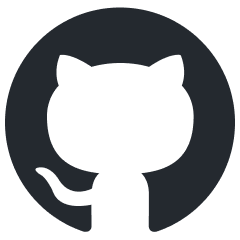
lloco
The official repo for "LLoCo: Learning Long Contexts Offline"
Stars: 60
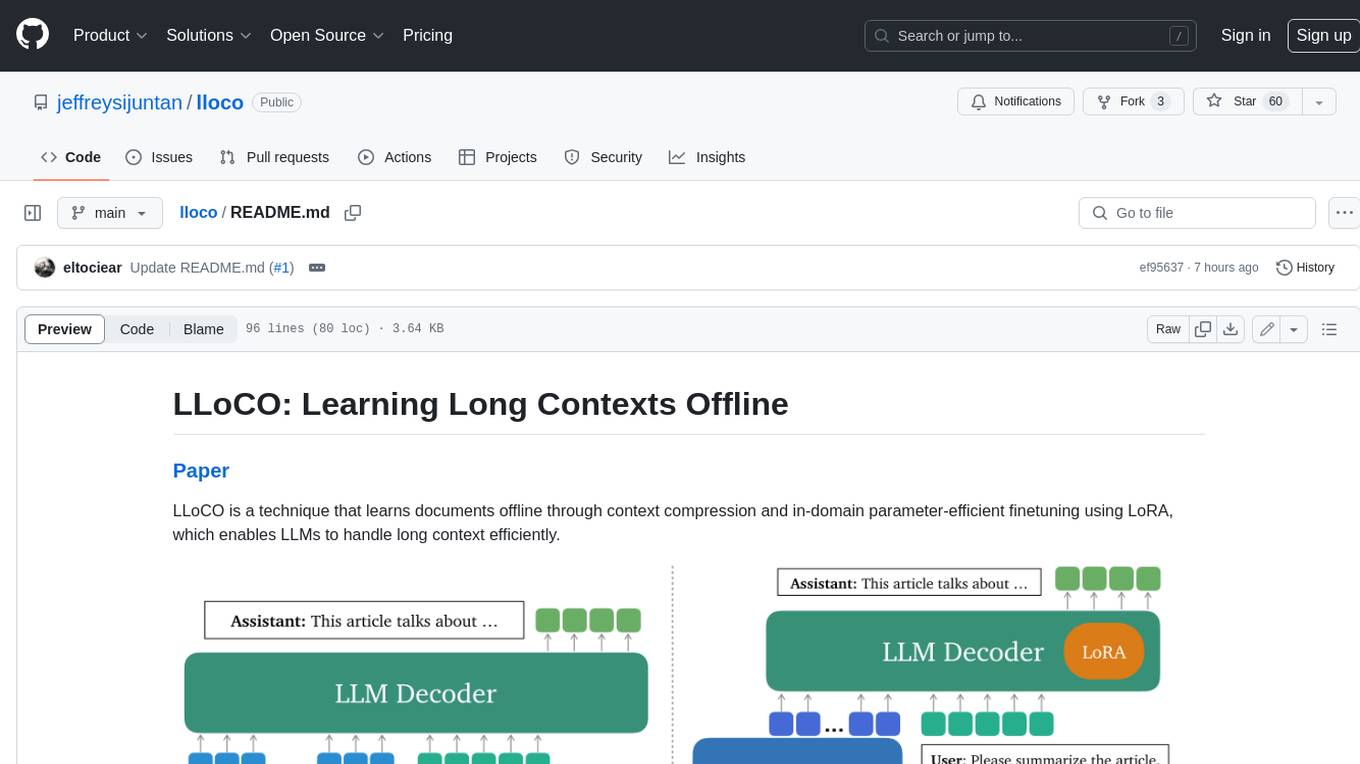
LLoCO is a technique that learns documents offline through context compression and in-domain parameter-efficient finetuning using LoRA, which enables LLMs to handle long context efficiently.
README:
LLoCO is a technique that learns documents offline through context compression and in-domain parameter-efficient finetuning using LoRA, which enables LLMs to handle long context efficiently.
Setup a new environment and run:
pip install -r requirements.txt
Use the following command to download the QuALITY dataset. Other datasets are loaded from HuggingFace and can be downloaded automatically during data loading.
cd data
wget https://raw.githubusercontent.com/nyu-mll/quality/main/data/v1.0.1/QuALITY.v1.0.1.htmlstripped.train
wget https://raw.githubusercontent.com/nyu-mll/quality/main/data/v1.0.1/QuALITY.v1.0.1.htmlstripped.dev
First generate summary embeddings for the datasets. An example bash script is stored in scripts/preproc_emb.sh
, which preprocess the training dataset of QuALITY:
python3 preproc_embs.py \
--emb_model_name "autocomp" \
--dataset quality \
--split train \
--data_path ./data/QuALITY.v1.0.1.htmlstripped.train \
--out_path ./embeddings/quality_train_embs.pth \
--truncation False \
This script will generate summary embeddings for QuALITY training set, and store the embeddings in the /embeddings
folder. Embedding generation for other datasets works similarly.
Here is an example bash script to finetune the QuALITY dataset. This script is in scripts/finetune_quality.sh
.
torchrun --nproc_per_node=4 finetune_quality.py \
--output_dir output/lloco_quality \
--run_name lloco_quality \
--data_path ./data/QuALITY.v1.0.1.htmlstripped.train \
--embedding_path ./embeddings/quality_train_embs.pth \
...
Below is a bash script to run inference over the validation sets are contained in script/inference.sh
. Evaluation results are stored in out_path
, and the finetuned model is specified by peft_model
.
python3 inference.py \
--model_name_or_path meta-llama/Llama-2-7b-chat-hf \
--dataset_name qmsum \
--eval_mode autocomp \
--out_path ./eval/qmsum_lloco.json \
--peft_model output/lloco_qmsum \
--embedding_path ./embeddings/qmsum_val_embs.pth \
...
After obtaining the prediction files, use the following evaluation scripts in the /eval folder to get the scores for each dataset.
Evaluate QuALITY:
python3 quality_evaluator.py --quality_path {quality_path} --pred_path {prediction_file}
Evaluate QMSum, Qasper, NarrativeQA:
python3 scroll_evaluator.py --split validation --dataset_name {dataset_name} --predictions {prediction_file} --metrics_output_dir .
Evaluate HotpotQA:
python3 hotpot_evaluator.py --pred_path {prediction_fild}
- [x] Release finetuning and inference code.
- [ ] Release pre-trained LoRA weights on HuggingFace.
- [ ] Integrate to VLLM.
If you find LLoCO useful or relevant to your project and research, please kindly cite our paper:
@article{tan2024lloco,
title = {LLoCO: Learning Long Contexts Offline},
author = {Sijun Tan and Xiuyu Li and Shishir Patil and Ziyang Wu and Tianjun Zhang and Kurt Keutzer and Joseph E. Gonzalez and Raluca Ada Popa},
year = {2024},
journal = {arXiv preprint arXiv: 2404.07979}
}
We referred to AutoCompressors for the context encoder implementation.
For Tasks:
Click tags to check more tools for each tasksFor Jobs:
Alternative AI tools for lloco
Similar Open Source Tools
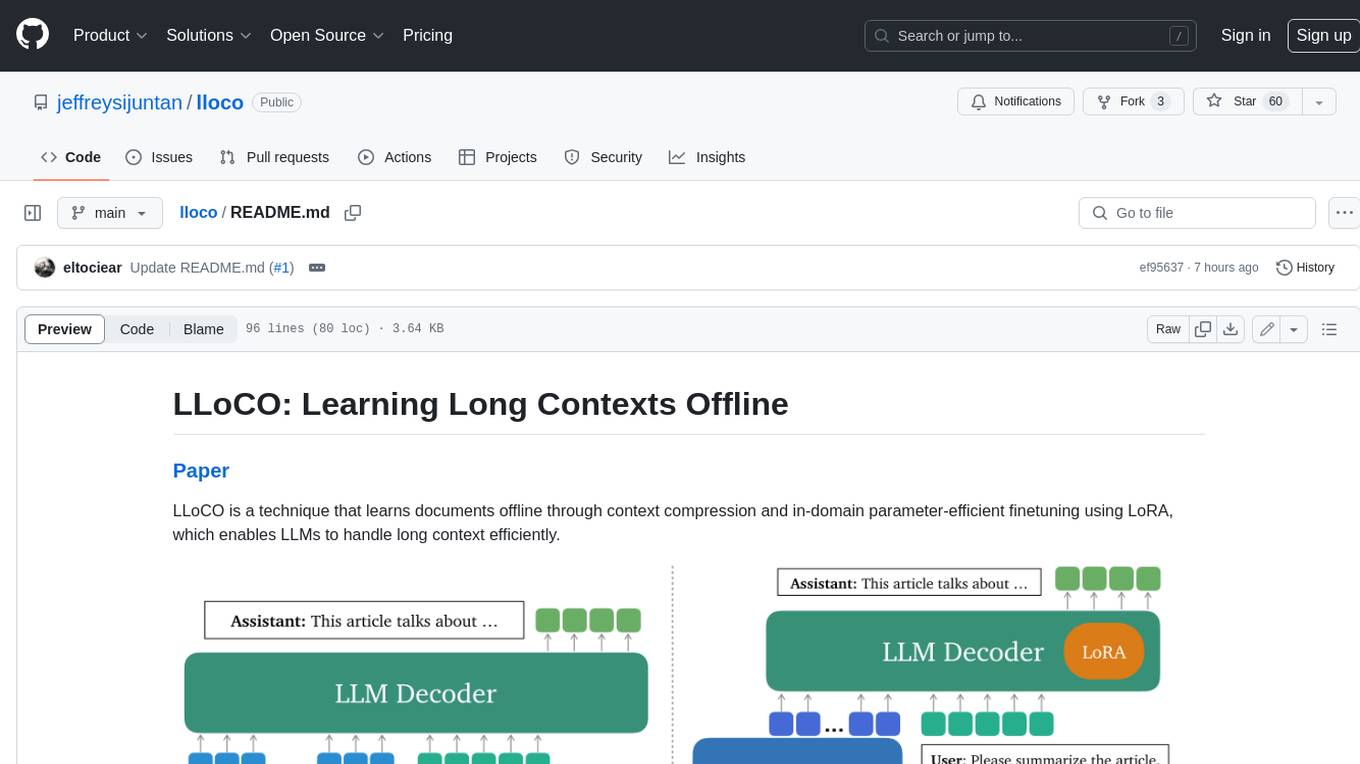
lloco
LLoCO is a technique that learns documents offline through context compression and in-domain parameter-efficient finetuning using LoRA, which enables LLMs to handle long context efficiently.
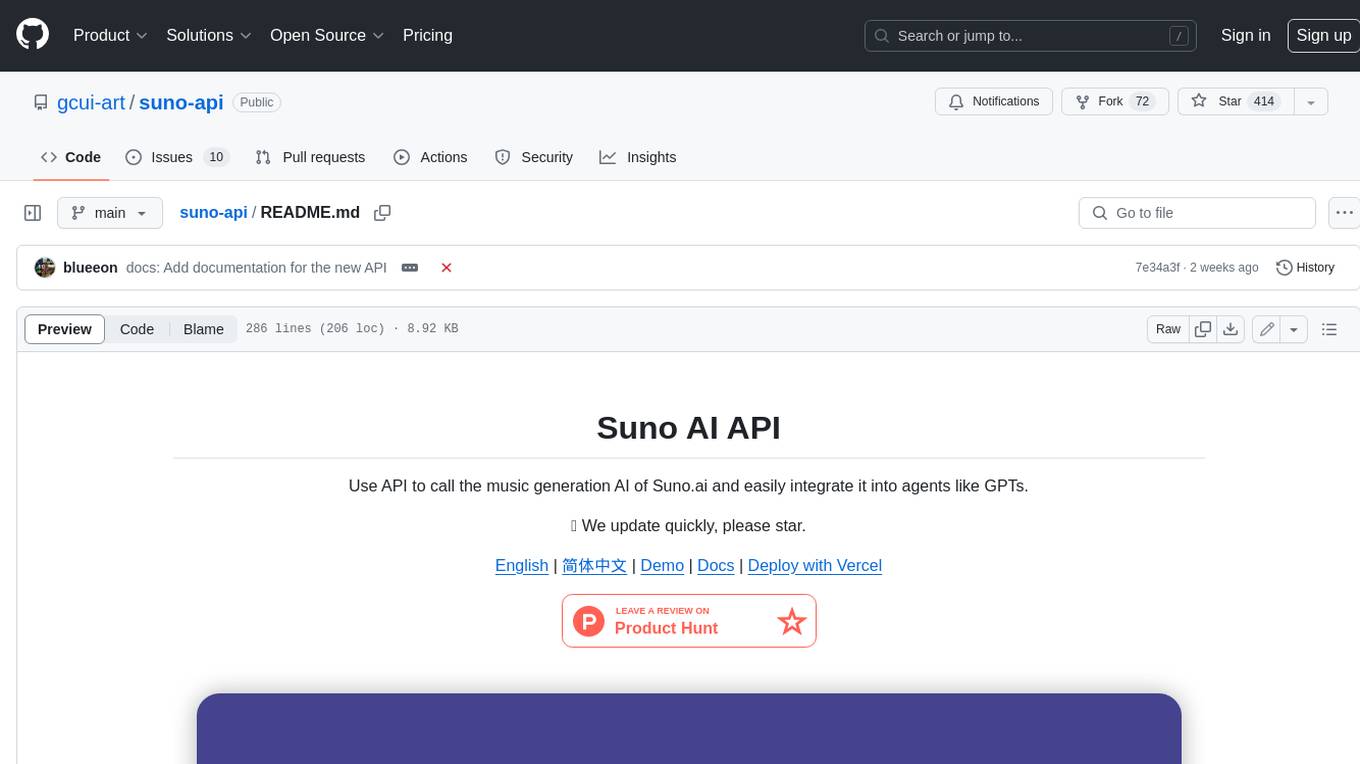
suno-api
Suno AI API is an open-source project that allows developers to integrate the music generation capabilities of Suno.ai into their own applications. The API provides a simple and convenient way to generate music, lyrics, and other audio content using Suno.ai's powerful AI models. With Suno AI API, developers can easily add music generation functionality to their apps, websites, and other projects.
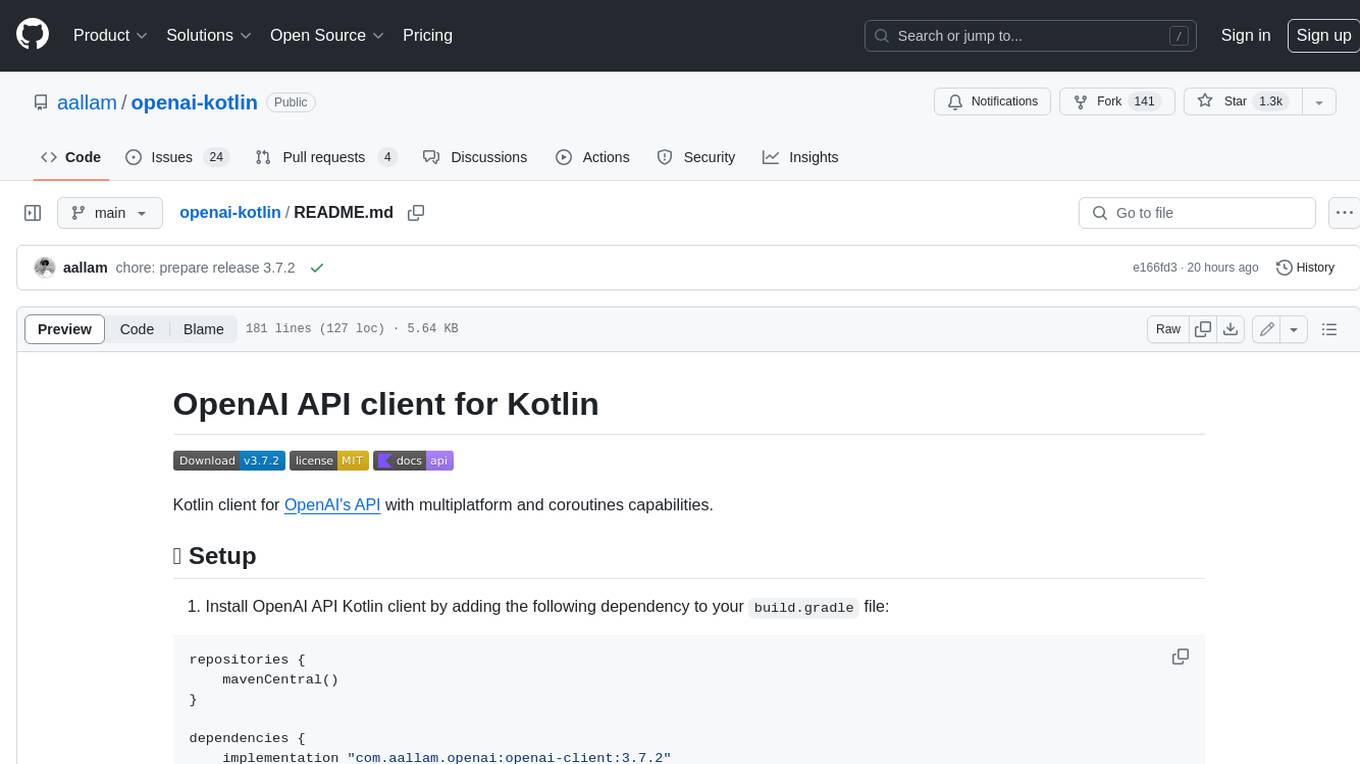
openai-kotlin
OpenAI Kotlin API client is a Kotlin client for OpenAI's API with multiplatform and coroutines capabilities. It allows users to interact with OpenAI's API using Kotlin programming language. The client supports various features such as models, chat, images, embeddings, files, fine-tuning, moderations, audio, assistants, threads, messages, and runs. It also provides guides on getting started, chat & function call, file source guide, and assistants. Sample apps are available for reference, and troubleshooting guides are provided for common issues. The project is open-source and licensed under the MIT license, allowing contributions from the community.
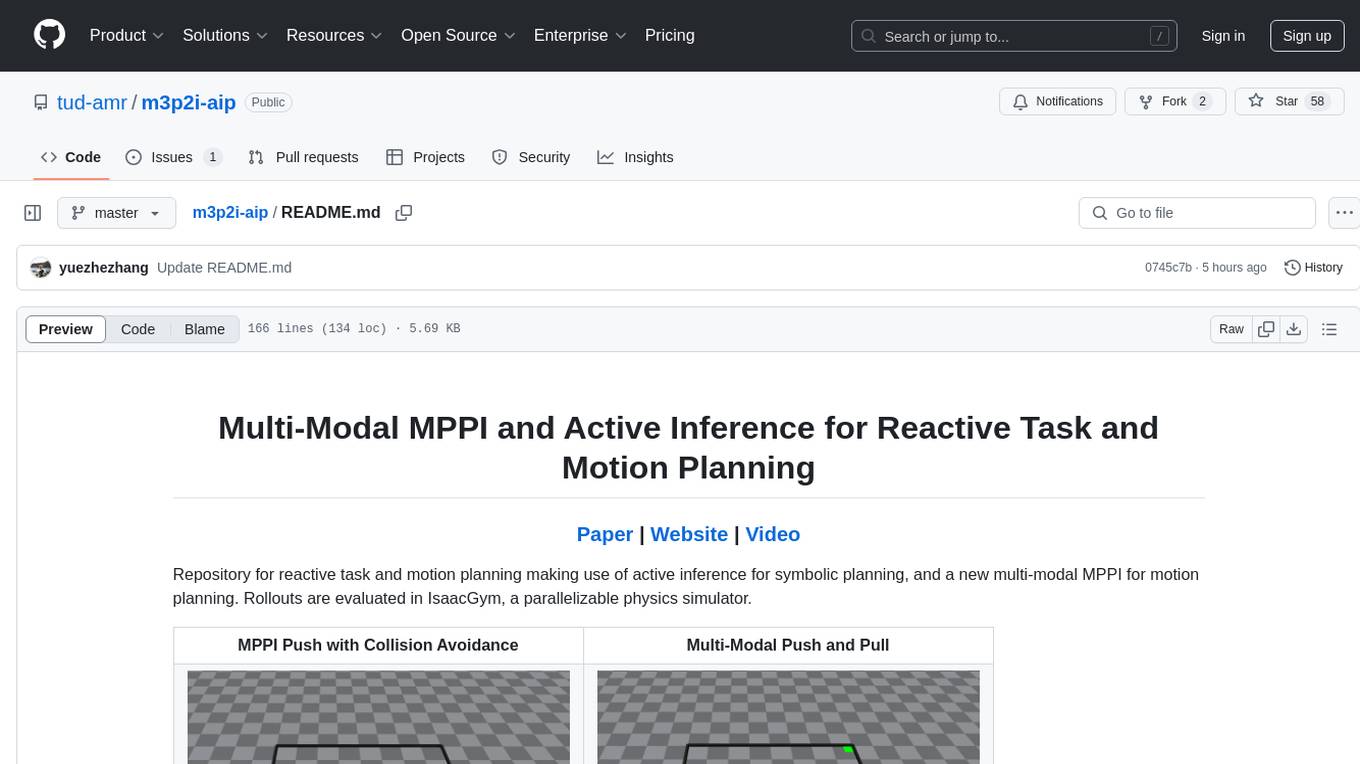
m3p2i-aip
Repository for reactive task and motion planning using active inference for symbolic planning and multi-modal MPPI for motion planning. Rollouts are evaluated in IsaacGym, a parallelizable physics simulator. The tool provides functionalities for push, pull, pick, and multi-modal push-pull tasks with collision avoidance.
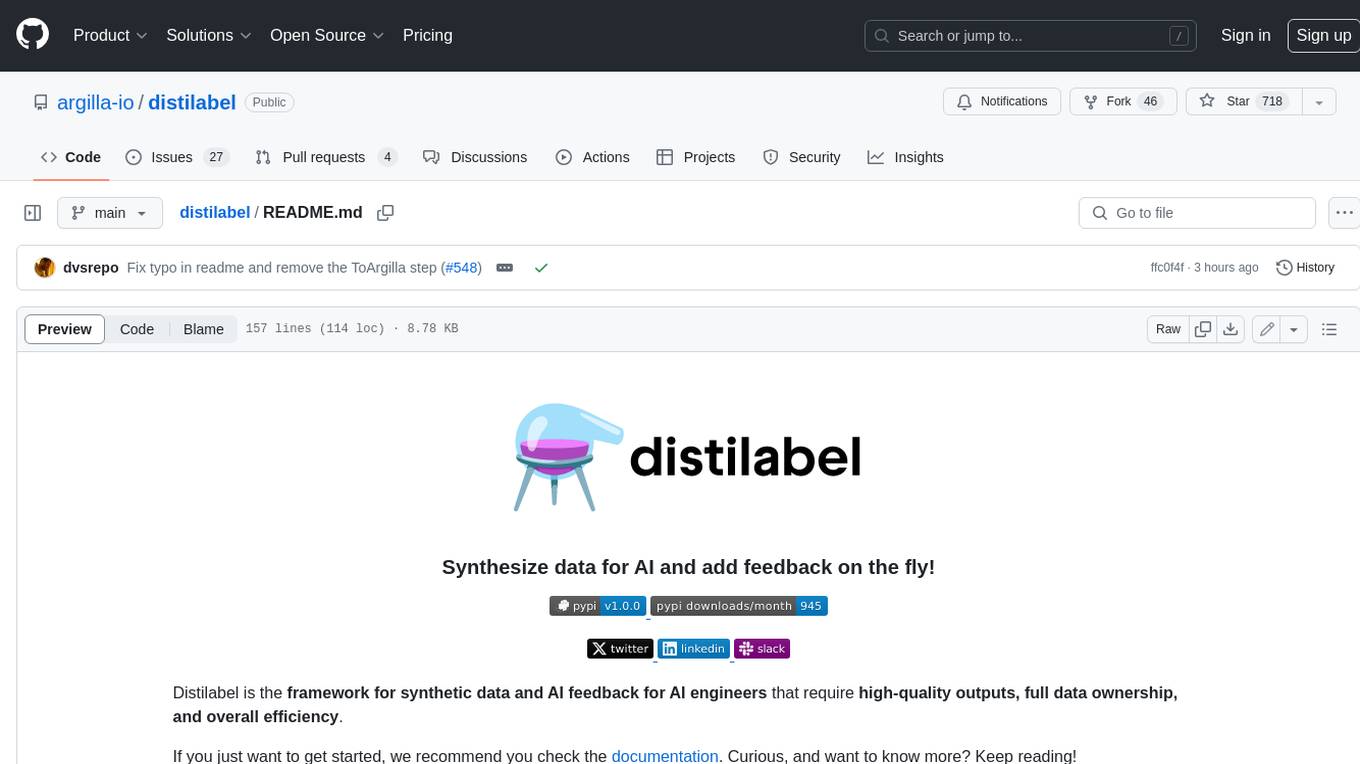
distilabel
Distilabel is a framework for synthetic data and AI feedback for AI engineers that require high-quality outputs, full data ownership, and overall efficiency. It helps you synthesize data and provide AI feedback to improve the quality of your AI models. With Distilabel, you can: * **Synthesize data:** Generate synthetic data to train your AI models. This can help you to overcome the challenges of data scarcity and bias. * **Provide AI feedback:** Get feedback from AI models on your data. This can help you to identify errors and improve the quality of your data. * **Improve your AI output quality:** By using Distilabel to synthesize data and provide AI feedback, you can improve the quality of your AI models and get better results.
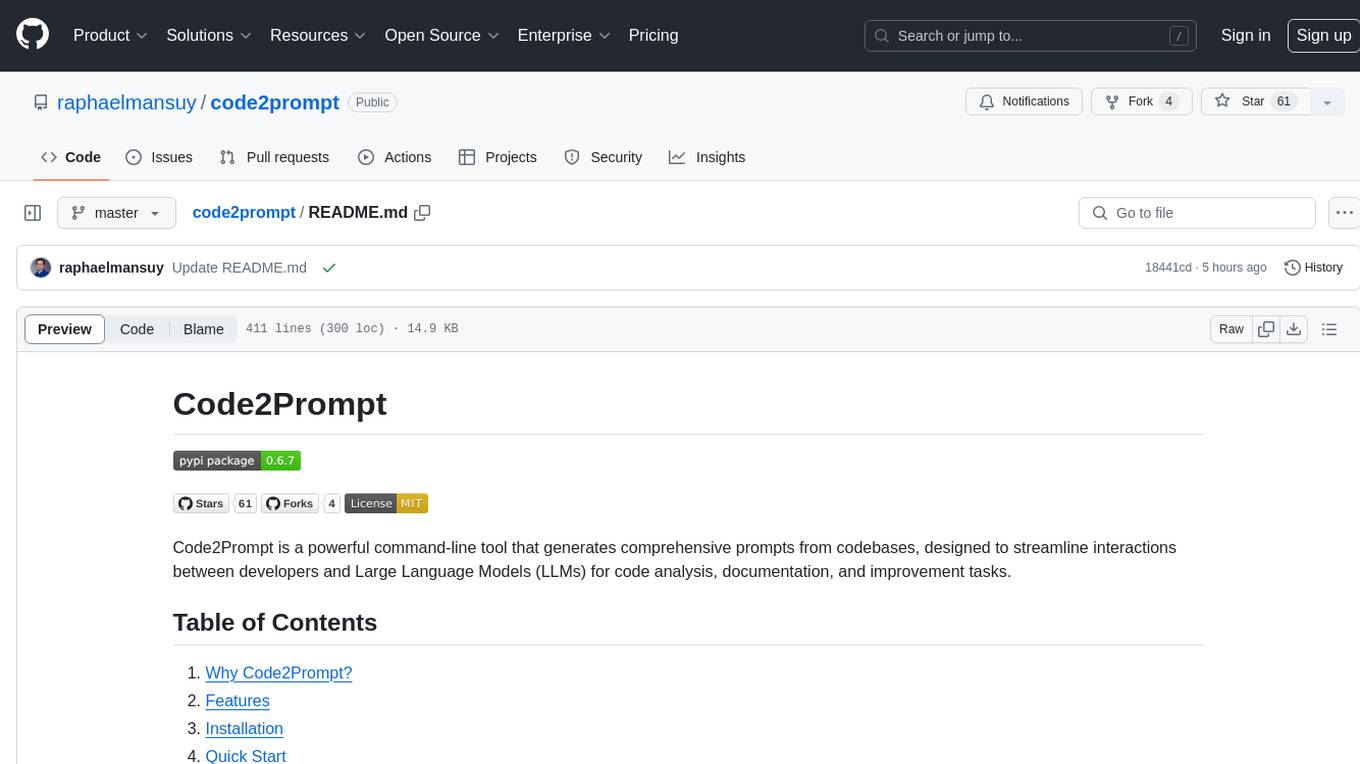
code2prompt
Code2Prompt is a powerful command-line tool that generates comprehensive prompts from codebases, designed to streamline interactions between developers and Large Language Models (LLMs) for code analysis, documentation, and improvement tasks. It bridges the gap between codebases and LLMs by converting projects into AI-friendly prompts, enabling users to leverage AI for various software development tasks. The tool offers features like holistic codebase representation, intelligent source tree generation, customizable prompt templates, smart token management, Gitignore integration, flexible file handling, clipboard-ready output, multiple output options, and enhanced code readability.
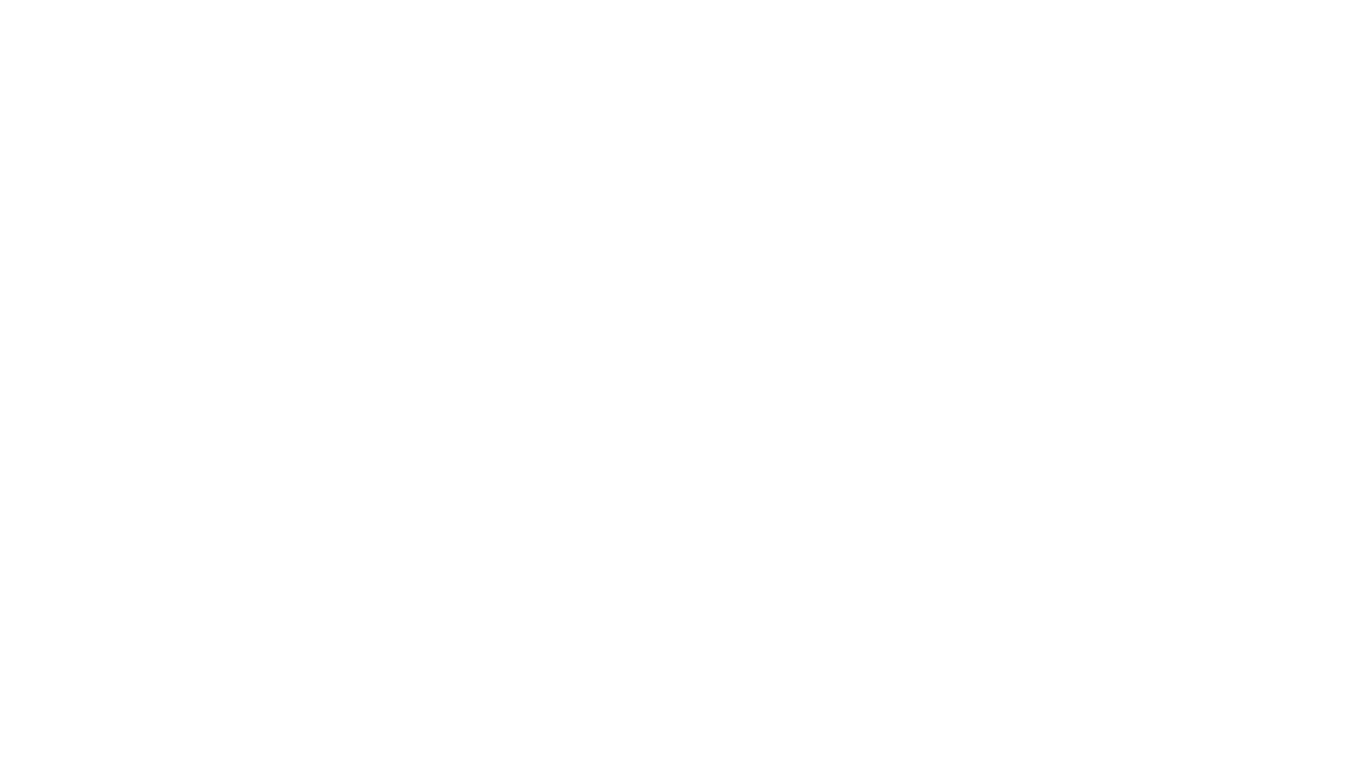
upgini
Upgini is an intelligent data search engine with a Python library that helps users find and add relevant features to their ML pipeline from various public, community, and premium external data sources. It automates the optimization of connected data sources by generating an optimal set of machine learning features using large language models, GraphNNs, and recurrent neural networks. The tool aims to simplify feature search and enrichment for external data to make it a standard approach in machine learning pipelines. It democratizes access to data sources for the data science community.
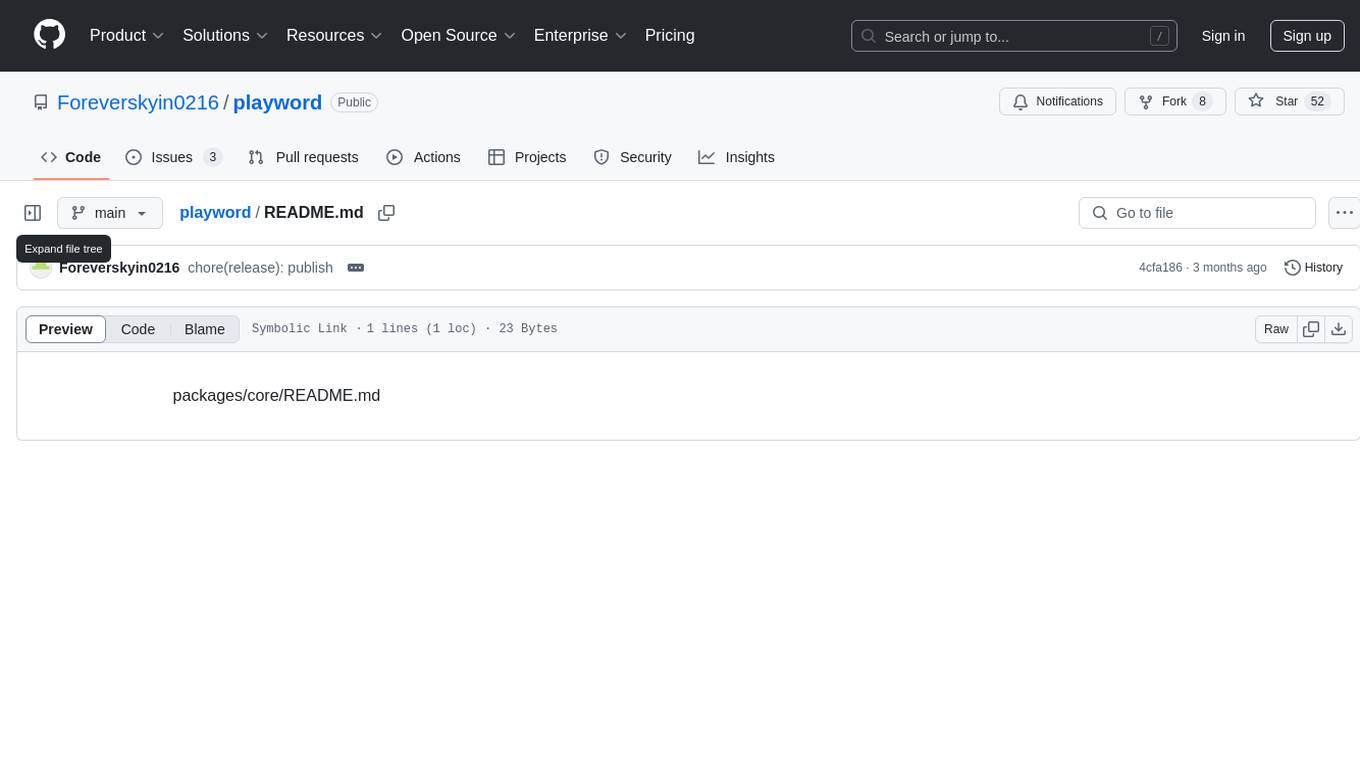
playword
PlayWord is a tool designed to supercharge web test automation experience with AI. It provides core features such as enabling browser operations and validations using natural language inputs, as well as monitoring interface to record and dry-run test steps. PlayWord supports multiple AI services including Anthropic, Google, and OpenAI, allowing users to select the appropriate provider based on their requirements. The tool also offers features like assertion handling, frame handling, custom variables, test recordings, and an Observer module to track user interactions on web pages. With PlayWord, users can interact with web pages using natural language commands, reducing the need to worry about element locators and providing AI-powered adaptation to UI changes.
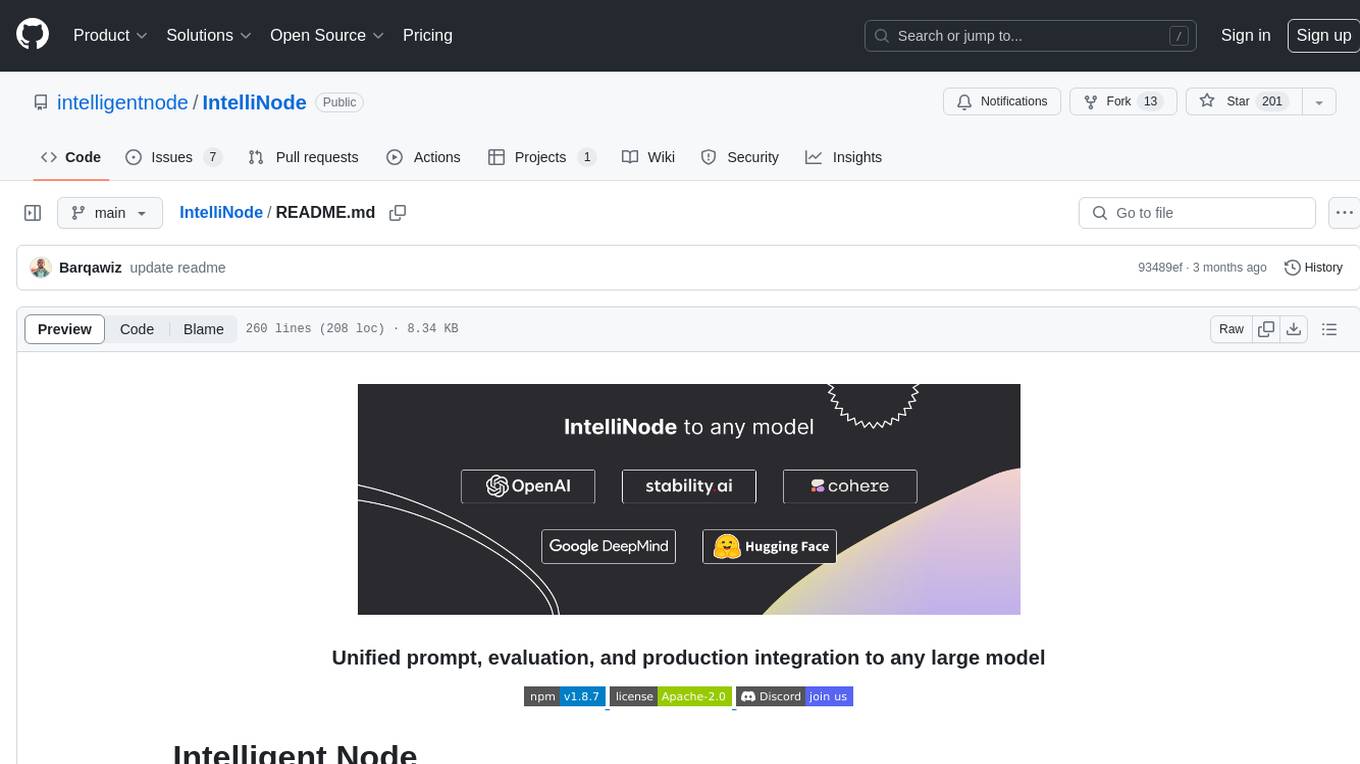
IntelliNode
IntelliNode is a javascript module that integrates cutting-edge AI models like ChatGPT, LLaMA, WaveNet, Gemini, and Stable diffusion into projects. It offers functions for generating text, speech, and images, as well as semantic search, multi-model evaluation, and chatbot capabilities. The module provides a wrapper layer for low-level model access, a controller layer for unified input handling, and a function layer for abstract functionality tailored to various use cases.
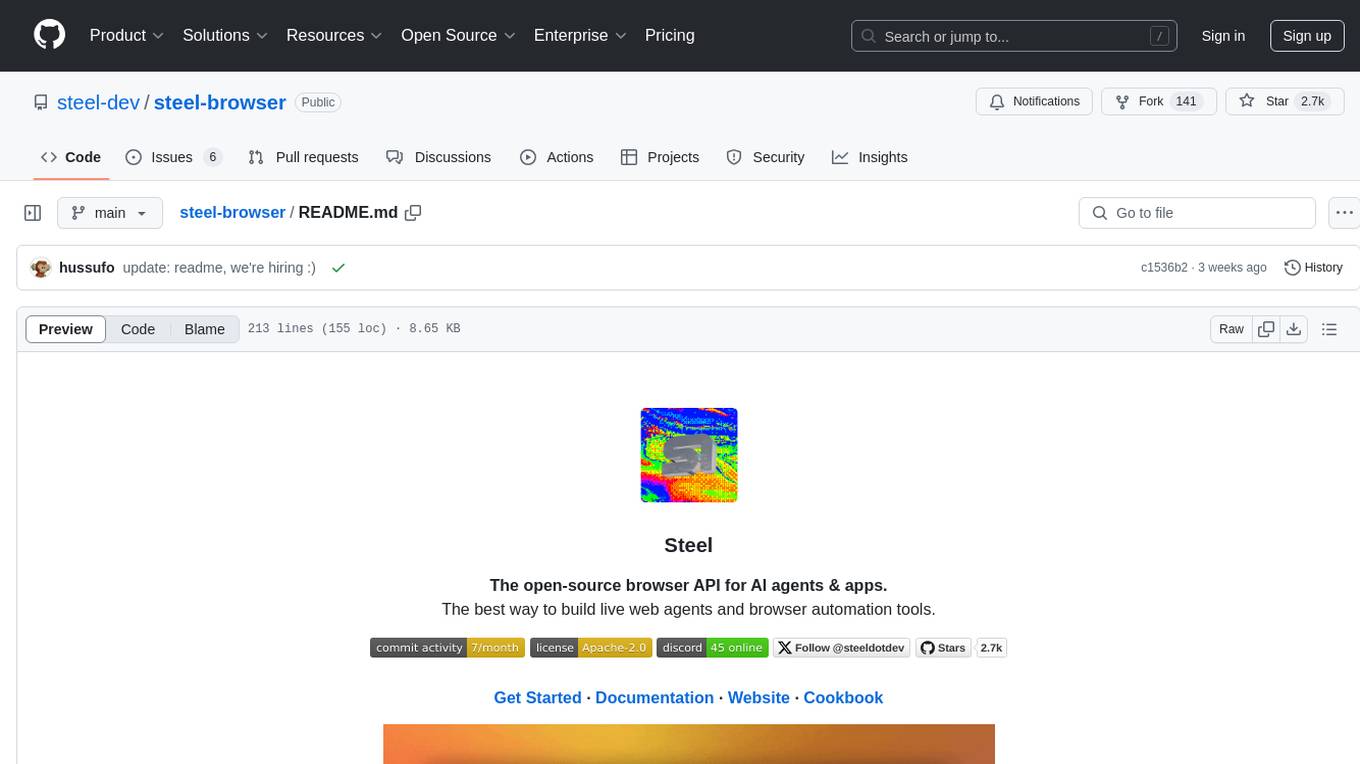
steel-browser
Steel is an open-source browser API designed for AI agents and applications, simplifying the process of building live web agents and browser automation tools. It serves as a core building block for a production-ready, containerized browser sandbox with features like stealth capabilities, text-to-markdown session management, UI for session viewing/debugging, and full browser control through popular automation frameworks. Steel allows users to control, run, and manage a production-ready browser environment via a REST API, offering features such as full browser control, session management, proxy support, extension support, debugging tools, anti-detection mechanisms, resource management, and various browser tools. It aims to streamline complex browsing tasks programmatically, enabling users to focus on their AI applications while Steel handles the underlying complexity.
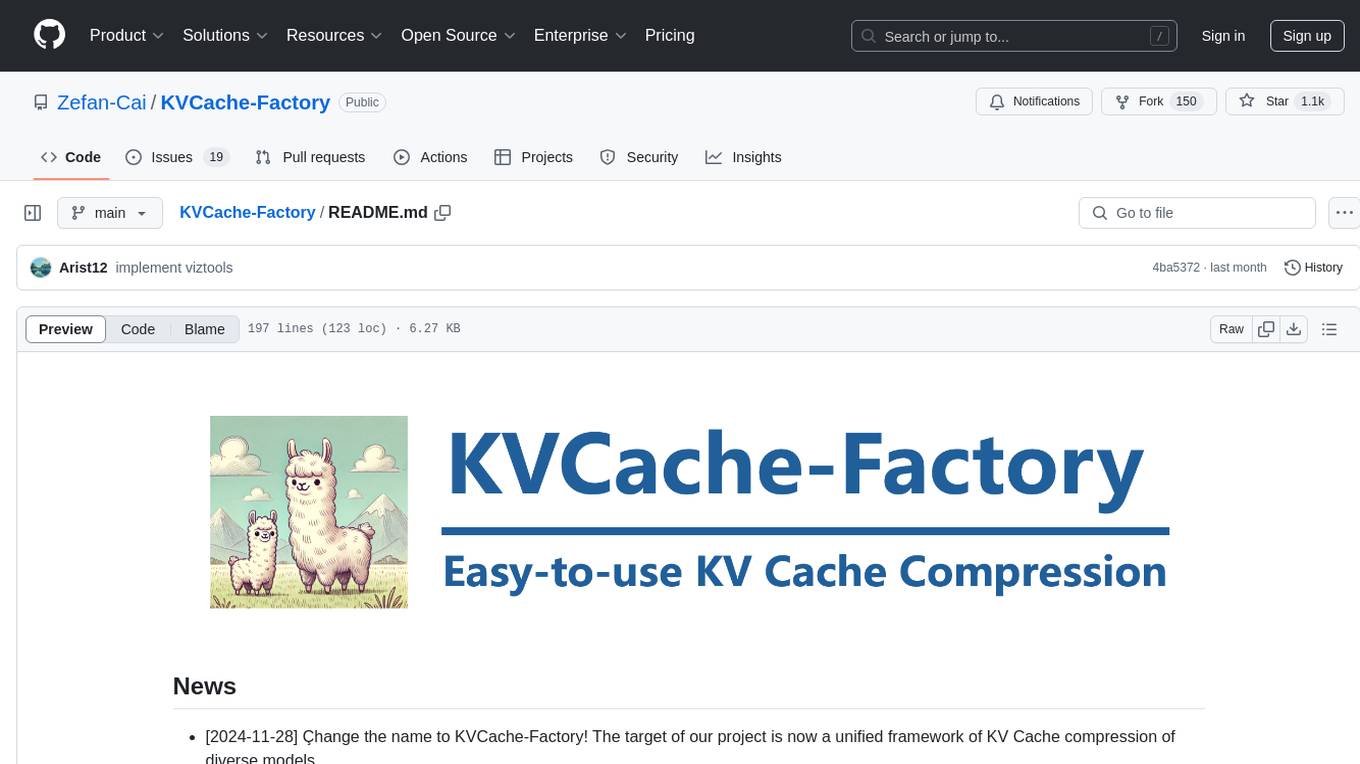
KVCache-Factory
KVCache-Factory is a unified framework for KV Cache compression of diverse models. It supports multi-GPUs inference with big LLMs and various attention implementations. The tool enables KV cache compression without Flash Attention v2, multi-GPU inference, and specific models like Mistral. It also provides functions for KV cache budget allocation and batch inference. The visualization tools help in understanding the attention patterns of models.
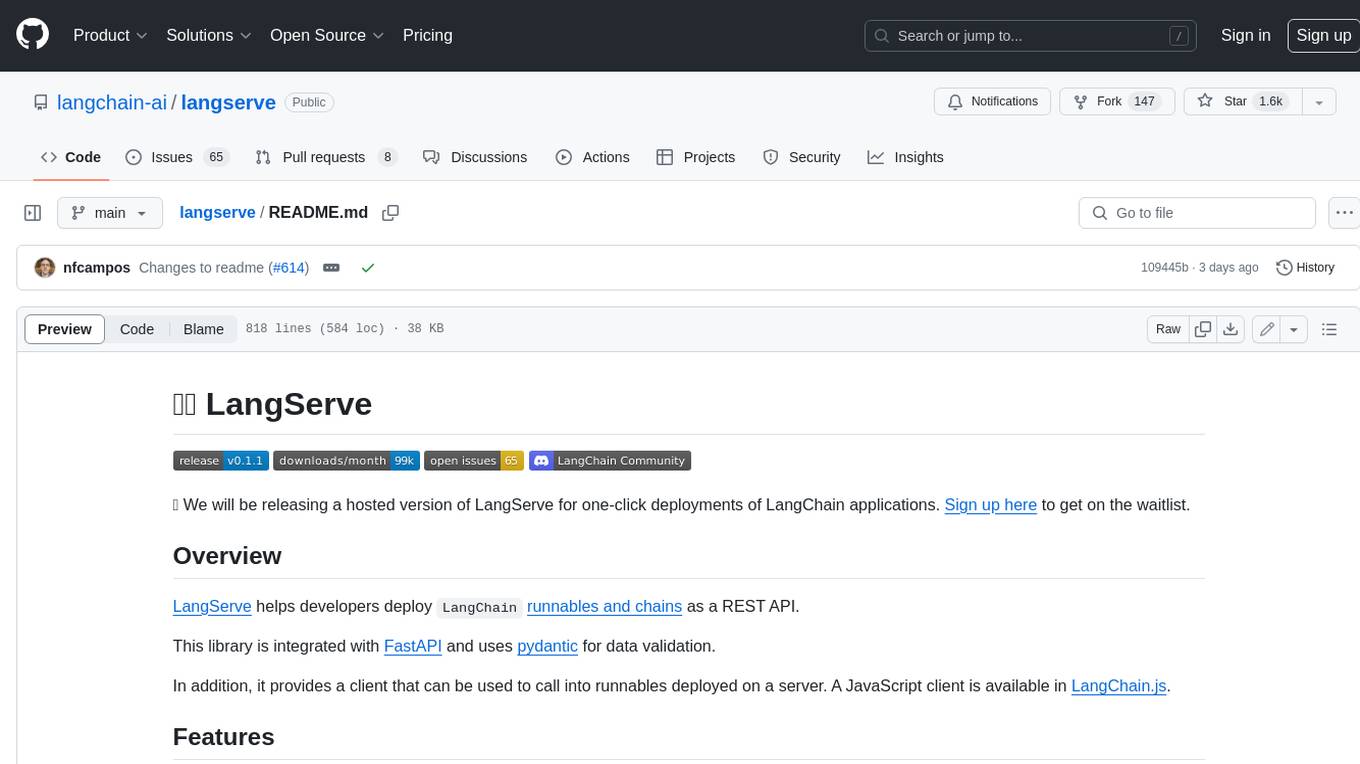
langserve
LangServe helps developers deploy `LangChain` runnables and chains as a REST API. This library is integrated with FastAPI and uses pydantic for data validation. In addition, it provides a client that can be used to call into runnables deployed on a server. A JavaScript client is available in LangChain.js.
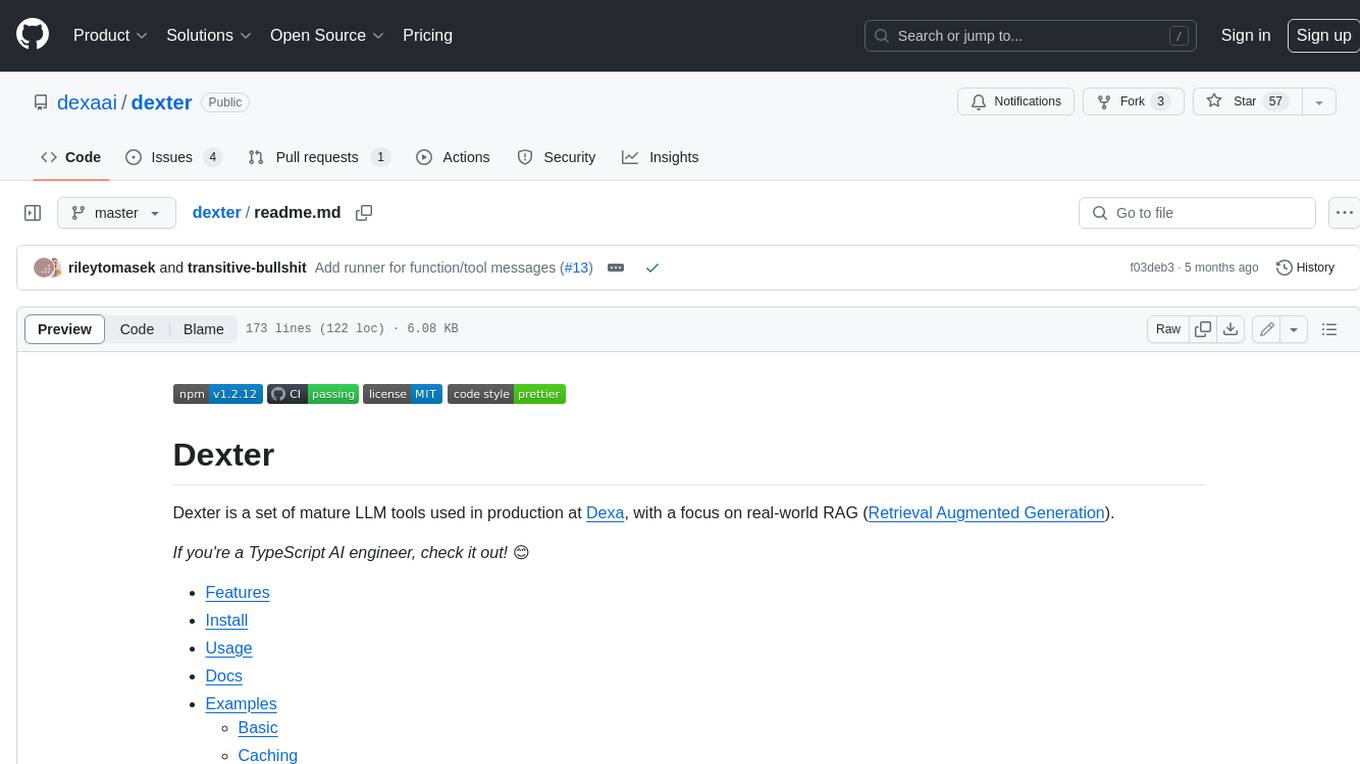
dexter
Dexter is a set of mature LLM tools used in production at Dexa, with a focus on real-world RAG (Retrieval Augmented Generation). It is a production-quality RAG that is extremely fast and minimal, and handles caching, throttling, and batching for ingesting large datasets. It also supports optional hybrid search with SPLADE embeddings, and is a minimal TS package with full typing that uses `fetch` everywhere and supports Node.js 18+, Deno, Cloudflare Workers, Vercel edge functions, etc. Dexter has full docs and includes examples for basic usage, caching, Redis caching, AI function, AI runner, and chatbot.
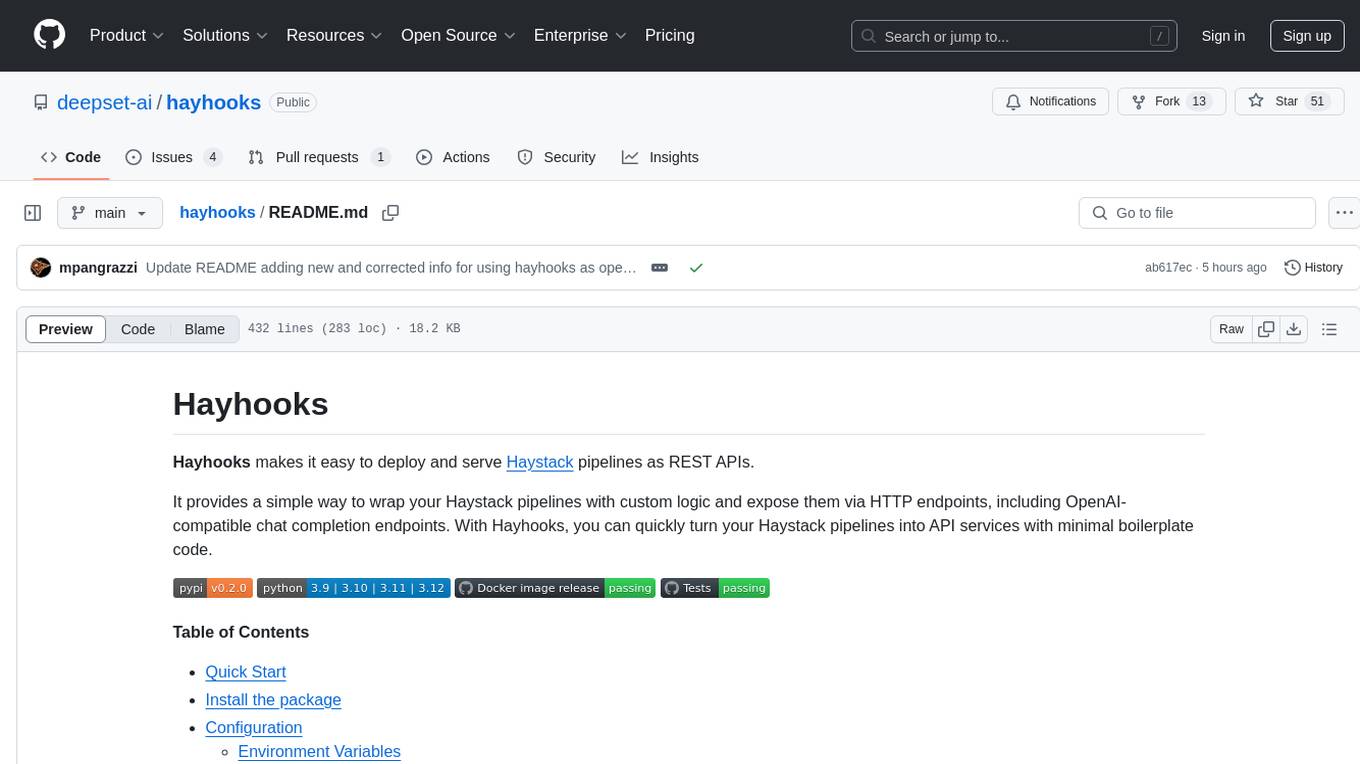
hayhooks
Hayhooks is a tool that simplifies the deployment and serving of Haystack pipelines as REST APIs. It allows users to wrap their pipelines with custom logic and expose them via HTTP endpoints, including OpenAI-compatible chat completion endpoints. With Hayhooks, users can easily convert their Haystack pipelines into API services with minimal boilerplate code.
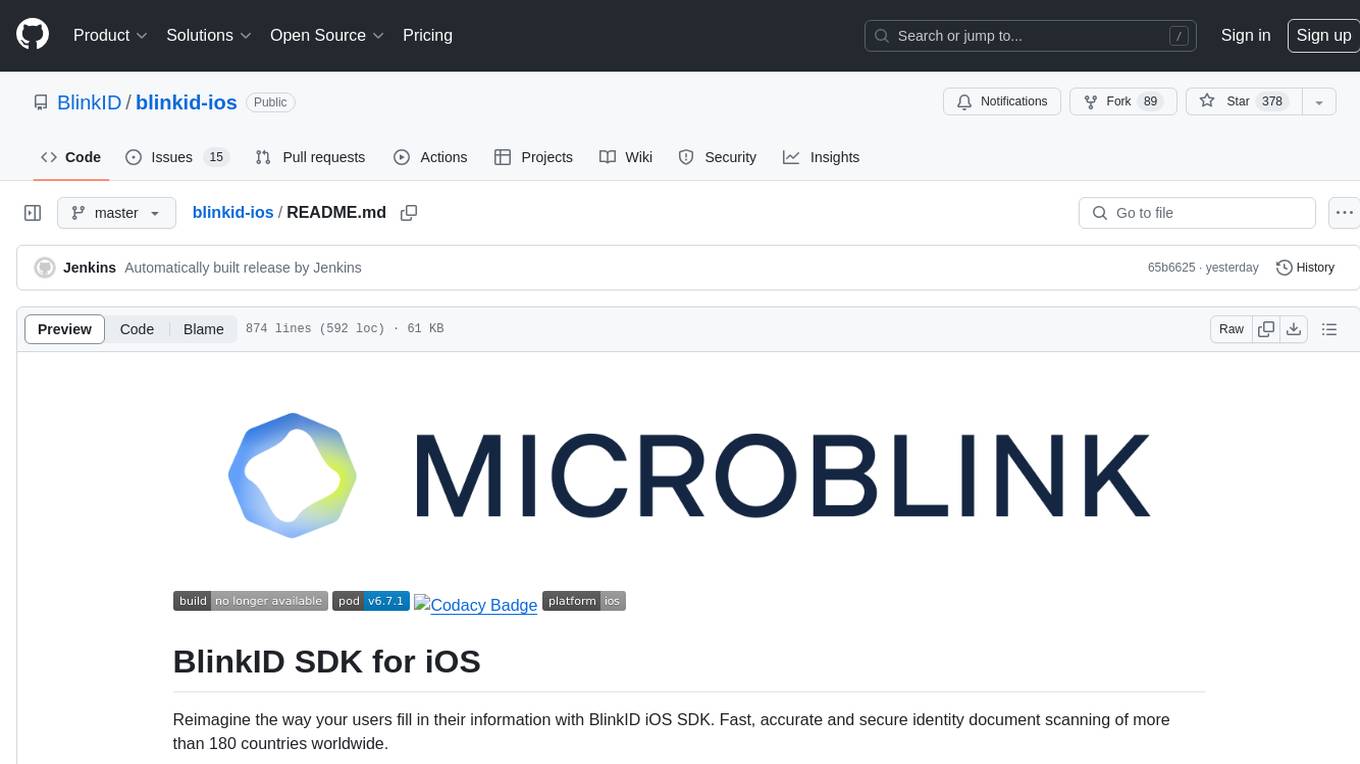
blinkid-ios
BlinkID iOS is a mobile SDK that enables developers to easily integrate ID scanning and data extraction capabilities into their iOS applications. The SDK supports scanning and processing various types of identity documents, such as passports, driver's licenses, and ID cards. It provides accurate and fast data extraction, including personal information and document details. With BlinkID iOS, developers can enhance their apps with secure and reliable ID verification functionality, improving user experience and streamlining identity verification processes.
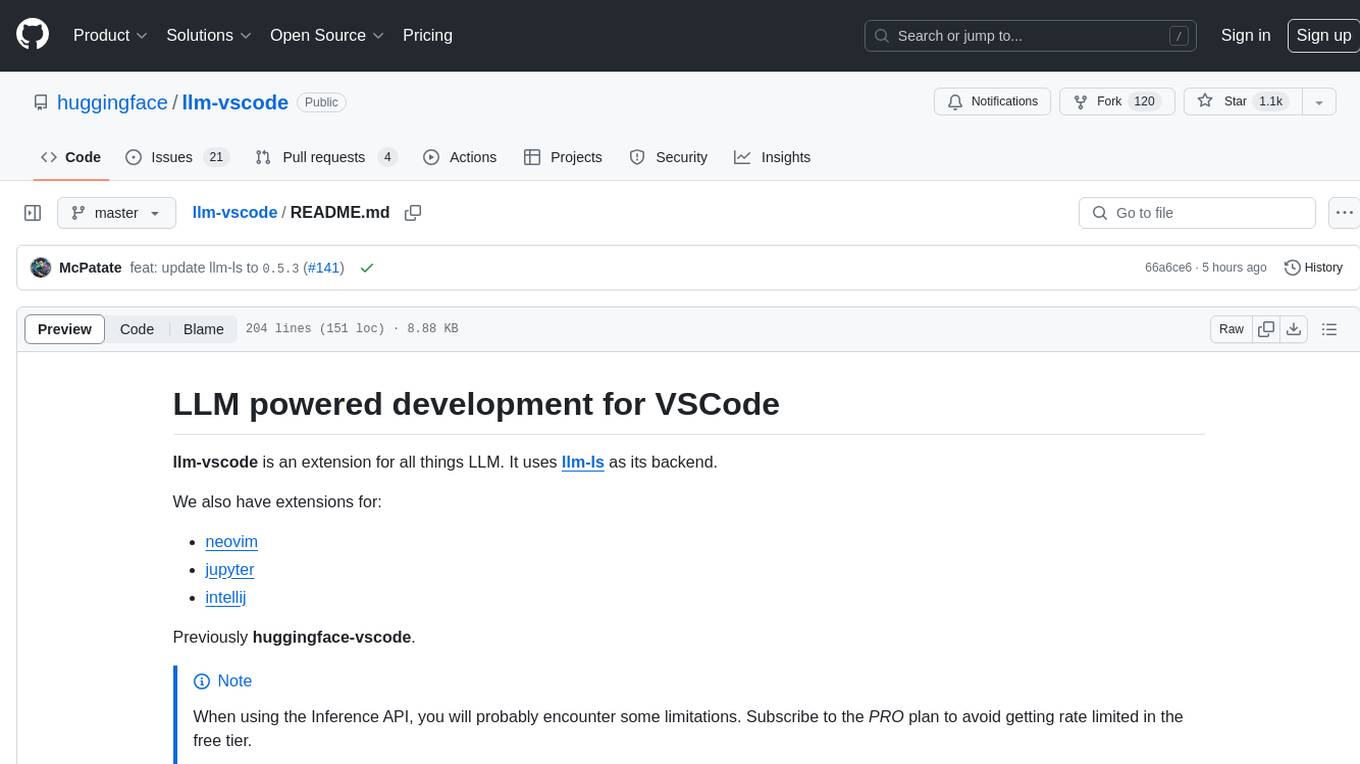
llm-vscode
llm-vscode is an extension designed for all things LLM, utilizing llm-ls as its backend. It offers features such as code completion with 'ghost-text' suggestions, the ability to choose models for code generation via HTTP requests, ensuring prompt size fits within the context window, and code attribution checks. Users can configure the backend, suggestion behavior, keybindings, llm-ls settings, and tokenization options. Additionally, the extension supports testing models like Code Llama 13B, Phind/Phind-CodeLlama-34B-v2, and WizardLM/WizardCoder-Python-34B-V1.0. Development involves cloning llm-ls, building it, and setting up the llm-vscode extension for use.
For similar tasks
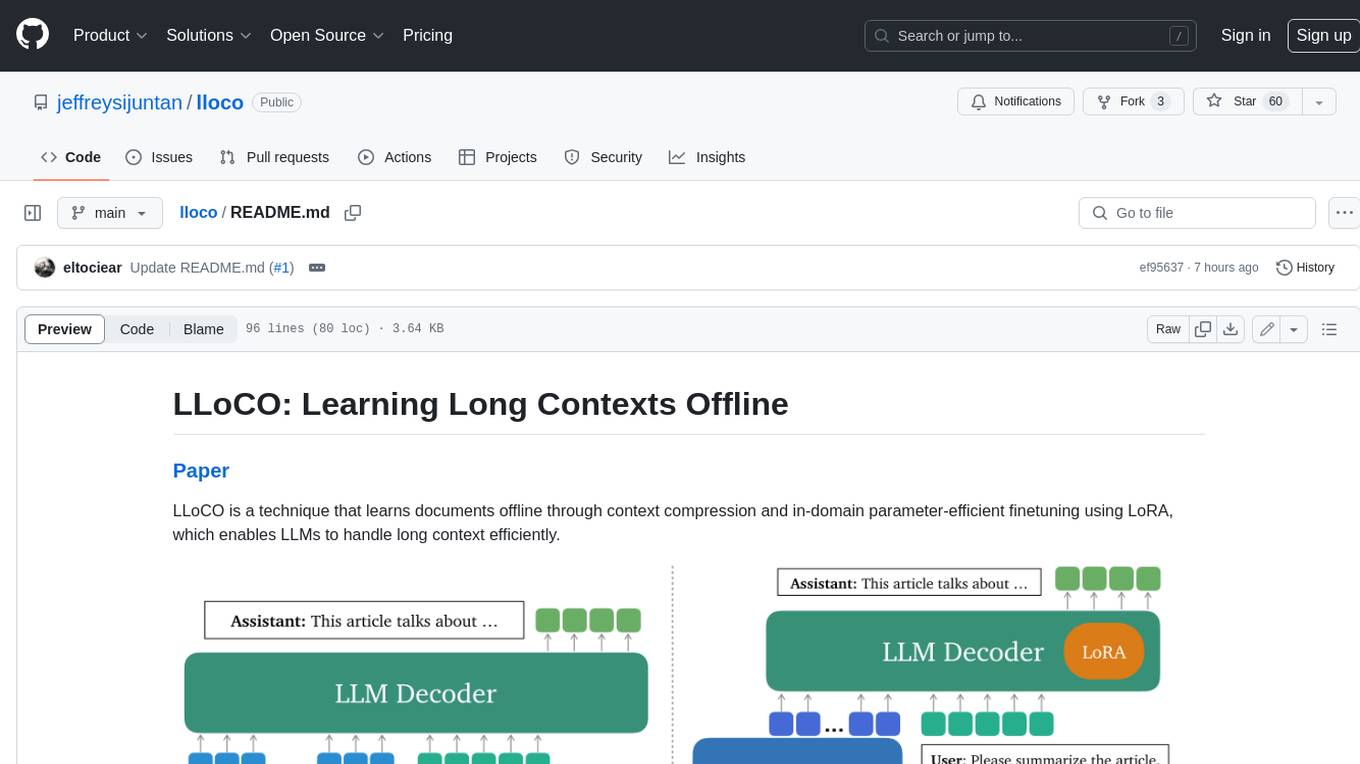
lloco
LLoCO is a technique that learns documents offline through context compression and in-domain parameter-efficient finetuning using LoRA, which enables LLMs to handle long context efficiently.
For similar jobs
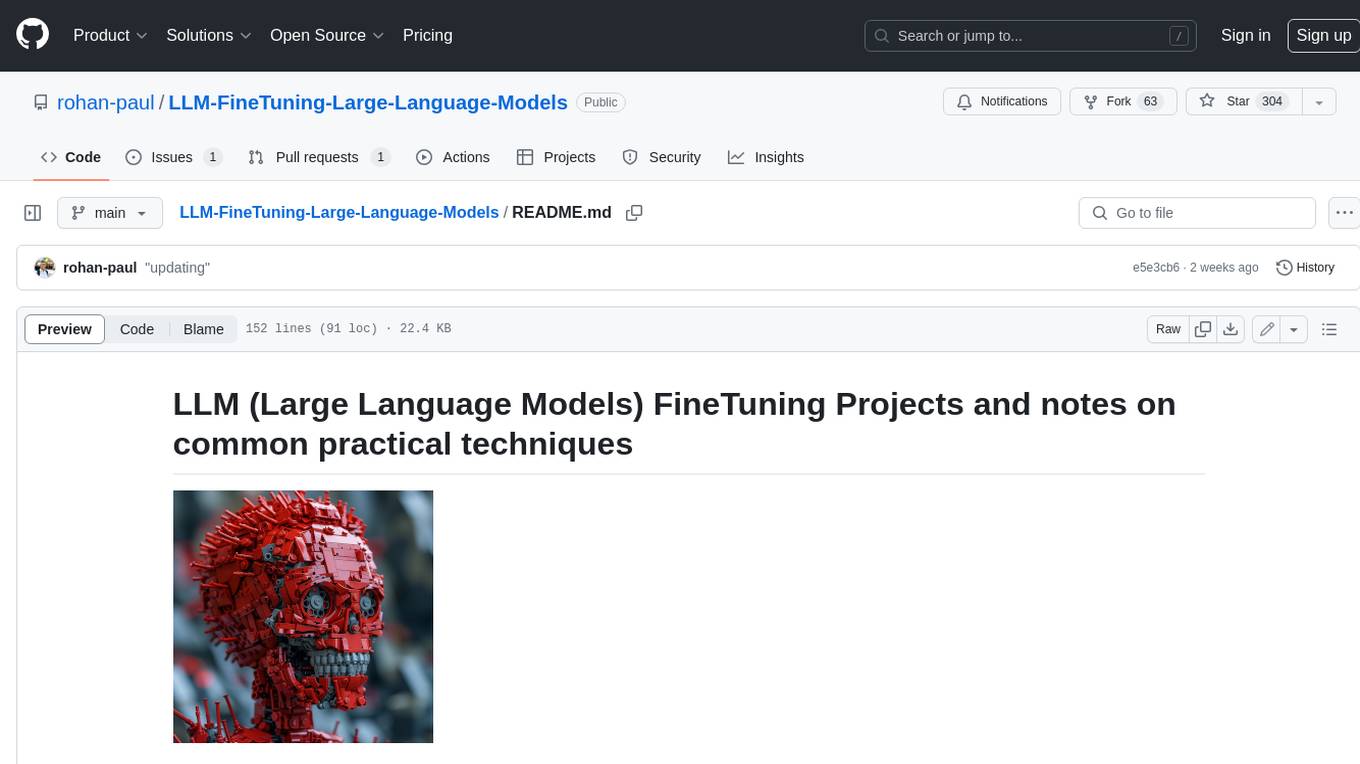
LLM-FineTuning-Large-Language-Models
This repository contains projects and notes on common practical techniques for fine-tuning Large Language Models (LLMs). It includes fine-tuning LLM notebooks, Colab links, LLM techniques and utils, and other smaller language models. The repository also provides links to YouTube videos explaining the concepts and techniques discussed in the notebooks.
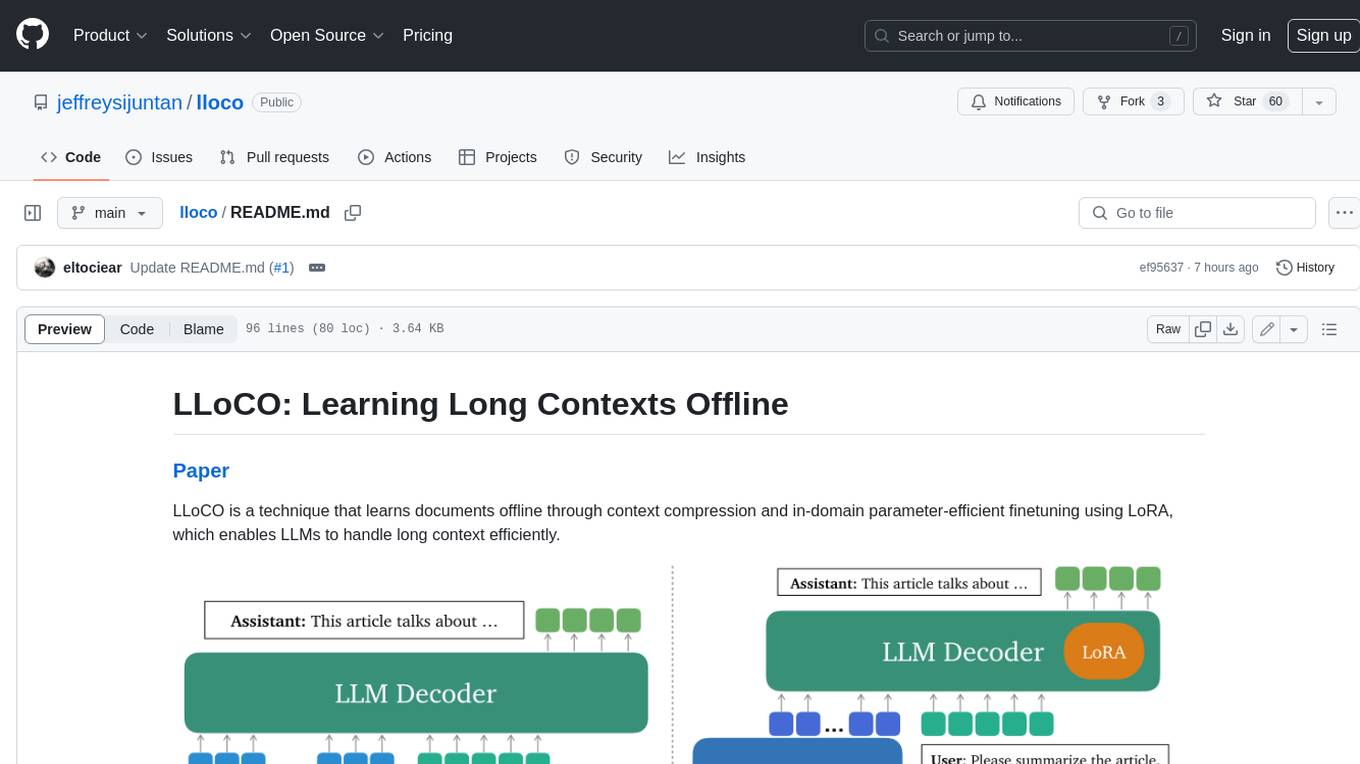
lloco
LLoCO is a technique that learns documents offline through context compression and in-domain parameter-efficient finetuning using LoRA, which enables LLMs to handle long context efficiently.
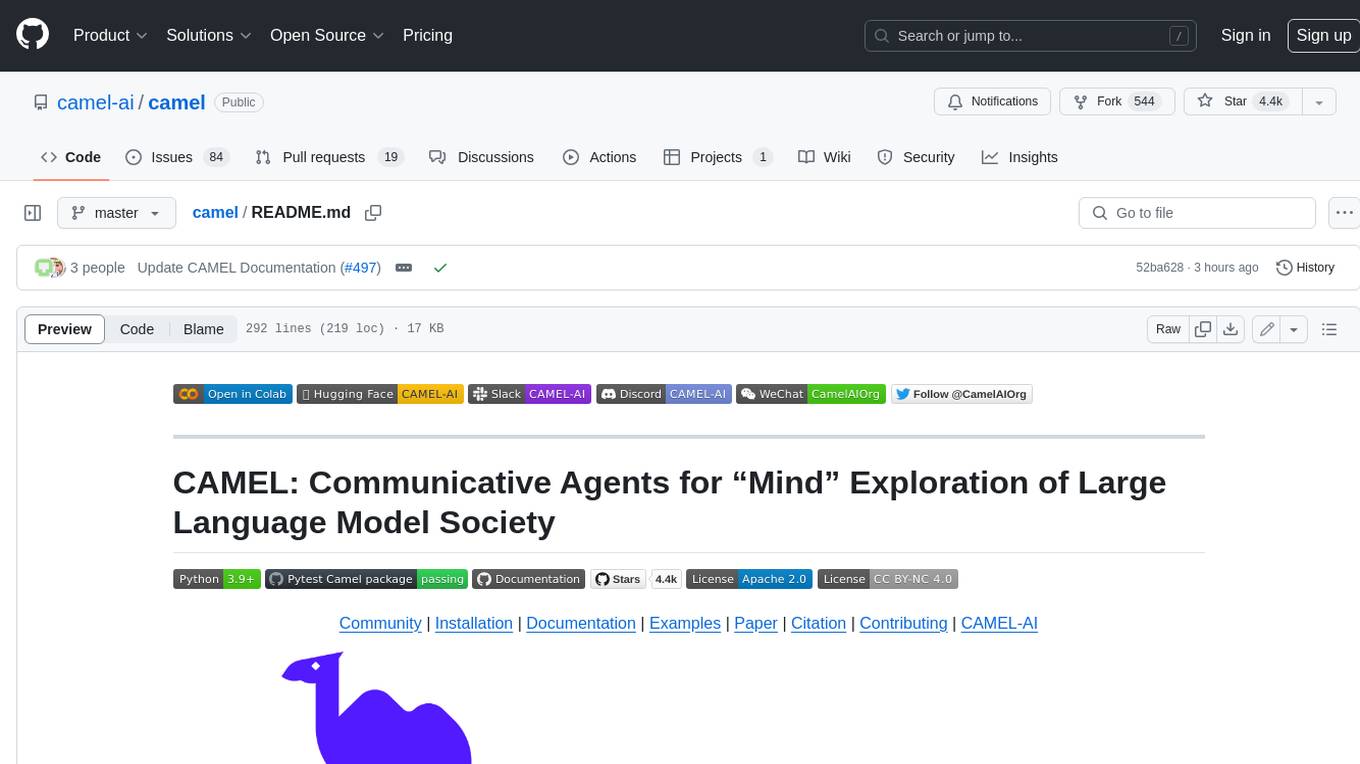
camel
CAMEL is an open-source library designed for the study of autonomous and communicative agents. We believe that studying these agents on a large scale offers valuable insights into their behaviors, capabilities, and potential risks. To facilitate research in this field, we implement and support various types of agents, tasks, prompts, models, and simulated environments.
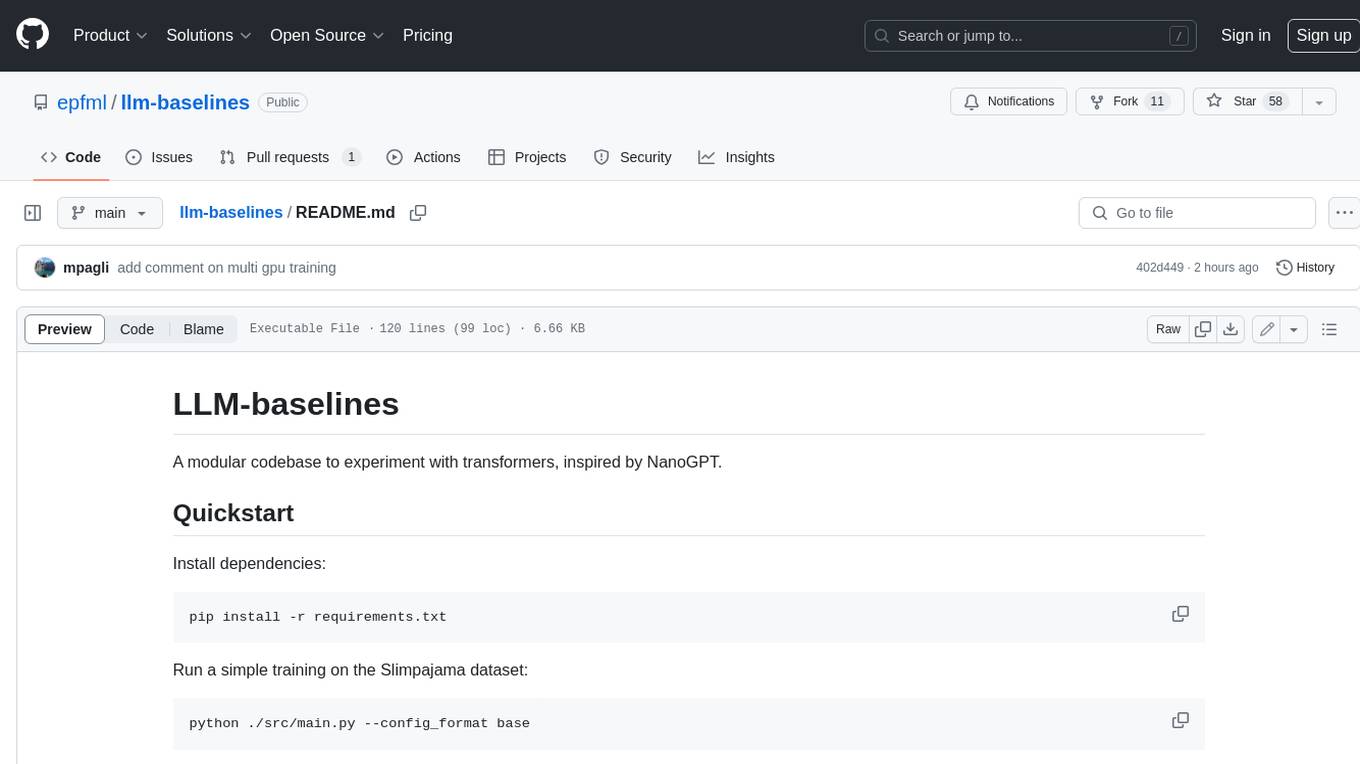
llm-baselines
LLM-baselines is a modular codebase to experiment with transformers, inspired from NanoGPT. It provides a quick and easy way to train and evaluate transformer models on a variety of datasets. The codebase is well-documented and easy to use, making it a great resource for researchers and practitioners alike.
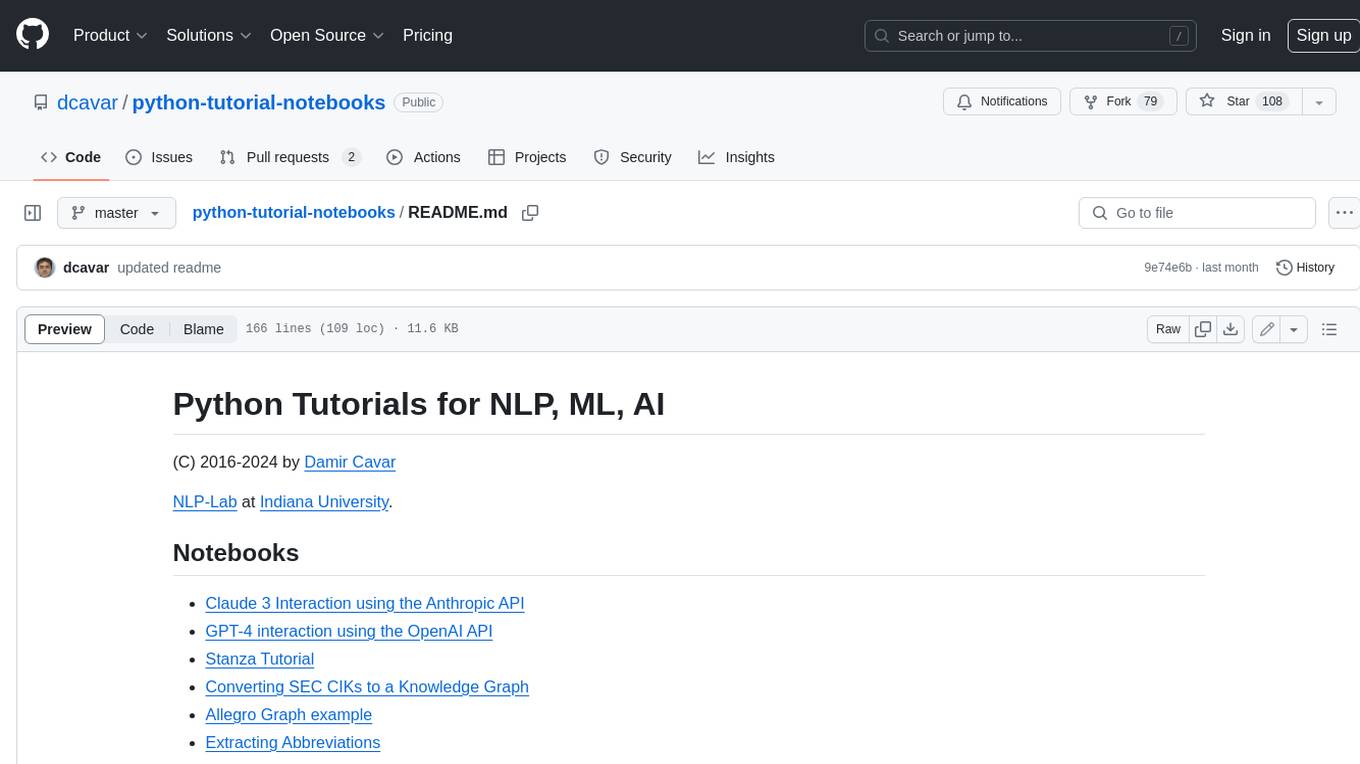
python-tutorial-notebooks
This repository contains Jupyter-based tutorials for NLP, ML, AI in Python for classes in Computational Linguistics, Natural Language Processing (NLP), Machine Learning (ML), and Artificial Intelligence (AI) at Indiana University.
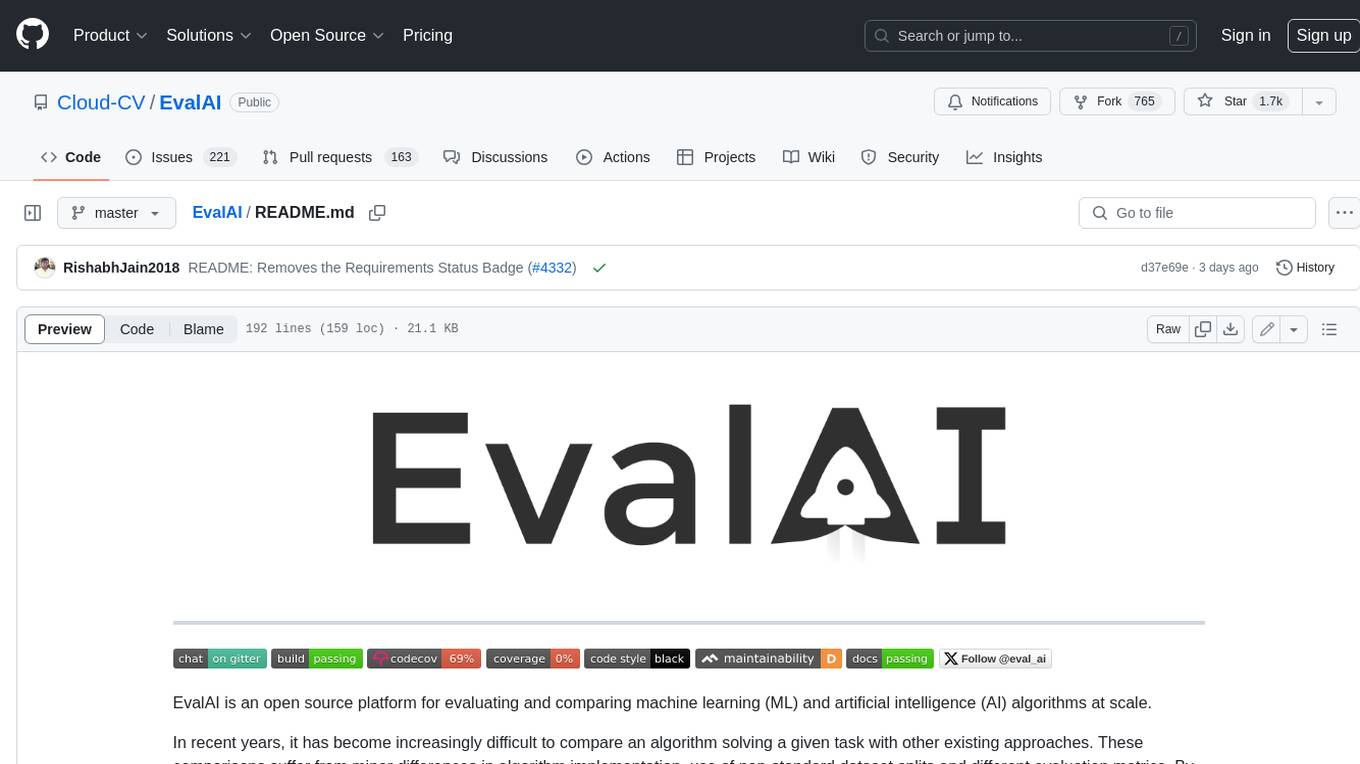
EvalAI
EvalAI is an open-source platform for evaluating and comparing machine learning (ML) and artificial intelligence (AI) algorithms at scale. It provides a central leaderboard and submission interface, making it easier for researchers to reproduce results mentioned in papers and perform reliable & accurate quantitative analysis. EvalAI also offers features such as custom evaluation protocols and phases, remote evaluation, evaluation inside environments, CLI support, portability, and faster evaluation.
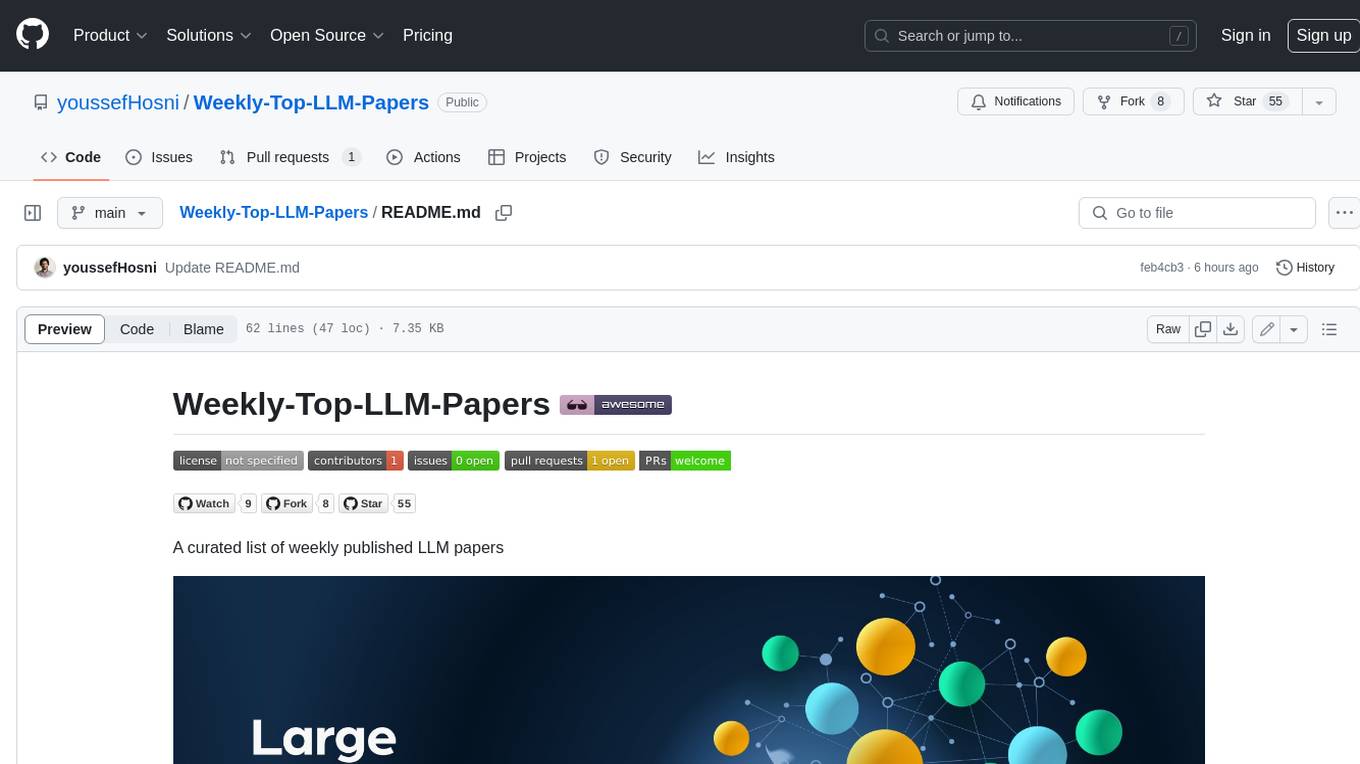
Weekly-Top-LLM-Papers
This repository provides a curated list of weekly published Large Language Model (LLM) papers. It includes top important LLM papers for each week, organized by month and year. The papers are categorized into different time periods, making it easy to find the most recent and relevant research in the field of LLM.
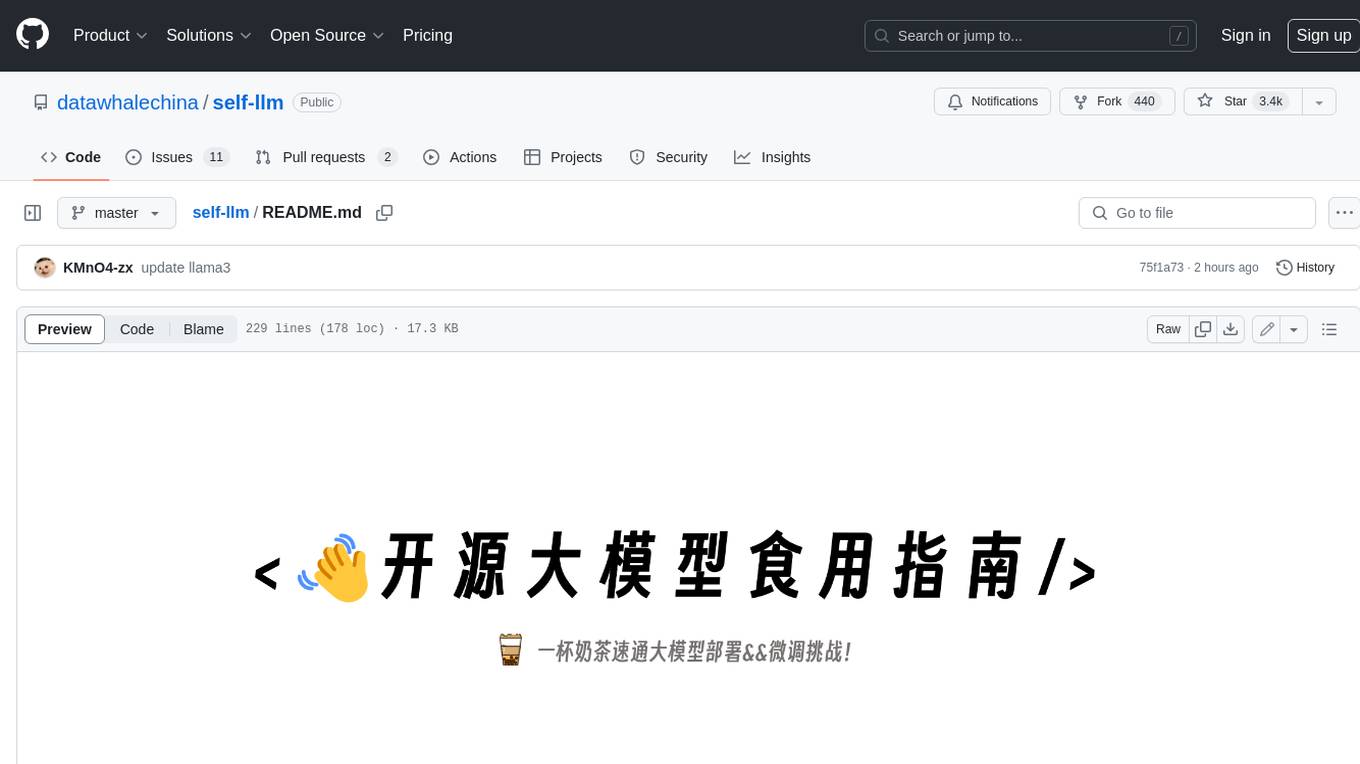
self-llm
This project is a Chinese tutorial for domestic beginners based on the AutoDL platform, providing full-process guidance for various open-source large models, including environment configuration, local deployment, and efficient fine-tuning. It simplifies the deployment, use, and application process of open-source large models, enabling more ordinary students and researchers to better use open-source large models and helping open and free large models integrate into the lives of ordinary learners faster.