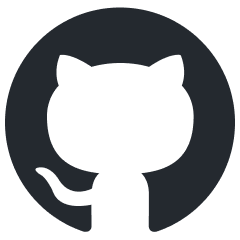
turnkeyml
The no-code AI toolchain
Stars: 58
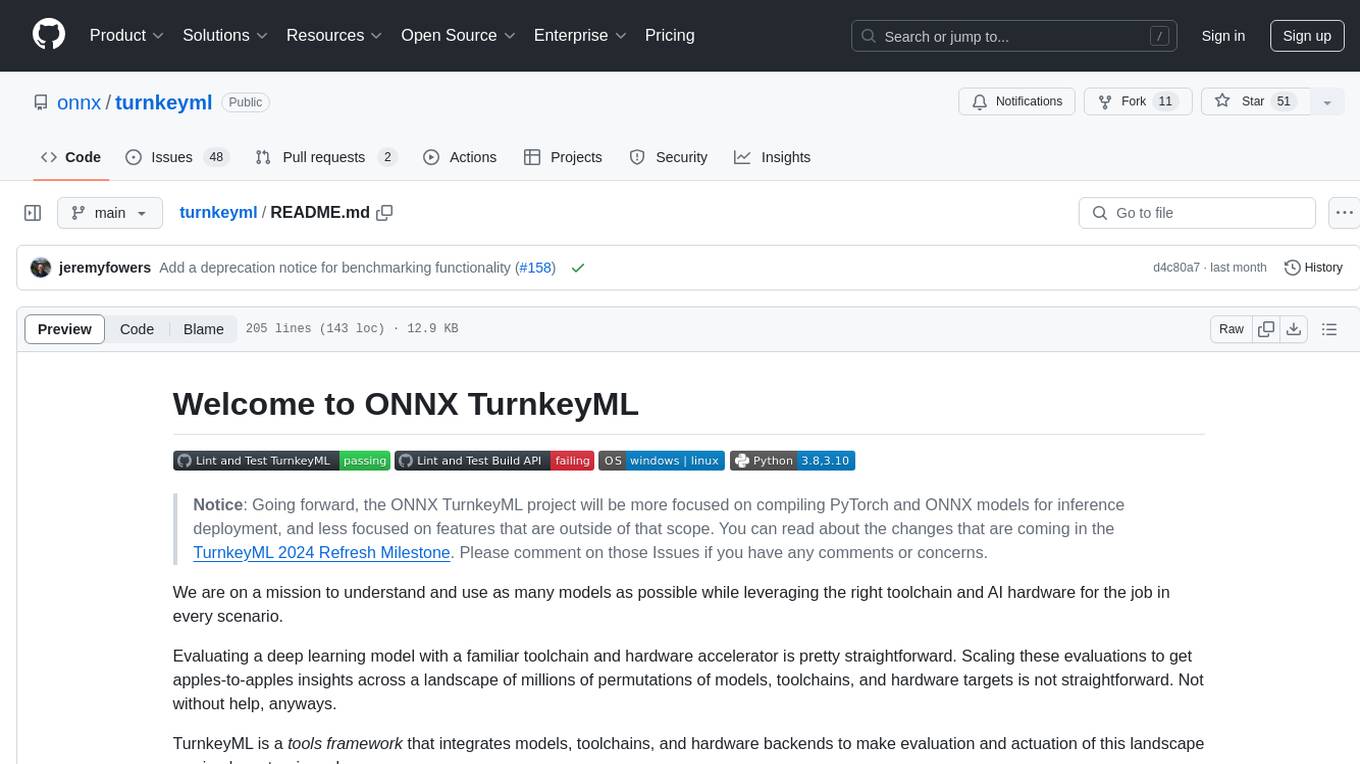
TurnkeyML is a tools framework that integrates models, toolchains, and hardware backends to simplify the evaluation and actuation of deep learning models. It supports use cases like exporting ONNX files, performance validation, functional coverage measurement, stress testing, and model insights analysis. The framework consists of analysis, build, runtime, reporting tools, and a models corpus, seamlessly integrated to provide comprehensive functionality with simple commands. Extensible through plugins, it offers support for various export and optimization tools and AI runtimes. The project is actively seeking collaborators and is licensed under Apache 2.0.
README:
We are on a mission to make it easy to use the most important tools in the ONNX ecosystem. TurnkeyML accomplishes this by providing a no-code CLI, turnkey
, as well as a low-code API, that provide seamless integration of these tools.
We also provide turnkey-llm
, which has LLM-specific tools for prompting, accuracy measurement, and serving on a variety of runtimes (Huggingface, onnxruntime-genai) and hardware (CPU, GPU, and NPU).
The easiest way to get started is:
pip install turnkeyml
- Copy a PyTorch example of a model, like the one on this Huggingface BERT model card, into a file named
bert.py
.
from transformers import BertTokenizer, BertModel
tokenizer = BertTokenizer.from_pretrained('bert-base-uncased')
model = BertModel.from_pretrained("bert-base-uncased")
text = "Replace me by any text you'd like."
encoded_input = tokenizer(text, return_tensors='pt')
output = model(**encoded_input)
-
turnkey -i bert.py discover export-pytorch
: make a BERT ONNX file from thisbert.py
example.
For LLM setup instructions, see turnkey-llm
.
Here's turnkey
in action: BERT-Base is exported from PyTorch to ONNX using torch.onnx.export
, optimized for inference with onnxruntime
, and converted to fp16 with onnxmltools
:
Breaking down the command turnkey -i bert.py discover export-pytorch optimize-ort convert-fp16
:
-
turnkey -i bert.py
feedsbert.py
, a minimal PyTorch script that instantiates BERT, into the tool sequence, starting with... -
discover
is a tool that finds the PyTorch model in a script and passes it to the next tool, which is... -
export-pytorch
, which takes a PyTorch model and converts it to an ONNX model, then passes it to... -
optimize-ort
, which usesonnxruntime
to optimize the model's compute graph, then passes it to... -
convert-fp16
, which usesonnxmltools
to convert the ONNX file into fp16. - Finally, the result is printed, and we can see that the requested
.onnx
files have been produced.
All without writing a single line of code or learning how to use any of the underlying ONNX ecosystem tools 🚀
The turnkey
CLI provides a set of Tools
that users can invoke in a Sequence
. The first Tool
takes the input (-i
), performs some action, and passes its state to the next Tool
in the Sequence
.
You can read the Sequence
out like a sentence. For example, the demo command above was:
> turnkey -i bert.py discover export-pytorch optimize-ort convert-fp16
Which you can read like:
Use
turnkey
onbert.py
todiscover
the model,export
thepytorch
to ONNX,optimize
the ONNX withort
, andconvert
the ONNX tofp16
.
You can configure each Tool
by passing it arguments. For example, export-pytorch --opset 18
would set the opset of the resulting ONNX model to 18.
A full command with an argument looks like:
> turnkey -i bert.py discover export-pytorch --opset 18 optimize-ort conver-fp16
The easiest way to learn more about turnkey
is to explore the help menu with turnkey -h
. To learn about a specific tool, run turnkey <tool name> -h
, for example turnkey export-pytorch -h
.
We also provide the following resources:
- Installation guide: how to install from source, set up Slurm, etc.
-
User guide: explains the concepts of
turnkey's
, including the syntax for making your own tool sequence. -
Examples: PyTorch scripts and ONNX files that can be used to try out
turnkey
concepts. - Code organization guide: learn how this repository is structured.
-
Models: PyTorch model scripts that work with
turnkey
.
turnkey
is used in multiple projects where many hundreds of models are being evaluated. For example, the ONNX Model Zoo was created using turnkey
.
We provide several helpful tools to facilitate this kind of mass-evaluation.
turnkey
will iterate over multiple inputs if you pass it a wildcard input.
For example, to export ~1000 built-in models to ONNX:
> turnkey models/*/*.py discover export-pytorch
All build results, such as .onnx
files, are collected into a cache directory, which you can learn about with turnkey cache -h
.
turnkey
collects statistics about each model and build into the corresponding build directory in the cache. Use turnkey report -h
to see how those statistics can be exported into a CSV file.
This repository is home to a diverse corpus of hundreds of models, which are meant to be a convenient input to turnkey -i <model>.py discover
. We are actively working on increasing the number of models in our model library. You can see the set of models in each category by clicking on the corresponding badge.
Evaluating a new model is as simple as taking a Python script that instantiates and invokes a PyTorch torch.nn.module
and call turnkey
on it. Read about model contributions here.
The build tool has built-in support for a variety of interoperable Tools
. If you need more, the TurnkeyML plugin API lets you add your own installable tools with any functionality you like:
> pip install -e my_custom_plugin
> turnkey -i my_model.py discover export-pytorch my-custom-tool --my-args
All of the built-in Tools
are implemented against the plugin API. Check out the example plugins and the plugin API guide to learn more about creating an installable plugin.
We are actively seeking collaborators from across the industry. If you would like to contribute to this project, please check out our contribution guide.
This project is sponsored by the ONNX Model Zoo special interest group (SIG). It is maintained by @danielholanda @jeremyfowers @ramkrishna @vgodsoe in equal measure. You can reach us by filing an issue.
This project is licensed under the Apache 2.0 License.
TurnkeyML used code from other open source projects as a starting point (see NOTICE.md). Thank you Philip Colangelo, Derek Elkins, Jeremy Fowers, Dan Gard, Victoria Godsoe, Mark Heaps, Daniel Holanda, Brian Kurtz, Mariah Larwood, Philip Lassen, Andrew Ling, Adrian Macias, Gary Malik, Sarah Massengill, Ashwin Murthy, Hatice Ozen, Tim Sears, Sean Settle, Krishna Sivakumar, Aviv Weinstein, Xueli Xao, Bill Xing, and Lev Zlotnik for your contributions to that work.
For Tasks:
Click tags to check more tools for each tasksFor Jobs:
Alternative AI tools for turnkeyml
Similar Open Source Tools
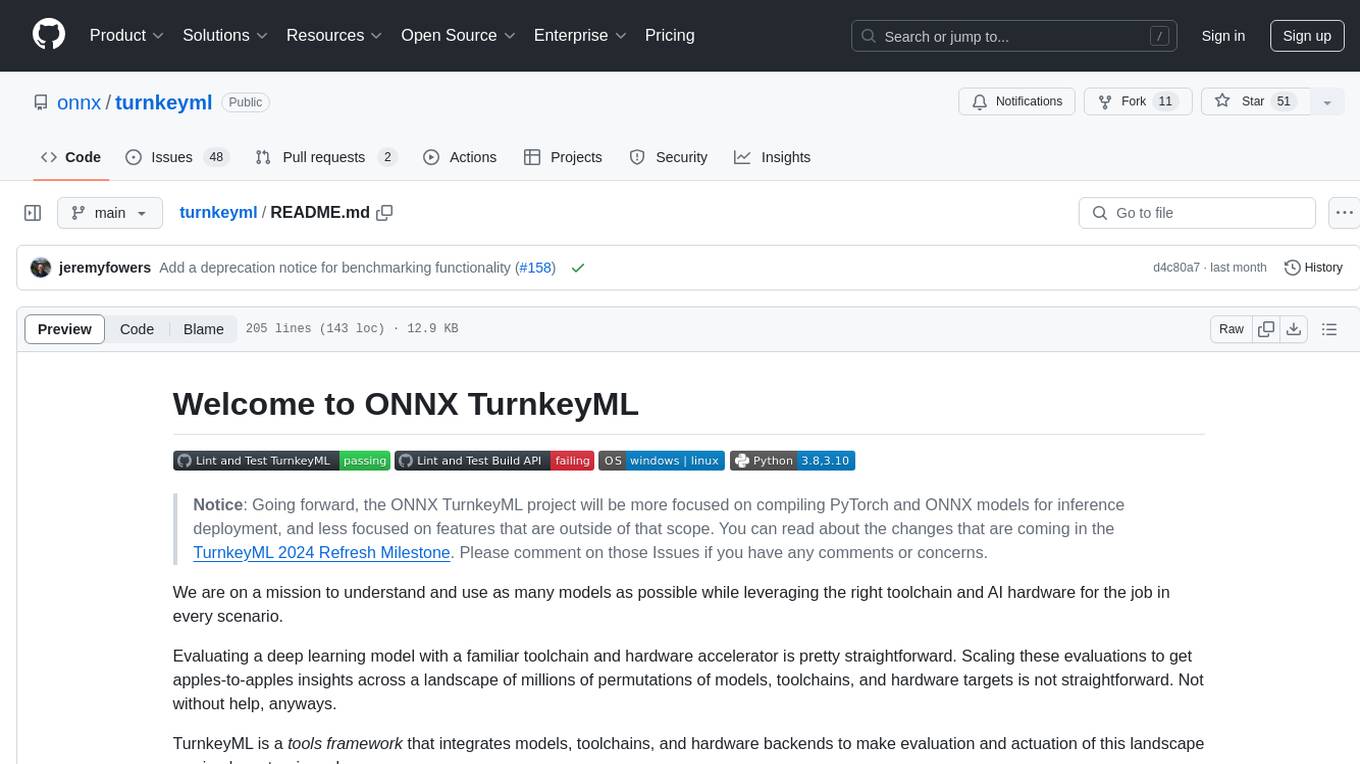
turnkeyml
TurnkeyML is a tools framework that integrates models, toolchains, and hardware backends to simplify the evaluation and actuation of deep learning models. It supports use cases like exporting ONNX files, performance validation, functional coverage measurement, stress testing, and model insights analysis. The framework consists of analysis, build, runtime, reporting tools, and a models corpus, seamlessly integrated to provide comprehensive functionality with simple commands. Extensible through plugins, it offers support for various export and optimization tools and AI runtimes. The project is actively seeking collaborators and is licensed under Apache 2.0.
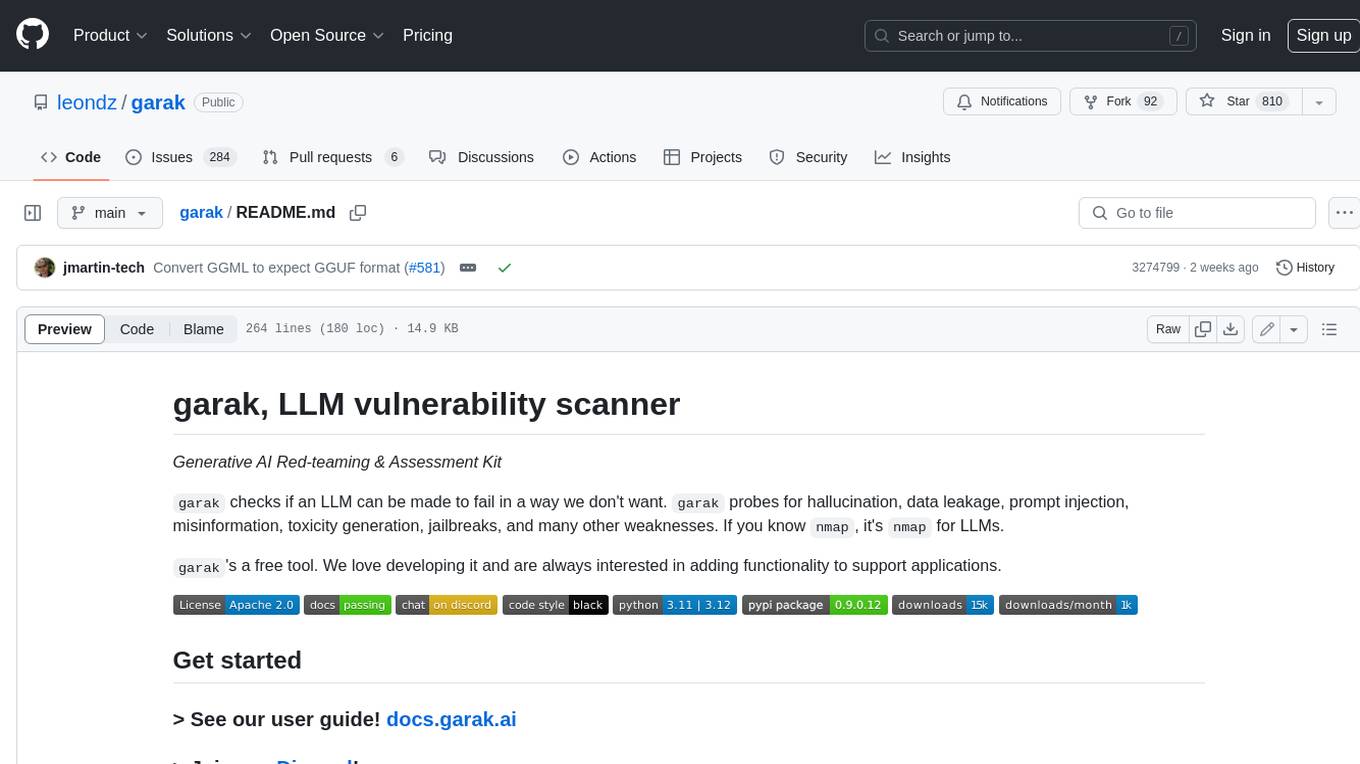
garak
Garak is a free tool that checks if a Large Language Model (LLM) can be made to fail in a way that is undesirable. It probes for hallucination, data leakage, prompt injection, misinformation, toxicity generation, jailbreaks, and many other weaknesses. Garak's a free tool. We love developing it and are always interested in adding functionality to support applications.
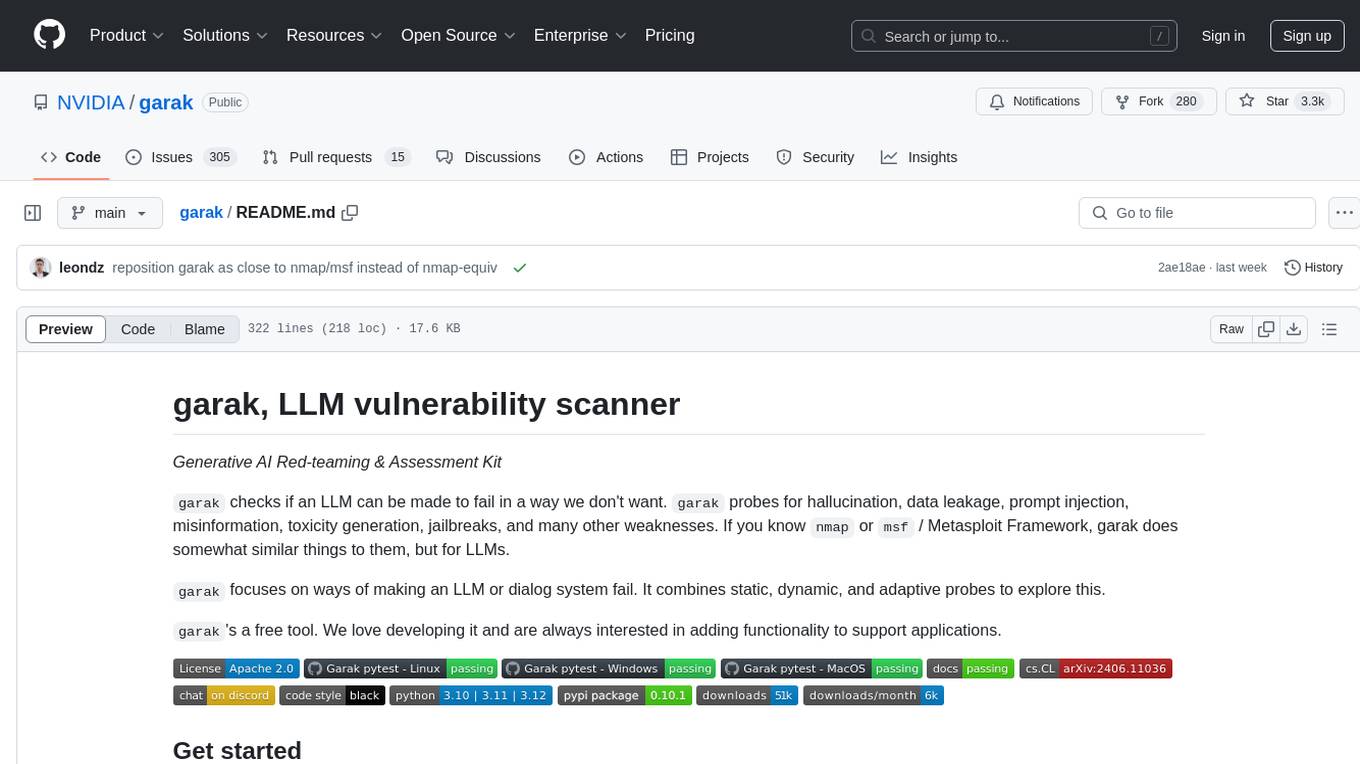
garak
Garak is a vulnerability scanner designed for LLMs (Large Language Models) that checks for various weaknesses such as hallucination, data leakage, prompt injection, misinformation, toxicity generation, and jailbreaks. It combines static, dynamic, and adaptive probes to explore vulnerabilities in LLMs. Garak is a free tool developed for red-teaming and assessment purposes, focusing on making LLMs or dialog systems fail. It supports various LLM models and can be used to assess their security and robustness.
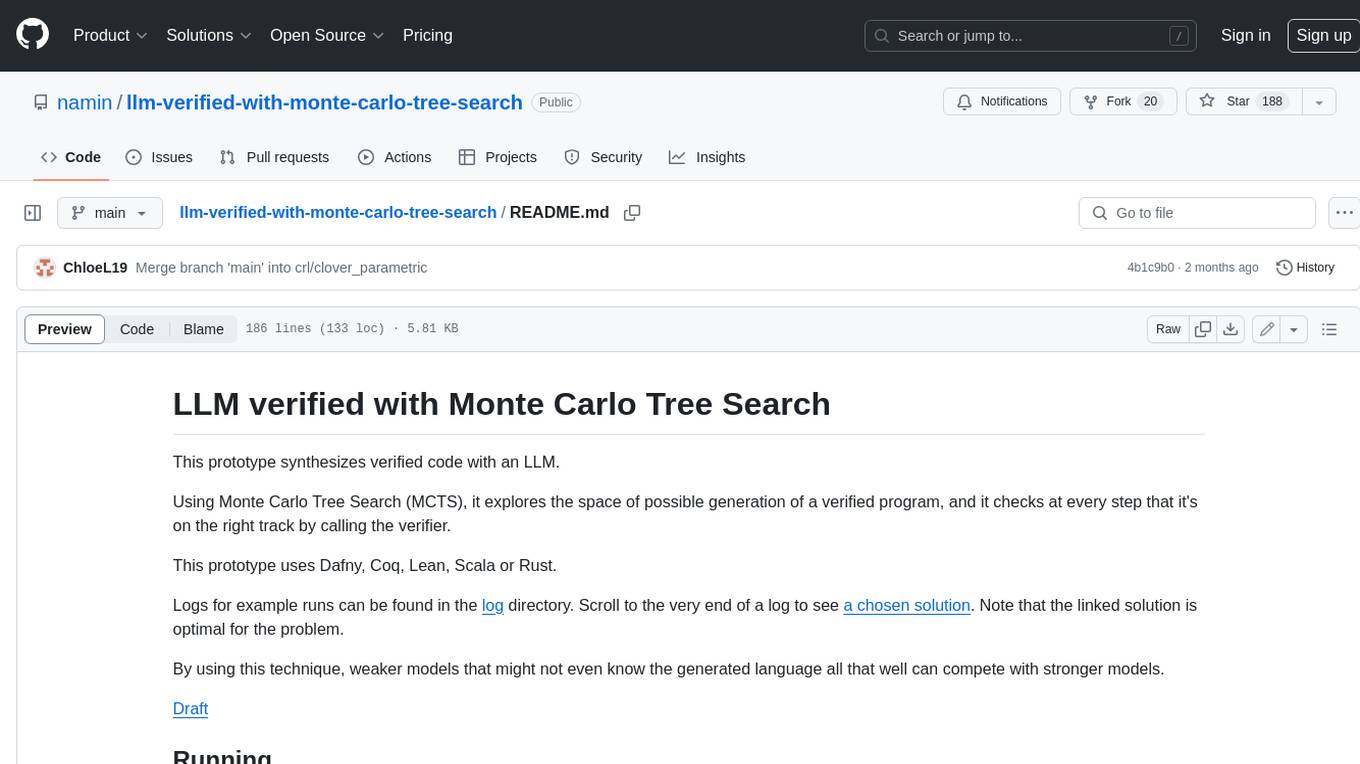
llm-verified-with-monte-carlo-tree-search
This prototype synthesizes verified code with an LLM using Monte Carlo Tree Search (MCTS). It explores the space of possible generation of a verified program and checks at every step that it's on the right track by calling the verifier. This prototype uses Dafny, Coq, Lean, Scala, or Rust. By using this technique, weaker models that might not even know the generated language all that well can compete with stronger models.
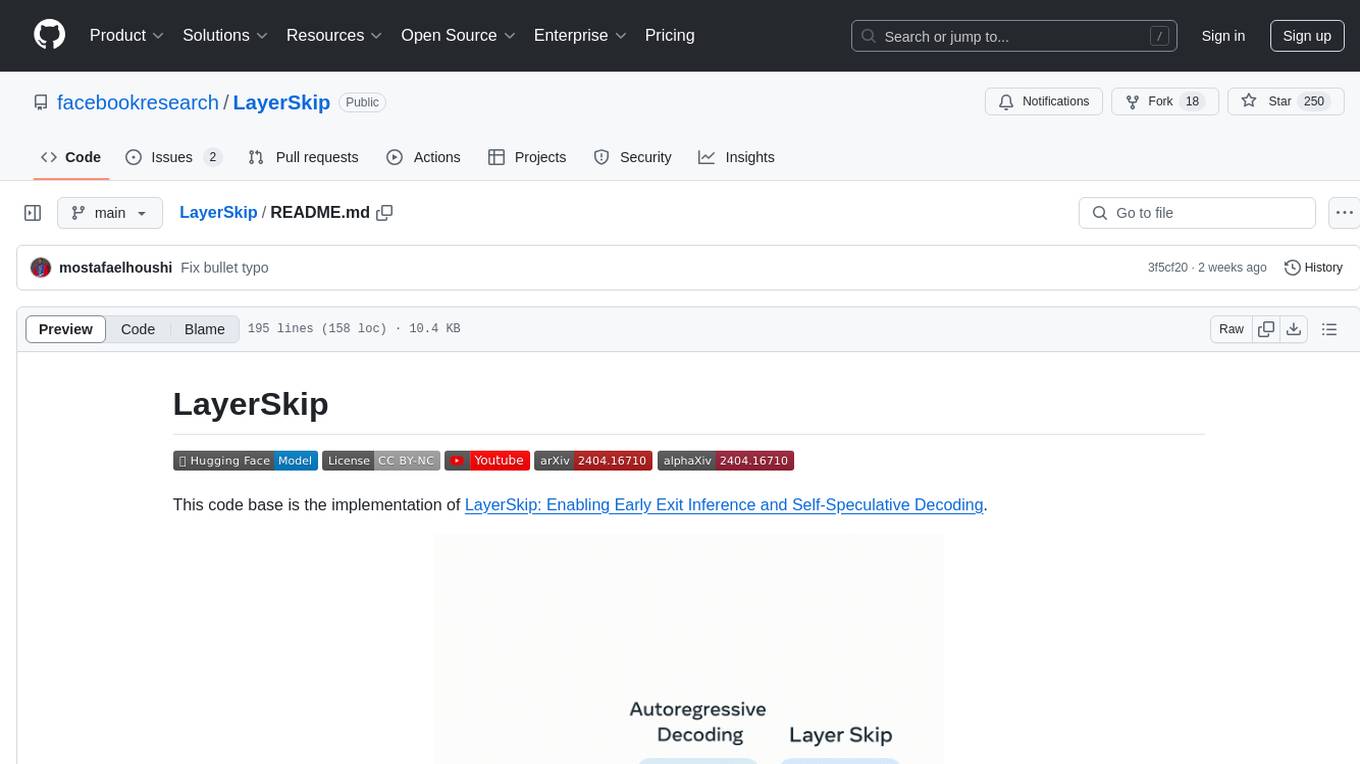
LayerSkip
LayerSkip is an implementation enabling early exit inference and self-speculative decoding. It provides a code base for running models trained using the LayerSkip recipe, offering speedup through self-speculative decoding. The tool integrates with Hugging Face transformers and provides checkpoints for various LLMs. Users can generate tokens, benchmark on datasets, evaluate tasks, and sweep over hyperparameters to optimize inference speed. The tool also includes correctness verification scripts and Docker setup instructions. Additionally, other implementations like gpt-fast and Native HuggingFace are available. Training implementation is a work-in-progress, and contributions are welcome under the CC BY-NC license.
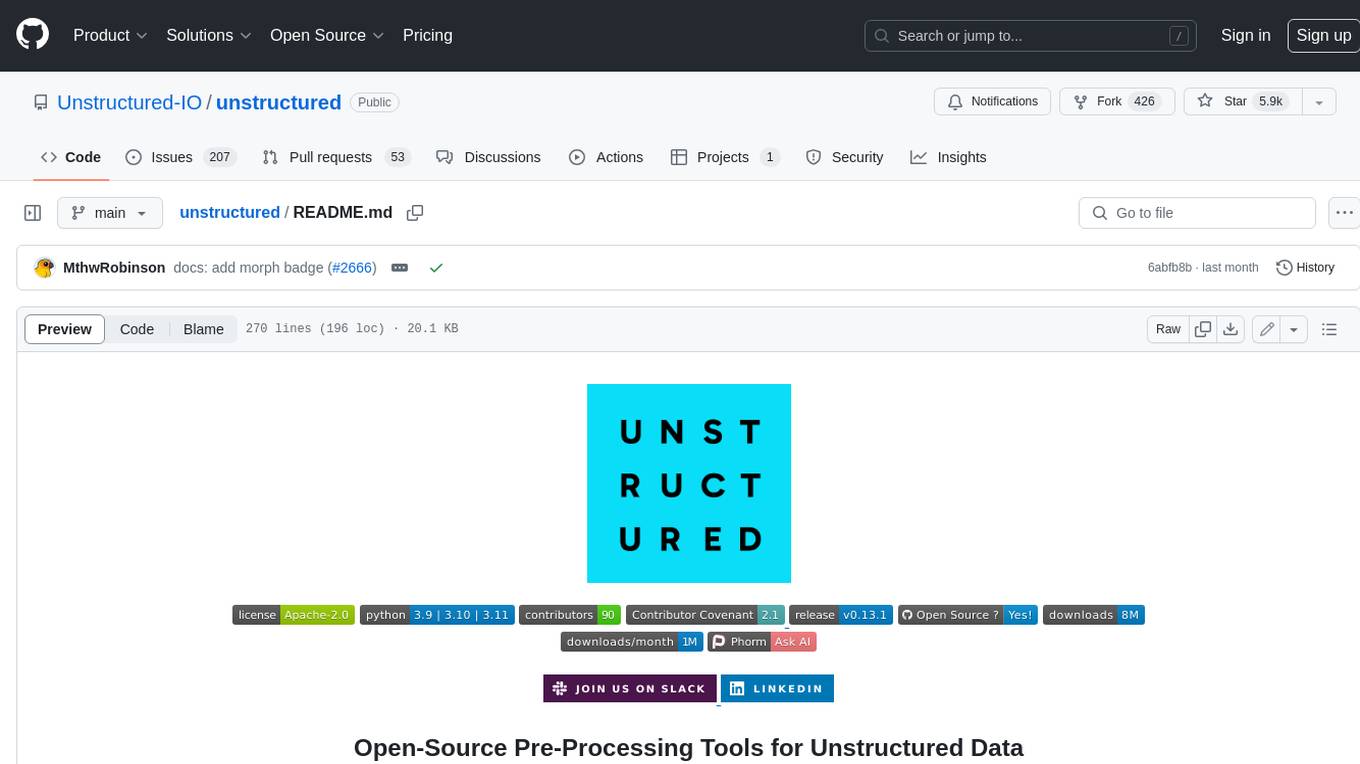
unstructured
The `unstructured` library provides open-source components for ingesting and pre-processing images and text documents, such as PDFs, HTML, Word docs, and many more. The use cases of `unstructured` revolve around streamlining and optimizing the data processing workflow for LLMs. `unstructured` modular functions and connectors form a cohesive system that simplifies data ingestion and pre-processing, making it adaptable to different platforms and efficient in transforming unstructured data into structured outputs.
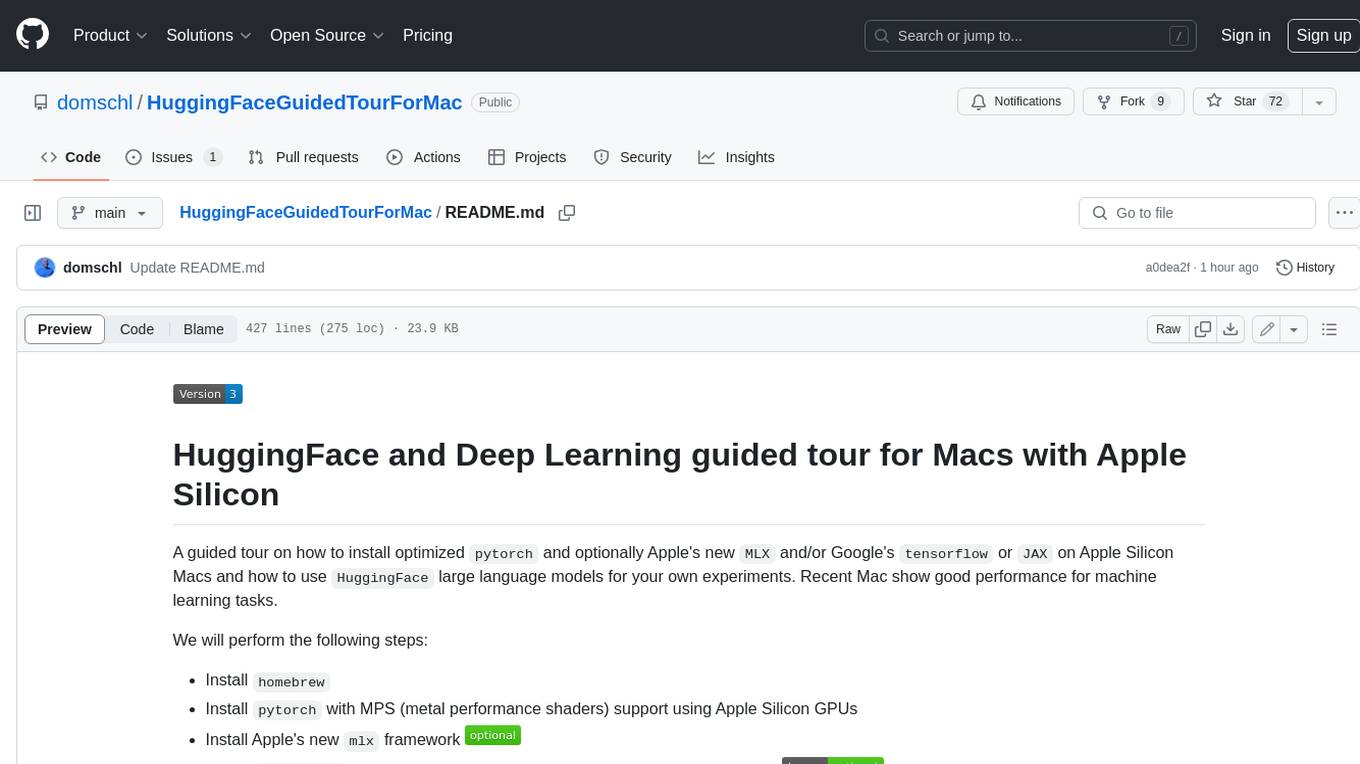
HuggingFaceGuidedTourForMac
HuggingFaceGuidedTourForMac is a guided tour on how to install optimized pytorch and optionally Apple's new MLX, JAX, and TensorFlow on Apple Silicon Macs. The repository provides steps to install homebrew, pytorch with MPS support, MLX, JAX, TensorFlow, and Jupyter lab. It also includes instructions on running large language models using HuggingFace transformers. The repository aims to help users set up their Macs for deep learning experiments with optimized performance.
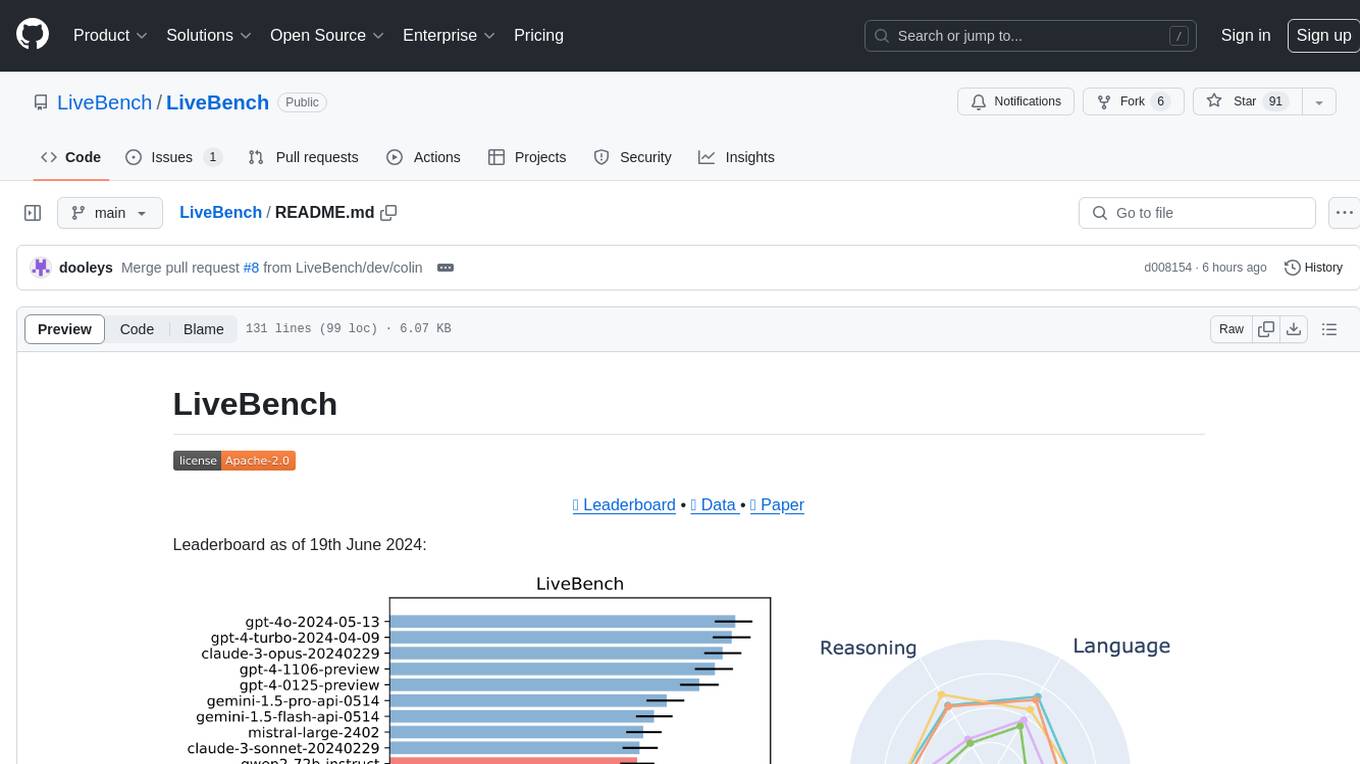
LiveBench
LiveBench is a benchmark tool designed for Language Model Models (LLMs) with a focus on limiting contamination through monthly new questions based on recent datasets, arXiv papers, news articles, and IMDb movie synopses. It provides verifiable, objective ground-truth answers for accurate scoring without an LLM judge. The tool offers 18 diverse tasks across 6 categories and promises to release more challenging tasks over time. LiveBench is built on FastChat's llm_judge module and incorporates code from LiveCodeBench and IFEval.
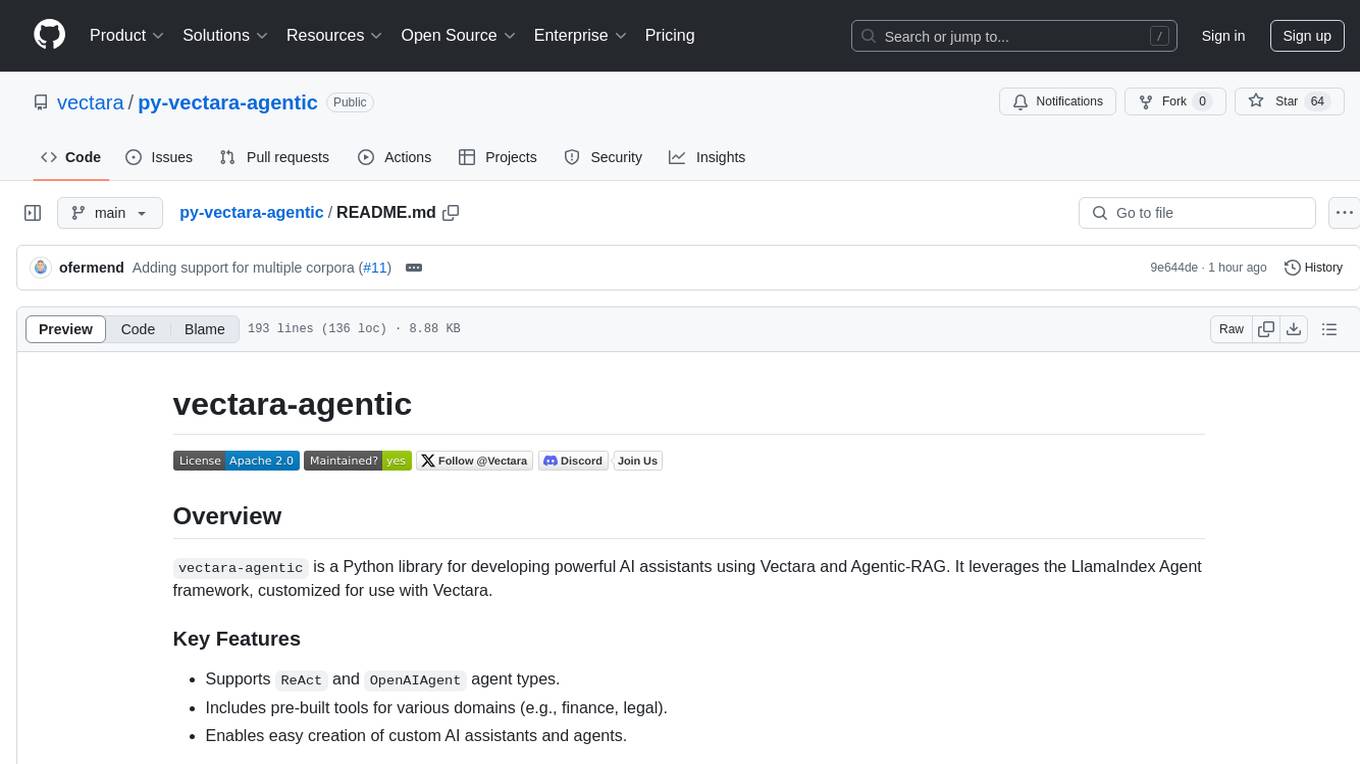
py-vectara-agentic
The `vectara-agentic` Python library is designed for developing powerful AI assistants using Vectara and Agentic-RAG. It supports various agent types, includes pre-built tools for domains like finance and legal, and enables easy creation of custom AI assistants and agents. The library provides tools for summarizing text, rephrasing text, legal tasks like summarizing legal text and critiquing as a judge, financial tasks like analyzing balance sheets and income statements, and database tools for inspecting and querying databases. It also supports observability via LlamaIndex and Arize Phoenix integration.
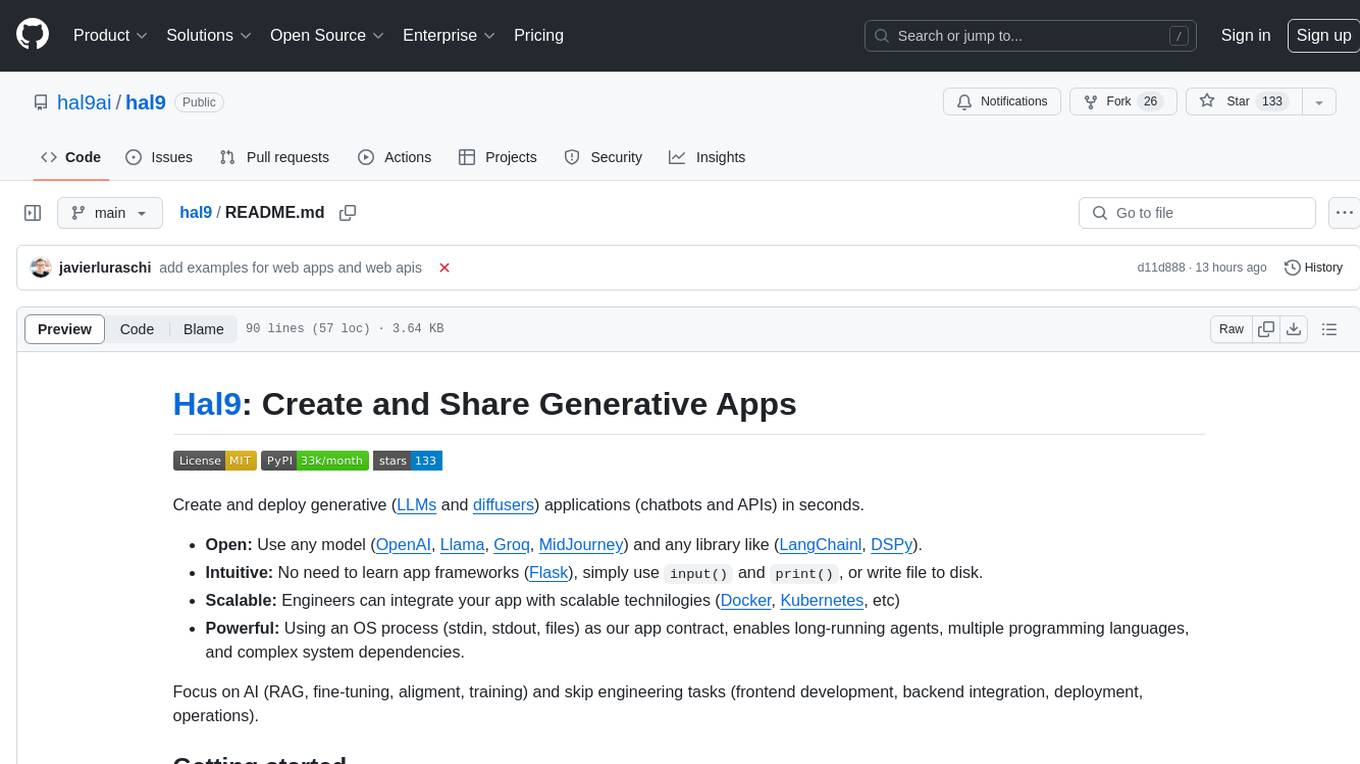
hal9
Hal9 is a tool that allows users to create and deploy generative applications such as chatbots and APIs quickly. It is open, intuitive, scalable, and powerful, enabling users to use various models and libraries without the need to learn complex app frameworks. With a focus on AI tasks like RAG, fine-tuning, alignment, and training, Hal9 simplifies the development process by skipping engineering tasks like frontend development, backend integration, deployment, and operations.
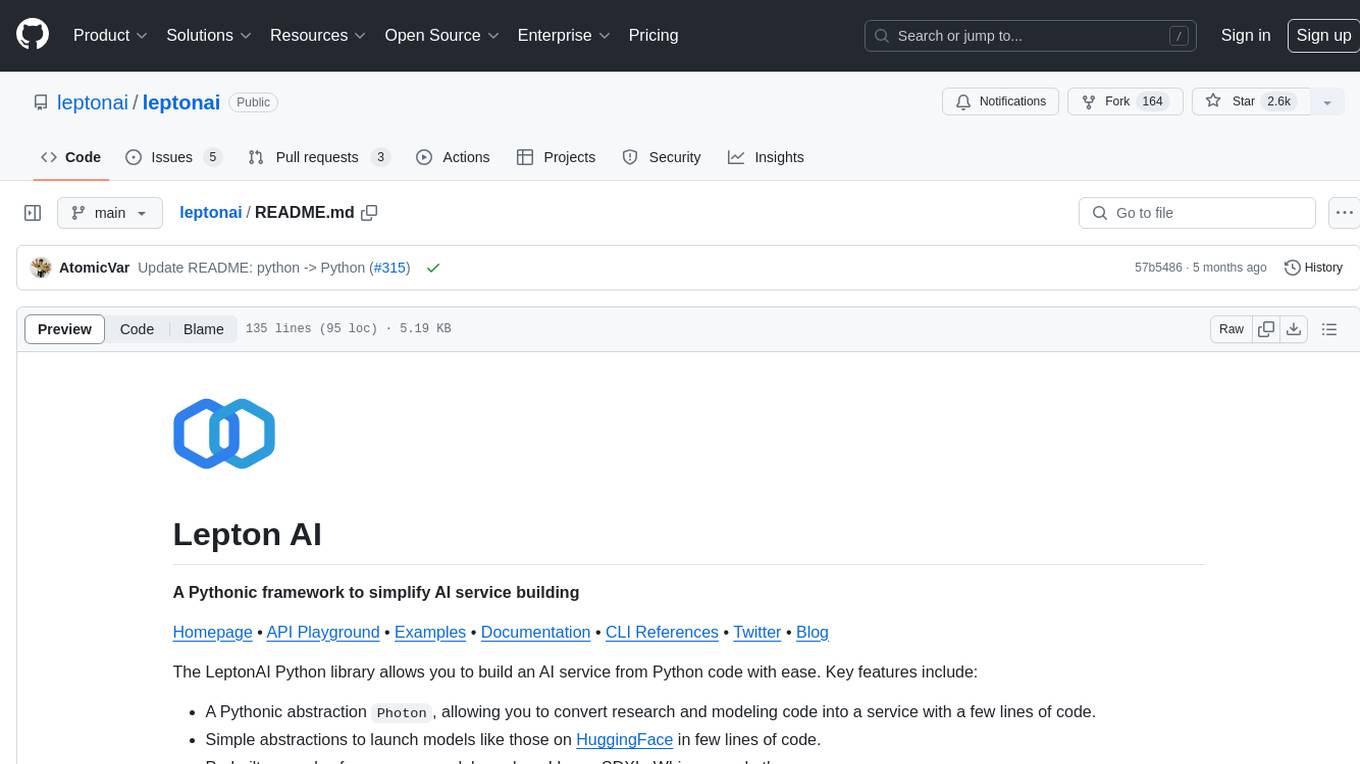
leptonai
A Pythonic framework to simplify AI service building. The LeptonAI Python library allows you to build an AI service from Python code with ease. Key features include a Pythonic abstraction Photon, simple abstractions to launch models like those on HuggingFace, prebuilt examples for common models, AI tailored batteries, a client to automatically call your service like native Python functions, and Pythonic configuration specs to be readily shipped in a cloud environment.
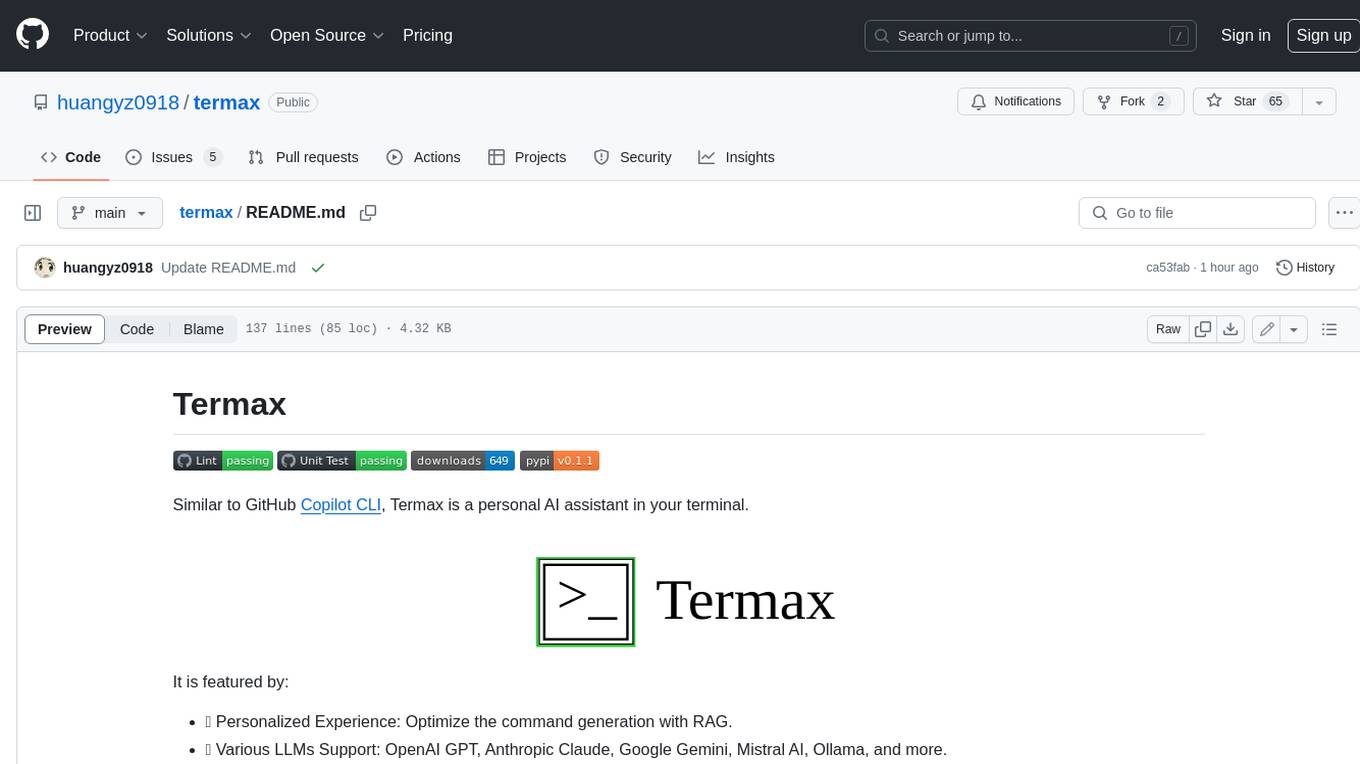
termax
Termax is an LLM agent in your terminal that converts natural language to commands. It is featured by: - Personalized Experience: Optimize the command generation with RAG. - Various LLMs Support: OpenAI GPT, Anthropic Claude, Google Gemini, Mistral AI, and more. - Shell Extensions: Plugin with popular shells like `zsh`, `bash` and `fish`. - Cross Platform: Able to run on Windows, macOS, and Linux.
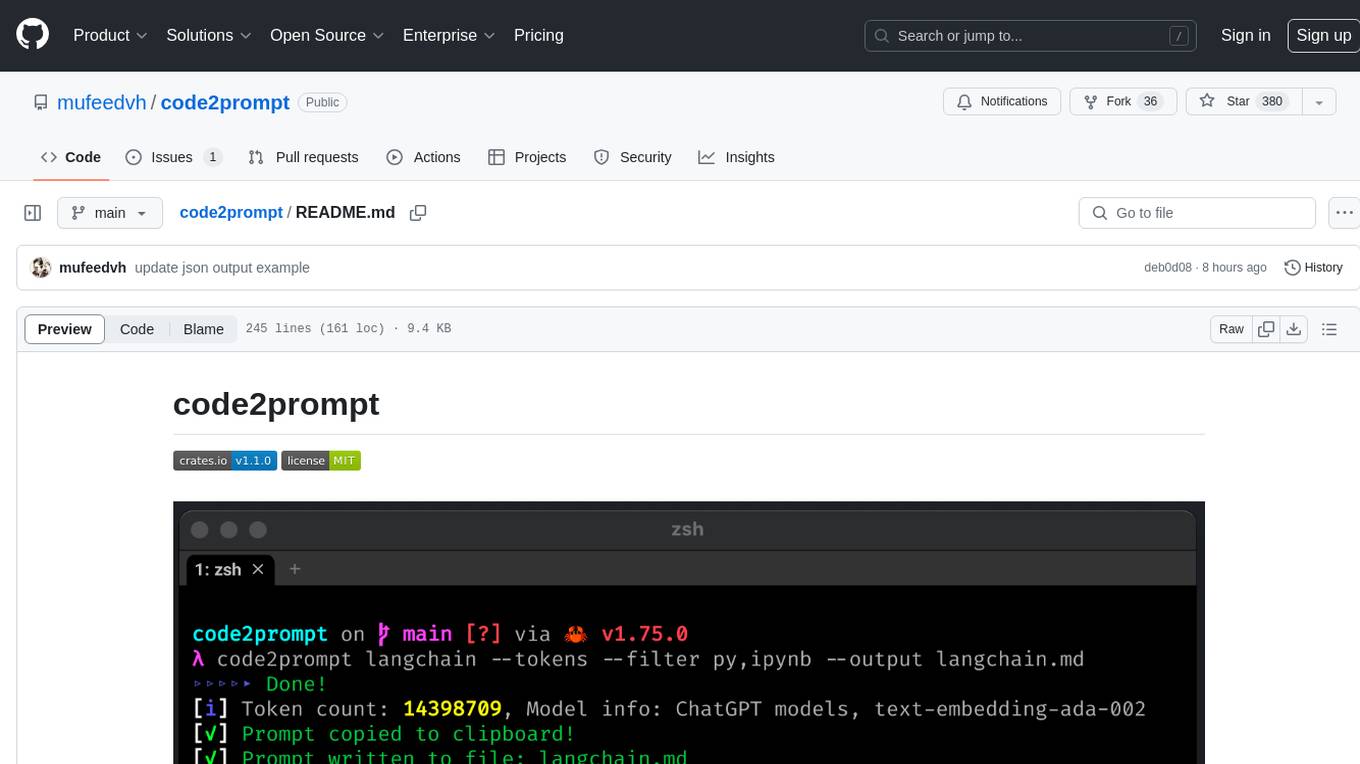
code2prompt
code2prompt is a command-line tool that converts your codebase into a single LLM prompt with a source tree, prompt templating, and token counting. It automates generating LLM prompts from codebases of any size, customizing prompt generation with Handlebars templates, respecting .gitignore, filtering and excluding files using glob patterns, displaying token count, including Git diff output, copying prompt to clipboard, saving prompt to an output file, excluding files and folders, adding line numbers to source code blocks, and more. It helps streamline the process of creating LLM prompts for code analysis, generation, and other tasks.
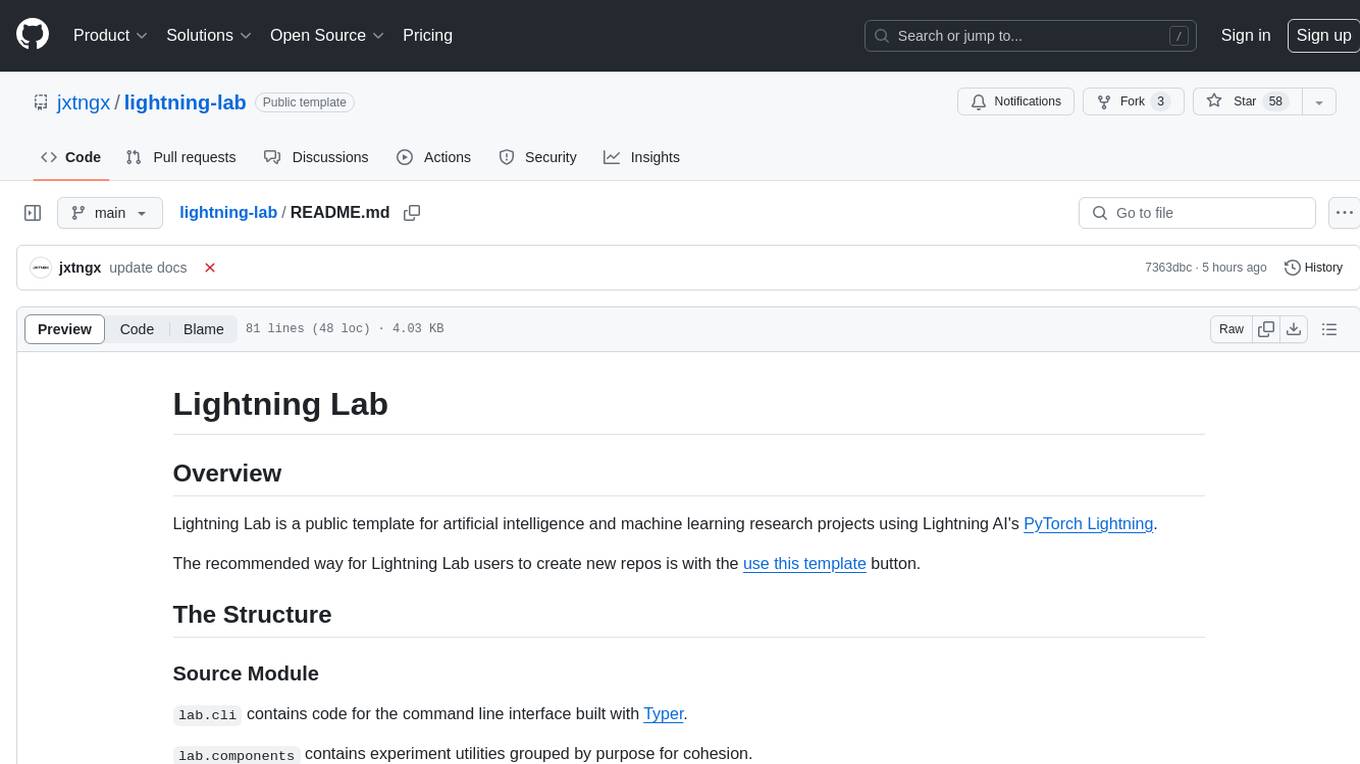
lightning-lab
Lightning Lab is a public template for artificial intelligence and machine learning research projects using Lightning AI's PyTorch Lightning. It provides a structured project layout with modules for command line interface, experiment utilities, Lightning Module and Trainer, data acquisition and preprocessing, model serving APIs, project configurations, training checkpoints, technical documentation, logs, notebooks for data analysis, requirements management, testing, and packaging. The template simplifies the setup of deep learning projects and offers extras for different domains like vision, text, audio, reinforcement learning, and forecasting.
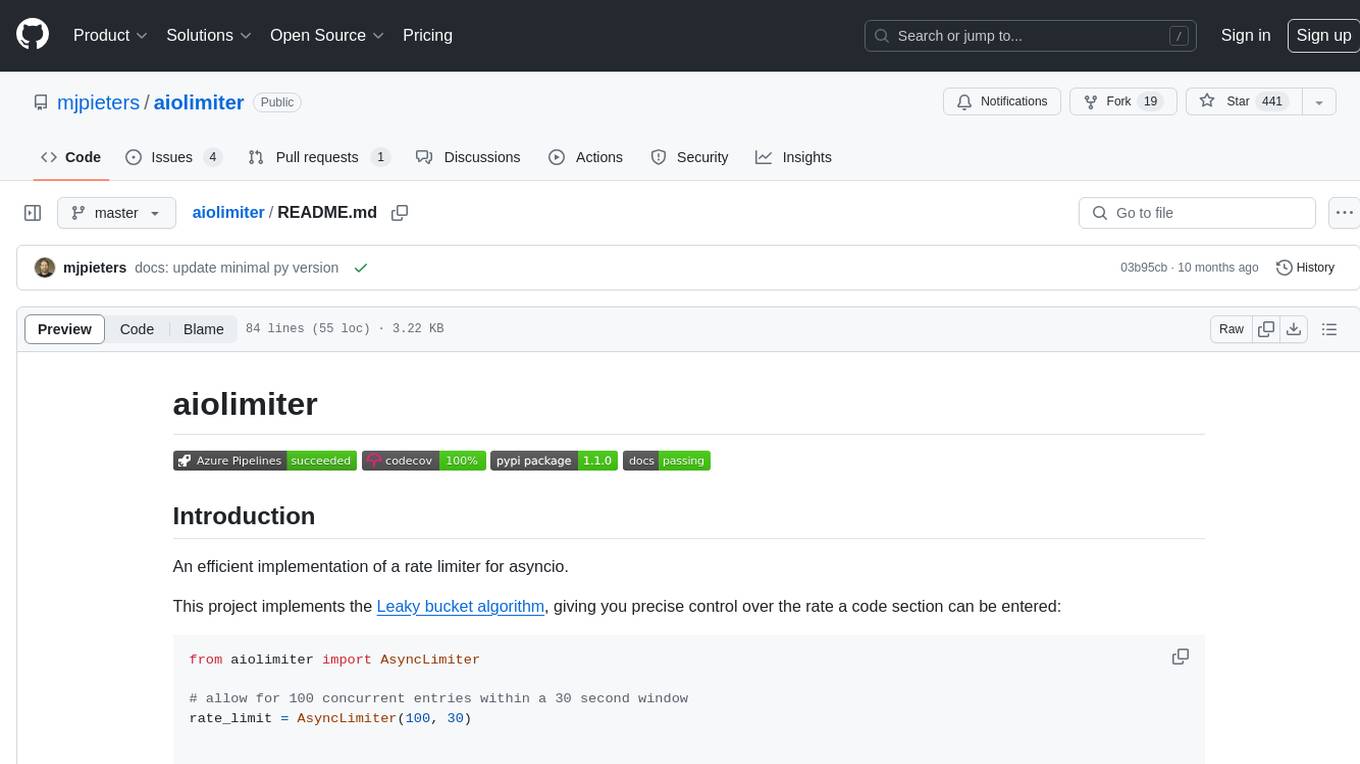
aiolimiter
An efficient implementation of a rate limiter for asyncio using the Leaky bucket algorithm, providing precise control over the rate a code section can be entered. It allows for limiting the number of concurrent entries within a specified time window, ensuring that a section of code is executed a maximum number of times in that period.
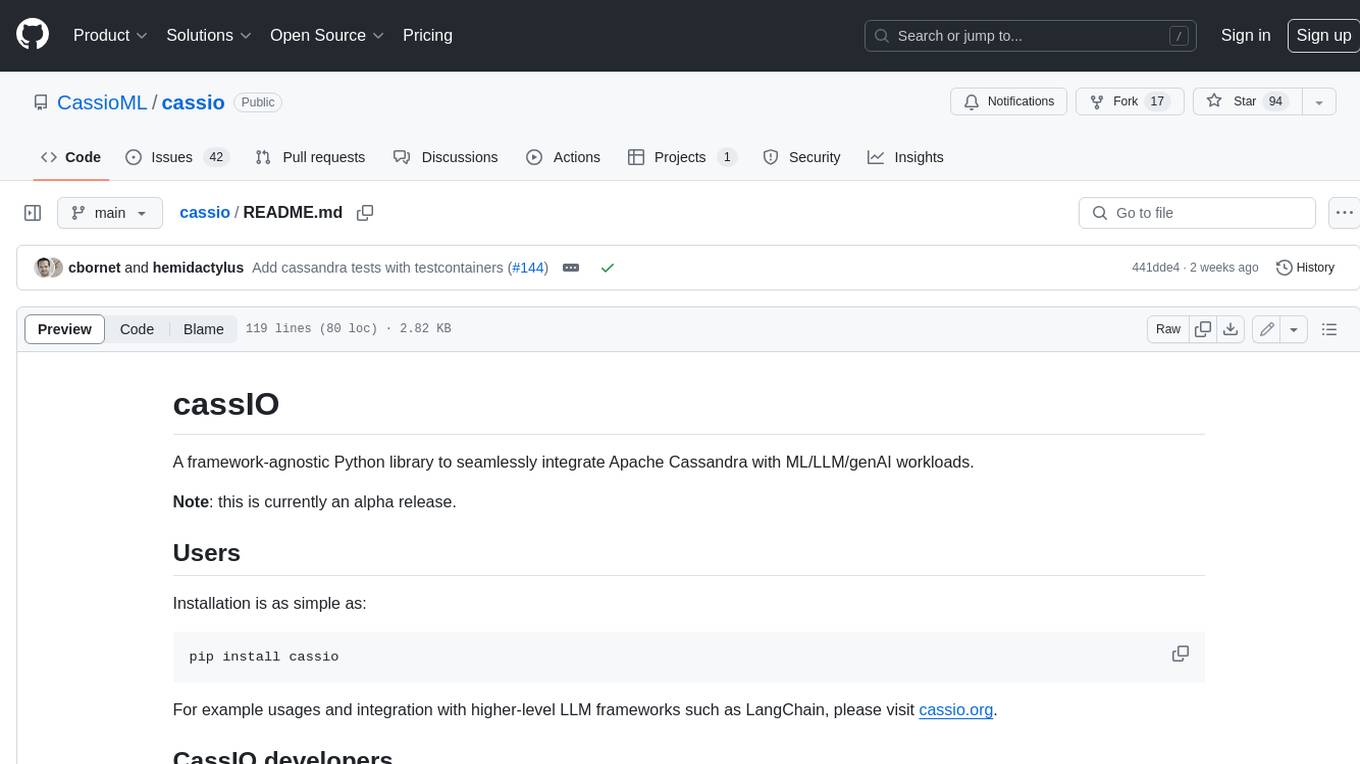
cassio
cassIO is a framework-agnostic Python library that seamlessly integrates Apache Cassandra with ML/LLM/genAI workloads. It provides an easy-to-use interface for developers to connect their Cassandra databases to machine learning models, allowing them to perform complex data analysis and AI-powered tasks directly on their Cassandra data. cassIO is designed to be flexible and extensible, making it suitable for a wide range of use cases, from data exploration and visualization to predictive modeling and natural language processing.
For similar tasks
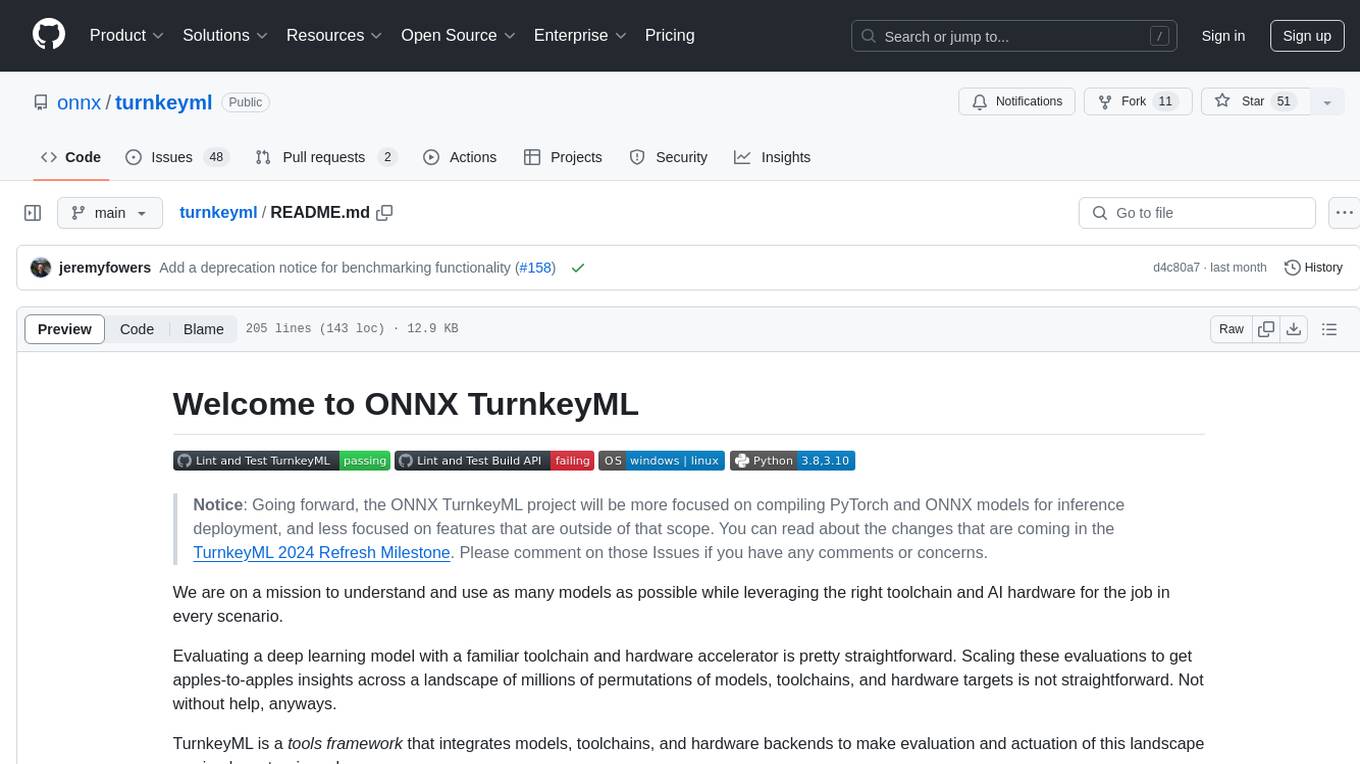
turnkeyml
TurnkeyML is a tools framework that integrates models, toolchains, and hardware backends to simplify the evaluation and actuation of deep learning models. It supports use cases like exporting ONNX files, performance validation, functional coverage measurement, stress testing, and model insights analysis. The framework consists of analysis, build, runtime, reporting tools, and a models corpus, seamlessly integrated to provide comprehensive functionality with simple commands. Extensible through plugins, it offers support for various export and optimization tools and AI runtimes. The project is actively seeking collaborators and is licensed under Apache 2.0.
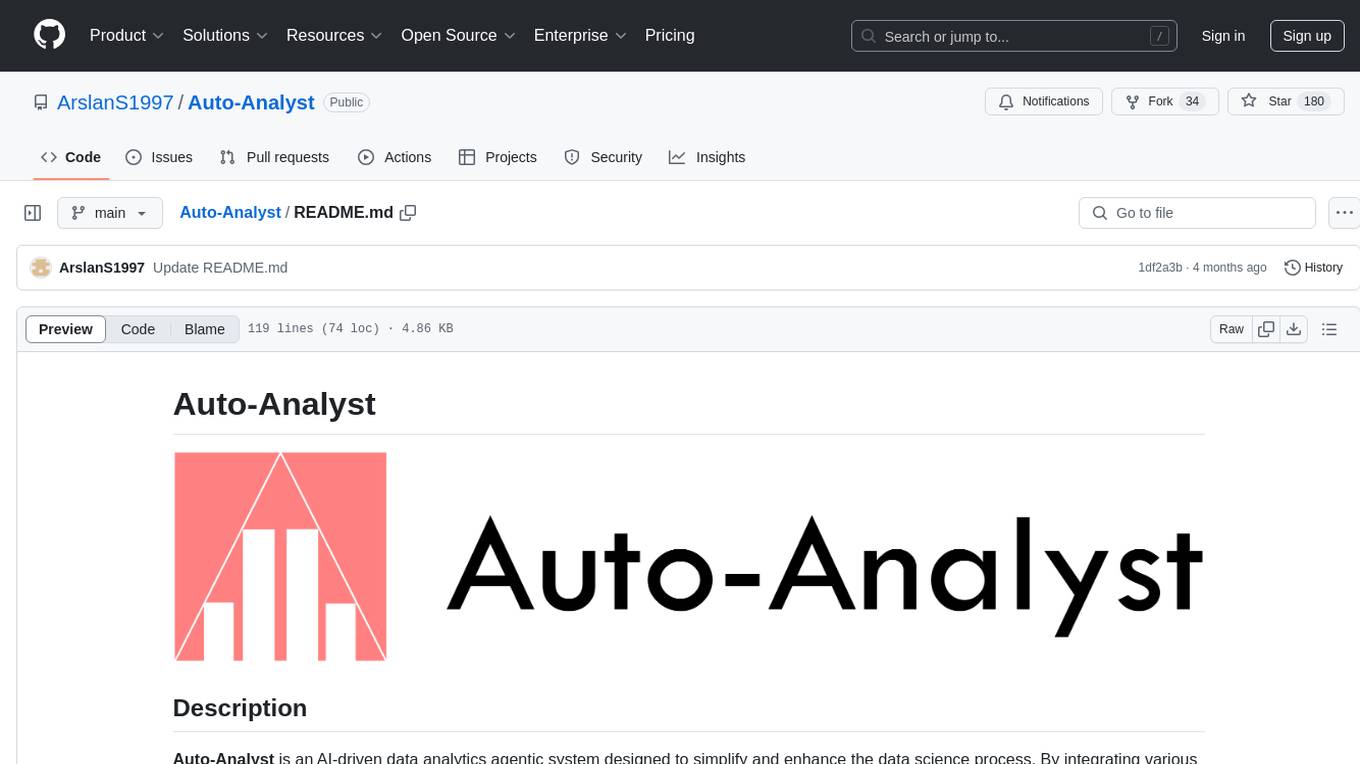
Auto-Analyst
Auto-Analyst is an AI-driven data analytics agentic system designed to simplify and enhance the data science process. By integrating various specialized AI agents, this tool aims to make complex data analysis tasks more accessible and efficient for data analysts and scientists. Auto-Analyst provides a streamlined approach to data preprocessing, statistical analysis, machine learning, and visualization, all within an interactive Streamlit interface. It offers plug and play Streamlit UI, agents with data science speciality, complete automation, LLM agnostic operation, and is built using lightweight frameworks.
For similar jobs
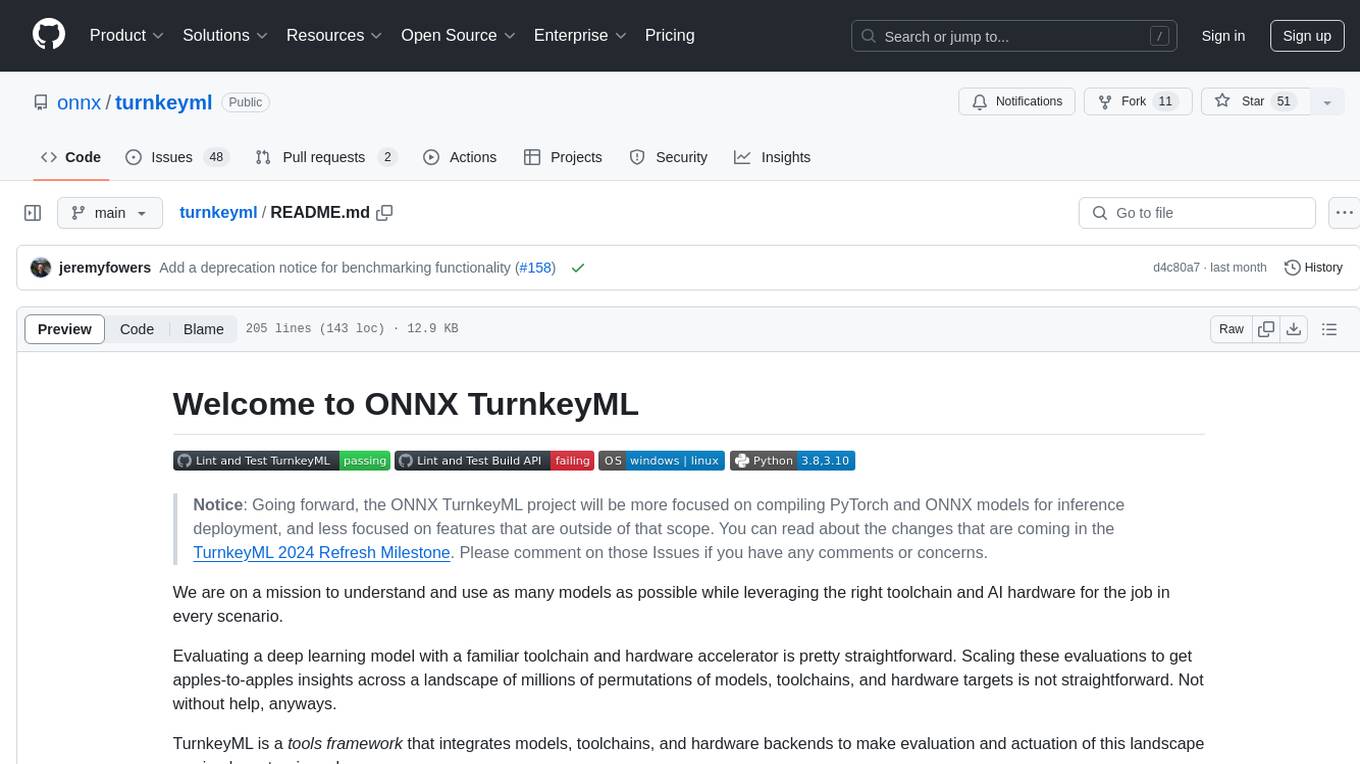
turnkeyml
TurnkeyML is a tools framework that integrates models, toolchains, and hardware backends to simplify the evaluation and actuation of deep learning models. It supports use cases like exporting ONNX files, performance validation, functional coverage measurement, stress testing, and model insights analysis. The framework consists of analysis, build, runtime, reporting tools, and a models corpus, seamlessly integrated to provide comprehensive functionality with simple commands. Extensible through plugins, it offers support for various export and optimization tools and AI runtimes. The project is actively seeking collaborators and is licensed under Apache 2.0.
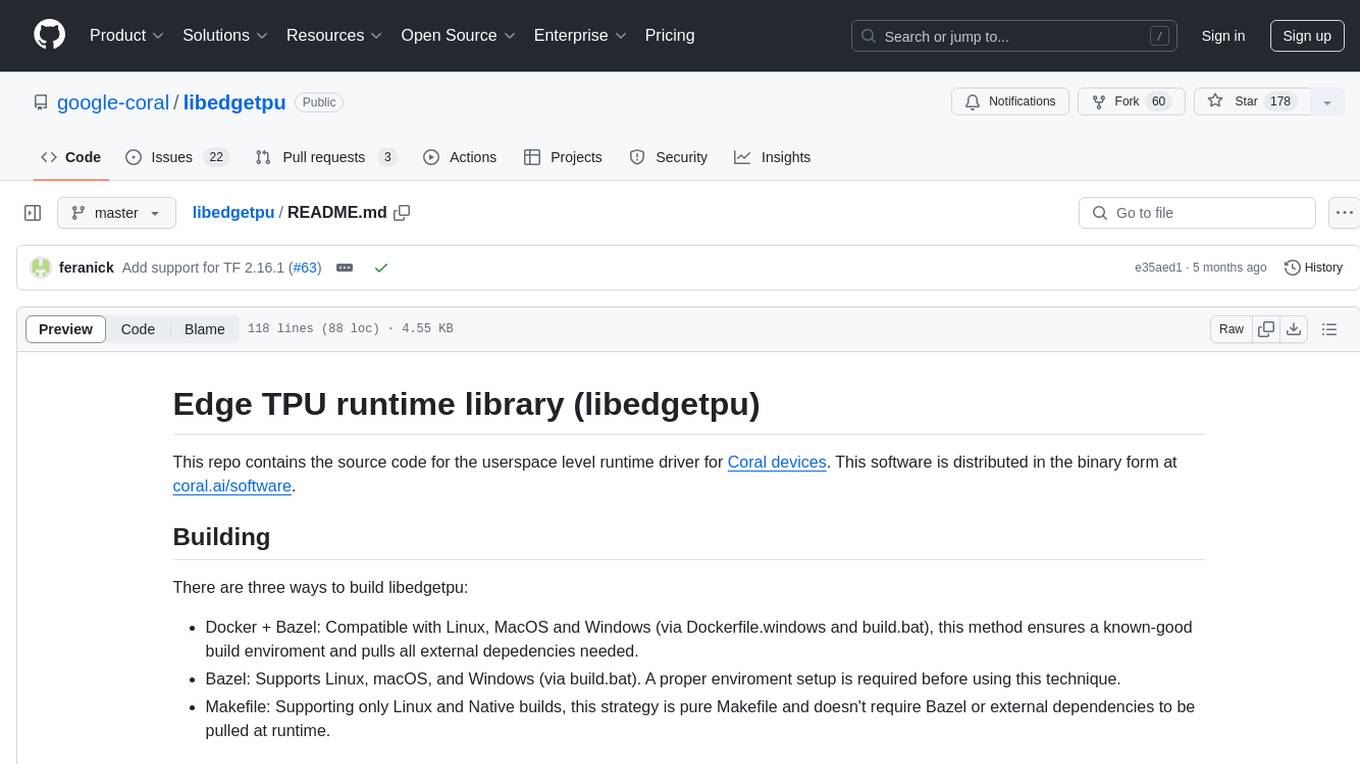
libedgetpu
This repository contains the source code for the userspace level runtime driver for Coral devices. The software is distributed in binary form at coral.ai/software. Users can build the library using Docker + Bazel, Bazel, or Makefile methods. It supports building on Linux, macOS, and Windows. The library is used to enable the Edge TPU runtime, which may heat up during operation. Google does not accept responsibility for any loss or damage if the device is operated outside the recommended ambient temperature range.
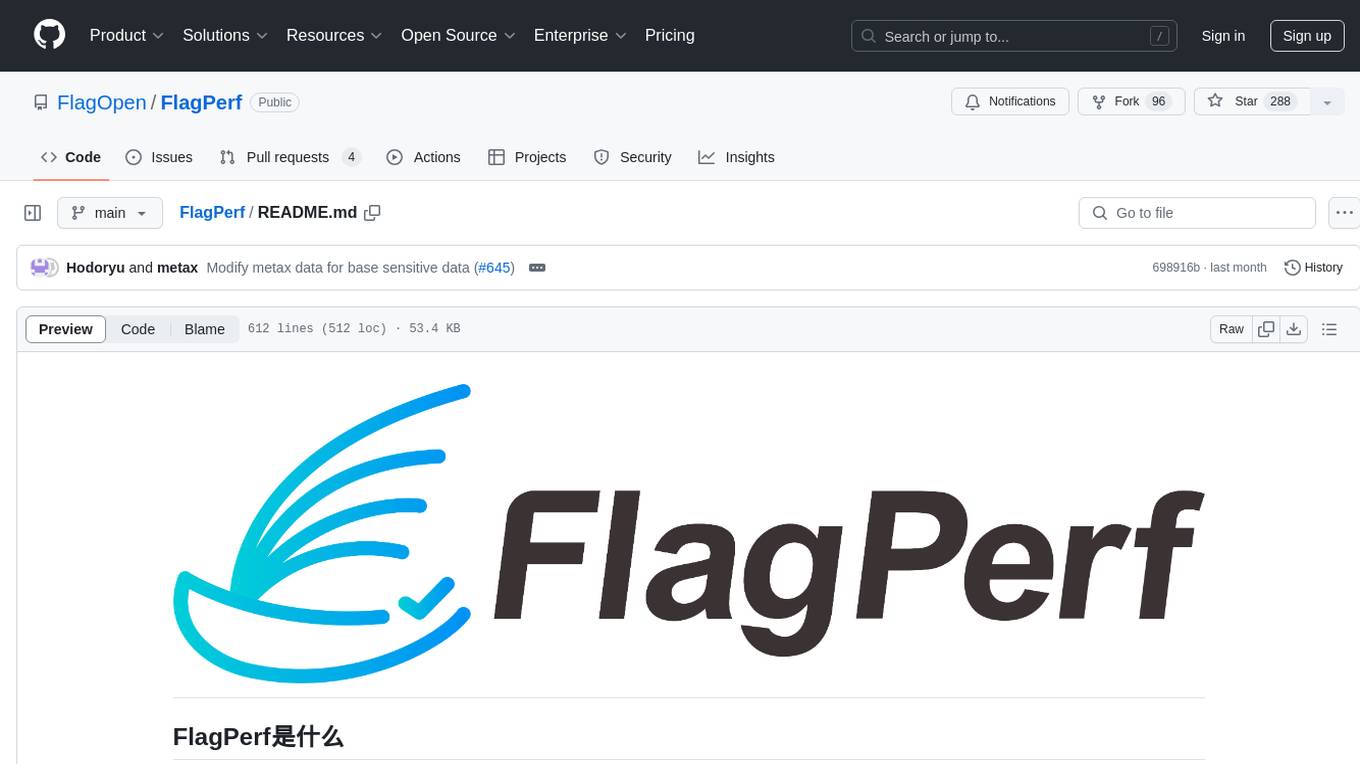
FlagPerf
FlagPerf is an integrated AI hardware evaluation engine jointly built by the Institute of Intelligence and AI hardware manufacturers. It aims to establish an industry-oriented metric system to evaluate the actual capabilities of AI hardware under software stack combinations (model + framework + compiler). FlagPerf features a multidimensional evaluation metric system that goes beyond just measuring 'whether the chip can support specific model training.' It covers various scenarios and tasks, including computer vision, natural language processing, speech, multimodal, with support for multiple training frameworks and inference engines to connect AI hardware with software ecosystems. It also supports various testing environments to comprehensively assess the performance of domestic AI chips in different scenarios.
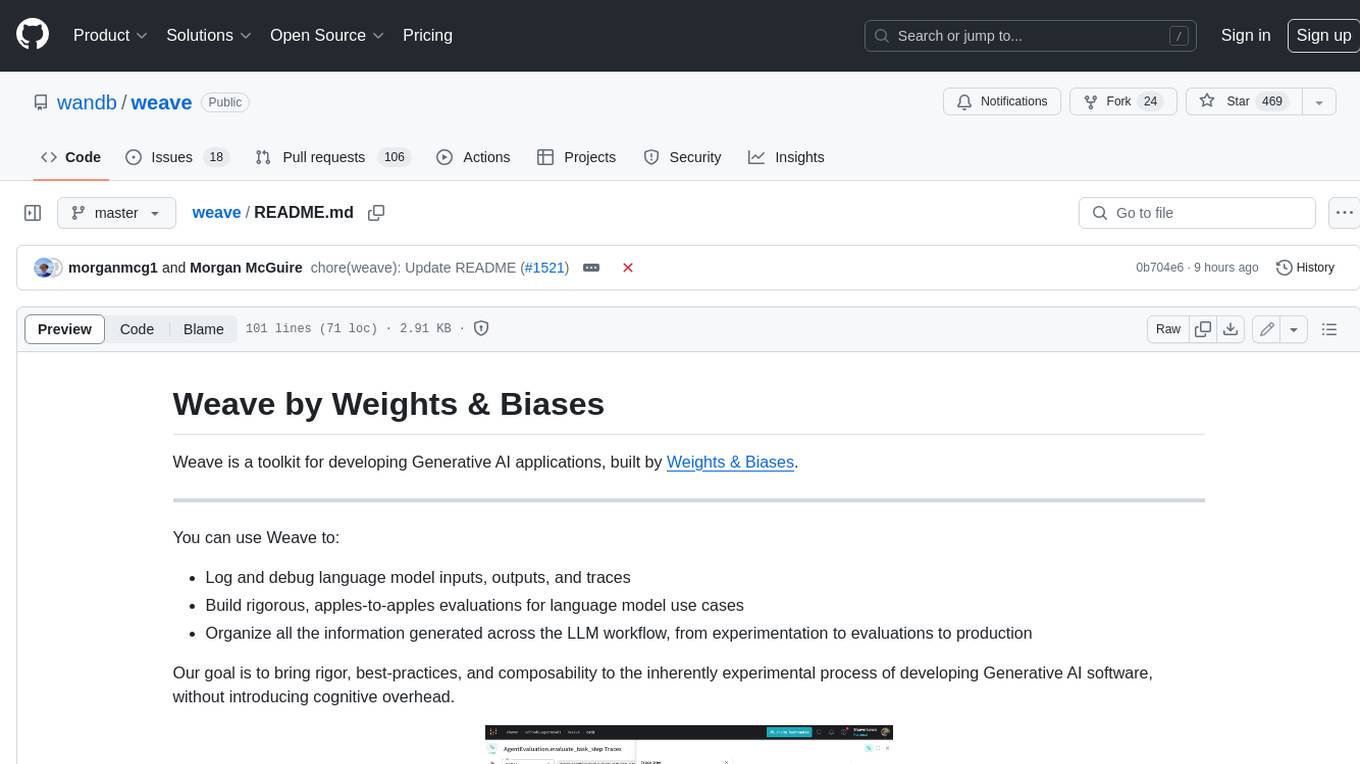
weave
Weave is a toolkit for developing Generative AI applications, built by Weights & Biases. With Weave, you can log and debug language model inputs, outputs, and traces; build rigorous, apples-to-apples evaluations for language model use cases; and organize all the information generated across the LLM workflow, from experimentation to evaluations to production. Weave aims to bring rigor, best-practices, and composability to the inherently experimental process of developing Generative AI software, without introducing cognitive overhead.
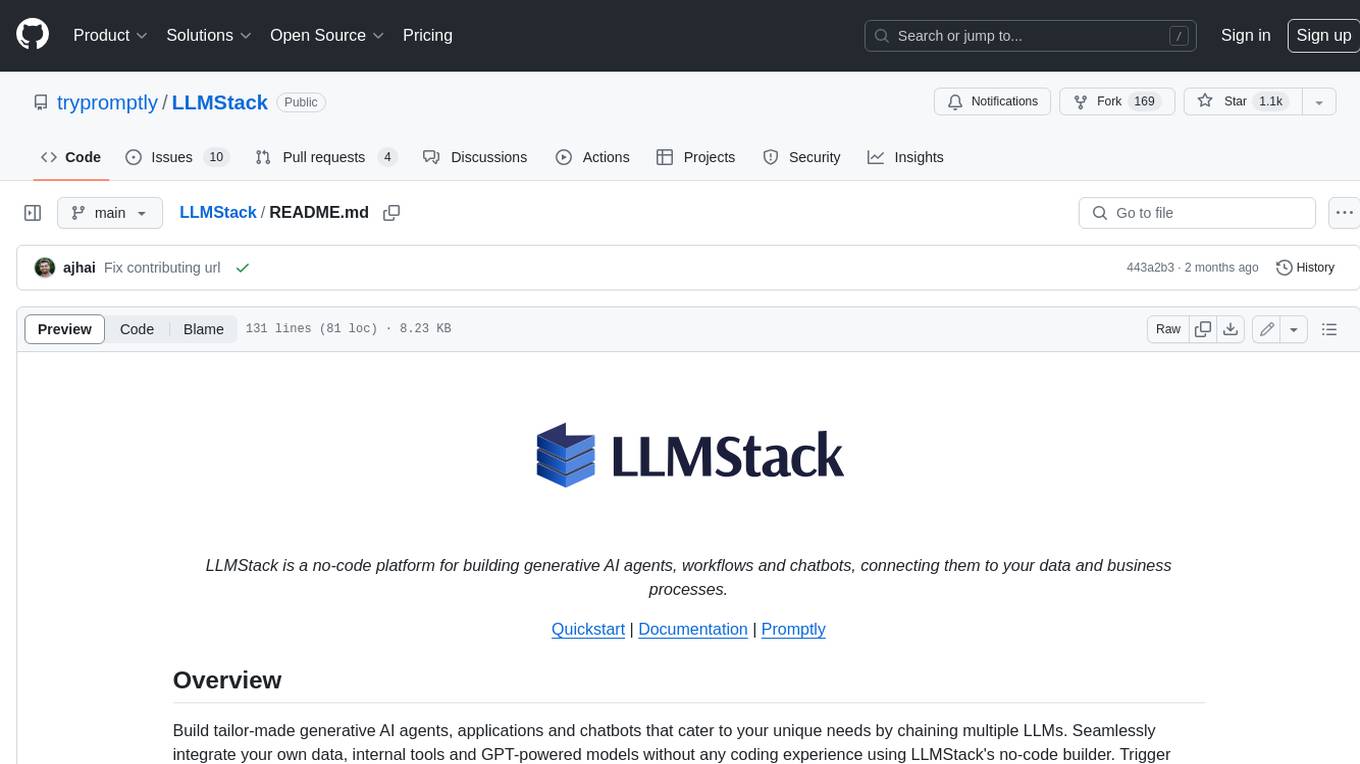
LLMStack
LLMStack is a no-code platform for building generative AI agents, workflows, and chatbots. It allows users to connect their own data, internal tools, and GPT-powered models without any coding experience. LLMStack can be deployed to the cloud or on-premise and can be accessed via HTTP API or triggered from Slack or Discord.
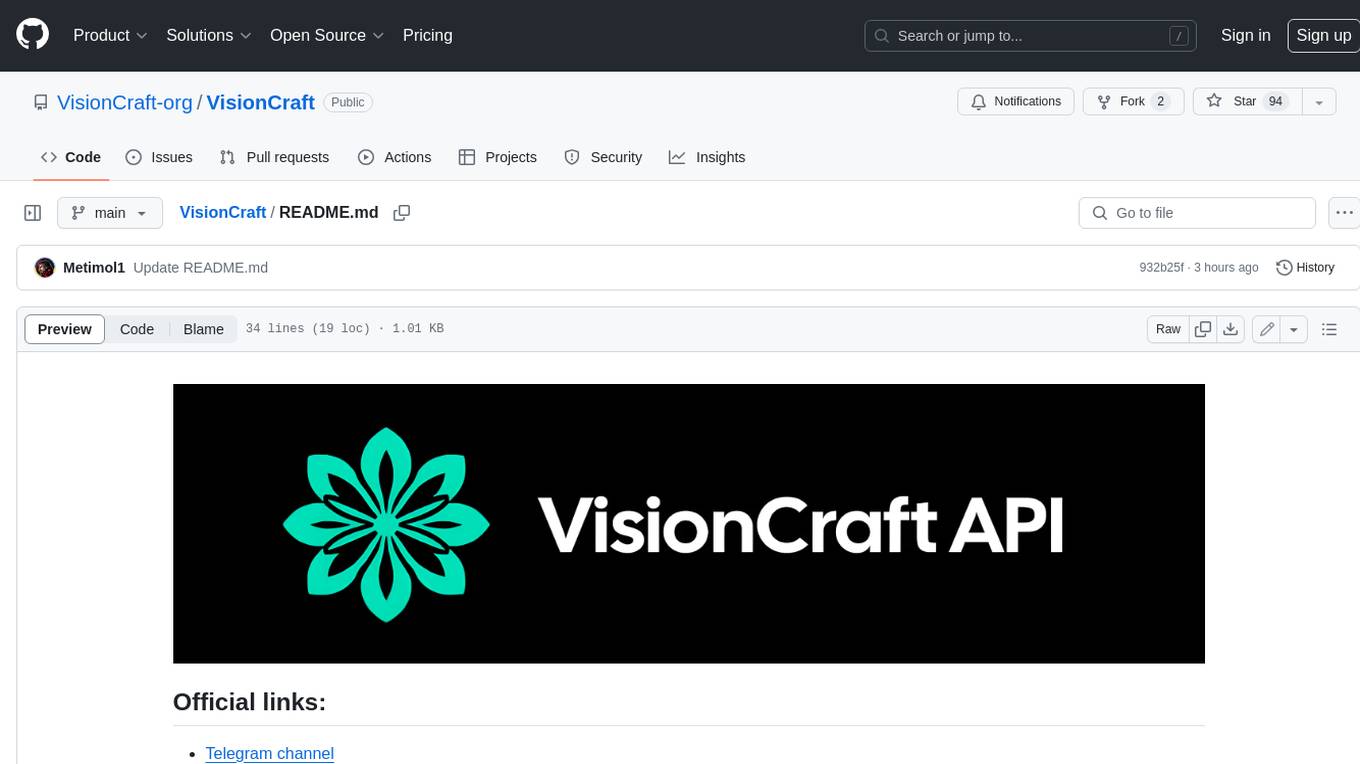
VisionCraft
The VisionCraft API is a free API for using over 100 different AI models. From images to sound.
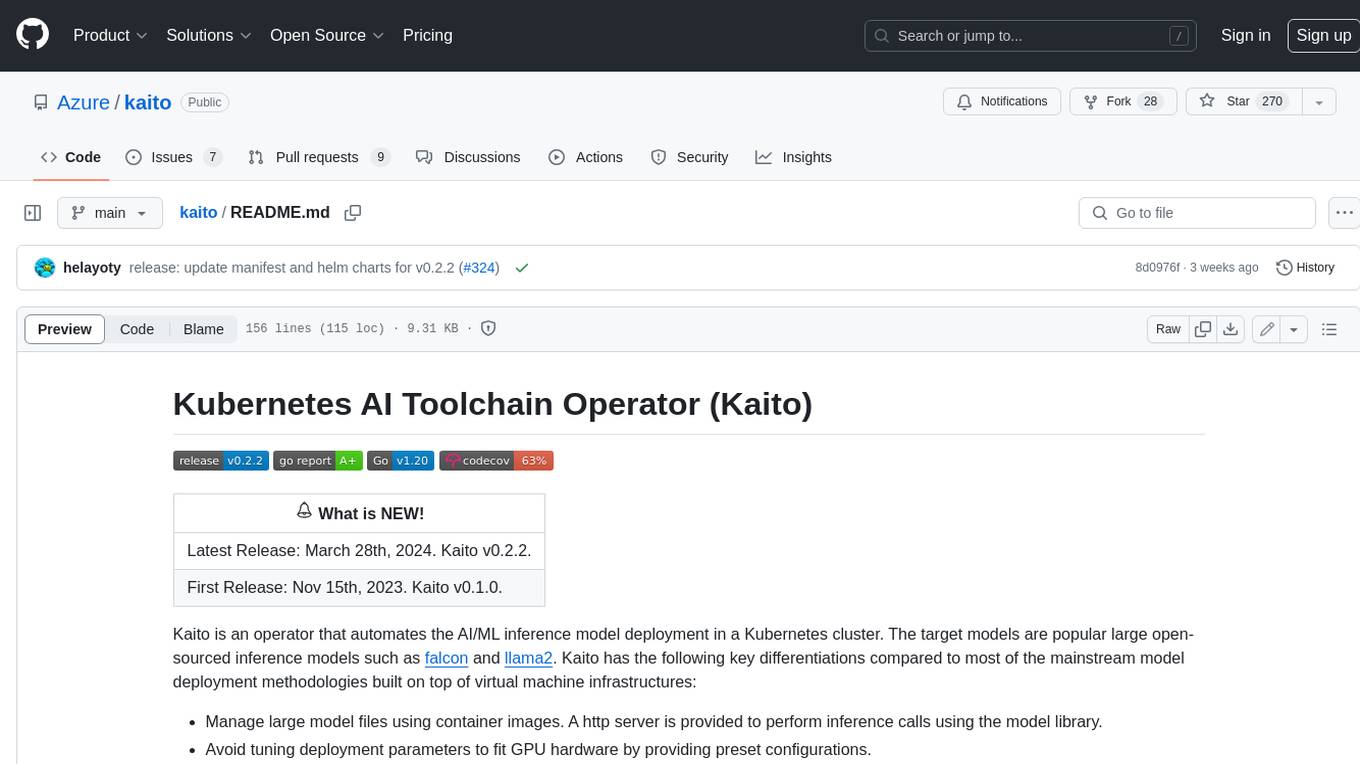
kaito
Kaito is an operator that automates the AI/ML inference model deployment in a Kubernetes cluster. It manages large model files using container images, avoids tuning deployment parameters to fit GPU hardware by providing preset configurations, auto-provisions GPU nodes based on model requirements, and hosts large model images in the public Microsoft Container Registry (MCR) if the license allows. Using Kaito, the workflow of onboarding large AI inference models in Kubernetes is largely simplified.
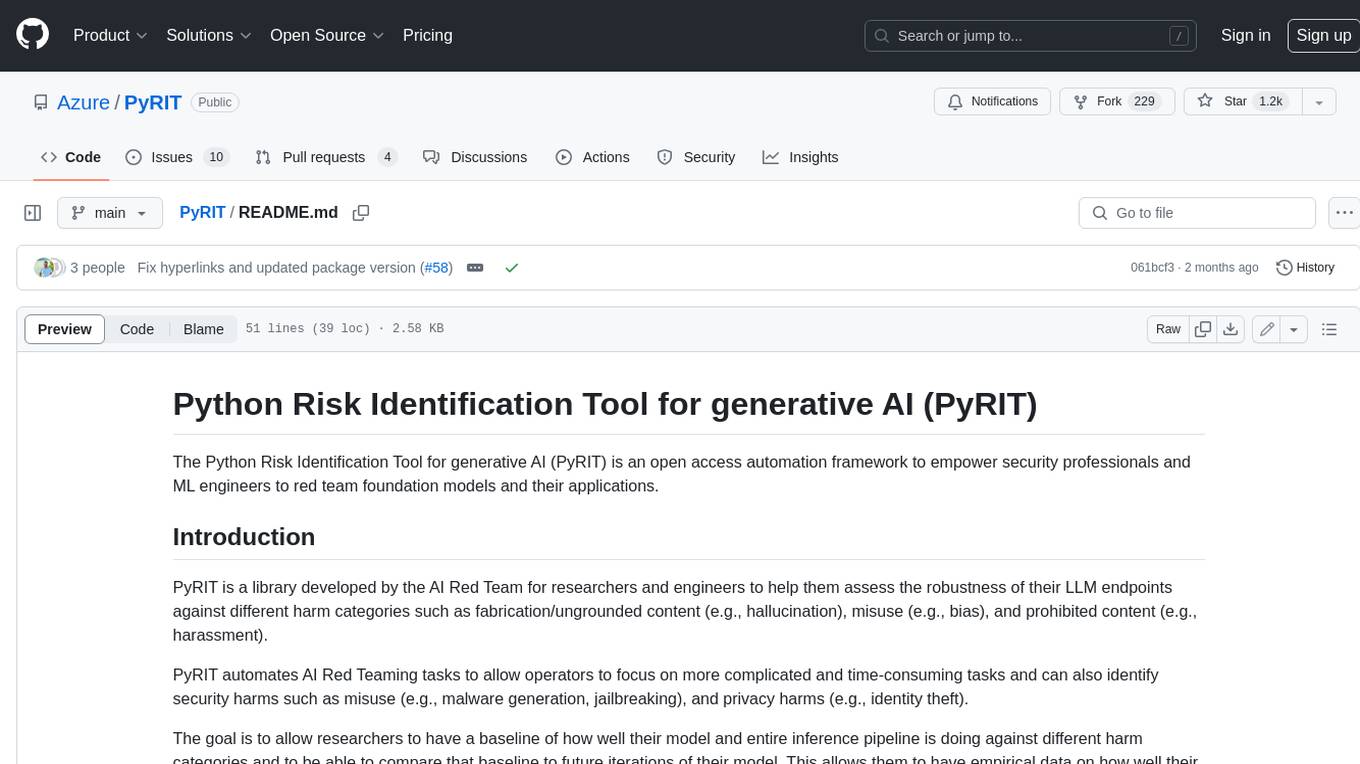
PyRIT
PyRIT is an open access automation framework designed to empower security professionals and ML engineers to red team foundation models and their applications. It automates AI Red Teaming tasks to allow operators to focus on more complicated and time-consuming tasks and can also identify security harms such as misuse (e.g., malware generation, jailbreaking), and privacy harms (e.g., identity theft). The goal is to allow researchers to have a baseline of how well their model and entire inference pipeline is doing against different harm categories and to be able to compare that baseline to future iterations of their model. This allows them to have empirical data on how well their model is doing today, and detect any degradation of performance based on future improvements.