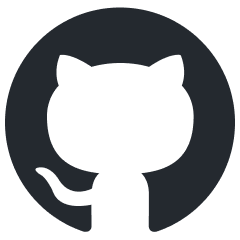
KVCache-Factory
Unified KV Cache Compression Methods for Auto-Regressive Models
Stars: 1109
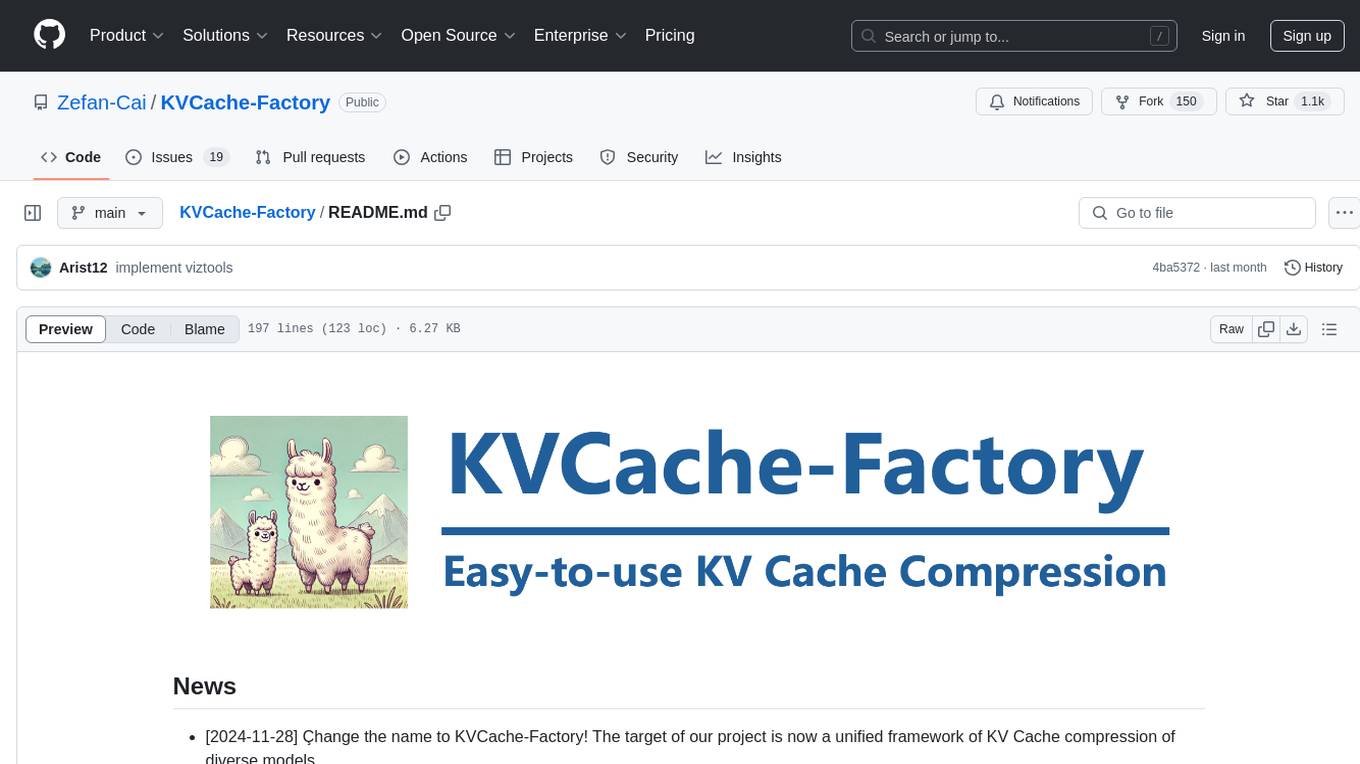
KVCache-Factory is a unified framework for KV Cache compression of diverse models. It supports multi-GPUs inference with big LLMs and various attention implementations. The tool enables KV cache compression without Flash Attention v2, multi-GPU inference, and specific models like Mistral. It also provides functions for KV cache budget allocation and batch inference. The visualization tools help in understanding the attention patterns of models.
README:
-
[2024-11-28] Çhange the name to KVCache-Factory! The target of our project is now a unified framework of KV Cache compression of diverse models.
-
[2024-06-25] Support multi-GPUs inference with big LLMs now! Try out PyramidKV on LlaMa-3-70B-Instruct!
-
[2024-06-10] Support PyramidKV, SnapKV, H2O and StreamingLLM at Flash Attention v2, Sdpa Attention now! If your devices (i.e., V100, 3090) does not support Flash Attention v2, you can set attn_implementation=sdpa to try PyramidKV at Sdpa Attention!
-
[x] Support implementation of Streaming LLM, H2O and SnapKV
-
[x] Support Mistral model
-
[x] Support implementation of Needle
-
[x] Support KV cache compression without Flash Attention v2 (i.e. Sdpa Attention) for V100
-
[x] Support multi-GPU inference for 70B LlaMa-3
-
[ ] Introduce new functions to support kv cache budget allocation (i.e., supports for percentage.)
-
[ ] Support Mixtral
-
[ ] Support Batch Inference
-
[ ] Support KV cache compression at decoding stage
The Llama model attention map with 3 documents is represented as follows:
./visualization-tools/vis.ipynb
reproduces the visualization results in the paper. We provide more visualization tools under ./visualization
that supports different levels of kv-cache visualization.
Model attention maps for different layers would be stored at ./attention
transformers >= 4.41
flash-attn >= 2.4.0.post1
git clone https://github.com/Zefan-Cai/PyramidKV.git
cd PyramidKV
pip install -r requirements.txt .
We support inference code on LongBench
to repuduce our result.
Please refer to scripts/scripts_longBench/eval.sh
to modify the parameters according to your requirements.
Our codebase support Flash Attention v2, Sdpa Attention, etc. The results presented in our paper in based on Flash Attention v2.
export CUDA_VISIBLE_DEVICES=$1
method=$2 # Support PyramidKV, SnapKV, H2O, StreamingLLM
max_capacity_prompts=64 # 128,2048 in paper
attn_implementation=$3 # Support "flash_attention_2", "sdpa", "eager".
source_path=$4
model_path=$5
save_dir=${source_path}"results_long_bench" # path to result save_dir
python3 run_longbench.py \
--method ${method} \
--model_path ${model_path} \
--max_capacity_prompts ${max_capacity_prompts} \
--attn_implementation ${attn_implementation} \
--save_dir ${save_dir} \
--use_cache True
- CUDA_VISIBLE_DEVICES: For multi-GPU inference for big LLMs, just need to specify CUDA_VISIBLE_DEVICES=0,1,2,3,4,5,6,7. For single GPU inference, just need to specify CUDA_VISIBLE_DEVICES=0.
- model_path: Path to your model. Support "Llama-3-8B-Instruct" for now.
- method: Support
PyramidKV
,SnapKV
,StreamingLLM
,H2O
. - max_capacity_prompts: Selected KV Size in each layer. (e.g. 128, 2048 in paper). When method is "PyramidKV", given that the total number of KV remains unchanged, the specific KV length for each layer will be modified accordingly
- save_dir: Path to your dir to save LongBench result.
After modifying parameters, run:
sh scripts/scripts_longBench/eval.sh
We support inference code on Needle in haystack
to repuduce our result.
Please refer to scripts/scripts_needle/eval.sh
to modify the parameters according to your requirements.
Our codebase support Flash Attention v2, Sdpa Attention, etc. The results presented in our paper in based on Flash Attention v2.
METHOD='pyramidkv' # ['full', 'pyramidkv', 'snapkv', 'streamingllm', 'h2o']
MAX_CAPACITY_PROMPT=96 # [64, 96, 128, 256, 512, 1024, 2048, ...]
attn_implementation="flash_attention_2" # Support "flash_attention_2", "sdpa", "".
TAG=test
# For Llama3-8b
(
python -u run_needle_in_haystack.py --s_len 1000 --e_len 8001\
--model_provider LLaMA3 \
--model_name /mnt/workspace/zhiyuanhu/yuliang/models/llama3-8b_raw \
--attn_implementation ${attn_implementation} \
--step 100 \
--method $METHOD \
--max_capacity_prompt $MAX_CAPACITY_PROMPT \
--model_version LlaMA3_${METHOD}_${MAX_CAPACITY_PROMPT}_${TAG}
) 2>&1 | tee results_needle/logs/LlaMA3_${METHOD}_${MAX_CAPACITY_PROMPT}_${TAG}.log
- Both LLaMA3 and Mistral2 inference support on single GPU.
- model_provider: LLaMA3 or Mistral2
- model_name: Path to your model. Support "Llama-3-8B-Instruct" "Mistral-7B-Instruct-v0.2" and for now.
- step: The increase of context length.
- method: Support
PyramidKV
,SnapKV
,StreamingLLM
,H2O
. - max_capacity_prompt: Selected KV Size in each layer. (e.g. 128, 2048 in paper). When method is "PyramidKV", given that the total number of KV remains unchanged, the specific KV length for each layer will be modified accordingly
To reproduce our results, run
bash scripts/scripts_needle/eval.sh
After inference, run
python scripts/scripts_needle/visualize.py
to draw the img, you should change FOLDER_PATH
in visualize.py
to your output path (the argument of --model_version
in eval.sh
).
If you find PyramidKV useful for your research and applications, please kindly cite using this BibTeX:
@article{cai2024pyramidkv,
title={Pyramidkv: Dynamic kv cache compression based on pyramidal information funneling},
author={Cai, Zefan and Zhang, Yichi and Gao, Bofei and Liu, Yuliang and Liu, Tianyu and Lu, Keming and Xiong, Wayne and Dong, Yue and Chang, Baobao and Hu, Junjie and Xiao Wen},
journal={arXiv preprint arXiv:2406.02069},
year={2024}
}
@article{fu2024not,
title={Not All Heads Matter: A Head-Level KV Cache Compression Method with Integrated Retrieval and Reasoning},
author={Fu, Yu and Cai, Zefan and Asi, Abedelkadir and Xiong, Wayne and Dong, Yue and Xiao, Wen},
journal={arXiv preprint arXiv:2410.19258},
year={2024}
}
Thanks [SnapKV] SnapKV: LLM Knows What You are Looking for Before Generation for providing open-source code to support the expansion of this project.
For Tasks:
Click tags to check more tools for each tasksFor Jobs:
Alternative AI tools for KVCache-Factory
Similar Open Source Tools
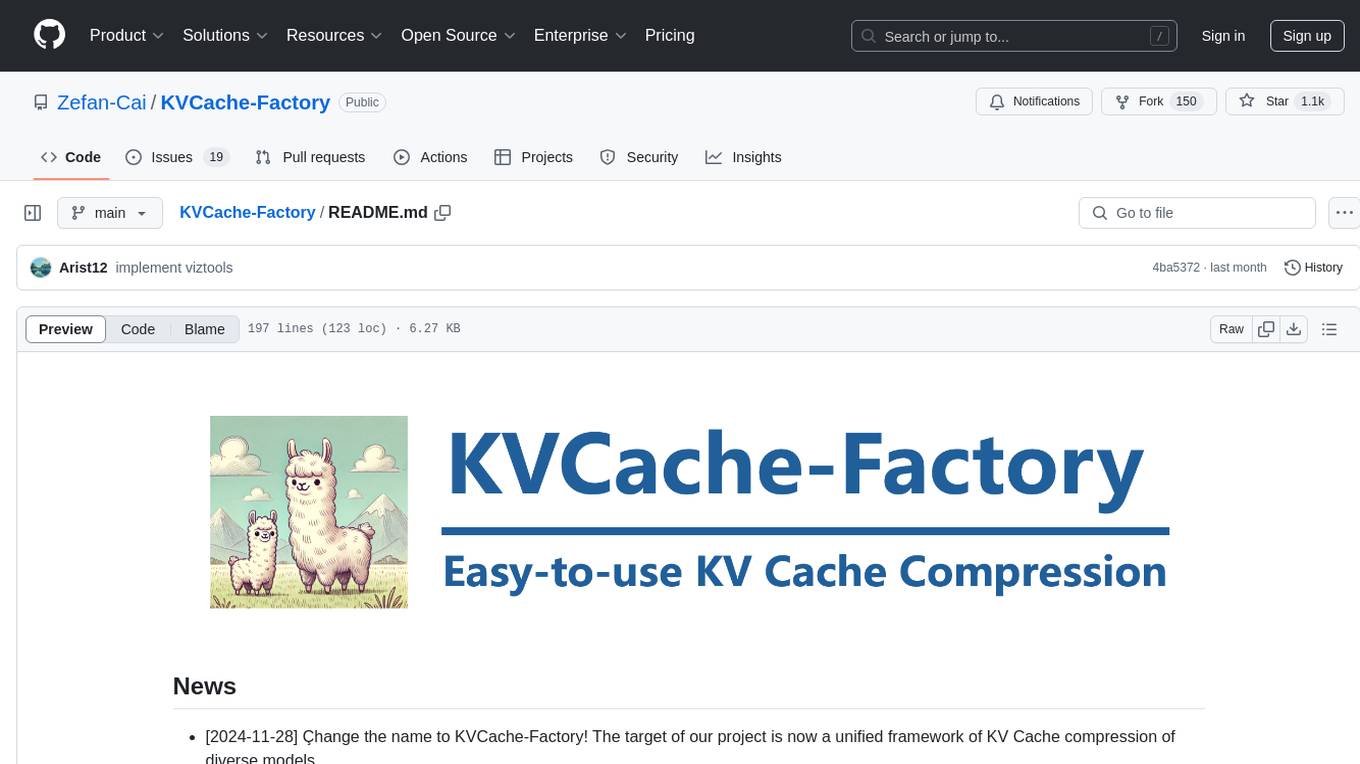
KVCache-Factory
KVCache-Factory is a unified framework for KV Cache compression of diverse models. It supports multi-GPUs inference with big LLMs and various attention implementations. The tool enables KV cache compression without Flash Attention v2, multi-GPU inference, and specific models like Mistral. It also provides functions for KV cache budget allocation and batch inference. The visualization tools help in understanding the attention patterns of models.
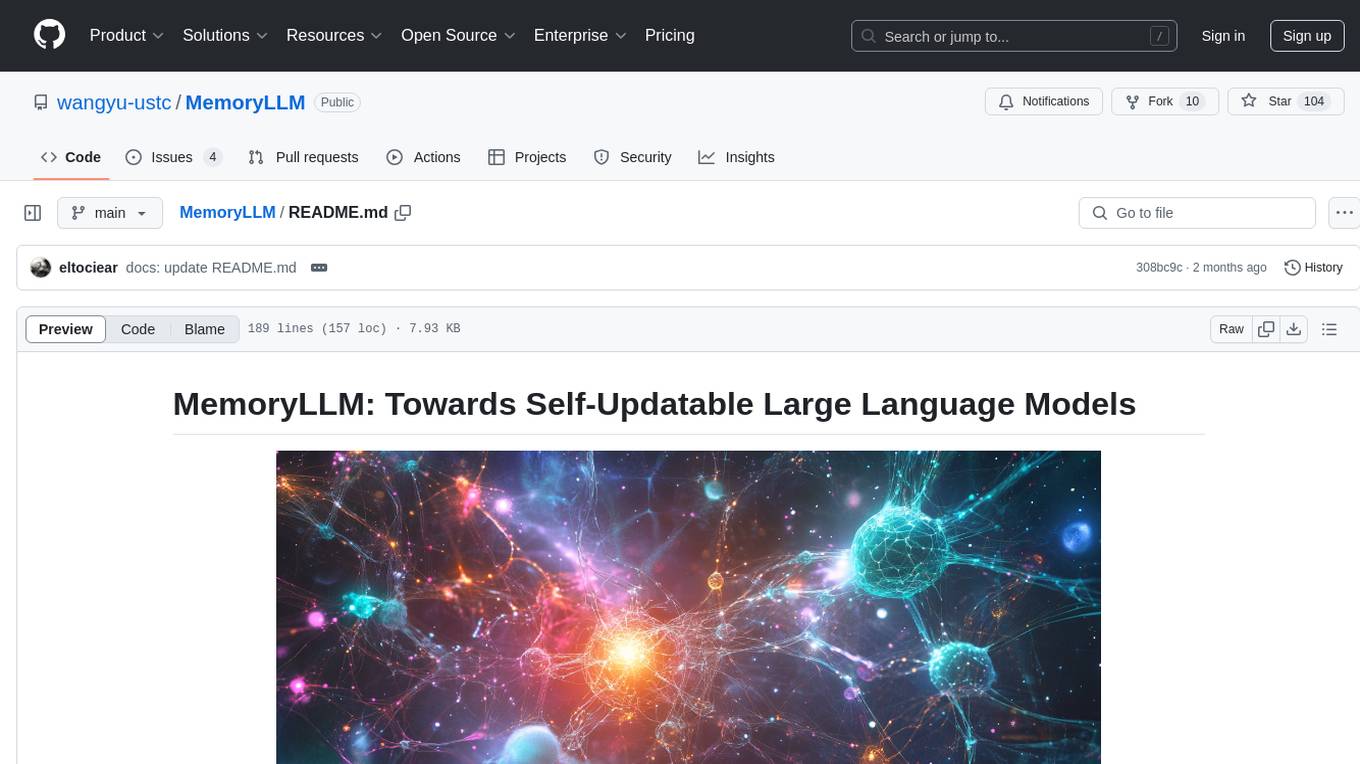
MemoryLLM
MemoryLLM is a large language model designed for self-updating capabilities. It offers pretrained models with different memory capacities and features, such as chat models. The repository provides training code, evaluation scripts, and datasets for custom experiments. MemoryLLM aims to enhance knowledge retention and performance on various natural language processing tasks.
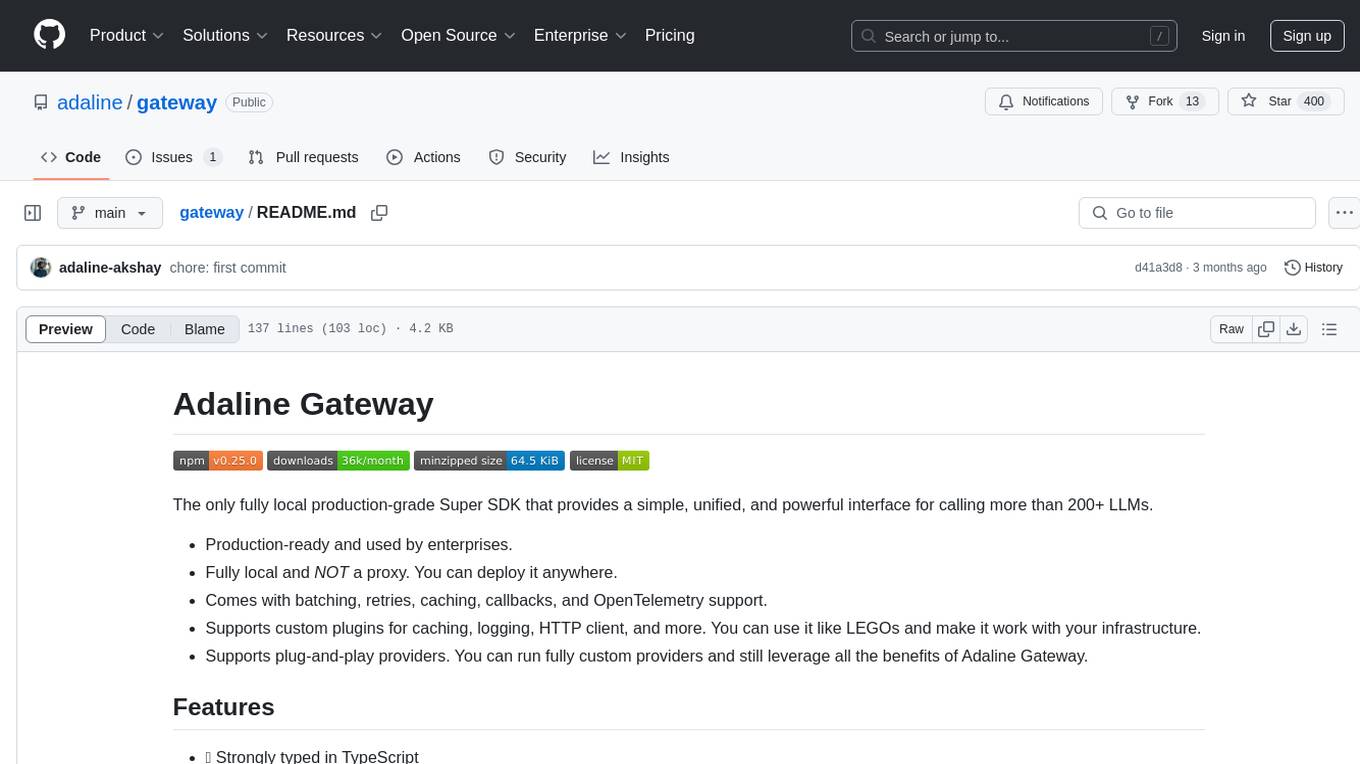
gateway
Adaline Gateway is a fully local production-grade Super SDK that offers a unified interface for calling over 200+ LLMs. It is production-ready, supports batching, retries, caching, callbacks, and OpenTelemetry. Users can create custom plugins and providers for seamless integration with their infrastructure.
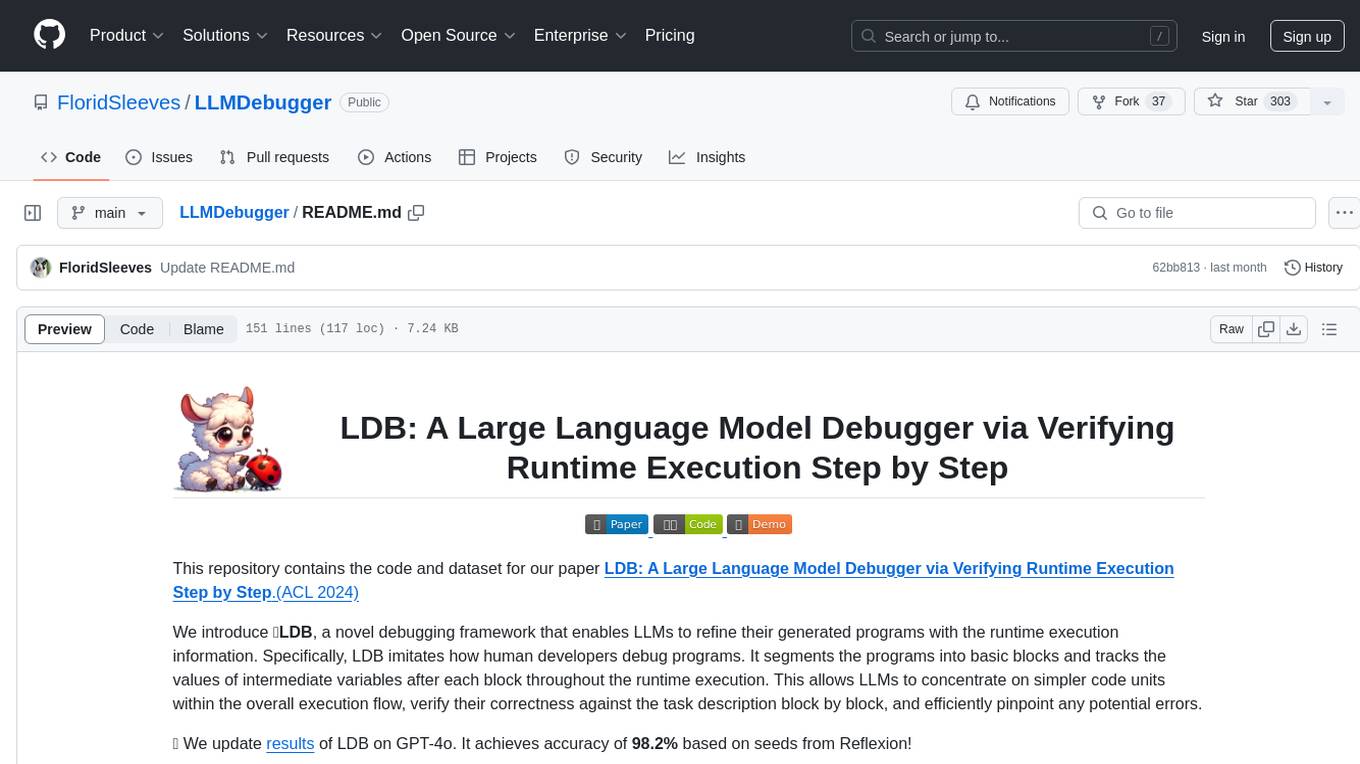
LLMDebugger
This repository contains the code and dataset for LDB, a novel debugging framework that enables Large Language Models (LLMs) to refine their generated programs by tracking the values of intermediate variables throughout the runtime execution. LDB segments programs into basic blocks, allowing LLMs to concentrate on simpler code units, verify correctness block by block, and pinpoint errors efficiently. The tool provides APIs for debugging and generating code with debugging messages, mimicking how human developers debug programs.
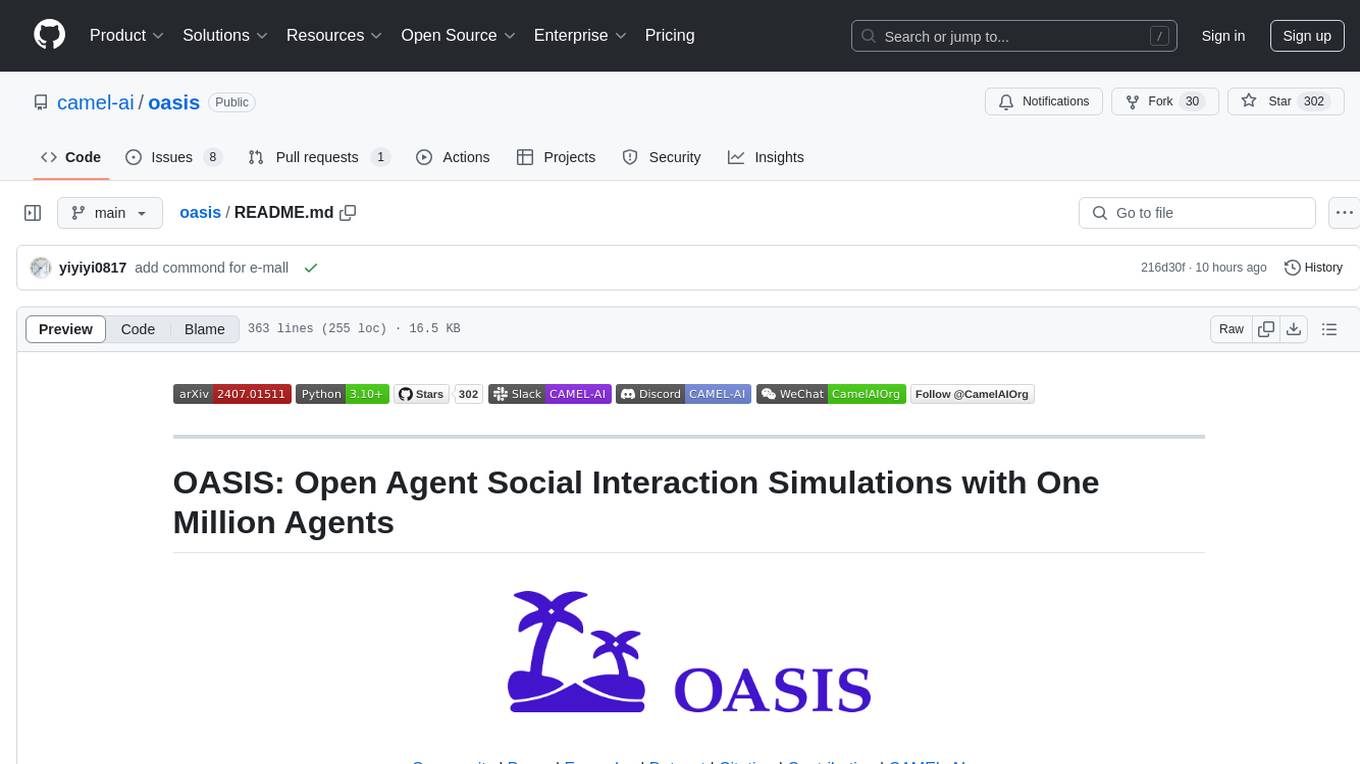
oasis
OASIS is a scalable, open-source social media simulator that integrates large language models with rule-based agents to realistically mimic the behavior of up to one million users on platforms like Twitter and Reddit. It facilitates the study of complex social phenomena such as information spread, group polarization, and herd behavior, offering a versatile tool for exploring diverse social dynamics and user interactions in digital environments. With features like scalability, dynamic environments, diverse action spaces, and integrated recommendation systems, OASIS provides a comprehensive platform for simulating social media interactions at a large scale.
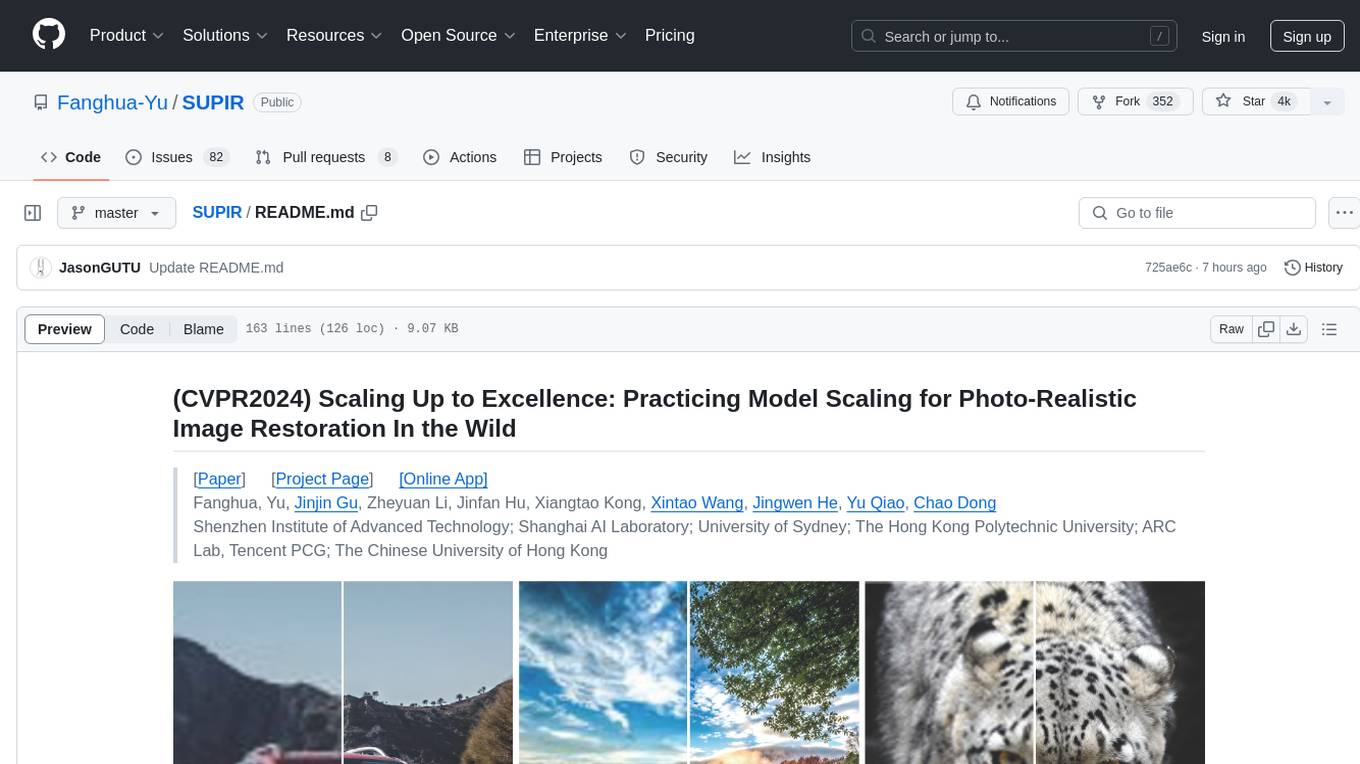
SUPIR
SUPIR is an AI-based image processing and upscaling tool that leverages cutting-edge technology to enhance image quality and resolution. The tool provides users with the ability to upscale images with high generalization and quality, as well as specific settings for light degradation scenarios. It offers a range of models and checkpoints for different use cases, along with detailed instructions for installation and usage. SUPIR also includes features for color fixing, linear CFG adjustments, and various prompts for image enhancement. The tool is designed for non-commercial use only and comes with a contact email for inquiries and permission requests for commercial use.
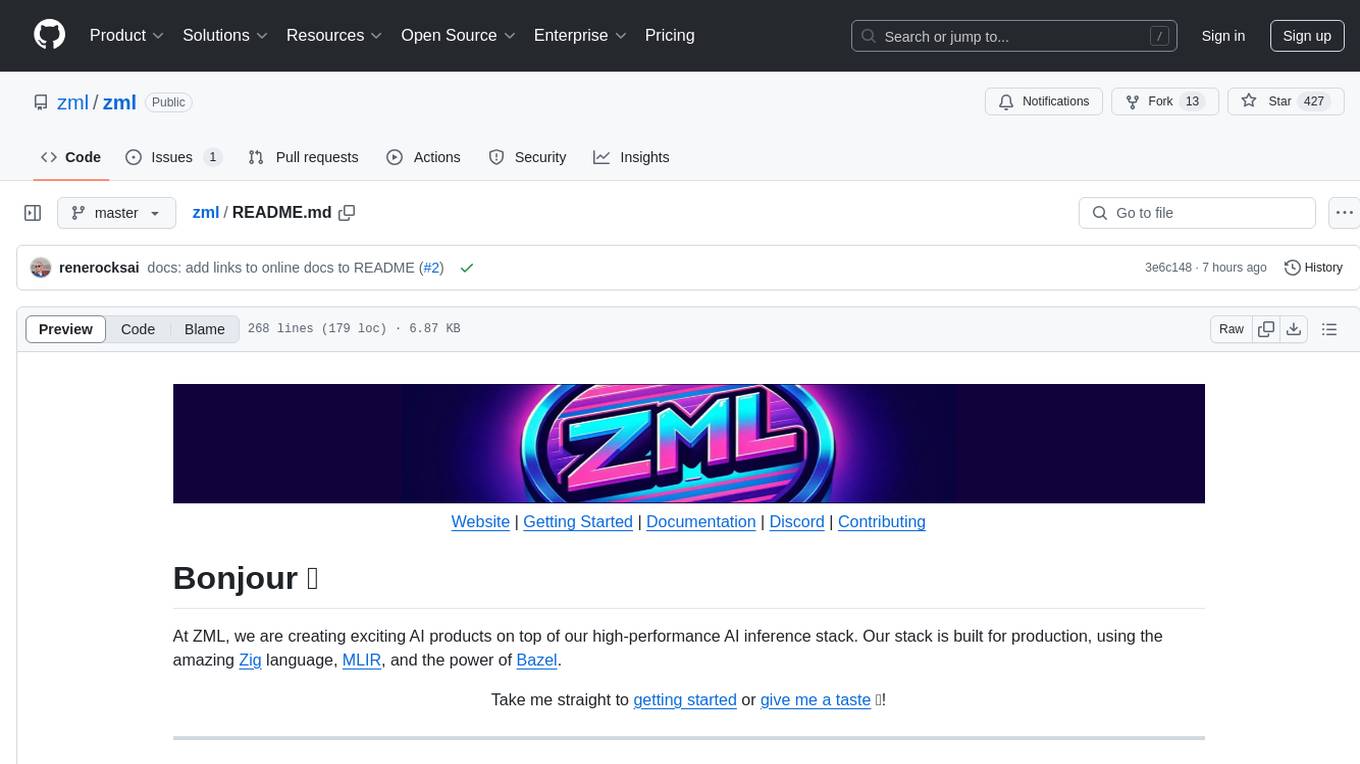
zml
ZML is a high-performance AI inference stack built for production, using Zig language, MLIR, and Bazel. It allows users to create exciting AI projects, run pre-packaged models like MNIST, TinyLlama, OpenLLama, and Meta Llama, and compile models for accelerator runtimes. Users can also run tests, explore examples, and contribute to the project. ZML is licensed under the Apache 2.0 license.
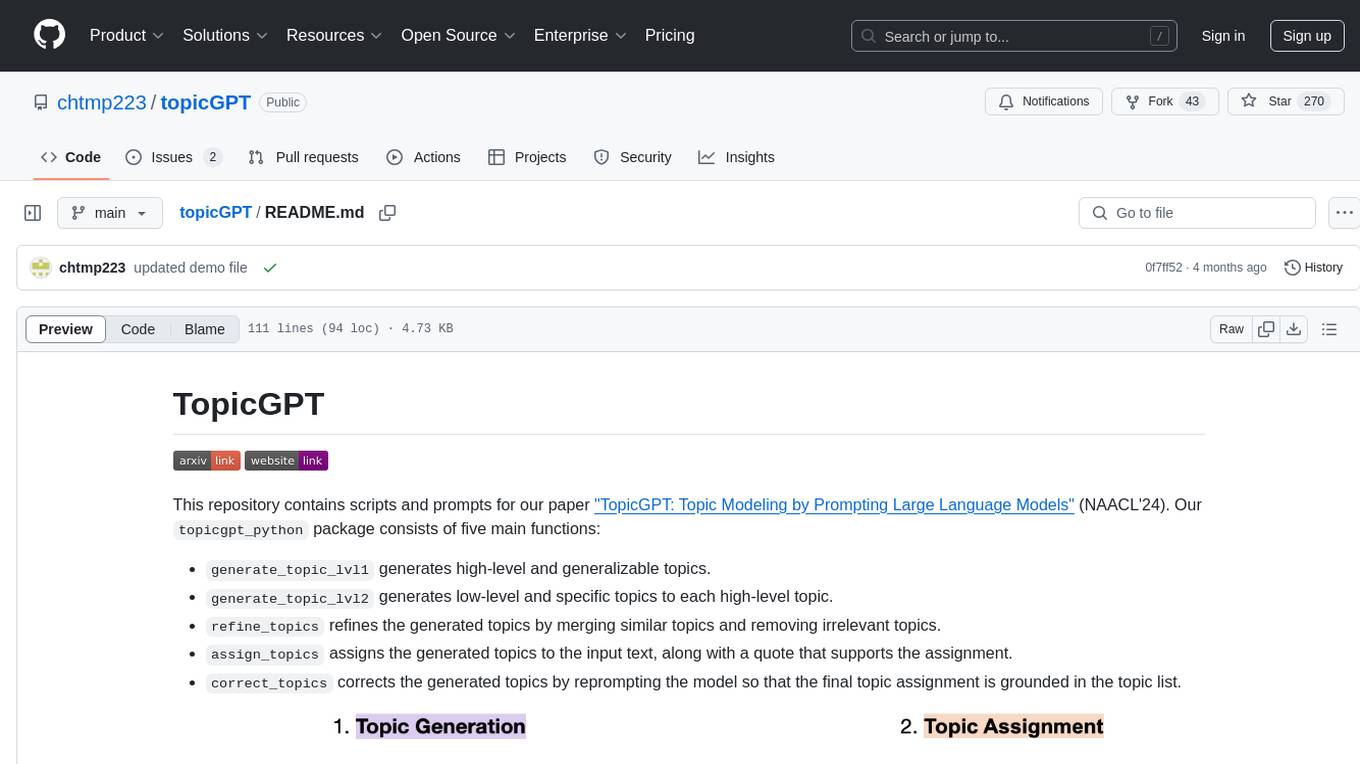
topicGPT
TopicGPT is a repository containing scripts and prompts for the paper 'TopicGPT: Topic Modeling by Prompting Large Language Models' (NAACL'24). The 'topicgpt_python' package offers functions to generate high-level and specific topics, refine topics, assign topics to input text, and correct generated topics. It supports various APIs like OpenAI, VertexAI, Azure, Gemini, and vLLM for inference. Users can prepare data in JSONL format, run the pipeline using provided scripts, and evaluate topic alignment with ground-truth labels.
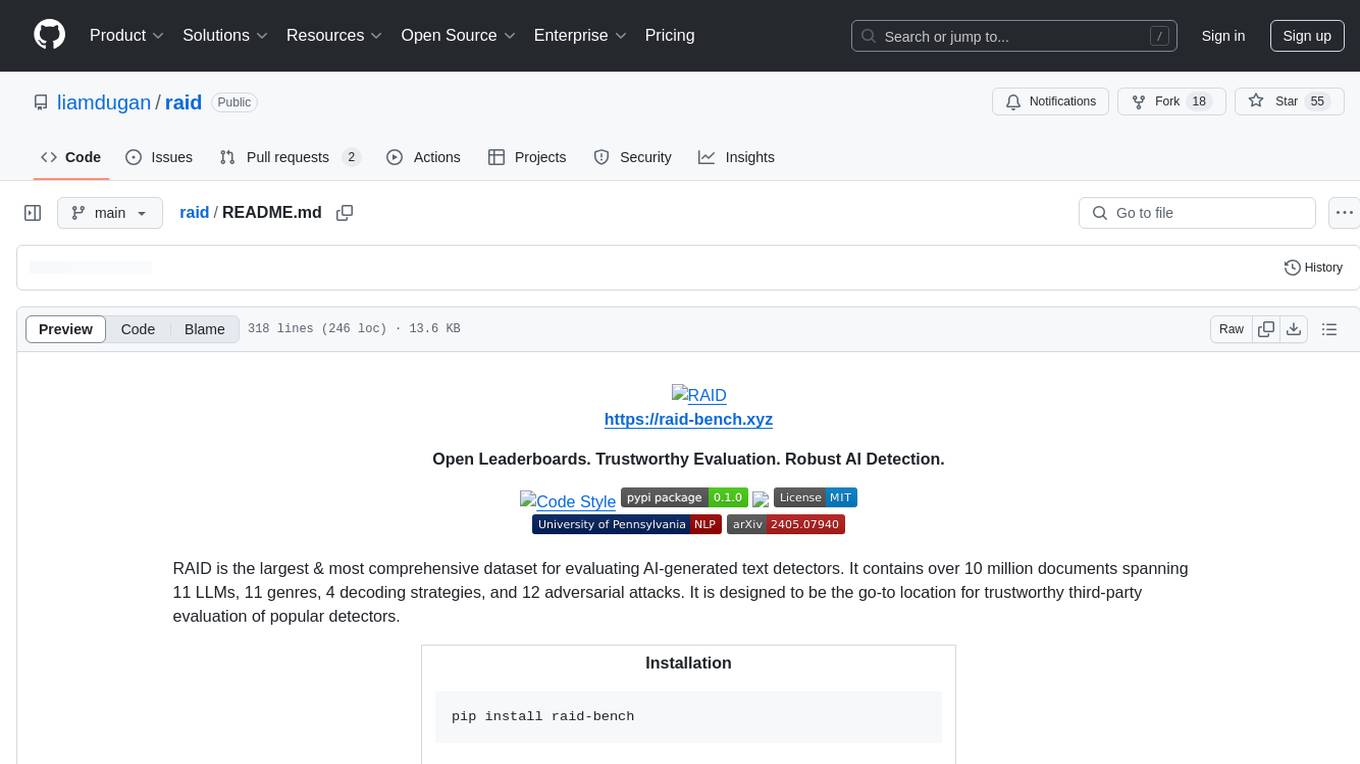
raid
RAID is the largest and most comprehensive dataset for evaluating AI-generated text detectors. It contains over 10 million documents spanning 11 LLMs, 11 genres, 4 decoding strategies, and 12 adversarial attacks. RAID is designed to be the go-to location for trustworthy third-party evaluation of popular detectors. The dataset covers diverse models, domains, sampling strategies, and attacks, making it a valuable resource for training detectors, evaluating generalization, protecting against adversaries, and comparing to state-of-the-art models from academia and industry.
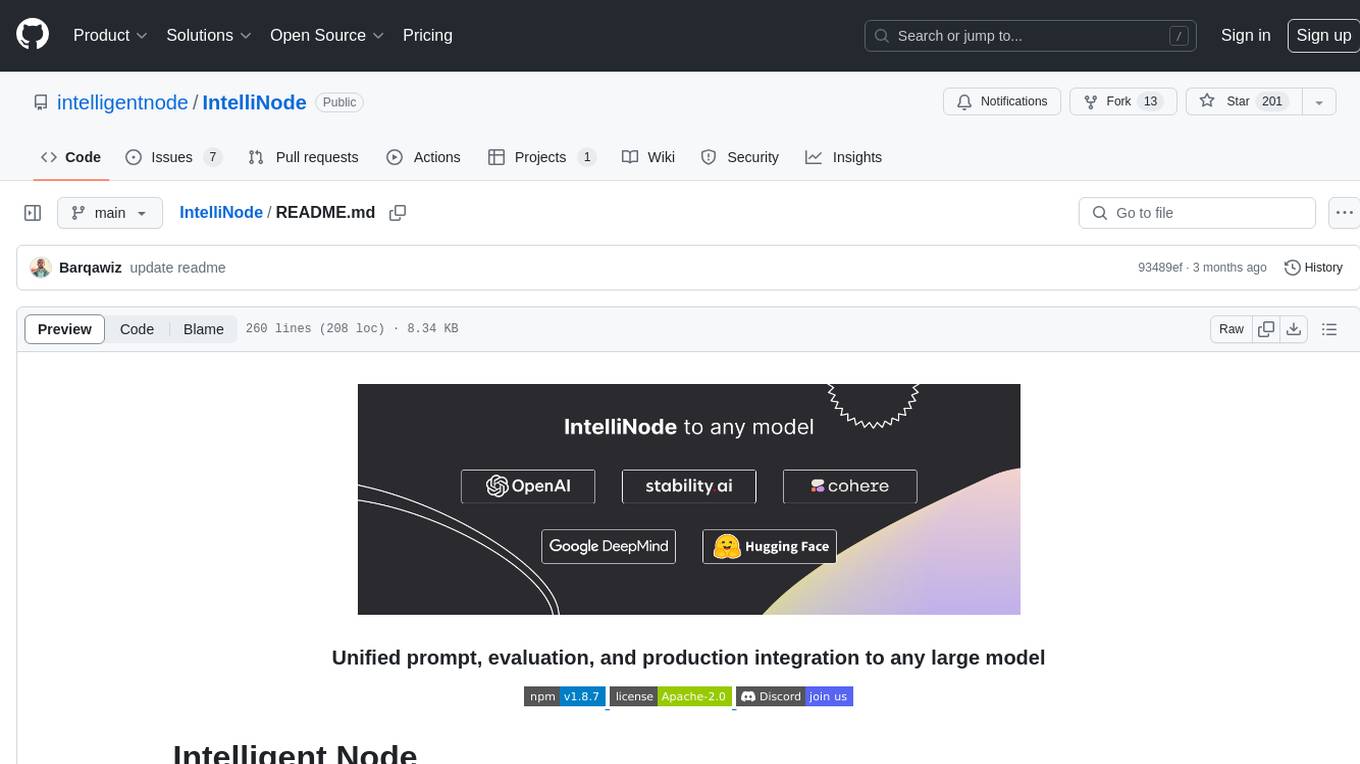
IntelliNode
IntelliNode is a javascript module that integrates cutting-edge AI models like ChatGPT, LLaMA, WaveNet, Gemini, and Stable diffusion into projects. It offers functions for generating text, speech, and images, as well as semantic search, multi-model evaluation, and chatbot capabilities. The module provides a wrapper layer for low-level model access, a controller layer for unified input handling, and a function layer for abstract functionality tailored to various use cases.
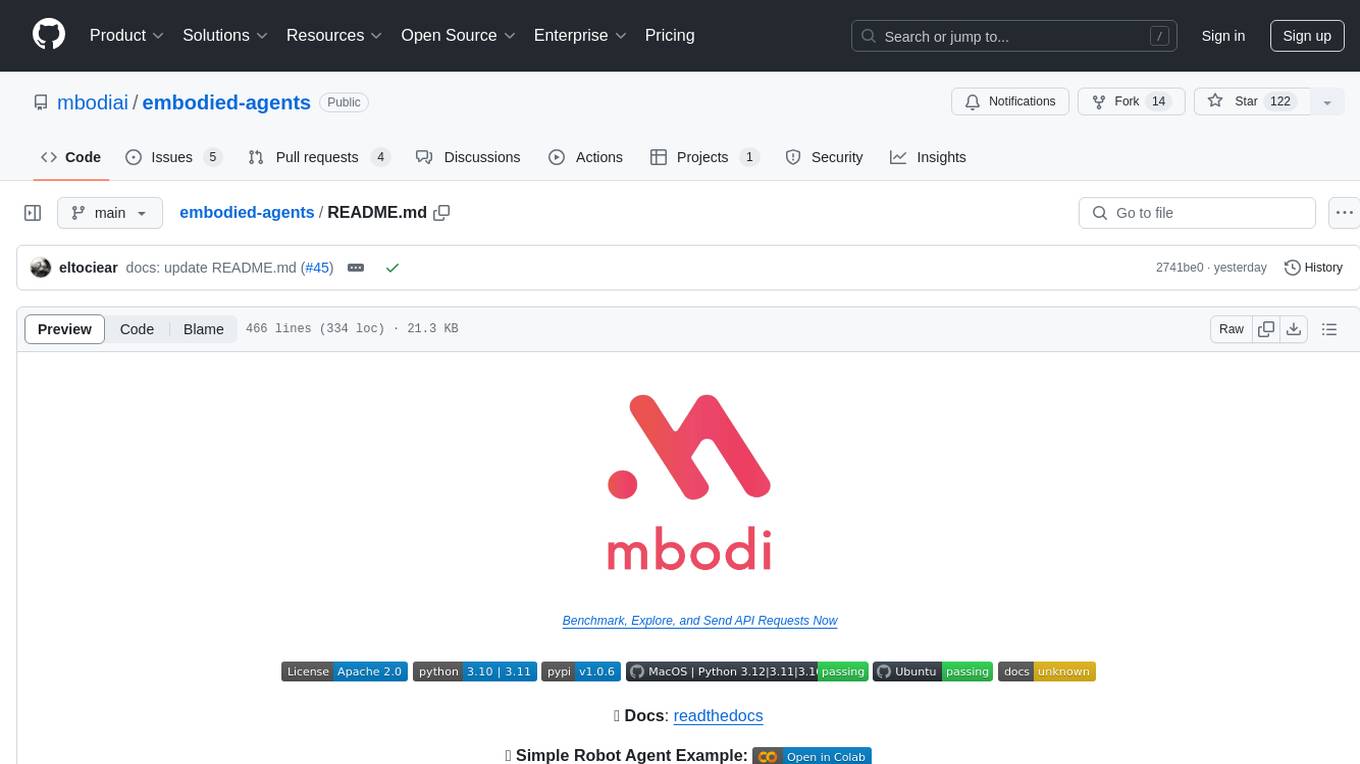
embodied-agents
Embodied Agents is a toolkit for integrating large multi-modal models into existing robot stacks with just a few lines of code. It provides consistency, reliability, scalability, and is configurable to any observation and action space. The toolkit is designed to reduce complexities involved in setting up inference endpoints, converting between different model formats, and collecting/storing datasets. It aims to facilitate data collection and sharing among roboticists by providing Python-first abstractions that are modular, extensible, and applicable to a wide range of tasks. The toolkit supports asynchronous and remote thread-safe agent execution for maximal responsiveness and scalability, and is compatible with various APIs like HuggingFace Spaces, Datasets, Gymnasium Spaces, Ollama, and OpenAI. It also offers automatic dataset recording and optional uploads to the HuggingFace hub.
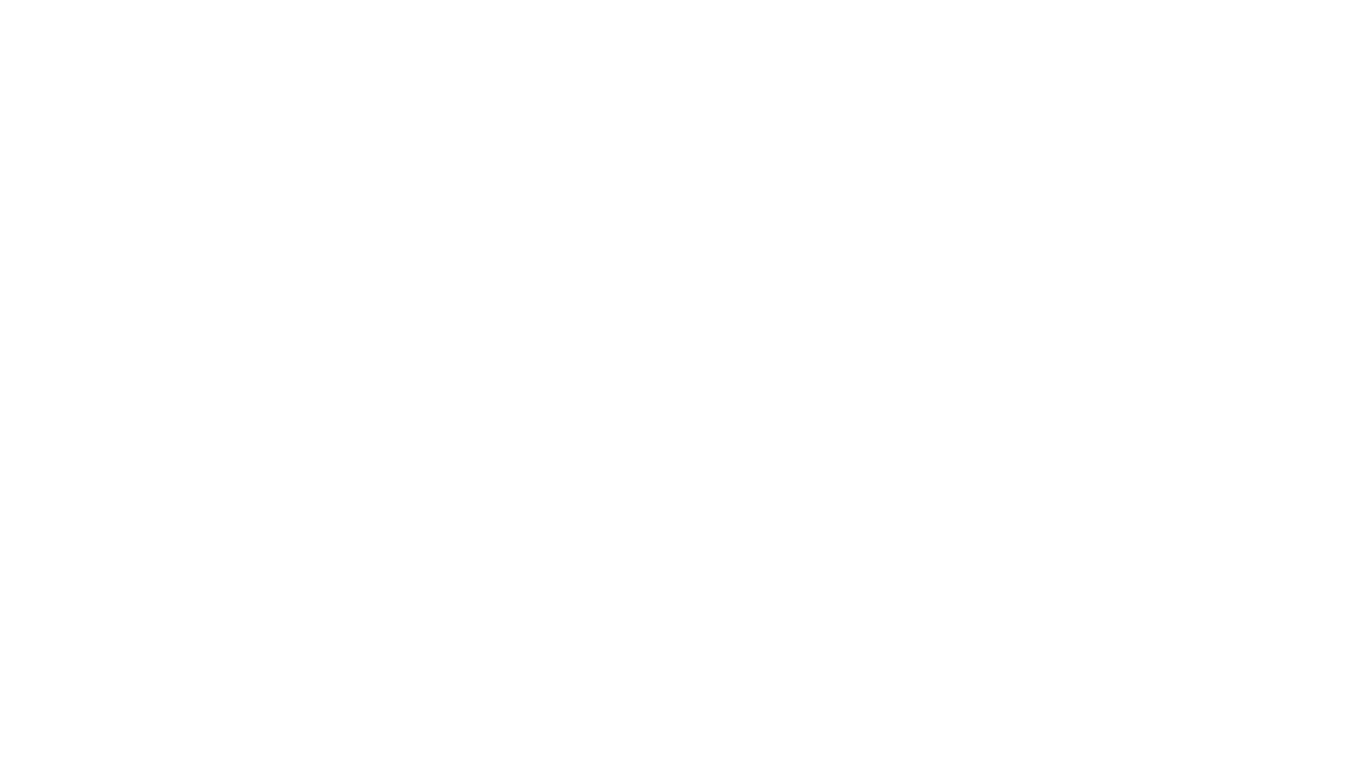
upgini
Upgini is an intelligent data search engine with a Python library that helps users find and add relevant features to their ML pipeline from various public, community, and premium external data sources. It automates the optimization of connected data sources by generating an optimal set of machine learning features using large language models, GraphNNs, and recurrent neural networks. The tool aims to simplify feature search and enrichment for external data to make it a standard approach in machine learning pipelines. It democratizes access to data sources for the data science community.
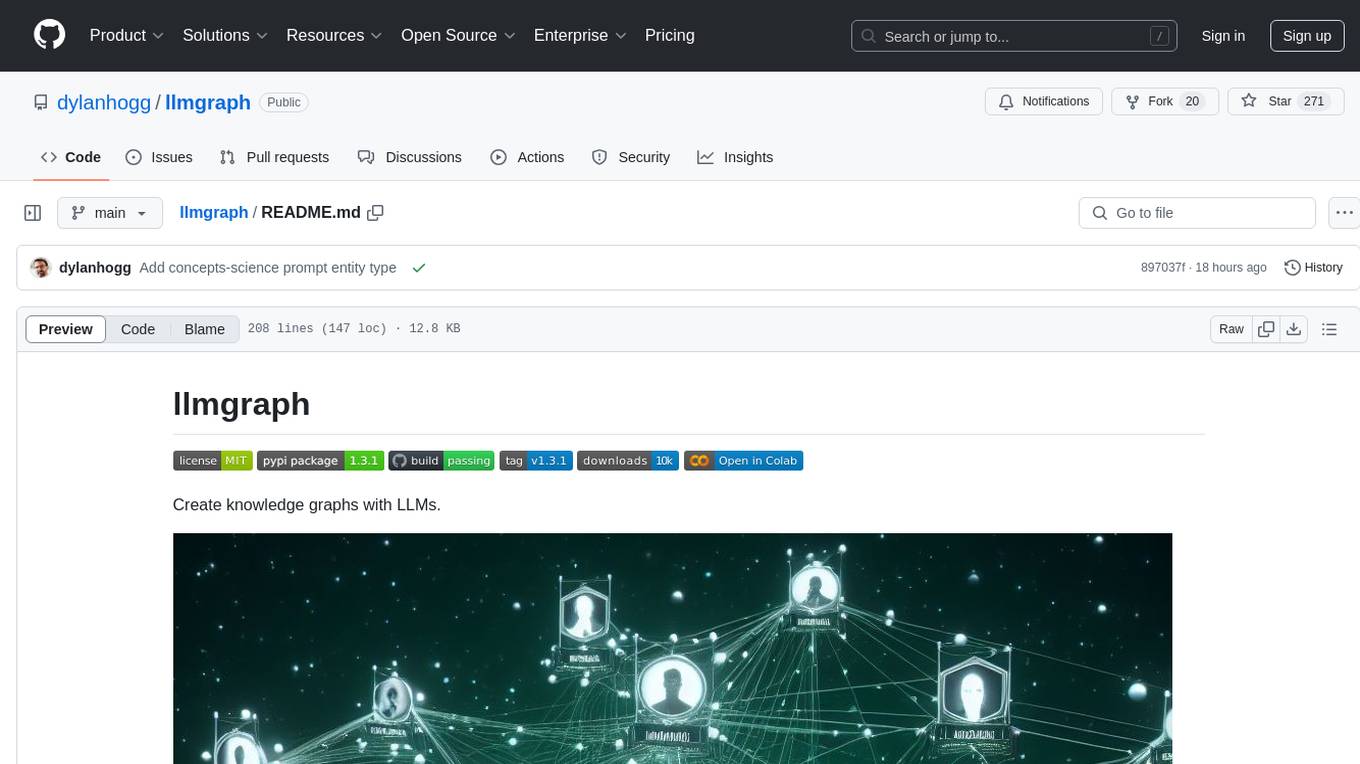
llmgraph
llmgraph is a tool that enables users to create knowledge graphs in GraphML, GEXF, and HTML formats by extracting world knowledge from large language models (LLMs) like ChatGPT. It supports various entity types and relationships, offers cache support for efficient graph growth, and provides insights into LLM costs. Users can customize the model used and interact with different LLM providers. The tool allows users to generate interactive graphs based on a specified entity type and Wikipedia link, making it a valuable resource for knowledge graph creation and exploration.
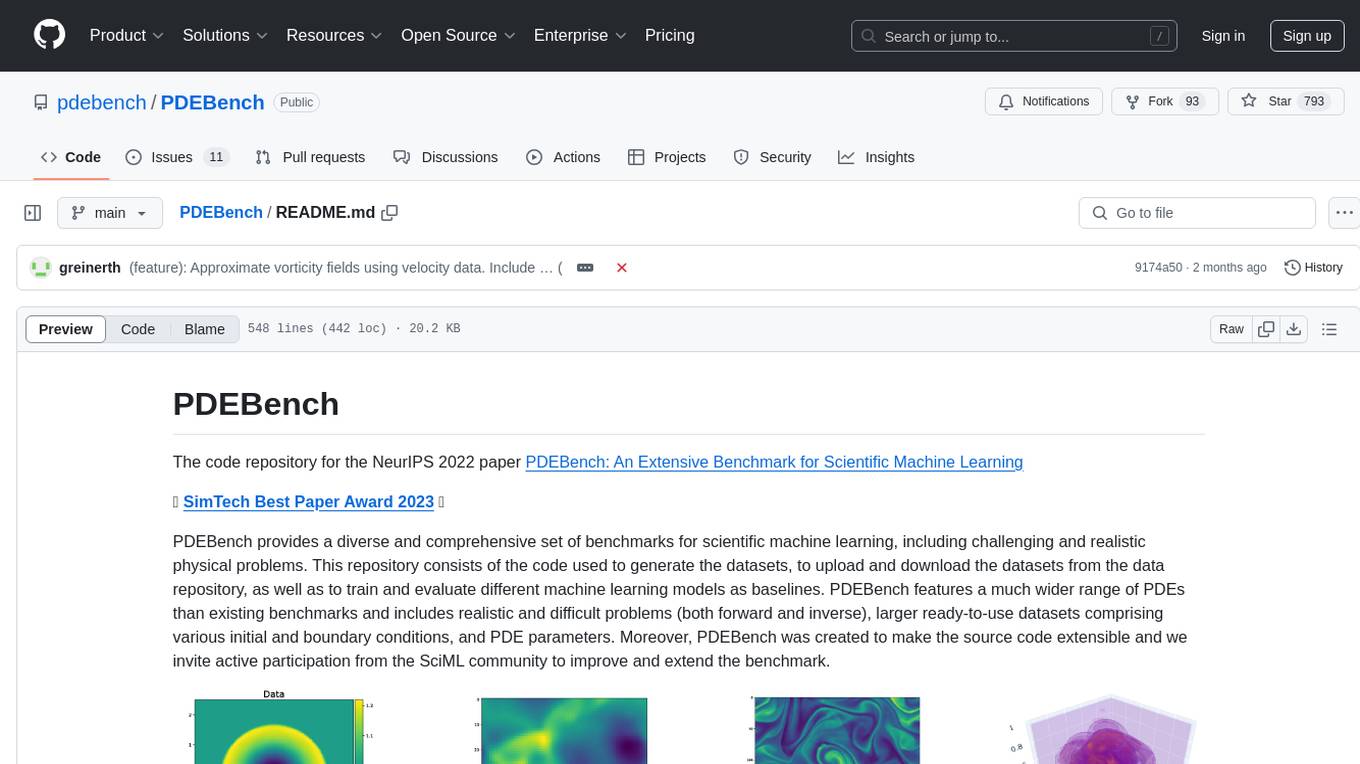
PDEBench
PDEBench provides a diverse and comprehensive set of benchmarks for scientific machine learning, including challenging and realistic physical problems. The repository consists of code for generating datasets, uploading and downloading datasets, training and evaluating machine learning models as baselines. It features a wide range of PDEs, realistic and difficult problems, ready-to-use datasets with various conditions and parameters. PDEBench aims for extensibility and invites participation from the SciML community to improve and extend the benchmark.
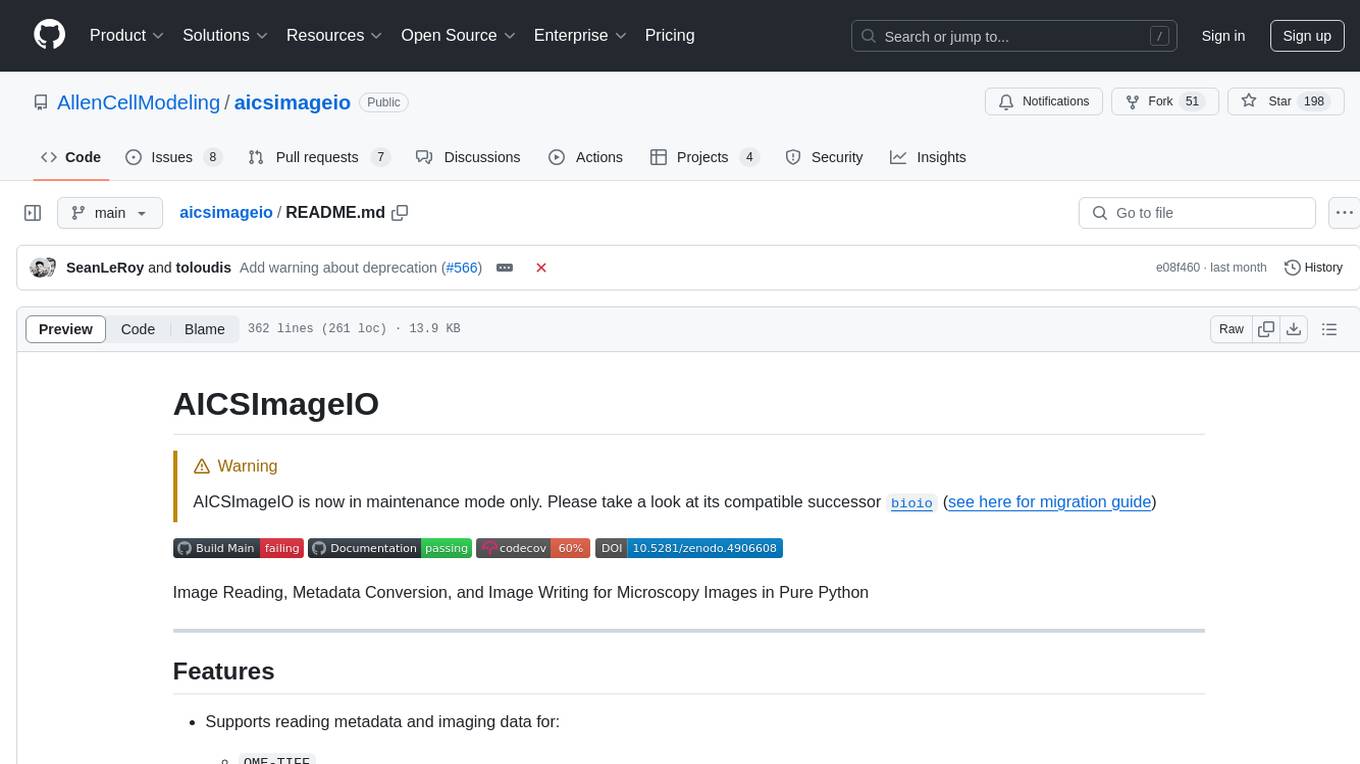
aicsimageio
AICSImageIO is a Python tool for Image Reading, Metadata Conversion, and Image Writing for Microscopy Images. It supports various file formats like OME-TIFF, TIFF, ND2, DV, CZI, LIF, PNG, GIF, and Bio-Formats. Users can read and write metadata and imaging data, work with different file systems like local paths, HTTP URLs, s3fs, and gcsfs. The tool provides functionalities for full image reading, delayed image reading, mosaic image reading, metadata reading, xarray coordinate plane attachment, cloud IO support, and saving to OME-TIFF. It also offers benchmarking and developer resources.
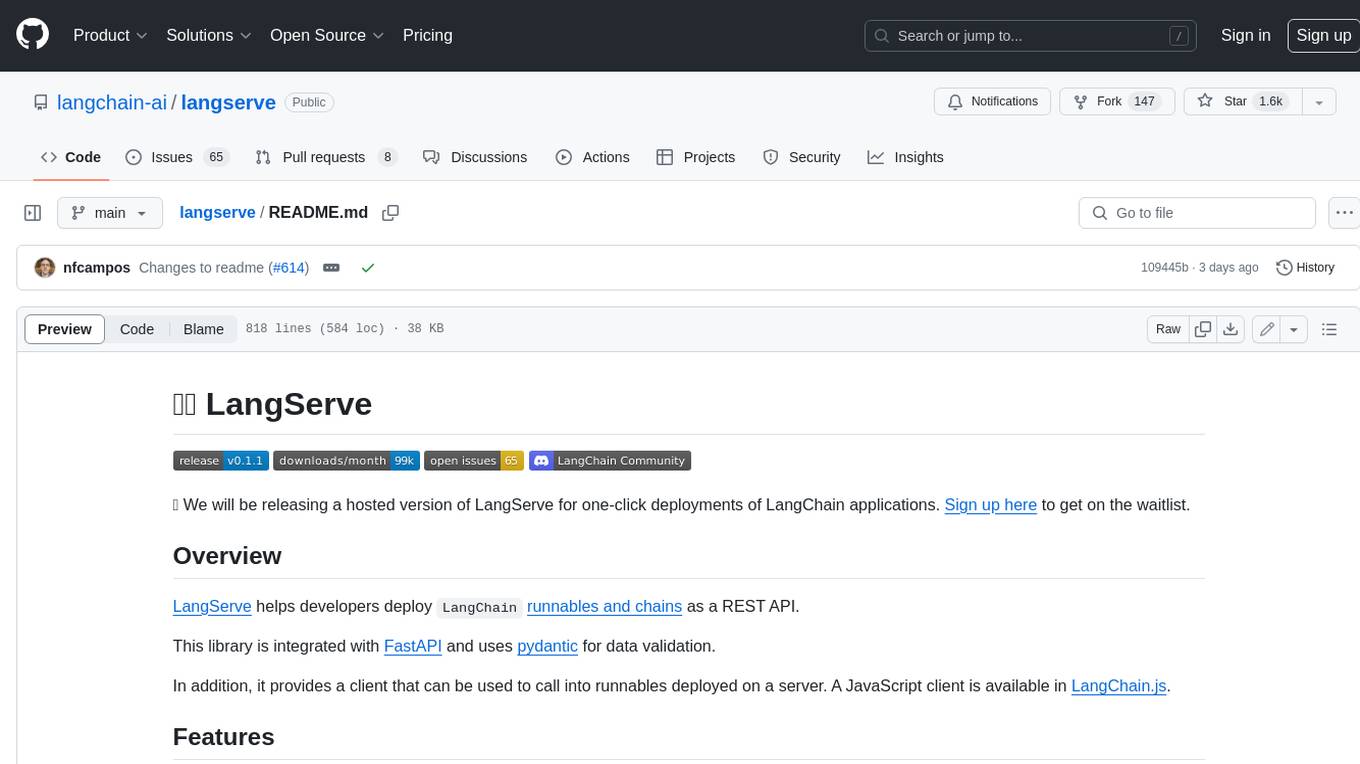
langserve
LangServe helps developers deploy `LangChain` runnables and chains as a REST API. This library is integrated with FastAPI and uses pydantic for data validation. In addition, it provides a client that can be used to call into runnables deployed on a server. A JavaScript client is available in LangChain.js.
For similar tasks
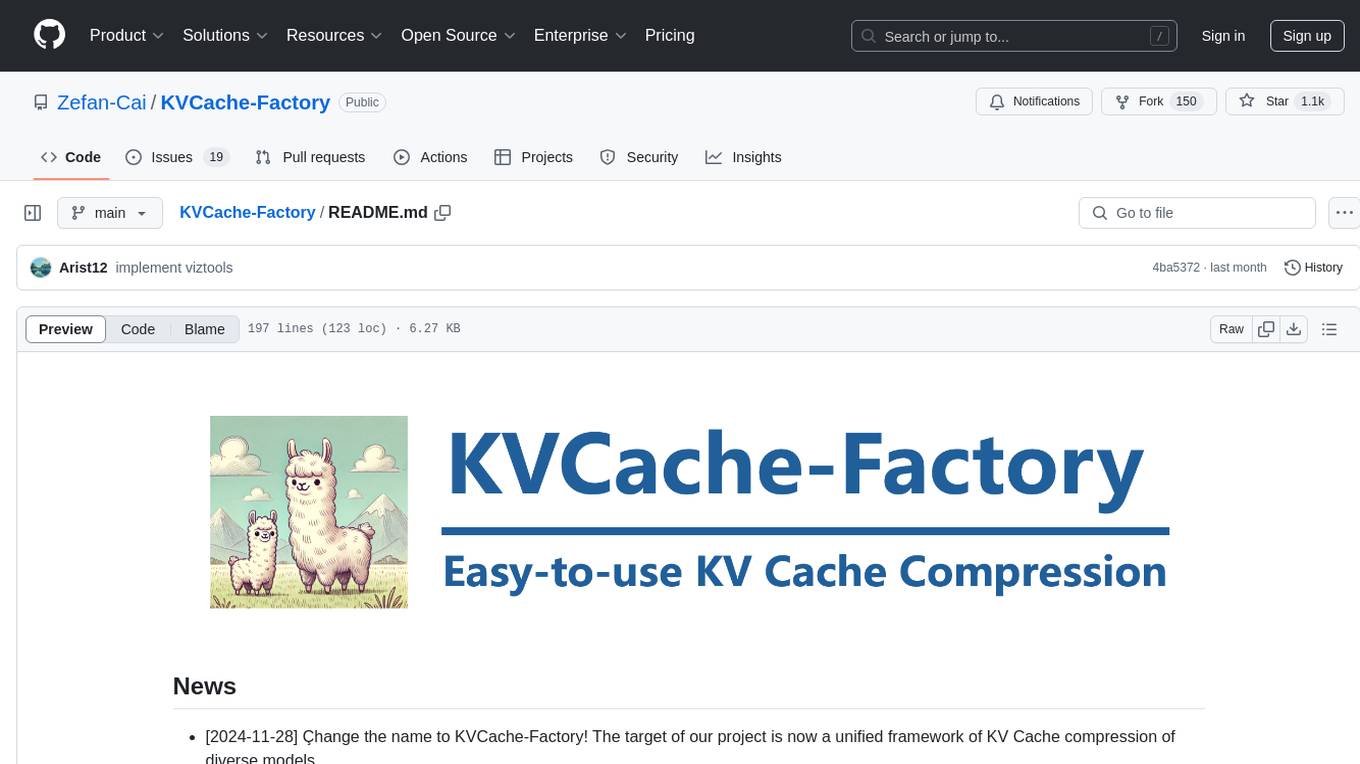
KVCache-Factory
KVCache-Factory is a unified framework for KV Cache compression of diverse models. It supports multi-GPUs inference with big LLMs and various attention implementations. The tool enables KV cache compression without Flash Attention v2, multi-GPU inference, and specific models like Mistral. It also provides functions for KV cache budget allocation and batch inference. The visualization tools help in understanding the attention patterns of models.
For similar jobs
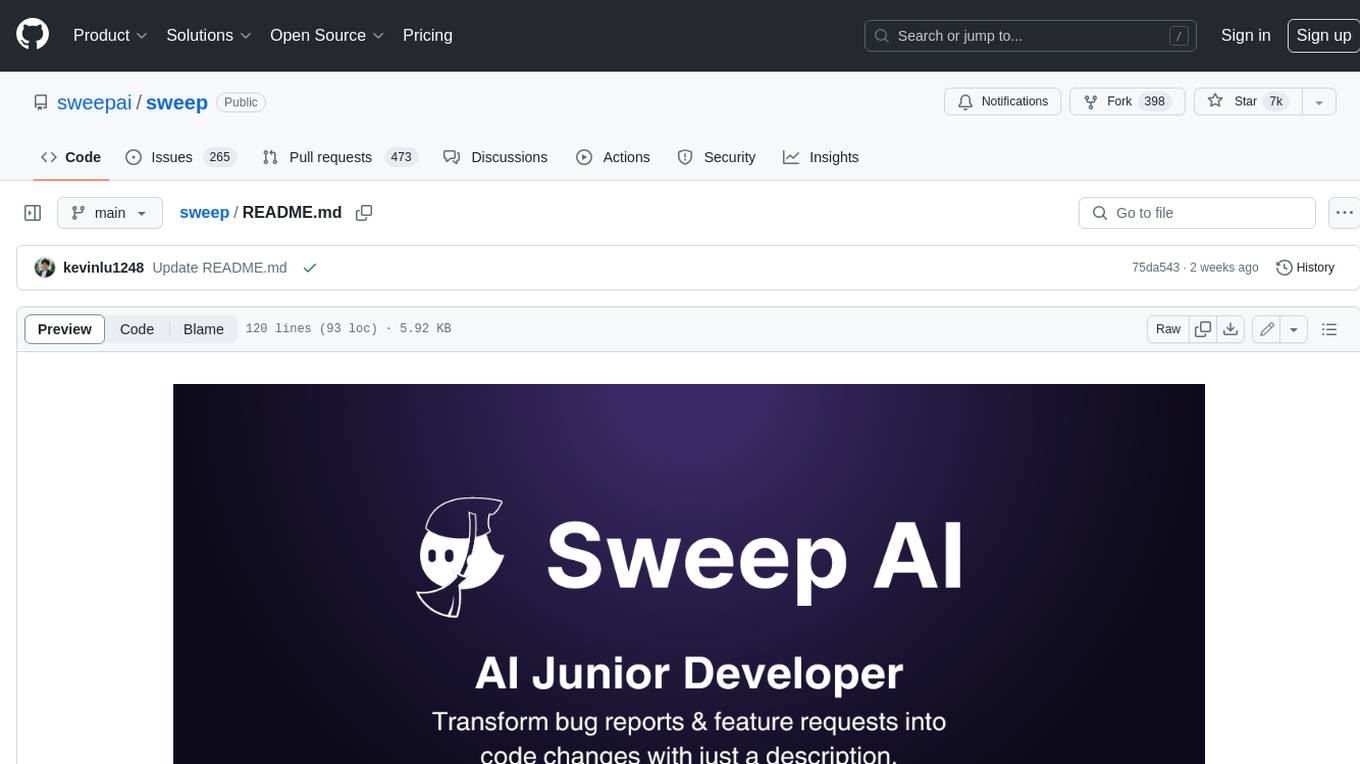
sweep
Sweep is an AI junior developer that turns bugs and feature requests into code changes. It automatically handles developer experience improvements like adding type hints and improving test coverage.
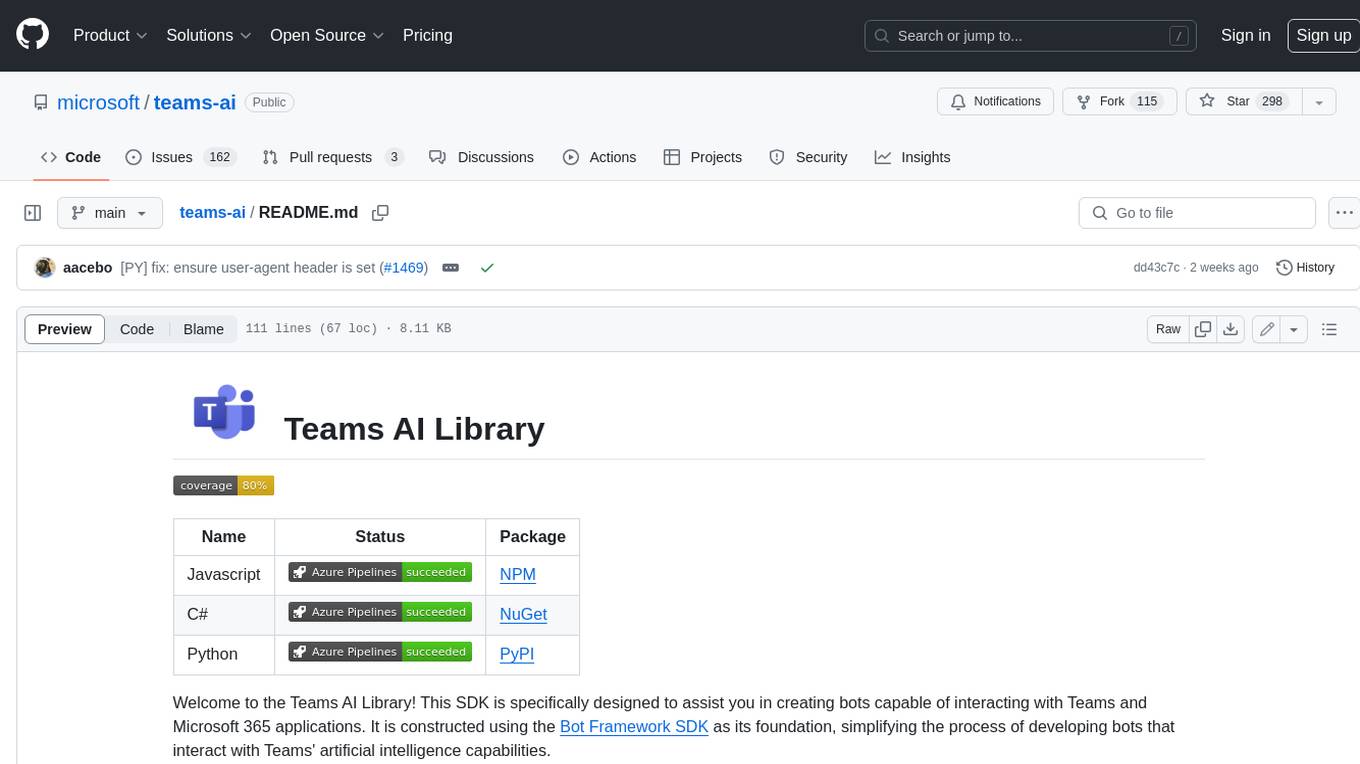
teams-ai
The Teams AI Library is a software development kit (SDK) that helps developers create bots that can interact with Teams and Microsoft 365 applications. It is built on top of the Bot Framework SDK and simplifies the process of developing bots that interact with Teams' artificial intelligence capabilities. The SDK is available for JavaScript/TypeScript, .NET, and Python.
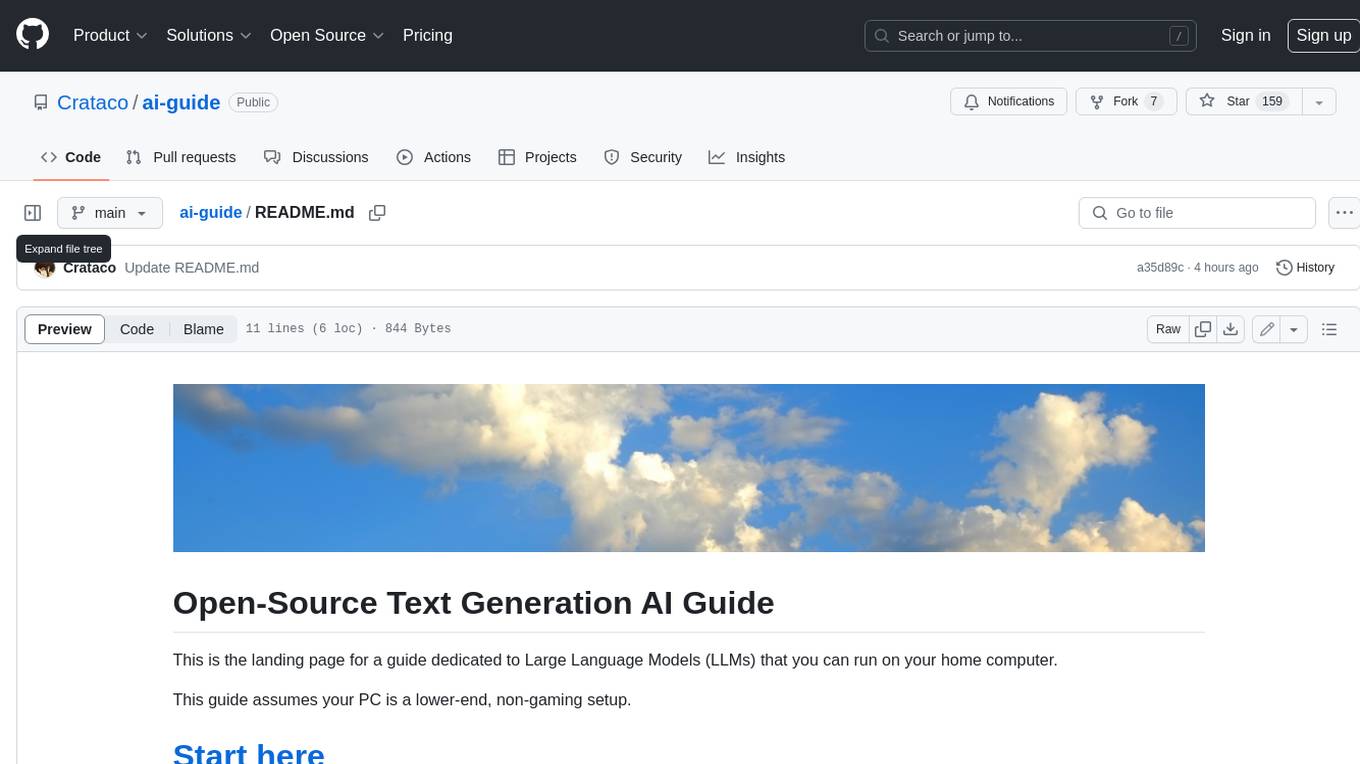
ai-guide
This guide is dedicated to Large Language Models (LLMs) that you can run on your home computer. It assumes your PC is a lower-end, non-gaming setup.
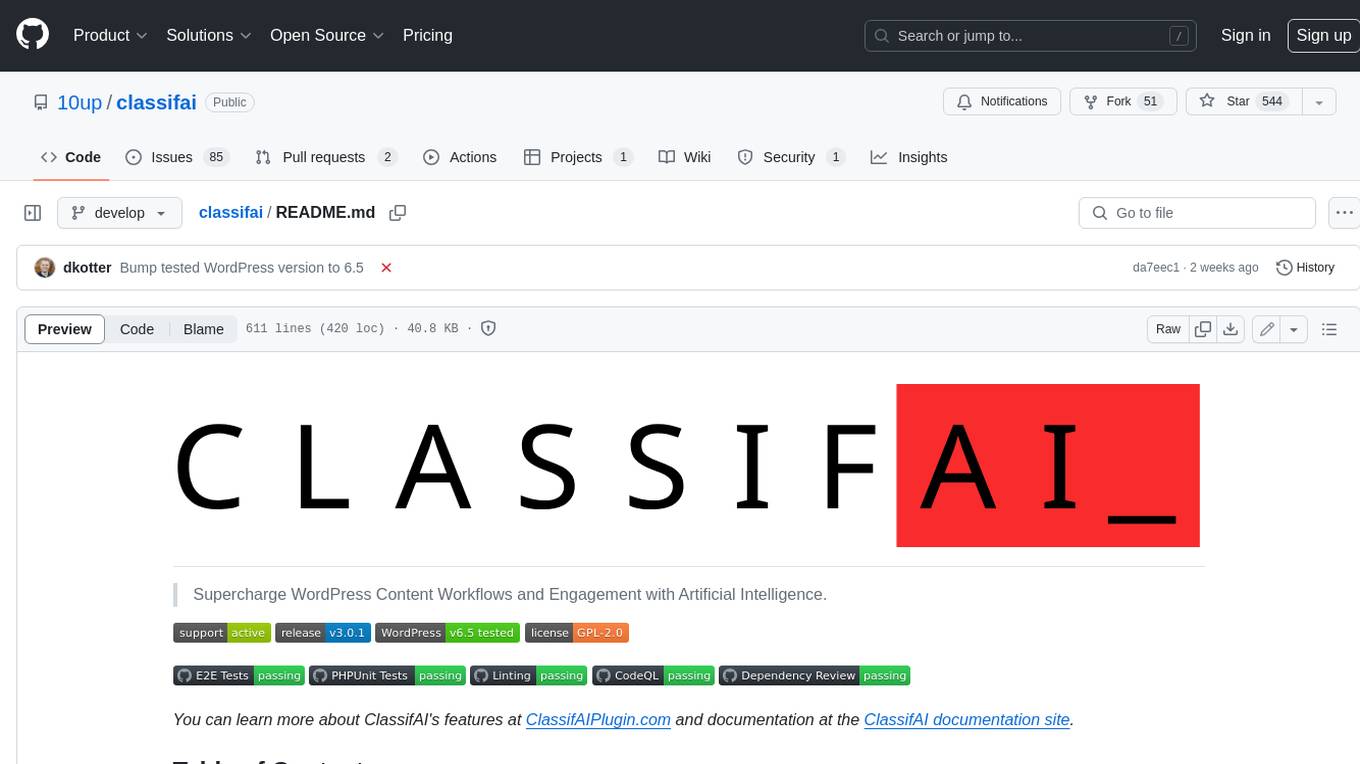
classifai
Supercharge WordPress Content Workflows and Engagement with Artificial Intelligence. Tap into leading cloud-based services like OpenAI, Microsoft Azure AI, Google Gemini and IBM Watson to augment your WordPress-powered websites. Publish content faster while improving SEO performance and increasing audience engagement. ClassifAI integrates Artificial Intelligence and Machine Learning technologies to lighten your workload and eliminate tedious tasks, giving you more time to create original content that matters.
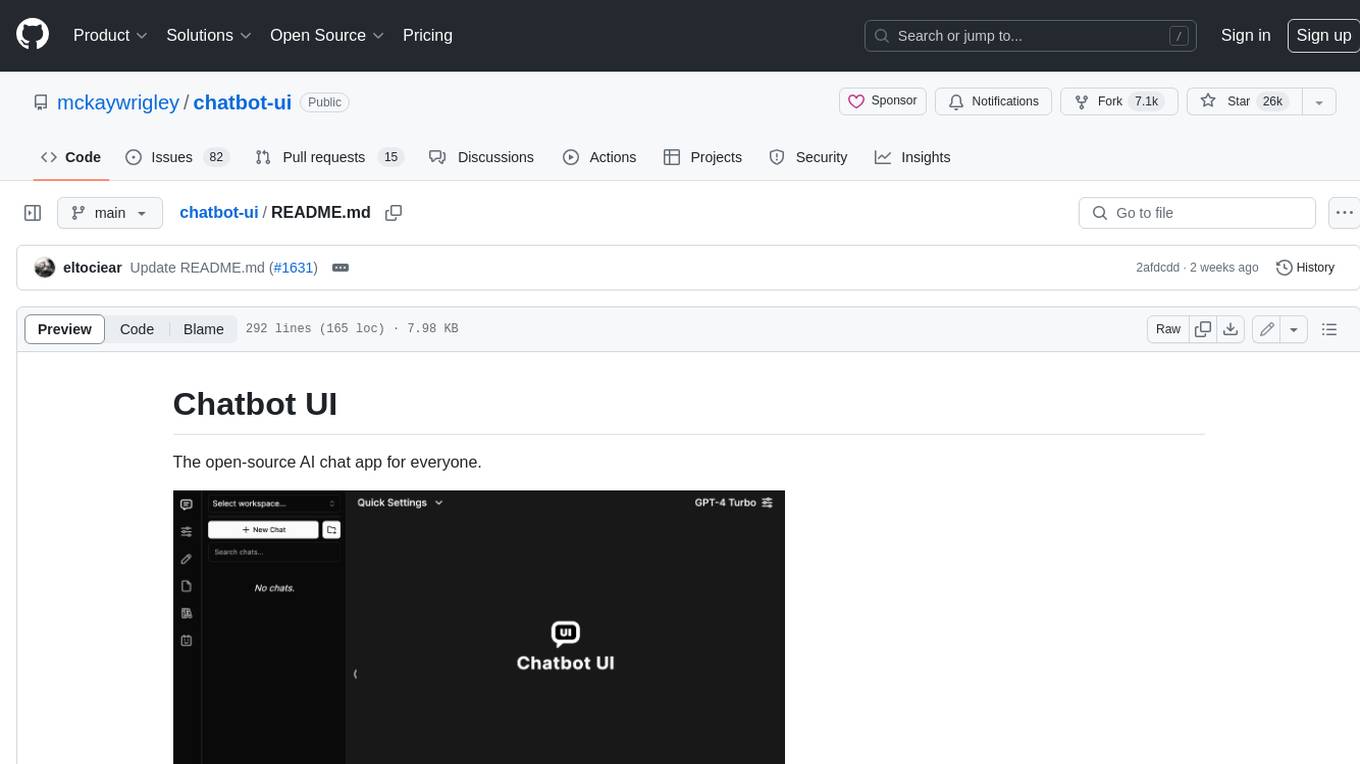
chatbot-ui
Chatbot UI is an open-source AI chat app that allows users to create and deploy their own AI chatbots. It is easy to use and can be customized to fit any need. Chatbot UI is perfect for businesses, developers, and anyone who wants to create a chatbot.
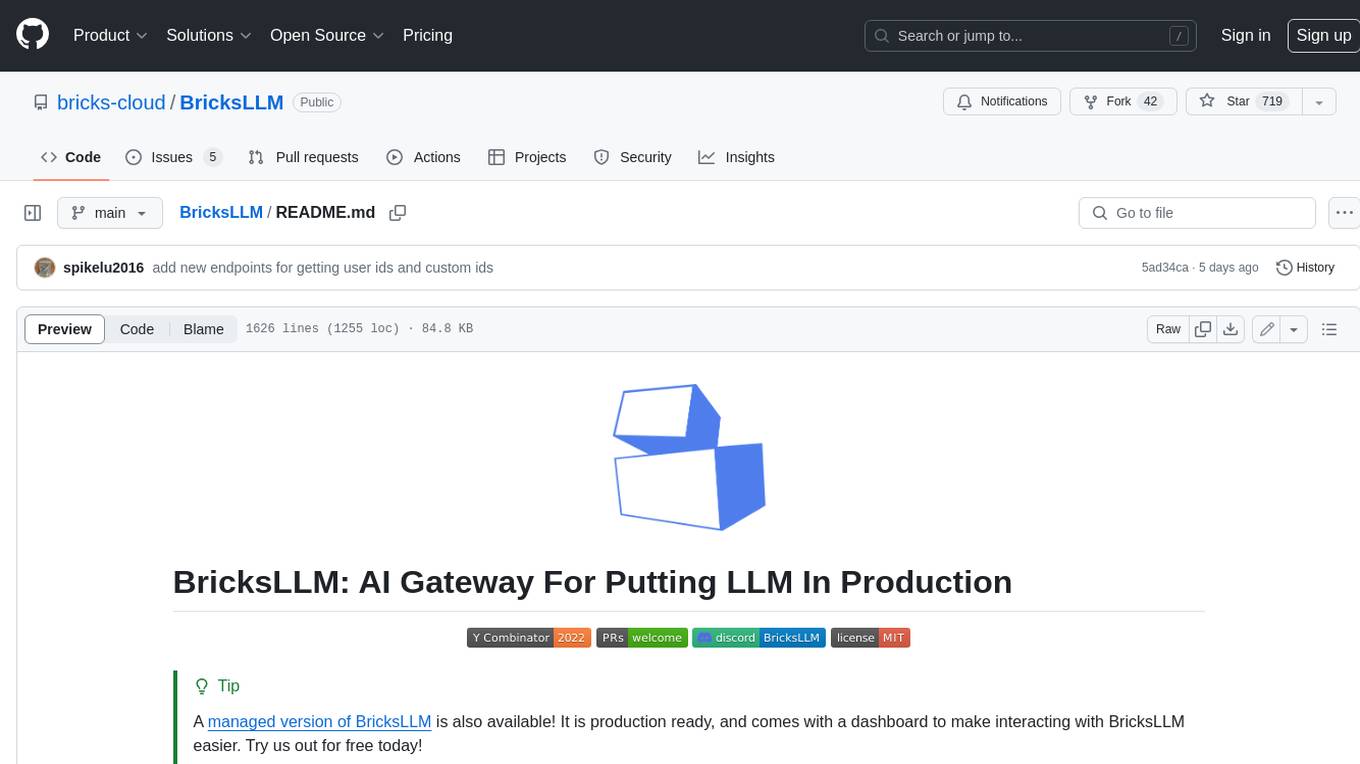
BricksLLM
BricksLLM is a cloud native AI gateway written in Go. Currently, it provides native support for OpenAI, Anthropic, Azure OpenAI and vLLM. BricksLLM aims to provide enterprise level infrastructure that can power any LLM production use cases. Here are some use cases for BricksLLM: * Set LLM usage limits for users on different pricing tiers * Track LLM usage on a per user and per organization basis * Block or redact requests containing PIIs * Improve LLM reliability with failovers, retries and caching * Distribute API keys with rate limits and cost limits for internal development/production use cases * Distribute API keys with rate limits and cost limits for students
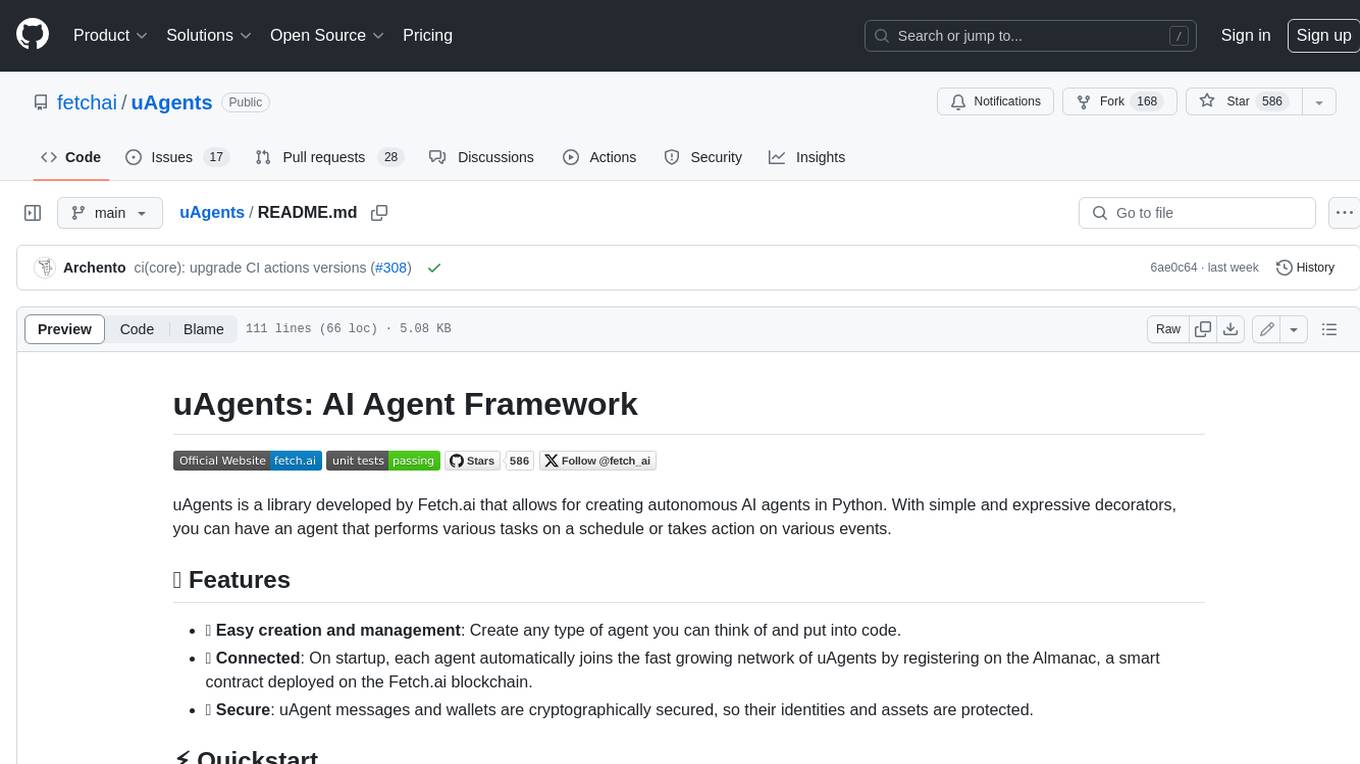
uAgents
uAgents is a Python library developed by Fetch.ai that allows for the creation of autonomous AI agents. These agents can perform various tasks on a schedule or take action on various events. uAgents are easy to create and manage, and they are connected to a fast-growing network of other uAgents. They are also secure, with cryptographically secured messages and wallets.
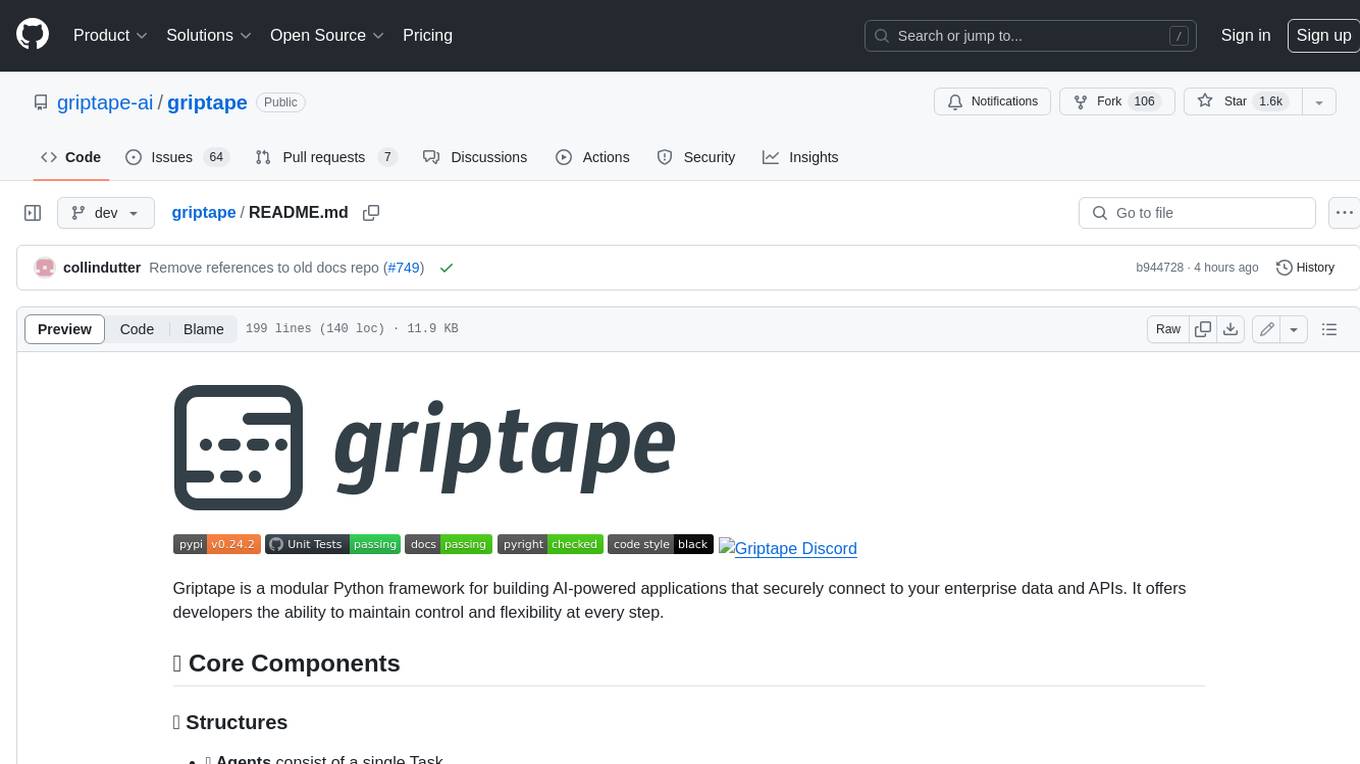
griptape
Griptape is a modular Python framework for building AI-powered applications that securely connect to your enterprise data and APIs. It offers developers the ability to maintain control and flexibility at every step. Griptape's core components include Structures (Agents, Pipelines, and Workflows), Tasks, Tools, Memory (Conversation Memory, Task Memory, and Meta Memory), Drivers (Prompt and Embedding Drivers, Vector Store Drivers, Image Generation Drivers, Image Query Drivers, SQL Drivers, Web Scraper Drivers, and Conversation Memory Drivers), Engines (Query Engines, Extraction Engines, Summary Engines, Image Generation Engines, and Image Query Engines), and additional components (Rulesets, Loaders, Artifacts, Chunkers, and Tokenizers). Griptape enables developers to create AI-powered applications with ease and efficiency.