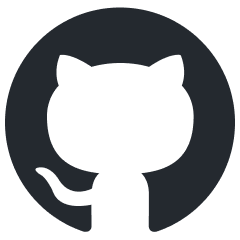
upgini
Data search & enrichment library for Machine Learning → Easily find and add relevant features to your ML & AI pipeline from hundreds of public and premium external data sources, including open & commercial LLMs
Stars: 327
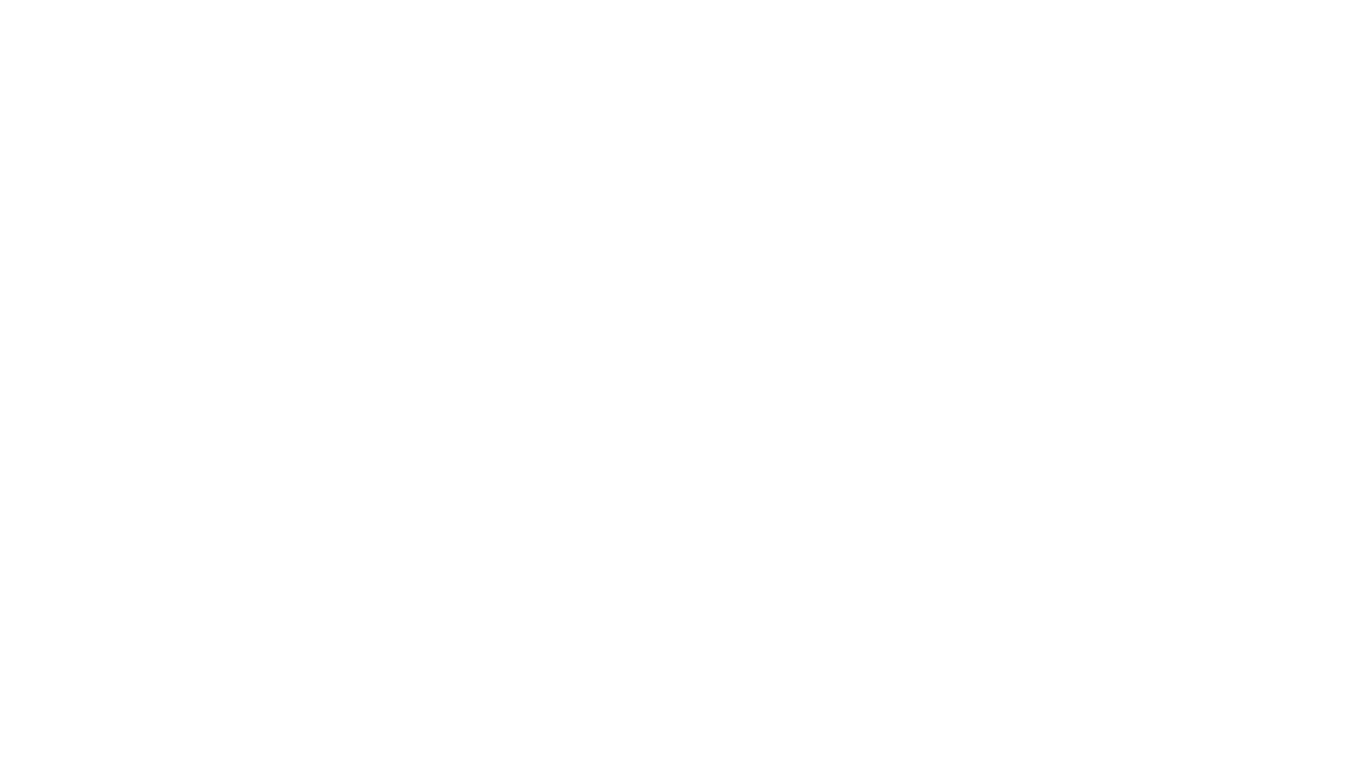
Upgini is an intelligent data search engine with a Python library that helps users find and add relevant features to their ML pipeline from various public, community, and premium external data sources. It automates the optimization of connected data sources by generating an optimal set of machine learning features using large language models, GraphNNs, and recurrent neural networks. The tool aims to simplify feature search and enrichment for external data to make it a standard approach in machine learning pipelines. It democratizes access to data sources for the data science community.
README:
Easily find and add relevant features to your ML & AI pipeline from hundreds of public, community and premium external data sources, including open & commercial LLMs
Quick Start in Colab » |
Register / Sign In |
Slack Community |
Propose new Data source
Upgini is an intelligent data search engine with a Python library that helps you find and add relevant features to your ML pipeline from hundreds of public, community, and premium external data sources. Under the hood, Upgini automatically optimizes all connected data sources by generating an optimal set of machine ML features using large language models (LLMs), GraphNNs and recurrent neural networks (RNNs).
Motivation: for most supervised ML models external data & features boost accuracy significantly better than any hyperparameters tuning. But lack of automated and time-efficient enrichment tools for external data blocks massive adoption of external features in ML pipelines. We want radically simplify features search and enrichment to make external data a standard approach. Like a hyperparameter tuning for machine learning nowadays.
Mission: Democratize access to data sources for data science community.
⭐️ Automatically find only relevant features that give accuracy improvement for ML model. Not just correlated with target variable, what 9 out of 10 cases gives zero accuracy improvement
⭐️ Automated feature generation from the sources: feature generation with Large Language Models' data augmentation, RNNs, GraphNN; multiple data source ensembling
⭐️ Automatic search key augmentation from all connected sources. If you do not have all search keys in your search request, such as postal/zip code, Upgini will try to add those keys based on the provided set of search keys. This will broaden the search across all available data sources
⭐️ Calculate accuracy metrics and uplifts after enrichment existing ML model with external features
⭐️ Check the stability of accuracy gain from external data on out-of-time intervals and verification datasets. Mitigate risks of unstable external data dependencies in ML pipeline
⭐️ Easy to use - single request to enrich training dataset with all of the keys at once:
date / datetime | phone number |
postal / ZIP code | hashed email / HEM |
country | IP-address |
⭐️ Scikit-learn compatible interface for quick data integration with existing ML pipelines
⭐️ Support for most common supervised ML tasks on tabular data:
☑️ binary classification | ☑️ multiclass classification |
☑️ regression | ☑️ time series prediction |
⭐️ Simple Drag & Drop Search UI:
- Public data : public sector, academic institutions, other sources through open data portals. Curated and updated by the Upgini team
- Community shared data: royalty / license free datasets or features from Data science community (our users). It's both a public and a scraped data
- Premium data providers: commercial data sources verified by the Upgini team in real-world use cases
👉 Details on datasets and features
Data sources | Countries | History, years | # sources for ensemble | Update | Search keys | API Key required |
---|---|---|---|---|---|---|
Historical weather & Climate normals | 68 | 22 | - | Monthly | date, country, postal/ZIP code | No |
Location/Places/POI/Area/Proximity information from OpenStreetMap | 221 | 2 | - | Monthly | date, country, postal/ZIP code | No |
International holidays & events, Workweek calendar | 232 | 22 | - | Monthly | date, country | No |
Consumer Confidence index | 44 | 22 | - | Monthly | date, country | No |
World economic indicators | 191 | 41 | - | Monthly | date, country | No |
Markets data | - | 17 | - | Monthly | date, datetime | No |
World mobile & fixed broadband network coverage and performance | 167 | - | 3 | Monthly | country, postal/ZIP code | No |
World demographic data | 90 | - | 2 | Annual | country, postal/ZIP code | No |
World house prices | 44 | - | 3 | Annual | country, postal/ZIP code | No |
Public social media profile data | 104 | - | - | Monthly | date, email/HEM, phone | Yes |
Car ownership data and Parking statistics | 3 | - | - | Annual | country, postal/ZIP code, email/HEM, phone | Yes |
Geolocation profile for phone & IPv4 & email | 239 | - | 6 | Monthly | date, email/HEM, phone, IPv4 | Yes |
🔜 Email/WWW domain profile | - | - | - | - |
❓Know other useful data sources for machine learning? Give us a hint and we'll add it for free.
Search of relevant external features & Automated feature generation for Salary prediction task (use as a template)
- The goal is to predict salary for data science job postning based on information about employer and job description.
- Following this guide, you'll learn how to search & auto generate new relevant features with Upgini library
- The evaluation metric is Mean Absolute Error (MAE).
Run Feature search & generation notebook inside your browser:
- The goal is to predict future sales of different goods in stores based on a 5-year history of sales.
- Kaggle Competition Store Item Demand Forecasting Challenge is a product sales forecasting. The evaluation metric is SMAPE.
Run Simple sales prediction for retail stores inside your browser:
- The goal is accuracy improvement for TOP1 winning Kaggle solution from new relevant external features & data.
- Kaggle Competition is a product sales forecasting, evaluation metric is SMAPE.
- Save time on feature search and engineering. Use ready-to-use external features and data sources to maximize overall AutoML accuracy, right out of the box.
- Kaggle Competition is a product sales forecasting, evaluation metric is SMAPE.
- Low-code AutoML tools: Upgini and PyCaret
- The goal is accuracy improvement of Multivariate Time Series prediction from new relevant external features & data. The main challenge here is a strategy of data & feature enrichment, when a component of Multivariate TS depends not only on its past values but also has some dependency on other components.
- Kaggle Competition is a product sales forecasting, evaluation metric is RMSLE.
- Save time on external data wrangling and feature calculation code for hypothesis tests. The key challenge here is a time-dependent representation of information in a training dataset, which is uncommon for credit default prediction tasks. As a result, special data enrichment strategy is used.
- Kaggle Competition is a credit default prediction, evaluation metric is normalized Gini coefficient.
%pip install upgini
🐳 Docker-way
Clone $ git clone https://github.com/upgini/upgini or download upgini git repo locally and follow steps below to build docker container 👇 1. Build docker image from cloned git repo: cd upgini docker build -t upgini . ...or directly from GitHub: DOCKER_BUILDKIT=0 docker build -t upgini [email protected]:upgini/upgini.git#main 2. Run docker image: docker run -p 8888:8888 upgini 3. Open http://localhost:8888?token="<"your_token_from_console_output">" in your browserYou can use your labeled training datasets "as is" to initiate the search. Under the hood, we'll search for relevant data using:
- search keys from training dataset to match records from potential data sources with a new features
- labels from training dataset to estimate relevancy of feature or dataset for your ML task and calculate feature importance metrics
- your features from training dataset to find external datasets and features which only give accuracy improvement to your existing data and estimate accuracy uplift (optional)
Load training dataset into pandas dataframe and separate features' columns from label column in a Scikit-learn way:
import pandas as pd
# labeled training dataset - customer_churn_prediction_train.csv
train_df = pd.read_csv("customer_churn_prediction_train.csv")
X = train_df.drop(columns="churn_flag")
y = train_df["churn_flag"]
We do dataset verification and cleaning under the hood, but still there are some requirements to follow: 1. pandas.DataFrame, pandas.Series or numpy.ndarray representation; 2. correct label column types: boolean/integers/strings for binary and multiclass labels, floats for regression; 3. at least one column selected as a search key; 4. min size after deduplication by search key column and NaNs removal: 100 records |
Search keys columns will be used to match records from all potential external data sources / features.
Define one or multiple columns as a search keys with FeaturesEnricher
class initialization.
from upgini.features_enricher import FeaturesEnricher
from upgini.metadata import SearchKey
enricher = FeaturesEnricher(
search_keys={
"subscription_activation_date": SearchKey.DATE,
"country": SearchKey.COUNTRY,
"zip_code": SearchKey.POSTAL_CODE,
"hashed_email": SearchKey.HEM,
"last_visit_ip_address": SearchKey.IP,
"registered_with_phone": SearchKey.PHONE
})
Search Key Meaning Type |
Description | Allowed pandas dtypes (python types) | Example |
---|---|---|---|
SearchKey.EMAIL | object(str) string |
[email protected] | |
SearchKey.HEM | sha256(lowercase(email)) | object(str) string |
0e2dfefcddc929933dcec9a5c7db7b172482814e63c80b8460b36a791384e955 |
SearchKey.IP | IP address (version 4) | object(str, ipaddress.IPv4Address) string int64 |
192.168.0.1 |
SearchKey.PHONE | phone number, E.164 standard | object(str) string int64 float64 |
443451925138 |
SearchKey.DATE | date |
object(str) string datetime64[ns] period[D] |
2020-02-12 (ISO-8601 standard)
12.02.2020 (non standard notation) |
SearchKey.DATETIME | datetime |
object(str) string datetime64[ns] period[D] |
2020-02-12 12:46:18 12:46:18 12.02.2020 |
SearchKey.COUNTRY | Country ISO-3166 code, Country name | object(str) string |
GB US IN |
SearchKey.POSTAL_CODE | Postal code a.k.a. ZIP code. Could be used only with SearchKey.COUNTRY | object(str) string |
21174 061107 SE-999-99 |
For the meaning types SearchKey.DATE/SearchKey.DATETIME with dtypes object or string you have to clarify date/datetime format by passing date_format parameter to FeaturesEnricher
. For example:
from upgini.features_enricher import FeaturesEnricher
from upgini.metadata import SearchKey
enricher = FeaturesEnricher(
search_keys={
"subscription_activation_date": SearchKey.DATE,
"country": SearchKey.COUNTRY,
"zip_code": SearchKey.POSTAL_CODE,
"hashed_email": SearchKey.HEM,
"last_visit_ip_address": SearchKey.IP,
"registered_with_phone": SearchKey.PHONE
},
date_format = "%Y-%d-%m"
)
To use datetime not in UTC timezone, you can cast datetime column explicitly to your timezone (example for Warsaw):
df["date"] = df.date.astype("datetime64").dt.tz_localize("Europe/Warsaw")
Single country for the whole training dataset can be passed with country_code
parameter:
from upgini.features_enricher import FeaturesEnricher
from upgini.metadata import SearchKey
enricher = FeaturesEnricher(
search_keys={
"subscription_activation_date": SearchKey.DATE,
"zip_code": SearchKey.POSTAL_CODE,
},
country_code = "US",
date_format = "%Y-%d-%m"
)
The main abstraction you interact is FeaturesEnricher
, a Scikit-learn compatible estimator. You can easily add it into your existing ML pipelines.
Create instance of the FeaturesEnricher
class and call:
-
fit
to search relevant datasets & features - than
transform
to enrich your dataset with features from search result
Let's try it out!
import pandas as pd
from upgini.features_enricher import FeaturesEnricher
from upgini.metadata import SearchKey
# load labeled training dataset to initiate search
train_df = pd.read_csv("customer_churn_prediction_train.csv")
X = train_df.drop(columns="churn_flag")
y = train_df["churn_flag"]
# now we're going to create `FeaturesEnricher` class
enricher = FeaturesEnricher(
search_keys={
"subscription_activation_date": SearchKey.DATE,
"country": SearchKey.COUNTRY,
"zip_code": SearchKey.POSTAL_CODE
})
# everything is ready to fit! For 200к records fitting should take around 10 minutes,
# we send email notification, just register on profile.upgini.com
enricher.fit(X, y)
That's all! We've fit FeaturesEnricher
.
FeaturesEnricher
class has two properties for feature importances, which will be filled after fit - feature_names_
and feature_importances_
:
-
feature_names_
- feature names from the search result, and if parameterkeep_input=True
was used, initial columns from search dataset as well -
feature_importances_
- SHAP values for features from the search result, same order as infeature_names_
Method get_features_info()
returns pandas dataframe with features and full statistics after fit, including SHAP values and match rates:
enricher.get_features_info()
Get more details about FeaturesEnricher
at runtime using docstrings via help(FeaturesEnricher)
or help(FeaturesEnricher.fit)
.
FeaturesEnricher
is a Scikit-learn compatible estimator, so any pandas dataframe can be enriched with external features from a search result (after fit
).
Use transform
method of FeaturesEnricher
, and let magic to do the rest 🪄
# load dataset for enrichment
test_x = pd.read_csv("test.csv")
# enrich it!
enriched_test_features = enricher.transform(test_x)
FeaturesEnricher
can be initiated with a search_id
parameter from completed search after fit method call.
Just use enricher.get_search_id()
or copy search id string from the fit()
output.
Search keys and features in X should be the same as for fit()
enricher = FeaturesEnricher(
#same set of a search keys as for the fit step
search_keys={"date": SearchKey.DATE},
api_key="<YOUR API_KEY>", # if you fit enricher with api_key then you should use it here
search_id = "abcdef00-0000-0000-0000-999999999999"
)
enriched_prod_dataframe=enricher.transform(input_dataframe)
For most of the ML cases, training step requires labeled dataset with a historical observations from the past. But for production step you'll need an updated and actual data sources and features for the present time, to calculate a prediction.
FeaturesEnricher
, when initiated with set of search keys which includes SearchKey.DATE
, will match records from all potential external data sources exactly on a the specific date/datetime based on SearchKey.DATE
. To avoid enrichment with features "form the future" for the fit
step.
And then, for transform
in a production ML pipeline, you'll get enrichment with relevant features, actual for the present date.
FeaturesEnricher
with SearchKey.DATE
search key in a key set to get actual features for production and avoid features from the future for the training:
enricher = FeaturesEnricher(
search_keys={
"subscription_activation_date": SearchKey.DATE,
"country": SearchKey.COUNTRY,
"zip_code": SearchKey.POSTAL_CODE,
},
)
We validate and clean search initialization dataset under the hood:
- сheck you search keys columns format;
- check zero variance for label column;
- check dataset for full row duplicates. If we find any, we remove duplicated rows and make a note on share of row duplicates;
- check inconsistent labels - rows with the same features and keys but different labels, we remove them and make a note on share of row duplicates;
- remove columns with zero variance - we treat any non search key column in search dataset as a feature, so columns with zero variance will be removed
We detect ML task under the hood based on label column values. Currently we support:
- ModelTaskType.BINARY
- ModelTaskType.MULTICLASS
- ModelTaskType.REGRESSION
But for certain search datasets you can pass parameter to FeaturesEnricher
with correct ML taks type:
from upgini.features_enricher import FeaturesEnricher
from upgini.metadata import SearchKey, ModelTaskType
enricher = FeaturesEnricher(
search_keys={"subscription_activation_date": SearchKey.DATE},
model_task_type=ModelTaskType.REGRESSION
)
Time series prediction supported as ModelTaskType.REGRESSION
or ModelTaskType.BINARY
tasks with time series specific cross-validation split:
-
Scikit-learn time series cross-validation -
CVType.time_series
parameter -
Blocked time series cross-validation -
CVType.blocked_time_series
parameter
To initiate feature search you can pass cross-validation type parameter to FeaturesEnricher
with time series specific CV type:
from upgini.features_enricher import FeaturesEnricher
from upgini.metadata import SearchKey, CVType
enricher = FeaturesEnricher(
search_keys={"sales_date": SearchKey.DATE},
cv=CVType.time_series
)
If you're working with multivariate time series, you should specify id columns of individual univariate series in FeaturesEnricher
. For example, if you have a dataset predicting sales for different stores and products, you should specify store and product id columns as follows:
enricher = FeaturesEnricher(
search_keys={
"sales_date": SearchKey.DATE,
},
id_columns=["store_id", "product_id"],
cv=CVType.time_series
)
sort rows in dataset according to observation order, in most cases - ascending order by date/datetime.
FeaturesEnricher
automaticaly calculates model metrics and uplift from new relevant features either using calculate_metrics()
method or calculate_metrics=True
parameter in fit
or fit_transform
methods (example below).
You can use any model estimator with scikit-learn compartible interface, some examples are:
👈 Evaluation metric should be passed to calculate_metrics() by scoring parameter,
out-of-the box Upgini supports
Metric | Description |
---|---|
explained_variance | Explained variance regression score function |
r2 | R2 (coefficient of determination) regression score function |
max_error | Calculates the maximum residual error (negative - greater is better) |
median_absolute_error | Median absolute error regression loss |
mean_absolute_error | Mean absolute error regression loss |
mean_absolute_percentage_error | Mean absolute percentage error regression loss |
mean_squared_error | Mean squared error regression loss |
mean_squared_log_error (or aliases: msle, MSLE) | Mean squared logarithmic error regression loss |
root_mean_squared_log_error (or aliases: rmsle, RMSLE) | Root mean squared logarithmic error regression loss |
root_mean_squared_error | Root mean squared error regression loss |
mean_poisson_deviance | Mean Poisson deviance regression loss |
mean_gamma_deviance | Mean Gamma deviance regression loss |
accuracy | Accuracy classification score |
top_k_accuracy | Top-k Accuracy classification score |
roc_auc | Area Under the Receiver Operating Characteristic Curve (ROC AUC) from prediction scores |
roc_auc_ovr | Area Under the Receiver Operating Characteristic Curve (ROC AUC) from prediction scores (multi_class="ovr") |
roc_auc_ovo | Area Under the Receiver Operating Characteristic Curve (ROC AUC) from prediction scores (multi_class="ovo") |
roc_auc_ovr_weighted | Area Under the Receiver Operating Characteristic Curve (ROC AUC) from prediction scores (multi_class="ovr", average="weighted") |
roc_auc_ovo_weighted | Area Under the Receiver Operating Characteristic Curve (ROC AUC) from prediction scores (multi_class="ovo", average="weighted") |
balanced_accuracy | Compute the balanced accuracy |
average_precision | Compute average precision (AP) from prediction scores |
log_loss | Log loss, aka logistic loss or cross-entropy loss |
brier_score | Compute the Brier score loss |
In addition to that list, you can define custom evaluation metric function using scikit-learn make_scorer, for example SMAPE.
By default, calculate_metrics()
method calculates evaluation metric with the same cross-validation split as selected for FeaturesEnricher.fit()
by parameter cv = CVType.<cross-validation-split>
.
But you can easily define new split by passing child of BaseCrossValidator to parameter cv
in calculate_metrics()
.
Example with more tips-and-tricks:
from upgini.features_enricher import FeaturesEnricher
from upgini.metadata import SearchKey
enricher = FeaturesEnricher(search_keys={"registration_date": SearchKey.DATE})
# Fit with default setup for metrics calculation
# CatBoost will be used
enricher.fit(X, y, eval_set=eval_set, calculate_metrics=True)
# LightGBM estimator for metrics
custom_estimator = LGBMRegressor()
enricher.calculate_metrics(estimator=custom_estimator)
# Custom metric function to scoring param (callable or name)
custom_scoring = "RMSLE"
enricher.calculate_metrics(scoring=custom_scoring)
# Custom cross validator
custom_cv = TimeSeriesSplit(n_splits=5)
enricher.calculate_metrics(cv=custom_cv)
# All this custom parameters could be combined in both methods: fit, fit_transform and calculate_metrics:
enricher.fit(X, y, eval_set, calculate_metrics=True, estimator=custom_estimator, scoring=custom_scoring, cv=custom_cv)
If a training dataset has a text column, you can generate additional embeddings from it using instructed embeddings generation with LLMs and data augmentation from external sources, just like Upgini does for all records from connected data sources.
For most cases, this gives better results than direct embeddings generation from a text field. Currently, Upgini has two LLMs connected to a search engine - GPT-3.5 from OpenAI and GPT-J.
To use this feature, pass the column names as arguments to the generate_features
parameter. You can use up to 2 columns.
Here's an example for generating features from the "description" and "summary" columns:
enricher = FeaturesEnricher(
search_keys={"date": SearchKey.DATE},
generate_features=["description", "summary"]
)
With this code, Upgini will generate LLM embeddings from text columns and then check them for predictive power for your ML task.
Finally, Upgini will return a dataset enriched by only relevant components of LLM embeddings.
If you already have features or other external data sources, you can specifically search new datasets & features only give accuracy gain "on top" of them.
Just leave all these existing features in the labeled training dataset and Upgini library automatically use them during feature search process and as a baseline ML model to calculate accuracy metric uplift. Only features which improve accuracy will return.
You can validate external features robustness on out-of-time dataset using eval_set
parameter:
# load train dataset
train_df = pd.read_csv("train.csv")
train_ids_and_features = train_df.drop(columns="label")
train_label = train_df["label"]
# load out-of-time validation dataset
eval_df = pd.read_csv("validation.csv")
eval_ids_and_features = eval_df.drop(columns="label")
eval_label = eval_df["label"]
# create FeaturesEnricher
enricher = FeaturesEnricher(search_keys={"registration_date": SearchKey.DATE})
# now we fit WITH eval_set parameter to calculate accuracy metrics on Out-of-time dataset.
# the output will contain quality metrics for both the training data set and
# the eval set (validation OOT data set)
enricher.fit(
train_ids_and_features,
train_label,
eval_set = [(eval_ids_and_features, eval_label)]
)
- Same data schema as for search initialization dataset
- Pandas dataframe representation
FeaturesEnricher
can be initialized with additional string parameter loss
.
Depending on ML-task, you can use the following loss functions:
-
regression
: regression, regression_l1, huber, poisson, quantile, mape, gamma, tweedie; -
binary
: binary; -
multiclass
: multiclass, multiclassova.
For instance, if your target variable has a Poisson distribution (count of events, number of customers in the shop and so on), you should try to use loss="poisson"
to improve quality of feature selection and get better evaluation metrics.
Usage example:
enricher = FeaturesEnricher(
search_keys={"date": SearchKey.DATE},
loss="poisson",
model_task_type=ModelTaskType.REGRESSION
)
enriched_dataframe.fit(X, y)
transform
and fit_transform
methods of FeaturesEnricher
can be used with two additional parameters:
-
importance_threshold
: float = 0 - only features with importance >= threshold will be added to the output dataframe -
max_features
: int - only first TOP N features by importance will be returned, where N = max_features
And keep_input=True
will keep all initial columns from search dataset X:
enricher = FeaturesEnricher(
search_keys={"subscription_activation_date": SearchKey.DATE}
)
enriched_dataframe.fit_transform(X, y, keep_input=True, max_features=2)
fit
, fit_transform
, transform
and calculate_metrics
methods of FeaturesEnricher
can be used with parameter exclude_features_sources
that allows to exclude Trial or Paid features from Premium data sources:
enricher = FeaturesEnricher(
search_keys={"subscription_activation_date": SearchKey.DATE}
)
enricher.fit(X, y, calculate_metrics=False)
trial_features = enricher.get_features_info()[enricher.get_features_info()["Feature type"] == "Trial"]["Feature name"].values.tolist()
paid_features = enricher.get_features_info()[enricher.get_features_info()["Feature type"] == "Paid"]["Feature name"].values.tolist()
enricher.calculate_metrics(exclude_features_sources=(trial_features + paid_features))
enricher.transform(X, exclude_features_sources=(trial_features + paid_features))
Upgini has autodetection of search keys on by default.
To turn off use detect_missing_search_keys=False
:
enricher = FeaturesEnricher(
search_keys={"date": SearchKey.DATE},
detect_missing_search_keys=False,
)
enricher.fit(X, y)
Upgini detect rows with target outlier for regression tasks. By default such rows are dropped on metrics calculation. To turn off removing of target outlier rows use parameter remove_outliers_calc_metrics=False
in fit, fit_transform or calculate_metrics methods:
enricher = FeaturesEnricher(
search_keys={"date": SearchKey.DATE},
)
enricher.fit(X, y, remove_outliers_calc_metrics=False)
Register and get a free API key for exclusive data sources and features: 600 mln+ phone numbers, 350 mln+ emails, 2^32 IP addresses
Benefit | No Sign-up | Registered user |
---|---|---|
Enrichment with date/datetime, postal/ZIP code and country keys | Yes | Yes |
Enrichment with phone number, hashed email/HEM and IP-address keys | No | Yes |
Email notification on search task completion | No | Yes |
Automated feature generation with LLMs from columns in a search dataset | Yes, till 12/05/23 | Yes |
Email notification on new data source activation 🔜 | No | Yes |
You may publish ANY data which you consider as royalty / license free (Open Data) and potentially valuable for ML applications for community usage:
- Please Sign Up here
- Copy Upgini API key from profile and upload your data from Upgini python library with this key:
import pandas as pd
from upgini.metadata import SearchKey
from upgini.ads import upload_user_ads
import os
os.environ["UPGINI_API_KEY"] = "your_long_string_api_key_goes_here"
#you can define custom search key which might not be supported yet, just use SearchKey.CUSTOM_KEY type
sample_df = pd.read_csv("path_to_data_sample_file")
upload_user_ads("test", sample_df, {
"city": SearchKey.CUSTOM_KEY,
"stats_date": SearchKey.DATE
})
- After data verification, search results on community data will be available usual way.
Please note, that we are still in a beta stage.
Requests and support, in preferred order
❗Please try to create bug reports that are:
- reproducible - include steps to reproduce the problem.
- specific - include as much detail as possible: which Python version, what environment, etc.
- unique - do not duplicate existing opened issues.
- scoped to a Single Bug - one bug per report.
We are not a large team, so we probably won't be able to:
- implement smooth integration with most common low-code ML libraries and platforms (PyCaret, H2O AutoML, etc. )
- implement all possible data verification and normalization capabilities for different types of search keys And we need some help from the community!
So, we'll be happy about every pull request you open and issue you find to make this library more incredible. Please note that it might sometimes take us a while to get back to you. For major changes, please open an issue first to discuss what you would like to change
Some convenient ways to start contributing are:
⚙️ Open in Visual Studio Code You can remotely open this repo in VS Code without cloning or automatically clone and open it inside a docker container.
⚙️ Gitpod You can use Gitpod to launch a fully functional development environment right in your browser.
- Simple sales predictions as a template notebook
- Full list of Kaggle Guides & Examples
- Project on PyPI
- More perks for registered users
😔 Found mistype or a bug in code snippet? Our bad! Please report it here
For Tasks:
Click tags to check more tools for each tasksFor Jobs:
Alternative AI tools for upgini
Similar Open Source Tools
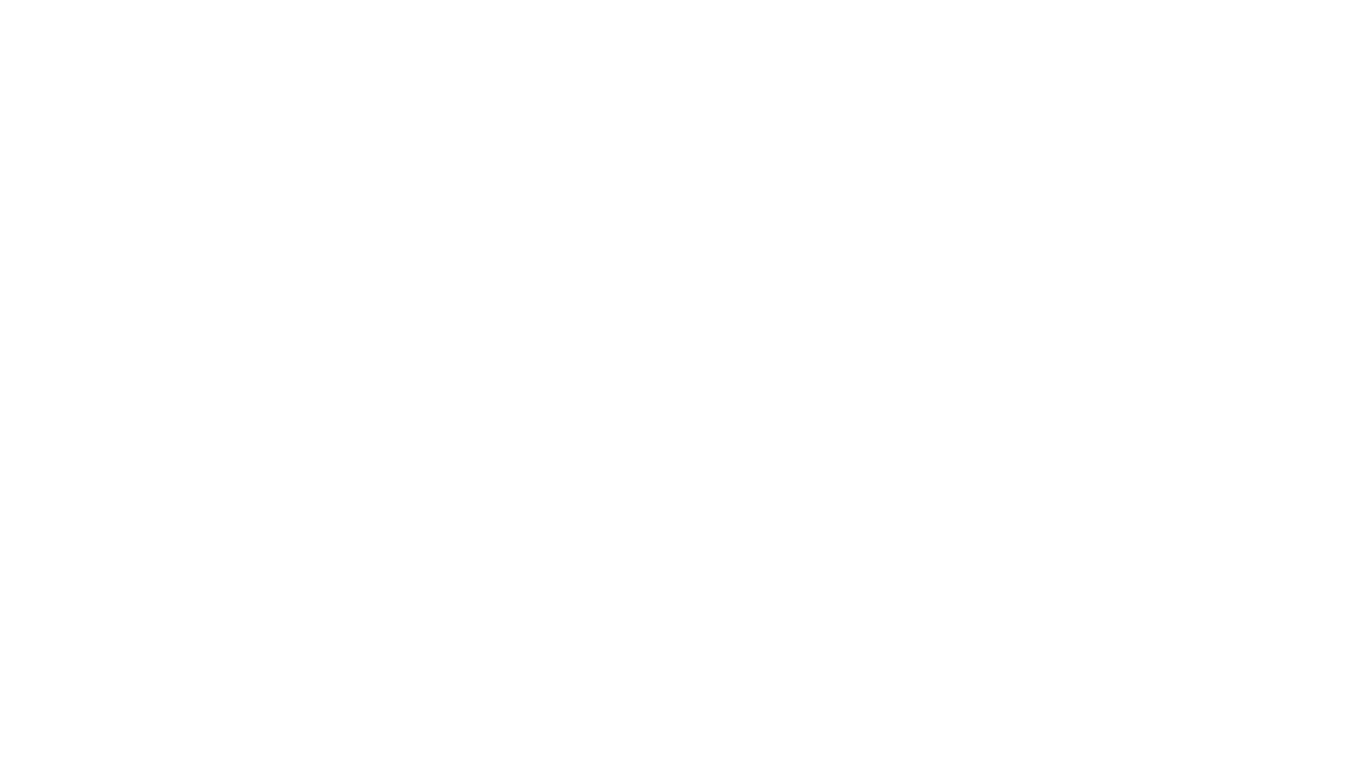
upgini
Upgini is an intelligent data search engine with a Python library that helps users find and add relevant features to their ML pipeline from various public, community, and premium external data sources. It automates the optimization of connected data sources by generating an optimal set of machine learning features using large language models, GraphNNs, and recurrent neural networks. The tool aims to simplify feature search and enrichment for external data to make it a standard approach in machine learning pipelines. It democratizes access to data sources for the data science community.
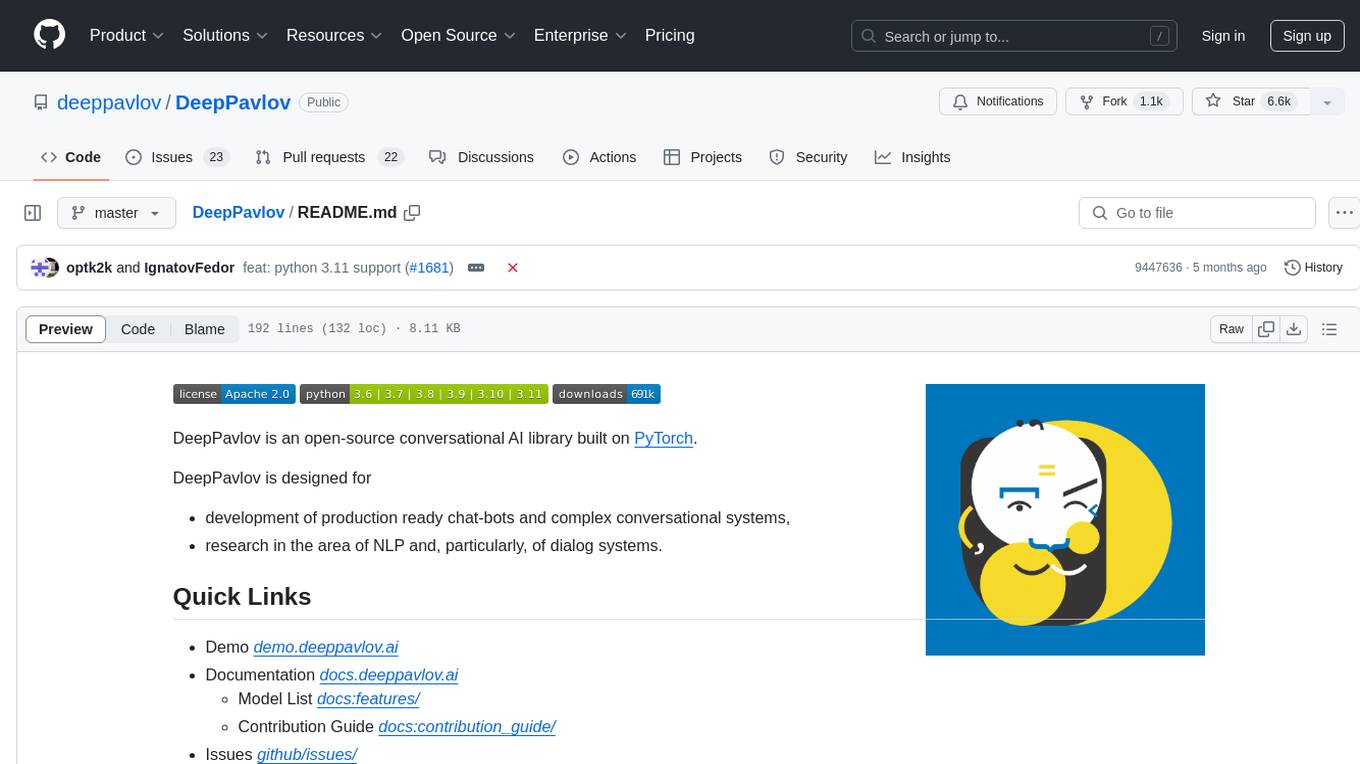
DeepPavlov
DeepPavlov is an open-source conversational AI library built on PyTorch. It is designed for the development of production-ready chatbots and complex conversational systems, as well as for research in the area of NLP and dialog systems. The library offers a wide range of models for tasks such as Named Entity Recognition, Intent/Sentence Classification, Question Answering, Sentence Similarity/Ranking, Syntactic Parsing, and more. DeepPavlov also provides embeddings like BERT, ELMo, and FastText for various languages, along with AutoML capabilities and integrations with REST API, Socket API, and Amazon AWS.
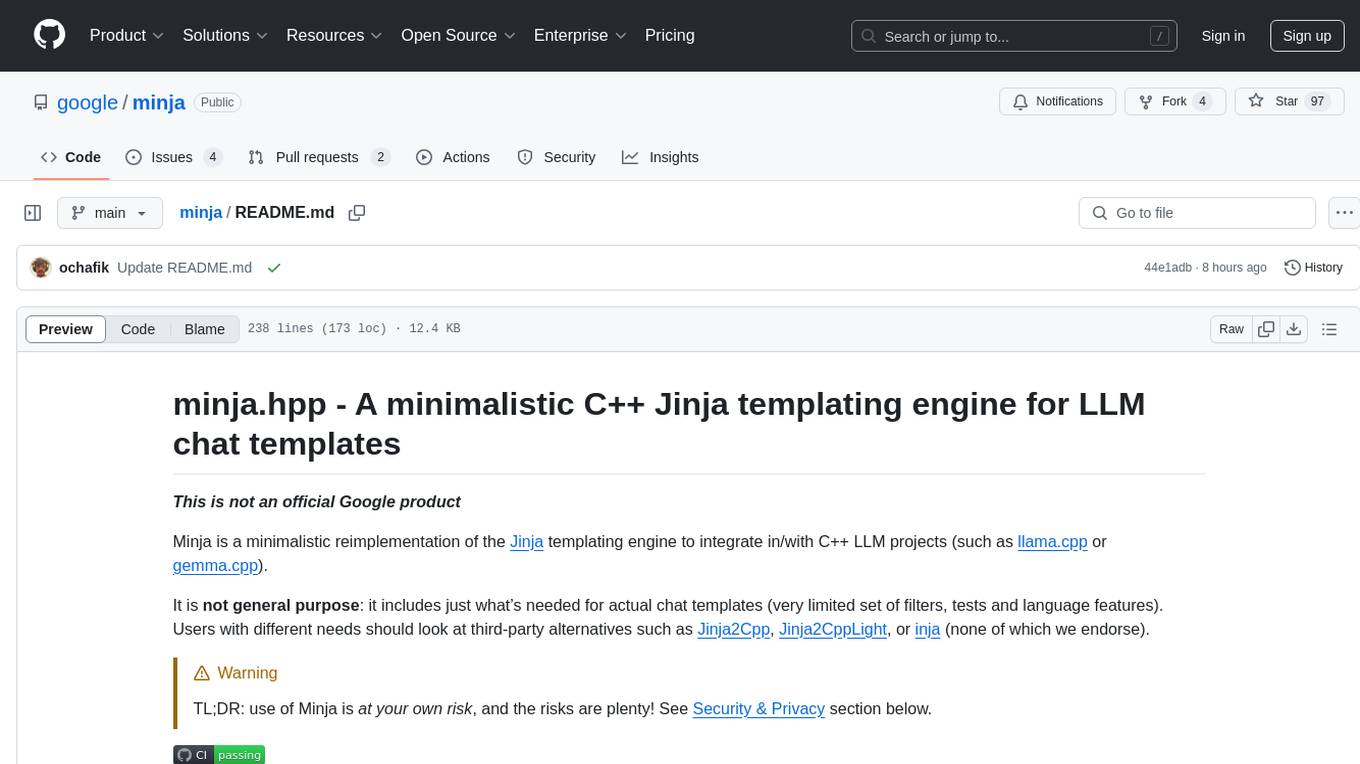
minja
Minja is a minimalistic C++ Jinja templating engine designed specifically for integration with C++ LLM projects, such as llama.cpp or gemma.cpp. It is not a general-purpose tool but focuses on providing a limited set of filters, tests, and language features tailored for chat templates. The library is header-only, requires C++17, and depends only on nlohmann::json. Minja aims to keep the codebase small, easy to understand, and offers decent performance compared to Python. Users should be cautious when using Minja due to potential security risks, and it is not intended for producing HTML or JavaScript output.
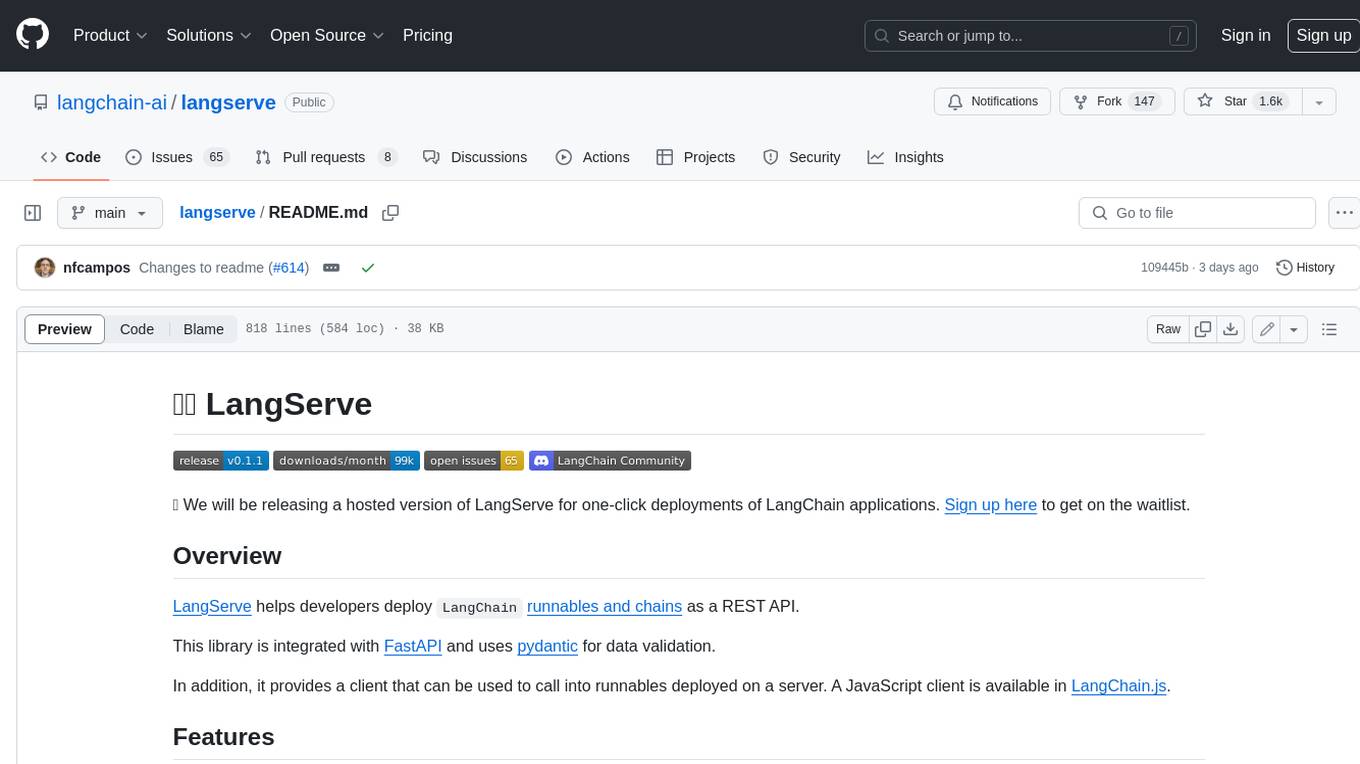
langserve
LangServe helps developers deploy `LangChain` runnables and chains as a REST API. This library is integrated with FastAPI and uses pydantic for data validation. In addition, it provides a client that can be used to call into runnables deployed on a server. A JavaScript client is available in LangChain.js.
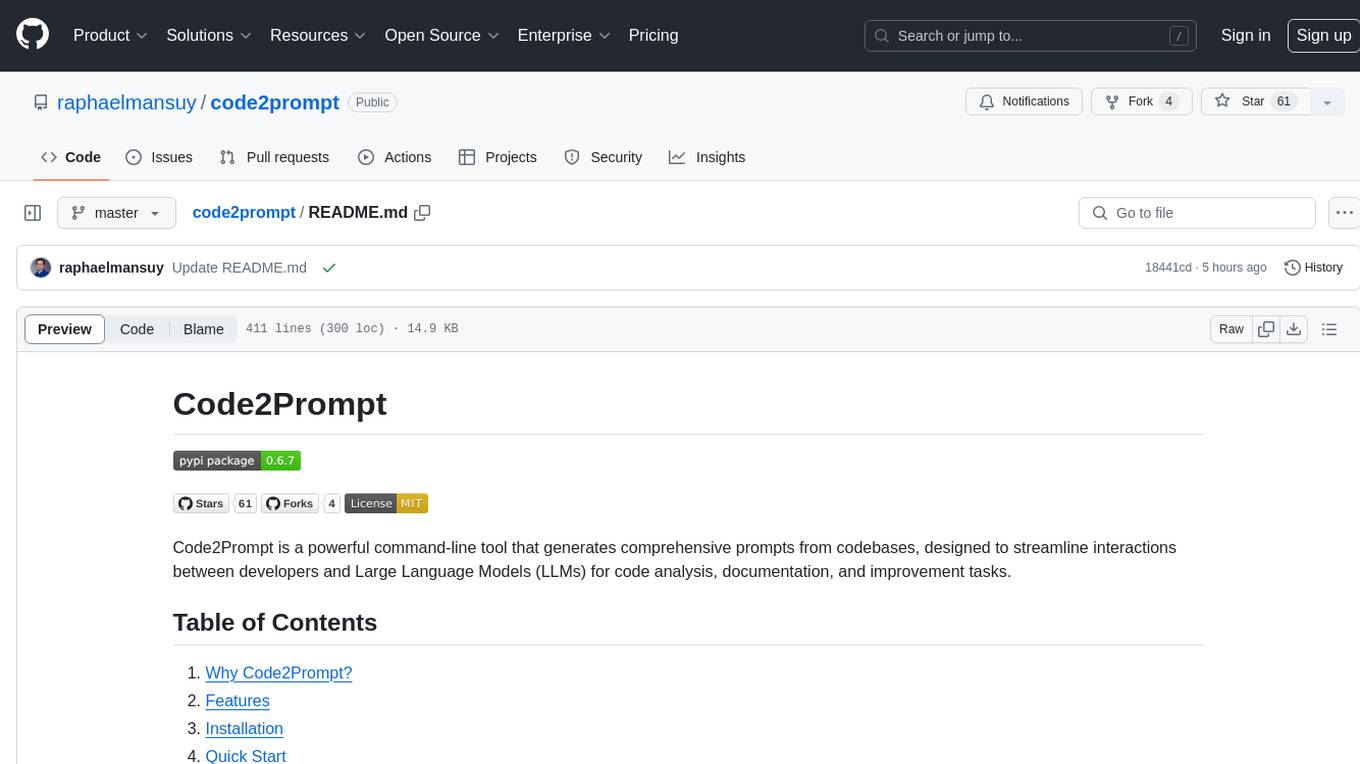
code2prompt
Code2Prompt is a powerful command-line tool that generates comprehensive prompts from codebases, designed to streamline interactions between developers and Large Language Models (LLMs) for code analysis, documentation, and improvement tasks. It bridges the gap between codebases and LLMs by converting projects into AI-friendly prompts, enabling users to leverage AI for various software development tasks. The tool offers features like holistic codebase representation, intelligent source tree generation, customizable prompt templates, smart token management, Gitignore integration, flexible file handling, clipboard-ready output, multiple output options, and enhanced code readability.
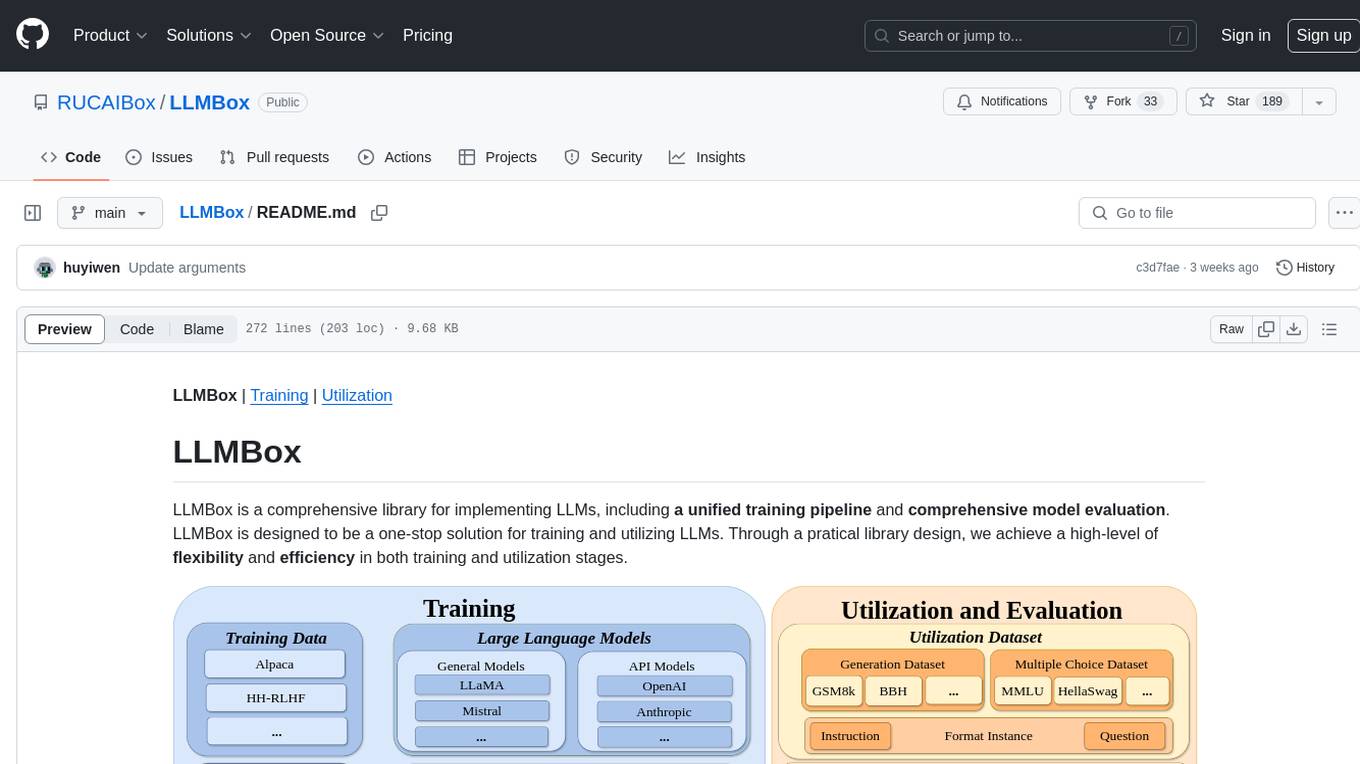
LLMBox
LLMBox is a comprehensive library designed for implementing Large Language Models (LLMs) with a focus on a unified training pipeline and comprehensive model evaluation. It serves as a one-stop solution for training and utilizing LLMs, offering flexibility and efficiency in both training and utilization stages. The library supports diverse training strategies, comprehensive datasets, tokenizer vocabulary merging, data construction strategies, parameter efficient fine-tuning, and efficient training methods. For utilization, LLMBox provides comprehensive evaluation on various datasets, in-context learning strategies, chain-of-thought evaluation, evaluation methods, prefix caching for faster inference, support for specific LLM models like vLLM and Flash Attention, and quantization options. The tool is suitable for researchers and developers working with LLMs for natural language processing tasks.
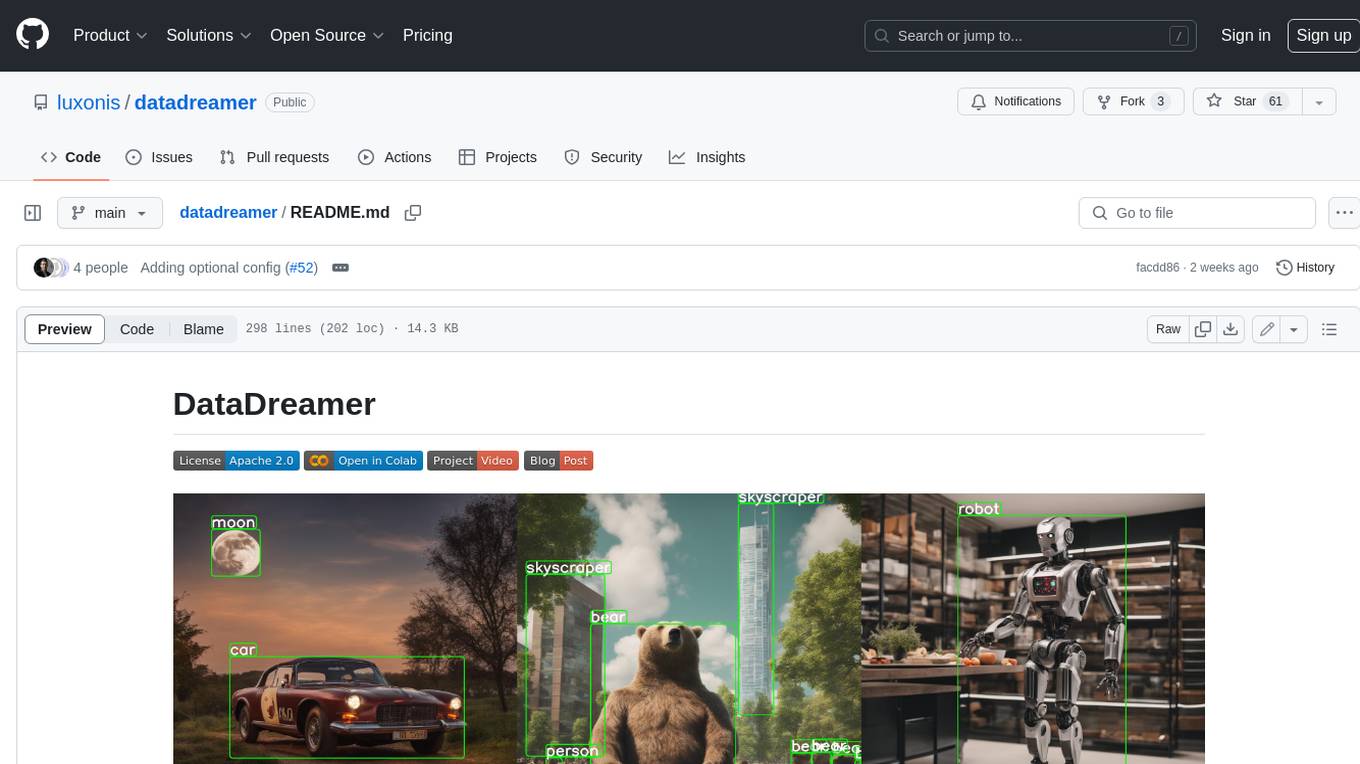
datadreamer
DataDreamer is an advanced toolkit designed to facilitate the development of edge AI models by enabling synthetic data generation, knowledge extraction from pre-trained models, and creation of efficient and potent models. It eliminates the need for extensive datasets by generating synthetic datasets, leverages latent knowledge from pre-trained models, and focuses on creating compact models suitable for integration into any device and performance for specialized tasks. The toolkit offers features like prompt generation, image generation, dataset annotation, and tools for training small-scale neural networks for edge deployment. It provides hardware requirements, usage instructions, available models, and limitations to consider while using the library.
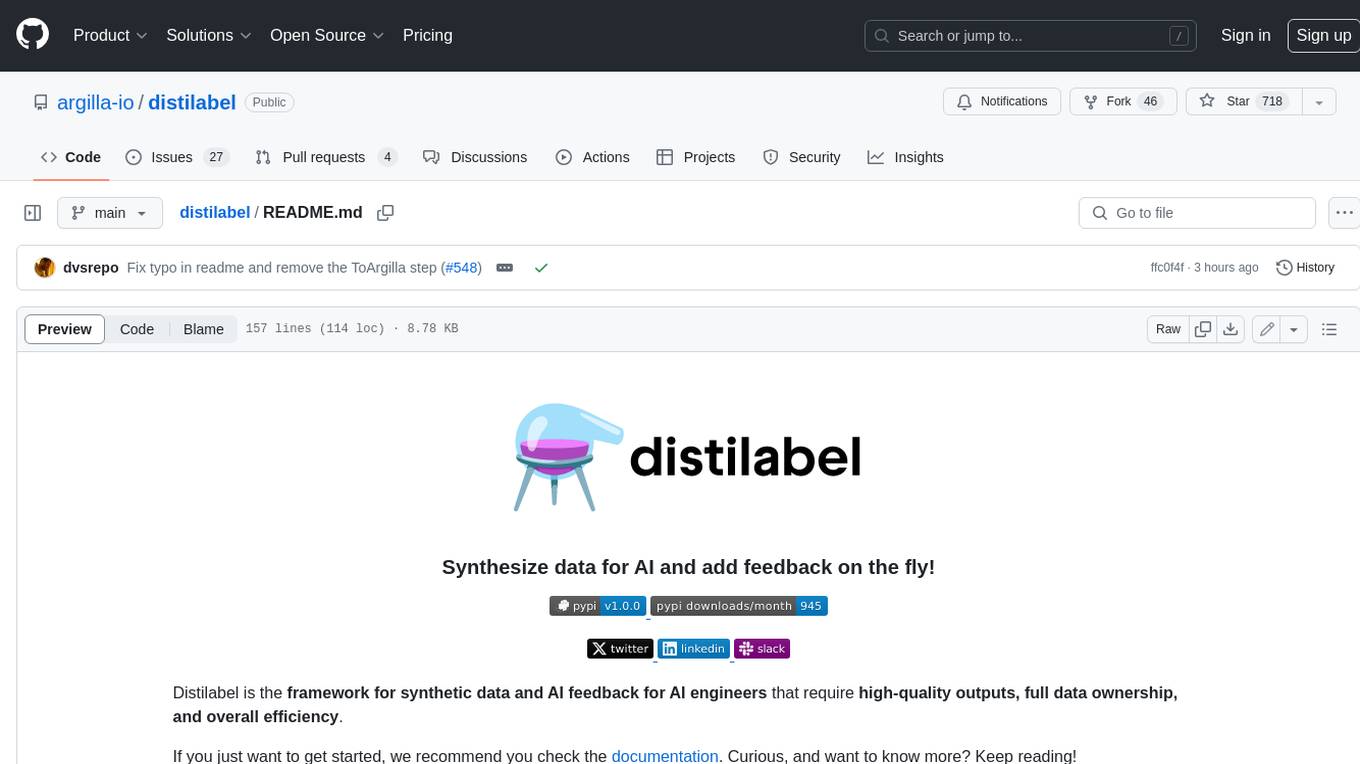
distilabel
Distilabel is a framework for synthetic data and AI feedback for AI engineers that require high-quality outputs, full data ownership, and overall efficiency. It helps you synthesize data and provide AI feedback to improve the quality of your AI models. With Distilabel, you can: * **Synthesize data:** Generate synthetic data to train your AI models. This can help you to overcome the challenges of data scarcity and bias. * **Provide AI feedback:** Get feedback from AI models on your data. This can help you to identify errors and improve the quality of your data. * **Improve your AI output quality:** By using Distilabel to synthesize data and provide AI feedback, you can improve the quality of your AI models and get better results.
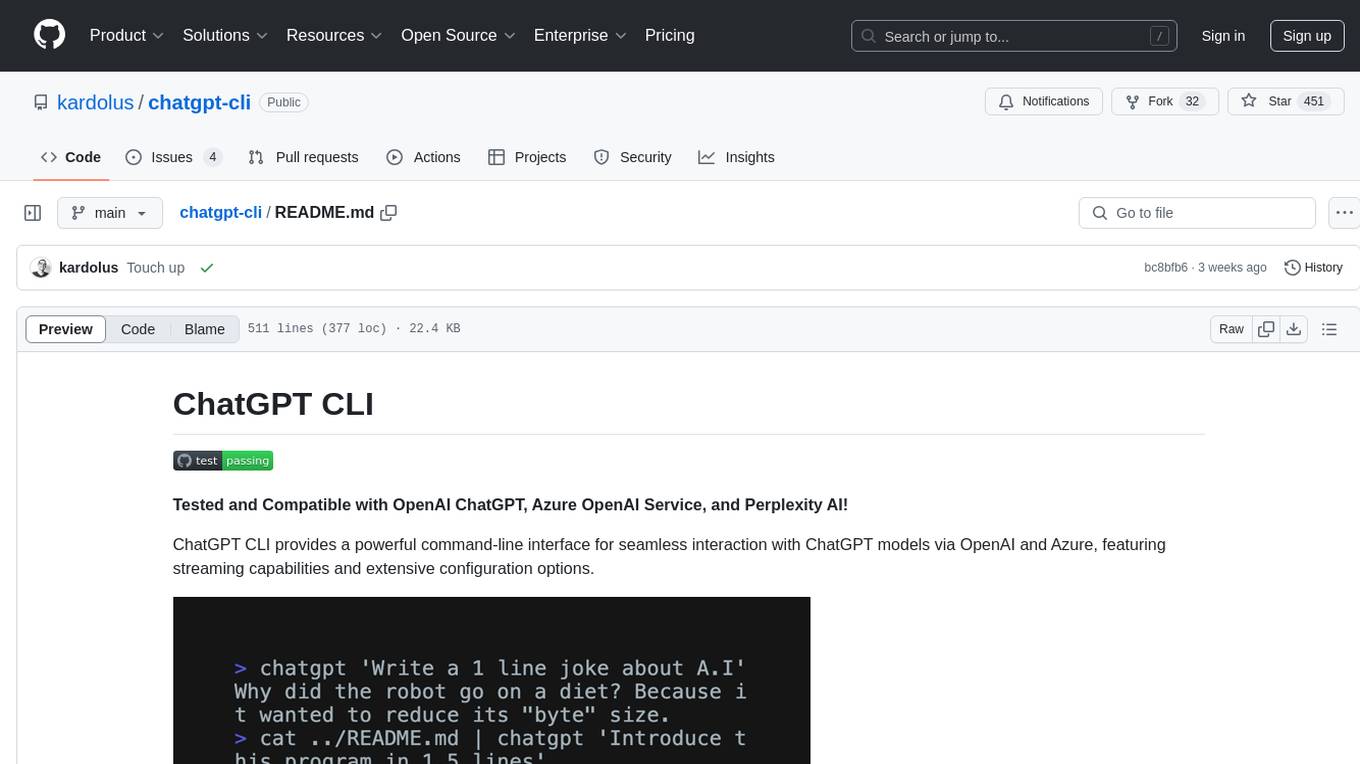
chatgpt-cli
ChatGPT CLI provides a powerful command-line interface for seamless interaction with ChatGPT models via OpenAI and Azure. It features streaming capabilities, extensive configuration options, and supports various modes like streaming, query, and interactive mode. Users can manage thread-based context, sliding window history, and provide custom context from any source. The CLI also offers model and thread listing, advanced configuration options, and supports GPT-4, GPT-3.5-turbo, and Perplexity's models. Installation is available via Homebrew or direct download, and users can configure settings through default values, a config.yaml file, or environment variables.
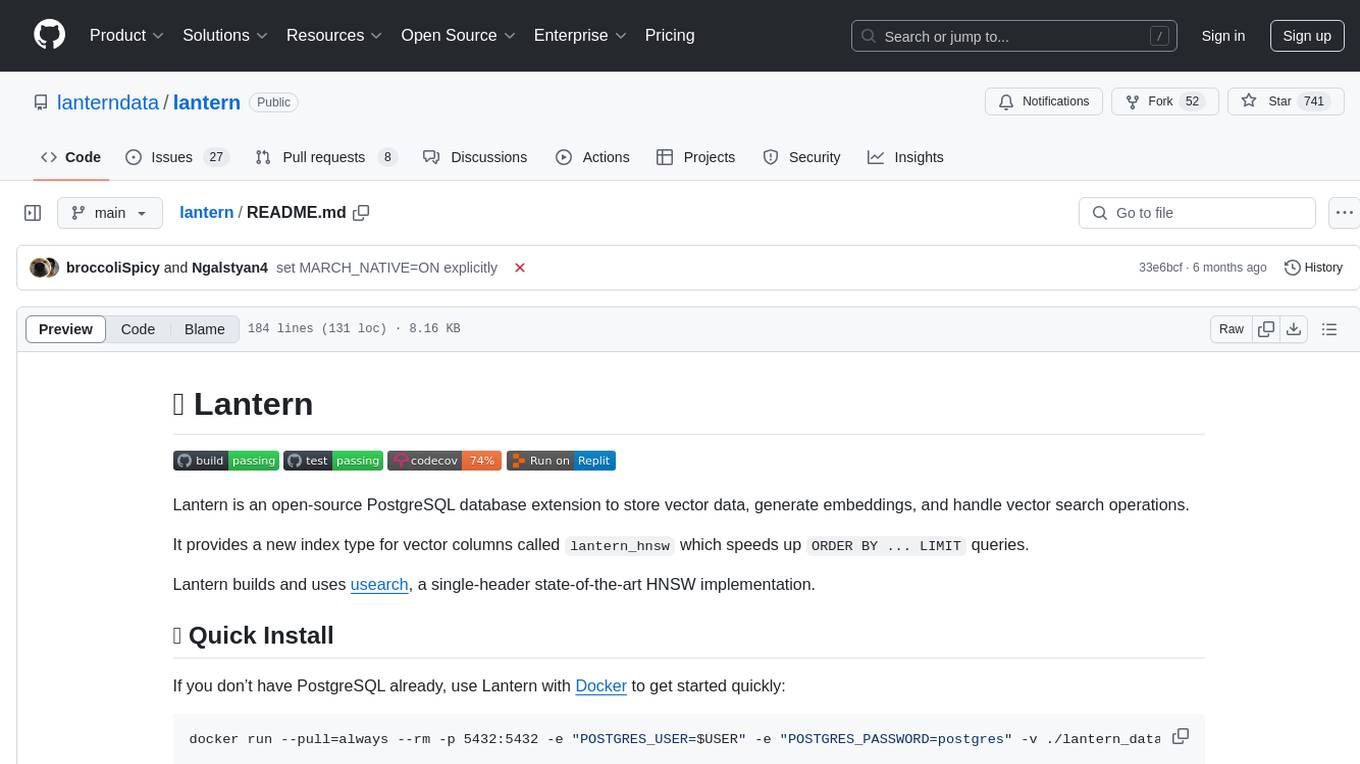
lantern
Lantern is an open-source PostgreSQL database extension designed to store vector data, generate embeddings, and handle vector search operations efficiently. It introduces a new index type called 'lantern_hnsw' for vector columns, which speeds up 'ORDER BY ... LIMIT' queries. Lantern utilizes the state-of-the-art HNSW implementation called usearch. Users can easily install Lantern using Docker, Homebrew, or precompiled binaries. The tool supports various distance functions, index construction parameters, and operator classes for efficient querying. Lantern offers features like embedding generation, interoperability with pgvector, parallel index creation, and external index graph generation. It aims to provide superior performance metrics compared to other similar tools and has a roadmap for future enhancements such as cloud-hosted version, hardware-accelerated distance metrics, industry-specific application templates, and support for version control and A/B testing of embeddings.
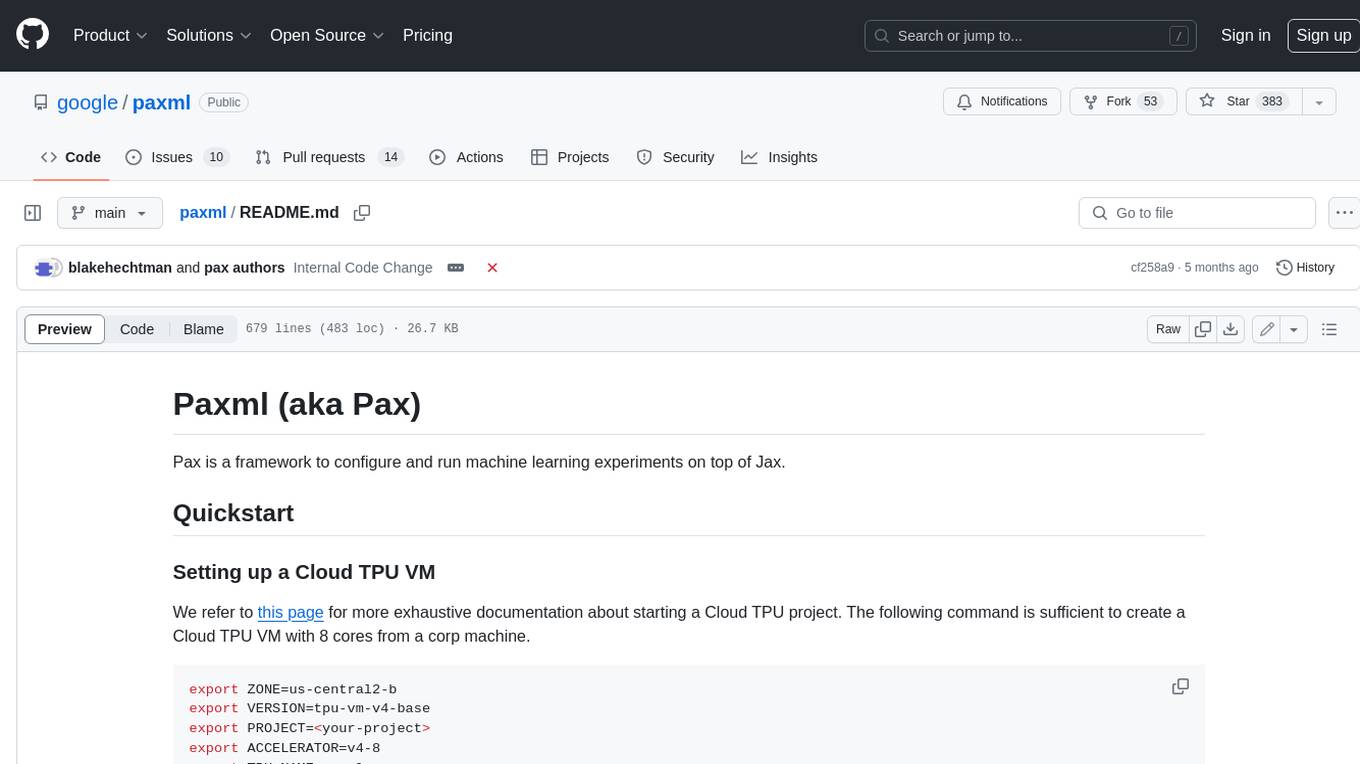
paxml
Pax is a framework to configure and run machine learning experiments on top of Jax.
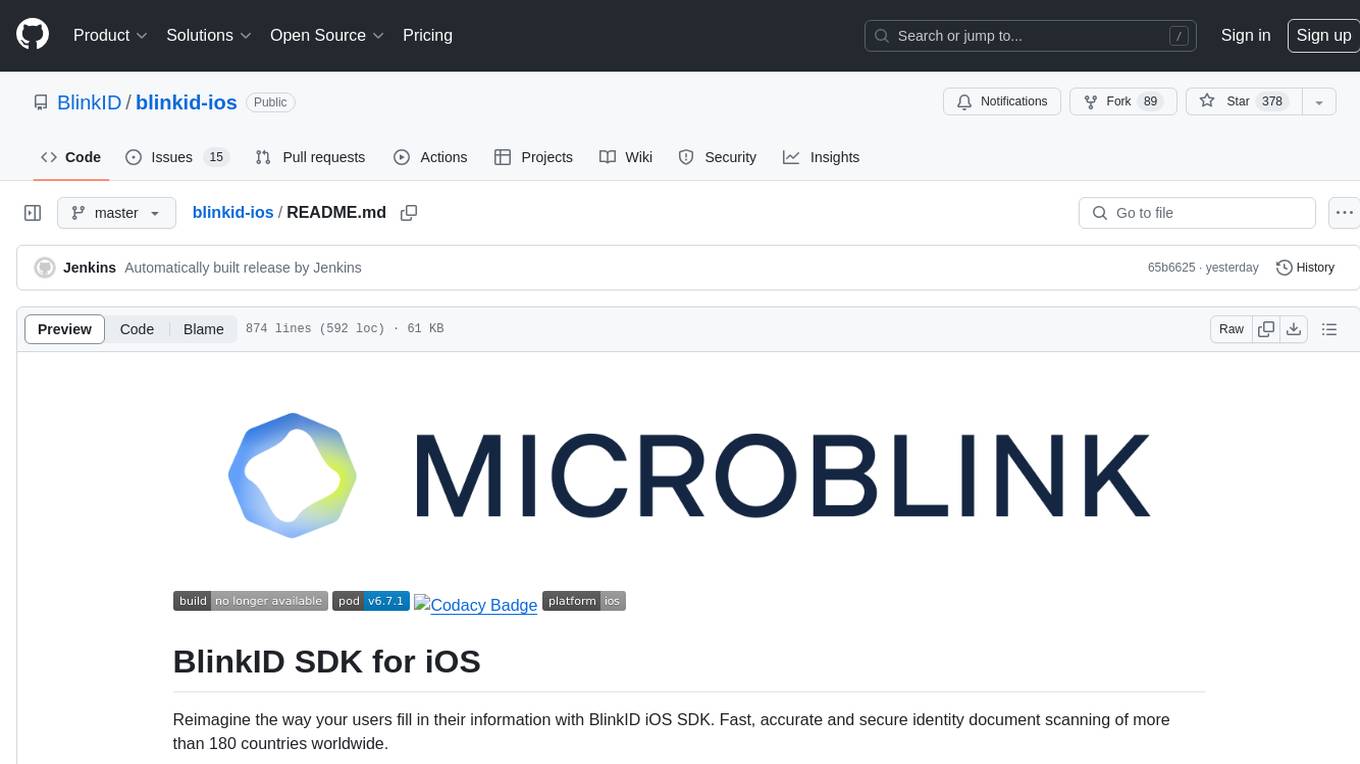
blinkid-ios
BlinkID iOS is a mobile SDK that enables developers to easily integrate ID scanning and data extraction capabilities into their iOS applications. The SDK supports scanning and processing various types of identity documents, such as passports, driver's licenses, and ID cards. It provides accurate and fast data extraction, including personal information and document details. With BlinkID iOS, developers can enhance their apps with secure and reliable ID verification functionality, improving user experience and streamlining identity verification processes.
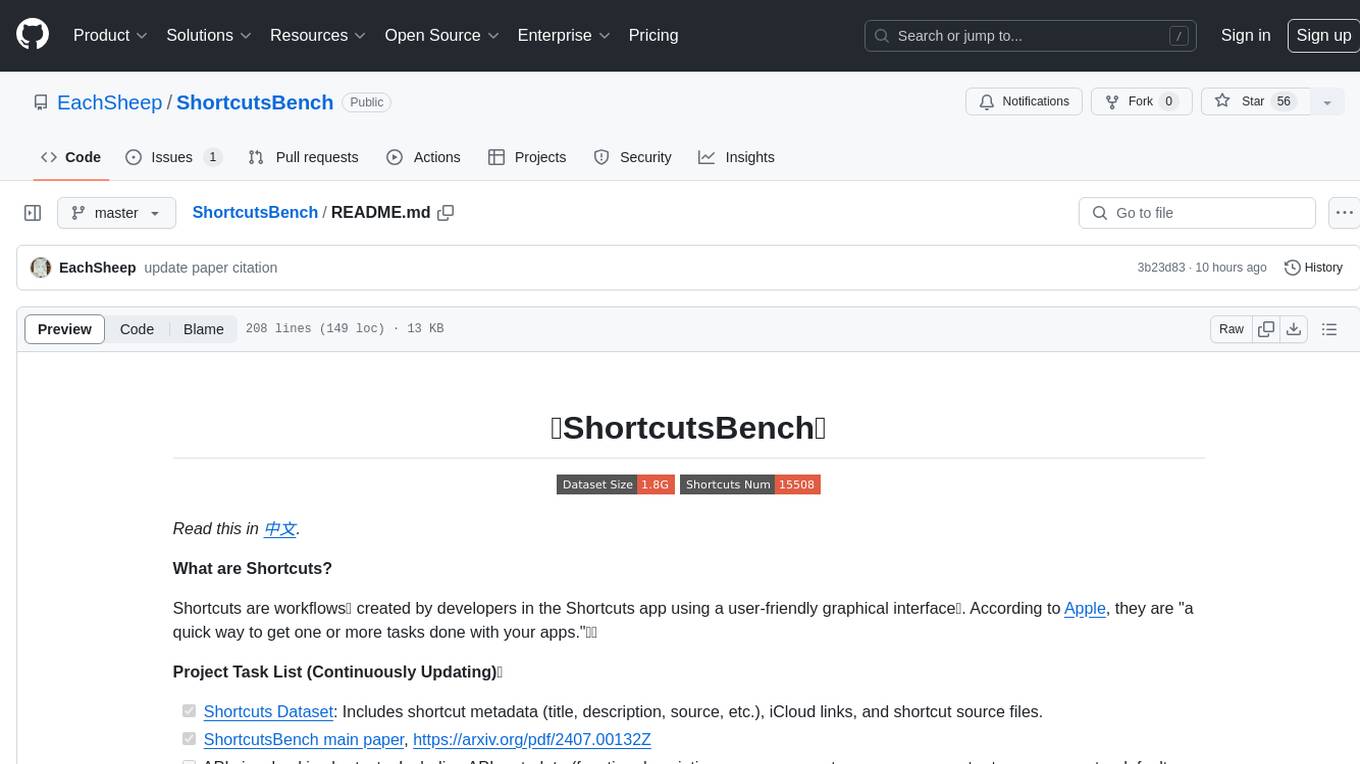
ShortcutsBench
ShortcutsBench is a project focused on collecting and analyzing workflows created in the Shortcuts app, providing a dataset of shortcut metadata, source files, and API information. It aims to study the integration of large language models with Apple devices, particularly focusing on the role of shortcuts in enhancing user experience. The project offers insights for Shortcuts users, enthusiasts, and researchers to explore, customize workflows, and study automated workflows, low-code programming, and API-based agents.
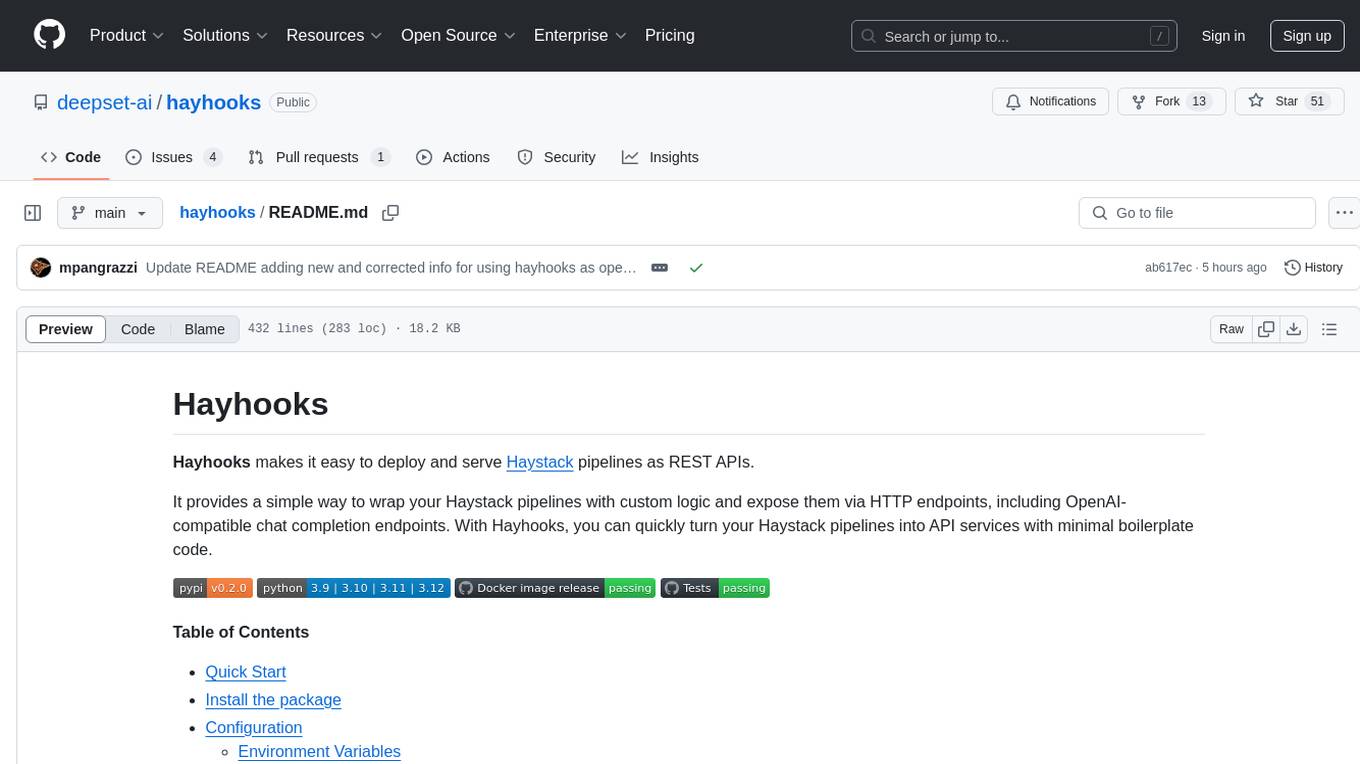
hayhooks
Hayhooks is a tool that simplifies the deployment and serving of Haystack pipelines as REST APIs. It allows users to wrap their pipelines with custom logic and expose them via HTTP endpoints, including OpenAI-compatible chat completion endpoints. With Hayhooks, users can easily convert their Haystack pipelines into API services with minimal boilerplate code.
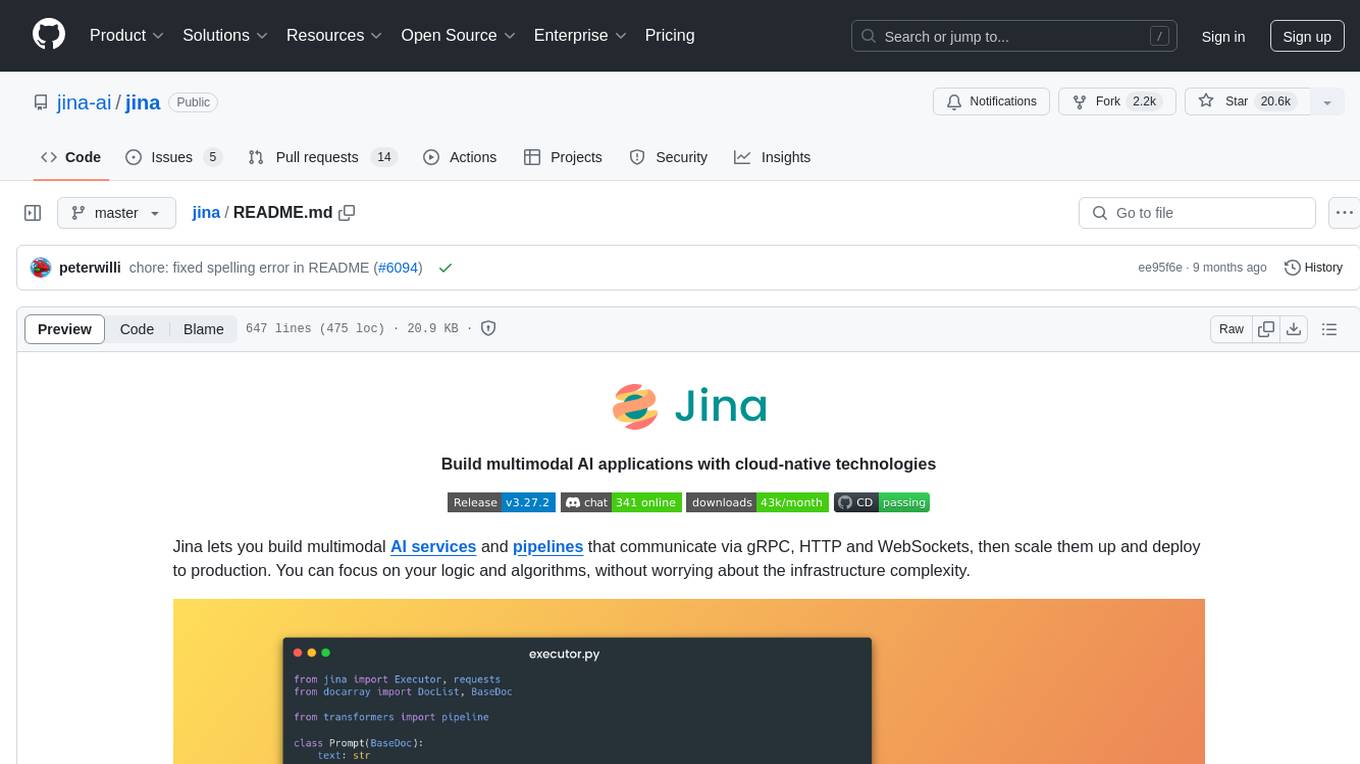
jina
Jina is a tool that allows users to build multimodal AI services and pipelines using cloud-native technologies. It provides a Pythonic experience for serving ML models and transitioning from local deployment to advanced orchestration frameworks like Docker-Compose, Kubernetes, or Jina AI Cloud. Users can build and serve models for any data type and deep learning framework, design high-performance services with easy scaling, serve LLM models while streaming their output, integrate with Docker containers via Executor Hub, and host on CPU/GPU using Jina AI Cloud. Jina also offers advanced orchestration and scaling capabilities, a smooth transition to the cloud, and easy scalability and concurrency features for applications. Users can deploy to their own cloud or system with Kubernetes and Docker Compose integration, and even deploy to JCloud for autoscaling and monitoring.
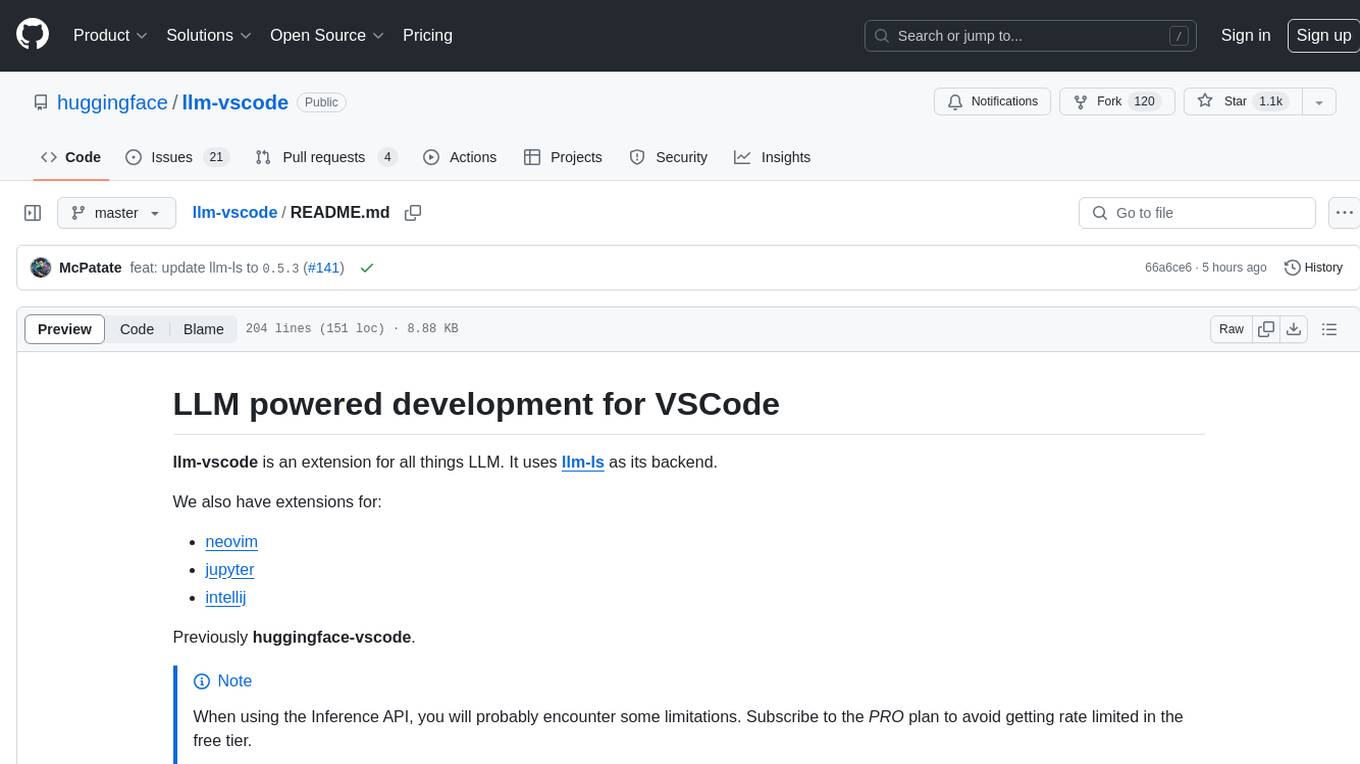
llm-vscode
llm-vscode is an extension designed for all things LLM, utilizing llm-ls as its backend. It offers features such as code completion with 'ghost-text' suggestions, the ability to choose models for code generation via HTTP requests, ensuring prompt size fits within the context window, and code attribution checks. Users can configure the backend, suggestion behavior, keybindings, llm-ls settings, and tokenization options. Additionally, the extension supports testing models like Code Llama 13B, Phind/Phind-CodeLlama-34B-v2, and WizardLM/WizardCoder-Python-34B-V1.0. Development involves cloning llm-ls, building it, and setting up the llm-vscode extension for use.
For similar tasks
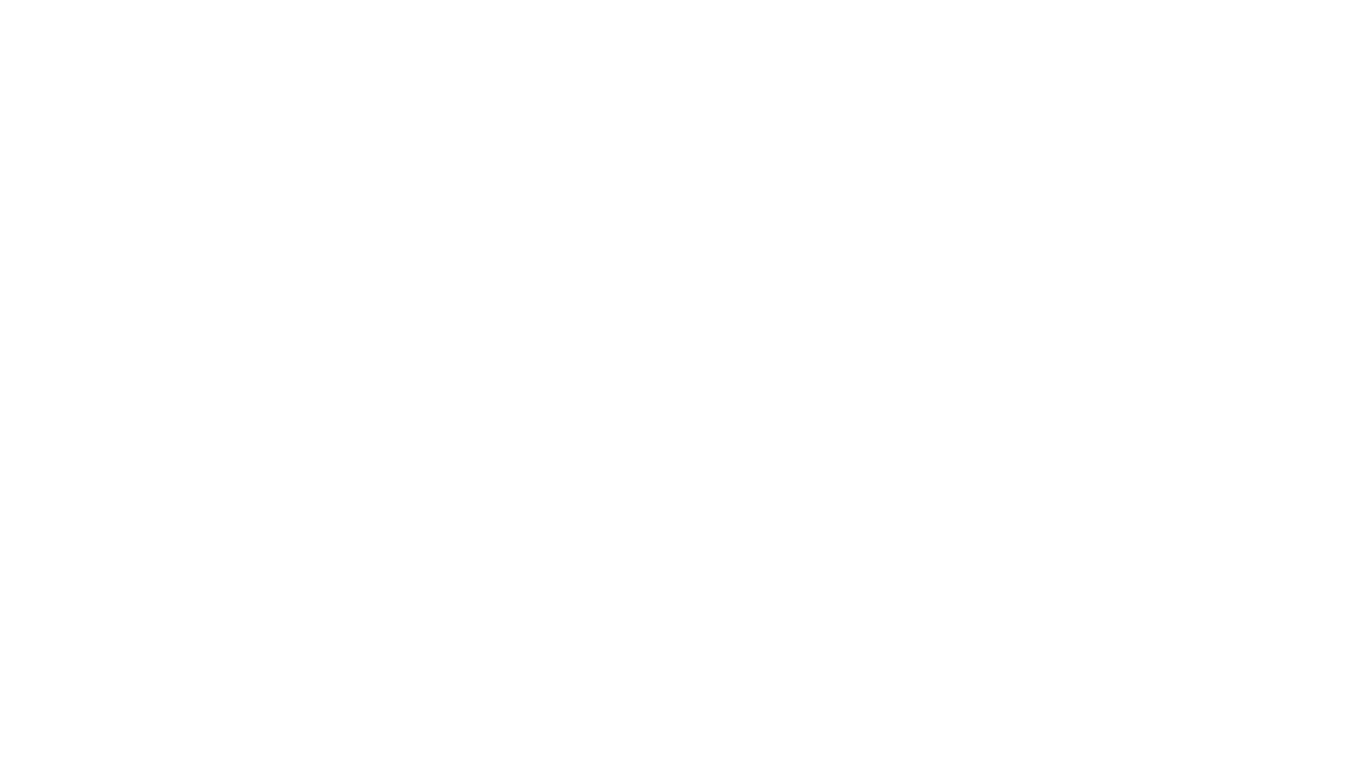
upgini
Upgini is an intelligent data search engine with a Python library that helps users find and add relevant features to their ML pipeline from various public, community, and premium external data sources. It automates the optimization of connected data sources by generating an optimal set of machine learning features using large language models, GraphNNs, and recurrent neural networks. The tool aims to simplify feature search and enrichment for external data to make it a standard approach in machine learning pipelines. It democratizes access to data sources for the data science community.
For similar jobs
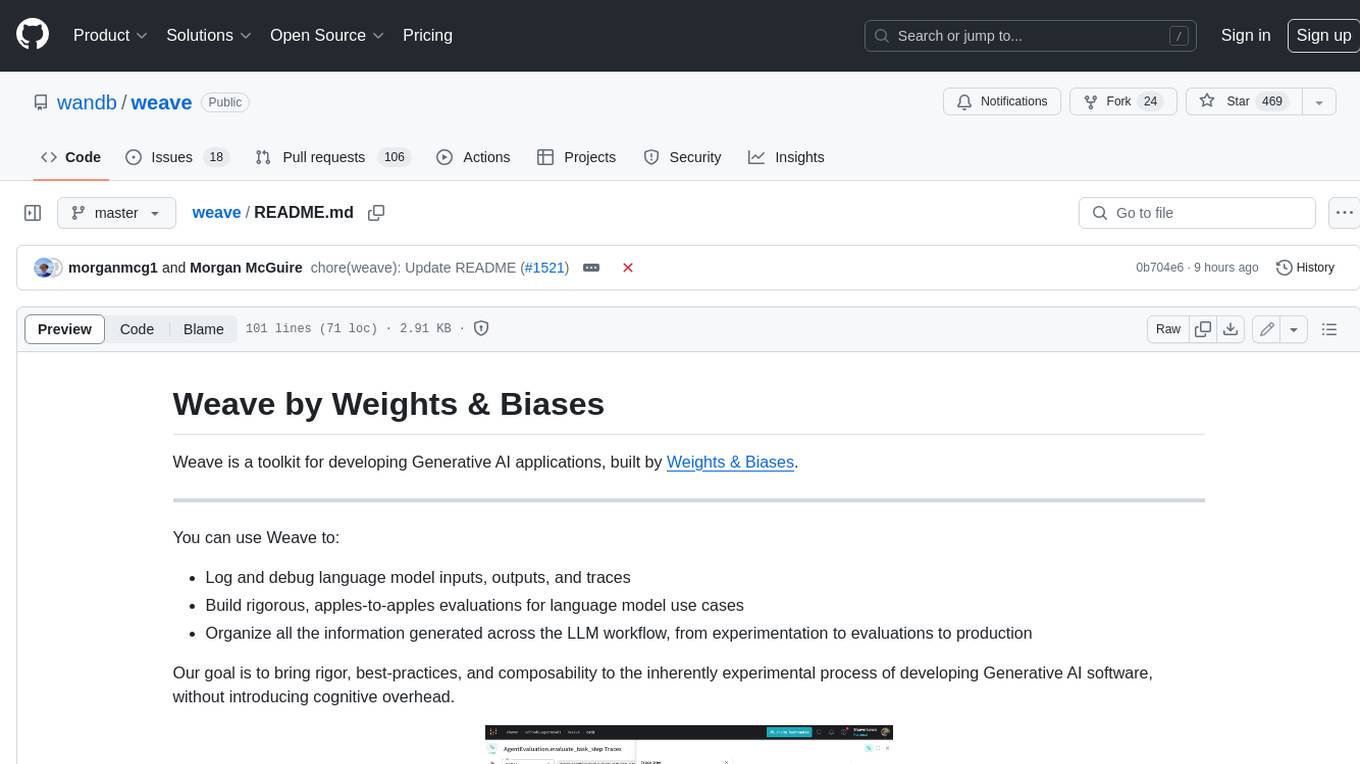
weave
Weave is a toolkit for developing Generative AI applications, built by Weights & Biases. With Weave, you can log and debug language model inputs, outputs, and traces; build rigorous, apples-to-apples evaluations for language model use cases; and organize all the information generated across the LLM workflow, from experimentation to evaluations to production. Weave aims to bring rigor, best-practices, and composability to the inherently experimental process of developing Generative AI software, without introducing cognitive overhead.
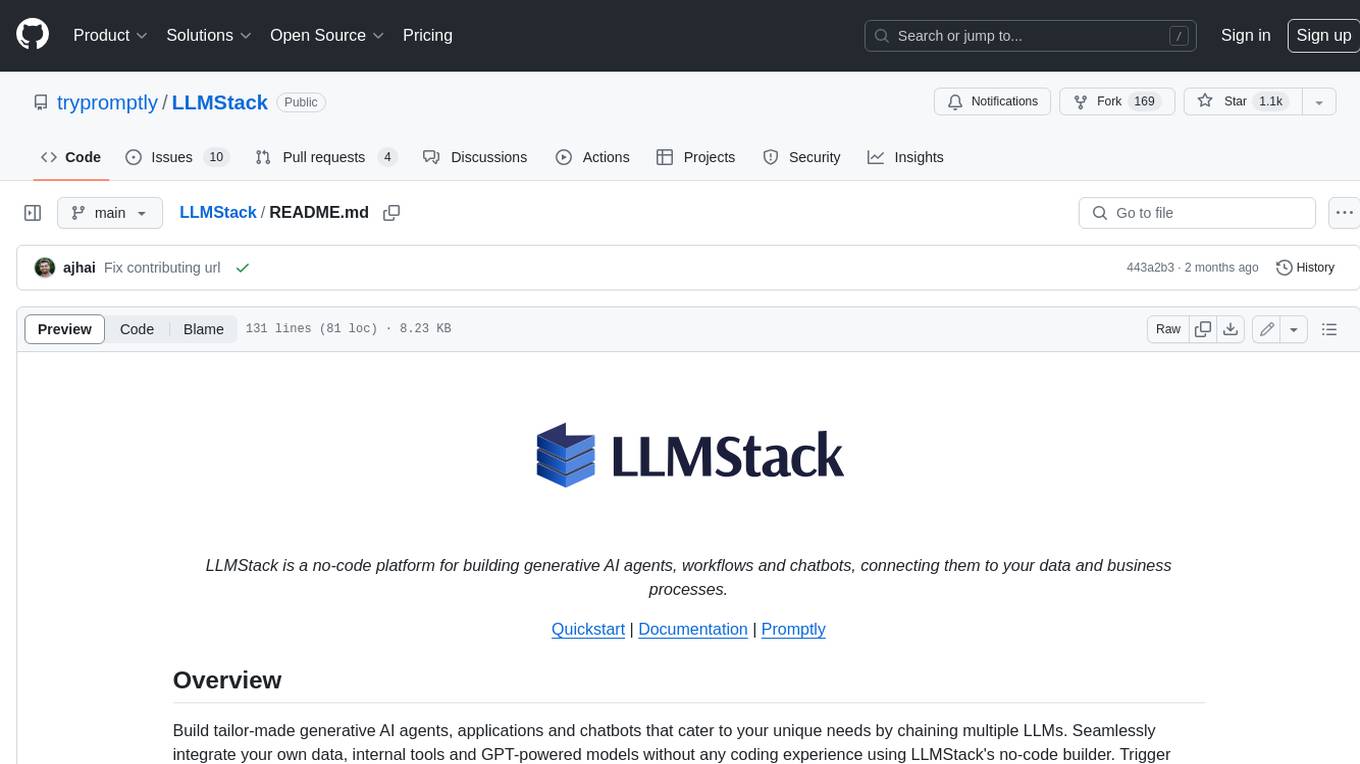
LLMStack
LLMStack is a no-code platform for building generative AI agents, workflows, and chatbots. It allows users to connect their own data, internal tools, and GPT-powered models without any coding experience. LLMStack can be deployed to the cloud or on-premise and can be accessed via HTTP API or triggered from Slack or Discord.
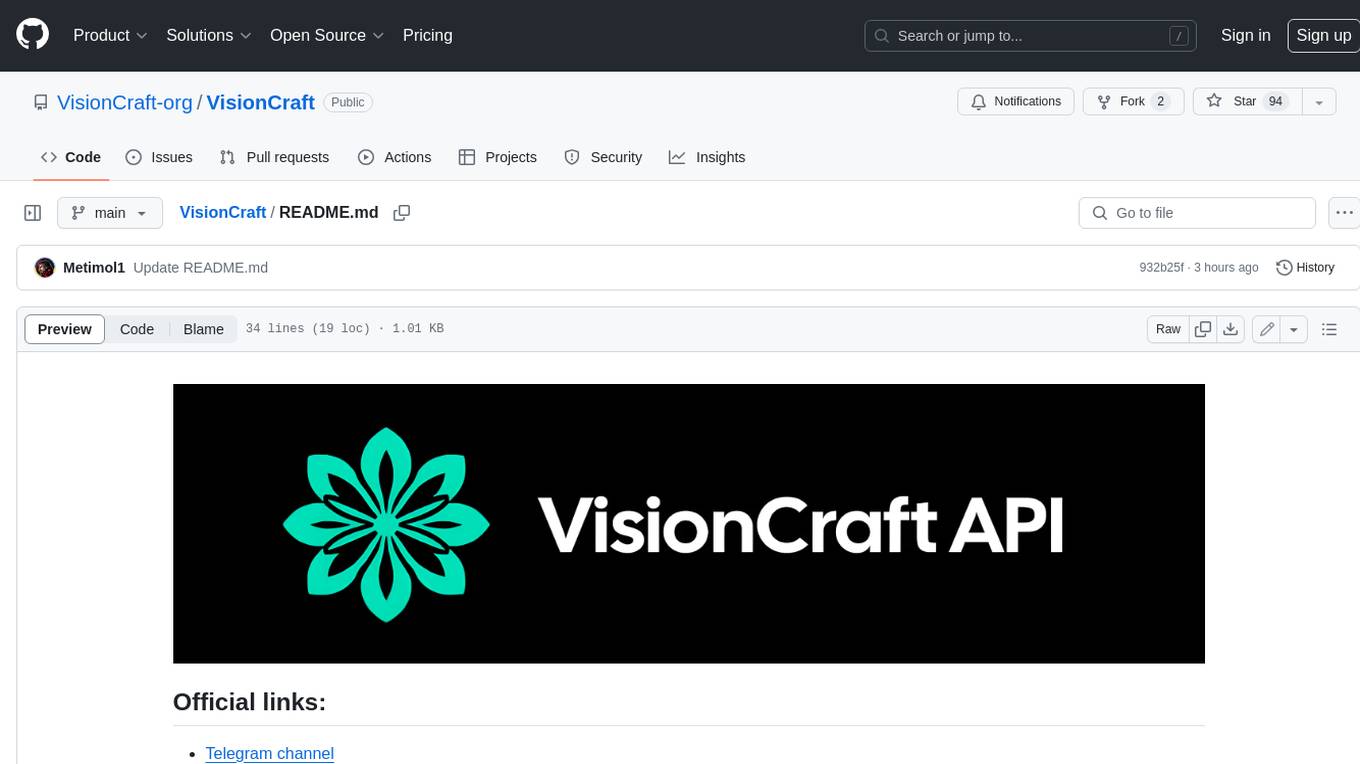
VisionCraft
The VisionCraft API is a free API for using over 100 different AI models. From images to sound.
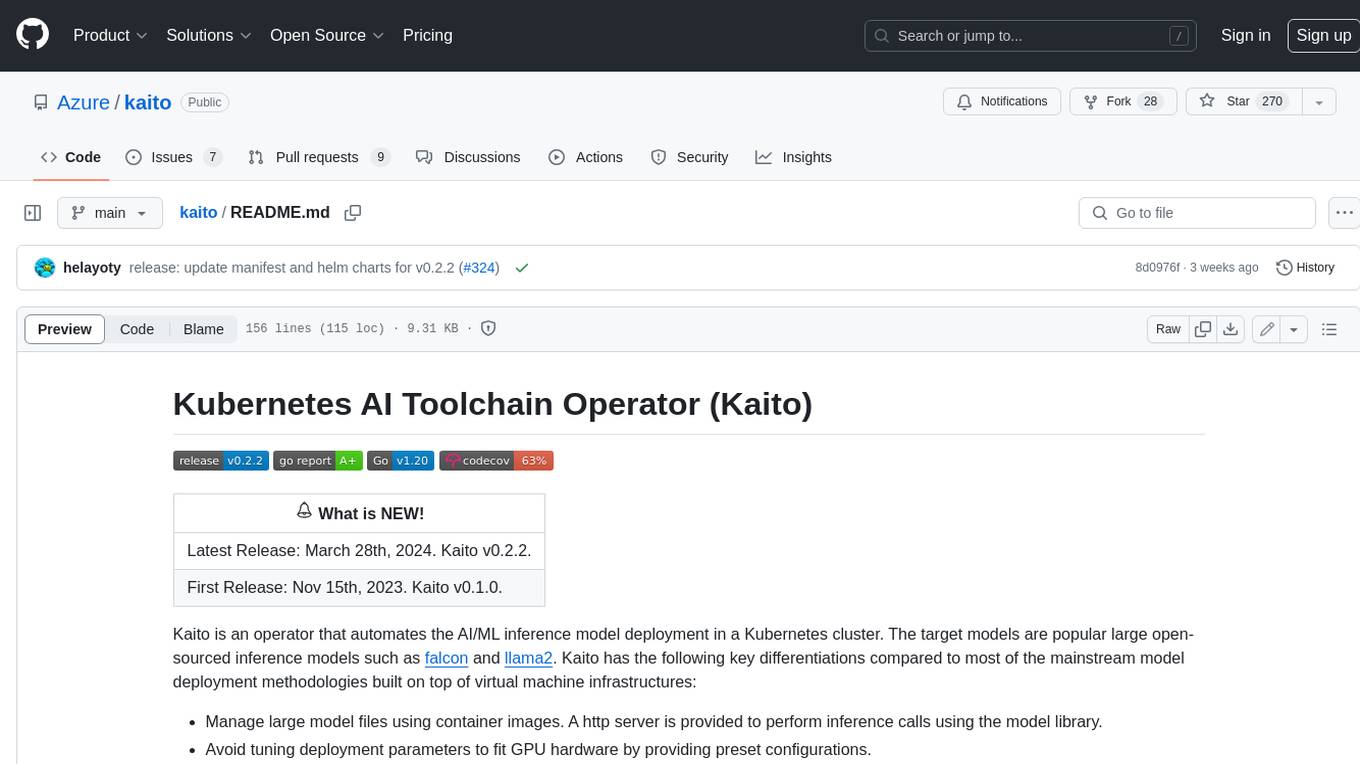
kaito
Kaito is an operator that automates the AI/ML inference model deployment in a Kubernetes cluster. It manages large model files using container images, avoids tuning deployment parameters to fit GPU hardware by providing preset configurations, auto-provisions GPU nodes based on model requirements, and hosts large model images in the public Microsoft Container Registry (MCR) if the license allows. Using Kaito, the workflow of onboarding large AI inference models in Kubernetes is largely simplified.
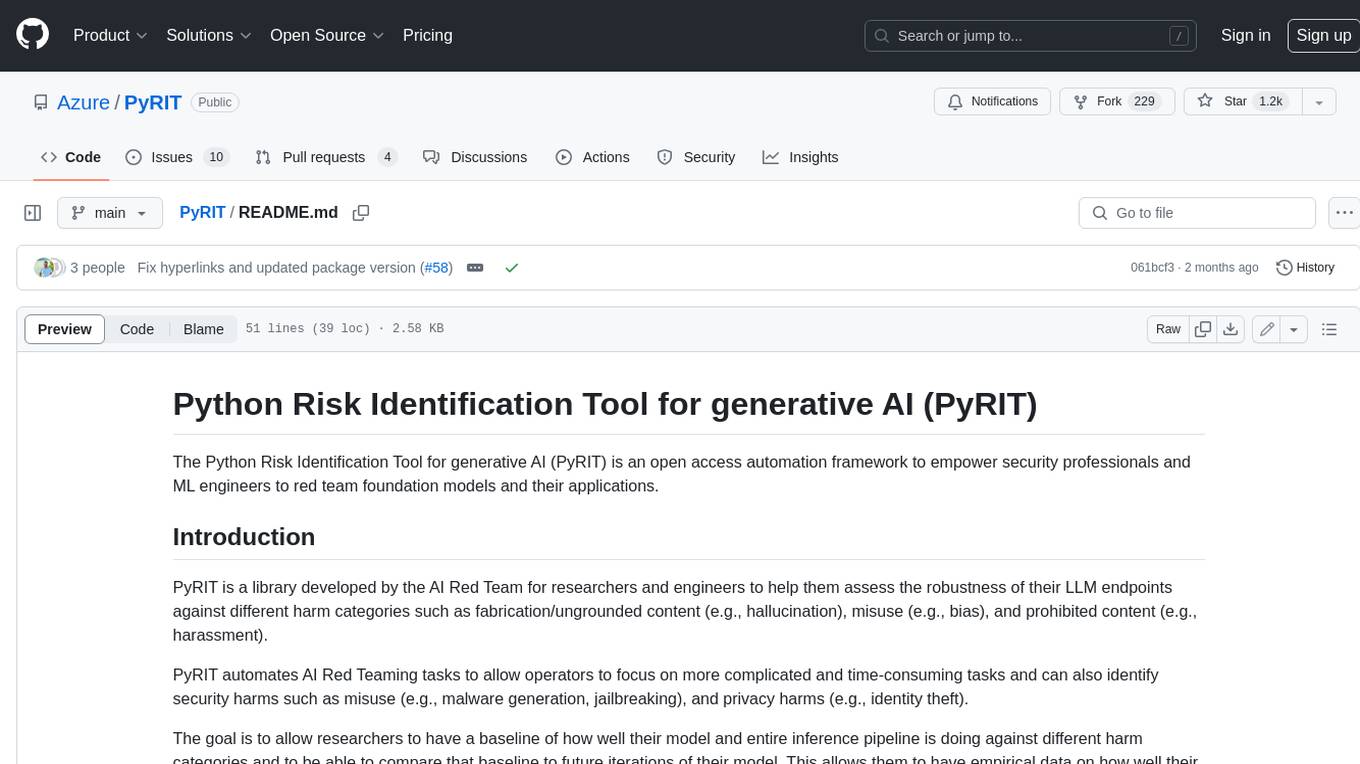
PyRIT
PyRIT is an open access automation framework designed to empower security professionals and ML engineers to red team foundation models and their applications. It automates AI Red Teaming tasks to allow operators to focus on more complicated and time-consuming tasks and can also identify security harms such as misuse (e.g., malware generation, jailbreaking), and privacy harms (e.g., identity theft). The goal is to allow researchers to have a baseline of how well their model and entire inference pipeline is doing against different harm categories and to be able to compare that baseline to future iterations of their model. This allows them to have empirical data on how well their model is doing today, and detect any degradation of performance based on future improvements.
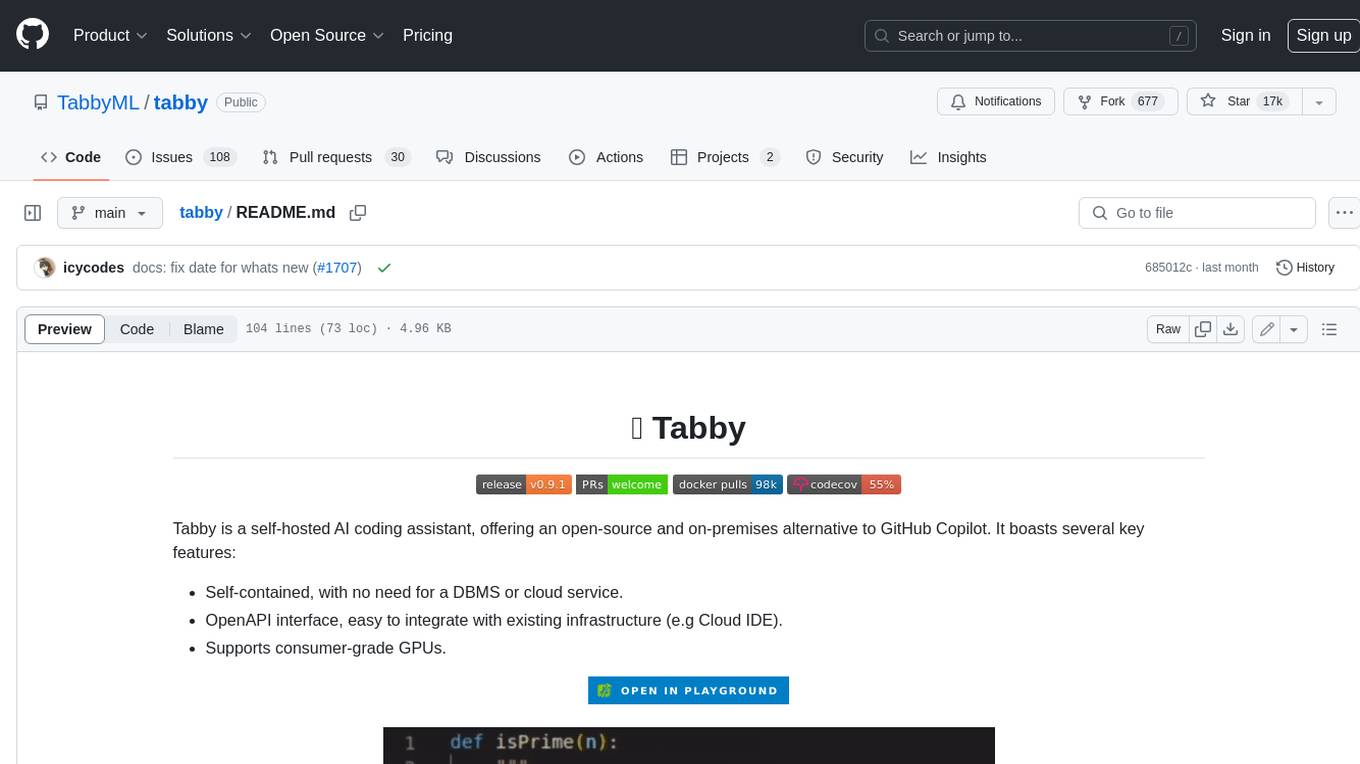
tabby
Tabby is a self-hosted AI coding assistant, offering an open-source and on-premises alternative to GitHub Copilot. It boasts several key features: * Self-contained, with no need for a DBMS or cloud service. * OpenAPI interface, easy to integrate with existing infrastructure (e.g Cloud IDE). * Supports consumer-grade GPUs.
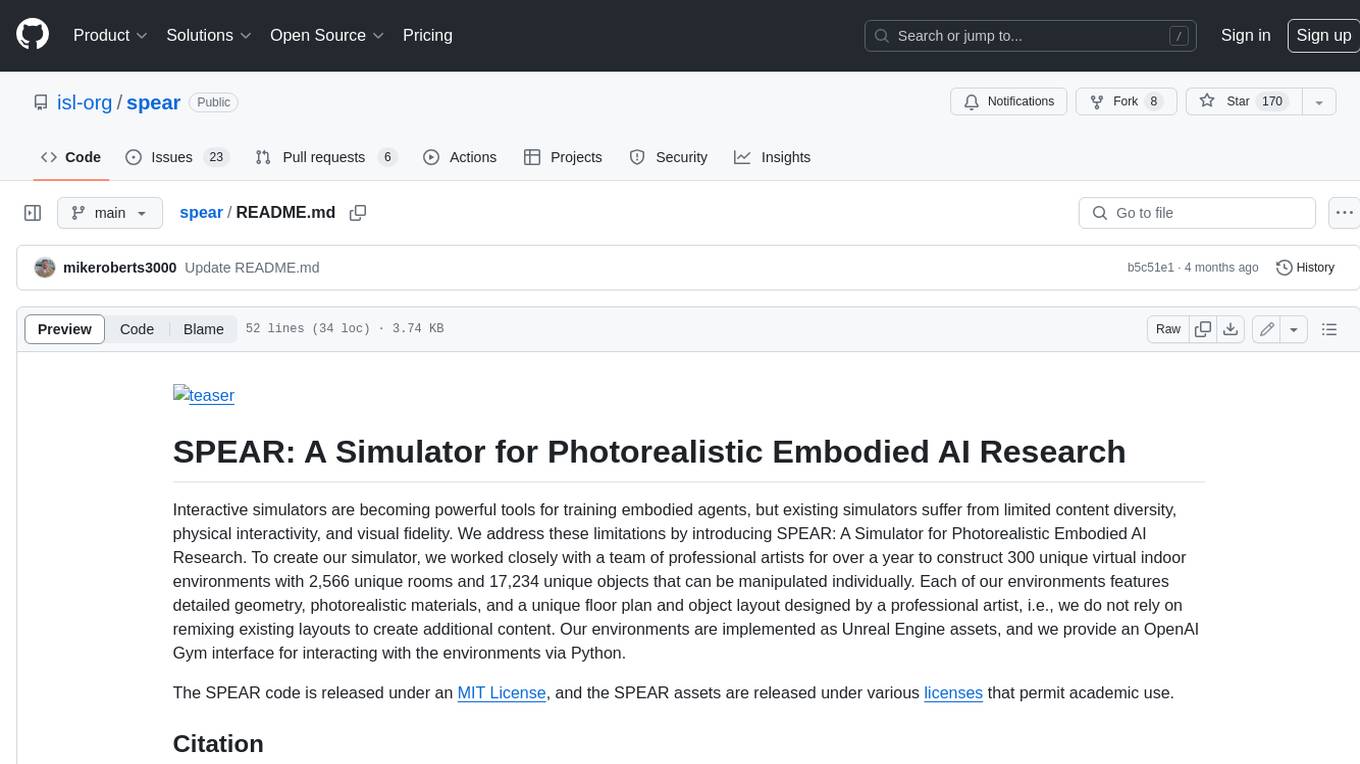
spear
SPEAR (Simulator for Photorealistic Embodied AI Research) is a powerful tool for training embodied agents. It features 300 unique virtual indoor environments with 2,566 unique rooms and 17,234 unique objects that can be manipulated individually. Each environment is designed by a professional artist and features detailed geometry, photorealistic materials, and a unique floor plan and object layout. SPEAR is implemented as Unreal Engine assets and provides an OpenAI Gym interface for interacting with the environments via Python.
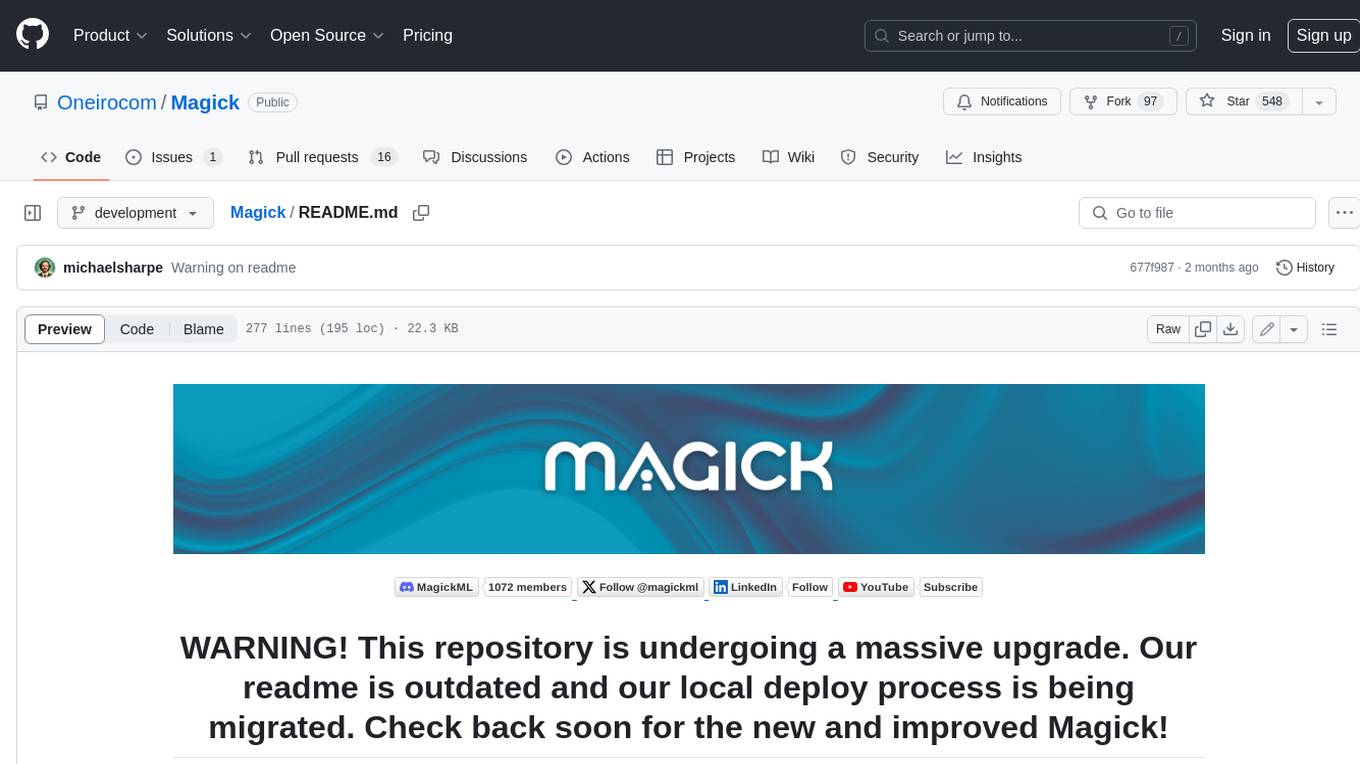
Magick
Magick is a groundbreaking visual AIDE (Artificial Intelligence Development Environment) for no-code data pipelines and multimodal agents. Magick can connect to other services and comes with nodes and templates well-suited for intelligent agents, chatbots, complex reasoning systems and realistic characters.