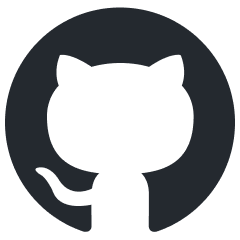
code2prompt
A CLI tool to convert your codebase into a single LLM prompt with source tree, prompt templating, and token counting.
Stars: 5055
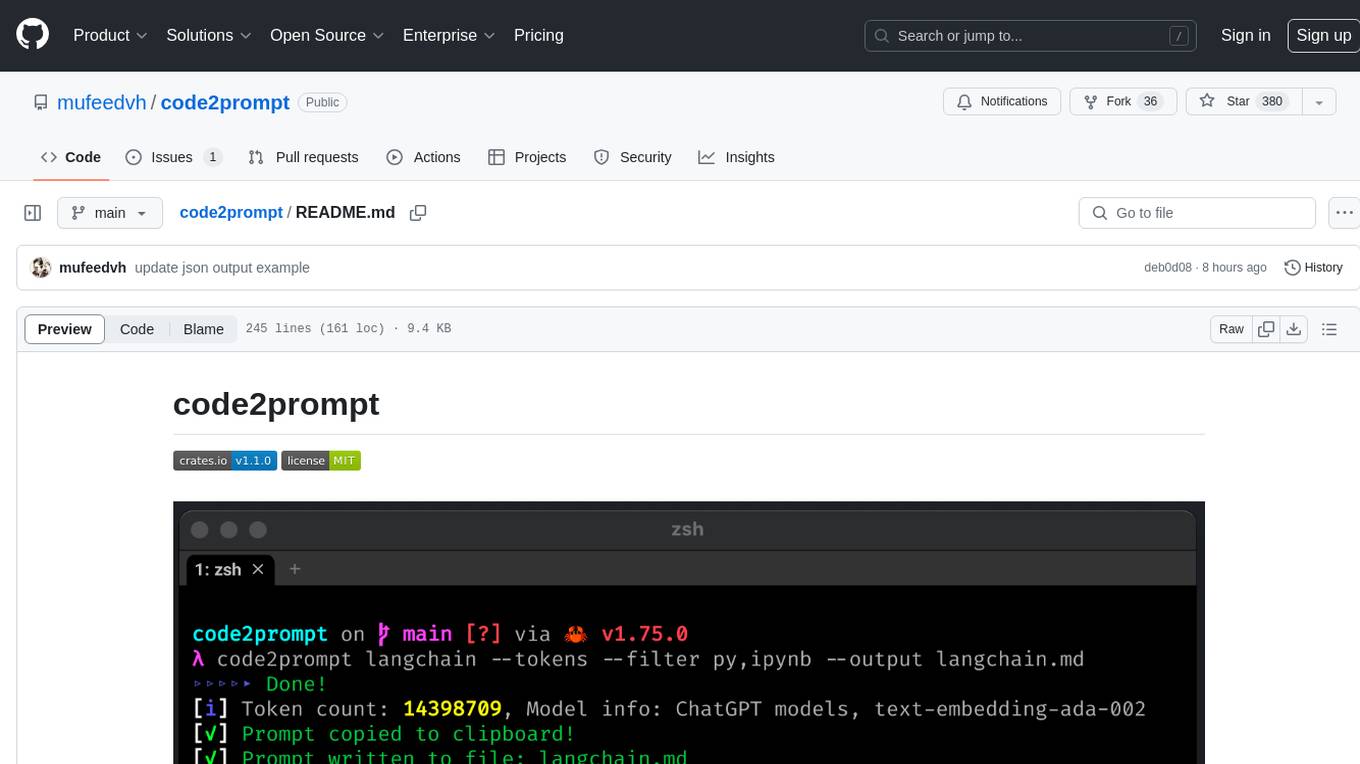
code2prompt is a command-line tool that converts your codebase into a single LLM prompt with a source tree, prompt templating, and token counting. It automates generating LLM prompts from codebases of any size, customizing prompt generation with Handlebars templates, respecting .gitignore, filtering and excluding files using glob patterns, displaying token count, including Git diff output, copying prompt to clipboard, saving prompt to an output file, excluding files and folders, adding line numbers to source code blocks, and more. It helps streamline the process of creating LLM prompts for code analysis, generation, and other tasks.
README:
Want to engage with us ? Join our Discord channel!
Stay updated on new features đĸ
Give your insight and suggestion đŦ
Get help with configuration and usage đ ī¸
Report Bug đ
CLI with cargo đĻ
cargo install code2prompt
SDK with pip đ
pip install code2prompt-rs
CLI
code2prompt
makes it easy to generate prompts for LLMs from your codebase. It traverses the directory, builds a tree structure, and collects information about each file. You can customize the prompt generation using Handlebars templates. The generated prompt is automatically copied to your clipboard and can also be saved to an output file. code2prompt
helps streamline the process of creating LLM prompts for code analysis, generation, and other tasks.
SDK
code2prompt
python binding helps programs to interact with codebase. It's useful to ingest codebase for AI agent or automation scripts.
Check our online documentation for usage
- Features
- Installation
- Usage
- Templates
- User Defined Variables
- Tokenizers
- Python SDK
- Contribution
- License
You can run this tool on the entire directory and it would generate a well-formatted Markdown prompt detailing the source tree structure, and all the code. You can then upload this document to either GPT or Claude models with higher context windows and ask it to:
- Quickly generate LLM prompts from codebases of any size.
- Customize prompt generation with Handlebars templates. (See the default template)
- Respects
.gitignore
(can be disabled with--no-ignore
). - Filter and exclude files using glob patterns.
- Control hidden file inclusion with
--hidden
flag. - Display the token count of the generated prompt. (See Tokenizers for more details)
- Optionally include Git diff output (staged files) in the generated prompt.
- Automatically copy the generated prompt to the clipboard.
- Save the generated prompt to an output file.
- Exclude files and folders by name or path.
- Add line numbers to source code blocks.
You can customize the prompt template to achieve any of the desired use cases. It essentially traverses a codebase and creates a prompt with all source files combined. In short, it automates copy-pasting multiple source files into your prompt and formatting them along with letting you know how many tokens your code consumes.
Download the latest binary for your OS from Releases.
Requires:
git clone https://github.com/mufeedvh/code2prompt.git
cd code2prompt/
cargo build --release
installs from the crates.io
registry.
cargo install code2prompt
For unpublished builds:
cargo install --git https://github.com/mufeedvh/code2prompt --force
code2prompt
is available in the AUR
. Install it via any AUR helpers.
paru/yay -S code2prompt
If you are on nix, You can use nix-env
or profile
to install.
# without flakes:
nix-env -iA nixpkgs.code2prompt
# with flakes:
nix profile install nixpkgs#code2prompt
Generate a prompt from a codebase directory:
code2prompt path/to/codebase
Use a custom Handlebars template file:
code2prompt path/to/codebase --template path/to/template.hbs
Filter files using glob patterns:
code2prompt path/to/codebase --include="*.rs,*.toml"
Exclude files using glob patterns:
code2prompt path/to/codebase --exclude="*.txt,*.md"
Display the token count of the generated prompt:
code2prompt path/to/codebase --tokens
Specify a tokenizer for token count:
code2prompt path/to/codebase --tokens --encoding=p50k
Supported tokenizers: cl100k
, p50k
, p50k_edit
, r50k_bas
.
[!NOTE]
See Tokenizers for more details.
Save the generated prompt to an output file:
code2prompt path/to/codebase --output-file=output.txt
Print output as JSON:
code2prompt path/to/codebase -O json
The JSON output will have the following structure:
{
"prompt": "<Generated Prompt>",
"directory_name": "codebase",
"token_count": 1234,
"model_info": "ChatGPT models, text-embedding-ada-002",
"files": []
}
Generate a Git commit message (for staged files):
code2prompt path/to/codebase --diff --template templates/write-git-commit.hbs
Generate a Pull Request with branch comparing (for staged files):
code2prompt path/to/codebase --git-diff-branch 'main, development' --git-log-branch 'main, development' --template templates/write-github-pull-request.hbs
Add line numbers to source code blocks:
code2prompt path/to/codebase --line-number
Disable wrapping code inside markdown code blocks:
code2prompt path/to/codebase --no-codeblock
Include hidden files and directories:
code2prompt path/to/codebase --hidden
Skip .gitignore rules:
code2prompt path/to/codebase --no-ignore
code2prompt
comes with a set of built-in templates for common use cases. You can find them in the templates
directory.
Use this template to generate prompts for documenting the code. It will add documentation comments to all public functions, methods, classes and modules in the codebase.
Use this template to generate prompts for finding potential security vulnerabilities in the codebase. It will look for common security issues and provide recommendations on how to fix or mitigate them.
Use this template to generate prompts for cleaning up and improving the code quality. It will look for opportunities to improve readability, adherence to best practices, efficiency, error handling, and more.
Use this template to generate prompts for fixing bugs in the codebase. It will help diagnose issues, provide fix suggestions, and update the code with proposed fixes.
Use this template to create GitHub pull request description in markdown by comparing the git diff and git log of two branches.
Use this template to generate a high-quality README file for the project, suitable for hosting on GitHub. It will analyze the codebase to understand its purpose and functionality, and generate the README content in Markdown format.
Use this template to generate git commits from the staged files in your git directory. It will analyze the codebase to understand its purpose and functionality, and generate the git commit message content in Markdown format.
Use this template to generate prompts for improving the performance of the codebase. It will look for optimization opportunities, provide specific suggestions, and update the code with the changes.
You can use these templates by passing the -t
flag followed by the path to the template file. For example:
code2prompt path/to/codebase -t templates/document-the-code.hbs
code2prompt
supports the use of user defined variables in the Handlebars templates. Any variables in the template that are not part of the default context (absolute_code_path
, source_tree
, files
) will be treated as user defined variables.
During prompt generation, code2prompt
will prompt the user to enter values for these user defined variables. This allows for further customization of the generated prompts based on user input.
For example, if your template includes {{challenge_name}}
and {{challenge_description}}
, you will be prompted to enter values for these variables when running code2prompt
.
This feature enables creating reusable templates that can be adapted to different scenarios based on user provided information.
Tokenization is implemented using tiktoken-rs
. tiktoken
supports these encodings used by OpenAI models:
Encoding name | OpenAI models |
---|---|
cl100k_base |
ChatGPT models, text-embedding-ada-002
|
p50k_base |
Code models, text-davinci-002 , text-davinci-003
|
p50k_edit |
Use for edit models like text-davinci-edit-001 , code-davinci-edit-001
|
r50k_base (or gpt2 ) |
GPT-3 models like davinci
|
o200k_base |
GPT-4o models |
For more context on the different tokenizers, see the OpenAI Cookbook
code2prompt also provides Python bindings for seamless integration into Python applications. The Python SDK offers all the functionality of the CLI tool through an intuitive object-oriented interface.
See python-sdk/README.md for detailed documentation and usage examples.
Example usage:
from code2prompt import CodePrompt
prompt = CodePrompt("./my_project", include_patterns=["*.py"])
result = prompt.generate(encoding="cl100k")
print(result["prompt"])
Licensed under the MIT License, see LICENSE for more information.
If you liked the project and found it useful, please give it a â !
Ways to contribute:
- Suggest a feature
- Report a bug
- Fix something and open a pull request
- Help me document the code
- Spread the word
For Tasks:
Click tags to check more tools for each tasksFor Jobs:
Alternative AI tools for code2prompt
Similar Open Source Tools
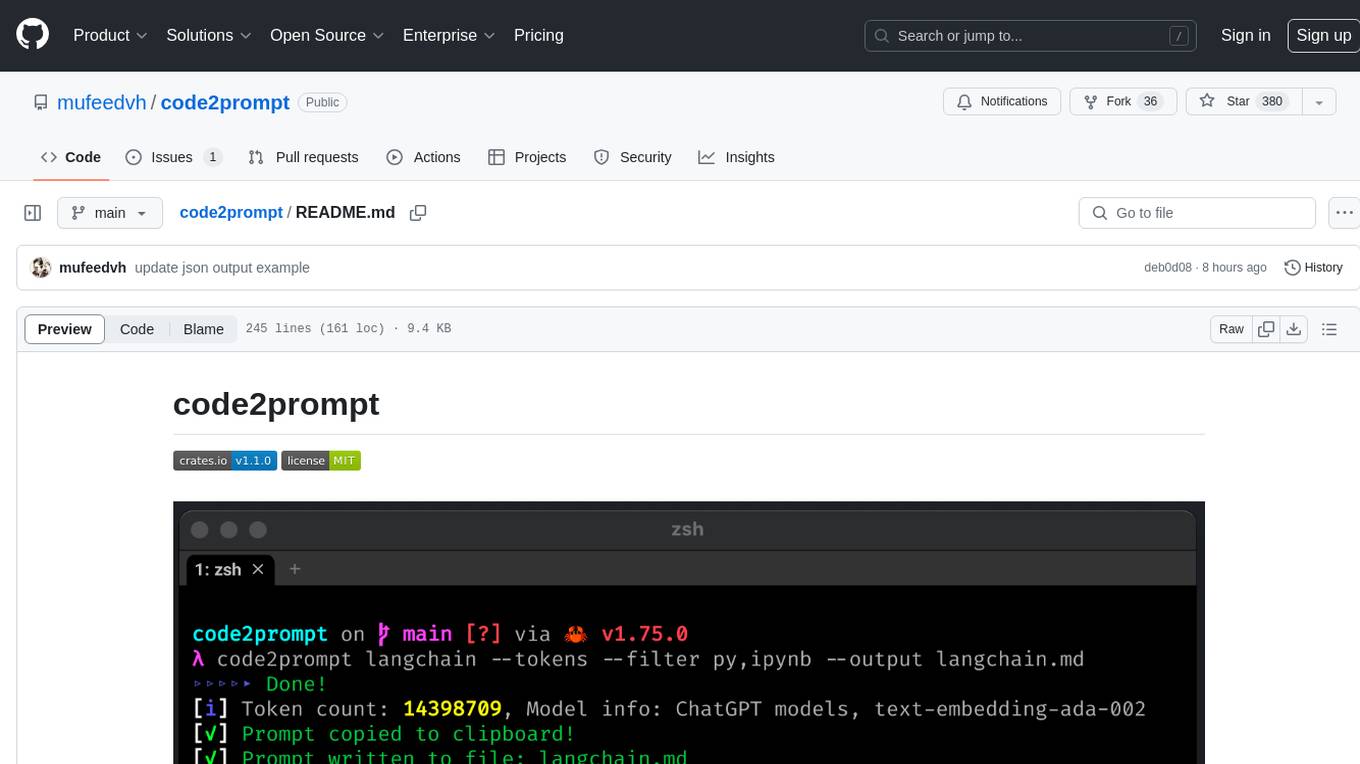
code2prompt
code2prompt is a command-line tool that converts your codebase into a single LLM prompt with a source tree, prompt templating, and token counting. It automates generating LLM prompts from codebases of any size, customizing prompt generation with Handlebars templates, respecting .gitignore, filtering and excluding files using glob patterns, displaying token count, including Git diff output, copying prompt to clipboard, saving prompt to an output file, excluding files and folders, adding line numbers to source code blocks, and more. It helps streamline the process of creating LLM prompts for code analysis, generation, and other tasks.
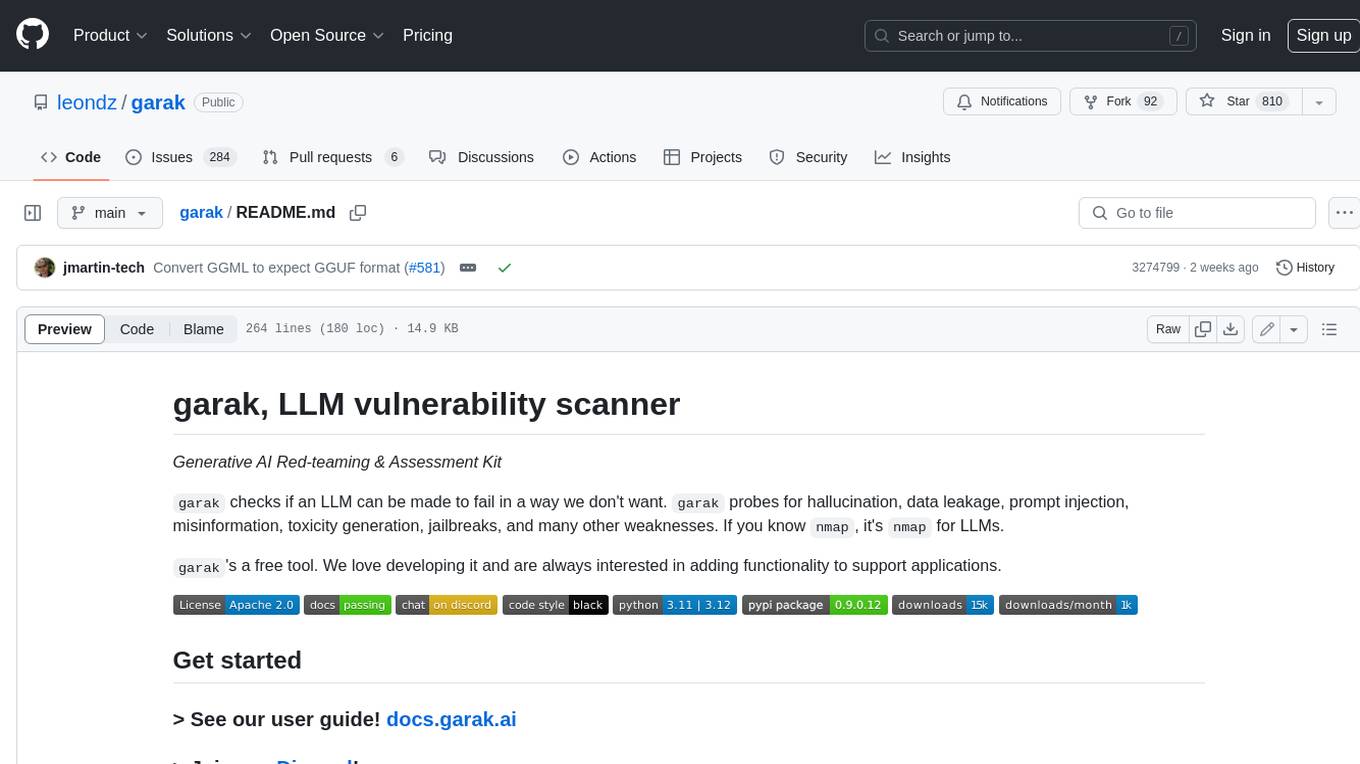
garak
Garak is a free tool that checks if a Large Language Model (LLM) can be made to fail in a way that is undesirable. It probes for hallucination, data leakage, prompt injection, misinformation, toxicity generation, jailbreaks, and many other weaknesses. Garak's a free tool. We love developing it and are always interested in adding functionality to support applications.
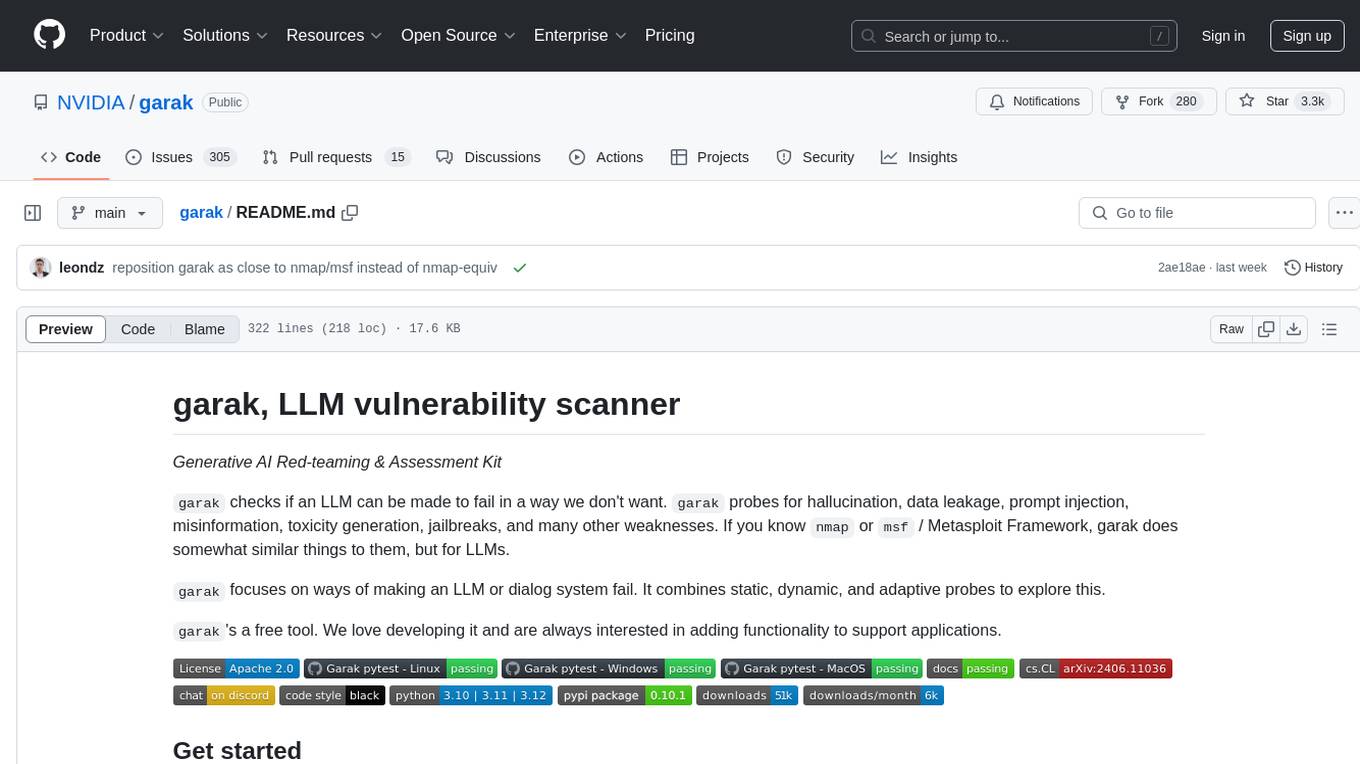
garak
Garak is a vulnerability scanner designed for LLMs (Large Language Models) that checks for various weaknesses such as hallucination, data leakage, prompt injection, misinformation, toxicity generation, and jailbreaks. It combines static, dynamic, and adaptive probes to explore vulnerabilities in LLMs. Garak is a free tool developed for red-teaming and assessment purposes, focusing on making LLMs or dialog systems fail. It supports various LLM models and can be used to assess their security and robustness.
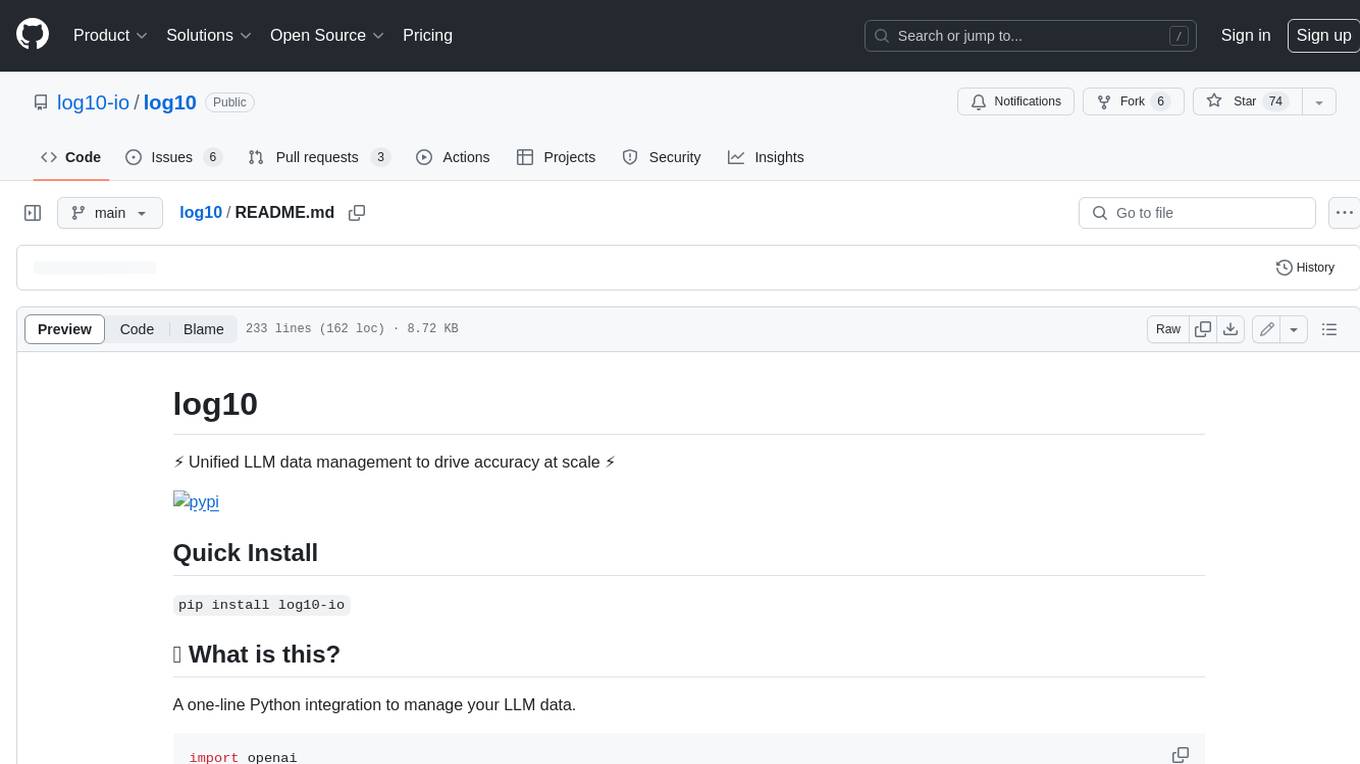
log10
Log10 is a one-line Python integration to manage your LLM data. It helps you log both closed and open-source LLM calls, compare and identify the best models and prompts, store feedback for fine-tuning, collect performance metrics such as latency and usage, and perform analytics and monitor compliance for LLM powered applications. Log10 offers various integration methods, including a python LLM library wrapper, the Log10 LLM abstraction, and callbacks, to facilitate its use in both existing production environments and new projects. Pick the one that works best for you. Log10 also provides a copilot that can help you with suggestions on how to optimize your prompt, and a feedback feature that allows you to add feedback to your completions. Additionally, Log10 provides prompt provenance, session tracking and call stack functionality to help debug prompt chains. With Log10, you can use your data and feedback from users to fine-tune custom models with RLHF, and build and deploy more reliable, accurate and efficient self-hosted models. Log10 also supports collaboration, allowing you to create flexible groups to share and collaborate over all of the above features.
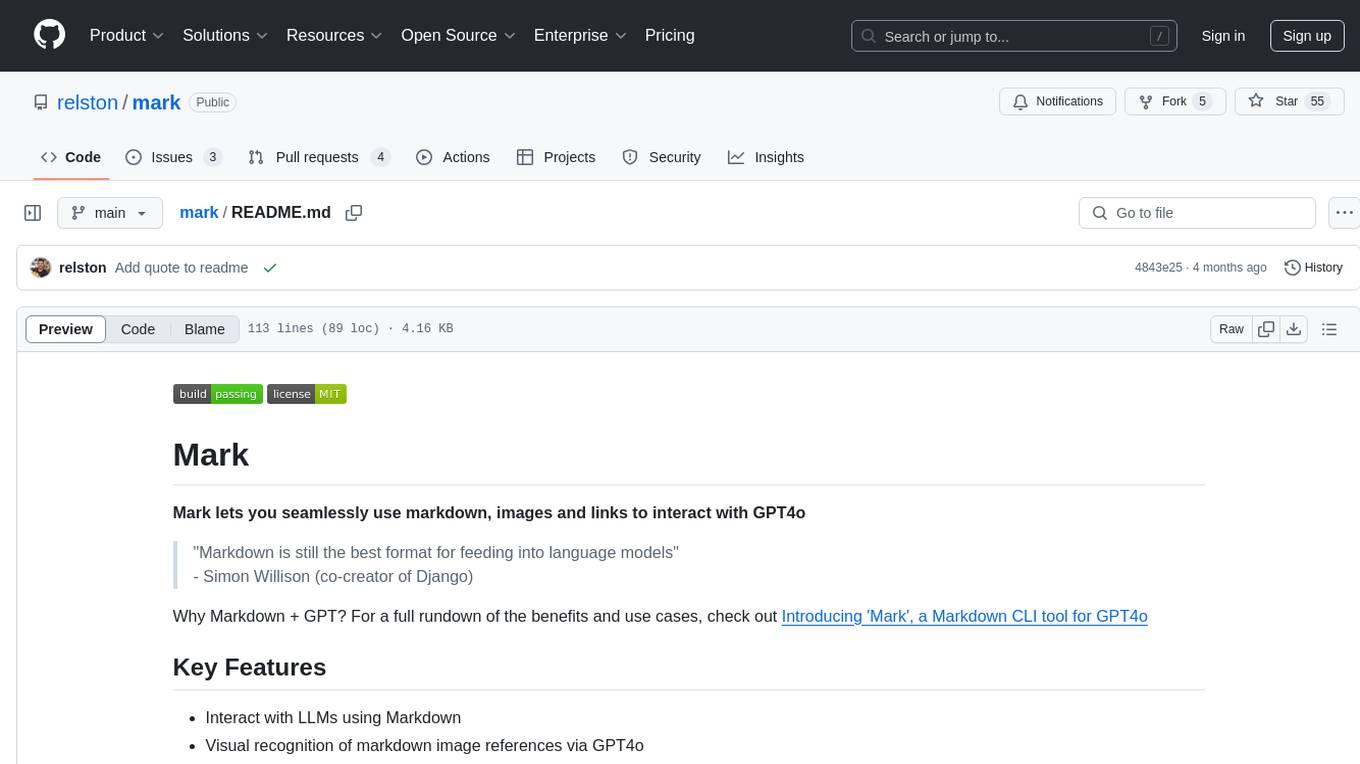
mark
Mark is a CLI tool that allows users to interact with large language models (LLMs) using Markdown format. It enables users to seamlessly integrate GPT responses into Markdown files, supports image recognition, scraping of local and remote links, and image generation. Mark focuses on using Markdown as both a prompt and response medium for LLMs, offering a unique and flexible way to interact with language models for various use cases in development and documentation processes.
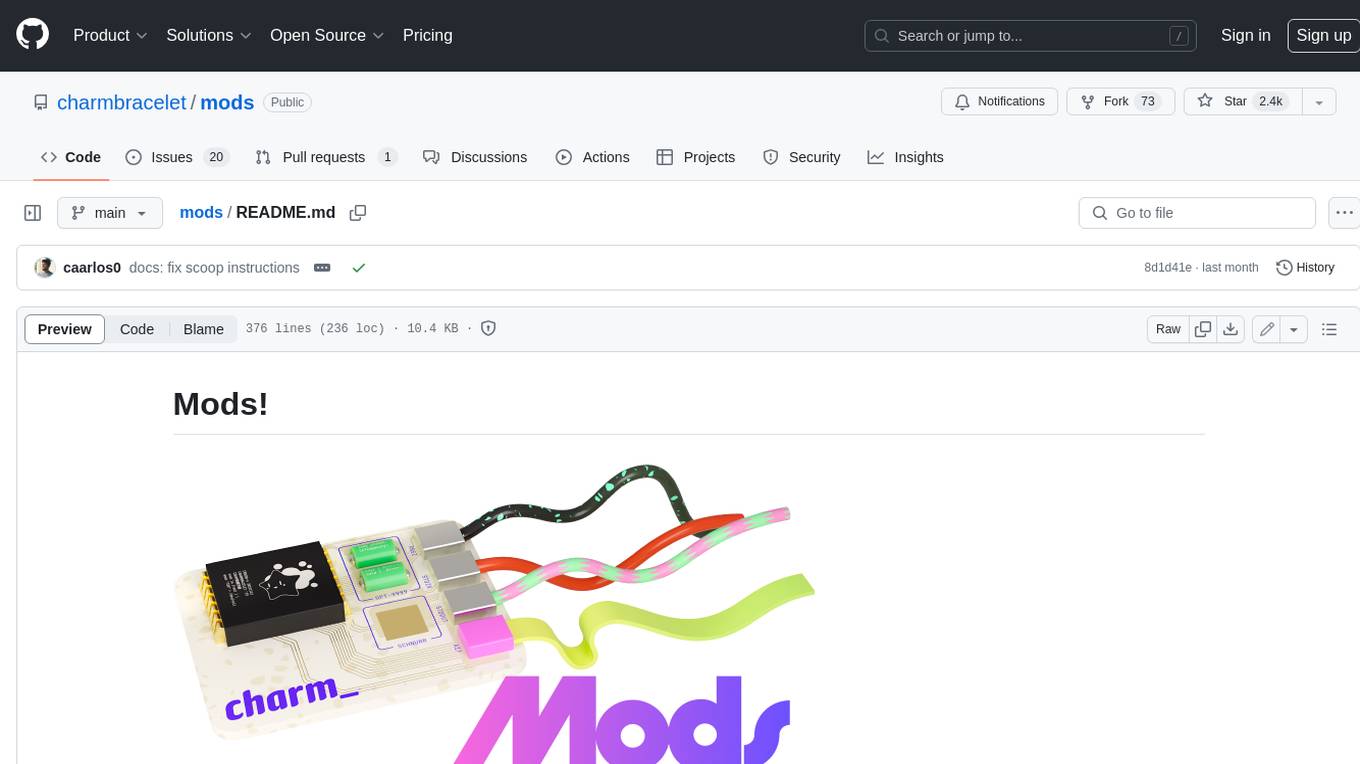
mods
AI for the command line, built for pipelines. LLM based AI is really good at interpreting the output of commands and returning the results in CLI friendly text formats like Markdown. Mods is a simple tool that makes it super easy to use AI on the command line and in your pipelines. Mods works with OpenAI, Groq, Azure OpenAI, and LocalAI To get started, install Mods and check out some of the examples below. Since Mods has built-in Markdown formatting, you may also want to grab Glow to give the output some _pizzazz_.
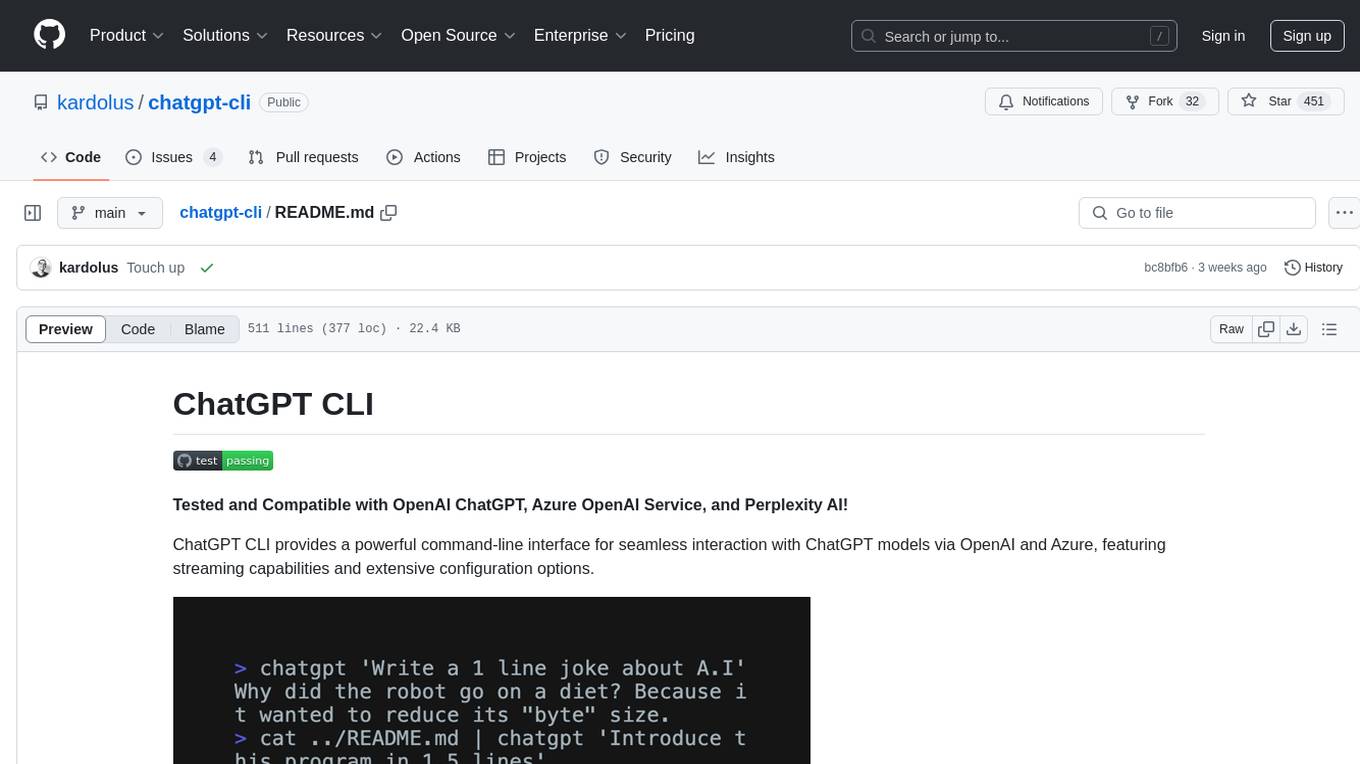
chatgpt-cli
ChatGPT CLI provides a powerful command-line interface for seamless interaction with ChatGPT models via OpenAI and Azure. It features streaming capabilities, extensive configuration options, and supports various modes like streaming, query, and interactive mode. Users can manage thread-based context, sliding window history, and provide custom context from any source. The CLI also offers model and thread listing, advanced configuration options, and supports GPT-4, GPT-3.5-turbo, and Perplexity's models. Installation is available via Homebrew or direct download, and users can configure settings through default values, a config.yaml file, or environment variables.
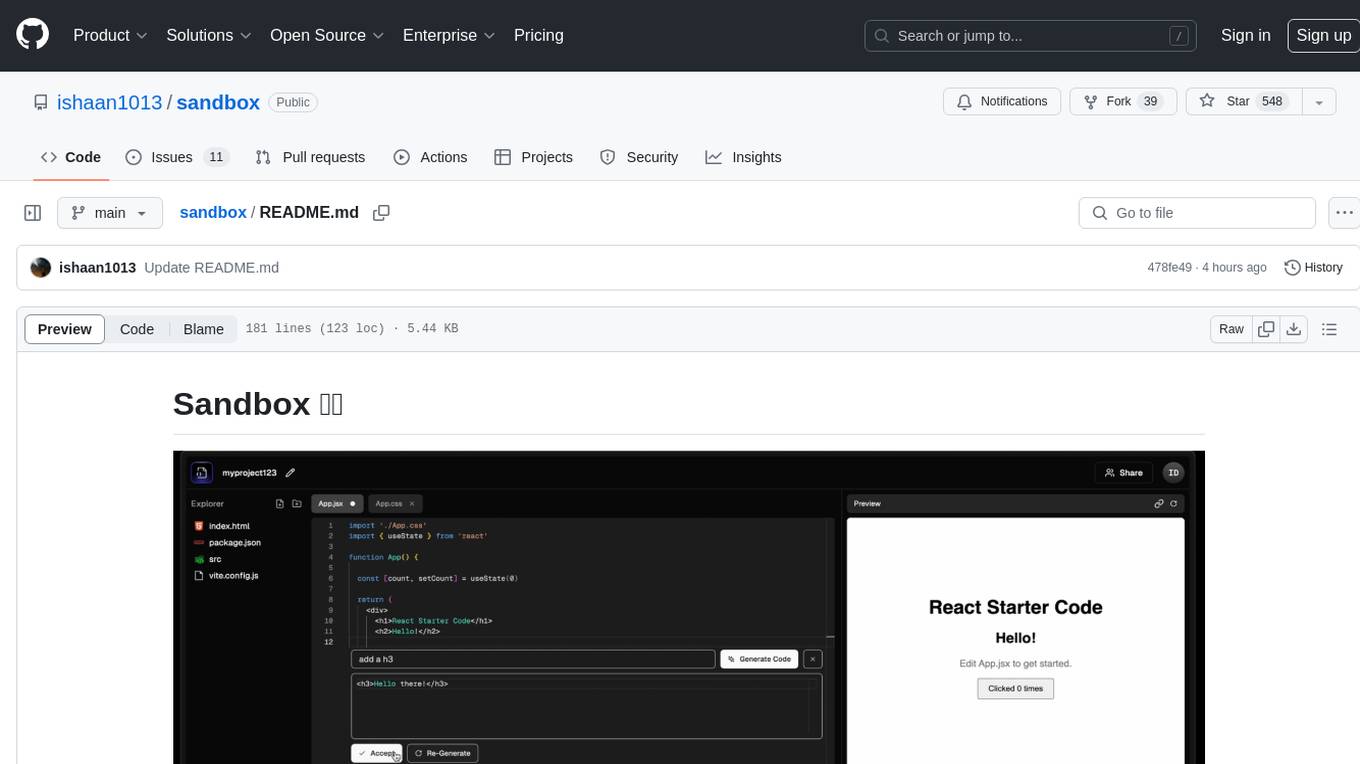
sandbox
Sandbox is an open-source cloud-based code editing environment with custom AI code autocompletion and real-time collaboration. It consists of a frontend built with Next.js, TailwindCSS, Shadcn UI, Clerk, Monaco, and Liveblocks, and a backend with Express, Socket.io, Cloudflare Workers, D1 database, R2 storage, Workers AI, and Drizzle ORM. The backend includes microservices for database, storage, and AI functionalities. Users can run the project locally by setting up environment variables and deploying the containers. Contributions are welcome following the commit convention and structure provided in the repository.
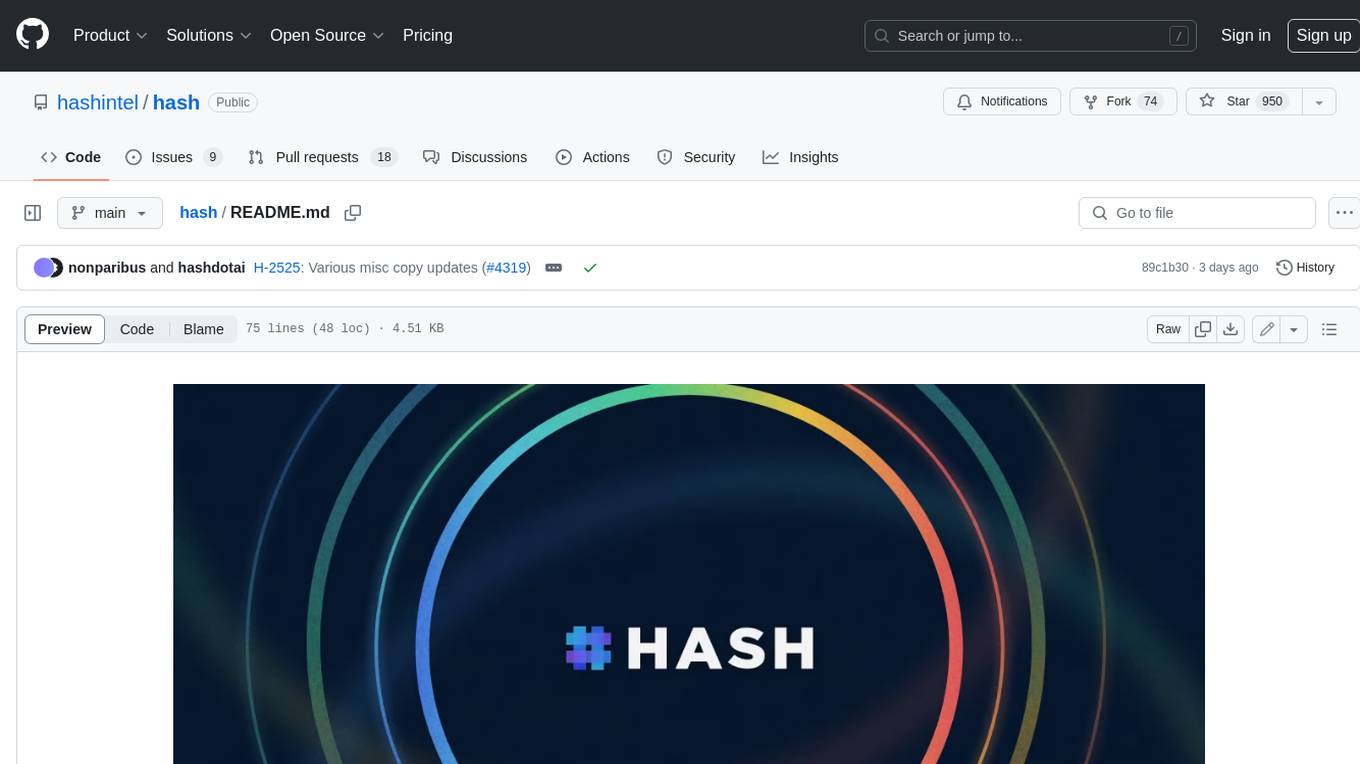
hash
HASH is a self-building, open-source database which grows, structures and checks itself. With it, we're creating a platform for decision-making, which helps you integrate, understand and use data in a variety of different ways.
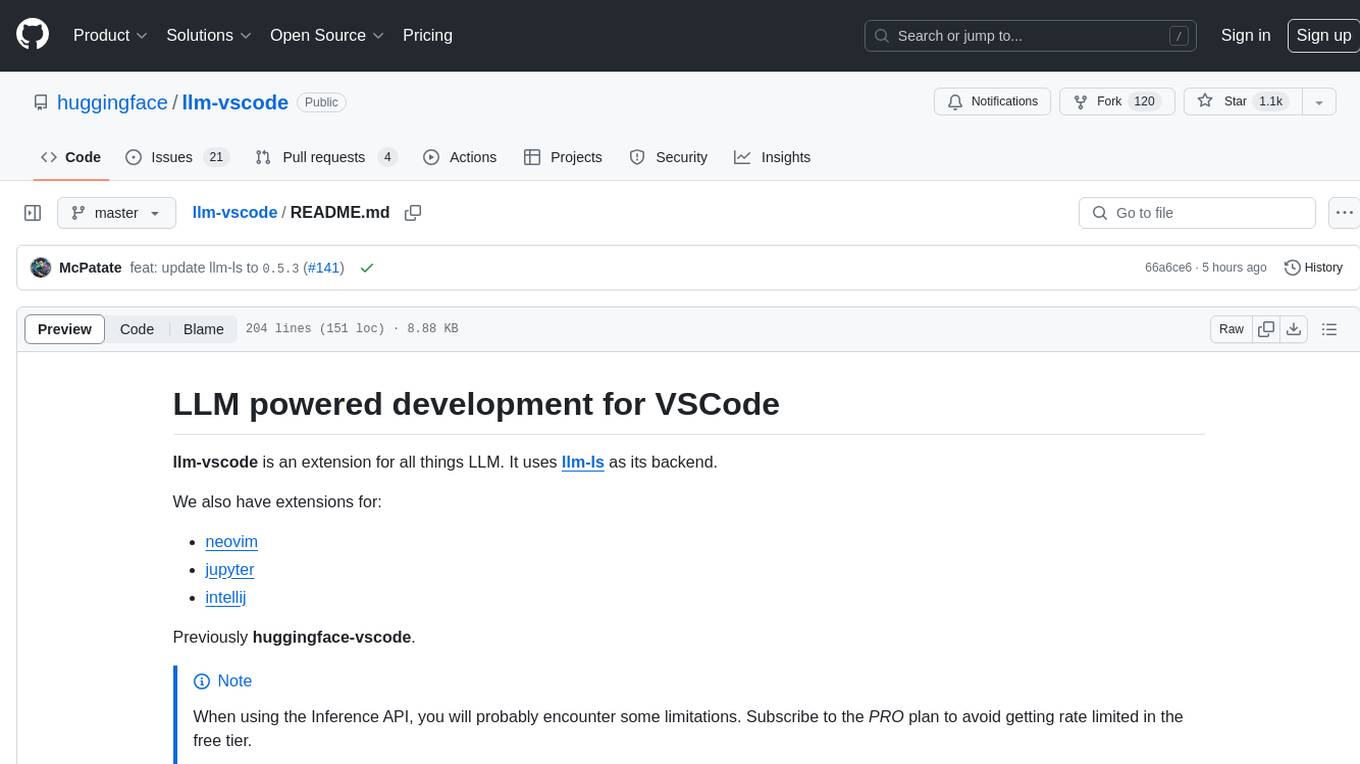
llm-vscode
llm-vscode is an extension designed for all things LLM, utilizing llm-ls as its backend. It offers features such as code completion with 'ghost-text' suggestions, the ability to choose models for code generation via HTTP requests, ensuring prompt size fits within the context window, and code attribution checks. Users can configure the backend, suggestion behavior, keybindings, llm-ls settings, and tokenization options. Additionally, the extension supports testing models like Code Llama 13B, Phind/Phind-CodeLlama-34B-v2, and WizardLM/WizardCoder-Python-34B-V1.0. Development involves cloning llm-ls, building it, and setting up the llm-vscode extension for use.
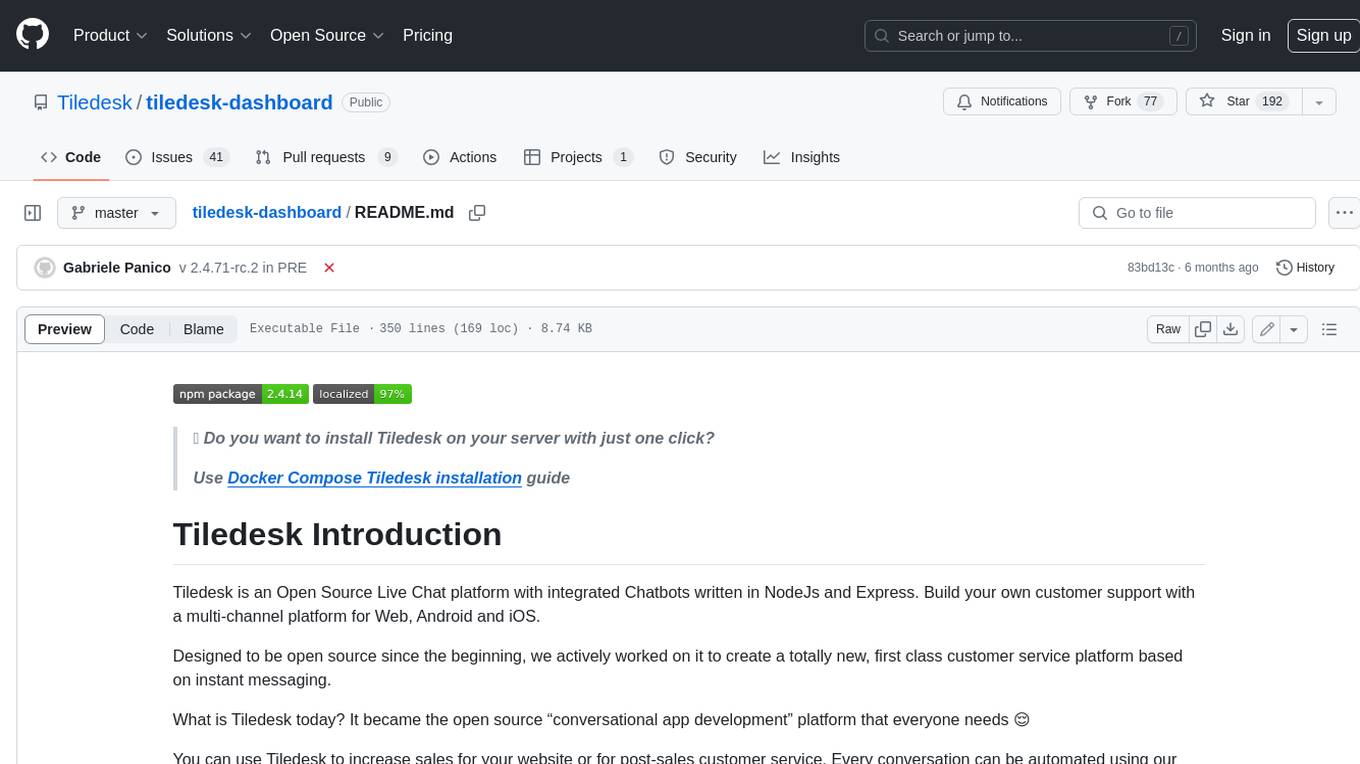
tiledesk-dashboard
Tiledesk is an open-source live chat platform with integrated chatbots written in Node.js and Express. It is designed to be a multi-channel platform for web, Android, and iOS, and it can be used to increase sales or provide post-sales customer service. Tiledesk's chatbot technology allows for automation of conversations, and it also provides APIs and webhooks for connecting external applications. Additionally, it offers a marketplace for apps and features such as CRM, ticketing, and data export.
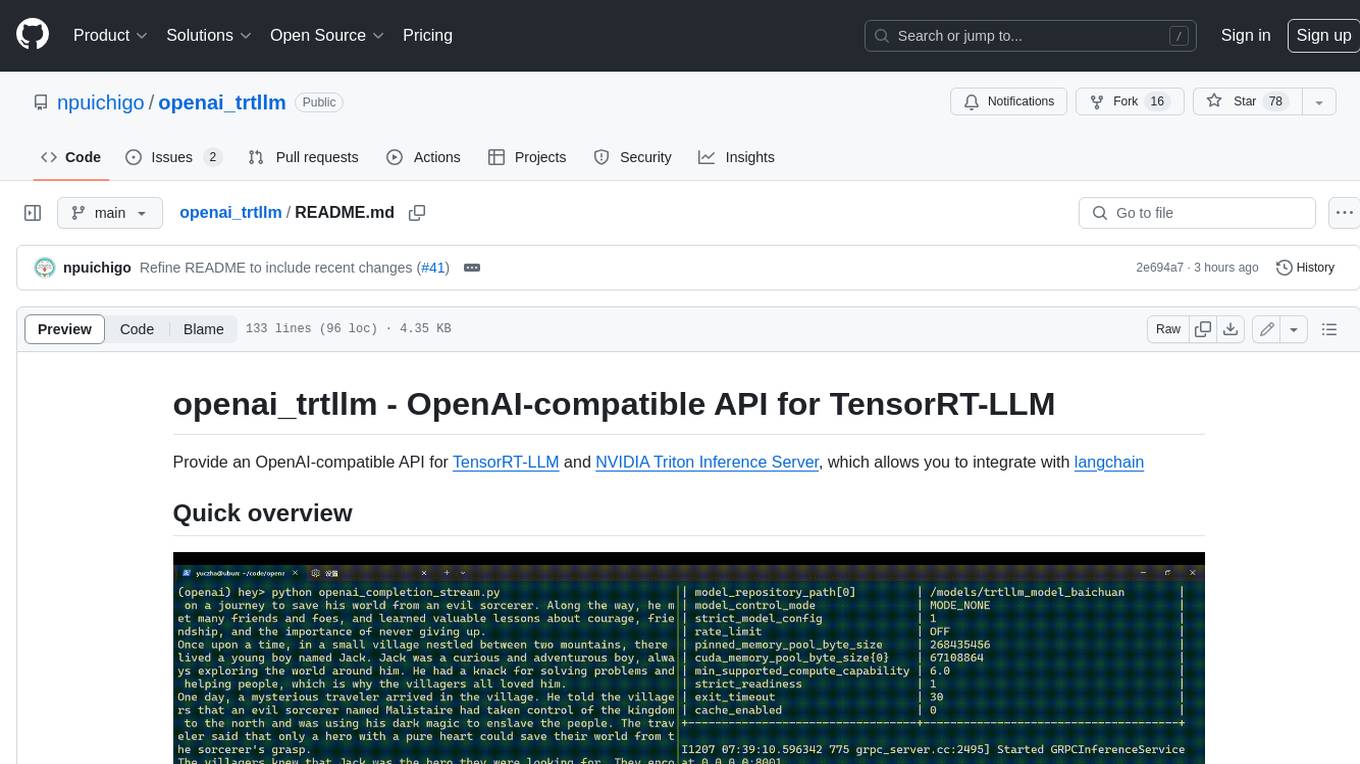
openai_trtllm
OpenAI-compatible API for TensorRT-LLM and NVIDIA Triton Inference Server, which allows you to integrate with langchain
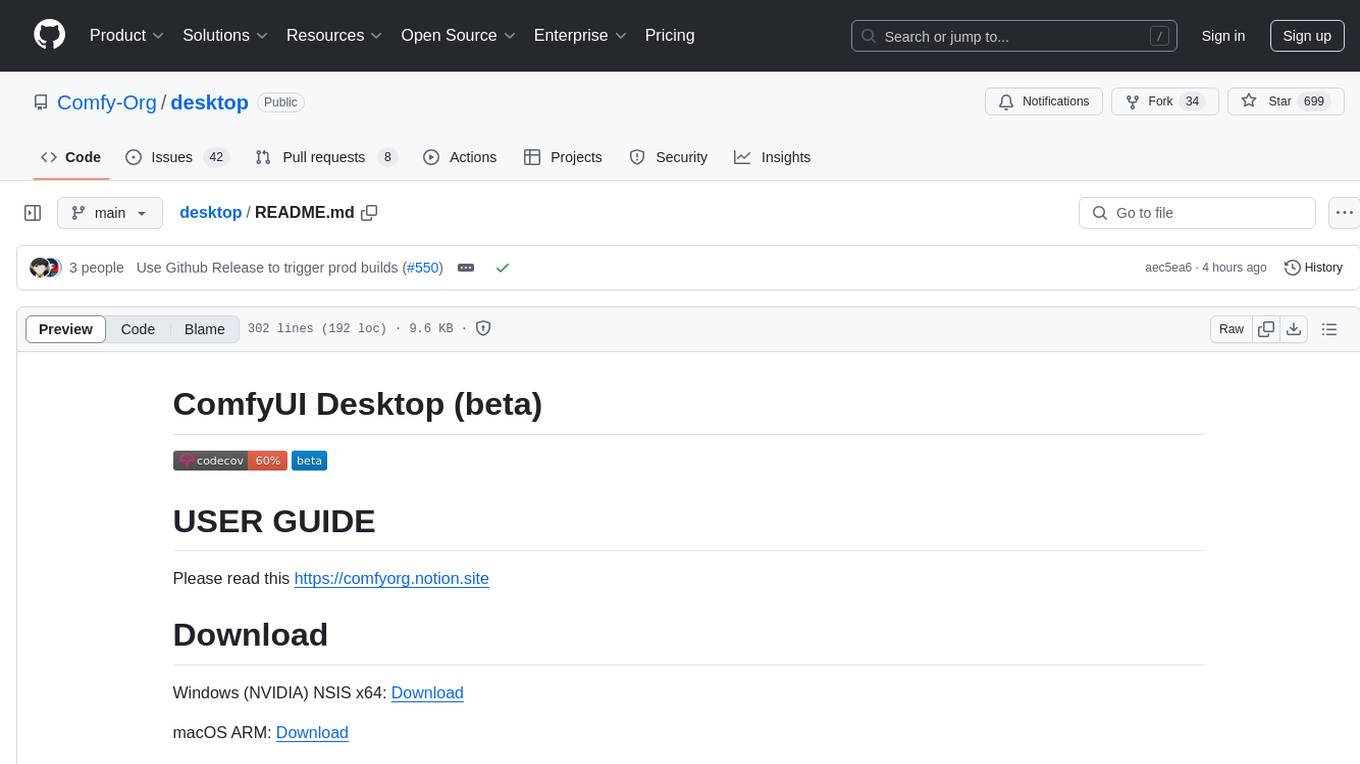
desktop
ComfyUI Desktop is a packaged desktop application that allows users to easily use ComfyUI with bundled features like ComfyUI source code, ComfyUI-Manager, and uv. It automatically installs necessary Python dependencies and updates with stable releases. The app comes with Electron, Chromium binaries, and node modules. Users can store ComfyUI files in a specified location and manage model paths. The tool requires Python 3.12+ and Visual Studio with Desktop C++ workload for Windows. It uses nvm to manage node versions and yarn as the package manager. Users can install ComfyUI and dependencies using comfy-cli, download uv, and build/launch the code. Troubleshooting steps include rebuilding modules and installing missing libraries. The tool supports debugging in VSCode and provides utility scripts for cleanup. Crash reports can be sent to help debug issues, but no personal data is included.
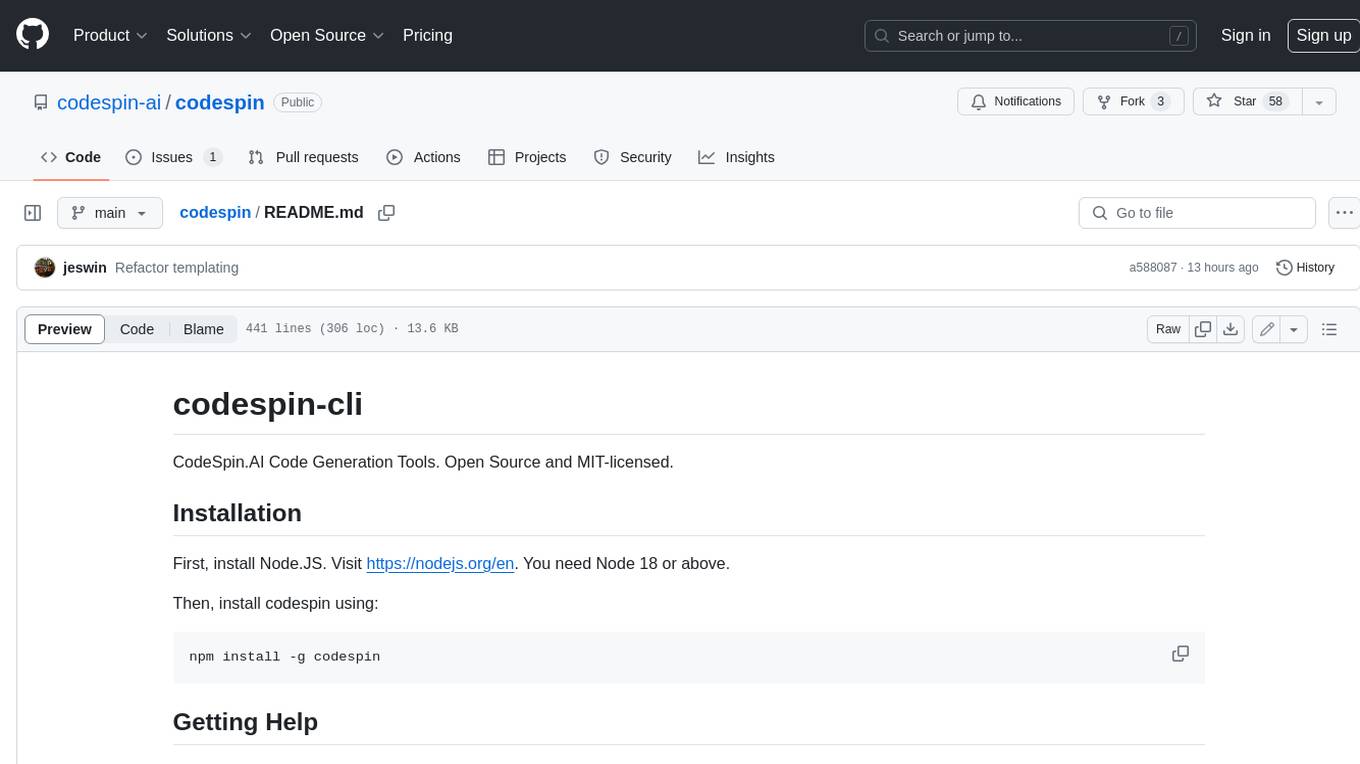
codespin
CodeSpin.AI is a set of open-source code generation tools that leverage large language models (LLMs) to automate coding tasks. With CodeSpin, you can generate code in various programming languages, including Python, JavaScript, Java, and C++, by providing natural language prompts. CodeSpin offers a range of features to enhance code generation, such as custom templates, inline prompting, and the ability to use ChatGPT as an alternative to API keys. Additionally, CodeSpin provides options for regenerating code, executing code in prompt files, and piping data into the LLM for processing. By utilizing CodeSpin, developers can save time and effort in coding tasks, improve code quality, and explore new possibilities in code generation.
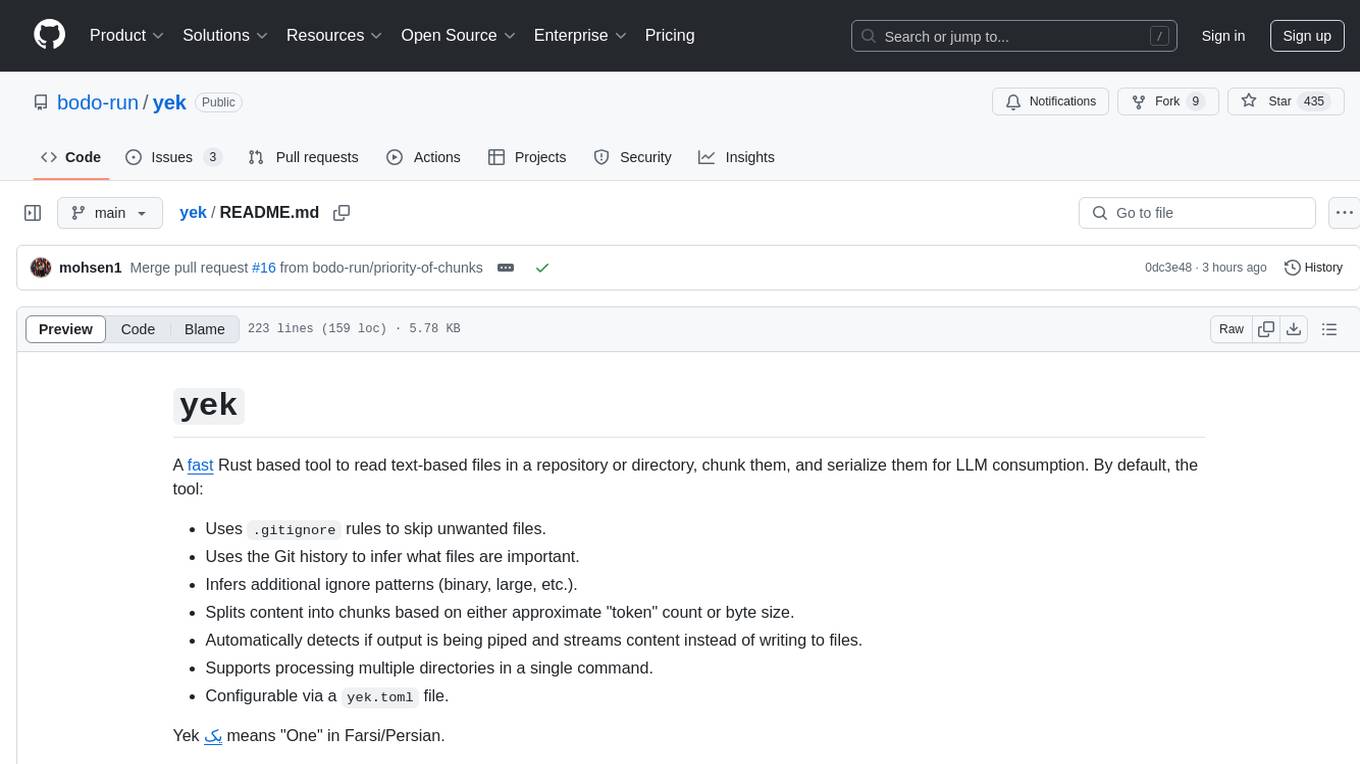
yek
Yek is a fast Rust-based tool designed to read text-based files in a repository or directory, chunk them, and serialize them for Large Language Models (LLM) consumption. It utilizes .gitignore rules to skip unwanted files, Git history to infer important files, and additional ignore patterns. Yek splits content into chunks based on token count or byte size, supports processing multiple directories, and can stream content when output is piped. It is configurable via a 'yek.toml' file and prioritizes important files at the end of the output.
For similar tasks
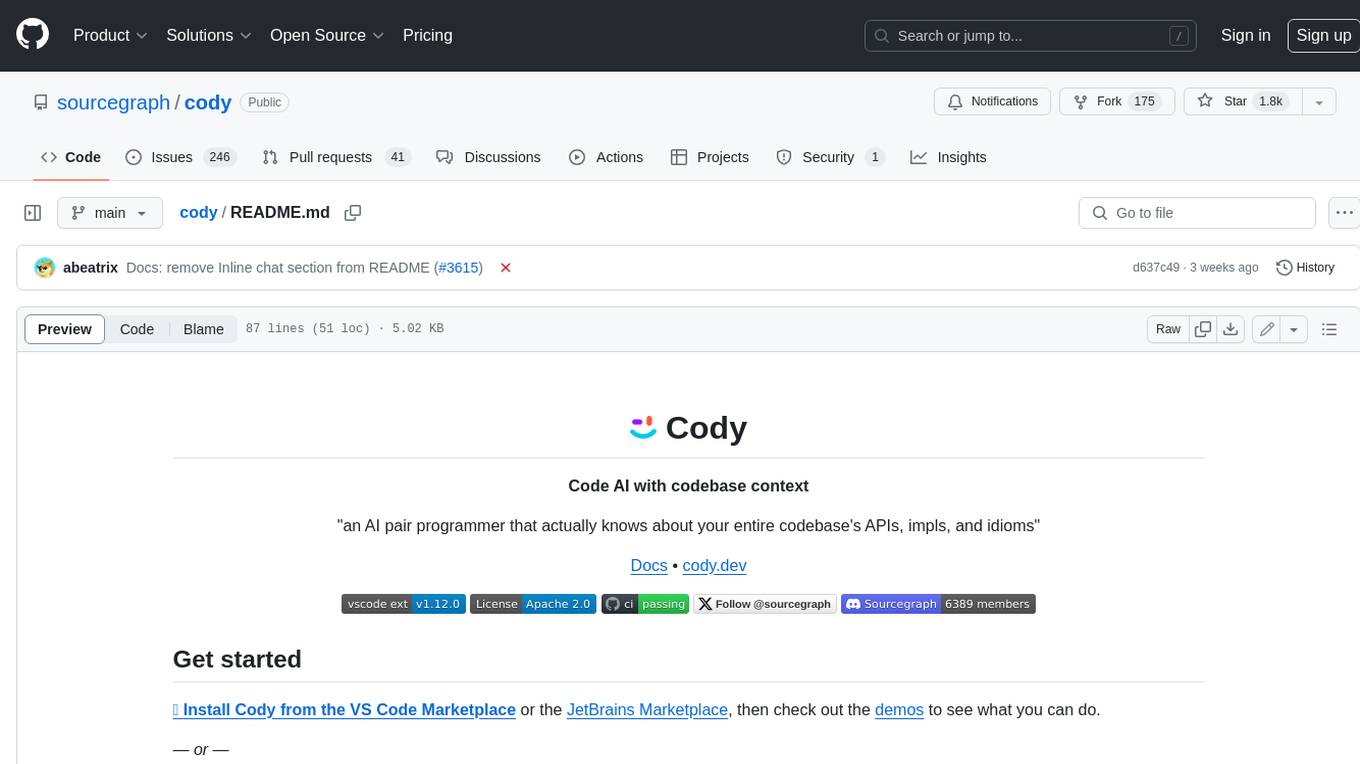
cody
Cody is a free, open-source AI coding assistant that can write and fix code, provide AI-generated autocomplete, and answer your coding questions. Cody fetches relevant code context from across your entire codebase to write better code that uses more of your codebase's APIs, impls, and idioms, with less hallucination.
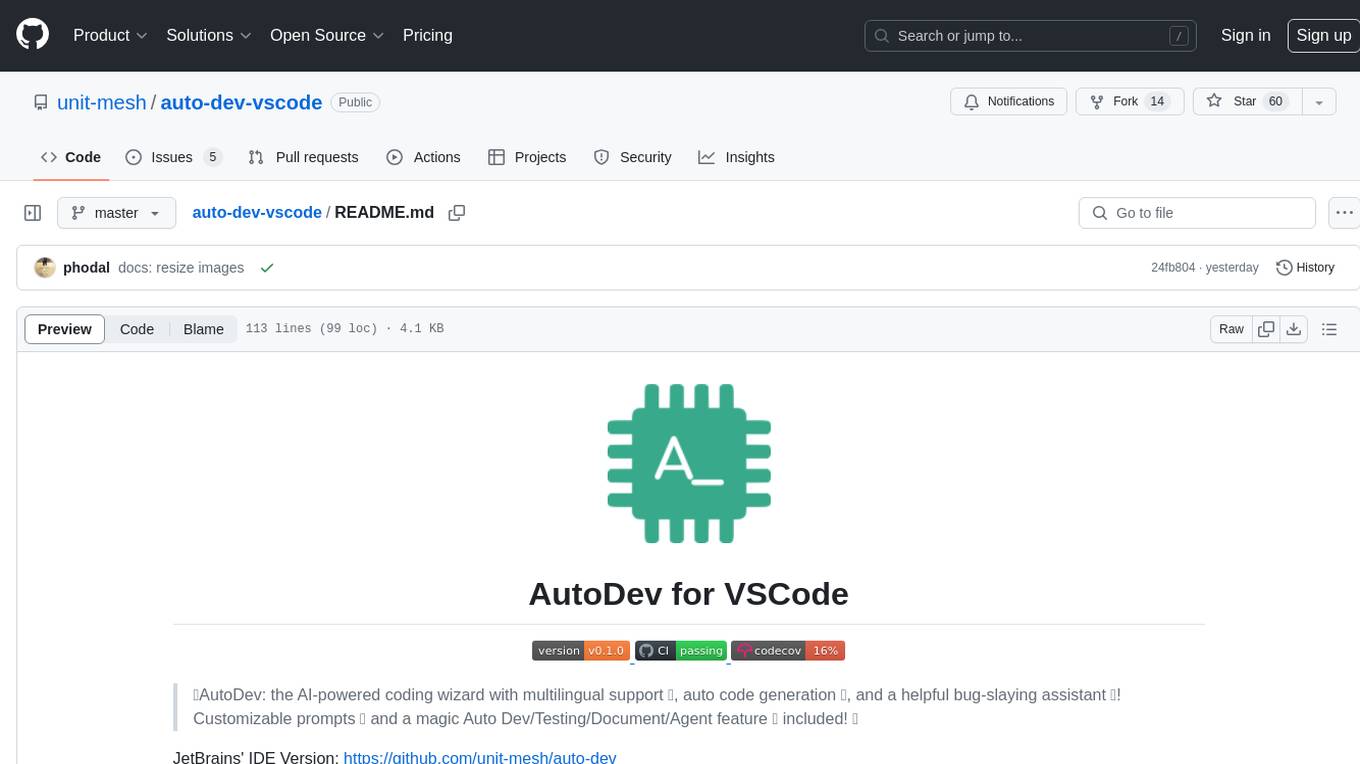
auto-dev-vscode
AutoDev for VSCode is an AI-powered coding wizard with multilingual support, auto code generation, and a bug-slaying assistant. It offers customizable prompts and features like Auto Dev/Testing/Document/Agent. The tool aims to enhance coding productivity and efficiency by providing intelligent assistance and automation capabilities within the Visual Studio Code environment.
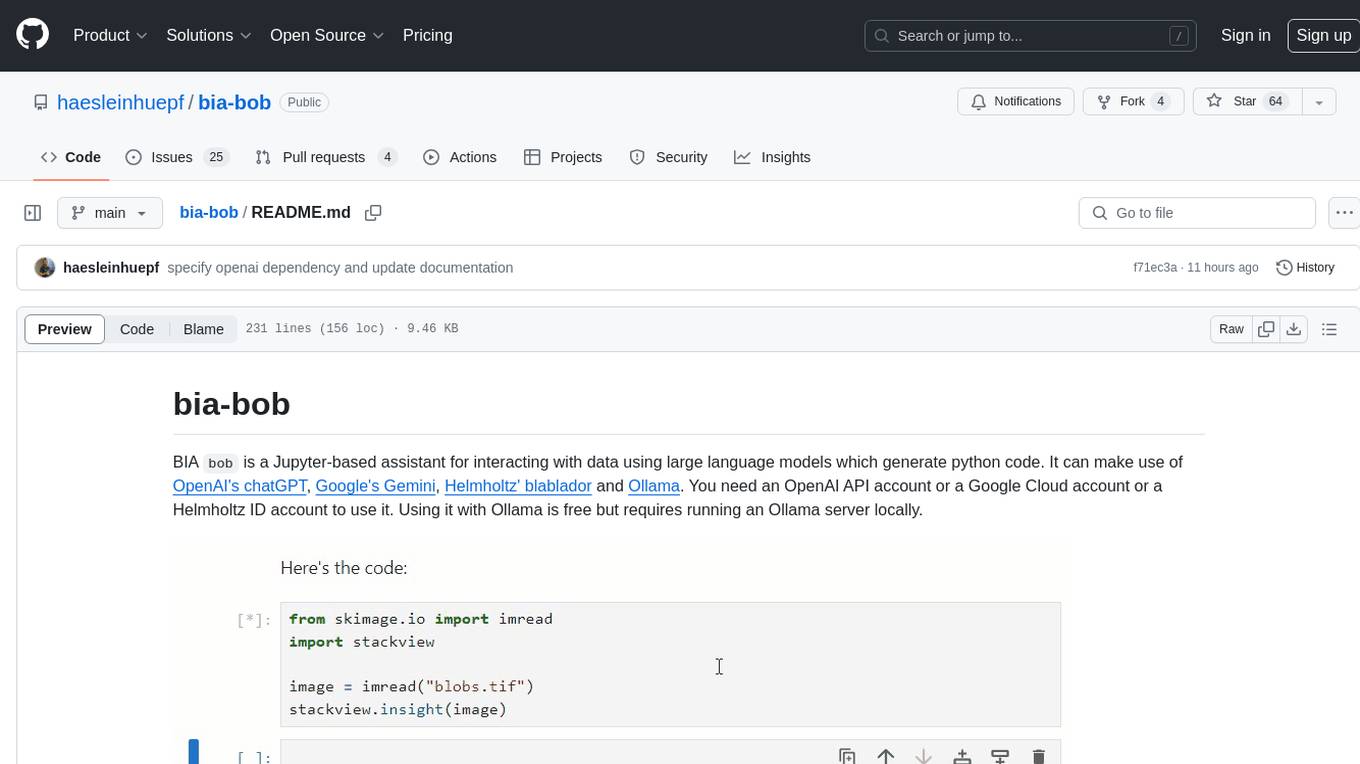
bia-bob
BIA `bob` is a Jupyter-based assistant for interacting with data using large language models to generate Python code. It can utilize OpenAI's chatGPT, Google's Gemini, Helmholtz' blablador, and Ollama. Users need respective accounts to access these services. Bob can assist in code generation, bug fixing, code documentation, GPU-acceleration, and offers a no-code custom Jupyter Kernel. It provides example notebooks for various tasks like bio-image analysis, model selection, and bug fixing. Installation is recommended via conda/mamba environment. Custom endpoints like blablador and ollama can be used. Google Cloud AI API integration is also supported. The tool is extensible for Python libraries to enhance Bob's functionality.
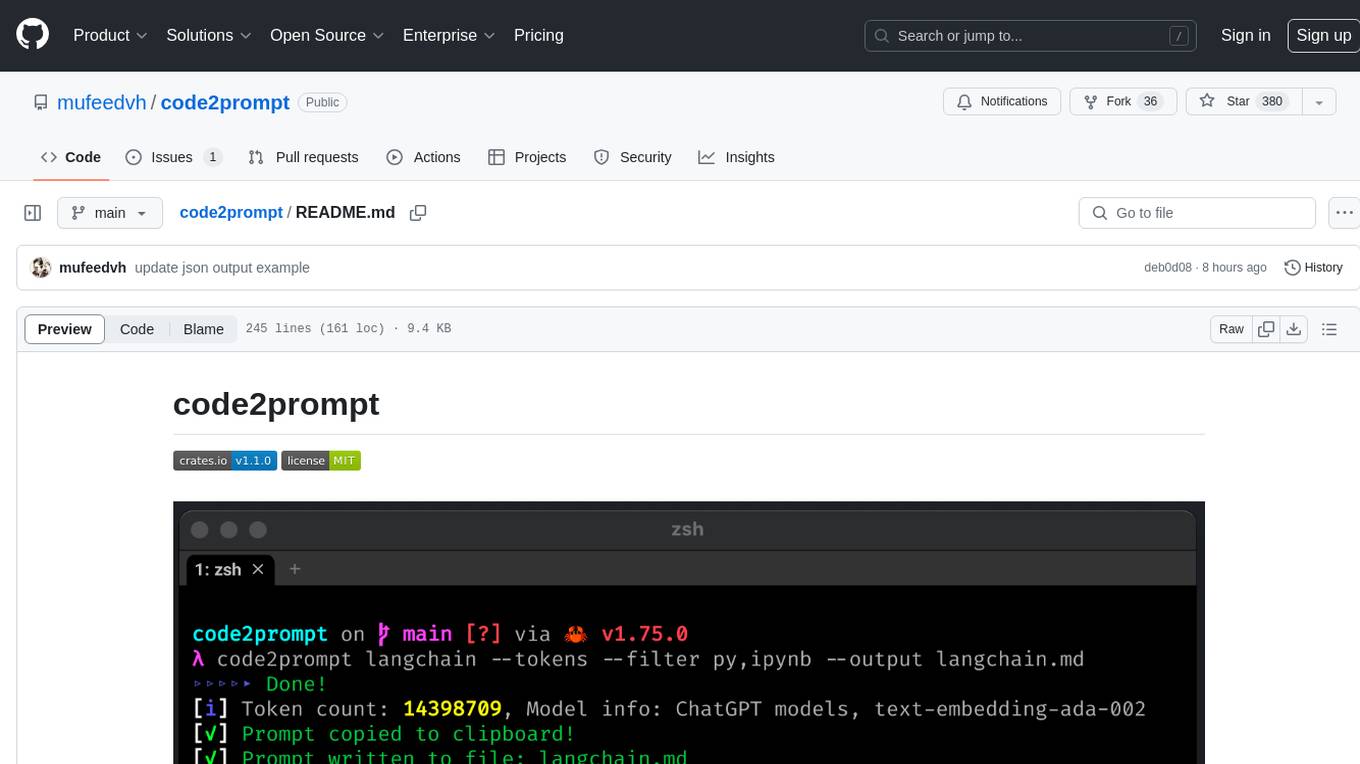
code2prompt
code2prompt is a command-line tool that converts your codebase into a single LLM prompt with a source tree, prompt templating, and token counting. It automates generating LLM prompts from codebases of any size, customizing prompt generation with Handlebars templates, respecting .gitignore, filtering and excluding files using glob patterns, displaying token count, including Git diff output, copying prompt to clipboard, saving prompt to an output file, excluding files and folders, adding line numbers to source code blocks, and more. It helps streamline the process of creating LLM prompts for code analysis, generation, and other tasks.
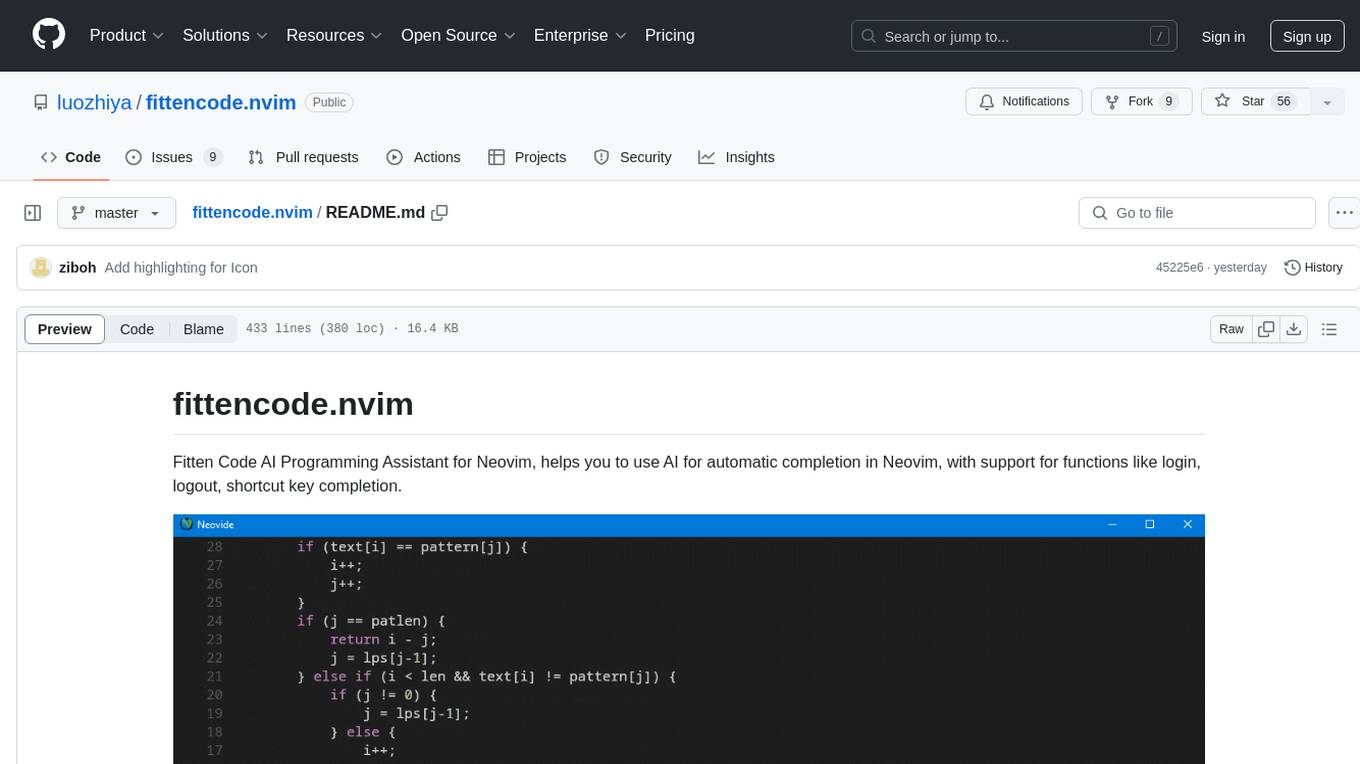
fittencode.nvim
Fitten Code AI Programming Assistant for Neovim provides fast completion using AI, asynchronous I/O, and support for various actions like document code, edit code, explain code, find bugs, generate unit test, implement features, optimize code, refactor code, start chat, and more. It offers features like accepting suggestions with Tab, accepting line with Ctrl + Down, accepting word with Ctrl + Right, undoing accepted text, automatic scrolling, and multiple HTTP/REST backends. It can run as a coc.nvim source or nvim-cmp source.
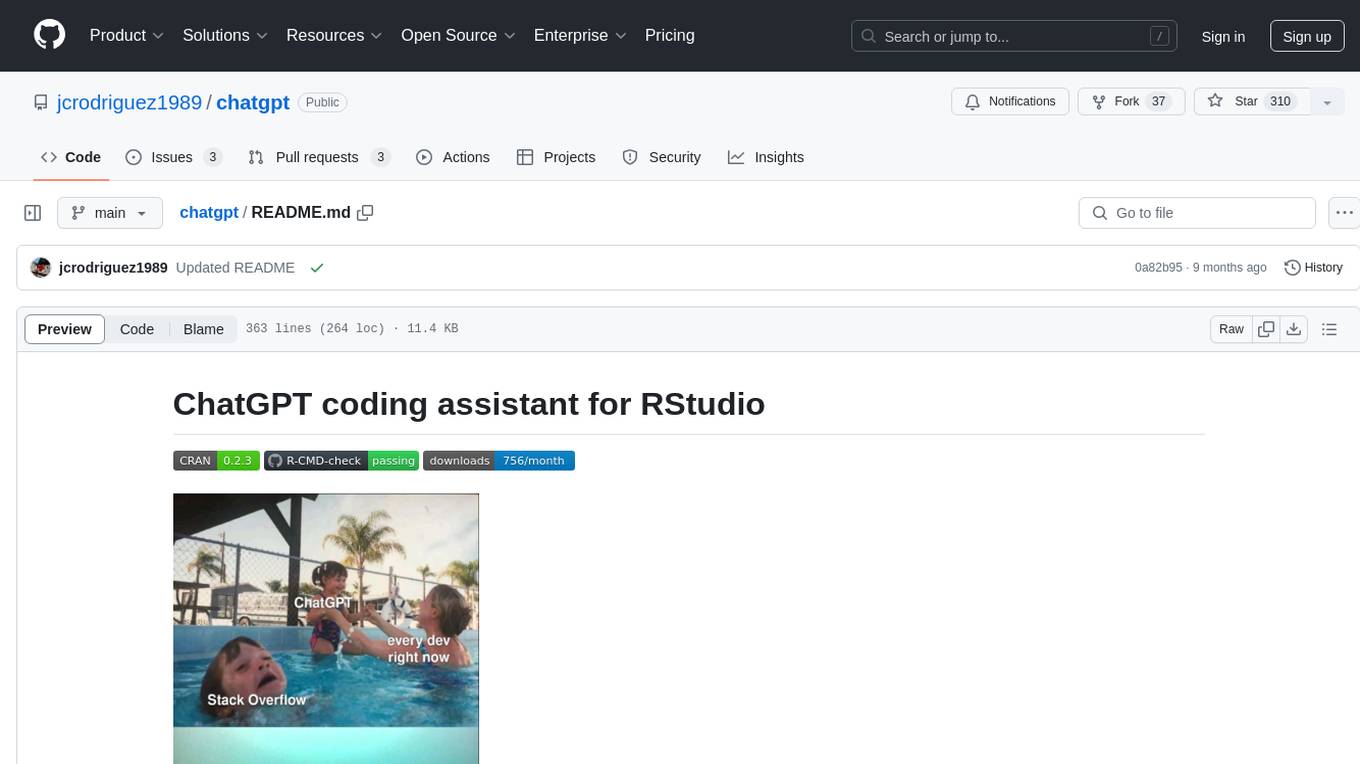
chatgpt
The ChatGPT R package provides a set of features to assist in R coding. It includes addins like Ask ChatGPT, Comment selected code, Complete selected code, Create unit tests, Create variable name, Document code, Explain selected code, Find issues in the selected code, Optimize selected code, and Refactor selected code. Users can interact with ChatGPT to get code suggestions, explanations, and optimizations. The package helps in improving coding efficiency and quality by providing AI-powered assistance within the RStudio environment.
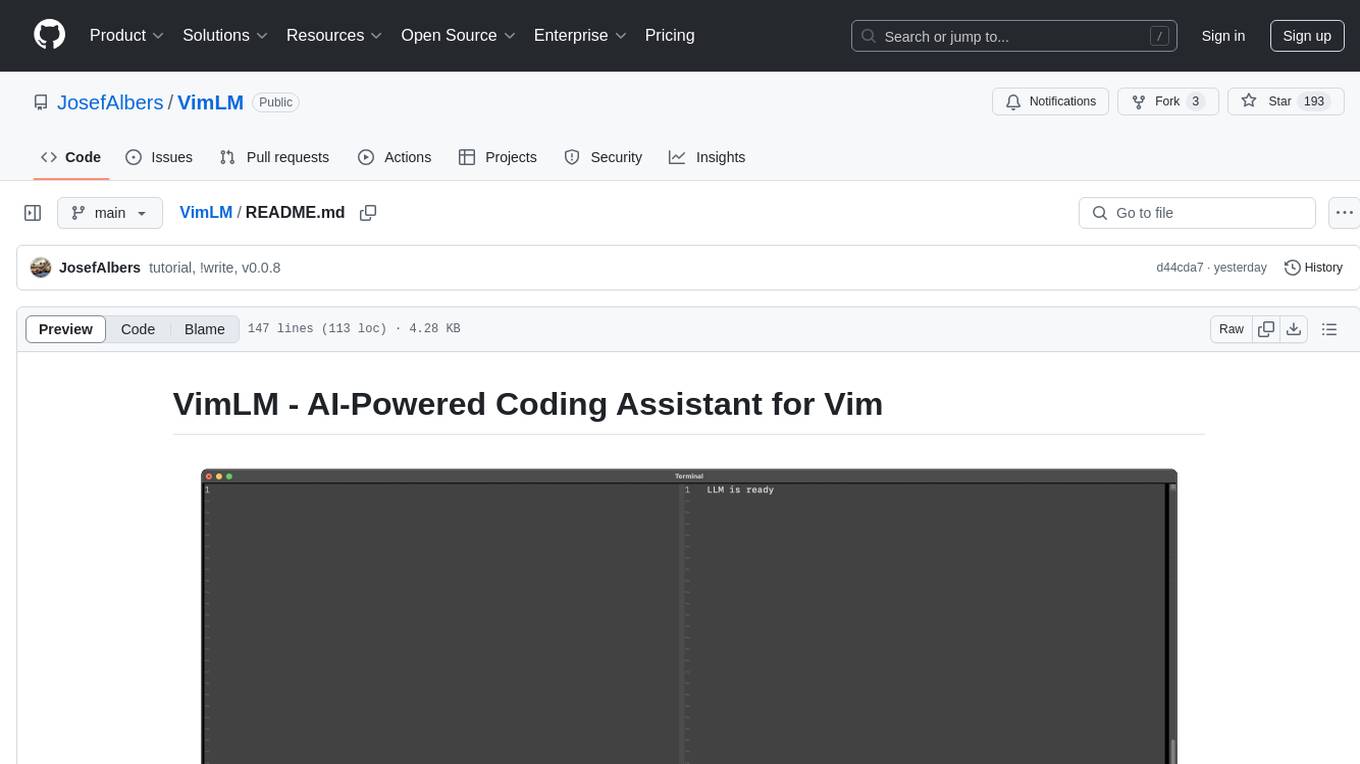
VimLM
VimLM is an AI-powered coding assistant for Vim that integrates AI for code generation, refactoring, and documentation directly into your Vim workflow. It offers native Vim integration with split-window responses and intuitive keybindings, offline first execution with MLX-compatible models, contextual awareness with seamless integration with codebase and external resources, conversational workflow for iterating on responses, project scaffolding for generating and deploying code blocks, and extensibility for creating custom LLM workflows with command chains.
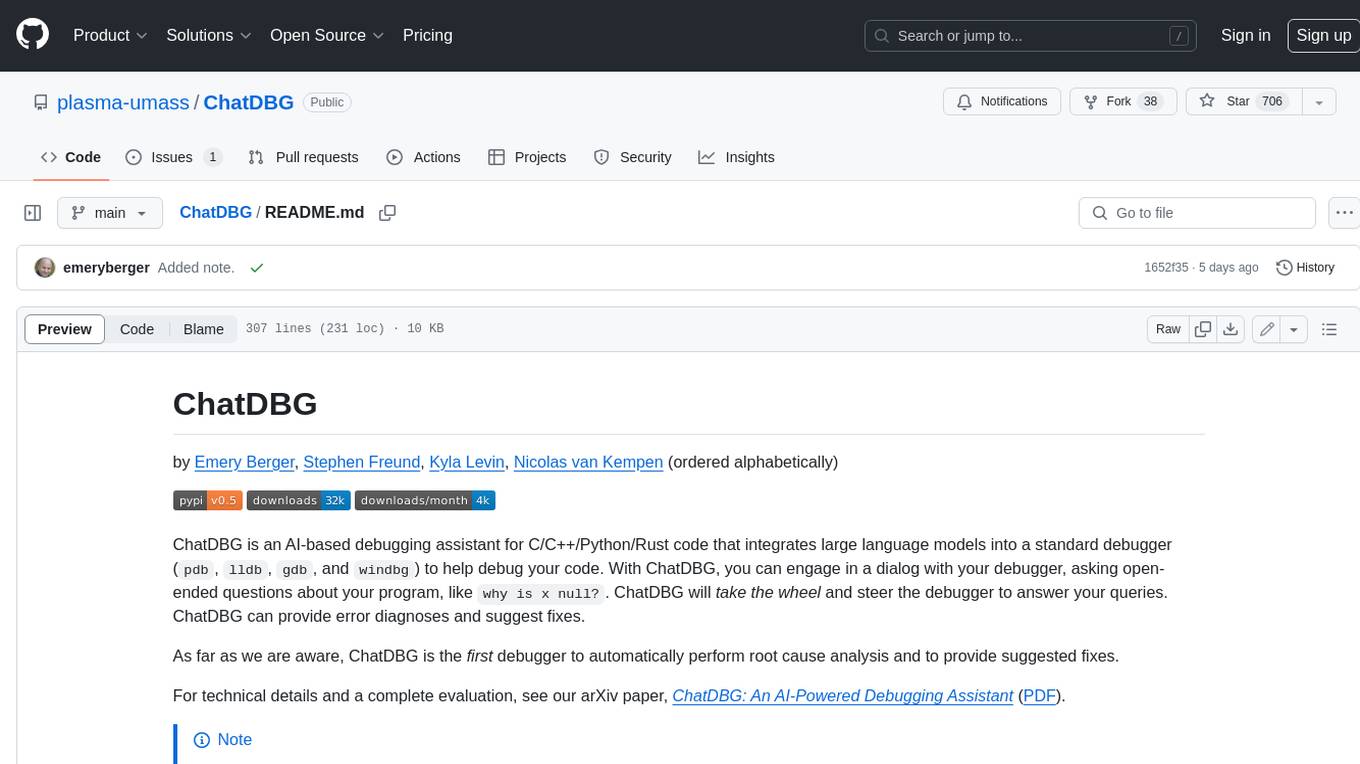
ChatDBG
ChatDBG is an AI-based debugging assistant for C/C++/Python/Rust code that integrates large language models into a standard debugger (`pdb`, `lldb`, `gdb`, and `windbg`) to help debug your code. With ChatDBG, you can engage in a dialog with your debugger, asking open-ended questions about your program, like `why is x null?`. ChatDBG will _take the wheel_ and steer the debugger to answer your queries. ChatDBG can provide error diagnoses and suggest fixes. As far as we are aware, ChatDBG is the _first_ debugger to automatically perform root cause analysis and to provide suggested fixes.
For similar jobs
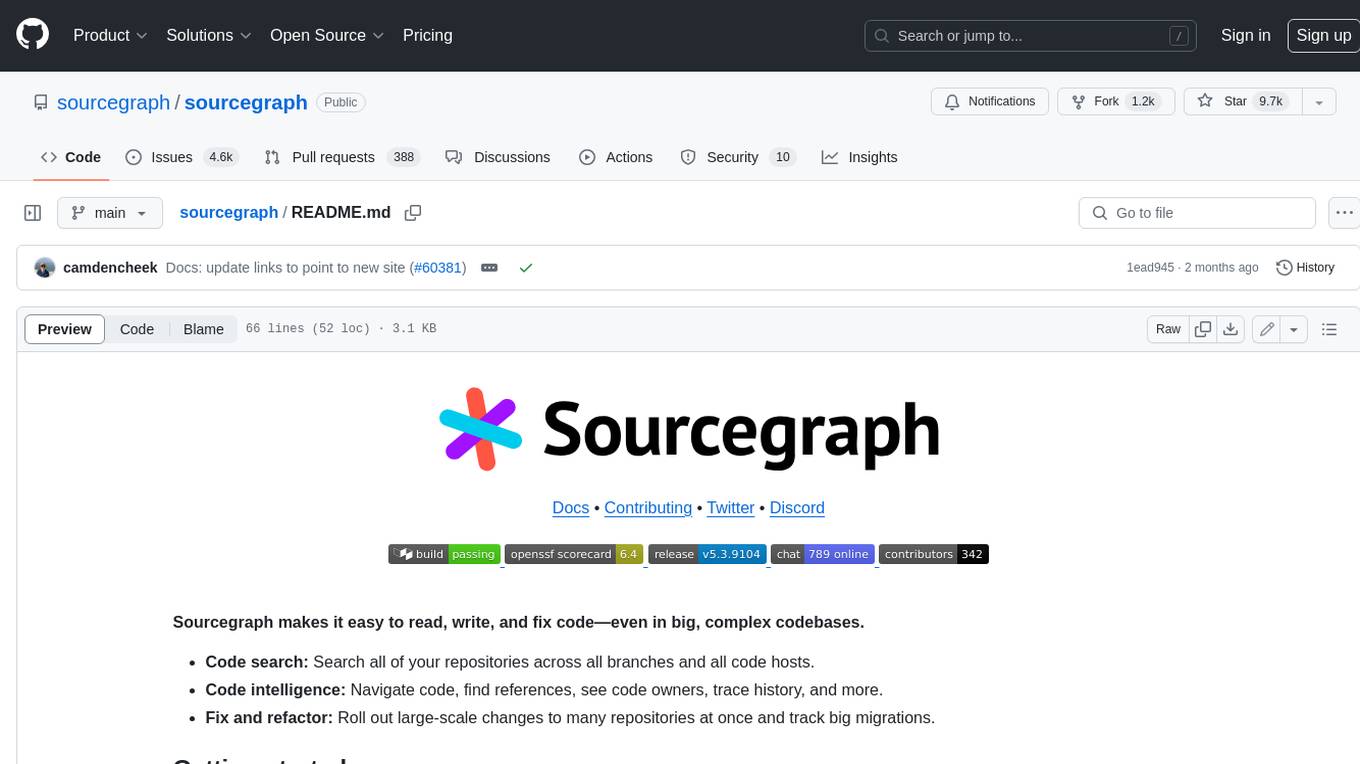
sourcegraph
Sourcegraph is a code search and navigation tool that helps developers read, write, and fix code in large, complex codebases. It provides features such as code search across all repositories and branches, code intelligence for navigation and refactoring, and the ability to fix and refactor code across multiple repositories at once.
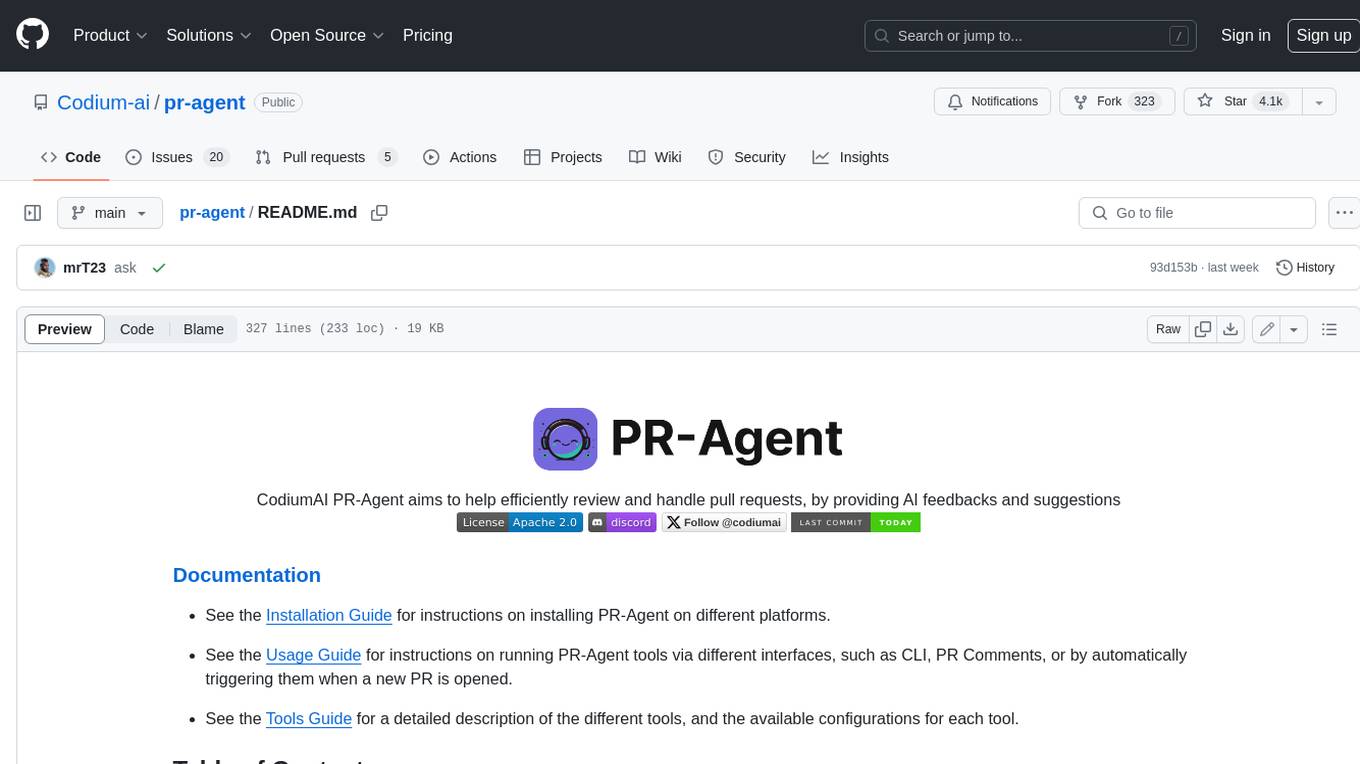
pr-agent
PR-Agent is a tool that helps to efficiently review and handle pull requests by providing AI feedbacks and suggestions. It supports various commands such as generating PR descriptions, providing code suggestions, answering questions about the PR, and updating the CHANGELOG.md file. PR-Agent can be used via CLI, GitHub Action, GitHub App, Docker, and supports multiple git providers and models. It emphasizes real-life practical usage, with each tool having a single GPT-4 call for quick and affordable responses. The PR Compression strategy enables effective handling of both short and long PRs, while the JSON prompting strategy allows for modular and customizable tools. PR-Agent Pro, the hosted version by CodiumAI, provides additional benefits such as full management, improved privacy, priority support, and extra features.
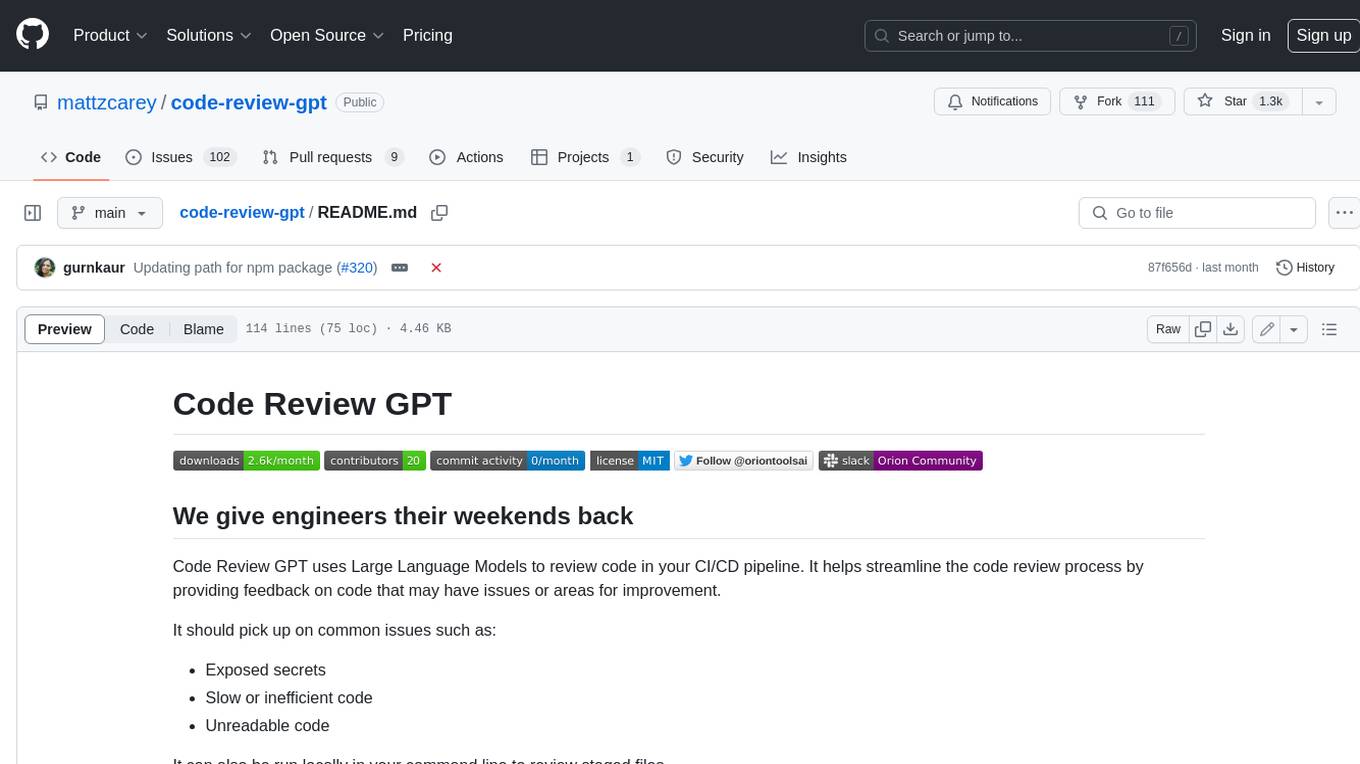
code-review-gpt
Code Review GPT uses Large Language Models to review code in your CI/CD pipeline. It helps streamline the code review process by providing feedback on code that may have issues or areas for improvement. It should pick up on common issues such as exposed secrets, slow or inefficient code, and unreadable code. It can also be run locally in your command line to review staged files. Code Review GPT is in alpha and should be used for fun only. It may provide useful feedback but please check any suggestions thoroughly.
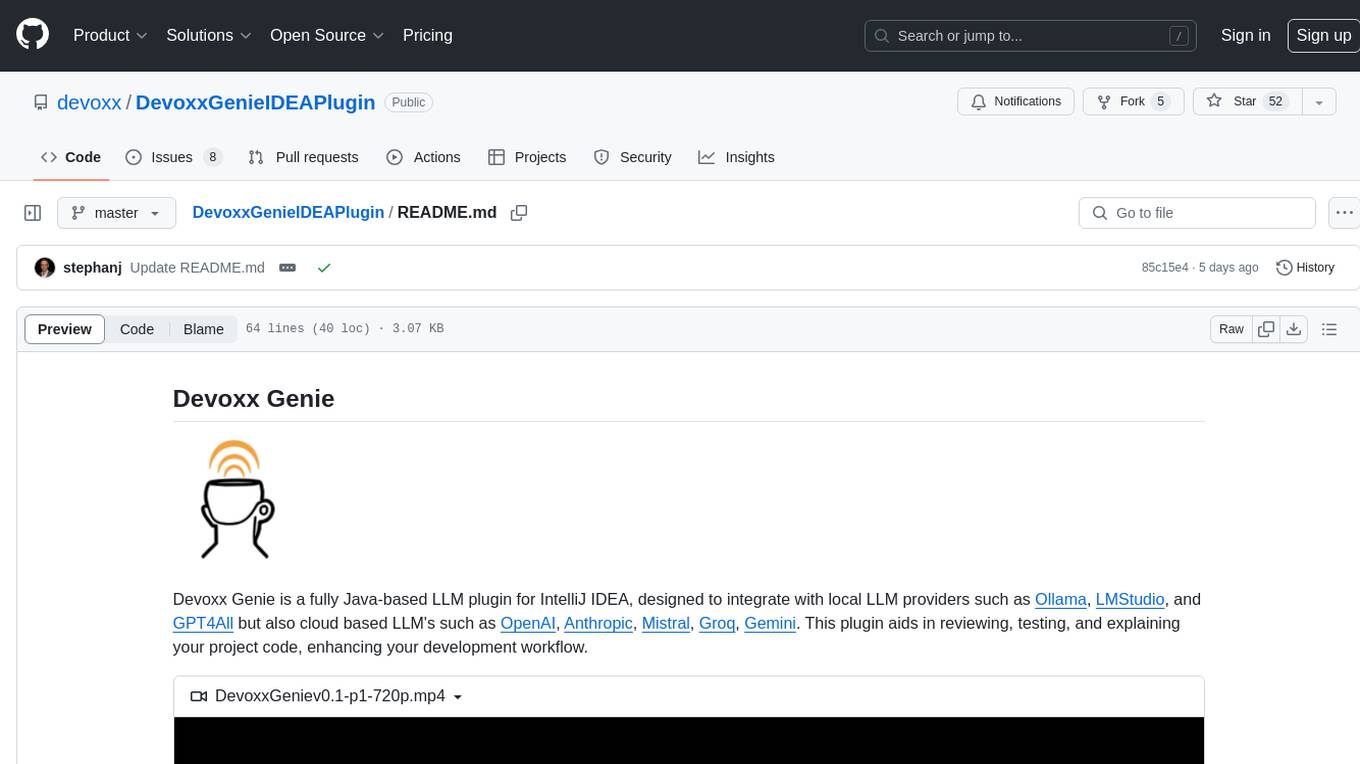
DevoxxGenieIDEAPlugin
Devoxx Genie is a Java-based IntelliJ IDEA plugin that integrates with local and cloud-based LLM providers to aid in reviewing, testing, and explaining project code. It supports features like code highlighting, chat conversations, and adding files/code snippets to context. Users can modify REST endpoints and LLM parameters in settings, including support for cloud-based LLMs. The plugin requires IntelliJ version 2023.3.4 and JDK 17. Building and publishing the plugin is done using Gradle tasks. Users can select an LLM provider, choose code, and use commands like review, explain, or generate unit tests for code analysis.
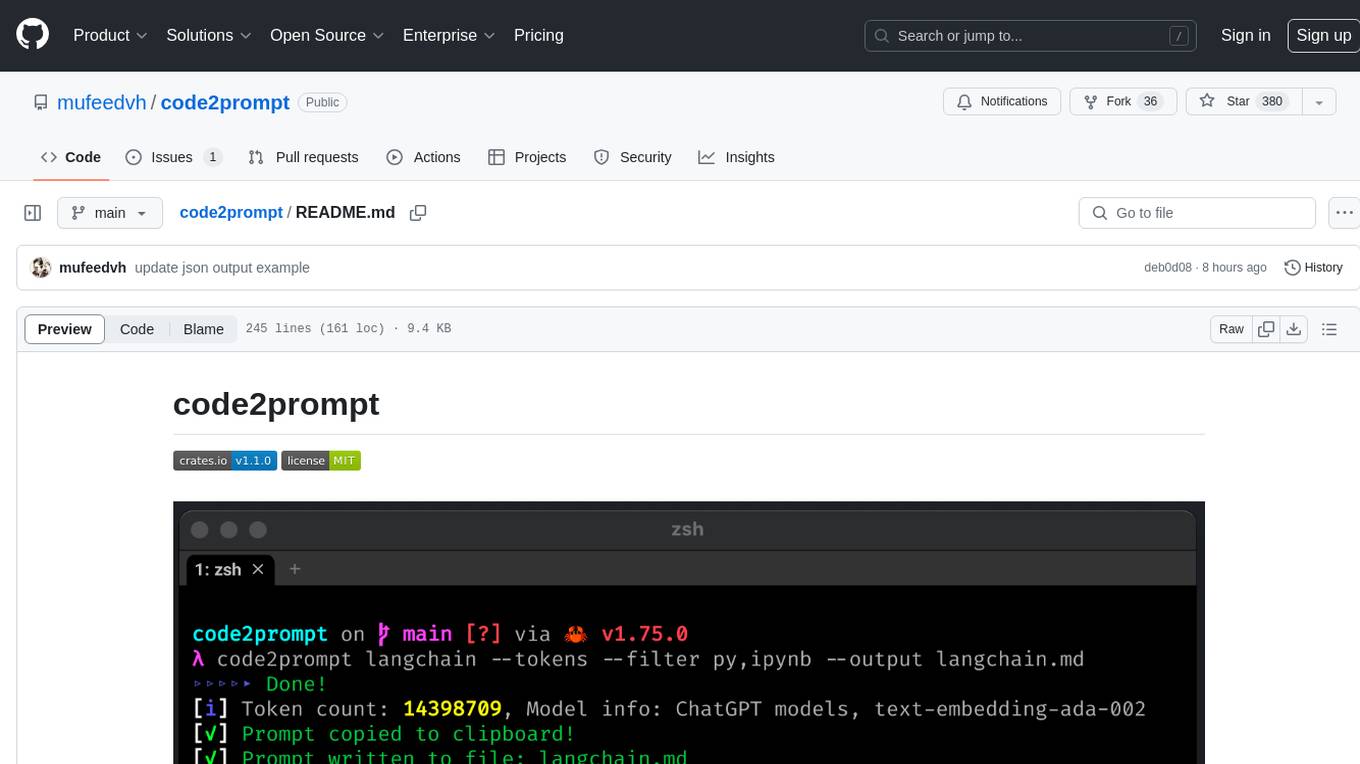
code2prompt
code2prompt is a command-line tool that converts your codebase into a single LLM prompt with a source tree, prompt templating, and token counting. It automates generating LLM prompts from codebases of any size, customizing prompt generation with Handlebars templates, respecting .gitignore, filtering and excluding files using glob patterns, displaying token count, including Git diff output, copying prompt to clipboard, saving prompt to an output file, excluding files and folders, adding line numbers to source code blocks, and more. It helps streamline the process of creating LLM prompts for code analysis, generation, and other tasks.
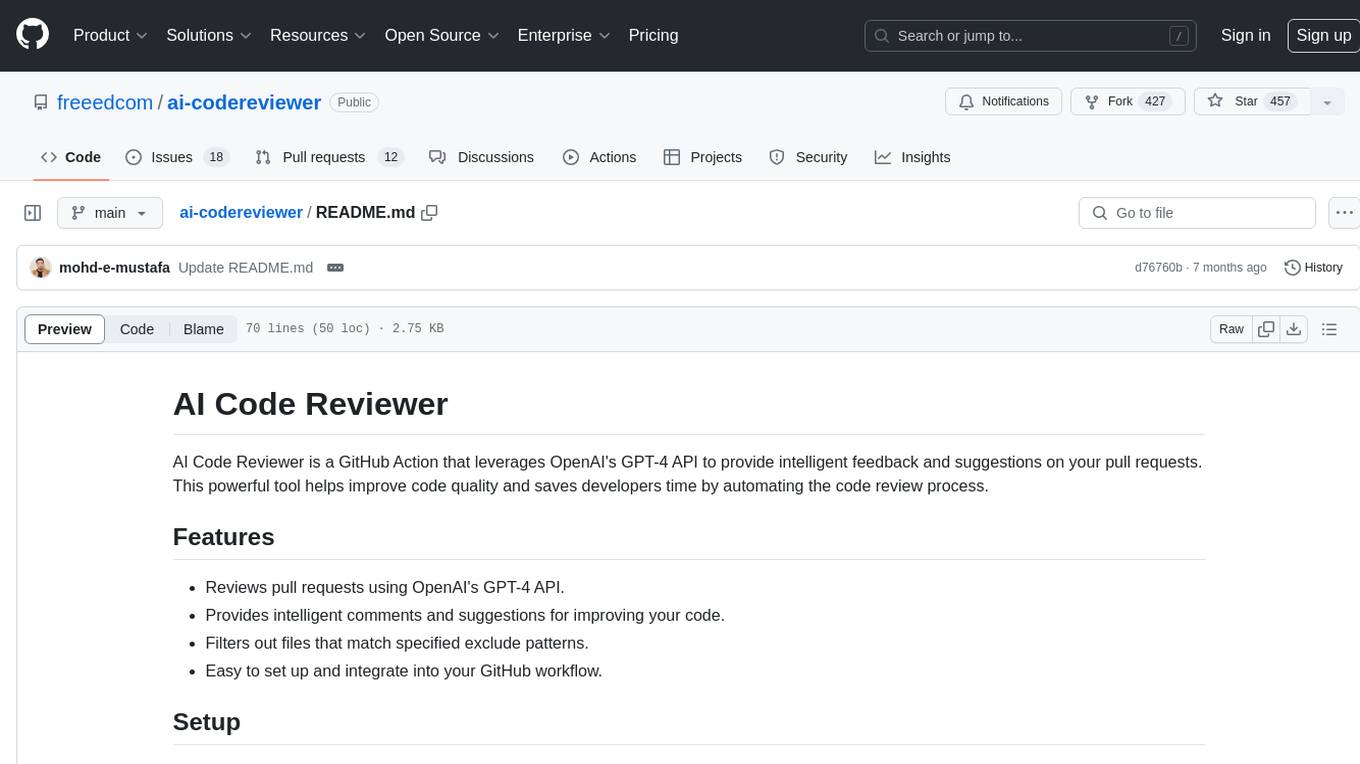
ai-codereviewer
AI Code Reviewer is a GitHub Action that utilizes OpenAI's GPT-4 API to provide intelligent feedback and suggestions on pull requests. It helps enhance code quality and streamline the code review process by offering insightful comments and filtering out specified files. The tool is easy to set up and integrate into GitHub workflows.
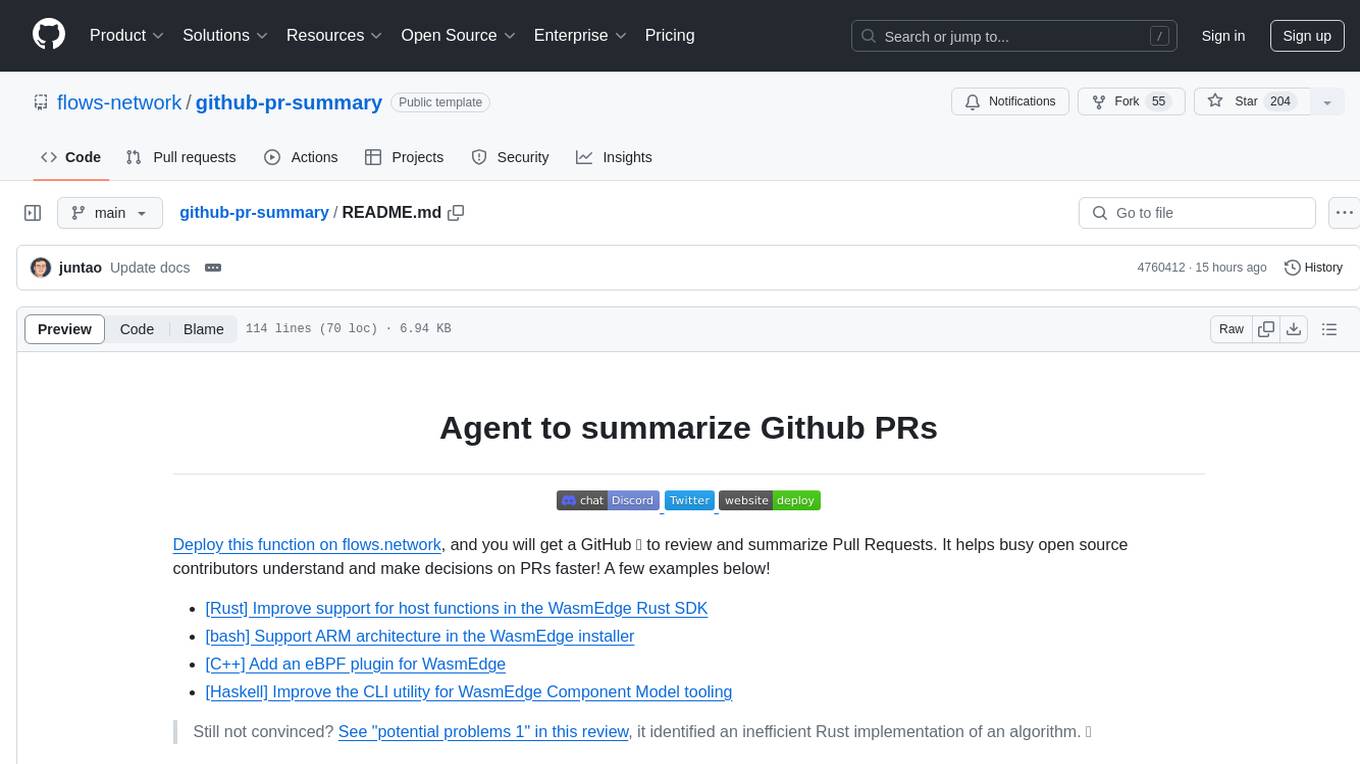
github-pr-summary
github-pr-summary is a bot designed to summarize GitHub Pull Requests, helping open source contributors make faster decisions. It automatically summarizes commits and changed files in PRs, triggered by new commits or a magic trigger phrase. Users can deploy their own code review bot in 3 steps: create a bot from their GitHub repo, configure it to review PRs, and connect to GitHub for access to the target repo. The bot runs on flows.network using Rust and WasmEdge Runtimes. It utilizes ChatGPT/4 to review and summarize PR content, posting the result back as a comment on the PR. The bot can be used on multiple repos by creating new flows and importing the source code repo, specifying the target repo using flow config. Users can also change the magic phrase to trigger a review from a PR comment.
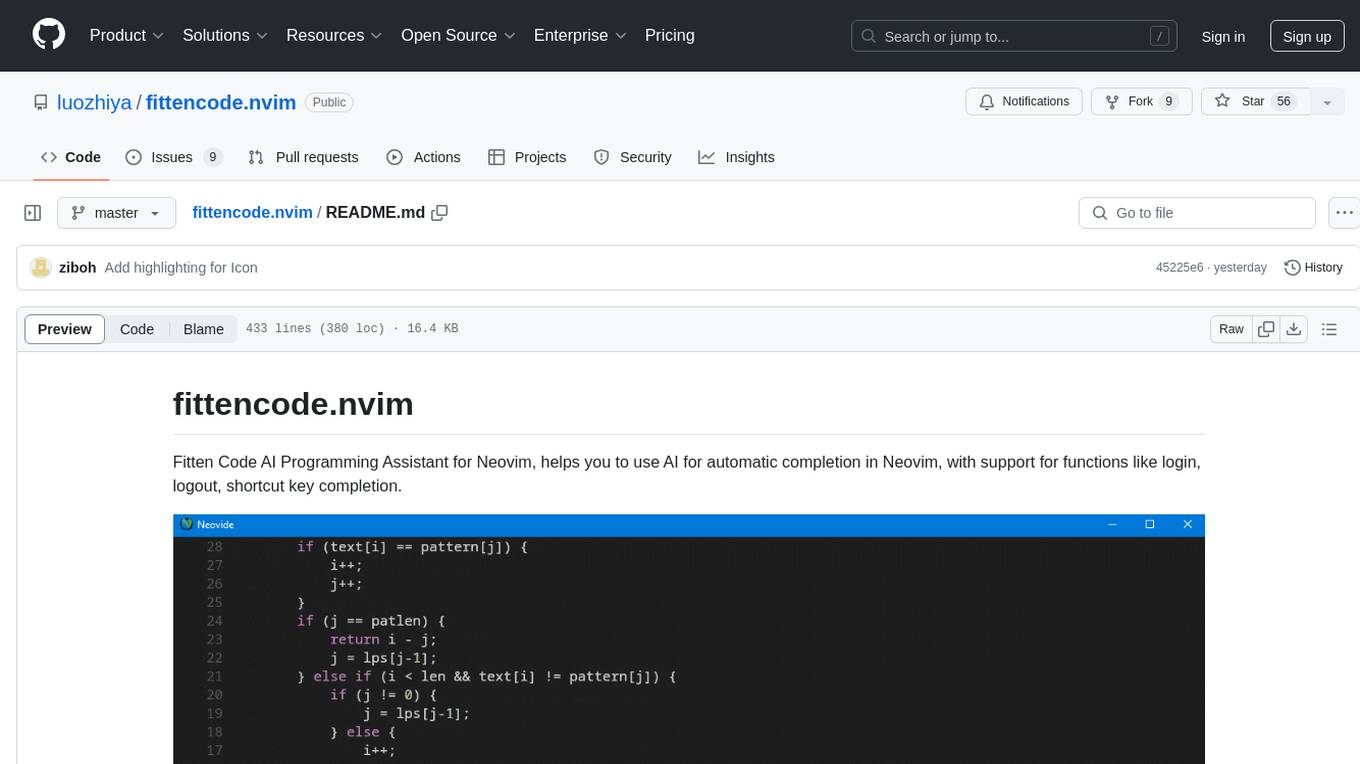
fittencode.nvim
Fitten Code AI Programming Assistant for Neovim provides fast completion using AI, asynchronous I/O, and support for various actions like document code, edit code, explain code, find bugs, generate unit test, implement features, optimize code, refactor code, start chat, and more. It offers features like accepting suggestions with Tab, accepting line with Ctrl + Down, accepting word with Ctrl + Right, undoing accepted text, automatic scrolling, and multiple HTTP/REST backends. It can run as a coc.nvim source or nvim-cmp source.