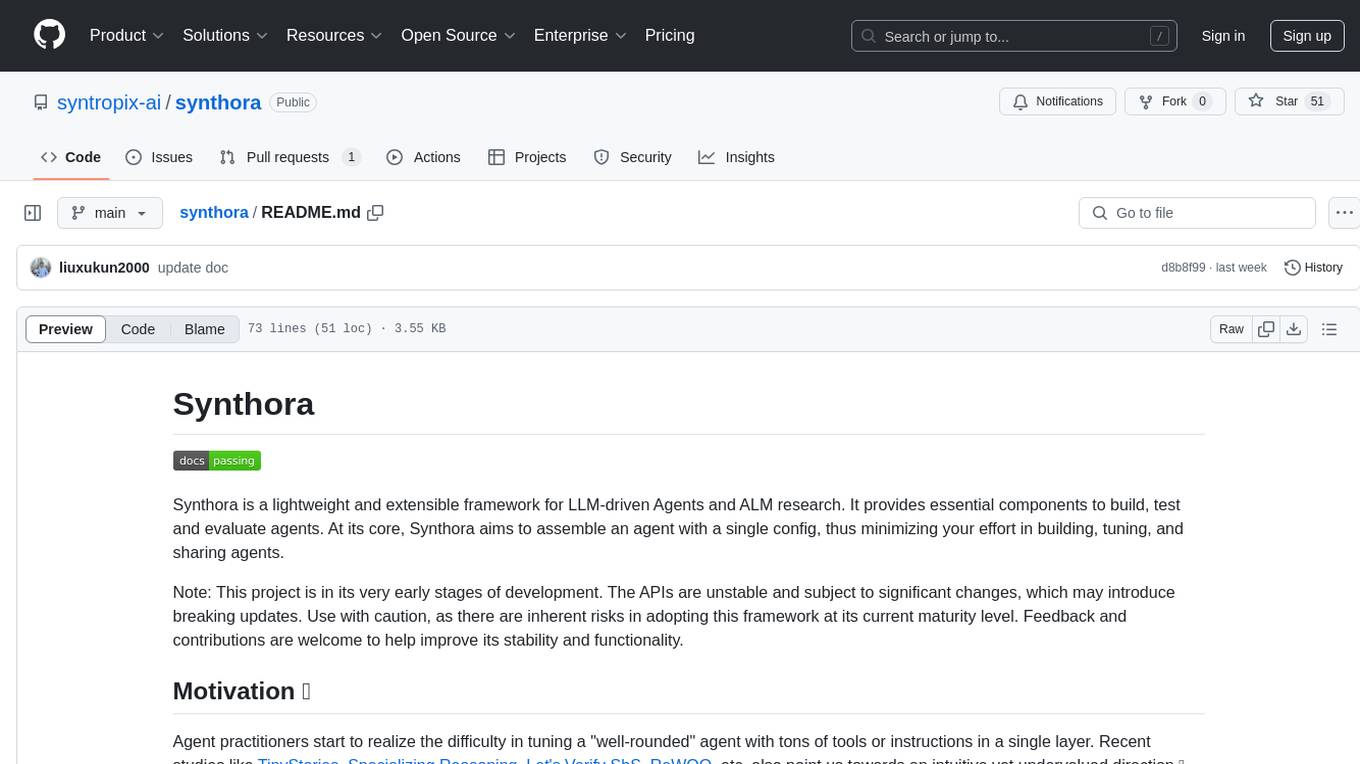
synthora
Synthora is a lightweight and extensible framework for LLM-driven Agents and ALM research. It aims to simplify the process of building, testing, and evaluating agents by providing essential components. The framework allows for easy agent assembly with a single config, reducing the effort required for tuning and sharing agents. Although in early development stages with unstable APIs, Synthora welcomes feedback and contributions to enhance its stability and functionality.
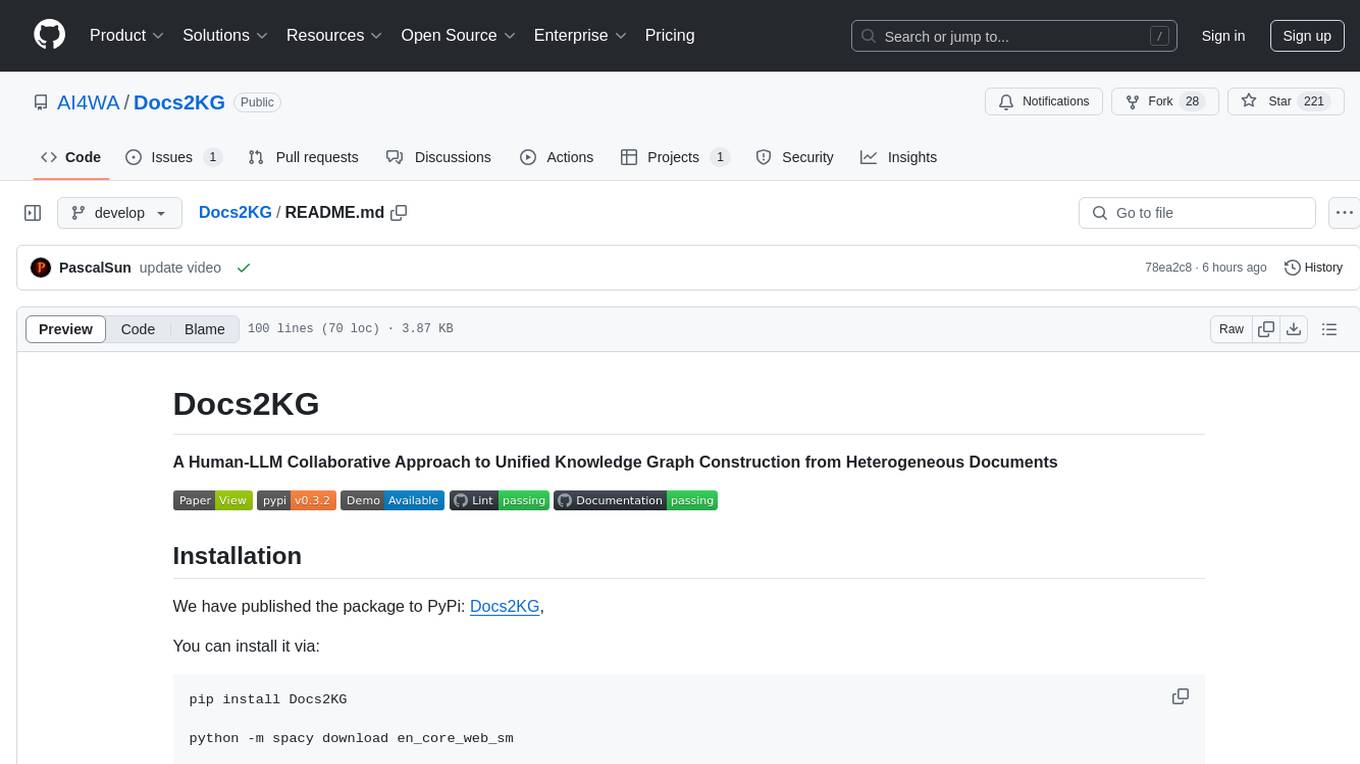
Docs2KG
Docs2KG is a tool designed for constructing a unified knowledge graph from heterogeneous documents. It addresses the challenges of digitizing diverse unstructured documents and constructing a high-quality knowledge graph with less effort. The tool combines bottom-up and top-down approaches, utilizing a human-LLM collaborative interface to enhance the generated knowledge graph. It organizes the knowledge graph into MetaKG, LayoutKG, and SemanticKG, providing a comprehensive view of document content. Docs2KG aims to streamline the process of knowledge graph construction and offers metrics for evaluating the quality of automatic construction.
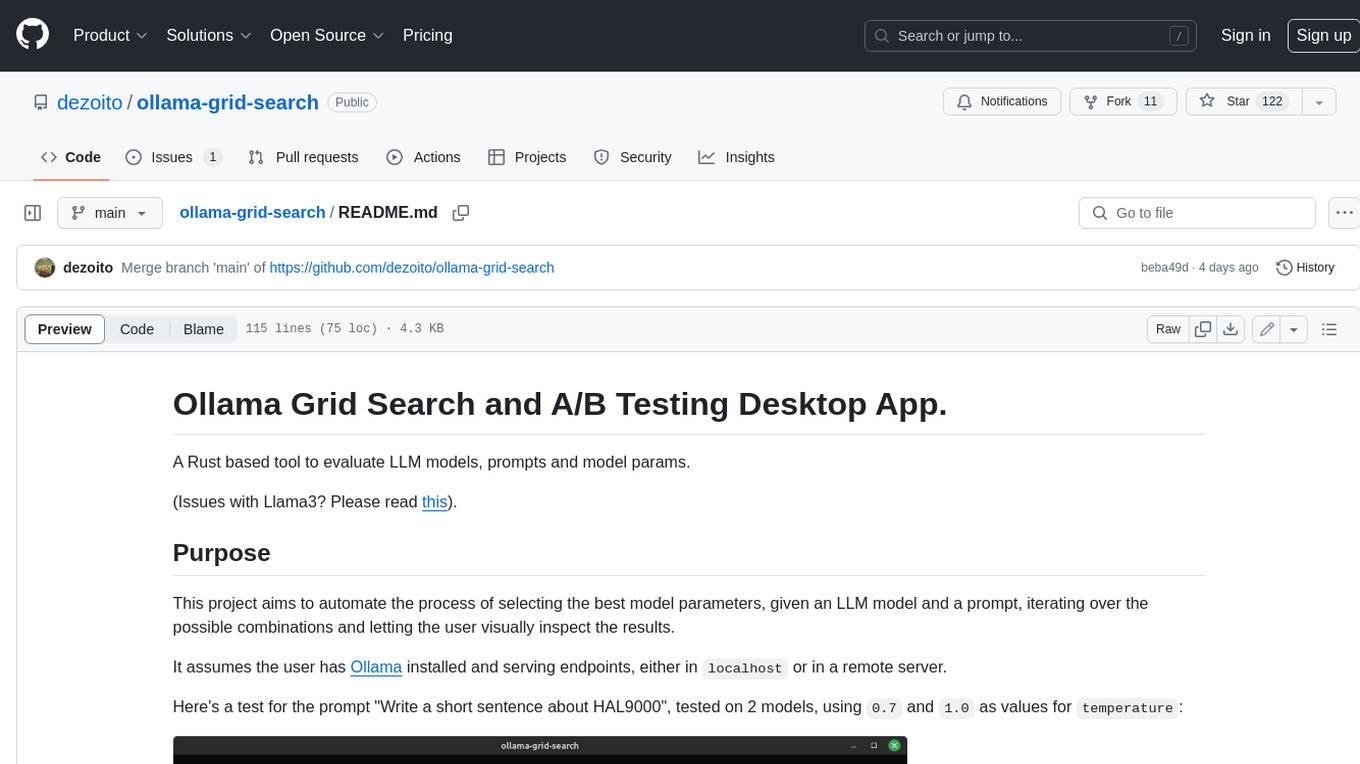
ollama-grid-search
A Rust based tool to evaluate LLM models, prompts and model params. It automates the process of selecting the best model parameters, given an LLM model and a prompt, iterating over the possible combinations and letting the user visually inspect the results. The tool assumes the user has Ollama installed and serving endpoints, either in `localhost` or in a remote server. Key features include: * Automatically fetches models from local or remote Ollama servers * Iterates over different models and params to generate inferences * A/B test prompts on different models simultaneously * Allows multiple iterations for each combination of parameters * Makes synchronous inference calls to avoid spamming servers * Optionally outputs inference parameters and response metadata (inference time, tokens and tokens/s) * Refetching of individual inference calls * Model selection can be filtered by name * List experiments which can be downloaded in JSON format * Configurable inference timeout * Custom default parameters and system prompts can be defined in settings
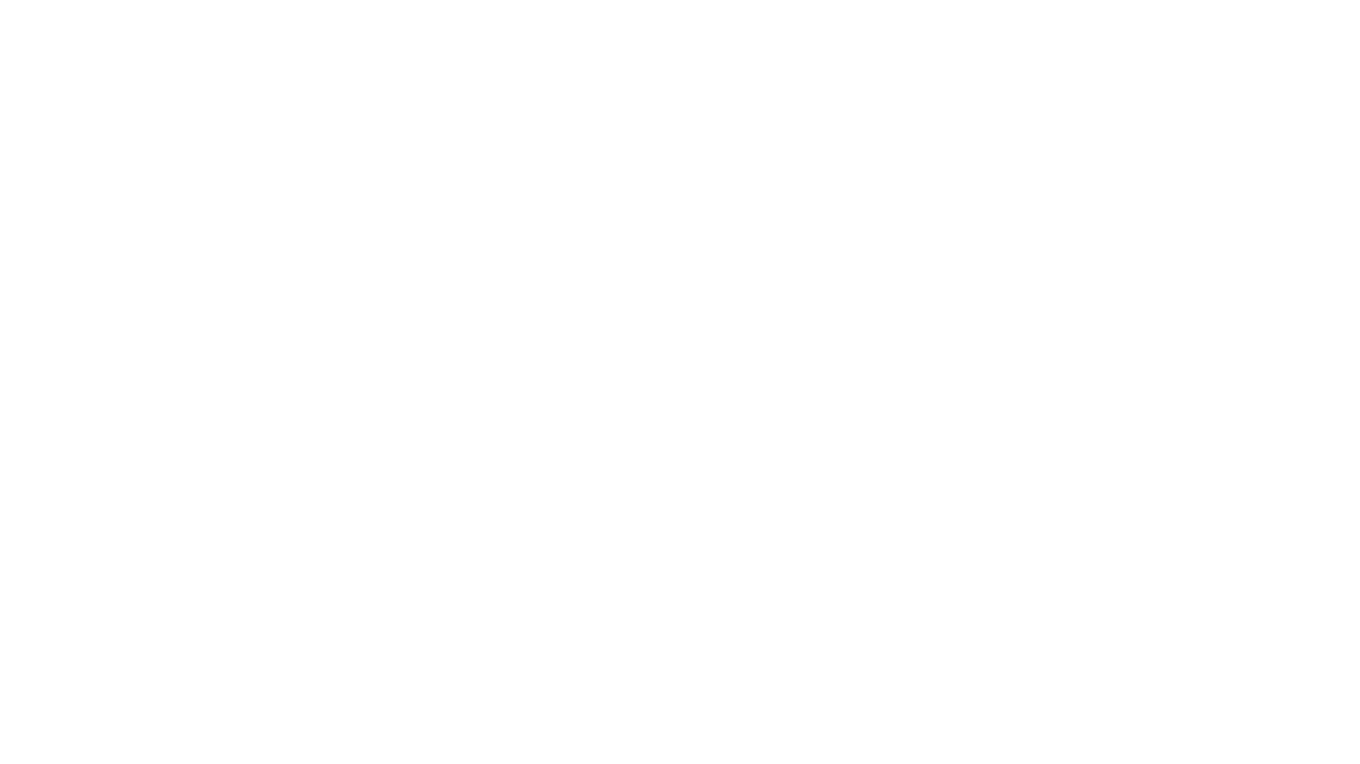
llm_client
llm_client is a Rust interface designed for Local Large Language Models (LLMs) that offers automated build support for CPU, CUDA, MacOS, easy model presets, and a novel cascading prompt workflow for controlled generation. It provides a breadth of configuration options and API support for various OpenAI compatible APIs. The tool is primarily focused on deterministic signals from probabilistic LLM vibes, enabling specialized workflows for specific tasks and reproducible outcomes.
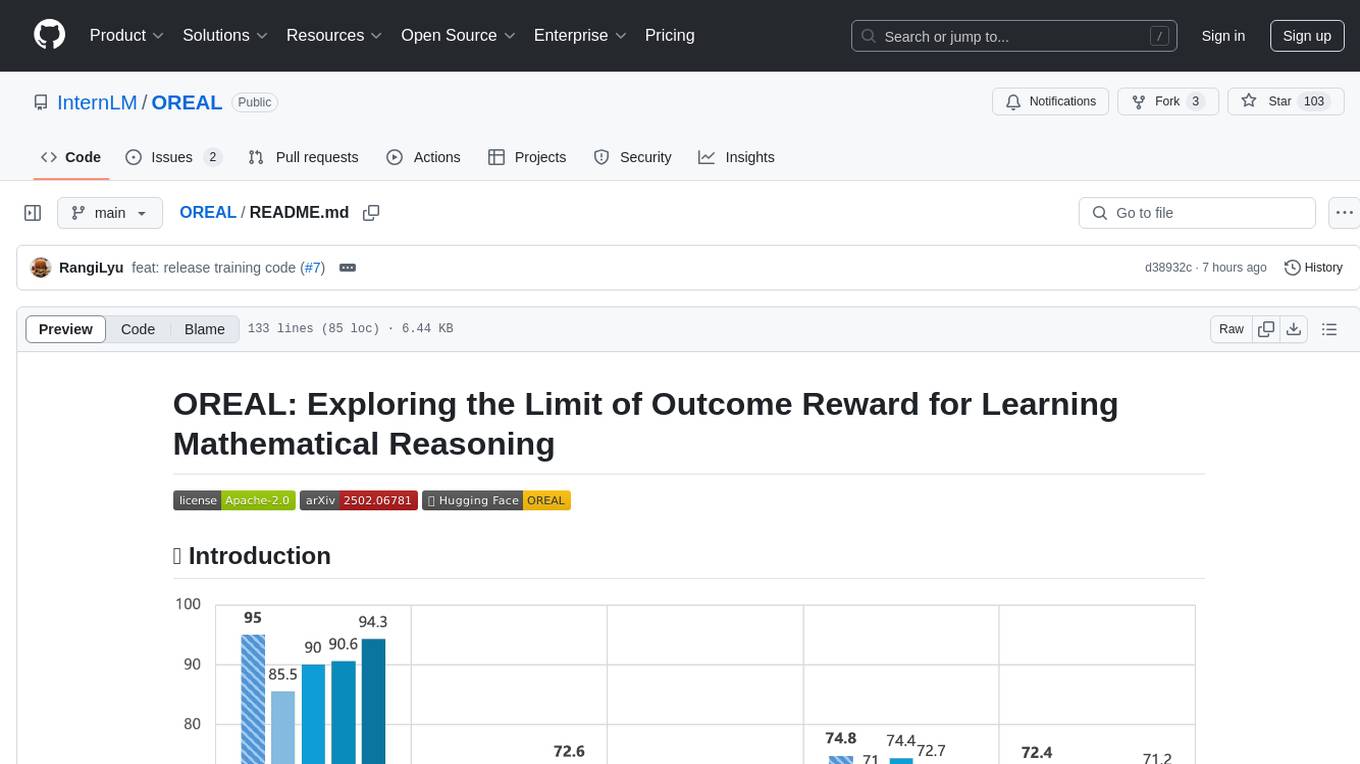
OREAL
OREAL is a reinforcement learning framework designed for mathematical reasoning tasks, aiming to achieve optimal performance through outcome reward-based learning. The framework utilizes behavior cloning, reshaping rewards, and token-level reward models to address challenges in sparse rewards and partial correctness. OREAL has achieved significant results, with a 7B model reaching 94.0 pass@1 accuracy on MATH-500 and surpassing previous 32B models. The tool provides training tutorials and Hugging Face model repositories for easy access and implementation.
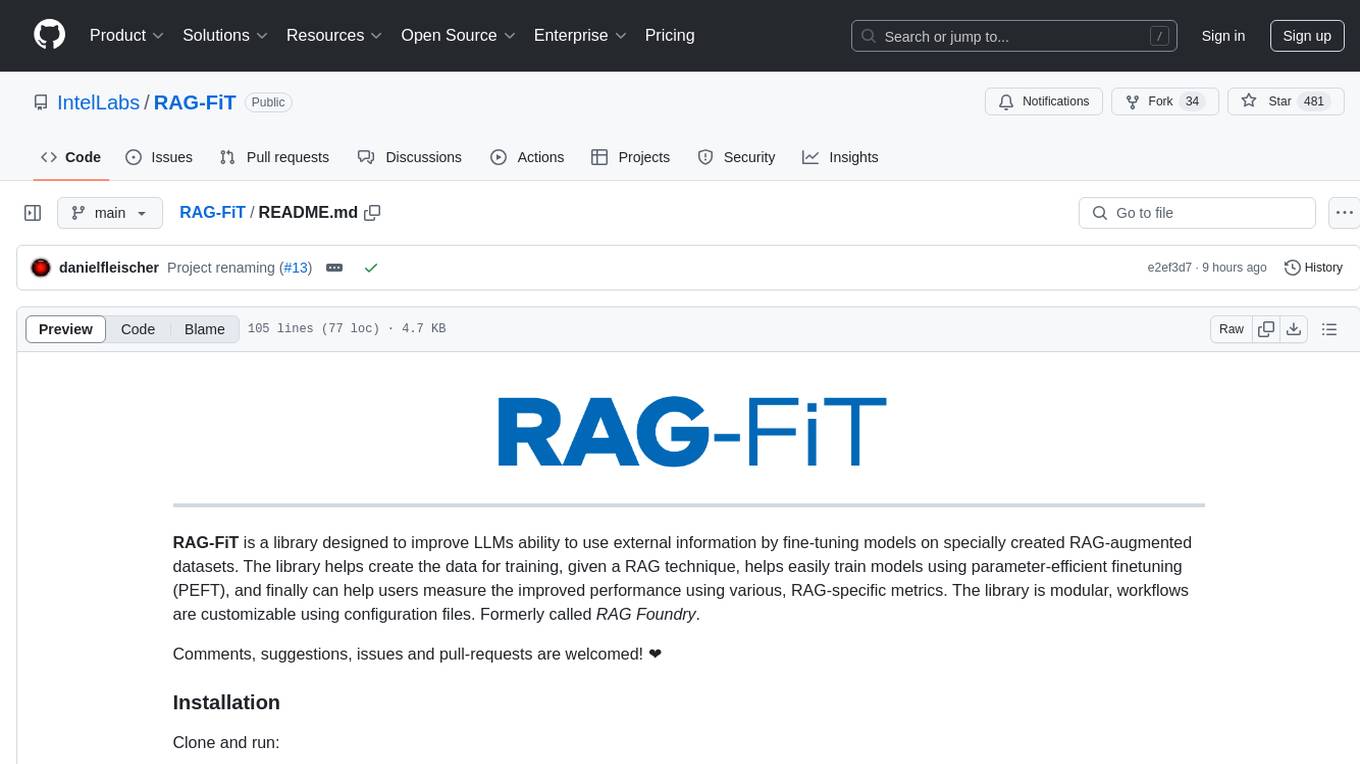
RAG-FiT
RAG-FiT is a library designed to improve Language Models' ability to use external information by fine-tuning models on specially created RAG-augmented datasets. The library assists in creating training data, training models using parameter-efficient finetuning (PEFT), and evaluating performance using RAG-specific metrics. It is modular, customizable via configuration files, and facilitates fast prototyping and experimentation with various RAG settings and configurations.
For similar tasks
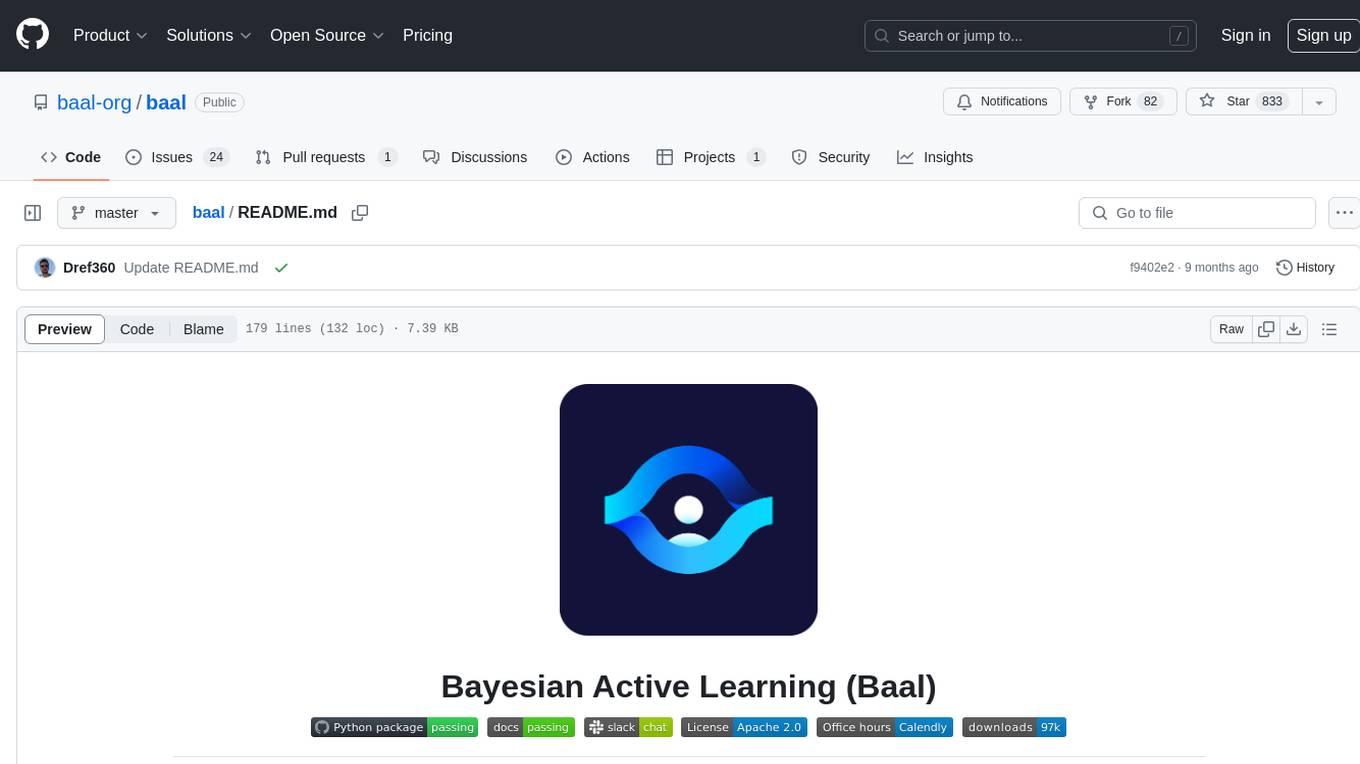
baal
Baal is an active learning library that supports both industrial applications and research use cases. It provides a framework for Bayesian active learning methods such as Monte-Carlo Dropout, MCDropConnect, Deep ensembles, and Semi-supervised learning. Baal helps in labeling the most uncertain items in the dataset pool to improve model performance and reduce annotation effort. The library is actively maintained by a dedicated team and has been used in various research papers for production and experimentation.
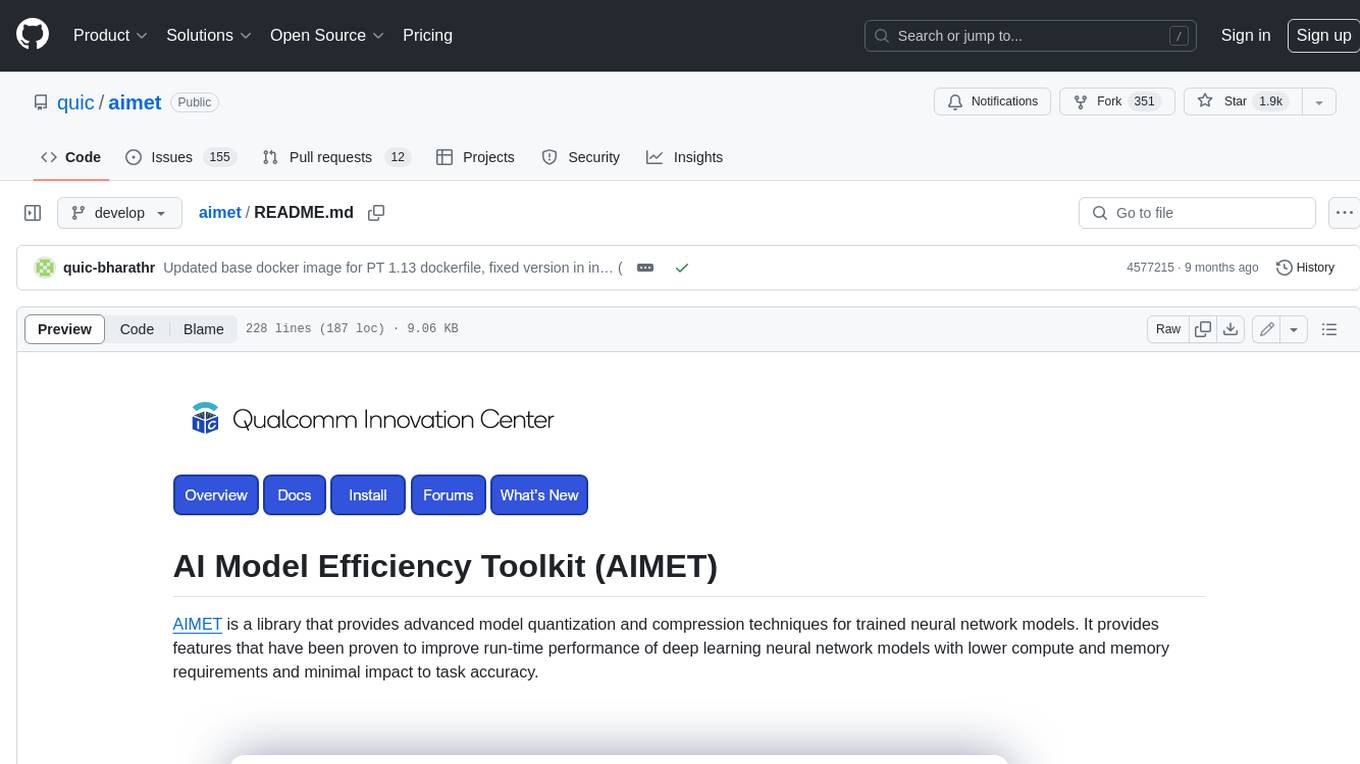
aimet
AIMET is a library that provides advanced model quantization and compression techniques for trained neural network models. It provides features that have been proven to improve run-time performance of deep learning neural network models with lower compute and memory requirements and minimal impact to task accuracy. AIMET is designed to work with PyTorch, TensorFlow and ONNX models. We also host the AIMET Model Zoo - a collection of popular neural network models optimized for 8-bit inference. We also provide recipes for users to quantize floating point models using AIMET.
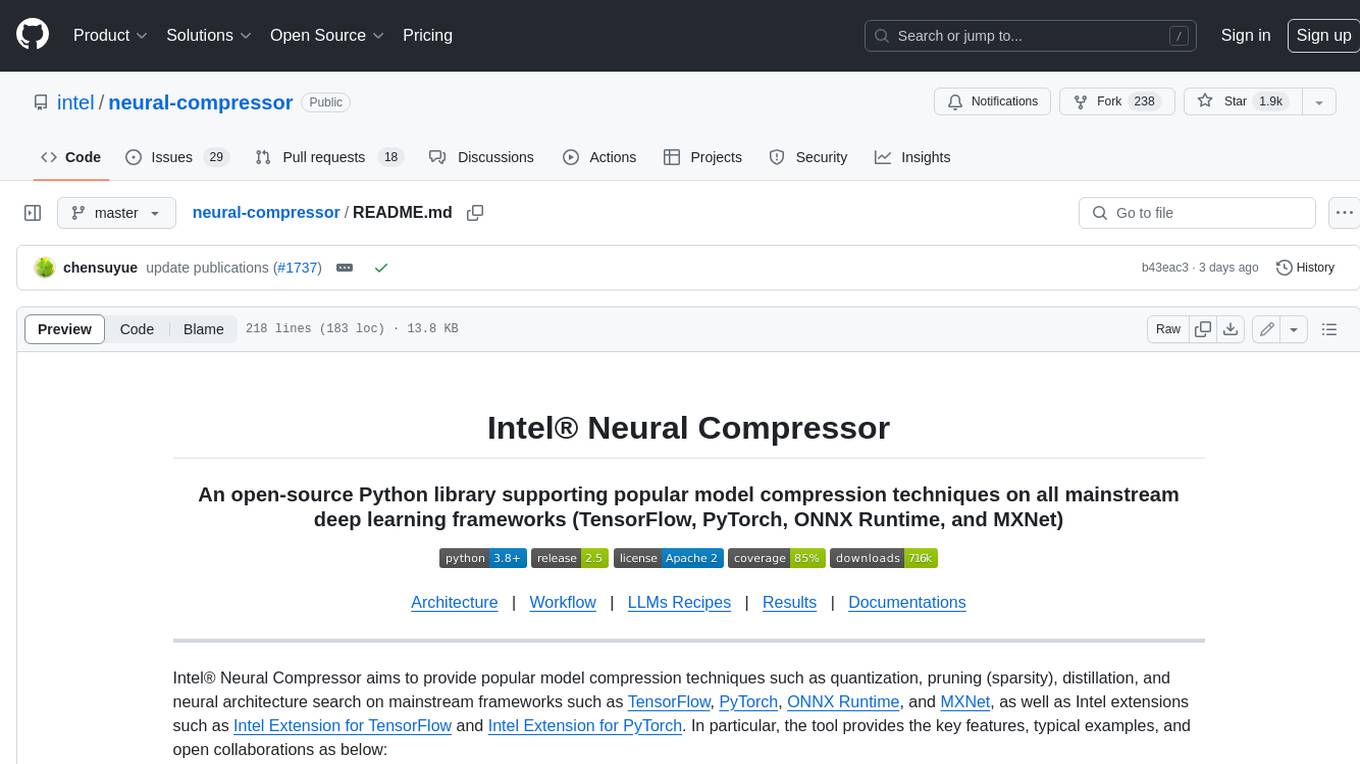
neural-compressor
Intel® Neural Compressor is an open-source Python library that supports popular model compression techniques such as quantization, pruning (sparsity), distillation, and neural architecture search on mainstream frameworks such as TensorFlow, PyTorch, ONNX Runtime, and MXNet. It provides key features, typical examples, and open collaborations, including support for a wide range of Intel hardware, validation of popular LLMs, and collaboration with cloud marketplaces, software platforms, and open AI ecosystems.
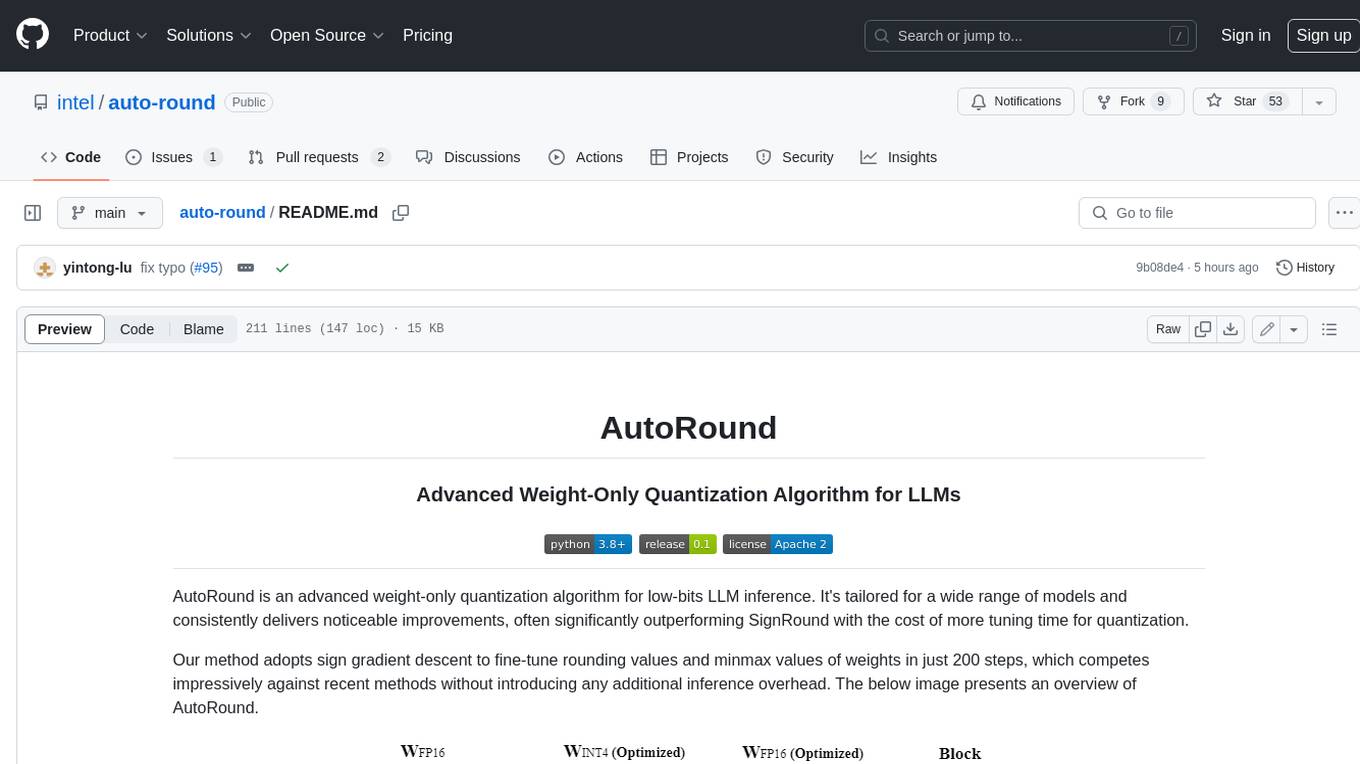
auto-round
AutoRound is an advanced weight-only quantization algorithm for low-bits LLM inference. It competes impressively against recent methods without introducing any additional inference overhead. The method adopts sign gradient descent to fine-tune rounding values and minmax values of weights in just 200 steps, often significantly outperforming SignRound with the cost of more tuning time for quantization. AutoRound is tailored for a wide range of models and consistently delivers noticeable improvements.
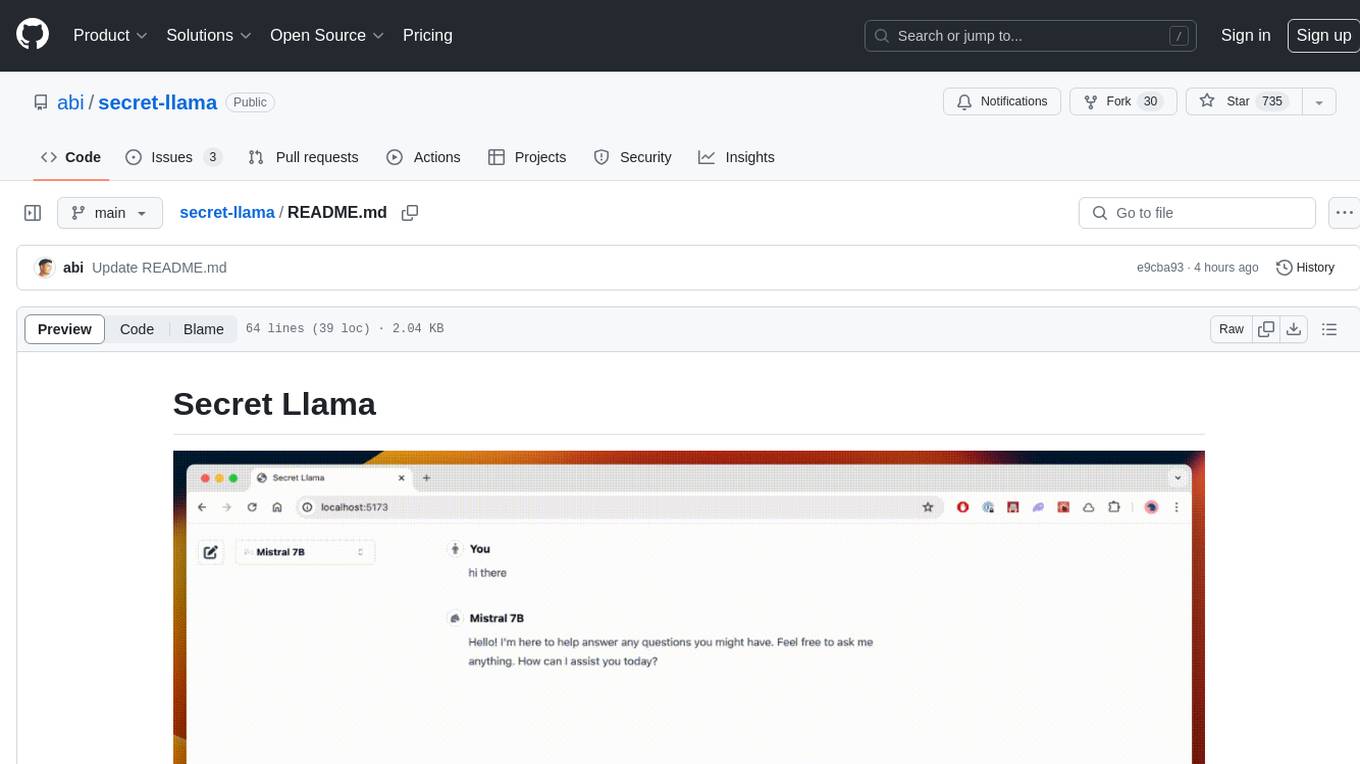
secret-llama
Entirely-in-browser, fully private LLM chatbot supporting Llama 3, Mistral and other open source models. Fully private = No conversation data ever leaves your computer. Runs in the browser = No server needed and no install needed! Works offline. Easy-to-use interface on par with ChatGPT, but for open source LLMs. System requirements include a modern browser with WebGPU support. Supported models include TinyLlama-1.1B-Chat-v0.4-q4f32_1-1k, Llama-3-8B-Instruct-q4f16_1, Phi1.5-q4f16_1-1k, and Mistral-7B-Instruct-v0.2-q4f16_1. Looking for contributors to improve the interface, support more models, speed up initial model loading time, and fix bugs.
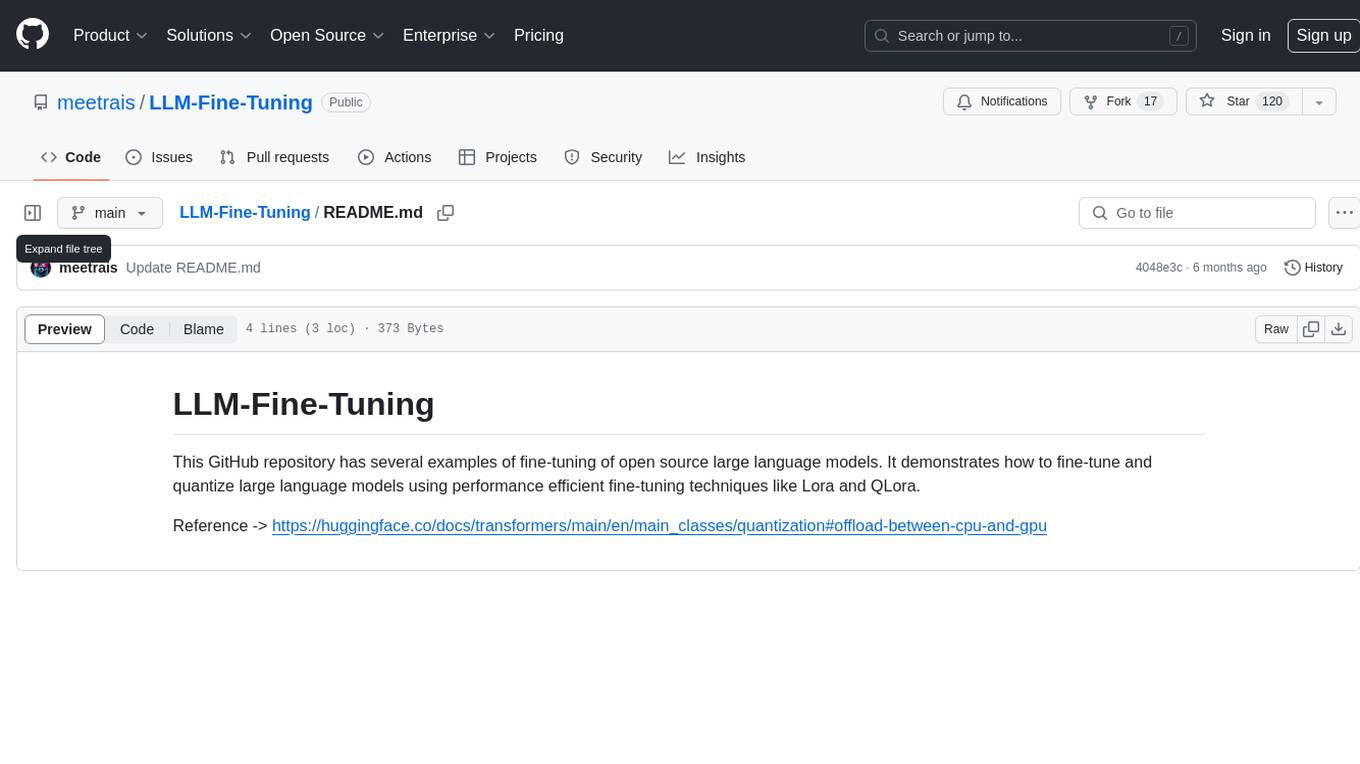
LLM-Fine-Tuning
This GitHub repository contains examples of fine-tuning open source large language models. It showcases the process of fine-tuning and quantizing large language models using efficient techniques like Lora and QLora. The repository serves as a practical guide for individuals looking to optimize the performance of language models through fine-tuning.
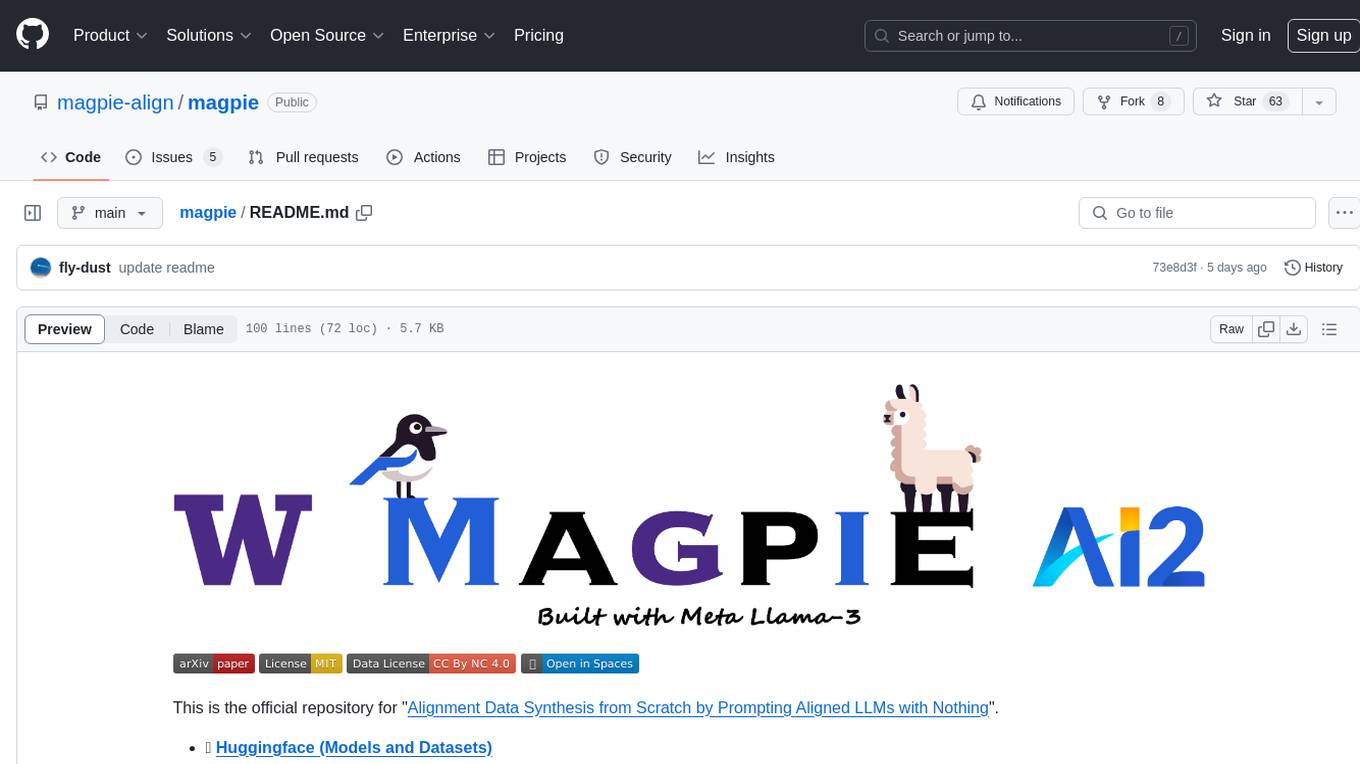
magpie
This is the official repository for 'Alignment Data Synthesis from Scratch by Prompting Aligned LLMs with Nothing'. Magpie is a tool designed to synthesize high-quality instruction data at scale by extracting it directly from an aligned Large Language Models (LLMs). It aims to democratize AI by generating large-scale alignment data and enhancing the transparency of model alignment processes. Magpie has been tested on various model families and can be used to fine-tune models for improved performance on alignment benchmarks such as AlpacaEval, ArenaHard, and WildBench.
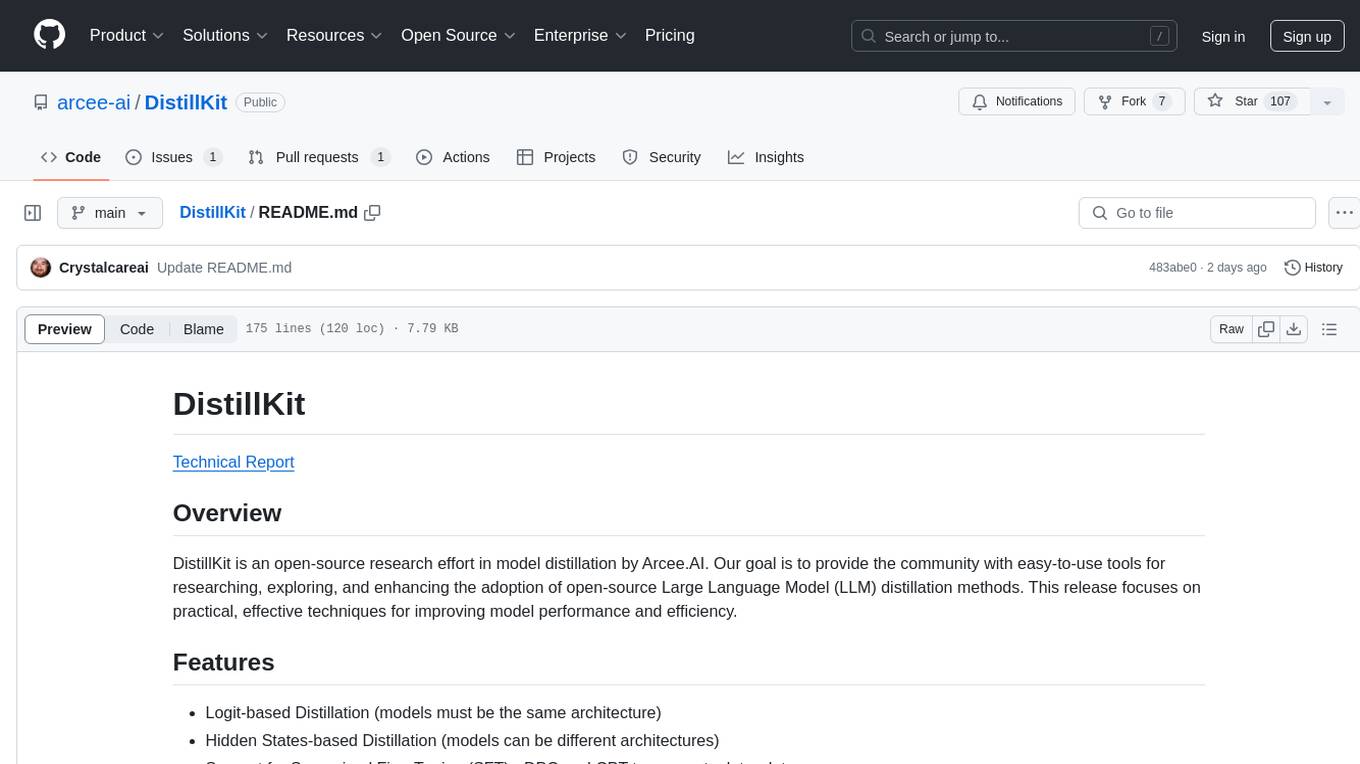
DistillKit
DistillKit is an open-source research effort by Arcee.AI focusing on model distillation methods for Large Language Models (LLMs). It provides tools for improving model performance and efficiency through logit-based and hidden states-based distillation methods. The tool supports supervised fine-tuning and aims to enhance the adoption of open-source LLM distillation techniques.
For similar jobs
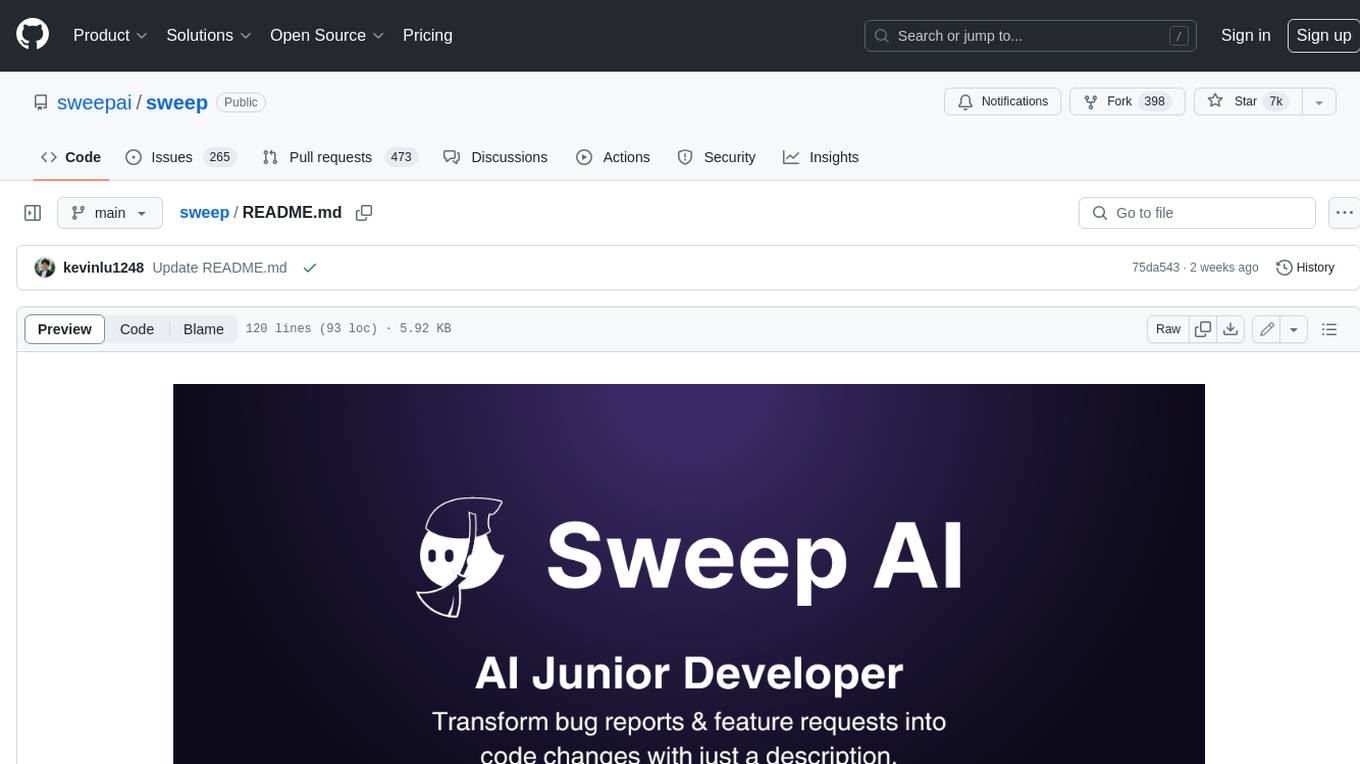
sweep
Sweep is an AI junior developer that turns bugs and feature requests into code changes. It automatically handles developer experience improvements like adding type hints and improving test coverage.
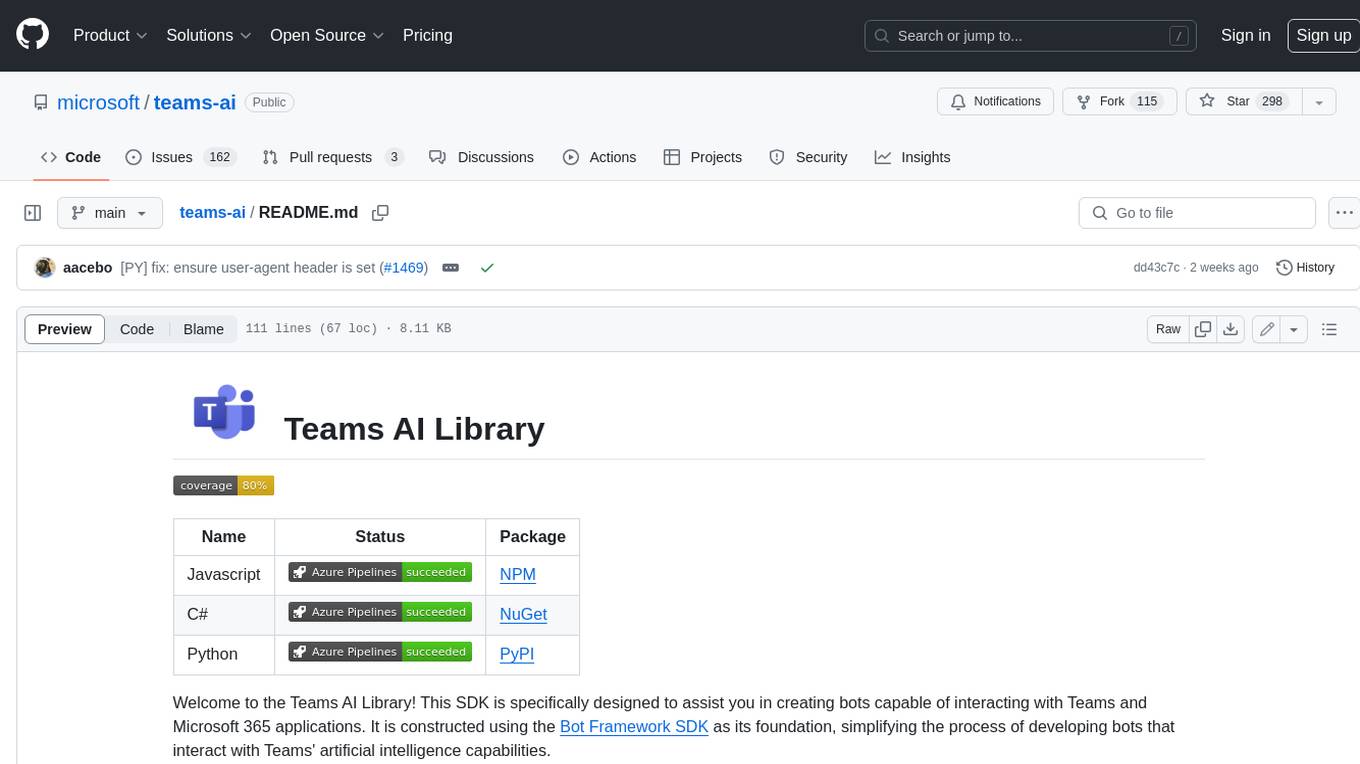
teams-ai
The Teams AI Library is a software development kit (SDK) that helps developers create bots that can interact with Teams and Microsoft 365 applications. It is built on top of the Bot Framework SDK and simplifies the process of developing bots that interact with Teams' artificial intelligence capabilities. The SDK is available for JavaScript/TypeScript, .NET, and Python.
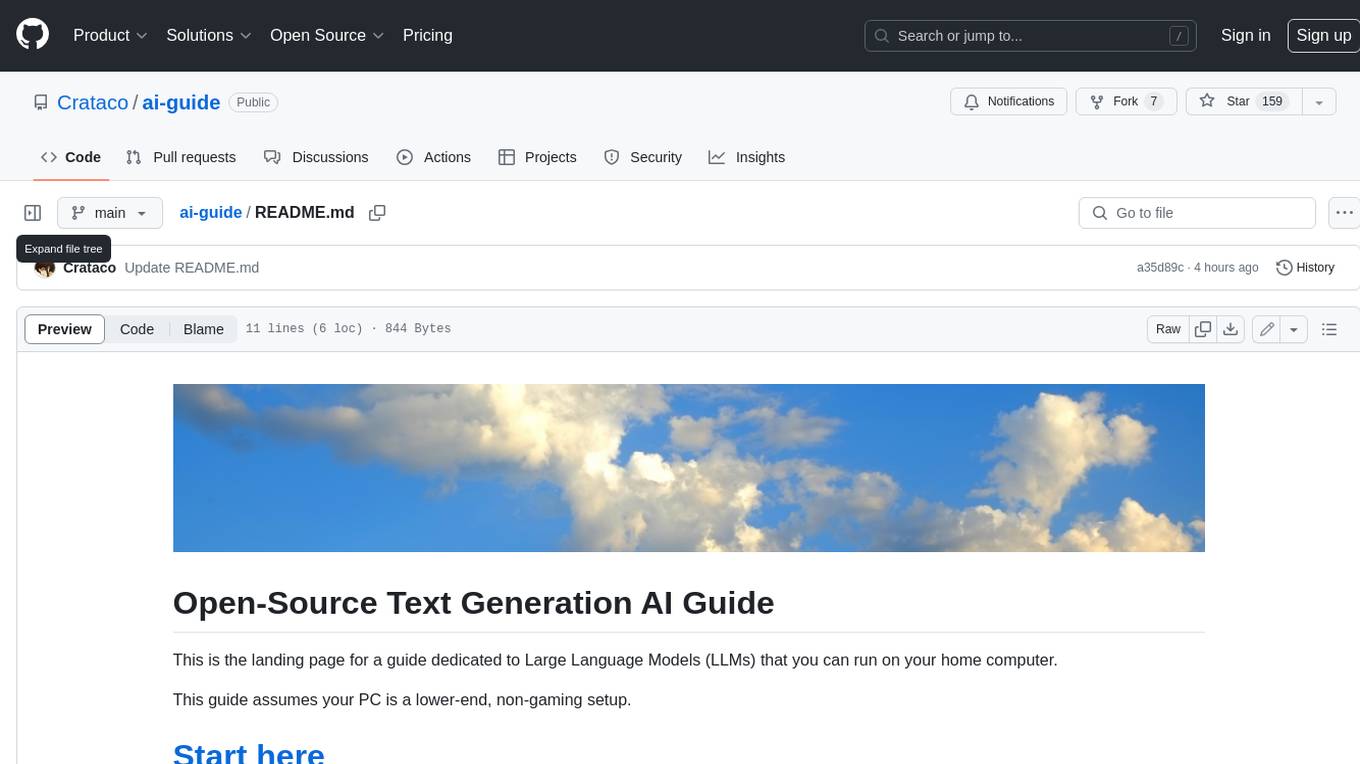
ai-guide
This guide is dedicated to Large Language Models (LLMs) that you can run on your home computer. It assumes your PC is a lower-end, non-gaming setup.
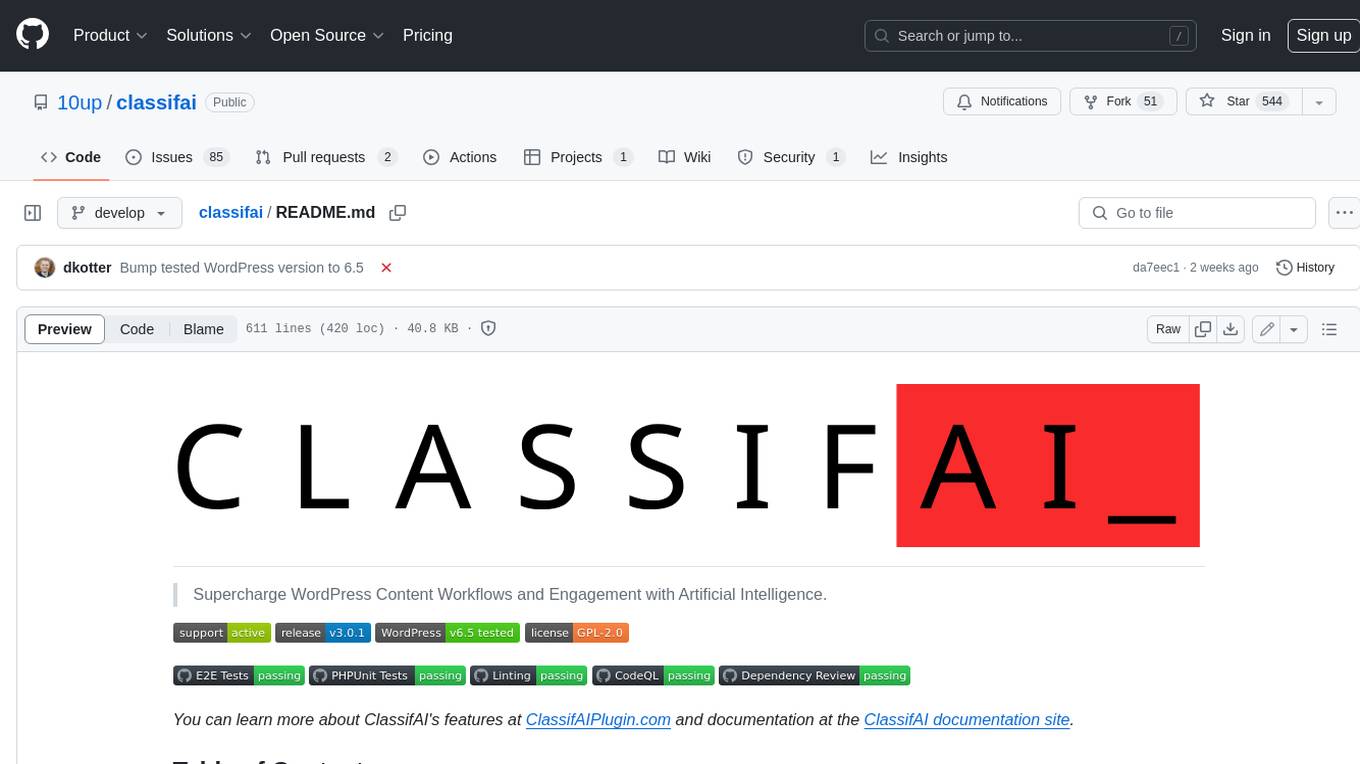
classifai
Supercharge WordPress Content Workflows and Engagement with Artificial Intelligence. Tap into leading cloud-based services like OpenAI, Microsoft Azure AI, Google Gemini and IBM Watson to augment your WordPress-powered websites. Publish content faster while improving SEO performance and increasing audience engagement. ClassifAI integrates Artificial Intelligence and Machine Learning technologies to lighten your workload and eliminate tedious tasks, giving you more time to create original content that matters.
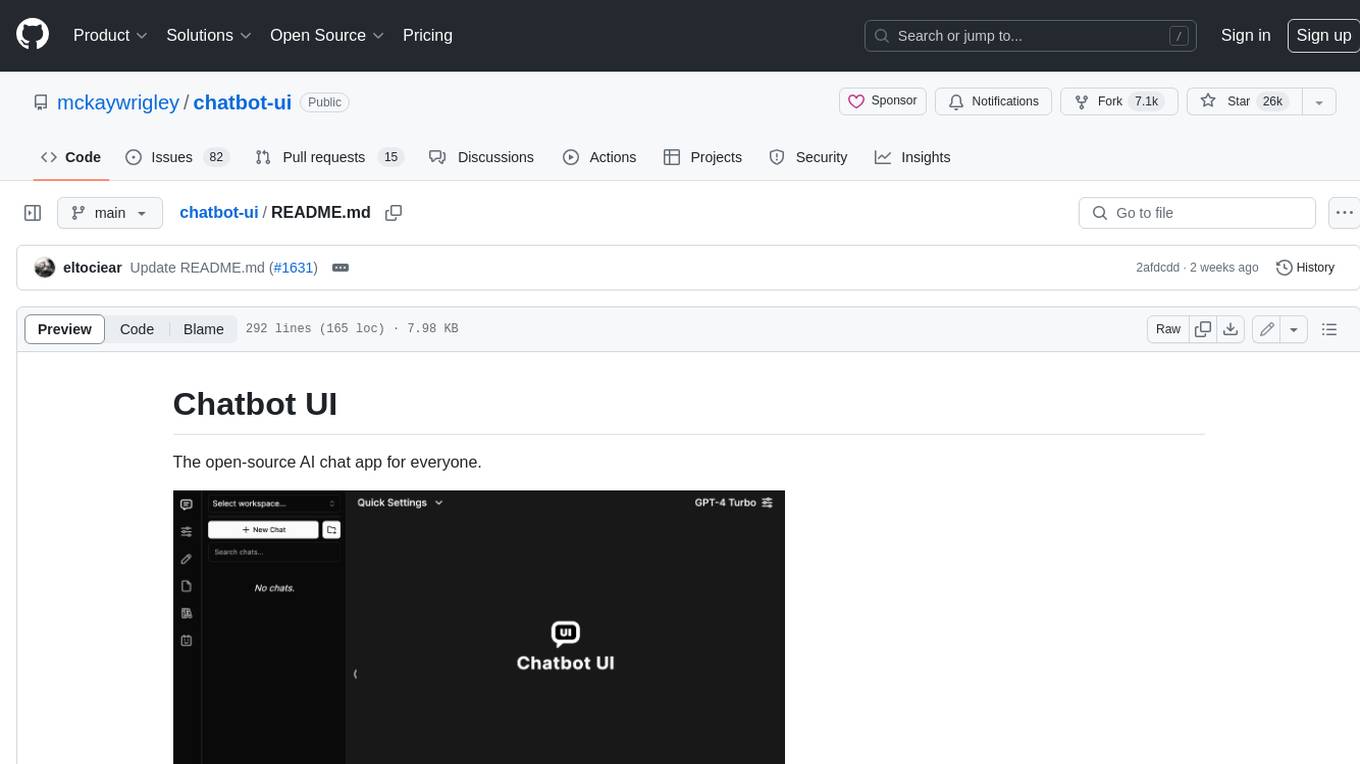
chatbot-ui
Chatbot UI is an open-source AI chat app that allows users to create and deploy their own AI chatbots. It is easy to use and can be customized to fit any need. Chatbot UI is perfect for businesses, developers, and anyone who wants to create a chatbot.
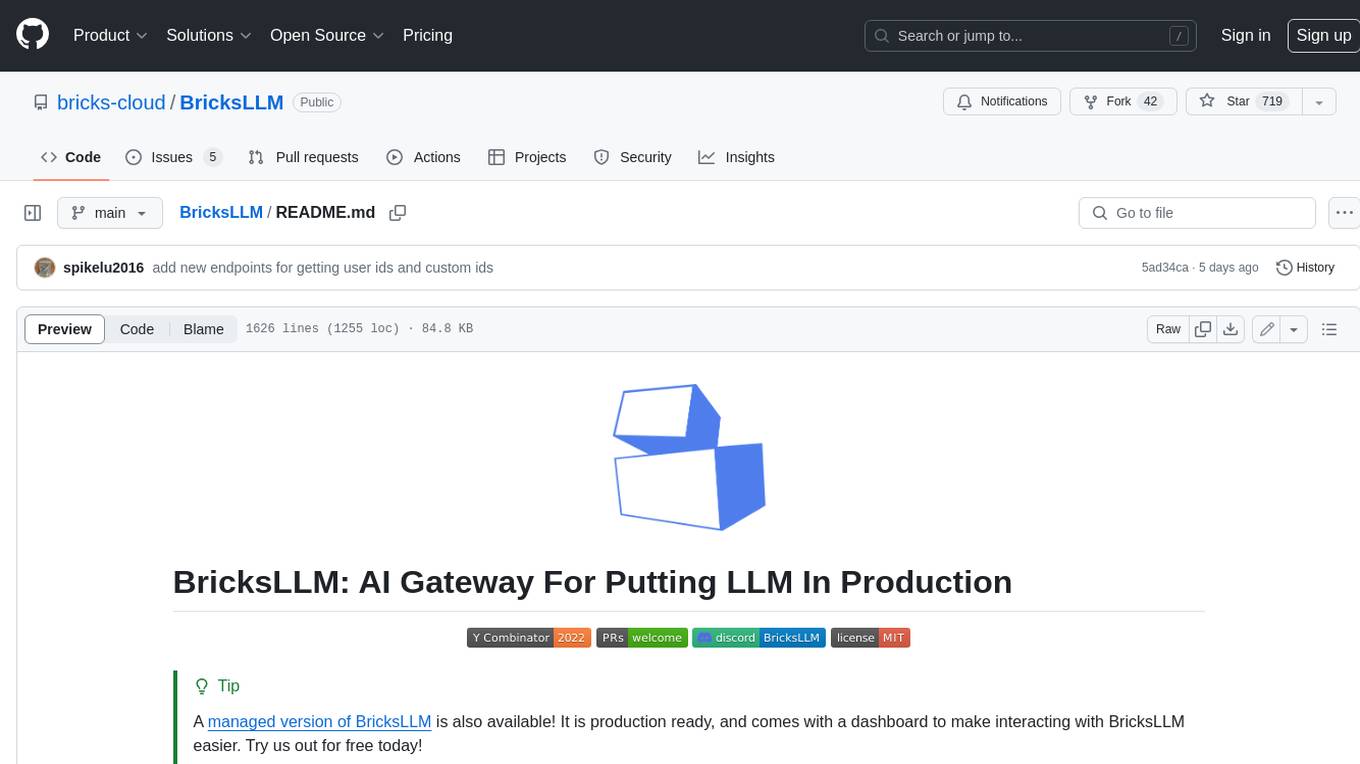
BricksLLM
BricksLLM is a cloud native AI gateway written in Go. Currently, it provides native support for OpenAI, Anthropic, Azure OpenAI and vLLM. BricksLLM aims to provide enterprise level infrastructure that can power any LLM production use cases. Here are some use cases for BricksLLM: * Set LLM usage limits for users on different pricing tiers * Track LLM usage on a per user and per organization basis * Block or redact requests containing PIIs * Improve LLM reliability with failovers, retries and caching * Distribute API keys with rate limits and cost limits for internal development/production use cases * Distribute API keys with rate limits and cost limits for students
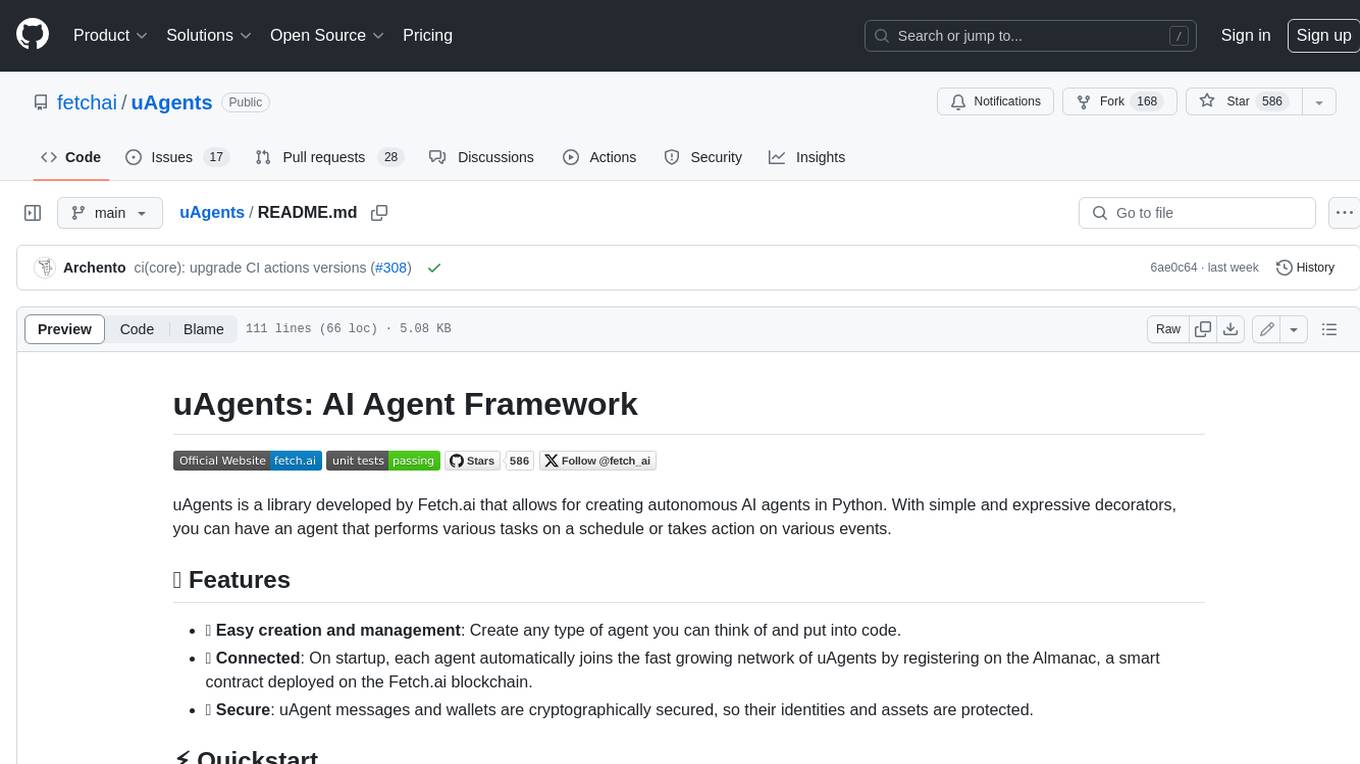
uAgents
uAgents is a Python library developed by Fetch.ai that allows for the creation of autonomous AI agents. These agents can perform various tasks on a schedule or take action on various events. uAgents are easy to create and manage, and they are connected to a fast-growing network of other uAgents. They are also secure, with cryptographically secured messages and wallets.
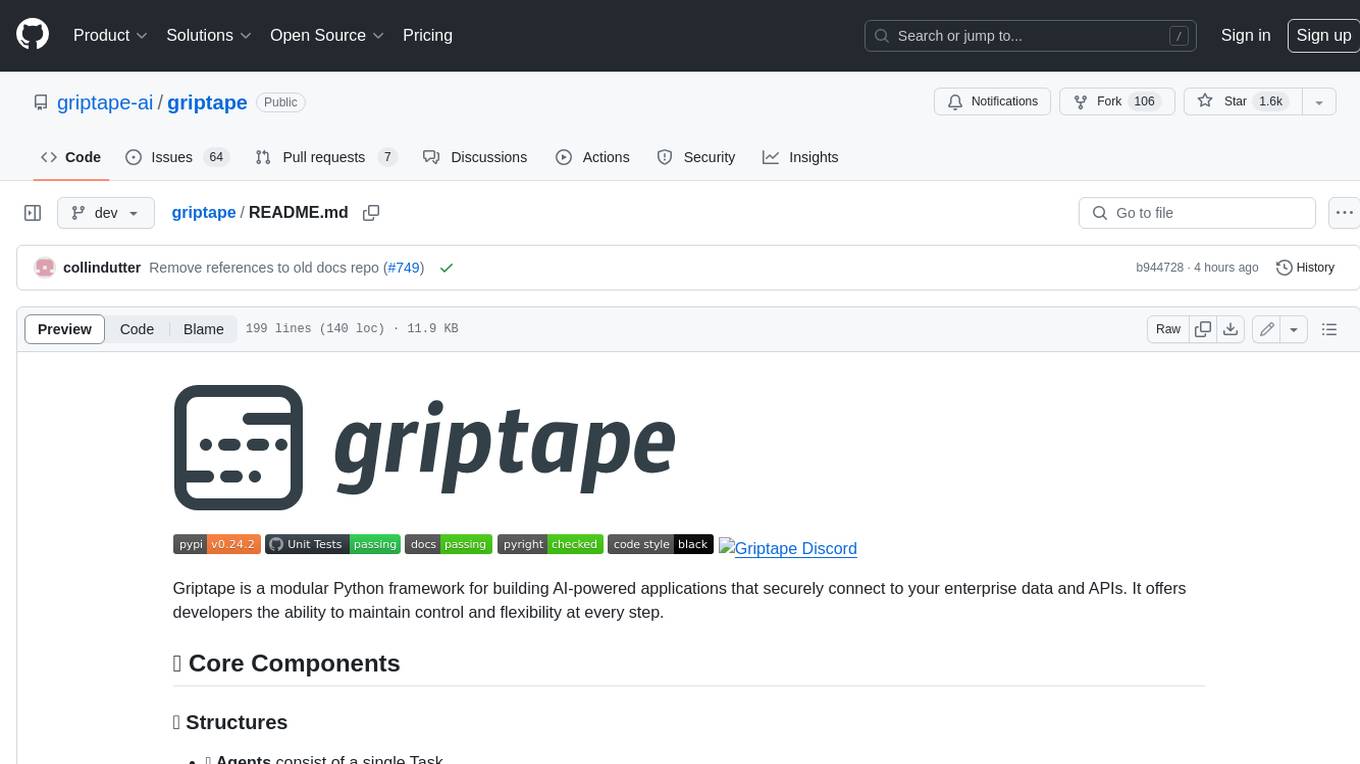
griptape
Griptape is a modular Python framework for building AI-powered applications that securely connect to your enterprise data and APIs. It offers developers the ability to maintain control and flexibility at every step. Griptape's core components include Structures (Agents, Pipelines, and Workflows), Tasks, Tools, Memory (Conversation Memory, Task Memory, and Meta Memory), Drivers (Prompt and Embedding Drivers, Vector Store Drivers, Image Generation Drivers, Image Query Drivers, SQL Drivers, Web Scraper Drivers, and Conversation Memory Drivers), Engines (Query Engines, Extraction Engines, Summary Engines, Image Generation Engines, and Image Query Engines), and additional components (Rulesets, Loaders, Artifacts, Chunkers, and Tokenizers). Griptape enables developers to create AI-powered applications with ease and efficiency.