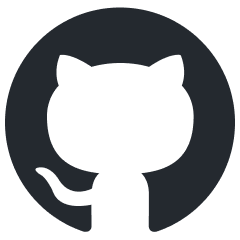
log10
Python client library for improving your LLM app accuracy
Stars: 96
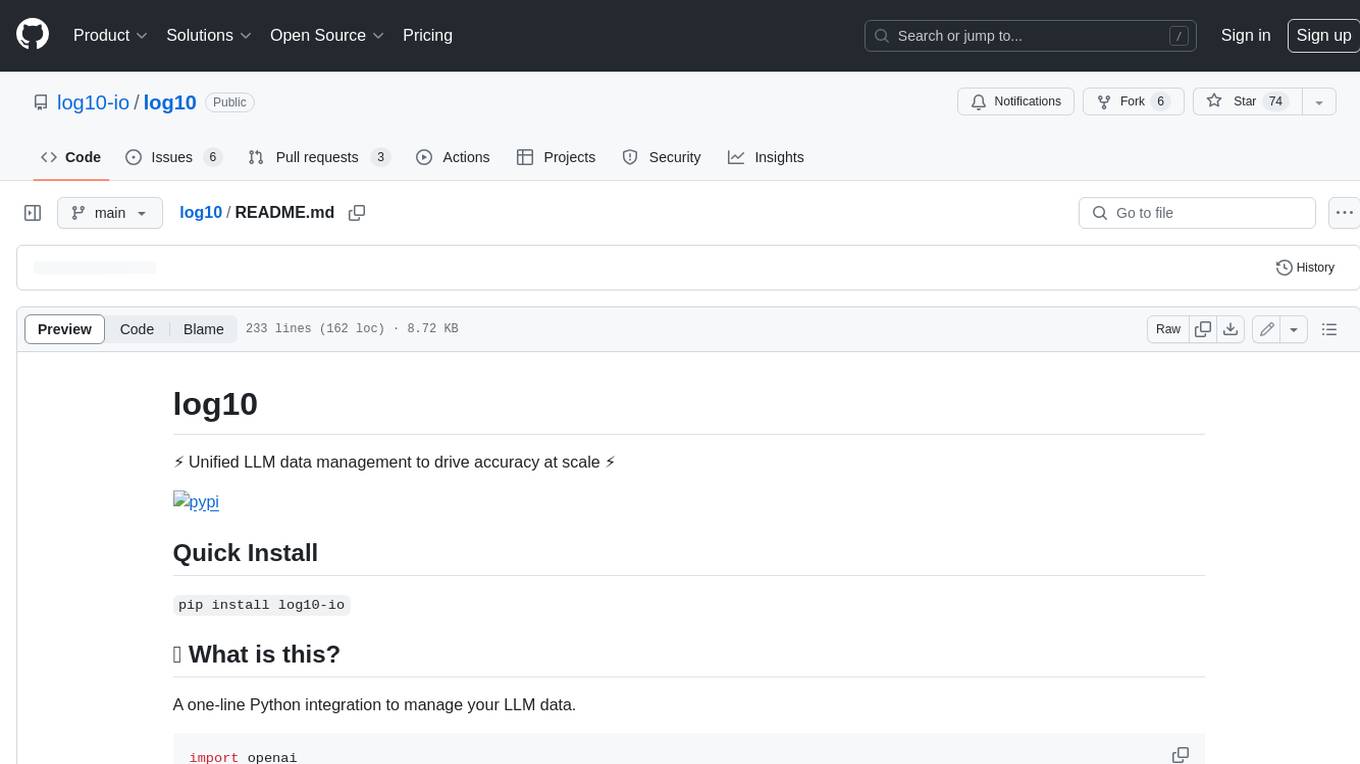
Log10 is a one-line Python integration to manage your LLM data. It helps you log both closed and open-source LLM calls, compare and identify the best models and prompts, store feedback for fine-tuning, collect performance metrics such as latency and usage, and perform analytics and monitor compliance for LLM powered applications. Log10 offers various integration methods, including a python LLM library wrapper, the Log10 LLM abstraction, and callbacks, to facilitate its use in both existing production environments and new projects. Pick the one that works best for you. Log10 also provides a copilot that can help you with suggestions on how to optimize your prompt, and a feedback feature that allows you to add feedback to your completions. Additionally, Log10 provides prompt provenance, session tracking and call stack functionality to help debug prompt chains. With Log10, you can use your data and feedback from users to fine-tune custom models with RLHF, and build and deploy more reliable, accurate and efficient self-hosted models. Log10 also supports collaboration, allowing you to create flexible groups to share and collaborate over all of the above features.
README:
β‘ Unified LLM data management to drive accuracy at scale β‘
pip install log10-io
A one-line Python integration to manage your LLM data.
import openai
from log10.load import log10
log10(openai)
# all your openai calls are now logged - including 3rd party libs using openai
For OpenAI v1, use from log10.load import OpenAI
instead of from openai import OpenAI
from log10.load import OpenAI
client = OpenAI()
Access your LLM data at log10.io
Use Log10 to log both closed and open-source LLM calls, e.g. OpenAI, Anthropic, Google Gemini, Llama, Mistral, etc. It helps you:
- Compare and identify the best models and prompts (try playground and llmeval)
- Store feedback for fine-tuning
- Collect performance metrics such as latency and usage
- Perform analytics and monitor compliance for LLM powered applications
Log10 offers various integration methods, including a python LLM library wrapper, the Log10 LLM abstraction, and callbacks, to facilitate its use in both existing production environments and new projects. Pick the one that works best for you.
log10 ver | openai v0 | openai v1 |
---|---|---|
0.4 |
log10(openai) β
|
β |
0.5+ |
log10(openai) β
|
from log10.load import OpenAI β
|
OpenAI v0 - Use library wrapper log10(openai)
. Check out examples/logging
in log10 version 0.4.6
.
import openai
from log10.load import log10
log10(openai)
# openai calls are now logged - including 3rd party libs using openai such as magentic or langchain
OpenAI v1
NOTE: We added OpenAI v1 API support in log10
0.5.0
release.load.log10(openai)
still works for openai v1. This also enables logging LLM completions from providers which support OpenAI API, such as Ollama.
from log10.load import OpenAI
# from openai import OpenAI
client = OpenAI()
completion = client.completions.create(model="gpt-3.5-turbo-instruct", prompt="Once upon a time")
# All completions.create and chat.completions.create calls will be logged
Full script here.
Use Log10 LLM abstraction
from log10.openai import OpenAI
llm = OpenAI({"model": "gpt-3.5-turbo"}, log10_config=Log10Config())
openai v1+ lib required. Full script here.
Use library wrapper log10(anthropic)
.
Full script here.
import anthropic
from log10.load import log10
log10(anthropic)
# anthropic calls are now logged
Use Log10 LLM abstraction. Full script here.
from log10.anthropic import Anthropic
llm = Anthropic({"model": "claude-2"}, log10_config=Log10Config())
We support OpenAI and Anthropic Async-client (e.g. AsyncOpenAI and AsyncAnthropic client) in their Python SDK
You could use the same code log10(openai)
or log10(anthropic)
and then call the async-client to start logging asynchronous mode (including streaming).
Release 0.9.0
includes significant improvements in how we handle concurrency while using LLM in asynchronous streaming mode.
This update is designed to ensure that logging at steady state incurs no overhead (previously up to 1-2 seconds), providing a smoother and more efficient experience in latency critical settings.
Important Considerations for Short-Lived Scripts:
π‘For short-lived scripts using asynchronous streaming, it's important to note that you may need to wait until all logging requests have been completed before terminating your script. We have provided a convenient method called
finalize()
to handle this. Here's how you can implement this in your code:
from log10._httpx_utils import finalize
...
await finalize()
Ensure finalize()
is called once, at the very end of your event loop to guarantee that all pending logging requests are processed before the script exits.
For more details, check async logging examples.
Log open-source LLM calls, e.g. Llama, Mistral, etc from providers. Currently we support inference endpoints on Together.AI and MosaicML (ranked on the top based on our benchmarking on Llama-2 inference providers). Adding other providers is on the roadmap.
If the providers support OpenAI API (e.g. Groq, vLLM, Together), you can easily starting logging using log10(openai)
.
MosaicML with LLM abstraction. Full script here.
from log10.mosaicml import MosaicML
llm = MosaicML({"model": "llama2-70b-chat/v1"}, log10_config=Log10Config())
Together with LLM abstraction. Full script here.
from log10.together import Together
llm = Together({"model": "togethercomputer/llama-2-70b-chat"}, log10_config=Log10Config())
Use Log10 callbacks if you use LangChain's LLM abstraction. Full script here.
from langchain.chat_models import ChatOpenAI
from langchain.schema import HumanMessage
from log10.langchain import Log10Callback
from log10.llm import Log10Config
log10_callback = Log10Callback(log10_config=Log10Config())
messages = [
HumanMessage(content="You are a ping pong machine"),
HumanMessage(content="Ping?"),
]
llm = ChatOpenAI(model_name="gpt-3.5-turbo", callbacks=[log10_callback])
Read more here for options for logging using library wrapper, langchain callback logger and how to apply log10 tags here.
Optimizing prompts requires a lot of manual effort. Log10 provides a copilot that can help you with suggestions on how to optimize your prompt.
Add feedback to your completions. Checkout the Python example
or use CLI log10 feedback-task create
and log10 feedback create
. Please check our doc for more details.
Leverage your current feedback and AI by using our AutoFeedback feature to generate feedback automatically. Hereβs a quick guide:
- Summary feedback: Use TLDR summary feedback rubics to rate summarization. E.g.
log10 feedback predict --task_id $FEEDBACK_TASK_ID --content '{"prompt": "this is article", "response": "summary of the article."}'
.- You can pass a file containing the context with
--file
or pass a completion from your Log10 logs with--completion_id
.
- You can pass a file containing the context with
- Custom Feedback Rubrics: Integrate your own feedback criteria for personalized assessments.
- Getting Started: To explore all options and usage details, use CLI
log10 feedback predict --help
.
Feel free to integrate AutoFeedback into your workflow to enhance the feedback and evaluation process.
Easily benchmark your logged completions using LLM models from OpenAI, Anthropic, Mistral, Meta, etc., by using the log10 completions benchmark_models
command in the log10 CLI.
Generate detailed reports and gain insights to enhance your model's performance and cost.
Please refer to the cli doc or the demo video for details.
Prompt chains such as those in Langchain can be difficult to debug. Log10 provides prompt provenance, session tracking and call stack functionality to help debug chains.
Use your data and feedback from users to fine-tune custom models with RLHF with the option of building and deploying more reliable, accurate and efficient self-hosted models.
Create flexible groups to share and collaborate over all of the above features
- Create a free account at log10.io
- Set the following environment variables:
LOG10_URL=https://log10.io
-
LOG10_TOKEN
: From the Settings tab in log10.io -
LOG10_ORG_ID
: From the Organization tab in log10.io -
OPENAI_API_KEY
: OpenAI API key -
ANTHROPIC_API_KEY
: Anthropic API key
You can find and run examples under folder examples
, e.g. run a logging example:
python examples/logging/chatcompletion.py
Also you can run some end-to-end tests with xdocttest
installed (pip install xdoctest
).
# list all tests
python -m xdoctest log10 list
# run all tests
python -m xdoctest log10 all
# run a single test, e.g.
python -m xdoctest /Users/wenzhe/dev/log10/log10/load.py log10:0
Few options to enable debug logging:
- set environment varible
export LOG10_DEBUG=1
- set
log10.load.log10(DEBUG_=True)
when usinglog10.load
- set
log10_config(DEBUG=True)
when using llm abstraction classes or callback.
log10 provides a managed data store, but if you'd prefer to manage data in your own environment, you can use data stores like google big query.
Install the big query client library with:
pip install log10-io[bigquery]
And provide the following configuration in either a .env
file, or as environment variables:
Name | Description |
---|---|
LOG10_DATA_STORE |
Either log10 or bigquery
|
LOG10_BQ_PROJECT_ID |
Your google cloud project id |
LOG10_BQ_DATASET_ID |
The big query dataset id |
LOG10_BQ_COMPLETIONS_TABLE_ID |
The name of the table to store completions in |
Note that your environment should have been setup with google cloud credentials. Read more here about authenticating.
We add CLI to manage your completions and feedback. Read more here.
We welcome community participation and feedback. Please leave an issue, submit a PR or join our Discord. For enterprise use cases, please contact us to set up a shared slack channel.
For Tasks:
Click tags to check more tools for each tasksFor Jobs:
Alternative AI tools for log10
Similar Open Source Tools
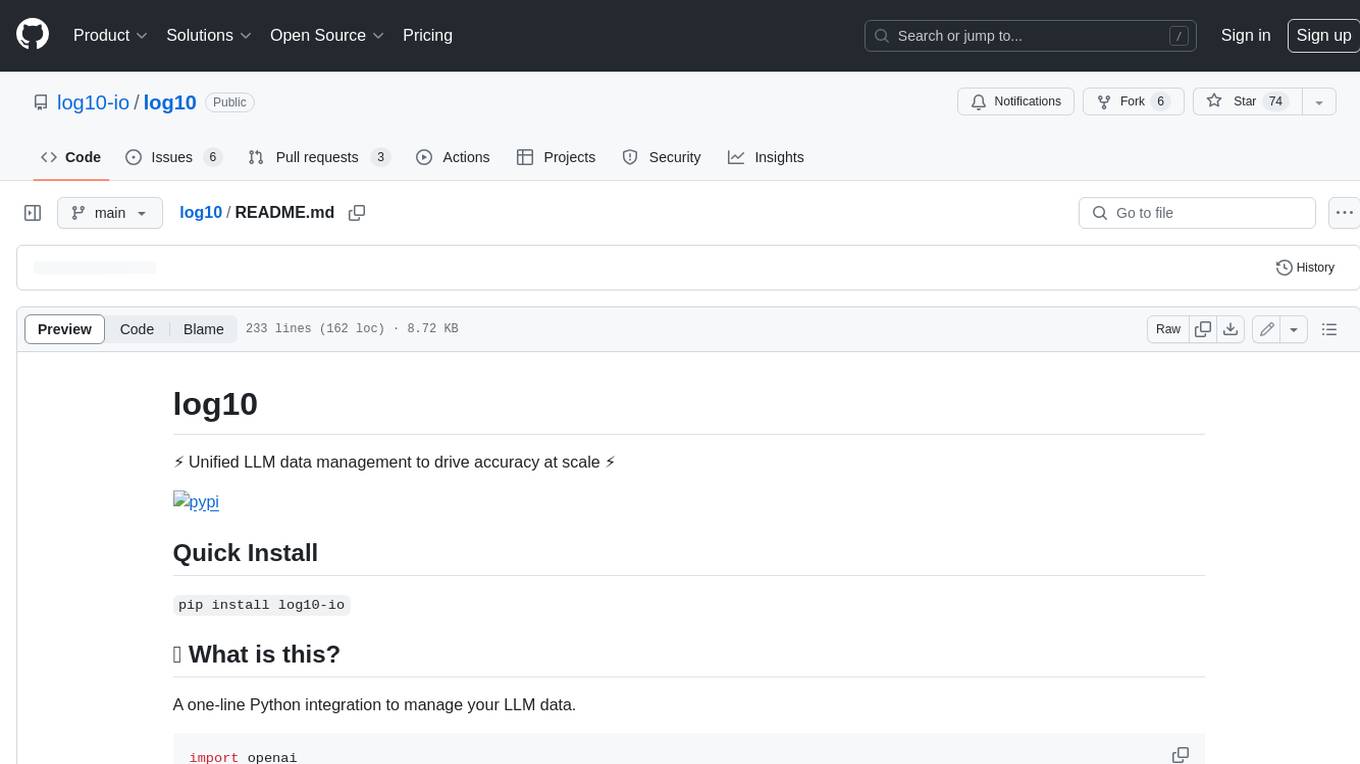
log10
Log10 is a one-line Python integration to manage your LLM data. It helps you log both closed and open-source LLM calls, compare and identify the best models and prompts, store feedback for fine-tuning, collect performance metrics such as latency and usage, and perform analytics and monitor compliance for LLM powered applications. Log10 offers various integration methods, including a python LLM library wrapper, the Log10 LLM abstraction, and callbacks, to facilitate its use in both existing production environments and new projects. Pick the one that works best for you. Log10 also provides a copilot that can help you with suggestions on how to optimize your prompt, and a feedback feature that allows you to add feedback to your completions. Additionally, Log10 provides prompt provenance, session tracking and call stack functionality to help debug prompt chains. With Log10, you can use your data and feedback from users to fine-tune custom models with RLHF, and build and deploy more reliable, accurate and efficient self-hosted models. Log10 also supports collaboration, allowing you to create flexible groups to share and collaborate over all of the above features.
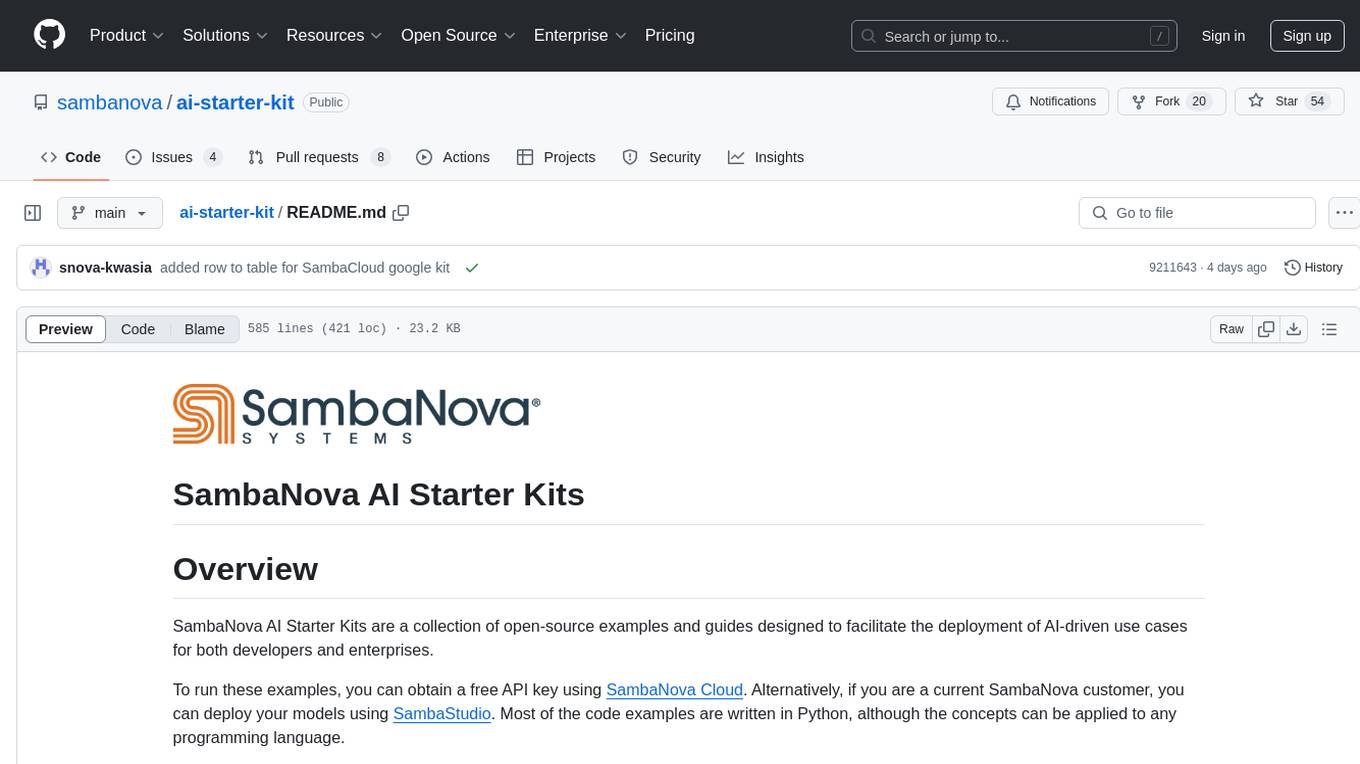
ai-starter-kit
SambaNova AI Starter Kits is a collection of open-source examples and guides designed to facilitate the deployment of AI-driven use cases for developers and enterprises. The kits cover various categories such as Data Ingestion & Preparation, Model Development & Optimization, Intelligent Information Retrieval, and Advanced AI Capabilities. Users can obtain a free API key using SambaNova Cloud or deploy models using SambaStudio. Most examples are written in Python but can be applied to any programming language. The kits provide resources for tasks like text extraction, fine-tuning embeddings, prompt engineering, question-answering, image search, post-call analysis, and more.
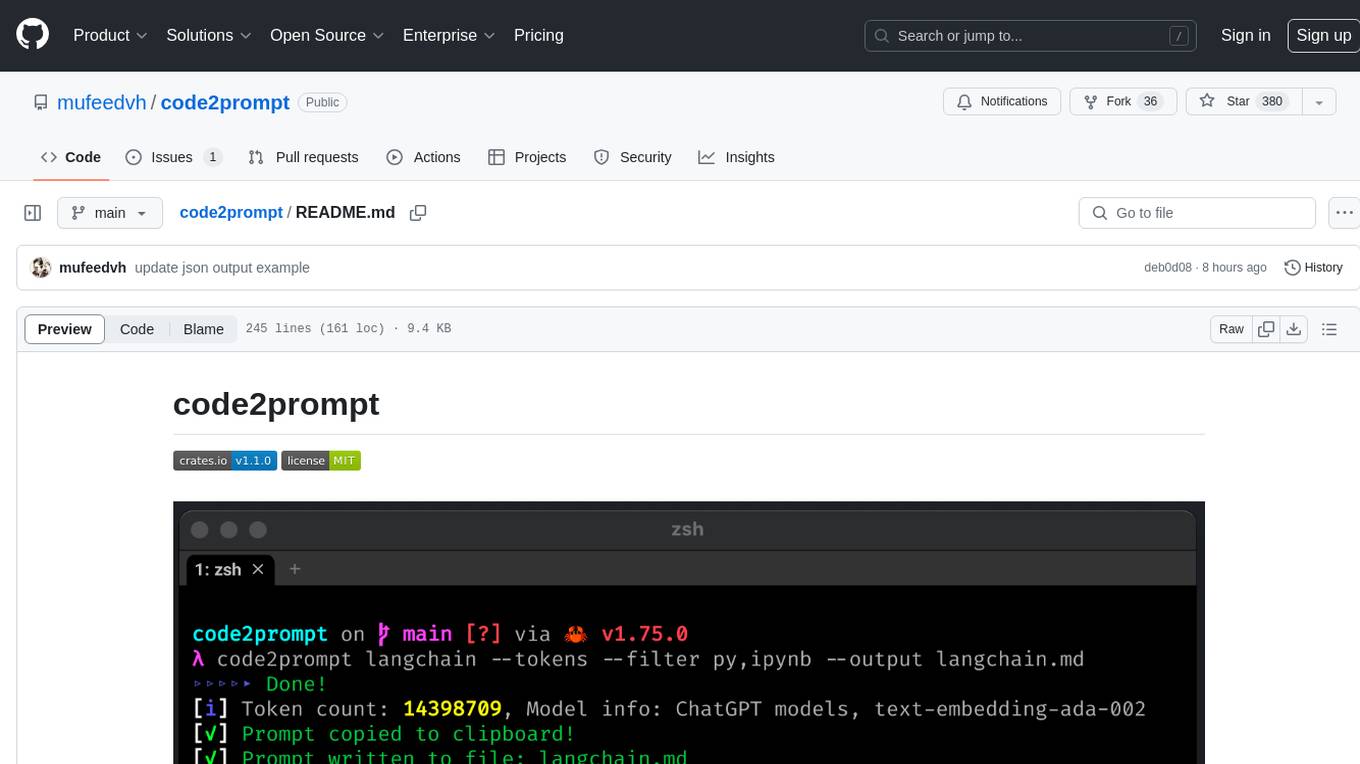
code2prompt
code2prompt is a command-line tool that converts your codebase into a single LLM prompt with a source tree, prompt templating, and token counting. It automates generating LLM prompts from codebases of any size, customizing prompt generation with Handlebars templates, respecting .gitignore, filtering and excluding files using glob patterns, displaying token count, including Git diff output, copying prompt to clipboard, saving prompt to an output file, excluding files and folders, adding line numbers to source code blocks, and more. It helps streamline the process of creating LLM prompts for code analysis, generation, and other tasks.
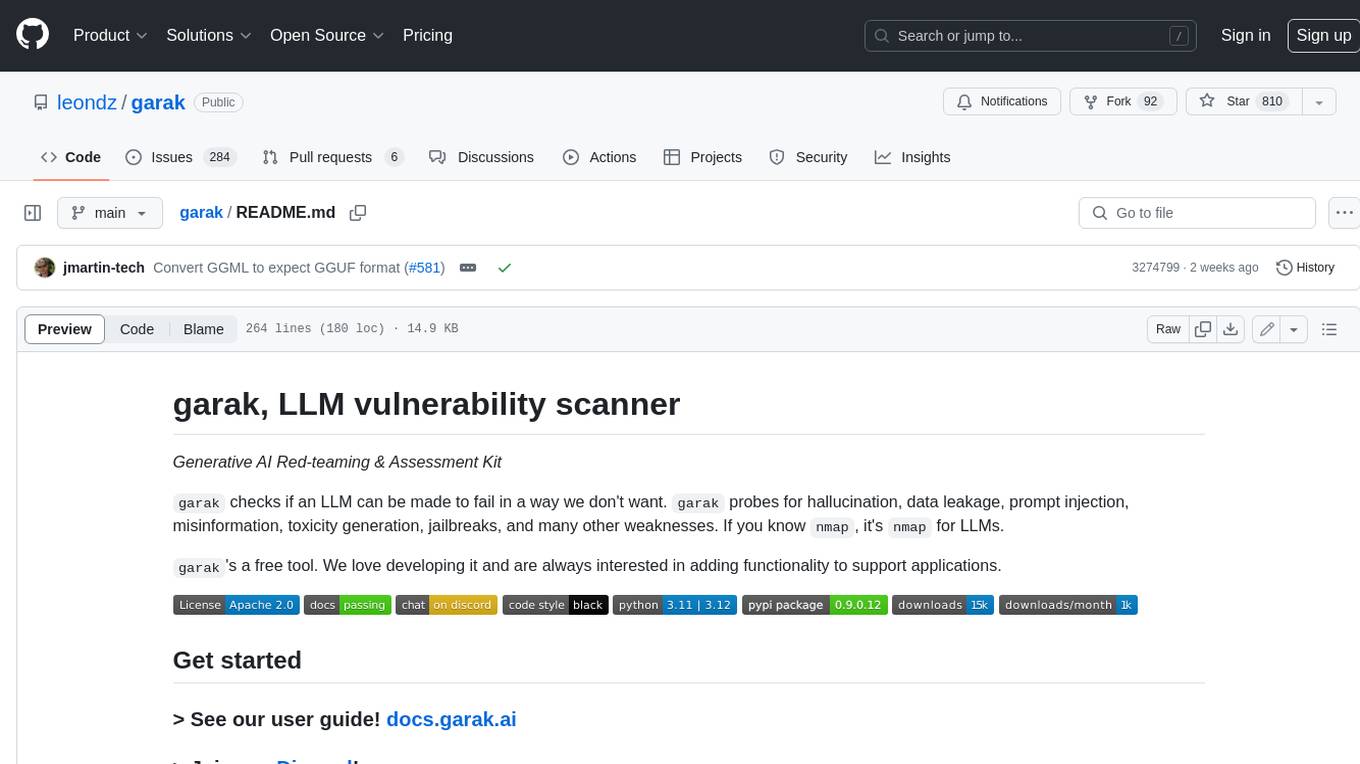
garak
Garak is a free tool that checks if a Large Language Model (LLM) can be made to fail in a way that is undesirable. It probes for hallucination, data leakage, prompt injection, misinformation, toxicity generation, jailbreaks, and many other weaknesses. Garak's a free tool. We love developing it and are always interested in adding functionality to support applications.
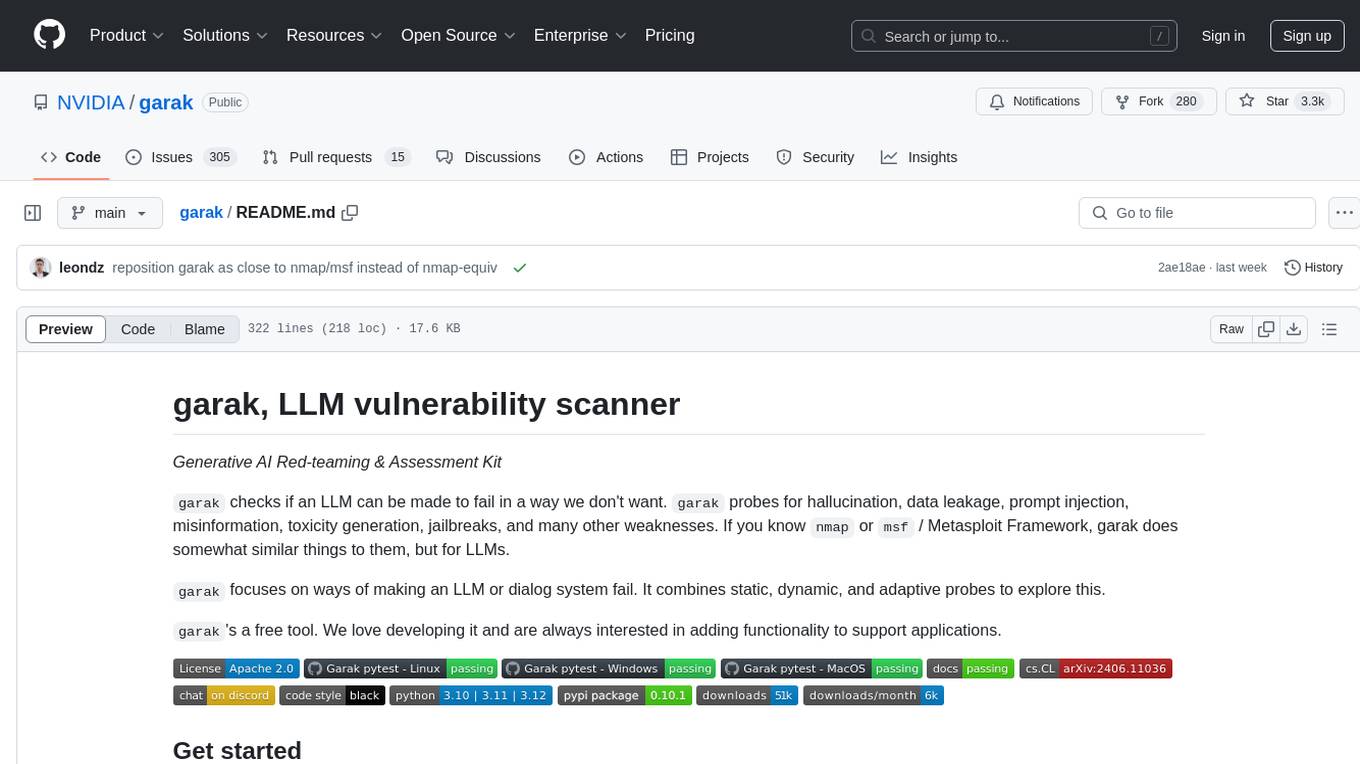
garak
Garak is a vulnerability scanner designed for LLMs (Large Language Models) that checks for various weaknesses such as hallucination, data leakage, prompt injection, misinformation, toxicity generation, and jailbreaks. It combines static, dynamic, and adaptive probes to explore vulnerabilities in LLMs. Garak is a free tool developed for red-teaming and assessment purposes, focusing on making LLMs or dialog systems fail. It supports various LLM models and can be used to assess their security and robustness.
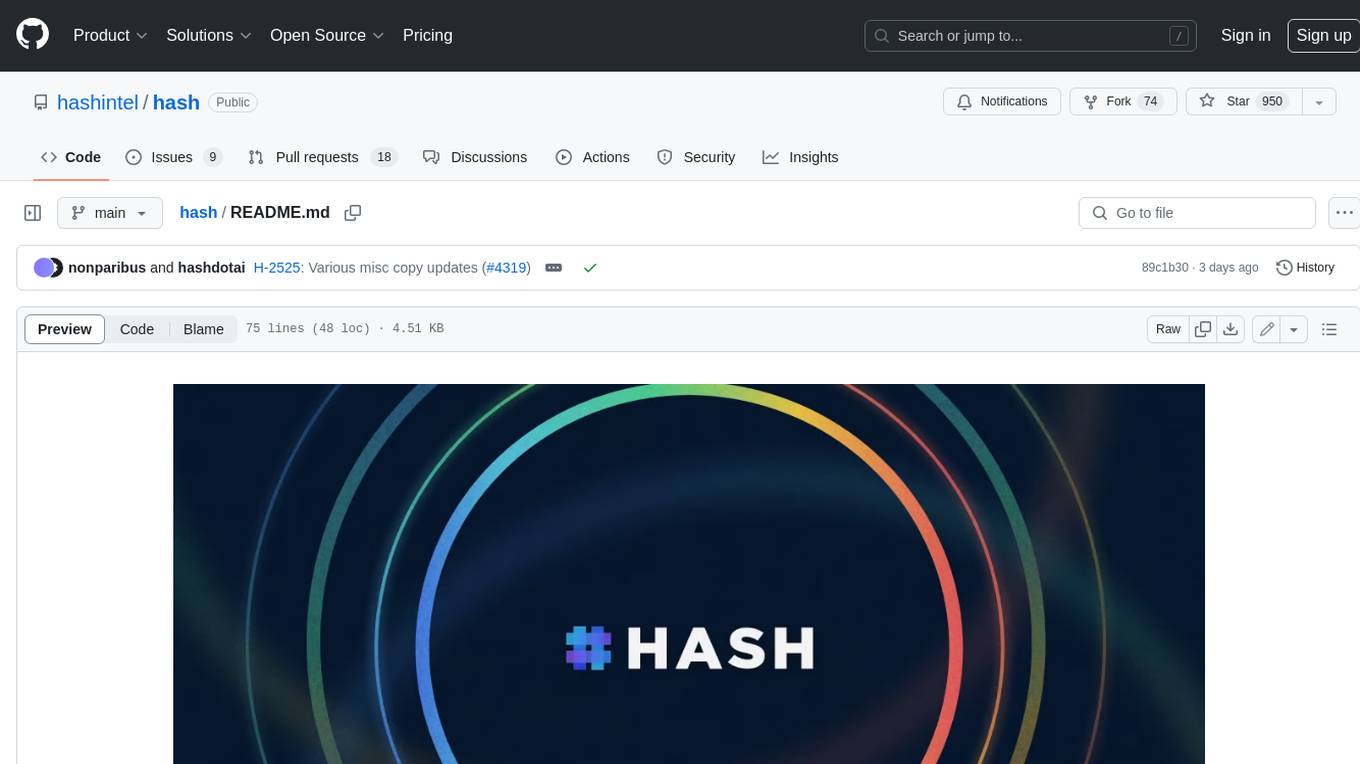
hash
HASH is a self-building, open-source database which grows, structures and checks itself. With it, we're creating a platform for decision-making, which helps you integrate, understand and use data in a variety of different ways.
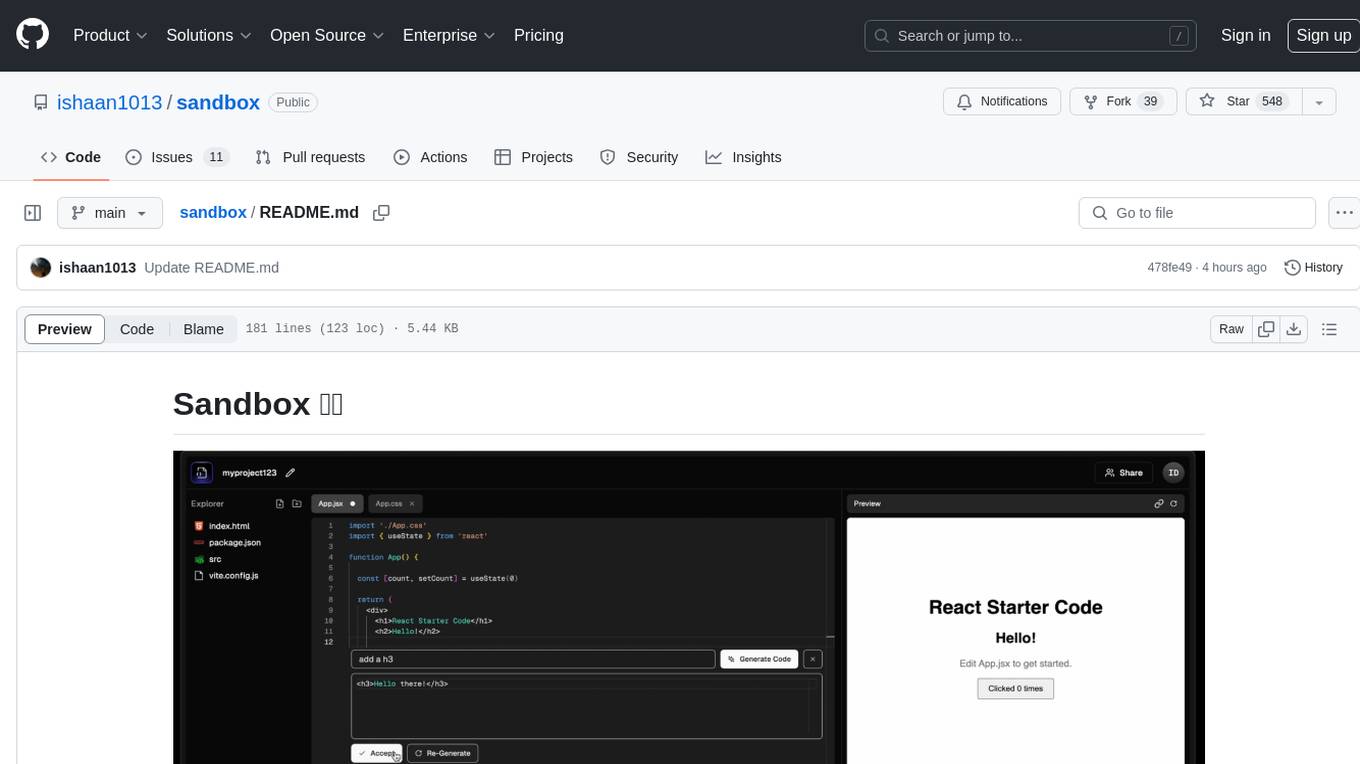
sandbox
Sandbox is an open-source cloud-based code editing environment with custom AI code autocompletion and real-time collaboration. It consists of a frontend built with Next.js, TailwindCSS, Shadcn UI, Clerk, Monaco, and Liveblocks, and a backend with Express, Socket.io, Cloudflare Workers, D1 database, R2 storage, Workers AI, and Drizzle ORM. The backend includes microservices for database, storage, and AI functionalities. Users can run the project locally by setting up environment variables and deploying the containers. Contributions are welcome following the commit convention and structure provided in the repository.
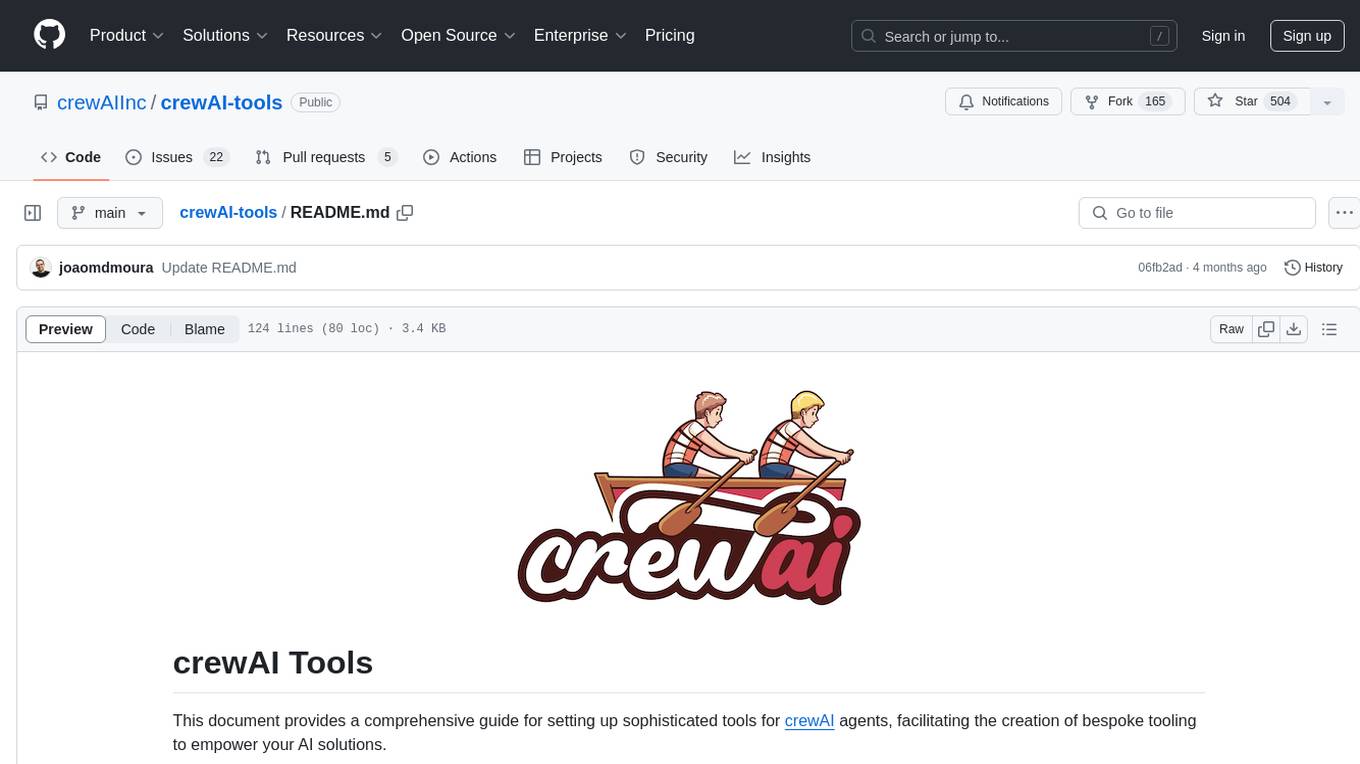
crewAI-tools
This repository provides a guide for setting up tools for crewAI agents to enhance functionality. It offers steps to equip agents with ready-to-use tools and create custom ones. Tools are expected to return strings for generating responses. Users can create tools by subclassing BaseTool or using the tool decorator. Contributions are welcome to enrich the toolset, and guidelines are provided for contributing. The development setup includes installing dependencies, activating virtual environment, setting up pre-commit hooks, running tests, static type checking, packaging, and local installation. The goal is to empower AI solutions through advanced tooling.
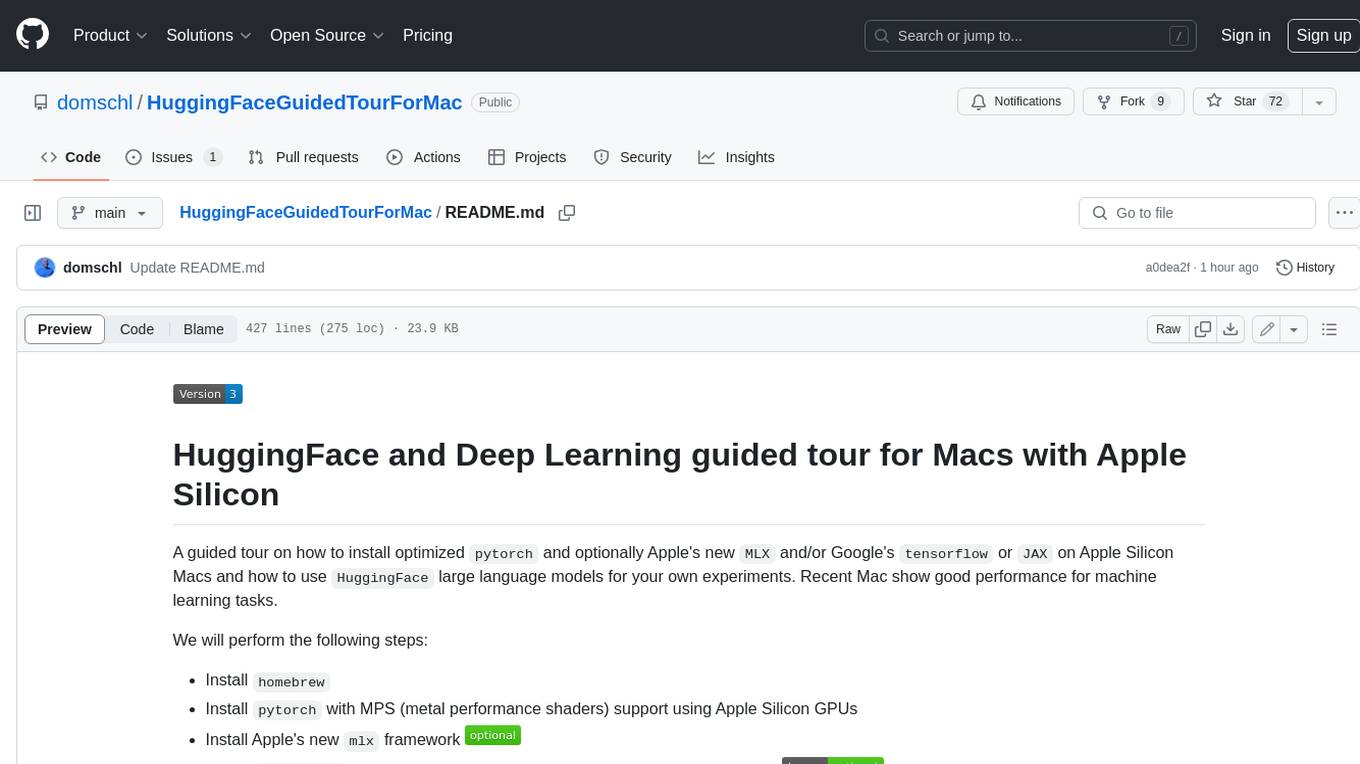
HuggingFaceGuidedTourForMac
HuggingFaceGuidedTourForMac is a guided tour on how to install optimized pytorch and optionally Apple's new MLX, JAX, and TensorFlow on Apple Silicon Macs. The repository provides steps to install homebrew, pytorch with MPS support, MLX, JAX, TensorFlow, and Jupyter lab. It also includes instructions on running large language models using HuggingFace transformers. The repository aims to help users set up their Macs for deep learning experiments with optimized performance.
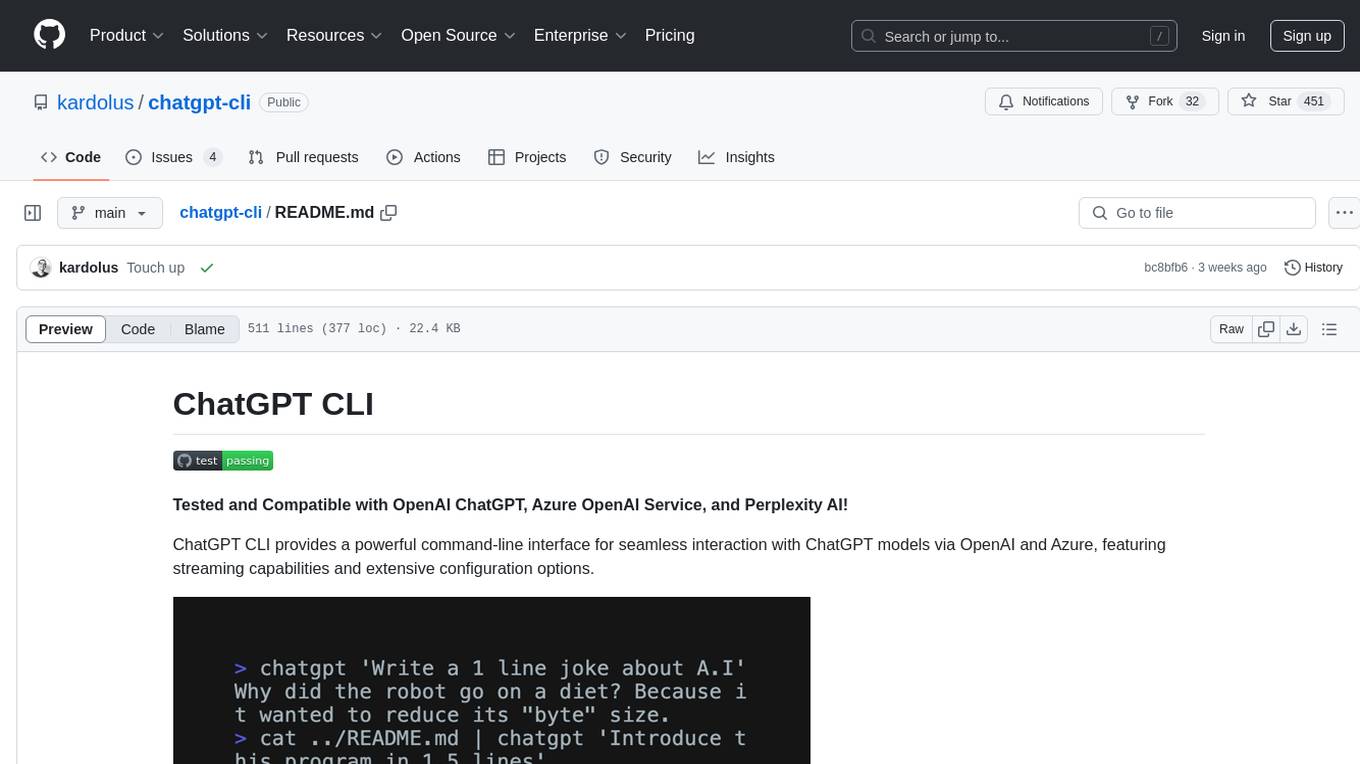
chatgpt-cli
ChatGPT CLI provides a powerful command-line interface for seamless interaction with ChatGPT models via OpenAI and Azure. It features streaming capabilities, extensive configuration options, and supports various modes like streaming, query, and interactive mode. Users can manage thread-based context, sliding window history, and provide custom context from any source. The CLI also offers model and thread listing, advanced configuration options, and supports GPT-4, GPT-3.5-turbo, and Perplexity's models. Installation is available via Homebrew or direct download, and users can configure settings through default values, a config.yaml file, or environment variables.
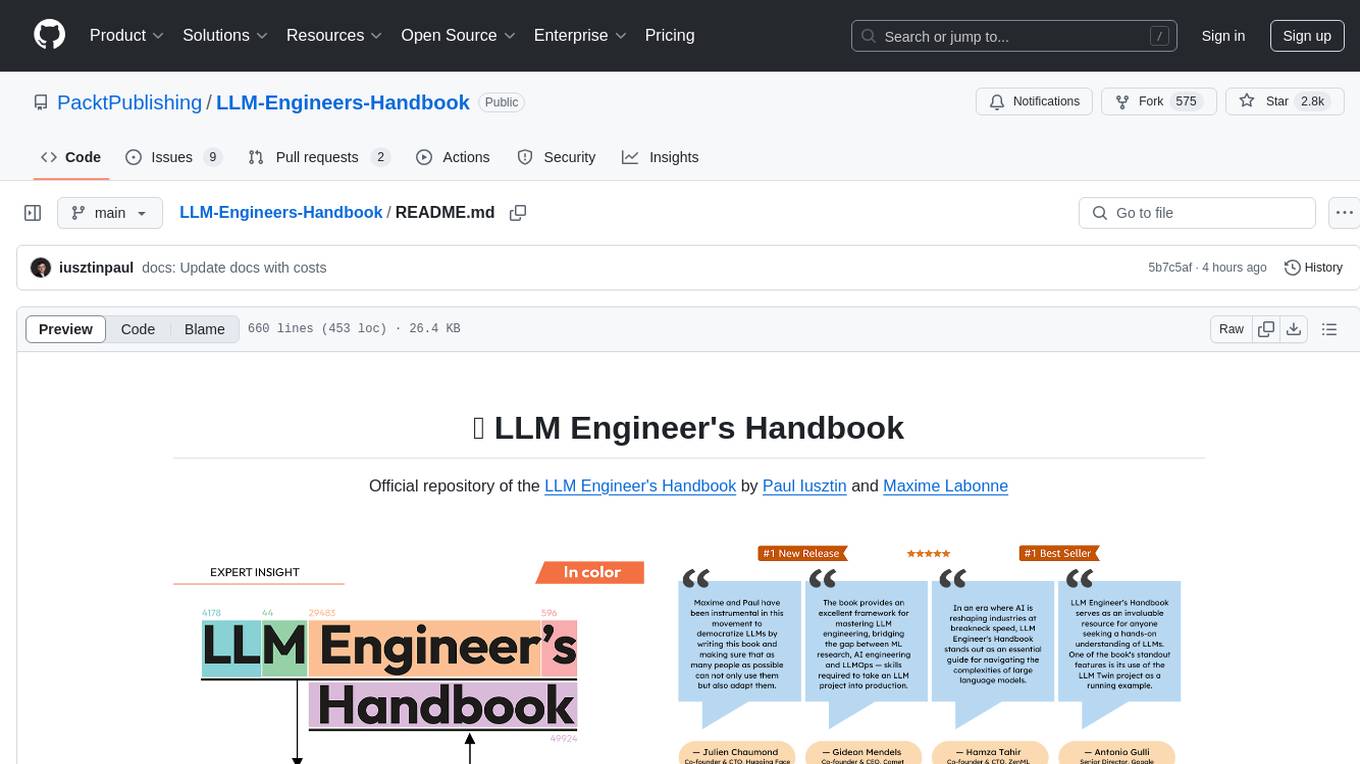
LLM-Engineers-Handbook
The LLM Engineer's Handbook is an official repository containing a comprehensive guide on creating an end-to-end LLM-based system using best practices. It covers data collection & generation, LLM training pipeline, a simple RAG system, production-ready AWS deployment, comprehensive monitoring, and testing and evaluation framework. The repository includes detailed instructions on setting up local and cloud dependencies, project structure, installation steps, infrastructure setup, pipelines for data processing, training, and inference, as well as QA, tests, and running the project end-to-end.
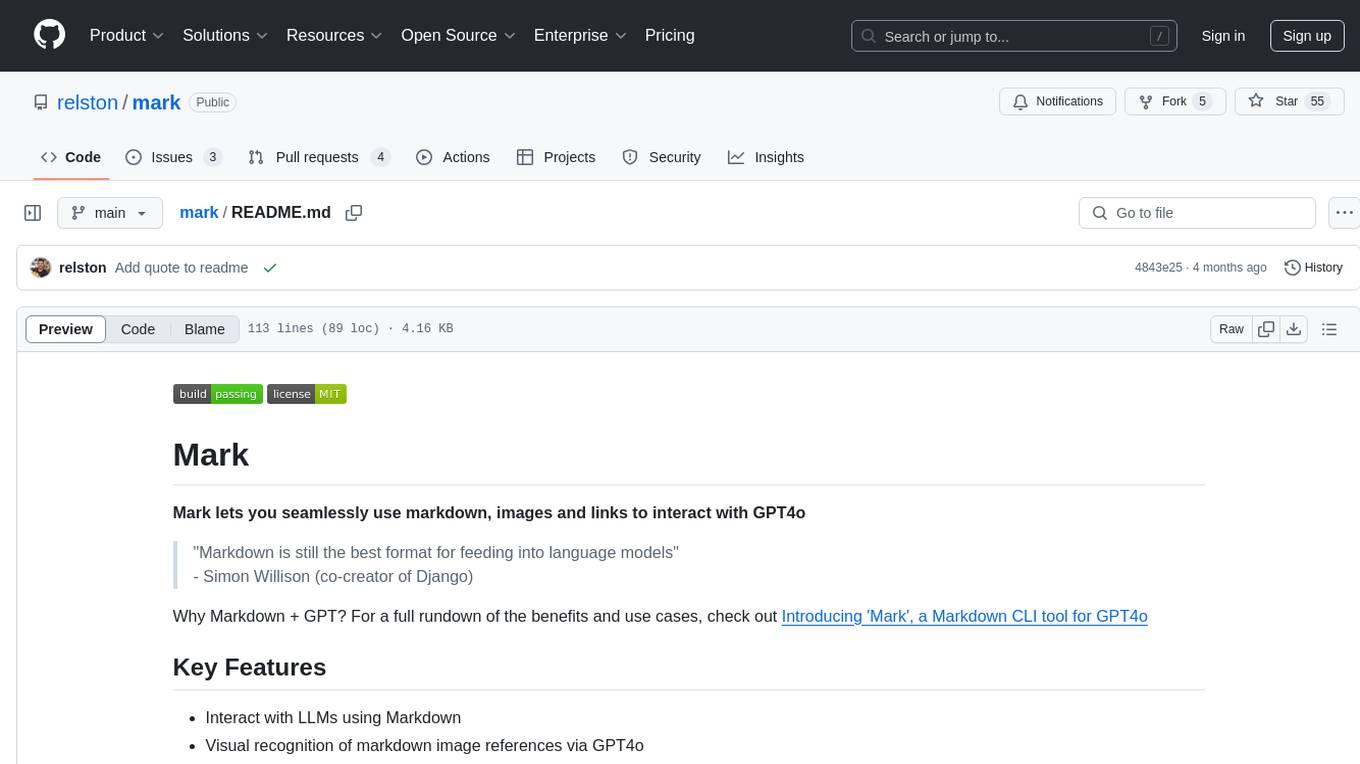
mark
Mark is a CLI tool that allows users to interact with large language models (LLMs) using Markdown format. It enables users to seamlessly integrate GPT responses into Markdown files, supports image recognition, scraping of local and remote links, and image generation. Mark focuses on using Markdown as both a prompt and response medium for LLMs, offering a unique and flexible way to interact with language models for various use cases in development and documentation processes.
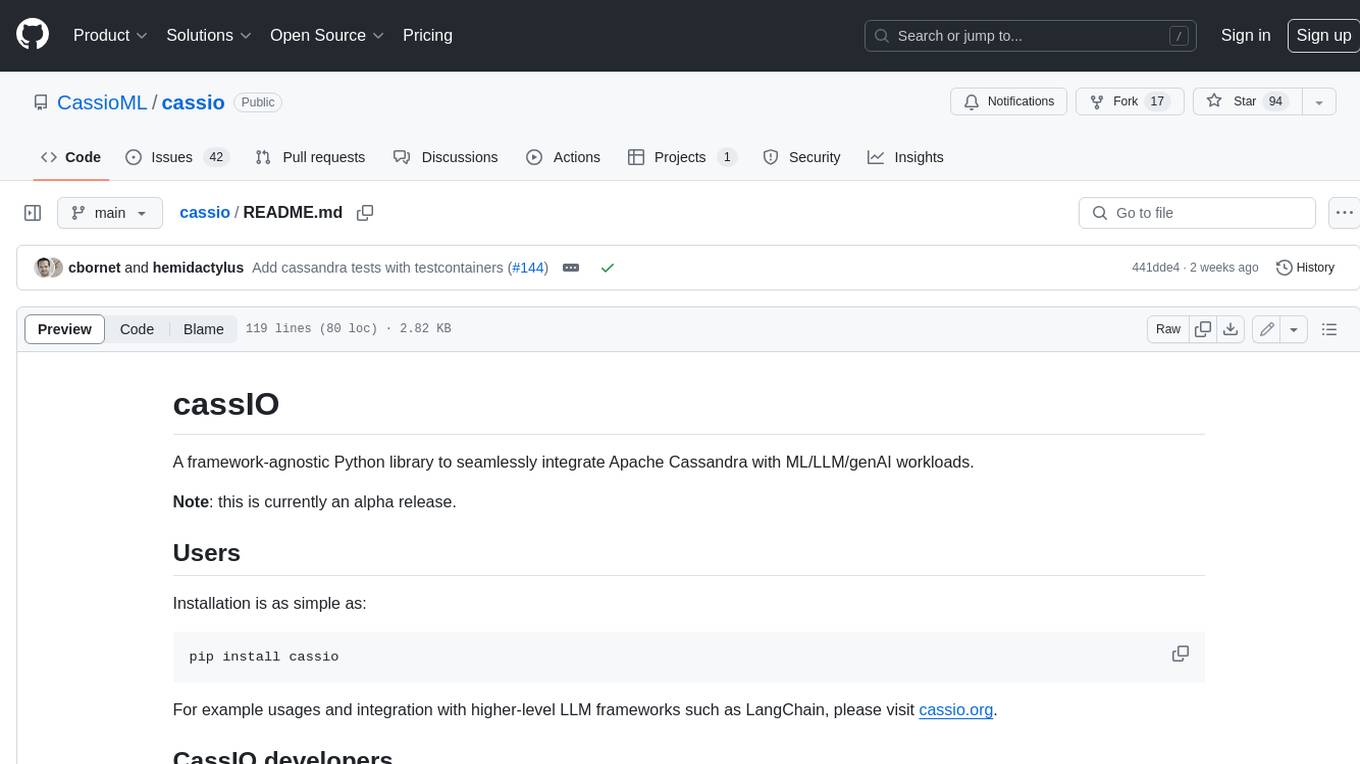
cassio
cassIO is a framework-agnostic Python library that seamlessly integrates Apache Cassandra with ML/LLM/genAI workloads. It provides an easy-to-use interface for developers to connect their Cassandra databases to machine learning models, allowing them to perform complex data analysis and AI-powered tasks directly on their Cassandra data. cassIO is designed to be flexible and extensible, making it suitable for a wide range of use cases, from data exploration and visualization to predictive modeling and natural language processing.
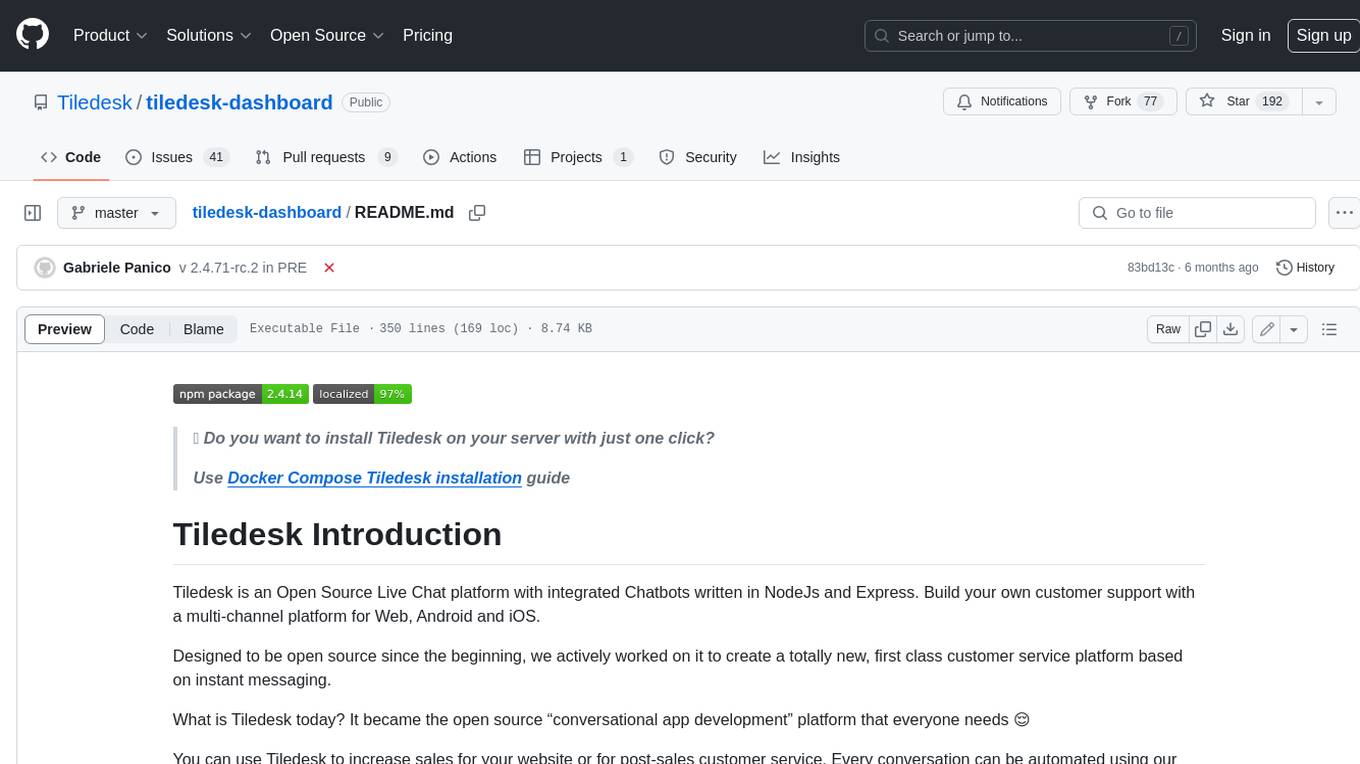
tiledesk-dashboard
Tiledesk is an open-source live chat platform with integrated chatbots written in Node.js and Express. It is designed to be a multi-channel platform for web, Android, and iOS, and it can be used to increase sales or provide post-sales customer service. Tiledesk's chatbot technology allows for automation of conversations, and it also provides APIs and webhooks for connecting external applications. Additionally, it offers a marketplace for apps and features such as CRM, ticketing, and data export.
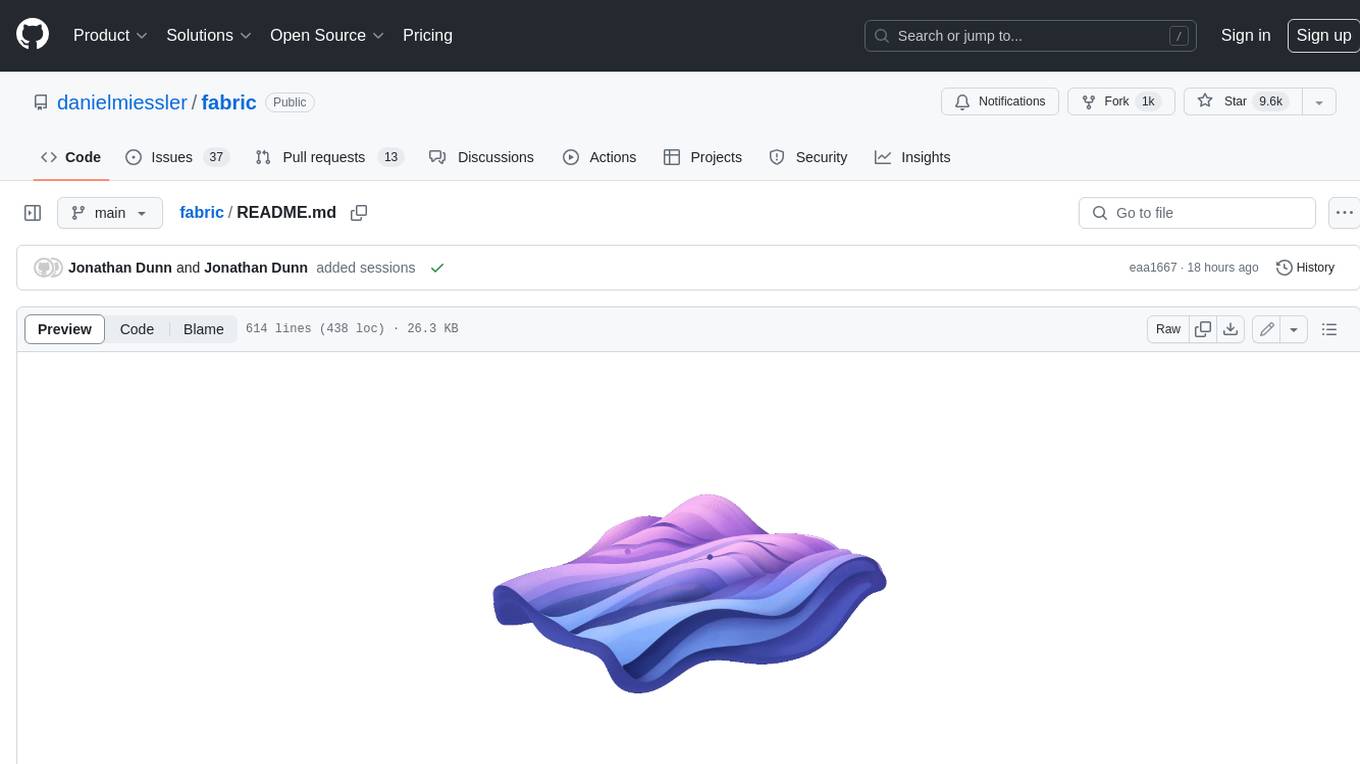
fabric
Fabric is an open-source framework for augmenting humans using AI. It provides a structured approach to breaking down problems into individual components and applying AI to them one at a time. Fabric includes a collection of pre-defined Patterns (prompts) that can be used for a variety of tasks, such as extracting the most interesting parts of YouTube videos and podcasts, writing essays, summarizing academic papers, creating AI art prompts, and more. Users can also create their own custom Patterns. Fabric is designed to be easy to use, with a command-line interface and a variety of helper apps. It is also extensible, allowing users to integrate it with their own AI applications and infrastructure.
For similar tasks
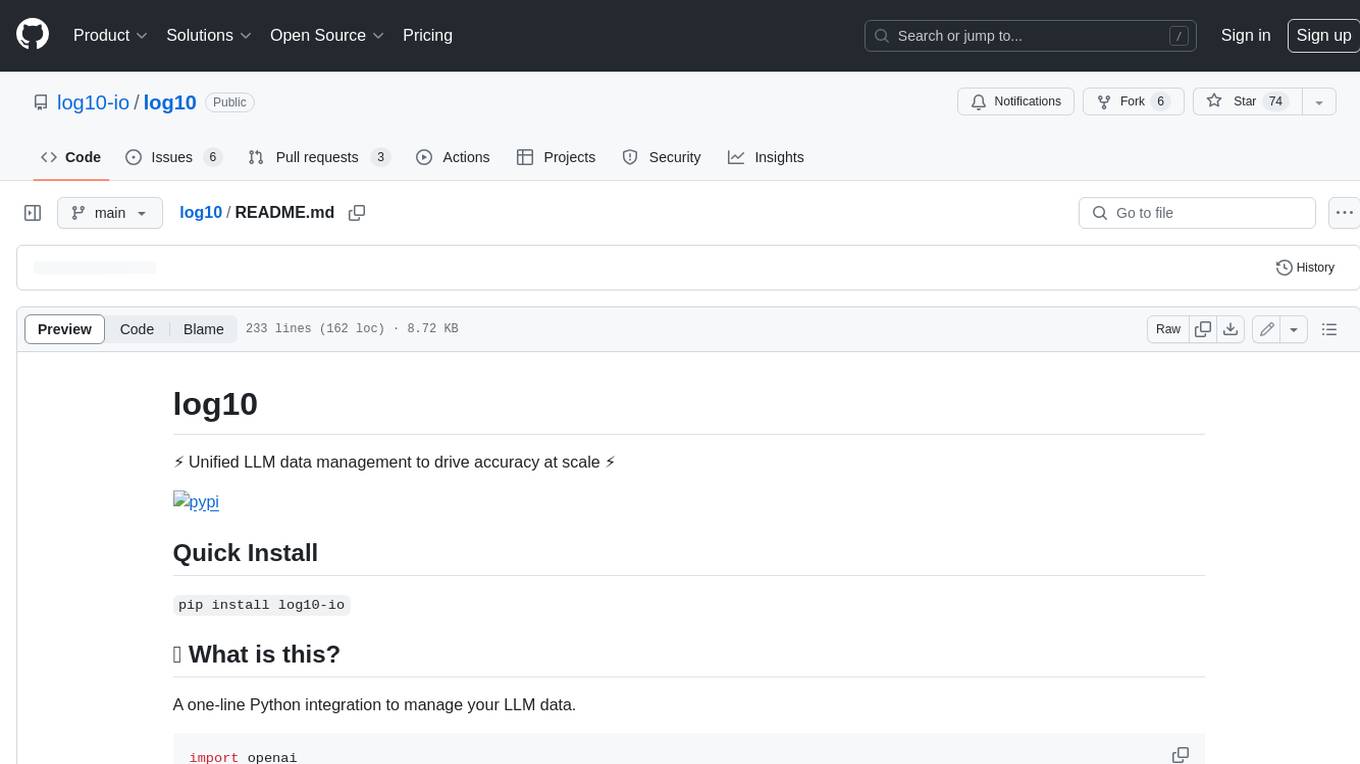
log10
Log10 is a one-line Python integration to manage your LLM data. It helps you log both closed and open-source LLM calls, compare and identify the best models and prompts, store feedback for fine-tuning, collect performance metrics such as latency and usage, and perform analytics and monitor compliance for LLM powered applications. Log10 offers various integration methods, including a python LLM library wrapper, the Log10 LLM abstraction, and callbacks, to facilitate its use in both existing production environments and new projects. Pick the one that works best for you. Log10 also provides a copilot that can help you with suggestions on how to optimize your prompt, and a feedback feature that allows you to add feedback to your completions. Additionally, Log10 provides prompt provenance, session tracking and call stack functionality to help debug prompt chains. With Log10, you can use your data and feedback from users to fine-tune custom models with RLHF, and build and deploy more reliable, accurate and efficient self-hosted models. Log10 also supports collaboration, allowing you to create flexible groups to share and collaborate over all of the above features.
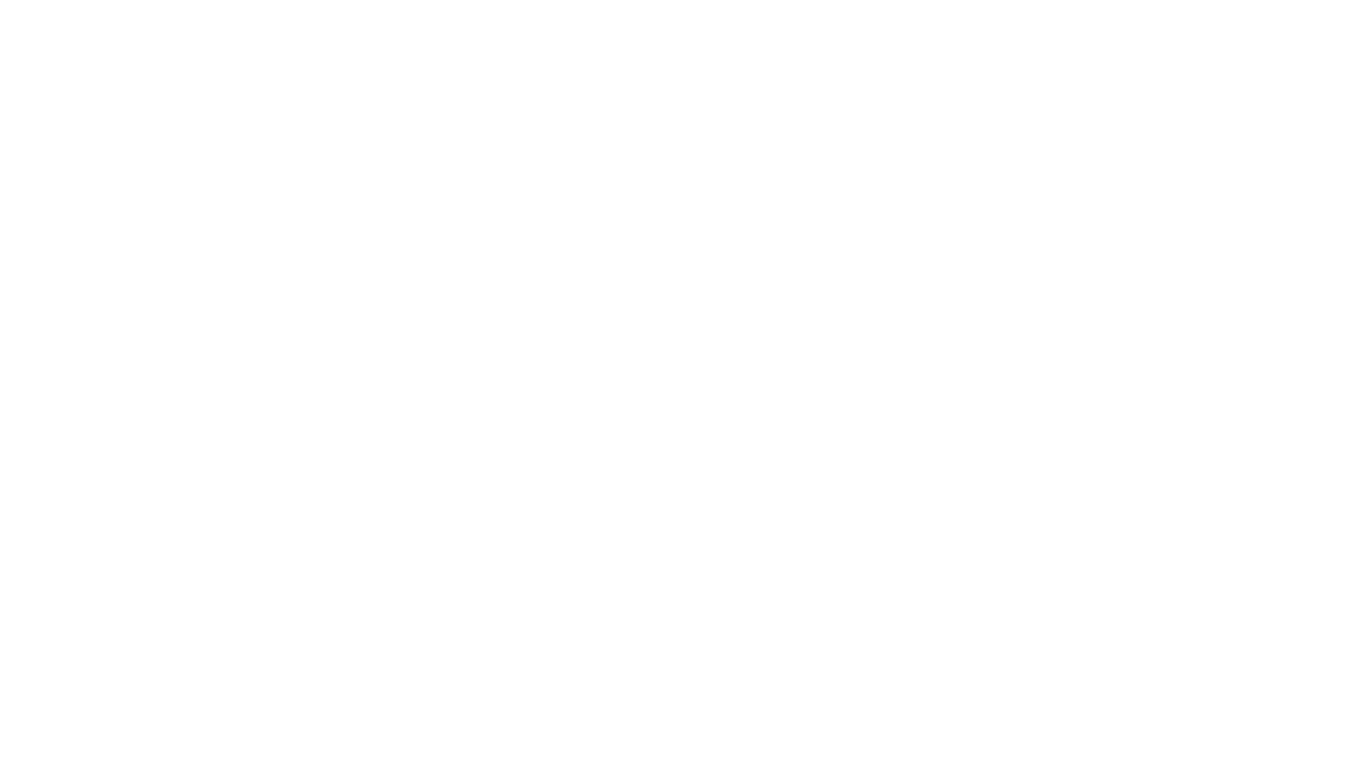
LMOps
LMOps is a research initiative focusing on fundamental research and technology for building AI products with foundation models, particularly enabling AI capabilities with Large Language Models (LLMs) and Generative AI models. The project explores various aspects such as prompt optimization, longer context handling, LLM alignment, acceleration of LLMs, LLM customization, and understanding in-context learning. It also includes tools like Promptist for automatic prompt optimization, Structured Prompting for efficient long-sequence prompts consumption, and X-Prompt for extensible prompts beyond natural language. Additionally, LLMA accelerators are developed to speed up LLM inference by referencing and copying text spans from documents. The project aims to advance technologies that facilitate prompting language models and enhance the performance of LLMs in various scenarios.
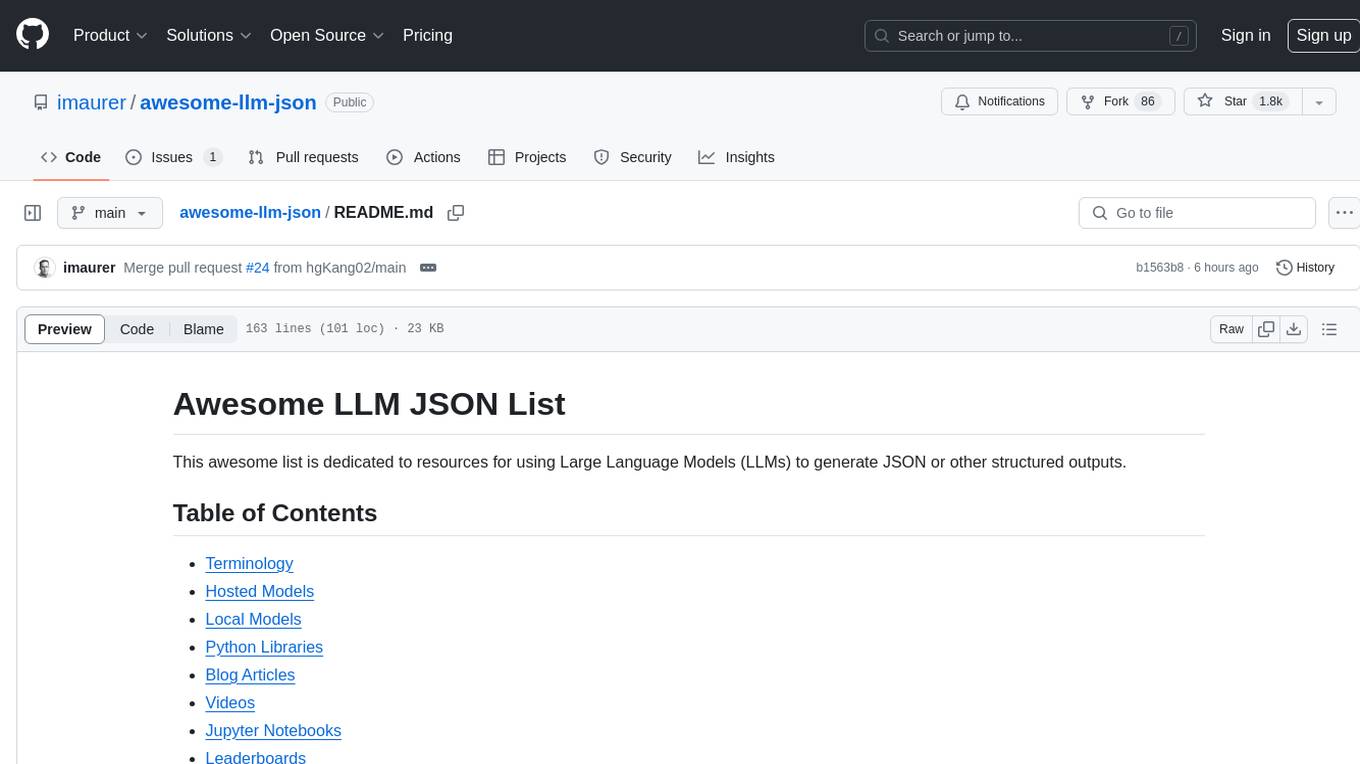
awesome-llm-json
This repository is an awesome list dedicated to resources for using Large Language Models (LLMs) to generate JSON or other structured outputs. It includes terminology explanations, hosted and local models, Python libraries, blog articles, videos, Jupyter notebooks, and leaderboards related to LLMs and JSON generation. The repository covers various aspects such as function calling, JSON mode, guided generation, and tool usage with different providers and models.
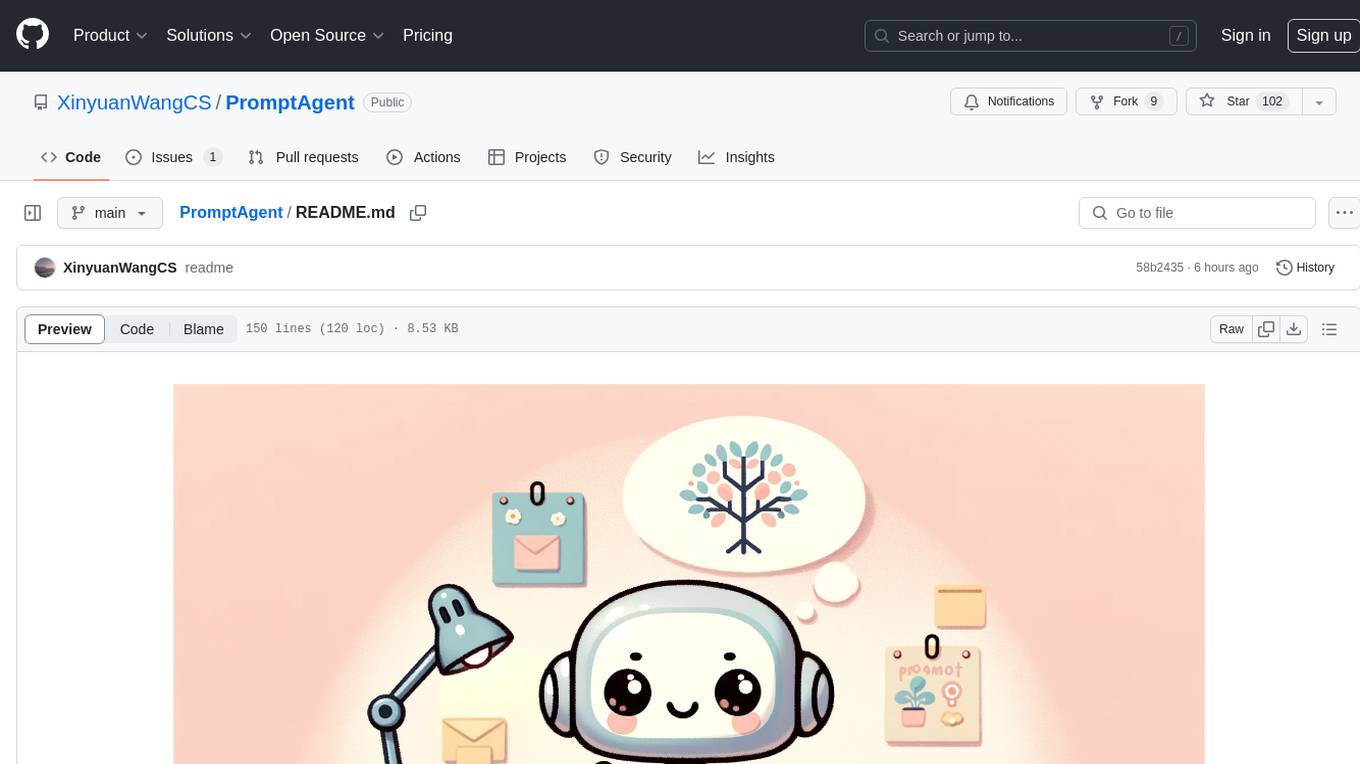
PromptAgent
PromptAgent is a repository for a novel automatic prompt optimization method that crafts expert-level prompts using language models. It provides a principled framework for prompt optimization by unifying prompt sampling and rewarding using MCTS algorithm. The tool supports different models like openai, palm, and huggingface models. Users can run PromptAgent to optimize prompts for specific tasks by strategically sampling model errors, generating error feedbacks, simulating future rewards, and searching for high-reward paths leading to expert prompts.
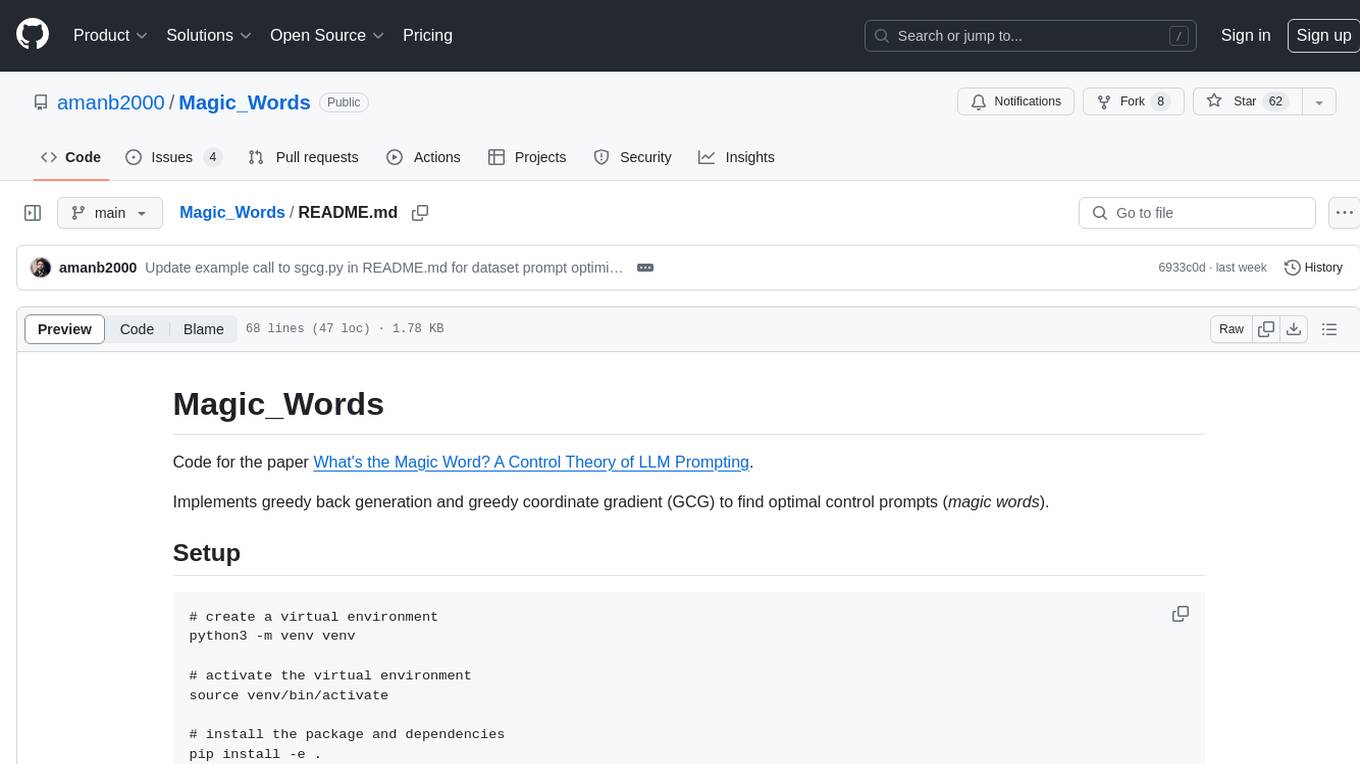
Magic_Words
Magic_Words is a repository containing code for the paper 'What's the Magic Word? A Control Theory of LLM Prompting'. It implements greedy back generation and greedy coordinate gradient (GCG) to find optimal control prompts (magic words). Users can set up a virtual environment, install the package and dependencies, and run example scripts for pointwise control and optimizing prompts for datasets. The repository provides scripts for finding optimal control prompts for question-answer pairs and dataset optimization using the GCG algorithm.
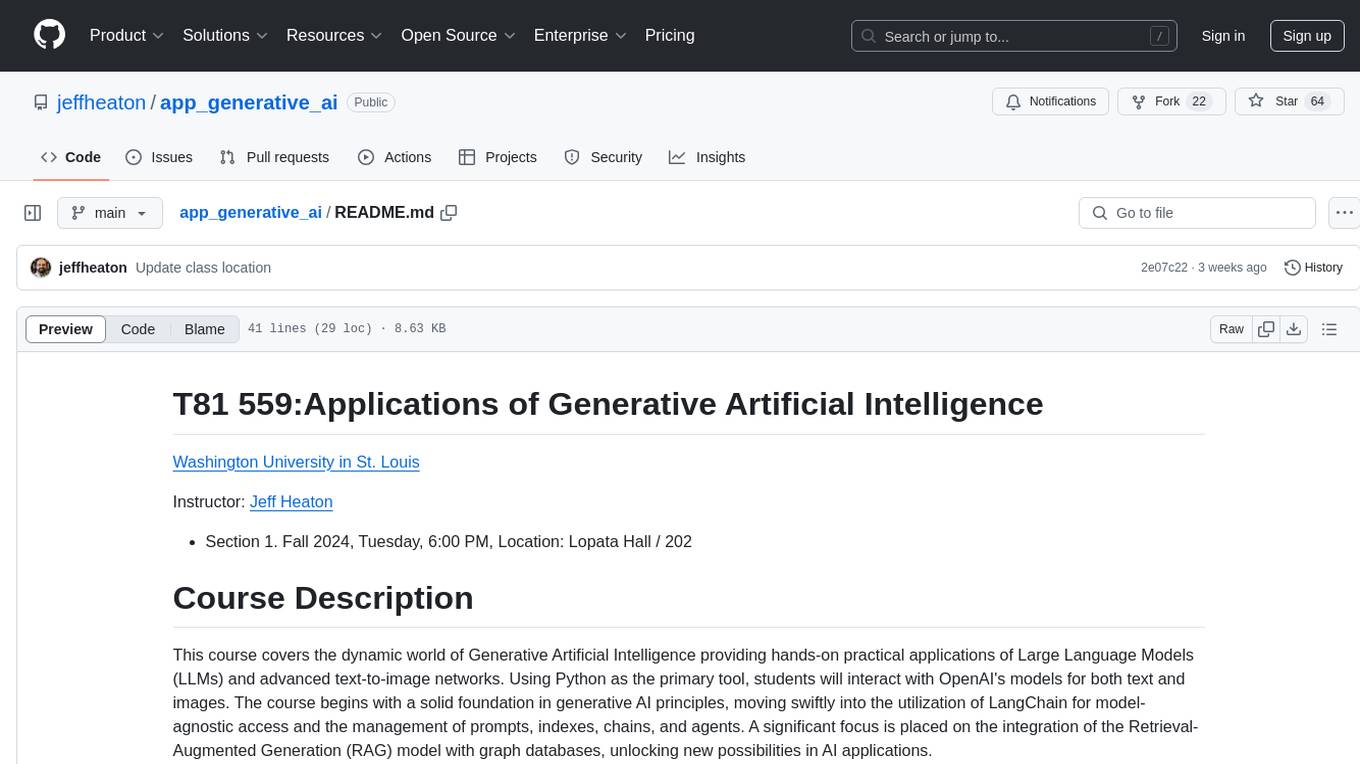
app_generative_ai
This repository contains course materials for T81 559: Applications of Generative Artificial Intelligence at Washington University in St. Louis. The course covers practical applications of Large Language Models (LLMs) and text-to-image networks using Python. Students learn about generative AI principles, LangChain, Retrieval-Augmented Generation (RAG) model, image generation techniques, fine-tuning neural networks, and prompt engineering. Ideal for students, researchers, and professionals in computer science, the course offers a transformative learning experience in the realm of Generative AI.
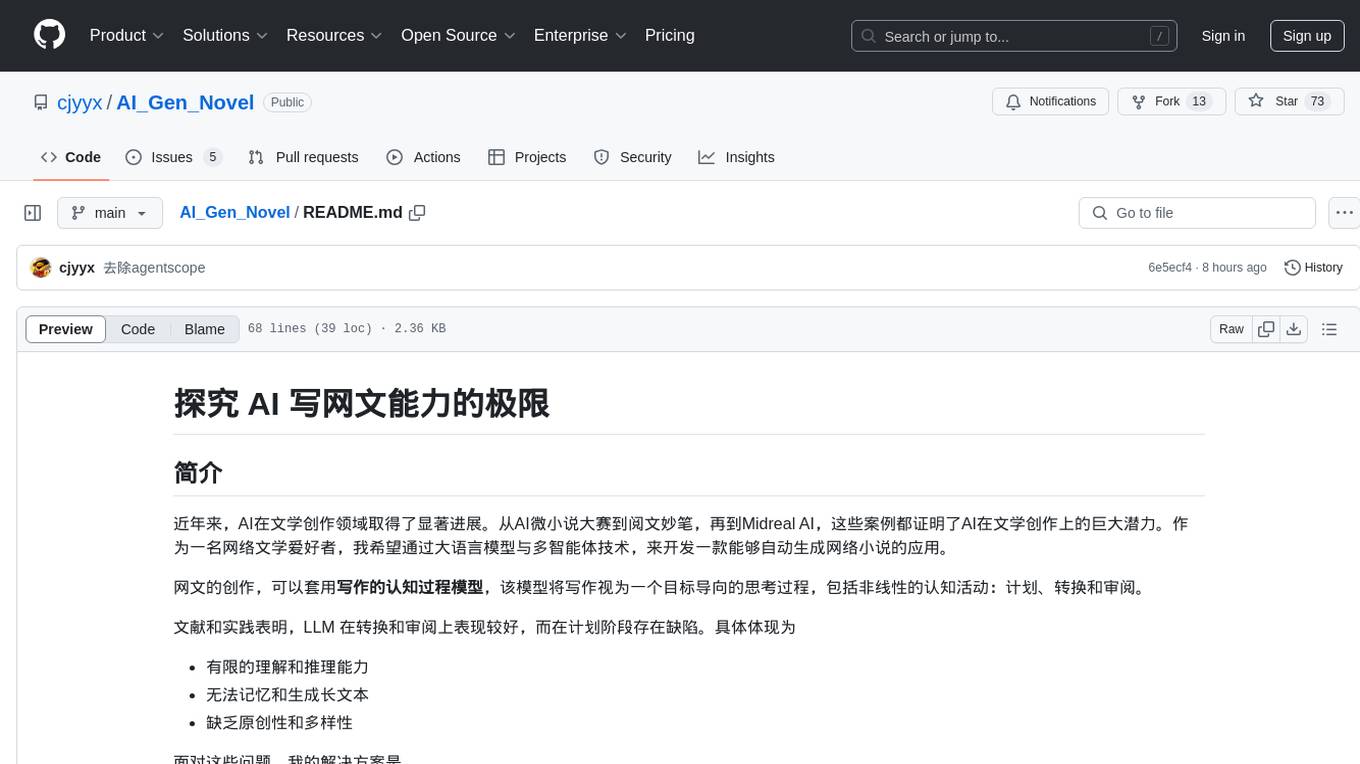
AI_Gen_Novel
AI_Gen_Novel is a project exploring the limits of AI in writing online fiction. Leveraging large language models and multi-agent technology, the tool aims to automatically generate web novels by compressing long texts, optimizing prompts, and enhancing originality. The tool combines the core idea of RecurrentGPT with language-based iterative computation to create texts of any length. Future directions include enhancing model capabilities, optimizing program architecture, and introducing more prior knowledge for structured storytelling.
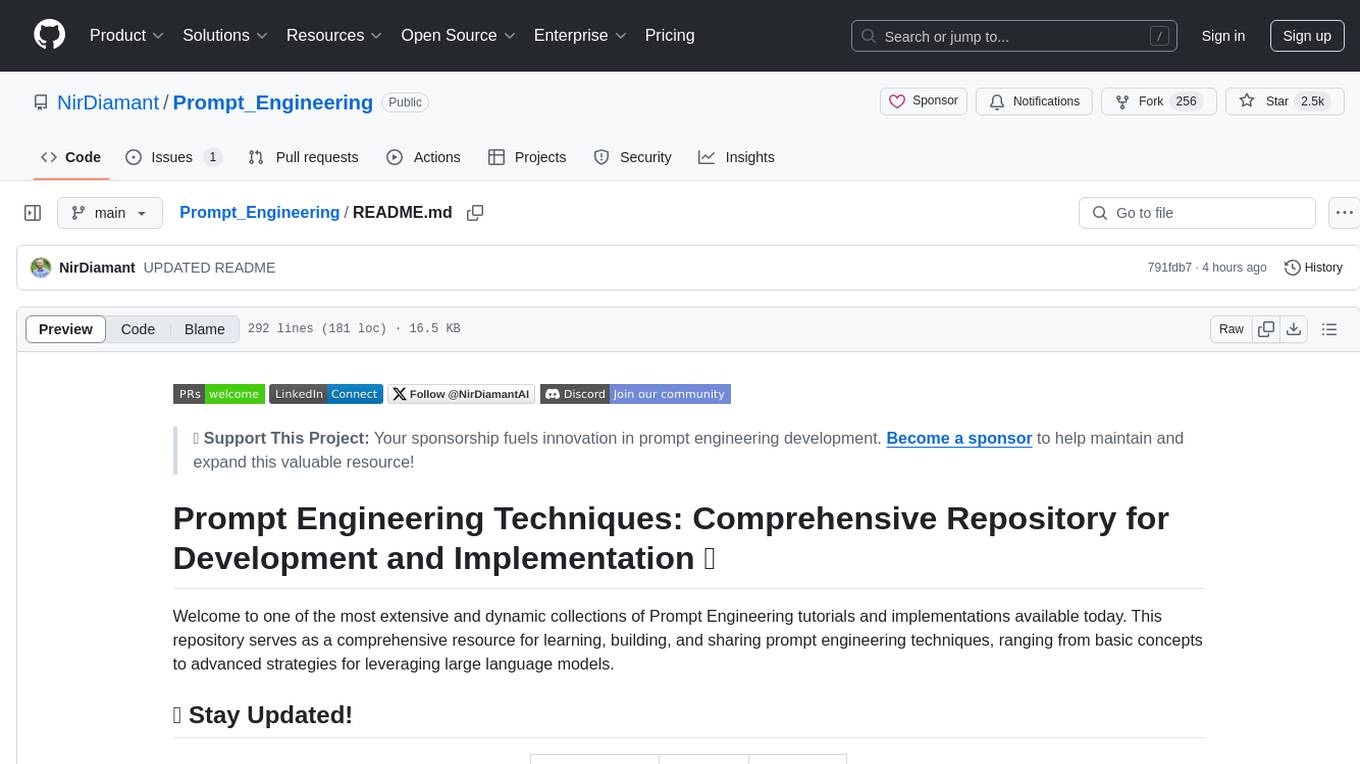
Prompt_Engineering
Prompt Engineering Techniques is a comprehensive repository for learning, building, and sharing prompt engineering techniques, from basic concepts to advanced strategies for leveraging large language models. It provides step-by-step tutorials, practical implementations, and a platform for showcasing innovative prompt engineering techniques. The repository covers fundamental concepts, core techniques, advanced strategies, optimization and refinement, specialized applications, and advanced applications in prompt engineering.
For similar jobs
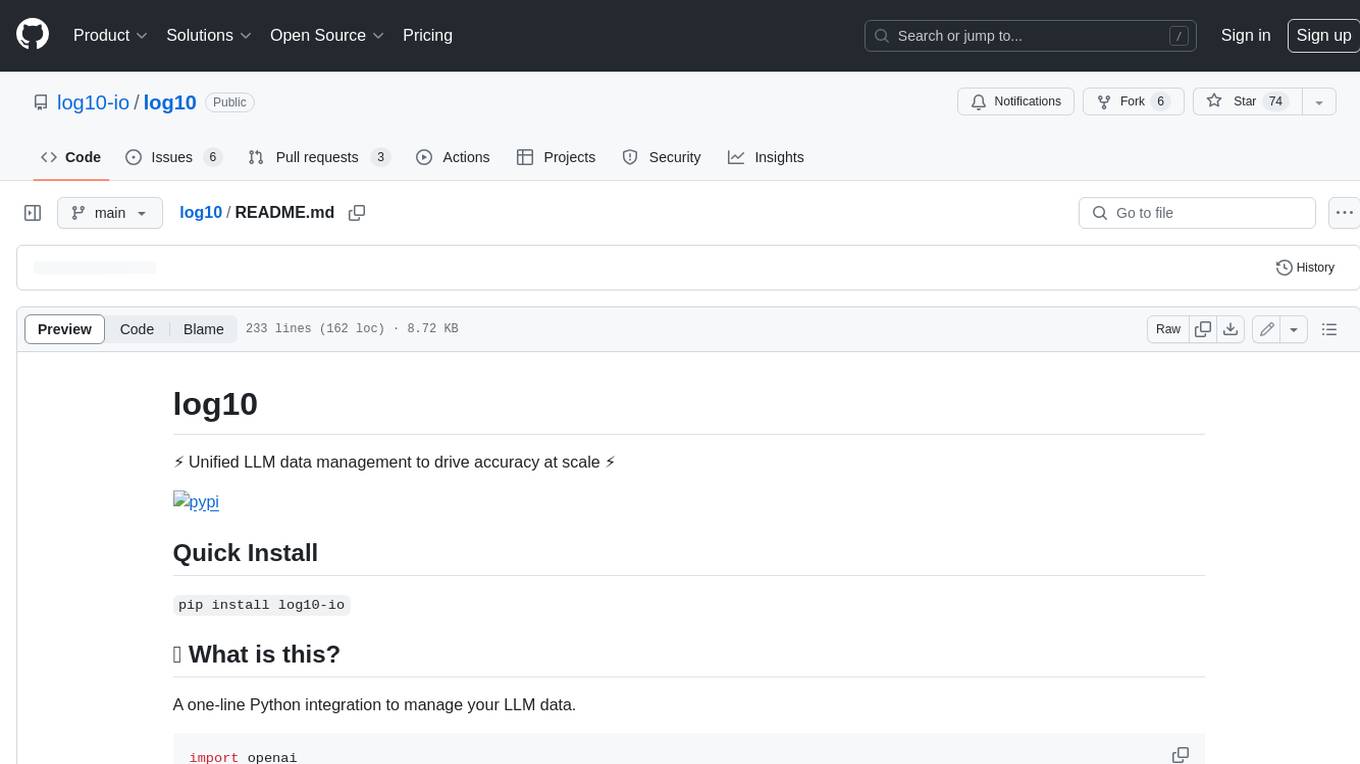
log10
Log10 is a one-line Python integration to manage your LLM data. It helps you log both closed and open-source LLM calls, compare and identify the best models and prompts, store feedback for fine-tuning, collect performance metrics such as latency and usage, and perform analytics and monitor compliance for LLM powered applications. Log10 offers various integration methods, including a python LLM library wrapper, the Log10 LLM abstraction, and callbacks, to facilitate its use in both existing production environments and new projects. Pick the one that works best for you. Log10 also provides a copilot that can help you with suggestions on how to optimize your prompt, and a feedback feature that allows you to add feedback to your completions. Additionally, Log10 provides prompt provenance, session tracking and call stack functionality to help debug prompt chains. With Log10, you can use your data and feedback from users to fine-tune custom models with RLHF, and build and deploy more reliable, accurate and efficient self-hosted models. Log10 also supports collaboration, allowing you to create flexible groups to share and collaborate over all of the above features.
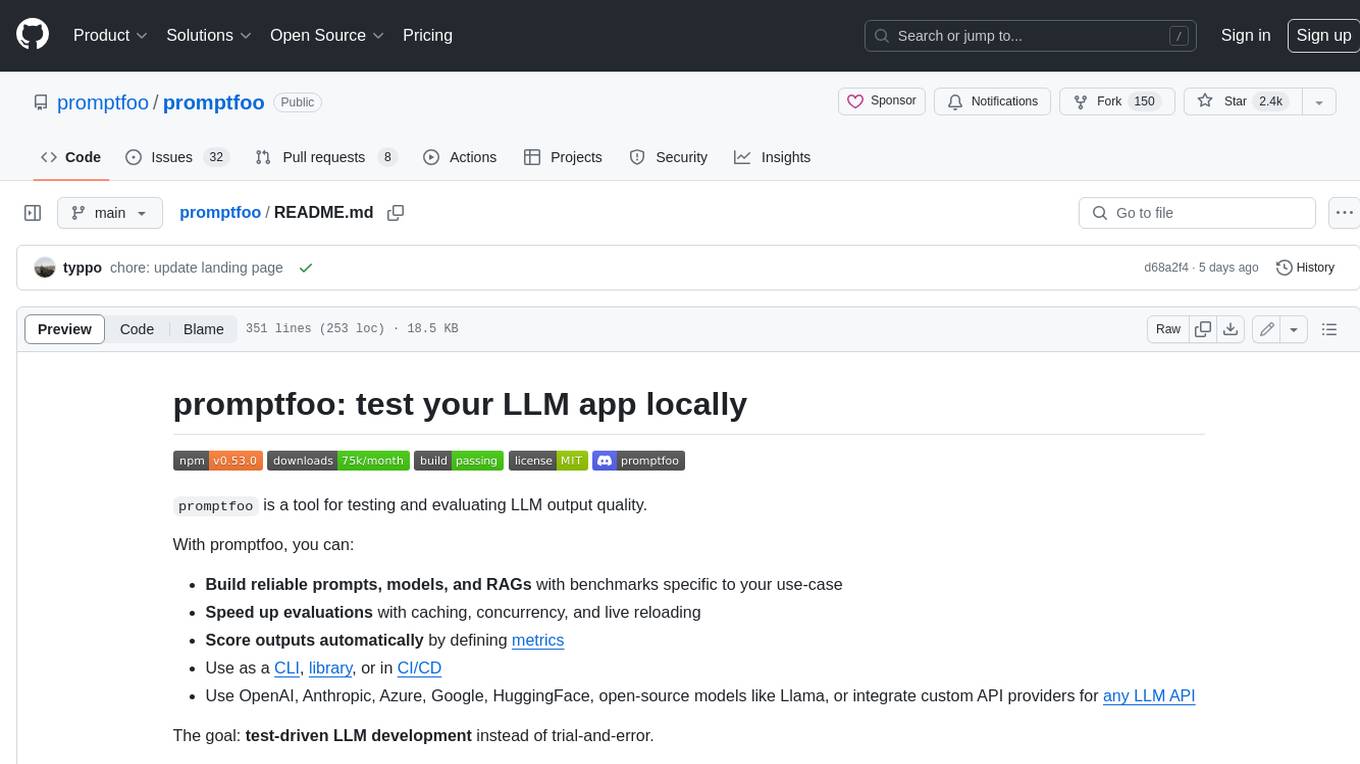
promptfoo
Promptfoo is a tool for testing and evaluating LLM output quality. With promptfoo, you can build reliable prompts, models, and RAGs with benchmarks specific to your use-case, speed up evaluations with caching, concurrency, and live reloading, score outputs automatically by defining metrics, use as a CLI, library, or in CI/CD, and use OpenAI, Anthropic, Azure, Google, HuggingFace, open-source models like Llama, or integrate custom API providers for any LLM API.
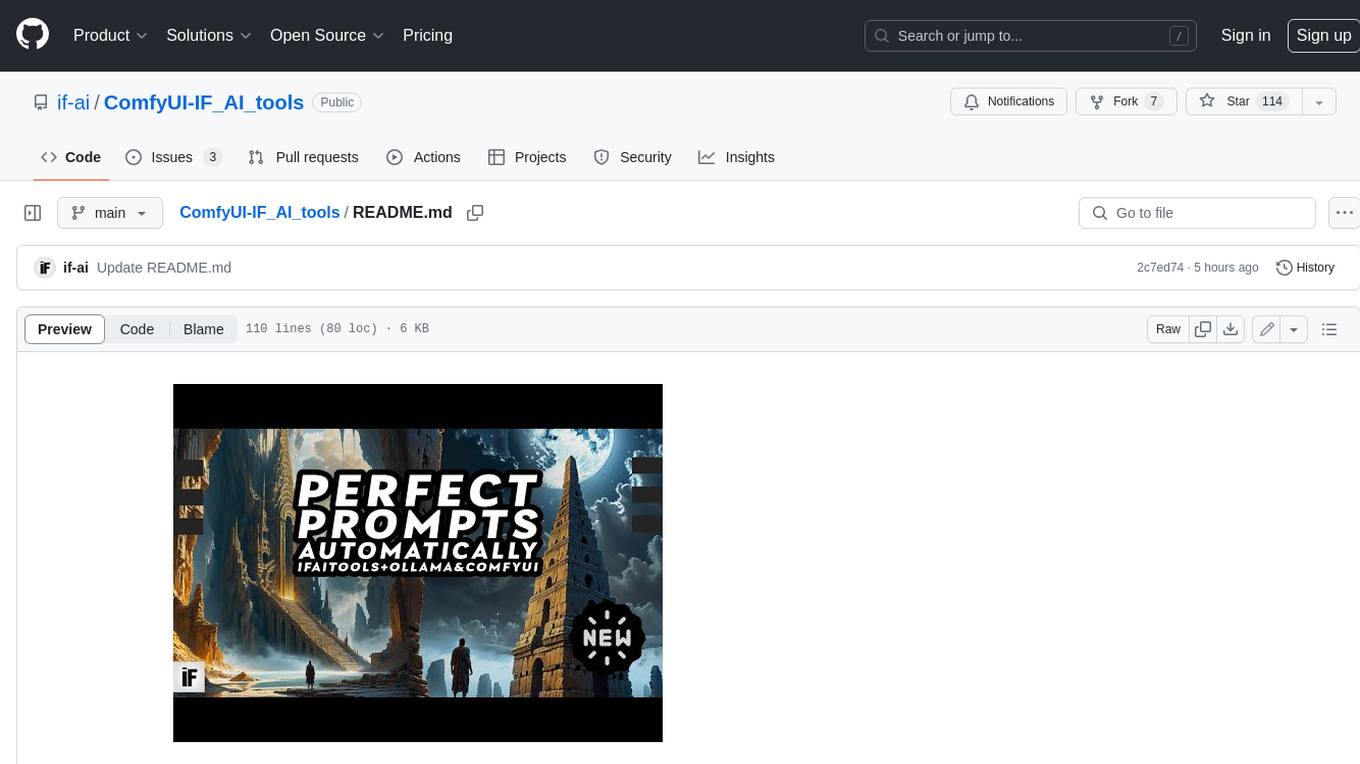
ComfyUI-IF_AI_tools
ComfyUI-IF_AI_tools is a set of custom nodes for ComfyUI that allows you to generate prompts using a local Large Language Model (LLM) via Ollama. This tool enables you to enhance your image generation workflow by leveraging the power of language models.
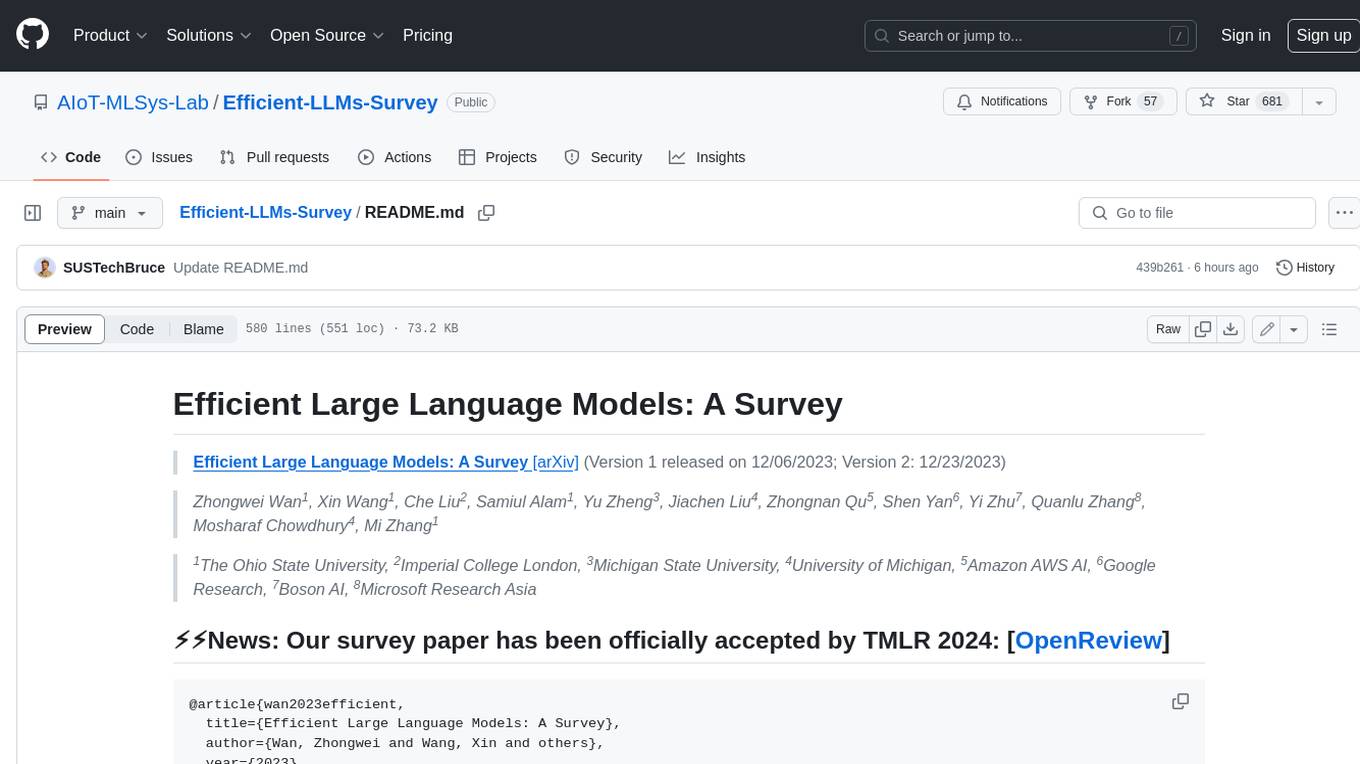
Efficient-LLMs-Survey
This repository provides a systematic and comprehensive review of efficient LLMs research. We organize the literature in a taxonomy consisting of three main categories, covering distinct yet interconnected efficient LLMs topics from **model-centric** , **data-centric** , and **framework-centric** perspective, respectively. We hope our survey and this GitHub repository can serve as valuable resources to help researchers and practitioners gain a systematic understanding of the research developments in efficient LLMs and inspire them to contribute to this important and exciting field.
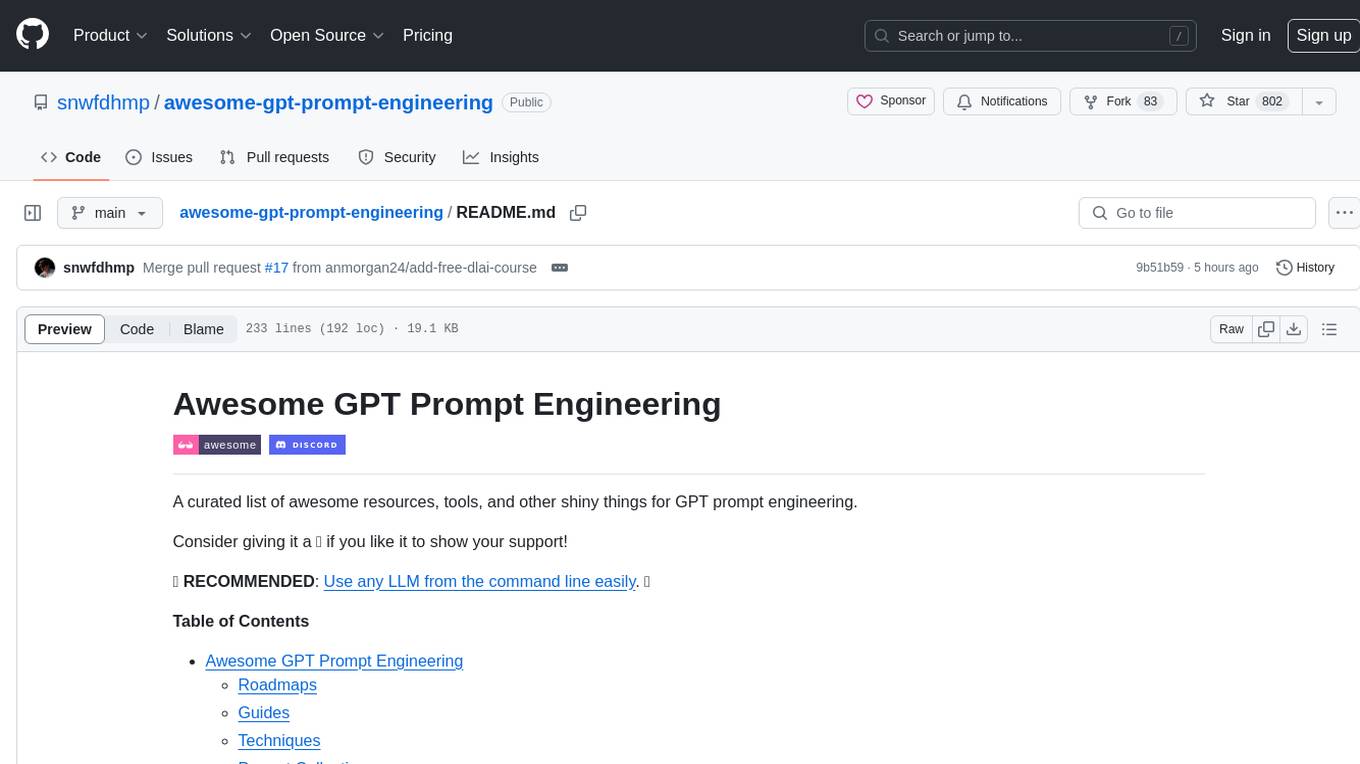
awesome-gpt-prompt-engineering
Awesome GPT Prompt Engineering is a curated list of resources, tools, and shiny things for GPT prompt engineering. It includes roadmaps, guides, techniques, prompt collections, papers, books, communities, prompt generators, Auto-GPT related tools, prompt injection information, ChatGPT plug-ins, prompt engineering job offers, and AI links directories. The repository aims to provide a comprehensive guide for prompt engineering enthusiasts, covering various aspects of working with GPT models and improving communication with AI tools.