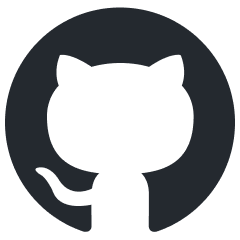
codespin
CodeSpin.AI Code Generation Tools
Stars: 60
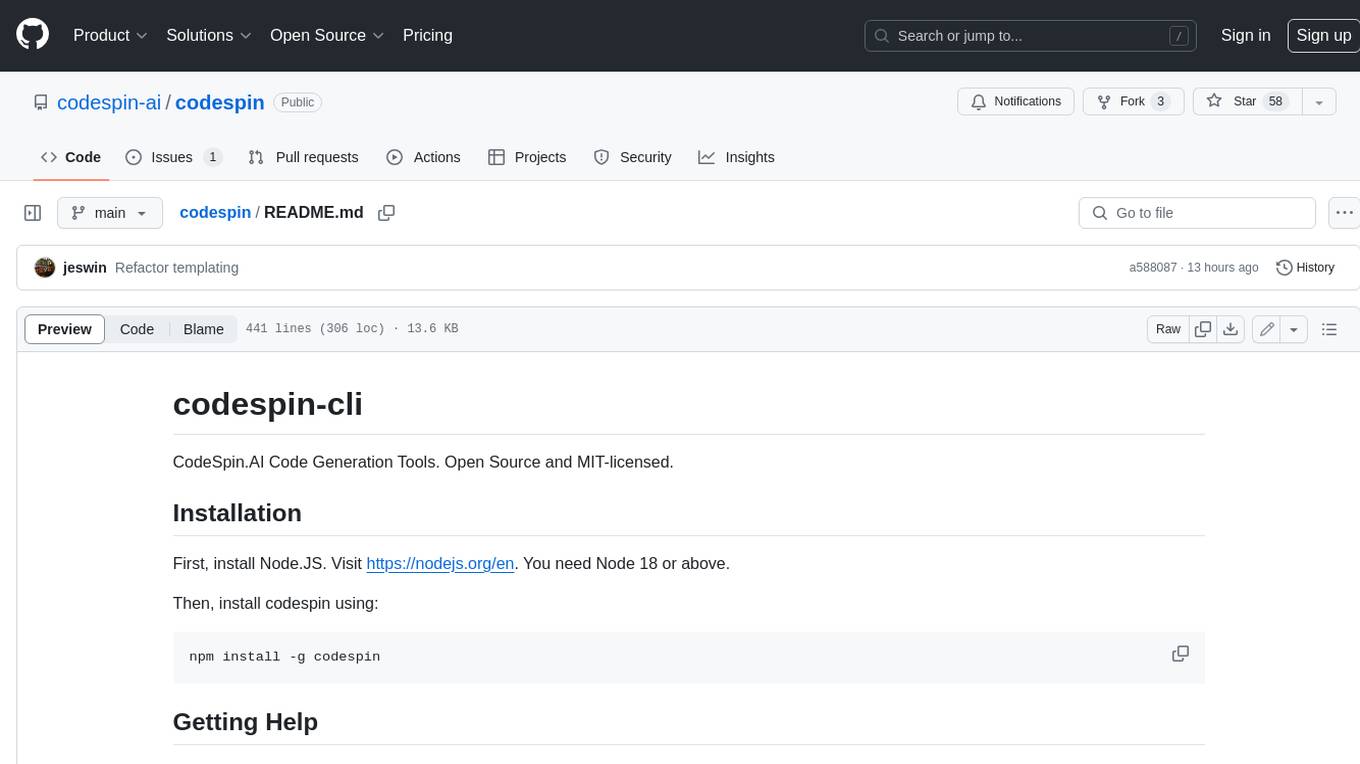
CodeSpin.AI is a set of open-source code generation tools that leverage large language models (LLMs) to automate coding tasks. With CodeSpin, you can generate code in various programming languages, including Python, JavaScript, Java, and C++, by providing natural language prompts. CodeSpin offers a range of features to enhance code generation, such as custom templates, inline prompting, and the ability to use ChatGPT as an alternative to API keys. Additionally, CodeSpin provides options for regenerating code, executing code in prompt files, and piping data into the LLM for processing. By utilizing CodeSpin, developers can save time and effort in coding tasks, improve code quality, and explore new possibilities in code generation.
README:
CodeSpin.AI Code Generation Tools. Open Source and MIT-licensed.
First, install Node.JS. Visit https://nodejs.org/en. You need Node 18 or above.
Then, install codespin using:
npm install -g codespin
💡 The easiest way to use codespin is via the CodeSpin.AI vscode plugin.
To list all available commands:
codespin help
For specific help on a command, type codespin [command] help
.
For instance:
codespin gen help # or codespin generate help
Also, check the Discord Channel.
Set the OPENAI_API_KEY
(AND/OR ANTHROPIC_API_KEY
) environment variable. If you don't have an OpenAI account, register at https://platform.openai.com/signup. For Anthropic, register at https://www.anthropic.com.
If you don't want to get an API key, you may also use it with ChatGPT.
Ready to try? The following command generates code for a Hello World app and displays it:
codespin gen --prompt 'Make a python program (in main.py) that prints Hello, World!'
To save the generated code to a file, use the --write
(or -w
) option:
codespin gen --prompt 'Make a python program (in main.py) that prints Hello, World!' --write
Simple, right? That's just the beginning.
Most features of codespin are available only once you initialize your project like this:
codespin init
This command creates a .codespin directory containing some default templates and configuration files. You may edit these templates as required, but the default template is fairly good.
In addition, it is also recommended to do a global init, which stores the config files under $HOME/.codespin. The global init also creates openai.json and anthropic.json under $HOME/.codespin where you can save your api keys.
codespin init --global
💡 It is recommeded to store the api keys globally, to avoid accidentally committing the api keys to git.
Use the codespin generate
command to produce source code.
You may also use the short alias codespin gen
. The following examples will use codespin gen
.
First, create a "prompt file" to describe the source code. A prompt file is simply a markdown file containing instructions on how to generate the code.
Let's start with something simple.
Create a file called main.py.md
as follows:
out: main.py
includes:
- main.py
Print Hello, World!
Include a shebang to make it directly executable.
Then generate the code by calling codespin:
codespin gen main.py.md --write
Alternatively, you could specify the file paths in CLI with --out
(or -o
shorthand) and --include
(or -o
) instead of using front-matter.
codespin gen main.py.md --out main.py --include main.py --write
This is just as simple. Here's an example of how you'd scaffold a new Node.JS blog app:
blogapp.md
:
Create a Node.JS application for a blog.
Split the code into multiple files for maintainability.
Use ExpressJS. Use Postgres for the database.
Place database code in a different file (a database layer).
For the code generator to better understand the context, you must pass the relevant external files (such as dependencies) with the --include
(or -i
) option.
For example, if main.py
depends on dep1.py
and dep2.py
:
codespin gen main.py.md --out main.py --include main.py --include dep1.py --include dep2.py --write
With --include
you can specify wildcards. The following will include all ".py" files:
codespin gen main.py.md --out main.py -d "*.py" --write
You can also define the --include
, --template
, --parser
, --model
, and --max-tokens
parameters in front-matter like this:
---
model: gpt-4o
maxTokens: 8000
out: main.py
includes:
- dep1.py
- dep2.py
---
Generate a Python CLI script named index.py that accepts arguments, calls calculate() function in dep1.py and prints their sum with print() in dep2.py.
It's quite a common requirement to mention a standard set of rules in all prompt files; such as mentioning coding conventions for a project. The include directive (codespin:include:<path>
) let's you write common rules in a file, and include them in prompts as needed.
For example, if you had a conventions.txt
file:
- Use snake_case for variables
- Generate extensive comments
You can include it like this:
Generate a Python CLI script named index.py that accepts arguments and prints their sum.
codespin:include:/conventions.txt
Spec files are another way to handle coding conventions and other instructions.
A "spec" is a template file containing a placeholder "{prompt}". The placeholder will be replaced by the prompt supplied (via the prompt file, or the --prompt argument).
For example, if you have the following spec called myrules.txt
:
{prompt}
Rules:
- Use snake_case for variables
- Generate extensive comments
You can include it like this:
codespin gen main.py.md --spec myrules.txt
The exec directive executes a command and replaces the line with the output of the command. This powerful technique can be used to make your templates smarter.
For example, if you want to include the diff of a file in your prompt, you could do this:
codespin:exec:git diff HEAD~1 HEAD -- main.py
The easiest way to regenerate code (for a single file) is by changing the original prompt to mention just the required modifications.
For example, if you originally had this in calculate_area.py.md
:
Write a function named calculate_area(l, b) which returns l\*b.
You could rewrite it as:
Change the function calculate_area to take an additional parameter shape_type (as the first param), and return the correct calculations. The subsequent parameters are dimensions of the shape, and there could be one (for a circle) or more dimensions (for a multi-sided shape).
And run the gen command as usual:
codespin gen calculate_area.py.md --out calculate_area.py --include calculate_area.py -w
Sometimes you want to ignore the latest modifications while generating code, and use previously committed file contents. The include parameter (both as a CLI arg and in frontmatter) understands git revisions.
You can do that by specifying the version like this.
codespin gen calculate_area.py.md --out calculate_area.py --include HEAD:calculate_area.py -w
You can include diffs as well:
# Diff a file between two versions
codespin gen main.py.md --out main.py --include HEAD~2+HEAD:main.py -w
There are some convenient shortcuts.
# include HEAD:main.py
codespin gen main.py.md --out main.py --include :main.py -w
# diff between HEAD and Working Copy
codespin gen main.py.md --out main.py --include +:main.py -w
# diff between HEAD~2 and Working Copy
codespin gen main.py.md --out main.py --include HEAD~2+:main.py -w
This command above will ignore the latest edits to main.py and use content from git's HEAD.
-
-c, --config <file path>
: Path to a config directory (.codespin). -
-e, --exec <script path>
: Execute a command for each generated file. -
-i, --include <file path>
: List of files to include in the prompt for additional context. Supports version specifiers and wildcards. -
-o, --out <output file path>
: Specify the output file name to generate. -
-p, --prompt <some text>
: Specify the prompt directly on the command line. -
-t, --template <template name or path>
: Path to the template file. -
-w, --write
: Write generated code to source file(s). -
--debug
: Enable debug mode. Prints debug messages for every step. -
--exclude <file path>
: List of files to exclude from the prompt. Used to override automatically included source files. -
--maxTokens <number>
: Maximum number of tokens for generated code. -
--model <model name>
: Name of the model to use, such as 'gpt-4o' or 'claude-3-5-haiku-latest'. -
--multi <number>
: Maximum number of API calls to make if the output exceeds token limit. -
--outDir <dir path>
: Path to directory relative to which files are generated. Defaults to the directory of the prompt file. -
--parse
: Whether the LLM response needs to be processed. Defaults to true. Use--no-parse
to disable parsing. -
--parser <path to js file>
: Use a custom script to parse LLM response. -
--pp, --printPrompt
: Print the generated prompt to the screen. Does not call the API. -
--spec <spec file>
: Specify a spec (prompt template) file. -
-a, --templateArgs <argument>
: An argument passed to a custom template. Can pass multiple by repeating-a
. -
--writePrompt <file>
: Write the generated prompt out to the specified path. Does not call the API. -
--maxi, --maxInput <bytes>
: Maximum number of input bytes to send to the API. -
-h, --help
: Display help.
As shown earlier, you can specify the prompt directly in the command line:
codespin gen --prompt 'Create a file main.py with a function to add two numbers.'
Remember to use --write
to save the generated files.
A CodeSpin Template is a JS file (an ES6 Module) exporting a default function with the following signature:
// The templating function that generates the LLM prompt.
export default async function generate(
args: TemplateArgs,
config: CodeSpinConfig
): Promise<TemplateResult> {
// Return the prompt to send to the LLM.
}
where TemplateResult and TemplateArgs are defined as:
// Output of the template
export type TemplateResult = {
// The generated prompt
prompt: string;
// Defaults to "file-block", and that's the only available option.
responseParser?: "file-block";
};
// Arguments to the templating function
export type TemplateArgs = {
prompt: string;
promptWithLineNumbers: string;
includes: VersionedFileInfo[];
generatedFiles: FileContent[];
outPath: string | undefined;
promptSettings: unknown;
customArgs: string[] | undefined;
workingDir: string;
debug: boolean | undefined;
};
export type FileContent = {
path: string;
content: string;
};
export type VersionedFileInfo =
| {
path: string;
type: "content";
content: string;
version: string;
}
| {
path: string;
type: "diff";
diff: string;
version1: string | undefined;
version2: string | undefined;
};
When generating code, specify custom templates with the --template
(or -t
) option:
codespin gen main.py.md --out main.py --template mypythontemplate.mjs --include main.py -w
💡 Your template should have the extension mjs
instead of js
.
Once you do codespin init
, you should be able to see example templates under the "codespin/templates" directory.
There are two ways to pass custom args to a custom template:
- frontMatter in a prompt file goes under
args.promptSettings
---
model: gpt-4o
maxTokens: 8000
useJDK: true //custom arg
out: main.py
---
- CLI args can be passed to the template with the
-a
(or--template-args
) option, and they'll be available inargs.customArgs
as a string array.
codespin gen main.py.md \
--template mypythontemplate.mjs \
-a useAWS \
-a swagger \
--out main.py \
--include main.py \
--write
While using codespin with an API key is straightforward, if you don't have one but have access to ChatGPT, there are alternatives.
Use the --pp
(or --print-prompt
) option to display the final LLM prompt, or --write-prompt
to save it to a file:
# Display on screen
codespin gen something.py.md --print-prompt
# Or save to a file
codespin gen something.py.md --write-prompt /path/to/file.txt
Copy and paste the prompt into ChatGPT. Save ChatGPT's response in a file, e.g., gptresponse.txt
.
Then, use the codespin parse
command to parse the content:
# As always, use --write for writing to the disk
codespin parse gptresponse.txt --write
💡 When copying the response from ChatGPT, use the copy icon. Selecting text and copying doesn't retain formatting.
You can pipe into codespin with the codespin go
command:
ls | codespin go 'Convert to uppercase each line in the following text'
If you find more effective templates or prompts, please open a Pull Request.
For Tasks:
Click tags to check more tools for each tasksFor Jobs:
Alternative AI tools for codespin
Similar Open Source Tools
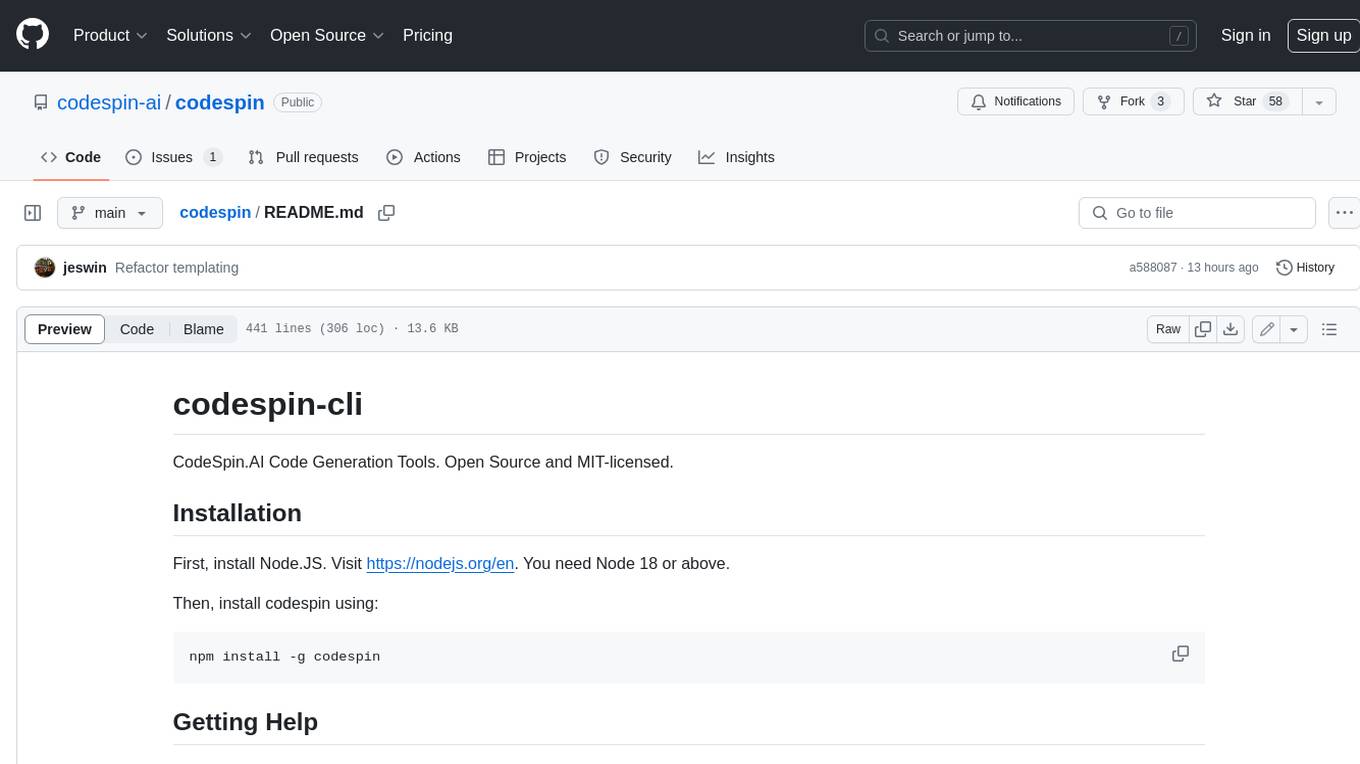
codespin
CodeSpin.AI is a set of open-source code generation tools that leverage large language models (LLMs) to automate coding tasks. With CodeSpin, you can generate code in various programming languages, including Python, JavaScript, Java, and C++, by providing natural language prompts. CodeSpin offers a range of features to enhance code generation, such as custom templates, inline prompting, and the ability to use ChatGPT as an alternative to API keys. Additionally, CodeSpin provides options for regenerating code, executing code in prompt files, and piping data into the LLM for processing. By utilizing CodeSpin, developers can save time and effort in coding tasks, improve code quality, and explore new possibilities in code generation.
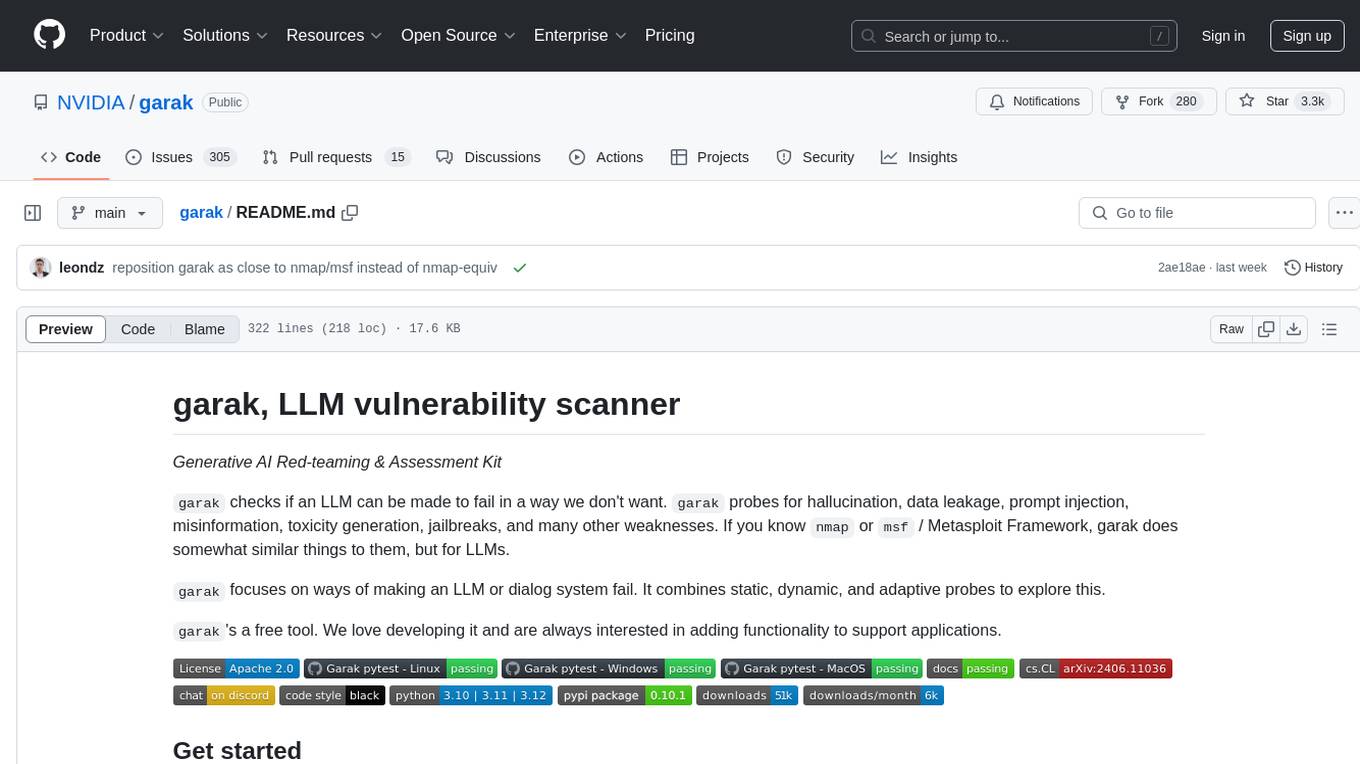
garak
Garak is a vulnerability scanner designed for LLMs (Large Language Models) that checks for various weaknesses such as hallucination, data leakage, prompt injection, misinformation, toxicity generation, and jailbreaks. It combines static, dynamic, and adaptive probes to explore vulnerabilities in LLMs. Garak is a free tool developed for red-teaming and assessment purposes, focusing on making LLMs or dialog systems fail. It supports various LLM models and can be used to assess their security and robustness.
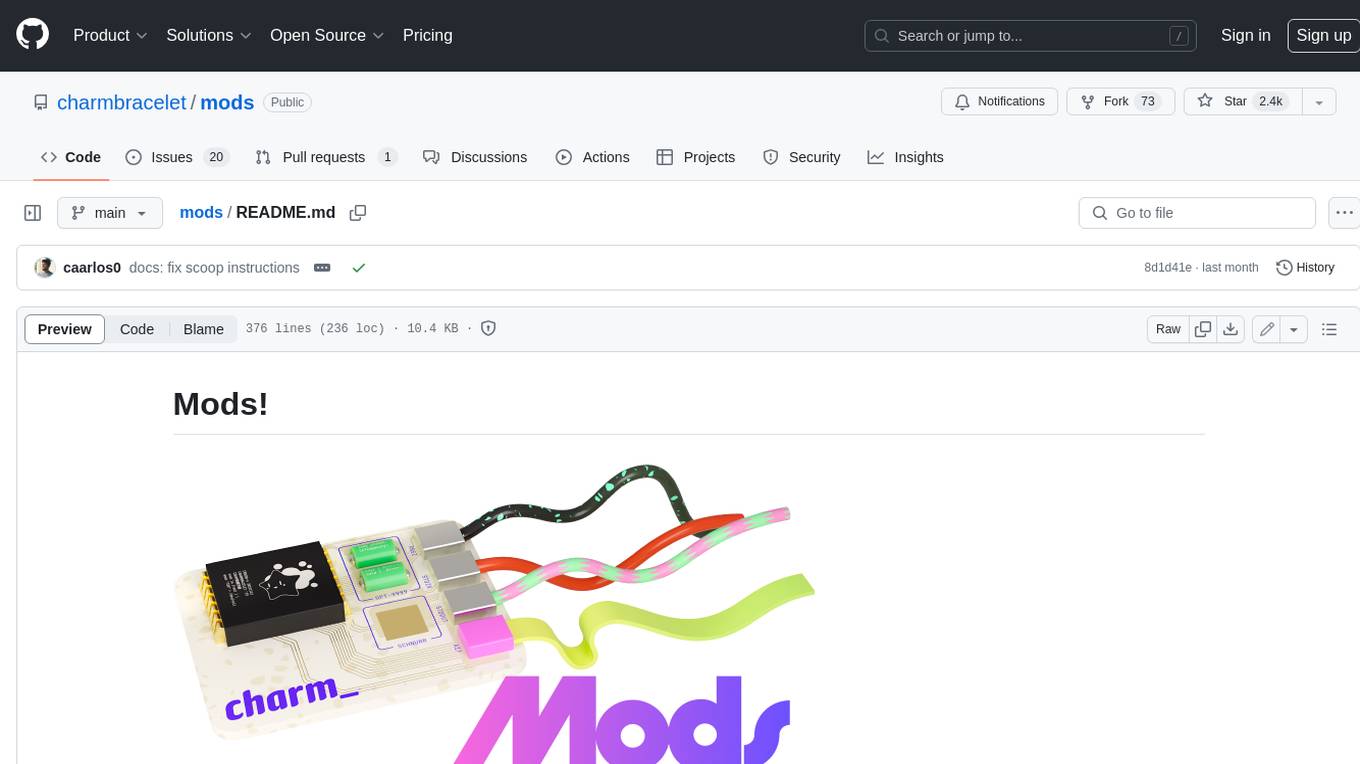
mods
AI for the command line, built for pipelines. LLM based AI is really good at interpreting the output of commands and returning the results in CLI friendly text formats like Markdown. Mods is a simple tool that makes it super easy to use AI on the command line and in your pipelines. Mods works with OpenAI, Groq, Azure OpenAI, and LocalAI To get started, install Mods and check out some of the examples below. Since Mods has built-in Markdown formatting, you may also want to grab Glow to give the output some _pizzazz_.
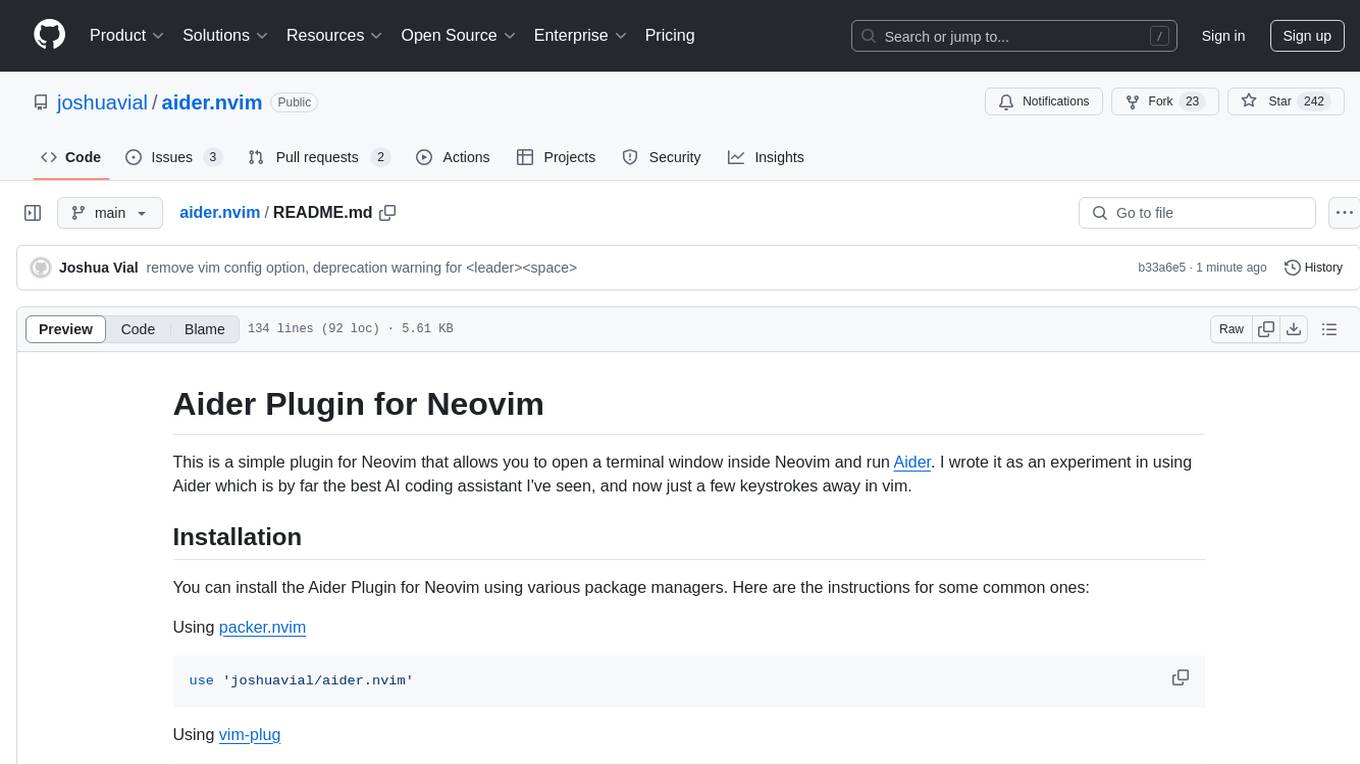
aider.nvim
Aider.nvim is a Neovim plugin that integrates the Aider AI coding assistant, allowing users to open a terminal window within Neovim to run Aider. It provides functions like AiderOpen to open the terminal window, AiderAddModifiedFiles to add git-modified files to the Aider chat, and customizable keybindings. Users can configure the plugin using the setup function to manage context, keybindings, debug logging, and ignore specific buffer names.
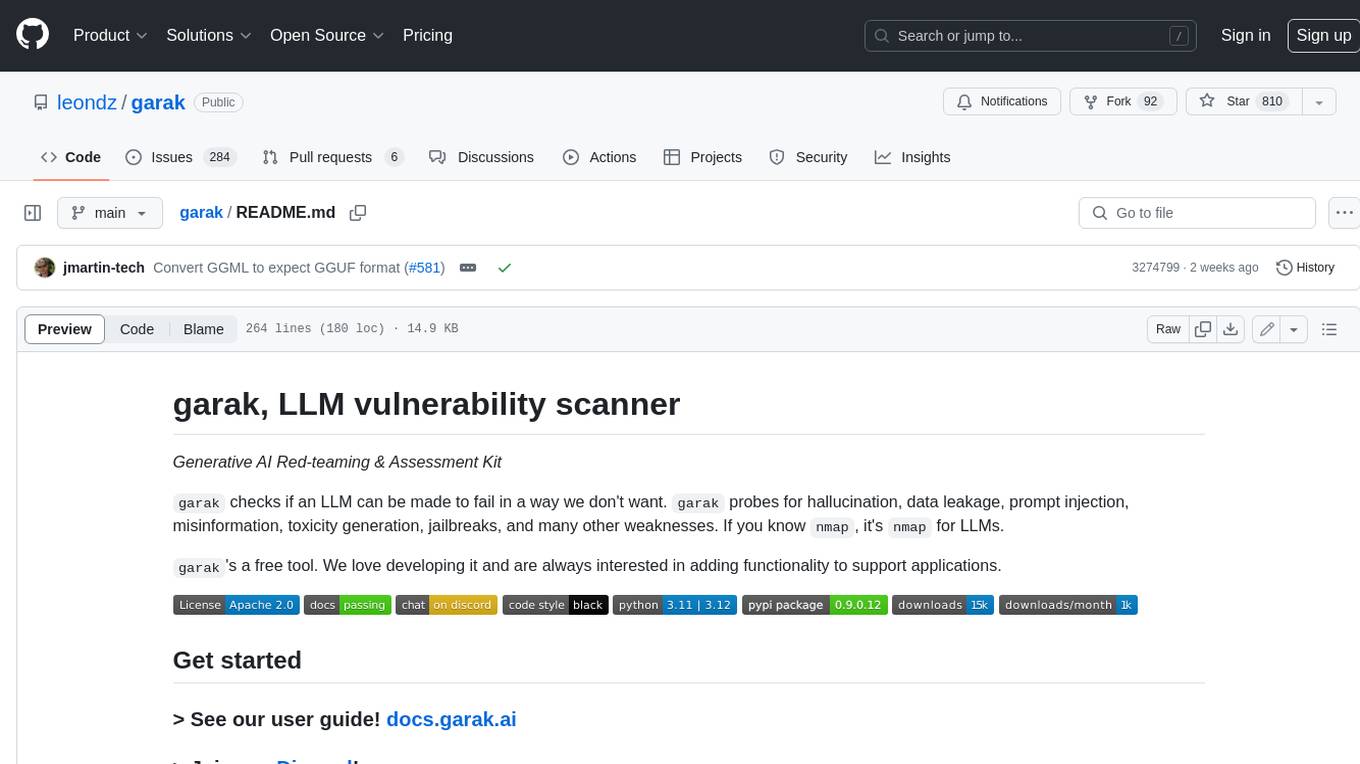
garak
Garak is a free tool that checks if a Large Language Model (LLM) can be made to fail in a way that is undesirable. It probes for hallucination, data leakage, prompt injection, misinformation, toxicity generation, jailbreaks, and many other weaknesses. Garak's a free tool. We love developing it and are always interested in adding functionality to support applications.
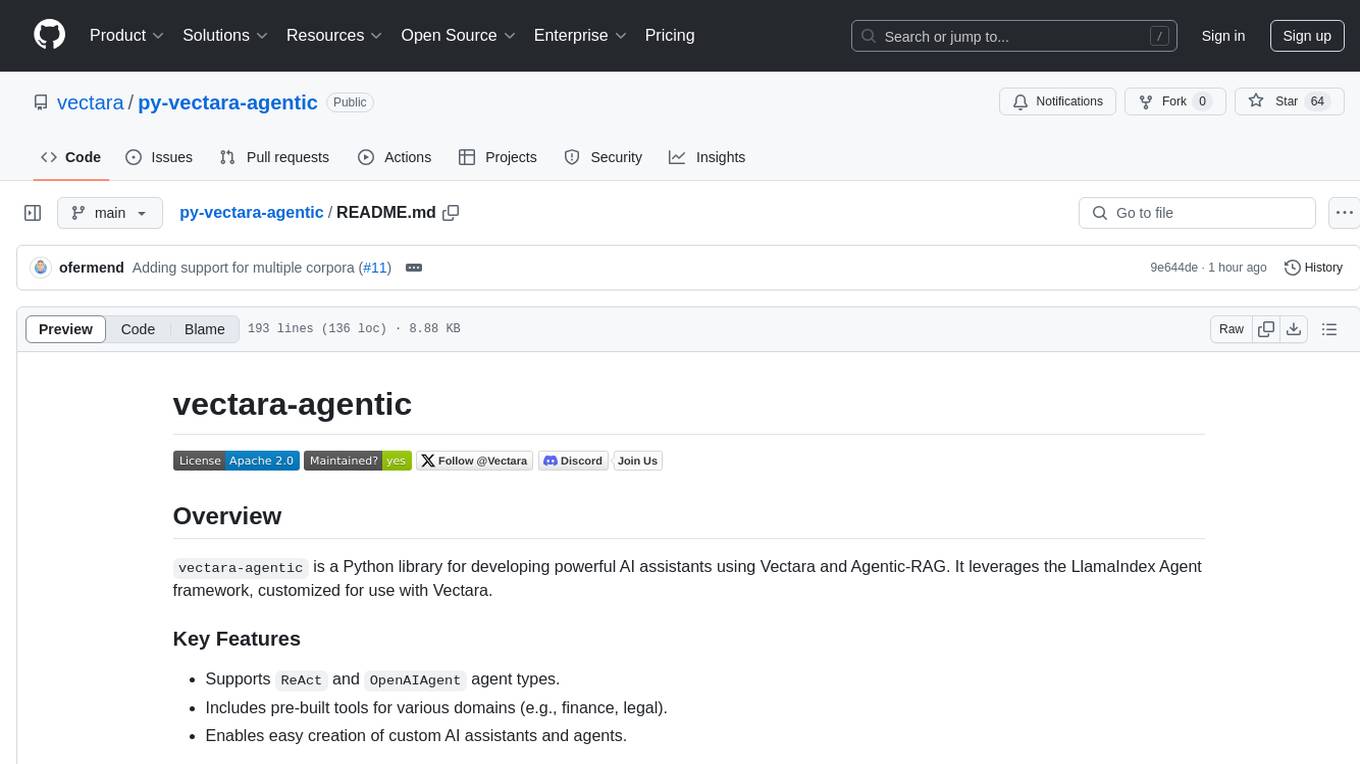
py-vectara-agentic
The `vectara-agentic` Python library is designed for developing powerful AI assistants using Vectara and Agentic-RAG. It supports various agent types, includes pre-built tools for domains like finance and legal, and enables easy creation of custom AI assistants and agents. The library provides tools for summarizing text, rephrasing text, legal tasks like summarizing legal text and critiquing as a judge, financial tasks like analyzing balance sheets and income statements, and database tools for inspecting and querying databases. It also supports observability via LlamaIndex and Arize Phoenix integration.
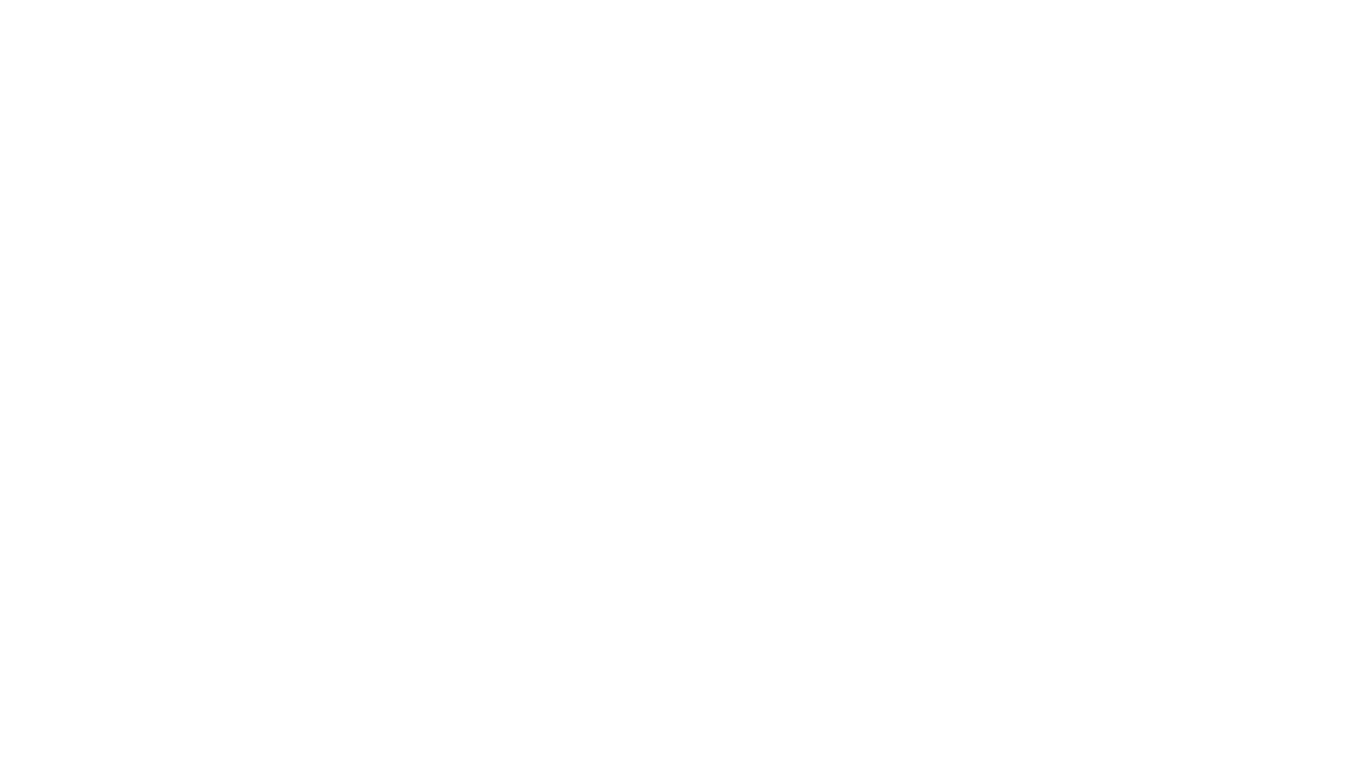
appworld
AppWorld is a high-fidelity execution environment of 9 day-to-day apps, operable via 457 APIs, populated with digital activities of ~100 people living in a simulated world. It provides a benchmark of natural, diverse, and challenging autonomous agent tasks requiring rich and interactive coding. The repository includes implementations of AppWorld apps and APIs, along with tests. It also introduces safety features for code execution and provides guides for building agents and extending the benchmark.
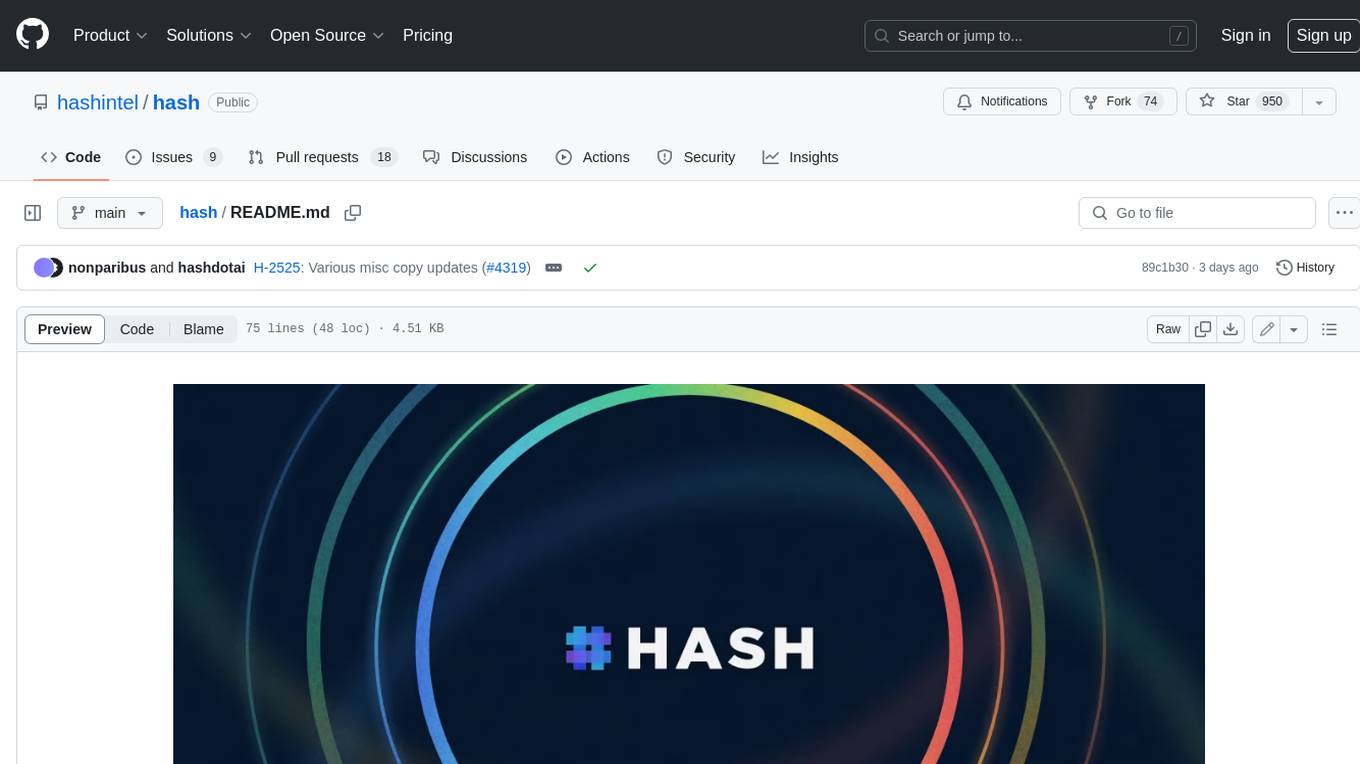
hash
HASH is a self-building, open-source database which grows, structures and checks itself. With it, we're creating a platform for decision-making, which helps you integrate, understand and use data in a variety of different ways.
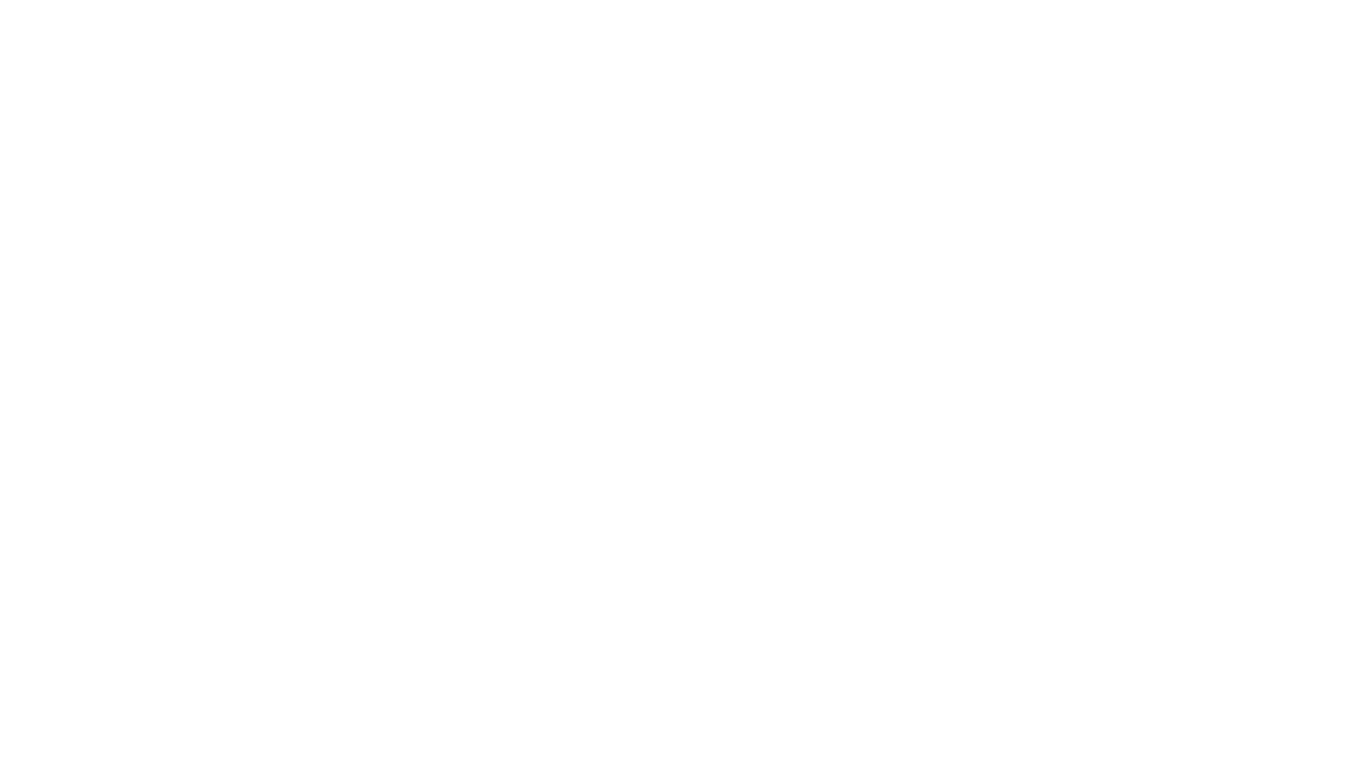
meta-prompt
Meta-Prompt is a tool designed for interacting with Jina Search Foundation APIs. It provides a way to access different versions of the API, pipe into 'llm' for specific tasks, and handle programmatic access for clean responses. Developers can upload prompts, fetch prompts using curl commands, and utilize response templates and headers for AI applications.
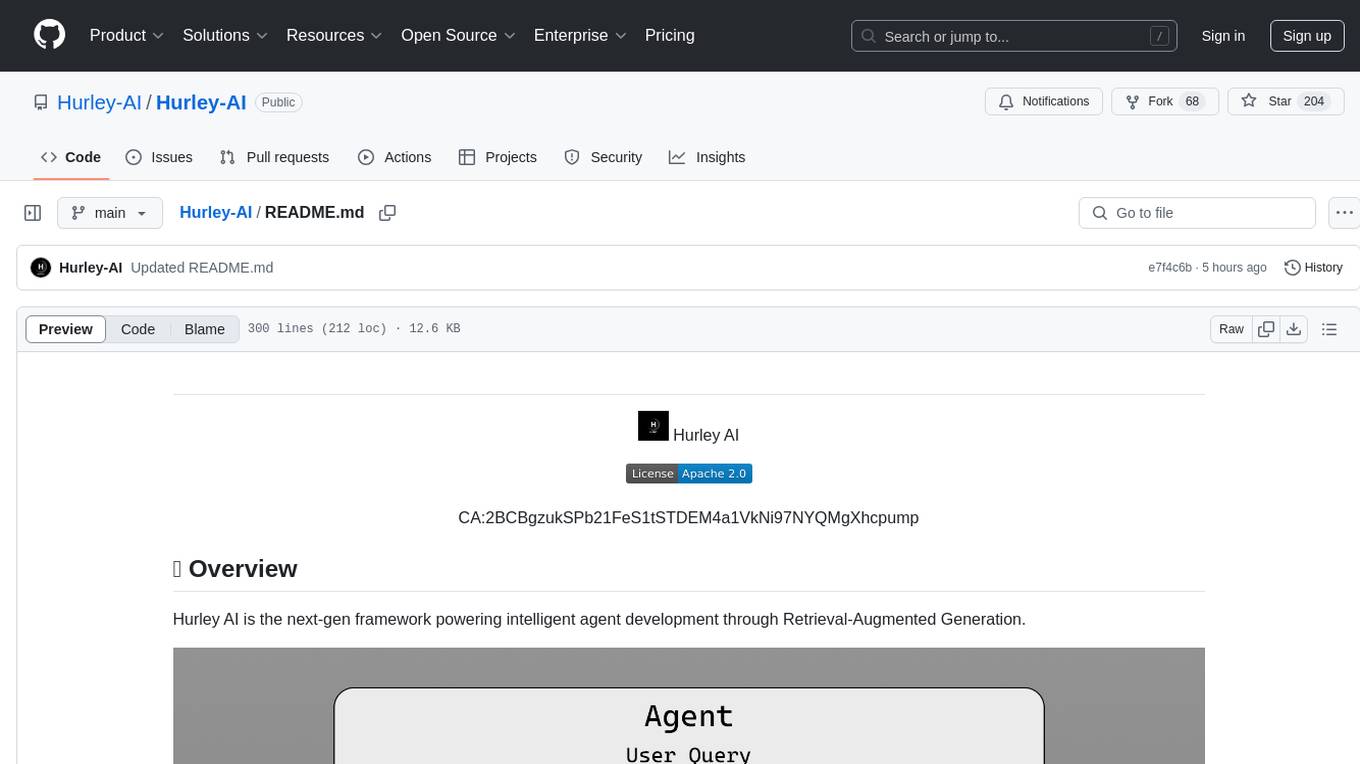
Hurley-AI
Hurley AI is a next-gen framework for developing intelligent agents through Retrieval-Augmented Generation. It enables easy creation of custom AI assistants and agents, supports various agent types, and includes pre-built tools for domains like finance and legal. Hurley AI integrates with LLM inference services and provides observability with Arize Phoenix. Users can create Hurley RAG tools with a single line of code and customize agents with specific instructions. The tool also offers various helper functions to connect with Hurley RAG and search tools, along with pre-built tools for tasks like summarizing text, rephrasing text, understanding memecoins, and querying databases.
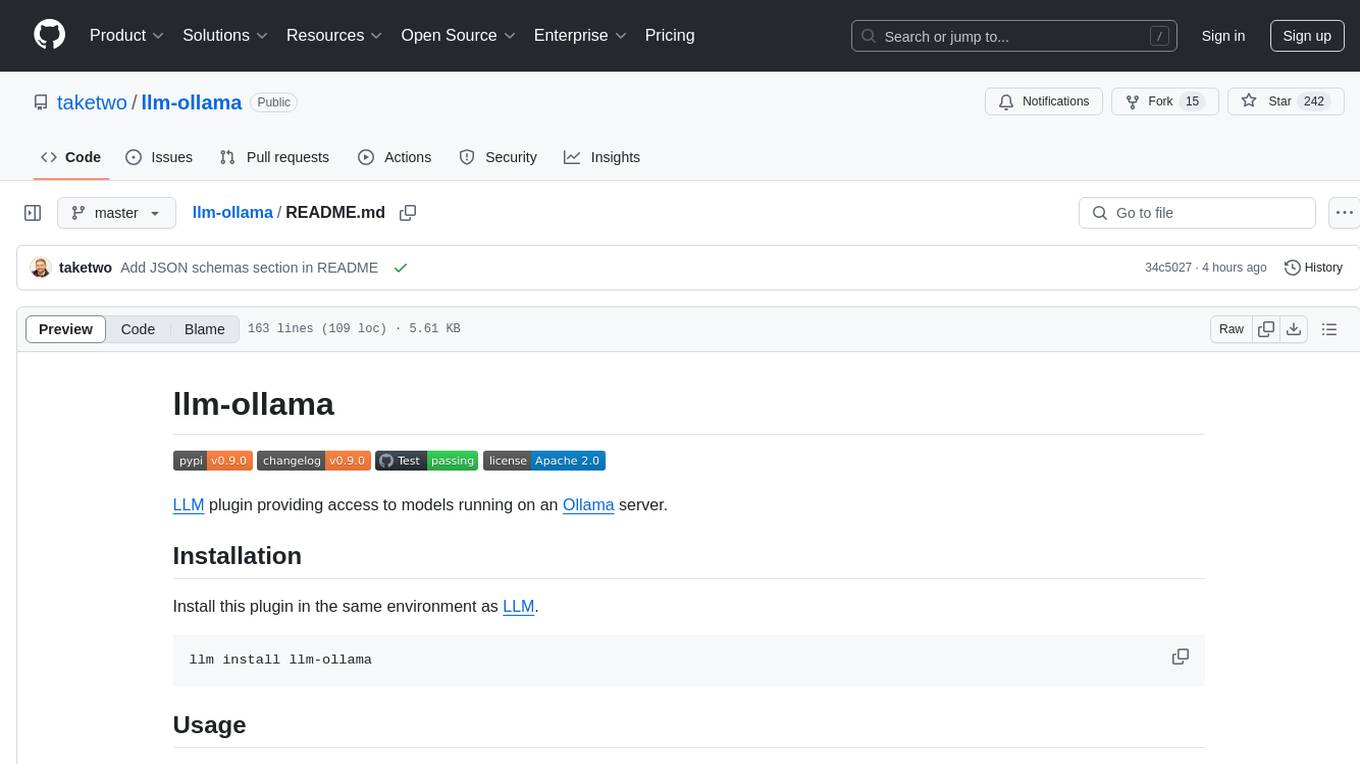
llm-ollama
LLM-ollama is a plugin that provides access to models running on an Ollama server. It allows users to query the Ollama server for a list of models, register them with LLM, and use them for prompting, chatting, and embedding. The plugin supports image attachments, embeddings, JSON schemas, async models, model aliases, and model options. Users can interact with Ollama models through the plugin in a seamless and efficient manner.
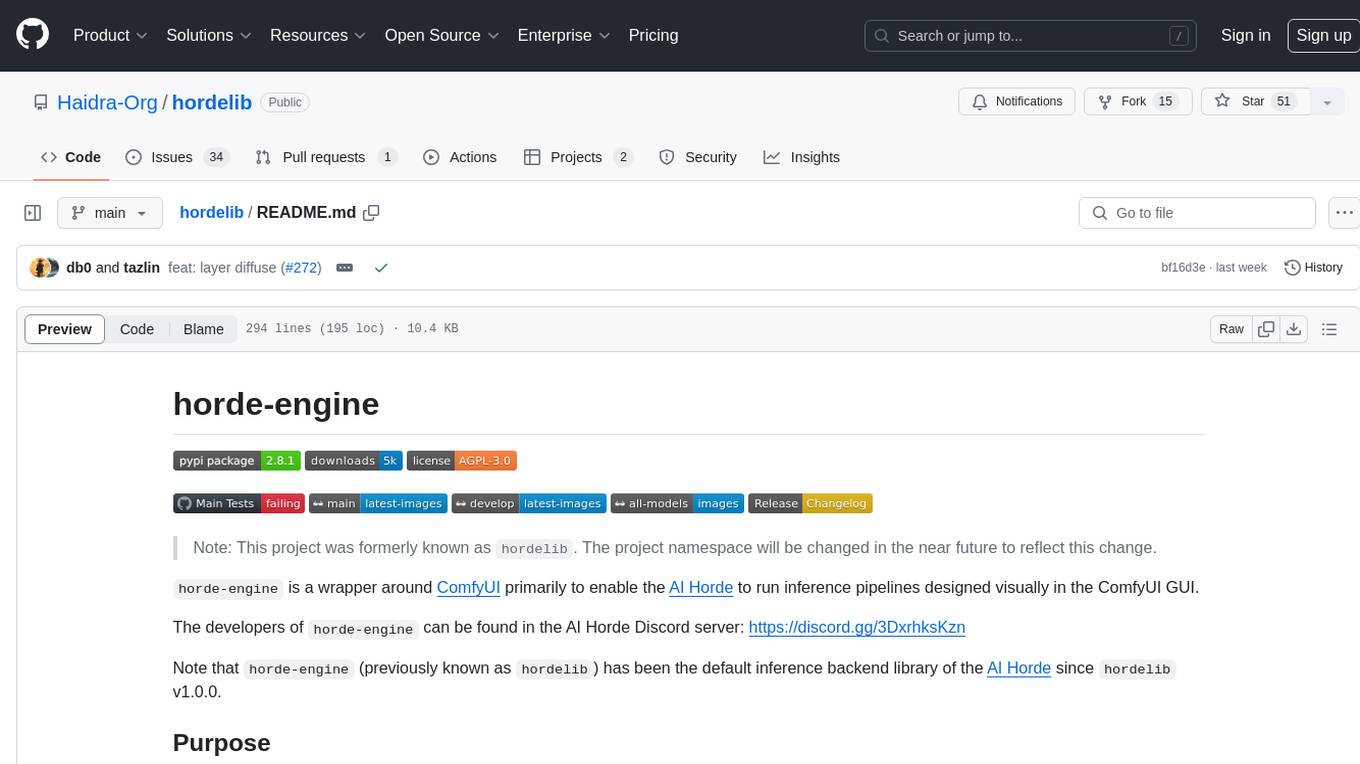
hordelib
horde-engine is a wrapper around ComfyUI designed to run inference pipelines visually designed in the ComfyUI GUI. It enables users to design inference pipelines in ComfyUI and then call them programmatically, maintaining compatibility with the existing horde implementation. The library provides features for processing Horde payloads, initializing the library, downloading and validating models, and generating images based on input data. It also includes custom nodes for preprocessing and tasks such as face restoration and QR code generation. The project depends on various open source projects and bundles some dependencies within the library itself. Users can design ComfyUI pipelines, convert them to the backend format, and run them using the run_image_pipeline() method in hordelib.comfy.Comfy(). The project is actively developed and tested using git, tox, and a specific model directory structure.
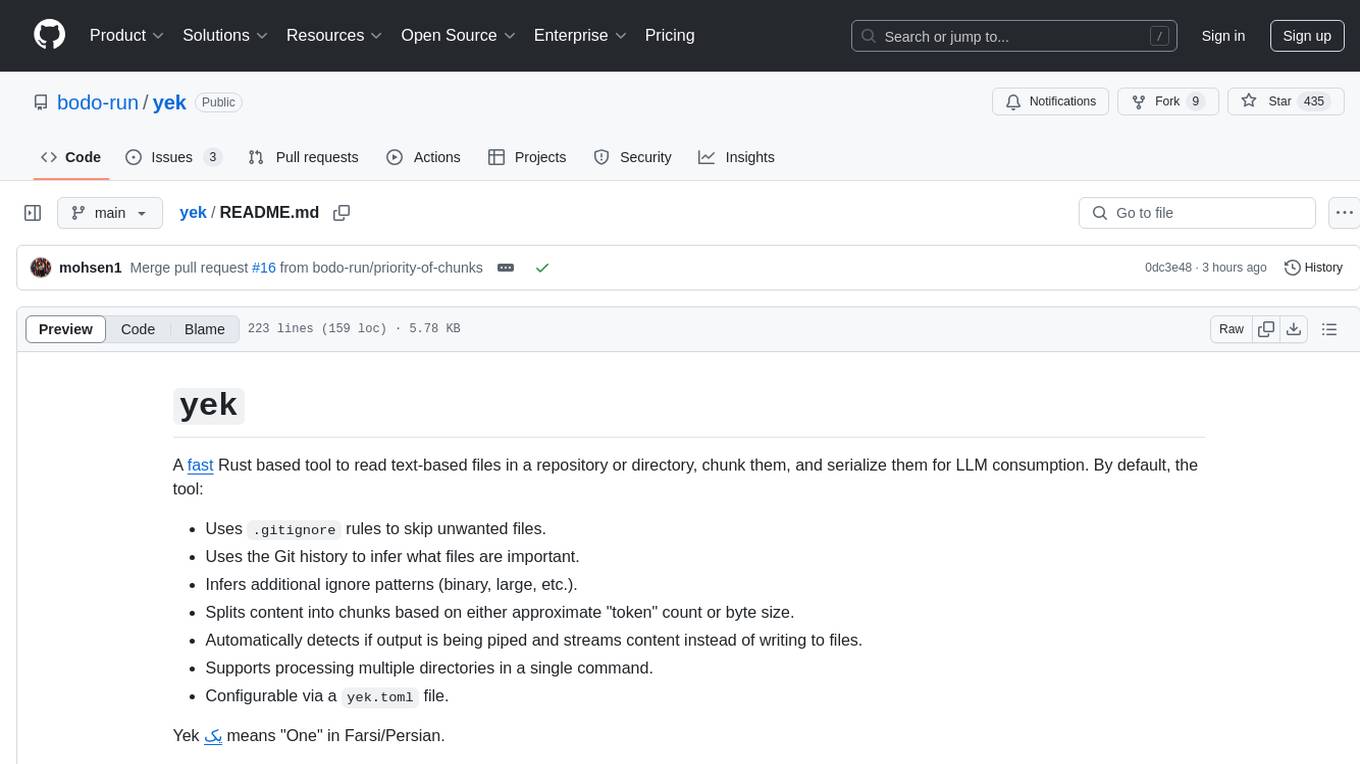
yek
Yek is a fast Rust-based tool designed to read text-based files in a repository or directory, chunk them, and serialize them for Large Language Models (LLM) consumption. It utilizes .gitignore rules to skip unwanted files, Git history to infer important files, and additional ignore patterns. Yek splits content into chunks based on token count or byte size, supports processing multiple directories, and can stream content when output is piped. It is configurable via a 'yek.toml' file and prioritizes important files at the end of the output.
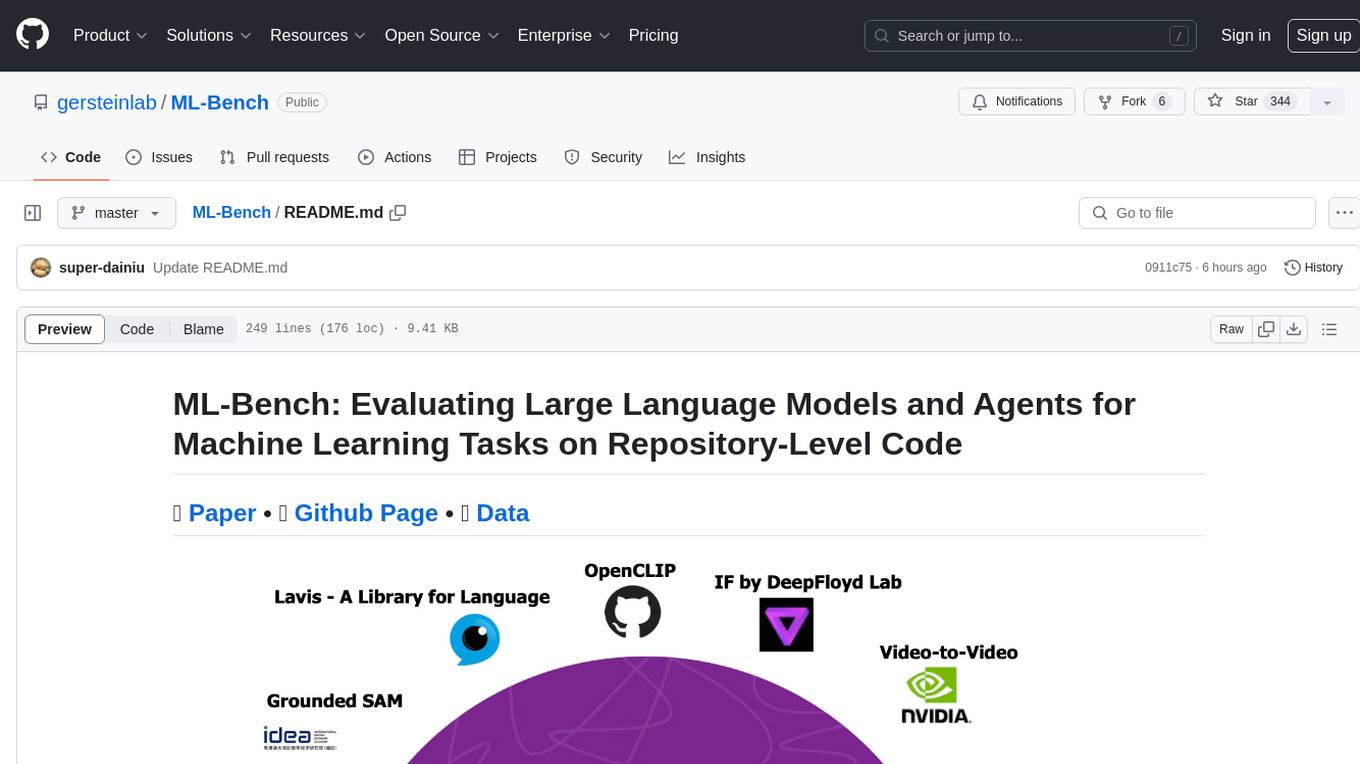
ML-Bench
ML-Bench is a tool designed to evaluate large language models and agents for machine learning tasks on repository-level code. It provides functionalities for data preparation, environment setup, usage, API calling, open source model fine-tuning, and inference. Users can clone the repository, load datasets, run ML-LLM-Bench, prepare data, fine-tune models, and perform inference tasks. The tool aims to facilitate the evaluation of language models and agents in the context of machine learning tasks on code repositories.
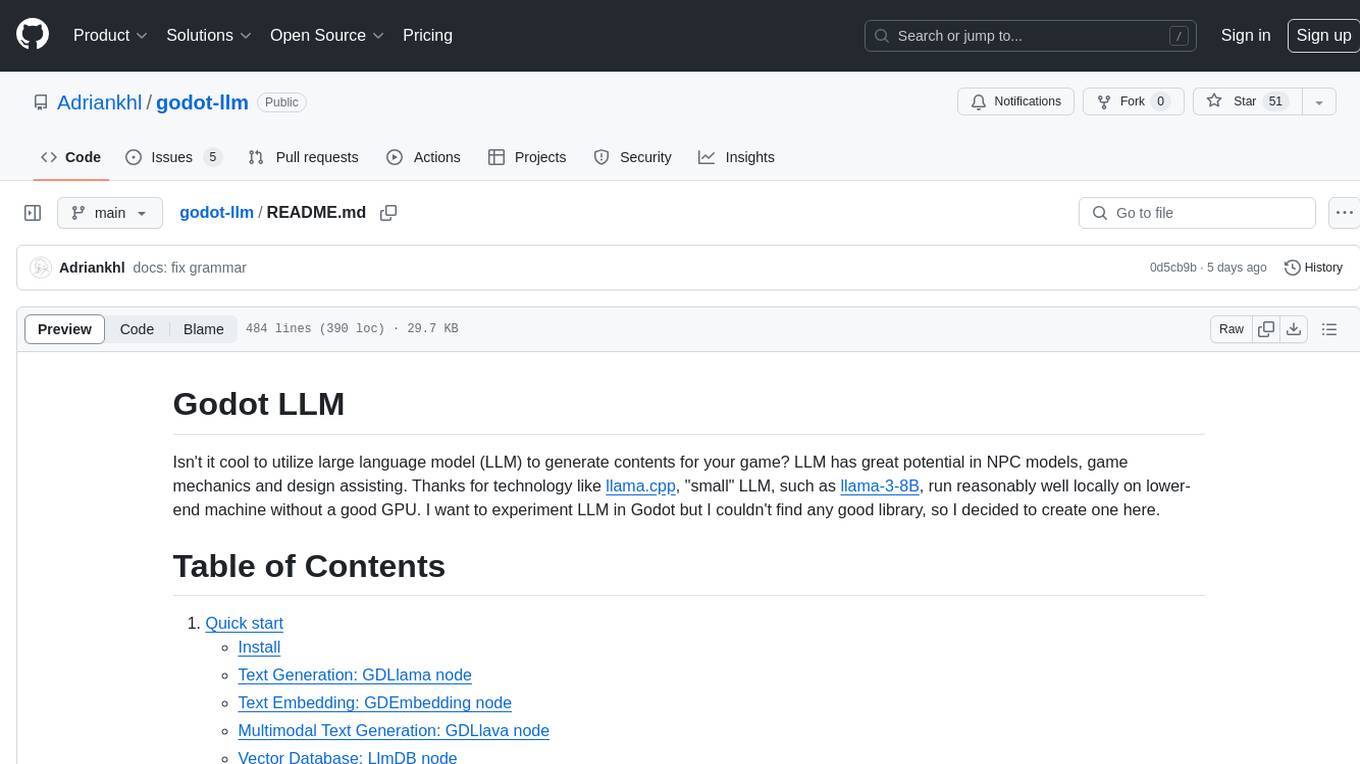
godot-llm
Godot LLM is a plugin that enables the utilization of large language models (LLM) for generating content in games. It provides functionality for text generation, text embedding, multimodal text generation, and vector database management within the Godot game engine. The plugin supports features like Retrieval Augmented Generation (RAG) and integrates llama.cpp-based functionalities for text generation, embedding, and multimodal capabilities. It offers support for various platforms and allows users to experiment with LLM models in their game development projects.
For similar tasks
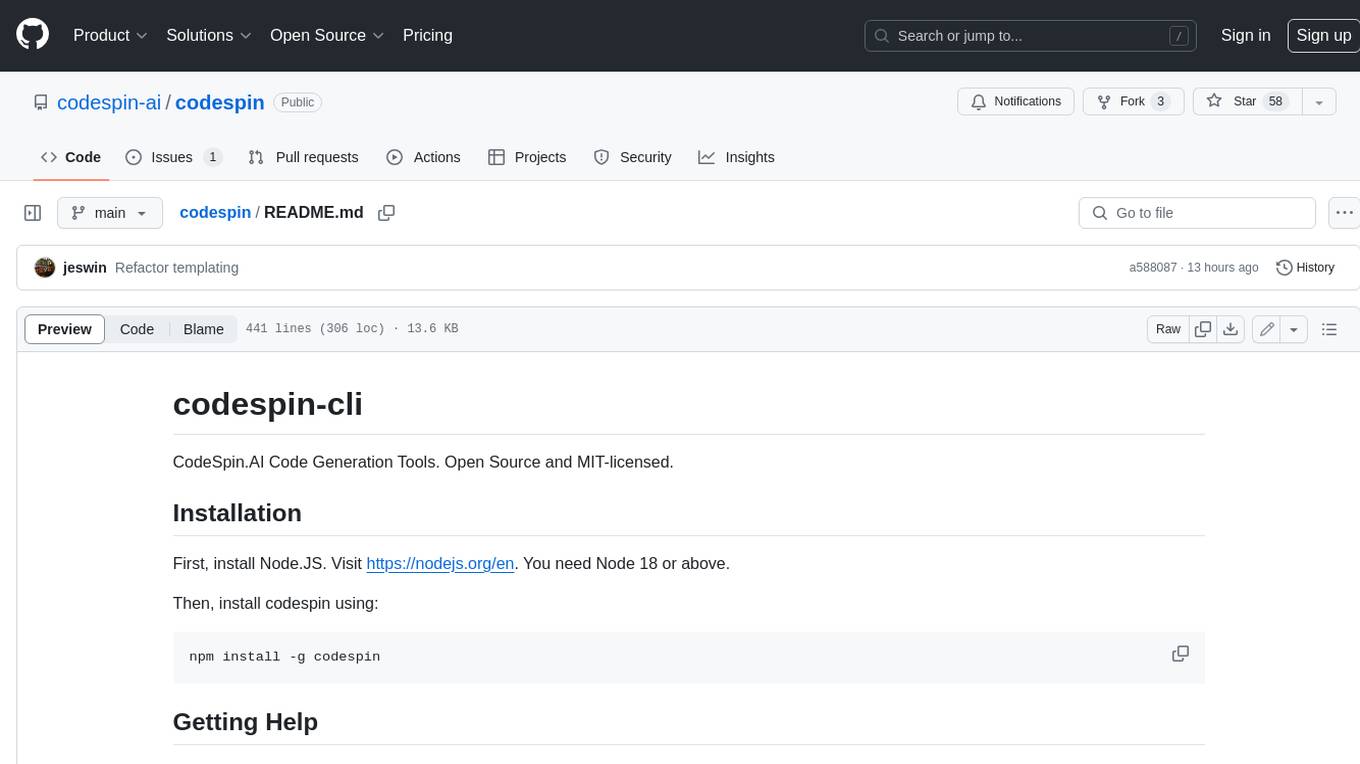
codespin
CodeSpin.AI is a set of open-source code generation tools that leverage large language models (LLMs) to automate coding tasks. With CodeSpin, you can generate code in various programming languages, including Python, JavaScript, Java, and C++, by providing natural language prompts. CodeSpin offers a range of features to enhance code generation, such as custom templates, inline prompting, and the ability to use ChatGPT as an alternative to API keys. Additionally, CodeSpin provides options for regenerating code, executing code in prompt files, and piping data into the LLM for processing. By utilizing CodeSpin, developers can save time and effort in coding tasks, improve code quality, and explore new possibilities in code generation.
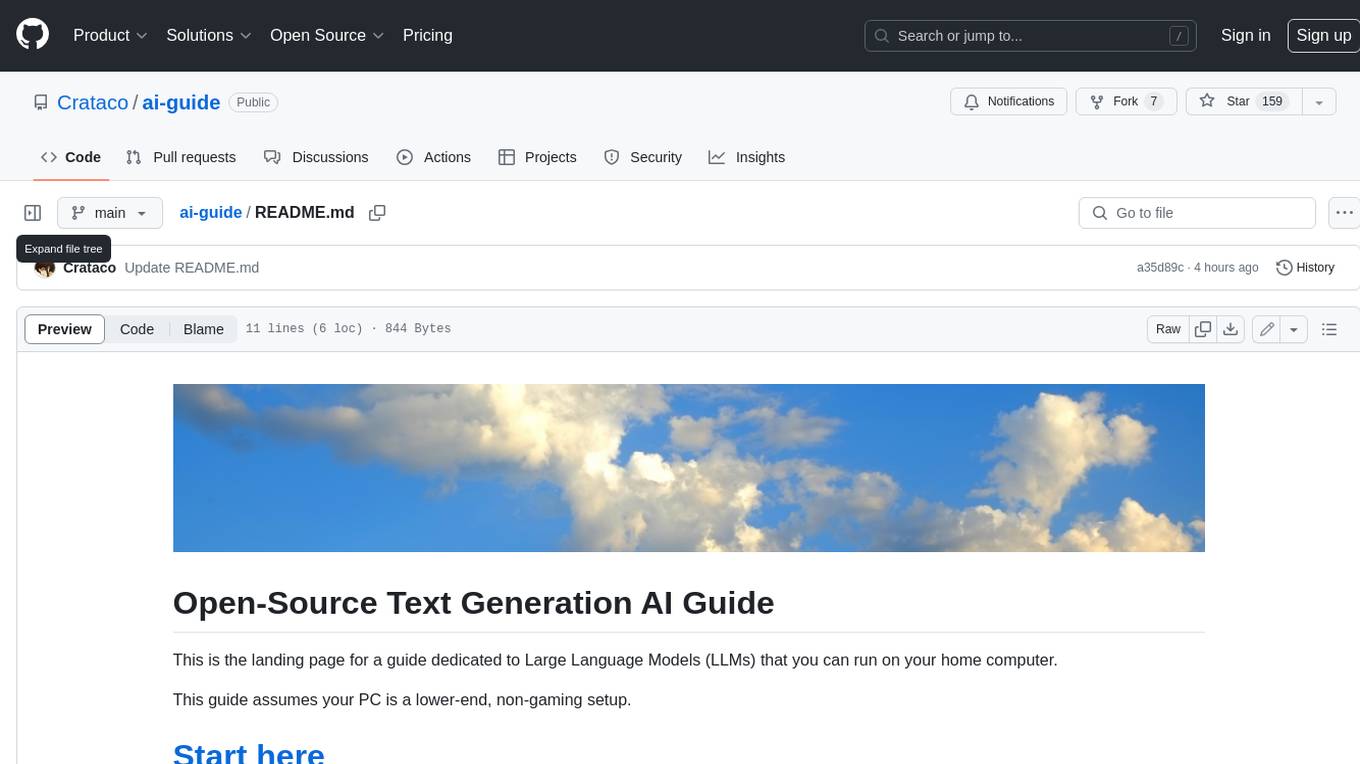
ai-guide
This guide is dedicated to Large Language Models (LLMs) that you can run on your home computer. It assumes your PC is a lower-end, non-gaming setup.
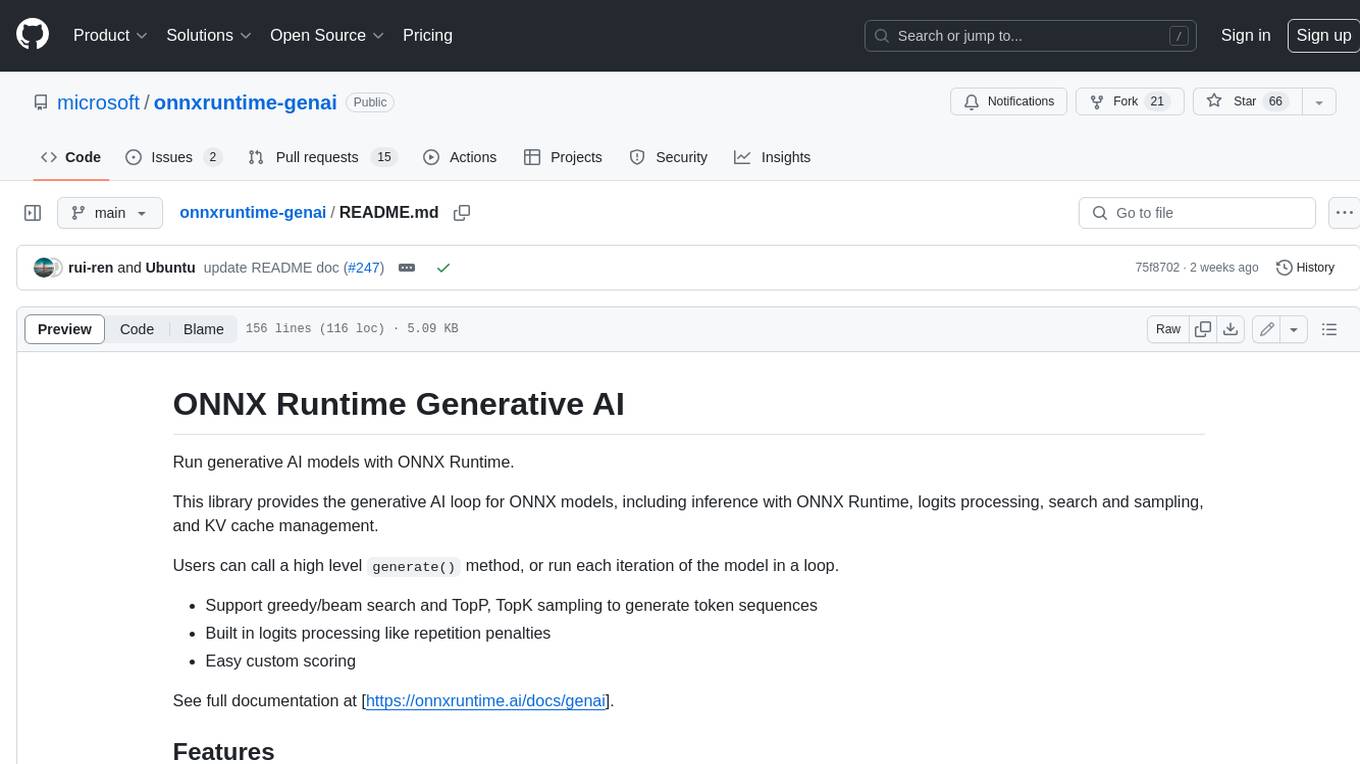
onnxruntime-genai
ONNX Runtime Generative AI is a library that provides the generative AI loop for ONNX models, including inference with ONNX Runtime, logits processing, search and sampling, and KV cache management. Users can call a high level `generate()` method, or run each iteration of the model in a loop. It supports greedy/beam search and TopP, TopK sampling to generate token sequences, has built in logits processing like repetition penalties, and allows for easy custom scoring.
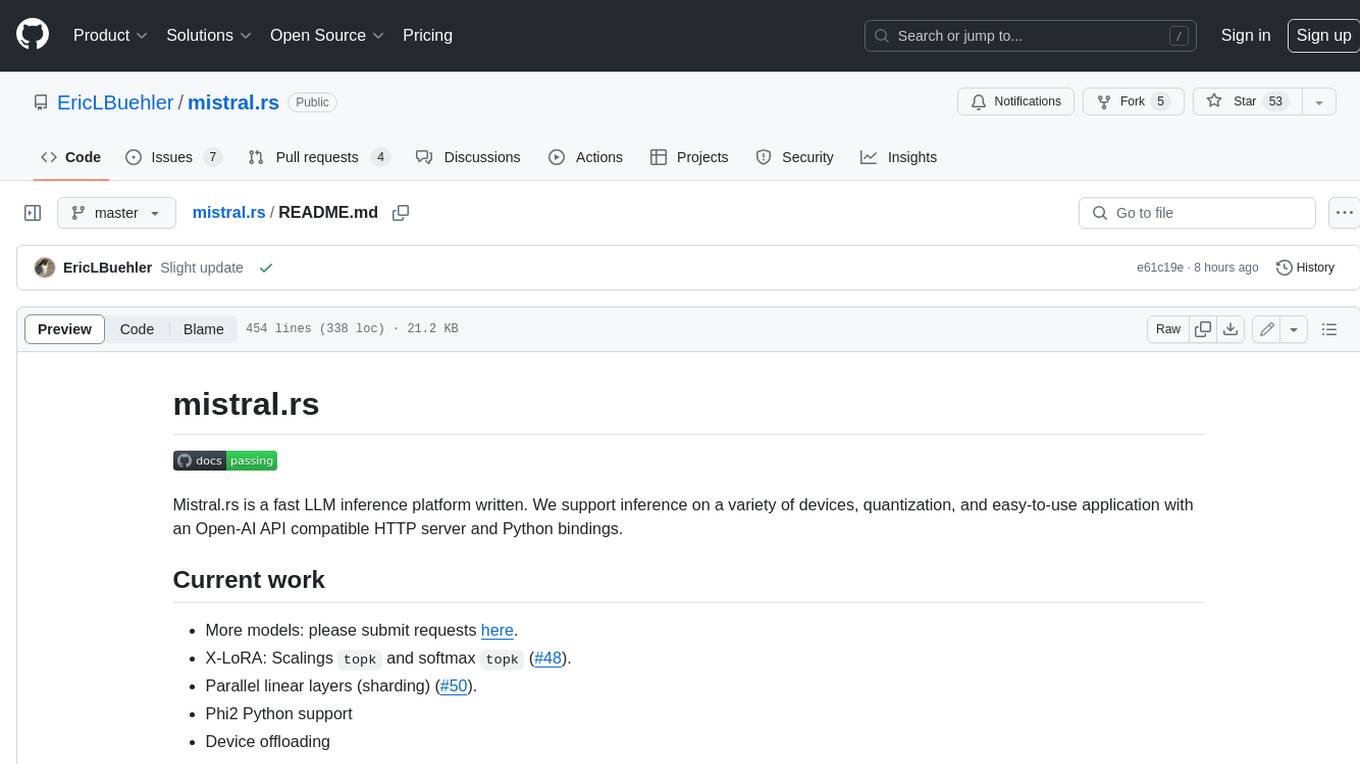
mistral.rs
Mistral.rs is a fast LLM inference platform written in Rust. We support inference on a variety of devices, quantization, and easy-to-use application with an Open-AI API compatible HTTP server and Python bindings.
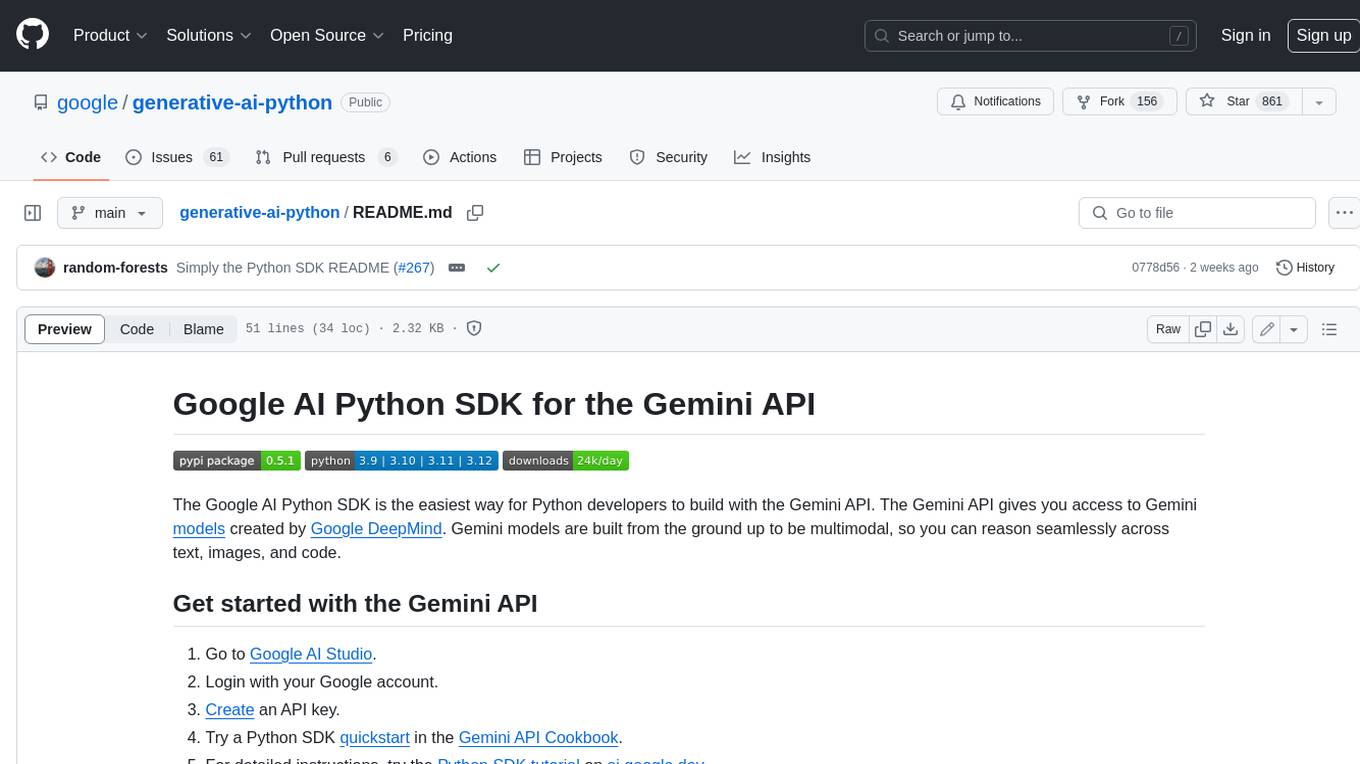
generative-ai-python
The Google AI Python SDK is the easiest way for Python developers to build with the Gemini API. The Gemini API gives you access to Gemini models created by Google DeepMind. Gemini models are built from the ground up to be multimodal, so you can reason seamlessly across text, images, and code.
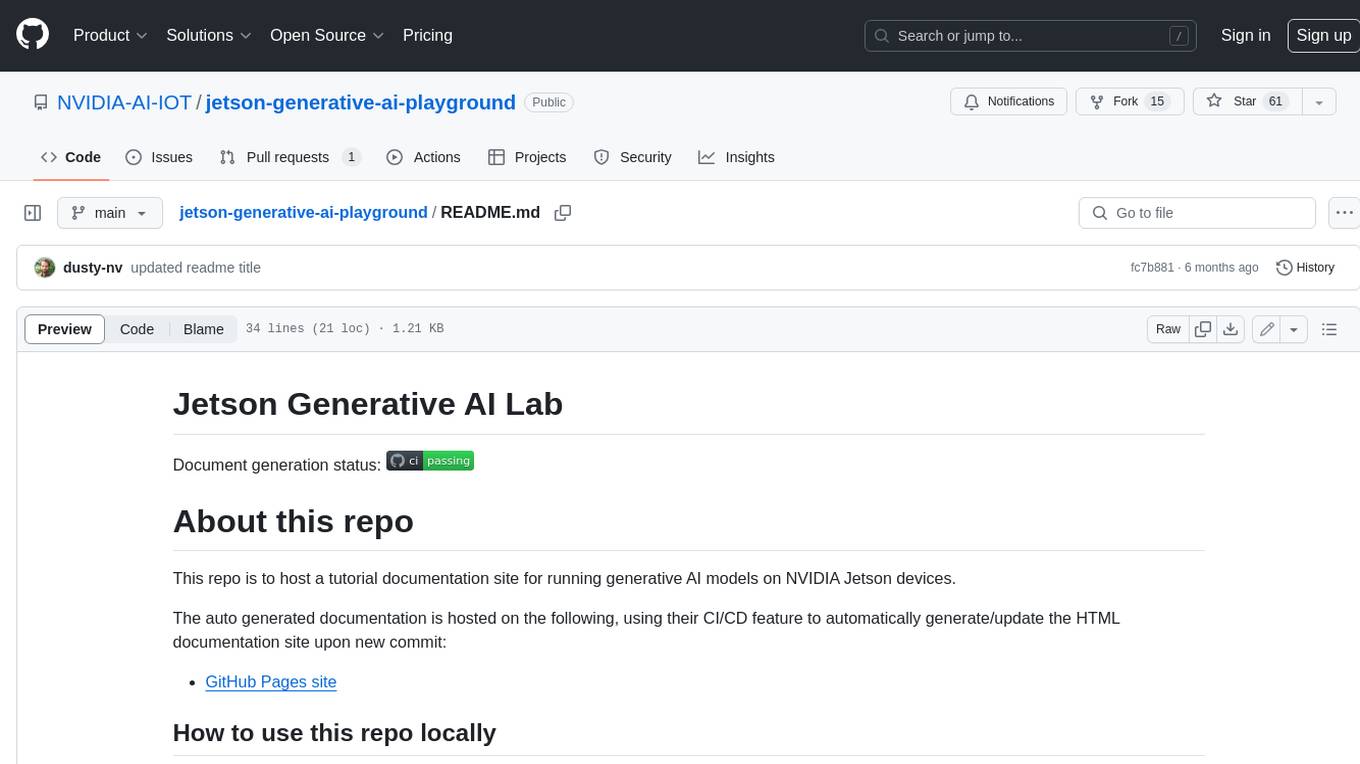
jetson-generative-ai-playground
This repo hosts tutorial documentation for running generative AI models on NVIDIA Jetson devices. The documentation is auto-generated and hosted on GitHub Pages using their CI/CD feature to automatically generate/update the HTML documentation site upon new commits.
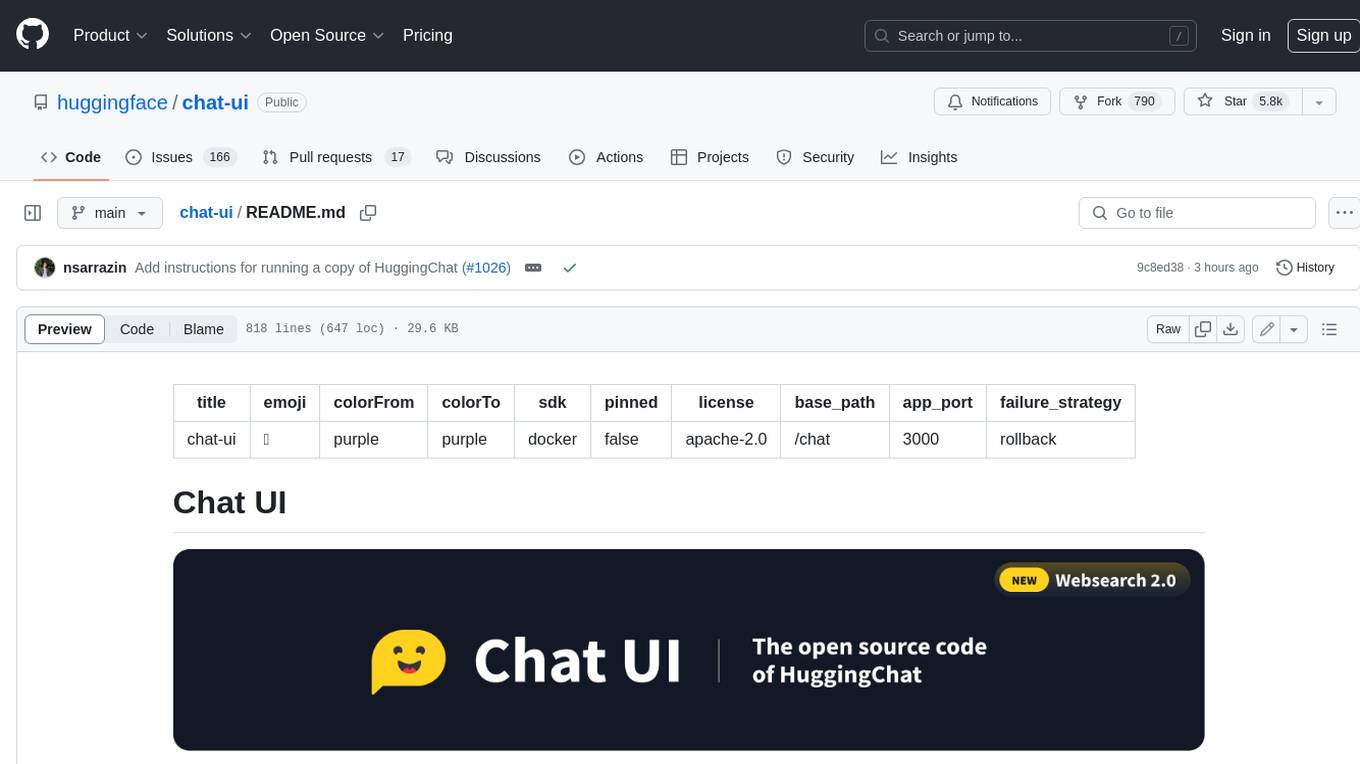
chat-ui
A chat interface using open source models, eg OpenAssistant or Llama. It is a SvelteKit app and it powers the HuggingChat app on hf.co/chat.
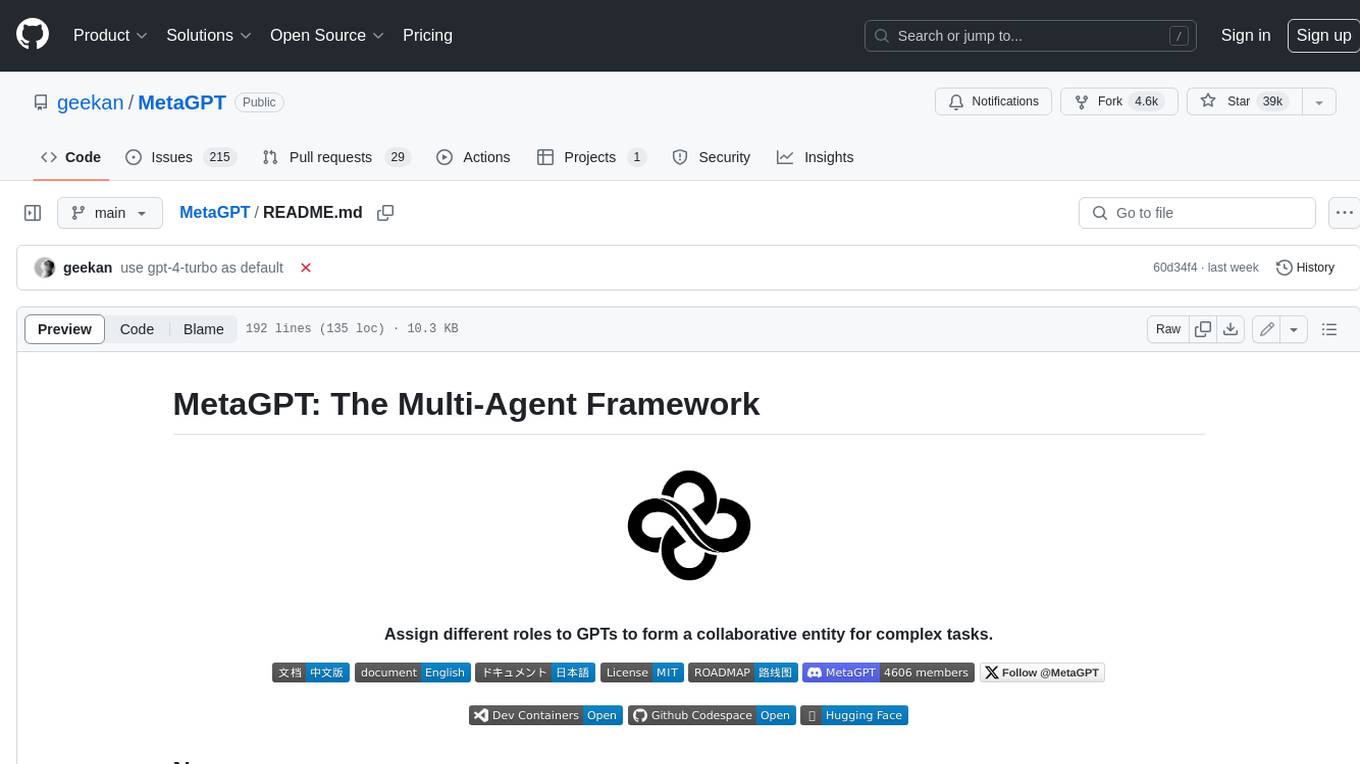
MetaGPT
MetaGPT is a multi-agent framework that enables GPT to work in a software company, collaborating to tackle more complex tasks. It assigns different roles to GPTs to form a collaborative entity for complex tasks. MetaGPT takes a one-line requirement as input and outputs user stories, competitive analysis, requirements, data structures, APIs, documents, etc. Internally, MetaGPT includes product managers, architects, project managers, and engineers. It provides the entire process of a software company along with carefully orchestrated SOPs. MetaGPT's core philosophy is "Code = SOP(Team)", materializing SOP and applying it to teams composed of LLMs.
For similar jobs
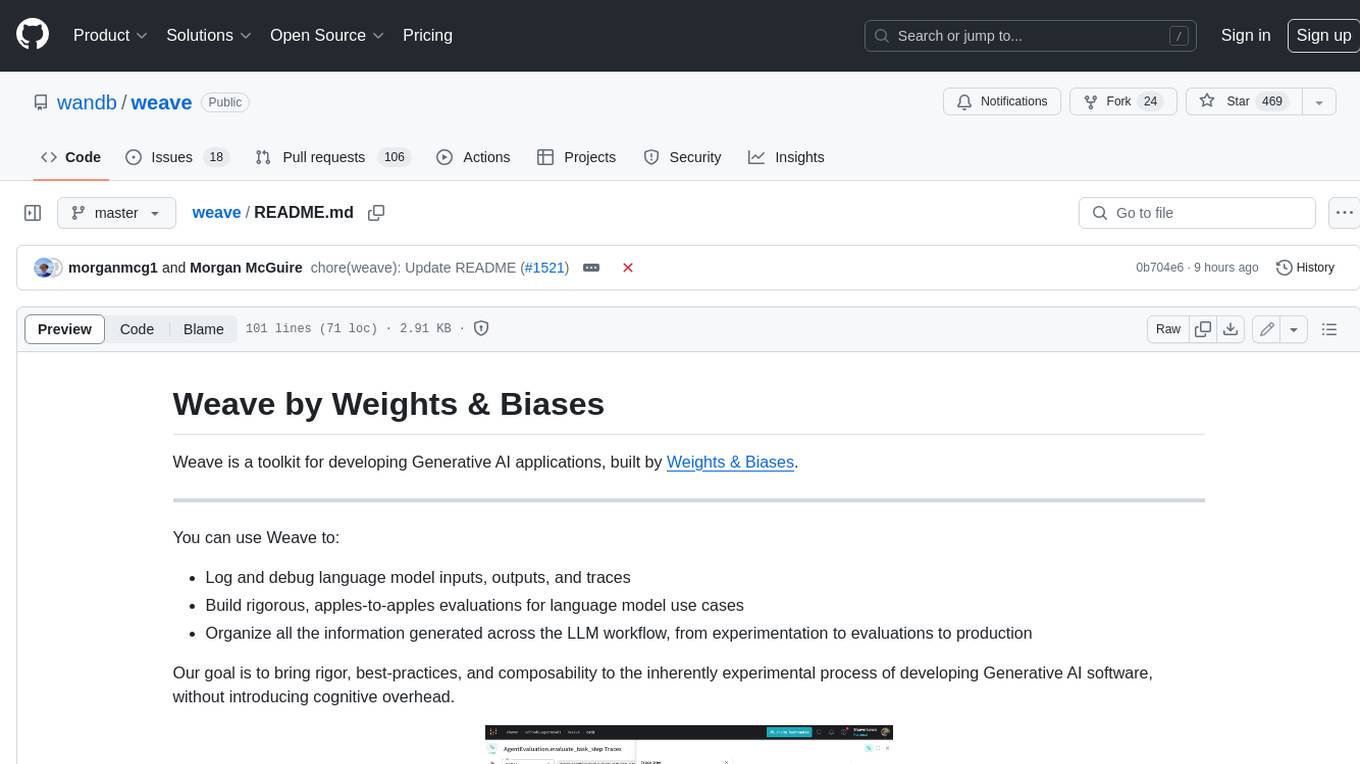
weave
Weave is a toolkit for developing Generative AI applications, built by Weights & Biases. With Weave, you can log and debug language model inputs, outputs, and traces; build rigorous, apples-to-apples evaluations for language model use cases; and organize all the information generated across the LLM workflow, from experimentation to evaluations to production. Weave aims to bring rigor, best-practices, and composability to the inherently experimental process of developing Generative AI software, without introducing cognitive overhead.
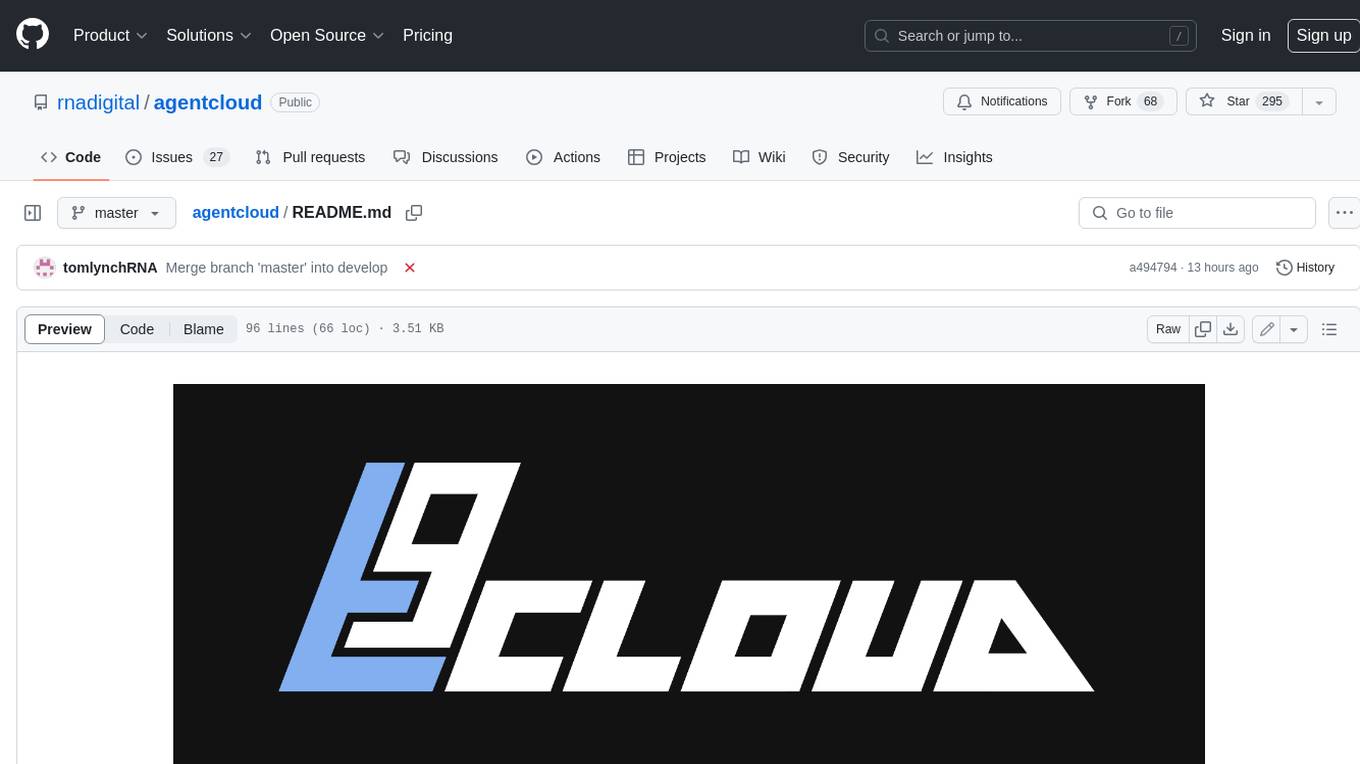
agentcloud
AgentCloud is an open-source platform that enables companies to build and deploy private LLM chat apps, empowering teams to securely interact with their data. It comprises three main components: Agent Backend, Webapp, and Vector Proxy. To run this project locally, clone the repository, install Docker, and start the services. The project is licensed under the GNU Affero General Public License, version 3 only. Contributions and feedback are welcome from the community.
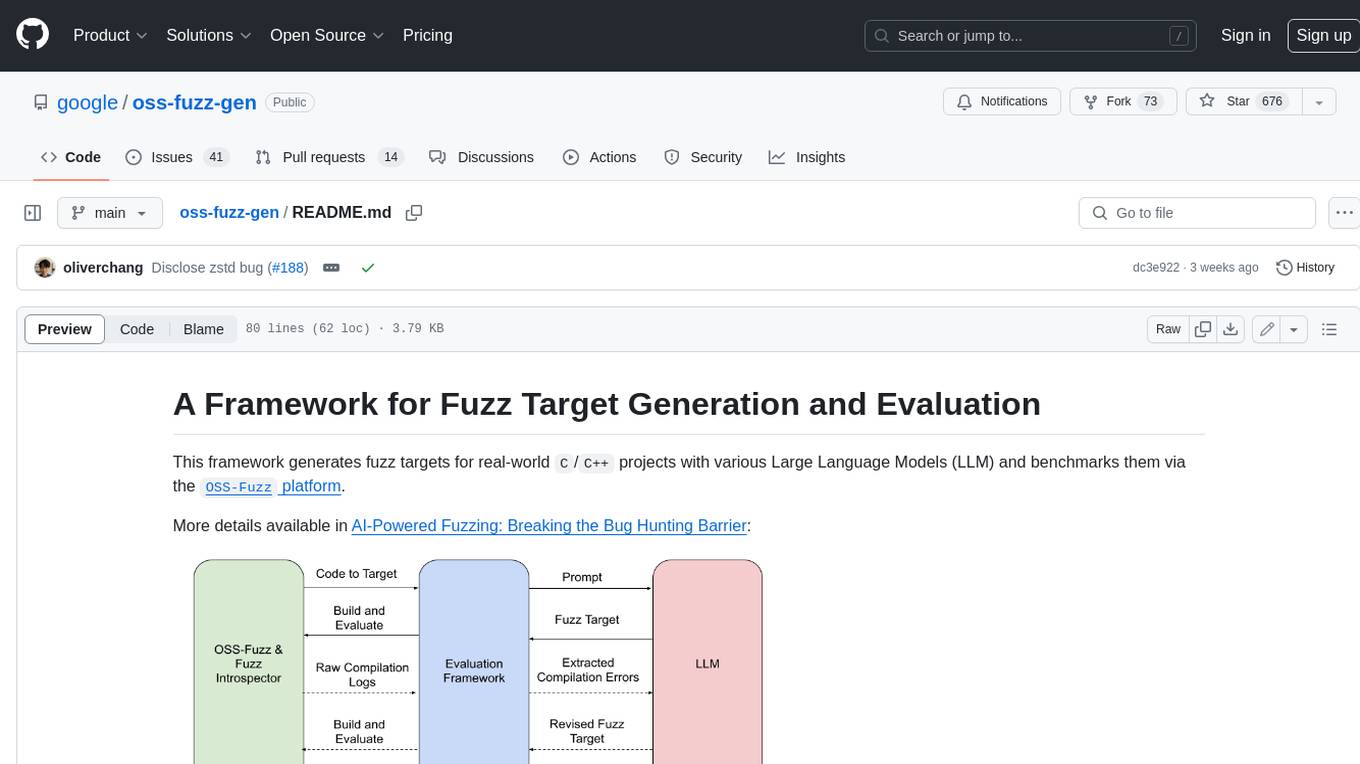
oss-fuzz-gen
This framework generates fuzz targets for real-world `C`/`C++` projects with various Large Language Models (LLM) and benchmarks them via the `OSS-Fuzz` platform. It manages to successfully leverage LLMs to generate valid fuzz targets (which generate non-zero coverage increase) for 160 C/C++ projects. The maximum line coverage increase is 29% from the existing human-written targets.
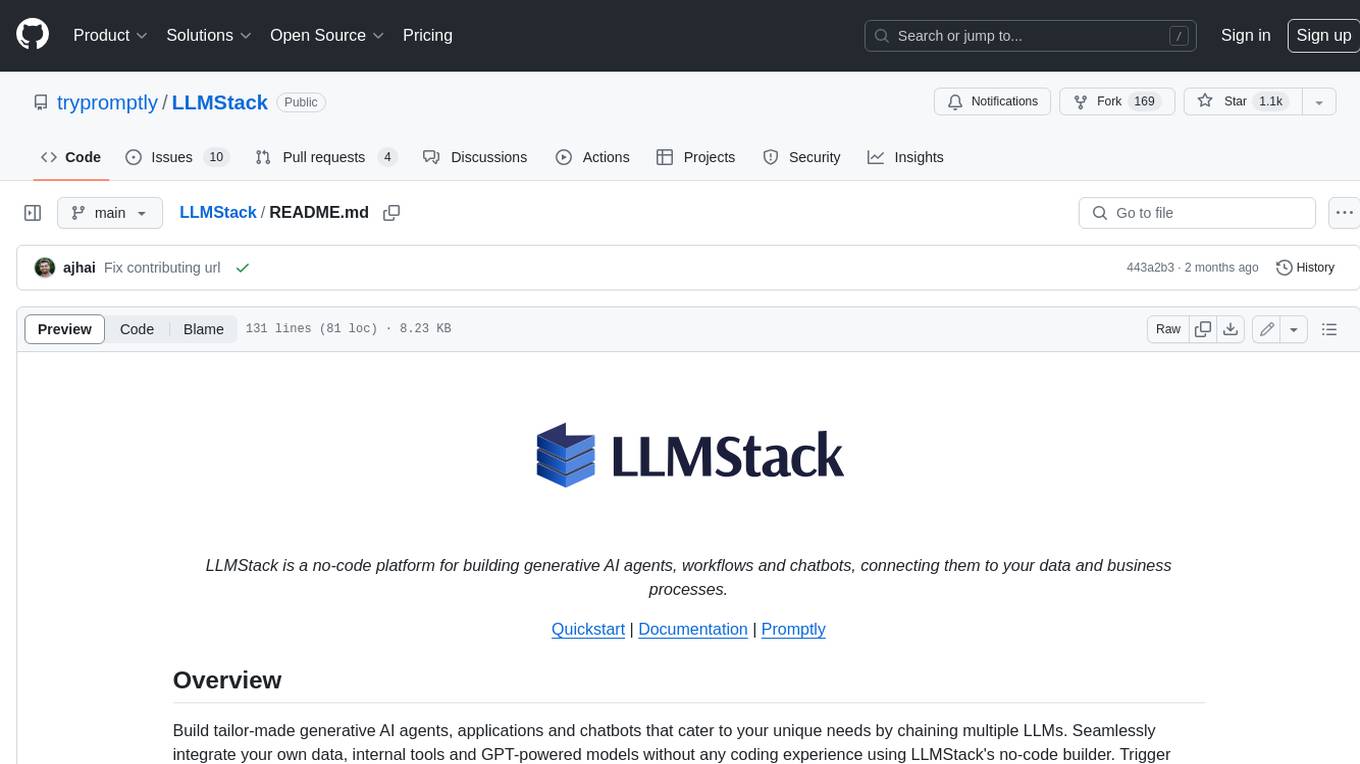
LLMStack
LLMStack is a no-code platform for building generative AI agents, workflows, and chatbots. It allows users to connect their own data, internal tools, and GPT-powered models without any coding experience. LLMStack can be deployed to the cloud or on-premise and can be accessed via HTTP API or triggered from Slack or Discord.
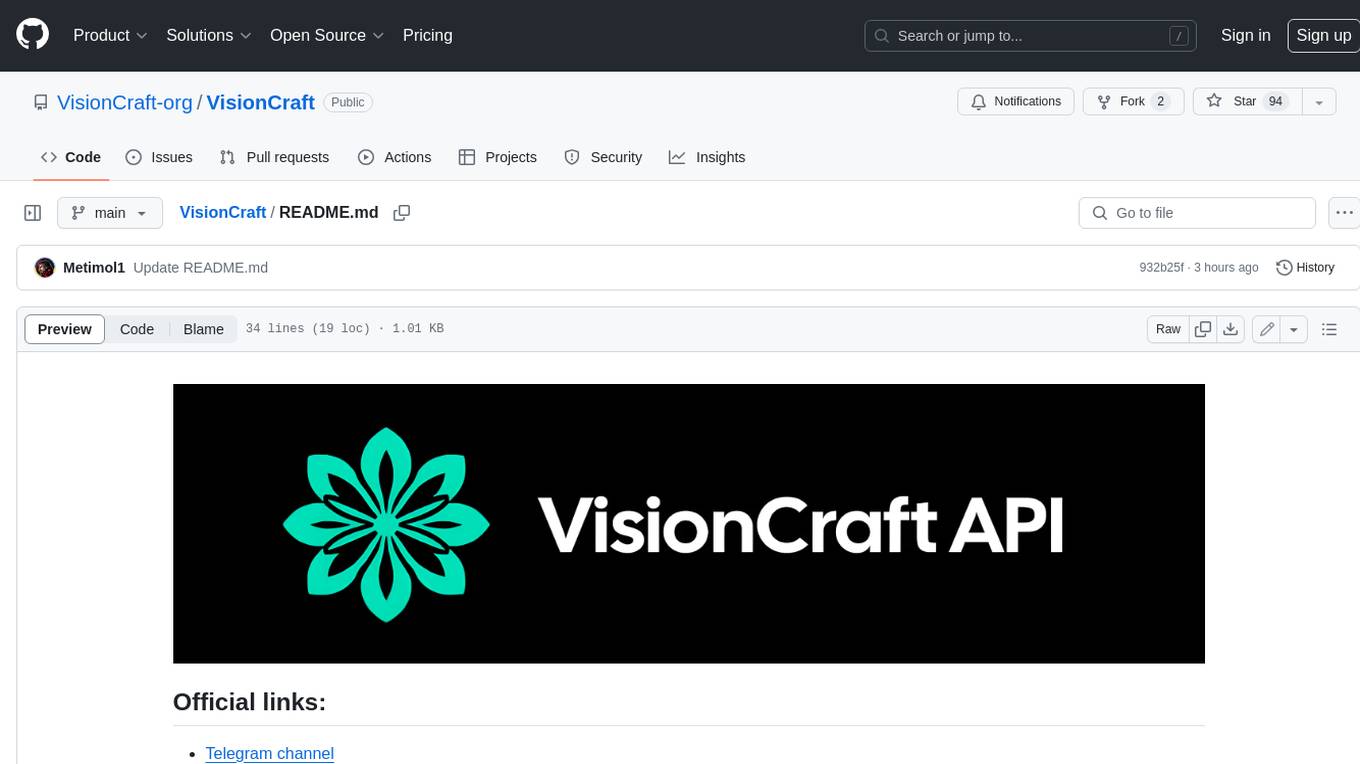
VisionCraft
The VisionCraft API is a free API for using over 100 different AI models. From images to sound.
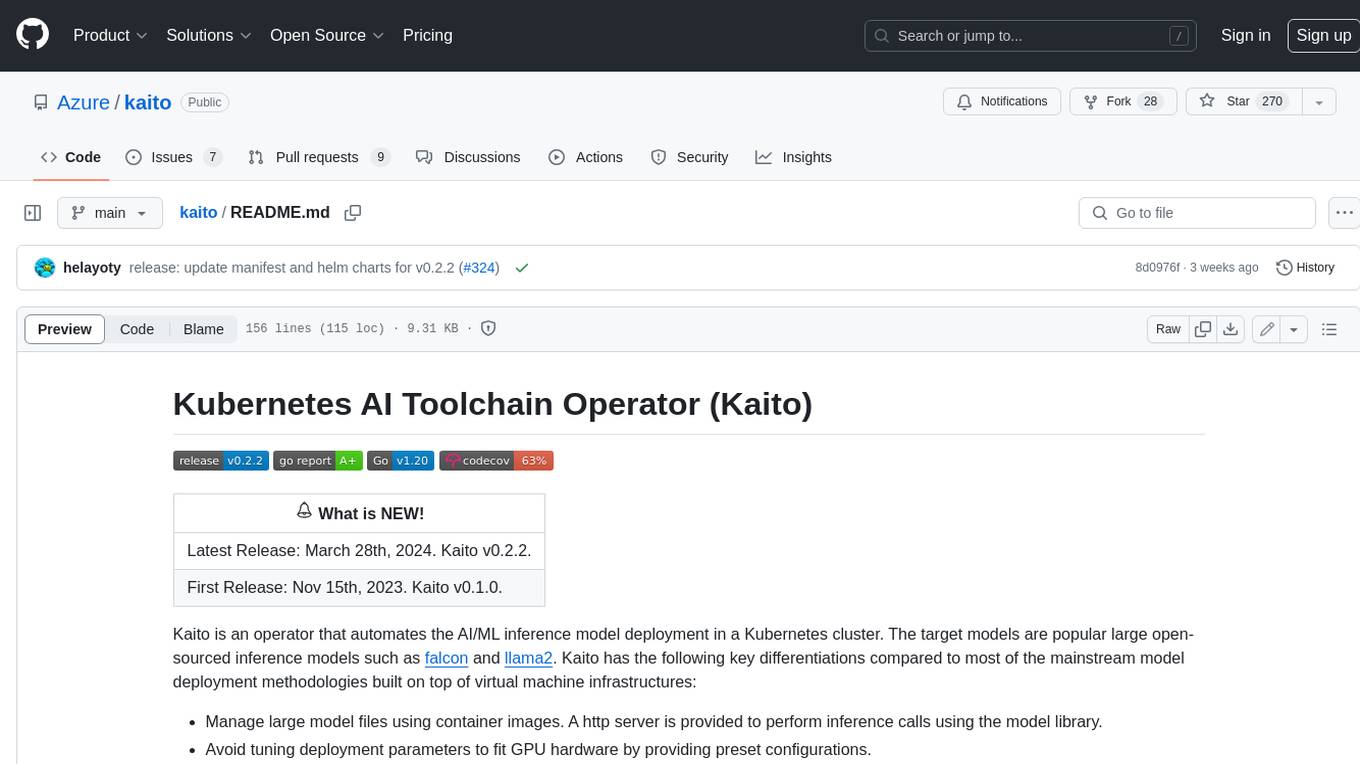
kaito
Kaito is an operator that automates the AI/ML inference model deployment in a Kubernetes cluster. It manages large model files using container images, avoids tuning deployment parameters to fit GPU hardware by providing preset configurations, auto-provisions GPU nodes based on model requirements, and hosts large model images in the public Microsoft Container Registry (MCR) if the license allows. Using Kaito, the workflow of onboarding large AI inference models in Kubernetes is largely simplified.
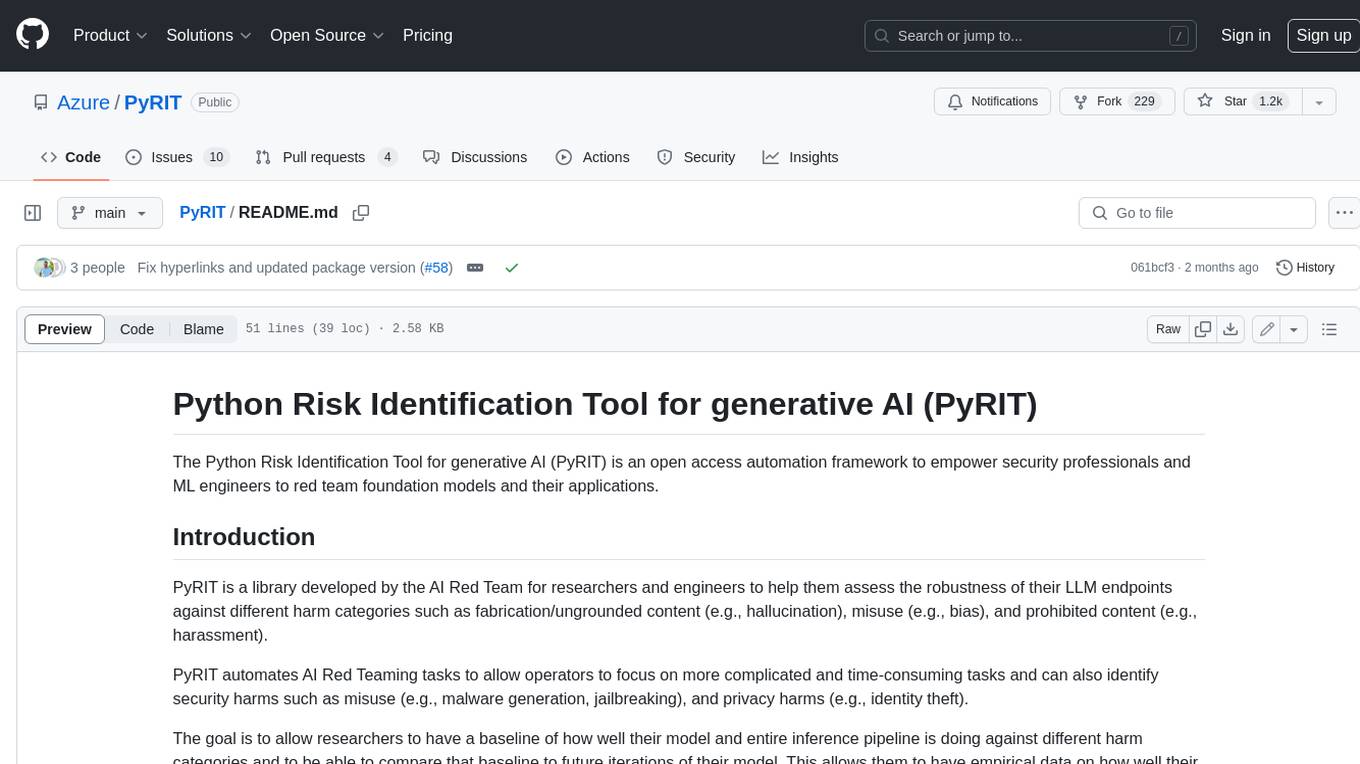
PyRIT
PyRIT is an open access automation framework designed to empower security professionals and ML engineers to red team foundation models and their applications. It automates AI Red Teaming tasks to allow operators to focus on more complicated and time-consuming tasks and can also identify security harms such as misuse (e.g., malware generation, jailbreaking), and privacy harms (e.g., identity theft). The goal is to allow researchers to have a baseline of how well their model and entire inference pipeline is doing against different harm categories and to be able to compare that baseline to future iterations of their model. This allows them to have empirical data on how well their model is doing today, and detect any degradation of performance based on future improvements.
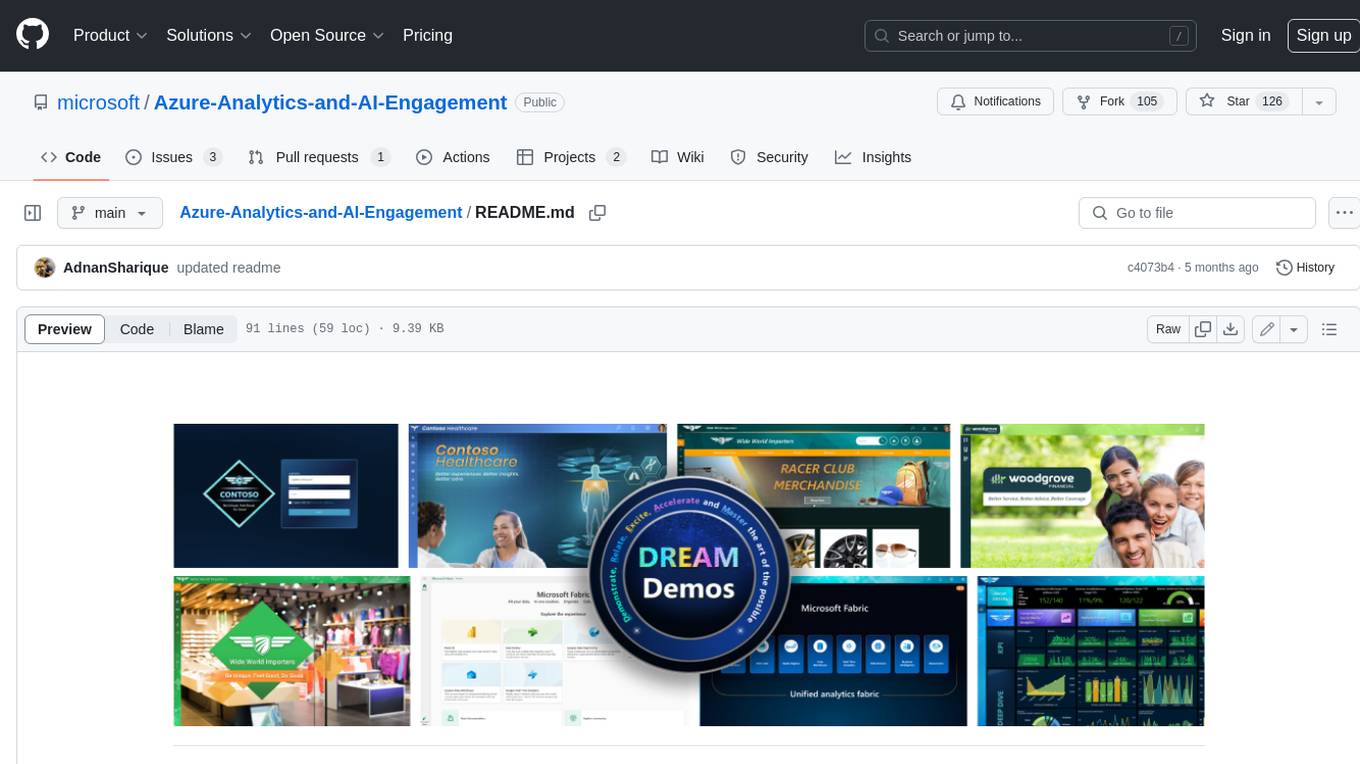
Azure-Analytics-and-AI-Engagement
The Azure-Analytics-and-AI-Engagement repository provides packaged Industry Scenario DREAM Demos with ARM templates (Containing a demo web application, Power BI reports, Synapse resources, AML Notebooks etc.) that can be deployed in a customer’s subscription using the CAPE tool within a matter of few hours. Partners can also deploy DREAM Demos in their own subscriptions using DPoC.