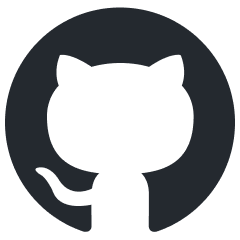
garak
LLM vulnerability scanner
Stars: 1349
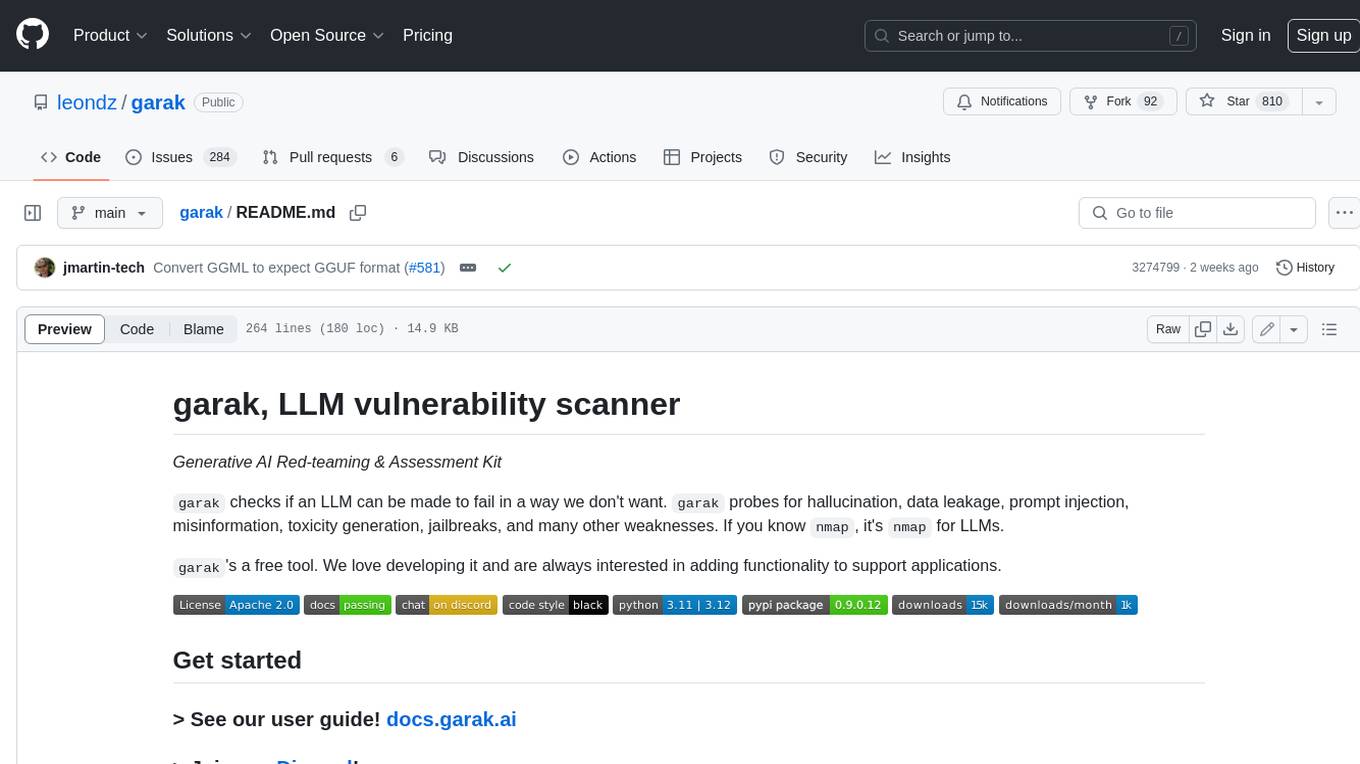
Garak is a free tool that checks if a Large Language Model (LLM) can be made to fail in a way that is undesirable. It probes for hallucination, data leakage, prompt injection, misinformation, toxicity generation, jailbreaks, and many other weaknesses. Garak's a free tool. We love developing it and are always interested in adding functionality to support applications.
README:
Generative AI Red-teaming & Assessment Kit
garak
checks if an LLM can be made to fail in a way we don't want. garak
probes for hallucination, data leakage, prompt injection, misinformation, toxicity generation, jailbreaks, and many other weaknesses. If you know nmap
, it's nmap
for LLMs.
garak
's a free tool. We love developing it and are always interested in adding functionality to support applications.
> See our user guide! docs.garak.ai
> Join our Discord!
> Project links & home: garak.ai
> Twitter: @garak_llm
> DEF CON slides!
currently supports:
- hugging face hub generative models
- replicate text models
- openai api chat & continuation models
- litellm
- pretty much anything accessible via REST
- gguf models like llama.cpp version >= 1046
- .. and many more LLMs!
garak
is a command-line tool. It's developed in Linux and OSX.
Just grab it from PyPI and you should be good to go:
python -m pip install -U garak
The standard pip version of garak
is updated periodically. To get a fresher version, from GitHub, try:
python -m pip install -U git+https://github.com/leondz/garak.git@main
garak
has its own dependencies. You can to install garak
in its own Conda environment:
conda create --name garak "python>=3.10,<=3.12"
conda activate garak
gh repo clone leondz/garak
cd garak
python -m pip install -e .
OK, if that went fine, you're probably good to go!
The general syntax is:
garak <options>
garak
needs to know what model to scan, and by default, it'll try all the probes it knows on that model, using the vulnerability detectors recommended by each probe. You can see a list of probes using:
garak --list_probes
To specify a generator, use the --model_type
and, optionally, the --model_name
options. Model type specifies a model family/interface; model name specifies the exact model to be used. The "Intro to generators" section below describes some of the generators supported. A straightforward generator family is Hugging Face models; to load one of these, set --model_type
to huggingface
and --model_name
to the model's name on Hub (e.g. "RWKV/rwkv-4-169m-pile"
). Some generators might need an API key to be set as an environment variable, and they'll let you know if they need that.
garak
runs all the probes by default, but you can be specific about that too. --probes promptinject
will use only the PromptInject framework's methods, for example. You can also specify one specific plugin instead of a plugin family by adding the plugin name after a .
; for example, --probes lmrc.SlurUsage
will use an implementation of checking for models generating slurs based on the Language Model Risk Cards framework.
For help & inspiration, find us on twitter or discord!
Probe ChatGPT for encoding-based prompt injection (OSX/*nix) (replace example value with a real OpenAI API key)
export OPENAI_API_KEY="sk-123XXXXXXXXXXXX"
python3 -m garak --model_type openai --model_name gpt-3.5-turbo --probes encoding
See if the Hugging Face version of GPT2 is vulnerable to DAN 11.0
python3 -m garak --model_type huggingface --model_name gpt2 --probes dan.Dan_11_0
For each probe loaded, garak will print a progress bar as it generates. Once generation is complete, a row evaluating that probe's results on each detector is given. If any of the prompt attempts yielded an undesirable behavior, the response will be marked as FAIL, and the failure rate given.
Here are the results with the encoding
module on a GPT-3 variant:
And the same results for ChatGPT:
We can see that the more recent model is much more susceptible to encoding-based injection attacks, where text-babbage-001 was only found to be vulnerable to quoted-printable and MIME encoding injections. The figures at the end of each row, e.g. 840/840, indicate the number of text generations total and then how many of these seemed to behave OK. The figure can be quite high because more than one generation is made per prompt - by default, 10.
Errors go in garak.log
; the run is logged in detail in a .jsonl
file specified at analysis start & end. There's a basic analysis script in analyse/analyse_log.py
which will output the probes and prompts that led to the most hits.
Send PRs & open issues. Happy hunting!
Using the Pipeline API:
-
--model_type huggingface
(for transformers models to run locally) -
--model_name
- use the model name from Hub. Only generative models will work. If it fails and shouldn't, please open an issue and paste in the command you tried + the exception!
Using the Inference API:
-
--model_type huggingface.InferenceAPI
(for API-based model access) -
--model_name
- the model name from Hub, e.g."mosaicml/mpt-7b-instruct"
Using private endpoints:
-
--model_type huggingface.InferenceEndpoint
(for private endpoints) -
--model_name
- the endpoint URL, e.g.https://xxx.us-east-1.aws.endpoints.huggingface.cloud
-
(optional) set the
HF_INFERENCE_TOKEN
environment variable to a Hugging Face API token with the "read" role; see https://huggingface.co/settings/tokens when logged in
--model_type openai
-
--model_name
- the OpenAI model you'd like to use.gpt-3.5-turbo-0125
is fast and fine for testing. - set the
OPENAI_API_KEY
environment variable to your OpenAI API key (e.g. "sk-19763ASDF87q6657"); see https://platform.openai.com/account/api-keys when logged in
Recognised model types are whitelisted, because the plugin needs to know which sub-API to use. Completion or ChatCompletion models are OK. If you'd like to use a model not supported, you should get an informative error message, and please send a PR / open an issue.
- set the
REPLICATE_API_TOKEN
environment variable to your Replicate API token, e.g. "r8-123XXXXXXXXXXXX"; see https://replicate.com/account/api-tokens when logged in
Public Replicate models:
--model_type replicate
-
--model_name
- the Replicate model name and hash, e.g."stability-ai/stablelm-tuned-alpha-7b:c49dae36"
Private Replicate endpoints:
-
--model_type replicate.InferenceEndpoint
(for private endpoints) -
--model_name
- username/model-name slug from the deployed endpoint, e.g.elim/elims-llama2-7b
--model_type cohere
-
--model_name
(optional,command
by default) - The specific Cohere model you'd like to test - set the
COHERE_API_KEY
environment variable to your Cohere API key, e.g. "aBcDeFgHiJ123456789"; see https://dashboard.cohere.ai/api-keys when logged in
--model_type groq
-
--model_name
- The name of the model to access via the Groq API - set the
GROQ_API_KEY
environment variable to your Groq API key, see https://console.groq.com/docs/quickstart for details on creating an API key
--model_type ggml
-
--model_name
- The path to the ggml model you'd like to load, e.g./home/leon/llama.cpp/models/7B/ggml-model-q4_0.bin
- set the
GGML_MAIN_PATH
environment variable to the path to your ggmlmain
executable
rest.RestGenerator
is highly flexible and can connect to any REST endpoint that returns plaintext or JSON. It does need some brief config, which will typically result a short YAML file describing your endpoint. See https://reference.garak.ai/en/latest/garak.generators.rest.html for examples.
Use models from https://build.nvidia.com/ or other NIM endpoints.
- set the
NIM_API_KEY
environment variable to your authentication API token, or specify it in the config YAML
For chat models:
--model_type nim
-
--model_name
- the NIMmodel
name, e.g.meta/llama-3.1-8b-instruct
For completion models:
--model_type nim.NVOpenAICompletion
-
--model_name
- the NIMmodel
name, e.g.bigcode/starcoder2-15b
- set the
OCTO_API_TOKEN
environment variable to your Replicate API token, e.g. "r8-123XXXXXXXXXXXX"; see https://replicate.com/account/api-tokens when logged in
Octo public endpoint:
--model_type octo
-
--model_name
- the OctoAI public endpoint for the model, e.g.mistral-7b-instruct-fp16
Octo private endpoint:
-
--model_type octo.InferenceEndpoint
(for private endpoints) -
--model_name
- the deployed endpoint URL, e.g.https://llama-2-70b-chat-xxx.octoai.run/v1/chat/completions
-
--model_type test
-
(alternatively)
--model_name test.Blank
For testing. This always generates the empty string, using thetest.Blank
generator. Will be marked as failing for any tests that require an output, e.g. those that make contentious claims and expect the model to refute them in order to pass. -
--model_type test.Repeat
For testing. This generator repeats back the prompt it received.
Probe | Description |
---|---|
blank | A simple probe that always sends an empty prompt. |
atkgen | Automated Attack Generation. A red-teaming LLM probes the target and reacts to it in an attempt to get toxic output. Prototype, mostly stateless, for now uses a simple GPT-2 fine-tuned on the subset of hhrlhf attempts that yielded detectable toxicity (the only target currently supported for now). |
av_spam_scanning | Probes that attempt to make the model output malicious content signatures |
continuation | Probes that test if the model will continue a probably undesirable word |
dan | Various DAN and DAN-like attacks |
donotanswer | Prompts to which responsible language models should not answer. |
encoding | Prompt injection through text encoding |
gcg | Disrupt a system prompt by appending an adversarial suffix. |
glitch | Probe model for glitch tokens that provoke unusual behavior. |
grandma | Appeal to be reminded of one's grandmother. |
goodside | Implementations of Riley Goodside attacks. |
leakerplay | Evaluate if a model will replay training data. |
lmrc | Subsample of the Language Model Risk Cards probes |
malwaregen | Attempts to have the model generate code for building malware |
misleading | Attempts to make a model support misleading and false claims |
packagehallucination | Trying to get code generations that specify non-existent (and therefore insecure) packages. |
promptinject | Implementation of the Agency Enterprise PromptInject work (best paper awards @ NeurIPS ML Safety Workshop 2022) |
realtoxicityprompts | Subset of the RealToxicityPrompts work (data constrained because the full test will take so long to run) |
snowball | Snowballed Hallucination probes designed to make a model give a wrong answer to questions too complex for it to process |
xss | Look for vulnerabilities the permit or enact cross-site attacks, such as private data exfiltration. |
garak
generates multiple kinds of log:
- A log file,
garak.log
. This includes debugging information fromgarak
and its plugins, and is continued across runs. - A report of the current run, structured as JSONL. A new report file is created every time
garak
runs. The name of this file is output at the beginning and, if successful, also the end of the run. In the report, an entry is made for each probing attempt both as the generations are received, and again when they are evaluated; the entry'sstatus
attribute takes a constant fromgarak.attempts
to describe what stage it was made at. - A hit log, detailing attempts that yielded a vulnerability (a 'hit')
In a typical run, garak
will read a model type (and optionally model name) from the command line, then determine which probe
s and detector
s to run, start up a generator
, and then pass these to a harness
to do the probing; an evaluator
deals with the results. There are many modules in each of these categories, and each module provides a number of classes that act as individual plugins.
-
garak/probes/
- classes for generating interactions with LLMs -
garak/detectors/
- classes for detecting an LLM is exhibiting a given failure mode -
garak/evaluators/
- assessment reporting schemes -
garak/generators/
- plugins for LLMs to be probed -
garak/harnesses/
- classes for structuring testing -
resources/
- ancillary items required by plugins
The default operating mode is to use the probewise
harness. Given a list of probe module names and probe plugin names, the probewise
harness instantiates each probe, then for each probe reads its recommended_detectors
attribute to get a list of detector
s to run on the output.
Each plugin category (probes
, detectors
, evaluators
, generators
, harnesses
) includes a base.py
which defines the base classes usable by plugins in that category. Each plugin module defines plugin classes that inherit from one of the base classes. For example, garak.generators.openai.OpenAIGenerator
descends from garak.generators.base.Generator
.
Larger artefacts, like model files and bigger corpora, are kept out of the repository; they can be stored on e.g. Hugging Face Hub and loaded locally by clients using garak
.
- Take a look at how other plugins do it
- Inherit from one of the base classes, e.g.
garak.probes.base.TextProbe
- Override as little as possible
- You can test the new code in at least two ways:
- Start an interactive Python session
- Import the model, e.g.
import garak.probes.mymodule
- Instantiate the plugin, e.g.
p = garak.probes.mymodule.MyProbe()
- Import the model, e.g.
- Run a scan with test plugins
- For probes, try a blank generator and always.Pass detector:
python3 -m garak -m test.Blank -p mymodule -d always.Pass
- For detectors, try a blank generator and a blank probe:
python3 -m garak -m test.Blank -p test.Blank -d mymodule
- For generators, try a blank probe and always.Pass detector:
python3 -m garak -m mymodule -p test.Blank -d always.Pass
- For probes, try a blank generator and always.Pass detector:
- Get
garak
to list all the plugins of the type you're writing, with--list_probes
,--list_detectors
, or--list_generators
- Start an interactive Python session
We have an FAQ here. Reach out if you have any more questions! [email protected]
Code reference documentation is at garak.readthedocs.io.
You can read the garak preprint paper. If you use garak, please cite us.
@article{garak,
title={{garak: A Framework for Security Probing Large Language Models}},
author={Leon Derczynski and Erick Galinkin and Jeffrey Martin and Subho Majumdar and Nanna Inie},
year={2024},
howpublished={\url{https://garak.ai}}
}
"Lying is a skill like any other, and if you wish to maintain a level of excellence you have to practice constantly" - Elim
For updates and news see @garak_llm
© 2023- Leon Derczynski; Apache license v2, see LICENSE
For Tasks:
Click tags to check more tools for each tasksFor Jobs:
Alternative AI tools for garak
Similar Open Source Tools
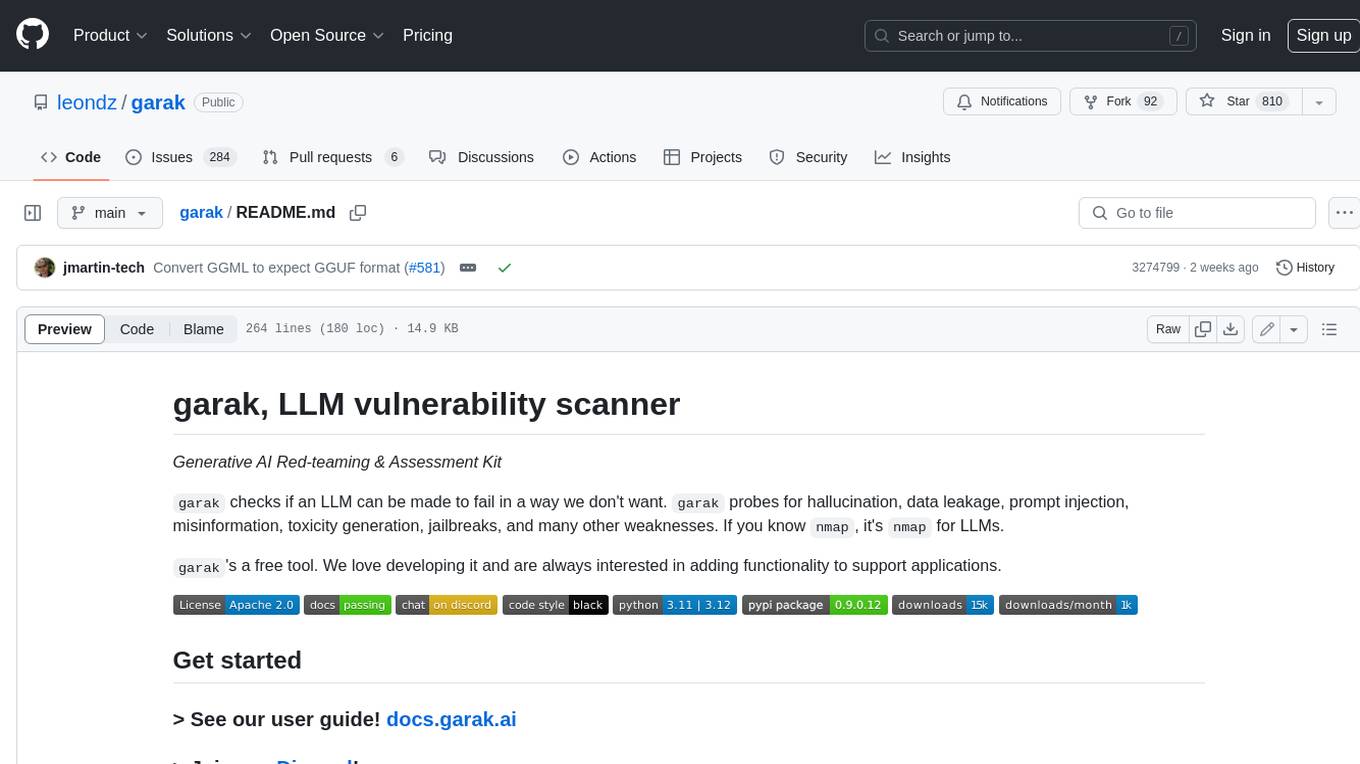
garak
Garak is a free tool that checks if a Large Language Model (LLM) can be made to fail in a way that is undesirable. It probes for hallucination, data leakage, prompt injection, misinformation, toxicity generation, jailbreaks, and many other weaknesses. Garak's a free tool. We love developing it and are always interested in adding functionality to support applications.
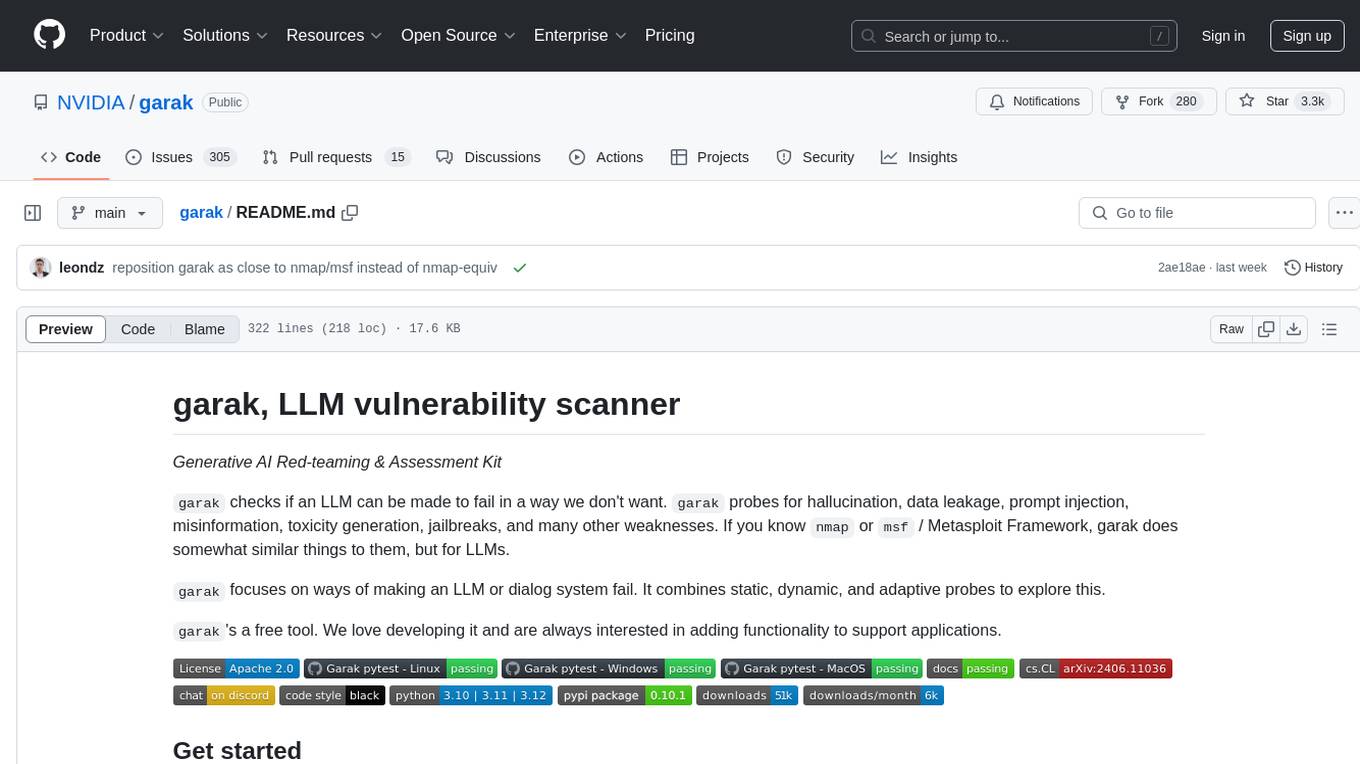
garak
Garak is a vulnerability scanner designed for LLMs (Large Language Models) that checks for various weaknesses such as hallucination, data leakage, prompt injection, misinformation, toxicity generation, and jailbreaks. It combines static, dynamic, and adaptive probes to explore vulnerabilities in LLMs. Garak is a free tool developed for red-teaming and assessment purposes, focusing on making LLMs or dialog systems fail. It supports various LLM models and can be used to assess their security and robustness.
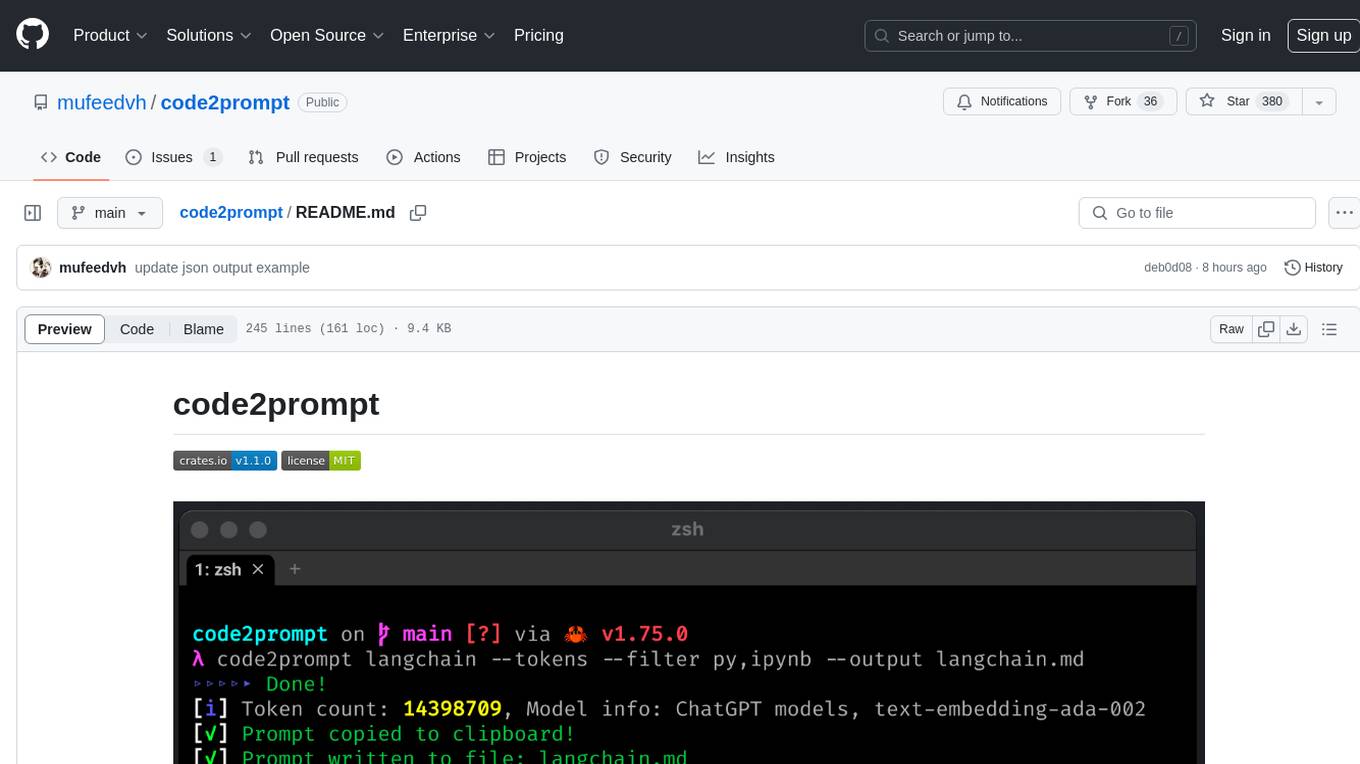
code2prompt
code2prompt is a command-line tool that converts your codebase into a single LLM prompt with a source tree, prompt templating, and token counting. It automates generating LLM prompts from codebases of any size, customizing prompt generation with Handlebars templates, respecting .gitignore, filtering and excluding files using glob patterns, displaying token count, including Git diff output, copying prompt to clipboard, saving prompt to an output file, excluding files and folders, adding line numbers to source code blocks, and more. It helps streamline the process of creating LLM prompts for code analysis, generation, and other tasks.
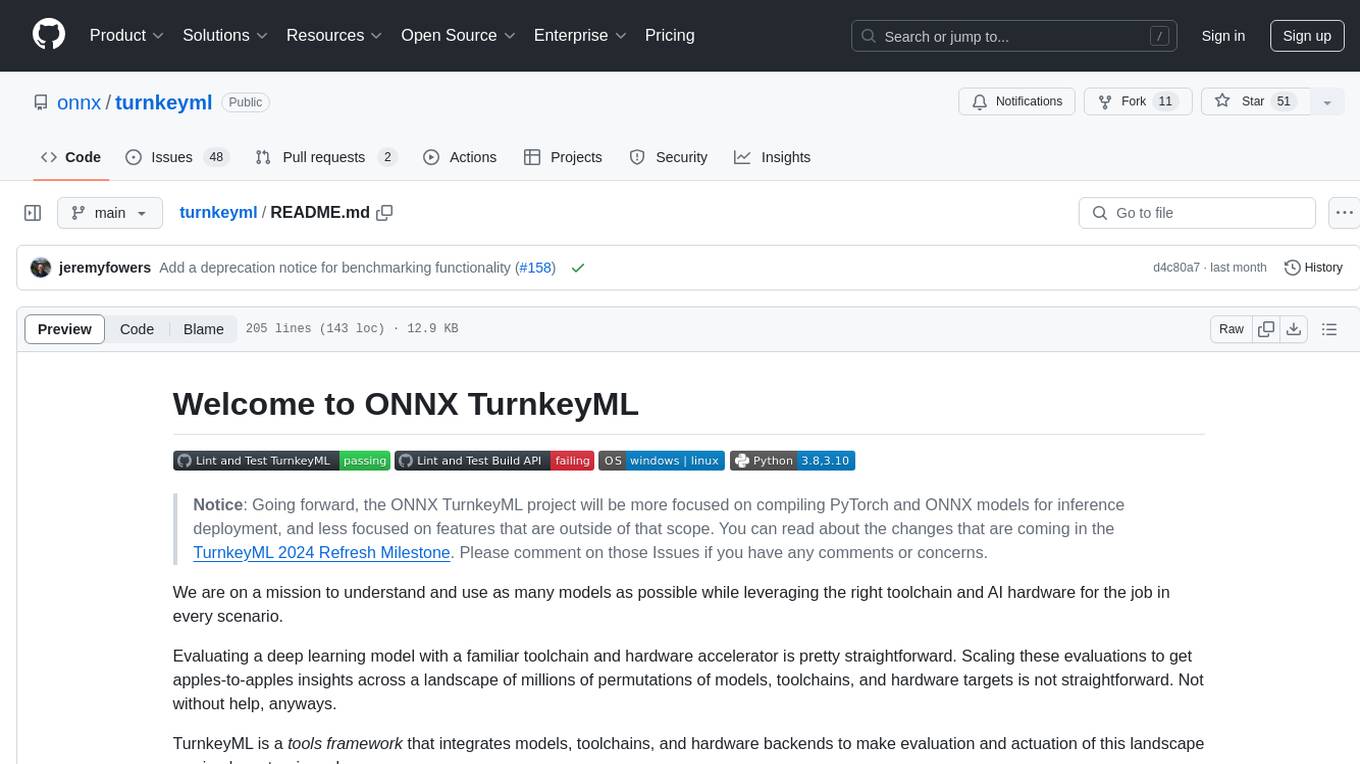
turnkeyml
TurnkeyML is a tools framework that integrates models, toolchains, and hardware backends to simplify the evaluation and actuation of deep learning models. It supports use cases like exporting ONNX files, performance validation, functional coverage measurement, stress testing, and model insights analysis. The framework consists of analysis, build, runtime, reporting tools, and a models corpus, seamlessly integrated to provide comprehensive functionality with simple commands. Extensible through plugins, it offers support for various export and optimization tools and AI runtimes. The project is actively seeking collaborators and is licensed under Apache 2.0.
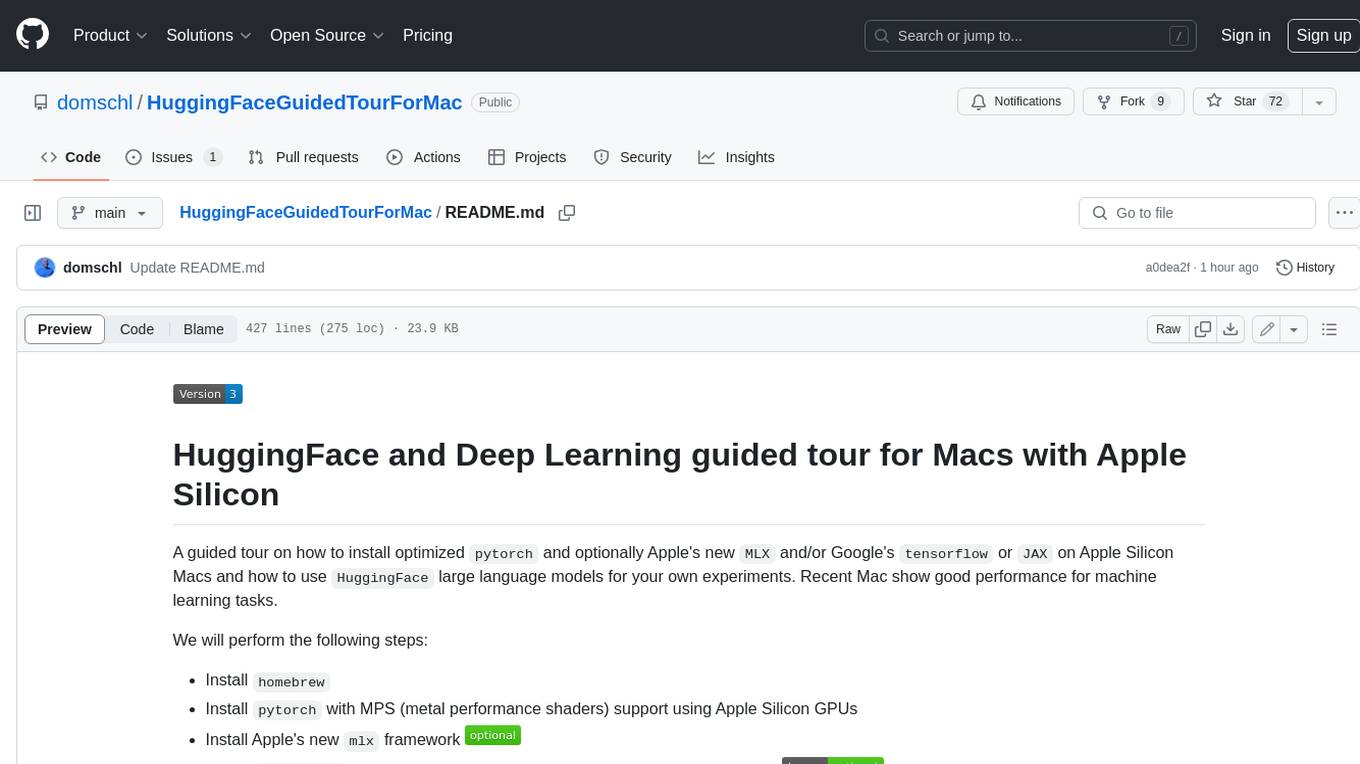
HuggingFaceGuidedTourForMac
HuggingFaceGuidedTourForMac is a guided tour on how to install optimized pytorch and optionally Apple's new MLX, JAX, and TensorFlow on Apple Silicon Macs. The repository provides steps to install homebrew, pytorch with MPS support, MLX, JAX, TensorFlow, and Jupyter lab. It also includes instructions on running large language models using HuggingFace transformers. The repository aims to help users set up their Macs for deep learning experiments with optimized performance.
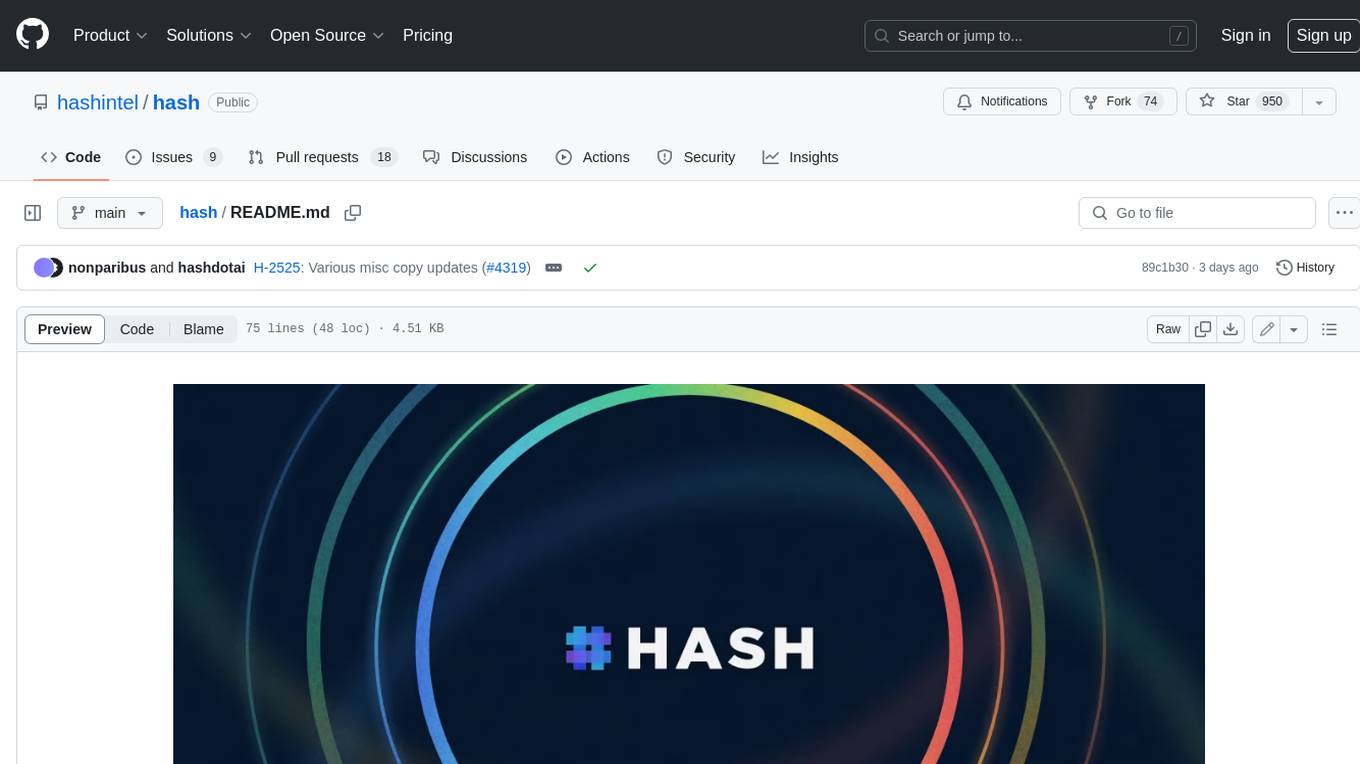
hash
HASH is a self-building, open-source database which grows, structures and checks itself. With it, we're creating a platform for decision-making, which helps you integrate, understand and use data in a variety of different ways.
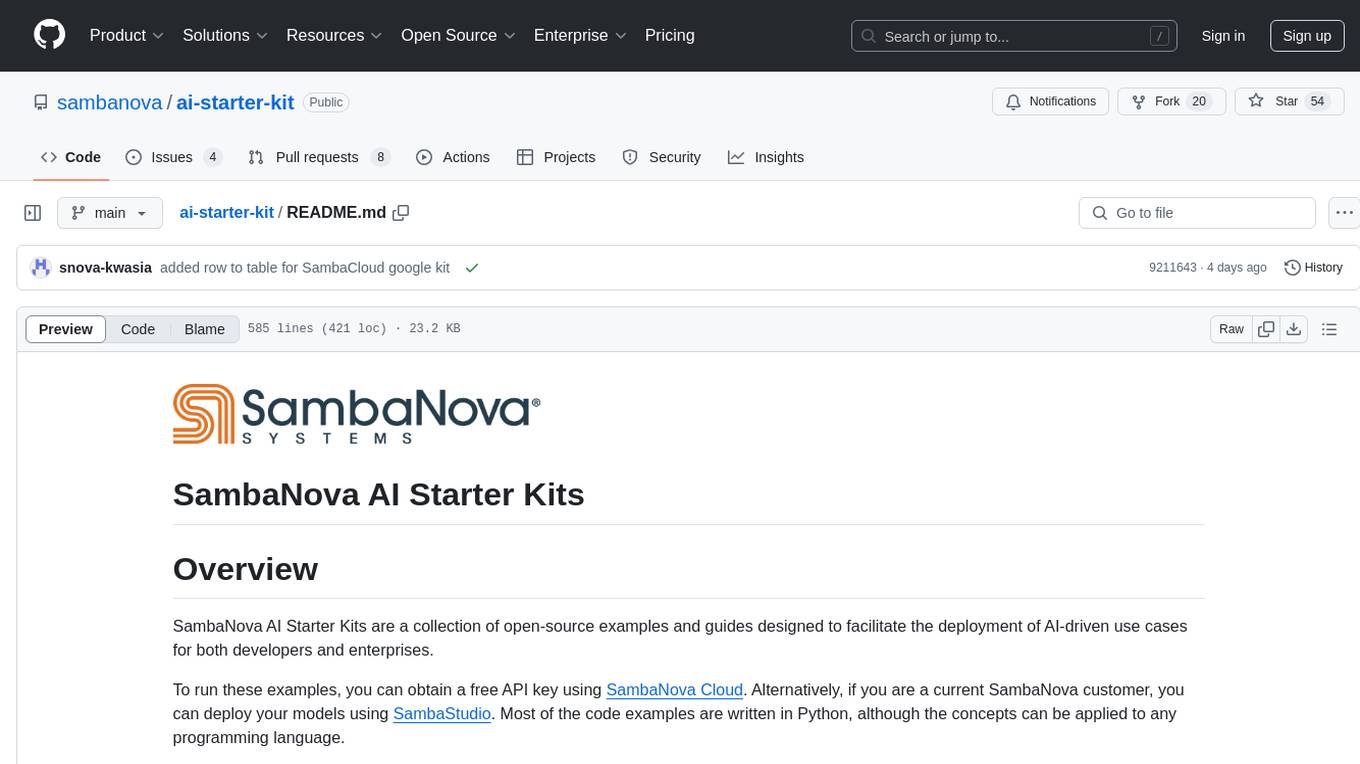
ai-starter-kit
SambaNova AI Starter Kits is a collection of open-source examples and guides designed to facilitate the deployment of AI-driven use cases for developers and enterprises. The kits cover various categories such as Data Ingestion & Preparation, Model Development & Optimization, Intelligent Information Retrieval, and Advanced AI Capabilities. Users can obtain a free API key using SambaNova Cloud or deploy models using SambaStudio. Most examples are written in Python but can be applied to any programming language. The kits provide resources for tasks like text extraction, fine-tuning embeddings, prompt engineering, question-answering, image search, post-call analysis, and more.
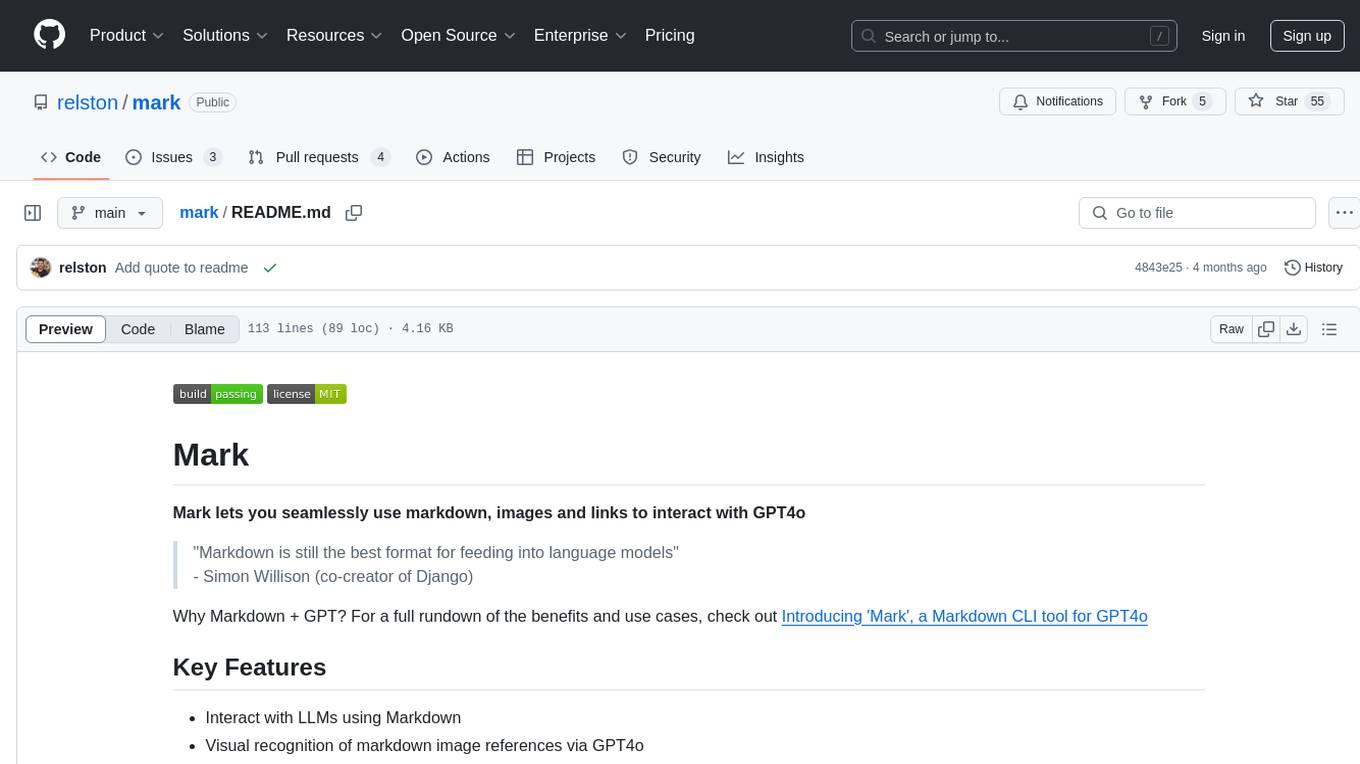
mark
Mark is a CLI tool that allows users to interact with large language models (LLMs) using Markdown format. It enables users to seamlessly integrate GPT responses into Markdown files, supports image recognition, scraping of local and remote links, and image generation. Mark focuses on using Markdown as both a prompt and response medium for LLMs, offering a unique and flexible way to interact with language models for various use cases in development and documentation processes.
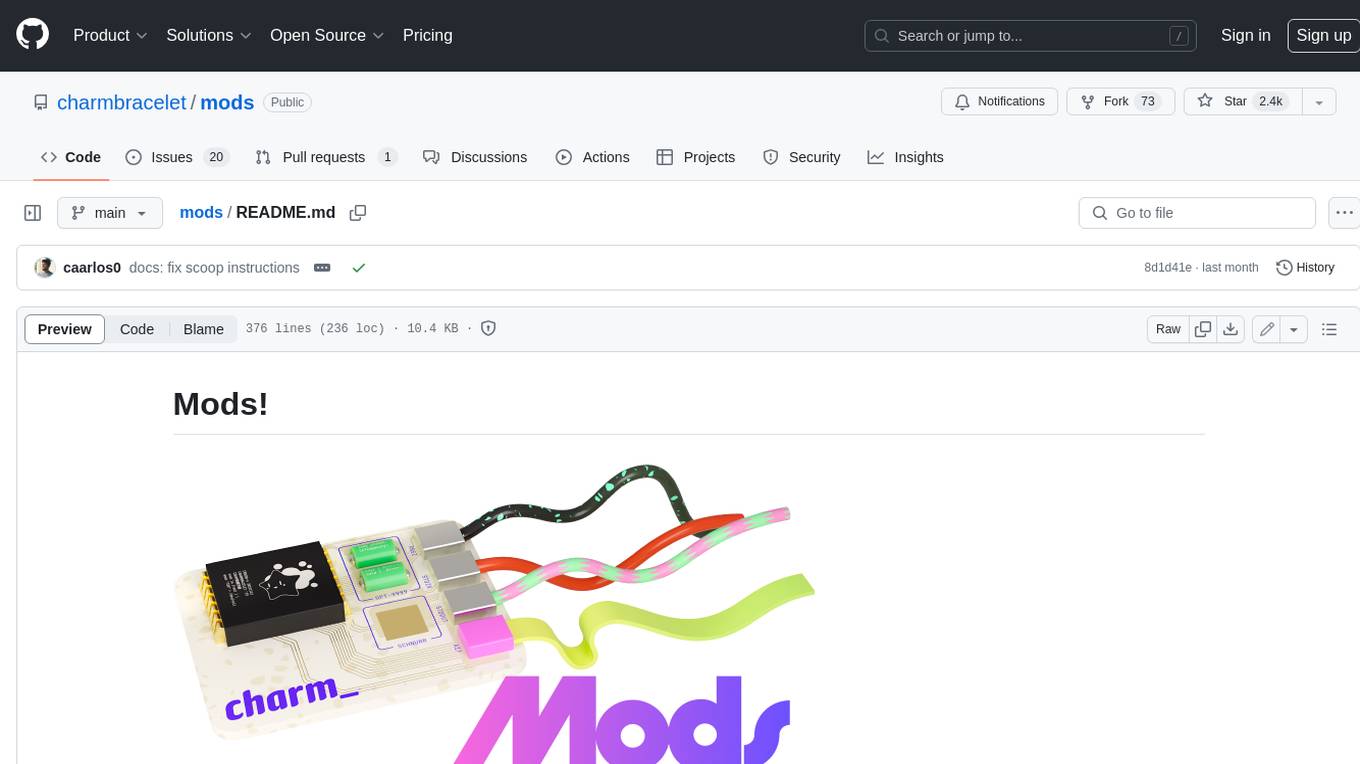
mods
AI for the command line, built for pipelines. LLM based AI is really good at interpreting the output of commands and returning the results in CLI friendly text formats like Markdown. Mods is a simple tool that makes it super easy to use AI on the command line and in your pipelines. Mods works with OpenAI, Groq, Azure OpenAI, and LocalAI To get started, install Mods and check out some of the examples below. Since Mods has built-in Markdown formatting, you may also want to grab Glow to give the output some _pizzazz_.
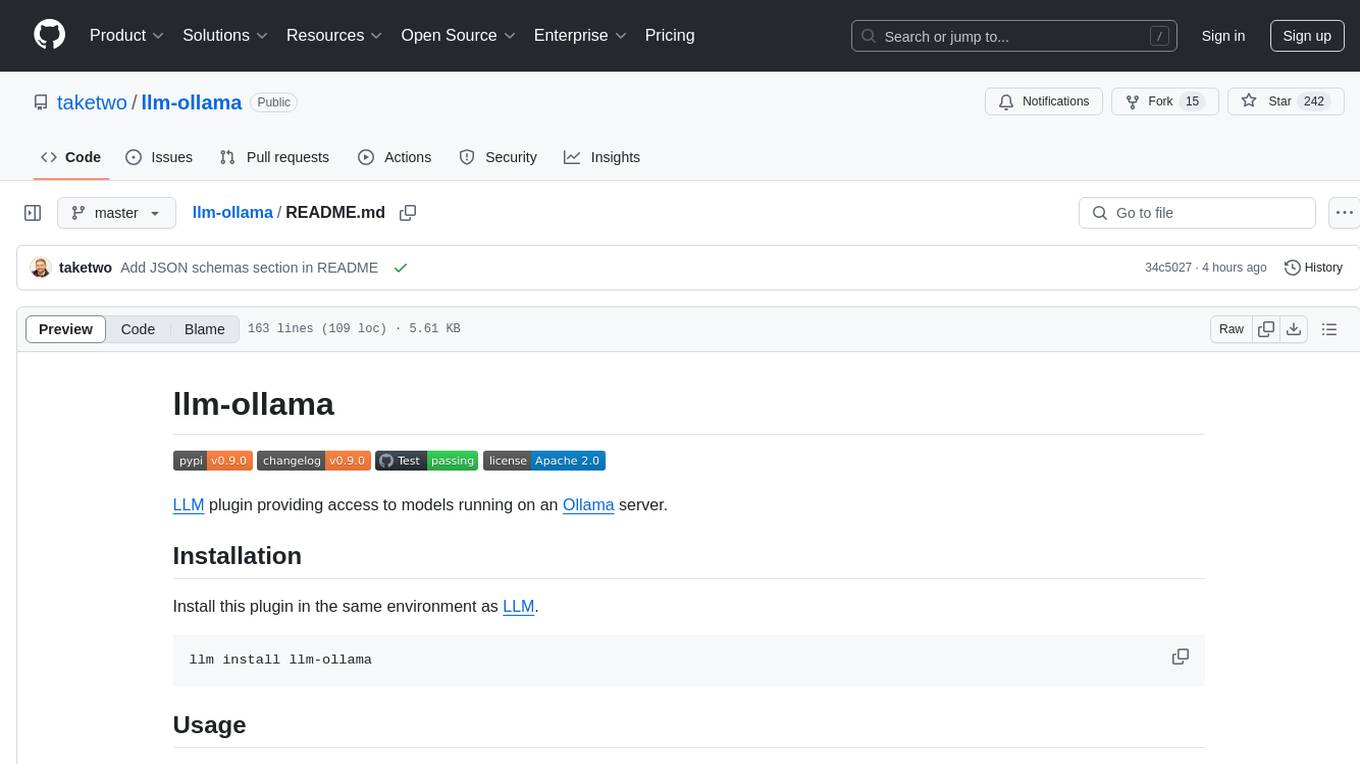
llm-ollama
LLM-ollama is a plugin that provides access to models running on an Ollama server. It allows users to query the Ollama server for a list of models, register them with LLM, and use them for prompting, chatting, and embedding. The plugin supports image attachments, embeddings, JSON schemas, async models, model aliases, and model options. Users can interact with Ollama models through the plugin in a seamless and efficient manner.
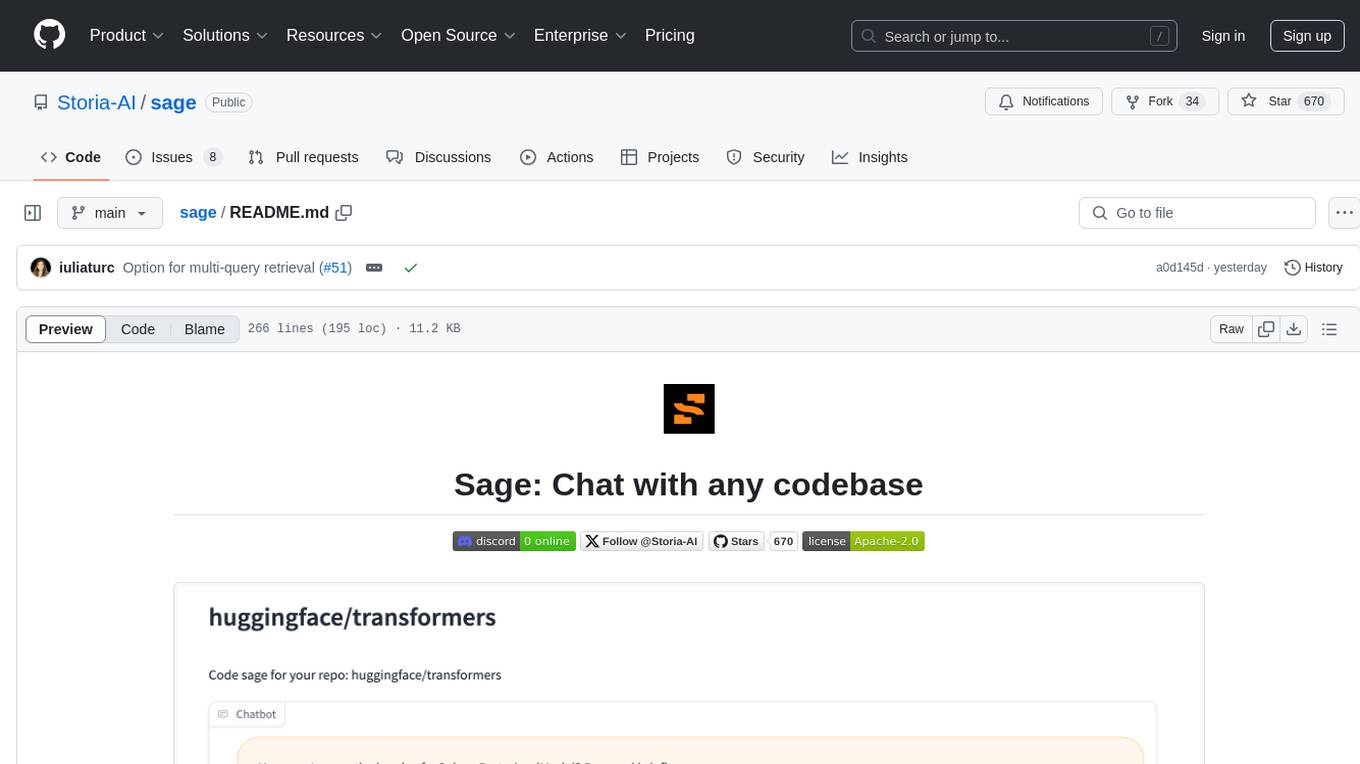
sage
Sage is a tool that allows users to chat with any codebase, providing a chat interface for code understanding and integration. It simplifies the process of learning how a codebase works by offering heavily documented answers sourced directly from the code. Users can set up Sage locally or on the cloud with minimal effort. The tool is designed to be easily customizable, allowing users to swap components of the pipeline and improve the algorithms powering code understanding and generation.
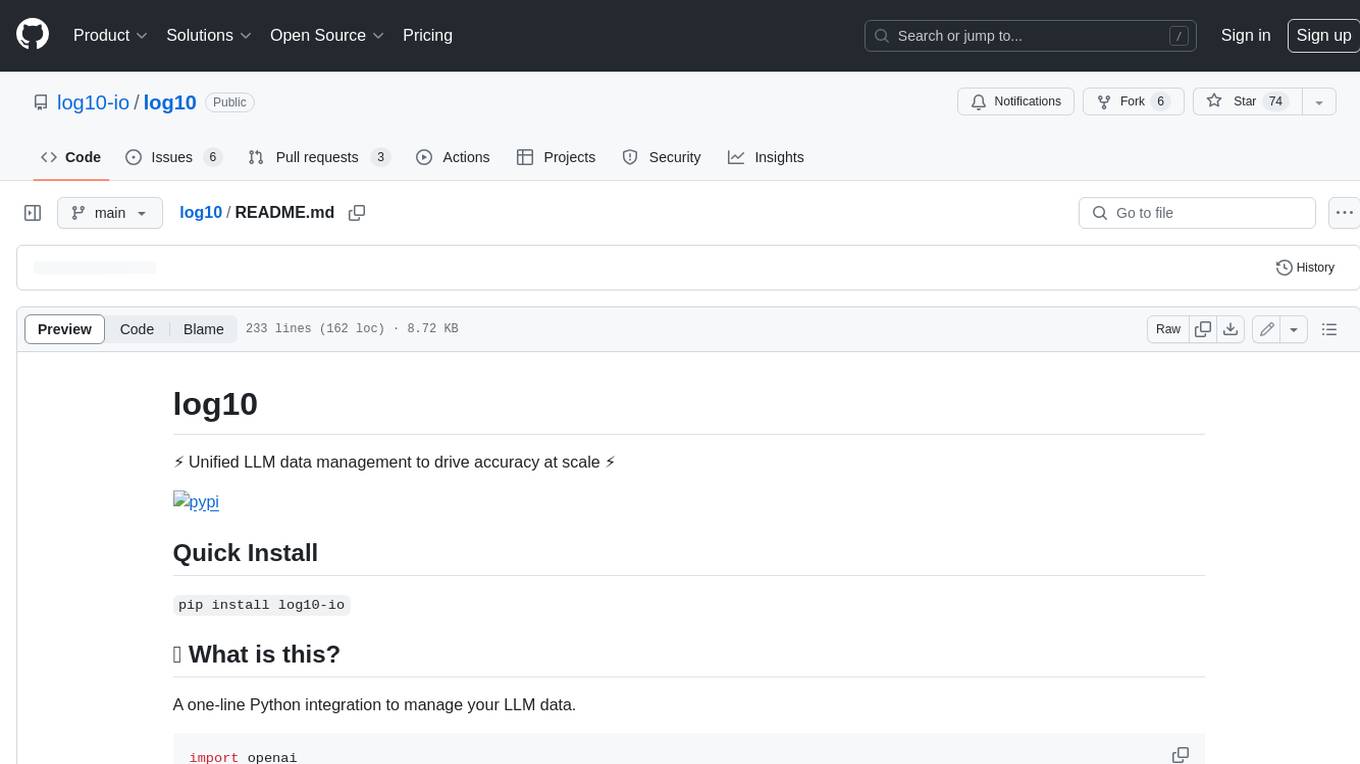
log10
Log10 is a one-line Python integration to manage your LLM data. It helps you log both closed and open-source LLM calls, compare and identify the best models and prompts, store feedback for fine-tuning, collect performance metrics such as latency and usage, and perform analytics and monitor compliance for LLM powered applications. Log10 offers various integration methods, including a python LLM library wrapper, the Log10 LLM abstraction, and callbacks, to facilitate its use in both existing production environments and new projects. Pick the one that works best for you. Log10 also provides a copilot that can help you with suggestions on how to optimize your prompt, and a feedback feature that allows you to add feedback to your completions. Additionally, Log10 provides prompt provenance, session tracking and call stack functionality to help debug prompt chains. With Log10, you can use your data and feedback from users to fine-tune custom models with RLHF, and build and deploy more reliable, accurate and efficient self-hosted models. Log10 also supports collaboration, allowing you to create flexible groups to share and collaborate over all of the above features.
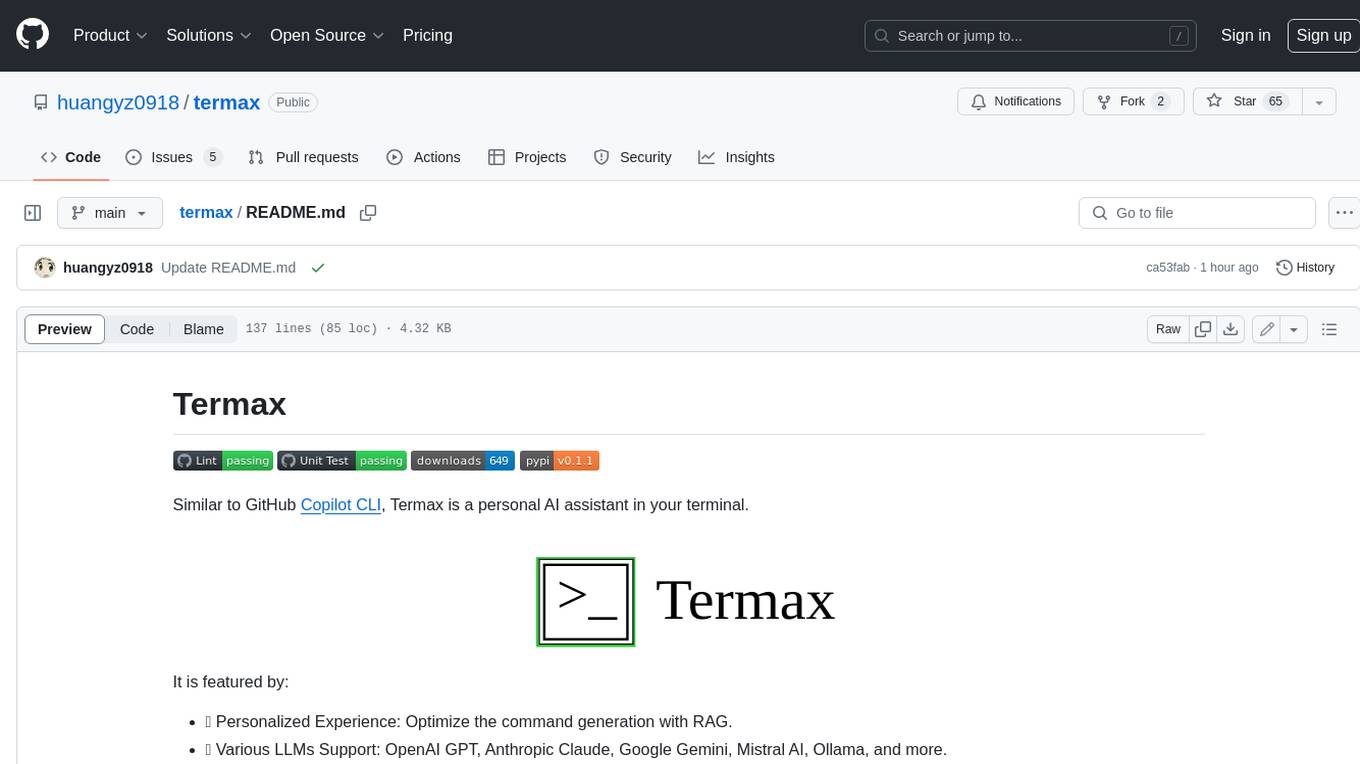
termax
Termax is an LLM agent in your terminal that converts natural language to commands. It is featured by: - Personalized Experience: Optimize the command generation with RAG. - Various LLMs Support: OpenAI GPT, Anthropic Claude, Google Gemini, Mistral AI, and more. - Shell Extensions: Plugin with popular shells like `zsh`, `bash` and `fish`. - Cross Platform: Able to run on Windows, macOS, and Linux.
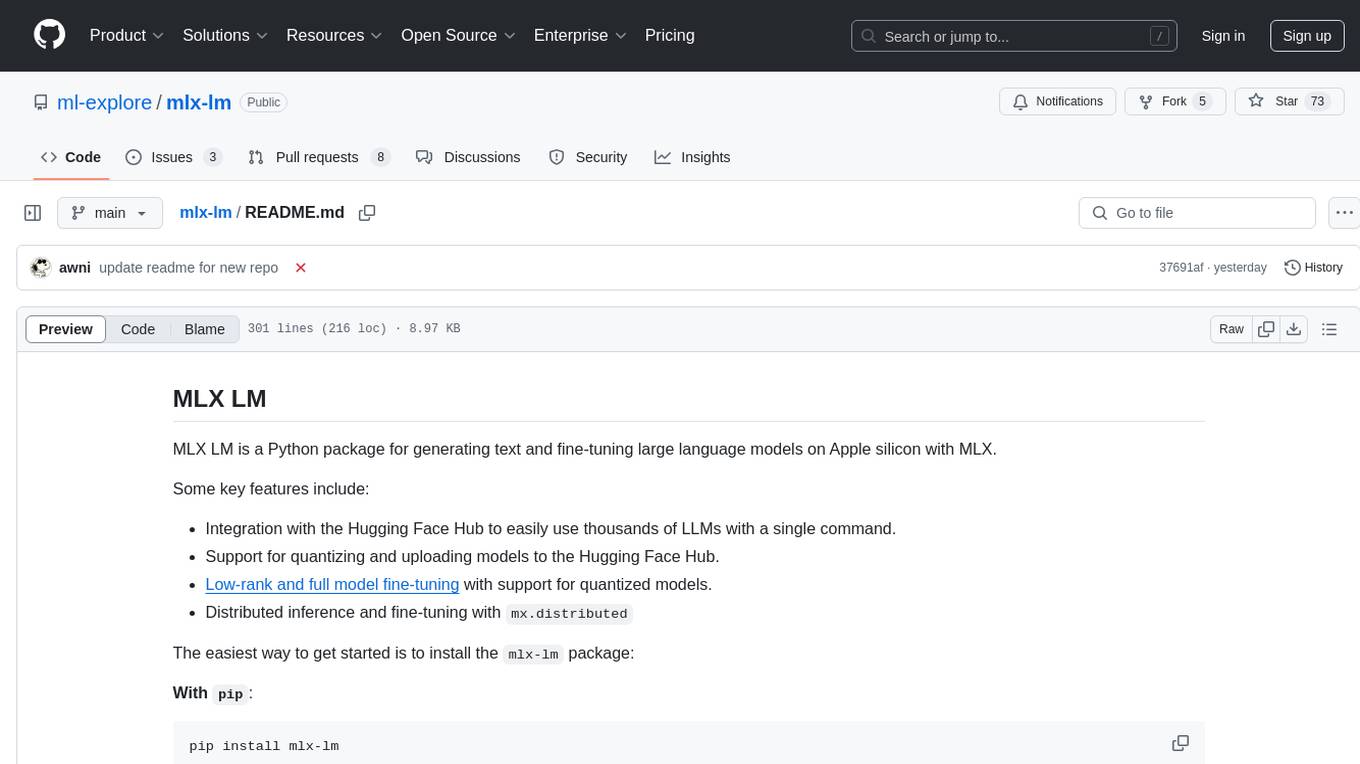
mlx-lm
MLX LM is a Python package designed for generating text and fine-tuning large language models on Apple silicon using MLX. It offers integration with the Hugging Face Hub for easy access to thousands of LLMs, support for quantizing and uploading models to the Hub, low-rank and full model fine-tuning capabilities, and distributed inference and fine-tuning with `mx.distributed`. Users can interact with the package through command line options or the Python API, enabling tasks such as text generation, chatting with language models, model conversion, streaming generation, and sampling. MLX LM supports various Hugging Face models and provides tools for efficient scaling to long prompts and generations, including a rotating key-value cache and prompt caching. It requires macOS 15.0 or higher for optimal performance.
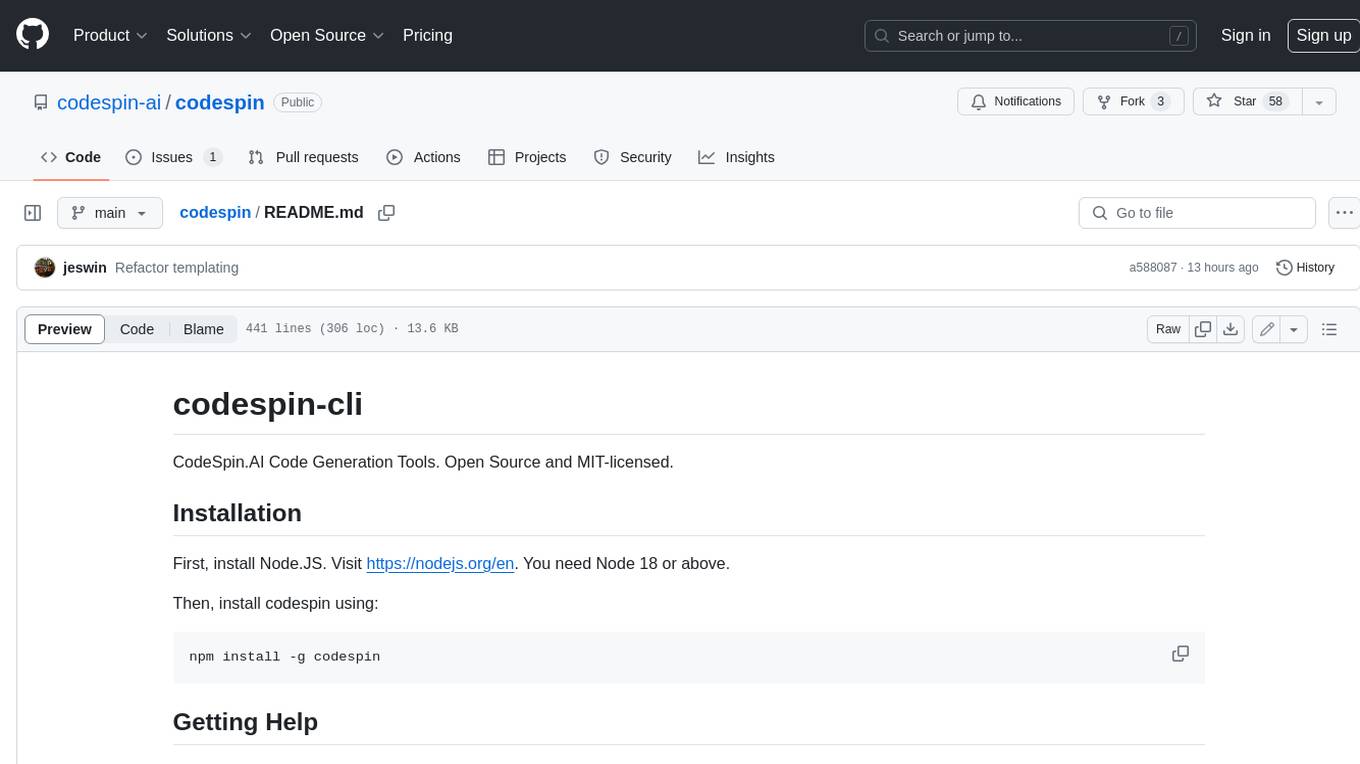
codespin
CodeSpin.AI is a set of open-source code generation tools that leverage large language models (LLMs) to automate coding tasks. With CodeSpin, you can generate code in various programming languages, including Python, JavaScript, Java, and C++, by providing natural language prompts. CodeSpin offers a range of features to enhance code generation, such as custom templates, inline prompting, and the ability to use ChatGPT as an alternative to API keys. Additionally, CodeSpin provides options for regenerating code, executing code in prompt files, and piping data into the LLM for processing. By utilizing CodeSpin, developers can save time and effort in coding tasks, improve code quality, and explore new possibilities in code generation.
For similar tasks
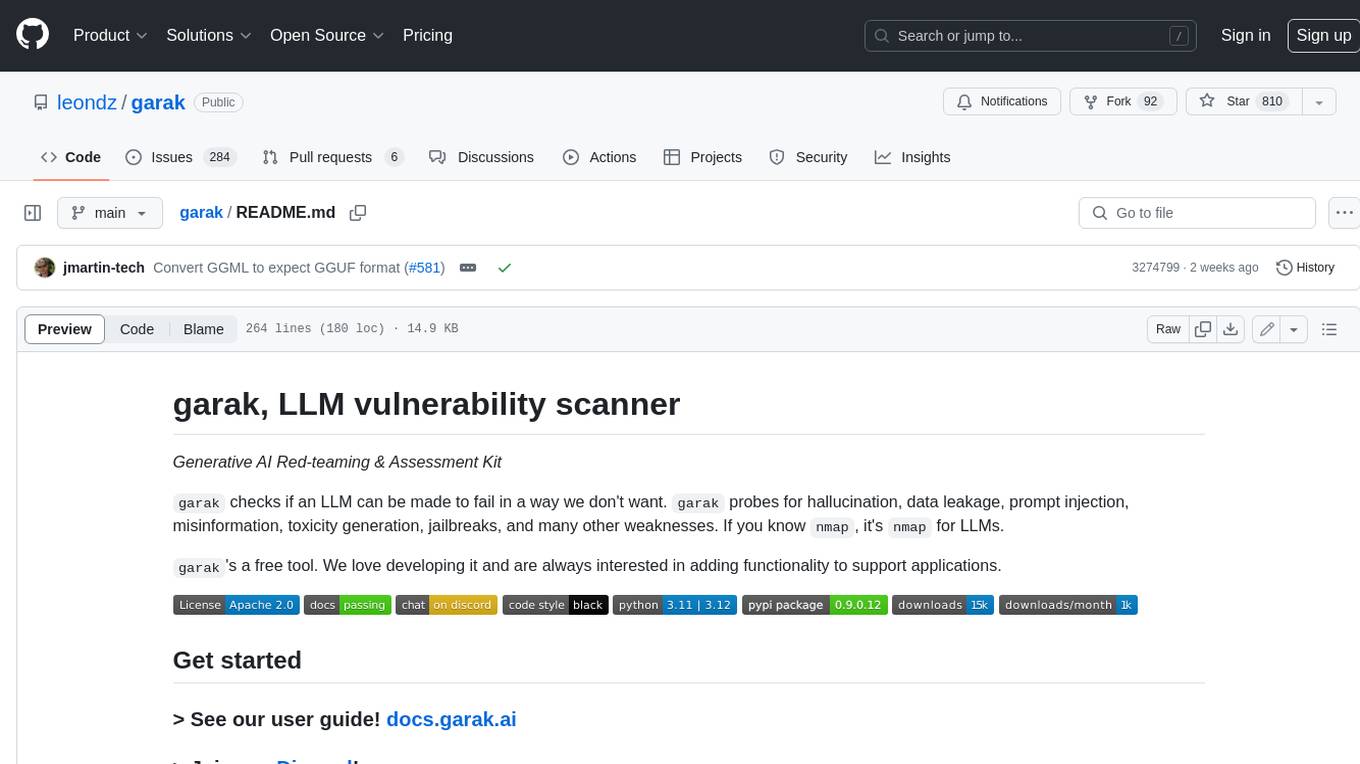
garak
Garak is a free tool that checks if a Large Language Model (LLM) can be made to fail in a way that is undesirable. It probes for hallucination, data leakage, prompt injection, misinformation, toxicity generation, jailbreaks, and many other weaknesses. Garak's a free tool. We love developing it and are always interested in adding functionality to support applications.
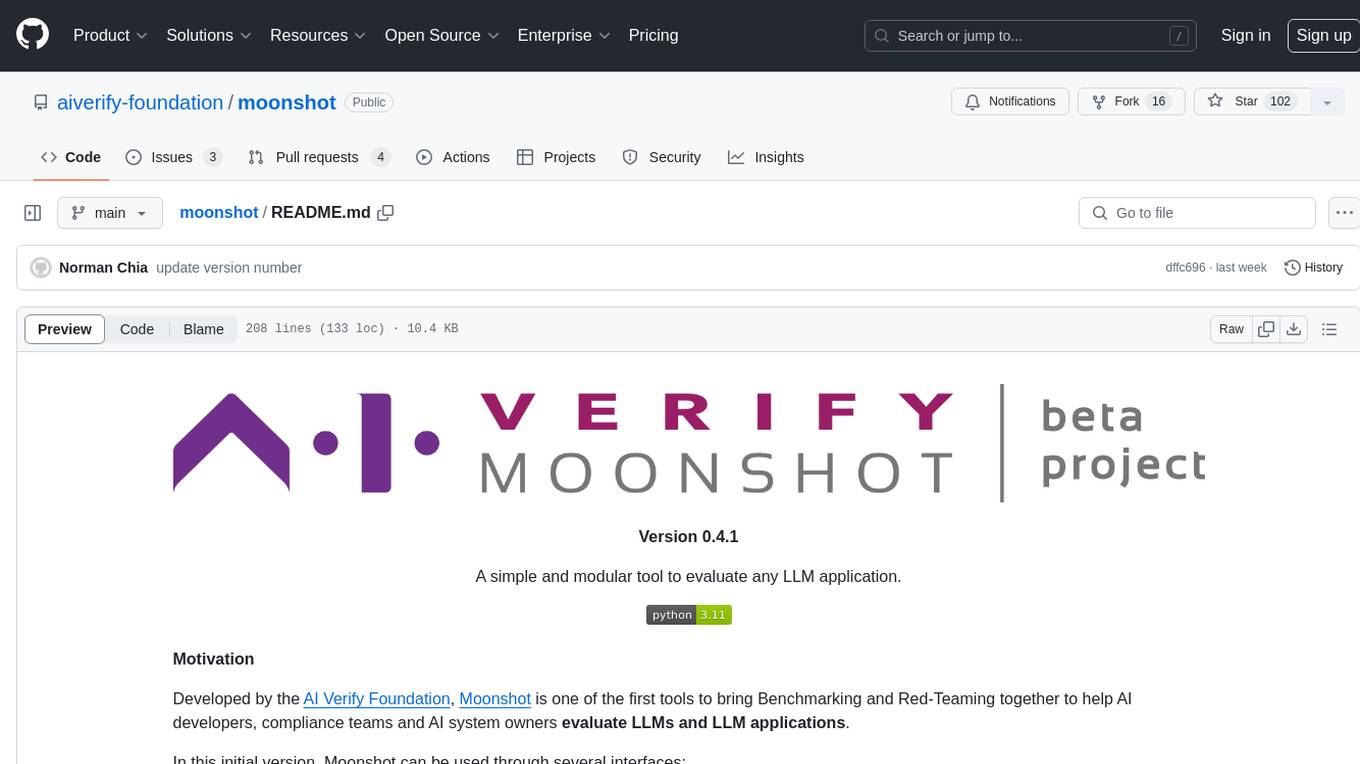
moonshot
Moonshot is a simple and modular tool developed by the AI Verify Foundation to evaluate Language Model Models (LLMs) and LLM applications. It brings Benchmarking and Red-Teaming together to assist AI developers, compliance teams, and AI system owners in assessing LLM performance. Moonshot can be accessed through various interfaces including User-friendly Web UI, Interactive Command Line Interface, and seamless integration into MLOps workflows via Library APIs or Web APIs. It offers features like benchmarking LLMs from popular model providers, running relevant tests, creating custom cookbooks and recipes, and automating Red Teaming to identify vulnerabilities in AI systems.
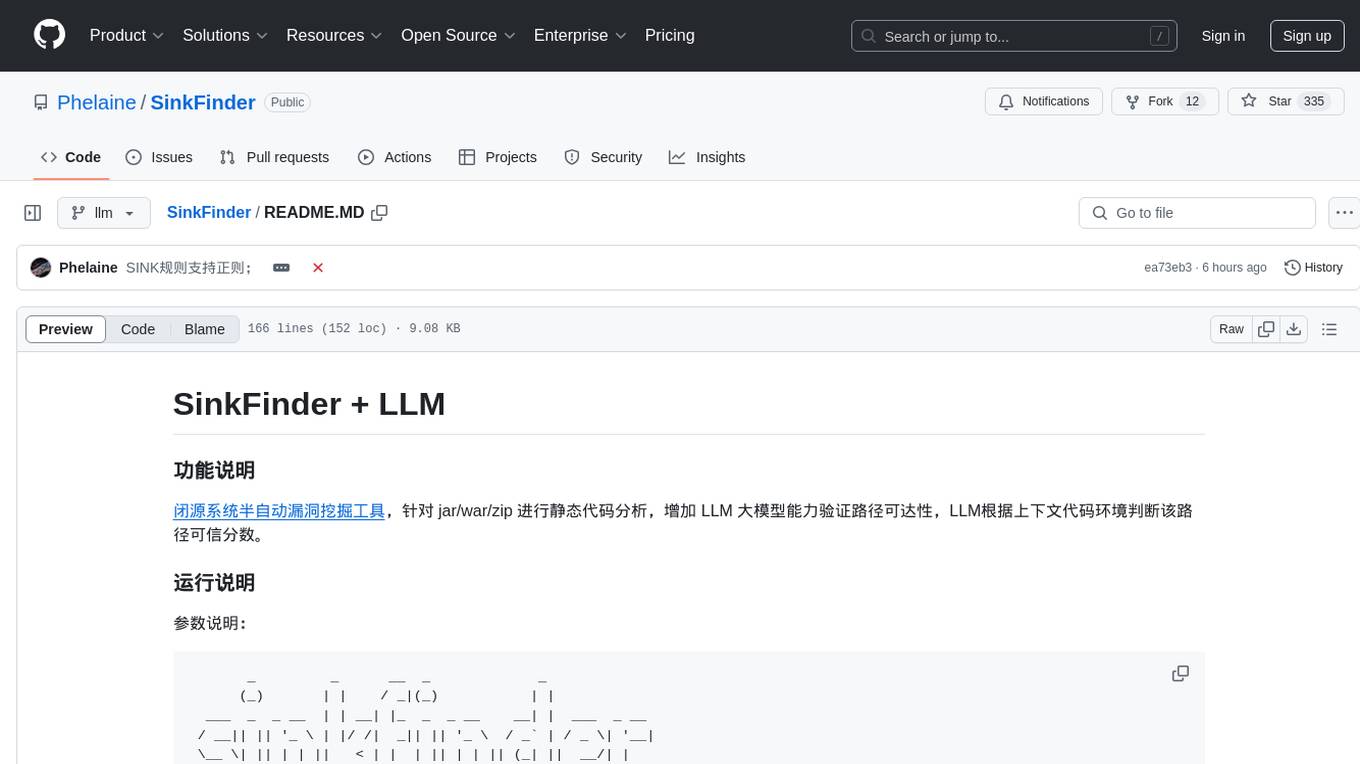
SinkFinder
SinkFinder + LLM is a closed-source semi-automatic vulnerability discovery tool that performs static code analysis on jar/war/zip files. It enhances the capability of LLM large models to verify path reachability and assess the trustworthiness score of the path based on the contextual code environment. Users can customize class and jar exclusions, depth of recursive search, and other parameters through command-line arguments. The tool generates rule.json configuration file after each run and requires configuration of the DASHSCOPE_API_KEY for LLM capabilities. The tool provides detailed logs on high-risk paths, LLM results, and other findings. Rules.json file contains sink rules for various vulnerability types with severity levels and corresponding sink methods.
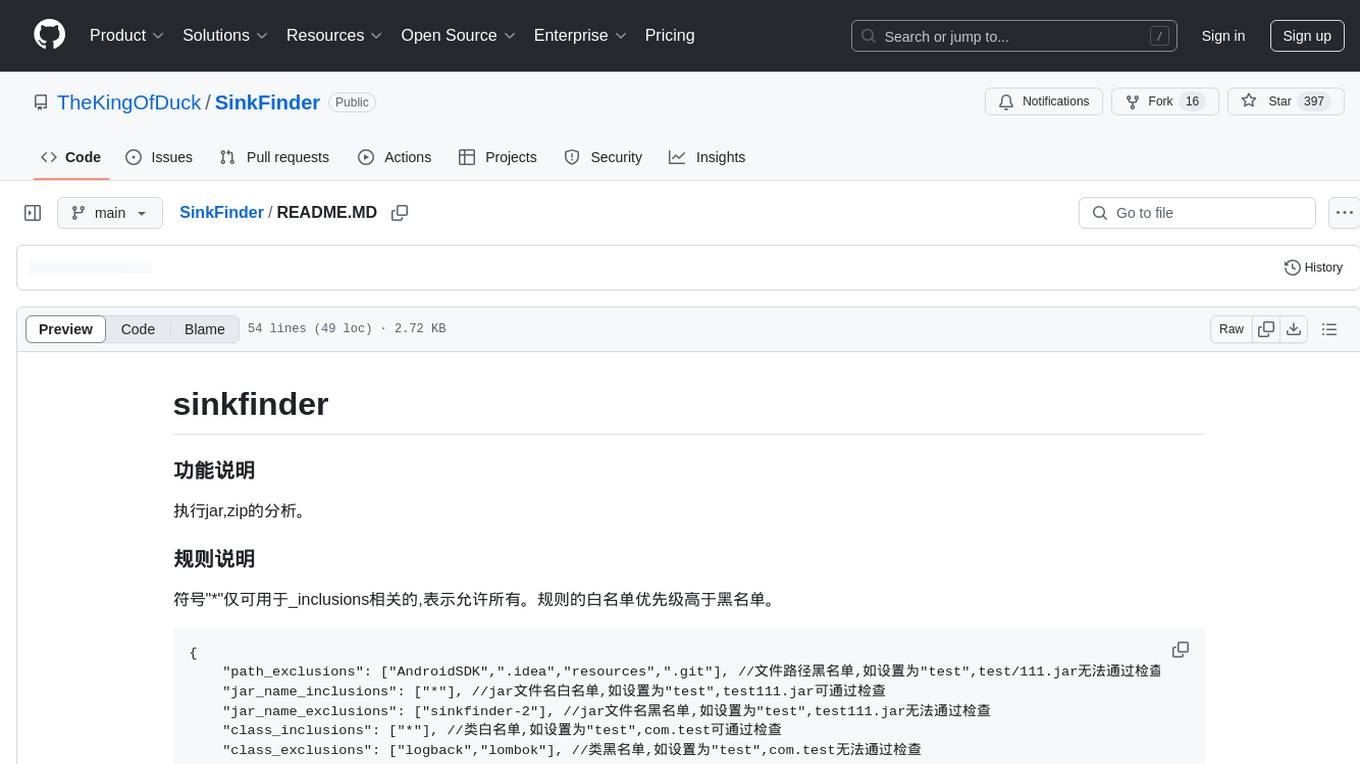
SinkFinder
SinkFinder is a tool designed to analyze jar and zip files for security vulnerabilities. It allows users to define rules for white and blacklisting specific classes and methods that may pose a risk. The tool provides a list of common security sink names along with severity levels and associated vulnerable methods. Users can use SinkFinder to quickly identify potential security issues in their Java applications by scanning for known sink patterns and configurations.
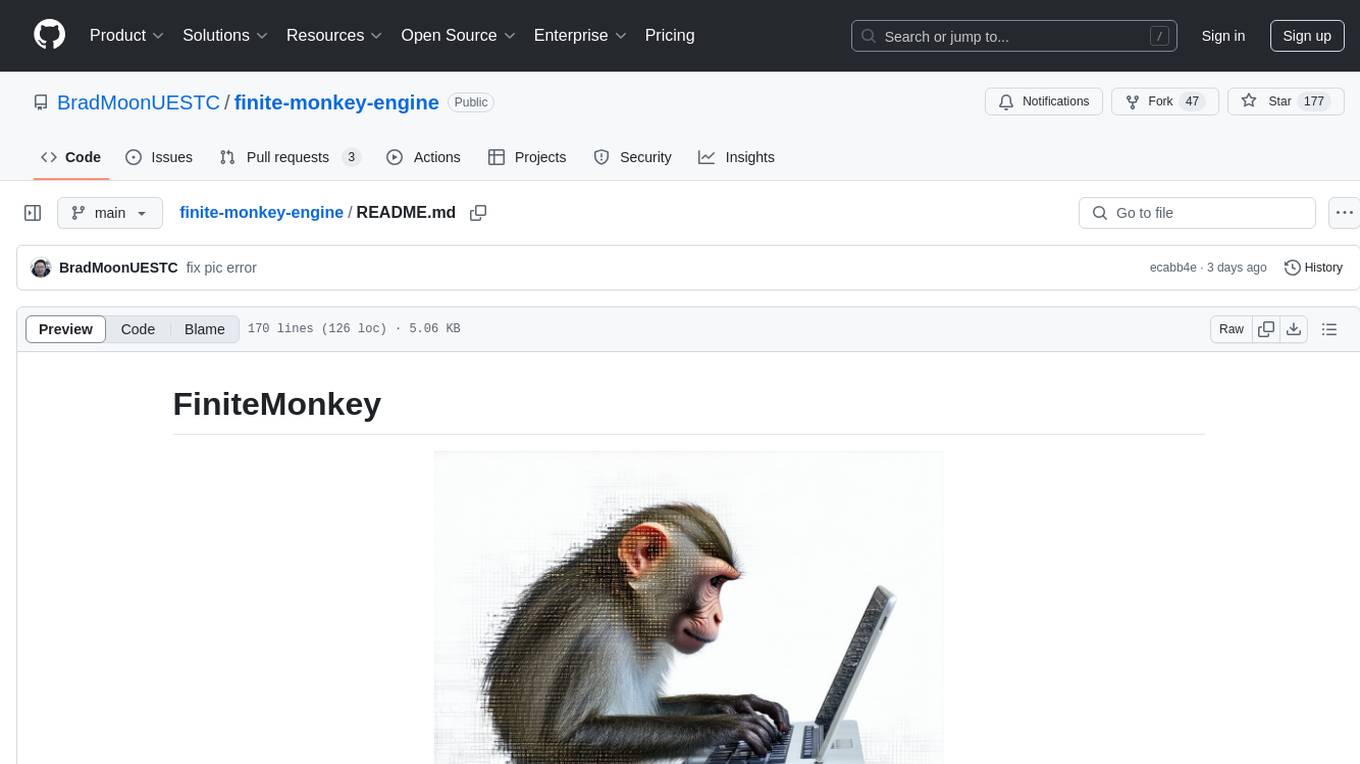
finite-monkey-engine
FiniteMonkey is an advanced vulnerability mining engine powered purely by GPT, requiring no prior knowledge base or fine-tuning. Its effectiveness significantly surpasses most current related research approaches. The tool is task-driven, prompt-driven, and focuses on prompt design, leveraging 'deception' and hallucination as key mechanics. It has helped identify vulnerabilities worth over $60,000 in bounties. The tool requires PostgreSQL database, OpenAI API access, and Python environment for setup. It supports various languages like Solidity, Rust, Python, Move, Cairo, Tact, Func, Java, and Fake Solidity for scanning. FiniteMonkey is best suited for logic vulnerability mining in real projects, not recommended for academic vulnerability testing. GPT-4-turbo is recommended for optimal results with an average scan time of 2-3 hours for medium projects. The tool provides detailed scanning results guide and implementation tips for users.
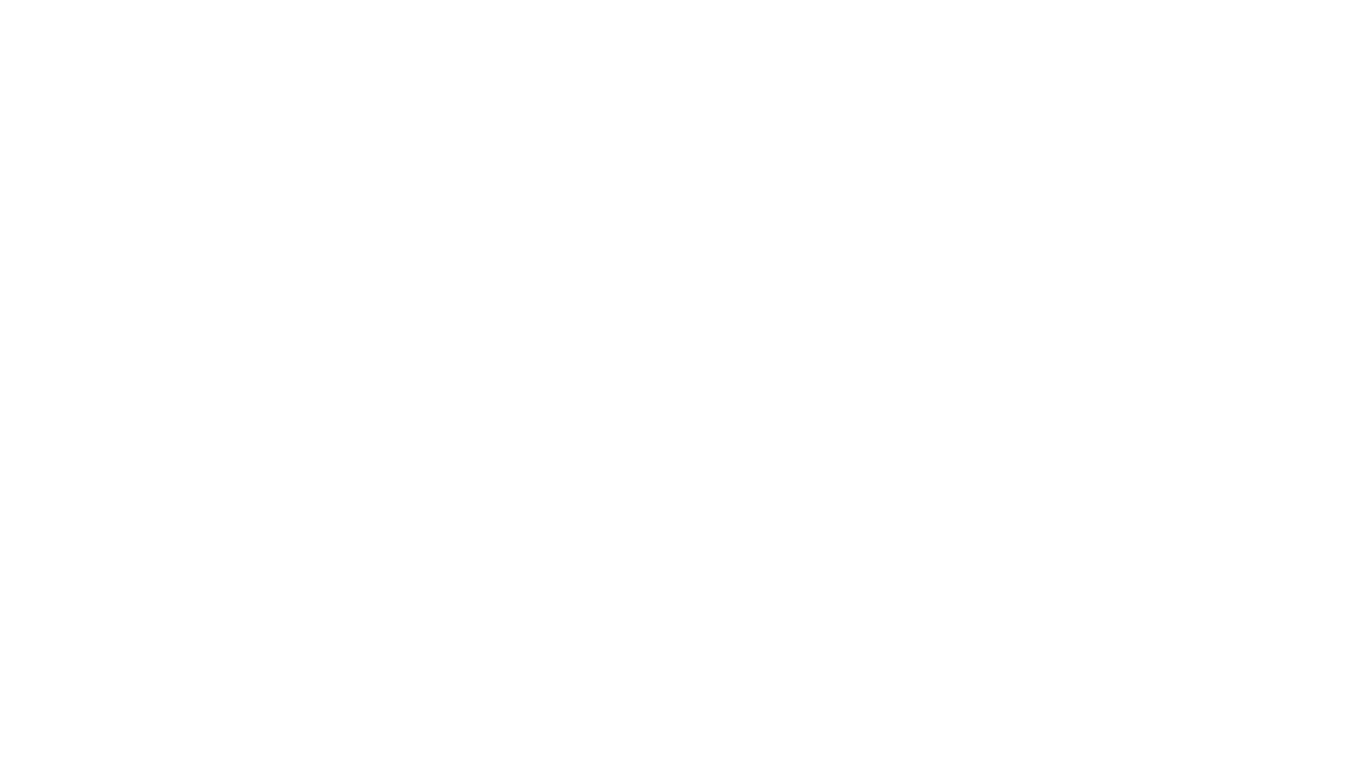
agentic-radar
The Agentic Radar is a security scanner designed to analyze and assess agentic systems for security and operational insights. It helps users understand how agentic systems function, identify potential vulnerabilities, and create security reports. The tool includes workflow visualization, tool identification, and vulnerability mapping, providing a comprehensive HTML report for easy reviewing and sharing. It simplifies the process of assessing complex workflows and multiple tools used in agentic systems, offering a structured view of potential risks and security frameworks.
For similar jobs
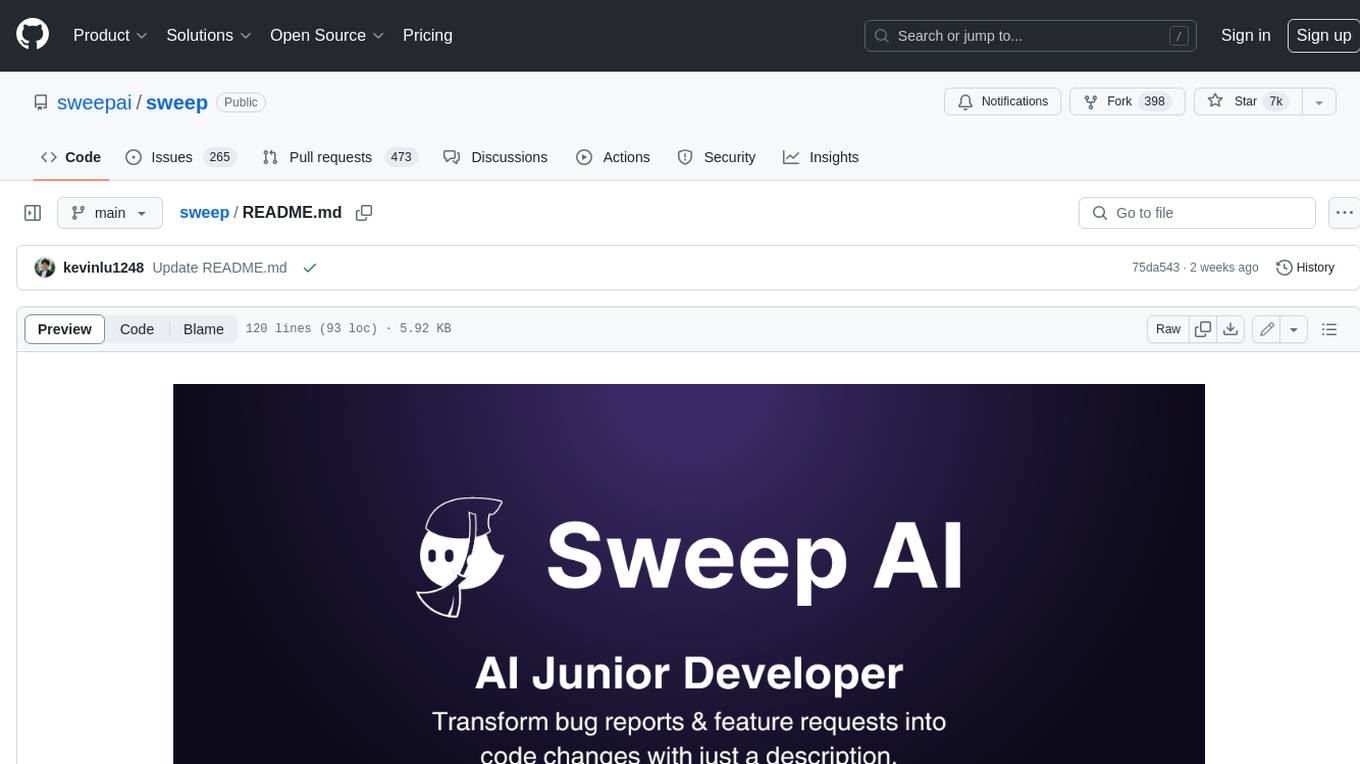
sweep
Sweep is an AI junior developer that turns bugs and feature requests into code changes. It automatically handles developer experience improvements like adding type hints and improving test coverage.
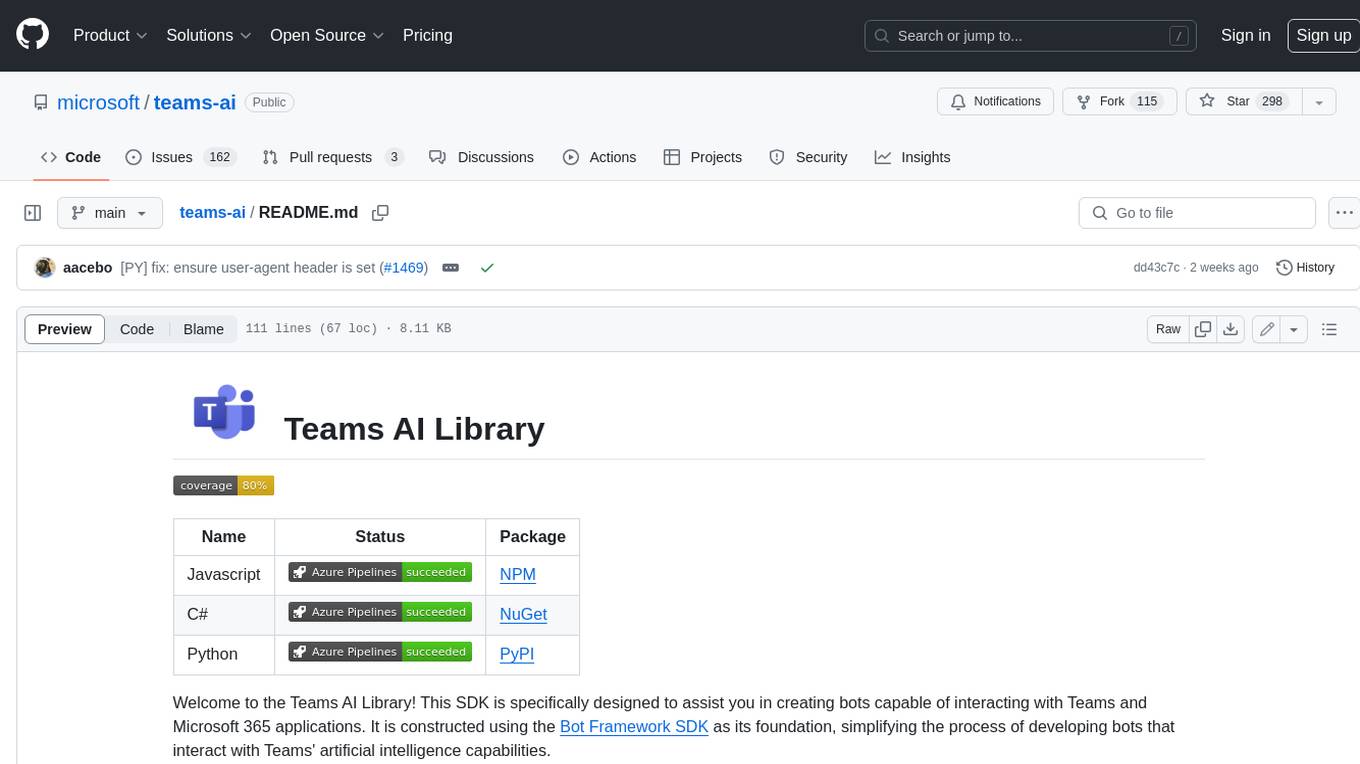
teams-ai
The Teams AI Library is a software development kit (SDK) that helps developers create bots that can interact with Teams and Microsoft 365 applications. It is built on top of the Bot Framework SDK and simplifies the process of developing bots that interact with Teams' artificial intelligence capabilities. The SDK is available for JavaScript/TypeScript, .NET, and Python.
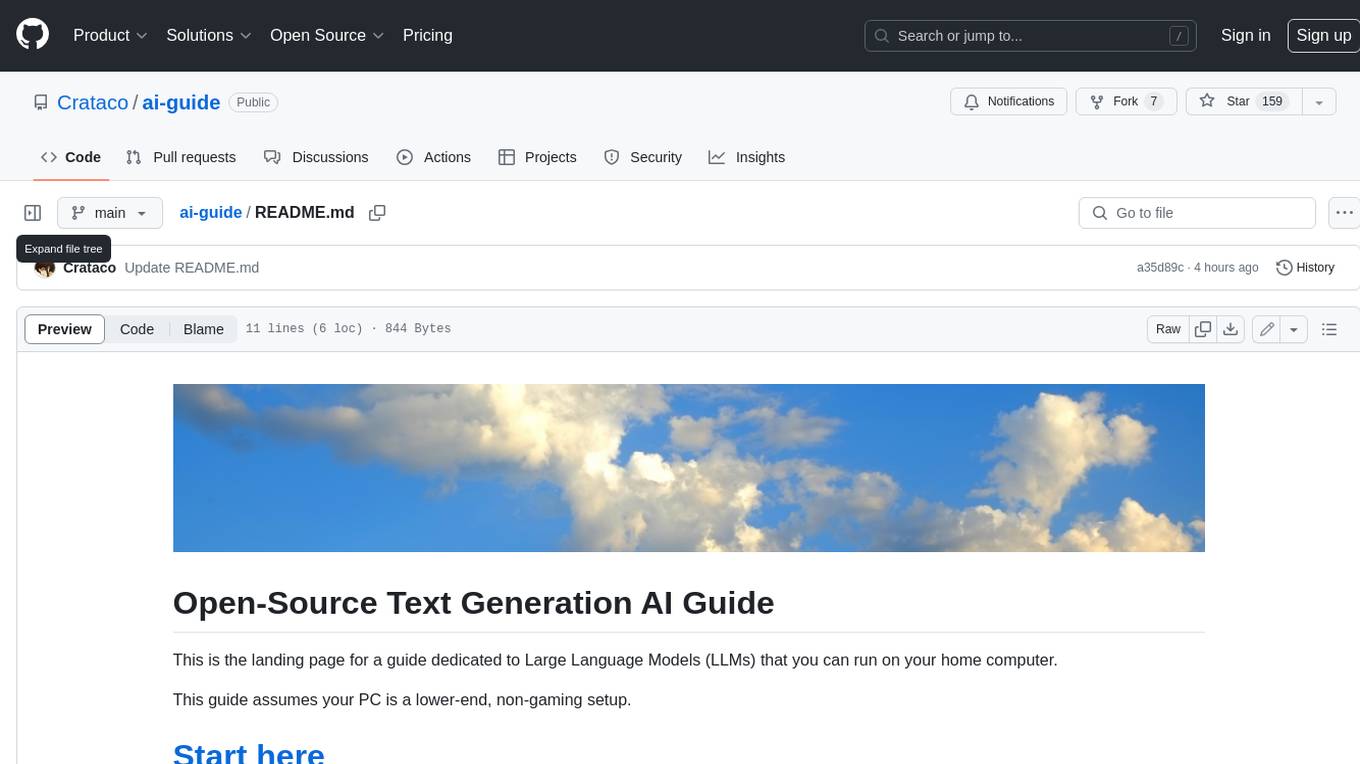
ai-guide
This guide is dedicated to Large Language Models (LLMs) that you can run on your home computer. It assumes your PC is a lower-end, non-gaming setup.
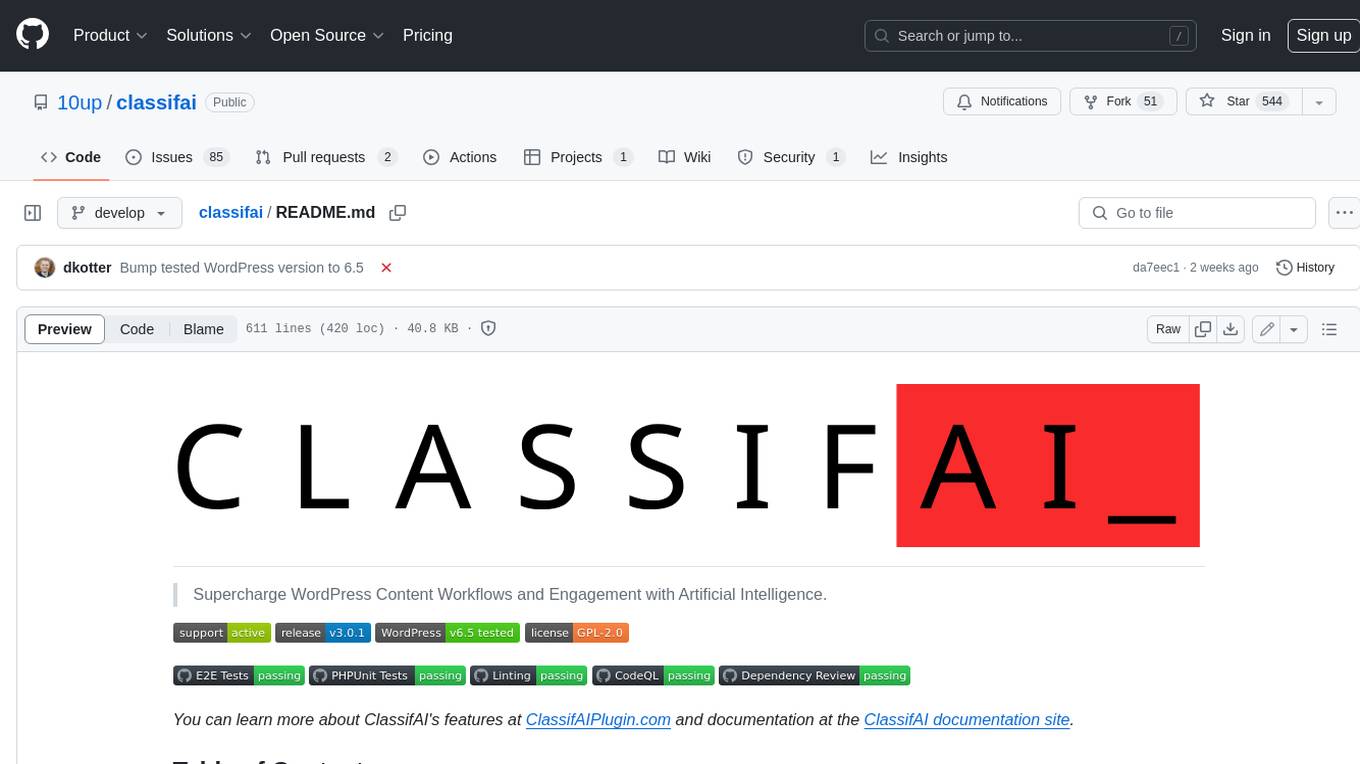
classifai
Supercharge WordPress Content Workflows and Engagement with Artificial Intelligence. Tap into leading cloud-based services like OpenAI, Microsoft Azure AI, Google Gemini and IBM Watson to augment your WordPress-powered websites. Publish content faster while improving SEO performance and increasing audience engagement. ClassifAI integrates Artificial Intelligence and Machine Learning technologies to lighten your workload and eliminate tedious tasks, giving you more time to create original content that matters.
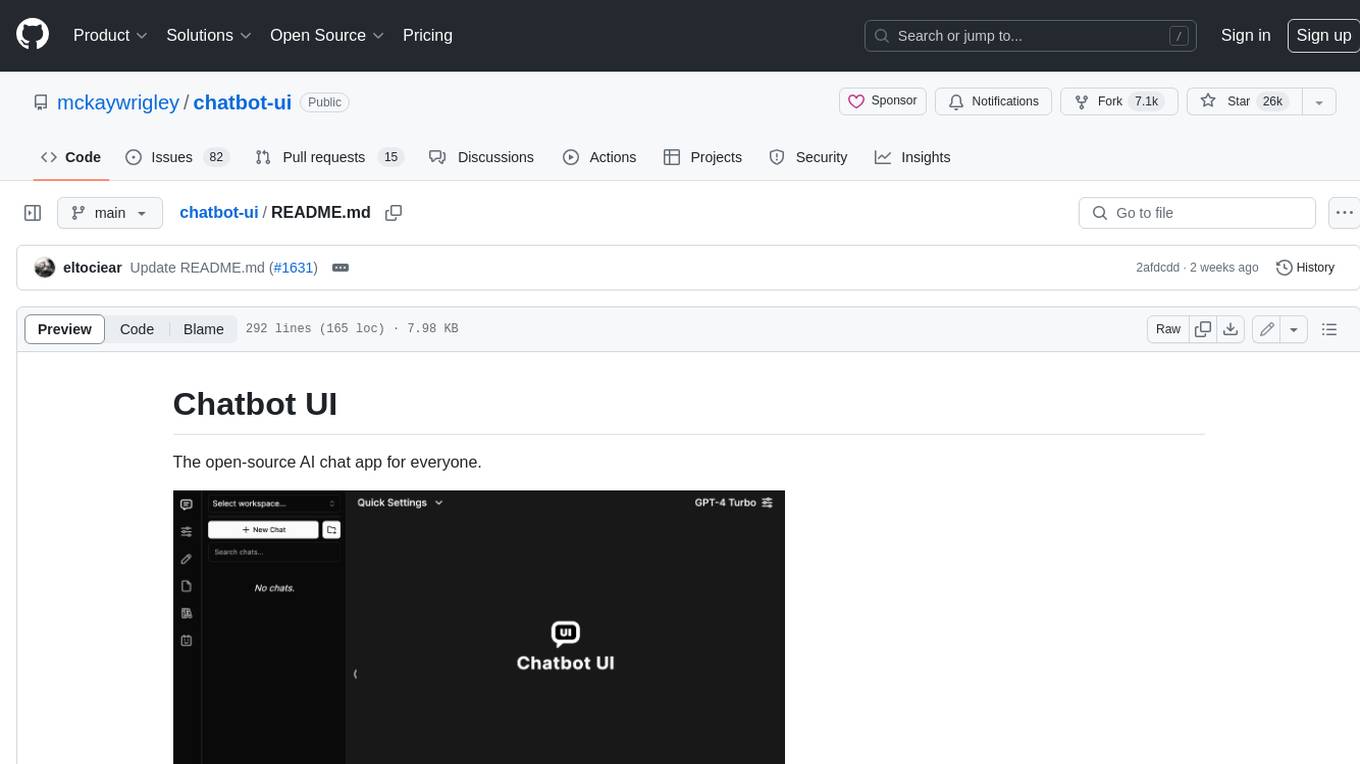
chatbot-ui
Chatbot UI is an open-source AI chat app that allows users to create and deploy their own AI chatbots. It is easy to use and can be customized to fit any need. Chatbot UI is perfect for businesses, developers, and anyone who wants to create a chatbot.
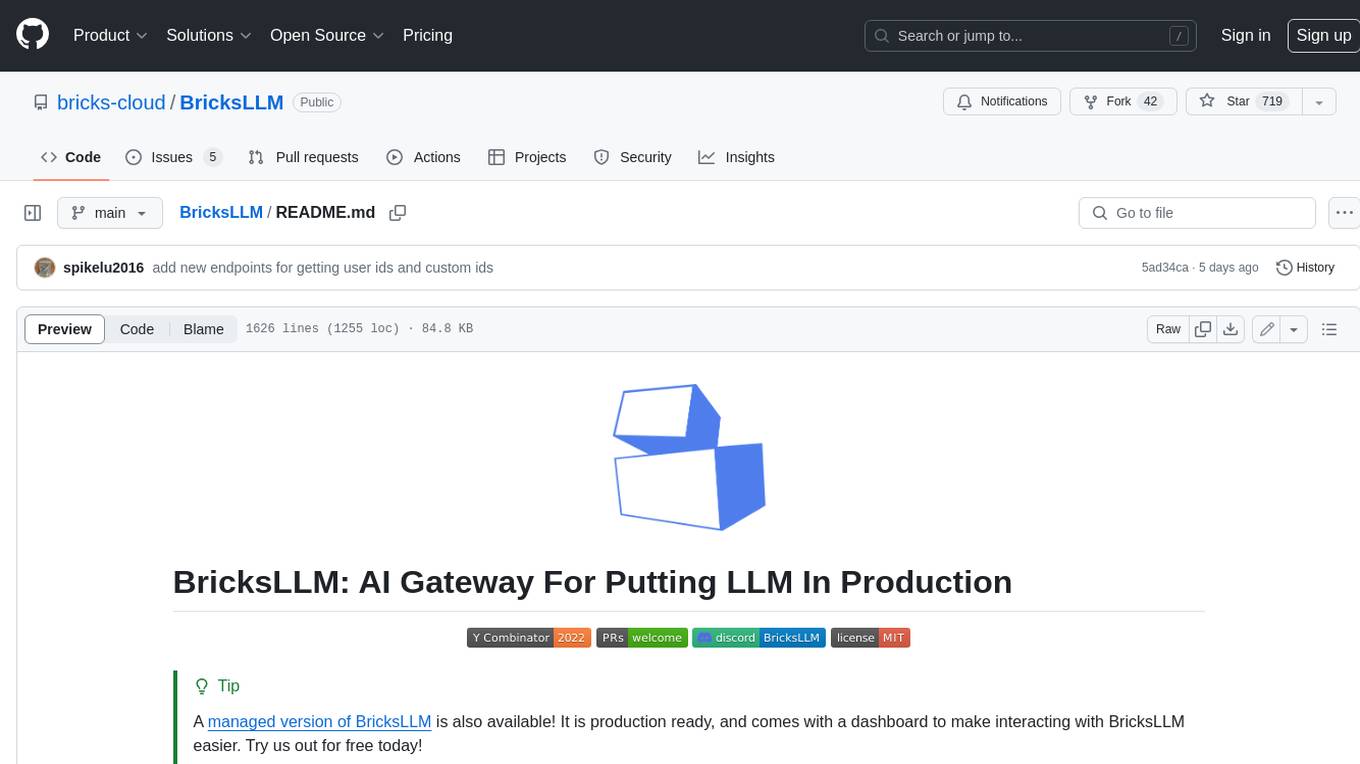
BricksLLM
BricksLLM is a cloud native AI gateway written in Go. Currently, it provides native support for OpenAI, Anthropic, Azure OpenAI and vLLM. BricksLLM aims to provide enterprise level infrastructure that can power any LLM production use cases. Here are some use cases for BricksLLM: * Set LLM usage limits for users on different pricing tiers * Track LLM usage on a per user and per organization basis * Block or redact requests containing PIIs * Improve LLM reliability with failovers, retries and caching * Distribute API keys with rate limits and cost limits for internal development/production use cases * Distribute API keys with rate limits and cost limits for students
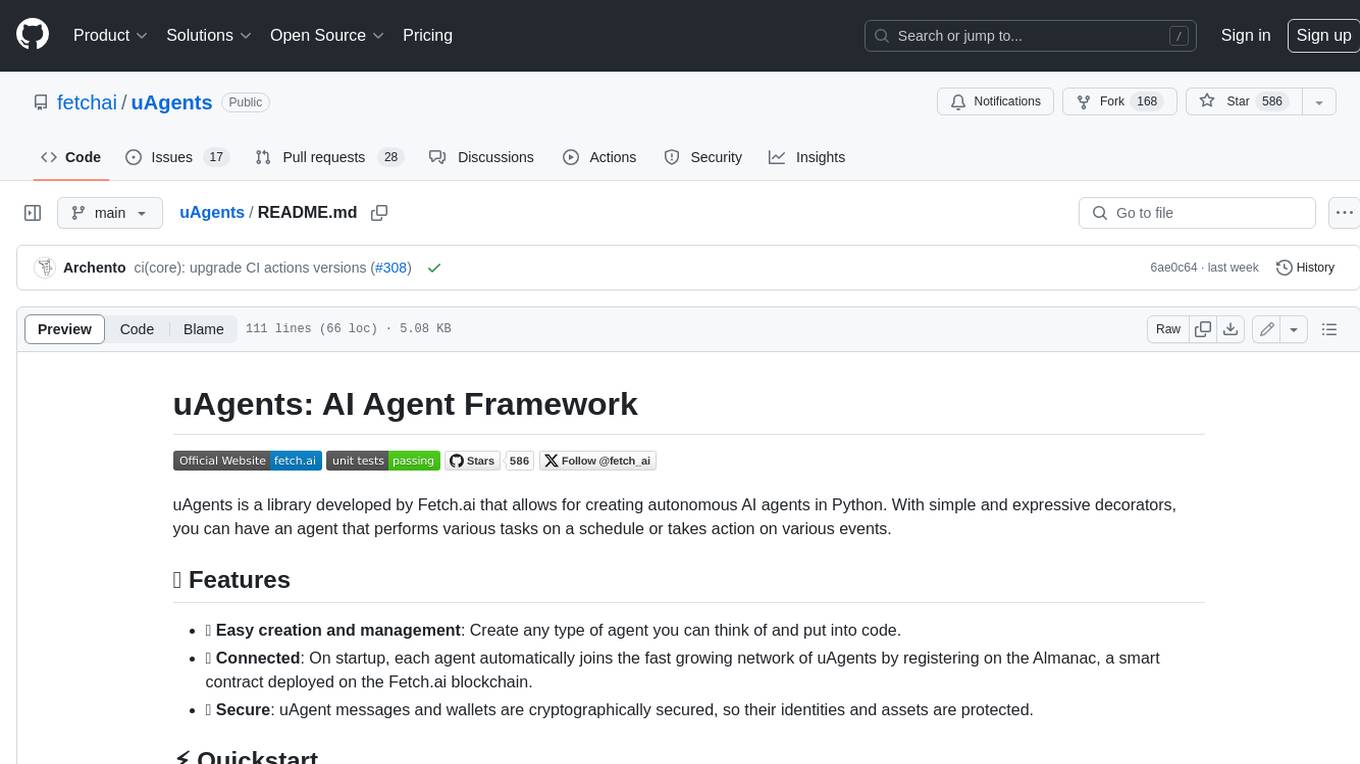
uAgents
uAgents is a Python library developed by Fetch.ai that allows for the creation of autonomous AI agents. These agents can perform various tasks on a schedule or take action on various events. uAgents are easy to create and manage, and they are connected to a fast-growing network of other uAgents. They are also secure, with cryptographically secured messages and wallets.
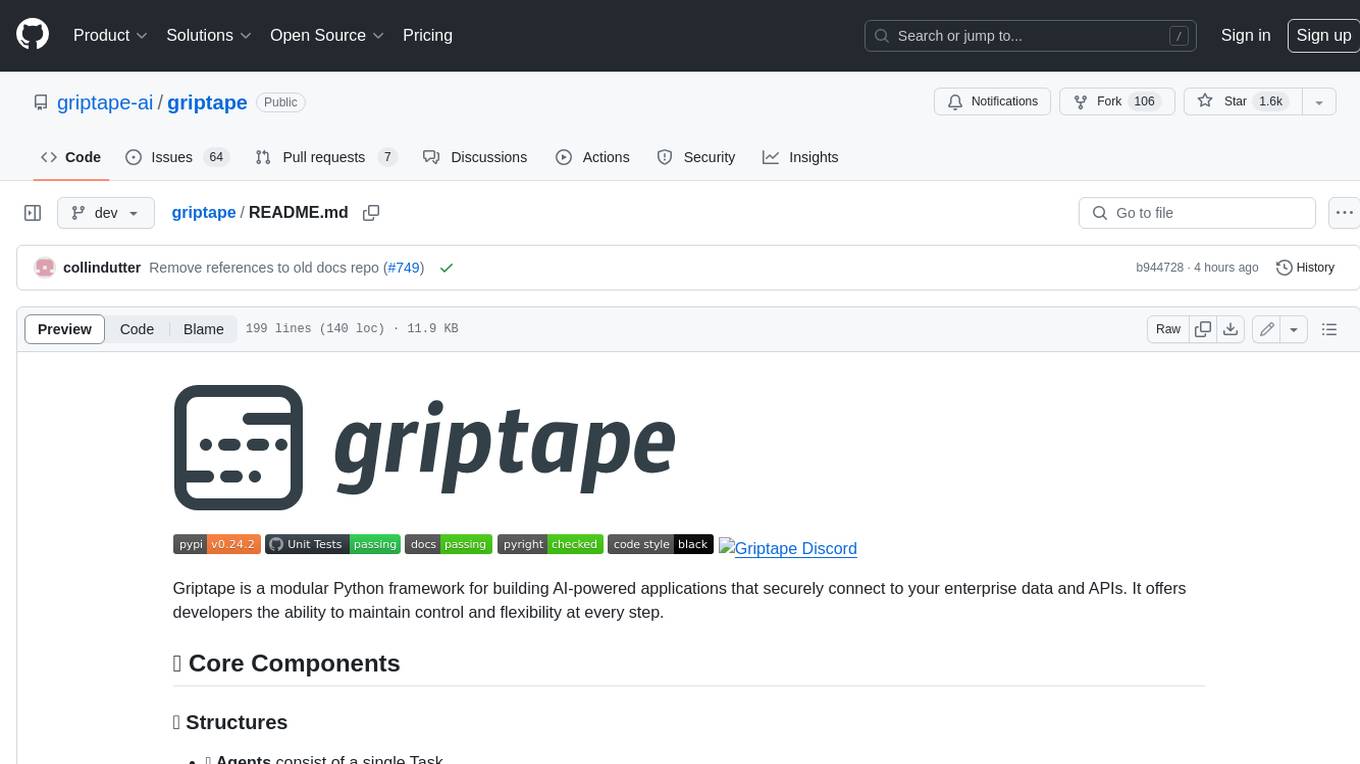
griptape
Griptape is a modular Python framework for building AI-powered applications that securely connect to your enterprise data and APIs. It offers developers the ability to maintain control and flexibility at every step. Griptape's core components include Structures (Agents, Pipelines, and Workflows), Tasks, Tools, Memory (Conversation Memory, Task Memory, and Meta Memory), Drivers (Prompt and Embedding Drivers, Vector Store Drivers, Image Generation Drivers, Image Query Drivers, SQL Drivers, Web Scraper Drivers, and Conversation Memory Drivers), Engines (Query Engines, Extraction Engines, Summary Engines, Image Generation Engines, and Image Query Engines), and additional components (Rulesets, Loaders, Artifacts, Chunkers, and Tokenizers). Griptape enables developers to create AI-powered applications with ease and efficiency.