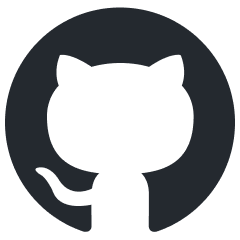
llm-reasoners
A library for advanced large language model reasoning
Stars: 1021
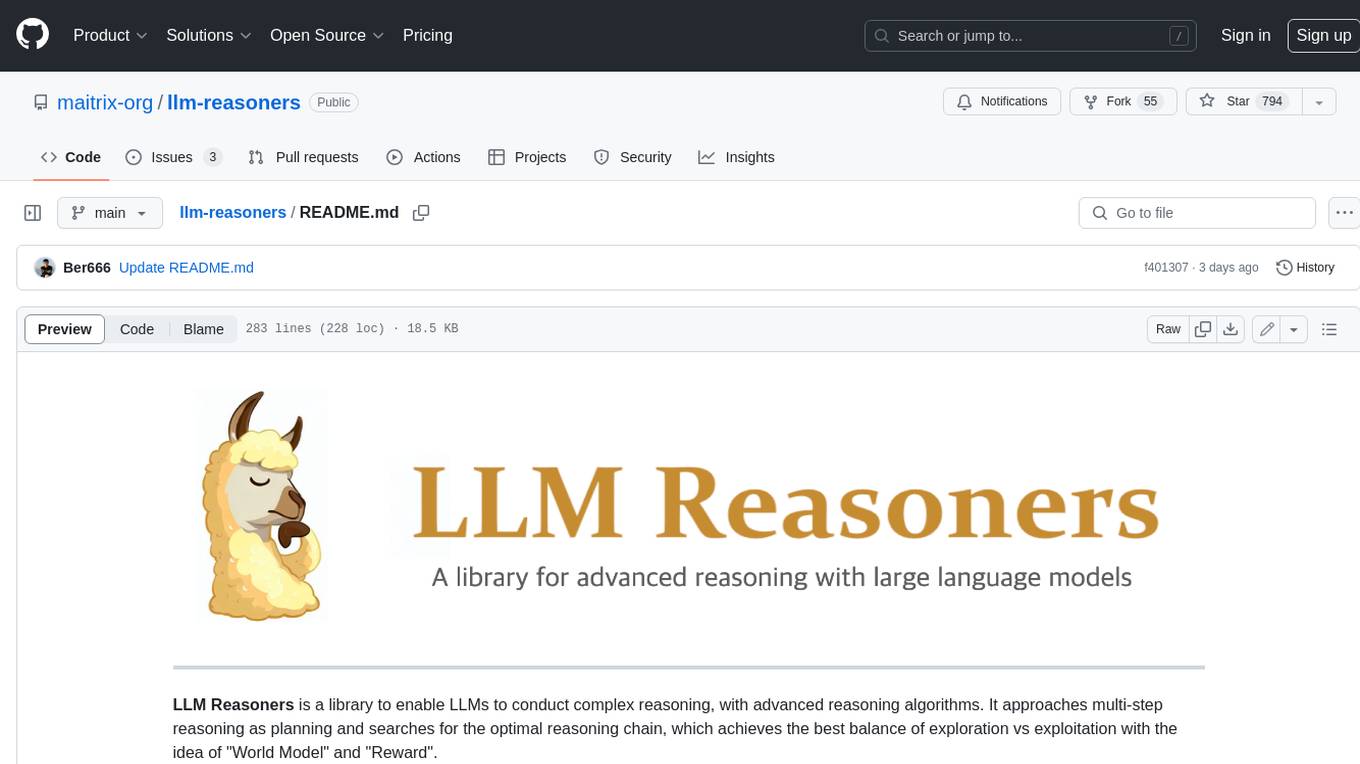
LLM Reasoners is a library that enables LLMs to conduct complex reasoning, with advanced reasoning algorithms. It approaches multi-step reasoning as planning and searches for the optimal reasoning chain, which achieves the best balance of exploration vs exploitation with the idea of "World Model" and "Reward". Given any reasoning problem, simply define the reward function and an optional world model (explained below), and let LLM reasoners take care of the rest, including Reasoning Algorithms, Visualization, LLM calling, and more!
README:
[Home] [Paper (COLM2024)] [Blog]
LLM Reasoners is a library to enable LLMs to conduct complex reasoning, with advanced reasoning algorithms. It approaches multi-step reasoning as planning and searches for the optimal reasoning chain, which achieves the best balance of exploration vs exploitation with the idea of "World Model" and "Reward".
Given any reasoning problem, simply define the reward function and an optional world model (explained below), and let LLM reasoners take care of the rest, including Reasoning Algorithms, Visualization, LLM calling, and more!
-
Jul. 10, 2024: The paper of LLM Reasoners is accepted to COLM 2024!
-
Jun. 24, 2024: PromptAgent is in LLM Reasoners! Let it help you write down a super detailed prompt for your task (here).
-
May. 14, 2024: Check out Eurus, a suit of LLMs optimized for reasoning. With LLM Reasoners, Eurus-RM can easily boost Llama-8B from 0.49 to 0.73 📈 on GSM8k (code).
-
May. 2, 2024: We have integrated our first reasoning method for scientific reasoning, StructChem! Check it out here.
-
Apr. 22, 2024: We integrated Llama-3, with additional useful APIs (e.g., customizing EOS tokens, calculating likelihood)
-
Apr. 8, 2024: Our new paper introducing LLM Reasoners is available!
-
Mar. 29, 2024: Grace Decoding has been incoporated!
-
Oct. 25, 2023: A video tutorial on the visualizer of LLM Reasoners are available.
-
Oct. 23, 2023: Reasoning-via-Planning is accepted to EMNLP 2023! Check our paper with updated results and discussion!
-
Aug. 21, 2023: A batch of quantized Llama-2 models has arrived! BitsandBytes with huggingface API, GPT-Q with exllama are available. Now you can try llama-2-70B with 2 x 24G GPUs.
-
Aug. 10, 2023: Llama-2 is supported! You can run examples with Llama-2 now.
-
Cutting-Edge Reasoning Algorithms: We offer the most up-to-date search algorithms for reasoning with LLMs, such as:
- Reasoning-via-Planning, MCTS (Hao et al., 2023)
- StructChem (Ouyang et al., 2023)
- Chain-of-thoughts (Wei et al., 202)
- Least-to-most prompting (Zhou et al., 2022)
- Tree-of-Thoughts, BFS (Yao et al., 2023)
- Tree-of-Thoughts, DFS (Yao et al., 2023)
- Self-Eval Guided Decoding, Beam Search (Xie et al., 2023)
- Grace Decoding (Khalifa et al., 2023)
- Eurus (Yuan et al., 2024)
- PromptAgent (Wang et al., 2023)
-
Intuitive Visualization and Interpretation: Our library provides a visualization tool to aid users in comprehending the reasoning process. Even for complex reasoning algorithms like Monte-Carlo Tree Search, users can easily diagnose and understand the process with one line of python code. See an exmaple in the tutorial notebook.
-
Compatibility with popular LLM libraries: Our framework is compatible with popular LLM frameworks, e.g.
Huggingface transformers
,OpenAI
/Google
/Anthropic
API, etc. Specifically, we have integrated LLaMA-1/2/3 with the option of usingfairscale
(1,2, 3), LLaMA.cpp, Exllama orhuggingface
for different needs, e.g., fastest inference speed, minimal hardware requirements, etc.
-
LLM Reasoners is applied to analyze the reasoning abilities of LLMs and the performance of multiple reasoning algorithms. See the comprehensive experiment results in the AutoRace Leaderboard, and more analysis in the blog and paper.
-
It has been tested to successfully reproduce the performance of Tree-of-Thoughts, Guided Decoding and GRACE Decoding with their official implementation. We list the results reported in their paper / reproduced from their official repositories for reference (†). Some results are on the subsets of the first 100 examples (*).
Method | Base LLM | GSM8k |
---|---|---|
Guided Decoding†| CodeX (PAL) | 0.80 |
Guided Decoding | CodeX (PAL) | 0.83* |
Method | Base LLM | Game of 24 |
---|---|---|
Tree-of-Thoughts†| GPT-3.5-turbo | 0.22 |
Tree-of-Thoughts | GPT-3.5-turbo | 0.22 |
Method | Base LLM | GSM8k |
---|---|---|
GRACE Decoding†| Flan-T5-Large (Fine-tuned) | 0.34 |
GRACE Decoding | Flan-T5-Large (Fine-tuned) | 0.33* |
Consider the following problem:
Let's start with a naive method for LLM reasoning: Prompted with a few examples of problem-solving step by step, an LLM can generate a chain of thoughts (or a sequence of actions) to solve a new problem. For the problem above, the prompt inputted to the LLM and the expected output (in bold) is shown below:
I am playing with a set of blocks where I need to arrange the blocks into stacks. (Example problems and solutions * 4) [STATEMENT] As initial conditions I have that, the red block is clear, the blue block is clear, the orange block is clear, the hand is empty, the red block is on the yellow block, the yellow block is on the table, the blue block is on the table and the orange block is on the table. My goal is to have that the orange block is on top of the blue block and the yellow block on top of the orange block. [PLAN] pick up the orange block stack the orange block on top of the blue block unstack the red block from on top of the yellow block put the red block on the table pick up the yellow block stack the yellow block on top of the orange block
Regarding each reasoning step as an action, we have $a_1=$"pick up the orange block", $a_2=$"stack the orange block on top of the blue block", and so on. At each time step, the next action is sampled from the LLM conditioned on the previous actions. This simple method is often referred to as Chain-of-thoughts reasoning. Unfortunately, it doesn't always work for complex reasoning problems. For Blocksworld dataset where the problem above comes from, even the strongest GPT-4 model can only reach the success rate of ~30%.
LLM Reasoners formulate reasoning as planning (RAP). Different from Chain-of-thoughts reasoning which autoregressively samples the next action, our goal is to efficiently search in the reasoning space for the optimal reasoning chain. To achieve this, two components need to be defined: a world model and a reward function.
-
World model defines the state transition, formally $P(s_{i+1} | s_i, a_i)$. A default world model regards the partial solution as the state and simply appends a new action/thought to the state as the state transition (the same formulation of Tree-of-Thoughts). However, you’ll have the option to design a better world model which predicts and keeps track of a more meaningful state (e.g., environment status, intermediate variable values, etc. Check RAP for more examples), thus enhancing the reasoning. For the example shown above, we can naturally define the state as the condition of blocks (e.g., the red block is on the yellow block...), and a world model is to predict the condition of blocks after every potential action.
-
Reward function provides a criterion to evaluate a reasoning step. Ideally, a reasoning chain with a higher accumulated reward should be more likely to be correct. For the example shown above, we can reward actions based on the increased number of accomplished subgoals they lead to. Besides, the likelihood of LLMs generating the action can also be used as a reward, to give the search a good prior.
After we have the world model and reward function, it's time to apply an algorithm to search for the optimal reasoning trace. Here, we show the process of Monte-Carlo Tree Search with a gif:
The three key components in a reasoning algorithm, reward function, world model, and search algorithm in the formulation (top), correspond to three classes in the library, SearchConfig, WorldModel and SearchAlgorithm respectively. Besides, there are LLM APIs to power other modules, Benchmark, and Visualization to evaluate or debug the reasoning algorithm (middle). To implement a reasoning algorithm for a certain domain (a Reasoner object), a user may inherit the SearchConfig and WorldModel class, and import a pre-implemented SearchAlgorithm. We also show a concrete example of solving Blocksworld with RAP using LLM Reasoners (bottom).
Let's go through the code of reasoning over Blocksworld problems. Note that the code is simplified for demonstration (check here for a runnable notebook).
The first step is to define the world model: you will set up an initial state given a question in init_state
, judge whether a state is terminal in is_terminal
, and most importantly, define the world dynamics with step
:
from typing import NamedTuple
import utils
from reasoners import WorldModel, LanguageModel
import copy
BWState = str
BWAction = str
class BlocksWorldModel(WorldModel[BWState, BWAction]):
def __init__(self,
base_model: LanguageModel,
prompt: dict) -> None:
super().__init__()
self.base_model = base_model
self.prompt = prompt
def init_state(self) -> BWState:
# extract the statement from a given problem
# e.g., "the red block is clear, the blue block is clear..."
return BWState(utils.extract_init_state(self.example))
def step(self, state: BWState, action: BWAction) -> tuple[BWState, dict]:
# call the LLM to predict the state transition
state = copy.deepcopy(state)
# load the prompt for the LLM to predict the next state
# e.g. "... I have that <state>, if I <action>, then ..."
world_update_prompt = self.prompt["update"].replace("<state>", state).replace("<action>", action)
world_output = self.base_model.generate([world_update_prompt],
eos_token_id="\n", hide_input=True, temperature=0).text[0].strip()
new_state = utils.process_new_state(world_output)
# till now, we have the new state after the action
# the following part is to speed up the reward calculation
# we want to check the portion of the satisfied subgoals, and use it as a part of the reward
# since we have predicted the new state already, we can just check it here at convenience
goal_reached = utils.goal_check(utils.extract_goals(self.example, new_state))
# return the new state and the additional dictionary (to be passed to the reward function)
return new_state, {"goal_reached": goal_reached}
def is_terminal(self, state: BWState) -> bool:
# define the condition the terminal state to stop the search
# e.g., all the subgoals are met
if utils.goal_check(utils.extract_goals(self.example), state.blocks_state) == 1:
return True
return False
Then, it's time to consider how to search for the optimal reasoning chain. It involves get_actions
to get the action space given a state, and the most important reward
as the guidance for reasoning. For Monte-Carlo Tree Search, we can additionally define a fast_reward
to speed up the roll-out stage.
import utils
from world_model import BWState, BWAction
from reasoners import SearchConfig, LanguageModel
class BWConfig(SearchConfig):
def __init__(self,
base_model: LanguageModel,
prompt: dict,
reward_alpha=0.5,
goal_reward_default=0.,
goal_reached_reward=100) -> None:
super().__init__()
self.base_model = base_model
self.example = None
self.prompt = prompt
# some parameters to calculate the fast reward or reward (explained below)
self.reward_alpha = reward_alpha
self.goal_reward_default = goal_reward_default
self.goal_reached_reward = goal_reached_reward
def get_actions(self, state: BWState) -> list[BWAction]:
# use a rule-based function to extract all legal actions
return utils.generate_all_actions(state)
def fast_reward(self, state: BWState, action: BWAction) -> tuple[float, dict]:
# build an in-context learning prompt (similar to the one used in Chain-of-thoughts reasoning)
inputs = self.prompt["icl"].replace("<init_state>", state)\
.replace("<goals>", utils.extract_goals(self.example))
# concatenate a candidate action after the prompt, and test its loglikelihood
intuition = self.base_model.get_loglikelihood(inputs, [inputs + action])[0]
# the reward is a combination of intuition and goal satisfaction
# in fast_reward, we skip the calculation of goal satisfaction and use a default value
fast_reward = intuition * self.reward_alpha + self.goal_reward_default * (1 - self.reward_alpha)
# cache some information for the reward calculation later (will be passed to `reward` function)
details = {'intuition': intuition}
return fast_reward, details
def reward(self, state: BWState, action: BWAction,
intuition: float = None,
goal_reached: tuple[bool, float] = None) -> float:
# note that `intuition` (cached in `fast_reward`) and `goal_reached` (cached in `step`) are automatically passed as parameters to this reward function
if goal_reached == 1:
# if the goal state is reached, we will assign a large reward
goal_reward = self.goal_reached_reward
else:
# otherwise assign the reward based on the portion of satisfied subgoals
goal_reward = goal_reached
# the reward is a combination of intuition and goal satisfaction
reward = intuition * self.reward_alpha + goal_reward * (1 - self.reward_alpha)
# return the reward and an additional dictionary (to be saved in the log for visualization later)
return reward, {'intuition': intuition, 'goal_reached': goal_reached}
Now, we are ready to apply a reasoning algorithm to solve the problem:
from reasoners.algorithm import MCTS
from reasoners.lm import LLaMAModel
from world_model import BlocksWorldModel
from search_config import BWConfig
llama_model = LLaMAModel(llama_ckpts, llama_size, max_batch_size=1)
with open(prompt_path) as f:
prompt = json.load(f)
world_model = BlocksWorldModel(base_model=base_model, prompt=prompt)
config = BWConfig(base_model=llama_model, prompt=prompt)
# save the history of every iteration for visualization
search_algo = MCTS(output_trace_in_each_iter=True)
reasoner = Reasoner(world_model=world_model, search_config=config, search_algo=search_algo)
for i, example in enumerate(dataset):
algo_output = reasoner(example)
# save the MCTS results as pickle files
with open(os.path.join(log_dir, 'algo_output', f'{resume + i + 1}.pkl'), 'wb') as f:
pickle.dump(algo_output, f)
Finally, we can easily visualize the reasoning process:
import pickle
from reasoners.visualization import visualize
with open("logs/bw_MCTS/xxx/algo_output/1.pkl", 'rb') as f:
mcts_result = pickle.load(f)
from reasoners.visualization.tree_snapshot import NodeData
from reasoners.algorithm.mcts import MCTSNode
# by default, a state will be presented along with the node, and the reward with saved dictionary in `SearchConfig.reward` will be presented along with the edge.
# we can also define a helper function to customize what we want to see in the visualizer.
def blocksworld_node_data_factory(n: MCTSNode) -> NodeData:
return NodeData({"block state": n.state.blocks_state if n.state else None,
"satisfied": n.fast_reward_details if n.fast_reward_details else "Not expanded"})
def blocksworld_edge_data_factory(n: MCTSNode) -> EdgeData:
return EdgeData({"reward": n.reward, "intuition": n.fast_reward_details["intuition"]})
visualize(mcts_result, node_data_factory=blocksworld_node_data_factory,
edge_data_factory=blocksworld_edge_data_factory)
Then a URL of the visualized results will pop up. The figure will be interactive and look like the examples shown on our demo website.
Make sure to use Python 3.10 or later.
conda create -n reasoners python=3.10
conda activate reasoners
Clone the repository and install the package:
git clone https://github.com/Ber666/llm-reasoners --recursive
cd llm-reasoners
pip install -e .
Adding --recursive
will help you clone exllama automatically. Note that some other optional modules may need other dependencies. Please refer to the error message for details.
This project is an extension of the following paper:
@inproceedings{hao2023reasoning,
title={Reasoning with Language Model is Planning with World Model},
author={Hao, Shibo and Gu, Yi and Ma, Haodi and Hong, Joshua and Wang, Zhen and Wang, Daisy and Hu, Zhiting},
booktitle={Proceedings of the 2023 Conference on Empirical Methods in Natural Language Processing},
pages={8154--8173},
year={2023}
}
@article{hao2024llm,
title={LLM Reasoners: New Evaluation, Library, and Analysis of Step-by-Step Reasoning with Large Language Models},
author={Hao, Shibo and Gu, Yi and Luo, Haotian and Liu, Tianyang and Shao, Xiyan and Wang, Xinyuan and Xie, Shuhua and Ma, Haodi and Samavedhi, Adithya and Gao, Qiyue and others},
journal={arXiv preprint arXiv:2404.05221},
year={2024}
}
For Tasks:
Click tags to check more tools for each tasksFor Jobs:
Alternative AI tools for llm-reasoners
Similar Open Source Tools
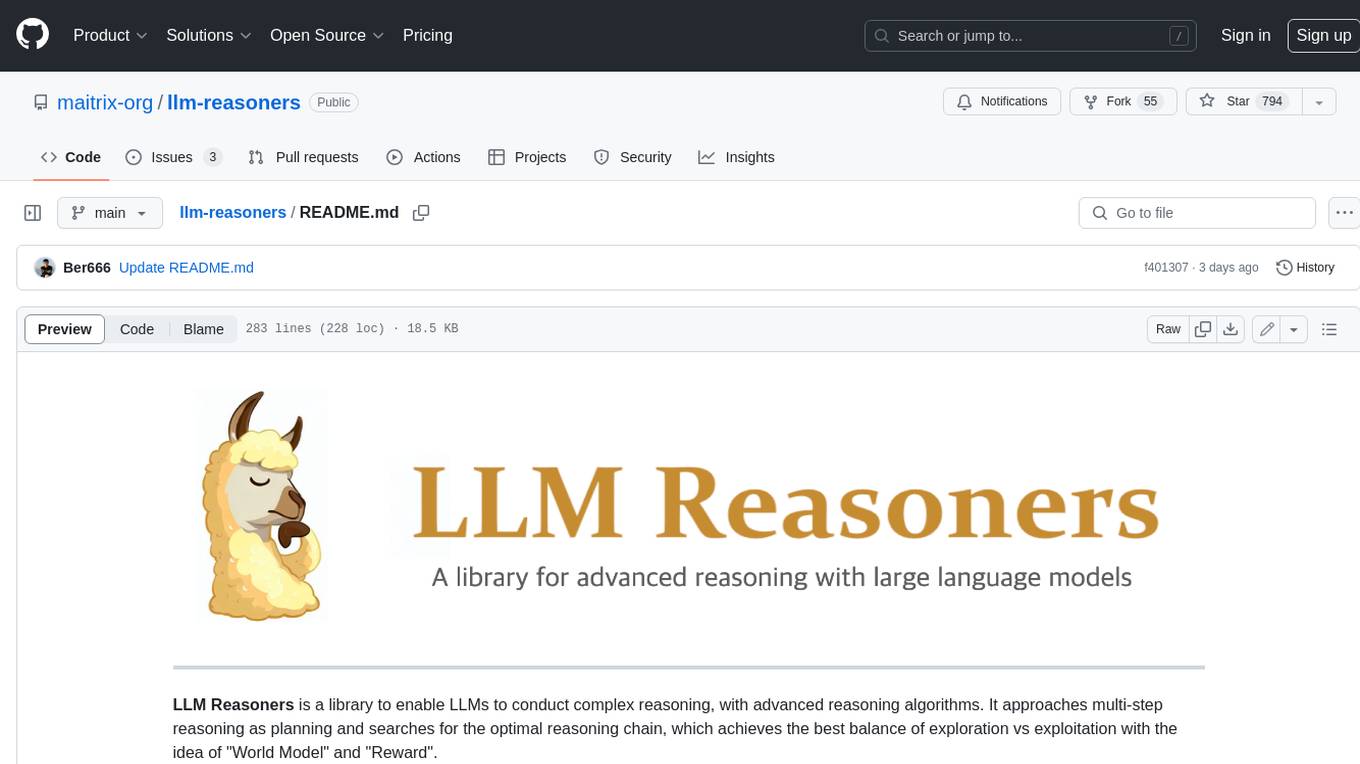
llm-reasoners
LLM Reasoners is a library that enables LLMs to conduct complex reasoning, with advanced reasoning algorithms. It approaches multi-step reasoning as planning and searches for the optimal reasoning chain, which achieves the best balance of exploration vs exploitation with the idea of "World Model" and "Reward". Given any reasoning problem, simply define the reward function and an optional world model (explained below), and let LLM reasoners take care of the rest, including Reasoning Algorithms, Visualization, LLM calling, and more!
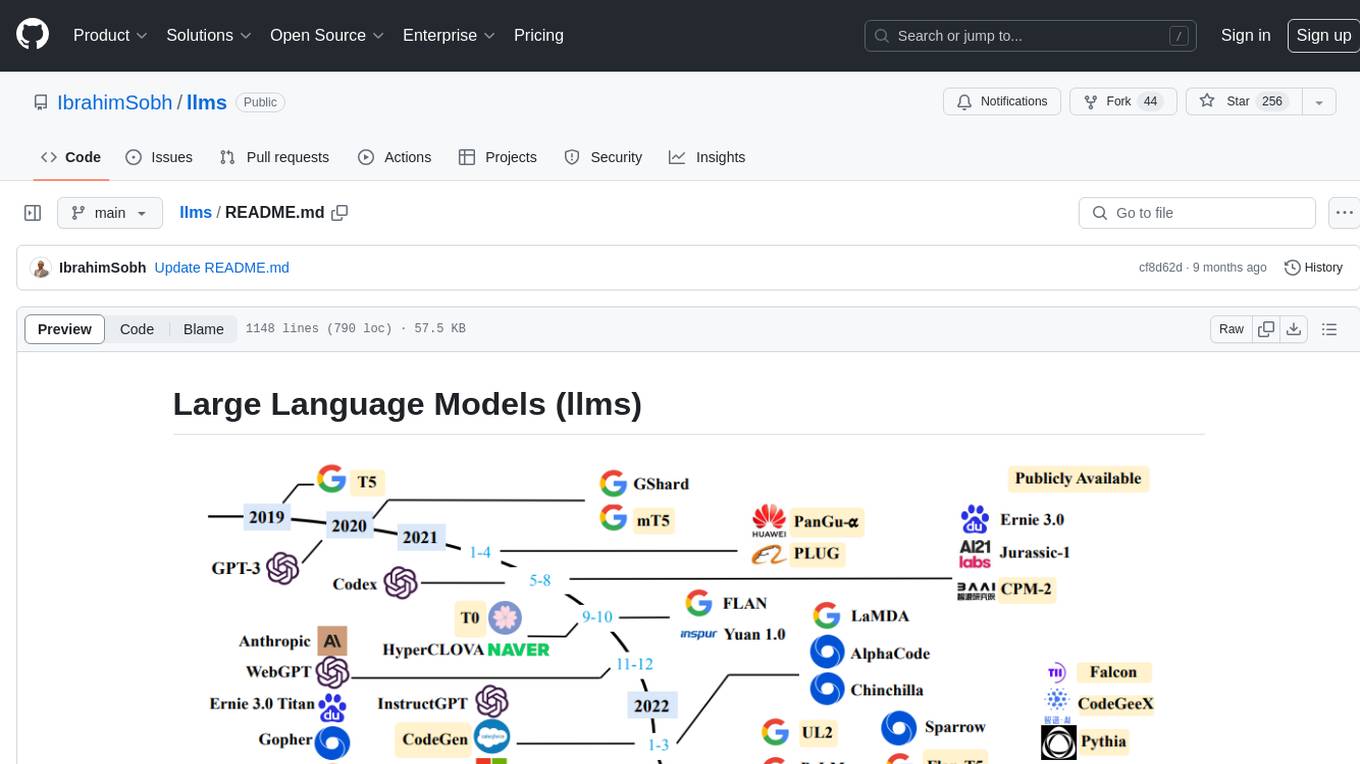
llms
The 'llms' repository is a comprehensive guide on Large Language Models (LLMs), covering topics such as language modeling, applications of LLMs, statistical language modeling, neural language models, conditional language models, evaluation methods, transformer-based language models, practical LLMs like GPT and BERT, prompt engineering, fine-tuning LLMs, retrieval augmented generation, AI agents, and LLMs for computer vision. The repository provides detailed explanations, examples, and tools for working with LLMs.
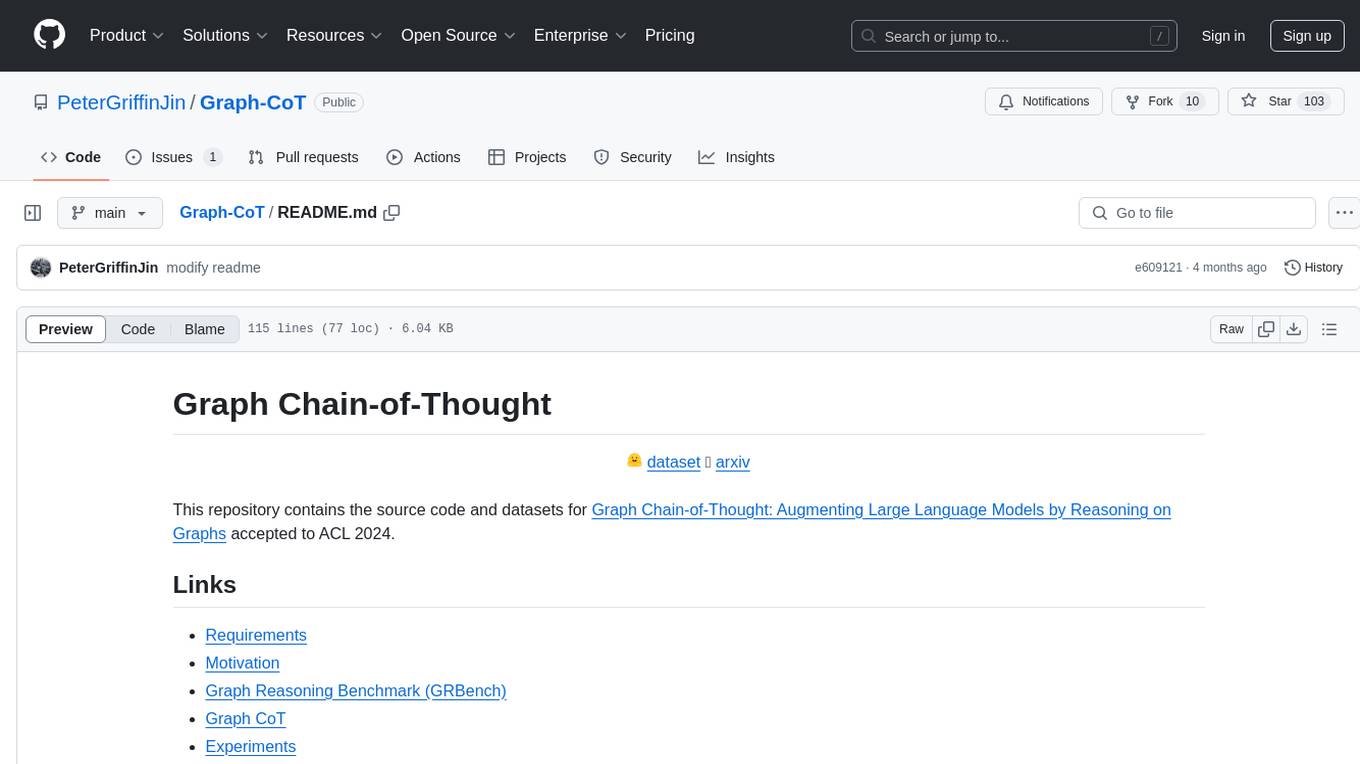
Graph-CoT
This repository contains the source code and datasets for Graph Chain-of-Thought: Augmenting Large Language Models by Reasoning on Graphs accepted to ACL 2024. It proposes a framework called Graph Chain-of-thought (Graph-CoT) to enable Language Models to traverse graphs step-by-step for reasoning, interaction, and execution. The motivation is to alleviate hallucination issues in Language Models by augmenting them with structured knowledge sources represented as graphs.
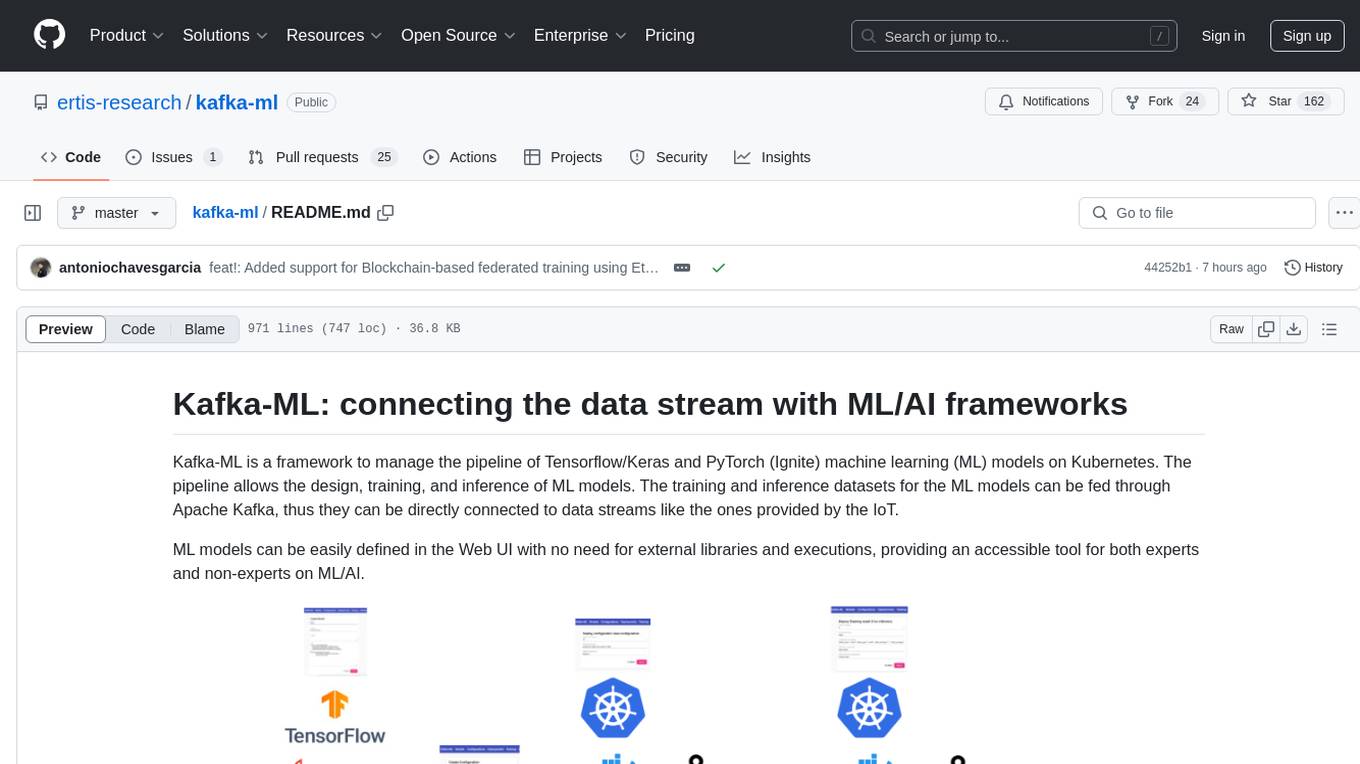
kafka-ml
Kafka-ML is a framework designed to manage the pipeline of Tensorflow/Keras and PyTorch machine learning models on Kubernetes. It enables the design, training, and inference of ML models with datasets fed through Apache Kafka, connecting them directly to data streams like those from IoT devices. The Web UI allows easy definition of ML models without external libraries, catering to both experts and non-experts in ML/AI.
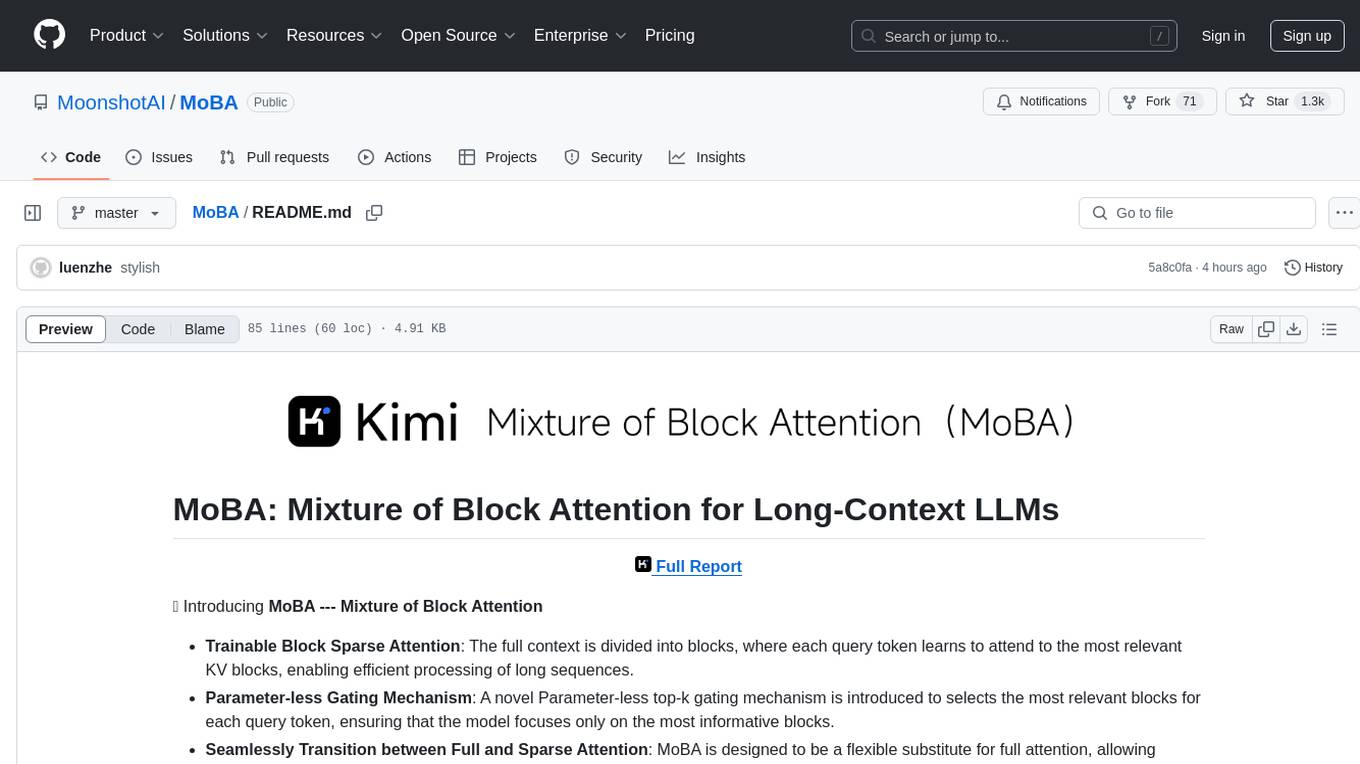
MoBA
MoBA (Mixture of Block Attention) is an innovative approach for long-context language models, enabling efficient processing of long sequences by dividing the full context into blocks and introducing a parameter-less gating mechanism. It allows seamless transitions between full and sparse attention modes, enhancing efficiency without compromising performance. MoBA has been deployed to support long-context requests and demonstrates significant advancements in efficient attention computation for large language models.
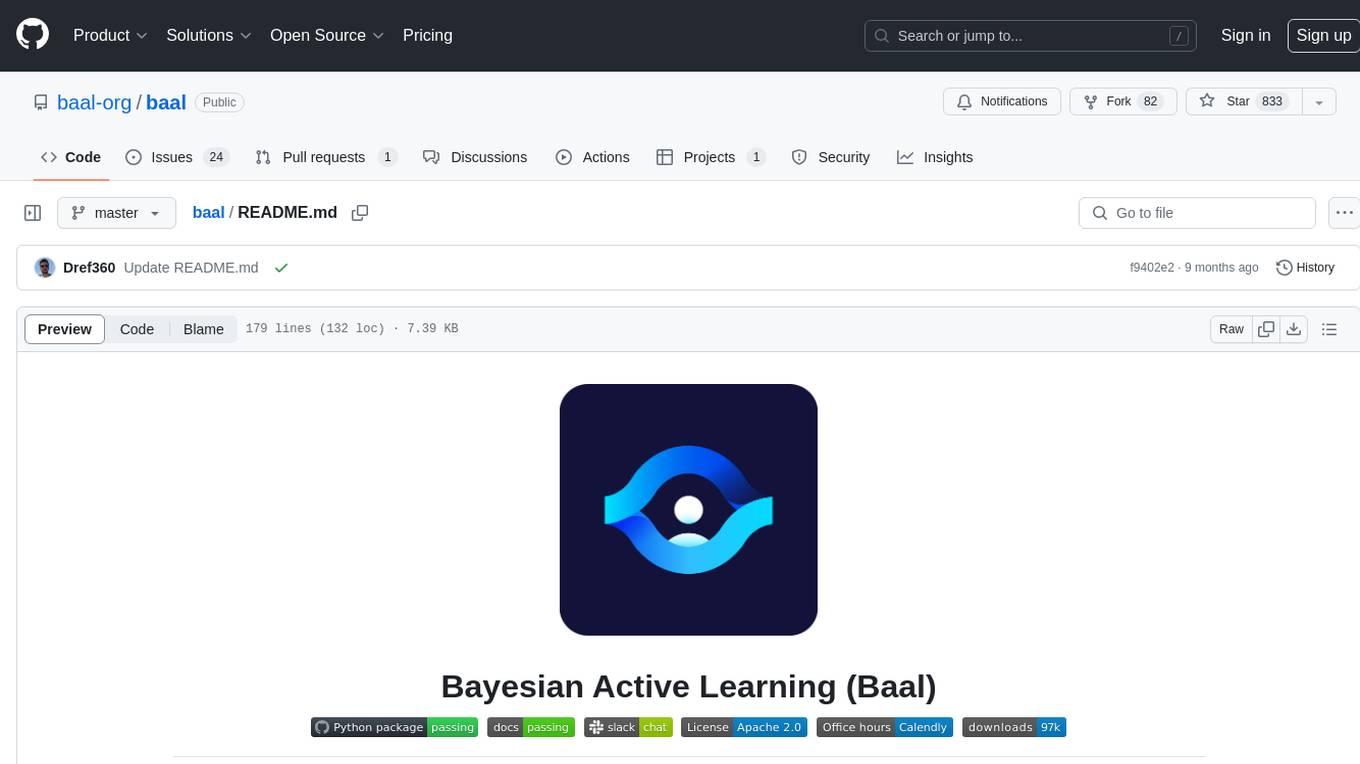
baal
Baal is an active learning library that supports both industrial applications and research use cases. It provides a framework for Bayesian active learning methods such as Monte-Carlo Dropout, MCDropConnect, Deep ensembles, and Semi-supervised learning. Baal helps in labeling the most uncertain items in the dataset pool to improve model performance and reduce annotation effort. The library is actively maintained by a dedicated team and has been used in various research papers for production and experimentation.
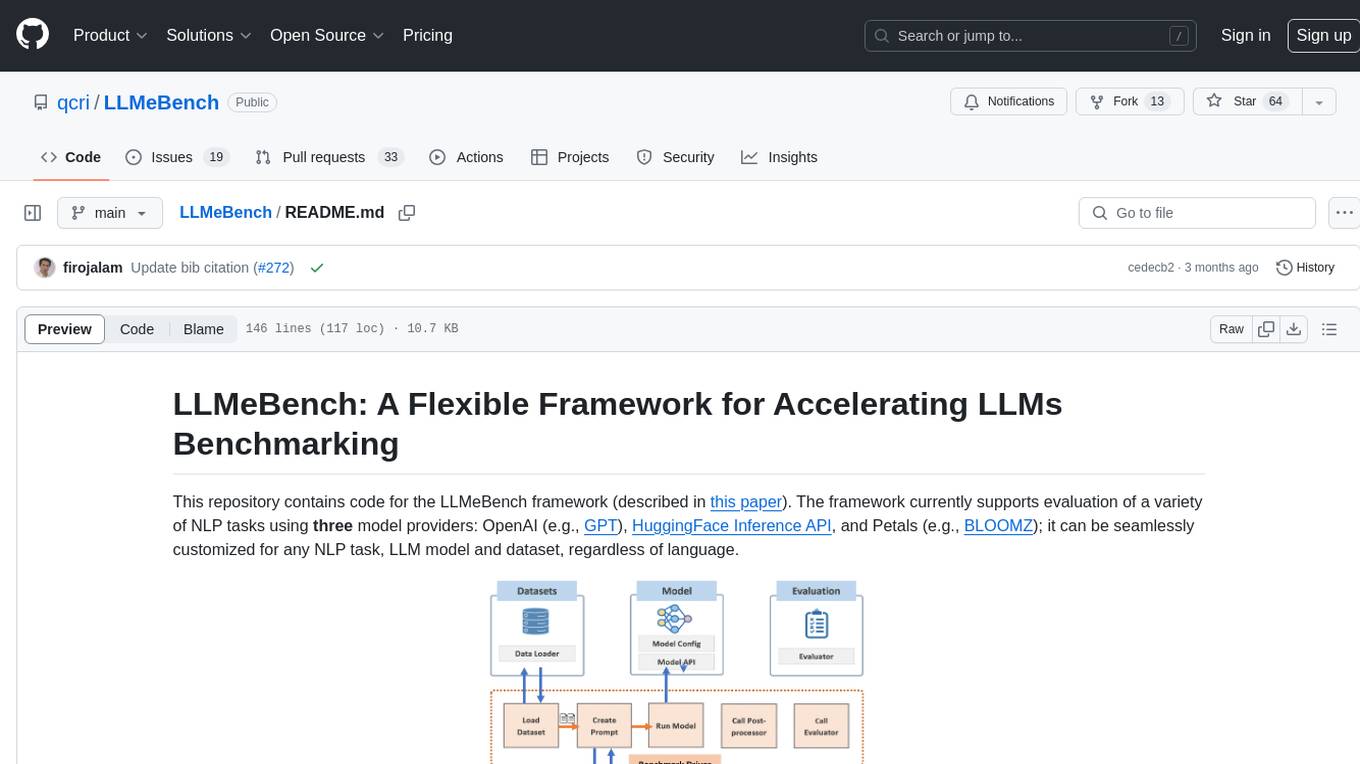
LLMeBench
LLMeBench is a flexible framework designed for accelerating benchmarking of Large Language Models (LLMs) in the field of Natural Language Processing (NLP). It supports evaluation of various NLP tasks using model providers like OpenAI, HuggingFace Inference API, and Petals. The framework is customizable for different NLP tasks, LLM models, and datasets across multiple languages. It features extensive caching capabilities, supports zero- and few-shot learning paradigms, and allows on-the-fly dataset download and caching. LLMeBench is open-source and continuously expanding to support new models accessible through APIs.
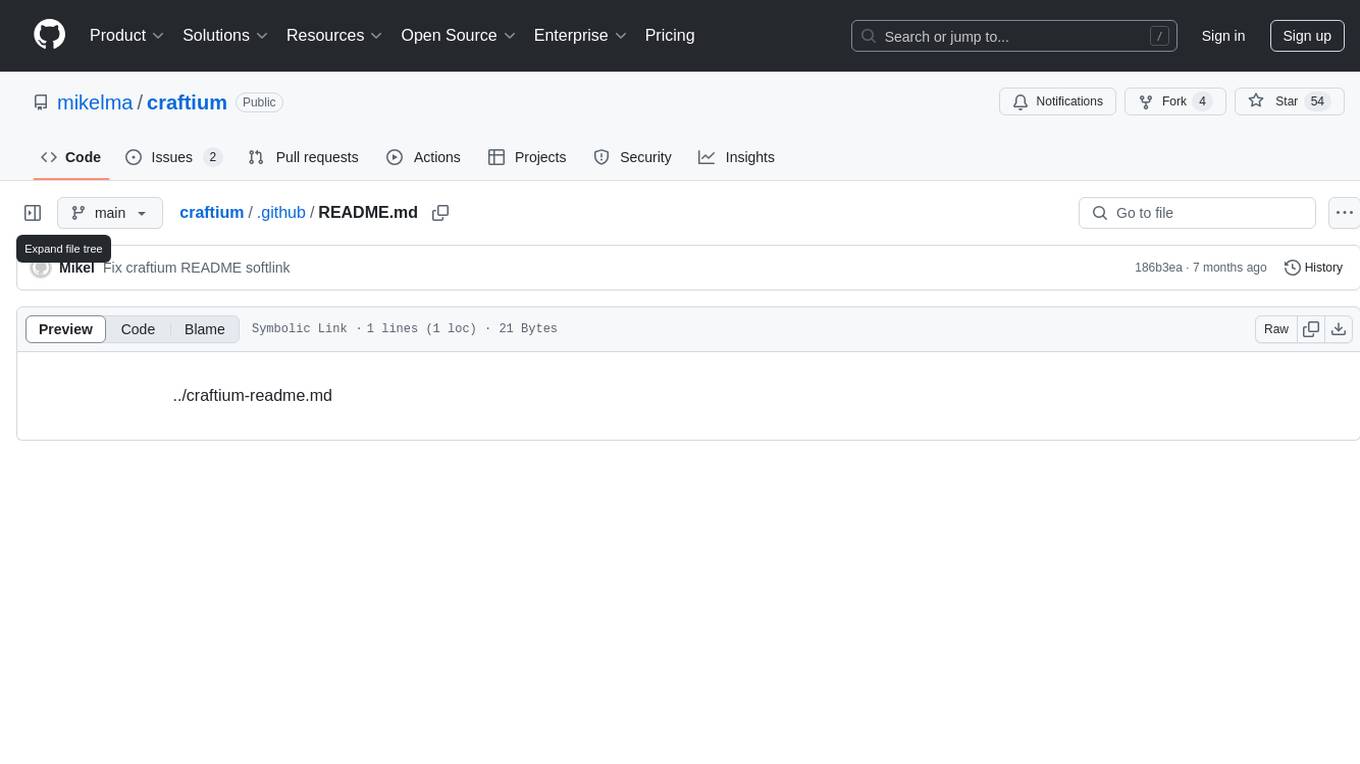
craftium
Craftium is an open-source platform based on the Minetest voxel game engine and the Gymnasium and PettingZoo APIs, designed for creating fast, rich, and diverse single and multi-agent environments. It allows for connecting to Craftium's Python process, executing actions as keyboard and mouse controls, extending the Lua API for creating RL environments and tasks, and supporting client/server synchronization for slow agents. Craftium is fully extensible, extensively documented, modern RL API compatible, fully open source, and eliminates the need for Java. It offers a variety of environments for research and development in reinforcement learning.
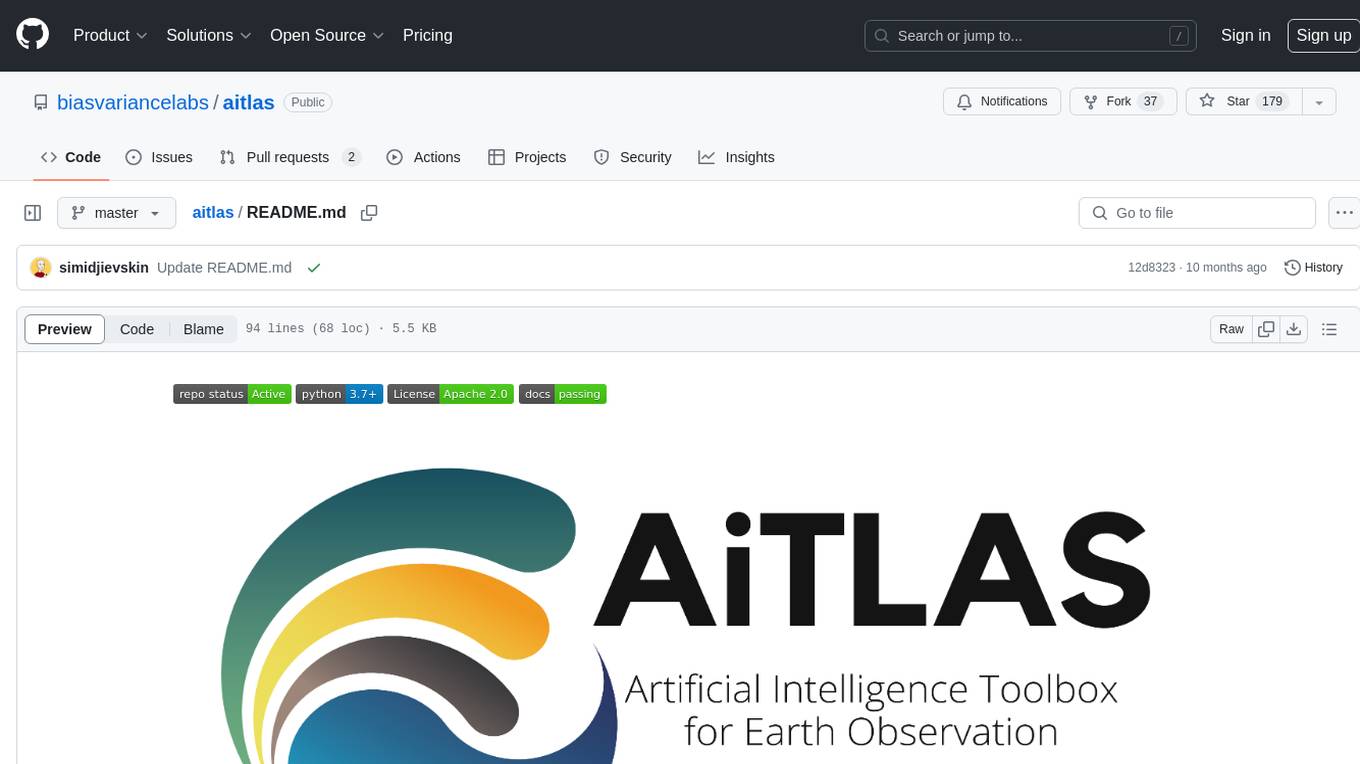
aitlas
The AiTLAS toolbox (Artificial Intelligence Toolbox for Earth Observation) includes state-of-the-art machine learning methods for exploratory and predictive analysis of satellite imagery as well as a repository of AI-ready Earth Observation (EO) datasets. It can be easily applied for a variety of Earth Observation tasks, such as land use and cover classification, crop type prediction, localization of specific objects (semantic segmentation), etc. The main goal of AiTLAS is to facilitate better usability and adoption of novel AI methods (and models) by EO experts, while offering easy access and standardized format of EO datasets to AI experts which allows benchmarking of various existing and novel AI methods tailored for EO data.
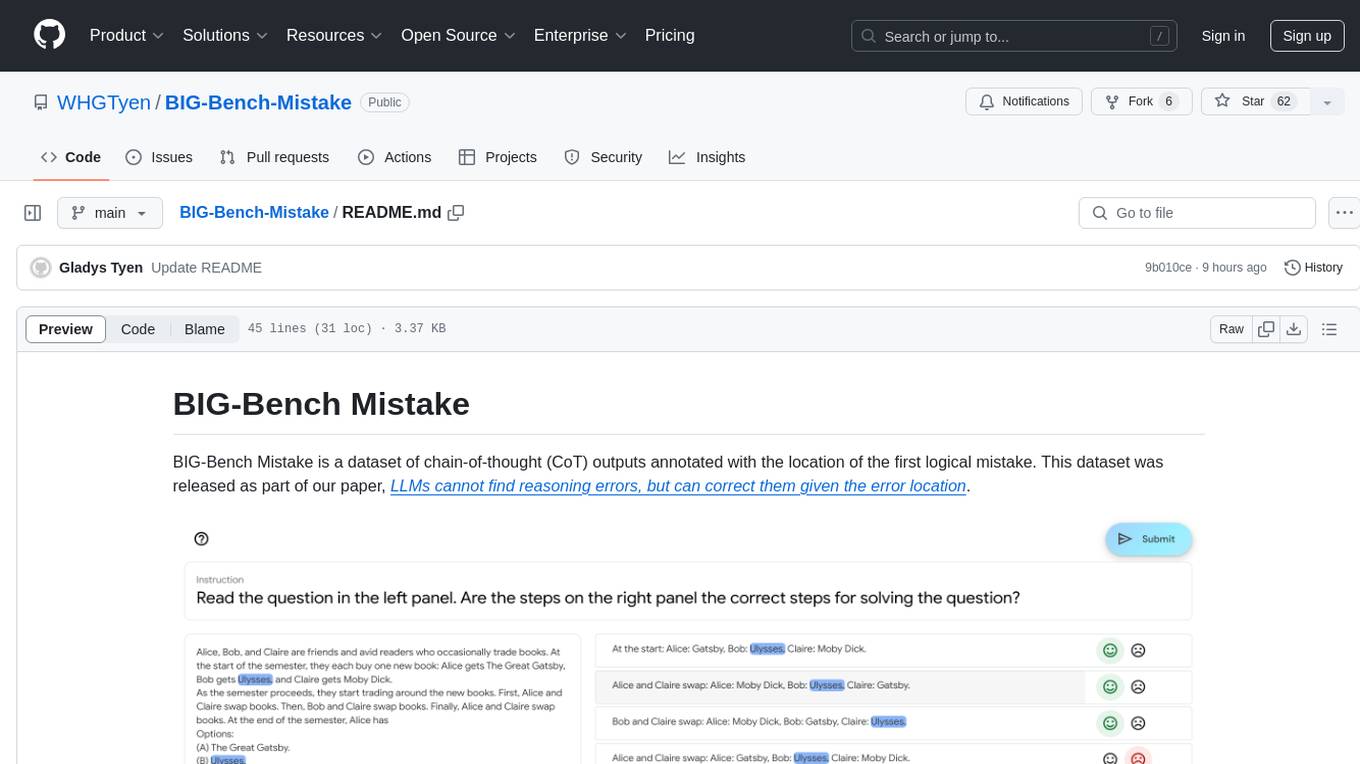
BIG-Bench-Mistake
BIG-Bench Mistake is a dataset of chain-of-thought (CoT) outputs annotated with the location of the first logical mistake. It was released as part of a research paper focusing on benchmarking LLMs in terms of their mistake-finding ability. The dataset includes CoT traces for tasks like Word Sorting, Tracking Shuffled Objects, Logical Deduction, Multistep Arithmetic, and Dyck Languages. Human annotators were recruited to identify mistake steps in these tasks, with automated annotation for Dyck Languages. Each JSONL file contains input questions, steps in the chain of thoughts, model's answer, correct answer, and the index of the first logical mistake.
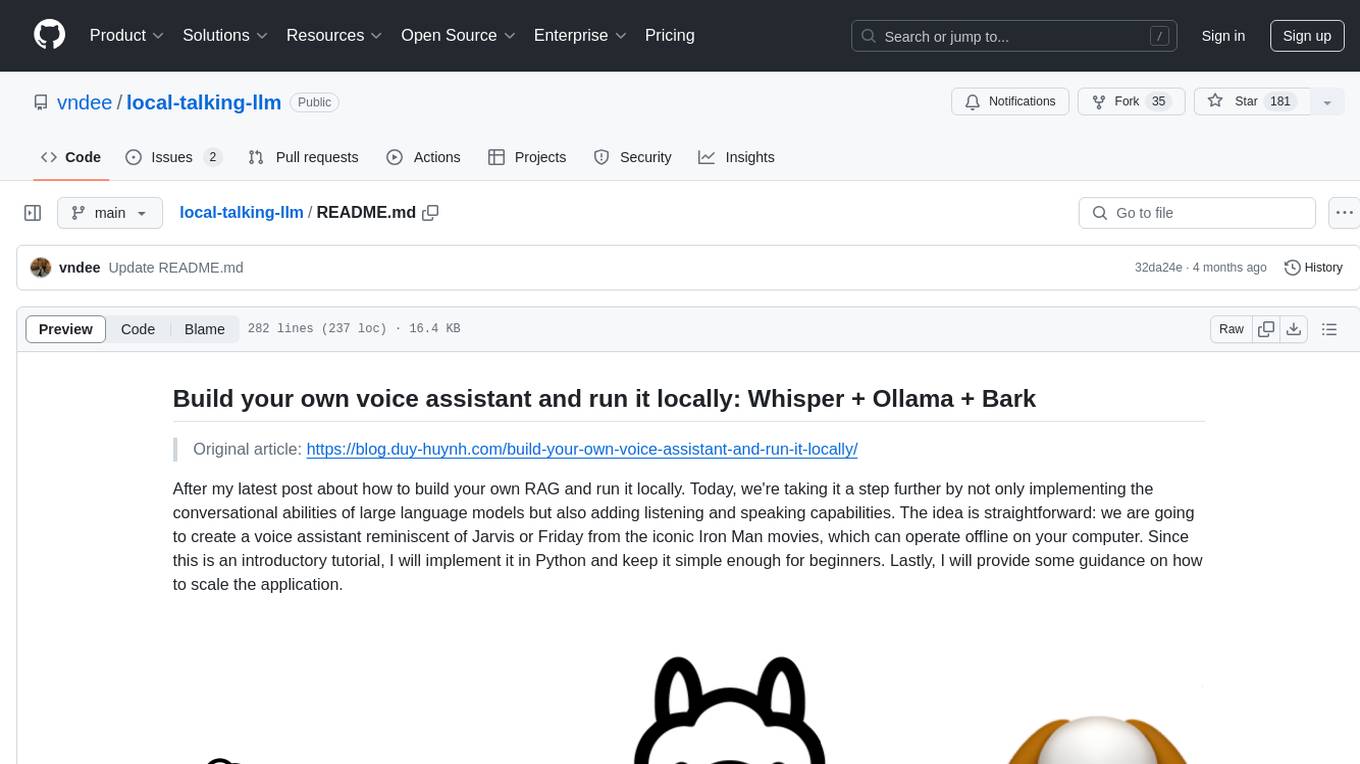
local-talking-llm
The 'local-talking-llm' repository provides a tutorial on building a voice assistant similar to Jarvis or Friday from Iron Man movies, capable of offline operation on a computer. The tutorial covers setting up a Python environment, installing necessary libraries like rich, openai-whisper, suno-bark, langchain, sounddevice, pyaudio, and speechrecognition. It utilizes Ollama for Large Language Model (LLM) serving and includes components for speech recognition, conversational chain, and speech synthesis. The implementation involves creating a TextToSpeechService class for Bark, defining functions for audio recording, transcription, LLM response generation, and audio playback. The main application loop guides users through interactive voice-based conversations with the assistant.
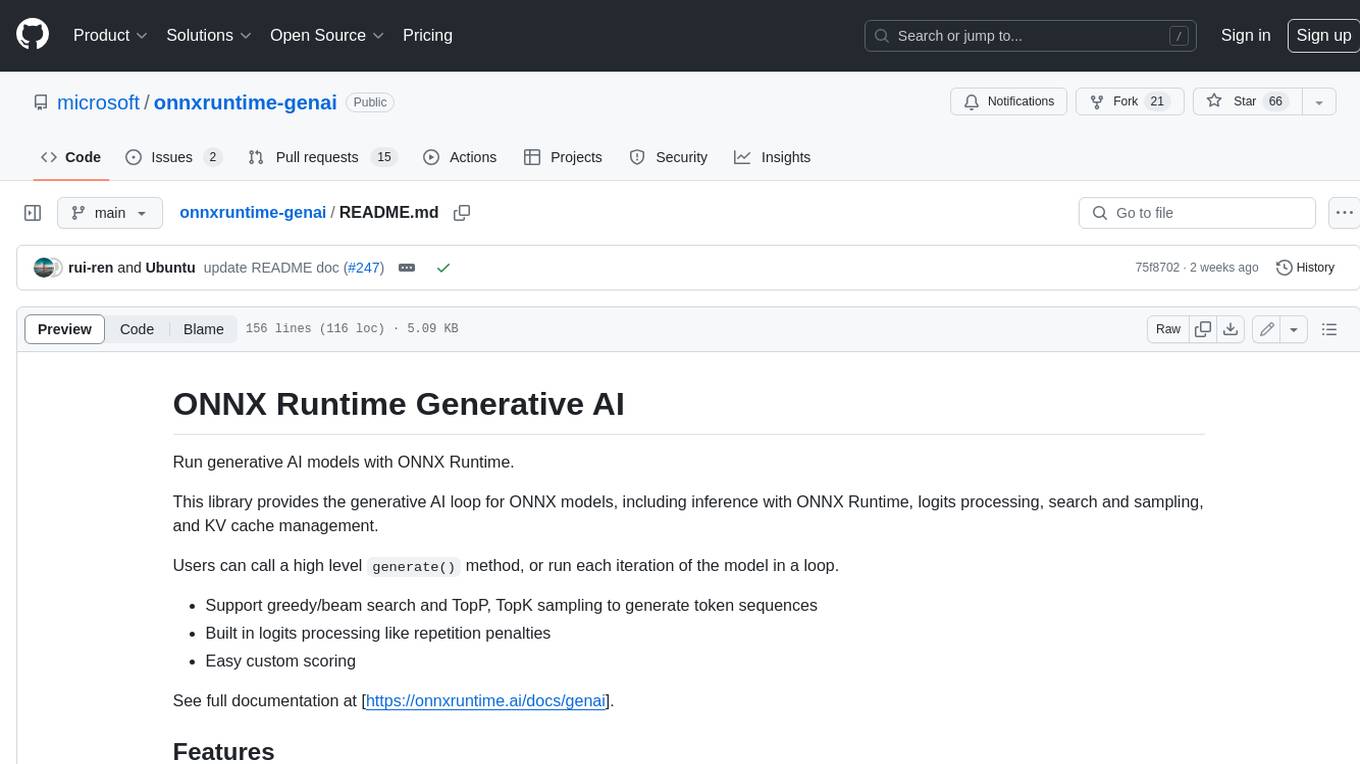
onnxruntime-genai
ONNX Runtime Generative AI is a library that provides the generative AI loop for ONNX models, including inference with ONNX Runtime, logits processing, search and sampling, and KV cache management. Users can call a high level `generate()` method, or run each iteration of the model in a loop. It supports greedy/beam search and TopP, TopK sampling to generate token sequences, has built in logits processing like repetition penalties, and allows for easy custom scoring.
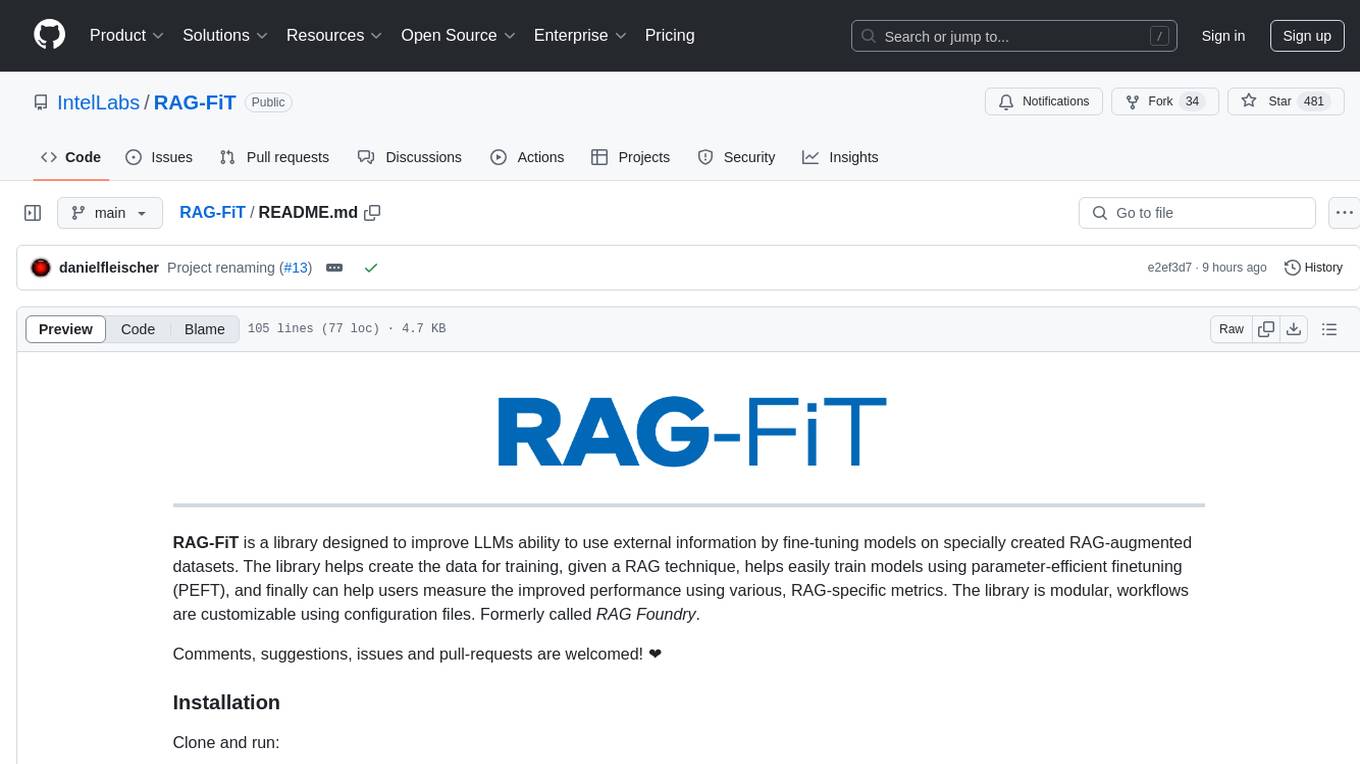
RAG-FiT
RAG-FiT is a library designed to improve Language Models' ability to use external information by fine-tuning models on specially created RAG-augmented datasets. The library assists in creating training data, training models using parameter-efficient finetuning (PEFT), and evaluating performance using RAG-specific metrics. It is modular, customizable via configuration files, and facilitates fast prototyping and experimentation with various RAG settings and configurations.
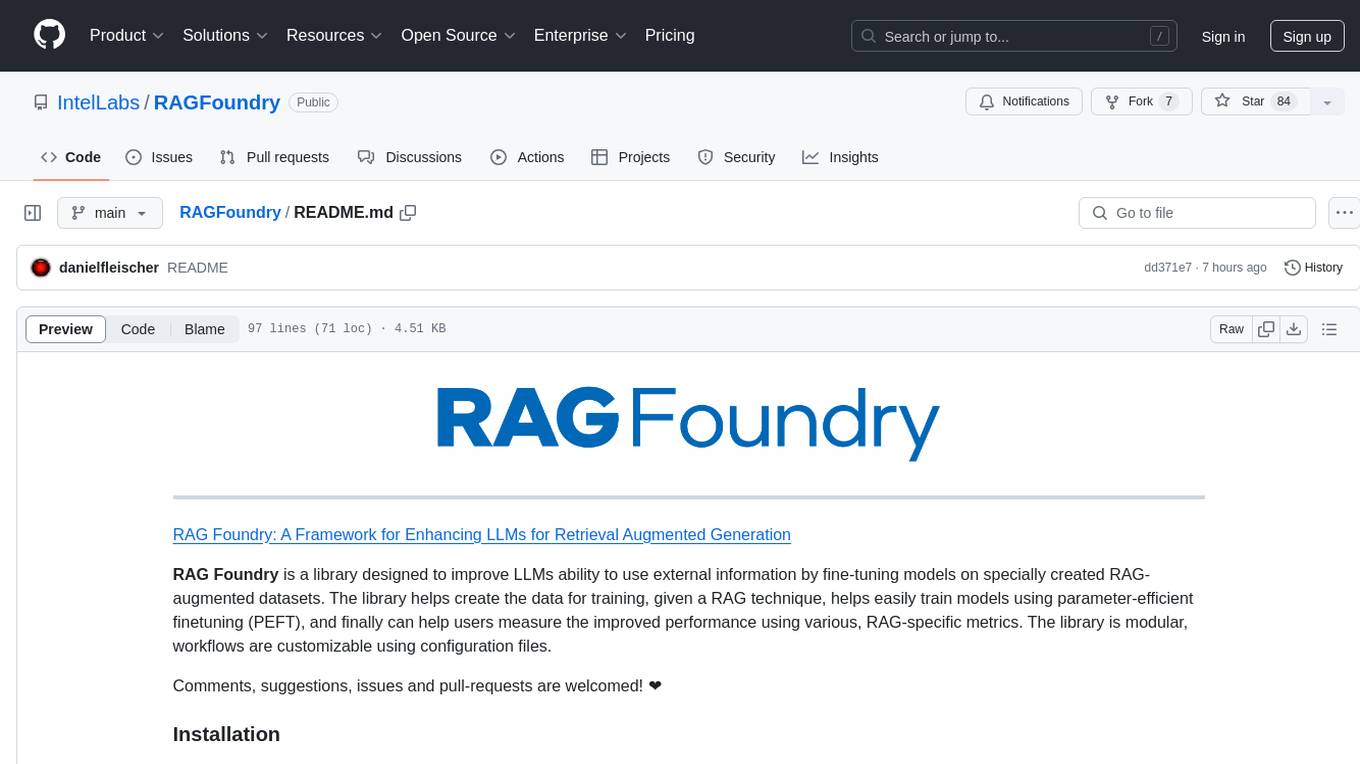
RAGFoundry
RAG Foundry is a library designed to enhance Large Language Models (LLMs) by fine-tuning models on RAG-augmented datasets. It helps create training data, train models using parameter-efficient finetuning (PEFT), and measure performance using RAG-specific metrics. The library is modular, customizable using configuration files, and facilitates prototyping with various RAG settings and configurations for tasks like data processing, retrieval, training, inference, and evaluation.
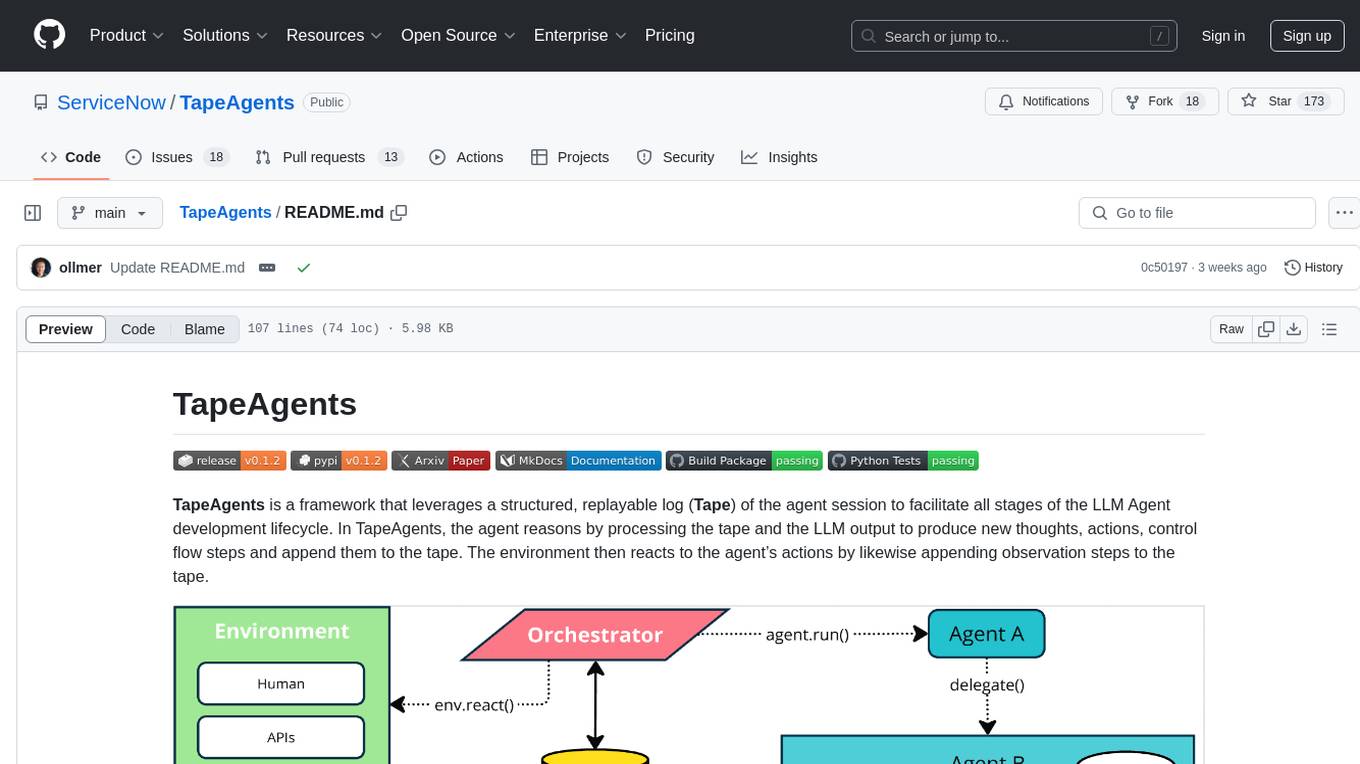
TapeAgents
TapeAgents is a framework that leverages a structured, replayable log of the agent session to facilitate all stages of the LLM Agent development lifecycle. The agent reasons by processing the tape and the LLM output to produce new thoughts, actions, control flow steps, and append them to the tape. Key features include building agents as low-level state machines or high-level multi-agent team configurations, debugging agents with TapeAgent studio or TapeBrowser apps, serving agents with response streaming, and optimizing agent configurations using successful tapes. The Tape-centric design of TapeAgents provides ultimate flexibility in project development, allowing access to tapes for making prompts, generating next steps, and controlling agent behavior.
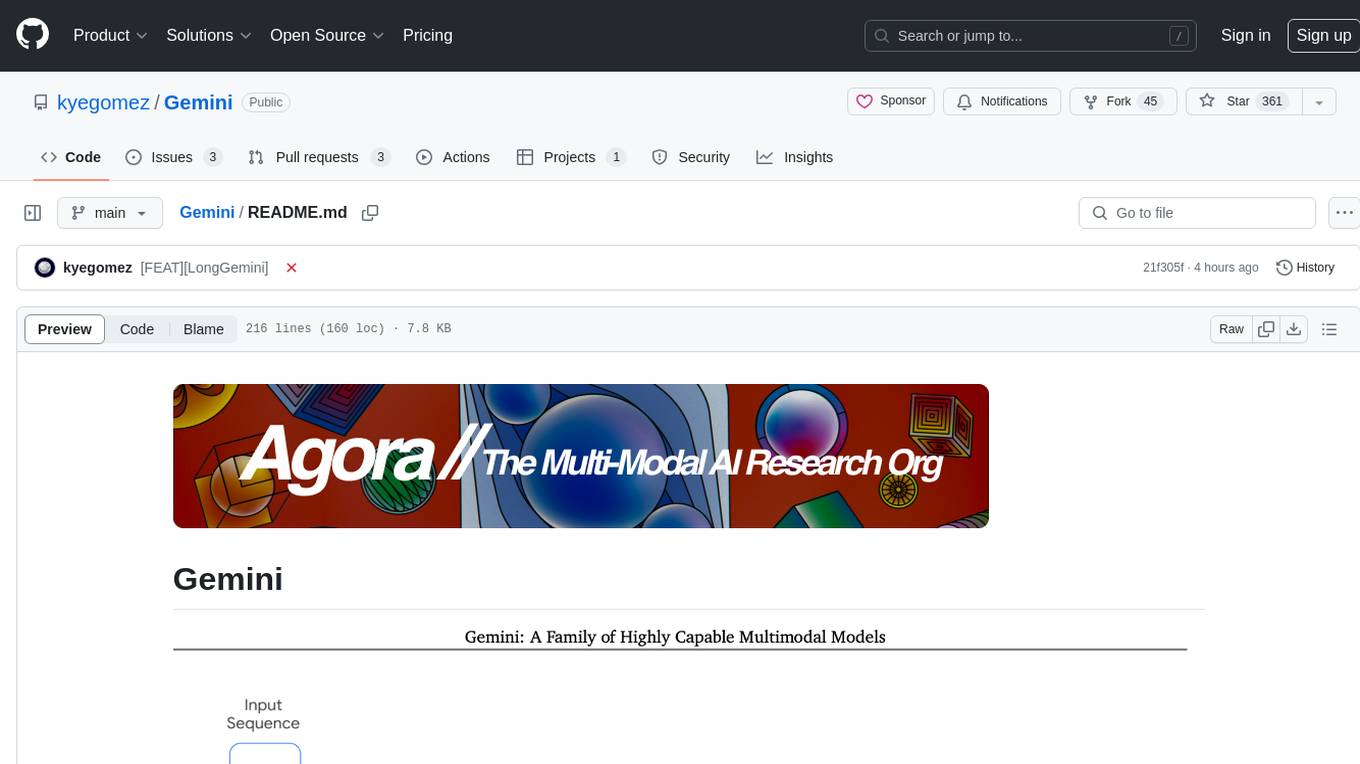
Gemini
Gemini is an open-source model designed to handle multiple modalities such as text, audio, images, and videos. It utilizes a transformer architecture with special decoders for text and image generation. The model processes input sequences by transforming them into tokens and then decoding them to generate image outputs. Gemini differs from other models by directly feeding image embeddings into the transformer instead of using a visual transformer encoder. The model also includes a component called Codi for conditional generation. Gemini aims to effectively integrate image, audio, and video embeddings to enhance its performance.
For similar tasks
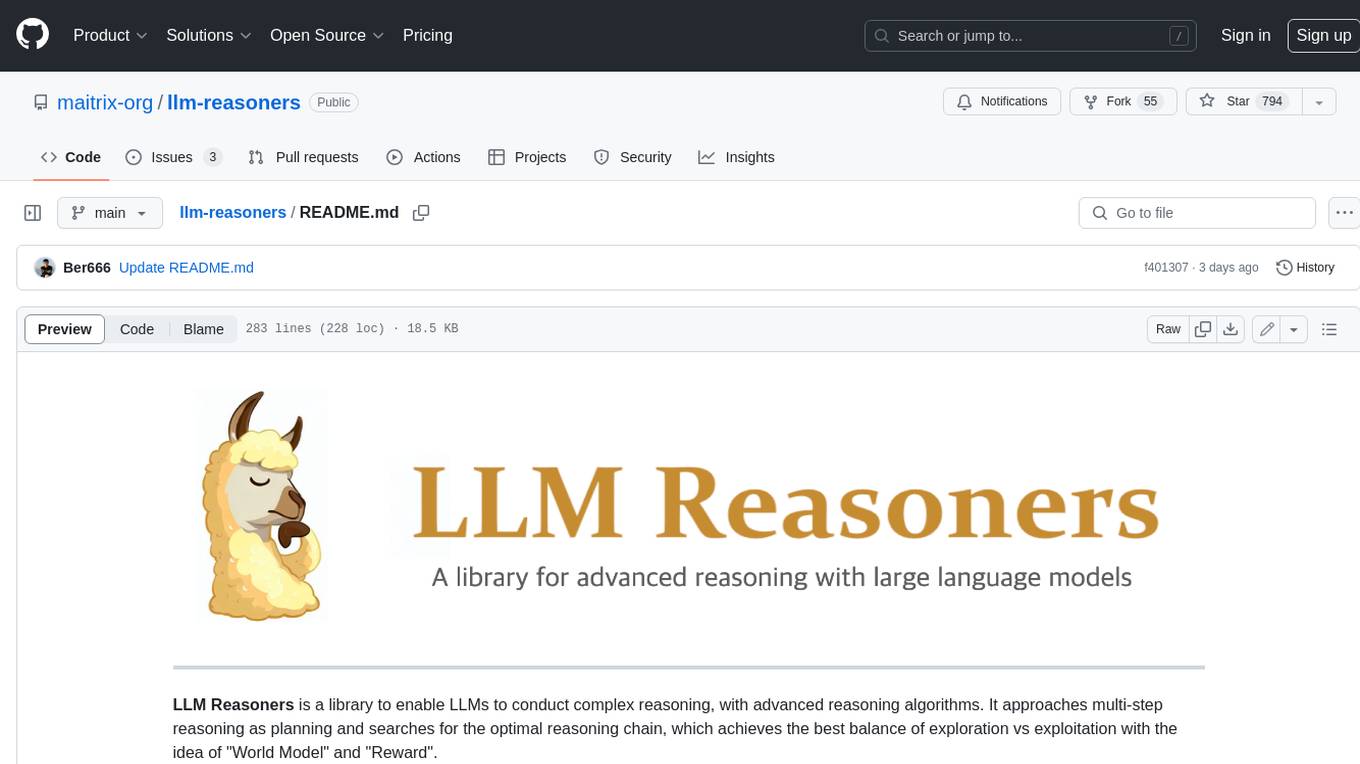
llm-reasoners
LLM Reasoners is a library that enables LLMs to conduct complex reasoning, with advanced reasoning algorithms. It approaches multi-step reasoning as planning and searches for the optimal reasoning chain, which achieves the best balance of exploration vs exploitation with the idea of "World Model" and "Reward". Given any reasoning problem, simply define the reward function and an optional world model (explained below), and let LLM reasoners take care of the rest, including Reasoning Algorithms, Visualization, LLM calling, and more!
For similar jobs
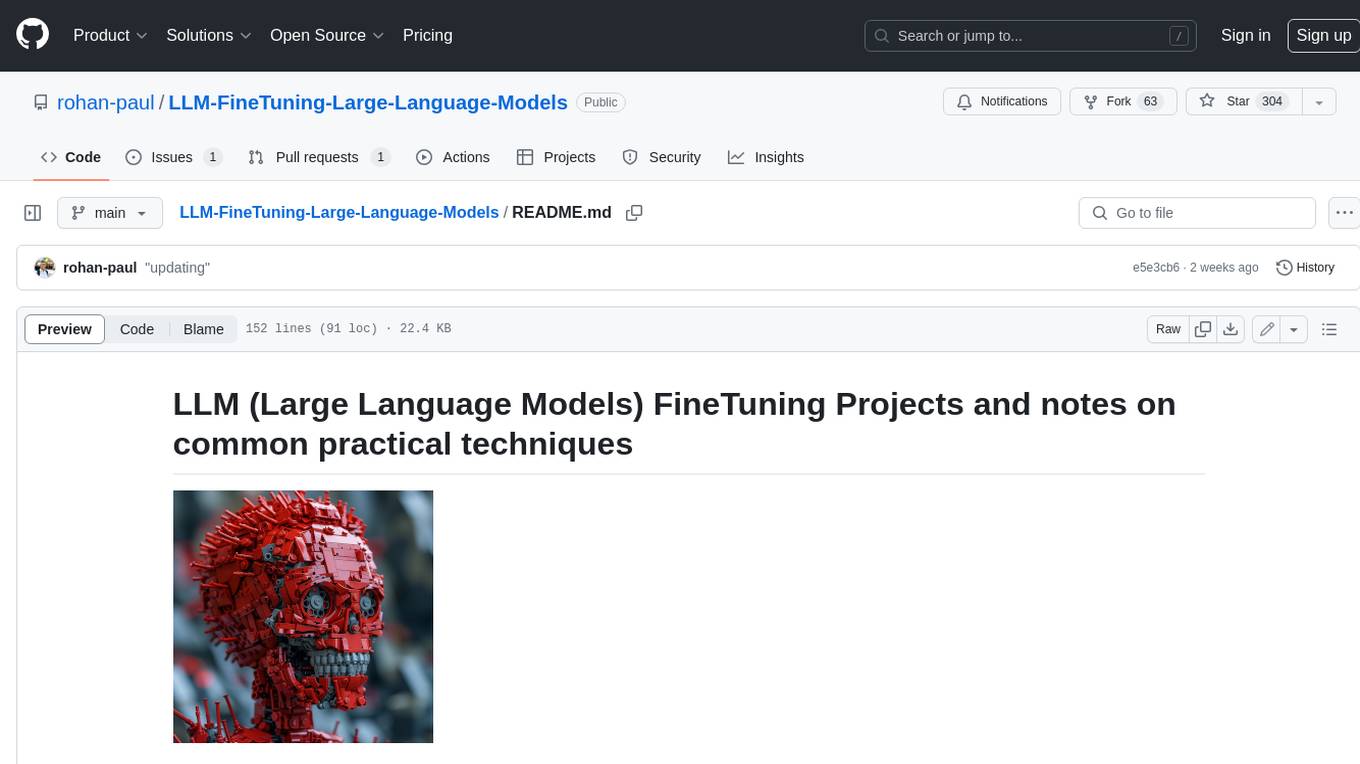
LLM-FineTuning-Large-Language-Models
This repository contains projects and notes on common practical techniques for fine-tuning Large Language Models (LLMs). It includes fine-tuning LLM notebooks, Colab links, LLM techniques and utils, and other smaller language models. The repository also provides links to YouTube videos explaining the concepts and techniques discussed in the notebooks.
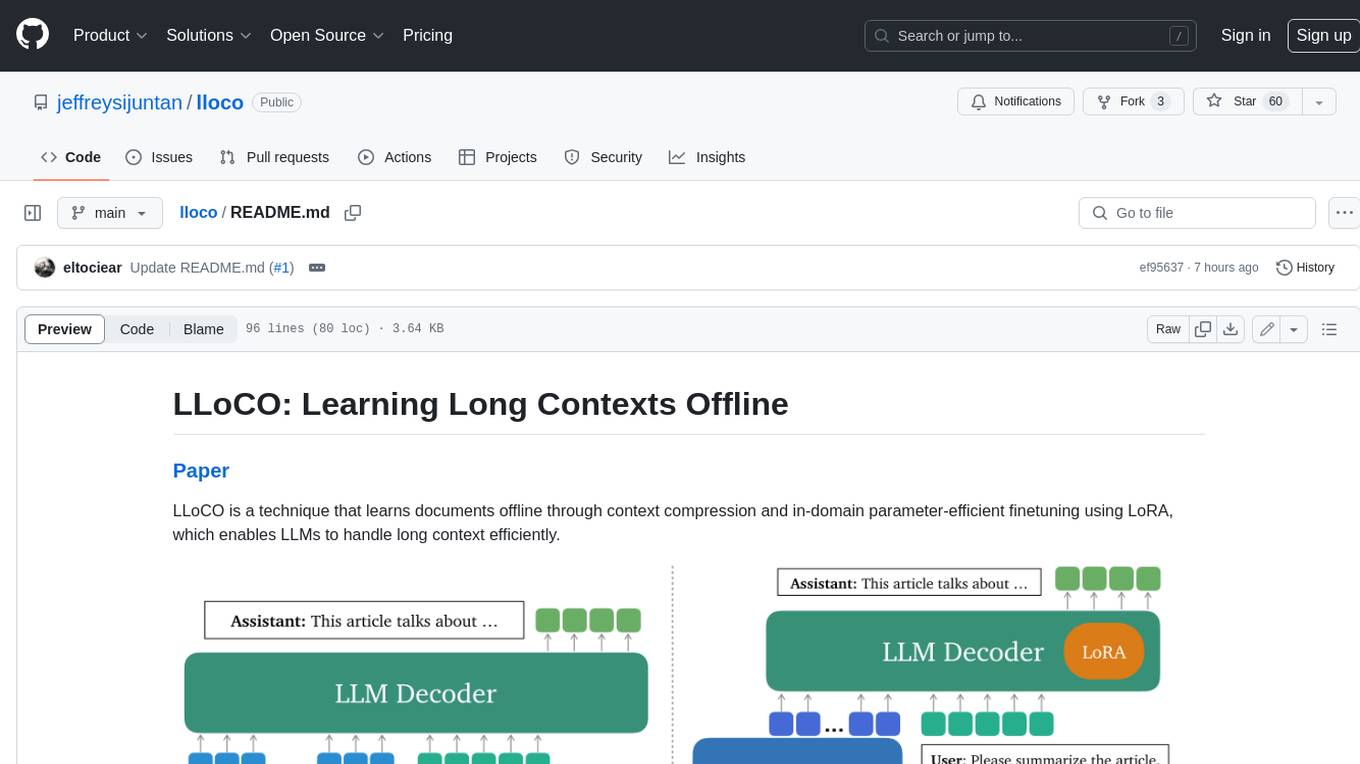
lloco
LLoCO is a technique that learns documents offline through context compression and in-domain parameter-efficient finetuning using LoRA, which enables LLMs to handle long context efficiently.
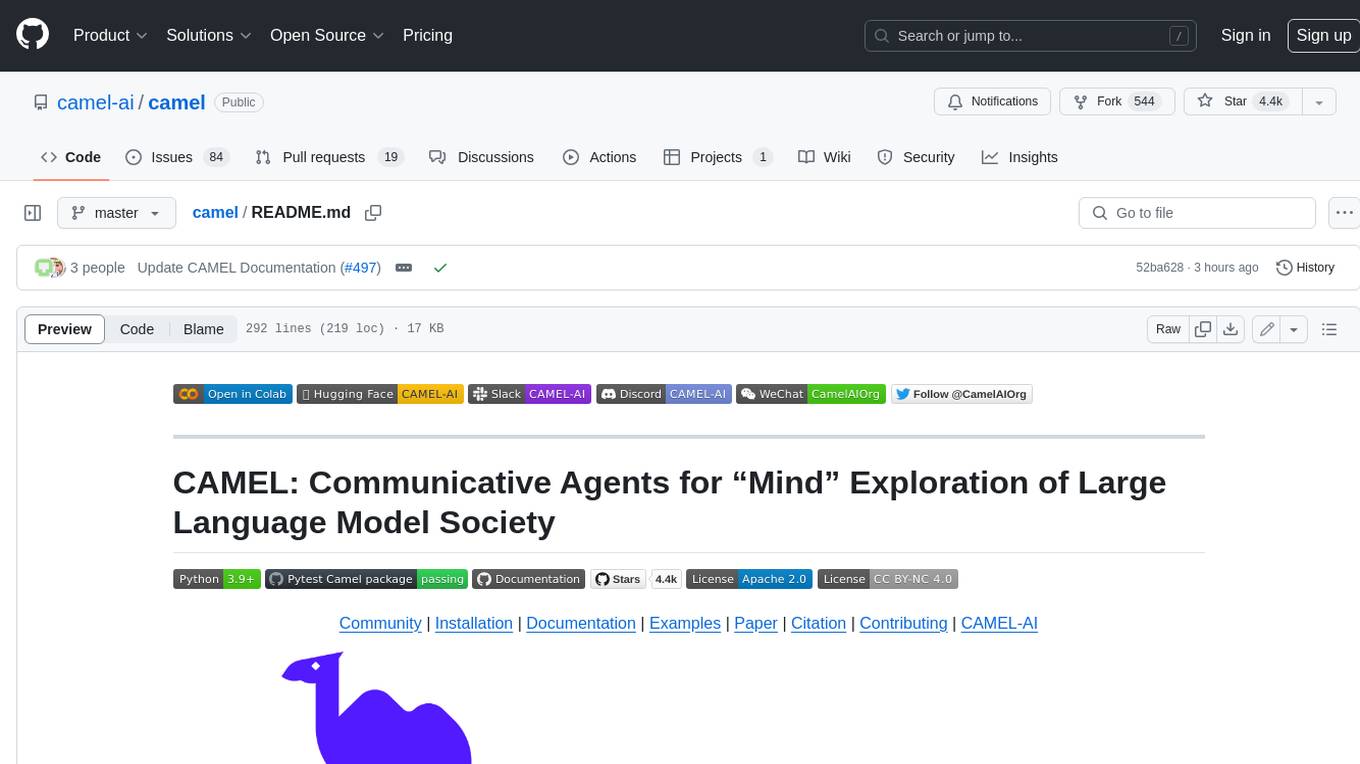
camel
CAMEL is an open-source library designed for the study of autonomous and communicative agents. We believe that studying these agents on a large scale offers valuable insights into their behaviors, capabilities, and potential risks. To facilitate research in this field, we implement and support various types of agents, tasks, prompts, models, and simulated environments.
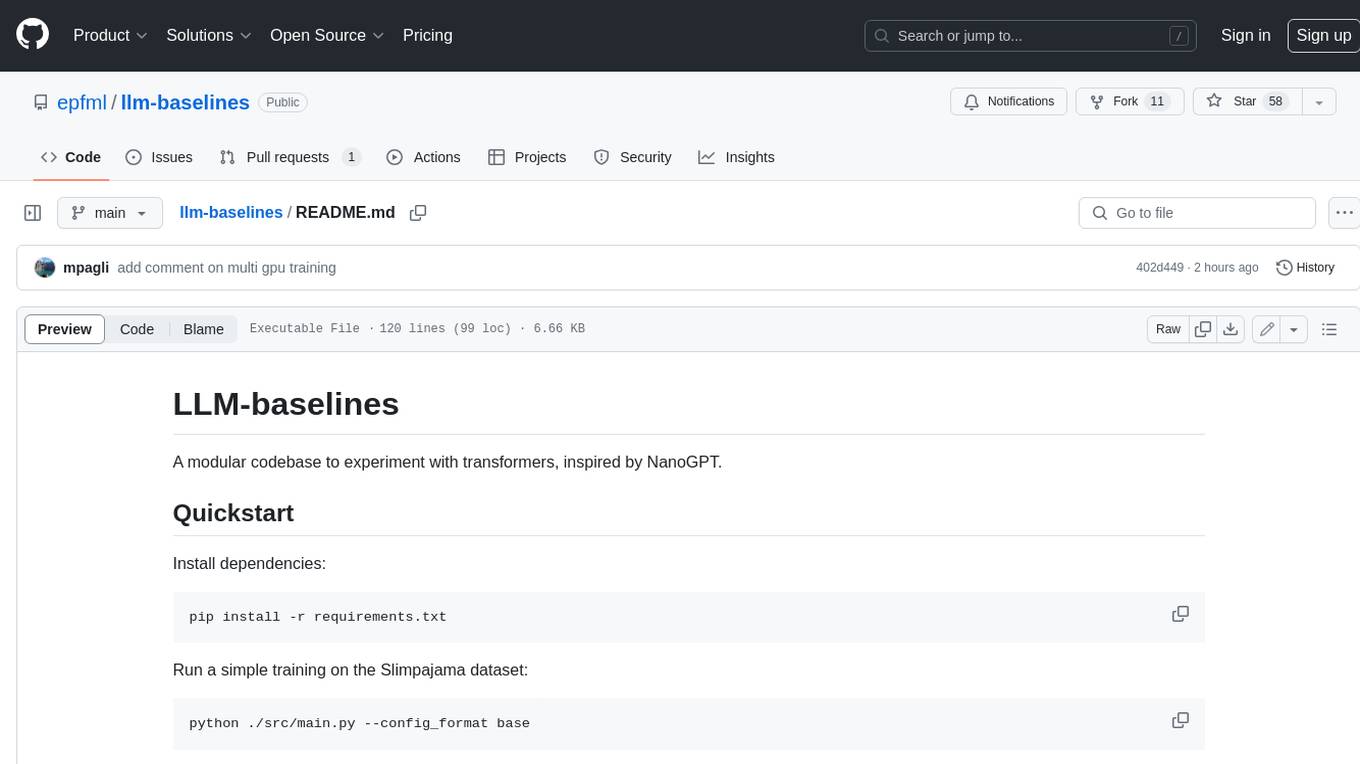
llm-baselines
LLM-baselines is a modular codebase to experiment with transformers, inspired from NanoGPT. It provides a quick and easy way to train and evaluate transformer models on a variety of datasets. The codebase is well-documented and easy to use, making it a great resource for researchers and practitioners alike.
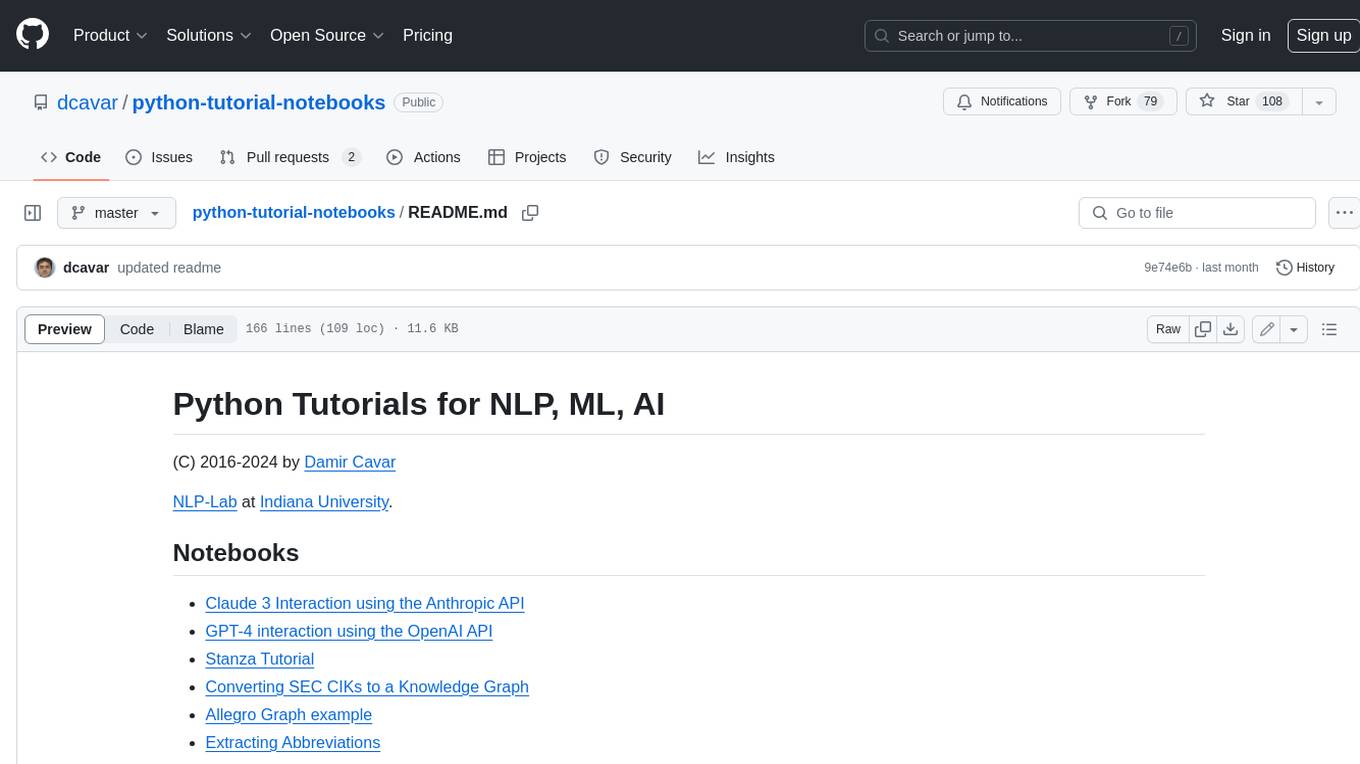
python-tutorial-notebooks
This repository contains Jupyter-based tutorials for NLP, ML, AI in Python for classes in Computational Linguistics, Natural Language Processing (NLP), Machine Learning (ML), and Artificial Intelligence (AI) at Indiana University.
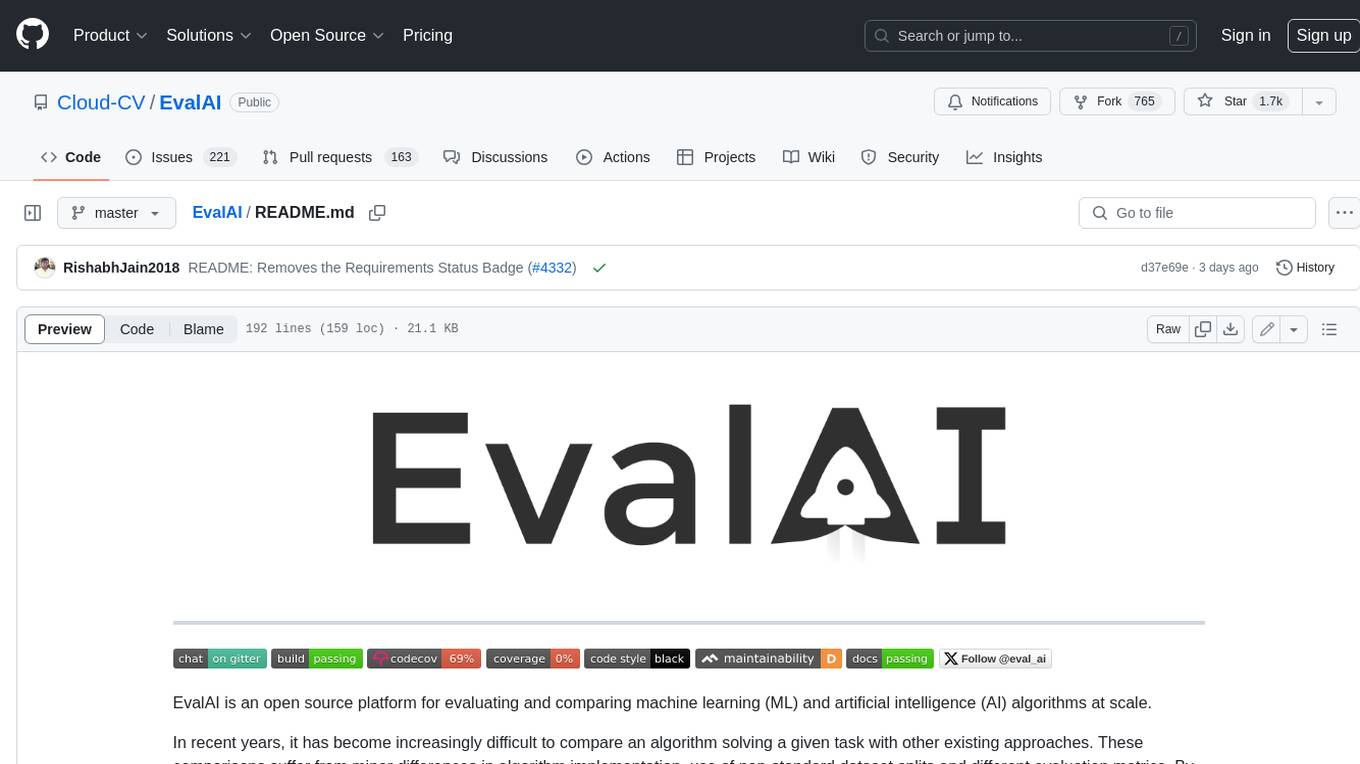
EvalAI
EvalAI is an open-source platform for evaluating and comparing machine learning (ML) and artificial intelligence (AI) algorithms at scale. It provides a central leaderboard and submission interface, making it easier for researchers to reproduce results mentioned in papers and perform reliable & accurate quantitative analysis. EvalAI also offers features such as custom evaluation protocols and phases, remote evaluation, evaluation inside environments, CLI support, portability, and faster evaluation.
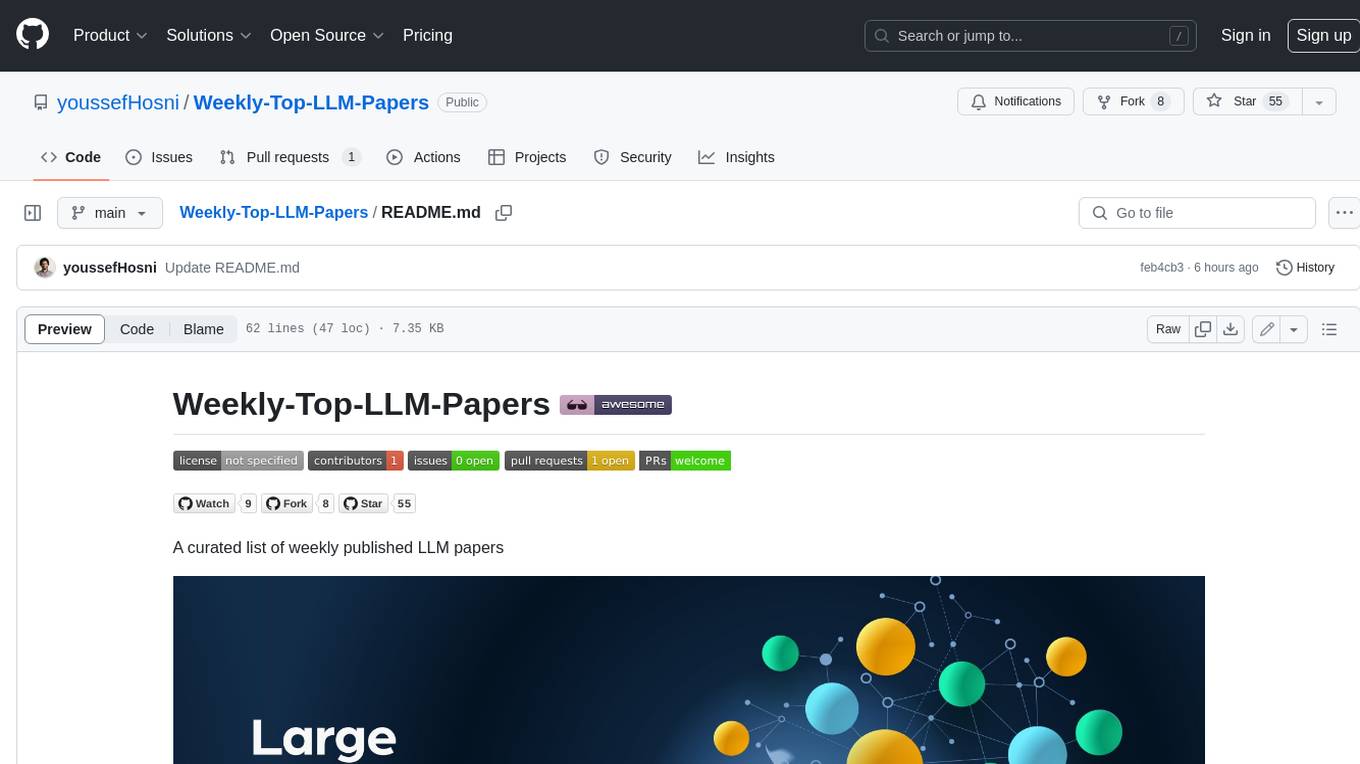
Weekly-Top-LLM-Papers
This repository provides a curated list of weekly published Large Language Model (LLM) papers. It includes top important LLM papers for each week, organized by month and year. The papers are categorized into different time periods, making it easy to find the most recent and relevant research in the field of LLM.
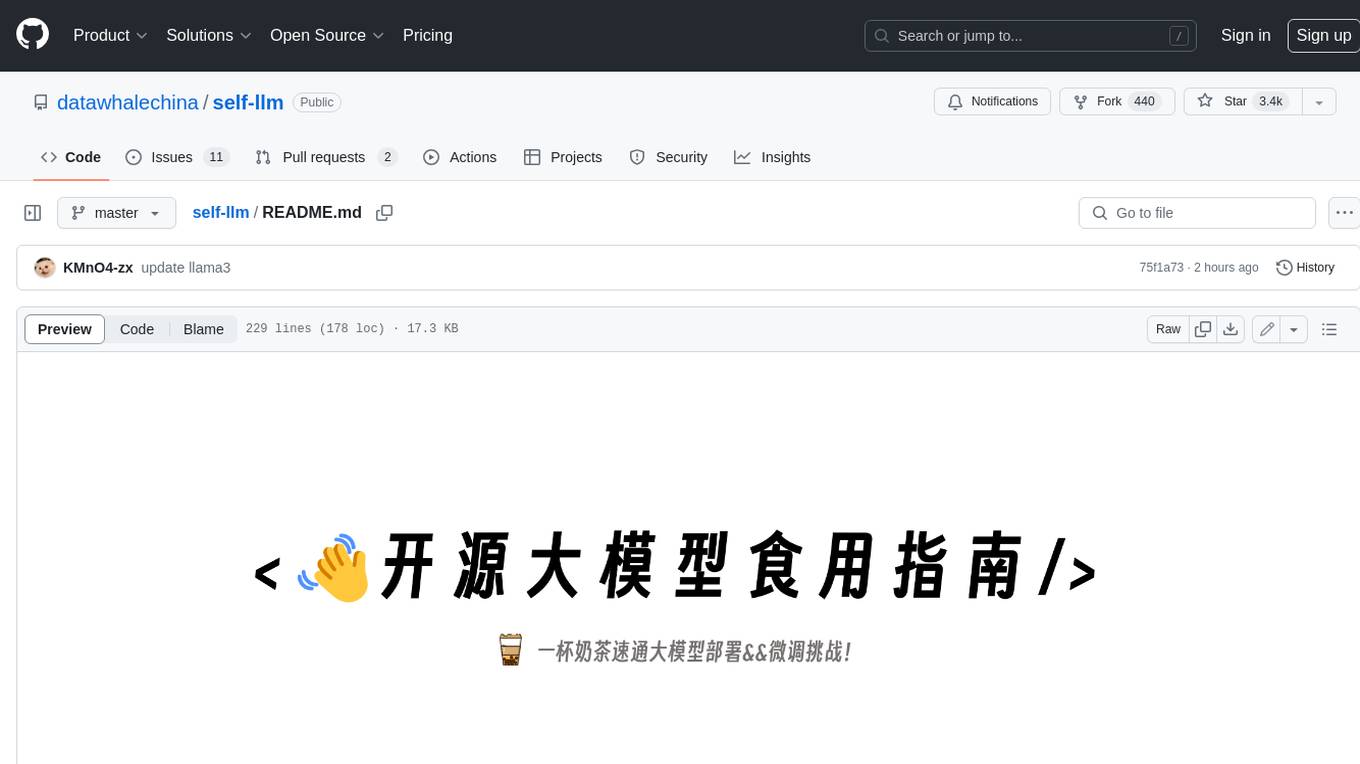
self-llm
This project is a Chinese tutorial for domestic beginners based on the AutoDL platform, providing full-process guidance for various open-source large models, including environment configuration, local deployment, and efficient fine-tuning. It simplifies the deployment, use, and application process of open-source large models, enabling more ordinary students and researchers to better use open-source large models and helping open and free large models integrate into the lives of ordinary learners faster.