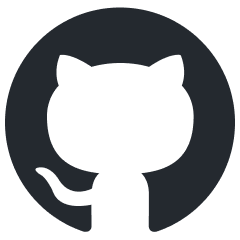
FlagPerf
FlagPerf is an open-source software platform for benchmarking AI chips.
Stars: 301
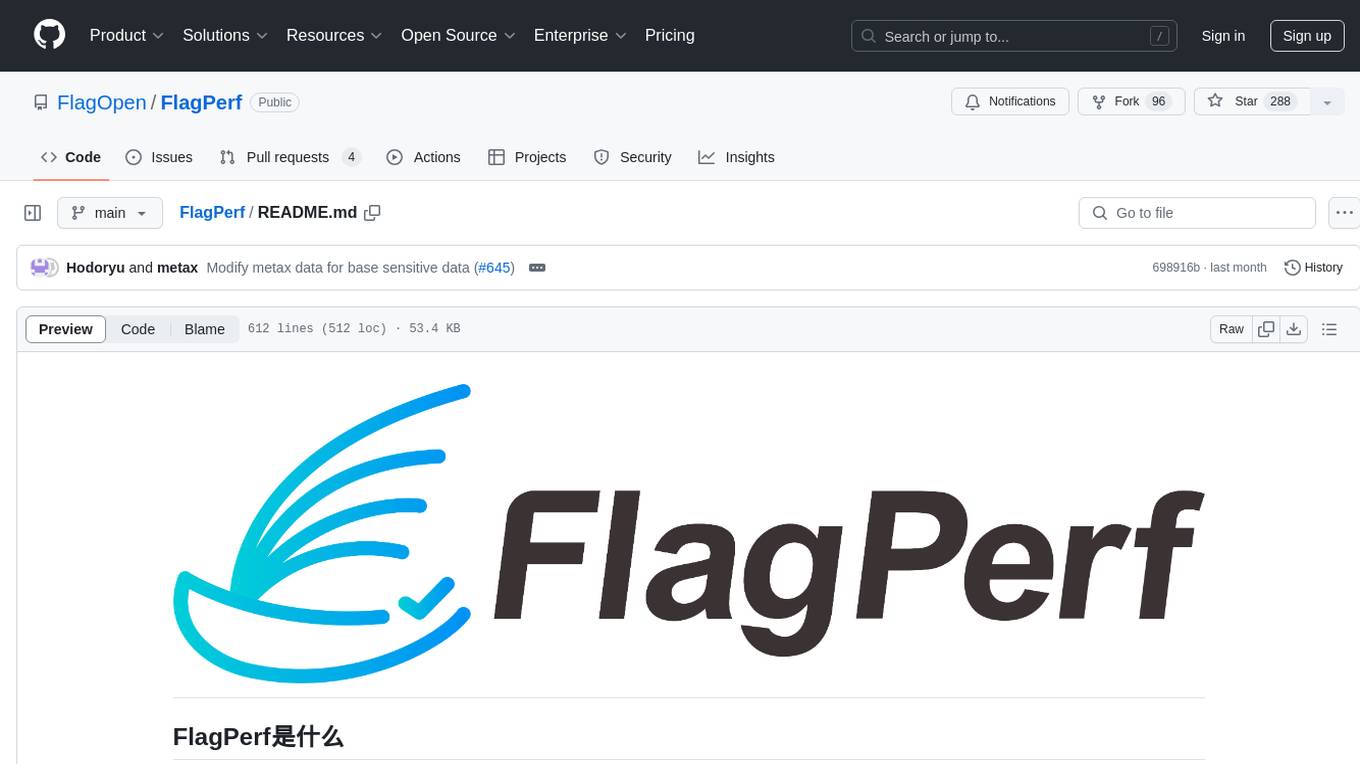
FlagPerf is an integrated AI hardware evaluation engine jointly built by the Institute of Intelligence and AI hardware manufacturers. It aims to establish an industry-oriented metric system to evaluate the actual capabilities of AI hardware under software stack combinations (model + framework + compiler). FlagPerf features a multidimensional evaluation metric system that goes beyond just measuring 'whether the chip can support specific model training.' It covers various scenarios and tasks, including computer vision, natural language processing, speech, multimodal, with support for multiple training frameworks and inference engines to connect AI hardware with software ecosystems. It also supports various testing environments to comprehensively assess the performance of domestic AI chips in different scenarios.
README:
FlagPerf是智源研究院联合AI硬件厂商共建的一体化AI硬件评测引擎,旨在建立以产业实践为导向的指标体系,评测AI硬件在软件栈组合(模型+框架+编译器)下的实际能力。
-
构建多维度评测指标体系,不止关注“耗时”:
FlagPerf 指标体系除了衡量“芯片能否支持特定模型训练”的功能正确性指标之外,还包含更多维度的性能指标、资源使用指标以及生态适配能力指标等。
指标详细介绍见 这篇文章
-
支持多样例场景及任务,覆盖大模型训练推理场景
FlagPerf 已经涵盖计算机视觉、自然语言处理、语音、多模态等领域的**30余个经典模型,80余个训练样例,**支持评测AI硬件的训练和推理能力,以及大模型场景的推理任务评测。
-
支持多训练框架及推理引擎,灵活连接AI硬件与软件生态
在训练任务场景中,除了支持 PyTorch、TensorFlow,FlagPerf 还在积极与 PaddlePaddle、MindSpore 研发团队密切配合。作为国产训练框架的领军者,百度 Paddle团队、华为昇思MindSpore 团队正在将 Llama、GPT3 等明星模型集成至 FlagPerf 测试样例集。
在推理任务场景中,FlagPerf 适配了多家芯片厂商和训练框架研发团队的推理加速引擎,以更灵活地连接AI硬件与软件生态,拓宽评测的边界和效率,如英伟达TensorRT、昆仑芯XTCL(XPU Tensor Compilation Library)、天数智芯IxRT(Iluvatar CoreX RunTime)、PyTorch TorchInductor。
-
支持多测试环境,综合考察单卡、单机、多机性能
为全面评估国产AI芯片多样性、可扩展性、实际应用模拟情况,FlagPerf 设定了单卡、单机(通常是8卡)、多机三个测试环境,为不同的测试环境匹配了不同测试样例场景和任务。
注:当前FlagPerf在保证测试环境除芯片外其他条件一致的情况下,进行芯片本身的离线批处理评测,暂不支持集群和客户端的性能评估。
-
严格审核参评代码,关注“结果公平”,更关注“过程公正”
测试由智源研究院与众多芯片厂商联合展开。总体原则是确保客观、公平地评估芯片的通用性能,限制厂商开展有针对性的定制优化。在确定测试模型之后,首先由芯片厂商进行模型适配,这个过程中只允许厂商进行分布式通信、批数据量(batch size)等和硬件执行强相关的方面的代码修改,以确保模型能够在芯片上高效运行。其次由智源研究院依托基准测试平台FlagPerf对芯片能力开展测试,并确保测试过程顺利,芯片性能和稳定性得到最佳发挥。同时,所有测试代码均已开源,测试过程、数据可复现。
🎯 未来智源及众多AI硬件、框架团队还将共同拓展FlagPerf的评测场景,如开展集群性能的整体评估,以更全面的评估国产软硬件的性能。
- [4 Jun 2024]支持算子评测板块. #562
- [20 May 2024]支持FlagPerf在容器内启动评测. #542
- [6 May 2024]支持LLaMA3-8B megatron-core预训练. #526
- [1 Apr 2024]支持基础规格评测板块. #496
- [15 Jan 2024]支持megatron-Llama 70B预训练. #389
- [27 Oct 2023]支持Torch-llama2 7B预训练,#289
- [7 Oct 2023]支持Paddle-GPT3 预训练,#233
- [27 Sep 2023]发布v1.0版本,支持20余个经典模型,50余个训练样例,支持多家芯片厂商的训练或推理评测 #v1.0
- [3 Aug 2023]支持推理框架, 支持常见基础模型的离线批推理评测 #136
Full News
基础规格列表:
编号 | 规格名称 | 规格类型 | 英伟达 | 沐曦 | 昇腾 |
---|---|---|---|---|---|
1 | FP64算力 | 算力 |
算子或原语, 厂商专用工具 |
N/A | N/A |
2 | FP32算力 | 算力 |
算子或原语, 厂商专用工具 |
算子或原语 | 厂商专用工具 |
3 | TF32算力 | 算力 |
算子或原语, 厂商专用工具 |
算子或原语 | N/A |
4 | FP16算力 | 算力 |
算子或原语, 厂商专用工具 |
算子或原语 | 厂商专用工具 |
5 | BF16算力 | 算力 |
算子或原语, 厂商专用工具 |
算子或原语 | 厂商专用工具 |
6 | INT8算力 | 算力 | 厂商专用工具 | 厂商专用工具 | 厂商专用工具 |
7 | 主存储带宽 | 存储 |
算子或原语, 厂商专用工具 |
算子或原语 | N/A |
8 | 主存储容量 | 存储 |
算子或原语, 厂商专用工具 |
算子或原语 | N/A |
9 | CPU-芯片互连 | 互联 |
算子或原语, 厂商专用工具 |
N/A | 厂商专用工具 |
10 | 服务器内P2P直连 | 互联 |
算子或原语, 厂商专用工具 |
N/A | 厂商专用工具 |
11 | 服务器内MPI直连 | 互联 |
算子或原语, 厂商专用工具 |
N/A | N/A |
12 | 跨服务器P2P直连 | 互联 |
算子或原语, 厂商专用工具 |
N/A | N/A |
13 | 跨服务器MPI直连 | 互联 |
算子或原语, 厂商专用工具 |
N/A | N/A |
算子列表:
编号 | 规格名称 | 算子库 | 英伟达 |
---|---|---|---|
1 | mm-FP16 | nativetorch flaggems |
A100_40_SXM |
2 | sum-FP32 | nativetorch flaggems |
A100_40_SXM |
3 | linear-FP16 | nativetorch flaggems |
A100_40_SXM |
... | ... | ... | ... |
训练列表:
[!TIP] 请在表格下方向右滑动查看更多厂商
推理列表:
编号 | 模型名称 | 模型类型 | 英伟达 | 昆仑芯 | 天数智芯 | 腾讯九霄 | 沐曦 | 海飞科 |
1 | resnet50 | CV | f32/f16 | f32/f16 | f16 | f16 | f32/f16 | N/A |
2 | BertLarge | NLP | f32/f16 | W32A16 | f16 | N/A | f32/f16 | N/A |
3 | VisionTransformer | CV | f32/f16 | W32A16 | N/A | N/A | f32/f16 | N/A |
4 | Yolov5_large | CV | f32/f16 | f32 | f16 | N/A | f32/f16 | N/A |
5 | Stable Diffusion v1.4 | MultiModal | f32/f16 | f32 | N/A | N/A | f32/f16 | N/A |
6 | SwinTransformer | CV | f32/f16 | W32A16 | N/A | N/A | f32/f16 | N/A |
7 | Llama2-7B-mmlu | NLP | f32/f16 | N/A | N/A | N/A | f32/f16 | f32/f16 |
8 | Aquila-7B-mmlu | LLM | fp16 | N/A | N/A | N/A | f16 | N/A |
9 | SegmentAnything | MultiModal | fp16 | W32A16 | N/A | N/A | f32/f16 | N/A |
10 | DeepSeek-7B MMLU | LLM | fp16 | N/A | N/A | N/A | N/A | N/A |
11 | LLaMA3-8B MMLU | LLM | fp16 | N/A | N/A | N/A | N/A | N/A |
- 安装docker,python
- 确保硬件驱动、网络、硬件虚拟化等服务器基础配置齐全
- 确保可连中国大陆可访问网站,速率正常
- 确保可在容器内找到硬件
- 确保各服务器间root帐号的ssh信任关系和sudo免密
- 确保monitor相关工具已安装:包括cpu(sysstat)、内存(free)、功耗(ipmitool)、系统信息(加速卡状态查看命令)。例如ubuntu系统中,使用apt install [sysstat/ipmitool]安装
- 设置环境变量
export EXEC_IN_CONTAINER=True
- 确保容器内硬件驱动、网络、硬件虚拟化等服务器基础配置齐全
- 确保可连中国大陆可访问网站,速率正常
- 确保容器镜像、容器内软件包对应版本安装正确
- 确保可在容器内找到硬件
- 确保各服务器间root帐号的ssh信任关系和sudo免密
- 确保monitor相关工具已安装:包括cpu(sysstat)、内存(free)、功耗(ipmitool)、系统信息(加速卡状态查看命令)。例如ubuntu系统中,使用apt install [sysstat/ipmitool]安装
更多阅读:
- 下载FlagPerf并部署
# 先各服务器间root帐号的ssh信任关系和sudo免密配置
git clone https://github.com/FlagOpen/FlagPerf.git
cd FlagPerf/base/
- 修改机器配置文件
cd FlagPerf/base/
vim configs/host.yaml
具体项修改方式及原则见基础规格文档中的运行时流程章节
- 启动测试
cd FlagPerf/base/
sudo python3 run.py
- 同基础规格评测
- 下载FlagPerf并部署
# 先各服务器间root帐号的ssh信任关系和sudo免密配置
git clone https://github.com/FlagOpen/FlagPerf.git
cd FlagPerf/training/
pip3 install -r requirements.txt
- 修改机器配置文件
cd Flagperf/training/
vim run_benchmarks/config/cluster_conf.py
集群配置文件主要包括集群主机列表和SSH端口,修改HOSTS
和SSH_PORT
为机器实际地址
'''Cluster configs'''
#Hosts to run the benchmark. Each item is an IP address or a hostname.
HOSTS = ["10.1.2.3", "10.1.2.4", "10.1.2.5", "10.1.2.6"]
#ssh connection port
SSH_PORT = "22"
- 修改模型配置文件
cd Flagperf/training/
vim run_benchmarks/config/test_conf.py
必改项:
VENDOR = "nvidia" #选择本次运行的硬件
FLAGPERF_PATH="" # FlagPerf项目路径,如"/home/FlagPerf/training"
CASES={} # 本次运行的测例,按照对应模型readme准备好数据,修改模型对应的地址
#如运行"bert:pytorch_1.8:A100:1:8:1": "/raid/home_datasets_ckpt/bert/train/",需要把:后面的路径替换为本地路径
- 启动测试
python3 ./run_benchmarks/run.py
sudo python3 ./run_benchmarks/run.py
- 查看日志
cd result/run2023XXXX/运行模型/
# ls
round1
# ls round1/
10.1.2.2_noderank0
# cd 10.1.2.2_noderank0/
# ls
cpu_monitor.log pwr_monitor.log rank2.out.log rank5.out.log start_pytorch_task.log
mem_monitor.log rank0.out.log rank3.out.log rank6.out.log
nvidia_monitor.log rank1.out.log rank4.out.log rank7.out.log
# tail -n 6 rank0.out.log
[PerfLog] {"event": "STEP_END", "value": {"loss": 2.679504871368408, "embedding_average": 0.916015625, "epoch": 1, "end_training": true, "global_steps": 3397, "num_trained_samples": 869632, "learning_rate": 0.000175375, "seq/s": 822.455385237589}, "metadata": {"file": "/workspace/flagperf/training/benchmarks/cpm/pytorch/run_pretraining.py", "lineno": 127, "time_ms": 1669034171032, "rank": 0}}
[PerfLog] {"event": "EVALUATE", "metadata": {"file": "/workspace/flagperf/training/benchmarks/cpm/pytorch/run_pretraining.py", "lineno": 127, "time_ms": 1669034171032, "rank": 0}}
[PerfLog] {"event": "EPOCH_END", "metadata": {"file": "/workspace/flagperf/training/benchmarks/cpm/pytorch/run_pretraining.py", "lineno": 127, "time_ms": 1669034171159, "rank": 0}}
[PerfLog] {"event": "TRAIN_END", "metadata": {"file": "/workspace/flagperf/training/benchmarks/cpm/pytorch/run_pretraining.py", "lineno": 136, "time_ms": 1669034171159, "rank": 0}}
[PerfLog] {"event": "FINISHED", "value": {"e2e_time": 1661.6114165782928, "training_sequences_per_second": 579.0933420700227, "converged": true, "final_loss": 3.066718101501465, "final_mlm_accuracy": 0.920166015625, "raw_train_time": 1501.713, "init_time": 148.937}, "metadata": {"file": "/workspace/flagperf/training/benchmarks/cpm/pytorch/run_pretraining.py", "lineno": 158, "time_ms": 1669034171646, "rank": 0}}
- 下载FlagPerf并部署
# 先各服务器间root帐号的ssh信任关系和sudo免密配置
git clone https://github.com/FlagOpen/FlagPerf.git
cd FlagPerf/inference/
pip3 install -r requirements.txt
- 修改机器配置文件
cd Flagperf/inference/
vim configs/host.yaml
集群配置文件主要包括集群主机列表和SSH端口,修改HOSTS
和SSH_PORT
为机器实际地址
#必须修改项
FLAGPERF_PATH: "/home/FlagPerf/inference" #FlagPerf inference 路径
HOSTS: ["127.0.0.1"] # 机器地址
VENDOR = "nvidia" #测试机器对象,nvidia/kunlunxin/iluvatar
CASES: #待测case,记得修改数据地址
"resnet50:pytorch_1.13": "/raid/dataset/ImageNet/imagenet/val"
- 用户需要根据评测对象,配置configs//configuration.yaml,如不修改可用默认配置
batch_size: 256
# 1 item(like 1 sequence, 1 image) flops
# Attention! For transformer decoder like bert, 1 token cause 2*param flops, so we need 2*length*params like 2*512*0.33B here
# format: a_1*a*2*...*a_nea_0,like 2*512*0.33e9(bert) or 4.12e9(resnet50)
flops: 4.12e9
fp16: true
compiler: tensorrt
num_workers: 8
log_freq: 30
repeat: 5
# skip validation(will also skip create_model, export onnx). Assert exist_onnx_path != null
no_validation: false
# set a real onnx_path to use exist, or set it to anything but null to avoid export onnx manually(like torch-tensorrt)
exist_onnx_path: null
# set a exist path of engine file like resnet50.trt/resnet50.plan/resnet50.engine
exist_compiler_path: null
必改项:
VENDOR = "nvidia" #选择本次运行的硬件
FLAGPERF_PATH="" # FlagPerf项目路径,如"/home/FlagPerf/training"
CASES={} # 本次运行的测例,按照对应模型readme准备好数据,修改模型对应的地址
#如运行"bert:pytorch_1.8:A100:1:8:1": "/raid/home_datasets_ckpt/bert/train/",需要把:后面的路径替换为本地路径
- 启动测试
sudo python inference/run.py
如需参与共建FlagPerf基础规格、训练、推理评测,请参考详细文档,依次位于基础规格文档目录、训练文档目录、推理文档目录。
为了更直观的展示厂商参与共建的实际工作量,下面给出6个已经合并进FlagPerf,面向不同特征厂商的Pull Request。
-
模型训练适配适配
-
模型推理适配
- 第一次参与推理适配的工作内容较多。除了适配case外,还包括厂商的dockerfile、编译器实现方式、monitor等,如 #256
- 后续参与推理适配时,通常不需要适配工作量、仅需运行软件完成测试。如 #227
本项目基于Apache 2.0 license。
本项目的代码来源于不同的代码仓库,关于各模型测试Case的情况,请参考各模型测试Case目录的文档。
如有疑问,可以发送邮件至[email protected],或在issue中说明情况
For Tasks:
Click tags to check more tools for each tasksFor Jobs:
Alternative AI tools for FlagPerf
Similar Open Source Tools
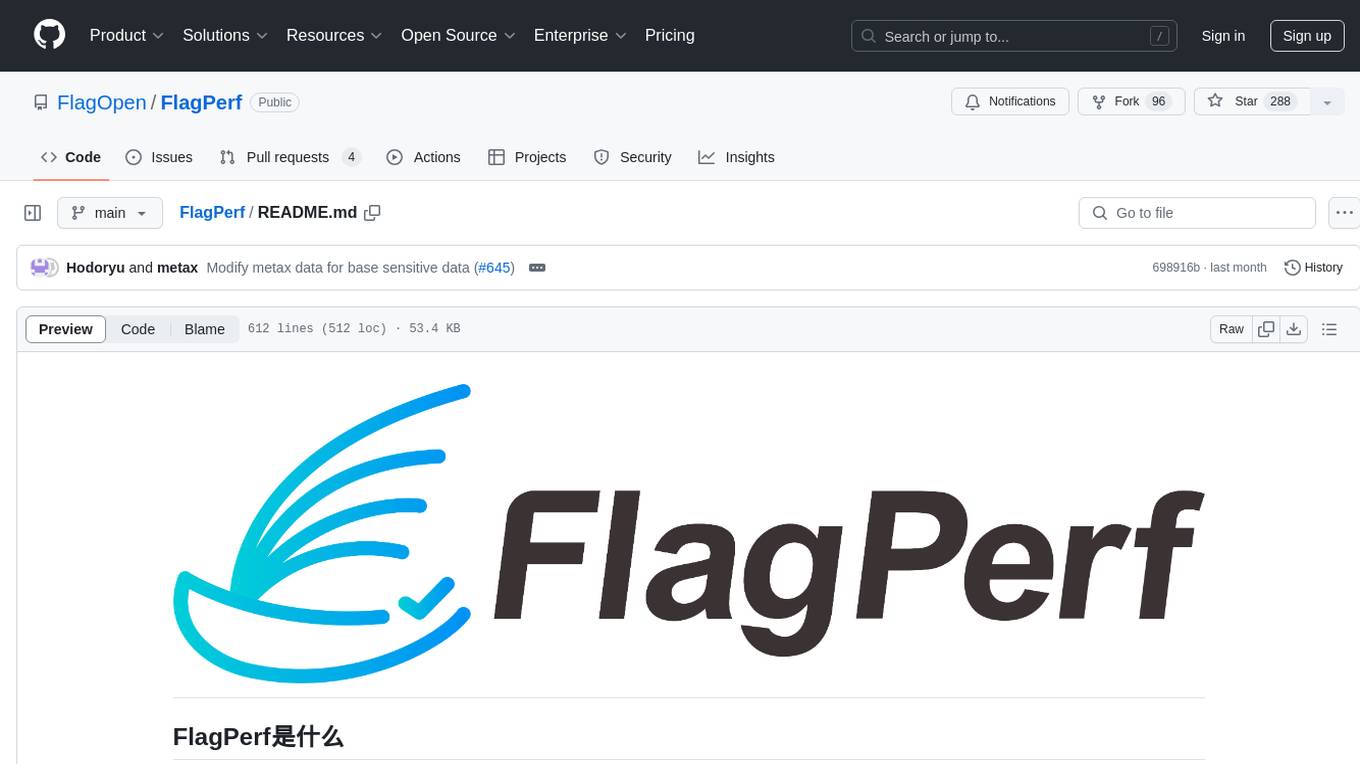
FlagPerf
FlagPerf is an integrated AI hardware evaluation engine jointly built by the Institute of Intelligence and AI hardware manufacturers. It aims to establish an industry-oriented metric system to evaluate the actual capabilities of AI hardware under software stack combinations (model + framework + compiler). FlagPerf features a multidimensional evaluation metric system that goes beyond just measuring 'whether the chip can support specific model training.' It covers various scenarios and tasks, including computer vision, natural language processing, speech, multimodal, with support for multiple training frameworks and inference engines to connect AI hardware with software ecosystems. It also supports various testing environments to comprehensively assess the performance of domestic AI chips in different scenarios.
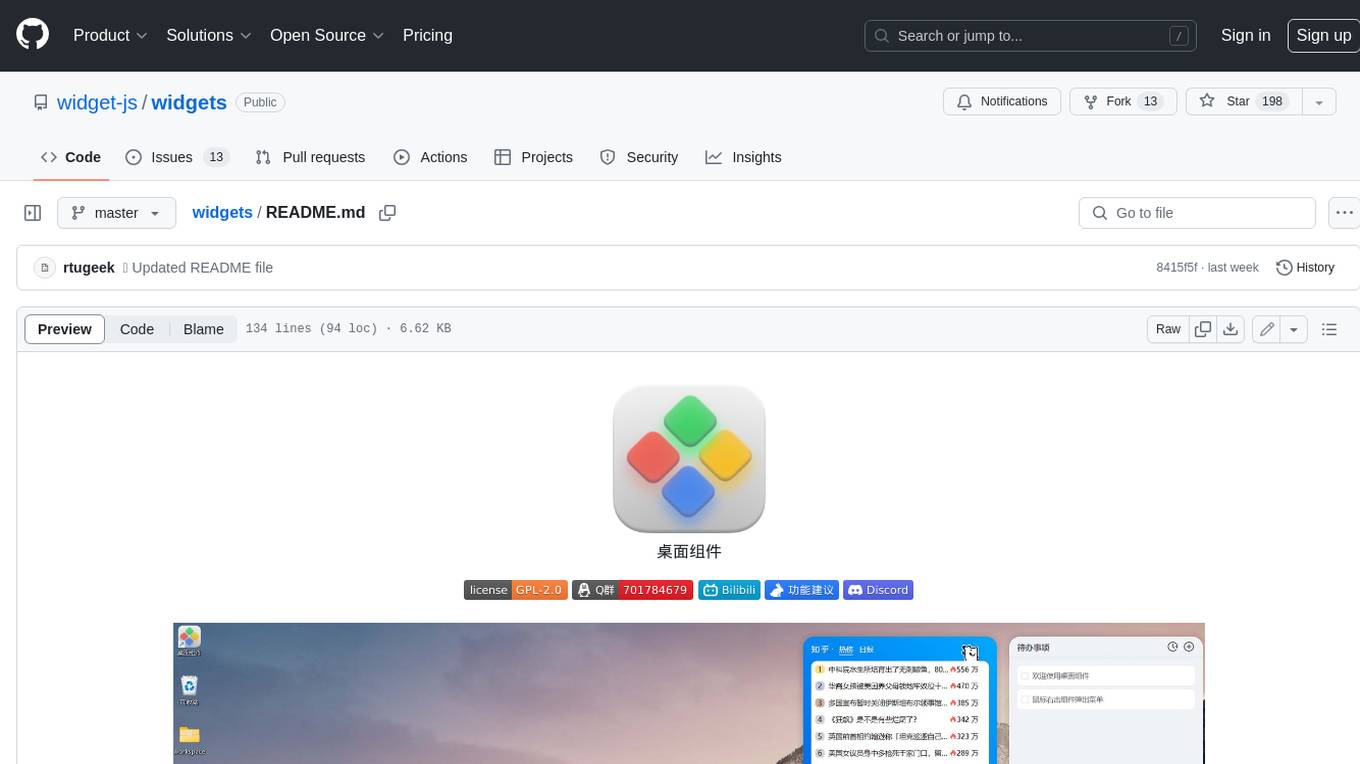
widgets
Widgets is a desktop component front-end open source component. The project is still being continuously improved. The desktop component client can be downloaded and run in two ways: 1. https://www.microsoft.com/store/productId/9NPR50GQ7T53 2. https://widgetjs.cn After cloning the code, you need to download the dependency in the project directory: `shell pnpm install` and run: `shell pnpm serve`
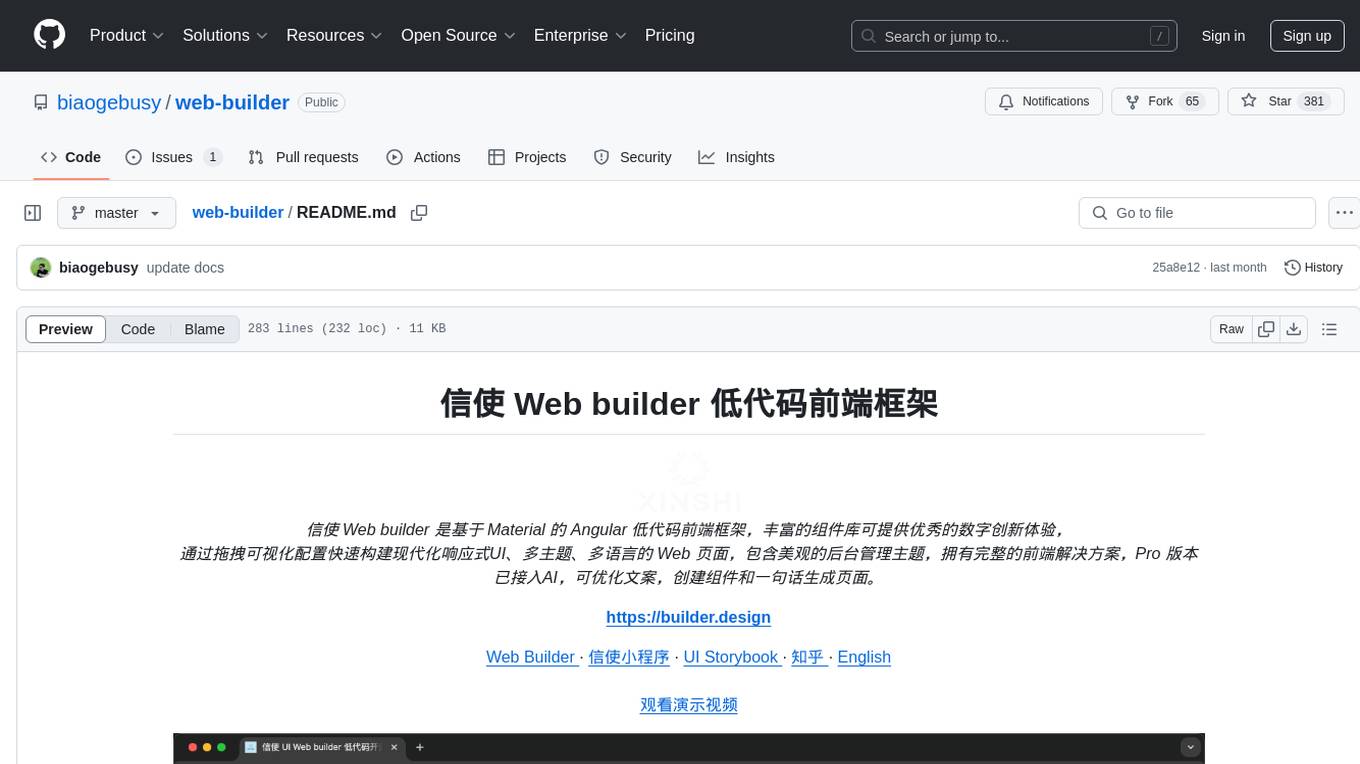
web-builder
Web Builder is a low-code front-end framework based on Material for Angular, offering a rich component library for excellent digital innovation experience. It allows rapid construction of modern responsive UI, multi-theme, multi-language web pages through drag-and-drop visual configuration. The framework includes a beautiful admin theme, complete front-end solutions, and AI integration in the Pro version for optimizing copy, creating components, and generating pages with a single sentence.
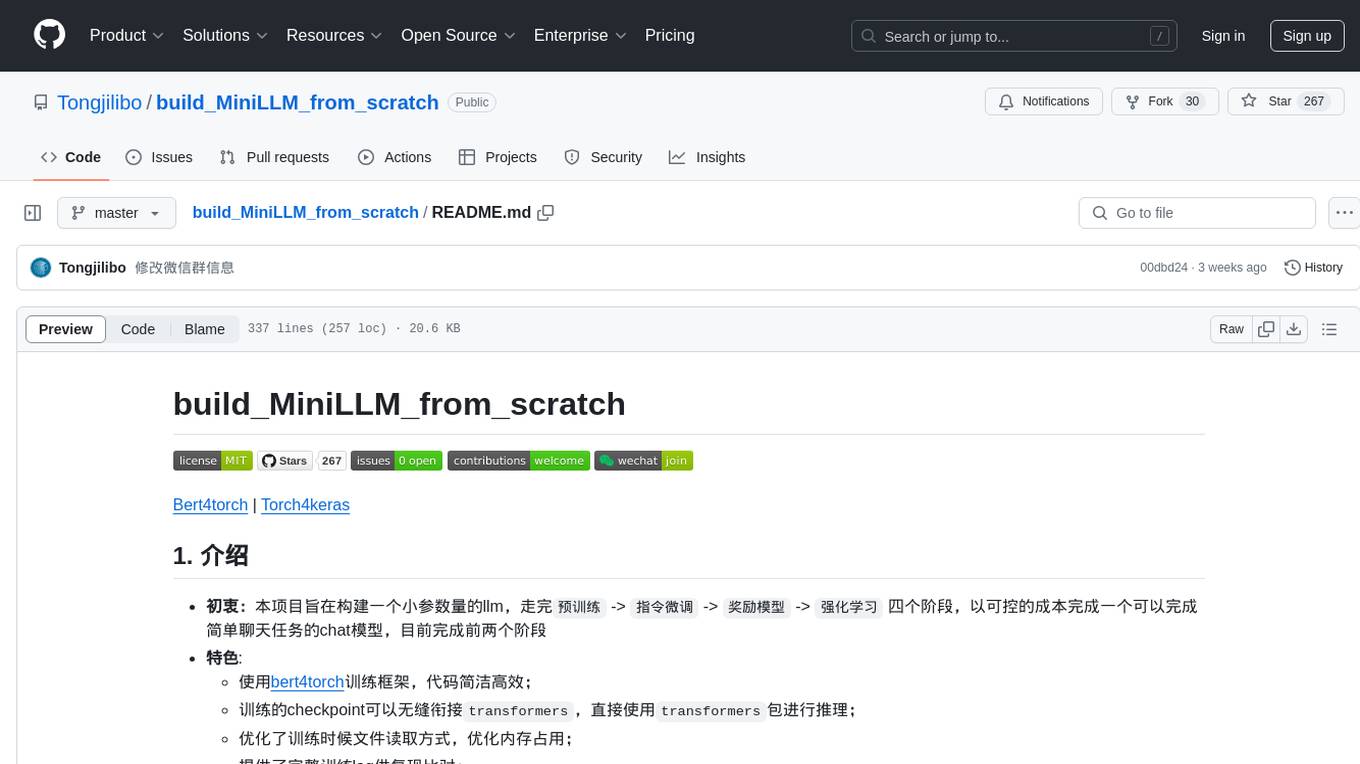
build_MiniLLM_from_scratch
This repository aims to build a low-parameter LLM model through pretraining, fine-tuning, model rewarding, and reinforcement learning stages to create a chat model capable of simple conversation tasks. It features using the bert4torch training framework, seamless integration with transformers package for inference, optimized file reading during training to reduce memory usage, providing complete training logs for reproducibility, and the ability to customize robot attributes. The chat model supports multi-turn conversations. The trained model currently only supports basic chat functionality due to limitations in corpus size, model scale, SFT corpus size, and quality.
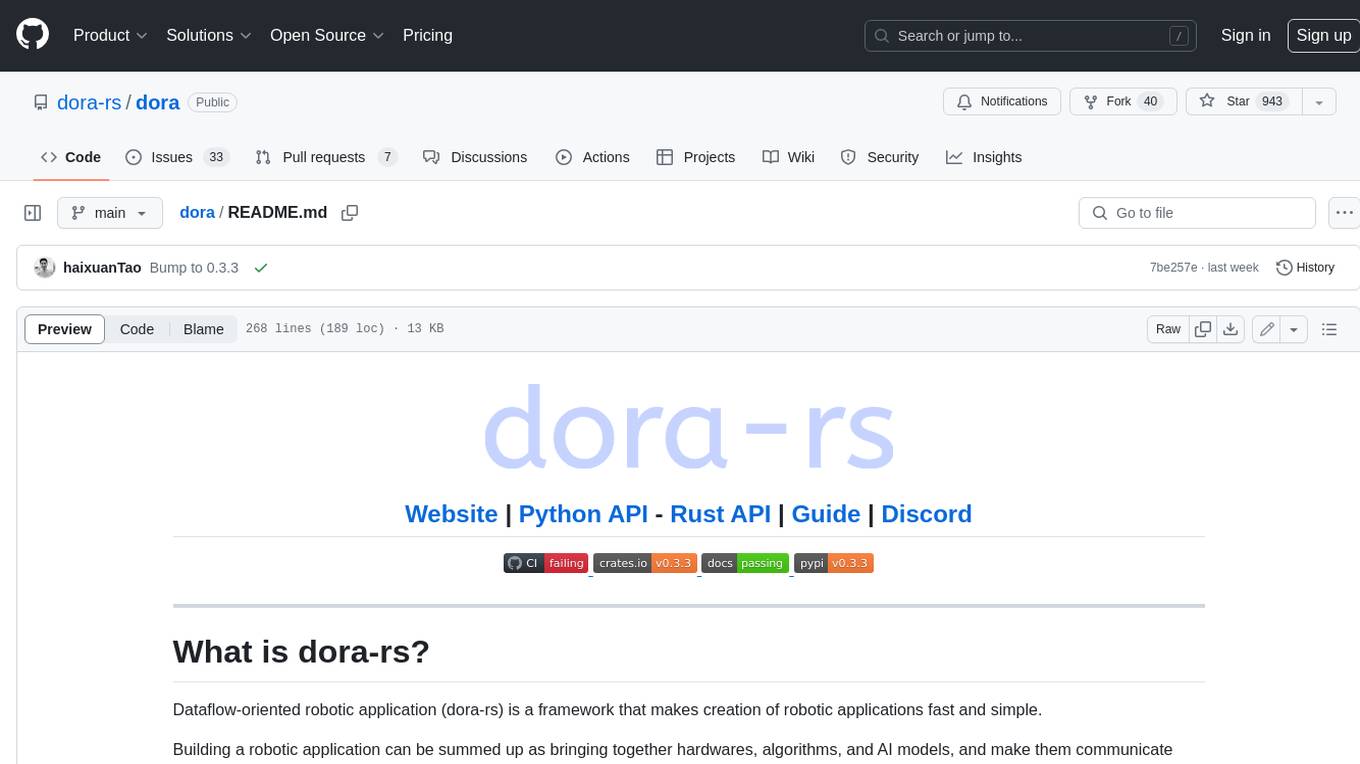
dora
Dataflow-oriented robotic application (dora-rs) is a framework that makes creation of robotic applications fast and simple. Building a robotic application can be summed up as bringing together hardwares, algorithms, and AI models, and make them communicate with each others. At dora-rs, we try to: make integration of hardware and software easy by supporting Python, C, C++, and also ROS2. make communication low latency by using zero-copy Arrow messages. dora-rs is still experimental and you might experience bugs, but we're working very hard to make it stable as possible.
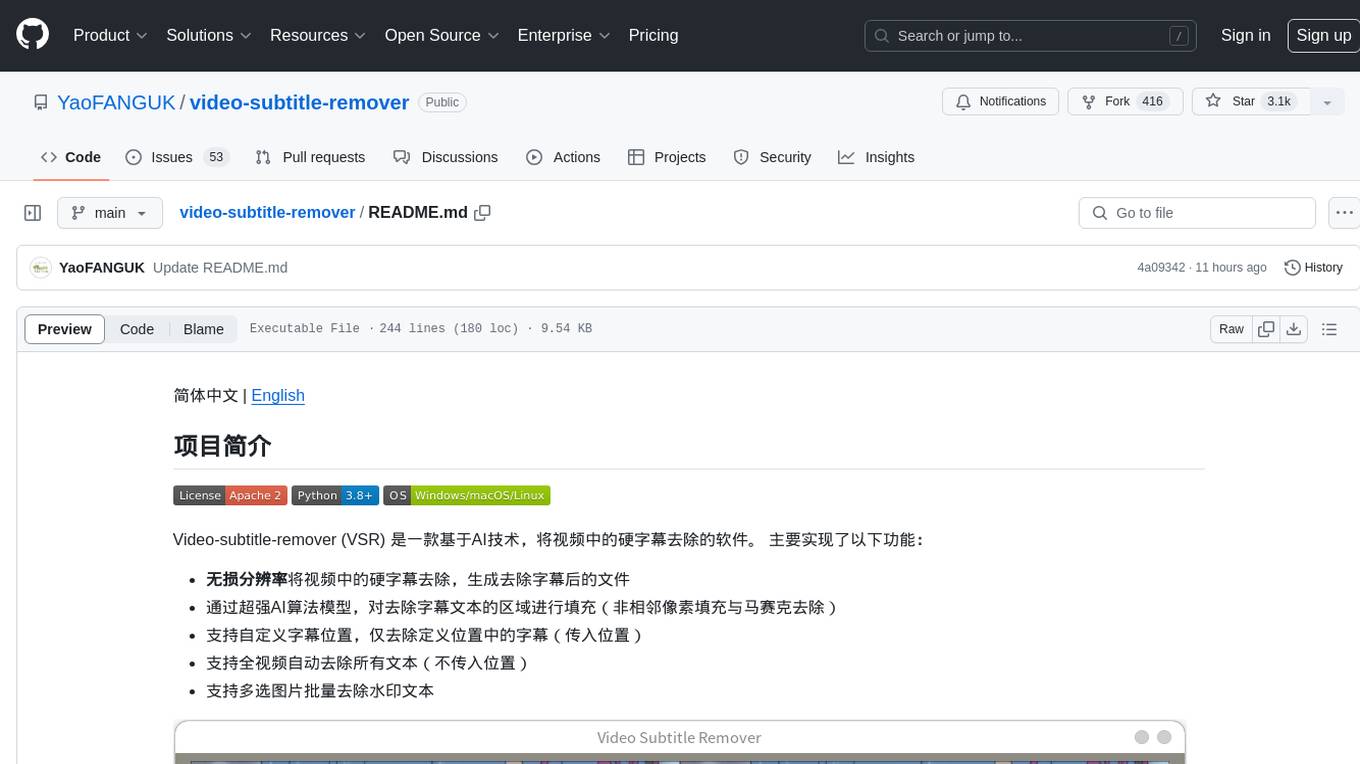
video-subtitle-remover
Video-subtitle-remover (VSR) is a software based on AI technology that removes hard subtitles from videos. It achieves the following functions: - Lossless resolution: Remove hard subtitles from videos, generate files with subtitles removed - Fill the region of removed subtitles using a powerful AI algorithm model (non-adjacent pixel filling and mosaic removal) - Support custom subtitle positions, only remove subtitles in defined positions (input position) - Support automatic removal of all text in the entire video (no input position required) - Support batch removal of watermark text from multiple images.
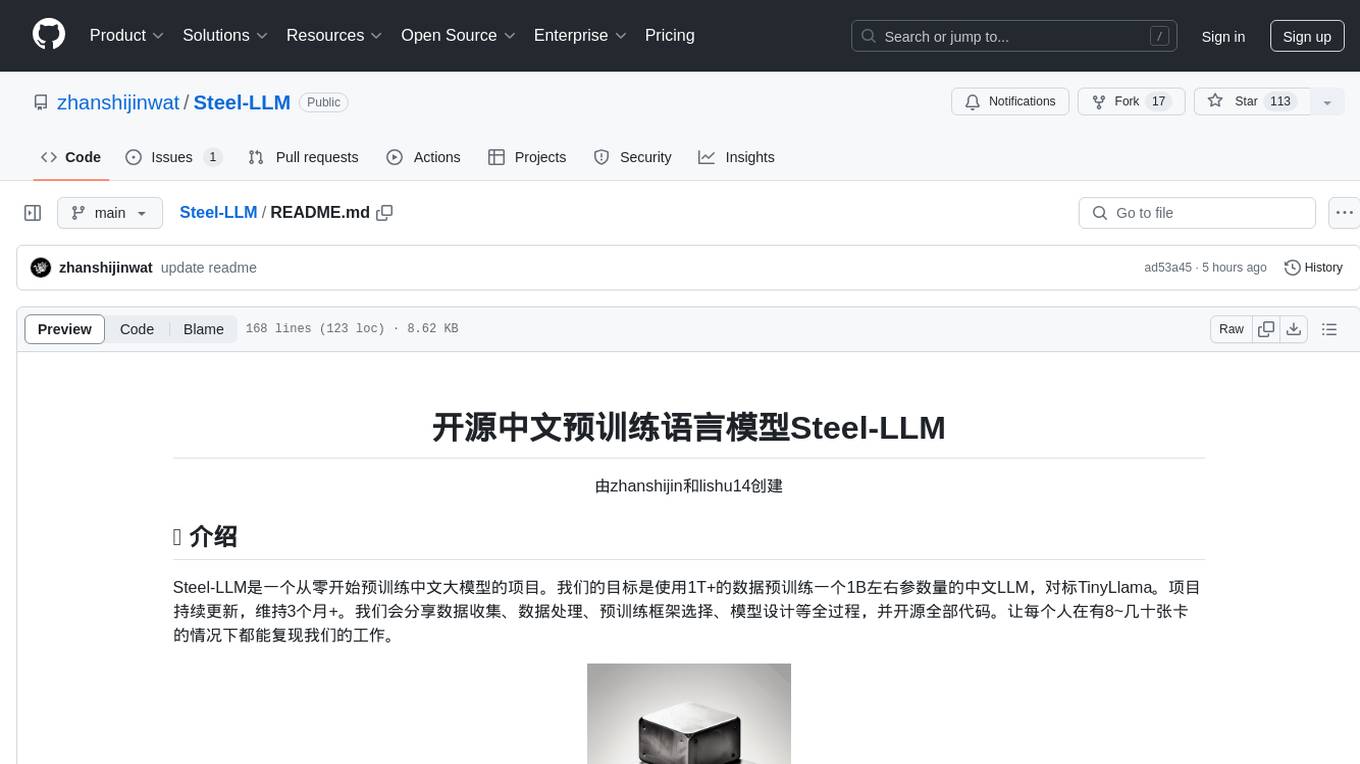
Steel-LLM
Steel-LLM is a project to pre-train a large Chinese language model from scratch using over 1T of data to achieve a parameter size of around 1B, similar to TinyLlama. The project aims to share the entire process including data collection, data processing, pre-training framework selection, model design, and open-source all the code. The goal is to enable reproducibility of the work even with limited resources. The name 'Steel' is inspired by a band '万能青年旅店' and signifies the desire to create a strong model despite limited conditions. The project involves continuous data collection of various cultural elements, trivia, lyrics, niche literature, and personal secrets to train the LLM. The ultimate aim is to fill the model with diverse data and leave room for individual input, fostering collaboration among users.
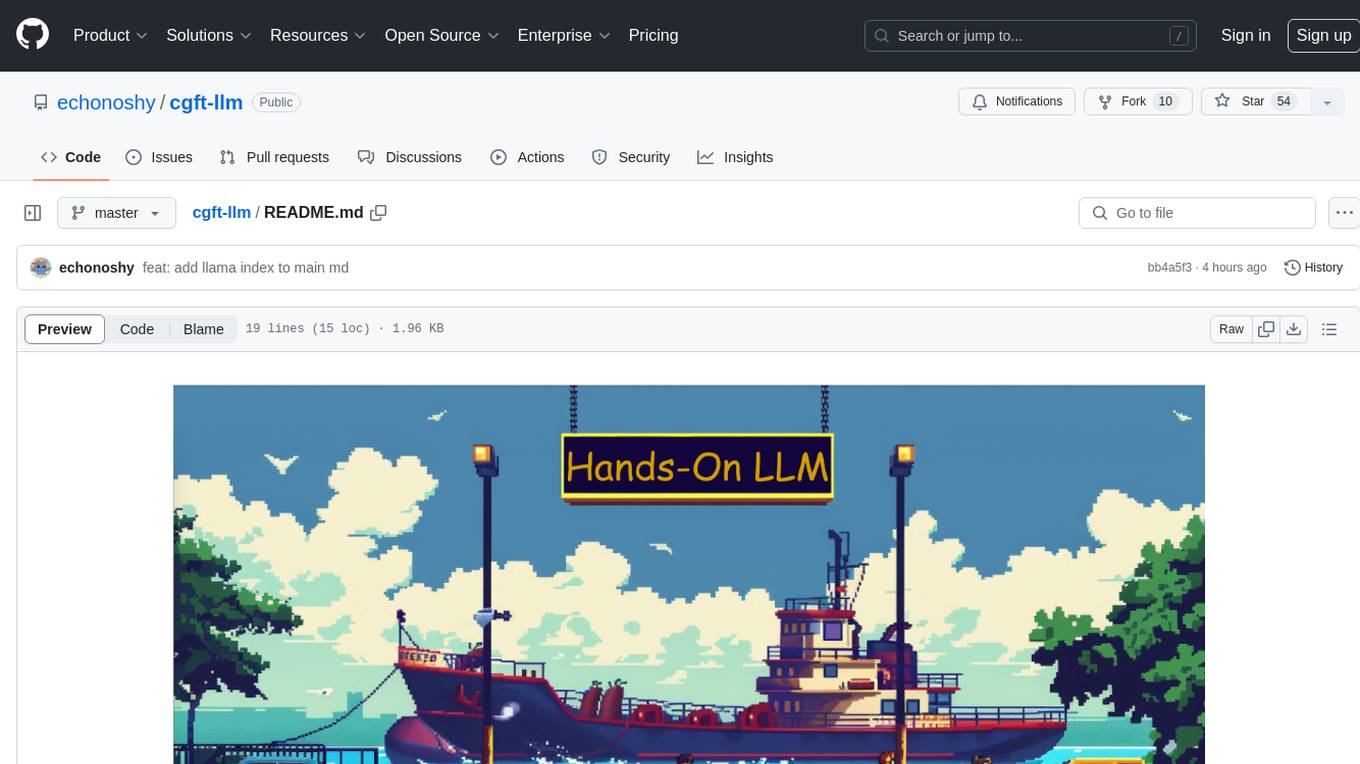
cgft-llm
The cgft-llm repository is a collection of video tutorials and documentation for implementing large models. It provides guidance on topics such as fine-tuning llama3 with llama-factory, lightweight deployment and quantization using llama.cpp, speech generation with ChatTTS, introduction to Ollama for large model deployment, deployment tools for vllm and paged attention, and implementing RAG with llama-index. Users can find detailed code documentation and video tutorials for each project in the repository.
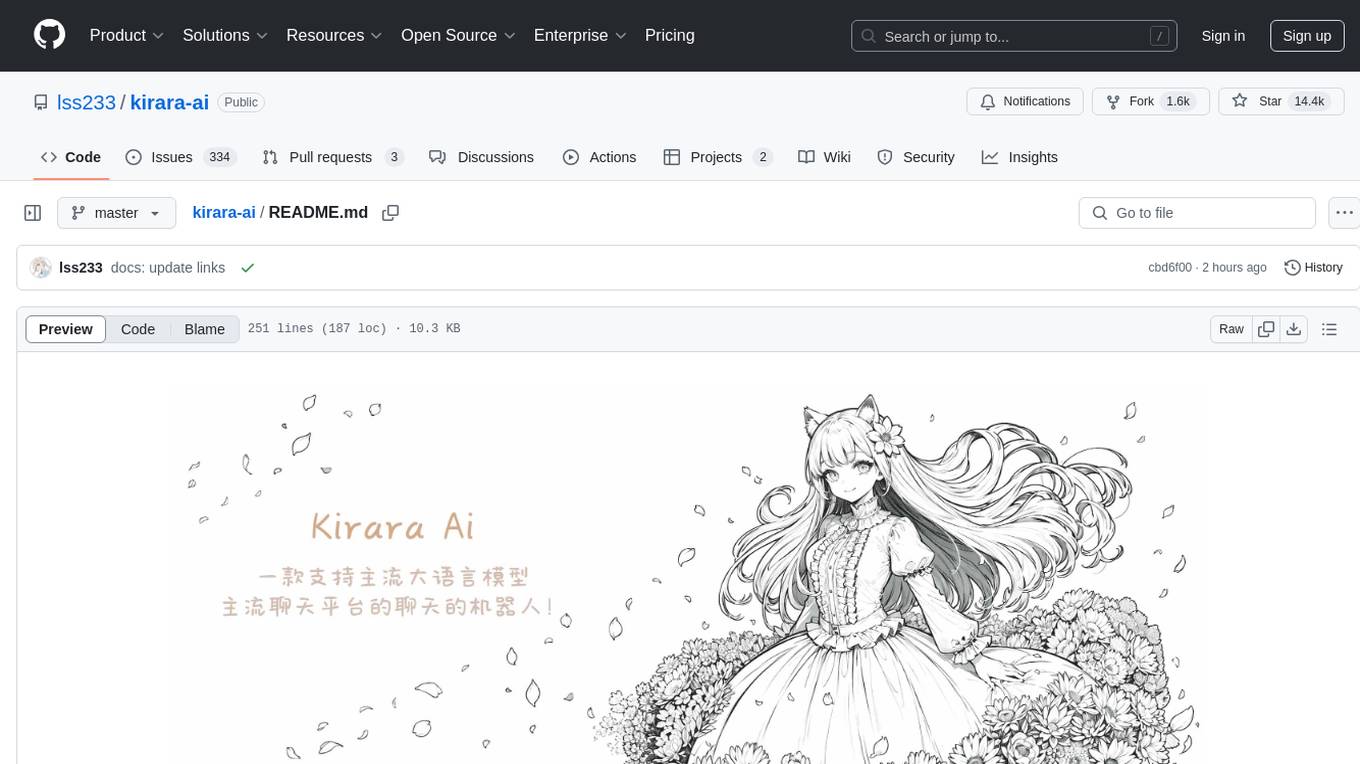
kirara-ai
Kirara AI is a chatbot that supports mainstream large language models and chat platforms. It provides features such as image sending, keyword-triggered replies, multi-account support, personality settings, and support for various chat platforms like QQ, Telegram, Discord, and WeChat. The tool also supports HTTP server for Web API, popular large models like OpenAI and DeepSeek, plugin mechanism, conditional triggers, admin commands, drawing models, voice replies, multi-turn conversations, cross-platform message sending, custom workflows, web management interface, and built-in Frpc intranet penetration.
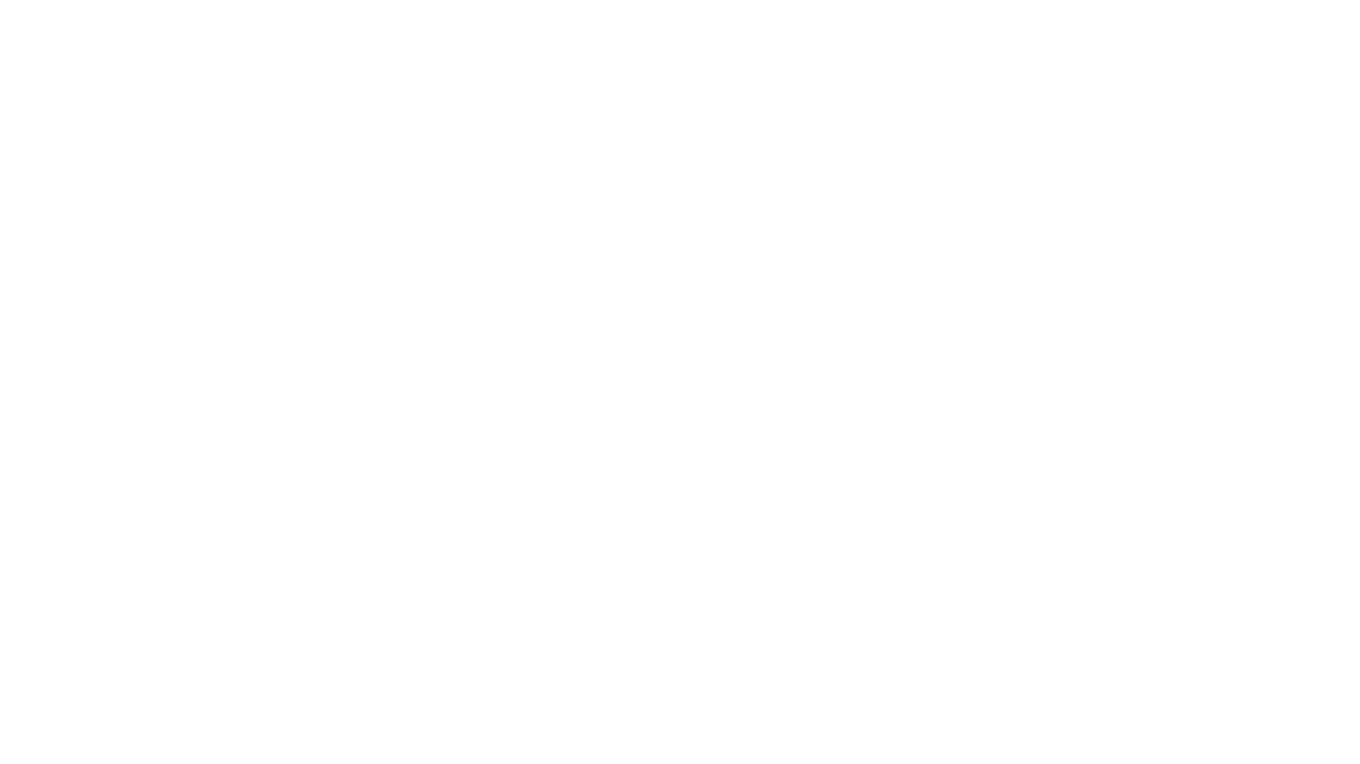
chatluna
Chatluna is a machine learning model plugin that provides chat services with large language models. It is highly extensible, supports multiple output formats, and offers features like custom conversation presets, rate limiting, and context awareness. Users can deploy Chatluna under Koishi without additional configuration. The plugin supports various models/platforms like OpenAI, Azure OpenAI, Google Gemini, and more. It also provides preset customization using YAML files and allows for easy forking and development within Koishi projects. However, the project lacks web UI, HTTP server, and project documentation, inviting contributions from the community.
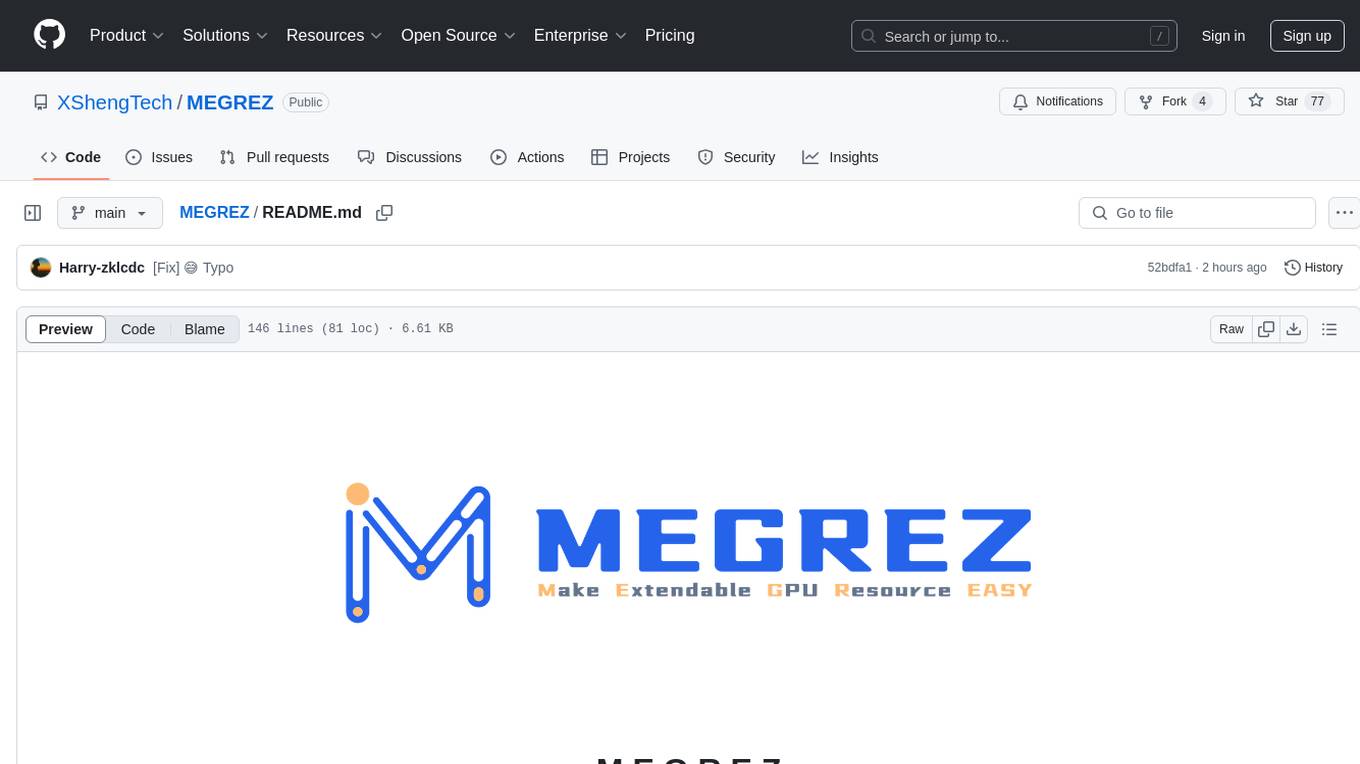
MEGREZ
MEGREZ is a modern and elegant open-source high-performance computing platform that efficiently manages GPU resources. It allows for easy container instance creation, supports multiple nodes/multiple GPUs, modern UI environment isolation, customizable performance configurations, and user data isolation. The platform also comes with pre-installed deep learning environments, supports multiple users, features a VSCode web version, resource performance monitoring dashboard, and Jupyter Notebook support.
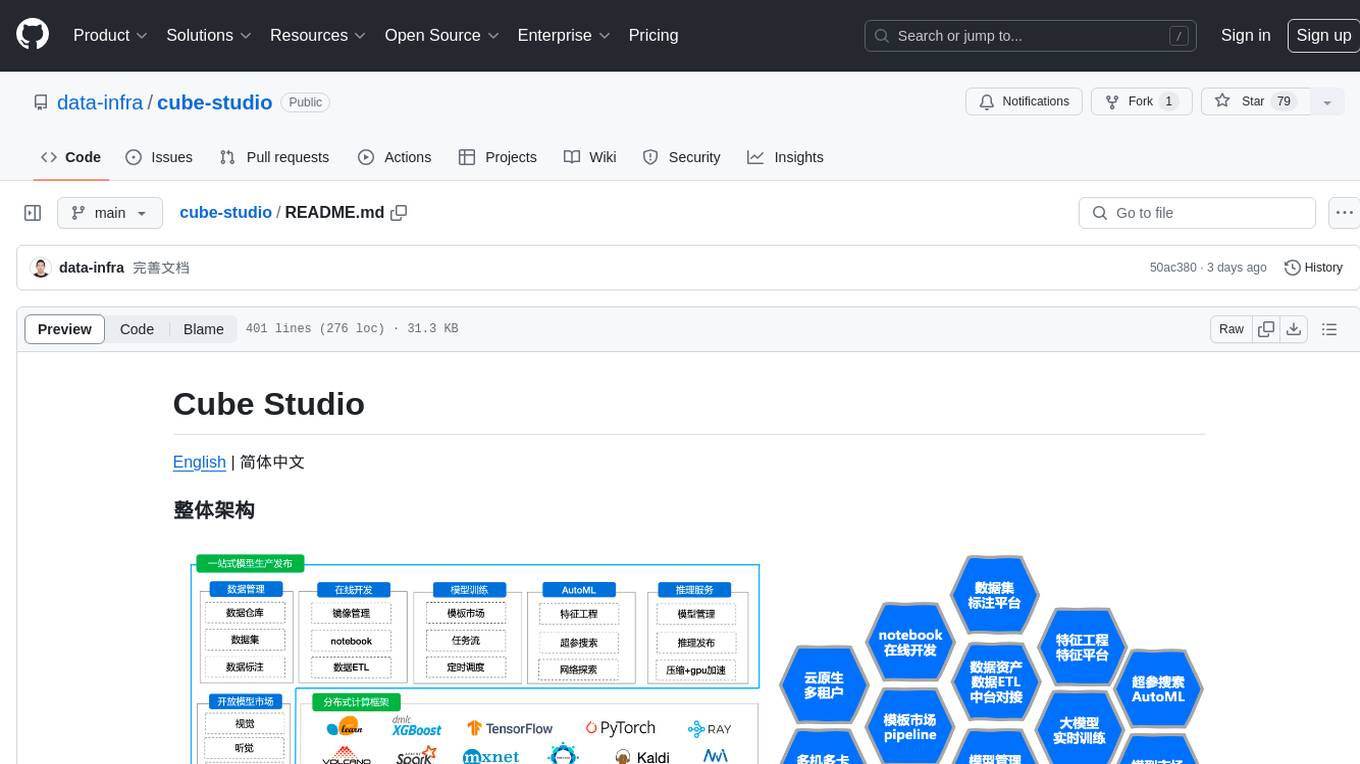
cube-studio
Cube Studio is an open-source all-in-one cloud-native machine learning platform that provides various functionalities such as project group management, network configuration, user management, role management, billing functions, SSO single sign-on, support for multiple computing power types, support for multiple resource groups and clusters, edge cluster support, serverless cluster mode support, database storage support, machine resource management, storage disk management, internationalization capabilities, data map management, data calculation, ETL orchestration, data set management, data annotation, image/audio/text dataset support, feature processing, traditional machine learning algorithms, distributed deep learning frameworks, distributed acceleration frameworks, model evaluation, model format conversion, model registration, model deployment, distributed media processing, custom operators, automatic learning, custom training images, automatic parameter tuning, TensorBoard jobs, internal services, model management, inference services, monitoring, model application management, model marketplace, model development, model fine-tuning, web model deployment, automated annotation, dataset SDK, notebook SDK, pipeline training SDK, inference service SDK, large model distributed training, large model inference, large model fine-tuning, intelligent conversation, private knowledge base, model deployment for WeChat public accounts, enterprise WeChat group chatbot integration, DingTalk group chatbot integration, and more. Cube Studio offers template-based functionality for data import/export, data processing, feature processing, machine learning frameworks, machine learning algorithms, deep learning frameworks, model processing, model serving, monitoring, and more.
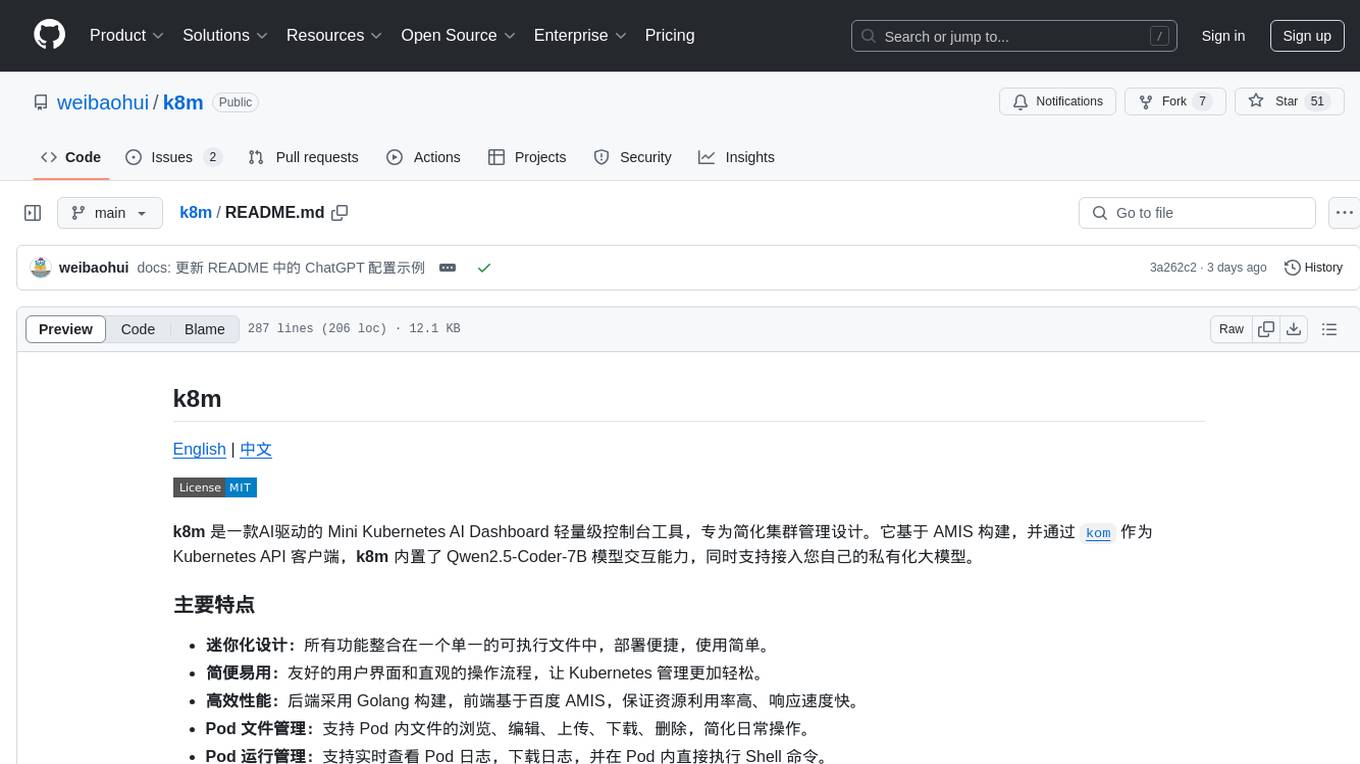
k8m
k8m is an AI-driven Mini Kubernetes AI Dashboard lightweight console tool designed to simplify cluster management. It is built on AMIS and uses 'kom' as the Kubernetes API client. k8m has built-in Qwen2.5-Coder-7B model interaction capabilities and supports integration with your own private large models. Its key features include miniaturized design for easy deployment, user-friendly interface for intuitive operation, efficient performance with backend in Golang and frontend based on Baidu AMIS, pod file management for browsing, editing, uploading, downloading, and deleting files, pod runtime management for real-time log viewing, log downloading, and executing shell commands within pods, CRD management for automatic discovery and management of CRD resources, and intelligent translation and diagnosis based on ChatGPT for YAML property translation, Describe information interpretation, AI log diagnosis, and command recommendations, providing intelligent support for managing k8s. It is cross-platform compatible with Linux, macOS, and Windows, supporting multiple architectures like x86 and ARM for seamless operation. k8m's design philosophy is 'AI-driven, lightweight and efficient, simplifying complexity,' helping developers and operators quickly get started and easily manage Kubernetes clusters.
For similar tasks
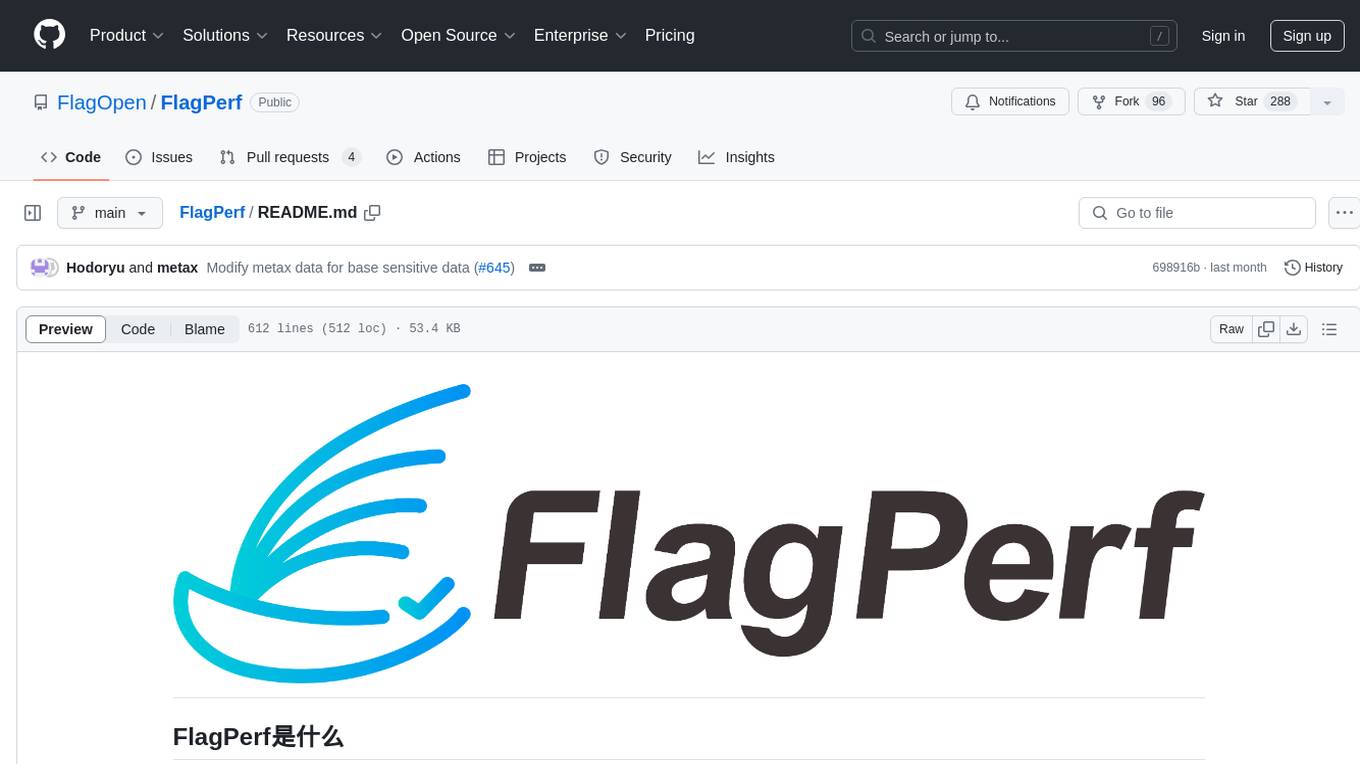
FlagPerf
FlagPerf is an integrated AI hardware evaluation engine jointly built by the Institute of Intelligence and AI hardware manufacturers. It aims to establish an industry-oriented metric system to evaluate the actual capabilities of AI hardware under software stack combinations (model + framework + compiler). FlagPerf features a multidimensional evaluation metric system that goes beyond just measuring 'whether the chip can support specific model training.' It covers various scenarios and tasks, including computer vision, natural language processing, speech, multimodal, with support for multiple training frameworks and inference engines to connect AI hardware with software ecosystems. It also supports various testing environments to comprehensively assess the performance of domestic AI chips in different scenarios.
For similar jobs
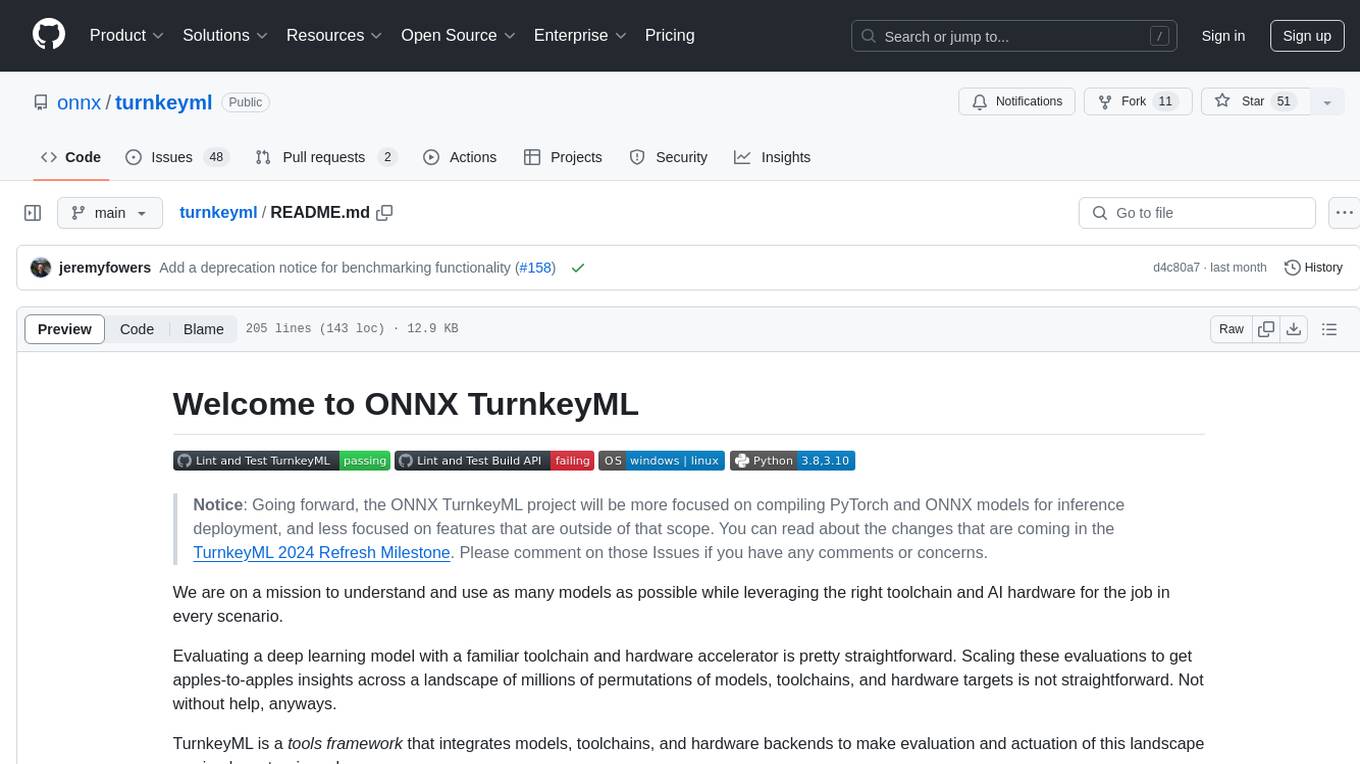
turnkeyml
TurnkeyML is a tools framework that integrates models, toolchains, and hardware backends to simplify the evaluation and actuation of deep learning models. It supports use cases like exporting ONNX files, performance validation, functional coverage measurement, stress testing, and model insights analysis. The framework consists of analysis, build, runtime, reporting tools, and a models corpus, seamlessly integrated to provide comprehensive functionality with simple commands. Extensible through plugins, it offers support for various export and optimization tools and AI runtimes. The project is actively seeking collaborators and is licensed under Apache 2.0.
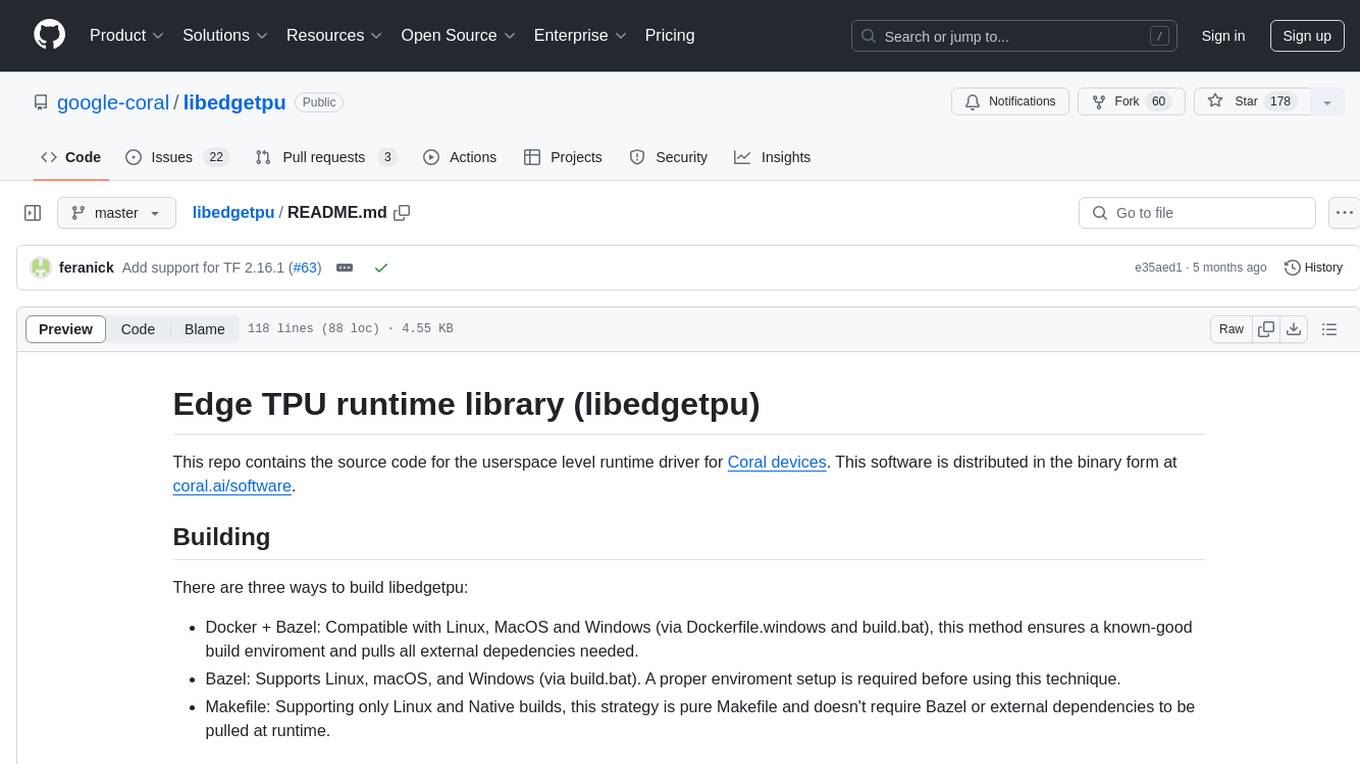
libedgetpu
This repository contains the source code for the userspace level runtime driver for Coral devices. The software is distributed in binary form at coral.ai/software. Users can build the library using Docker + Bazel, Bazel, or Makefile methods. It supports building on Linux, macOS, and Windows. The library is used to enable the Edge TPU runtime, which may heat up during operation. Google does not accept responsibility for any loss or damage if the device is operated outside the recommended ambient temperature range.
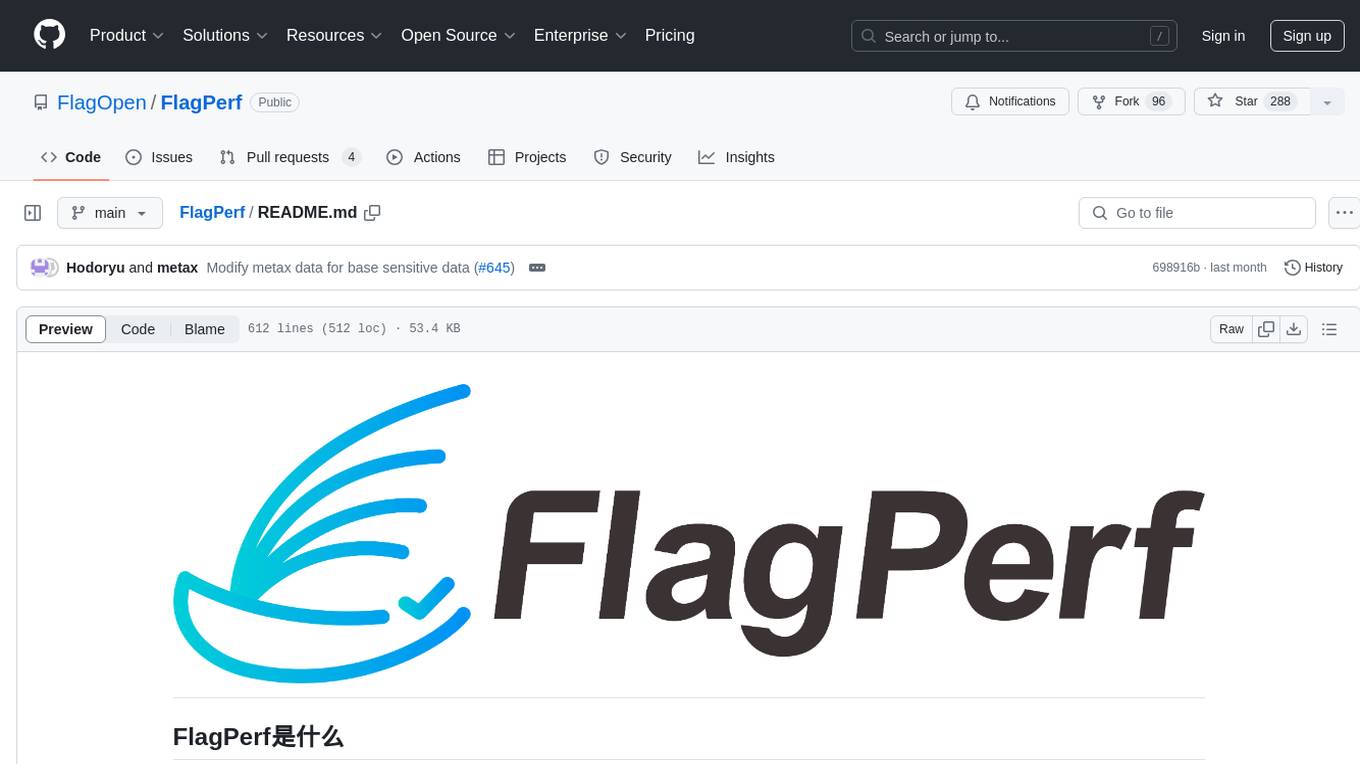
FlagPerf
FlagPerf is an integrated AI hardware evaluation engine jointly built by the Institute of Intelligence and AI hardware manufacturers. It aims to establish an industry-oriented metric system to evaluate the actual capabilities of AI hardware under software stack combinations (model + framework + compiler). FlagPerf features a multidimensional evaluation metric system that goes beyond just measuring 'whether the chip can support specific model training.' It covers various scenarios and tasks, including computer vision, natural language processing, speech, multimodal, with support for multiple training frameworks and inference engines to connect AI hardware with software ecosystems. It also supports various testing environments to comprehensively assess the performance of domestic AI chips in different scenarios.
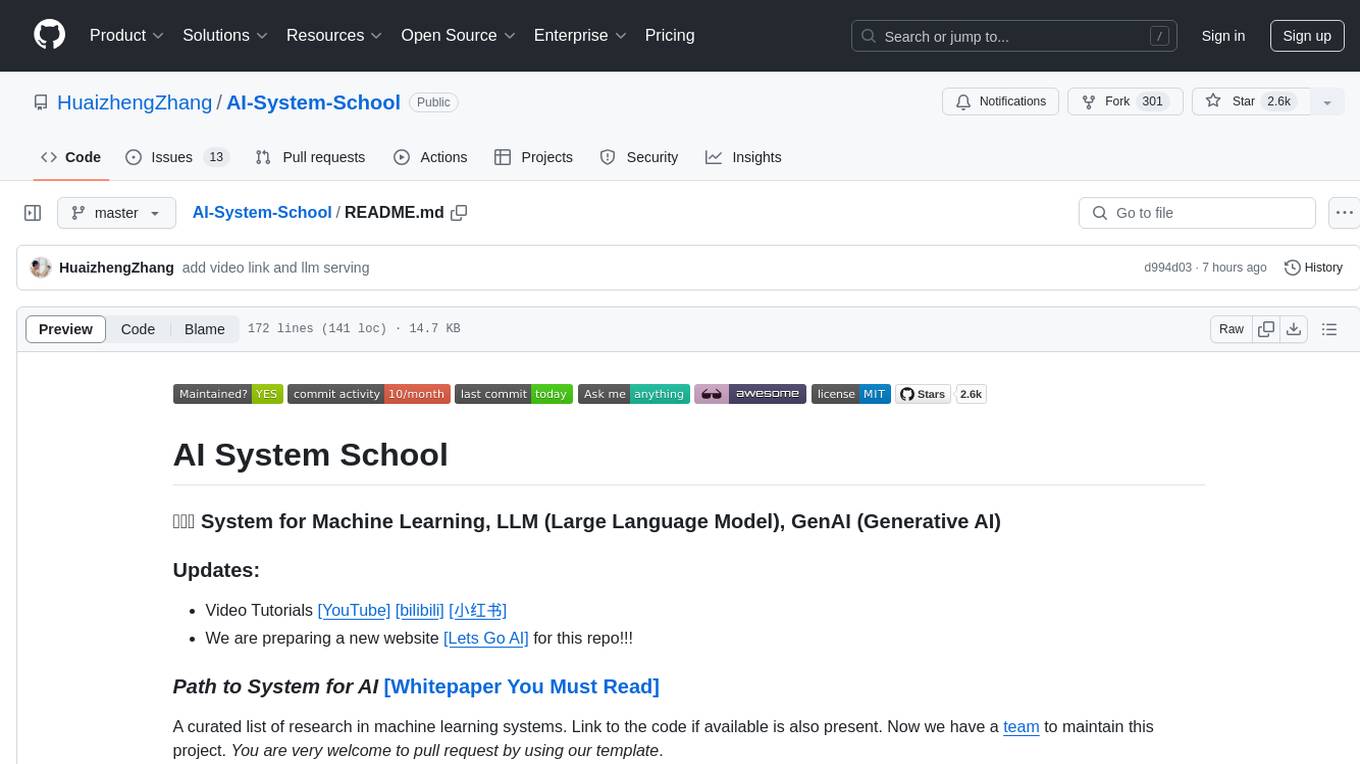
AI-System-School
AI System School is a curated list of research in machine learning systems, focusing on ML/DL infra, LLM infra, domain-specific infra, ML/LLM conferences, and general resources. It provides resources such as data processing, training systems, video systems, autoML systems, and more. The repository aims to help users navigate the landscape of AI systems and machine learning infrastructure, offering insights into conferences, surveys, books, videos, courses, and blogs related to the field.
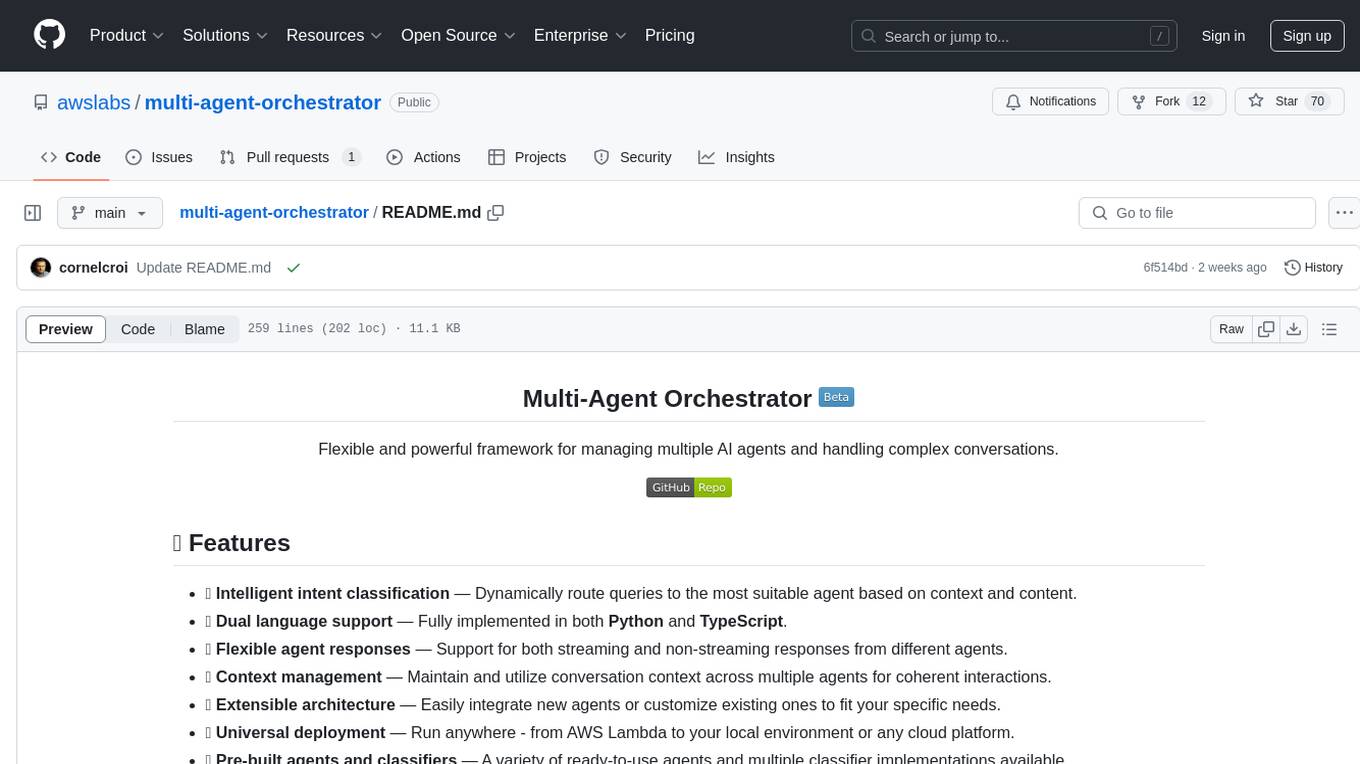
multi-agent-orchestrator
Multi-Agent Orchestrator is a flexible and powerful framework for managing multiple AI agents and handling complex conversations. It intelligently routes queries to the most suitable agent based on context and content, supports dual language implementation in Python and TypeScript, offers flexible agent responses, context management across agents, extensible architecture for customization, universal deployment options, and pre-built agents and classifiers. It is suitable for various applications, from simple chatbots to sophisticated AI systems, accommodating diverse requirements and scaling efficiently.
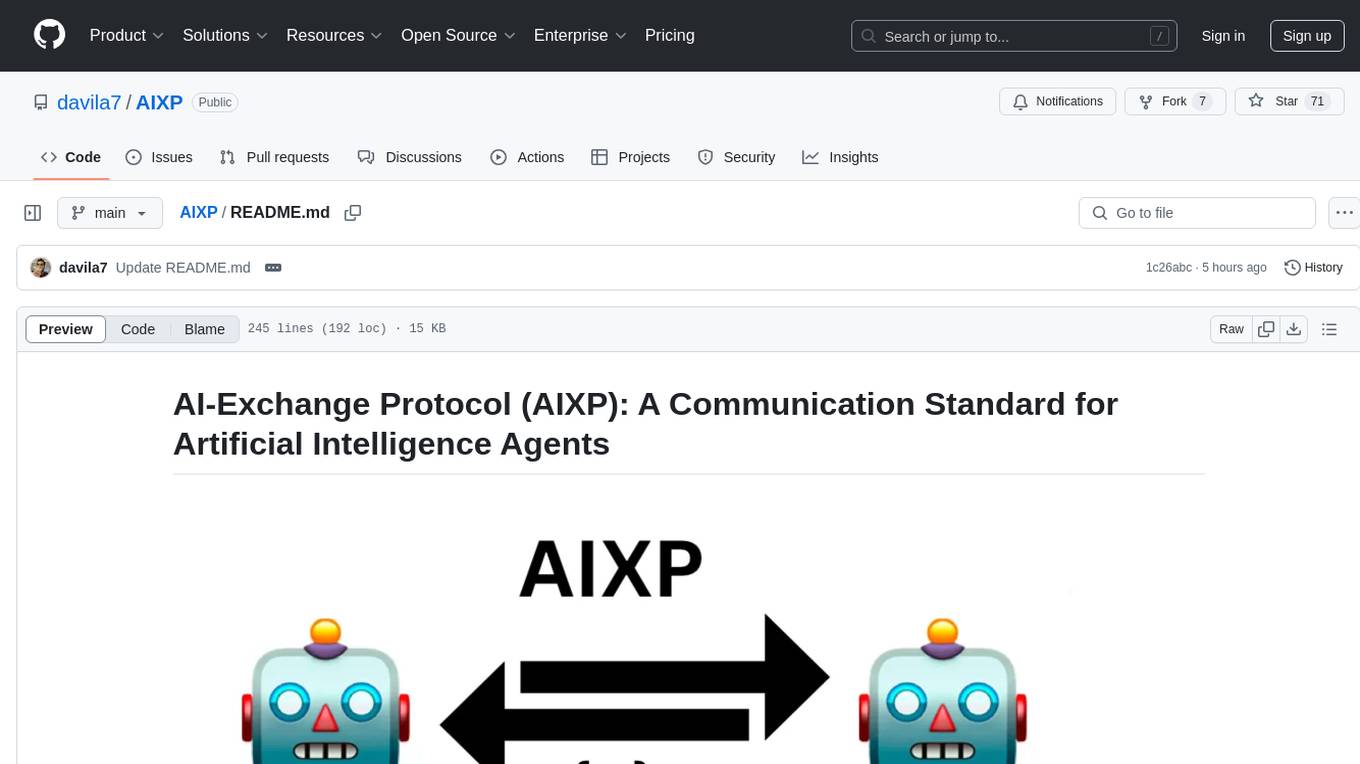
AIXP
The AI-Exchange Protocol (AIXP) is a communication standard designed to facilitate information and result exchange between artificial intelligence agents. It aims to enhance interoperability and collaboration among various AI systems by establishing a common framework for communication. AIXP includes components for communication, loop prevention, and task finalization, ensuring secure and efficient collaboration while avoiding infinite communication loops. The protocol defines access points, data formats, authentication, authorization, versioning, loop detection, status codes, error messages, and task completion verification. AIXP enables AI agents to collaborate seamlessly and complete tasks effectively, contributing to the overall efficiency and reliability of AI systems.
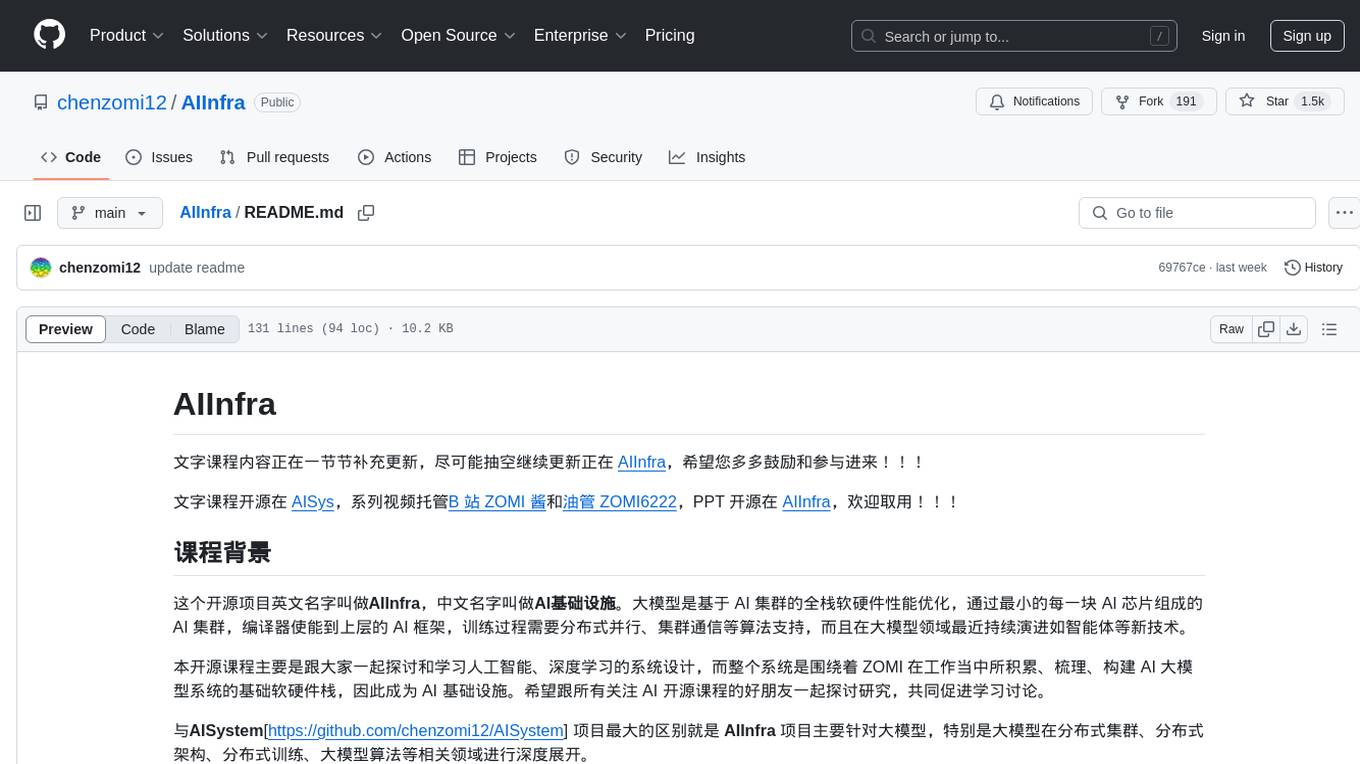
AIInfra
AIInfra is an open-source project focused on AI infrastructure, specifically targeting large models in distributed clusters, distributed architecture, distributed training, and algorithms related to large models. The project aims to explore and study system design in artificial intelligence and deep learning, with a focus on the hardware and software stack for building AI large model systems. It provides a comprehensive curriculum covering topics such as AI chip principles, communication and storage, AI clusters, large model training, and inference, as well as algorithms for large models. The course is designed for undergraduate and graduate students, as well as professionals working with AI large model systems, to gain a deep understanding of AI computer system architecture and design.
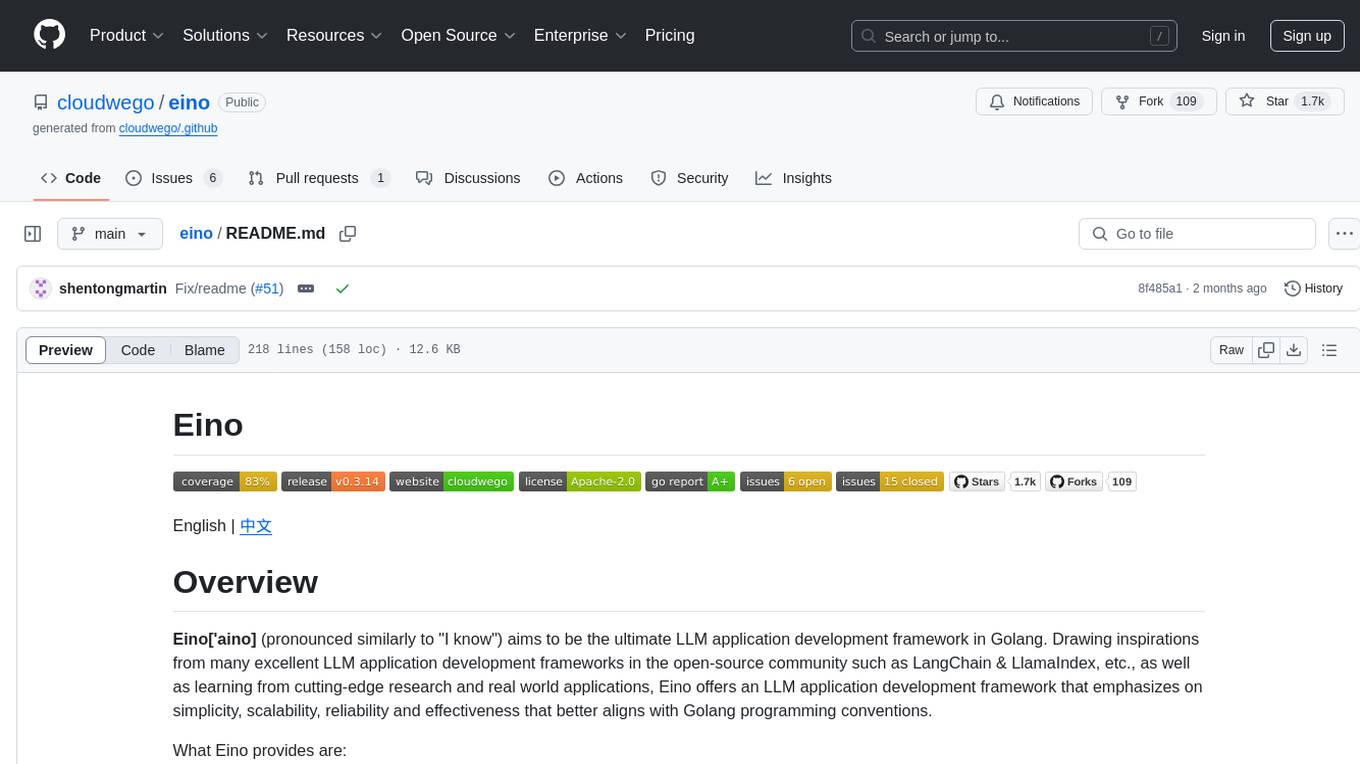
eino
Eino is an ultimate LLM application development framework in Golang, emphasizing simplicity, scalability, reliability, and effectiveness. It provides a curated list of component abstractions, a powerful composition framework, meticulously designed APIs, best practices, and tools covering the entire development cycle. Eino standardizes and improves efficiency in AI application development by offering rich components, powerful orchestration, complete stream processing, highly extensible aspects, and a comprehensive framework structure.